- Go to ILO main site
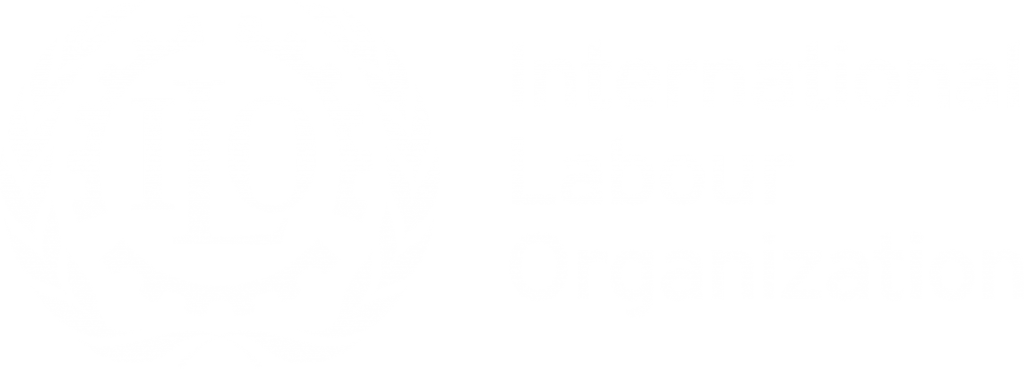
- Country profiles
Concepts and definitions
Database descriptions, statistical standards (resolutions and guidelines), and guides and manuals – all the metadata to better understand the labour statistics presented on ILOSTAT.
Forms of work: An overview of the new statistical standards
An introduction to the conceptual frameworks for forms of work and labour force statistics, including labour underutilization.
ICLS documents
Find resolutions, guidelines, meeting room documents and reports related to the International Conference of Labour Statisticians (ICLS).
Labour force survey (LFS) resources
Online resources for data producers to build or improve their labour force survey, such as PAPI and CAPI model questionnaires.
Population Census resources
Online resources for data producers to improve their population census to be in line with the latest international statistical standards for labour.
Labour market information systems (LMIS)
Learn more about LMIS and what the ILO can offer in terms of tools, capacity building activities and technical assistance in labour statistics.
Selected data tools
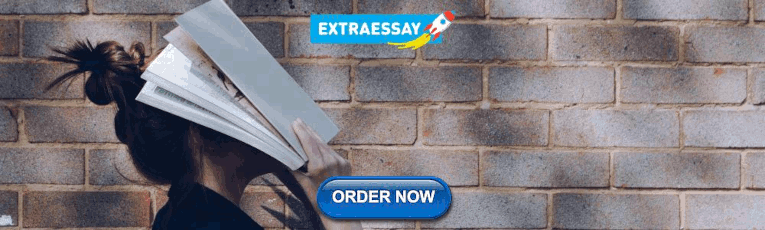
Bulk download facility
Access all ILOSTAT data, including detailed cross-tabulations and special requests not made available through other data tools. Recommended for researchers!
Quickly find, filter, pivot, map and download data in various formats.
Data explorer
Data catalogues by region

Statistics in Africa

Statistics in the Americas

Statistics in Arab States

Statistics in Asia and the Pacific

Statistics in Europe and Central Asia
International Conference of Labour Statisticians (ICLS)
The ICLS meets every 5 years to establish international standards on labour statistics. The latest (21st) ICLS was held in October 2023.
See the upcoming schedule for major events, training and workshops in labour statistics.
Capacity building and technical assistance
We provide training and support with the implementation of international statistical standards, data production, analysis and dissemination.
Learn about the ILO Department of Statistics’ role in the field of labour statistics, including contributions to capacity building and SDGs.
Standards and guidelines on labour statistics
Learn more about the international standards on labour statistics (a. conventions and recommendations and b. resolutions and guidelines).
LFS research and development
Learn more about the ILO’s programme of methodological research to identify and promote good practices in the collection and reporting of labour statistics.
Data collection and production
Discover the main methods to compile and produce ILOSTAT data: automated data collection, microdata processing and an annual questionnaire.
Dissemination and analysis
Learn about ILOSTAT dissemination tools, including those available to data producers, and analytical products.
Partnerships
The ILO seeks partners to improve the production and dissemination of labour statistics for better evidence-based policy. See our current partnerships.
Get started
Instructions on how to make best use of the ILOSTAT website to find data, methods and resources for labour statistics.
Catalogue of ILOSTAT sources
This catalogue of national data sources presented in ILOSTAT provides an overview of worldwide sources available for labour statistics.
Analytical publications
Find selected publications featuring ILOSTAT data.
Learn about the latest labour statistics trends using ILOSTAT data and get insights into methodological issues.
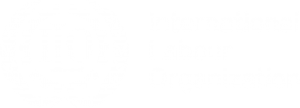
Quick Guide on Measuring Economic Characteristics in the Population Census
Thank you for visiting nature.com. You are using a browser version with limited support for CSS. To obtain the best experience, we recommend you use a more up to date browser (or turn off compatibility mode in Internet Explorer). In the meantime, to ensure continued support, we are displaying the site without styles and JavaScript.
- View all journals
- My Account Login
- Explore content
- About the journal
- Publish with us
- Sign up for alerts
- Open access
- Published: 17 April 2024
The economic commitment of climate change
- Maximilian Kotz ORCID: orcid.org/0000-0003-2564-5043 1 , 2 ,
- Anders Levermann ORCID: orcid.org/0000-0003-4432-4704 1 , 2 &
- Leonie Wenz ORCID: orcid.org/0000-0002-8500-1568 1 , 3
Nature volume 628 , pages 551–557 ( 2024 ) Cite this article
63k Accesses
3473 Altmetric
Metrics details
- Environmental economics
- Environmental health
- Interdisciplinary studies
- Projection and prediction
Global projections of macroeconomic climate-change damages typically consider impacts from average annual and national temperatures over long time horizons 1 , 2 , 3 , 4 , 5 , 6 . Here we use recent empirical findings from more than 1,600 regions worldwide over the past 40 years to project sub-national damages from temperature and precipitation, including daily variability and extremes 7 , 8 . Using an empirical approach that provides a robust lower bound on the persistence of impacts on economic growth, we find that the world economy is committed to an income reduction of 19% within the next 26 years independent of future emission choices (relative to a baseline without climate impacts, likely range of 11–29% accounting for physical climate and empirical uncertainty). These damages already outweigh the mitigation costs required to limit global warming to 2 °C by sixfold over this near-term time frame and thereafter diverge strongly dependent on emission choices. Committed damages arise predominantly through changes in average temperature, but accounting for further climatic components raises estimates by approximately 50% and leads to stronger regional heterogeneity. Committed losses are projected for all regions except those at very high latitudes, at which reductions in temperature variability bring benefits. The largest losses are committed at lower latitudes in regions with lower cumulative historical emissions and lower present-day income.
Similar content being viewed by others
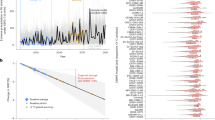
Climate damage projections beyond annual temperature
Paul Waidelich, Fulden Batibeniz, … Sonia I. Seneviratne
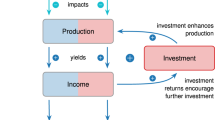
Investment incentive reduced by climate damages can be restored by optimal policy
Sven N. Willner, Nicole Glanemann & Anders Levermann
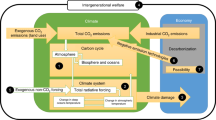
Climate economics support for the UN climate targets
Martin C. Hänsel, Moritz A. Drupp, … Thomas Sterner
Projections of the macroeconomic damage caused by future climate change are crucial to informing public and policy debates about adaptation, mitigation and climate justice. On the one hand, adaptation against climate impacts must be justified and planned on the basis of an understanding of their future magnitude and spatial distribution 9 . This is also of importance in the context of climate justice 10 , as well as to key societal actors, including governments, central banks and private businesses, which increasingly require the inclusion of climate risks in their macroeconomic forecasts to aid adaptive decision-making 11 , 12 . On the other hand, climate mitigation policy such as the Paris Climate Agreement is often evaluated by balancing the costs of its implementation against the benefits of avoiding projected physical damages. This evaluation occurs both formally through cost–benefit analyses 1 , 4 , 5 , 6 , as well as informally through public perception of mitigation and damage costs 13 .
Projections of future damages meet challenges when informing these debates, in particular the human biases relating to uncertainty and remoteness that are raised by long-term perspectives 14 . Here we aim to overcome such challenges by assessing the extent of economic damages from climate change to which the world is already committed by historical emissions and socio-economic inertia (the range of future emission scenarios that are considered socio-economically plausible 15 ). Such a focus on the near term limits the large uncertainties about diverging future emission trajectories, the resulting long-term climate response and the validity of applying historically observed climate–economic relations over long timescales during which socio-technical conditions may change considerably. As such, this focus aims to simplify the communication and maximize the credibility of projected economic damages from future climate change.
In projecting the future economic damages from climate change, we make use of recent advances in climate econometrics that provide evidence for impacts on sub-national economic growth from numerous components of the distribution of daily temperature and precipitation 3 , 7 , 8 . Using fixed-effects panel regression models to control for potential confounders, these studies exploit within-region variation in local temperature and precipitation in a panel of more than 1,600 regions worldwide, comprising climate and income data over the past 40 years, to identify the plausibly causal effects of changes in several climate variables on economic productivity 16 , 17 . Specifically, macroeconomic impacts have been identified from changing daily temperature variability, total annual precipitation, the annual number of wet days and extreme daily rainfall that occur in addition to those already identified from changing average temperature 2 , 3 , 18 . Moreover, regional heterogeneity in these effects based on the prevailing local climatic conditions has been found using interactions terms. The selection of these climate variables follows micro-level evidence for mechanisms related to the impacts of average temperatures on labour and agricultural productivity 2 , of temperature variability on agricultural productivity and health 7 , as well as of precipitation on agricultural productivity, labour outcomes and flood damages 8 (see Extended Data Table 1 for an overview, including more detailed references). References 7 , 8 contain a more detailed motivation for the use of these particular climate variables and provide extensive empirical tests about the robustness and nature of their effects on economic output, which are summarized in Methods . By accounting for these extra climatic variables at the sub-national level, we aim for a more comprehensive description of climate impacts with greater detail across both time and space.
Constraining the persistence of impacts
A key determinant and source of discrepancy in estimates of the magnitude of future climate damages is the extent to which the impact of a climate variable on economic growth rates persists. The two extreme cases in which these impacts persist indefinitely or only instantaneously are commonly referred to as growth or level effects 19 , 20 (see Methods section ‘Empirical model specification: fixed-effects distributed lag models’ for mathematical definitions). Recent work shows that future damages from climate change depend strongly on whether growth or level effects are assumed 20 . Following refs. 2 , 18 , we provide constraints on this persistence by using distributed lag models to test the significance of delayed effects separately for each climate variable. Notably, and in contrast to refs. 2 , 18 , we use climate variables in their first-differenced form following ref. 3 , implying a dependence of the growth rate on a change in climate variables. This choice means that a baseline specification without any lags constitutes a model prior of purely level effects, in which a permanent change in the climate has only an instantaneous effect on the growth rate 3 , 19 , 21 . By including lags, one can then test whether any effects may persist further. This is in contrast to the specification used by refs. 2 , 18 , in which climate variables are used without taking the first difference, implying a dependence of the growth rate on the level of climate variables. In this alternative case, the baseline specification without any lags constitutes a model prior of pure growth effects, in which a change in climate has an infinitely persistent effect on the growth rate. Consequently, including further lags in this alternative case tests whether the initial growth impact is recovered 18 , 19 , 21 . Both of these specifications suffer from the limiting possibility that, if too few lags are included, one might falsely accept the model prior. The limitations of including a very large number of lags, including loss of data and increasing statistical uncertainty with an increasing number of parameters, mean that such a possibility is likely. By choosing a specification in which the model prior is one of level effects, our approach is therefore conservative by design, avoiding assumptions of infinite persistence of climate impacts on growth and instead providing a lower bound on this persistence based on what is observable empirically (see Methods section ‘Empirical model specification: fixed-effects distributed lag models’ for further exposition of this framework). The conservative nature of such a choice is probably the reason that ref. 19 finds much greater consistency between the impacts projected by models that use the first difference of climate variables, as opposed to their levels.
We begin our empirical analysis of the persistence of climate impacts on growth using ten lags of the first-differenced climate variables in fixed-effects distributed lag models. We detect substantial effects on economic growth at time lags of up to approximately 8–10 years for the temperature terms and up to approximately 4 years for the precipitation terms (Extended Data Fig. 1 and Extended Data Table 2 ). Furthermore, evaluation by means of information criteria indicates that the inclusion of all five climate variables and the use of these numbers of lags provide a preferable trade-off between best-fitting the data and including further terms that could cause overfitting, in comparison with model specifications excluding climate variables or including more or fewer lags (Extended Data Fig. 3 , Supplementary Methods Section 1 and Supplementary Table 1 ). We therefore remove statistically insignificant terms at later lags (Supplementary Figs. 1 – 3 and Supplementary Tables 2 – 4 ). Further tests using Monte Carlo simulations demonstrate that the empirical models are robust to autocorrelation in the lagged climate variables (Supplementary Methods Section 2 and Supplementary Figs. 4 and 5 ), that information criteria provide an effective indicator for lag selection (Supplementary Methods Section 2 and Supplementary Fig. 6 ), that the results are robust to concerns of imperfect multicollinearity between climate variables and that including several climate variables is actually necessary to isolate their separate effects (Supplementary Methods Section 3 and Supplementary Fig. 7 ). We provide a further robustness check using a restricted distributed lag model to limit oscillations in the lagged parameter estimates that may result from autocorrelation, finding that it provides similar estimates of cumulative marginal effects to the unrestricted model (Supplementary Methods Section 4 and Supplementary Figs. 8 and 9 ). Finally, to explicitly account for any outstanding uncertainty arising from the precise choice of the number of lags, we include empirical models with marginally different numbers of lags in the error-sampling procedure of our projection of future damages. On the basis of the lag-selection procedure (the significance of lagged terms in Extended Data Fig. 1 and Extended Data Table 2 , as well as information criteria in Extended Data Fig. 3 ), we sample from models with eight to ten lags for temperature and four for precipitation (models shown in Supplementary Figs. 1 – 3 and Supplementary Tables 2 – 4 ). In summary, this empirical approach to constrain the persistence of climate impacts on economic growth rates is conservative by design in avoiding assumptions of infinite persistence, but nevertheless provides a lower bound on the extent of impact persistence that is robust to the numerous tests outlined above.
Committed damages until mid-century
We combine these empirical economic response functions (Supplementary Figs. 1 – 3 and Supplementary Tables 2 – 4 ) with an ensemble of 21 climate models (see Supplementary Table 5 ) from the Coupled Model Intercomparison Project Phase 6 (CMIP-6) 22 to project the macroeconomic damages from these components of physical climate change (see Methods for further details). Bias-adjusted climate models that provide a highly accurate reproduction of observed climatological patterns with limited uncertainty (Supplementary Table 6 ) are used to avoid introducing biases in the projections. Following a well-developed literature 2 , 3 , 19 , these projections do not aim to provide a prediction of future economic growth. Instead, they are a projection of the exogenous impact of future climate conditions on the economy relative to the baselines specified by socio-economic projections, based on the plausibly causal relationships inferred by the empirical models and assuming ceteris paribus. Other exogenous factors relevant for the prediction of economic output are purposefully assumed constant.
A Monte Carlo procedure that samples from climate model projections, empirical models with different numbers of lags and model parameter estimates (obtained by 1,000 block-bootstrap resamples of each of the regressions in Supplementary Figs. 1 – 3 and Supplementary Tables 2 – 4 ) is used to estimate the combined uncertainty from these sources. Given these uncertainty distributions, we find that projected global damages are statistically indistinguishable across the two most extreme emission scenarios until 2049 (at the 5% significance level; Fig. 1 ). As such, the climate damages occurring before this time constitute those to which the world is already committed owing to the combination of past emissions and the range of future emission scenarios that are considered socio-economically plausible 15 . These committed damages comprise a permanent income reduction of 19% on average globally (population-weighted average) in comparison with a baseline without climate-change impacts (with a likely range of 11–29%, following the likelihood classification adopted by the Intergovernmental Panel on Climate Change (IPCC); see caption of Fig. 1 ). Even though levels of income per capita generally still increase relative to those of today, this constitutes a permanent income reduction for most regions, including North America and Europe (each with median income reductions of approximately 11%) and with South Asia and Africa being the most strongly affected (each with median income reductions of approximately 22%; Fig. 1 ). Under a middle-of-the road scenario of future income development (SSP2, in which SSP stands for Shared Socio-economic Pathway), this corresponds to global annual damages in 2049 of 38 trillion in 2005 international dollars (likely range of 19–59 trillion 2005 international dollars). Compared with empirical specifications that assume pure growth or pure level effects, our preferred specification that provides a robust lower bound on the extent of climate impact persistence produces damages between these two extreme assumptions (Extended Data Fig. 3 ).
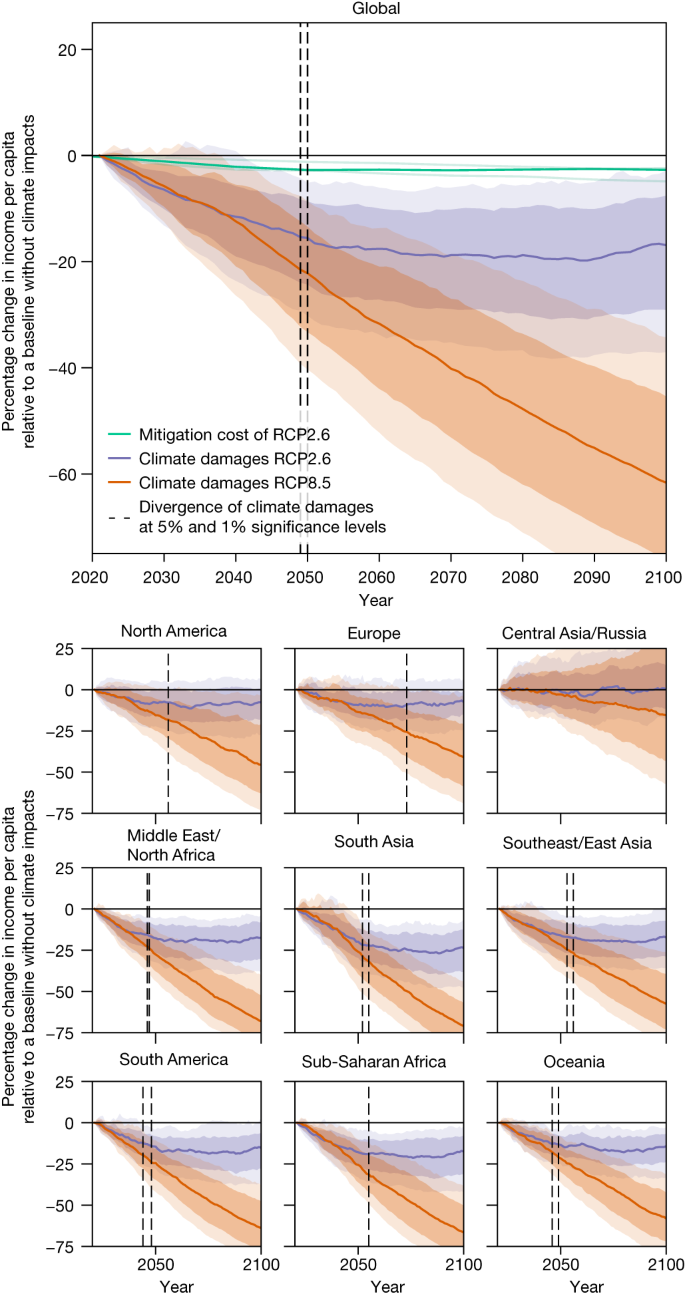
Estimates of the projected reduction in income per capita from changes in all climate variables based on empirical models of climate impacts on economic output with a robust lower bound on their persistence (Extended Data Fig. 1 ) under a low-emission scenario compatible with the 2 °C warming target and a high-emission scenario (SSP2-RCP2.6 and SSP5-RCP8.5, respectively) are shown in purple and orange, respectively. Shading represents the 34% and 10% confidence intervals reflecting the likely and very likely ranges, respectively (following the likelihood classification adopted by the IPCC), having estimated uncertainty from a Monte Carlo procedure, which samples the uncertainty from the choice of physical climate models, empirical models with different numbers of lags and bootstrapped estimates of the regression parameters shown in Supplementary Figs. 1 – 3 . Vertical dashed lines show the time at which the climate damages of the two emission scenarios diverge at the 5% and 1% significance levels based on the distribution of differences between emission scenarios arising from the uncertainty sampling discussed above. Note that uncertainty in the difference of the two scenarios is smaller than the combined uncertainty of the two respective scenarios because samples of the uncertainty (climate model and empirical model choice, as well as model parameter bootstrap) are consistent across the two emission scenarios, hence the divergence of damages occurs while the uncertainty bounds of the two separate damage scenarios still overlap. Estimates of global mitigation costs from the three IAMs that provide results for the SSP2 baseline and SSP2-RCP2.6 scenario are shown in light green in the top panel, with the median of these estimates shown in bold.
Damages already outweigh mitigation costs
We compare the damages to which the world is committed over the next 25 years to estimates of the mitigation costs required to achieve the Paris Climate Agreement. Taking estimates of mitigation costs from the three integrated assessment models (IAMs) in the IPCC AR6 database 23 that provide results under comparable scenarios (SSP2 baseline and SSP2-RCP2.6, in which RCP stands for Representative Concentration Pathway), we find that the median committed climate damages are larger than the median mitigation costs in 2050 (six trillion in 2005 international dollars) by a factor of approximately six (note that estimates of mitigation costs are only provided every 10 years by the IAMs and so a comparison in 2049 is not possible). This comparison simply aims to compare the magnitude of future damages against mitigation costs, rather than to conduct a formal cost–benefit analysis of transitioning from one emission path to another. Formal cost–benefit analyses typically find that the net benefits of mitigation only emerge after 2050 (ref. 5 ), which may lead some to conclude that physical damages from climate change are simply not large enough to outweigh mitigation costs until the second half of the century. Our simple comparison of their magnitudes makes clear that damages are actually already considerably larger than mitigation costs and the delayed emergence of net mitigation benefits results primarily from the fact that damages across different emission paths are indistinguishable until mid-century (Fig. 1 ).
Although these near-term damages constitute those to which the world is already committed, we note that damage estimates diverge strongly across emission scenarios after 2049, conveying the clear benefits of mitigation from a purely economic point of view that have been emphasized in previous studies 4 , 24 . As well as the uncertainties assessed in Fig. 1 , these conclusions are robust to structural choices, such as the timescale with which changes in the moderating variables of the empirical models are estimated (Supplementary Figs. 10 and 11 ), as well as the order in which one accounts for the intertemporal and international components of currency comparison (Supplementary Fig. 12 ; see Methods for further details).
Damages from variability and extremes
Committed damages primarily arise through changes in average temperature (Fig. 2 ). This reflects the fact that projected changes in average temperature are larger than those in other climate variables when expressed as a function of their historical interannual variability (Extended Data Fig. 4 ). Because the historical variability is that on which the empirical models are estimated, larger projected changes in comparison with this variability probably lead to larger future impacts in a purely statistical sense. From a mechanistic perspective, one may plausibly interpret this result as implying that future changes in average temperature are the most unprecedented from the perspective of the historical fluctuations to which the economy is accustomed and therefore will cause the most damage. This insight may prove useful in terms of guiding adaptation measures to the sources of greatest damage.
