Evolution of the Graphics Processing Unit (GPU)
Ieee account.
- Change Username/Password
- Update Address
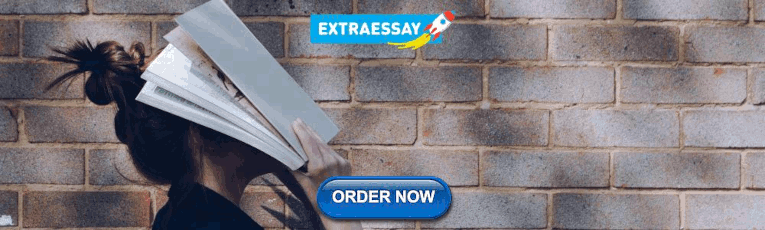
Purchase Details
- Payment Options
- Order History
- View Purchased Documents
Profile Information
- Communications Preferences
- Profession and Education
- Technical Interests
- US & Canada: +1 800 678 4333
- Worldwide: +1 732 981 0060
- Contact & Support
- About IEEE Xplore
- Accessibility
- Terms of Use
- Nondiscrimination Policy
- Privacy & Opting Out of Cookies
A not-for-profit organization, IEEE is the world's largest technical professional organization dedicated to advancing technology for the benefit of humanity. © Copyright 2024 IEEE - All rights reserved. Use of this web site signifies your agreement to the terms and conditions.
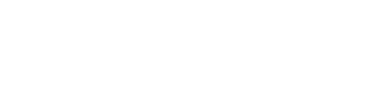
A complete anatomy of a graphics card: Case study of the NVIDIA A100
In our latest blogpost, we shine a spotlight on the Nvidia A100 to take a technical examination of the technology behind them, their components, architecture, and how the innovations within have made them the best tool for deep learning.
2 years ago • 13 min read
Add speed and simplicity to your Machine Learning workflow today
In this article, we'll take a technical examination of the technology behind graphics cards, their components, architecture, and how they relate to machine learning.
The task of a graphics card is very complex, yet its concepts and components are simple to comprehend. We will look at the essential components of a video card and what they accomplish. And at each stage, we'll use the NVIDIA A100 - 40 GB as an example of the current state of the art of graphics cards. The A100 arguably represents the best single GPU available for deep learning on the market.
Graphics card breakdown
A graphics card, often known as a video card, graphics adapter, display card, or display adapter, is a type of expansion card that processes data and produces graphical output. As a result, it's commonly used for video editing, gaming, and 3D rendering. However, it's become the go-to powerhouse for machine learning applications and cryptocurrency mining in recent years. A graphics card accomplishes these highly demanding tasks with the help of the following components:
Graphics Processing Unit (GPU)
Data processing unit (dpu), video memory (vram), video bios (vbios), voltage regulator module (vrm), motherboard interface, interconnection interface.
- Network interface & controller
Output Interfaces
Cooling system.
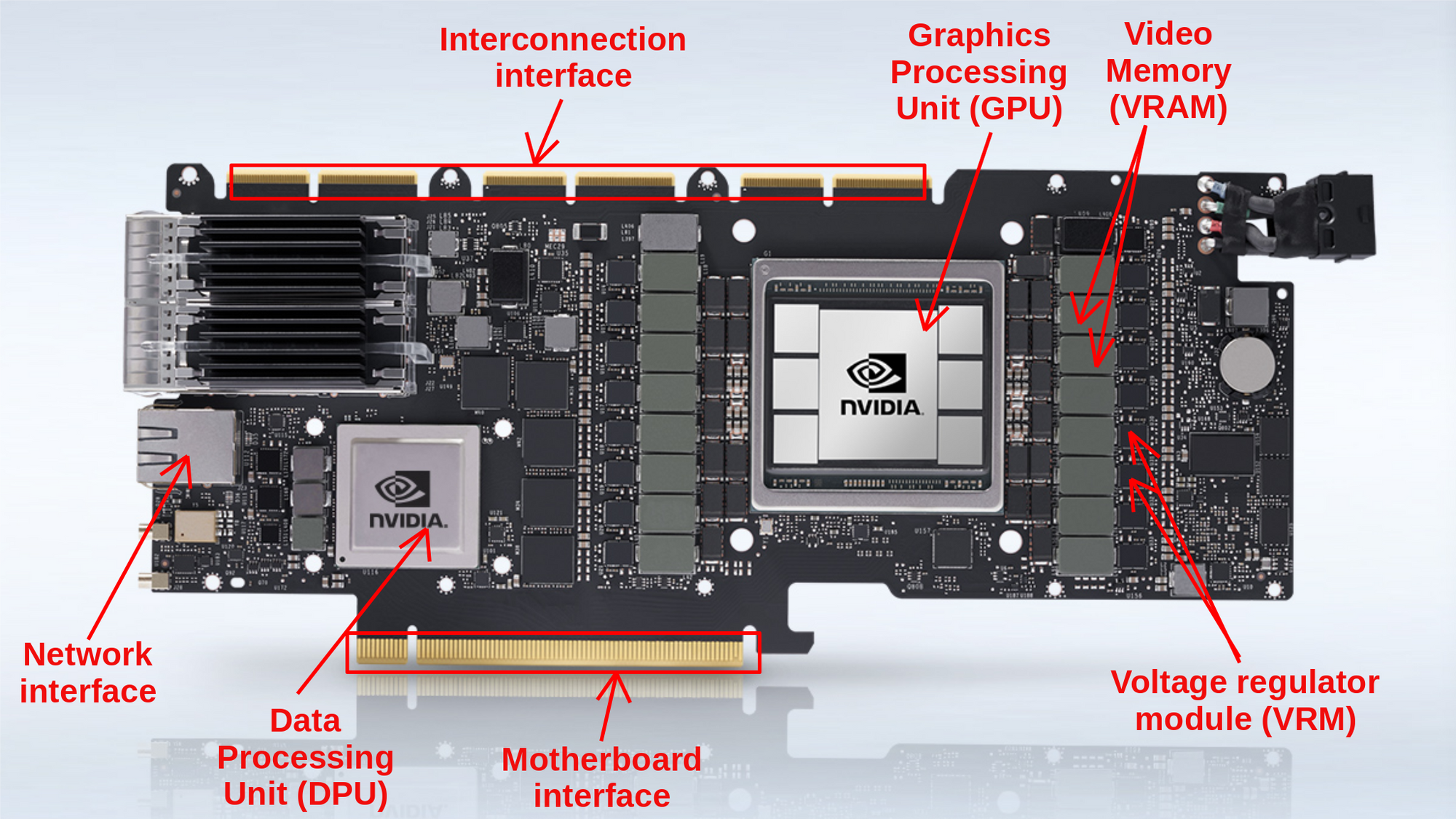
Frequently mistaken for the graphics card itself. The GPU, unlike a computer's CPU, is designed to handle more complex mathematical and geometric calculations required for graphics rendering. GPUs, on average, have more transistors and a larger density of computing cores with more Arithmetic Logic Units (ALU) than a normal CPU.
There are four classifications of these units:
- Streaming Multiprocessors (SMs)
- Load/Store (LD/ST) units
- Special Function Units (SFU)
- Texture Mapping Unit (TMU)
1) A Streaming Multiprocessor (SM) is a type of execution entity that consists of a collection of cores that share a register space, as well as shared memory and an L1 cache. Multiple threads can be simultaneously executed by a core in an SM. When it comes to SM's core, there are two major rivals:
- Compute Unified Device Architecture (CUDA) or Tensor cores by NVIDIA
- Stream Processors by AMD
NVIDIA's CUDA Cores and Tensor Cores, in general, are believed to be more stable and optimized, particularly for machine learning applications. CUDA cores have been present on every Nvidia GPU released in the last decade, but Tensor Cores are a newer addition. Tensor cores are much quicker than CUDA cores at computing. In fact, CUDA cores can only do one operation every clock cycle, but tensor cores can perform several operations per cycle. In terms of accuracy and processing speed, CUDA cores are not as powerful as Tensor cores for machine learning models, but for some applications they are more than enough. As a result, these are the best options for training machine learning models.
The performances of these cores are measured in the FLOPS unit (floating point operations per second). For these measurements, The NVIDIA A100 achieves record breaking values:
According the NVIDIA documentation , using sparsity format for data representation can even help double some of these values.
Inside the A100, cache management is done in a particular way to make data transfer between cores and VRAM as fast and smooth as possible. For this purpose, the A100 GPU has 3 levels of cache L0, L1 and L2:
The L0 instruction cache is private to a single streaming multiprocessor sub-processing block, the L1 instruction cache is private to an SM, and the L2 cache is unified, shared across all SMs, and reserved for both instruction and data. The L2 cache in the A100 is larger than all previous GPU's caches and comes with a size of 40MB and it acts as the bridge between the L1 private cache and the 40GB HBM2 VRAM which we will see in details later in this article.
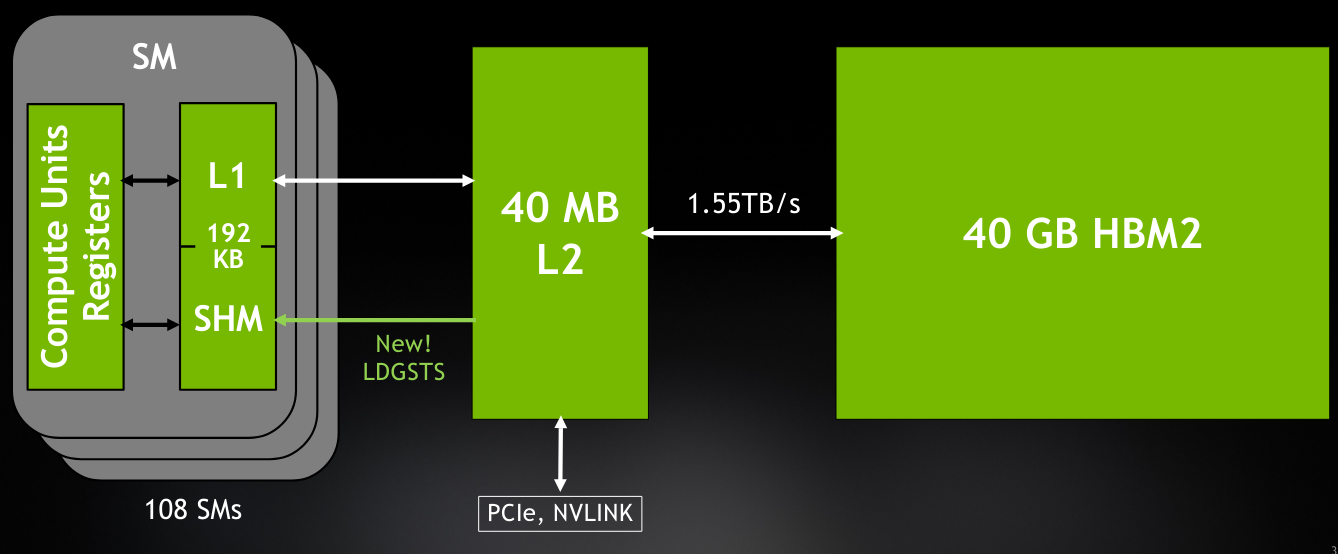
2) Load/Store (LD/ST) units allow threads to perform multiple data loading and storing to memory operations per single clock cycle. In the A100, these unit introduce a new method for asynchronous copy of data, which gives the possibility to load data that can be shared globally between threads without consuming extra thread resources. This newly introduced method offers an increase of around 20% of data loading times between shared memory and local caches.
3) Special Function Units (SFUs) efficiently perform structured arithmetic or mathematical functions on vectored data, – for example sine, cosine, reciprocal, and square root.
4) Texture Mapping Unit (TMU) handles application-specific tasks such image rotation, resizing, adding distortion and noise, and moving 3D plane objects.
The DPU is a non-standard component of the graphics card. Data Processing units are a newly introduced class of programmable processor that joined CPUs and GPUs as the three main components of computing. So, a DPU is a stand-alone processor that is generally implemented in ML and Data centers. It offers a set of accelerated software abilities to manage: networking, storage, security. The A100 graphics card has on board the latest BlueField-2 DPU, which can give great advantages when it comes to handling workloads with massive multiple-input multiple-outputs (MIMO), AI-on-5G deployments, and even more specialized workloads such as signal processing or multi-node training.
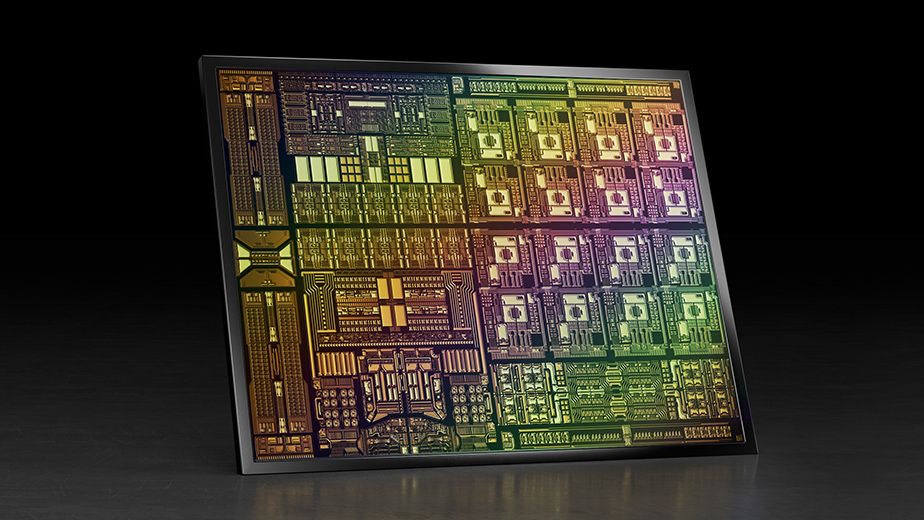
In its broadest definition, video random-access memory (VRAM) is analogous to system RAM. VRAM is a sort of cache utilized by the GPU to hold massive amounts of data required for graphics or other applications. All data saved in VRAM is transitory. Traditional VRAM is frequently much faster than the system RAM. And, more importantly, it's physically close to the GPU. It's directly soldered to the graphics card's PCB. This enables remarkably fast data transport with minimal latency, allowing for high-resolution graphics rendering or deep learning model training.
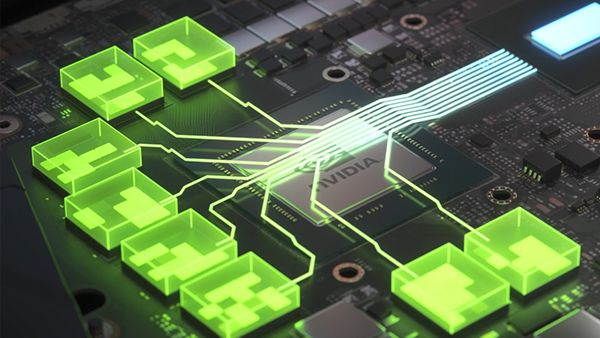
On current graphics cards, VRAM comes in a variety of sizes, speeds, and bus widths. Currently, multiple technologies are implemented; GDDR and HMB have their own respective variations. GDDR (SGRAM Double Data Rate) has been the industry standard for more than a decade. It achieves high clock speeds, but at the expense of physical space and higher than average power consumption. On the other hand, HBM (High Bandwidth Memory) is the state of the art for VRAM technologies. It consumes less power and has the ability to be stacked to increase memory size while taking less real estate on the graphics card. It also allows higher bandwidth and lower clock speeds. The NVIDIA A100 is backed with the latest generation of HBM memories, the HBM2e with a size of 80GB, and a bandwidth up to 1935 GB/s. This is a 73% increase in comparison with the previous version Tesla V100.
It ensures that the GPU receives the necessary power at a constant voltage. A low-quality VRM can create a series of problems, including GPU shutdowns under stress, limited overclocking performances, and even shortened GPU lifespan. The graphics card receives 12 volts of electricity from a modern power supply unit (PSU). GPUs, on the other hand, are sensitive to voltage and cannot sustain that value. This is where the VRM comes into play. It reduces the 12-volt power supply to 1.1 volts before sending it to the GPU cores and memory. The power stage of the A100 with all its VRMs can sustain a power delivery up to 300 Watts.
Using the 8-Pin power connector the A100 receives power from the power supply unit, then forwards the current to the VRMs, that supplies the power to the GPU and DPU, as a 1.1 VDC current, rated at a maximum enforced limit of 300 W and a theoretical limit of 400 W.
This is the sub-component of the graphics card that plugs into the system's motherboard. It is via this interface, or 'slot’, that the graphics card and the computer interchange data and control commands. At the start of the 2000s, many types of interfaces were implemented by different manufacturers: PCI, PCIe, PCI-X or AGP. But, now PCIe has become the go-to interface for mainly all graphics card manufacturers.
PCIe or PCI Express, short for Peripheral Component Interconnect Express, is the most common standardized motherboard interface for connection with graphics cards, hard disk drive, host adapters, SSDs, Wi-Fi and other Ethernet hardware connections.
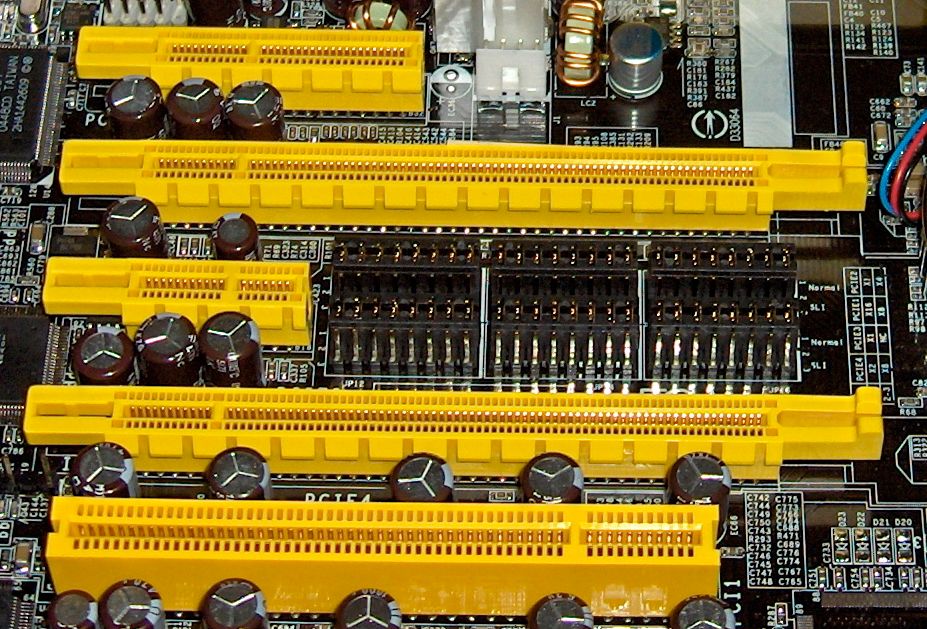
PCIe standards have different generations, and by each generation there is a major increase in speed and bandwidth:
PCIe slots can be implemented in different physical configurations: x1, x4, x8, x16, x32. The number represents how many lanes are implemented in the slot. The more lanes we have the higher bandwidth we can transfer between the graphics card and the motherboard. The NVidia A100 comes with a PCIe 4.0 x16 interface, which is the most performant commercially available generation of the interface.
The interconnection interface is a bus that gives system builders the possibility to connect multiple graphics cards mounted on a single motherboard, to allow scaling of the processing power through multiple cards. This multi-card scaling can be done through the PCIe bus on the motherboard or through a dedicated interconnection interface that acts as a data bridge. AMD and NVIDIA both present their graphics cards with proprietary methods of scaling, AMD with its CrossFireX technology and NVIDIA with its SLI technology. SLI was deprecated during the Turing generation with the introduction of NVLink, which is considered as the top of the line of multi-card scaling technologies.
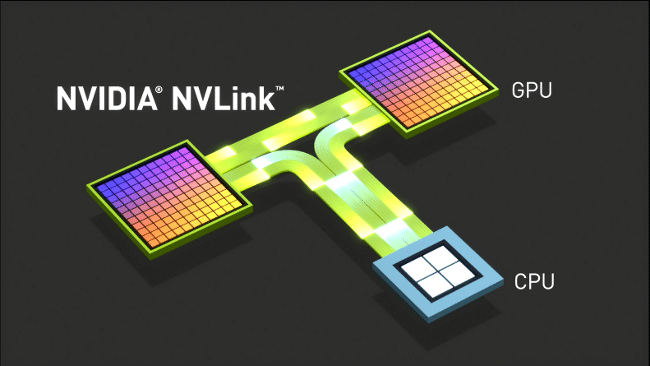
The NVIDIA A100 uses the 3rd generation of NVLink that can offer up to 600 GB/s speed between the two GPUs. Also, it represent a more energy efficient way, than PCI Express, to deliver data between GPUs.
Network interface
The network interface is not a standard component of the graphics card. It's only available for high performance cards that require direct data tunneling to its DPU and GPU. In the case of the A100, the network interface is comprised of 2x 100Gbps Ethernet ports that allows faster processing especially for applications involving AI-based networking.
The output interfaces are the ports that are built on the graphics card and gives it the ability to connect to a monitor. Multiple connection types can be implemented.
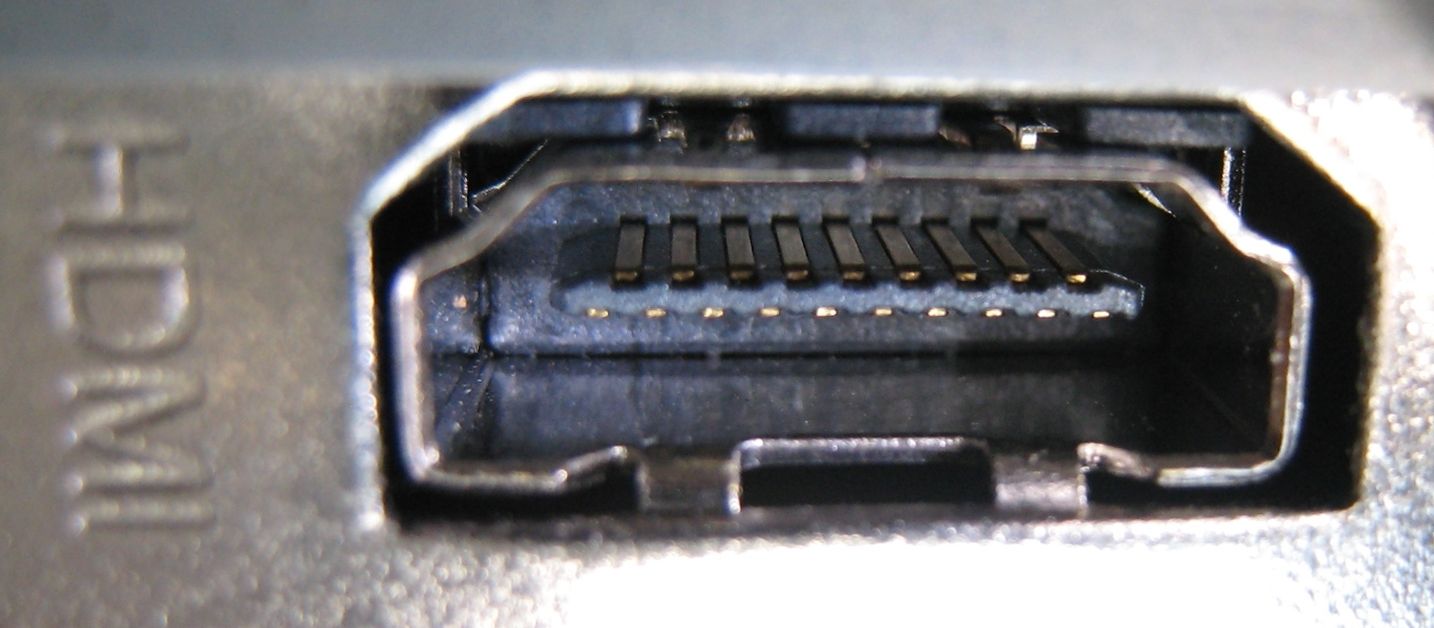
For older systems, VGA and DVI were used, while recently manufacturers tend to use HDMI and Display-Port while some portable systems implement the USB Type-C as the main port.
As for the card under our microscope in this article, the A100 does not have an output interface. Since, it was designed from the start as professional card for ML/DL and use in data centers, so there is no reason for it have a display connectivity.
A video BIOS, often known as VBIOS, is a graphics card's Basic Input Output System (BIOS). The video BIOS, like the system BIOS, provides a set of video-related informations that programs can use to access the graphics card, as well as maintaining vendor-specific settings such as the card name, clock frequencies, VRAM types, voltages, and fan speed control parameters.
The cooling is generally not considered as a part of the graphics card components listing. But, due to its importance it cannot be neglected in this technical deep dive.
Due of the amount of energy consumed by graphics cards, a high amount of thermal energy is generated. And, to keep the performances during the activity of the card and to preserve the long term usability, core temperature values should be limited to ovoid thermal throttling which is the performance reduction due to high temperature at GPU and VRAM level.
For this, two techniques are mainly used: Air cooling and liquid cooling. We'll take a look at the liquid cooling method used by the A100.
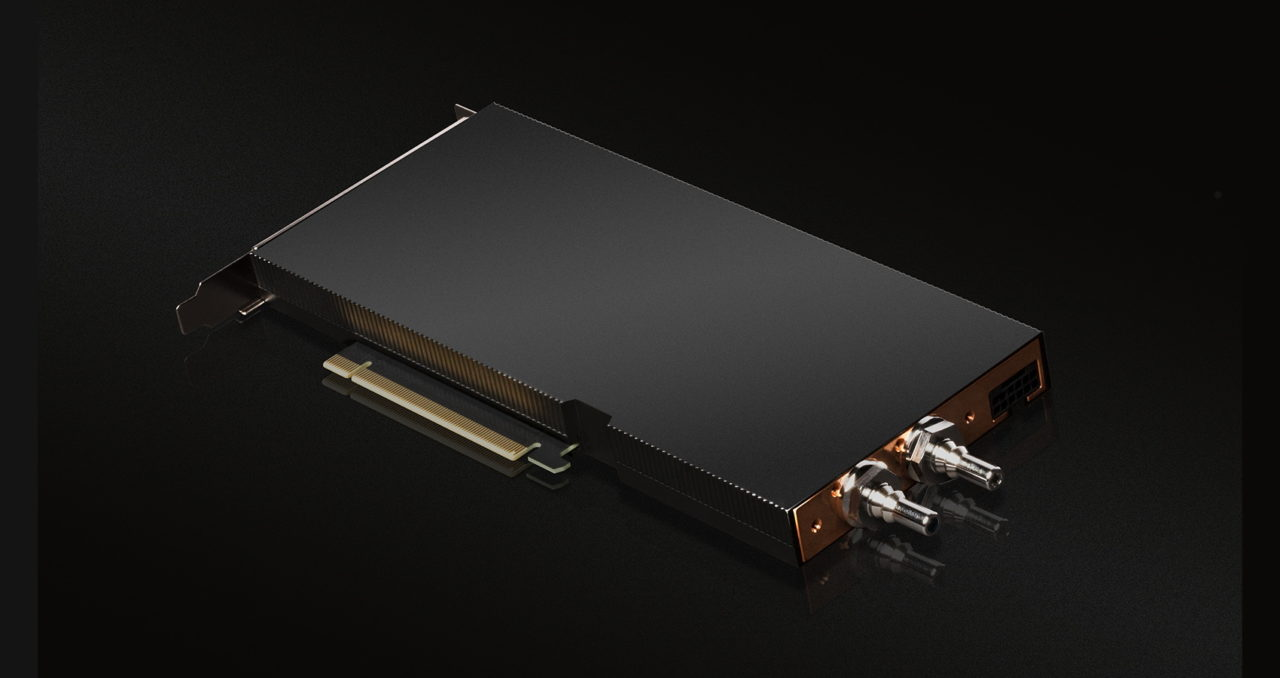
The coolant enters the graphics cards through heat-conductive pipes and absorbs the heat when going through the system. Then, the coolant is pulled using liquid pumping toward the radiator that acts as a heat exchanger between the liquid in the pipes and the air surrounding the radiator. Cloud GPU services often come built in with tools to monitor this temperature, like Paperspace Gradient Notebook's monitoring tools. This helps prevent overheating if you are running particularly expensive programs by serving as a warning system.
How to measure the performance of a graphics card?
Now that we know the major components and parts of a graphics card, we will see how the performance of a given card can be measured so it can be compared to other cards.
To evaluate a graphics card two scheme can be followed: evaluate the technical specifications of the sub-components and compare them to the results of other cards, or perform a test (a.k.a a benchmark) on the cards and compare the scores.
Specifications based evaluation
Graphics card have tens of technical specifications that can help determine its performance. We'll list the most important ones to look for when making a choice based on this evaluation method:
Core counts: the number of cores on a GPU can be a good measurement to start with when looking at the potential performance of a card. However, this can give biased comparison when comparing GPUs with different core types and architectures.
Core speed : It indicates the number of individual elementary computations that cores perform every second, measured in MHz or GHz. Another measurement to look for when building a personal system is the overclock maximum core speed, which is generally much higher than the non-overclocked speed.
Memory Size : The more RAM a card have, the more data it can handle a given time. But, it does not mean that by increasing VRAM the performance will increase, since this also depends on other components that can bottleneck.
Memory type : Memory chips with the same size can present different performances based on the technology implemented. HBM, HBM2 and HBM2e memory chips perform generally better that GDDR5 and GDDR6.
Memory Bandwidth: Memory bandwidth can be viewed as a broader method for evaluating a graphics card's VRAM performance. Memory bandwidth is basically how quickly your card's VRAM can be accessed and used at any one time.
Thermal design power (TDP): It shows how much electricity is needed to produce the most heat that the cooling system is capable of handling. When building systems, TDP is an important element for evaluating the power needs of the card.
Benchmark based evaluation
While the technical specifications can offer a broad idea on where the graphics card stands in comparison with others, it does not give a definite quantifiable mean of comparison.
Enter the benchmark , which is a test that gives a quantifiable result that can be clearly comparable between cards. For machine learning oriented graphics cards, a logical benchmark would be an ML model that is trained and evaluated across the cards to be compared. On Paperspace, multiple DL model benchmarks (YOLOR, StyleGAN_XL and EfficientNet) were performed on all cards available on either Core or Gradient. And, for each one the completion time of the benchmark was the quantifiable variable used.
Spoiler alert ! the A100 had the best results across all three benchmark test scenarios.
The advantage of the benchmark based evaluation is that it produces a single measurable element that can simply be used for comparison. Unlike the specification based evaluation, this method allows for a more complete evaluation of the graphics card as a unified system.
Why are graphics cards suitable for machine learning ?
In contrast to CPUs, GPUs are built from the ground up to process large amounts of data and carry out complicated tasks. Parallel computing is another benefit of GPUs. While CPU manufacturers strive for performance increases, which are recently starting to plateau, GPUs get around this by tailoring hardware and compute arrangements to a particular need. The Single Instruction, Multiple Data (SIMD) architecture used in this kind of parallel computing makes it possible to effectively spread workloads among GPU cores.
So, since the goal of machine learning is to enhance and improve the capabilities of algorithms, greater continuous data sets are required to be input. More data means these algorithms can learn from it more effectively and create more reliable models. Parallel computing capabilities, offered by graphics cards, can facilitate complex multi-step processes like deep learning algorithms and neural networks, in particular.
What are the best graphics cards for machine learning?
Short answer: The NVIDIA A100 - 80GB is the best single GPU available.
Long answer: Machine learning applications are a perfect match for the architecture of the NVIDIA A100 in particular and the Ampere series in general. Traffic moving to and from the DPU will be directly treated by the A100 GPU cores. This opens up a completely new class of networking and security applications that use AI, such as data leak detection, network performance optimization, and prediction.
While the A100 is the nuclear option when it comes to machine learning applications, more power does not always mean better. Depending on the ML model, the size of the dataset, the training and evaluation time constraints, sometimes a lower tier graphics card can be more than enough while keeping the cost as low as it can be. That's why having a cloud platform that offers a variety of graphics cards is important for an ML expert. For each mission, there is a perfect weapon.
Be sure to check out the Paperspace Cloud GPU comparison site to find the best deals available for the GPU you need! The A100 80 GB is currently only available in the cloud from Paperspace.
Add speed and simplicity to your Machine Learning workflow today.
https://images.nvidia.com/aem-dam/en-zz/Solutions/data-center/nvidia-ampere-architecture-whitepaper.pdf
https://www.nvidia.com/en-us/data-center/a100/
https://developer.nvidia.com/blog/nvidia-ampere-architecture-in-depth/
https://www.nvidia.com/content/dam/en-zz/Solutions/Data-Center/a100/pdf/nvidia-a100-datasheet-nvidia-us-2188504-web.pdf
https://www.nvidia.com/en-in/networking/products/data-processing-unit/
https://images.nvidia.com/aem-dam/en-zz/Solutions/data-center/dgx-a100/dgxa100-system-architecture-white-paper.pdf
Spread the word
Padding in convolutional neural networks, alternative to colab pro: comparing google's jupyter notebooks to gradient notebooks (updated), keep reading, understanding parallel computing: gpus vs cpus explained simply with role of cuda, exploring the evolution of gpus: nvidia hopper vs. ampere architectures, gpu computing for accelerating drug discovery processes, subscribe to our newsletter.
Stay updated with Paperspace Blog by signing up for our newsletter.
🎉 Awesome! Now check your inbox and click the link to confirm your subscription.
Please enter a valid email address
Oops! There was an error sending the email, please try later

