- Merci professeur
- Devenir expert
- Ne plus se tromper
- Voyager en français

- Dictionnaire
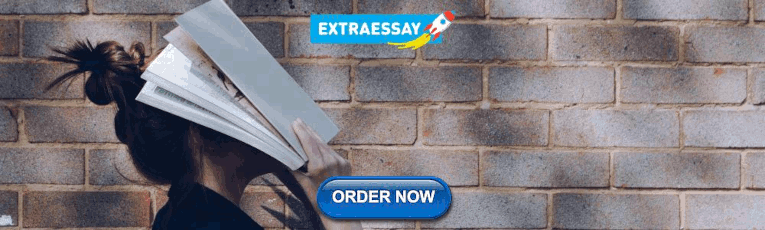
Définition "speech"
- Petit discours improvisé.
Synonyme "speech"
allocution , baratin , causerie , compliment , conférence , discours , éloge , harangue , laïus , toast
Rate of Speech
Published Date : November 5, 2020
Reading Time :
Rate of speech , also known as speaking rate or tempo, refers to the speed at which you speak, measured in words per minute (wpm). It plays a crucial role in effective communication, impacting comprehension, engagement, and overall delivery in various contexts, including public speaking . While speech coaches can offer personalized guidance, public speaking courses can also provide valuable techniques for controlling your speaking rate for clear and impactful communication.
Factors Influencing Rate of Speech:
- Nervousness: Anxiety can increase speaking rate, making it harder for listeners to understand.
- Complexity of information: Technical vocabulary or complex concepts may require a slower pace.
- Target audience: Tailor your rate to the audience’s understanding level and cultural norms.
- Purpose of the speech : Informative speeches benefit from a moderate pace, while persuasive speeches might involve strategic variations.
Ideal Rate of Speech:
There’s no single “perfect” rate, depending on various factors. However, research suggests an ideal range between 150-180 wpm for optimal comprehension and engagement in general communication. This range may vary in public speaking depending on the specific context and purpose.
Benefits of a Controlled Rate of Speech:
- Improved clarity and understanding: Allows listeners to process information easily, reducing confusion.
- Enhanced emphasis and impact: Strategic slowing down can highlight key points and evoke emotions.
- Increased audience engagement: A balanced pace keeps listeners focused and prevents tune-out.
- Greater credibility and professionalism: Projects confidence and control over your message.
Tips for Controlling Rate of Speech:
- Be mindful of your pace: Pay attention to how fast you speak and consciously slow down if needed.
- Practice with a recording: Listen back to identify areas where you can adjust your rate.
- Use pauses effectively: Strategic pauses for emphasis and audience reflection can also pace your speech .
- Focus on breathing: Deep breaths help control vocal cords and naturally slow your speech .
- Join a public speaking course: Gain feedback and practice exercises to refine your pace control.
- Consider working with a speech coach : They can provide personalized guidance and tailored techniques for specific settings.
The rate of speech is a powerful tool for optimizing communication. By understanding its importance, mastering pace control through dedicated practice, and considering resources like public speaking courses and speech coaches, you can deliver your message with clarity and impact and connect with your audience more effectively.
You might also like
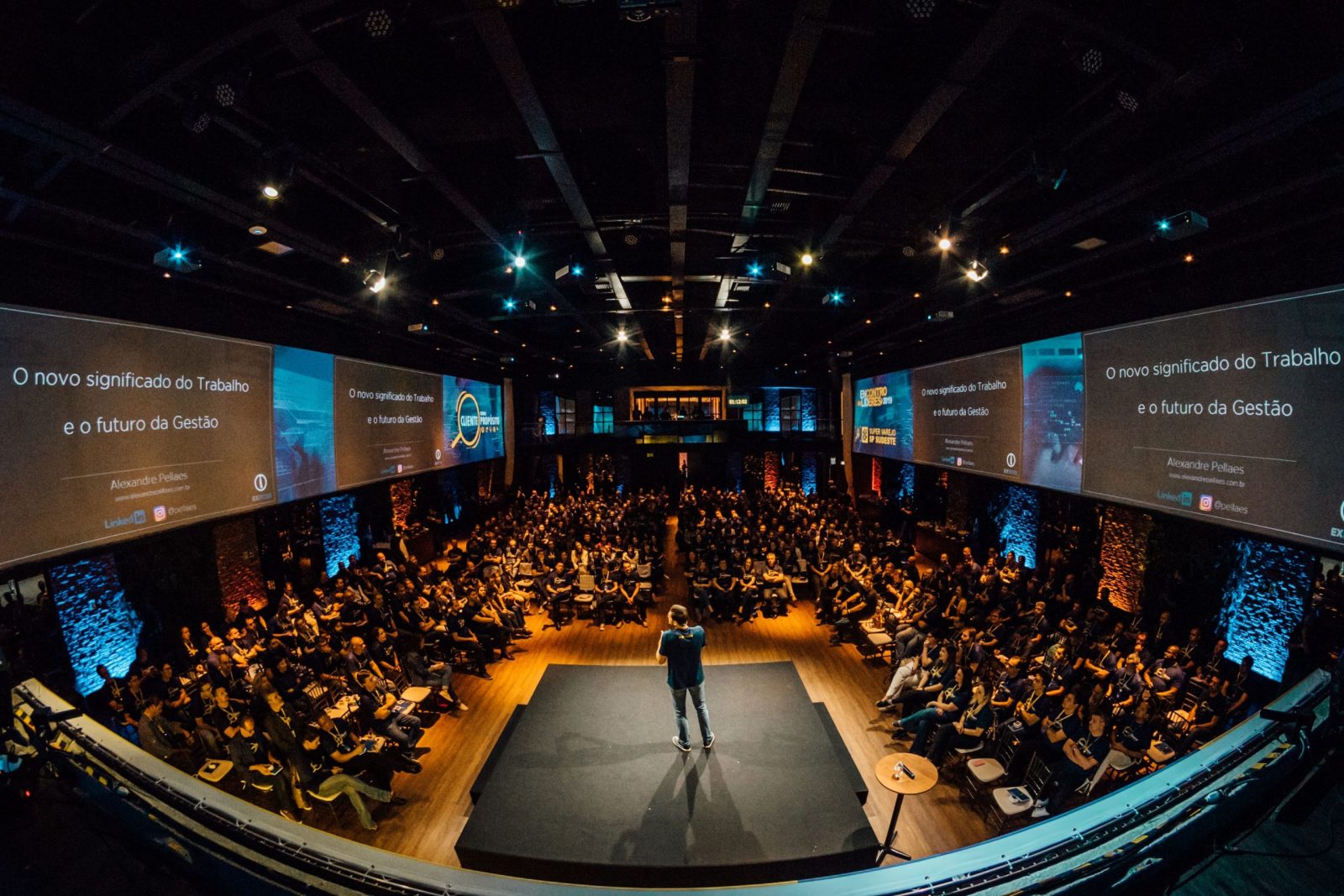
How Many Words is a 5-Minute Speech
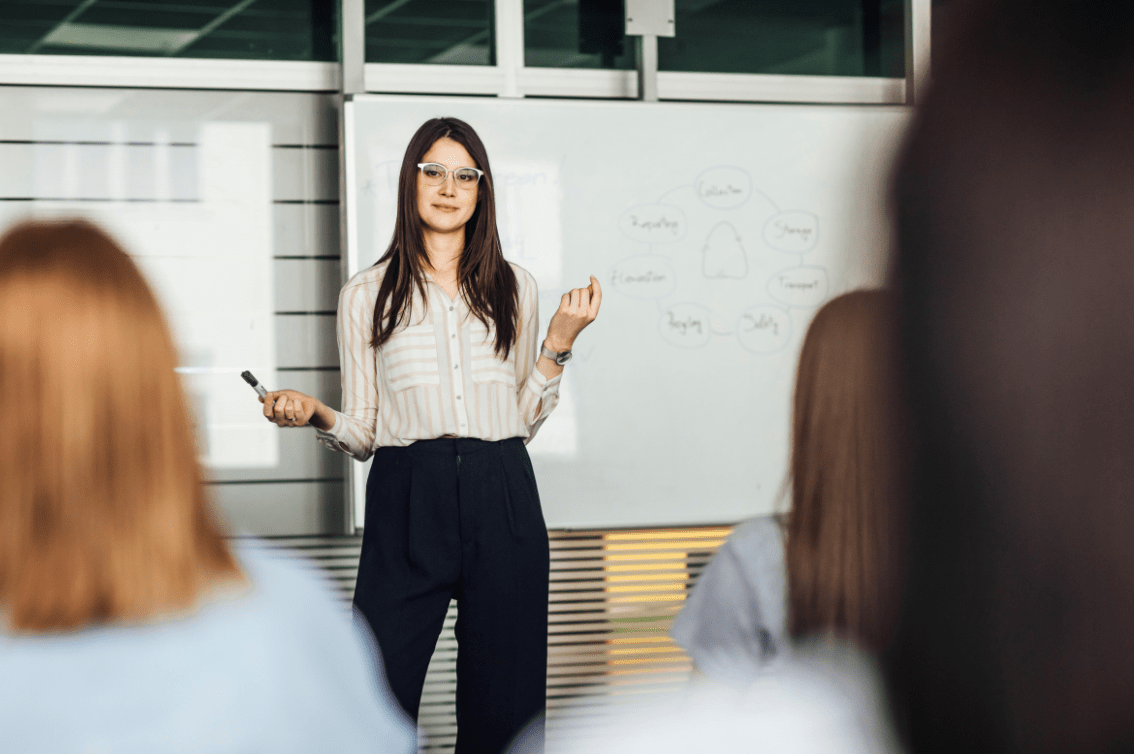
Good Attention Getters for Speeches with 10+ Examples!
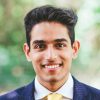
Quick Links
- Presentation Topics
Useful Links
- Start free trial
- The art of public speaking
- improve public speaking
- mastering public speaking
- public speaking coach
- professional speaking
- public speaking classes - Courses
- public speaking anxiety
- © Orai 2023
- Games, topic printables & more
- The 4 main speech types
- Example speeches
- Commemorative
- Declamation
- Demonstration
- Informative
- Introduction
- Student Council
- Speech topics
- Poems to read aloud
- How to write a speech
- Using props/visual aids
- Acute anxiety help
- Breathing exercises
- Letting go - free e-course
- Using self-hypnosis
- Delivery overview
- 4 modes of delivery
- How to make cue cards
- How to read a speech
- 9 vocal aspects
- Vocal variety
- Diction/articulation
- Pronunciation
- Speaking rate
- How to use pauses
- Eye contact
- Body language
- Voice image
- Voice health
- Public speaking activities and games
- About me/contact
- Speech delivery
- Speech rate
What's your speech rate?
Why a flexible speaking rate is important.
By: Susan Dugdale
Is your speech rate too fast, too slow, or just right?
And what is, a normal speaking pace?
The answers to both questions are not straight forward. They fall into the 'it depends' category. And what they're depending on is context.
Context is everything when it comes to deciding whether the speed you speak at is good, extremely good, or poor.
What you'll find on this page
- why, and when, speech rate becomes important
- what speech rate is and how it is calculated
- 2 ways of finding out your own speech rate
- speech rate guidelines - what's fast or slow?
- reasons to change your speech rate
- exercises to develop a flexible speaking rate
- a link to a free printable: a diagnostic resource used by speech therapists to test speech fluency and rate, The Rainbow Passage
- a link to a quick reference guide: how many words per minute are in 1 through to 10 minute speeches .
- links to authoritative references for more information