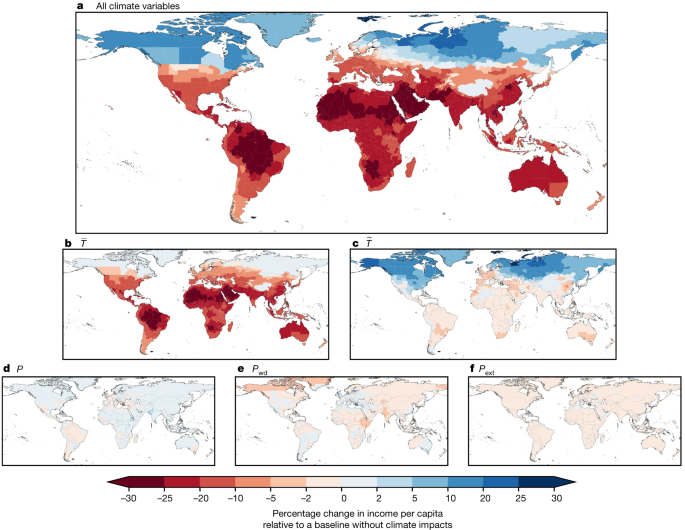
Estimates of the median projected reduction in sub-national income per capita across emission scenarios (SSP2-RCP2.6 and SSP2-RCP8.5) as well as climate model, empirical model and model parameter uncertainty in the year in which climate damages diverge at the 5% level (2049, as identified in Fig. 1 ). a , Impacts arising from all climate variables. b – f , Impacts arising separately from changes in annual mean temperature ( b ), daily temperature variability ( c ), total annual precipitation ( d ), the annual number of wet days (>1 mm) ( e ) and extreme daily rainfall ( f ) (see Methods for further definitions). Data on national administrative boundaries are obtained from the GADM database version 3.6 and are freely available for academic use ( https://gadm.org/ ).
Nevertheless, future damages based on empirical models that consider changes in annual average temperature only and exclude the other climate variables constitute income reductions of only 13% in 2049 (Extended Data Fig. 5a , likely range 5–21%). This suggests that accounting for the other components of the distribution of temperature and precipitation raises net damages by nearly 50%. This increase arises through the further damages that these climatic components cause, but also because their inclusion reveals a stronger negative economic response to average temperatures (Extended Data Fig. 5b ). The latter finding is consistent with our Monte Carlo simulations, which suggest that the magnitude of the effect of average temperature on economic growth is underestimated unless accounting for the impacts of other correlated climate variables (Supplementary Fig. 7 ).
In terms of the relative contributions of the different climatic components to overall damages, we find that accounting for daily temperature variability causes the largest increase in overall damages relative to empirical frameworks that only consider changes in annual average temperature (4.9 percentage points, likely range 2.4–8.7 percentage points, equivalent to approximately 10 trillion international dollars). Accounting for precipitation causes smaller increases in overall damages, which are—nevertheless—equivalent to approximately 1.2 trillion international dollars: 0.01 percentage points (−0.37–0.33 percentage points), 0.34 percentage points (0.07–0.90 percentage points) and 0.36 percentage points (0.13–0.65 percentage points) from total annual precipitation, the number of wet days and extreme daily precipitation, respectively. Moreover, climate models seem to underestimate future changes in temperature variability 25 and extreme precipitation 26 , 27 in response to anthropogenic forcing as compared with that observed historically, suggesting that the true impacts from these variables may be larger.
The distribution of committed damages
The spatial distribution of committed damages (Fig. 2a ) reflects a complex interplay between the patterns of future change in several climatic components and those of historical economic vulnerability to changes in those variables. Damages resulting from increasing annual mean temperature (Fig. 2b ) are negative almost everywhere globally, and larger at lower latitudes in regions in which temperatures are already higher and economic vulnerability to temperature increases is greatest (see the response heterogeneity to mean temperature embodied in Extended Data Fig. 1a ). This occurs despite the amplified warming projected at higher latitudes 28 , suggesting that regional heterogeneity in economic vulnerability to temperature changes outweighs heterogeneity in the magnitude of future warming (Supplementary Fig. 13a ). Economic damages owing to daily temperature variability (Fig. 2c ) exhibit a strong latitudinal polarisation, primarily reflecting the physical response of daily variability to greenhouse forcing in which increases in variability across lower latitudes (and Europe) contrast decreases at high latitudes 25 (Supplementary Fig. 13b ). These two temperature terms are the dominant determinants of the pattern of overall damages (Fig. 2a ), which exhibits a strong polarity with damages across most of the globe except at the highest northern latitudes. Future changes in total annual precipitation mainly bring economic benefits except in regions of drying, such as the Mediterranean and central South America (Fig. 2d and Supplementary Fig. 13c ), but these benefits are opposed by changes in the number of wet days, which produce damages with a similar pattern of opposite sign (Fig. 2e and Supplementary Fig. 13d ). By contrast, changes in extreme daily rainfall produce damages in all regions, reflecting the intensification of daily rainfall extremes over global land areas 29 , 30 (Fig. 2f and Supplementary Fig. 13e ).
The spatial distribution of committed damages implies considerable injustice along two dimensions: culpability for the historical emissions that have caused climate change and pre-existing levels of socio-economic welfare. Spearman’s rank correlations indicate that committed damages are significantly larger in countries with smaller historical cumulative emissions, as well as in regions with lower current income per capita (Fig. 3 ). This implies that those countries that will suffer the most from the damages already committed are those that are least responsible for climate change and which also have the least resources to adapt to it.
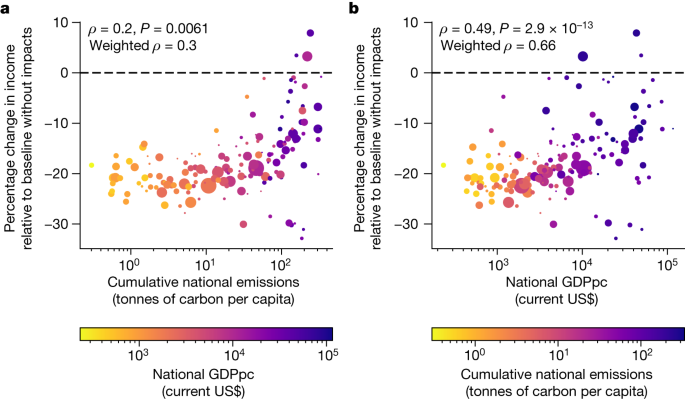
Estimates of the median projected change in national income per capita across emission scenarios (RCP2.6 and RCP8.5) as well as climate model, empirical model and model parameter uncertainty in the year in which climate damages diverge at the 5% level (2049, as identified in Fig. 1 ) are plotted against cumulative national emissions per capita in 2020 (from the Global Carbon Project) and coloured by national income per capita in 2020 (from the World Bank) in a and vice versa in b . In each panel, the size of each scatter point is weighted by the national population in 2020 (from the World Bank). Inset numbers indicate the Spearman’s rank correlation ρ and P -values for a hypothesis test whose null hypothesis is of no correlation, as well as the Spearman’s rank correlation weighted by national population.
To further quantify this heterogeneity, we assess the difference in committed damages between the upper and lower quartiles of regions when ranked by present income levels and historical cumulative emissions (using a population weighting to both define the quartiles and estimate the group averages). On average, the quartile of countries with lower income are committed to an income loss that is 8.9 percentage points (or 61%) greater than the upper quartile (Extended Data Fig. 6 ), with a likely range of 3.8–14.7 percentage points across the uncertainty sampling of our damage projections (following the likelihood classification adopted by the IPCC). Similarly, the quartile of countries with lower historical cumulative emissions are committed to an income loss that is 6.9 percentage points (or 40%) greater than the upper quartile, with a likely range of 0.27–12 percentage points. These patterns reemphasize the prevalence of injustice in climate impacts 31 , 32 , 33 in the context of the damages to which the world is already committed by historical emissions and socio-economic inertia.
Contextualizing the magnitude of damages
The magnitude of projected economic damages exceeds previous literature estimates 2 , 3 , arising from several developments made on previous approaches. Our estimates are larger than those of ref. 2 (see first row of Extended Data Table 3 ), primarily because of the facts that sub-national estimates typically show a steeper temperature response (see also refs. 3 , 34 ) and that accounting for other climatic components raises damage estimates (Extended Data Fig. 5 ). However, we note that our empirical approach using first-differenced climate variables is conservative compared with that of ref. 2 in regard to the persistence of climate impacts on growth (see introduction and Methods section ‘Empirical model specification: fixed-effects distributed lag models’), an important determinant of the magnitude of long-term damages 19 , 21 . Using a similar empirical specification to ref. 2 , which assumes infinite persistence while maintaining the rest of our approach (sub-national data and further climate variables), produces considerably larger damages (purple curve of Extended Data Fig. 3 ). Compared with studies that do take the first difference of climate variables 3 , 35 , our estimates are also larger (see second and third rows of Extended Data Table 3 ). The inclusion of further climate variables (Extended Data Fig. 5 ) and a sufficient number of lags to more adequately capture the extent of impact persistence (Extended Data Figs. 1 and 2 ) are the main sources of this difference, as is the use of specifications that capture nonlinearities in the temperature response when compared with ref. 35 . In summary, our estimates develop on previous studies by incorporating the latest data and empirical insights 7 , 8 , as well as in providing a robust empirical lower bound on the persistence of impacts on economic growth, which constitutes a middle ground between the extremes of the growth-versus-levels debate 19 , 21 (Extended Data Fig. 3 ).
Compared with the fraction of variance explained by the empirical models historically (<5%), the projection of reductions in income of 19% may seem large. This arises owing to the fact that projected changes in climatic conditions are much larger than those that were experienced historically, particularly for changes in average temperature (Extended Data Fig. 4 ). As such, any assessment of future climate-change impacts necessarily requires an extrapolation outside the range of the historical data on which the empirical impact models were evaluated. Nevertheless, these models constitute the most state-of-the-art methods for inference of plausibly causal climate impacts based on observed data. Moreover, we take explicit steps to limit out-of-sample extrapolation by capping the moderating variables of the interaction terms at the 95th percentile of the historical distribution (see Methods ). This avoids extrapolating the marginal effects outside what was observed historically. Given the nonlinear response of economic output to annual mean temperature (Extended Data Fig. 1 and Extended Data Table 2 ), this is a conservative choice that limits the magnitude of damages that we project. Furthermore, back-of-the-envelope calculations indicate that the projected damages are consistent with the magnitude and patterns of historical economic development (see Supplementary Discussion Section 5 ).
Missing impacts and spatial spillovers
Despite assessing several climatic components from which economic impacts have recently been identified 3 , 7 , 8 , this assessment of aggregate climate damages should not be considered comprehensive. Important channels such as impacts from heatwaves 31 , sea-level rise 36 , tropical cyclones 37 and tipping points 38 , 39 , as well as non-market damages such as those to ecosystems 40 and human health 41 , are not considered in these estimates. Sea-level rise is unlikely to be feasibly incorporated into empirical assessments such as this because historical sea-level variability is mostly small. Non-market damages are inherently intractable within our estimates of impacts on aggregate monetary output and estimates of these impacts could arguably be considered as extra to those identified here. Recent empirical work suggests that accounting for these channels would probably raise estimates of these committed damages, with larger damages continuing to arise in the global south 31 , 36 , 37 , 38 , 39 , 40 , 41 , 42 .
Moreover, our main empirical analysis does not explicitly evaluate the potential for impacts in local regions to produce effects that ‘spill over’ into other regions. Such effects may further mitigate or amplify the impacts we estimate, for example, if companies relocate production from one affected region to another or if impacts propagate along supply chains. The current literature indicates that trade plays a substantial role in propagating spillover effects 43 , 44 , making their assessment at the sub-national level challenging without available data on sub-national trade dependencies. Studies accounting for only spatially adjacent neighbours indicate that negative impacts in one region induce further negative impacts in neighbouring regions 45 , 46 , 47 , 48 , suggesting that our projected damages are probably conservative by excluding these effects. In Supplementary Fig. 14 , we assess spillovers from neighbouring regions using a spatial-lag model. For simplicity, this analysis excludes temporal lags, focusing only on contemporaneous effects. The results show that accounting for spatial spillovers can amplify the overall magnitude, and also the heterogeneity, of impacts. Consistent with previous literature, this indicates that the overall magnitude (Fig. 1 ) and heterogeneity (Fig. 3 ) of damages that we project in our main specification may be conservative without explicitly accounting for spillovers. We note that further analysis that addresses both spatially and trade-connected spillovers, while also accounting for delayed impacts using temporal lags, would be necessary to adequately address this question fully. These approaches offer fruitful avenues for further research but are beyond the scope of this manuscript, which primarily aims to explore the impacts of different climate conditions and their persistence.
Policy implications
We find that the economic damages resulting from climate change until 2049 are those to which the world economy is already committed and that these greatly outweigh the costs required to mitigate emissions in line with the 2 °C target of the Paris Climate Agreement (Fig. 1 ). This assessment is complementary to formal analyses of the net costs and benefits associated with moving from one emission path to another, which typically find that net benefits of mitigation only emerge in the second half of the century 5 . Our simple comparison of the magnitude of damages and mitigation costs makes clear that this is primarily because damages are indistinguishable across emissions scenarios—that is, committed—until mid-century (Fig. 1 ) and that they are actually already much larger than mitigation costs. For simplicity, and owing to the availability of data, we compare damages to mitigation costs at the global level. Regional estimates of mitigation costs may shed further light on the national incentives for mitigation to which our results already hint, of relevance for international climate policy. Although these damages are committed from a mitigation perspective, adaptation may provide an opportunity to reduce them. Moreover, the strong divergence of damages after mid-century reemphasizes the clear benefits of mitigation from a purely economic perspective, as highlighted in previous studies 1 , 4 , 6 , 24 .
Historical climate data
Historical daily 2-m temperature and precipitation totals (in mm) are obtained for the period 1979–2019 from the W5E5 database. The W5E5 dataset comes from ERA-5, a state-of-the-art reanalysis of historical observations, but has been bias-adjusted by applying version 2.0 of the WATCH Forcing Data to ERA-5 reanalysis data and precipitation data from version 2.3 of the Global Precipitation Climatology Project to better reflect ground-based measurements 49 , 50 , 51 . We obtain these data on a 0.5° × 0.5° grid from the Inter-Sectoral Impact Model Intercomparison Project (ISIMIP) database. Notably, these historical data have been used to bias-adjust future climate projections from CMIP-6 (see the following section), ensuring consistency between the distribution of historical daily weather on which our empirical models were estimated and the climate projections used to estimate future damages. These data are publicly available from the ISIMIP database. See refs. 7 , 8 for robustness tests of the empirical models to the choice of climate data reanalysis products.
Future climate data
Daily 2-m temperature and precipitation totals (in mm) are taken from 21 climate models participating in CMIP-6 under a high (RCP8.5) and a low (RCP2.6) greenhouse gas emission scenario from 2015 to 2100. The data have been bias-adjusted and statistically downscaled to a common half-degree grid to reflect the historical distribution of daily temperature and precipitation of the W5E5 dataset using the trend-preserving method developed by the ISIMIP 50 , 52 . As such, the climate model data reproduce observed climatological patterns exceptionally well (Supplementary Table 5 ). Gridded data are publicly available from the ISIMIP database.
Historical economic data
Historical economic data come from the DOSE database of sub-national economic output 53 . We use a recent revision to the DOSE dataset that provides data across 83 countries, 1,660 sub-national regions with varying temporal coverage from 1960 to 2019. Sub-national units constitute the first administrative division below national, for example, states for the USA and provinces for China. Data come from measures of gross regional product per capita (GRPpc) or income per capita in local currencies, reflecting the values reported in national statistical agencies, yearbooks and, in some cases, academic literature. We follow previous literature 3 , 7 , 8 , 54 and assess real sub-national output per capita by first converting values from local currencies to US dollars to account for diverging national inflationary tendencies and then account for US inflation using a US deflator. Alternatively, one might first account for national inflation and then convert between currencies. Supplementary Fig. 12 demonstrates that our conclusions are consistent when accounting for price changes in the reversed order, although the magnitude of estimated damages varies. See the documentation of the DOSE dataset for further discussion of these choices. Conversions between currencies are conducted using exchange rates from the FRED database of the Federal Reserve Bank of St. Louis 55 and the national deflators from the World Bank 56 .
Future socio-economic data
Baseline gridded gross domestic product (GDP) and population data for the period 2015–2100 are taken from the middle-of-the-road scenario SSP2 (ref. 15 ). Population data have been downscaled to a half-degree grid by the ISIMIP following the methodologies of refs. 57 , 58 , which we then aggregate to the sub-national level of our economic data using the spatial aggregation procedure described below. Because current methodologies for downscaling the GDP of the SSPs use downscaled population to do so, per-capita estimates of GDP with a realistic distribution at the sub-national level are not readily available for the SSPs. We therefore use national-level GDP per capita (GDPpc) projections for all sub-national regions of a given country, assuming homogeneity within countries in terms of baseline GDPpc. Here we use projections that have been updated to account for the impact of the COVID-19 pandemic on the trajectory of future income, while remaining consistent with the long-term development of the SSPs 59 . The choice of baseline SSP alters the magnitude of projected climate damages in monetary terms, but when assessed in terms of percentage change from the baseline, the choice of socio-economic scenario is inconsequential. Gridded SSP population data and national-level GDPpc data are publicly available from the ISIMIP database. Sub-national estimates as used in this study are available in the code and data replication files.
Climate variables
Following recent literature 3 , 7 , 8 , we calculate an array of climate variables for which substantial impacts on macroeconomic output have been identified empirically, supported by further evidence at the micro level for plausible underlying mechanisms. See refs. 7 , 8 for an extensive motivation for the use of these particular climate variables and for detailed empirical tests on the nature and robustness of their effects on economic output. To summarize, these studies have found evidence for independent impacts on economic growth rates from annual average temperature, daily temperature variability, total annual precipitation, the annual number of wet days and extreme daily rainfall. Assessments of daily temperature variability were motivated by evidence of impacts on agricultural output and human health, as well as macroeconomic literature on the impacts of volatility on growth when manifest in different dimensions, such as government spending, exchange rates and even output itself 7 . Assessments of precipitation impacts were motivated by evidence of impacts on agricultural productivity, metropolitan labour outcomes and conflict, as well as damages caused by flash flooding 8 . See Extended Data Table 1 for detailed references to empirical studies of these physical mechanisms. Marked impacts of daily temperature variability, total annual precipitation, the number of wet days and extreme daily rainfall on macroeconomic output were identified robustly across different climate datasets, spatial aggregation schemes, specifications of regional time trends and error-clustering approaches. They were also found to be robust to the consideration of temperature extremes 7 , 8 . Furthermore, these climate variables were identified as having independent effects on economic output 7 , 8 , which we further explain here using Monte Carlo simulations to demonstrate the robustness of the results to concerns of imperfect multicollinearity between climate variables (Supplementary Methods Section 2 ), as well as by using information criteria (Supplementary Table 1 ) to demonstrate that including several lagged climate variables provides a preferable trade-off between optimally describing the data and limiting the possibility of overfitting.
We calculate these variables from the distribution of daily, d , temperature, T x , d , and precipitation, P x , d , at the grid-cell, x , level for both the historical and future climate data. As well as annual mean temperature, \({\bar{T}}_{x,y}\) , and annual total precipitation, P x , y , we calculate annual, y , measures of daily temperature variability, \({\widetilde{T}}_{x,y}\) :
the number of wet days, Pwd x , y :
and extreme daily rainfall:
in which T x , d , m , y is the grid-cell-specific daily temperature in month m and year y , \({\bar{T}}_{x,m,{y}}\) is the year and grid-cell-specific monthly, m , mean temperature, D m and D y the number of days in a given month m or year y , respectively, H the Heaviside step function, 1 mm the threshold used to define wet days and P 99.9 x is the 99.9th percentile of historical (1979–2019) daily precipitation at the grid-cell level. Units of the climate measures are degrees Celsius for annual mean temperature and daily temperature variability, millimetres for total annual precipitation and extreme daily precipitation, and simply the number of days for the annual number of wet days.
We also calculated weighted standard deviations of monthly rainfall totals as also used in ref. 8 but do not include them in our projections as we find that, when accounting for delayed effects, their effect becomes statistically indistinct and is better captured by changes in total annual rainfall.
Spatial aggregation
We aggregate grid-cell-level historical and future climate measures, as well as grid-cell-level future GDPpc and population, to the level of the first administrative unit below national level of the GADM database, using an area-weighting algorithm that estimates the portion of each grid cell falling within an administrative boundary. We use this as our baseline specification following previous findings that the effect of area or population weighting at the sub-national level is negligible 7 , 8 .
Empirical model specification: fixed-effects distributed lag models
Following a wide range of climate econometric literature 16 , 60 , we use panel regression models with a selection of fixed effects and time trends to isolate plausibly exogenous variation with which to maximize confidence in a causal interpretation of the effects of climate on economic growth rates. The use of region fixed effects, μ r , accounts for unobserved time-invariant differences between regions, such as prevailing climatic norms and growth rates owing to historical and geopolitical factors. The use of yearly fixed effects, η y , accounts for regionally invariant annual shocks to the global climate or economy such as the El Niño–Southern Oscillation or global recessions. In our baseline specification, we also include region-specific linear time trends, k r y , to exclude the possibility of spurious correlations resulting from common slow-moving trends in climate and growth.
The persistence of climate impacts on economic growth rates is a key determinant of the long-term magnitude of damages. Methods for inferring the extent of persistence in impacts on growth rates have typically used lagged climate variables to evaluate the presence of delayed effects or catch-up dynamics 2 , 18 . For example, consider starting from a model in which a climate condition, C r , y , (for example, annual mean temperature) affects the growth rate, Δlgrp r , y (the first difference of the logarithm of gross regional product) of region r in year y :
which we refer to as a ‘pure growth effects’ model in the main text. Typically, further lags are included,
and the cumulative effect of all lagged terms is evaluated to assess the extent to which climate impacts on growth rates persist. Following ref. 18 , in the case that,
the implication is that impacts on the growth rate persist up to NL years after the initial shock (possibly to a weaker or a stronger extent), whereas if
then the initial impact on the growth rate is recovered after NL years and the effect is only one on the level of output. However, we note that such approaches are limited by the fact that, when including an insufficient number of lags to detect a recovery of the growth rates, one may find equation ( 6 ) to be satisfied and incorrectly assume that a change in climatic conditions affects the growth rate indefinitely. In practice, given a limited record of historical data, including too few lags to confidently conclude in an infinitely persistent impact on the growth rate is likely, particularly over the long timescales over which future climate damages are often projected 2 , 24 . To avoid this issue, we instead begin our analysis with a model for which the level of output, lgrp r , y , depends on the level of a climate variable, C r , y :
Given the non-stationarity of the level of output, we follow the literature 19 and estimate such an equation in first-differenced form as,
which we refer to as a model of ‘pure level effects’ in the main text. This model constitutes a baseline specification in which a permanent change in the climate variable produces an instantaneous impact on the growth rate and a permanent effect only on the level of output. By including lagged variables in this specification,
we are able to test whether the impacts on the growth rate persist any further than instantaneously by evaluating whether α L > 0 are statistically significantly different from zero. Even though this framework is also limited by the possibility of including too few lags, the choice of a baseline model specification in which impacts on the growth rate do not persist means that, in the case of including too few lags, the framework reverts to the baseline specification of level effects. As such, this framework is conservative with respect to the persistence of impacts and the magnitude of future damages. It naturally avoids assumptions of infinite persistence and we are able to interpret any persistence that we identify with equation ( 9 ) as a lower bound on the extent of climate impact persistence on growth rates. See the main text for further discussion of this specification choice, in particular about its conservative nature compared with previous literature estimates, such as refs. 2 , 18 .