GPU Architecture
- Living reference work entry
- Later version available View entry history
- First Online: 16 May 2023
- Cite this living reference work entry
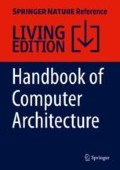
- Hyeran Jeon 2
267 Accesses
The graphics processing unit (GPU) became an undoubtedly important computing engine for high-performance computing. With massive parallelism and easy programmability, GPU has been quickly adopted by various emerging computing domains including gaming, artificial intelligence, security, virtual reality, and so on. With its huge success in the market, GPU execution and its architecture became one of the essential topics in parallel computing today. The goal of this chapter is to provide readers with a basic understanding of GPU architecture and its programming model. This chapter explores the historical background of current GPU architecture, basics of various programming interfaces, core architecture components such as shader pipeline, schedulers and memories that support SIMT execution, various types of GPU device memories and their performance characteristics, and some examples of optimal data mapping to memories. Several recent studies are also discussed that helped advance the GPU architecture from the perspectives of performance, energy efficiency, and reliability.
- Parallel computing platform
This is a preview of subscription content, log in via an institution to check access.
Access this chapter
Institutional subscriptions
Abdel-Majeed M, Dweik W, Jeon H, Annavaram M (2015) Warped-RE: low-cost error detection and correction in GPUs. In: Proceedings of the 45th annual IEEE/IFIP international conference on dependable systems and networks, 2015 June 22–25, Rio de Janeiro, Brazil
Google Scholar
Abdel-Majeed M, Shafaei A, Jeon H, Pedram M, Annavaram M (2017) Pilot register file: energy efficient partitioned register file for GPUs. In: Proceedings of the IEEE international symposium on High performance computer architecture (HPCA), 2017 Feb 4–8, Austin, TX, USA
Alverson R, Callahan D, Cummings D, Koblenz B, Porterfield A, Smith B (1990) The tera computer system. In: ACM SIGARCH computer architecture news, 1990 Sept, vol 18(3b), pp 1–6
AMD (2021) AMD HIP programming guide v1.0. [Internet]. Available from: https://github.com/RadeonOpenCompute/ROCm/blob/master/AMD_HIP_Programming_Guide.pdf
Esfeden HA, Khorasani F, Jeon H, Wong D, Abu-Ghazaleh NB (2019) CORF: Coalescing Operand Register File for GPUs. In: international conference on architectural support for programming languages and operating systems, April 2019, Providence, RI
Gebhart M, Keckler SW, Dally WJ (2011) A compile-time managed multi-level register file hierarchy. In: Proceedings of the 45th annual IEEE/ACM international symposium on microarchitecture (MICRO), 2011 Dec 3–7, Porto Alegre Brazil
Hower DR, Hechtman BA, Beckmann BM, Gaster BR, Hill MD, Reinhardt SK, Wood DA (2014) Heterogeneous-race-free memory models. In: Proceedings of the international conference on architectural support for programming languages and operating systems (ASPLOS), Mar 1–5 2014, Salt Lake City, Utah, USA
Ibrahim MA, Kayiran O, Eckert Y, Loh GH, Jog A (2021) Analyzing and leveraging decoupled L1 caches in GPUs. In: Proceedings of the IEEE international symposium on high-performance computer architecture (HPCA), Feb 27–Mar 3 2021, Seoul, Korea
Jeon H, Annavaram M (2012) Warped-DMR: light-weight error detection for GPGPU. In: Proceedings of the 45th annual IEEE/ACM international symposium on microarchitecture (MICRO), 2012 Dec 1–5, Vancouver, BC, Canada
Jeon H, Ravi GS, Kim NS, Annavaram M (2015) GPU register file virtualization. In: Proceedings of the 48th annual IEEE/ACM international symposium on microarchitecture (MICRO), 2015 Dec 5–9, Waikiki, HI, USA
Jeon H, Esfeden HA, Abu-Ghazaleh NB, Wong D, Elango S (2019) Locality-aware GPU register file. IEEE Comput Archit Lett 18(2):153–156
Jog A, Kayiran O, Mishra AK, Kandemir MT, Mutlu O, Iyer R, Das CR (2013) Orchestrated scheduling and prefetching for GPGPUs. In: Proceedings of the 40th annual international symposium on computer architecture (ISCA), 2013 June 23, Tel Aviv, Israel
Kim K, Wo RW (2018) WIR: warp instruction reuse to minimize repeated computations in GPUs. In: Proceedings of the IEEE international symposium on High Performance Computer Architecture (HPCA), 2018 Feb 24–28, Vienna, Austria
Kim K, Lee S, Yoon MK, Koo G, Ro WW, Annavaram M (2016) Warped-preexecution: a GPU pre-execution approach for improving latency hiding. In: Proceedings of the IEEE international symposium on high performance computer architecture (HPCA), 2016 Mar 12–16, Barcelona, Spain
Kim H, Ahn S, Oh Y, Bo K, Ro WW, Song W (2020) Duplo: lifting redundant memory accesses of deep neural networks for GPU tensor cores. In: Proceedings of the 53rd annual IEEE/ACM international symposium on microarchitecture (MICRO), 2020 Oct 17–21, Athens, Greece
Koo G, Oh Y, Ro WW, Annavaram M (2017) Access pattern-aware cache management for improving data utilization in GPU. In: Proceedings of the ACM/IEEE 44th annual international symposium on computer architecture (ISCA), 2017 June 24–28, Toronto, ON, Canada
Lai J, Seznec A (2013) Performance upper bound analysis and optimization of SGEMM on Fermi and Kepler GPUs. In: Proceedings of the 2013 IEEE/ACM international symposium on code generation and optimization (CGO), 2013 Feb 23, pp 1–10
Lee S, Kim K, Koo G, Jeon H, Ro WW, Annavaram M (2015) Warped-compression: enabling power efficient GPUs through register compression. In: Proceedings of the ACM/IEEE 42nd annual international symposium on computer architecture (ISCA), 2015 June 13–17, Portland, OR, USA
Lee S, Arunkumar A, Wu C (2015b) CAWA: coordinated warp scheduling and cache prioritization for critical warp acceleration of GPGPU workloads. In: Proceedings of the ACM/IEEE 42nd annual international symposium on computer architecture (ISCA), 2015 June 13–17, Portland, OR, USA
Lee S, Kim K, Koo G, Jeon H, Annavaram M, Ro WW (2017) Improving energy efficiency of GPUs through data compression and compressed execution. IEEE Trans Comp 66(5):834–847
Nie B, Yang L, Jog A, Smirni E (2018) Fault site pruning for practical reliability analysis of GPGPU applications. In: Proceedings of the 51st international symposium on microarchitecture (MICRO), 2018 Oct 20–24, Fukuoka, Japan
NVIDIA (2012) NVIDIA Geforce GTX 680 white paper v1.0. [Internet]. Available from: https://www.nvidia.com/content/PDF/product-specifications/GeForce_GTX_680_Whitepaper_FINAL.pdf
NVIDIA (2016) NVIDIA Tesla P100 white paper v1.1. [Internet]. Available from: https://images.nvidia.com/content/pdf/tesla/whitepaper/pascal-architecture-whitepaper.pdf
NVIDIA (2022) CUDA C++ Programming Guide v11.6. [Internet]. Available from: https://docs.nvidia.com/cuda/pdf/CUDA_C_Programming_Guide.pdf
Oh Y, Koo G, Annavaram M, Ro WW (2019) Linebacker: preserving victim cache lines in idle register files of GPUs. In: Proceedings of the ACM/IEEE 46th annual international symposium on computer architecture (ISCA), 2019 June 22–26, Phoenix, AZ, USA
Pattnaik A, Tang X, Kayiran O, Jog A, Mishra A, Kandemir MT, Sivasubramaniam A, Das CR (2019) Opportunistic computing in GPU architectures. In: Proceedings of the 46th international symposium on computer architecture (ISCA), 2019 June 22, Phoenix, Arizona
Rogers TG, O’Connor M, Aamodt TM (2012) Cache-conscious wavefront scheduling. In: Proceedings of the IEEE/ACM 45th annual international symposium on microarchitecture (MICRO), 2012 Dec 1–5, Vancouver, BC, Canada
Rogers TG, O’Connor M, Aamodt TM (2013) Divergence-aware warp scheduling. In: Proceedings of the IEEE/ACM 45th annual international symposium on microarchitecture (MICRO), 2013 Dec 7–11, Davis, CA, USA
Sethia A, Jamshidi D A, Mahlke S (2015) Mascar: speeding up GPU warps by reducing memory pitstops. In: IEEE 21st international symposium on high performance computer architecture (HPCA), 2015 Feb 7–11, Burlingame, CA, USA
Tan J, Fu X (2012) RISE: improving the streaming processors reliability against soft errors in GPGPUs. In: Proceedings of the 21st international conference on parallel architectures and compilation techniques (PACT), 2012 Sept 19–23, Minneapolis, Minnesota, USA
Top500 (2021) Top 500 supercomputer lists. [Internet]. Available from: https://www.top500.org/
Wong D, Kim NS, Annavaram M (2016) Approximating warps with intra-warp operand value similarity. In: IEEE international symposium on high performance computer architecture, March 2016, Barcelona, Spain
Download references
Author information
Authors and affiliations.
University of California Merced, Merced, CA, USA
Hyeran Jeon
You can also search for this author in PubMed Google Scholar
Corresponding author
Correspondence to Hyeran Jeon .
Editor information
Editors and affiliations.
Sch of Computer Science & Engineering, Nanyang Technological University, Singapore, Singapore
Anupam Chattopadhyay
Section Editor information
The Sirindhorn International Thai-German Graduate School of Engineering, King Mongkut's University of Technology North Bangkok, Bangkok, Thailand
Rachata Ausvarungnirun
Rights and permissions
Reprints and permissions
Copyright information
© 2023 Springer Nature Singapore Pte Ltd.
About this entry
Cite this entry.
Jeon, H. (2023). GPU Architecture. In: Chattopadhyay, A. (eds) Handbook of Computer Architecture. Springer, Singapore. https://doi.org/10.1007/978-981-15-6401-7_66-1
Download citation
DOI : https://doi.org/10.1007/978-981-15-6401-7_66-1
Received : 02 February 2022
Accepted : 24 October 2022
Published : 16 May 2023
Publisher Name : Springer, Singapore
Print ISBN : 978-981-15-6401-7
Online ISBN : 978-981-15-6401-7
eBook Packages : Springer Reference Engineering Reference Module Computer Science and Engineering
- Publish with us
Policies and ethics
Chapter history
DOI: https://doi.org/10.1007/978-981-15-6401-7_66-2
DOI: https://doi.org/10.1007/978-981-15-6401-7_66-1
- Find a journal
- Track your research
- All Research Labs
- 3D Deep Learning
- Applied Research
- Autonomous Vehicles
- Deep Imagination
- New and Featured
- AI Art Gallery
- AI & Machine Learning
- Computer Vision
- Academic Collaborations
- Government Collaborations
- Graduate Fellowship
- Internships
- Research Openings
- Research Scientists
- Meet the Team
Research Areas
Computer graphics, associated publications, researchers.
Research at NVIDIA
Groundbreaking technology begins right here with the world's leading researchers.
- Introduction
- Discover more
Explore State of the Art Generative AI, Graphics, and More
NVIDIA Research is passionate about developing the technology and finding the breakthroughs that bring positive change to the world. Beyond publishing our work in papers and at conferences, we apply it to NVIDIA solutions and services, share resources and code, and offer hands-on experiences with technical demos.
Inside the Work
Take a deeper dive into nvidia research.
- Research Areas
Advancing Generative AI, Robotics, and Rendering
NVIDIA investigations span a wide range of fields and often encompass more than one research area.
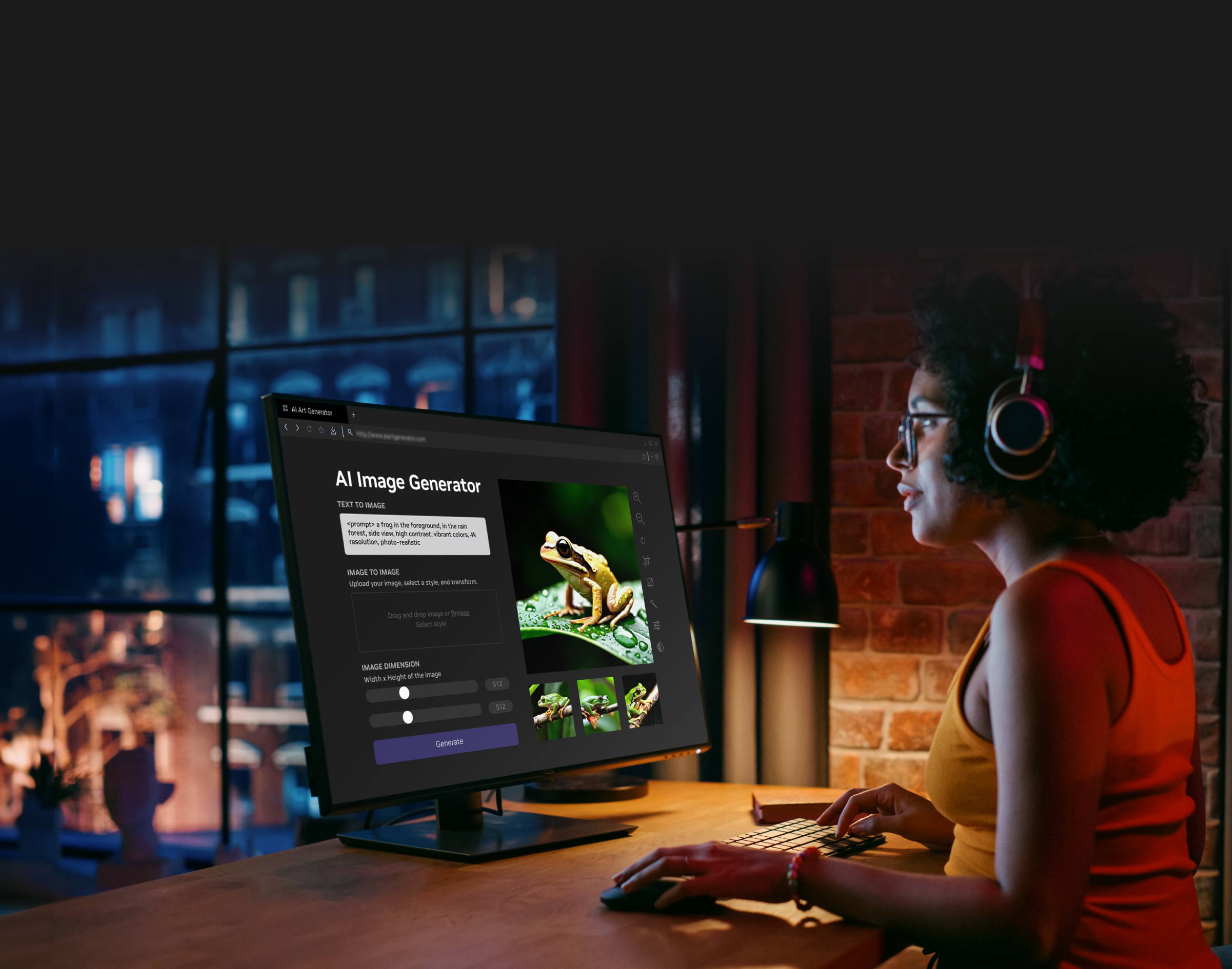
Research Labs
Advancements from our Research Labs
Here, our teams share more than just their cutting-edge research. They highlight examples, provide additional resources, and even code.
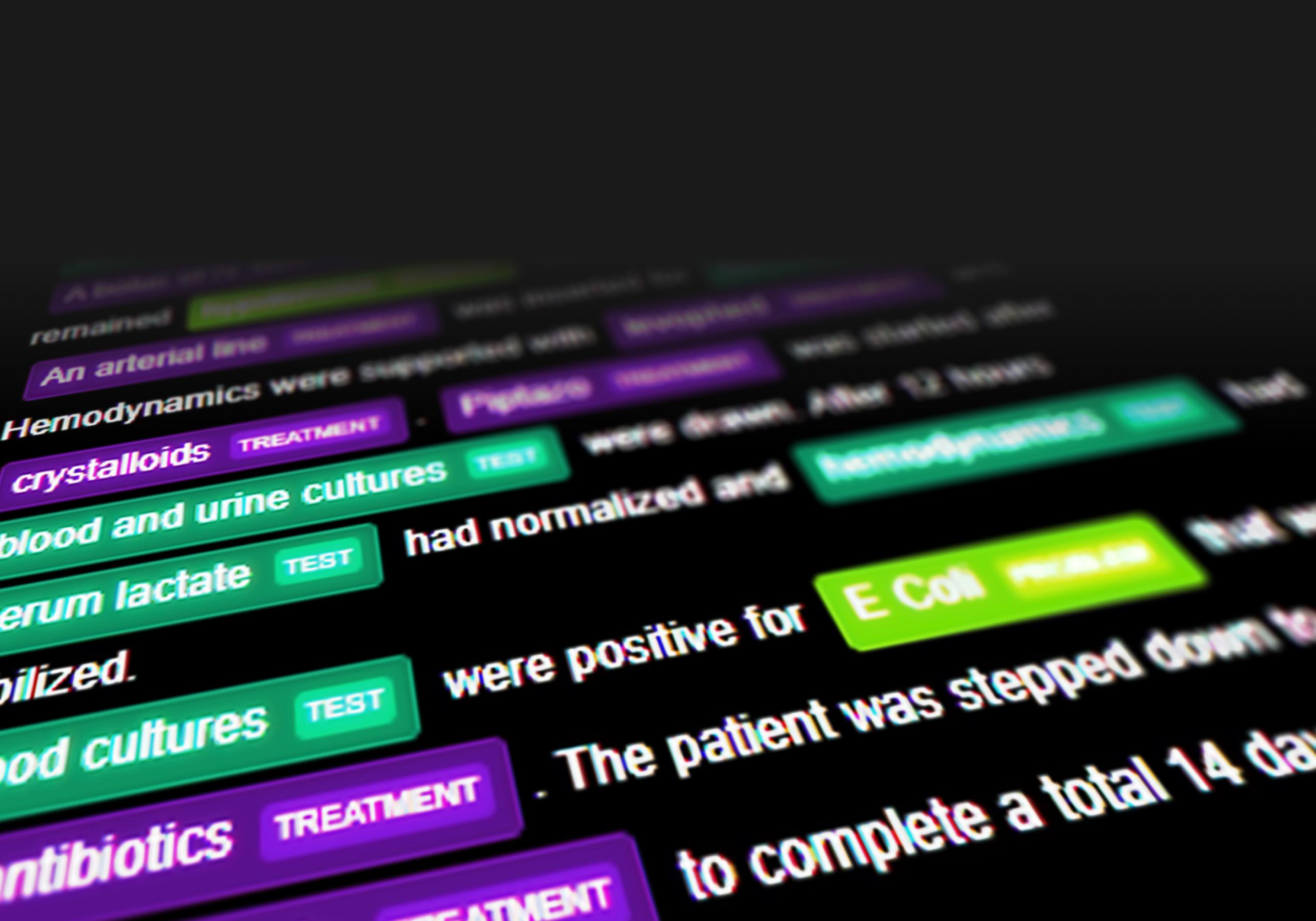
AI Playground
The Intersection of AI, Art, and Science
In the AI Playground, you can play with the latest innovations from our research teams. Take a few minutes to explore the various demos that push the boundaries of technology.
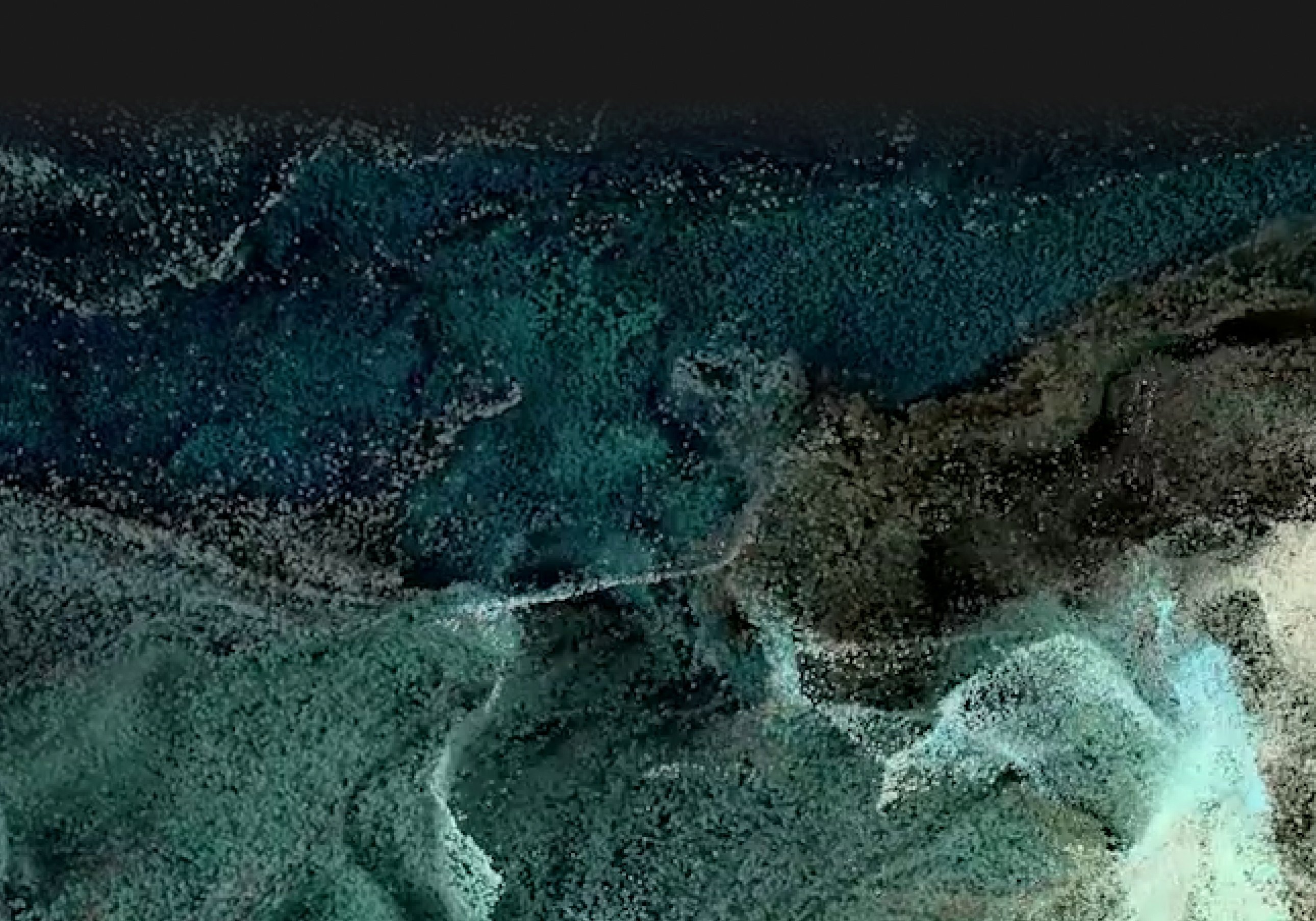
- Publications
A Collection of NVIDIA Research Papers
Our publications provide insight into some of our leading-edge research. Published regularly to world-renowned conferences and academic journals, we also host these papers here to provide easy access to all NVIDIA Research publications.
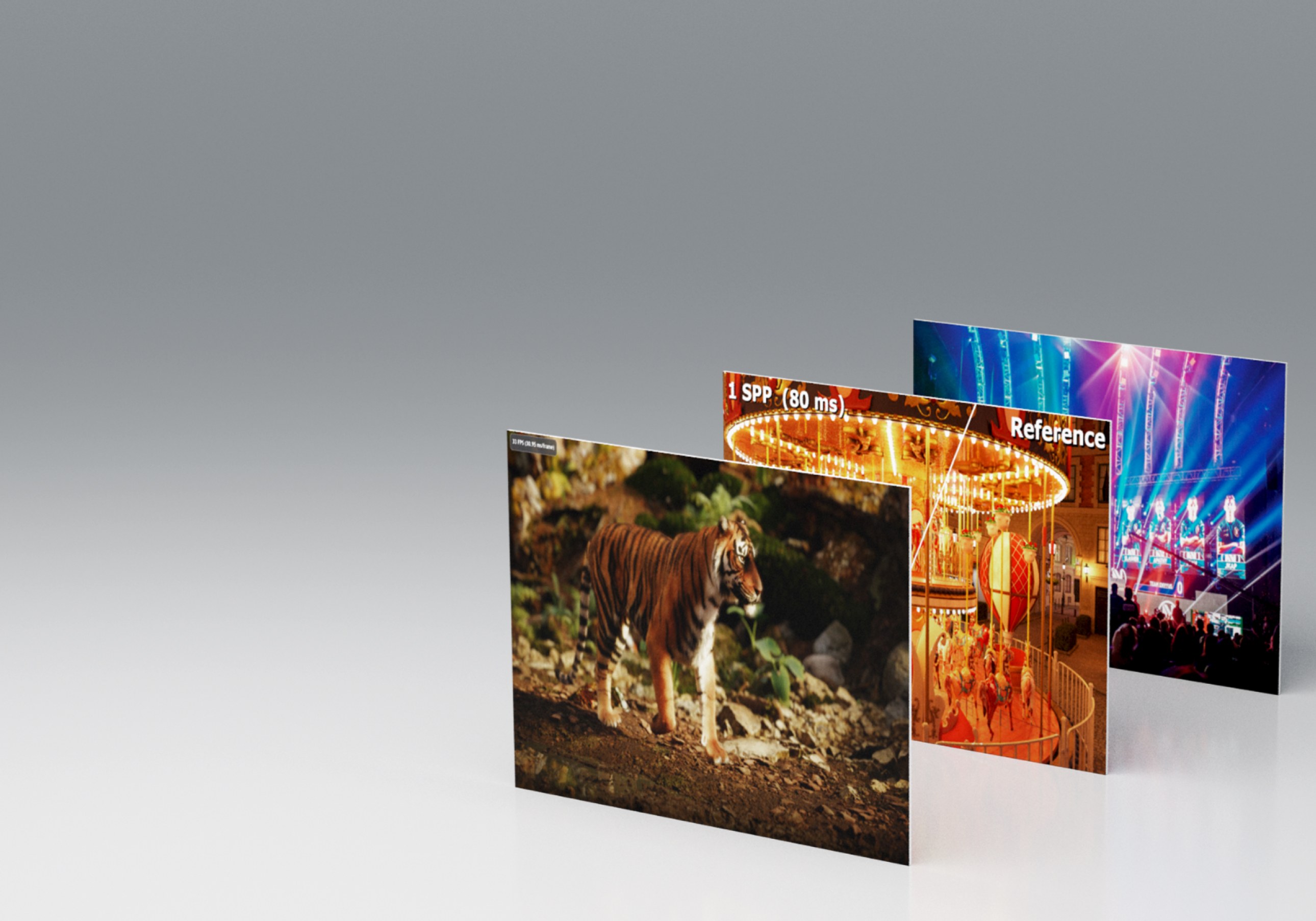
Access the Power of NVIDIA's Proprietary Models
NVIDIA researchers are known for developing game-changing research models. Startups, corporations, and researchers can request an NVIDIA Research proprietary software license and, if approved, use these models in their products, services, or internal workflows.
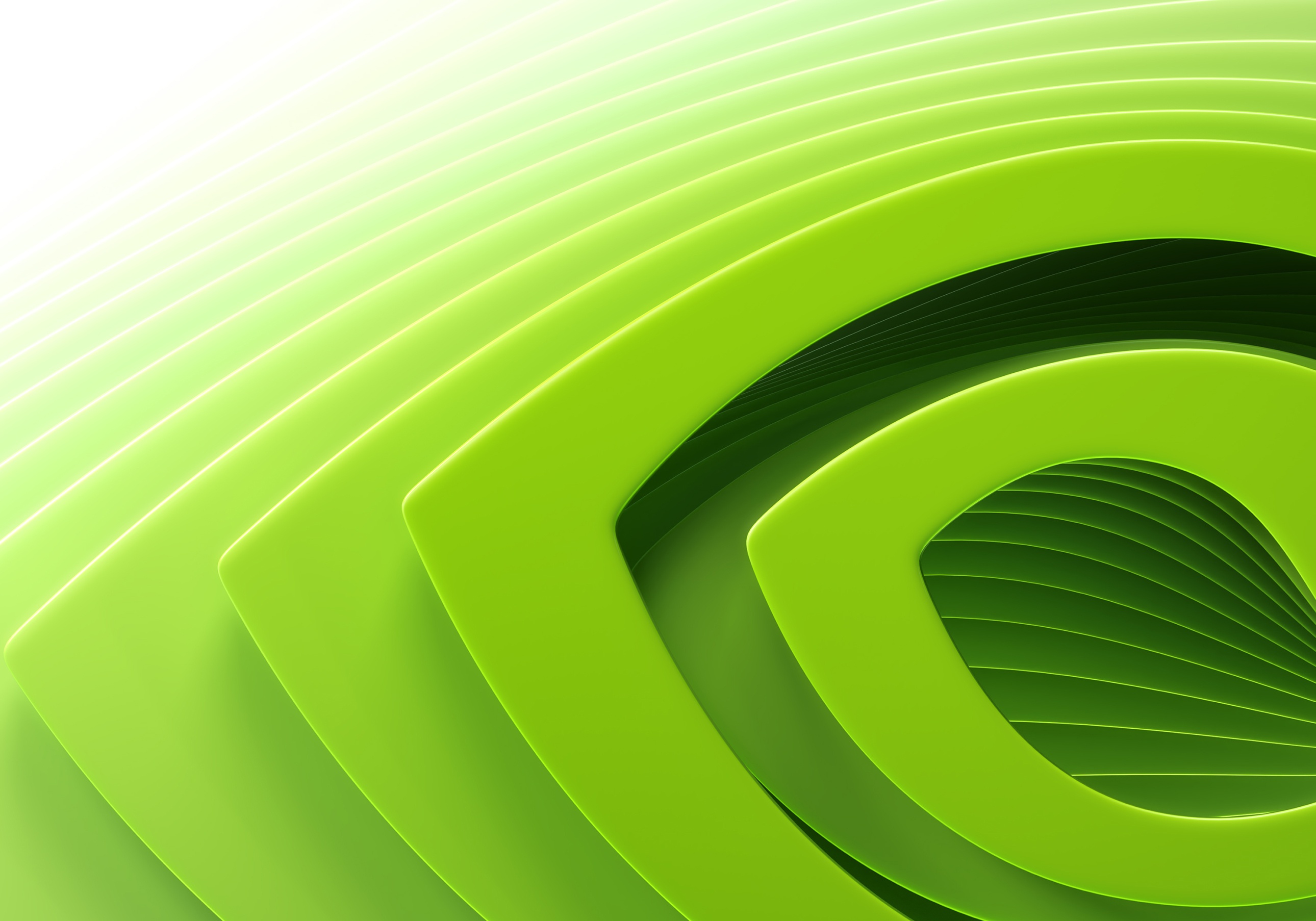
Research News
Stay up to date with the always-advancing world of AI.
NVIDIA Research Announces Array of AI Advancements at NeurIPS
NVIDIA presented around 20 research papers at SIGGRAPH, the year’s most important computer graphics conference.