Why, and when, is speech rate important?
Speech rate – how fast, or how slowly a person talks, only becomes important when the speed of their speech becomes a barrier to effective communication.
If people listening are not able to fully take in or comprehend what is being said and a large part of the reason for that is speech rate, then it's time to take action.
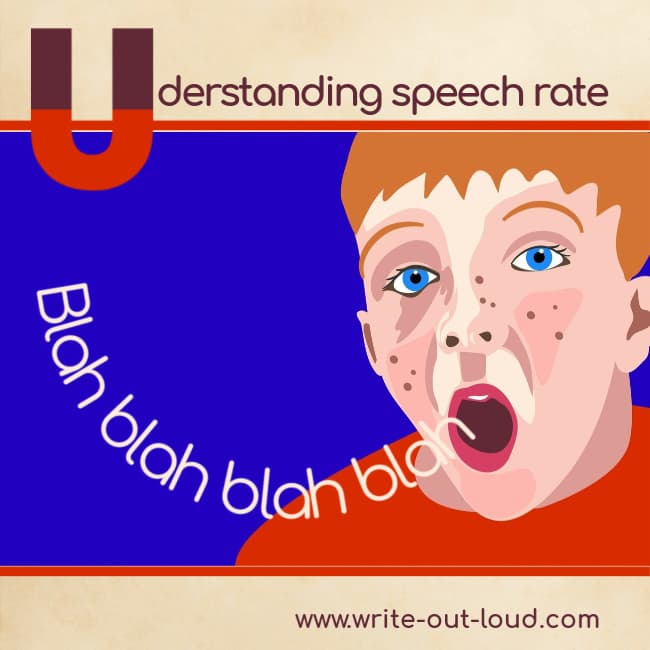
What is speech rate? How is it calculated?
Speech rate refers to a person's habitual speaking speed. It's calculated through counting the normal number of words they say per minute, and just like people, words per minute (wpm) can vary hugely.
Additionally, because all words are not equal, wpm can only ever be an approximate measure. For instance, a word can be as simple as a single syllable like "it" or a single letter like “I”, or a collection of many syllables such as “hippopotamus” or “tintinnabulation” - the ringing of bells.
One syllable is considerably quicker to say than many, just as a simple short sentence is faster to say than a complex longer one.
How to work out your own speech rate
Here are two ways of working out your habitual speech rate.
The first is to read aloud The Rainbow Passage. This piece of text is frequently used by speech language therapists as a diagnostic tool to test a person's ability to produce connected speech .
Record yourself as you read it aloud at your regular speaking rate for one minute.
How far you get through the passage will give you an indication * of your rate of speech.
Here are the first 175 words. The entire piece has 330 words.
(There's a printable pdf of the whole Rainbow Passage for you to download at the bottom of the page.)
The Rainbow Passage
When the sunlight strikes raindrops in the air, they act as a prism and form a rainbow. The rainbow is a division of white light into many beautiful colors. These take the shape of a long round arch, with its path high above, and its two ends apparently beyond the horizon. (51 words)
There is, according to legend, a boiling pot of gold at one end. People look, but no one ever finds it. When a man looks for something beyond his reach, his friends say he is looking for the pot of gold at the end of the rainbow. (99 words)
Throughout the centuries people have explained the rainbow in various ways. Some have accepted it as a miracle without physical explanation. To the Hebrews it was a token that there would be no more universal floods. The Greeks used to imagine that it was a sign from the gods to foretell war or heavy rain. The Norsemen considered the rainbow as a bridge over which the gods passed from earth to their home in the sky. (175 words)
* I've used the word 'indication' because you are reading aloud rather than giving a speech or talking to a friend. There is a difference.
You'll also need to take into account your familiarity with the text. A 'cold' reading, that is reading the passage without seeing it before hand will probably influence how much of it you get through in a minute.
Record yourself delivering a speech
The second way to test yourself is to record one of your own speeches or presentations. This will give you a much more accurate measure of your actual speech rate.
If you have the text of your speech in a word document you'll have access under the Tools tab (see image below) to the total word count.
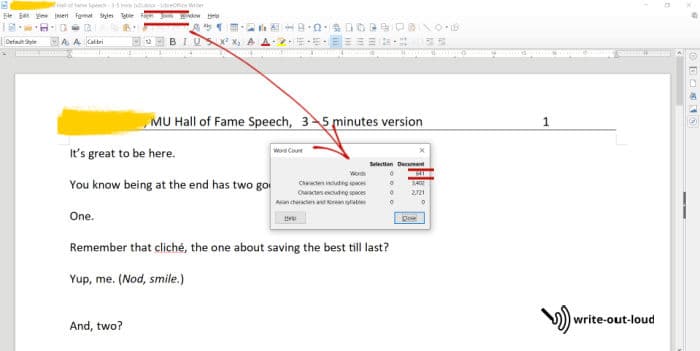
Record the speech. Then take the time you took to deliver it and use it to divide the number of words.
To give you an example I recorded the 'Hall of Fame' speech I wrote for a client a couple of years ago. I took 4.9 minutes to say it through. The total word count of the speech is 641.
Therefore, 641 words divided by 4.9 minutes = a speaking rate of 130 words per minute.
Speech rate guidelines
Studies show speech rate alters depending on the speaker's culture, geographical location, subject matter, choice of vocabulary and its usage (simple short sentences v complex), fluency, use of pauses, gender, age, emotional state, health, profession, audience, and whether or not they're using their primary, or native, language.
However, despite these variables, there are widely accepted guidelines. These are:
- Slow speech is usually regarded as less than 110 wpm, or words per minute.
- Conversational speech generally falls between 120 wpm at the slow end, to 160 - 200 wpm in the fast range.
- People who read books for radio or podcasts are often asked to speak at 150-160 wpm.
- Auctioneers or commentators who practice speed speech are usually in the 250 to 400 wpm range.
Why change your speech rate?
Generally people are not conscious of their habitual speaking speed and if they are easily understood by those listening to them there is little reason to change. Their speech could be considered too slow or too fast by people outside of their normal environment but if they are not routinely communicating with them it doesn't really matter.
However changes of audience and speech purpose can force a need to become more aware of speaking speed.
For example - a shift from one part of a country to another, from a slower speaking area to a faster speaking one, will, through audience response, make a habitually slower speaker aware of their speech rate.
Similarly someone with naturally fast speech who takes a job requiring presentations to colleagues or customers, will find themselves having to slow down in order to communicate effectively.
Having an accent makes a difference too. If the language you're using is not your first one there may be pronunciation issues which make it harder for your audience to understand you. Slowing down your rate of speech will help.
Public speaking and rate of speech
If you're giving a speech or presentation, the concept of a normal speaking speed doesn't apply.
What does is flexibility - the ability of the speaker to mix and match pace appropriately with speech content and the audience's ability to comprehend it.
Experience and audience reaction will teach you that a one-size-fits-all approach will be far less effective than careful variation in rate.
Exercises to change speaking rate
If you know you speak either too fast, too slowly or without speed variation then exercises to develop flexibility are what you need.
Here are Quick and Easy Effective Tips for Speaking Rate Flexibility
These six exercises specifically address the undesirable audience responses brought on by a speaker either talking too quickly or too slowly. Have fun with them!
How many words per minute in a speech?
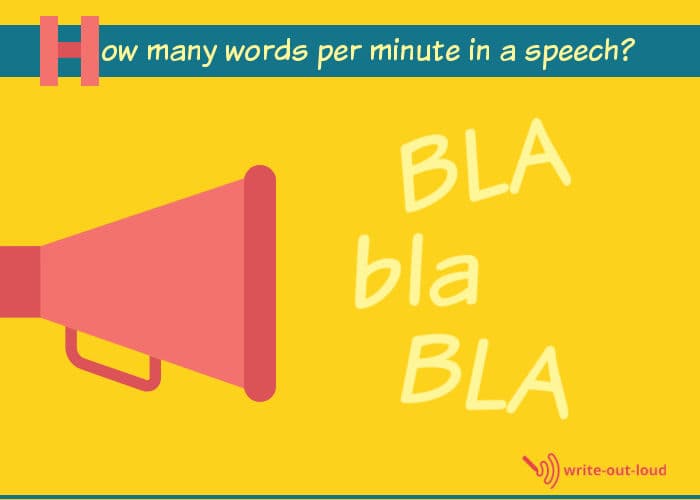
When you have a speech to give with a strict time limit it's useful to have an estimate of how many words will fit comfortably into the time allocated, before you begin to write.
For more see: How many words per minute in a speech: a quick reference guide for 1 through to 10 minute speeches.
Do you know what your voice says about you?
Find out about Voice Image First impressions count and they're not only about looking good, but sounding good too!
References and additional information
Miller, N., Maruyama, G., Beaber, R. J., & Valone, K. (1976). Speed of speech and persuasion . Journal of Personality and Social Psychology, 34(4), 615–624.
Smith, S. M., & Shaffer, D. R. (1991). Celerity and cajolery: Rapid speech may promote or inhibit persuasion through its impact on message elaboration . Personality and Social Psychology Bulletin, 17(6), 663–669.
Rodero, E. (2012). A comparative analysis of speech rate and perception in radio bulletins . Text & Talk, 32 (3), pp. 391–411
Apple, W., Streeter, L.A., & Krauss, R. M. (1979). Effects of Pitch and Speech Rate on Personal Attributions . Journal of Personality and Social Psychology, 37( 5), 715-727
Optimal Podcast Words per Minute Rate for Biggest Impact - an extremely thorough article by Chris Land of improvepodcast.com
What is the ideal rate of speech? Public speaking coach Lynda Stucky 'shows and tells' about speech rate. She's made 7 variations of The Rainbow Passage so that you can hear the difference speed makes.
Speech Pace: do you talk too fast or too slow? Take this test . - a YouTube video by speech teacher Laura Bergells.
Perfect Your Speed Talking at This Auction School - a YouTube video showing how The Missouri Auction School teaches speed speech. ☺
Download The Rainbow Passage
Click the link to download a printable pdf of The Rainbow Passage .
- Return to top of speech rate page
speaking out loud
Subscribe for FREE weekly alerts about what's new For more see speaking out loud
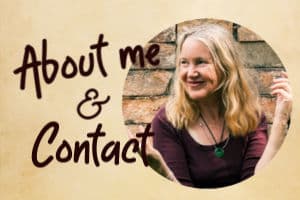
Top 10 popular pages
- Welcome speech
- Demonstration speech topics
- Impromptu speech topic cards
- Thank you quotes
- Impromptu public speaking topics
- Farewell speeches
- Phrases for welcome speeches
- Student council speeches
- Free sample eulogies
From fear to fun in 28 ways
A complete one stop resource to scuttle fear in the best of all possible ways - with laughter.
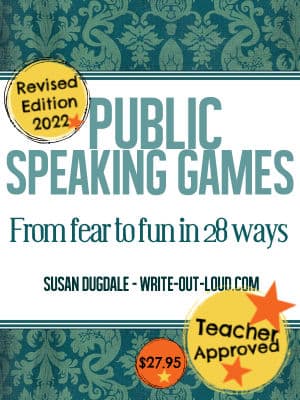
Useful pages
- Search this site
- About me & Contact
- Blogging Aloud
- Free e-course
- Privacy policy
©Copyright 2006-24 www.write-out-loud.com
Designed and built by Clickstream Designs
Module 8: Delivering Your Speech
Articulation, pitch, and rate, learning objectives.
- Identify techniques to use effective articulation.
- Identify effective rates of speaking.
Articulation
Once you’ve mastered controlling your breath as you speak, next let’s look at how you speak. If you have ever had someone ask you to repeat a word, you may suffer from poor diction. Articulation , or diction , is what helps the listener not just hear the spoken word but also understand it.
Articulation is how clearly the speaker pronounces words. When some sounds are slurred together or dropped out of a word, the word may not be understood by the audience. To use proper articulation, a speaker must use their articulators : tongue, teeth, and lips. When a speaker uses improper diction, the hearer cannot make out the word spoken and often requests a repeat of what was said. In public speaking, a hearer cannot request a repeat and therefore poor articulation can make a listener tune out. It is important to say all parts of the word in order to speak clearly. This often requires slowing down your speaking pace , more on that topic to follow, and using your lips, teeth, and tongue to their full capacity.
Tongue twisters are a great way to force the speaker to slow down and pronounce each part of the word. Try saying, “Seven silly swans swam silently seaward” three times quickly. If that was easy for you, s’s may be your forte! Each individual speaker will struggle with certain sounds specific to them, or have developed a regionalism that makes them pronounce a word the way they’ve always heard it that doesn’t work in other parts of the country. A technique to making sure your speech isn’t affected by problem words is to note which sounds are struggles and circling the parts of the word on the speech outline. This serves as a reminder to take extra care when speaking that word out loud. Identifying these barriers to communication will improve the understanding of the audience and give polish to your speech.
In addition to speaking clearly, finding vocal variety in your speaking voice will help the audience stay awake. A voice that lacks variety can be described as monotone. In comedies, teachers are often portrayed as having a monotone voice, as in this famous scene in Ferris Bueller’s Day Off :
You can view the transcript for “Bueller Bueller Bueller” here (opens in new window) .
When the audience hears a monotone voice, they don’t stay engaged.
Much like a keyboard, your voice has many notes to it called pitches. Your voice can speak on higher notes and lower notes much like when someone sings. To explore the notes in your voice, try this exercise. Stand up on your toes and lift your hands in the air. Say ah at the highest point of your voice, which makes sound come out, and drop your wrists, elbows, and head over as you slide down to your lowest note. Reverse it and come back up trying to go higher and lower each time. Having discovered how much pitch variety you have to work with, you can now put arrows into your speech outline reminding you to raise the pitch or lower it on some words or phrases to be more effective.
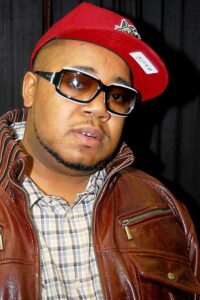
Chicago rapper Twista can clock 280 words per minute or 598 syllables in 55 seconds (a Guinness record). Don’t try to do this in your speech.
Next to being loud enough, the most commonly identified speech problem is speaking too quickly. Raise your hand if you’ve ever been told you’re a fast talker. Controlling the rate at which one speaks is often one of the most challenging things a speaker has to do. When nerves kick in, it can be really hard to pull back on the speed that you’re talking at as sometimes you just want to finish and get out of the spotlight. Speaking too quickly can also make your audience tune out from listening to the speech. You’ve put all this time into the speech, so let’s make sure the audience hears it. According to The National Center for Voice and Speech, the average speaking rate for English speakers in the U.S. is around 150 words per minute. In a public speaking situation, you’ll want to speak slower than average, around 125–150 words per minute.
One of the ways to control your rate of speech is to make sure you are taking enough breaths. As we discussed before, if you lose control of your breathing, the rate of speech also gets out of control. One of the ways to make sure you breathe enough is to place a mark next to the word in a sentence on your outline to remind yourself to breathe there. A backwards slash (/) is a good signal to use. In order to see if the breaths selected work, read it out loud. If you find yourself gasping for air at the end of that sentence, there should be another breath added. Punctuations are the clues for where to breathe in a sentence too, so let those be your guide.
Recording yourself is one way to get a sense of how quickly you’re going. Play the recording back and listen to see if you can hear and understand every word. If not, write notes on your notecards that say SLOW DOWN or BREATHE to remind yourself to do so. Once you’ve mastered a controlled rate of speech, then you are able to play with speeding up and slowing down certain sections. Finding this variety of speed will further engage your audience. Think about telling the climax of a story. Sometimes you pause at certain moments to build suspense. That’s what you want to do in public speaking too. Sometimes you speed up to tell a story with momentum so the audience goes along for the ride too. Finding variety in your rate can be thrilling and the icing to a great speech.
To watch: Rébecca Kleinberger, “Why you don’t like the sound of your own voice”
In this talk, MIT voice expert and researcher Rébecca Kleinberger talks about the three voices humans have: the outward voice, the inward voice, and the inner voice. Kleinberger’s account here helps to explain why our own voice—which we hear all the time—sounds so unfamiliar to us when we hear it in a recording. It also speaks to the need to practice listening to your voice in recordings.
You can view the transcript for “Why you don’t like the sound of your own voice | Rébecca Kleinberger” here (opens in new window) .
What to watch for:
Kleinberger’s speech is fascinating, and offers a great deal of insight into the way we perceive (or fail to perceive) our own voices. Interestingly, although she speaks at length about why we don’t recognize our voice, Kleinberger doesn’t really answer the question of why we don’t like our voices. At the end of the speech, some listeners may still be wondering why they don’t like the voice they hear in recordings of themselves, and what they could do about it. This should serve as a reminder that if you have a catchy title with a question in it, you have to make sure you answer the question in your speech!
- Twista. Authored by : Adam Bielawski. Located at : https://en.wikipedia.org/wiki/Twista#/media/File:Twista_101109_photoby_Adam-Bielawski.jpg . License : CC BY-SA: Attribution-ShareAlike
- Bueller Bueller Bueller. Authored by : blc3211. Located at : https://youtu.be/f4zyjLyBp64 . License : Other . License Terms : Standard YouTube License
- Why you don't like the sound of your own voice | Rebecca Kleinberger. Provided by : TED. Located at : https://youtu.be/g3vSYbT1Aco . License : Other . License Terms : Standard YouTube License
- Articulation, Pitch, and Rate. Authored by : Misti Wills with Lumen Learning. License : CC BY: Attribution
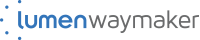
Voice speed
Text translation, source text, translation results, document translation, drag and drop.
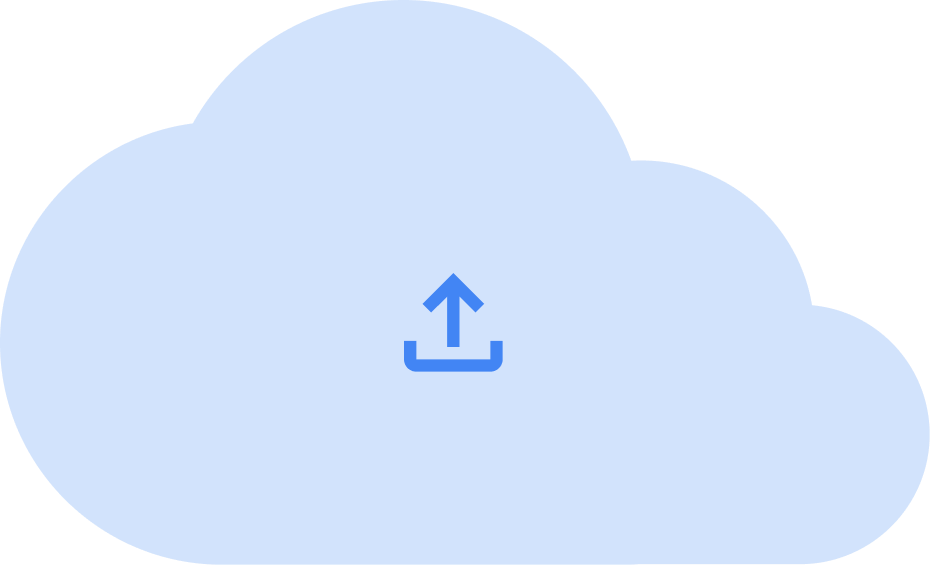
Website translation
Enter a URL
Image translation