We allow the response to climatic changes to vary across regions, using interactions of the climate variables with historical average (1979–2019) climatic conditions reflecting heterogenous effects identified in previous work 7 , 8 . Following this previous work, the moderating variables of these interaction terms constitute the historical average of either the variable itself or of the seasonal temperature difference, \({\hat{T}}_{r}\) , or annual mean temperature, \({\bar{T}}_{r}\) , in the case of daily temperature variability 7 and extreme daily rainfall, respectively 8 .
The resulting regression equation with N and M lagged variables, respectively, reads:
in which Δlgrp r , y is the annual, regional GRPpc growth rate, measured as the first difference of the logarithm of real GRPpc, following previous work 2 , 3 , 7 , 8 , 18 , 19 . Fixed-effects regressions were run using the fixest package in R (ref. 61 ).
Estimates of the coefficients of interest α i , L are shown in Extended Data Fig. 1 for N = M = 10 lags and for our preferred choice of the number of lags in Supplementary Figs. 1 – 3 . In Extended Data Fig. 1 , errors are shown clustered at the regional level, but for the construction of damage projections, we block-bootstrap the regressions by region 1,000 times to provide a range of parameter estimates with which to sample the projection uncertainty (following refs. 2 , 31 ).
Spatial-lag model
In Supplementary Fig. 14 , we present the results from a spatial-lag model that explores the potential for climate impacts to ‘spill over’ into spatially neighbouring regions. We measure the distance between centroids of each pair of sub-national regions and construct spatial lags that take the average of the first-differenced climate variables and their interaction terms over neighbouring regions that are at distances of 0–500, 500–1,000, 1,000–1,500 and 1,500–2000 km (spatial lags, ‘SL’, 1 to 4). For simplicity, we then assess a spatial-lag model without temporal lags to assess spatial spillovers of contemporaneous climate impacts. This model takes the form:
in which SL indicates the spatial lag of each climate variable and interaction term. In Supplementary Fig. 14 , we plot the cumulative marginal effect of each climate variable at different baseline climate conditions by summing the coefficients for each climate variable and interaction term, for example, for average temperature impacts as:
These cumulative marginal effects can be regarded as the overall spatially dependent impact to an individual region given a one-unit shock to a climate variable in that region and all neighbouring regions at a given value of the moderating variable of the interaction term.
Constructing projections of economic damage from future climate change
We construct projections of future climate damages by applying the coefficients estimated in equation ( 10 ) and shown in Supplementary Tables 2 – 4 (when including only lags with statistically significant effects in specifications that limit overfitting; see Supplementary Methods Section 1 ) to projections of future climate change from the CMIP-6 models. Year-on-year changes in each primary climate variable of interest are calculated to reflect the year-to-year variations used in the empirical models. 30-year moving averages of the moderating variables of the interaction terms are calculated to reflect the long-term average of climatic conditions that were used for the moderating variables in the empirical models. By using moving averages in the projections, we account for the changing vulnerability to climate shocks based on the evolving long-term conditions (Supplementary Figs. 10 and 11 show that the results are robust to the precise choice of the window of this moving average). Although these climate variables are not differenced, the fact that the bias-adjusted climate models reproduce observed climatological patterns across regions for these moderating variables very accurately (Supplementary Table 6 ) with limited spread across models (<3%) precludes the possibility that any considerable bias or uncertainty is introduced by this methodological choice. However, we impose caps on these moderating variables at the 95th percentile at which they were observed in the historical data to prevent extrapolation of the marginal effects outside the range in which the regressions were estimated. This is a conservative choice that limits the magnitude of our damage projections.
Time series of primary climate variables and moderating climate variables are then combined with estimates of the empirical model parameters to evaluate the regression coefficients in equation ( 10 ), producing a time series of annual GRPpc growth-rate reductions for a given emission scenario, climate model and set of empirical model parameters. The resulting time series of growth-rate impacts reflects those occurring owing to future climate change. By contrast, a future scenario with no climate change would be one in which climate variables do not change (other than with random year-to-year fluctuations) and hence the time-averaged evaluation of equation ( 10 ) would be zero. Our approach therefore implicitly compares the future climate-change scenario to this no-climate-change baseline scenario.
The time series of growth-rate impacts owing to future climate change in region r and year y , δ r , y , are then added to the future baseline growth rates, π r , y (in log-diff form), obtained from the SSP2 scenario to yield trajectories of damaged GRPpc growth rates, ρ r , y . These trajectories are aggregated over time to estimate the future trajectory of GRPpc with future climate impacts:
in which GRPpc r , y =2020 is the initial log level of GRPpc. We begin damage estimates in 2020 to reflect the damages occurring since the end of the period for which we estimate the empirical models (1979–2019) and to match the timing of mitigation-cost estimates from most IAMs (see below).
For each emission scenario, this procedure is repeated 1,000 times while randomly sampling from the selection of climate models, the selection of empirical models with different numbers of lags (shown in Supplementary Figs. 1 – 3 and Supplementary Tables 2 – 4 ) and bootstrapped estimates of the regression parameters. The result is an ensemble of future GRPpc trajectories that reflect uncertainty from both physical climate change and the structural and sampling uncertainty of the empirical models.
Estimates of mitigation costs
We obtain IPCC estimates of the aggregate costs of emission mitigation from the AR6 Scenario Explorer and Database hosted by IIASA 23 . Specifically, we search the AR6 Scenarios Database World v1.1 for IAMs that provided estimates of global GDP and population under both a SSP2 baseline and a SSP2-RCP2.6 scenario to maintain consistency with the socio-economic and emission scenarios of the climate damage projections. We find five IAMs that provide data for these scenarios, namely, MESSAGE-GLOBIOM 1.0, REMIND-MAgPIE 1.5, AIM/GCE 2.0, GCAM 4.2 and WITCH-GLOBIOM 3.1. Of these five IAMs, we use the results only from the first three that passed the IPCC vetting procedure for reproducing historical emission and climate trajectories. We then estimate global mitigation costs as the percentage difference in global per capita GDP between the SSP2 baseline and the SSP2-RCP2.6 emission scenario. In the case of one of these IAMs, estimates of mitigation costs begin in 2020, whereas in the case of two others, mitigation costs begin in 2010. The mitigation cost estimates before 2020 in these two IAMs are mostly negligible, and our choice to begin comparison with damage estimates in 2020 is conservative with respect to the relative weight of climate damages compared with mitigation costs for these two IAMs.
Data availability
Data on economic production and ERA-5 climate data are publicly available at https://doi.org/10.5281/zenodo.4681306 (ref. 62 ) and https://www.ecmwf.int/en/forecasts/datasets/reanalysis-datasets/era5 , respectively. Data on mitigation costs are publicly available at https://data.ene.iiasa.ac.at/ar6/#/downloads . Processed climate and economic data, as well as all other necessary data for reproduction of the results, are available at the public repository https://doi.org/10.5281/zenodo.10562951 (ref. 63 ).
Code availability
All code necessary for reproduction of the results is available at the public repository https://doi.org/10.5281/zenodo.10562951 (ref. 63 ).
Glanemann, N., Willner, S. N. & Levermann, A. Paris Climate Agreement passes the cost-benefit test. Nat. Commun. 11 , 110 (2020).
Article ADS CAS PubMed PubMed Central Google Scholar
Burke, M., Hsiang, S. M. & Miguel, E. Global non-linear effect of temperature on economic production. Nature 527 , 235–239 (2015).
Article ADS CAS PubMed Google Scholar
Kalkuhl, M. & Wenz, L. The impact of climate conditions on economic production. Evidence from a global panel of regions. J. Environ. Econ. Manag. 103 , 102360 (2020).
Article Google Scholar
Moore, F. C. & Diaz, D. B. Temperature impacts on economic growth warrant stringent mitigation policy. Nat. Clim. Change 5 , 127–131 (2015).
Article ADS Google Scholar
Drouet, L., Bosetti, V. & Tavoni, M. Net economic benefits of well-below 2°C scenarios and associated uncertainties. Oxf. Open Clim. Change 2 , kgac003 (2022).
Ueckerdt, F. et al. The economically optimal warming limit of the planet. Earth Syst. Dyn. 10 , 741–763 (2019).
Kotz, M., Wenz, L., Stechemesser, A., Kalkuhl, M. & Levermann, A. Day-to-day temperature variability reduces economic growth. Nat. Clim. Change 11 , 319–325 (2021).
Kotz, M., Levermann, A. & Wenz, L. The effect of rainfall changes on economic production. Nature 601 , 223–227 (2022).
Kousky, C. Informing climate adaptation: a review of the economic costs of natural disasters. Energy Econ. 46 , 576–592 (2014).
Harlan, S. L. et al. in Climate Change and Society: Sociological Perspectives (eds Dunlap, R. E. & Brulle, R. J.) 127–163 (Oxford Univ. Press, 2015).
Bolton, P. et al. The Green Swan (BIS Books, 2020).
Alogoskoufis, S. et al. ECB Economy-wide Climate Stress Test: Methodology and Results European Central Bank, 2021).
Weber, E. U. What shapes perceptions of climate change? Wiley Interdiscip. Rev. Clim. Change 1 , 332–342 (2010).
Markowitz, E. M. & Shariff, A. F. Climate change and moral judgement. Nat. Clim. Change 2 , 243–247 (2012).
Riahi, K. et al. The shared socioeconomic pathways and their energy, land use, and greenhouse gas emissions implications: an overview. Glob. Environ. Change 42 , 153–168 (2017).
Auffhammer, M., Hsiang, S. M., Schlenker, W. & Sobel, A. Using weather data and climate model output in economic analyses of climate change. Rev. Environ. Econ. Policy 7 , 181–198 (2013).
Kolstad, C. D. & Moore, F. C. Estimating the economic impacts of climate change using weather observations. Rev. Environ. Econ. Policy 14 , 1–24 (2020).
Dell, M., Jones, B. F. & Olken, B. A. Temperature shocks and economic growth: evidence from the last half century. Am. Econ. J. Macroecon. 4 , 66–95 (2012).
Newell, R. G., Prest, B. C. & Sexton, S. E. The GDP-temperature relationship: implications for climate change damages. J. Environ. Econ. Manag. 108 , 102445 (2021).
Kikstra, J. S. et al. The social cost of carbon dioxide under climate-economy feedbacks and temperature variability. Environ. Res. Lett. 16 , 094037 (2021).
Article ADS CAS Google Scholar
Bastien-Olvera, B. & Moore, F. Persistent effect of temperature on GDP identified from lower frequency temperature variability. Environ. Res. Lett. 17 , 084038 (2022).
Eyring, V. et al. Overview of the Coupled Model Intercomparison Project Phase 6 (CMIP6) experimental design and organization. Geosci. Model Dev. 9 , 1937–1958 (2016).
Byers, E. et al. AR6 scenarios database. Zenodo https://zenodo.org/records/7197970 (2022).
Burke, M., Davis, W. M. & Diffenbaugh, N. S. Large potential reduction in economic damages under UN mitigation targets. Nature 557 , 549–553 (2018).
Kotz, M., Wenz, L. & Levermann, A. Footprint of greenhouse forcing in daily temperature variability. Proc. Natl Acad. Sci. 118 , e2103294118 (2021).
Article CAS PubMed PubMed Central Google Scholar
Myhre, G. et al. Frequency of extreme precipitation increases extensively with event rareness under global warming. Sci. Rep. 9 , 16063 (2019).
Min, S.-K., Zhang, X., Zwiers, F. W. & Hegerl, G. C. Human contribution to more-intense precipitation extremes. Nature 470 , 378–381 (2011).
England, M. R., Eisenman, I., Lutsko, N. J. & Wagner, T. J. The recent emergence of Arctic Amplification. Geophys. Res. Lett. 48 , e2021GL094086 (2021).
Fischer, E. M. & Knutti, R. Anthropogenic contribution to global occurrence of heavy-precipitation and high-temperature extremes. Nat. Clim. Change 5 , 560–564 (2015).
Pfahl, S., O’Gorman, P. A. & Fischer, E. M. Understanding the regional pattern of projected future changes in extreme precipitation. Nat. Clim. Change 7 , 423–427 (2017).
Callahan, C. W. & Mankin, J. S. Globally unequal effect of extreme heat on economic growth. Sci. Adv. 8 , eadd3726 (2022).
Diffenbaugh, N. S. & Burke, M. Global warming has increased global economic inequality. Proc. Natl Acad. Sci. 116 , 9808–9813 (2019).
Callahan, C. W. & Mankin, J. S. National attribution of historical climate damages. Clim. Change 172 , 40 (2022).
Burke, M. & Tanutama, V. Climatic constraints on aggregate economic output. National Bureau of Economic Research, Working Paper 25779. https://doi.org/10.3386/w25779 (2019).
Kahn, M. E. et al. Long-term macroeconomic effects of climate change: a cross-country analysis. Energy Econ. 104 , 105624 (2021).
Desmet, K. et al. Evaluating the economic cost of coastal flooding. National Bureau of Economic Research, Working Paper 24918. https://doi.org/10.3386/w24918 (2018).
Hsiang, S. M. & Jina, A. S. The causal effect of environmental catastrophe on long-run economic growth: evidence from 6,700 cyclones. National Bureau of Economic Research, Working Paper 20352. https://doi.org/10.3386/w2035 (2014).
Ritchie, P. D. et al. Shifts in national land use and food production in Great Britain after a climate tipping point. Nat. Food 1 , 76–83 (2020).
Dietz, S., Rising, J., Stoerk, T. & Wagner, G. Economic impacts of tipping points in the climate system. Proc. Natl Acad. Sci. 118 , e2103081118 (2021).
Bastien-Olvera, B. A. & Moore, F. C. Use and non-use value of nature and the social cost of carbon. Nat. Sustain. 4 , 101–108 (2021).
Carleton, T. et al. Valuing the global mortality consequences of climate change accounting for adaptation costs and benefits. Q. J. Econ. 137 , 2037–2105 (2022).
Bastien-Olvera, B. A. et al. Unequal climate impacts on global values of natural capital. Nature 625 , 722–727 (2024).
Malik, A. et al. Impacts of climate change and extreme weather on food supply chains cascade across sectors and regions in Australia. Nat. Food 3 , 631–643 (2022).
Article ADS PubMed Google Scholar
Kuhla, K., Willner, S. N., Otto, C., Geiger, T. & Levermann, A. Ripple resonance amplifies economic welfare loss from weather extremes. Environ. Res. Lett. 16 , 114010 (2021).
Schleypen, J. R., Mistry, M. N., Saeed, F. & Dasgupta, S. Sharing the burden: quantifying climate change spillovers in the European Union under the Paris Agreement. Spat. Econ. Anal. 17 , 67–82 (2022).
Dasgupta, S., Bosello, F., De Cian, E. & Mistry, M. Global temperature effects on economic activity and equity: a spatial analysis. European Institute on Economics and the Environment, Working Paper 22-1 (2022).
Neal, T. The importance of external weather effects in projecting the macroeconomic impacts of climate change. UNSW Economics Working Paper 2023-09 (2023).
Deryugina, T. & Hsiang, S. M. Does the environment still matter? Daily temperature and income in the United States. National Bureau of Economic Research, Working Paper 20750. https://doi.org/10.3386/w20750 (2014).
Hersbach, H. et al. The ERA5 global reanalysis. Q. J. R. Meteorol. Soc. 146 , 1999–2049 (2020).
Cucchi, M. et al. WFDE5: bias-adjusted ERA5 reanalysis data for impact studies. Earth Syst. Sci. Data 12 , 2097–2120 (2020).
Adler, R. et al. The New Version 2.3 of the Global Precipitation Climatology Project (GPCP) Monthly Analysis Product 1072–1084 (University of Maryland, 2016).
Lange, S. Trend-preserving bias adjustment and statistical downscaling with ISIMIP3BASD (v1.0). Geosci. Model Dev. 12 , 3055–3070 (2019).
Wenz, L., Carr, R. D., Kögel, N., Kotz, M. & Kalkuhl, M. DOSE – global data set of reported sub-national economic output. Sci. Data 10 , 425 (2023).
Article PubMed PubMed Central Google Scholar
Gennaioli, N., La Porta, R., Lopez De Silanes, F. & Shleifer, A. Growth in regions. J. Econ. Growth 19 , 259–309 (2014).
Board of Governors of the Federal Reserve System (US). U.S. dollars to euro spot exchange rate. https://fred.stlouisfed.org/series/AEXUSEU (2022).
World Bank. GDP deflator. https://data.worldbank.org/indicator/NY.GDP.DEFL.ZS (2022).
Jones, B. & O’Neill, B. C. Spatially explicit global population scenarios consistent with the Shared Socioeconomic Pathways. Environ. Res. Lett. 11 , 084003 (2016).
Murakami, D. & Yamagata, Y. Estimation of gridded population and GDP scenarios with spatially explicit statistical downscaling. Sustainability 11 , 2106 (2019).
Koch, J. & Leimbach, M. Update of SSP GDP projections: capturing recent changes in national accounting, PPP conversion and Covid 19 impacts. Ecol. Econ. 206 (2023).
Carleton, T. A. & Hsiang, S. M. Social and economic impacts of climate. Science 353 , aad9837 (2016).
Article PubMed Google Scholar
Bergé, L. Efficient estimation of maximum likelihood models with multiple fixed-effects: the R package FENmlm. DEM Discussion Paper Series 18-13 (2018).
Kalkuhl, M., Kotz, M. & Wenz, L. DOSE - The MCC-PIK Database Of Subnational Economic output. Zenodo https://zenodo.org/doi/10.5281/zenodo.4681305 (2021).
Kotz, M., Wenz, L. & Levermann, A. Data and code for “The economic commitment of climate change”. Zenodo https://zenodo.org/doi/10.5281/zenodo.10562951 (2024).
Dasgupta, S. et al. Effects of climate change on combined labour productivity and supply: an empirical, multi-model study. Lancet Planet. Health 5 , e455–e465 (2021).
Lobell, D. B. et al. The critical role of extreme heat for maize production in the United States. Nat. Clim. Change 3 , 497–501 (2013).
Zhao, C. et al. Temperature increase reduces global yields of major crops in four independent estimates. Proc. Natl Acad. Sci. 114 , 9326–9331 (2017).
Wheeler, T. R., Craufurd, P. Q., Ellis, R. H., Porter, J. R. & Prasad, P. V. Temperature variability and the yield of annual crops. Agric. Ecosyst. Environ. 82 , 159–167 (2000).
Rowhani, P., Lobell, D. B., Linderman, M. & Ramankutty, N. Climate variability and crop production in Tanzania. Agric. For. Meteorol. 151 , 449–460 (2011).
Ceglar, A., Toreti, A., Lecerf, R., Van der Velde, M. & Dentener, F. Impact of meteorological drivers on regional inter-annual crop yield variability in France. Agric. For. Meteorol. 216 , 58–67 (2016).
Shi, L., Kloog, I., Zanobetti, A., Liu, P. & Schwartz, J. D. Impacts of temperature and its variability on mortality in New England. Nat. Clim. Change 5 , 988–991 (2015).
Xue, T., Zhu, T., Zheng, Y. & Zhang, Q. Declines in mental health associated with air pollution and temperature variability in China. Nat. Commun. 10 , 2165 (2019).
Article ADS PubMed PubMed Central Google Scholar
Liang, X.-Z. et al. Determining climate effects on US total agricultural productivity. Proc. Natl Acad. Sci. 114 , E2285–E2292 (2017).
Desbureaux, S. & Rodella, A.-S. Drought in the city: the economic impact of water scarcity in Latin American metropolitan areas. World Dev. 114 , 13–27 (2019).
Damania, R. The economics of water scarcity and variability. Oxf. Rev. Econ. Policy 36 , 24–44 (2020).
Davenport, F. V., Burke, M. & Diffenbaugh, N. S. Contribution of historical precipitation change to US flood damages. Proc. Natl Acad. Sci. 118 , e2017524118 (2021).
Dave, R., Subramanian, S. S. & Bhatia, U. Extreme precipitation induced concurrent events trigger prolonged disruptions in regional road networks. Environ. Res. Lett. 16 , 104050 (2021).
Download references
Acknowledgements
We gratefully acknowledge financing from the Volkswagen Foundation and the Deutsche Gesellschaft für Internationale Zusammenarbeit (GIZ) GmbH on behalf of the Government of the Federal Republic of Germany and Federal Ministry for Economic Cooperation and Development (BMZ).
Open access funding provided by Potsdam-Institut für Klimafolgenforschung (PIK) e.V.
Author information
Authors and affiliations.
Research Domain IV, Research Domain IV, Potsdam Institute for Climate Impact Research, Potsdam, Germany
Maximilian Kotz, Anders Levermann & Leonie Wenz
Institute of Physics, Potsdam University, Potsdam, Germany
Maximilian Kotz & Anders Levermann
Mercator Research Institute on Global Commons and Climate Change, Berlin, Germany
Leonie Wenz
You can also search for this author in PubMed Google Scholar
Contributions
All authors contributed to the design of the analysis. M.K. conducted the analysis and produced the figures. All authors contributed to the interpretation and presentation of the results. M.K. and L.W. wrote the manuscript.
Corresponding author
Correspondence to Leonie Wenz .
Ethics declarations
Competing interests.
The authors declare no competing interests.
Peer review
Peer review information.
Nature thanks Xin-Zhong Liang, Chad Thackeray and the other, anonymous, reviewer(s) for their contribution to the peer review of this work. Peer reviewer reports are available.
Additional information
Publisher’s note Springer Nature remains neutral with regard to jurisdictional claims in published maps and institutional affiliations.
Extended data figures and tables
Extended data fig. 1 constraining the persistence of historical climate impacts on economic growth rates..
The results of a panel-based fixed-effects distributed lag model for the effects of annual mean temperature ( a ), daily temperature variability ( b ), total annual precipitation ( c ), the number of wet days ( d ) and extreme daily precipitation ( e ) on sub-national economic growth rates. Point estimates show the effects of a 1 °C or one standard deviation increase (for temperature and precipitation variables, respectively) at the lower quartile, median and upper quartile of the relevant moderating variable (green, orange and purple, respectively) at different lagged periods after the initial shock (note that these are not cumulative effects). Climate variables are used in their first-differenced form (see main text for discussion) and the moderating climate variables are the annual mean temperature, seasonal temperature difference, total annual precipitation, number of wet days and annual mean temperature, respectively, in panels a – e (see Methods for further discussion). Error bars show the 95% confidence intervals having clustered standard errors by region. The within-region R 2 , Bayesian and Akaike information criteria for the model are shown at the top of the figure. This figure shows results with ten lags for each variable to demonstrate the observed levels of persistence, but our preferred specifications remove later lags based on the statistical significance of terms shown above and the information criteria shown in Extended Data Fig. 2 . The resulting models without later lags are shown in Supplementary Figs. 1 – 3 .
Extended Data Fig. 2 Incremental lag-selection procedure using information criteria and within-region R 2 .
Starting from a panel-based fixed-effects distributed lag model estimating the effects of climate on economic growth using the real historical data (as in equation ( 4 )) with ten lags for all climate variables (as shown in Extended Data Fig. 1 ), lags are incrementally removed for one climate variable at a time. The resulting Bayesian and Akaike information criteria are shown in a – e and f – j , respectively, and the within-region R 2 and number of observations in k – o and p – t , respectively. Different rows show the results when removing lags from different climate variables, ordered from top to bottom as annual mean temperature, daily temperature variability, total annual precipitation, the number of wet days and extreme annual precipitation. Information criteria show minima at approximately four lags for precipitation variables and ten to eight for temperature variables, indicating that including these numbers of lags does not lead to overfitting. See Supplementary Table 1 for an assessment using information criteria to determine whether including further climate variables causes overfitting.
Extended Data Fig. 3 Damages in our preferred specification that provides a robust lower bound on the persistence of climate impacts on economic growth versus damages in specifications of pure growth or pure level effects.