From Simulation to Data Generation: NVIDIA Research to Animate CoRL
This year in Atlanta, NVIDIA Research will be presenting their research findings in sim-to-real transfer, imitation learning, and language-guided tasks.
TIME Best Inventions 2023
TIME awarded Neuralangelo as one of 2023’s best inventions. It’s an AI model for 3D reconstruction that uses neural networks to turn 2D video clips into detailed 3D structures.

NVIDIA Picasso: Build and Deploy Gen AI-Powered Image, Video, and 3D Applications
NVIDIA Picasso is a generative AI foundry for visual design for building, customizing, and deploying foundation models with ease.

Like No Place You’ve Ever Worked
At NVIDIA, you’ll solve some of the world’s hardest problems and discover new ways to improve the quality of life for people everywhere. From healthcare to robots, self-driving cars to blockbuster movies—and a growing list of opportunities every single day—explore all of our open roles, including internships and new college graduate positions.

Research Code Libraries
Check out a collection of resources that simplify programming tasks for AI researchers. These open-source resources are available to all and include NVIDIA Sionna, Kaolin, Kaolin Wisp, Imaginaire, and CUDA-X™.

Try state-of-the-art generative AI model APIs from your browser.
NVIDIA AI Foundation Models offer an easy-to-use interface to quickly experience curated and optimized AI models directly from your browser. These AI models run on the NVIDIA accelerated computing stack, providing the best performance for experiencing NVIDIA AI.
NVIDIA Research Team at Events
Discover more ai inspiration.

Research On-Demand
See all the latest NVIDIA advances from GTC and other leading technology conferences—free and on demand.

Higher Education and Research
Supercharge your research with the latest higher-education discounts on NVIDIA’s state-of-the-art GPUs.
Stay Up to Date
Have the latest news in groundbreaking NVIDIA Research sent directly to your inbox.
Get The Latest NVIDIA Research News
NVIDIA Privacy Policy
NVIDIA Sionna
Sionna is a GPU-accelerated open-source library for physical-layer research and link-level simulations based on TensorFlow. It enables rapid prototyping of complex communication system architectures and provides native support for the integration of neural networks. Sionna ›
NVIDIA Kaolin
Kaolin is a PyTorch library that accelerates 3D deep learning research by providing efficient implementations of differentiable 3D modules. Importantly, a comprehensive model zoo comprising many state-of-the-art 3D deep learning architectures has been curated to serve as a starting point for future research endeavors. Kaolin ›
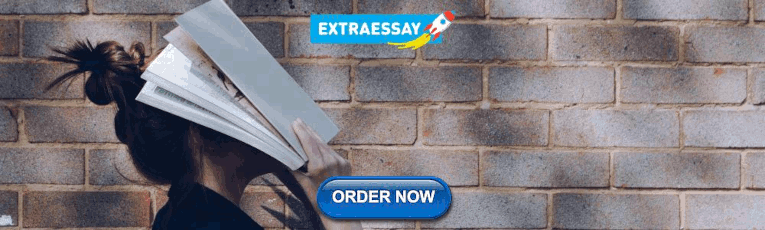
NVIDIA Kaolin Wisp
Kaolin Wisp is a PyTorch library powered by NVIDIA Kaolin to work with neural fields (including NeRFs, NGLOD, instant-ngp, and VQAD). Kaolin Wisp ›
NVIDIA Imaginaire
Imaginaire is a PyTorch library that contains optimized implementations of several image and video synthesis methods developed at NVIDIA. Imaginaire ›
NVIDIA CUDA-X
CUDA® is a parallel computing platform and programming model developed by NVIDIA for general computing on GPUs. CUDA-X™ is a collection of libraries for AI and high-performance computing built on top of CUDA that lets developers dramatically speed up their applications with the power of GPUs. CUDA-X ›
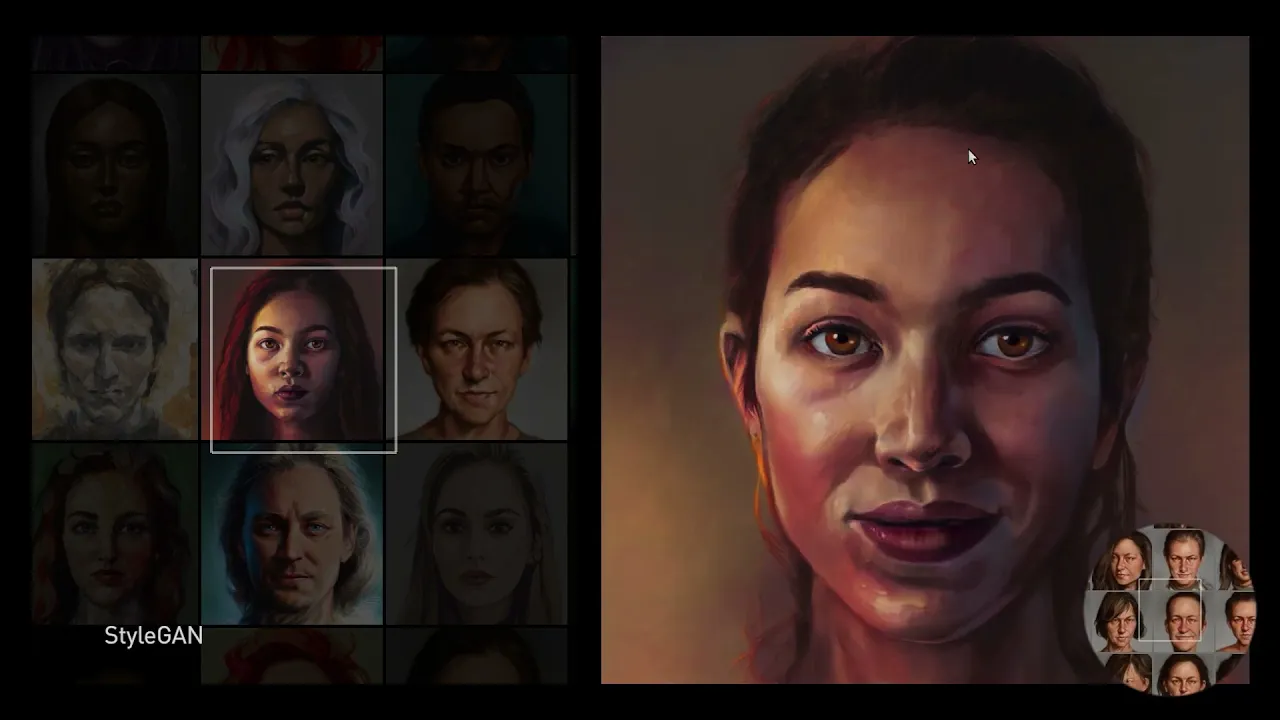
- Collaborations
- Business Inquires
- Technical Blog
- Deep Learning Institute
- AI Startups Program
- AI Computing Model