- Cambridge Dictionary +Plus
- Déconnexion
Définition de speech en anglais
Your browser doesn't support HTML5 audio
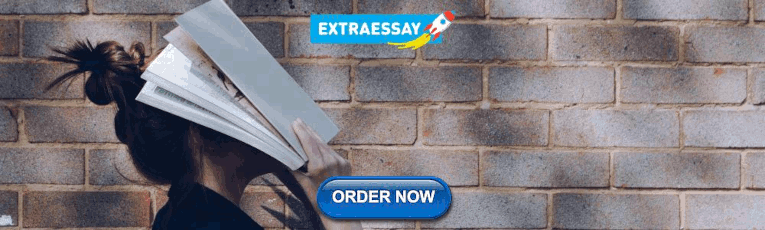
speech noun ( SAY WORDS )
- She suffers from a speech defect .
- From her slow , deliberate speech I guessed she must be drunk .
- Freedom of speech and freedom of thought were both denied under the dictatorship .
- As a child , she had some speech problems .
- We use these aids to develop speech in small children .
- a problem shared is a problem halved idiom
- banteringly
- bull session
- chew the fat idiom
- conversation
- conversational
- put the world to rights idiom
- take/lead someone on/to one side idiom
- tête-à-tête
Vous pouvez aussi trouver des mots apparentés, des expressions et des synonymes dans les thèmes :
speech noun ( FORMAL TALK )
- talk She will give a talk on keeping kids safe on the internet.
- lecture The lecture is entitled "War and the Modern American Presidency".
- presentation We were given a presentation of progress made to date.
- speech You might have to make a speech when you accept the award.
- address He took the oath of office then delivered his inaugural address.
- oration It was to become one of the most famous orations in American history.
- Her speech was received with cheers and a standing ovation .
- She closed the meeting with a short speech.
- The vicar's forgetting his lines in the middle of the speech provided some good comedy .
- Her speech caused outrage among the gay community .
- She concluded the speech by reminding us of our responsibility .
- call for papers
- extemporize
- maiden speech
- presentation
- talk at someone
«speech» en anglais américain
Speech noun ( talking ), exemples de speech, collocations avec speech.
Voici des mots souvent utilisés en combinaison avec speech .
Cliquez sur une collocation pour plus d'exemples.
Traductions de speech
Obtenez une traduction rapide et gratuite !
Mot du jour
acting or speaking together, or at the same time

Alike and analogous (Talking about similarities, Part 1)

Nouveaux mots
En apprendre plus avec +Plus
- Récent et Recommandé {{#preferredDictionaries}} {{name}} {{/preferredDictionaries}}
- Définitions Explications claires de l'anglais naturel écrit et parlé anglais dictionnaire des apprenants anglais britannique essentiel anglais américain essentiel
- Grammaire et Dictionnaire des synonymes Explications des usages de l'anglais naturel écrit et parlé grammaire synonymes et antonymes
- Pronunciation British and American pronunciations with audio English Pronunciation
- anglais-chinois (simplifié) Chinese (Simplified)–English
- anglais-chinois (traditionnel) Chinese (Traditional)–English
- anglais-néerlandais néerlandais-anglais
- anglais-français français-anglais
- anglais-allemand allemand-anglais
- anglais-indonésien indonésien-anglais
- anglais-italien italien-anglais
- anglais-japonais japonais-anglais
- anglais-norvégien norvégien-anglais
- anglais-polonais polonais-anglais
- anglais-portugais portugais-anglais
- anglais-espagnol espagnol-anglais
- English–Swedish Swedish–English
- Dictionary +Plus Listes de mots
- speech (SAY WORDS)
- speech (FORMAL TALK)
- speech (TALKING)
- Collocations
- Translations
- Toutes les traductions
Ajoutez speech à une de vos listes ci-dessous, ou créez une nouvelle liste.
{{message}}
Il y a eu un problème.
Votre commentaire n'a pas pu être envoyé dû à un problème.