Estimates of future damages as shown in Fig. 1 but under the emission scenario RCP8.5 for three separate empirical specifications: in orange our preferred specification, which provides an empirical lower bound on the persistence of climate impacts on economic growth rates while avoiding assumptions of infinite persistence (see main text for further discussion); in purple a specification of ‘pure growth effects’ in which the first difference of climate variables is not taken and no lagged climate variables are included (the baseline specification of ref. 2 ); and in pink a specification of ‘pure level effects’ in which the first difference of climate variables is taken but no lagged terms are included.
Extended Data Fig. 4 Climate changes in different variables as a function of historical interannual variability.
Changes in each climate variable of interest from 1979–2019 to 2035–2065 under the high-emission scenario SSP5-RCP8.5, expressed as a percentage of the historical variability of each measure. Historical variability is estimated as the standard deviation of each detrended climate variable over the period 1979–2019 during which the empirical models were identified (detrending is appropriate because of the inclusion of region-specific linear time trends in the empirical models). See Supplementary Fig. 13 for changes expressed in standard units. Data on national administrative boundaries are obtained from the GADM database version 3.6 and are freely available for academic use ( https://gadm.org/ ).
Extended Data Fig. 5 Contribution of different climate variables to overall committed damages.
a , Climate damages in 2049 when using empirical models that account for all climate variables, changes in annual mean temperature only or changes in both annual mean temperature and one other climate variable (daily temperature variability, total annual precipitation, the number of wet days and extreme daily precipitation, respectively). b , The cumulative marginal effects of an increase in annual mean temperature of 1 °C, at different baseline temperatures, estimated from empirical models including all climate variables or annual mean temperature only. Estimates and uncertainty bars represent the median and 95% confidence intervals obtained from 1,000 block-bootstrap resamples from each of three different empirical models using eight, nine or ten lags of temperature terms.
Extended Data Fig. 6 The difference in committed damages between the upper and lower quartiles of countries when ranked by GDP and cumulative historical emissions.
Quartiles are defined using a population weighting, as are the average committed damages across each quartile group. The violin plots indicate the distribution of differences between quartiles across the two extreme emission scenarios (RCP2.6 and RCP8.5) and the uncertainty sampling procedure outlined in Methods , which accounts for uncertainty arising from the choice of lags in the empirical models, uncertainty in the empirical model parameter estimates, as well as the climate model projections. Bars indicate the median, as well as the 10th and 90th percentiles and upper and lower sixths of the distribution reflecting the very likely and likely ranges following the likelihood classification adopted by the IPCC.
Supplementary information
Supplementary information, peer review file, rights and permissions.
Open Access This article is licensed under a Creative Commons Attribution 4.0 International License, which permits use, sharing, adaptation, distribution and reproduction in any medium or format, as long as you give appropriate credit to the original author(s) and the source, provide a link to the Creative Commons licence, and indicate if changes were made. The images or other third party material in this article are included in the article’s Creative Commons licence, unless indicated otherwise in a credit line to the material. If material is not included in the article’s Creative Commons licence and your intended use is not permitted by statutory regulation or exceeds the permitted use, you will need to obtain permission directly from the copyright holder. To view a copy of this licence, visit http://creativecommons.org/licenses/by/4.0/ .
Reprints and permissions
About this article
Cite this article.
Kotz, M., Levermann, A. & Wenz, L. The economic commitment of climate change. Nature 628 , 551–557 (2024). https://doi.org/10.1038/s41586-024-07219-0
Download citation
Received : 25 January 2023
Accepted : 21 February 2024
Published : 17 April 2024
Issue Date : 18 April 2024
DOI : https://doi.org/10.1038/s41586-024-07219-0
Share this article
Anyone you share the following link with will be able to read this content:
Sorry, a shareable link is not currently available for this article.
Provided by the Springer Nature SharedIt content-sharing initiative
By submitting a comment you agree to abide by our Terms and Community Guidelines . If you find something abusive or that does not comply with our terms or guidelines please flag it as inappropriate.
Quick links
- Explore articles by subject
- Guide to authors
- Editorial policies
Sign up for the Nature Briefing newsletter — what matters in science, free to your inbox daily.

Child Tax Benefits and Labor Supply: Evidence from California
The largest tax-based social welfare programs in the US limit their benefits to taxpayers with labor market income. Eliminating these work requirements would better target transfers to the neediest families but risks attenuating tax-based incentives to work. We study changes in labor force participation from the elimination of a work requirement in a tax credit for parents of young children, drawing on quasi-random variation in birth timing and administrative tax records. To do so, we develop and implement a novel approach for selecting an empirical specification to maximize the precision of our estimate. The unique design of the policy along with its subsequent reform allow us to isolate taxpayers' sensitivity to conditioning child tax benefits on work -- the parameter at the center of recent debates about the labor supply consequences of reforming federal tax policy for children. We estimate that eliminating the work requirement causes very few mothers to exit the labor force, with a 95% confidence interval excluding labor supply reductions of one-third of a percentage point or greater. Our results suggest expanding tax benefits for low-income children need not meaningfully reduce labor force participation.
For helpful comments and suggestions, we thank Connor Dowd, Joe Doyle, Kye Lippold, David Lee, Zhuan Pei, and seminar participants at the Upjohn Institute, the University of Wisconsin-Madison, and Rutgers University. Any taxpayer data used in this research was kept in a secured IRS data repository, and all results have been reviewed to ensure that no confidential information is disclosed. The views expressed herein are those of the authors and do not necessarily reflect the views of the U.S. Treasury Department or of the National Bureau of Economic Research.
MARC RIS BibTeΧ
Download Citation Data
More from NBER
In addition to working papers , the NBER disseminates affiliates’ latest findings through a range of free periodicals — the NBER Reporter , the NBER Digest , the Bulletin on Retirement and Disability , the Bulletin on Health , and the Bulletin on Entrepreneurship — as well as online conference reports , video lectures , and interviews .
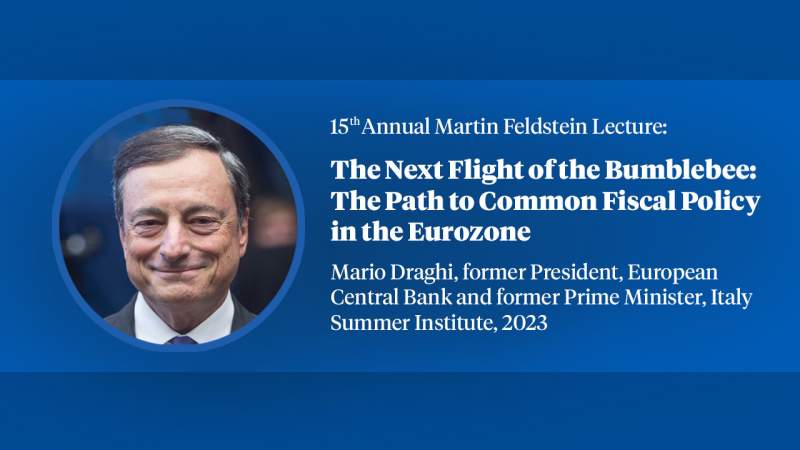
Economic characteristics of electricity
- Share via Email: Economic%20characteristics%20of%20electricity
- Share via Facebook: Economic%20characteristics%20of%20electricity
- Share via Twitter: Economic%20characteristics%20of%20electricity
The physical fundamentals of energy inform the economic fundamentals of electricity. Power plants that generate electricity face both fixed and variable costs. Fixed costs do not vary with the level of output; they primarily include capital costs and land costs associated with building a facility. The costs faced by transmission and distribution facilities are almost entirely fixed.
The variable costs of electricity – including fuel, labor and maintenance costs – depend on a power plant’s level of output. Over the short term, these are captured by looking to a plant’s marginal cost – that is, the cost to produce one more increment of output. Marginal cost provides the conceptual basis for cost-effective operation of the electricity system.
An illustration of typical energy supply curve

OPERATIONAL ECONOMICS
Unique short-term supply-and-demand characteristics make electricity an unusual product. Notably, all producers and consumers require access to a shared network (transmission), where the actions of some participants can affect the quality of service received by others. The challenge of balancing supply and demand is compounded by the lack of cost-effective storage options, like batteries. This makes the system very sensitive to short-term supply and demand shifts.
Historically, consumers had no way to assess real-time grid conditions for themselves, meaning they also had no way to adjust their consumption accordingly. Advances in technologies and services have given customers new information about grid conditions, like high costs on hot evenings or low costs on cool mornings. Nonetheless, most customers remain unresponsive to these changing grid conditions, which can cause rapid fluctuations in the marginal cost of generation. Some large consumers are a notable exception and change their demand according to grid conditions in order to save money on their bills.
Operating the grid in the “least-cost” manner requires minimizing generation costs. This involves dispatching generators in order of lowest to highest marginal cost to meet demand. The primary component of marginal cost for generators is fuel. This is determined both by fuel cost and by the efficiency with which a generator converts fuel into electricity. The result largely drives the shape of the supply curve. Fuel costs vary little over months for coal and nuclear generators, who usually sign long-term fuel contracts. Natural gas costs can vary substantially between and within a day, which shifts the supply curve considerably. Wind and solar, given their free fuel supply, have marginal costs near zero. Their dependence on the weather shifts the supply curve considerably. Most generators also incur substantial costs to start, shut down and adjust their output.
The marginal cost to serve demand, or “load,” in a particular area depends on the marginal cost of generation and the typically small “line loss” associated with transmitting power over transmission and distribution lines. Load also is limited by transmission availability. If power from the least-cost generator cannot flow to a load area because of constraints on the transmission system, the least-cost generator who can serve that load moves up in the dispatch order. The difference between these two generators’ marginal cost is referred to as the “marginal cost of transmission congestion.” This represents the extra cost to satisfy demand when transmission constraints require generation to be redispatched.
Economic characteristics by fuel type

Investment Economics
Historically, units with higher fixed costs – like nuclear, hydroelectric and coal – had low marginal costs. These units would provide the lowest average cost of electricity when they were operated frequently. As such, they were built to run at a steady level in a “baseload” role – that is, to meet minimum levels of demand. It proved more cost-effective to build low fixed-cost generation, like natural gas and oil, to meet less-frequent demand needs.
In recent years, cheap natural-gas prices have usually made it more cost-effective to build natural-gas power plants in a baseload role than either coal or nuclear. Wind and solar expansion has contributed to lowering marginal generation costs, but their variability limits those sources’ ability to replace conventional power plants. This is because their maximum output capability at peak periods is constrained by weather.
Electric infrastructure is capital-intensive, meaning its fixed costs are high relative to its variable costs. Spreading fixed costs out over a greater scale results in lower average per-unit costs, thus offering what are known as economies of scale. For example, building and operating a 600 MW plant is less expensive than two 300 MW plants.
Electric infrastructure also is long-lived, operating for decades at a time. It requires long lead times to build. Once built, the high fixed costs become large sunk costs (that is, costs that were already incurred) to generation owners. This magnifies the consequences of poor investment decisions. Furthermore, investment economics depends on a variety of conditions that are difficult to predict, such as fuel prices, technology advances and policy changes. All these factors make generation investment risky for investors.
These features have made long-term planning an indispensable tool to minimize cost and risk. Through a process commonly known as “integrated resource planning” (IRP), producers aim to determine the least-cost mix of resources. This involves evaluating the fuel type, size and timing of new resource investments and retirements to meet expected future demand. For example, many IRPs in recent years support expanded demand-side management programs, construction of new natural-gas-fired generation and retirement of coal-fired generation. Policy interventions that deviate from IRP principles, such as those dictating a part of the fuel mix, tend to result in higher-cost investments.
Characteristics like enormous economies of scale and the inefficiencies of duplicate transmission and distribution system investments led the electric power industry historically to be designated a natural monopoly. This meant that one firm would provide electricity at lower cost than multiple, competing firms. For example, a firm with an existing transmission network can expand its network at lower cost than a new entrant starting from scratch.
New technologies reduced the economies of scale, starting in the 1980s, as smaller generation facilities became more economical. These shifts called into question whether a single generation provider would deliver lower-cost service than multiple providers in a competitive market. Competition also could offer incentives for generators to manage risks, lower costs, innovate and provide superior customer service. This set the foundation for industry reforms in the 1990s, when electricity markets began signaling investment decisions for much of the United States.
Contact: If you have questions regarding this subject, please contact Electricity Policy Manager Devin Hartman or Outreach Director Lori Sanders at the R Street Institute at 202-525-5717.
Featured Publications
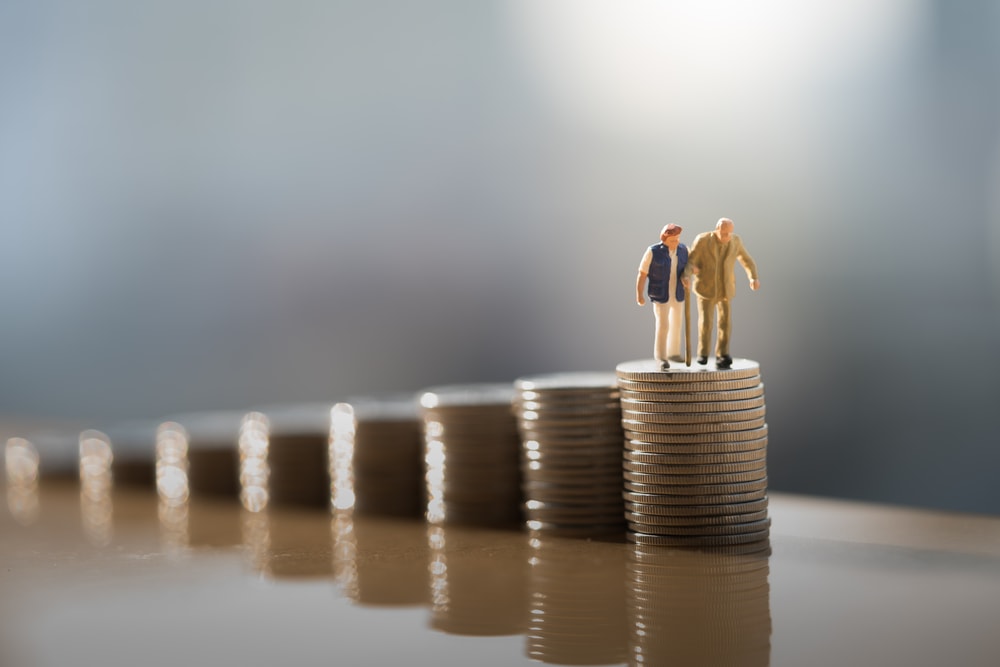
Lessons from the States on Entitlement Reform
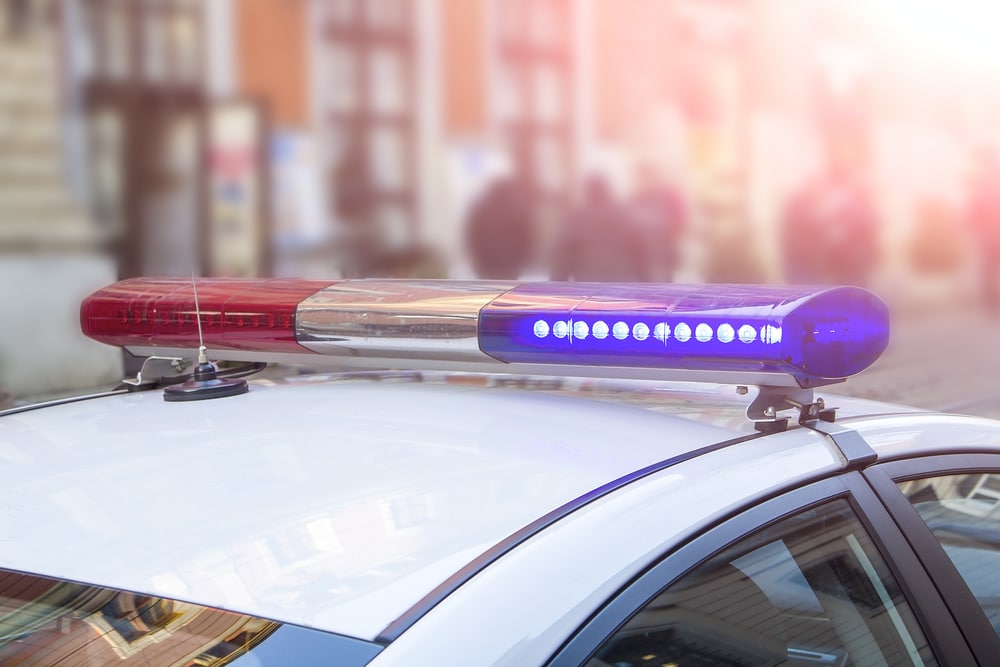