- Privacy Policy
- Manage My Privacy
- Do Not Sell or Share My Data
- Terms of Service
- Accessibility
- Corporate Policies
- Product Security
Thank you for visiting nature.com. You are using a browser version with limited support for CSS. To obtain the best experience, we recommend you use a more up to date browser (or turn off compatibility mode in Internet Explorer). In the meantime, to ensure continued support, we are displaying the site without styles and JavaScript.
- View all journals
- Explore content
- About the journal
- Publish with us
- Sign up for alerts
- Review Article
- Published: 23 March 2022
The transformational role of GPU computing and deep learning in drug discovery
- Mohit Pandey ORCID: orcid.org/0000-0002-2562-7155 1 na1 ,
- Michael Fernandez ORCID: orcid.org/0000-0003-2273-733X 1 na1 ,
- Francesco Gentile ORCID: orcid.org/0000-0001-8299-1976 1 ,
- Olexandr Isayev ORCID: orcid.org/0000-0001-7581-8497 2 ,
- Alexander Tropsha 3 ,
- Abraham C. Stern 4 &
- Artem Cherkasov 1
Nature Machine Intelligence volume 4 , pages 211–221 ( 2022 ) Cite this article
35k Accesses
72 Citations
100 Altmetric
Metrics details
- Cheminformatics
- Drug discovery
- High-throughput screening
Deep learning has disrupted nearly every field of research, including those of direct importance to drug discovery, such as medicinal chemistry and pharmacology. This revolution has largely been attributed to the unprecedented advances in highly parallelizable graphics processing units (GPUs) and the development of GPU-enabled algorithms. In this Review, we present a comprehensive overview of historical trends and recent advances in GPU algorithms and discuss their immediate impact on the discovery of new drugs and drug targets. We also cover the state-of-the-art of deep learning architectures that have found practical applications in both early drug discovery and consequent hit-to-lead optimization stages, including the acceleration of molecular docking, the evaluation of off-target effects and the prediction of pharmacological properties. We conclude by discussing the impacts of GPU acceleration and deep learning models on the global democratization of the field of drug discovery that may lead to efficient exploration of the ever-expanding chemical universe to accelerate the discovery of novel medicines.
Similar content being viewed by others
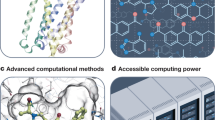
Computational approaches streamlining drug discovery
Anastasiia V. Sadybekov & Vsevolod Katritch
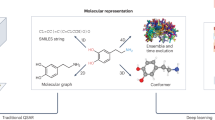
Integrating QSAR modelling and deep learning in drug discovery: the emergence of deep QSAR
Alexander Tropsha, Olexandr Isayev, … Artem Cherkasov
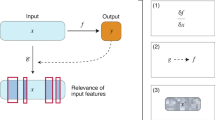
Drug discovery with explainable artificial intelligence
José Jiménez-Luna, Francesca Grisoni & Gisbert Schneider
Originally developed to accelerate three-dimensional graphics, the benefits of GPUs for powerful parallel computing were quickly praised by the scientific community. The earliest attempts to use GPUs for scientific purposes employed the programmable shader language to run calculations. In 2007, NVIDIA released Compute Unified Device Architecture (CUDA) as an extension of the C programming language, together with compilers and debuggers, opening the floodgates for porting computationally intensive workloads into GPU accelerators. Further advances came from the release of common maths libraries such as fast Fourier transforms and basic linear algebra subroutines, which were foundational to scientific computing. In the same year, the first computational chemistry programs were ported to GPUs, enabling efficient parallelization of molecular mechanics and quantum Monte Carlo 1 calculations.
In September 2014, NVIDIA released cuDNN, a GPU-accelerated library of primitives for deep neural networks (DNNs) implementing standard routines such as forward and backward convolution, pooling, normalization and activation layers. The architectural support for training and testing subprocesses enabled by GPUs seemed to be particularly effective for standard deep learning (DL) procedures. As a result, an entire ecosystem of GPU-accelerated DL 2 platforms has emerged. While NVIDIA’s CUDA is a more established GPU programming framework, AMD’s ROCm 3 represents a universal platform for GPU-accelerated computing. ROCm introduced new numerical formats to support common open-source machine learning libraries such as TensorFlow and PyTorch; it also provides the means for porting NVIDIA CUDA code into AMD hardware 4 . It is important to note that AMD not only is catching up to the ROCm platform in the GPU computing race, but also recently introduced the new flagship GPU architecture AMD Instinct MI200 Series 5 to compete with the latest NVIDIA Ampere A100 GPU architecture 6 .
The fields of bioinformatics, cheminformatics and chemogenomics in particular, including computer-aided drug discovery (CADD), have taken advantage of DL methods running on GPUs. Most challenges in CADD have routinely faced combinatorics and optimization problems, and machine learning has been effective at providing solutions for them 7 . Thus, major progress has been made in DL for CADD applications such as virtual screening, de novo drug design, absorption, distribution, metabolism, excretion and toxicity (ADMET) properties prediction and so on (Fig. 1 ).
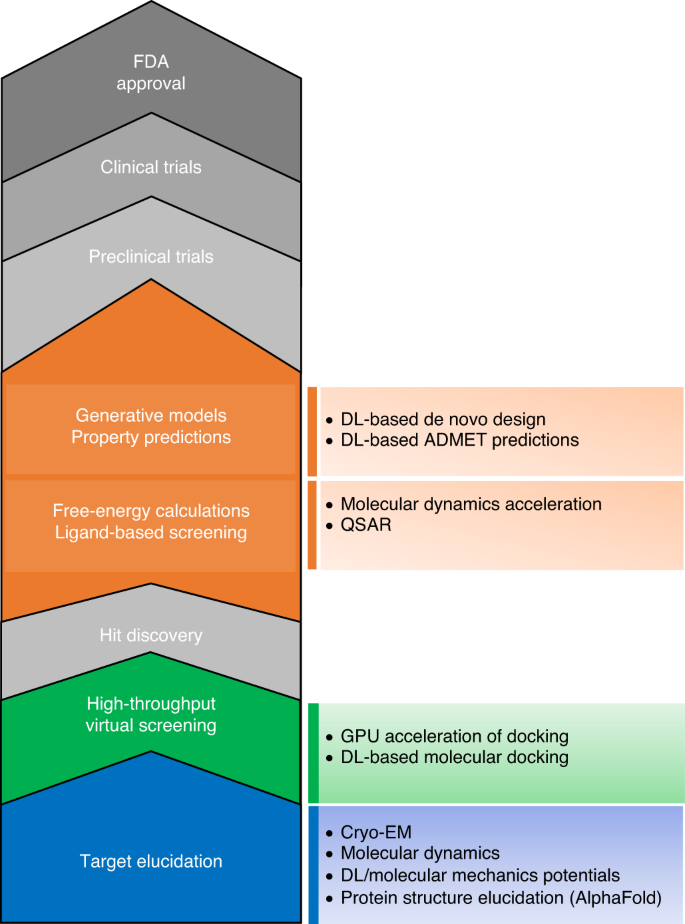
GPU accelerators find applications in each step of the drug discovery and development process (shaded in colour). FDA, US Food and Drug Administration.
Herein, we discuss the effects of GPU-supported parallelization and DL model development and application on the timescale and accuracy of simulations of proteins and protein–ligand complexes. We also provide examples of DL algorithms used for structure determination in cryo-electron microscopy (cryo-EM) and 3D structure prediction of proteins.
GPU computing and DL for molecular simulations
GPU acceleration comes from massive data parallelism, which arises from similar independent operations performed on many elements of the data. In graphics, an example of a common data parallel operation is the use of a rotation matrix across coordinates describing the positions of objects as a view is rotated. In a molecular simulation, data parallelism can be applied to independent calculation of atomic potential energies. Similarly, DL model training involves forward and backward passes that are commonly expressed as matrix transformations that are readily parallelizable (Fig. 2 ).
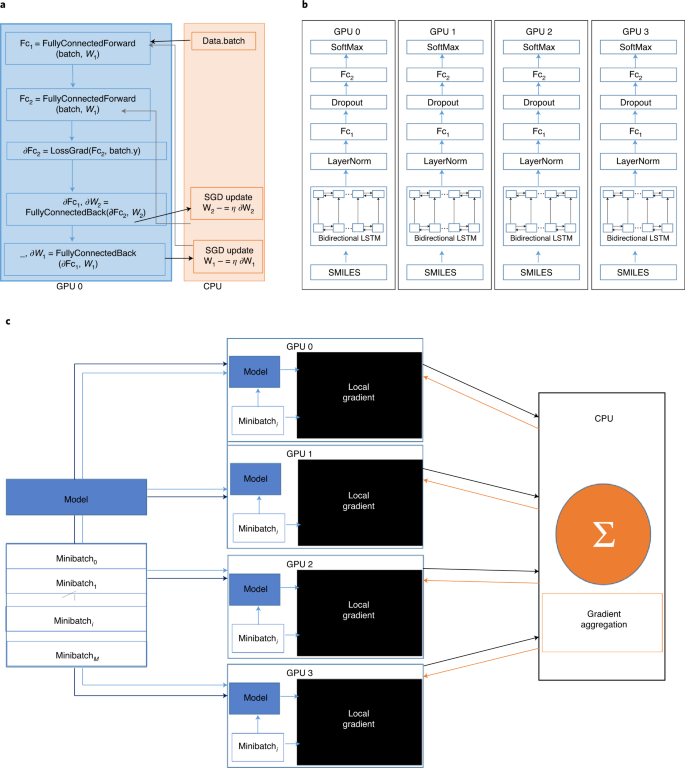
Neural network arithmetic operations are based on matrix multiplications that are parallelized by GPUs using block multiplication and aggregation 131 . a , Distribution of computational graph over one GPU for a two-layered multilayer perceptron (MLP). W , trainable parameters; SGD, stochastic gradient descent algorithm; η , learning rate of the stochastic gradient descent algorithm. b , Data parallelization. Each GPU stores a network copy. Data parallelization is the most commonly adopted GPU paradigm for accelerating DL 132 . A copy of the network resides in each GPU, and each GPU gets its own dedicated minibatch of data to train on. The computed gradients and losses are then transferred to a shared device (typically the CPU) for aggregation before being rebroadcast to GPUs for parameter updates. LayerNorm, Dropout, Fc, SoftMax and Bidirectional LSTM (long short-term memory) are modules of an arbitrary neural network topology used for demonstration. c , Forward and backpropagation for a gradient minibatch descent algorithm. M , total mini-batches for the data.
Accelerating molecular dynamics simulations on GPUs
The development of GPU-centred molecular dynamics codes in the past decade led hundred-fold reductions in the computational costs of simulations compared with central processing unit (CPU)-based algorithms 8 . Consequently, most molecular dynamics engines (such as AMBER (assisted model building with energy refinement) 9 , GROMACS (Groningen machine for chemical simulations) 10 and NAMD (nanoscale molecular dynamics) 11 ) now provide GPU-accelerated implementations. GPUs not only are well suited to accelerating molecular dynamics simulations but also scale well with system size using spatial domain decomposition 12 . As a result, molecular dynamics simulations extend to a broader range of biomolecular phenomena, approaching the viral and cell level and coming closer to experimental timescales. Recent methodological and algorithmic advances enabled molecular dynamics simulations of molecular assemblies of up to 2 × 10 9 atoms (Fig. 3 ) 13 , with overall simulation times of microseconds or even milliseconds.
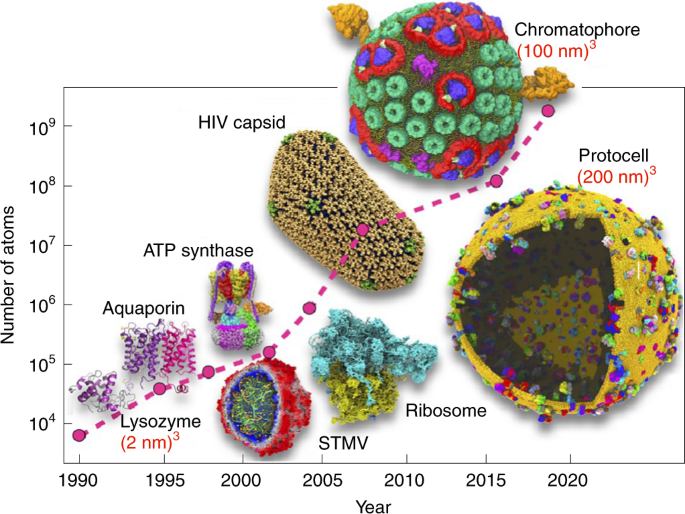
Continuous development effort over the years towards simulating with NAMD realistic biological objects of increasing complexity from a small, solvated protein, on the thousand-atom size scale, in the early 1990s, to a full protocell, on the billion-atom size scale, now. ATP, adenosine triphosphate; HIV, human immunodeficiency virus; STMV, satellite tobacco mosaic virus. Figure reproduced with permission from ref. 13 , AIP Publishing.
Free-energy simulations represent another area that continues to benefit from progress in GPU development. Methods such as relative binding free-energy calculations, thermodynamic integration and free-energy perturbation 14 now allow reliable binding affinities for a large number of protein–ligand complexes to be computed. In this regard, the recent development of neural network-based force fields such as ANI (accurate neural network engine for molecular energies) 15 and AIMNet (atoms-in-molecules net) 16 provides industry-standard accuracy of free-energy simulations. The benchmarks with inhibitors for tyrosine-protein kinase 2 from the Schrödinger Journal of the American Chemical Society benchmark set 17 showed that the simulations with ANI machine learning potential reduced the absolute binding free-energy errors by 50%. Frameworks such as ANI provide a systematic approach for generating atomistic potentials and drastically reduce the human effort required to fit a force field, thus automating force field development 18 . More recently, other DL frameworks have been proposed to further push the boundaries of molecular simulations in drug discovery 19 . Exemplifying these approaches, the reweighted autoencoder variational Bayes for enhanced sampling 20 method was employed successfully to simulate ligand–protein dissociation. It processed notably faster than conventional molecular dynamics, yet generated accurate estimates of binding free energies 21 and loop conformation sampling 22 . Similarly, Drew Bennett et al. 23 used DNNs to predict water-to-cyclohexane transfer energies of small molecules derived from molecular dynamics simulations. The use of hybrid DL and molecular mechanics potentials 24 for ligand–protein simulations has also been proposed, supported by the development of open-source frameworks 25 , 26 . These methods employ quantum mechanics-based DL potentials for the ligand and molecular mechanics for the surrounding environment, and have shown superior performances in reproducing binding poses 27 compared with conventional potentials.
Quantum mechanics and GPUs
The availability of CUDA 28 and OpenCL 29 application programming interfaces (APIs) has been key to the success of GPU applications, although programming GPUs to run chemistry codes efficiently is not trivial. To achieve high efficiency, computational threads that are grouped into blocks need to be executed simultaneously. TeraChem was the first quantum chemistry code to be written specifically for GPUs 30 . The mixed-precision arithmetic allowed very efficient computation of Coulomb and exchange matrices 31 . The latest algorithmic developments in TeraChem allowed entire proteins to be simulated with density functional theory (DFT) 32 . Hybrid quantum mechanics–molecular mechanics simulations of the nonadiabatic dynamics of Bacteriorhodopsin provided insight into the light-activation machinery and a molecular-level understanding of the conversion of light energy into work 33 . DFT calculations are now routine for studying protein–ligand interactions. For instance, the best calculations resulted in mean absolute errors of ~2 kcal mol −1 for protein–ligand interaction energies 33 . DFT calculations of serine protease factor X and tyrosine-protein kinase 2 showed that the obtained geometries are close to the co-crystallized protein–ligand structures 34 .
Future exascale supercomputers will provide high levels of parallelism in heterogeneous CPU and GPU environments. This scaling requires the development of new hybrid algorithms and, essentially, a complete rewrite of the scientific codes. These new developments are now being implemented as a part of the NWChemEx package 35 . NWChemEx will offer the possibility of performing quantum mechanics and molecular mechanics simulations for systems that are several orders of magnitude larger than those that are tractable by canonical formulations of theoretical methods 35 .
GPU acceleration of protein structure determination
High-throughput and automation of cryo-EM have become increasingly important as the state-of-the-art experimental technique used for protein structure determination for use in structure-based drug design 36 . DL-based approaches, such as DEFMap 37 and DeepPicker 38 , have been developed to accelerate processing of cryo-EM images. The DEFMap method directly extracts structure dynamics associated with hidden atomic fluctuations by combining DL and molecular dynamics simulations that learn the relationships between local density data. DeepPicker employs convolutional neural networks (CNNs) and cross-molecule training to capture common features of particles from previously analysed micrographs, which facilities automatic particle picking in single-particle analysis. This tool serves to illustrate that DL integration can successfully address current gaps towards fully automated cryo-EM pipelines, paving the way for a new multidisciplinary approach to protein science 37 , 38 .
In addition to accelerated experimental characterization of protein structures by cryo-EM, the recent ground-breaking success of DeepMind with the AlphaFold-2 method in the Critical Assessment of Protein Structure Prediction (CASP) challenge hints at the future impacts of DL algorithms in protein structural characterization and the expansion of the druggable proteome 39 . AlphaFold-2 can regularly predict protein geometry with atomic accuracy without being previously exposed to similar structures. The recently updated neural network-based model demonstrated an accuracy competitive with experiments in most cases, and greatly outperformed other methods at the 14th CASP competition. The DL model behind AlphaFold-2 incorporates physical and biological knowledge about protein structure, leveraging multi-sequence alignments to crack one of the oldest problems in biology. AlphaFold-2 was employed to predict the structures of nearly every known human protein and other organisms important to medical research, a total of 350,000 proteins, which represents an impressive achievement for biomedical research 39 .
The emergence of DL in CADD
Advances in DL, particularly in computer vision and language processing, revived the recent interest of CADD researchers in neural networks. Merck is credited with popularizing DL for CADD through the Kaggle competition on Molecular Activity Challenge in 2012 (ref. 40 ). The winning solution by Dahl et al. 41 leveraged a multitask learning approach to train a DNN. Thereafter, many researchers embraced such models for drug discovery problems. These include the evaluation of the predictors of the pharmacokinetic behaviour of therapeutics and their adverse effects 42 , the prediction of small molecule–protein binding 43 , the determination of chemotherapeutic responses of carcinogenic cells 44 , the quantitative estimation of drug sensitivity 45 and quantitative structure–activity relationship (QSAR) modelling 46 , among others.
The emergence of GPU-enabled DL architectures, along with the proliferation of chemical genomics data, has led to meaningful CADD-enabled discoveries of clinical drug candidates. Furthermore, artificial intelligence (AI)-driven companies (such as BenevolentAI, Insilico Medicine and Exscientia, among others) are reporting successes in augmented drug discovery. For example, Exscientia developed a drug candidate, DSP-1181, to be used against obsessive-compulsive disorder that entered phase 1 clinical trials less than 12 months from its conception using AI approaches 47 . Insilico Medicine just began a clinical trial with its first AI-developed drug candidate to treat idiopathic pulmonary fibrosis and BenevolentAI identified baricitinib 48 as a potential treatment for COVID-19 (ref. 49 ). These recent success cases indicate that further promotion and application of AI-driven approaches supported by GPU computing could greatly accelerate the discovery of novel and improved medicines.
DL architectures for CADD
From discriminative neural networks that find applications in virtual screening of existing or synthetically feasible chemical libraries to the recent success of DL generative models that has inspired their use in de novo drug design, Fig. 4 depicts the general scheme of commonly used state-of-the-art DL architectures. Table 1 enumerates their adoption in CADD.
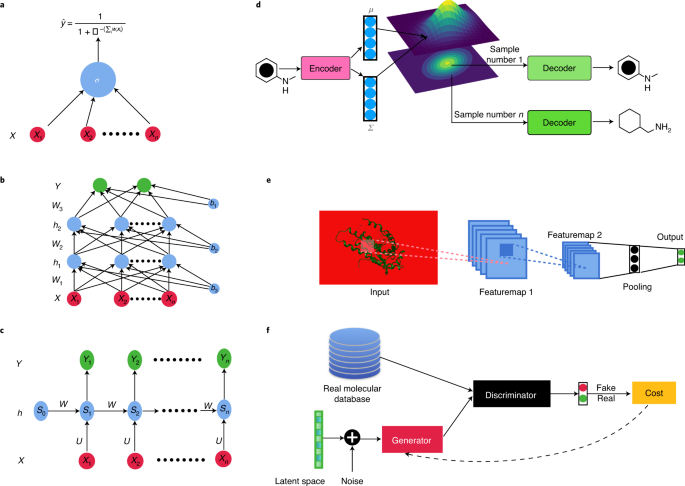
a , Sigmoid neuron as a building block for neural networks. A sigmoid neuron is a perceptron with sigmoid nonlinearity. b , A fully connected feed-forward neural network (MLP) consists of an input layer, hidden layer(s) and output layer with non-linear activations such as sigmoid. X and Y represent input and output, respectively, from the models. h , hidden layer; b , bias term. c , A simplified unfolded representation of an RNN. U and W are trainable model parameters; S i is the latent state at the ‘ith’ timestep of an RNN input. d , VAE. A probabilistic encoder maps the input into a latent space under a Gaussian assumption. µ and ∑ are the parameter vectors of learned multivariate Gaussian distribution. Samples are drawn from this latent space and decoder attempts to reconstruct original input from these samples. e , CNN. Kernels are convolved over input image and subsequently over feature maps to progressively generate higher-order feature maps. Pooling further reduces the dimensionality of the feature maps. f , GAN. The discriminator and generator are two arbitrary neural networks that compete in a zero-sum game to synthetically generate new samples. These large-capacity DL models cannot be reasonably trained without using a hardware accelerator such as a GPU. It is implied (unless otherwise stated) that such models are deployed on GPUs.
Multilayer perceptrons (MLPs) are fully connected networks with input, hidden and output layer(s) and nonlinear activation functions (sigmoid, tanh, ReLU (rectified linear unit) and so on) that are the basis of DNNs 50 . Their large learning capacity and relatively small numbers of parameters made MLPs the earliest successful application of artificial neural networks in drug discovery for QSAR studies 51 . Modern GPU machines render MLPs inexpensive models that are suitable for the large cheminformatics datasets that are having a renewed impact on CADD 52 .
Arguably the most utilized DNNs, CNNs are guided by hierarchical principles and utilize small receptive fields to process local subsections of the input. CNNs have been the go-to architecture for image and video processing, while they also enable success in biomedical text classification 53 . A typical CNN operates on a 3D volume (height, width, channel), generates translation-invariant feature maps based on learnable kernels and pools these maps to produce scale- and rotation-invariant outputs.
The parallelizable nature of convolution operation makes CNNs suitable for implementation on GPUs. The Toxic Color 54 method was first developed with the Tox21 benchmark data using simple 2D drawings of chemicals, demonstrating that GPU-enabled CNN predictions, without employing any chemical descriptors, were comparable to state-of-the-art machine learning methods. Goh et al. 55 subsequently introduced Chemception, a CNN trained on molecular drawings to predict chemical properties such as toxicity, activity and solvation, which showed comparable performance to MLPs trained with extended-connectivity fingerprints. Their model was further improved by encoding atom- and bond-specific chemical information into the CNN 55 .
Historically, computational chemists have relied extensively on topological fingerprints such as extended-connectivity fingerprints 56 or other descriptors for molecular characterization 57 . One popular linear Goh representation is SMILES (simplified molecular input line entry system) 58 . String representations of fixed length are useful because they can be treated as sequences and efficiently modelled within temporal networks such as recurrent neural networks (RNNs). RNNs may be viewed as an extension of Markov chains with memory that are capable of learning long-range dependencies through its internal states, and hence modelling autoregression in molecular sequences.
The capacity of DL algorithms to learn latent internal representations for the input molecules without the need for hand-crafted descriptors allows syntactically and semantically meaningful representations specific to the dataset and problem at hand. SMILES2vec 59 was trained to learn continuous embeddings from SMILES representations to make predictions for several datasets and tasks (toxicity, activity, solvation and solubility). The lower dimensionality of these vectors speeds training and reduces memory requirements—both of which are critical aspects of training neural networks. Inspired by the success of popular word-embedding algorithm word2vec, Jaeger et al. 60 developed mol2vec. Based on unsupervised pretraining of word2vec on ZINC and ChEMBL datasets, the learned representations achieved state-of-the-art performance and were better suited to regression tasks than Morgan fingerprints.