An official website of the United States government
The .gov means it’s official. Federal government websites often end in .gov or .mil. Before sharing sensitive information, make sure you’re on a federal government site.
The site is secure. The https:// ensures that you are connecting to the official website and that any information you provide is encrypted and transmitted securely.
- Publications
- Account settings
Preview improvements coming to the PMC website in October 2024. Learn More or Try it out now .
- Advanced Search
- Journal List
- HHS Author Manuscripts

Robust Speech Rate Estimation for Spontaneous Speech
Viterbi School of Engineering, University of Southern California (USC), Los Angeles, CA 90007 USA. He is now with the IBM T. J. Watson Research Center, Yorktown Heights, NY 10598 USA
Shrikanth S. Narayanan
Viterbi School of Engineering, University of Southern California (USC), Los Angeles, CA 90007 USA
In this paper, we propose a direct method for speech rate estimation from acoustic features without requiring any automatic speech transcription. We compare various spectral and temporal signal analysis and smoothing strategies to better characterize the underlying syllable structure to derive speech rate. The proposed algorithm extends the methods of spectral subband correlation by including temporal correlation and the use of prominent spectral subbands for improving the signal correlation essential for syllable detection. Furthermore, to address some of the practical robustness issues in previously proposed methods, we introduce some novel components into the algorithm such as the use of pitch confidence for filtering spurious syllable envelope peaks, magnifying window for tackling neighboring syllable smearing, and relative peak measure thresholds for pseudo peak rejection. We also describe an automated approach for learning algorithm parameters from data, and find the optimal settings through Monte Carlo simulations and parameter sensitivity analysis. Final experimental evaluations are conducted based on a portion of the Switchboard corpus for which manual phonetic segmentation information, and published results for direct comparison are available. The results show a correlation coefficient of 0.745 with respect to the ground truth based on manual segmentation. This result is about a 17% improvement compared to the current best single estimator and a 11% improvement over the multiestimator evaluated on the same Switchboard database.
I. Introduction
S PEECH has been considered an attractive input modality for human–computer interactions for a long time. More recently, there has also been increasing interest in automatically mining vast amounts of speech data to determine not just what was spoken but how and by whom as well. Much of the research focus over the past three decades has been on automatic speech recognition, with tremendous progress being made, especially with the adoption of hidden Markov model (HMM)-based architectures. However, speech technology is still far from achieving the goal of robust speech understanding. One reason, which is also reflected in the current research trends in human language technologies, is the inability to adequately capture and represent the rich information contained in speech that is beyond mere speech-to-text transcription, as provided by conventional automatic speech recognizers. Humans use a wide variety of cues for recognizing and understanding speech, including intonation, prominence, and speaking rate. Machine processing of natural speech may also benefit from using these cues. Hence, one key goal of present day spoken language processing research is to automatically and robustly characterize these suprasegmental aspects of speech. This paper focuses on the topic of automatic speech rate estimation.
A. Significance
Speech rate is primarily dependent on two factors: speaking style and the nature/scenario of speech production (e.g., scripted/spontaneous). Research in this domain has two distinct application-driven threads as to how speech rate variability is addressed. On the one hand, variation in speech rate tends to adversely impact automatic speech recognition (ASR) and needs to be mitigated. On the other hand, variation in speech rate carries information critical for speech understanding and needs to be quantified to determine contextual variables such as speaking context, audience, knowledge of the subjects, etc. Much of the early focus on speech rate estimation was targeted toward improving ASR robustness. Even though HMMs have the ability to accommodate some of the spectral–temporal variations in speech, recognition accuracy is still severely influenced by mismatches between training and testing conditions. Speech rate variability is one such contributing factor [ 1 ]. A first step toward addressing this issue, i.e., to help improve the match between the models used and the speech being processed for recognition, is to quantify the inherent speech rate variability. Then, once an estimation of the underlying speech rate is done, one could select appropriately pretrained acoustic models [ 25 ], [ 54 ] or adaptively set transition probabilities of the HMMs [ 4 ], [ 5 ] that appropriately reflect the rate of the speech being measured.
Speech rate information can also be used in other speech processing scenarios besides robust automatic speech recognition. Speech rate variance could be interpreted as a function of the cognitive load associated with processing the text transcription [ 27 ], [ 42 ]. Cognitive load could be defined as the level of effort for the speaker/user to select the words to speak (for the main task or concurrent subtask [ 42 ]). In spontaneous speech scenarios, the speaker typically has to address various tasks on the fly, as they unfold, with unknown cognitive loads. So, not surprisingly, the speech rate variability for spontaneous speech can be quite large [ 44 ].
With increasing interest in spontaneous speech recognition and interpretation in recent years, and challenges posed by the acoustic and linguistic characteristics of spontaneous speech that are highly variable and more unstructured than prepared speech, the role of speech rate estimates has become ever more important. Notably, instead of just relying on the text from ASR to arrive at speech rate estimates, which may be quite noisy, there is a need to use suprasegmental acoustic features to directly facilitate speech interpretation. Below, we highlight some specific applications.
Prior research has shown that local speech rate correlates with discourse structure. For example, global analysis of the discourse structure in paragraphs and clauses has revealed that for each of the speakers considered, the average syllable duration of the first run of a paragraph is longer than the overall mean value per speaker in more than 60% of the cases (50% is the chance value)[ 3 ]. Local speech rate variations may carry other crucial information as well. For example, speech rate plays an important role in the context of sentence boundary detection and disfluency detection. It has been suggested that people tend to have longer syllable duration, or equivalently slower local speaking rate, at these events [ 6 ], [ 7 ]. Speech rate also correlates with prosodic prominence. Detection and normalization of rate of speech has been found to be necessary in measuring such attributes [ 8 ], [ 21 ]. Global speech rate also works as a normalization factor for many prosody-based classifiers. For example, it was selected as a key prosodic feature in the machine learning process of dialog act detection [ 19 ], [ 23 ]. In summary, speech rate estimation can be useful in a number of spoken language processing contexts.
B. General Measurement Methods
There have been two major trends in measuring speech rate. Each has its advantages and limitations. The first represents the use of discrete categorization—frequently, “fast,” “normal” and “slow”—to describe speech rate [ 24 ]. Such perceptually chosen classes have been used in applications such as acoustic model selection [ 9 ], [ 25 ] and HMM normalization [ 15 ] in ASR. Even though it matches human intuition, the boundaries between these three categories are fuzzy. Most of the time, human knowledge is required to set the boundaries, and hence it is difficult to devise a completely automated engineering solution.
In the second approach, speech rate is measured in a quantitative way by counting the number of phonetic elements per second. Words, syllables [ 9 ], stressed syllables, and phonemes [ 10 ] are all possible candidates, and syllables are a popular choice [ 6 ], [ 9 ], [ 11 ]. Studies on speech rhythm, i.e., organization of prominent and less prominent speech units in time, offer some motivation in this regards. Evidence from reiterative speech studies [ 16 ] supports the idea that syllable evolution is a good estimate of speech rhythm. Specifically, while the classic isochrony (or rhythm class) hypothesis regarding stress-timed, syllable-timed, or mora-timed languages has been largely unsupported by acoustic–phonetic evidence, a form of the isochrony hypothesis for rhythm has been shown to be supported by speech measures based on syllable structure and vowel reduction [ 50 ], [ 51 ]. Definitions for the syllable have been offered from a variety of perspectives; phonetically, Roach [ 37 ] describes a syllable as “consisting of a center which has little or no obstruction to airflow and which sounds comparatively loud; before and after that center (…) there will be greater obstruction to airflow and/or less loud sound.” This definition allows for a plausible way for detecting syllables in speech. Intuitively, syllables, by these accounts, should have an even distribution under normal speech production, and their rate could be changed as a result of speech rate change. Given such characteristics of syllables, the syllable-based rate estimate appears to be a widely used choice among speech rate researchers [ 6 ], [ 9 ], [ 11 ]. In this paper, we use number of syllables per second as a measure of speech rate. We will further explore the syllable’s acoustic property in Section II.
C. Role of ASR in Speech Rate Estimation
We first need to detect syllable boundaries for speech rate estimation. A straightforward, and convenient, approach would be through the use of automatic speech recognition where syllable boundaries can be retrieved as a side product of phonetic segmentation such as through Viterbi decoding [ 10 ]. Furthermore, ASR errors could be minimized with a supervised alignment process if the correct transcription were known [ 6 ], [ 7 ]. However, such an approach has limitations, while alternative approaches can offer other advantages.
First, assuming that the reference transcription is not available in real applications, recognition errors—especially for spontaneous speech—are unavoidable. Recognition errors (particularly insertions and deletions) would have the effect of degrading the performance of ASR-reliant speech rate estimation methods [ 25 ]. Second, speech rate could work as an acoustic feature to help ASR instead of being dependent on it. Hence, it is better to detect it in parallel or even be used as a part of an ASR front end. In this way, we can combine the complementary information produced by speech rate estimation and ASR. Finally, we believe that direct speech rate estimation can be easily extended to languages with vowel-centric syllable structures similar to English. This would be especially useful when only sparse data is available and where building a high-performance ASR system is especially challenging.
In this paper, we investigate using acoustic-only features to derive speech rate. The rest of the paper is organized as follows: Section II reviews the previous work and identifies the challenges. Section III introduces the data for evaluation. Section IV introduces our algorithm. Section V describes the system and evaluation. The final section provides conclusion and discussion.
II. Previous Work
As stated in the previous section, we use number of syllables per second as the speech rate measure in this work. We therefore focus on identifying the correct number of syllables in an utterance.
A. Background
This task to identify the syllable structure in an utterance dates back to the very early stages of speech recognition research in the mid 1970s, where syllable detection was a popular first step in automatic speech recognition [ 41 ]. The HMM-based statistical framework for ASR had not been popularized then, and most of the research relied on knowledge (rule)-based acoustic signal processing. A variety of features had been proposed to capture the syllable nucleus. These included, for example, the use of linear predictive coding spectra [ 27 ], [ 28 ] or critical filter banks [ 32 ] to extract the low-to-high frequency energy ratios that characterize the acoustic properties of a syllable. Also, power spectra were used to derive a low-frequency profile in the region of first few formants of vowels [ 29 ], [ 30 ]. Due to restrictions of processing hardware and data availability at that time, these efforts were limited to read speech in quiet laboratory environments, usually produced as isolated words or slow, carefully read sentences. [ 41 ].
With the wide adoption of hidden Markov model-based speech recognition in the 1980s, there was a decreased focus on acoustic–phonetic studies for ASR. However, recently with the increased scope of spoken language processing (Section I) the need for processing meta-linguistic features has increased considerably, resulting in many interesting approaches, including for speech rate estimation [ 8 ], [ 12 ]. A significant advantage of present-day research is the ability to use large, spontaneous speech corpora to obtain statistically significant results. An influential recent effort on speech rate estimation is by Morgan and Fosler-Lussier [ 9 ]. Our paper was inspired, and builds upon on their work, which we will review further in Section II-C.
All of the previously proposed techniques share the basic knowledge-based feature extraction ideas. The strategy relies on converting the speech waveform to a lower (frequently, one)-dimensional representation. Following that step, the syllable nucleus is located by picking peak patterns in such a representation. There are alternatives to simple peak picking. For example, Mermelstein [ 29 ] used a “convex hull” algorithm to recursively detect peaks which are prominent relative to their surroundings. Rabiner used a static threshold on the total energy profile [ 31 ].
In addition to these rule-based approaches, there have been attempts to use statistical learning methods to derive syllable nuclei. Normally, a large number of features are extracted such as log energy spectra organized in critical bands [ 33 ], bark scale filter bank [ 34 ], and even auditory models (RASTA [ 35 ]). The learning methods are mostly based on hidden Markov models [ 34 ] or artificial neural networks [ 33 ] and are usually trained with appropriately annotated corpora.
B. Acoustic Characteristics of Syllables
The task of automatically detecting the syllable nucleus has a close relationship with vowel landmark detection [ 41 ] based on the assumption that a syllable is typically vowel centric and neighboring vowels are always separated by consonants. The use of the term “vowels” in this context can be in fact generalized to “sonorant segments,” in light of the discussion in Section I-B about the definition of syllable. Generally speaking, vowels form the nucleus of syllables, whereas consonants form the boundaries in between [ 40 ]. However, it should be noted that a more precise characterization of the syllable structure can be made in terms of sonority (a sound’s “loudness relative to that of other sounds with the same length, stress, and pitch.” [ 40 ]) which posits that syllables contain peaks of sonority that constitute their nuclei and may be surrounded by less sonorous sounds [ 52 ], [ 53 ]. According to the Sonority Sequencing Principle [ 52 ], vowels and consonant sounds span a sonority continuum with vowels being the most sonorous and obstruents being the least, with glides, liquids, and nasals in the middle. In this paper, we will use the term vowels to mean sonorant sounds in the nucleus of a syllable. We use this convention for simplicity and because vowels constitute the most sonorous and frequent members of syllable nuclei.
A vowel is characterized by an open configuration of the vocal tract so that, unlike consonants, there is no significant build-up of air pressure above the glottis [ 40 ]. Due to resonances in the vocal tract, a vowel exhibits clear formant structure in its spectrum. This contrasts with consonants, which are characterized by a constriction or closure at one or more locations along the vocal tract. We will use this general description to motivate our design of the algorithm for syllable nucleus detection.
C. Subband-Based Correlation Approach
As a preface to the description of our algorithm, we review the correlation based approach proposed by Morgan and Fosler-Lussier [ 9 ] and other related work. One classic way to get syllable counts is through performing full-band spectrum/energy analysis and measuring the dominant peak of the long-term envelope [ 13 ]. However, such an approach results in significant noise in the final envelope, making it difficult to obtain syllable counts robustly.
Many further improvements for the energy/spectrum idea have been proposed. For example, Pfitzinger [ 20 ] extracted a band-pass signal and applied rectifying and smoothing window to it before performing peak counting. In that work, a 21.8% error rate (a measure that uses syllable nucleus matching between test and transcription location) was reported. As an alternate approach to the same problem, the first spectral moment of the broadband energy envelope was used as a speech rate measure [ 12 ]. While this method provided improved performance with conversational speech, it was shown that using a one-hour subset of manually transcribed Switchboard data, the correlation between transcribed syllable rate and the experimental rate was only about 0.4 (when both were measured over between-pause spurts) [ 12 ].
All the aforementioned syllable detection approaches assume the rate of peaks on wide band energy envelope (see, e.g., Fig. 1 ) is a valid representation for speech rate measure. However, this assumption has its limitations. For instance, formant structure, which is crucial for syllable identification in fast speech, is lost when the wide band energy envelope representation is used. For example, the same magnitude on wide band energy envelope might correspond to different formant structure, thus different vowels. For fast speech, the transition between different vowels is difficult to identify by energy envelope. Since such a wide band energy envelope is only one of many possible representations of speech, researchers have proposed alternative measures. One of the major improvements was given in [ 9 ], where Morgan and Fosler-Lussier developed a subband-based module that computes a trajectory that is the average product over all pairs of compressed subband energy trajectories. That is, if x i ( n ) is the compressed energy envelope of the i th spectral band, a new trajectory y ( n ) is defined as
where N is the number of bands, and M = N ( N − 1)/2 is the number of unique pairs. The algorithm and the system of [ 9 ] is summarized in Fig. 2 . By this method alone, correlation coefficients greater than 0.6 were achieved between the referenced and measured speech rate values. Furthermore, it was shown in [ 9 ] that the performance would boost to 0.673 if multiple estimators were combined (with wideband energy peak count and spectral moment count; see Fig. 2 ). This method addresses the formant structure issues we discussed earlier by introducing band-wise correlation in the spectral domain, which accentuates the syllable peak in the correlation profile.