R Sheet On The Law Enforcement De-escalation Training Act of 2022: Purpose, Implementation, and Next Steps
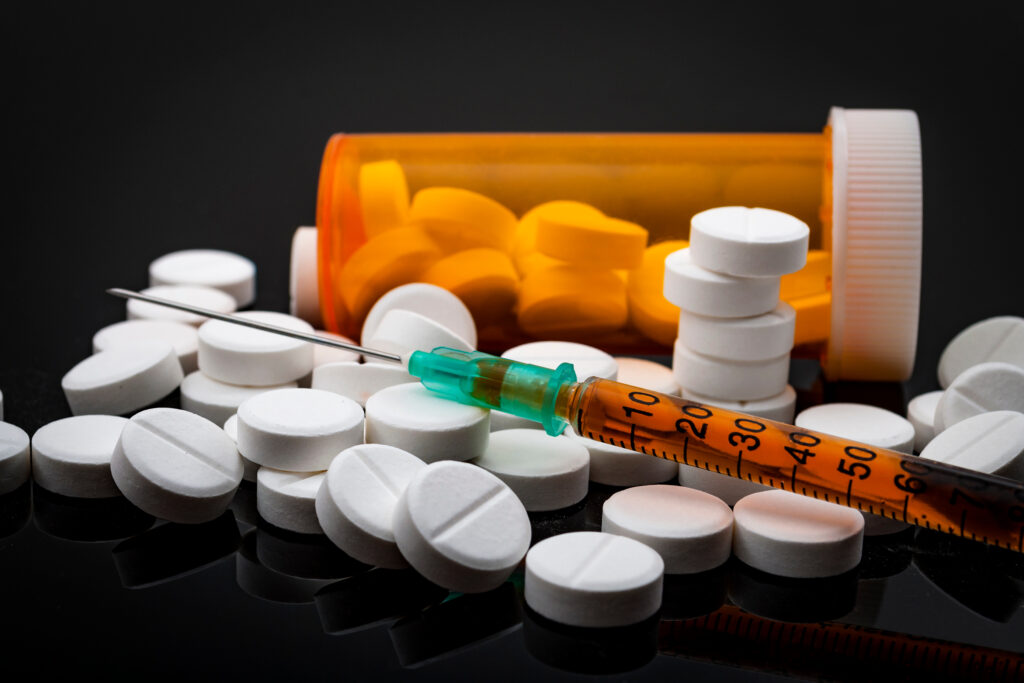
More Is Not Always Better: Challenging Calls for High-Dose Naloxone
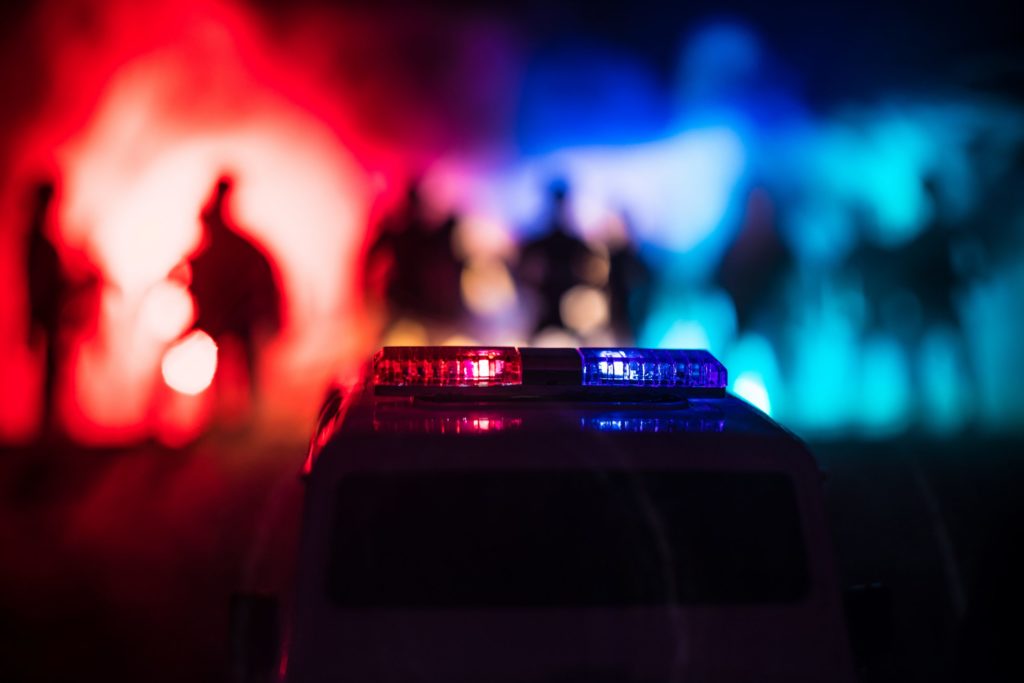
Measured Force: The Benefits of Police Data Transparency
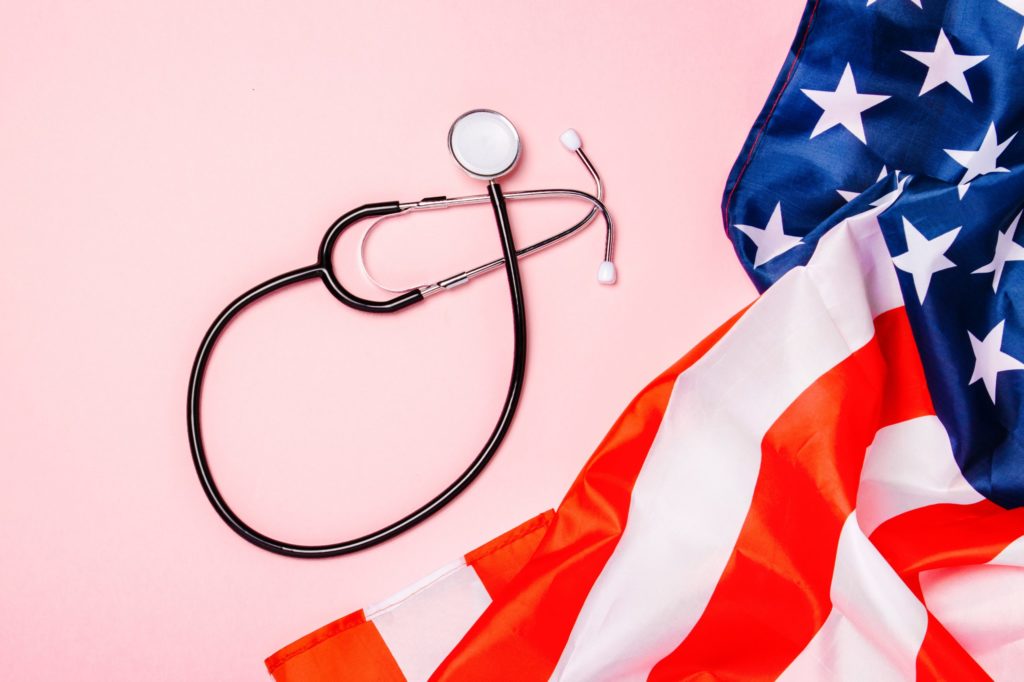
HIV Pre-exposure Prophylaxis (PrEP) and Post-exposure Prophylaxis (PEP) Basics
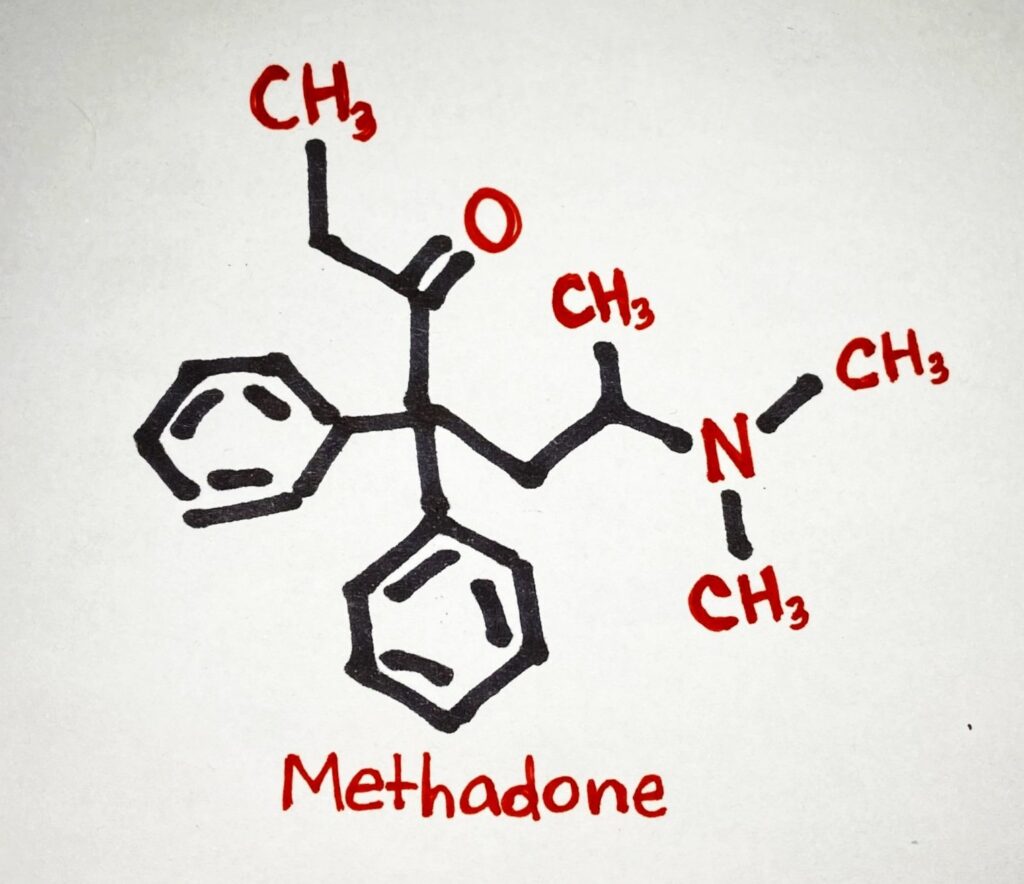
Unshackled from OTPs, Methadone Can Still Be Safe and Effective
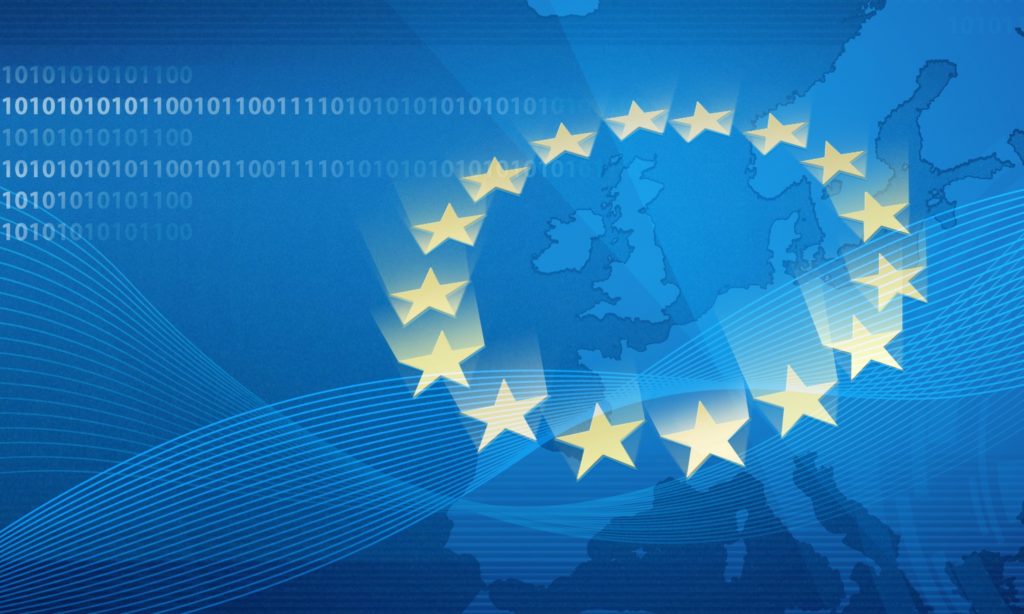
Cybersecurity Score — European Cybersecurity Certification Scheme For Cloud Services (EUCS)
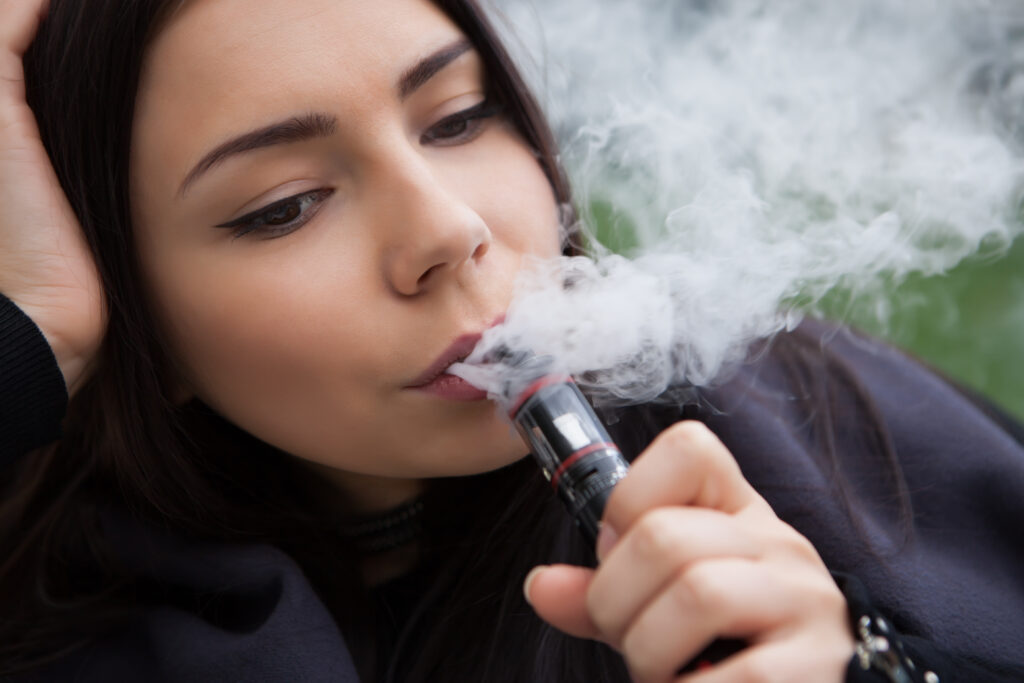
Progressive Except for Nicotine: A Discussion of States’ Inconsistent Adoption of Harm Reduction Public Policy
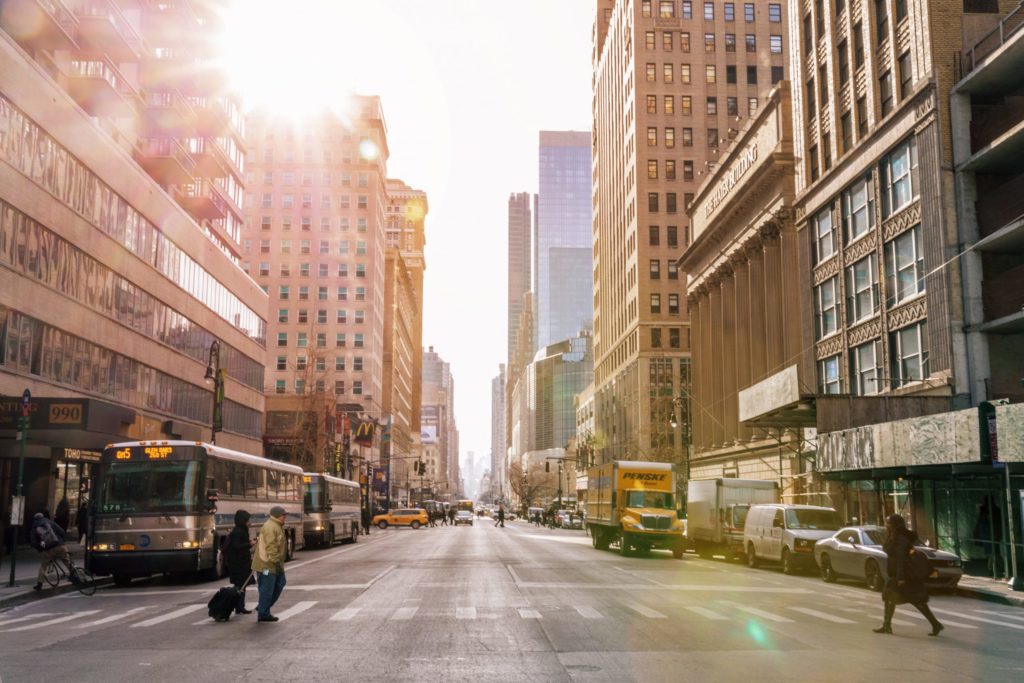
New York City’s OPCs Do Not Pose a Serious Threat to Public Safety
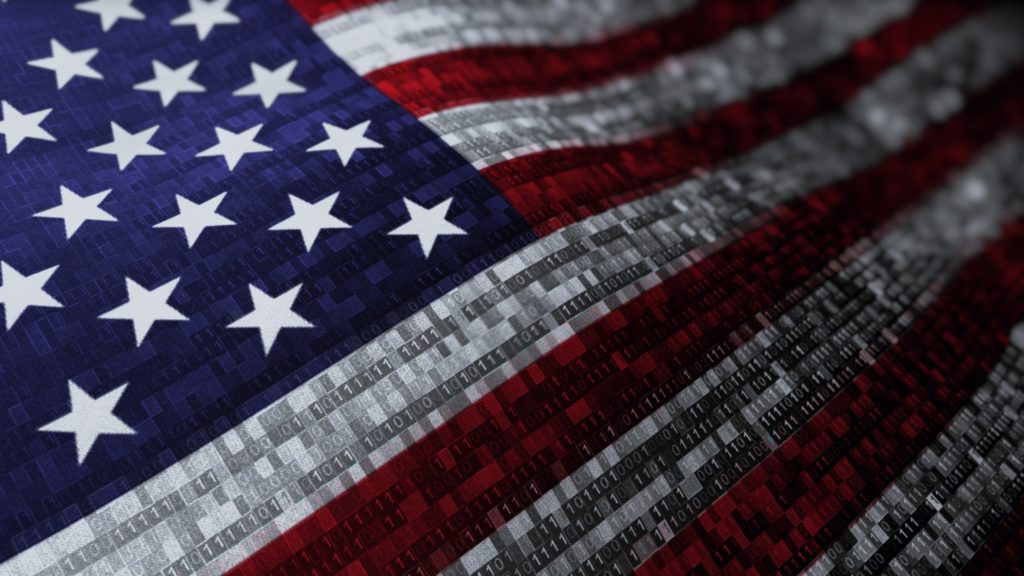
Lawmakers “Move Fast and Break Things” when Regulating Social Media, but AI Can (and Should) Be Handled Differently
Numbers, Facts and Trends Shaping Your World
Read our research on:
Full Topic List
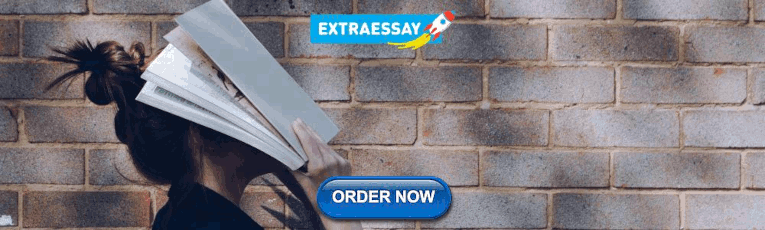
Regions & Countries
- Publications
- Our Methods
- Short Reads
- Tools & Resources
Read Our Research On:
Economic characteristics of U.S. Filipino population, 2015
% (unless otherwise noted)
Note: The household population excludes persons living in institutions, college dormitories and other group quarters. Households are classified by the race or detailed Asian group of the head. “Full-time, year-round workers” are defined as people ages 16 and older who usually worked at least 35 hours per week and at least 48 weeks in the past year. The share of the population ages 16 and older who are not employed differs from the unemployment rate because the share not employed is based on the total population, while the unemployment rate is based on those who are in the labor force (i.e. working or looking for work). Poverty status is determined for individuals in housing units and non-institutional group quarters. It is unavailable for children younger than 15 who are not related to the householder, people living in institutional group quarters and people living in college dormitories or military barracks. Due to the way in which the IPUMS assigns poverty values, these data will differ from those provided by the U.S. Census Bureau. Figures may not sum to 100% due to rounding. Figures for Filipinos and all Asians based on mixed-race and mixed-group populations, regardless of Hispanic origin. See methodology for more detail. Source: Pew Research Center analysis of 2013-2015 American Community Survey (IPUMS).
Sign up for The Briefing
Weekly updates on the world of news & information
Most Popular
1615 L St. NW, Suite 800 Washington, DC 20036 USA (+1) 202-419-4300 | Main (+1) 202-857-8562 | Fax (+1) 202-419-4372 | Media Inquiries
Research Topics
- Age & Generations
- Coronavirus (COVID-19)
- Economy & Work
- Family & Relationships
- Gender & LGBTQ
- Immigration & Migration
- International Affairs
- Internet & Technology
- Methodological Research
- News Habits & Media
- Non-U.S. Governments
- Other Topics
- Politics & Policy
- Race & Ethnicity
- Email Newsletters
ABOUT PEW RESEARCH CENTER Pew Research Center is a nonpartisan fact tank that informs the public about the issues, attitudes and trends shaping the world. It conducts public opinion polling, demographic research, media content analysis and other empirical social science research. Pew Research Center does not take policy positions. It is a subsidiary of The Pew Charitable Trusts .
Copyright 2024 Pew Research Center
Terms & Conditions
Privacy Policy
Cookie Settings
Reprints, Permissions & Use Policy

An official website of the United States government
The .gov means it’s official. Federal government websites often end in .gov or .mil. Before sharing sensitive information, make sure you’re on a federal government site.
The site is secure. The https:// ensures that you are connecting to the official website and that any information you provide is encrypted and transmitted securely.
- Publications
- Account settings
Preview improvements coming to the PMC website in October 2024. Learn More or Try it out now .
- Advanced Search
- Journal List
- Elsevier - PMC COVID-19 Collection

Exploring the impact of socio-demographic characteristics, health concerns, and product type on home delivery rates and expenditures during a strict COVID-19 lockdown period: A case study from Portland, OR
E-commerce volumes and home deliveries have experienced steady growth in the last two decades. Strict COVID-19 lockdowns made home delivery an essential service and a lifeline for many households that, for travel restrictions or health concerns, were not able to utilize traditional shopping methods. This research studies the impact of socio-demographic variables and e-commerce attitudes on household deliveries for seven product categories (groceries, meals, electronics, household and office goods, recreational items, and fashion, beauty and personal care products, and medicine/health-related products) during the lockdown period in the greater Portland metropolitan region. To understand these impacts, exploratory factor analysis and choice models with latent variables are estimated utilizing data collected from an online survey representing the population in the greater Portland metropolitan region. The results indicate that each factor has a unique profile in terms of significant socio-demographic variables. A novel contribution of this research is to study the impact on home deliveries of non-traditional variables like health and safety concerns and the presence of household members with disabilities during a pandemic. The results show that health concerns are very influential and that there are substantial differences across factors on delivery rate and expenditure levels. Key findings and perspectives regarding future delivery rates and implications for transportation agencies and logistics companies are discussed.
1. Introduction
The sudden onset of the COVID-19 pandemic and consequent lockdowns have significantly altered the way people work, educate themselves or their children, feed their families, and seek recreation. The government-imposed lockdowns and restrictions have changed traditional business patterns and activities. As a result, many businesses have been forced to shut down or impose strict social distancing guidelines. Many consumers have found that home deliveries are a solution to some of the lockdown challenges, leading to a surge in online shopping sales during the COVID-19 pandemic. According to the Adobe index of the digital economy, US e-commerce sales jumped approximately 49% in April 2020 and 60% in May 2020 with respect to expected pre-COVID figures ( Adobe, 2020 ). The highest growth is likely to have taken place in the online food, beverages, and grocery market, where companies like Instacart have experienced 500% year-over-year increases during the lockdown ( CNBC, 2020 ). In this research, the term home delivery refers to deliveries to households, places where people reside, in contrast to commercial building-related deliveries.
In the US, there has been a steady growth in e-commerce sales in the last two decades, but there is a lack of disaggregated data that can be utilized to understand home delivery attitudes and product preferences across different populations. The 2017 National Household Travel Survey (NHTS) collected a wide array of responses related to household travel patterns, land use, and socio-demographic variables. However, only one question in the latest NHTS focused on home deliveries, and it was limited to the number of deliveries in a 30-day period ( FHWA, 2018 ).
In the US, early studies have shown that consumers are less to shop at grocery stores when COVID-19 infections are surging ( Grashuis et al., 2020 ). In other countries, researchers have also documented dramatic changes in travel and car utilization patterns in Australia ( Beck and Hensher, 2020 ) and in Turkey ( Shakibaei et al., 2020 ), for example. Though the literature in this area is evolving fast, to the best of the authors’ knowledge, there is no published research study that has focused on how socio-demographic factors, health attitudes, and product type have impacted home delivery rates during the lockdown in the US.
Seven different products are studied in this research: groceries, meals, electronics, household and office goods, recreational items, and fashion, beauty and personal care products, and medicine and health products. This research explores answers for three novel research questions: (i) What is the impact of health concerns on home deliveries during the lockdown? (ii) What is the impact of socio-demographic variables on products and attitudes? and (iii) How home delivery rates do change with different levels of home delivery expenditures? To answer these questions, exploratory factor analysis and choice models with latent variables are estimated utilizing data collected from an online survey collected during a strict lockdown period.
This research is organized as follows: Section 2 presents an overview of relevant trends related to home product deliveries and a literature review. Section 3 describes the data collection effort. Section 4 utilizes exploratory factor analysis to group products and attitudes. Section 5 details the choice models with latent variables used to estimate the delivery rate and expenditure models. Section 6 presents the results of a model to explore the factors behind the changes in home delivery rates during the lockdown. Section 7 focuses on the results of models for and different levels of delivery rates and expenditures. Section 8 discusses implications of the results regarding future data collection efforts, modeling, and travel and logistic trends. Section 9 ends with a summary of key findings and conclusions.
2. Literature review
E-commerce sales in the US have increased at double-digit rates for the past two decades and have significantly outpaced brick-and-mortar retail growth ( USDC, 2020 ). Amazon is the largest player in market share, and Amazon Prime subscriptions have been steadily growing. An important draw to membership is the offer of free, fast shipping for many types of orders. Amazon Prime membership in the US has grown from 50 million members in late 2015 to 112 million in December 2019 ( Fortune, 2020 ). Since Amazon is the leader in online sales in the US, trends related to its sales per category are relevant background information for this research (see Table 1 ). Electronic and appliances lead in expenditures, followed by sporting goods and entertainment, personal use items such as clothing and apparel, furniture, and home decorations.
Amazon’s US sales product category (in $ billions), 2014–2018.
Source: PYMNTS (2018) .
Academic and government literature and consumer surveys have studied the effect of socio-demographics, product characteristics, and shopping experience on e-commerce adoption and home deliveries. Consumer surveys find that 43% of American consumers cite convenience as their strongest motivator for shopping online, and pricing is a distant second at 19% ( eMarketer, 2018a ). Age is also an important factor. Different age groups vary in their means of internet access and types of products purchased ( eMarketer, 2018b ; Schmid and Axhausen, 2019 ). In general, older people are less likely to adopt e-commerce compared to their younger counterparts ( Farag et al., 2005 , Farag et al., 2006a , Farag et al., 2007 , Krizek et al., 2005 , DE BLASIO, 2008 , Cao et al., 2012 , Crocco et al., 2013 , Zhou and Wang, 2014 , Clemes et al., 2014 , Irawan and Wirza, 2015 , Lee et al., 2015 , Ding and Lu, 2017 ). According to NHTS 2017 data, households above the poverty line are “almost twice as likely to make online purchases compared to respondents in households below the poverty level (i.e., 61% versus 33%)” ( FHWA, 2018 ). Income is a variable that is linked to other household characteristics such as internet access, credit card access, education levels, and the number of household workers ( Cao et al., 2012 ). Higher-income households are more likely to make purchases online ( Farag et al., 2005 , Farag et al., 2006a , Farag et al., 2007 , DE BLASIO, 2008 , Cao et al., 2012 , Crocco et al., 2013 , Zhou and Wang, 2014 , Lee et al., 2015 , Schmid and Axhausen, 2019 ). The 2017 NHTS results indicate online shopping is directly proportional to the frequency of Internet usage ( FHWA, 2018 ). Other studies have confirmed this result. Utilization of smartphones, access to the internet, computers increases the likelihood of online shopping ( Farag et al., 2005 , Farag et al., 2006a , Farag et al., 2007 , Krizek et al., 2005 , Cao et al., 2012 , Crocco et al., 2013 , Zhou and Wang, 2014 , Irawan and Wirza, 2015 , Lee et al., 2015 , Ding and Lu, 2017 , Schmid and Axhausen, 2019 , Alemi et al., 2019 ). Variables associated with a household structure such as the number of members with driver licenses ( Irawan and Wirza 2015 ), vehicle ownership ( Dias et al. 2020 ), presence of workers ( Farag et al., 2006a , Farag et al., 2006b , Zhou and Wang, 2014 , Irawan and Wirza, 2015 ), etc. affect the propensity to shop online. Outside of demographic characteristics, product type plays a vital role in e-commerce adoption ( Girard et al., 2003 , Dias et al., 2020 , Zhen et al., 2016 , Zhen et al., 2018 ; Zhai et al., 2017 , Maat and Konings, 2018 , Schmid and Axhausen, 2019 ). A novel contribution of this research is studying the impact of product types and groups on home delivery rates and expenditures in a pandemic setting.
The number of deliveries per household has not been widely studied. Based on a study of a large apartment building near New York City in 2016 and 2017, the average apartment received about 1.5 packages per week ( Rodrigue, 2017 ). This study showed that package deliveries peaked in December, which correlates with holiday spending trends. In South Korea, a country that has widely adopted e-commerce, the estimated annual package deliveries per person was 39.6. ( Seo and Lee, 2017 ).
Before COVID-19, there have been growing concerns about increasing home package deliveries and their associated externalities. Some researchers have studied different ways to minimize the impact of household deliveries utilizing newer and greener delivery modes ( Perboli and Rosano, 2019 ). Cherrett et al. (2017) analyzed the externalities brought about by parcel deliveries, using a survey of residence halls and university students in Southampton (UK). This research found that there are significant opportunities to increase delivery efficiency by consolidating trips. The magnitude of home deliveries is likely to be significant. A modeling effort and case study utilizing 2009 NHTS data estimated that the volume of freight trips destined for residences may be on par with the magnitude of freight trips to businesses ( Zhou and Wang, 2014 ).