Variational autoencoders (VAEs) 61 are deep generative models that are revolutionizing cheminformatics owing to their capacity to probabilistically learn latent space from observed data that can later be sampled to generate new molecules with fine-tuned functional properties. VAEs support direct sampling, and hence generation, of molecules from a learned distribution over the latent space without the need for expensive Monte Carlo sampling. Blaschke et al. 62 generated new molecules targeting dopamine receptor 2 using a VAE model. These molecules were further validated using a support vector machine model trained for activity prediction. Sattarov et al. 63 explored Seq2Seq VAEs to selectively design compounds with desired properties. A generative topographic mapping was used to sample from the latent representation learned by the VAE. Other studies investigated VAEs in conjunction with molecular graphs to generate new molecules 64 .
Recently, generative adversarial networks (GANs) have established themselves as powerful and diverse deep generative models. GANs are based on an adversarial game between a generator and a discriminator module. The objective of the discriminator network is to differentiate between real and fake datapoints generated by the generator network. A concurrently trained generator network attempts to create novel datapoints such that the discriminator is manipulated into believing the generated results to be real. Following the empirical success of GANs, several improvements and modifications were proposed 65 . These methods were promptly utilized by researchers in drug discovery to artificially synthesize data across subproblems 66 . Méndez-Lucio et al. 67 investigated a GAN-based generative modelling approach at the intersection of systems biology and molecular drug design. Their attempt to bring biology and chemistry together was demonstrated in the generation of active-like molecules given the gene expression signature of the target. To this end, they used a combination of conditional GANs and a Wasserstein GAN with a gradient penalty. GANs have also been explored in conjunction with genetic algorithms to combat mode collapse and hence incrementally explore a larger chemical space 68 .
Transformer networks
Inspired by tremendous success of the use of transformer networks 69 in natural language processing, DL researchers in drug discovery were motivated to explore its power for training long-term dependencies for sequences. Using self-attention, Shin et al. 70 performed end-to-end neural regressions to predict affinity scores between drug molecules and target proteins. In doing so, they learned molecular representations for the drug molecules by aggregating molecular token embedding with position embedding, as well as learning new representations for proteins using a CNN. In the same vein, Huang et al. 71 introduced MolTrans to predict drug–target interactions. Grechishnikova formulated target-specific molecular generation as a translation task between amino acid chains and their SMILES representations using a transformer encoder and decoder 72 .
A recent innovation in the use of DL on non-Euclidean data such as graphs, point clouds and manifolds promoted graph neural networks (GNNs) 71 . The central form taken by the majority of GNN variants is neural message parsing in which messages from each node in the graph are exchanged and updated iteratively using neural networks, thereby generating robust representations. PyTorch Geometric 73 provides CUDA kernels for message parsing APIs by leveraging sparse GPU acceleration. Deep Graph Library-LifeSci 74 unifies several seminal works to introduce a platform-agnostic API for the easy integration of GNNs in life sciences with a particular focus on drug discovery. The mathematical representation for graphs succinctly captures the graphical structure of molecules, meaning that GNNs are potentially of great use in CADD.
Duvenaud et al. 75 showed that learned graph representations for drugs outperform circular fingerprints on several benchmark datasets. Inspired by gated GNNs, PotentialNet 76 showed improved performance at ligand-based multitasks (electronic property, solubility and toxicity prediction). Several other studies demonstrated improved predictive performance when geometric features such as atomic distances were also considered 77 . Torng et al. 78 used graph autoencoders to learn protein representations from their amino acid residues, along with graph representations of protein pockets. These vectors were then concatenated with graph representations for drug molecules and fed into an MLP to predict drug–protein associations. Gao et al. 79 learned protein and drug embeddings using RNNs and GNNs on protein sequences and atomic graphs of drugs, respectively. One popular approach to the repurposing of drugs involves the completion of knowledge graphs; these large knowledge graphs are built from the known similarities between diseases, drugs and indications 80 . Gaudelet et al. presented an extensive review of GNNs for CADD applications 81 .
Reinforcement learning
Reinforcement learning is a branch of AI that simulates decision-making through the optimization of reward- and penalty-based policies. With the penetration of DL, deep reinforcement learning has found applications in CADD, particularly in de novo drug design, by enabling molecules to have desired chemical properties 82 , 83 . Deep reinforcement learning trained on GNNs was further shown to improve the validity of the molecular structures generated 84 . Enforcing chemically meaningful actions simultaneously with optimizing rewards around chemical properties generates useful leads while imparting chemistry domain knowledge to otherwise largely black-box DL solutions 85 .
Scaling up virtual screening with GPUs and DL
Structure-based virtual screening and ligand-based virtual screening aim to rank chemical compounds on the basis of their computed binding affinity to a target, and to extrapolate structural similarities between small molecules to functional equivalence, respectively. With the exponential growth of purchasable ligand libraries, already comprising tens of billions of synthesizable molecules 86 , there is increasing interest in expanding the scale at which conventional virtual screening operates with the parallelization of docking calculations or DL-based acceleration.
A number of structure-based virtual screening methods have been developed recently to efficiently screen billion-entry chemical libraries. VirtualFlow 87 represents the first example of such platforms, allowing a billion molecules to be screened on large CPU clusters (~10,000 cores) in a couple of weeks while displaying a linear scaling behaviour. Differently from VirtualFlow and other CPU-based methods 88 , GPU acceleration of docking algorithms using OpenCL and CUDA libraries has partially addressed the high-throughput bottleneck by dividing the whole protein surface into arbitrary independent regions (or spots) 89 or by combining both multicore CPU architectures and GPU accelerators in heterogeneous computing systems 90 . A recent example of such strategies is Autodock-GPU, which allows a billion molecules to be screened in a day on large GPU clusters such as the Summit supercomputer (~27,000 GPUs) by parallelizing the pose search process 91 . These approaches that leverage GPU computing on high-performance computing will therefore probably become instrumental in identifying novel lead compounds from large, diverse chemical libraries, or accelerating other structure-based methods such as inverse docking 92 . Still, the costs of computing remain high and can be prohibitive for drug discovery organizations that cannot access elite supercomputing clusters.
On the other hand, alternative structure-based virtual screening platforms have recently emerged, leveraging DL predictions and molecular docking to boost the selection of active compounds from large libraries with limited computational resources. The common strategy among these methods is the implementation of DL emulators of classical computational screening scores that rely on an order-of-magnitude higher inference speed than conventional docking. Predictive DL models are built using a variety of chemical structure representations, from molecular fingerprints to more sophisticated embeddings, to filter out large portions of a chemical library. One of the earliest developed methods, Deep Docking 93 , relies on a fully connected MLP model that is trained with chemical fingerprints and scores of a small portion of a library, then used to predict the docking score classes of the remaining molecules, allowing low-ranked entries to be removed without docking them. Deep Docking was initially deployed by Ton et al. 94 to screen 1.3 billion molecules from ZINC15 using Glide against SARS-CoV-2 main protease. More recently, it was also applied sequentially on different docking programs to screen 40 billion commercially available molecules against SARS-CoV-2 main protease by Gentile et al., leading to the identification of novel experimentally confirmed inhibitor scaffolds 95 . Other similar methods have been proposed that rely on DL models that predict docking outcomes, such as MolPAL (molecular pool-based active learning) 96 and AutoQSAR/DeepChem 97 . Hofmarcher et al. 98 also performed ligand-based virtual screening on the ZINC database with over 1 billion compounds to rank potential SARS-CoV-2 inhibitors using an RNN. Compared with brute-force methods, these DL-based approaches may play an important role in making the chemical space accessible to academic research groups and small/medium industry alike.
GPU-enabled DL promotes open science and the democratization of drug discovery
The integration of DL in CADD as presented here has contributed greatly to the global democratization of drug discovery and open science efforts. The open-source DL packages DeepChem 99 , ATOM 100 , Deep Docking 93 , MolPAL 96 , OpenChem 101 , GraphInvent 102 and MOSES 103 , among others, have simplified the integration of DL strategies into drug discovery pipelines using popular machine learning libraries including (but not limited to) scikit-learn, Tensorflow and Pytorch. The growing demand for large datasets for DL models is naturally encouraging data-sharing practices and calls for broader open data policies. Furthermore, GPU acceleration in cloud-native computing and micro-service-oriented architectures could make CADD methods free and widely available, contributing to standardizing computational modules and tools, as well as architectures, platforms and user interfaces. DL solutions can take advantage of public cloud services such as Amazon Web Services, Google Cloud Platform and Microsoft Azure to boost drug discovery by reducing the cost.
As exciting as these new DL-enabled modelling opportunities are, CADD scientists need to be cautious about the expected impact of DL technologies. Realistic expectations need to be derived from the lessons learned and best practices developed during more than 20 years of data-driven molecular modelling 104 . For example, the quality, quantity and diversity of data can hamper not only the accuracy but also the overall generality of CADD models. Thus, data cleaning and curation will continue to play a major role that can alone determine the success or failure of such DL applications 104 . On the other hand, the use of of dynamic datasets derived from guided experiments or high-level computer simulations can facilitate the utilization of active learning strategies. Interactive training and validation can substantially improve model quality, as implemented by the AutoQSAR tool 105 . Beyond predictive models, DL solutions are particularly useful when combining generative models and RL-based decision-making approaches. An optimization of reward- and penalty-based rules could enable unprecedented ‘à la carte’ design of chemical structures with desired chemical and functional properties 82 , 83 . This method of simultaneously enforcing chemically and biologically meaningful actions into de novo drug design represents a drastic departure from the more traditional black-box DL solutions.
Open science efforts are benefiting from recent end-to-end DL models that can be implemented at all stages of drug discovery using GPUs 106 . One such recently developed platform is IMPECABLE 107 , which integrates multiple CADD methods. Al Saadi et al. 107 combined the strength of molecular dynamics in predicting binding free energies with the strength of docking in pose prediction. Their solution automates not just virtual screening, but also lead refinement and optimization.
NVIDIA Clara Discovery is a collection of GPU-accelerated frameworks, tools and applications for computational drug discovery spanning molecular simulation, virtual screening, quantum chemistry, genomics, microscopy and natural language processing 108 . These platforms are intended to be open and cross-compatible, and are expected to accelerate the integration of different data sources across the biopharmaceutical spectrum from research papers, patient records, symptoms and biomedical images to genes, proteins and drug candidates.
Many major hardware producers now use their computing expertise to enter the realm of supercomputing by employing multiple GPU clusters to train large-capacity DL models for reaction prediction, molecular optimization and de novo molecular generation. The adoption of DL emulation of pharmaceutical endpoints 93 by CADD platforms can make drug discovery on libraries containing tens of billions of compounds affordable, even for small companies and academic labs without access to elite computational facilities.
Owing to the legal complexities, sharing of proprietary data between institutions continues to act as a bottleneck in streamlined drug discovery research. Federated learning allows participating institutions to perform localized training on their respective unshared data. Trained local models are then aggregated in a central server for broader accessibility. Federated learning thus supports democratization by alleviating data-exchange challenges to some degree, although effective model aggregation remains an active area of research.
Conclusions and outlook
Modern drug discovery has benefited from the recent explosion of DL models and GPU parallel computing. Driven by hardware advances, DL has demonstrated excellence in drug discovery problems ranging from virtual screening and QSAR analysis to generative drug design. De novo drug design in particular has been one of the major beneficiaries of advancements in GPU computation as it leverages large capacity and highly parameterized models such as VAE and GANs that cannot be reasonably deployed without using hardware accelerators such as GPUs. The ever-improving price-to-performance ratio of GPU hardware, reliance of DL on GPU and wide adoption of DL in CADD in recent years are all evident from the fact that over 50% of all ‘AI in chemistry’ documents in CAS Content Collection have been published in the past 4 years (ref. 109 ). Furthermore, hybrid AI methods have been adopted that combine conventional molecular simulations with DL for fast and accurate screening of ultra-large chemical libraries approaching hundreds of billions of molecules. We expect that the growing availability of increasingly powerful GPU architectures, together with the development of advanced DL strategies and GPU-accelerated algorithms, will help to make drug discovery affordable and accessible to the broader scientific community worldwide.
Another key driver of DL algorithms is the availability of ‘big data’. With the growing ease of genetic sequencing and high-throughput screening, large volumes of pristine data are now readily available to researchers in data-driven computational chemistry. However, the high-quality labelled data that are essential for supervised learning methods are still expensive to curate. Methods that build on learning from auxiliary datasets, knowledge transfer using transfer learning and label-conservative methods such as zero-shot learning have thus become a central piece of DL for drug discovery. The reliability and generalizability of any DL method developed for drug discovery critically depends on the quality of the sourced data. Thus, data cleaning and curation play a major role that can solely define the success or failure of such DL applications 110 and, consequently, in-depth exploration of the putative benefits of centralized, processed and well-labelled data repositories remains an open field of research.
Overall, researchers in drug discovery and machine learning have efficiently collaborated to identify CADD subproblems and corresponding DL tools. We believe that the next few years will see these applications be fine-tuned and mature, and this collaboration will further evolve to other underexplored areas of the life sciences. As such, federated learning and collaborative machine learning are gaining traction, and we believe they will be the forebears of the democratized drug discovery revolution.
Stone, J. E. et al. Accelerating molecular modeling applications with graphics processors. J. Comput. Chem. 28 , 2618–2640 (2007).
Article Google Scholar
LeCun, Y., Bengio, Y. & Hinton, G. Deep learning. Nature 521 , 436–444 (2015). This Review article succinctly captures key areas of DL and the most popular architectural paradigms used across domains and modalities .
ROCm, a New Era in Open GPU Computing (AMD Corporation, 2021); https://rocm.github.io/rocncloc.html
Shafie Khorassani, K. et al. Designing a ROCm-aware MPI library for AMD GPUs: early experiences. In High Performance Computing Lecture Notes in Computer Science Vol. 12728 (eds. Chamberlain, B. L., Varbanescu, A.-L., Ltaief, H. & Luszczek, P.) 118–136 (Springer, 2021).
AMD Instinct MI Series Accelerators (AMD Corporation, 2021); https://www.amd.com/en/graphics/instinct-server-accelerators
NVIDIA A100 Tensor Core GPU (NVIDIA Corporation, 2021); https://www.nvidia.com/en-us/data-center/a100/
Vamathevan, J. et al. Applications of machine learning in drug discovery and development. Nat. Rev. Drug Discov. 18 , 463–477 (2019).
Harvey, M. J. & De Fabritiis, G. High-throughput molecular dynamics: the powerful new tool for drug discovery. Drug Discov. Today 17 , 1059–1062 (2012).
Case, D. A. et al. The Amber biomolecular simulation programs. J. Comput. Chem. 26 , 1668–1688 (2005).
Abraham, M. J. et al. GROMACS: high performance molecular simulations through multi-level parallelism from laptops to supercomputers. SoftwareX 1–2 , 19–25 (2015).
Phillips, J. C. et al. Scalable molecular dynamics with NAMD. J. Comput. Chem. 26 , 1781–1802 (2005).
Nyland, L. et al. Achieving scalable parallel molecular dynamics using dynamic spatial domain decomposition techniques. J. Parallel Distrib. Comput. 47 , 125–138 (1997).
Phillips, J. C. et al. Scalable molecular dynamics on CPU and GPU architectures with NAMD. J. Chem. Phys. 153 , 44130 (2020).
Abel, R., Wang, L., Harder, E. D., Berne, B. J. & Friesner, R. A. Advancing drug discovery through enhanced free energy calculations. Acc. Chem. Res. 50 , 1625–1632 (2017).
Yoo, P. et al. Neural network reactive force field for C, H, N, and O systems. NPJ Comput. Mater. 7 , 9 (2021).
Zubatyuk, R., Smith, J.S., Leszczynski, J. & Isayev, O. Accurate and transferable multitask prediction of chemical properties with an atoms-in-molecules neural network. Sci. Adv. 5 , eaav6490 (2021).
Wang, L. et al. Accurate and reliable prediction of relative ligand binding potency in prospective drug discovery by way of a modern free-energy calculation protocol and force field. J. Am. Chem. Soc. 137 , 2695–2703 (2015).
Devereux, C. et al. Extending the applicability of the ANI deep learning molecular potential to sulfur and halogens. J. Chem. Theory Comput. 16 , 4192–4202 (2020).
Noé, F., Tkatchenko, A., Müller, K. R. & Clementi, C. Machine learning for molecular simulation. Ann. Rev. Phys. Chem. 71 , 361–390 (2020).
Ribeiro, J. M. L., Bravo, P., Wang, Y. & Tiwary, P. Reweighted autoencoded variational Bayes for enhanced sampling (RAVE). J. Chem. Phys. 149 , 72301 (2018).
Lamim Ribeiro, J. M. & Tiwary, P. Toward achieving efficient and accurate ligand-protein unbinding with deep learning and molecular dynamics through RAVE. J. Chem. Theory Comput. 15 , 708–719 (2019).
Smith, Z., Ravindra, P., Wang, Y., Cooley, R. & Tiwary, P. Discovering protein conformational flexibility through artificial-intelligence-aided molecular dynamics. J. Phys. Chem. B 124 , 8221–8229 (2020).
Drew Bennett, W. F. et al. Predicting small molecule transfer free energies by combining molecular dynamics simulations and deep learning. J. Chem. Inf. Model. 60 , 5375–5381 (2020).
von Lilienfeld, O. A. Quantum machine learning in chemical compound space. Angew. Chem. Int. Ed. 57 , 4164–4169 (2018).
Gao, X., Ramezanghorbani, F., Isayev, O., Smith, J. S. & Roitberg, A. E. TorchANI: a free and open source PyTorch-based deep learning implementation of the ANI neural network potentials. J. Chem. Inf. Model. 60 , 3408–3415 (2020).
Doerr, S. et al. TorchMD: a deep learning framework for molecular simulations. J. Chem. Theory Comput. 17 , 2355–2363 (2021).
Lahey, S. L. J. & Rowley, C. N. Simulating protein-ligand binding with neural network potentials. Chem. Sci. 11 , 2362–2368 (2020).
Vingelmann, P. & Fitzek, F. H. P. CUDA release 10.2.89 (NVIDIA, 2020).