Sample speech utterance “SOME FORM” from the Switchboard corpus: (a) Speech waveform. (b) Wideband spectrum. (c) Correlation envelope (approach in this paper). (d) Wideband energy envelope.

Major steps in computing “mrate” (adapted from [ 9 ]).
We build upon this method, and address two key challenges. The first one relates to choosing the robust feature set to identify the syllable nucleus. Solutions have been proposed from both signal processing [ 27 ], [ 29 ] and speech production [ 35 ] points of view. We consider both spectral and temporal features in characterizing the syllable envelope as described in Section IV. The second problem concerns optimal parameter selection. Heuristic methods have been popular, but they do not guarantee optimality or generalizability across domains [ 31 ]. Statistical learning schemes are attractive in the sense of objectively trying to seek optimal parameters. The challenges, however, include the availability of an appropriate training scheme, and effectively dealing with multiscale, multidimensional features such as those needed for the speech rate problem [ 34 ]. We adopt a Monte Carlo simulation-based method, followed by a systematic sensitivity analysis to facilitate parameter estimation. We evaluate our method on a database of spontaneous speech, which we describe in Section III.
III. Database
Our primary goal is to robustly detect speech rate on spontaneous speech. We use the phonetically transcribed ICSI Switchboard corpus subset (provided kindly by Fosler-Lussier [ 9 ]). Switchboard is a corpus of several hundred informal speech dialogs recorded over the telephone [ 11 ], [ 39 ]. The corpus is extensively used for development and testing of speech recognition algorithms and is considered to be fairly representative of spontaneous discourse. In contrast to carefully enunciated, read speech (such as TIMIT [ 43 ]), the speech contained in Switchboard tends to vary significantly in terms of rate, prominence, etc. A total of 5682 spurts were hand transcribed phonetically by linguists in the Switchboard Transcription Project at ICSI [ 2 ]. The transcription includes syllable boundary information (not manually segmented but hand-corrected machine derived segmentations). The cutoff marks (h#, sil) are taken care of to get the accurate reference syllable numbers. This corpus is the same as used in [ 9 ].
IV. Algorthm Design
Our proposed algorithm works by abstracting the speech waveform to a 1-D envelope and detecting syllables by peak picking. It consists of four stages: spectral processing, temporal processing, smoothing, and thresholding. This section is organized in the following way: First, we will summarize a number of practical issues that the algorithm needs to tackle. Second (in Sections IV-B–E), we will describe the four stages of our algorithm, clarifying which particular challenge each part is addressing. Finally, we will describe our strategy for choosing the optimal parameters for each algorithm setting.
A. Practical Challenges
Our algorithm is based on the speech subband correlation approach [ 9 ]. Peak picking on the resulting correlation envelope gives the syllable number estimation. A major challenge is due to noise in this envelope, that can result from a variety of sources as discussed below, and can interfere with the peak picking and degrade the accuracy of syllable number estimation.
1) Background Noise
Background noise is a significant contributing factor toward spurious peaks in the correlation envelope. For example, in Fig. 1 , there are instances of background noise in the regions between 0.78 and 0.85 and 1.05 and 1.15 s. Such noises tend to introduce extra peaks in the final correlation envelope. One traditional way is do noise cancellation or suppression. However, often, noise can be of disparate types, and difficult to characterize. Such noises also include soft breath and cross-channel voices that are not a part of the foreground speech. We apply pitch verification and relative thresholding techniques to address these problems.
2) Consonant “Noise”
Consonants are key components of speech. The particular correlation approach we consider here, however, relies on vowels to be the major contributor of the syllables and thus the peaks. As explained in Section II-B, additionally this includes sonorant consonants, such as /l/, /r/, which can also carry syllabic weights. However, other (obstruent) consonants, especially fricatives, also sometimes contribute extra peaks not related to the “syllable peak.” This is why they are categorized as “noise” here. The characteristic of such noise is that they do not have as much energy as a vowel. Furthermore, they may not have pitch associated with them when they are unvoiced. Lastly, they normally have short durations. We will show how these cues can be advantageously exploited to mitigate the effects of consonant “noise.”
3) Smearing
In our experiments, and also those in [ 9 ], there are a number of individual cases where a high speaking rate sometimes results in smearing neighboring energy peaks. This makes it particularly difficult to derive the correct number of syllables for that segment.
Fig. 3 shows an example of smearing of syllables “in” and “tro” (from the word “introduction”) showing only one peak. The possible reasons include effects of windowing used in the analysis and any smoothing of the envelope in a post processing step.

Illustration of peak smearing shown for the word “in-tro” (from the Switchboard corpus).
4) Overestimation Issues
It is also observed that for some slow segments, people tend to shift the vowel formant to express some prosodic content. Such phenomena will bring extra peak estimates in the direct application of the subband correlation method as proposed in [ 9 ].
In the example shown in Fig. 4 , “so” has only one syllable. With a fixed subband, when a formant shifts from one band to another, it will generate an additional peak.