Several research efforts have focused on how perceptions of online risk may be associated with consumers' purchasing decisions ( Potoglou et al., 2015 ). This research shows that privacy and online shopping concerns reduce the likelihood of online shopping. Schmid et al. (2016) studied tradeoffs between online and in-store shopping for groceries and electronic appliances. They found that socio-economic characteristics significantly impact shopping cost sensitivity and highlight the potential of online shopping services for some products depending on the relation between the value of time and delivery time savings. Both research efforts utilized integrated choice and latent variable (ICLV) models.
Despite the significant amount of work on e-commerce adoption, to the best of the authors’ knowledge, no published work has focused on the impact of socio-demographic variables, products, and health concerns on home delivery rate and expenditures during COVID-19 lockdowns or in a pandemic setting.
3. Data collection
The online survey for this research was administered in the last week of May and the first week of June 2020. Oregon Governor Brown issued a “stay at home” executive order on March 23, and the state of emergency was extended until July 6, 2020. During this time, the lockdown was widely accepted by the general population, and traffic levels on the main Portland freeways dropped between 40 and 60% ( ODOT, 2020 ). The focus of the study is on a single geographic region to reduce variability and uncertainty regarding lockdown enforcement rules and timing.
The data was collected utilizing an online survey targeting residents in the greater Portland metropolitan area that includes several counties and cities and is also called the Portland-Vancouver-Hillsboro Oregon-Washington Metro Area. This metro area has approximately 2.5 million people spread over nearly 7,000 square miles ( Census Reporter, 2020 ). To obtain a representative sample of the population, the following demographic quotas were imposed: (a) at least 40% representation of males or females in the sample, (b) a minimum quota of 20% was imposed for each of these household annual income categories: 0–$50,000, $50,000–$100,000, and greater than $100,000, and finally (c) an age-related quota mandating at least a 20% representation in the following categories 18–29, 30–44, and 45–64 and at least 8% in 65 and above. The data collection was limited to respondents above 18 years old.
The survey focused on questions related to (a) demographic and household-level information regarding age, income, household size, and presence of members with a disability who require assistance and (b) the number of home deliveries made 30 days before and during the lockdown, and lockdown delivery levels for the products analyzed in this research.
Table 2 provides the descriptive statistics of the relevant socio-demographic variables found to be significant in the model. Slightly more than 40% of the respondents are male. All age and income groups are well represented, with nearly one-third of the respondents between the ages of 31 and 45 and more than half of the respondents having an annual household income of greater than $ 50,000. This is consistent with the income distribution of the Portland metro region, which has a median household income of nearly $76,000 and a median age of 38.4 for the Metro region ( Census Reporter, 2020 ). One-fifth of the respondents live alone. Two people households are the most common group and compose nearly one-third of the samples.
Descriptive statistics of relevant demographic variables.
Unlike previous research efforts regarding e-commerce, this effort includes a question about “the presence of members with a disability who require assistance”. Home deliveries may be an important lifeline for populations with disabilities and/or health concerns. This question was included to gauge the impact of disabilities or special needs on home delivery rates during the lockdown period. Nearly one-fifth of the households have at least one member with a disability.
The numbers provided in Table 2 , right column, for gender, age, and household income and size were obtained from ACS (American Community Survey) data for the region surveyed ( USCB, 2021 ). The age distribution follows the regional data, and there are some differences at the top and bottom ends of the income and household size distributions. Males are underrepresented and show the largest percental deviation in absolute terms. Regarding disabilities and the number of subscriptions, it was not possible to get regional data. The number of adults with any disability in large urban areas is approximately 23% ( Zhao et al., 2019 ). Regarding subscriptions, precise numbers are difficult to obtain, but Amazon (the e-commerce leader in the US but not the only company offering subscriptions) in mid-2020 had 128 million subscribers, roughly 38% of the US population ( DC360, 2021 ). Also, the number of subscriptions in the US has been growing at double-digit rates and the COVID pandemic has accelerated this trend ( SUBTA, 2021 ). It was not possible to obtain figures for the period under study for other e-commerce and home delivery services.
The main reference used for the design of socio-demographic questions was the 2017 NHTS ( FHWA, 2018 ). However, due to the unprecedented nature of the data collection during the pandemic COVID-19 additional attitudinal questions related to health concerns and products (e.g., medicines, meal deliveries) were also included. After data cleaning, the dataset has 1,015 fully complete and clean responses that are utilized to estimate all the models presented in this research.
In the survey, the questions related to home delivery rates are the following: “In a typical month BEFORE COVID-19, how many times did you or members of your household purchase something online and have it delivered to your home?” and “In the last 30 days, AFTER COVID-19 lockdown started, how many times did you or members of your household purchase something online and have it delivered to your home?”. In these two questions, respondents had to choose one of the five categories shown in Table 3 . Comparing before and during answers, it is possible to observe that the number of households that received “0” (zero) or “3 to 5” deliveries barely changed. However, there was a large decrease in the “1 to 2” category and large increases in the “6 to10” and “More than 10” categories. In aggregate, there is a clear increase in delivery rates, but a more in-depth view shows that roughly 10% of the households decrease a category, 37% of the households kept the same level, 37% of the households increased one level, and 15% of the households increased two or more levels during the lockdown. The shift towards higher categories can be better observed in Table 4 ; for categories “1 to 2, “3 to 5”, and “6 to 10” the category with the highest percentage is the next level up.
Number of home deliveries in 30 days before and during COVID-19 lockdown.
Crosstabulation (row percentages) of home deliveries in 30 days before COVID-19 lockdown and after COVID-19 lockdown.
Table 5 presents the frequency and relative frequency of response to “In the last 30 days, how much money you or members of your household have spent on items that have been delivered to your household?”. More than 40% of households spent between $ 100 and $ 499 on household deliveries in 30 days. Slightly more than 10% of the respondents had household delivery expenditures exceeding $ 1000 per month. The right side of Table 5 presents the distribution of expenditures by the number of deliveries. For low levels of expenditure, the frequency of deliveries is also low. However, as the level of expenditure increases, the values are more evenly spread, and this is likely the result of factors such as household attitudes and products.
Descriptive Statistics of household delivery expenditures in 30 days and crosstabulation with home deliveries during COVID.
NA: not applicable.
4. Exploratory factor analysis
A key goal of the survey was to gather data about attitudes regarding safety and home delivery features as well the type of products purchased online and with home delivery. The survey asked, “If you have made online purchases with home delivery in the last 30 days, which of the following categories did you purchase most often? For each category, assign a number ranging from 0 to 5, assign 0 if a category is never ordered, and 5 for the most frequently ordered category.” The seven categories included were: (1) groceries, (2) meals home-delivered, (3) electronics and related products, (4) fashion, beauty, or personal care (FBPC) products, (5) recreational/entertainment items, (6) household or office goods, and (7) medicines/medical or health-related goods. The last category was included to gauge the relative importance of health-related items during the pandemic. Table 6 shows the distribution of delivery frequency by product. Given the sample size of 1015, the smallest number of observations per bin in Table 6 is 18, i.e., electronics, column 5 “most frequent” with 1.8%. In this and similar questions, the goal was to obtain an ordering frequency for each category being possible to have two categories with the same category or score. Herein, a shortened description of the categories is included in tables and text to improve readability and table format.
Distribution of Delivery Frequency by Product (rows sum to 100%).
In addition, four questions regarding the importance of delivery cost, delivery time, overall online experience, and health concerns to gauge attitudes in these areas during the lockdown. The wording of the question was the following: “When deciding between purchasing at a physical store or ordering online for a home delivered product, what factors are most important? For each factor assign a number ranging from 0 to 5, assign 0 if a factor is not relevant and 5 for the most important factor(s). Factors: (a) home delivery cost, (b) home delivery time, (c) easy overall online experience, and (d) personal health and safety concerns. The relative distribution frequencies for each of the factors are displayed in Table 7 .
Distribution of attitudinal questions (rows sum to 100%).
Since the product frequency and attitudinal variables are correlated, it is necessary to find groups of variables or factors that can allow the estimation of the models. Exploratory factor analysis (EFA) was performed to group the products into four factors. The Kaiser-Meyer-Olkin (KMO) test was applied to measure the adequacy of the data for EFA ( Fabrigar and Wegener, 2011 ). The overall score is 0.75, and for each variable, the scores are shown in Table 8 . As a general guideline, KMO values between 0.8 and 1 are usually considered very good and below 0.6 is considered inadequate for EFA. The values of the product data are between 0.67 and 0.8, mostly in the 0.7–0.8 range, which indicates that the data is acceptable for EFA.
Rotated factor loadings.
The eigenvalue and parallel analysis suggested that four factors would be adequate, and the results are shown in Table 8 . The EFA was performed using the Psych package in R version 2.1.5 ( Revelle and Revelle, 2015 ) and the “Varimax” rotation at its default parameters. The factor loadings are shown in Table 8 , with a standard cutoff value of 0.3 to ease interpretation.
The first factor could be labeled “food-related” categories. The second factor can be labeled non-food “consumer products”. The third and fourth factors can be labeled “home delivery attributes” and the last factor “health and safety” concerns. These factors are used to define the latent variables in the frequency and expenditure models. Factor 4 has only one indicator and Factor 1 two indicators. In many studies with more attitudinal questions, the factors are related to three or more indicators. However, it is valid to have for example two latent factors with one indicator each as shown in Vij and Walker (2016) .
5. Methodology
The model utilized in this research involves the simultaneous estimation of choice models for order frequency and/or expenditures, latent variables, attitudinal/ordered responses, and socio-demographic variables. The joint estimation of the models offers insights into the contribution of each factor, the different products/attitudes, and the socio-demographic variables ( Daly et al., 2012 ). The formulation presented herein roughly follows Daly et al., 2012 , Potoglou et al., 2015 models, but in this case, there is no panel data, and the upper-level choice model is either an ordered logit model or a binary logit instead of a multinomial logit model.
The latent variable model focuses on the four latent factors identified in the previous section. The measurement equations for each product or attitude s links the ordered response, y s , as follows:
where Z is the corresponding latent variable and υ s is the random (normal) component of the response for product or attitude. The four latent factors are assumed to be determined by linear structural relationships for each factor f and individual n as follows:
The vector of parameters b f is estimated for each factor, and the term W f , n represents a set of socio-demographic variables (delivery subscription, gender, income, age, household size, disability). The term σ f ψ f , n is a normally distributed error term with zero mean and standard deviation σ f for each factor.
Following Daly et al. (2012) , an ordered logit model is utilized to account for the ordinal character of the product purchased frequency response. The probability that an individual n generates the observed response q for a product or attitude s is estimated as follows:
where ⋀ is the closed cumulative form of the logistic distribution and with constraints:
for each response level. The threshold parameters τ are estimated, and to set the additive scale of the ordinal model, constants are omitted. To normalize the scale of the measurement equations, one of the parameters d p for each group of products is set to one ( Ben-Akiva et al., 2002 ). The likelihood of the set of S ( s ∈ S ) ordered responses for products and attitudes for respondent n is:
There is a final ordered logit or binary logit model. In the ordered model, the observed utility of individual n is given by this expression:
The parameter γ f is the contribution of the latent factor f and the parameter β i is the contribution of the independent variable x i , n . To differentiate thresholds, η is utilized to denote estimated threshold parameters in this final model. The dependent variable, u n (change in delivery frequency) is also naturally ordered, and the probability that an individual n generates the observed response k is estimated as follows:
In the binary logit model, the dependent variable is a given level of delivery and expenditure, the observed utility of individual n is given by this expression:
Without alternative specific variables and normalizing to zero the γ coefficients for the first alternative, the probability of the first alternative is:
Following Daly et al. (2012) , it is assumed that the disturbances are independent and that their covariance matrices are diagonal matrices. All the parameters ( d s , b f , σ f , τ y s , q , β i , γ f , η k ) are jointly estimated by maximizing the log-likelihood function utilizing the package Apollo version 0.2.1 ( Hess and Palma, 2019 ) in the R environment version 4.0.3 ( R Core Team, 2020 ). The coefficients of the estimated parameters are stable after approximately 100 draws, but the results presented are obtained with 500 draws per random parameter utilizing the Modified Latin Hypercube Sampling (MLHS) method ( Hess et al., 2006 ). The results of initial exploratory models were validated against the results of the “polr” function of the MASS package in R ( Ripley et al., 2013 ).
6. Change in delivery frequency results
This section presents the latent variable model results when applied to the increase in delivery rates per month. In all tables henceforth, t-ratios are shown, and to guide the reader, variable coefficients with t-ratios ≥ 1.96 (0.05 significant level) in absolute value are bold to facilitate the interpretation of the results. In addition, * and ** symbols have been added to indicate significant levels of 0.01 and 0.001 with t-ratios 2.58 and 3.30 respectively.
For the delivery rate model, a difference model is estimated, i.e., the dependent variable is the difference between COVID and pre-COVID delivery rates. The difference in delivery rates produces a dependent variable with four levels: decreased, the same level, increased by one, or increased by more than one category. This difference between ordered variables is not ideal, but it reduces biases in the estimated parameters because the error distributions of COVID and pre-COVID delivery rates are likely correlated (i.e., not independent over time). There are four levels; comparing before and during COVID-19 rates roughly 10% of the households decreased a category, 37% of the households kept the same level, 37% of the households increased one level, and 15% of the households increased two or more levels during the lockdown.
Regarding socio-demographic variables, mid-income comprises incomes in the range of $30,000–$100,000 and high income above $100,000. According to the literature review, age is likely to be negative, and mid/high-income groups are expected to be positive. Except for “Age”, all the socio-demographic variables are treated as binary variables, which means that no ordering or structure is imposed on them. For example, income level has a natural order, but each income level is estimated in relation to the baseline, which is low household annual income (less than $30,000). In all the results, high income has a higher coefficient than mid-income, but this is not the result of imposing a constraint. The whole model LL, AIC, and BIC is −17,647, 35,496, and 36,244 respectively.
6.1. Latent model results
The results are compared side by side in Table 9 . Factors 1, 2, and 4 are significant and positive, and Factor 3 (related to delivery time and cost and online experience) is not significant. Health and safety concerns had the largest contribution in terms of coefficient value followed by food products.
Ordered logit model results.
Bold p < .05, * p < .01, ** p < .001.
6.2. Socio-demographic variables
Table 10 presents the effect of socio-demographic variables on the four latent factors. Table 10 shows that for Factor 1 (food products), only delivery subscription, age, and households with a disability are positive and statistically significant factors. Same results are observed for Factor 2, but in addition, income variables and household size are positive and statistically significant variables. For Factor 3, only subscription, income, and age are significant. An important change is observed in Factor 4 (health and safety), age is no longer negative but positive, indicating a major reversal in trend regarding age. In addition, the variable male is significant and with a negative sign. These results seem to indicate that younger and/or males are less concerned about health and safety than other groups.
Socio-demographic variables.
The variable disability has a significant and positive coefficient for Factors 1, 2, and 4, whereas income variables are positive and significant for Factors 2, 3, and 4. In all cases, a delivery subscription is a significant variable, but the results are stronger in terms of coefficients and t-ratios for Factors 1, 2 and 3. Household size is positive and significant only for Factor 2 non-food products. Overall, the results confirm some previous expectations based on the literature review, but there are novel results regarding age and disability. A graph showing significant variables for the delivery frequency model is presented in Fig. 1 .

Delivery Rate Model. Note: only significant variables p < 0.05 are included with symbols * for p < 0.01 and ** for p < 0 s.001.
6.3. Latent variable results
Table 11 shows the impact of latent factors on product frequency. When comparing results in terms of d s parameters it is possible to see the sign and relative standing of each product. Groceries, electronic products, delivery cost, and online experience are set to 1 as required to have an efficient estimation process. All the estimated parameters are significant. The value of medicine/health products is significantly lower than household products and recreational items. Online experience is significantly lower than delivery time.
Latent variable results.
Finally, Table A1 , Table A2 in Appendix A show the threshold parameters for product and attitude models.
7. Exploring factors affecting high/low delivery rates and expenditures
Binary logit models with latent variables are estimated to further explore the factors that result in different levels of deliveries and expenditures. This section presents the results of the latent variable models when applied to customers with (a) low delivery rates and high level of expenditures – herein denoted “LD-HE” model – (b) high delivery rates and low level of expenditures – herein denoted “HD-LE” model – and (c) high delivery rates and high level of expenditures – herein denoted “HD-HE” model.
As shown in Table 3 and Table 5 , approximately 36% of the households reported expenditures larger than $500 per month, and 42% of the households reported more than six deliveries per month. These are the thresholds used to create binary variables with low and high levels of deliveries and expenditures. Approximately 9% of the households (92 observations) belong to the LD-HE case, 19% of the households (188 observations) belong to the HD-LE case, and 24% of the households (239 observations) belong to the HD-HE case. For the HD-LE model, the whole model LL is −16691, AIC 33579 and BIC 34313; for the LD-HE model, the whole model LL is −16862, AIC 33923 and BIC 34656; and for the HD-HE model, the whole model LL is −16843, AIC 33884 and BIC 34617.
7.1. Latent model results
The results are compared side by side in Table 12 . There are major differences among model results. In the LD-HE model, Factor 2 (non-food products) is the only significant latent factor that contributes to low delivery rates but high level of expenditures. High level of deliveries but low expenditures (HD-LE) has positive and significant contributions from safety/health followed by non-food products and delivery/purchase attributes. Finally, high levels of deliveries and expenditures (HD-HE) also has positive and significant contributions from safety/health but followed by non-food products and food-products. The results confirm that high delivery rates took place in households with higher levels of health and safety concerns, health and safety concerns is not a significant factor in households with low delivery rates.
7.2. Socio-demographic variables
Table 13 shows the results regarding socio-demographic variables. In most cases, the same variables are significant (using as a reference a t-ratio >2) and with the same sign when comparing with the results in Table 10 . For Factor 1 (food products), only delivery subscription, age, and households with a disability are positive and statistically significant factors with the sole addition of mid-income in the LD-HE model. Same significant variables are observed for Factors 2 and 3 in Table 13 (also similar to significant variables in Table 10 ).
Major changes are observed in Factor 4 (health and safety); the variable “age” is positive and significant only for the HD-HE model, indicating that only in households with more deliveries and expenditures there is a reversal in the sign of the variable “age”. In addition, the variable “male” is significant and with a negative sign only for models LD-HE and HD-LE. These results indicate that younger and/or males are less concerned about health and safety mostly in LD-HE and HD-LE households. The variable “high income” is only positive and significant in the models with high levels of expenditures. The variable “disability” has a significant and positive coefficient only in the LD-HE model.
Graphs showing significant variables for the three models are presented in Fig. 2 .

Frequency and Expenditure Models. Note: only significant variables p < 0.05 are included with symbols * for p < 0.01 and ** for p < 0.001.
7.3. Latent variable results
Latent variable results are displayed in Table 14 . All the estimated parameters are again positive and significant. In all cases, the values of the coefficients do not show a large variation.
Finally, Table B1 , Table B2 in Appendix B show the threshold parameters for product and attitude models.
8. Discussion
The unprecedented scope and speed of changes brought about by the COVID-19 lockdown on household activities, travel, and consumption patterns are still ongoing and likely to leave an indelible mark on attitudes towards online shopping and household deliveries. The extent and severity of the COVID-19 pandemic and its associated lockdown were mostly unexpected, and its speed was a shock to traditional ways of commerce and work for most businesses and workers. The lockdowns have accelerated a trend towards teleworking, remote schooling, and remote delivery of many services and activities that used to be only (or mostly) offered at brick-and-mortar locations.
A high degree of uncertainty has characterized the evolution of the COVID-19 pandemic and its ongoing and future impacts on transportation systems and, more specifically, on household shopping patterns. It is possible to conjecture that the post-COVID-19 world will strengthen trends clearly visible before the pandemics, such as teleworking and online shopping with home deliveries. Lessons learned from the early days of teleworking may provide an insight into future trends for home deliveries. The impacts of remote working have been studied since the late nineties, e.g., Varma et al. (1998) analyzing the adoption of telecommuting and telecenters and Mokhtarian and Meenakshisundaram (1999) showing that there was an early and “stable” segment of adopters of telecommuting but with a spectrum regarding long-term commitments. It is possible that a similar trend will be observed in terms of home deliveries but with sharp differences across product types and households. For example, home deliveries of electronic products may have a steadier growth. In contrast, home deliveries of products like groceries or meals may depend more on the evolution of the pandemic and population concerns about health and safety while shopping at a physical location (as well as costs and other factors found significant).
This section discusses insights related to home deliveries and speculates about different aspects of home deliveries that have implications for policy analysis and future research efforts. The discussion is divided into the following subsections: data collection, future demand trends, freight and logistics impacts, integration of freight and travel demand modeling, equity, and study limitations.
8.1. Data collection
There has been scant or no research regarding the type of products and household characteristics that trigger high levels of deliveries and expenditures. There are sources of e-commerce data at the government level ( USDC, 2020 ) and at the private level ( Adobe, 2020 ) that provide relatively fast and highly accurate information regarding e-commerce sales and online price inflation. However, there is little data about actual household delivery rates, at least in the US. The NHTS is conducted typically every eight years; the most recent surveys are dated 2009 and 2017, and the next release of data is years away.
This research has also shown that the factors driving household delivery expenditure levels are not necessarily the same as those driving household delivery frequencies. Expenditures are a useful proxy, but actual travel impacts regarding miles traveled, emissions, and crashes are related to actual delivery rates per household and the type of product. From a logistics perspective, the efficiency of household delivery systems is not affected directly by expenditure levels but instead by the overall number of deliveries, the number of deliveries per vehicle or route, and delivery constraints such as time windows and delivery time.
Home deliveries can be a substitution for in-person travel to stores. Hence, home deliveries are at the intersection of personal travel and commercial vehicle (last mile) travel. However, there are no datasets that can provide a joint comprehensive view of these two complementary travel modes. There is also a lack of long-term longitudinal data to track detailed consumer behavior regarding shopping trips, home deliveries, and e-commerce.
Data collection is now becoming even more complex with different ways to receive products shopped online. Alternatives to traditional home delivery include now ordering online (e-commerce) with in-store pickup or pickup at locker facilities. New acronyms like BOPAC (buy online pick-up at curbside) and BOPIS (buy online and pick up in-store) became mainstream in logistic circles during the pandemic. In addition, it is possible to add BOPL (buy online pick up at locker) which is more common in Europe but that Amazon is also introducing in the US. In some countries like Poland, with a high locker density, there are even more advanced alternatives regarding lockers. It is possible for companies (and consumers) to ship products from locker to locker and to request delivery of products from locker to locker.
8.2. Home delivery demand trends
The results of the latent models show clear variation across factors and socio-demographic variables. Unlike previous research efforts, there is a clear reversal regarding the sign of the variable age and the factor health and safety concerns (age is significant and positive). If older respondents are more likely to engage in home deliveries due to health and safety concerns, this has large future implications in terms of demographic changes and future home delivery demand.
Previous research efforts have alerted about long known demographics trends such as population aging and more specifically, the impact of the baby boomer generation on travel demand ( Goulias et al., 2007 , Metz, 2012 , Siren and Haustein, 2013 ). The potential impacts of population aging are not clear yet, and the travel propensity of baby boomers and future retirees is likely to depend on many factors. The COVID-19 pandemic has likely added another layer of complexity in terms of long-term household decisions such as housing location and vehicle ownership or level of comfort utilizing public transportation options. Future research should explicitly include health and safety (Factor 4 in this research) as well as disabilities as factors that impact transportation-related decisions in future research efforts.
Population aging is also likely to increase trips related to health and assistance services as total percentage of trips. However, telehealth or telemedicine received a boost during the pandemic; in the future, in-person visits could be reduced, but it is likely that this is going to trigger the demand for home deliveries related to ancillary services such as medicines, home tests, as well as devices to remotely monitor health indicators. Robotization of care may also impact the number or health care worker trips.