Stone, J. E., Gohara, D. & Shi, G. OpenCL: a parallel programming standard for heterogeneous computing systems. Comput. Sci. Eng. 12 , 66–72 (2010).
Ufimtsev, I. S. & Martínez, T. J. Quantum chemistry on graphical processing units. 1. Strategies for two-electron integral evaluation. J. Chem. Theory Comput. 4 , 222–231 (2008).
Asadchev, A. & Gordon, M. S. New multithreaded hybrid CPU/GPU approach to Hartree–Fock. J. Chem. Theory Comput. 8 , 4166–4176 (2012).
Seritan, S. et al. TeraChem: a graphical processing unit-accelerated electronic structure package for large-scale ab initio molecular dynamics. Wiley Interdiscip. Rev. Comput. Mol. Sci. 11 , e1494 (2021).
Yu, J. K., Liang, R., Liu, F. & Martínez, T. J. First-principles characterization of the elusive I fluorescent state and the structural evolution of retinal protonated Schiff base in bacteriorhodopsin. J. Am. Chem. Soc. 141 , 18193–18203 (2019).
Ehrlich, S., Göller, A. H. & Grimme, S. Towards full quantum-mechanics-based protein-ligand binding affinities. ChemPhysChem 18 , 898–905 (2017).
Kowalski, K. et al. From NWChem to NWChemEx: evolving with the computational chemistry landscape. Chem. Rev. 121 , 4962–4998 (2021).
Banerjee, S. et al. 2.3 Å resolution cryo-EM structure of human p97 and mechanism of allosteric inhibition. Science 351 , 871–875 (2016).
Matsumoto, S. et al. Extraction of protein dynamics information from cryo-EM maps using deep learning. Nat. Mach. Intell. 3 , 153–160 (2021).
Al-Azzawi, A. et al. DeepCryoPicker: fully automated deep neural network for single protein particle picking in cryo-EM. BMC Bioinform. 21 , 509 (2020).
Tunyasuvunakool, K. et al. Highly accurate protein structure prediction for the human proteome. Nature 596 , 590–596 (2021).
Markoff, J. Scientists see advances in deep learning a part of artificial intelligence. New York Times (23 November 2012).
Dahl, G. E., Jaitly, N. & Salakhutdinov, R. Multi-task neural networks for QSAR predictions. Preprint at https://arxiv.org/abs/1406.1231 (2014). Inspired by the winning solution of the Merck QSAR competition, this work used neural networks to predict activities of compounds for multiple assays. This was a pivotal work in popularizing DL in drug discovery.
Yang, M. et al. Linking drug target and pathway activation for effective therapy using multi-task learning. Sci. Rep. 8 , 18322 (2018).
Google Scholar
Lee, K. & Kim, D. In-silico molecular binding prediction for human drug targets using deep neural multi-task learning. Genes 10 , 906 (2019).
Tan, M. Prediction of anti-cancer drug response by kernelized multi-task learning. Artif. Intell. Med. 73 , 70–77 (2016).
Yuan, H., Paskov, I., Paskov, H., González, A. J. & Leslie, C. S. Multitask learning improves prediction of cancer drug sensitivity. Sci. Rep. 6 , 31619 (2016).
Simões, R. S., Maltarollo, V. G., Oliveira, P. R. & Honorio, K. M. Transfer and multi-task learning in QSAR modeling: advances and challenges. Front. Pharmacol. 9 , 74 (2018).
Burki, T. A new paradigm for drug development. Lancet Digit. Heal. 2 , e226–e227 (2020).
AI-discovered novel antifibrotic drug goes first-in-human. Insilico Medicine https://insilico.com/blog/fih (30 November 2021).
Richardson, P. et al. Baricitinib as potential treatment for 2019-nCoV acute respiratory disease. Lancet 395 , e30 (2020).
Ruppert, D. The elements of statistical learning: data mining, inference, and prediction. J. Am. Stat. Assoc. 99 , 567–567 (2004).
Article MathSciNet Google Scholar
Aoyama, T., Suzuki, Y. & Ichikawa, H. Neural networks applied to structure-activity relationships. J. Med. Chem. 33 , 905–908 (1990).
Bertoni, M. et al. Bioactivity descriptors for uncharacterized chemical compounds. Nat. Commun. 12 , 3932 (2021). The generation of bioactivity signatures or fingerprints is reported using a collection of DNNs derived from broadly released bioactivity data that are relevant to capturing known biological properties, showing a substantial improvement in performance across a series of biophysics and physiology activity prediction benchmarks .
Pandey, M. et al. Extraction of radiographic findings from unstructured thoracoabdominal computed tomography reports using convolutional neural network based natural language processing. PLoS ONE 15 , e0236827 (2020).
Fernandez, M. et al. Toxic colors: the use of deep learning for predicting toxicity of compounds merely from their graphic images. J. Chem. Inf. Model. 58 , 1533–1543 (2018).
Goh, G. B., Siegel, C., Vishnu, A., Hodas, N. & Baker, N. How much chemistry does a deep neural network need to know to make accurate predictions? In 2018 IEEE Winter Conference on Applications of Computer Vision 1340–1349 (IEEE, 2018).
Rogers, D. & Hahn, M. Extended-connectivity fingerprints. J. Chem. Inf. Model. 50 , 742–754 (2010).
Sahoo, S., Adhikari, C., Kuanar, M. & Mishra, B. A short review of the generation of molecular descriptors and their applications in quantitative structure property/activity relationships. Curr. Comput. Aid. Drug Des. 12 , 181–205 (2016).
Weininger, D. SMILES, a chemical language and information system. 1. Introduction to methodology and encoding rules. J. Chem. Inf. Comput. Sci. 28 , 31–36 (1988).
Goh, G. B., Hodas, N. O., Siegel, C. & Vishnu, A. SMILES2vec: an interpretable general-purpose deep neural network for predicting chemical properties. Preprint at https://arxiv.org/abs/1712.02034 (2017).
Jaeger, S., Fulle, S. & Turk, S. Mol2vec: unsupervised machine learning approach with chemical intuition. J. Chem. Inf. Model. 58 , 27–35 (2018).
Kingma, D. P. & Welling, M. Auto-encoding variational Bayes. Preprint at https://doi.org/10.48550/arXiv.1312.6114 (2013).
Blaschke, T., Olivecrona, M., Engkvist, O., Bajorath, J. & Chen, H. Application of generative autoencoder in de novo molecular design. Mol. Inf. 37 , 1700123 (2018).
Sattarov, B. et al. De novo molecular design by combining deep autoencoder recurrent neural networks with generative topographic mapping. J. Chem. Inf. Model. 59 , 1182–1196 (2019).
Samanta, B. et al. NEVAE: a deep generative model for molecular graphs. J. Mach. Learn. Res. https://www.jmlr.org/papers/volume21/19-671/19-671.pdf (2020).
Gui, J., Sun, Z., Wen, Y., Tao, D. & Ye, J. A review on generative adversarial networks: algorithms, theory, and applications. IEEE Trans. Knowl. Data Eng . https://doi.org/10.1109/TKDE.2021.3130191 (2022).
Lin, E., Lin, C.-H. & Lane, H.-Y. Relevant applications of generative adversarial networks in drug design and discovery: molecular de novo design, dimensionality reduction, and de novo peptide and protein design. Molecules 25 , 3250 (2020).
Méndez-Lucio, O., Baillif, B., Clevert, D.-A., Rouquié, D. & Wichard, J. De novo generation of hit-like molecules from gene expression signatures using artificial intelligence. Nat. Commun. 11 , 10 (2020).
Blanchard, A. E., Stanley, C. & Bhowmik, D. Using GANs with adaptive training data to search for new molecules. J. Cheminform. 13 , 14 (2021).
Ben-Baruch, E. et al. Attention Is All You Need. Adv. Neural Inf. Process. Syst. 16 , 687–694 (2019).
Shin, B., Park, S., Kang, K. & Ho, J. C. Self-attention based molecule representation for predicting drug-target interaction. In Proc. 4th Machine Learning for Healthcare Conference 106 , 230–248 (2019).
Huang, K., Xiao, C., Glass, L. M. & Sun, J. MolTrans: molecular interaction transformer for drug-target interaction prediction. Bioinformatics 37 , 830–836 (2021). A molecular interaction transformer (MolTrans) was developed that uses knowledge-inspired sub-structural pattern mining to better extract substructure semantic relations from massive unlabelled biomedical data to improve prediction of ligand–target interactions .
Grechishnikova, D. Transformer neural network for protein-specific de novo drug generation as a machine translation problem. Sci. Rep. 11 , 31619 (2021).
Fey, M. & Lenssen, J. E. Fast graph representation learning with PyTorch Geometric. Preprint at https://arxiv.org/abs/1903.02428 (2019).
Wang, M. et al. Deep Graph Library: a graph-centric, highly-performant package for graph neural networks. Preprint at https://doi.org/10.48550/arXiv.1909.01315 (2019).
Duvenaud, D. et al. Convolutional networks on graphs for learning molecular fingerprints. Adv Neural Inf. Process. Syst. https://proceedings.neurips.cc/paper/2015/file/f9be311e65d81a9ad8150a60844bb94c-Paper.pdf (2015).
Feinberg, E. N. et al. PotentialNet for molecular property prediction. ACS Cent. Sci. 4 , 1520–1530 (2018).
Klicpera, J., Groß, J. & Günnemann, S. Directional message passing for molecular graphs. Preprint at https://arxiv.org/abs/2003.03123 (2020).
Torng, W. & Altman, R. B. Graph convolutional neural networks for predicting drug-target interactions. J. Chem. Inf. Model. 59 , 4131–4149 (2019).
Gao, K. Y. et al. Interpretable drug target prediction using deep neural representation. Proc. 27th International Joint Conference on Artificial Intelligence 2018 , 3371–3377 (2018).
Yang, M., Luo, H., Li, Y. & Wang, J. Drug repositioning based on bounded nuclear norm regularization. Bioinformatics 35 , i455–i463 (2019).
Gaudelet, T. et al. Utilizing graph machine learning within drug discovery and development. Brief. Bioinform . https://doi.org/10.1093/bib/bbab159 (2021). This is an informed review of the applications of GNNs and their variants in various components of drug discovery.
Olivecrona, M., Blaschke, T., Engkvist, O. & Chen, H. Molecular de-novo design through deep reinforcement learning. J. Cheminform. 9 , 48 (2017).
Putin, E. et al. Reinforced adversarial neural computer for de novo molecular design. J. Chem. Inf. Model. 58 , 1194–1204 (2018).
You, J., Liu, B., Ying, R., Pande, V. S. & Leskovec, J. Graph convolutional policy network for goal-directed molecular graph generation. Preprint at https://doi.org/10.48550/arXiv.1806.02473 (2018).
Zhou, Z., Kearnes, S., Li, L., Zare, R. N. & Riley, P. Optimization of molecules via deep reinforcement learning. Sci. Rep. 9 , 10752 (2019).
Grygorenko, O. O. et al. Generating multibillion chemical space of readily accessible screening compounds. iScience 23 , 101681 (2020).
Gorgulla, C. et al. An open-source drug discovery platform enables ultra-large virtual screens. Nature 580 , 663–668 (2020).
Acharya, A. et al. Supercomputer-based ensemble docking drug discovery pipeline with application to Covid-19. J. Chem. Inf. Model. https://doi.org/10.1021/acs.jcim.0c01010 (2020).
McIntosh-Smith, S., Price, J., Sessions, R. B. & Ibarra, A. A. High performance in silico virtual drug screening on many-core processors. Int. J. High Perform. Comput. Appl. 29 , 119–134 (2015).
Pérez-Serrano, J., Imbernón, B., Cecilia, J. M. & Ujaldón, M. Energy-based tuning of metaheuristics for molecular docking on multi-GPUs. Concurr. Comput. 30 , e4684 (2018).
LeGrand, S. et al. GPU-accelerated drug discovery with docking on the summit supercomputer: porting, optimization, and application to COVID-19 research. In Proc. 11th ACM International Conference on Bioinformatics, Computational Biology and Health Informatics https://doi.org/10.1145/3388440.3412472 (2020).
Darme, P. et al. Amide v2: high-throughput screening based on AutoDock-GPU and improved workflow leading to better performance and reliability. Int. J. Mol. Sci. 22 , 7489 (2021).
Gentile, F. et al. Deep docking: a deep learning platform for augmentation of structure based drug discovery. ACS Cent. Sci. 6 , 939–949 (2020). The authors propose a novel DL-inspired paradigm that helps accelerate docking, enabling them to screen larger libraries .
Ton, A.-T., Gentile, F., Hsing, M., Ban, F. & Cherkasov, A. Rapid identification of potential inhibitors of SARS-CoV-2 main protease by deep docking of 1.3 billion compounds. Mol. Inf. 39 , 2000028 (2020).
Gentile, F. et al. Automated discovery of noncovalent inhibitors of SARS-CoV-2 main protease by consensus deep docking of 40 billion small molecules. Chem. Sci . https://doi.org/10.1039/D1SC05579H (2021). About 40 billion molecules were computational screened against SARS-CoV-2 main protease, returning a large number of experimentally confirmed inhibitors using a fully automated end-to-end drug discovery protocol that integrates machine learning and human expertise.
Graff, D. E., Shakhnovich, E. I. & Coley, C. W. Accelerating high-throughput virtual screening through molecular pool-based active learning. Chem. Sci. 12 , 7866–7881 (2021).
Yang, Y. et al. Efficient exploration of chemical space with docking and deep learning. J. Chem. Theory Comput. 17 , 7106–7119 (2021).
Hofmarcher, M. et al. Large-scale ligand-based virtual screening for SARS-CoV-2 inhibitors using deep neural networks. SSRN Electron. J . https://doi.org/10.2139/ssrn.3561442 (2020).
Ramsundar, B., Eastman, P., Walters, P. & Pande, V. Deep Learning for the Life Sciences: Applying Deep Learning to Genomics, Microscopy, Drug Discovery, and More (O’Reilly Media, Inc., 2019). This work attempts to democratize DL for life sciences and drug discovery by providing tools for representing data in DL-suitable formats for subsequent modelling.
Minnich, A. J. et al. AMPL: a data-driven modeling pipeline for drug discovery. J. Chem. Inf. Model. 60 , 1955–1968 (2020).
Korshunova, M., Ginsburg, B., Tropsha, A. & Isayev, O. OpenChem: a deep learning toolkit for computational chemistry and drug design. J. Chem. Inf. Model. 61 , 7–13 (2021).
Mercado, R. et al. Graph networks for molecular design. Mach. Learn. Sci. Technol 2 , 25023 (2021).
Polykovskiy, D. et al. Molecular Sets (MOSES): a benchmarking platform for molecular generation models. Front. Pharmacol. 11 , 1931 (2020).
Cherkasov, A. et al. QSAR modeling: where have you been? Where are you going to? J. Med. Chem. 57 , 4977–5010 (2014).
Dixon, S. L. et al. Medicinal chemistry AutoQSAR: an automated machine learning tool for best-practice QSAR modeling. Future Med. Chem. 8 , 1825–1839 (2016).
Ekins, S. et al. Exploiting machine learning for end-to-end drug discovery and development. Nat. Mater. 18 , 435–441 (2019).
Al Saadi, A. et al. IMPECCABLE: Integrated Modeling PipelinE for COVID Cure by Assessing Better LEads. In 50th International Conference on Parallel Processing 20 , 1–12 (ACM, 2021).
NVIDIA Clara. https://developer.nvidia.com/clara (NVIDIA Corporation, 2021).
Baum, Z. J. et al. Artificial intelligence in chemistry: current trends and future directions. J. Chem. Inf. Model . https://doi.org/10.1021/acs.jcim.1c00619 (2021).
Artrith, N. et al. Best practices in machine learning for chemistry. Nat. Chem. 13 , 505–508 (2021).
Feinberg, E. N., Joshi, E., Pande, V. S. & Cheng, A. C. Improvement in ADMET prediction with multitask deep featurization. J. Med. Chem. 63 , 8835–8848 (2020).
Klicpera, J., Yeshwanth, C. & Günnemann, S. Directional message passing on molecular graphs via synthetic coordinates. Preprint at https://doi.org/10.48550/arXiv.2111.04718 (2021).
Wieder, O. et al. A compact review of molecular property prediction with graph neural networks. Drug Disc. Today Technol. https://doi.org/10.1016/j.ddtec.2020.11.009 (2020).
Putin, E. et al. Adversarial threshold neural computer for molecular de novo design. Mol. Pharm. 15 , 4386–4397 (2018).
Samanta, B. et al. NeVAE: a deep generative model for molecular graphs. Proc. AAAI Conf. Artif. Intell. 33 , 1110–1117 (2019).
Asgari, E. & Mofrad, M. R. K. ProtVec: a continuous distributed representation of biological sequences. PLoS ONE 10 , 141287 (2015).
Imrie, F., Bradley, A. R., van der Schaar, M. & Deane, C. M. Protein family-specific models using deep neural networks and transfer learning improve virtual screening and highlight the need for more data. J. Chem. Inf. Model. 58 , 2319–2330 (2018).
Mayr, A., Klambauer, G., Unterthiner, T. & Hochreiter, S. DeepTox: toxicity prediction using deep learning. Front. Environ. Sci. 3 , 80 (2016).
Ye, Z., Yang, Y., Li, X., Cao, D. & Ouyang, D. An integrated transfer learning and multitask learning approach for pharmacokinetic parameter prediction. Mol. Pharm. 16 , 533–541 (2018).
Ashtawy, H. M. & Mahapatra, N. R. Task-specific scoring functions for predicting ligand binding poses and affinity and for screening enrichment. J. Chem. Inf. Model. 58 , 119–133 (2017).
Chen, S., Xue, D., Chuai, G., Yang, Q. & Liu, Q. FL-QSAR: a federated learning-based QSAR prototype for collaborative drug discovery. Bioinformatics 36 , 5492–5498 (2021).
Xiong, Z. et al. Facing small and biased data dilemma in drug discovery with enhanced federated learning approaches. Sci. China Life Sci . https://doi.org/10.1007/s11427-021-1946-0 (2021).
Popova, M., Isayev, O. & Tropsha, A. Deep reinforcement learning for de novo drug design. Sci. Adv. 4 , eaap7885 (2018).
Neil, D. et al. Exploring deep recurrent models with reinforcement learning for molecule design. Preprint at https://openreview.net/forum?id=HkcTe-bR- (2018).
Ståhl, N., Falkman, G., Karlsson, A., Mathiason, G. & Boström, J. Deep reinforcement learning for multiparameter optimization in de novo drug design. J. Chem. Inf. Model. 59 , 3166–3176 (2019).
Liu, R., Wang, H., Glover, K. P., Feasel, M. G. & Wallqvist, A. Dissecting machine-learning prediction of molecular activity: is an applicability domain needed for quantitative structure–activity relationship models based on deep neural networks? J. Chem. Inf. Model. 59 , 117–126 (2018).
Schwaller, P. et al. Molecular transformer: a model for uncertainty-calibrated chemical reaction prediction. ACS Cent. Sci. 5 , 1572–1583 (2019).
Zhang, Y. & Lee, A. A. Bayesian semi-supervised learning for uncertainty-calibrated prediction of molecular properties and active learning. Chem. Sci. 10 , 8154–8163 (2019).
Ryu, S., Lim, J., Hong, S. H. & Kim, W. Y. Deeply learning molecular structure-property relationships using attention- and gate-augmented graph convolutional network. Preprint at https://doi.org/10.48550/arXiv.1805.10988 (2018).
Coley, C. W. et al. A graph-convolutional neural network model for the prediction of chemical reactivity. Chem. Sci. 10 , 370–377 (2019).
Rajasekaran, S., Fiondella, L., Ahmed, M. & Ammar, R. A. (eds). Multicore Computing: Algorithms, Architectures, and Applications 1st edn (Chapman & Hall/CRC, 2013).
Li, M. et al. Scaling distributed machine learning with the parameter server. In Proc. 11th USENIX Conference on Operating Systems Design and Implementation 583–598 (USENIX Association, 2014).
Download references
Acknowledgements
This work was funded by the Canadian Institutes of Health Research (CIHR), Canadian 2019 Novel Coronavirus (2019-nCoV) Rapid Research grant numbers OV3-170631 and VR3-172639, and generous donations for COVID-19 research from TELUS, Teck Resources, the 625 Powell Street Foundation, the Tai Hung Fai Charitable Foundation and the Vancouver General Hospital Foundation. F.G. is supported by fellowships from the Canadian Institutes for Health Research (MFE-171324), Michael Smith Foundation for Health Research/VCHRI and VGH UBC Hospital Foundation (RT-2020-0408) and the Ermenegildo Zegna Foundation.
Author information
These authors contributed equally: Mohit Pandey, Michael Fernandez.
Authors and Affiliations
Vancouver Prostate Centre, Department of Urologic Sciences, University of British Columbia, Vancouver, British Columbia, Canada
Mohit Pandey, Michael Fernandez, Francesco Gentile & Artem Cherkasov
Department of Chemistry, Carnegie Mellon University, Pittsburgh, PA, USA
- Olexandr Isayev
Eshelman School of Pharmacy, University of North Carolina at Chapel Hill, Chapel Hill, NC, USA
- Alexander Tropsha
Nvidia Corporation, Santa Clara, CA, USA
Abraham C. Stern
You can also search for this author in PubMed Google Scholar
Corresponding authors
Correspondence to Abraham C. Stern or Artem Cherkasov .
Ethics declarations
Competing interests.
A.C.S. is employed by the NVIDIA corporation, a manufacturer of GPU technology. No other authors received funding from NVIDIA for this work.
Peer review
Peer review information.
Nature Machine Intelligence thanks Jeremy Smith, Leonardo Solis-Vasquez and the other, anonymous, reviewer(s) for their contribution to the peer review of this work.
Additional information
Publisher’s note Springer Nature remains neutral with regard to jurisdictional claims in published maps and institutional affiliations.
Rights and permissions
Reprints and permissions
About this article
Cite this article.
Pandey, M., Fernandez, M., Gentile, F. et al. The transformational role of GPU computing and deep learning in drug discovery. Nat Mach Intell 4 , 211–221 (2022). https://doi.org/10.1038/s42256-022-00463-x
Download citation
Received : 26 May 2021
Accepted : 15 February 2022
Published : 23 March 2022
Issue Date : March 2022
DOI : https://doi.org/10.1038/s42256-022-00463-x
Share this article
Anyone you share the following link with will be able to read this content:
Sorry, a shareable link is not currently available for this article.
Provided by the Springer Nature SharedIt content-sharing initiative
This article is cited by
- Artem Cherkasov
Nature Reviews Drug Discovery (2024)
Integrating sequence and graph information for enhanced drug-target affinity prediction
- Guanxing Chen
- Calvin Yu-Chian Chen
Science China Information Sciences (2024)
A primer on the use of machine learning to distil knowledge from data in biological psychiatry
- Thomas P. Quinn
- Jonathan L. Hess
- Stephen J. Glatt
Molecular Psychiatry (2024)
On the difficulty of validating molecular generative models realistically: a case study on public and proprietary data
- Koichi Handa
- Morgan C. Thomas
- Andreas Bender
Journal of Cheminformatics (2023)
First fully-automated AI/ML virtual screening cascade implemented at a drug discovery centre in Africa
- Gemma Turon
- Jason Hlozek
- Miquel Duran-Frigola
Nature Communications (2023)
Quick links
- Explore articles by subject
- Guide to authors
- Editorial policies
Sign up for the Nature Briefing: Translational Research newsletter — top stories in biotechnology, drug discovery and pharma.