Overestimation for “So” (from Switchboard).
5) Windowing Effect
In all these methods, a correlation envelope is generated and utilized. Like all short-time windowing methods, a larger window makes the envelope smoother but loses fine details. A smaller window provides more detail but makes the envelope noisy and in turn renders peak counting difficult. In the syllable scale we are considering, such windowing effects are unavoidable. We will address this problem by Gaussian filtering.
The aforementioned challenges are addressed in the four steps of the proposed method, as described below: spectral processing, temporal processing, overall smoothing, and thresholding.
The overall system flow chart is shown in Fig. 9 .

System flowchart for speech rate estimation.
B. Spectral Processing
1) selected subband correlation.
We believe formant structure is the major key to identifying vowels and thus locating the syllable nucleus. Our algorithm aims to abstract the speech waveform to a 1-D envelope, with a general strategy to let the center of the vowel to be maximized while not significantly increasing the contribution to the envelope from the consonants. As a consequence, the neighboring syllables (vowel centric) should have a deeper gap in between. The subband correlation addresses this issue. We wish to further improve its performance by doing a selected subband correlation.
In all previous approaches, spectral correlation is performed on the full bands. However, we find that if we concentrate on the prominent subbands where the formant structure lies, the vowel segments could be further boosted while the consonant contribution will be diminished comparatively. Such discrimination increase will be useful for later threshold setting. So we propose to do spectral correlation only on a selected subset of the subbands. First, instead of choosing only four subbands, we apply a 19 subband analysis (by a facility provided in the speech filing system tool [ 14 ]). We then keep the top M bands by subband energy for further temporal and spectral processing. M is a parameter we need to set appropriately and will be discussed in a later section.
In the example shown in Fig. 4 , slow speech incurs an overestimation of syllable number, and we noticed that the formant structure has shifted within the vowel segment. In this case, if we select the top M most prominent subbands to do correlation, the shifting effects could be automatically tracked and resolved.
2) Pitch Verification
In the previous section (Section IV-A), we outlined the characteristics of background noise and consonant noise. Typically, such regions do not have any voicing. The availability of pitch information could serve to identify this effect. Pitch estimation is a fairly mature signal processing technique and can be easily implemented using a variety of approaches. The use of pitch in conjunction with the correlation envelope could help eliminate the pseudopeaks where there is no pitch. In this paper, we apply the pitch estimation algorithm that is based on normalized cross correlation function and dynamic programming. It is similar to that as presented in [ 46 ]. Such an approach was found to be very effective as shown in the later evaluation section.
C. Temporal Processing
Few previous approaches incorporate temporal processing. However, we note that each landmark lasts over some period of time. For example, vowels and sonorant consonants which constitute the major body of a syllable extend over several tens of milliseconds. Silence and nonsonorant consonant sounds can also cause signal discontinuity in the temporal realm (consonant discontinuities are typically shorter). Temporal processing, aimed at achieving desirable smoothing effects, is carried out as described below.
1) Temporal Correlation
Inspired by spectral cross correlation, and also by the fact that each syllable (i.e., similar spectral pattern) typically lasts over several tens of milliseconds, we also perform a cross correlation in time domain.
Let x t , x t +1 , … , x t + K −1 represent an increasing time order of subband energy vectors with length K . We then compute correlation y t as
Through this correlation, each syllable has a peak at its center, because it spans most of the part of this syllable.
It also could be viewed as a type of filtering. However, compared to linear weighting of neighboring frames, the above approach uses products which will boost within-syllable frame similarities. This approach was found to effectively address the windowing effect of the envelope. The parameter we need to set here is K , the size of the window to do the correlation.
2) Weighting Window
Fig. 3 illustrates the case where fast speech has a smearing effect on neighboring syllables. Even though the major purpose of our algorithm is to smooth the correlation envelope, we do not want to lose important details in the process. In order to emphasize intersyllable discontinuities, we apply a Gaussian weighting window centered at the middle of the analysis frame before the process of self temporal correlation (as described in Section IV-CI). So the center part, in the case there is a small discontinuity, is amplified, and this frame has more weight in the correlation process. Such an approach could be mathematically described as follows.
Let w 0 , w 1 , … , w K −1 represent a series of window coefficients. We first perform a weighting operation on the subband energy temporal vector series x 0 , x 1 , … , x k −1
Here, we choose w to be a Gaussian window centered in the middle of the analysis segment. After this process, we plug in the updated vector series x 0 , x 1 , … , x k −1 to the temporal correlation process as described in Section IV-C1. We need to set the variance of the Gaussian window appropriately to control the shape of the window.
In order to illustrate the effects of such weighting window, we study the discontinuities of the step function in the 1-D case, and show the results in Fig. 5 . (The temporal correlation in Section IV-C1 is for M -dimensional vectors where M is the number of selected subbands.)

Weighting window effects for step functions. Correlation window length is set to 11, and the variance of Gaussian is 1.2.
The original step signal has the sharpest edge. The effect of the weighted windowing, as can be seen in Fig. 5 , is to help reach an acceptable tradeoff between amplifying the discontinuity while achieving the desirable smoothing effect suitable for rate detection. These parameters used in correlation and weighting the window are selected as optimal settings for the experiments in Section V where we will further discuss the implications of this algorithm.
It also needs to be mentioned that there are many possible filter selections to achieve similar smoothing effects. Gaussian windows offer desirable kernel characteristics and easy parametric control of their shape, and are widely popular in image processing for smoothing [ 47 ]. Also, both the Fourier transform and the derivative of a Gaussian window are Gaussian functions. We hence adopt the 1-D Gaussian window for our case.
D. Smoothing
After the spectral and temporal correlation, we obtain a 1-D correlation envelope. There still may be local peaks in the correlation envelope which result in spurious peak counts. As a result, some type of further smoothing becomes necessary. We apply the standard Gaussian filtering method. The parameter setting strategy for the filter is described in Section IV-F.
It needs to be clarified that our algorithm has two different Gaussian windows involved with different intended use. While the purpose of the one described in Section IV-C is to alleviate the smoothing effects of the temporal correlation by making the slope sharper, the purpose of the one in Section IV-D is purely to provide a low-pass smoothing filter.
E. Threshold Mechanism
In addition to smoothing, for handling spurious peaks in the correlation envelope, we could design further thresholding mechanisms to improve the overall robustness of the peak counting. Based on empirical analysis on several speech correlation envelopes, we categorized the observed spurious peaks into 2 two classes: First are those that occur when there is no voicing activity. We proposed in Section IV-BII to use pitch verification as a hard threshold where all peaks with no corresponding pitch activity are removed. However, there are limitations to pitch verification such as when there are voiced consonants, cross-channel voice, or pitch computation error. The major characteristic of such noisy peaks is that they are of relatively low amplitude. Such peaks could be removed by appropriate thresholding. The second class of noisy peaks appears in the voiced part. In this case, neither pitch verification nor absolute thresholds would be effective since those regions always have nonzero pitch, and the noisy peaks are of quite high amplitude. Most algorithms in Sections IV-A–D try to address this issue to some extent. As an additional step, we design a threshold mechanism which could deal with pseudovoiced peaks specifically.
1) Temporal and Magnitude Thresholds
To counter pseudopeaks that occur close in time, first, we set a threshold for the minimum distance in time between two neighboring peaks. The simple idea here is that two syllables could not be very close in the final correlation envelope with respect to the frame advance of 10 ms. Second, we still need to set thresholds on magnitude.
Fig. 6 illustrates a case where a single syllable displays two peaks (marked peak A and peak B) in the final correlation envelope. We propose to measure the minimum difference between a local peak and its larger neighboring minima instead of the ground zero, for setting temporal thresholds. For example, in Fig. 6 , the threshold magnitude of peak A is measured by the relative magnitude between A and C; similarly, for peak B it is measured between B and C. This method however could fail to report any peaks in specific cases such as in Fig. 6 (Since the relative magnitudes of peak A and peak B are all very small). Instead, we found that a modification that considers the magnitude of a peak with respect to its immediate preceding minimum to be more robust. This was based on observations about typical syllable-level acoustic characteristics that demonstrate larger ranges between neighboring syllables, i.e., high absolute magnitude (such as A or B) at the syllable and rather low absolute magnitude between the neighboring syllables (such as D, E). On the other hand, spurious peaks tend to have smaller ranges. Hence, in the new scheme, for example in Fig. 6 , peak A’s threshold magnitude is measured by the relative magnitude difference between A and D. Peak B’s threshold magnitude is measured by the relative magnitude difference between B and C. Peak A could thus pass the threshold since it is rather high in such magnitude. So, it returns the correct peak number.

Syllable “BAD” in Switchboard 3994B.
This scheme could also handle many other cases very well. In the case that A–D and B–C are very close and high, this most probably implies that they are two distinct syllables and the algorithm will keep both. If A–D and B–C are both of small magnitudes, considering D has low absolute magnitude, they are both removed as background noise. The other advantage is that this left-compare-only threshold is compatible with absolute thresholding: When we apply it on silence regions, this method works the same as absolute threshold.
It should be noted that there is potential failure possibility of this threshold mechanism in the case of very close syllables with no discernable boundaries such as in the words “reenter,” and “reenergize” which may appear as pseudovoiced peaks in fast speech. Nevertheless, overall, we expect that these cases to be relatively infrequent, and that the proposed threshold mechanism would be in general effective.
F. Parameter Selection
The previous sections described many approaches for improving the syllable detection performance robustness. One critical question that still needs to be answered is how to choose the different algorithm parameters to enable the various processing blocks to work well together. The manual heuristic method has its own merits in that it utilizes expert human knowledge for rapid parameter setting. This is especially useful when a single running cycle (even on development test set) is computationally intensive. However, the approach suffers from limitations of scalability. For instance, many iterations of tuning may be needed, and it may be difficult to tell when the algorithm reaches a local maximum or if we could find the global maximum. Furthermore, such an approach would be difficult to easily port to other data types and domains. Hence, we propose to use a principled way for parameter estimation relying on Monte Carlo-based initialization followed by a sensitivity analysis to set the parameters using a development set.
1) Monte Carlo Method
The algorithm we have proposed for speech rate estimation poses a multidimensional parameter setting problem. We adopt the Monte Carlo method to bootstrap the parameter value initialization. The first step is generating the possible ranges for the parameter values. We specify these initial ranges rather large (greedily) and then generate the parameter set by Monte Carlo sampling. Fig. 7 illustrates the sample histogram after 4446 runs on the development set. The algorithm’s performance with the selected parameters is then noted. The large initial parameter set requires that a large number of random parameter samples be generated in order to reach the optimal region, a computationally intensive process. We made this possible by optimizing the batch operation and offline front-end processing. Since Monte Carlo simulation draws parameters randomly within a large range, it is an important step towards detecting the global maxima. Though with a given number of simulations, we cannot guarantee to find the global maxima, we believe it at least provides an acceptable approximation to it.