Recent studies have indicated that public transportation is perceived to be associated with higher levels of risk and that risk perception might depend on characteristics of residential location ( Rahimi et al., 2021 ). A future research topic is the investigation of risk perception regarding transportation modes and their relationship with the adoption of home deliveries and e-commerce. A recent review of travel demand studies indicates that the literature has not yet reached a consensus on the dominant effect of online shopping ( Le et al., 2021 ). Although there is more evidence indicating that online shopping substitutes for shopping travel demand, there is also a lot of heterogeneity, and this research effort now indicates that the heterogeneity may be related to product types, home delivery attributes, and health/safety factors.
The long-term impact of the ‘urban exodus’ seen at the start of the COVID-19 pandemic is not clear. It is likely that a sizable percentage of people that moved out of cities had second homes and therefore higher incomes. It is also likely that another percentage of movers were teleworkers “escaping” from expensive urban areas and looking for cheaper and larger housing units in suburban and rural areas.
A movement of population towards urban or suburban areas is likely to put pressure on local services ( Gallent, 2020 ) in the short-term. But for home deliveries, a movement towards less dense and populated areas is likely to result in more home deliveries, i.e., more store trip substitutions since higher-income households buy more and more often online and because former city dwellers are more familiar with e-commerce and home deliveries. Changes in teleworking rates are also likely to have direct impacts on home delivery frequency but also potential indirect effects such as the need to continue improving homes that are also work environments. The term “home nesting” became popular during the pandemic as many consumers (and companies in some cases) invested in home furniture and equipment. Home nesting may result in redirecting deliveries that traditionally were shipped to office or company buildings in dense urban cores.
8.3. Impacts on freight and logistics operations
The type of product is likely to play a key role regarding home delivery frequency by type of household and by location. Some types of home deliveries like food or certain groceries (refrigerated or vegetables) may not be available in low-density areas. However, durable consumer products are likely to dominate home deliveries in rural and suburban areas with low density.
Type of product is also key in terms of logistics efficiency. Meals and food products for immediate or same-day consumption are not typically delivered by the same vehicles and/or companies. Furthermore, there are significant differences in supply/service chains and distribution networks for food-related (Factor 1 in this research) and non-food-related products (Factor 2 in this research). Hence, commercial trips and flows cannot be captured solely by focusing on traditional heavy-duty commercial vehicles. Data collection efforts such as the NHTS should include variables that better reflect the evolution of home deliveries and the attitudes and non-traditional products and services (like food delivery) that impact home delivery rates.
Demographic and spending habit changes and the growth of home delivery will trigger changes in the logistics networks needed to meet spatial changes of home deliveries demand (for example reshoring sourcing and the addition of warehouses and distribution centers) as well as changes in freight patterns. In addition, as a result of the pandemic and other disruptions (like electronic chips), supply chains will likely increase safety stocks or change sourcing locations to meet changing consumer expectations in terms of delivery time and reliability.
It is expected that the last mile of home deliveries will have to evolve to meet the growth of customer demands but also growing expectations regarding accessibility, affordability, and convenience. Logistics and shipping capacity in the last mile will have to increase to meet surges that are not limited to Black Friday events or the holiday season.
The growth of home delivery and new delivery modalities like BOPIS will require that many stores in urban areas will have to be redesigned to serve in-person customers as well as a growing demand at the curb (access by auto/transit/peds) and docks to facilitate delivery fleet access. For cities, especially where roadside space is scarce, it may be challenging to allocate space for growing logistical access. Road, parking, and sidewalk space reallocations may be needed, especially at locations where parking and access to automobiles were reduced during the pandemic to allow for on-street/sidewalk outdoor restaurant seating and other uses.
The aftermath of COVID-19 may not only change the geography of work and urban centers but also the geography of freight and logistics at regional, urban, and even at the block level.
8.4. Integrating freight and travel demand modeling
The previous discussion highlights the need for greater integration between freight and passenger demand modeling. E-commerce not only may reduce or change in-store shopping frequency (complementary or substitution effects), but it also impacts flows at the regional level (warehousing, freight flows), urban level (fulfillment centers and more delivery traffic), and even at the block or street level when considering parking and access for last-mile delivery vehicles and fulfillment utilizing BOPIS systems.
There is also a need for more freight and specifically last-mile models within urban areas. A paper analyzing best practices for urban freight management, an international survey, indicates that there are many opportunities for freight research, starting with the question of how many vehicles are engaged in freight activities ( Dablanc et al., 2013 ). Freight models are more undeveloped than passenger models, and there is no model that can integrate and forecast trips disaggregating by vehicle types such as heavy truck, light-duty truck, van, car, rideshare, and even less by modality type including parcel delivery, BOPIS, BOPAC, and BOPL. Traditional freight models have focused on heavy and medium trucks and business-to-business flows, neglecting home deliveries and the impacts of e-commerce at different geographic scales. The emergence of new vehicle types such as electric cargo bicycles or trikes, autonomous delivery robots, and drones may also play a significant role in future home delivery operations.
The connections and interactions among home deliveries, passenger trips, and commercial trips are growing stronger due to the COVID-19 pandemic. Still, current modeling approaches are rather elementary at best when representing these interactions. Part of the problem stems from the lack of suitable datasets, as mentioned earlier.
8.5. Home delivery and equity
Income is another key socio-demographic variable. Equity is becoming more relevant and increasingly affecting transportation programs and funding decisions. Home deliveries disproportionally benefit higher-income more educated sectors of the population ( Figliozzi and Unnikrishnan, 2021 ). In this regard policymakers may consider policy options that facilitate access to e-commerce and home deliveries. More specifically, measures to reduce the digital divide will increase e-commerce adoption among disadvantaged groups. In addition, the installation of common carrier lockers can reduce delivery costs and facilitate access to consumers that due to work constraints or residential locations do not feel comfortable with unattended home deliveries ( Keeling et al., 2021 ). The integration of transit services, that usually cater to disadvantaged populations or groups with low or no auto ownership, with common carrier lockers or other methods that reduce cost or facilitate access could also be beneficial to reduce home delivery inequality in urban and rural areas.
Equity can also be impacted from the supply and labor side of home deliveries. Higher delivery volumes and pressures to cut costs and become more competitive are likely to foster last-mile delivery innovations that increase productivity and reliability. At the same time, the logistics delivery system has shown some signs of fragility related to labor unrest. Protests motivated by low wages, equity issues, and workers' health at distribution centers have been a challenge for many companies that had to ramp up operations and swiftly hire an unprecedented number of new workers. Automatization and contact-free delivery systems may provide long-term advantages both in terms of delivery costs but also on the resiliency and sustainability of delivery systems. Research has found that the market penetration of autonomous trucks would, in part, depend on autonomous delivery technology ( Jennings and Figliozzi, 2019 , Jennings and Figliozzi, 2020 ) and public opinion ( Pani et al., 2020 ); higher income households are more likely to order home deliveries utilizing autonomous delivery vehicles. COVID-19 may accelerate the necessary public opinion changes to support autonomous home deliveries but this may also bring equity concerns regarding access to this type of service.
8.6. Limitations
The data used in this paper is one snapshot at a specific point in time. It is likely an important point in time though, COVID-19 may have created an inflection point and triggered many long-term changes in societal behavior and norms, passenger travel, freight and supply chains, and home deliveries. Research efforts that include longitudinal panel data would be key to complement the presented findings and analyze the evolution of attitudinal changes regarding travel and home deliveries. It would have been desirable to collect data at other locations with a different sociodemographic profile to compare results and derive more complex insights.
The findings regarding products and factors such as health and safety are very likely to be robust, especially the ones supported by variables with a stringent significant level. However, with the caveat that the results are robust but limited to a specific yet important point in time. It is difficult to speculate about the “new normal” and long-term “stickiness” of the changes brought up by the COVID-19 pandemic as other researchers have already discussed ( Salon et al., 2021 ).
9. Conclusions
This research is a first towards understanding how the lockdown has changed home delivery patterns by trying to disaggregate the impacts of a set of products and consumer attitudes utilizing latent variable models. The data used in this research effort was collected during the period of a strict lockdown in Oregon, when traffic levels on main freeways were 40–60% lower than usual.
An important finding of this research is that the factors that increase or decrease the adoption of home deliveries during a lockdown can vary widely across factors related to products and attitudes. Each factor has a unique profile regarding household characteristics.
Unlike previous research efforts related to e-commerce and home deliveries, this effort analyzed the impacts of health and safety concerns and the impact of household members with disabilities or special needs on home delivery rates and expenditures. Nearly one-fifth of the households surveyed have at least one member with a disability or a special need. Health and safety concerns is the latent factor with the largest contribution to home deliveries rates and in households with high levels of deliveries and expenditures.
Gender also plays a role in attitudes during the lockdown, the variable “male” only has a significant and negative sign in the health and safety concerns factor. Households with a member with a disability or special need are more likely to order food-products (meals and groceries), non-food products, and are also likely to be more concerned in terms of health and safety.
The results of the latent models show clear variation across factors and socio-demographic variables. Age is a significant and negative variable for factors related to products or home delivery attributes, i.e. younger respondents are more likely to engage in home deliveries related to food or non-food products as previously shown by many research efforts. However, there is clear reversal regarding health and safety concerns where age is significant and positive, indicating that older respondents are more likely to engage in home deliveries due to health and safety concerns.
Based on the modeling results, the number and type of deliveries are expected to vary widely across one urban area. Consumers and households evaluate tradeoffs regarding costs, convenience, and health concerns; these tradeoffs are affected by household and personal characteristics such as household size, age, income, and the presence of members with disabilities. The adoption of subscription services and the cost of deliveries (flat monthly fee or per purchase) is also likely to play an important role in the evolution of home delivery rates. If the home delivery sector continues to grow rapidly, this will also have implications for cities and planning organizations that will have to adapt and react to the changes and their broader implications in terms of accessibility, safety, and equity. The results of this research provide new insights into the adoption and utilization of home deliveries and may help inform future data collection and planning endeavors.
Regarding long-term trends, demographics, and the natural aging of the population also provide support to the hypothesis of sustained growth of e-commerce and home deliveries fueled by the continuous growth of the next generation of younger and tech-savvy consumers. Currently, there are billions of annual grocery shopping trips in the US ( FHWA, 2018 ); the growth of the home delivery sector with higher route efficiency and cleaner autonomous vehicles can have a significant and positive impact in terms of safety ( Sparrow and Howard, 2017 ) and emissions ( Figliozzi, 2020 ). It is likely that in the post-COVID world, access for residential deliveries may still be challenging in dense urban areas ( Chen et al., 2017 ). The extent of the pros and cons of a rapid increase in home delivery rates is likely to depend on long-term demographic, land use, and business patterns, which are particularly difficult to visualize at this point in time. Traditionally, most freight research has focused on modeling freight attraction and production rates as a function of land use and other variables, e.g., Lawson et al. (2012) . This type of study will remain important for planning organizations, but it is likely that the share of trips related home deliveries will grow in importance and this should be taken into account in future data collection, research, and planning efforts.
Potential implications of the findings in terms of data collection, modeling, freight and logistics, and equity were discussed. Given that the long-term impacts of the COVID-19 pandemic are still unraveling, better and more data should be collected to let future research efforts study the connections among home deliveries, travel demand, safety and risk perceptions, and sociodemographic and location factors.
Declaration of Competing Interest
The authors declare that they have no known competing financial interests or personal relationships that could have appeared to influence the work reported in this paper.
Appendix A.
See Table A1 , Table A2 .
Threshold levels for products.
Threshold levels for attitudes.
Appendix B.
See Table B1 , Table B2 .
- Adobe, 2020. Adobe Digital Economy Index - Adobe Analytics, June 2020 report. Accessed June 30, 2020. https://www.adobe.com/experience-cloud/digital-insights/digital-economy-index.html .
- Alemi F., Circella G., Mokhtarian P., Handy S. What drives the use of ridehailing in California? Ordered probit models of the usage frequency of Uber and Lyft. Transp. Res. Part C: Emerg. Technol. 2019; 102 :233–248. [ Google Scholar ]
- Beck M.J., Hensher D.A. Insights into the impact of COVID-19 on household travel and activities in Australia – The early days under restrictions. Transp. Policy. 2020; 96 :76–93. doi: 10.1016/j.tranpol.2020.07.001. [ PMC free article ] [ PubMed ] [ CrossRef ] [ Google Scholar ]
- Ben-Akiva M., Walker J., Bernardino A.T., Gopinath D.A., Morikawa T., Polydoropoulou A. In: In Perpetual Motion: Travel Behaviour Research Opportunities and Application Challenges. Mahmassani H.S., editor. Elsevier; Amsterdam: 2002. Integration of choice and latent variable models; pp. 431–470. [ Google Scholar ]
- Cao X.J., Xu Z., Douma F. The interactions between e-shopping and traditional in-store shopping: an application of structural equations model. Transportation. 2012; 39 (5):957–974. [ Google Scholar ]
- Census Reporter, 2020. Portland-Vancouver-Hillsboro, OR-WA Metro Area. Available at https://censusreporter.org/profiles/31000US38900-portland-vancouver-hillsboro-or-wa-metro-area/ , Last Accessed: July 2020.
- Chen Q., Conway A., Cheng J. Parking for residential delivery in New York City: Regulations and behavior. Transp. Policy. 2017; 54 :53–60. [ Google Scholar ]
- Clemes M.D., Gan C., Zhang J. An empirical analysis of online shopping adoption in Beijing, China. J. Retail. Consumer Serv. 2014; 21 (3):364–375. [ Google Scholar ]
- CNBC, 2020. Coronavirus is making grocery delivery services like Instacart really popular and they might be here to stay, https://www.cnbc.com/2020/05/13/coronavirus-making-grocery-delivery-services-like-instacart-popular.html . Last Accessed: July 2020.
- Cherrett T., Dickinson J., McLeod F., Sit J., Bailey G., Whittle G. Logistics impacts of student online shopping–evaluating delivery consolidation to halls of residence. Transp. Res. Part C: Emerg. Technol. 2017; 78 :111–128. [ Google Scholar ]
- Crocco F., Eboli L., Mazzulla G. Individual attitudes and shopping mode characteristics affecting the use of e-shopping and related travel. Transp. Telecommun. J. 2013; 14 (1):45–56. [ Google Scholar ]
- Daly A., Hess S., Patruni B., Potoglou D., Rohr C. Using ordered attitudinal indicators in a latent variable choice model: a study of the impact of security on rail travel behaviour. Transportation. 2012; 39 (2):267–297. doi: 10.1007/s11116-011-9351-z. [ CrossRef ] [ Google Scholar ]
- De Blasio G. Urban–rural differences in internet usage, e-commerce, and e-banking: Evidence from Italy. Growth Change. 2008; 39 (2):341–367. [ Google Scholar ]
- Dablanc L., Giuliano G., Holliday K., O'Brien T. Best Practices in Urban Freight Management: Lessons from an International Survey. Transp. Res. Rec. 2013; 2379 (1):29–38. doi: 10.3141/2379-04. [ CrossRef ] [ Google Scholar ]
- DC360, 2021. Amazon Prime reaches 200 million members worldwide [WWW Document]. Digital Commerce 360. URL https://www.digitalcommerce360.com/article/amazon-prime-membership/ (accessed 8.4.21).
- Dias F.F., Lavieri P.S., Sharda S., Khoeini S., Bhat C.R., Pendyala R.M., Pinjari A.R., Ramadurai G., Srinivasan K.K. A comparison of online and in-person activity engagement: The case of shopping and eating meals. Transp. Res. Part C: Emerg. Technol. 2020; 114 :643–656. [ Google Scholar ]
- Ding Y., Lu H. The interactions between online shopping and personal activity travel behavior: an analysis with a GPS-based activity travel diary. Transportation. 2017; 44 (2):311–324. [ Google Scholar ]
- eMarketer, 2018a. ‘Primary reason that internet users in the United States make digital purchases as of March 2018’, published by emarketer.com. Accessed at: https://www.statista.com/statistics/826773/primary-reasons-internet-users-make-digital-purchases/ (accessed on June 15 2019).
- eMarketer, 2018b. ‘Online shopping and buying-related activities performed by internet users in the United States as of March 2018, by age (Graph)’. Accessed at https://www.statista.com/statistics/868862/online-shopping-buying-related-activities-internet-users/ (accessed on June 5 2019).
- Fabrigar L.R., Wegener D.T. Oxford University Press; 2011. Exploratory Factor Analysis. [ Google Scholar ]
- Farag S., Schwanen T., Dijst M. Empirical investigation of online searching and buying and their relationship to shopping trips. Transp. Res. Rec. 2005; 1926 (1):242–251. [ Google Scholar ]
- Farag S., Krizek K.J., Dijst M. E-Shopping and its Relationship with In-store Shopping: Empirical Evidence from the Netherlands and the USA. Transp. Rev. 2006; 26 (1):43–61. [ Google Scholar ]
- Farag S., Weltevreden J., van Rietbergen T., Dijst M., van Oort F. E-shopping in the Netherlands: does geography matter? Environ. Plann. B: Plann. Design. 2006; 33 (1):59–74. [ Google Scholar ]
- Farag S., Schwanen T., Dijst M., Faber J. Shopping online and/or in-store? A structural equation model of the relationships between e-shopping and in-store shopping. Transp. Res. Part A: Policy Pract. 2007; 41 (2):125–141. [ Google Scholar ]
- FHWA, 2018. Federal Highway Administration FHWA NHTS Brief: Changes in Online Shopping Trends (online). Available at: https://nhts.ornl.gov/assets/NHTSBriefOnlineShopping081018.pdf (Accessed: June 28 2020).
- Figliozzi M.A. Carbon Emissions Reductions in Last Mile and Grocery Deliveries Utilizing Autonomous Vehicles. Transp. Res. Part D. Transp. Environ. 2020; 85 [ PMC free article ] [ PubMed ] [ Google Scholar ]
- Figliozzi M., Unnikrishnan A. Home-deliveries before-during COVID-19 lockdown: Accessibility, environmental justice, equity, and policy implications. Transp. Res. Part D: Transp. Environ. 2021; 93 :102760. doi: 10.1016/j.trd.2021.102760. [ PMC free article ] [ PubMed ] [ CrossRef ] [ Google Scholar ]
- Fortune, 2020. Amazon Prime’s numbers (and influence) continue to grow. https://fortune.com/2020/01/16/amazon-prime-subscriptions/ (Accessed: June 30, 2020).
- Gallent N. COVID-19 and the flight to second homes. Town Country Plann. 2020; 89 :141–144. [ Google Scholar ]
- Girard T., Korgaonkar P., Silverblatt R. Relationship of type of product, shopping orientations, and demographics with preference for shopping on the Internet. J. Bus. Psychol. 2003; 18 (1):101–120. [ Google Scholar ]
- Goulias K.G., Blain L., Kilgren N., Michalowski T., Murakami E. Catching the Next Big Wave: Do Observed Behavioral Dynamics of Baby Boomers Force Rethinking of Regional Travel Demand Models? Transp. Res. Rec. 2007; 2014 (1):67–75. doi: 10.3141/2014-09. [ CrossRef ] [ Google Scholar ]
- Grashuis J., Skevas T., Segovia M.S. Grocery Shopping Preferences during the COVID-19 Pandemic. Sustainability. 2020; 12 :5369. doi: 10.3390/su12135369. [ CrossRef ] [ Google Scholar ]
- Hess S., Palma D. Apollo: A flexible, powerful and customisable freeware package for choice model estimation and application. J. Choice Modell. 2019; 32 :100170. doi: 10.1016/j.jocm.2019.100170. [ CrossRef ] [ Google Scholar ]
- Hess S., Train K.E., Polak J.W. On the use of a Modified Latin Hypercube Sampling (MLHS) method in the estimation of a Mixed Logit Model for vehicle choice. Transp. Res. Part B: Methodol. 2006; 40 (2):147–163. doi: 10.1016/j.trb.2004.10.005. [ CrossRef ] [ Google Scholar ]
- Irawan M.Z., Wirza E. Understanding the effect of online shopping behavior on shopping travel demand through structural equation modeling. J. Eastern Asia Soc. Transp. Stud. 2015; 11 :614–625. [ Google Scholar ]
- Jennings D., Figliozzi M. Study of sidewalk autonomous delivery robots and their potential impacts on freight efficiency and travel. Transp. Res. Rec. 2019; 2673 (6):317–326. [ Google Scholar ]
- Jennings D., Figliozzi M. Study of Road Autonomous Delivery Robots and Their Potential Effects on Freight Efficiency and Travel. Transp. Res. Rec.: J. Transp. Res. Board. 2020; 2674 (9):1019–1029. [ Google Scholar ]
- Keeling K., Schaefer J.S., Figliozzi M.A. Accessibility and Equity Analysis of Transit Facility Sites for Common Carrier Parcel Lockers. Transp. Res. Rec.: J. Transp. Res. Board. 2021 doi: 10.1177/03611981211032214. [ CrossRef ] [ Google Scholar ]
- Krizek K.J., Li Y., Handy S.L. Spatial attributes and patterns of use in household-related information and communications technology activity. Transp. Res. Rec. 2005; 1926 (1):252–259. [ Google Scholar ]
- Lawson C.T., et al. Estimated generation of freight trips based on land use. Transp. Res. Rec. 2012; 2269 (1):65–72. [ Google Scholar ]
- Le H.T.K., Carrel A.L., Shah H. Impacts of online shopping on travel demand: a systematic review. Transp. Rev. 2021:1–23. doi: 10.1080/01441647.2021.1961917. [ CrossRef ] [ Google Scholar ]
- Lee R.J., Sener I.N., Handy S.L. Picture of online shoppers: Specific focus on Davis, California. Transp. Res. Rec. 2015; 2496 (1):55–63. [ Google Scholar ]
- Maat K., Konings R. Accessibility or innovation? store shopping trips versus online shopping. Transp. Res. Rec. 2018; 2672 (50):1–10. [ Google Scholar ]
- Metz D. Demographic determinants of daily travel demand. Transp. Policy. 2012; 21 :20–25. doi: 10.1016/j.tranpol.2012.01.007. [ CrossRef ] [ Google Scholar ]
- Mokhtarian P.L., Meenakshisundaram R. Beyond tele-substitution: disaggregate longitudinal structural equations modeling of communication impacts. Transp. Res. Part C: Emerg. Technol. 1999; 7 (1):33–52. [ Google Scholar ]
- ODOT, 2020. Oregon Department of Transportation. Impact of Covid-19 on Traffic: March 16 to May 6 and May 4 to June 24 2020 Reports.
- Pani A., Mishra S., Golias M., Figliozzi M. Evaluating Public Acceptance of Autonomous Delivery Robots During COVID-19 Pandemic. Transp. Res. Part D: Transp. Environ. 2020; 89 :102600. doi: 10.1016/j.trd.2020.102600. [ CrossRef ] [ Google Scholar ]
- Perboli G., Rosano M. Parcel delivery in urban areas: Opportunities and threats for the mix of traditional and green business models. Transp. Res. Part C: Emerg. Technol. 2019; 99 :19–36. [ Google Scholar ]
- Potoglou D., Palacios J.-F., Feijóo C. An integrated latent variable and choice model to explore the role of privacy concern on stated behavioural intentions in e-commerce. J. Choice Modell. 2015; 17 :10–27. doi: 10.1016/j.jocm.2015.12.002. [ CrossRef ] [ Google Scholar ]
- PYMNTS, 2018. Amazon retail e-commerce sales in the United States from 2014 to 2018, by product category (in million U.S. dollars). Statista. Statista Inc. Available at: https://www. statista.com/statistics/979781/amazon-retail-e-retail-sales-by-product-category-usa/ (Accessed: June 30, 2020.
- R Core Team, 2020. R: A language and environment for statistical computing. R Foundation for Statistical Computing, Vienna, Austria. https://www.R-project.org/ .