Ordered by Date
Graphic Card Industry and Evolution Essay
Introduction, significance of graphic card industry, works cited.
A graphic card, usually, referred to as a video card or a graphic accelerator card is an electronic circuit installed on the motherboard inside the central power unit, mostly on Laptops and desktop computers (David and Shoemake 500). The pictures or images seen on the screen of a monitor are made up of many pixels, which a computer uses to create images. To create images, a computer makes use of a graphic card which decides what needs to be done regarding all the pixels. The graphic card, as a translator, extracts binary data from the motherboard to produce a picture (Fehily 28). It is on the graphic card where this translation takes place.
A graphic card contains four main components:
- Graphics processor
- Video memory
- RAMDAC (Random Access Memory Digital-to-Analogue Converter
- Driver software
Below is a diagram of a graphic card.
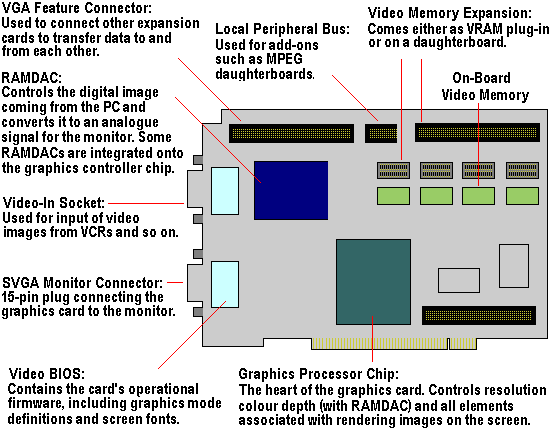
The overall performance of a computer depends on the performance of its subsystems. Video cards improve a computers performance by undertaking the largest duty of generating a picture from a model and finally presenting on the user interface, the screen, from the Central Power Unit to the Graphic card (Fehily 108). For instance, when working on large files, playing games or watching a video, a computer must be installed with a graphic card.
Video cards were developed following the invention of computers. As such, any integrated version of a computer made, would see a new version of a graphic card (David and Shoemake 700). In olden times, computers used printed cards. In the early 1960s, IBM was the first to innovate a graphic card which was aimed at replacing the printed cards. The first video card was used on a personal computer. At that time, the video cards were referred to as Monochrome Display adapters and were only applied when text mode was in use. It was an elementary kind of thing as green was the only color that appeared on the screen of a computer (Fehily 109). Later on, during the 1980s, another version of MDAs was invented which only used color red. This posed a problem since no pictures would appear to exhibit both colors. It was either green or red. Later in 1980s, several improved graphic cards were made.
Another generation of MDAs was introduced in the 1990’s and this was referred to as Video Graphic Adapter, today known as VGA. This had the ability of displaying images with different colors and would even show an image at a better resolution (Thorn 241). It was due to this innovation that many companies came into existence. A good example of a company which came into being as a result of the invention of the VGA is the Cirrus Logic.
The invention of another version of VGA, however, did not take long. Super VGA was more superior and upgraded compared to the other versions. It had 256 colors and an internal memory of 2 mega bytes (Thorn 247).
Video card development did not stop at this point and in the mid 1990s, another generation was introduced. This came in the form of 2D and 3D. 2D and 3D video cards showed graphics that were multi dimensional. The most recent innovation was the Voodoo. This was, and remains to be, the greatest innovation of all times in regards to graphic cards. It helped software developers come up with in-depth graphics with the help of a chip (Thorn 205).
Graphic cards have different internal memories and different computer applications require different memories. Those that involve low resolutions are executed using low memory graphic cards (David and Shoemake 426). A computer game usually entails high-resolution graphics and hence, using a low memory graphic card would make a game incompatible with the computer system. Therefore, to run a personal computer game, one must use a graphic card whose memory exceeds 512 megabytes. A graphic card eases the practice of playing computer. A computer system that runs a 3D title, which is above 90 frames per second, has GPU horsepower that is capacious enough and hence one could use for the improvement of the game’s image quality (Parsons and 12).
Invention of graphic cards has had a terrific significance in the life of an individual and to the society. In fact, due to its application on computers, it has led to improved lifestyles, both in social and economic perspectives (Fehily 48). The graphic card industry has led to the establishment of entities. Individuals view graphic cards as viable opportunities of starting ventures. These cards have helped companies in the entertainment industry to flourish. An example of such a company is EA GAMES. This gaming company is concerned with producing personal computers and playing station games. The initiators of this company took advantage of the innovation of graphic cards and established a gaming company (Parsons and 22). Graphic cards have not only boosted the entertainment industry, but have also employed people and, therefore, helping individuals to raise their standards of living.
It is argued that, without entertainment, human life can be immensely uninteresting. People buy visual devices such as personal computers, DVD players and television sets to be entertained. These electronic devices help improve the social life of an individual by breaking boredom (Cook and Keromytis 641). Pictures and the flow of events in the visual devices leave the person happy and entertained. As such, this is made possible by the presence of graphic cards in those devices. There would have been none if a graphic card were not in existence.
To a greater insight, graphic cards are perceived as helpful in alleviating misdemeanors of social life. An idle person is the source of evil deeds in the society and visual electronics make many people busy (Bjelland 192). Watching movies and playing computer games is vital and is a good source of entertainment. As such, one becomes glued to the screen, and thus he or she cannot think of evil deeds such as activities that cause problems to people in the society. Therefore, to some extent, graphic cards help improve security in a society and as a result, community members become safe (Cook and Keromytis 402).
Graphic cards can be attributed to the establishment of businesses. Ideas of starting entertainment spots such as play stations and cinemas arise due to visual capability of computers and other electronic devices. As such, graphic cards have proven to be social devices and entertainment spots where people, not only game but also interact. The best example is where graphic cards make people, unknown to each other, to meet and interact (Bjelland 92). They come to know each other and, that becomes the onset of a friendship. Consequently, for those using the cards to play games, it enhances their social life through knowing each other. The owners of the entertainment joints encounter diverse characters and this, not only enhances their social lives but also their economic welfare becomes proficient.
Commercial entertainment spots are recognized as elements that make up a country’s business unit. All commercial undertakings are governed by state policies such as tax policies and this helps in regulating the businesses. The entertainment spots submit monthly tax and, when the government pools these resources, a revenue base is established. As a result, different government engineered projects can be started from funds obtained from entertainment businesses associated with graphic cards. The projects are as a result, beneficial to the society. For instance, a government-engineered project includes the construction of modern roads which are beneficial to citizens in such a way that transport becomes easier and products reach a greater geographical area than it would have been if such a project was not initiated.It also becomes easier to transport agricultural products to various markets (Cook and Keromytis 511).
The graphic industry has also given rise to creativity and innovativeness when it comes to electronic dependent arts. By use of graphic cards, it is easier to come up with different aspects of art that are of high quality. Graphic cards installed in computers make it possible for cartoons are designed, and these are entertaining to the society. A person can create ideas in his mind which through the use of graphic cards can be translated into an observable piece of art.
The existence of graphic cards has led to improvements in the mobile phone industry. Old handsets were elementary and did not have pictures. The mobile industry has come up with graphic cards that are compatible with handsets and mobile phones can now display pictures and this enables people to watch visual clips (Bjelland 225). This, in turn, improves the performance of the handset and the user enjoys having the handset. Today, due to inbuilt graphic cards in mobile handsets, one can input and view videos as well as access and see pictures.
Graphic Cards are also pertinent to the advertisement industry. Advertisements are mostly used to promote events as well as promoting products. They design attractive advertisements and pass them to the public through the media. It is through graphic cards that this is made possible. As a result, the advertisements attract customers and hence the overall sales of a business entity are increased. The same case applies to advertisements that are designed for other purposes other than business purposes.
Graphic cards have also helped increase security in the society. Closed circuit televisions have been installed on buildings for security reasons (Bjelland 190). They operate in such a way that they capture everything that takes place both outside and inside their locations. They do this by showing live pictures, which help in boosting security as any ill motive is easily detected and acted accordingly.
A graphic card helps improve the understanding capabilities of learners in educational institutions. These institutions employ pictures from computers and other devices to aid or to act as teaching aids. It is said that when you learn while incorporating pictures as a learning strategy, it is easier to understand than just reading the theory. It is, therefore, due to graphic cards that pictures and videos are produced. Teachers and lecturers use the relevant ones as a way of helping impart knowledge on the students. Consequently, the students will comprehend what is being expressed, and this will last in their minds for a long time. Graphic cards, therefore, help students to remember what they learn (Bjelland 92).
Graphic cards are, however, associated with several shortcomings in the society. They are seen as chief objects that have critical influence to individuals living in the society. Graphic cards are the source of pictures seen in visual electronic devices and do not discriminate indecent or expedient contents. It is, therefore, through them that pornographic materials are produced. This has a negative influence to the individuals accessing such materials especially the young in the society.
Graphic cards encourage immorality (Null and Lobur 69). The youth after viewing immoral contents, start practicing what they see. Consequently, cases of irresponsible behaviors such as fornication and early pregnancies arise. Many people also suffer from infectious and dreadful diseases associated with sex.
The economic status of a country can be adversely affected if a large part of the population is unemployed. As depicted above, irresponsible behaviors arise resulting to layoffs in work places as well as deaths resulting from sex related diseases (Null and Lobur 326). This leads to increased unemployment levels in a country. Unemployment in any given country lowers the economic growth of a country. The deaths of people who are vital to the country, e.g. the young people, lead to loss in work forces. All these have a negative effect on a country’s economy. This argument rests on the issue of graphic cards and pornographic materials leading to economic degradation.
Computers, play stations, televisions, and other devices in which graphic cards are installed, make individuals to develop a tendency which later grows to addiction. Addiction is in itself a vice because, once a person becomes addicted, he or she forgets his responsibilities and the mind becomes unfocused. He or she becomes a social misfit (Null and Lobur 340). This is simply because he cannot get time for others because he is too much into the game. This follows a state of psychological illness.
Graphic cards are indispensable elements in the contemporary society. Through graphic cards, social and economic aspects of people have been improved, and as a result, the society has advanced. To reduce the adverse effects associated with the use of these devices on computers, different measures can be put forward. Such measures include educating the youth on the pros and cons of graphic cards and how they should use them to avoid instances of irresponsibility. This ensures that the individuals accessing materials produced as a result of graphic cards do not suffer the negative effects associated with them. It is, however, clear that graphic cards have made human life interesting through entertainment services. The invention of these cards has had a terrific significance in the life of individuals and to the society as a whole. In fact, its application on computers, has led to improved lifestyles, both in social and economic perspectives.
3DLABS Inc. Ltd. Legacy Graphic Cards . 2006. Web.
Bjelland, Harley, Using online scientific & engineering databases . Massachusetts: Morgan Kaufmann, 1992. Print
Cook, Debra and Keromytis, Angelos. Exploiting Graphics Cards for Security. Connecticut: Cengage Learning. 2010. Print.
David, Eberly and Shoemake, Ken. Game Physics . Massachusetts: Morgan Kaufmann, 2004. Print.
Fehily, Chris. Microsoft Windows Vista . California: Peach pit Press, 2008, Print.
Nul, Linda and Lobur, Julia. The essentials of computer organization and architecture . Massachusetts: Jones & Bartlett Learning, 2006. Print.
Thorn, Allan. DirectX 9 Graphics: The Definitive Guide to Direct3D. Texas: Wordware publishing Inc, 2005. Print.
- Chicago (A-D)
- Chicago (N-B)
IvyPanda. (2020, September 26). Graphic Card Industry and Evolution. https://ivypanda.com/essays/graphic-card-industry-and-evolution/
"Graphic Card Industry and Evolution." IvyPanda , 26 Sept. 2020, ivypanda.com/essays/graphic-card-industry-and-evolution/.
IvyPanda . (2020) 'Graphic Card Industry and Evolution'. 26 September.
IvyPanda . 2020. "Graphic Card Industry and Evolution." September 26, 2020. https://ivypanda.com/essays/graphic-card-industry-and-evolution/.
1. IvyPanda . "Graphic Card Industry and Evolution." September 26, 2020. https://ivypanda.com/essays/graphic-card-industry-and-evolution/.
Bibliography
IvyPanda . "Graphic Card Industry and Evolution." September 26, 2020. https://ivypanda.com/essays/graphic-card-industry-and-evolution/.
- Comparison: Nokia E73 Mode, Motorola EX115 and Samsung R360 Freeform II
- Apple Mobile Handset Improvement: Weather Resistance
- Kaixin Company's Business Environment
- Future for mobile handsets in UK
- BlackBerry Handset in Arab World
- Nokia Aims Way Beyond Handsets
- Graphics Card: Technical Description for Professionals
- Graphics Card: Technical Description for Non-Professionals
- Motorola Inc.'s Strategic Options
- Drivers of Automobiles Should Be Prohibited From Using Cellular Phones While Driving
- Life Without Computers Essay
- Graph Theory Application in Computer Science
- Paessler Router Traffic Grapher Network Monitoring
- Network Administration and Its Importance
- Qatar Intelligent Traffic Simulator in Driver Assistance