Monte Carlo simulation histogram.
2) Sensitivity Analysis
The chosen parameter values were then subjected to a sensitivity analysis. This was done through systematic perturbations to the parameter values (obtained from the Monte Carlo simulation) until a local maximum is reached. We first define an “atomic increment,” which specifies the smallest amount by which each parameter could change. We then perturb each parameter one by one with the atomic increment in each direction. Every time there is an improvement, we will update the relative parameter. This step is repeated until no further improvement is obtained for perturbations on all parameters.
In Fig. 8 , the X -axis shows the number of the perturbation trials. This number starts from 0 and increases by the aforementioned procedure. The Y -axis shows the correlation coefficient between speech rate estimates obtained from the test and reference data in the development set. The correlation coefficient is an indicator of speech rate estimation accuracy. Fig. 7 then illustrates how such perturbations could monotonically improve the performance. We found for fast convergence, the Monte Carlo method is essential to obtain a good rough estimate of the starting point. The sensitivity analysis is designed in such a way to efficiently but exhaustively search the parameter space to scan all possible local maxima in the given range.

Perturbation yields monotonic improvement on correlation coefficient between test and reference data.
V. System Description and Experimental Results
Given the description of the various components of our algorithm in Section IV, we will now describe the full system and report the evaluation results.
The overall speech rate estimation system is summarized in Fig. 9 . Each block therein was described in Section IV. The algorithm parameters are set systematically and automatically using the Monte Carlo simulation and sensitivity analysis described in the previous section.
The technical specification of each functional component is described below in order.
- The speech is passed through a 19-channel filter bank analyzer to get the energy vector series. We apply the utility “voc19” as provided by [ 14 ]. It is a straightforward implementation of a 19-channel filterbank analyzer using two second-order section Butterworth bandpass filters spaced as in [ 22 ]. Energy smoothing is done at 50 Hz to give a default 100-Hz frame rate. Here we do not apply any energy compression procedures as in [ 9 ].
- With such a 19-channel filter bank, we get a 19 stream subband energy series. Only the top bands are selected and kept.
- Then we choose K temporal frames. These K frames are weighted by a Gaussian Window as described in Section IV-C2. Temporal correlation is then applied as detailed in Section IV-C1. The overlap across successive Gaussian windows is K−1 frames.
- For the next step, the resulting subband energy vector is cross-correlated in a way identical to [ 9 ].
- Finally, peak counting is performed on the final smoothed envelope with pitch validation and various thresholding schemes as in Section IV.
In order to set the parameters, we randomly selected 568 speech spurts from the full ICSI Switchboard data set as the development set which represents about 10% of the data. Applying the Monte Carlo simulation and sensitivity analysis, we obtained the parameter values as listed in Table I .
Optimal Parameter Settings
While this is a multiparameter tuning problem, it is also desirable to understand the effect of the individual parameters. To experimentally obtain insights in this regards, we evaluated the performance by removing each of the proposed component and measured the resulting performance on the development test set. Following methods in [ 9 ], a transcribed syllable rate was computed by dividing the number of syllables occurring in the spurts by the length of the spurt. In this paper, we treat this rate as the reference rate. We use the detected rate to correlate with the reference rate to get the final agreement measure on the data set. We also computed the simple mean squared error (MSE) between the estimated and reference rates as follows:
The results are reported in Table II .
Experiments Run on the Development Set With Specific Components Removed From the Full System
All the components appear to provide improvements in the performance, but to varying degrees: Results show pitch validation to be the most effective, with thresholding strategies also contributing significantly on this data set. The use of reduced, instead of full, number of bands improves the error variance without degrading (in fact, slightly improving) the correlation rate and MSE, but with obvious reduced computation.
While interpreting the results of Table II , we should note that the algorithm was designed to have several mutually dependent components working together to locate the syllable nucleus correctly. As motivated in Section IV, each component attempts to address specific issues in rate estimation, and the Monte Carlo approach enabled us determine a compromise optimum of these parameters. Hence, the method of evaluating relative performances by turning off components with respect to a jointly tuned parameter set may not necessarily assure optimal settings for the remaining components. The only exception to this might be the pitch validation component. Since the computation of pitch is independent of all other components, its contribution is most likely also largely independent of the other modules. Table II shows that the performance degradation by turning this option off is the most significant. This implies that it could remove the effects of background and consonant noise (Section IV-B2) which are difficult to be mitigated by other components. The results also suggest that the pseudopeaks removed by pitch validation constitute a significant portion of the impediment to accurate rate estimation.
The results of Table II also indicate that the thresholding schemes contribute noticeably to the system performance. However, the contributions do not come just from the “threshold” selection but from the effects of other signal processing components that help isolate the “noise” that is then easily removable by thresholding. For example, subband correlation helps to boost the contribution of vowel and other sonorants while suppressing the intersyllable valleys. This makes the margins between true peaks and pseudopeaks accentuated, which in turn facilitates the thresholding schemes to work robustly.
Temporal correlation and Gaussian filtering both try to achieve the same goal of smoothing the syllable envelope. Table II shows that they contribute similarly to the overall system. We believe that the joint parameter setting with Monte Carlo approach would set these two subsystems to work optimally with the thresholding scheme. In sum, the experiment of studying the effects of the various components shows their relative importance, although it is understood their settings in this process may not be entirely optimal.
In the next step, we proceeded with the evaluation of the full system with all the available Switchboard data and the parameter settings obtained from the Monte Carlo simulation and sensitivity analysis, again following the methods as reported in [ 9 ]. We use the detected rate to correlate with the reference rate to get the final agreement measure on the full 5682 spurts set. Also, the mean error and standard deviation error were calculated. The results are reported in Table III . This result represents about 17% improvement compared to a single estimator and 11% improvement with respect to a multiestimator evaluated on the same database in [ 9 ].
Experimental Results Note: Enrate, Sub-Mrate, and Mrate are the Results From [ 9 ]
Also, instead of using all of the switchboard data and removing just the development part, the correlation coefficient is 0.734, which is slightly lower than the results in Table III .
In addition, we analyzed the influence of certain factors on the estimation of speech rate. In Section II-B, we noted that besides vowels, sonorant segments of syllable nuclei might include glides, liquids, and nasals. In Table IV , we report results for the two cases separately: speech spurts which have at least one syllable with glides/liquids/nasals as the sonorant elements, while the other class consists of spurts with only vowels as syllable nucleus. Results show that the inclusion of sonorant consonants is handled well by the algorithm.
Effects of Syllable Nucleus Type on Speech Rate Estimation
We also investigated the effect of the actual value of the speech rate itself. For that purpose, we heuristically categorized the speech data into three classes based on transcribed speech rate: fast (> 5 syllables/s, 711 spurts), normal (between 3 and 5 syllables/s, 3405 spurts), and slow (< 3 syllables/s, 1566 spurts). The estimated and reference values are shown in Fig. 10 for each of these data conditions. In general, the estimated values tend to be underestimates, with greater disagreements in the case of slow and fast speech (second and fourth panels in Fig. 10 ). We calculated the mean squared error between the reference and estimated values for each of these cases: the overall MSE rate was 5%, while the rates for slow, normal, and fast cases were 10.3%, 3.5%, and 6.8%, respectively. The major cause of this effect is due to two factors: overestimation and smearing, which occur often in slow and fast speech, respectively. (Refer to Sections IV-A3 and A4).

Estimated and reference rates for various data conditions (reference, blue; estimated, red). The top panel correspond to the results for the entire data, while the second, third, and last panels in the figure correspond to slow, normal, and fast speech, respectively. The horizontal axis is the ID of the spurts; the vertical axis is the MSE.
It needs to be clarified that the number of syllables per utterance might be an ill-defined quantity. Even though we use the normalized syllables per second as the rate measure, this quantity might not keep constant as the spurts length is varied. This should be taken into consideration for the justification in Fig. 10 .
Lastly, we wish to explore yet another property of our algorithm. Throughout this work, we have been assuming that the peak number is a valid indication of the syllable number. It assumes that the peak location on the correlation envelope should be consistent with the syllable location. Even though this is not part of the work of Fosler-Lussier and Morgan [ 9 ], and it might not be a necessary condition to make our algorithm work, we include these statistics for closer analysis. For this purpose, we treat the original syllable transcription in the ICSI Switchboard corpus subset as a “gold standard.” Then, we compare the peak location on the correlation envelope to this standard. If within a syllable, there is a one-to-one mapping, we treat this as “correct.” Otherwise, it is deemed as a “deletion” or “insertion.” The statistics are provided in Table V .
Comparison to the Transcribed Syllable Location
For a spontaneous speech corpus like Switchboard, more than 80% of the time the syllable gives a one-to-one mapping. As stated in Section IV-A, our algorithm has deletion and insertion errors under specific circumstances. Even though slow speech rate is slightly more difficult to estimate (as illustrated in Fig. 10 ), due to the preponderance of the number of fast-spoken syllables relative to the slow-spoken ones in the data, deletion errors dominate insertion errors.
It should be noted our algorithm is optimized towards improving the speech rate correlation between a reference and the measured, and it might not necessarily produce the optimal syllable location information. One reason, as discussed in Fig. 10 , is the ill-defined nature of syllables per utterance as a rate indicator.
VI. Summary and Conclusion
Our experiments show that the speech rate estimation methods proposed in this paper offer further improvements over previous methods. Such advantages are demonstrated by improved correlation coefficients and reduced mean error and standard deviation in the estimates with respect to the reference values. We have also systematized the heuristic parameter setting methodology originally used in [ 18 ]. The Monte Carlo method and dynamic parameter perturbation schemes provide ways for parameter tuning that guarantee finding the local maximum and approximating the global maximum. For the Monte Carlo method, the coverage is large, but the precision is low. Local convergence is achieved in postprocessing through sensitivity analysis implemented through systematic parameter perturbations. Such a dynamic perturbation scheme could help find the neighboring local maxima but cannot guarantee to enumerate all the local maxima.
The key part of the algorithm is in obtaining the correlation envelope. Such a signal envelope measure could disclose other useful information like syllable duration and spectrum intensity. For example, in [ 45 ], this envelope was used to derive a measure for word prominence.
There are further avenues that can be considered for improving the methods presented in this paper. For instance, it is well known that there are a number of factors that could affect the phonetic characteristics of a syllable (duration, f0), notably the underlying linguistic prosodic structure, which can impact the syllable detection accuracy, a critical aspect of the speech rate measure proposed in this paper. Specifically, lengthening at the edges of prosodic domains (boundaries) has been well documented both in read speech [ 49 ] as well as in spontaneous speech [ 48 ]. This includes the effect of utterance position: initial words are longer than noninitial words; utterance final words are longer than utterance medial words. These in turn can influence the quality of automatic syllable detection that relies on the acoustic characteristics of the syllable. Explicitly incorporating contextual information, such as the temporal structure, can further improve the proposed algorithm.
A possible alternative would be designing an adaptive algorithm for dynamic parameter adjustment such as through multipass rate estimation. For example, the first pass can give a rough estimate of the rate, while the second pass can use the results of the first pass to set relative parameters. Such an approach could be implemented iteratively. However, in applications of rate estimation that require real time processing, such multiple-pass methods may drastically limit the usefulness of rate estimation.
We described different types of noises which could render the syllable correlation envelope peak counting in the prone to error. Due to different characteristics of these noises, there is no one universal method to deal with all of them well. The approach we described in this paper was to design several different components, each addressing a specific subset of noise types. Finally, we tune the parameters and thresholds jointly to make these components work optimally through systematic multiparameter tuning. While removing a particular component from the system provided some insights into its relative effect on performance, such an approach does not ensure that the values of the other parameters are necessarily optimal. Further detailed experiments can help shed further light onto such details.
Evaluating the role of the estimates of speech rate derived in this work within specific application frameworks is outside the scope of the present work. Rate sensitive modeling in automatic speech recognition has been shown to provide performance improvements [ 54 ], and we expect that improved rate estimation to contribute toward improvement such models. Similarly, the results of the present work can contribute to other spoken language processing domains. In related work [ 45 ], acoustic measures of word prominence were shown to benefit from the algorithms presented in this paper. Further detailed application-specific evaluations of the proposed rate estimation remain as topics of future work.
Acknowledgments
This work was supported in part by grants from the Office of Naval Research (ONR), the National Science Foundation (NSF), and the U.S. Army.
Dagen Wang received the B.S. and M.S. degrees in electrical engineering from Peking University, Beijing, China, in 1997 and 2000, respectively, and the Ph.D degree in electrical engineering from the University of Southern California (USC), Los Angeles, in 2006.
He was with the Intel China Research Center, Beijing, China. He is now a Speech Scientist at the IBM T. J. Watson Research Center, Yorktown Heights, NY. His current research interest is on speech recognition and translation on limited-resource platforms. His general research interests include signal processing and artificial intelligence with applications to speech, language, and human–computer interaction problems.
Shrikanth S. Narayanan (S’88–M’95–SM’02) received the Ph.D. degree from the University of California, Los Angeles, in 1995.
He is Andrew J. Viterbi Professor of Engineering at the University of Southern California (USC), Los Angeles, where he holds appointments as Professor in electrical engineering and jointly in computer science, linguistics, and psychology. Prior to joining USC, he was with AT&T Bell Labs and AT&T Research, first as a Senior Member, and later as a Principal Member of its Technical Staff from 1995–2000. At USC, he is a member of the Signal and Image Processing Institute and a Research Area Director of the Integrated Media Systems Center, an NSF Engineering Research Center. He has published over 235 papers and has 14 granted/pending U.S. patents.
Dr. Narayanan is a recipient of an NSF CAREER Award, USC Engineering Junior Research Award, USC Electrical Engineering Northrop Grumman Research Award, a Provost Fellowship from the USC Center for Interdisciplinary Research, a Mellon Award for Excellence in Mentoring, and a recipient of a 2005 Best Paper Award from the IEEE Signal Processing Society. Papers by his students have won best student paper awards at ICSLP’02, ICASSP’05, and MMSP’06. He is an Editor for the Computer Speech and Language Journal (2007-present) and an Associate Editor for the IEEE Signal Processing Magazine . He was also an Associate Editor of the IEEE T ransactions on S peech and A udio P rocessing (2000–2004). He serves on the Speech Processing and Multimedia Signal Processing technical committees of the IEEE Signal Processing Society and the Speech Communication Committee of the Acoustical Society of America. He is a Fellow of the Acoustical Society of America and a member of Tau Beta Pi, Phi Kappa Phi, and Eta Kappa Nu.
Contributor Information
Dagen Wang, Viterbi School of Engineering, University of Southern California (USC), Los Angeles, CA 90007 USA. He is now with the IBM T. J. Watson Research Center, Yorktown Heights, NY 10598 USA.
Shrikanth S. Narayanan, Viterbi School of Engineering, University of Southern California (USC), Los Angeles, CA 90007 USA.
- Look up in Linguee
- Suggest as a translation of "rates"
Linguee Apps
▾ dictionary english-french, rates noun, plural ( singular: rate ) —, taux pl m (almost always used), rate noun —, taux m ( plural: taux m ), tarif m ( plural: tarifs m ), rythme m ( plural: rythmes m ), rate ( sb./sth. ) verb ( rated , rated ) —, évaluer qqch./qqn. v, rate noun as adjective —, tarifaire adj, exchange rate n —, freight rates pl —, mortgage rates pl —, competitive rates pl —, rate of growth n —, affordable rates pl —, different rates pl —, rates of growth pl —, short-term rates pl —, advertisement rates pl —, rooms rates pl —, exchange rates pl —, growth rates pl —, daily rates pl —, special rates pl —, attendance rates pl —, vat rates pl —, current rates pl —, room rates pl —, discounted rates pl —, suicide rates pl —, wage rates pl —, transformation rates pl —, dropout rates pl —, graduation rates pl —, closing rates pl —, depreciation rates pl —, remuneration rates pl —, sickness rates pl —, market rates pl —, scrap rates pl —, world rates pl —, completion rate n —, rate of change n —, production rate n —, refresh rate n —, cure rate n —, lease rate n —, cap rate n —, basal metabolic rate n —, higher rate n —, sink rate n —, seed rate n —, uniform rate n —, flat-rate contribution n —, ordinary rate n —, rate package n —, kilometre rate be n —, flat-rate quantity n —, development rate n —, reproduction rate n —, commencing rate n —, ▾ external sources (not reviewed).
- This is not a good example for the translation above.
- The wrong words are highlighted.
- It does not match my search.
- It should not be summed up with the orange entries
- The translation is wrong or of bad quality.
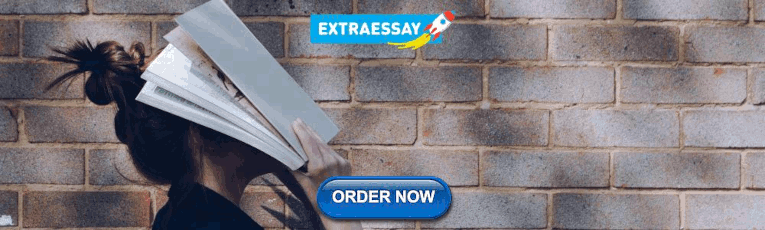
IMAGES
VIDEO
COMMENTS
speech n: uncountable (pronunciation) élocution nf : articulation nf : People tend to have slurred speech after a few beers. Après quelques bières, l'élocution des gens est moins bonne. Après quelques bières, l'articulation des gens est moins bonne. speech n: uncountable (way of speaking) langage nm : Most young people's speech is full of ...
The speech rate is the speed at which you talk.: Le débit de parole, c'est la vitesse à laquelle vous parlez.: The first one, speech rate acceleration, reduces most directly the absolute vowel duration. L'une, l'accélération du débit de parole réduit le plus directement la durée absolue des voyelles.: 7 steps to getting used to the speech rate of Spanish language
The speech rate is the speed at which you talk. Le débit de parole, c'est la vitesse à laquelle vous parlez. The first one, speech rate acceleration, reduces most directly the absolute vowel duration. L'une, l'accélération du débit de parole réduit le plus directement la durée absolue des voyelles. I thought I had a high speech rate, but ...
speech rate translation in English - French Reverso dictionary, see also 'acceptance speech, direct speech, free speech, indirect speech', examples, definition, conjugation
To select the default speech engine, speech rate, or to specify your default settings, go to Text-to-speech settings as described above. Pour sélectionner le moteur de reconnaissance vocale par défaut, la vitesse d'élocution , ou pour spécifier vos paramètres par défaut, aller à Text-to-speech paramètres comme décrit ci-dessus.
If you talk too fast, the person, you might lose the person you're pitching to. The accepted ideal speechrate is 140-160 words per minute. You must think about getting the facts to your listeners. If they can't comprehend what you're saying due to speaking fast, they will most likely lose interest in your speech.
discours m (plural: discours m) The president gave a speech during the meeting. Le président a prononcé un discours pendant la réunion. My speech was appropriate to the occasion. Mon discours était approprié à l'occasion.
Speech rate is the speed of one's articulation, including usually the pause time between sentences or between thought groups. There are several means for measuring speech rate; the most popular means calculates total words or syllables uttered per minute. However, determining a normal speech rate for a language can be rather complicated because ...
La définition de Speech dans le dictionnaire français de TV5MONDE. Découvrez également les synonymes de speech sur TV5MONDE. Aller au contenu principal ... FR, EN, ES; TV5MONDE Asie/Pacifique FR, EN; TV5MONDE Etats-Unis EN; TV5MONDE Europe FR, EN, NL, DE, RO; TV5MONDE Maghreb-Orient ...
Rate of speech, also known as speaking rate or tempo, refers to the speed at which you speak, measured in words per minute (wpm). It plays a crucial role in effective communication, impacting comprehension, engagement, and overall delivery in various contexts, including public speaking. While speechcoachescan offer personalized guidance, public ...
Slow speech is usually regarded as less than 110 wpm, or words per minute. Conversational speech generally falls between 120 wpm at the slow end, to 160 - 200 wpm in the fast range. People who read books for radio or podcasts are often asked to speak at 150-160 wpm. Auctioneers or commentators who practice speed speech are usually in the 250 to ...
According to The National Center for Voice and Speech, the average speaking rate for English speakers in the U.S. is around 150 words per minute. In a public speaking situation, you'll want to speak slower than average, around 125-150 words per minute. One of the ways to control your rate of speech is to make sure you are taking enough breaths.
Google's service, offered free of charge, instantly translates words, phrases, and web pages between English and over 100 other languages.
Speech rate has long been recognized as an important factor in an individual's ability to communicate a message in an effective manner. In fact, speech rate is a common target in speech intervention to improve speech production in individuals with speech motor involvement. As a result, a primary focus of the speech rate literature has been to ...
SPEECH définition, signification, ce qu'est SPEECH: 1. the ability to talk, the activity of talking, or a piece of spoken language: 2. the way a…. En savoir plus.
Speech rate is primarily dependent on two factors: speaking style and the nature/scenario of speech production (e.g., scripted/spontaneous). ... before and after that center (…) there will be greater obstruction to airflow and/or less loud sound." This definition allows for a plausible way for detecting syllables in speech. Intuitively ...
speech therapist ( speech therapists plural ) A speech therapist is a person whose job is to help people to overcome speech and language problems. n-count. speech therapy. Speech therapy is the treatment of people who have speech and language problems. n-uncount. A stammering child can benefit from speech therapy.
cadence f. It is less busy this week and the rate of work is more manageable. Cette semaine est moins chargée et la cadence de travail est plus gérable. less common: valeur f. ·. vitesse f. ·. train m.
It is less busy this week and the rate of work is more manageable. Cette semaine est moins chargée et la cadence de travail est plus gérable. less common: vitesse f. ·. valeur f. ·. train m. "rates" could be 3rd Person Present Tense.
speech rate translation in English - English Reverso dictionary, see also 'figure of speech, speechless, speed, speck', examples, definition, conjugation
reported speech n. (indirect quotation) style indirect nm. Mettez la phrase suivante au style indirect. Un oubli important ? Signalez une erreur ou suggérez une amélioration. 'reported speech' également trouvé dans ces entrées : Dans la description anglaise : indirect speech - would.