- Forums New posts Search forums
- What's new New posts Latest activity
- Members Current visitors
Research paper about graphics cards
- Thread starter Hoempapaa
- Start date Apr 7, 2007
- Graphics Cards
Distinguished
- Apr 7, 2007
Hi guys, I am a student at the technical university Eindhoven (in Holland) and I'm writing a paper about the technological trajectories of graphics cards. For this paper I'm looking for a graph that contains the speed of all, or the mayor, graphics cards from 1993 untill now. This graph could best be about the millions of triangles per second or something alike. I would be very glad if anybody here could help me finding a graph like this. Or maybe anyone nows where to find data about this so I can construct a graph by myself. Thanks!

TheGreatGrapeApe
As good as it gets IMO; http://www.techarp.com/showarticle.aspx?artno=88 http://users.erols.com/chare/video.htm
TRENDING THREADS

- Started by HarryDrews
- Today at 3:00 PM

- Started by Selynelar
- Today at 11:41 AM
- Replies: 11
- Started by jameshasty
- Today at 12:45 PM
- Started by isman77
- Today at 3:51 PM
- Started by Xbmitchx
- Today at 2:57 PM

- Started by Admin
- Yesterday at 2:25 PM
- Replies: 36

- Started by Anonymous72
- Today at 12:29 PM
Latest posts
- Latest: gnomicidal
- 6 minutes ago
- Latest: lantis3
- 7 minutes ago
- Latest: Lafong
- 8 minutes ago
- Latest: Nikzogg
- 10 minutes ago

- Latest: Teknoman2
- 11 minutes ago
- Latest: beyondlogic
- 12 minutes ago

- Latest: tennis2
Moderators online

Share this page
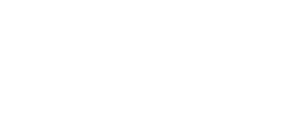
- Advertising
- Cookies Policies
- Term & Conditions

A Discrimination Report Card
We develop an empirical Bayes ranking procedure that assigns ordinal grades to noisy measurements, balancing the information content of the assigned grades against the expected frequency of ranking errors. Applying the method to a massive correspondence experiment, we grade the race and gender contact gaps of 97 U.S. employers, the identities of which we disclose for the first time. The grades are presented alongside measures of uncertainty about each firm’s contact gap in an accessible report card that is easily adaptable to other settings where ranks and levels are of simultaneous interest.
We thank Ben Scuderi for helpful feedback on an early draft of this paper and Hadar Avivi and Luca Adorni for outstanding research assistance. Seminar participants at Brown University, the 2022 California Econometrics Conference, Columbia University, CIREQ 2022 Montreal, Harvard University, Microsoft Research, Monash University, Peking University, Royal Holloway, UC Santa Barbara, UC Berkeley, The University of Virginia, the Cowles Econometrics Conference on Discrimination and Algorithmic Fairness, and The University of Chicago Interactions Conference provided useful comments. Routines for implementing the ranking procedures developed in this paper are available online at https://github.com/ekrose/drrank. The views expressed herein are those of the authors and do not necessarily reflect the views of the National Bureau of Economic Research.
Christopher Walters holds concurrent appointments as an Associate Professor of Economics at UC Berkeley and as an Amazon Scholar. This paper describes work performed at UC Berkeley and is not associated with Amazon.
MARC RIS BibTeΧ
Download Citation Data
- randomized controlled trials registry entry
- GitHub archive
Working Groups
Conferences, mentioned in the news, more from nber.
In addition to working papers , the NBER disseminates affiliates’ latest findings through a range of free periodicals — the NBER Reporter , the NBER Digest , the Bulletin on Retirement and Disability , the Bulletin on Health , and the Bulletin on Entrepreneurship — as well as online conference reports , video lectures , and interviews .
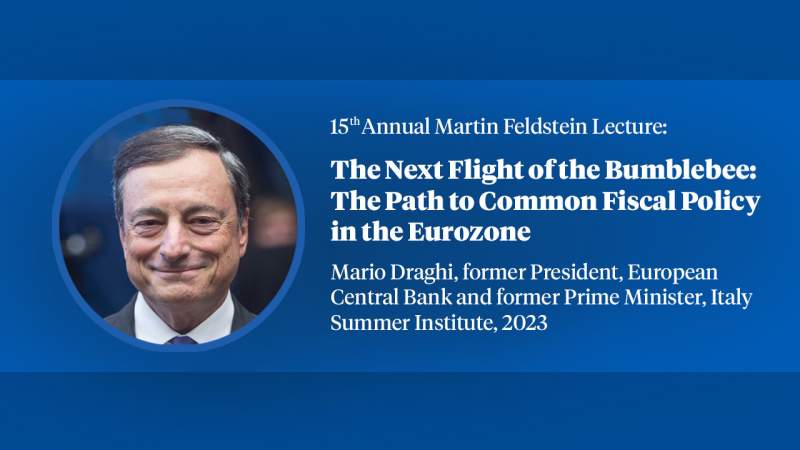
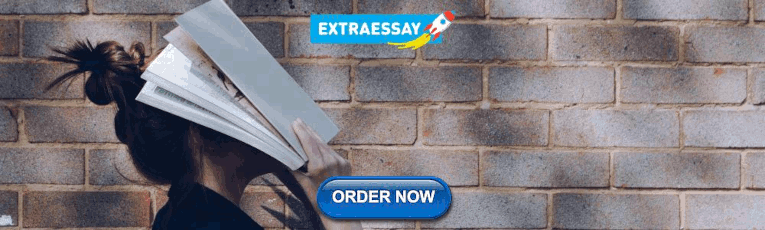
IMAGES
VIDEO
COMMENTS
This paper presents an effort to bring GPU computing closer to programmers and wider community of users. GPU computing is explored through NVIDIA Compute Unified Device Architecture (CUDA) that is ...
Published manuscript (IEEE Digital Library) Graphics processing units (GPUs) power today's fastest supercomputers, are the dominant platform for deep learning, and provide the intelligence for devices ranging from self-driving cars to robots and smart cameras. They also generate compelling photorealistic images at real-time frame rates.
Graphics processing units (GPUs) power today's fastest supercomputers, are the dominant platform for deep learning, and provide the intelligence for devices ranging from self-driving cars to robots and smart cameras. They also generate compelling photorealistic images at real-time frame rates. GPUs have evolved by adding features to support new use cases. NVIDIA's GeForce 256, the first ...
Introduction. Graphics processing units (GPUs) have for well over a decade been used for general-purpose computation, called GPU computing or GPGPU [8]. When the first GPU programs were written, the GPU was used much like a calculator: it had a set of fixed operations that were exploited to achieve some desired result.
Lanfear suggests experimenting with parallel processing on a cheaper GPU aimed at gamers and then deploying code on a more professional chip. A top-of-the-range gaming GPU can cost US$1,200 ...
Our publications provide insight into some of our leading-edge research. Research Labs . All Research Labs. 3D Deep Learning. Applied Research. Autonomous Vehicles ... Computer Graphics (294) Computer Architecture (222) Robotics (106) Circuits and VLSI Design (101) ... Best Paper Award. MedPart: A Multi-Level Evolutionary Differentiable ...
GPU-Card Performance Research in Satellite Imagery Classification Problems Using Machine Learning ... application of various machine learning models for solving practical problems largely depends on the performance of GPU cards. The paper compares the accuracy of image recognition based on the experiments with NVIDIA's GPU cards and gives ...
It's directly soldered to the graphics card's PCB. This enables remarkably fast data transport with minimal latency, allowing for high-resolution graphics rendering or deep learning model training. NVIDIA GeForce RTX 3050 VRAM Positionning . On current graphics cards, VRAM comes in a variety of sizes, speeds, and bus widths.
Examination of the Nvidia RTX. November 2019. DOI: 10.30987/graphicon-2019-2-7-12. Conference: 29th International Conference on Computer Graphics, Image Processing and Computer Vision ...
Graphics processing units (GPUs) are one of the most widely used accelerators today. As of 2021, 7 out of top 10 world-class super computers are powered by GPUs (Top500 2021).After gaining popularity in high-performance computing domain since mid-2000s, GPU has been conquering emerging domains such as deep learning, security, virtual reality, and so on by proving superior performance than ...
Top 25%. Instant Neural Graphics Primitives with a Multiresolution Hash Encoding. Thomas Müller, Alex Evans, Christoph Schied, Alex Keller. ACM Transactions on Graphics (SIGGRAPH 2022) Best Technical Paper, SIGGRAPH 2022, THE BEST INVENTIONS OF 2022, TIME. Unbiased and consistent rendering using biased estimators.
Explore State of the Art Generative AI, Graphics, and More. NVIDIA Research is passionate about developing the technology and finding the breakthroughs that bring positive change to the world. Beyond publishing our work in papers and at conferences, we apply it to NVIDIA solutions and services, share resources and code, and offer hands-on ...
Accelerating molecular dynamics simulations on GPUs. The development of GPU-centred molecular dynamics codes in the past decade led hundred-fold reductions in the computational costs of ...
Original Research Paper Graphics Pipeline Evolution: Problems and Solutions 1Ghazi Shakah, 1Mutasem Alkhasawneh, ... Graphics card Image DirectX OpenGL Hardware pipeline API . Ghazi Shakah et al . / Journal of Computer Science 2019, 15 (7): 880.885 DOI: 10.3844/jcssp.2019.880.885
GPU is a graphical processing unit which enables you to run high definitions graphics on your PC, which are the demand of modern computing. Like the CPU (Central Processing Unit), it is a single-chip processor. However, as shown in Fig. 1, the GPU has hundreds of cores as compared to the 4 or 8 in the latest CPUs.
In this research report, we'll examine the new Turing architecture and how its RT Cores enable real-time ray tracing. We'll discuss the enhancements to Turing's Streaming Multiprocessors, Tensor Cores, memory, I/O and more. We'll also introduce the new Turing-based RTX graphics cards, from the consumer GeForce RTX series to the ...
performance for graphics, AI, and compute workloads with exceptional architectural and power efficiency. After the baseline design for the Ada SM was established, the chip was scaled up to shatter records. Manufacturing innovations and materials research enabled NVIDIA engineers to craft a GPU with 76.3 billion transistors and 18,432 CUDA Cores
Paper (PDF) To appear in Graphics Interface 2009: Point-Based Approximate Color Bleeding Per H. Christensen July 2008 This technical memo describes a fast point-based method for computing diffuse global illumination (color bleeding). The computation is 4-10 times faster than ray tracing, uses less memory, has no noise, and its run-time does not ...
A graphic card, usually, referred to as a video card or a graphic accelerator card is an electronic circuit installed on the motherboard inside the central power unit, mostly on Laptops and desktop computers (David and Shoemake 500). The pictures or images seen on the screen of a monitor are made up of many pixels, which a computer uses to ...
Sue Walker is professor of Typography and a former head of the Department of Typography & Graphic Communication and dean of the Faculty of Arts and Humanities at the University of Reading for 10 years. She is a fellow of the Design Research Society and was a member of the art and design sub-panel for Research Assessment Exercise (RAE) 2008 and Research Excellence Framework (REF) 2014, the UK ...
18,510. Apr 7, 2007. #1. Hi guys, I am a student at the technical university Eindhoven (in Holland) and I'm writing a paper about the technological trajectories of graphics cards. For this paper I'm looking for a graph that contains the speed of all, or the mayor, graphics cards from 1993 untill now. This graph could best be about the millions ...
Therefore, this paper, aiming at the problem of imported cases, considers the problem of overturning cases, which is caused by the discordance between the stacking order and the operation order, an integer programming model with the objective functions of changing cost of reservation time window, waiting cost of outer gathering card and ...
Nagy, János. Maize (Zea mays L), as a major cereal crop produced in Hungary in addition to wheat, attracts enormous research from both educational and non-educational institutions. Research is aimed at addressing the key abiotic, biotic and social economic constraints. The stakeholders and institutions involved in research are spread all over ...
A Discrimination Report Card. Patrick M. Kline, Evan K. Rose & Christopher R. Walters. Working Paper 32313. DOI 10.3386/w32313. Issue Date April 2024. We develop an empirical Bayes ranking procedure that assigns ordinal grades to noisy measurements, balancing the information content of the assigned grades against the expected frequency of ...