- Rahimi, E., Shabanpour, R., Shamshiripour, A., (Kouros) Mohammadian, A., 2021. Perceived risk of using shared mobility services during the COVID-19 pandemic. Transp. Res. Part F: Traff. Psychol. Behav. 81, 271–281. 10.1016/j.trf.2021.06.01. [ CrossRef ]
- Revelle, W., Revelle, M.W., 2015. Package ‘psych.’ The comprehensive R archive network.
- Ripley B., Venables B., Bates D.M., Hornik K., Gebhardt A., Firth D., Ripley M.B. Package ‘mass’ Cran R. 2013; 538 [ Google Scholar ]
- Rodrigue, J., 2017. Residential Parcel Deliveries: Evidence from a Large Apartment Complex, Project Number: 17-5.1d, Final Report March 2017, MetroFreight, Accessed at : https://www.metrans.org/ (Accessed on June 1 2019).
- Salon, D., Conway, M.W., Capasso da Silva, D., Chauhan, R.S., Derrible, S., Mohammadian, A. (Kouros), Khoeini, S., Parker, N., Mirtich, L., Shamshiripour, A., Rahimi, E., Pendyala, R.M., 2021. The potential stickiness of pandemic-induced behavior changes in the United States. Proc. Natl. Acad. Sci. U.S.A. 118, e2106499118. 10.1073/pnas.2106499118. [ PMC free article ] [ PubMed ] [ CrossRef ]
- Schmid B., Axhausen K.W. In-store or online shopping of search and experience goods: A hybrid choice approach. J. Choice Modell. 2019; 31 :156–180. [ Google Scholar ]
- Schmid, B., Schmutz, S., Axhausen, K.W., 2016. Exploring the choice between in-store and online shopping 35 p. 10.3929/ETHZ-B-000114973. [ CrossRef ]
- Seo, S., Lee, J., 2017. An analysis on the changes in the parcel delivery services market with growing home-based consumption in Korea, by The Korea Transport Institute, presented at the 7th Metrans I-NUF Conference, Long Beach, CA. October 18–20, 2017. Slide 8.
- Shakibaei S., de Jong G.C., Alpkökin P., Rashidi T.H. Impact of the COVID-19 pandemic on travel behavior in Istanbul: A panel data analysis. Sustain. Cities Soc. 2020; 65 :102619. doi: 10.1016/j.scs.2020.102619. [ PMC free article ] [ PubMed ] [ CrossRef ] [ Google Scholar ]
- Siren A., Haustein S. Baby boomers’ mobility patterns and preferences: What are the implications for future transport? Transp. Policy. 2013; 29 :136–144. doi: 10.1016/j.tranpol.2013.05.001. [ CrossRef ] [ Google Scholar ]
- Sparrow R., Howard M. When human beings are like drunk robots: Driverless vehicles, ethics, and the future of transport. Transp. Res. Part C: Emergi. Technol. 2017; 80 :206–215. [ Google Scholar ]
- SUBTA, 2021. Subscriptions are Reshaping Industries During the Pandemic | Blog [WWW Document]. SUBTA. URL https://subta.com/subscriptions-are-reshaping-industries-during-the-pandemic/ (accessed 8.4.21).
- USCB, 2021. American Community Survey Data, US Census Bureau [WWW Document]. The United States Census Bureau. URL https://www.census.gov/programs-surveys/acs/data.html (accessed 8.7.21).
- USDC, 2020. U.S. Department of Commerce Quarterly retail e-commerce sales: 4th quarter 2019 (online). Available at: https://www.census.gov/retail/index.html#ecommerce (accessed July 1 2020).
- Varma K.V., Ho C.-I., Stanek D.M., Mokhtarian P.L. Duration and frequency of telecenter use: Once a telecommuter, always a telecommuter? Transp. Res. Part C: Emerg. Technol. 1998; 6 (1-2):47–68. [ Google Scholar ]
- Vij A., Walker J.L. How, when and why integrated choice and latent variable models are latently useful. Transp. Res. Part B: Methodol. 2016; 90 :192–217. doi: 10.1016/j.trb.2016.04.021. [ CrossRef ] [ Google Scholar ]
- Zhai Q., Cao X., Mokhtarian P.L., Zhen F. The interactions between e-shopping and store shopping in the shopping process for search goods and experience goods. Transportation. 2017; 44 (5):885–904. [ Google Scholar ]
- Zhao G., Okoro C.A., Hsia J., Garvin W.S., Town M. Prevalence of Disability and Disability Types by Urban-Rural County Classification—U.S., 2016. Am. J. Prev. Med. 2019; 57 (6):749–756. doi: 10.1016/j.amepre.2019.07.022. [ PMC free article ] [ PubMed ] [ CrossRef ] [ Google Scholar ]
- Zhen F., Cao X., Mokhtarian P.L., Xi G. Associations between online purchasing and store purchasing for four types of products in Nanjing, China. Transp. Res. Rec. 2016; 2566 (1):93–101. [ Google Scholar ]
- Zhen F., Du X., Cao J., Mokhtarian P.L. The association between spatial attributes and e-shopping in the shopping process for search goods and experience goods: Evidence from Nanjing. J. Transp. Geogr. 2018; 66 :291–299. [ Google Scholar ]
- Zhou Y., Wang X.C. Explore the relationship between online shopping and shopping trips: An analysis with the 2009 NHTS data. Transp. Res. Part A: Policy Pract. 2014; 70 :1–9. [ Google Scholar ]
Title characteristics and citations in economics
- Published: 03 January 2017
- Volume 110 , pages 1573–1578, ( 2017 )
Cite this article
- Matthias Gnewuch 1 &
- Klaus Wohlrabe 2
1077 Accesses
25 Citations
10 Altmetric
Explore all metrics
We investigate the relationship between article title characteristics and citations in economics using a large data set from Web of Science. Our results suggest that articles with a short title that also contains a non-alphanumeric character achieve a higher citation count.
This is a preview of subscription content, log in via an institution to check access.
Access this article
Price includes VAT (Russian Federation)
Instant access to the full article PDF.
Rent this article via DeepDyve
Institutional subscriptions
We conducted the same analysis with the number of characters instead of the number of words and obtained similar results. We focus on words to make the results more intuitive.
We also performed regressions including the quadratic term of words and obtained substantially the same results.
Alimoradi, F., Javadi, M., Mohammadpoorasl, A., Moulodi, F., Hajizadeh, M., et al. (2016). The effect of key characteristics of the title and morphological features of published articles on their citation rates. Annals of Library and Information Studies (ALIS) , 63 (1), 74–77.
Google Scholar
Buter, R., & van Raan, A. F. (2011). Non-alphanumeric characters in titles of scientific publications: An analysis of their occurrence and correlation with citation impact. Journal of Informetrics , 5 (4), 608–617.
Article Google Scholar
Ely, J. C. (2016). Beeps. American Economic Review (forthcoming).
Guo, S., Zhang, G., Ju, Q., Chen, Y., Chen, Q., & Li, L. (2015). The evolution of conceptual diversity in economics titles from 1890 to 2012. Scientometrics , 102 (3), 2073–2088.
Hartley, J. (2007). Planning that title: Practices and preferences for titles with colons in academic articles. Library and Information Science Research , 29 (4), 553–568. doi: 10.1016/j.lisr.2007.05.002 .
Nair, L. B., & Gibbert, M. (2016). What makes a good title and (how) does it matter for citations? A review and general model of article title attributes in management science. Scientometrics , 107 (3), 1331–1359.
Paiva, C. E., Lima, J. P. S. N., & Paiva, B. S. R. (2012). Articles with short titles describing the results are cited more often. Clinics , 67 (5), 509–513.
Rostami, F., Mohammadpoorasl, A., & Hajizadeh, M. (2014). The effect of characteristics of title on citation rates of articles. Scientometrics , 98 (3), 2007–2010.
Subotic, S., & Mukherjee, B. (2014). Short and amusing: The relationship between title characteristics, downloads, and citations in psychology articles. Journal of Information Science , 40 (1), 115–124.
Yitzhaki, M. (1994). Relation of title length of journal articles to number of authors. Scientometrics , 30 (1), 321–332.
Download references
Author information
Authors and affiliations.
University of Bonn, Bonn, Germany
Matthias Gnewuch
Ifo Institute for Economic Research, University of Munich, Poschingerstr. 5, 81769, Munich, Germany
Klaus Wohlrabe
You can also search for this author in PubMed Google Scholar
Corresponding author
Correspondence to Klaus Wohlrabe .
Rights and permissions
Reprints and permissions
About this article
Gnewuch, M., Wohlrabe, K. Title characteristics and citations in economics. Scientometrics 110 , 1573–1578 (2017). https://doi.org/10.1007/s11192-016-2216-7
Download citation
Received : 10 October 2016
Published : 03 January 2017
Issue Date : March 2017
DOI : https://doi.org/10.1007/s11192-016-2216-7
Share this article
Anyone you share the following link with will be able to read this content:
Sorry, a shareable link is not currently available for this article.
Provided by the Springer Nature SharedIt content-sharing initiative
- Title characteristics
- Non-alphanumeric characters
- Title length
JEL Classification
- Find a journal
- Publish with us
- Track your research
- Open access
- Published: 18 April 2024
Implementation of an audit and feedback module targeting low-value clinical practices in a provincial trauma quality assurance program: a cost-effectiveness study
- Blanchard Conombo 1 , 2 ,
- Jason R. Guertin 1 ,
- Jeffrey S. Hoch 3 ,
- Jeremy Grimshaw 4 ,
- Mélanie Bérubé 2 , 5 ,
- Christian Malo 5 ,
- Simon Berthelot 2 , 6 , 7 ,
- François Lauzier 8 ,
- Henry T. Stelfox 9 ,
- Alexis F. Turgeon 2 , 8 ,
- Patrick Archambault 10 , 6 ,
- Amina Belcaid 2 &
- Lynne Moore 1 , 2
BMC Health Services Research volume 24 , Article number: 479 ( 2024 ) Cite this article
132 Accesses
Metrics details
Audit and Feedback (A&F) interventions based on quality indicators have been shown to lead to significant improvements in compliance with evidence-based care including de-adoption of low-value practices (LVPs). Our primary aim was to evaluate the cost-effectiveness of adding a hypothetical A&F module targeting LVPs for trauma admissions to an existing quality assurance intervention targeting high-value care and risk-adjusted outcomes. A secondary aim was to assess how certain A&F characteristics might influence its cost-effectiveness.
We conducted a cost-effectiveness analysis using a probabilistic static decision analytic model in the Québec trauma care continuum. We considered the Québec Ministry of Health perspective. Our economic evaluation compared a hypothetical scenario in which the A&F module targeting LVPs is implemented in a Canadian provincial trauma quality assurance program to a status quo scenario in which the A&F module is not implemented. In scenarios analyses we assessed the impact of A&F characteristics on its cost-effectiveness. Results are presented in terms of incremental costs per LVP avoided.
Results suggest that the implementation of A&F module (Cost = $1,480,850; Number of LVPs = 6,005) is associated with higher costs and higher effectiveness compared to status quo (Cost = $1,124,661; Number of LVPs = 8,228). The A&F module would cost $160 per LVP avoided compared to status quo. The A&F module becomes more cost-effective with the addition of facilitation visits; more frequent evaluation; and when only high-volume trauma centers are considered.
A&F module targeting LVPs is associated with higher costs and higher effectiveness than status quo and has the potential to be cost-effective if the decision-makers’ willingness-to-pay is at least $160 per LVP avoided. This likely represents an underestimate of true ICER due to underestimated costs or missed opportunity costs. Results suggest that virtual facilitation visits, frequent evaluation, and implementing the module in high-volume centers can improve cost-effectiveness.
Peer Review reports
Introduction
Low-value practices (LVPs) are tests and treatments that are not supported by evidence and may expose patients to physical and psychological harm [ 1 , 2 ]. They have been estimated to consume up to 30% of healthcare resources in Canada [ 3 ] and in the US [ 4 ]. In 2013, an estimated $270 billion was wasted on excess healthcare services in the US [ 2 ]. From a patient and caregiver perspective, LVPs expose patients to physical and psychological harms, delays to effective treatment, and direct and indirect expenses [ 2 , 5 , 6 , 7 , 8 ]. From a healthcare system perspective, they put strain on tight healthcare budgets and decrease the availability of scarce resources.
Recent literature suggests that interventions targeting the de-implementation of ineffective or harmful health interventions have the potential to reduce overuse and improve clinically important outcomes [ 9 ]. Among these are Audit and Feedback (A&F) interventions, defined as ‘a summary of clinical performance of healthcare over a specified period aimed at providing information to health professionals to allow them to assess and adjust their performance’ [ 10 ]. We now have extensive evidence of the effectiveness of A&F interventions, including those targeting de-implementation of LVPs. A systematic review including 140 randomized controlled trials (RCTs) estimated that A&F interventions resulted in close to 4.3% absolute increase in adherence to evidence-based care (IQR 0.5% to 16.0%) [ 11 ]. The effect of an A&F intervention appears to be larger when it targets de-implementation of low-value practices (absolute decrease of 10.5%). This review also revealed that A&F effectiveness is influenced by its design and delivery [ 11 ]. The World Health Organisation recently expressed concern about the major knowledge gap on the cost and cost-effectiveness of A&F interventions [ 12 ], and recommended that implementation of these interventions be informed by data on their cost-effectiveness [ 13 ]. Despite this, most A&F interventions, including those used across Canadian trauma systems, are implemented without evidence on their cost-effectiveness [ 12 , 14 ]. A 2022 systematic review summarized evidence on the economic value of A&F interventions in healthcare [ 15 ] and found that they have a high potential to be cost-effective. However, authors only identified economic evaluations for 6% of A&F trials, methodological quality of these evaluations was low, and authors concluded that model-based simulations were urgently needed to assess the impact of A&F characteristics on cost-effectiveness to inform optimal A&F design.
Trauma systems are a favorable setting for de-implementation interventions as they possess many documented facilitators including quality improvement teams with medical leadership, routinely-collected clinical data, and performance linked to accreditation [ 16 ]. Furthermore, potential gains are huge due to the resource-intensive nature of trauma care. Trauma systems are thus the ideal setting to advance knowledge on de-implementation. Our research team recently published a list of quality indicators targeting LVPs in acute trauma care [ 17 , 18 ]. We aim to evaluate the cost-effectiveness of an A&F module targeting the de-implementation of these LVPs in an integrated Canadian trauma system and to assess the impact of A&F characteristics on cost-effectiveness.
We conducted an economic evaluation according to the Canadian guidelines for the Economic Evaluation of Health Technologies [ 19 ], and results are reported following the CHEERS 2022 statement [ 20 ]. The study protocol was developed with a project advisory committee including two emergency physicians (CM, EM), two trauma surgeons (TR, NY), three critical care physicians (FL, AFT, HTS), a neurosurgeon (PLB), a spine surgeon (JP), an orthopedic surgeon (ML), two trauma service managers (MB, CR), a trauma registry co-ordinator (AB), and epidemiologist (LM), and two health economists (JRG, JSH). The protocol was approved a priori by all co-authors, members of the advisory committee, a granting agency peer-review committee (Canadian Institutes of Health Research project #353374) and the CHU-de Québec – Université Laval research ethics committee.
Our economic evaluation is based on a hypothetical A&F module embedded in the Québec Trauma Care Continuum , a provincial regionalized trauma system comprising 57 adult trauma centers of which 3 are level I (highly specialized urban centers), 5 are level II (similar capacity to level I but in smaller cities), 21 are level III (hospitals in small towns transferring most major trauma to level I/II centers after stabilization), and 28 are level IV (rural community hospitals). All centers undergo mandatory, periodic verifications in line with designation, conducted by the provincial healthcare quality agency, Institut national d’excellence en santé et services sociaux ( INESSS ) and overseen by the Ministry of Health and Social Services [ 21 ]. Verification includes A&F on adherence to high-value care and risk-adjusted outcomes. Local trauma committees in each center are required to ensure the quality of the trauma program according to designation requirements. Committees include the program medical director (Chair), the program manager, heads of critical care, emergency and surgical departments, heads of multidisciplinary services, and a hospital administrator. Quality improvement activities include trimestral committee meetings with chart review, development of local care protocols, and discussions with clinical and administrative leads locally and at referring centers to identify improvement strategies. Formal letters of agreement are signed by heads of clinical departments to operate changes in their services when required.
Intervention and comparator
We compared a hypothetical scenario in which an A&F module targeting LVPs is implemented in the Québec trauma system to a status quo scenario in which the A&F module is not implemented.
Comparator (status quo scenario)
The study comparator is the A&F intervention currently in place in the Québec Trauma Care Continuum, designed by the provincial healthcare quality agency using the US Agency for Healthcare Research and Quality guidelines [ 22 ]. This A&F intervention targets trauma committees in each trauma center and, as explained above, currently includes modules for adherence to high-value practices (15 quality indicators) and optimal outcomes (3 quality indicators). The A&F intervention currently in place consists of:
Quality reports disseminated via a Web platform to local trauma committees and hospital boards of directors produced using trauma registry data.
Web links to user-friendly information sheets including definitions for quality indicators and references supporting each indicator.
Information sheets and Web capsules with guidelines on how the results should be interpreted and acted upon.
A case revision tool integrated into the trauma registry.
Within 6 months of reception of the report, committees are required to submit an action plan proposing improvement strategy for quality indicators for which they are identified as negative outliers.
Intervention
The study intervention is an A&F module targeting LVPs (6 quality indicators) ( http://www.ohri.ca/auditfeedback/laboratories/ ). The 6 quality indicators were selected using the results of an expert consensus study [ 23 ] and an indicator validation study using data from the Quebec trauma registy [ 24 ].
In the base case scenario, the module includes the components already in place described in the status quo scenario, applied to quality indicators on LVPs. We attributed a 5-year lifespan to the A&F module as current literature recommends that quality indicators be updated every five years [ 25 ]. To account for the 5-year lifespan of the A&F module and its potential benefits one year beyond its lifespan, we used a 6-year time horizon.
Type of economic evaluation
For this early economic evaluation, i.e., an evaluation prior to the implementation of the module, a probabilistic static decision analytic model was developed to estimate the incremental cost-effectiveness ratio (ICER) of the A&F module compared with status quo scenario in which the A&F module is not implemented for patients with acute injury (Fig. 1 ). We considered the Québec Ministry of Health perspective.
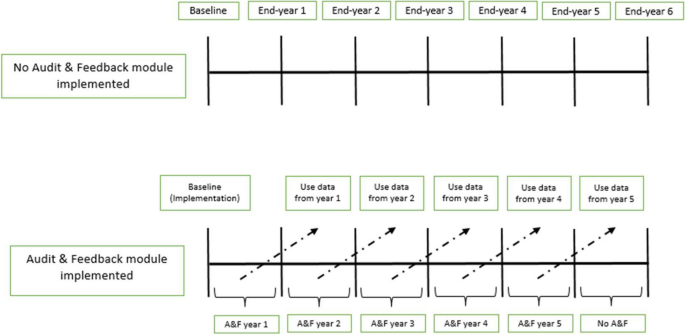
Decision-analytic model. In the status quo scenario, there is no implementation of the A&F module targeting LVPs. In the intervention scenario, an A&F module targeting LVPs is implemented at baseline (at the beginning of the 1st year). “Use data from year 1” means that data on the effectiveness and costs of A&F module from the 1st year are available at the beginning of the second year and so on for the following years
Effectiveness
The incremental effectiveness of the A&F module was estimated as the incremental number of LVPs avoided. Plausible ranges of percent reductions in LVPs were obtained from the 2012 Cochrane review, which presented effectiveness of A&F interventions as medians and interquartile ranges [ 26 ]. Specifically, we used the pooled estimate of effectiveness specific to deimplementation interventions based on 29 studies. For the purposes of our analysis, these values were used to estimate mean effectiveness and associated standard errors using a method based on highly-cited recommendations [ 27 , 28 ].
The incremental costs of the A&F module over the comparator were estimated by summing the implementation costs of the A&F module over its 5-year lifespan and the potential reduction in resource utilization for all LVPs, valued in costs and estimated between years 2 and 6. The implementation costs were determined by identifying all non-recurrent and recurrent costs related to the implementation of the A&F module including data validation and analyses, report production and validation, administrative costs, and follow-up in local trauma committees (Table 1 ). The potential reduction in resource utilization was estimated by multiplying the hypothesized reduction in the frequency of the LVPs by their average costs. Detailed information on how practices were costed are available elsewhere [ 29 ]. Briefly, we estimated direct healthcare costs for each LVP from the Ministry of Health perspective using an activity-based costing approach. Activity-based costing involves multiplying unit costs of specific activity centres by the corresponding units of resources used. This method provides an estimate of hospital resource use by activity center, consistent with Grading of Recommendations Assessment, Development and Evaluations guidelines [ 30 , 31 , 32 ]. All costs are expressed in 2020 Canadian dollars. We report our study following the Consolidated Health Economic Evaluation Reporting Standards statement [ 29 ].
Incremental cost-effectiveness ratio
The incremental cost-effectiveness ratio (ICER) was estimated by dividing the incremental costs (or savings) of the A&F module by its incremental effectiveness. Results are reported as the incremental cost per LVP avoided.
Discount rate
All future costs and benefits were discounted at a rate of 1.5% as recommended by current Canadian guidelines [ 19 ].
Scenario analyses
Our advisory committee identified 6 scenario-specific sensitivity analyses based on published evidence of A&F effectiveness and context-specific considerations (#1, 2, 3, 6) [ 24 , 26 ] as well as Canadian guidelines on economic evaluation (#4, 5):
Adding a virtual facilitation visit once per cycle to help trauma committees identify barriers and facilitators and use them to identify improvement strategies for their action plan; [ 15 , 26 ]
Increasing feedback frequency from annually to monthly, as assessed in the systematic review; [ 26 ]
Implementing the module only in high-volume trauma centers (i.e., level I and II);
Varying the discount rate between 0 and 5%, as recommended by current Canadian guidelines; [ 19 ]
Increasing the lifespan of the A&F module to 10 years (Supplementary Fig. 1 ) to take into account the effect of time on module effectiveness.
Increasing the costs of LVPs by 100% to account for lack of complete data on physician billing and unit costs that underestimate market prices. This is based on evidence that physician billing represents approximately 56% of hospital costs in Canada ( https://www.cihi.ca/sites/default/files/document/nhex-trends-2020-narrative-report-en.pdf ).
We present the ICER based on the results of probabilistic sensitivity analysis (PSA) as recommended in the Canadian Guidelines for the Economic Evaluation of Health Technologies [ 19 ]. In the PSA, model parameters were represented by distributions of possible values rather than point estimates to address parameter uncertainty. All parameters and their distributions are presented in Table 2 . Parameter distributions were randomly sampled 10,000 times. Results were summarized using cost-effectiveness acceptability curves (CEACs) and the cost-effectiveness acceptability frontier (CEAF) [ 33 ]. We used Excel software (Microsoft Office 2019 Professional Plus) to construct a decision model, to analyze base case results, and conduct PSA.
The mean costs of the A&F module and status quo scenario were $1,480,850 and $1,124,661 respectively. The associated average number of LVPs were 6,005 for the A&F module and 8,228 for status quo scenario. The implementation of the A&F module is associated with a reduction of approximately 2,223 LVPs. The ICER for the A&F module versus status quo scenario was $160 per LVP avoided (Table 3 ). The results of the PSA plotted on a cost-effectiveness plane (Fig. 2 ) show that most of the points in the scatter plot are located in the Northeast quadrant, indicating that the A&F module has a potential to be cost-effective given a decision maker’s willingness-to-pay (WTP). The cost-effectiveness acceptability frontier indicates that A&F module is cost-effective in 50% of our iterations at a WTP of $160 per LVP avoided (Fig. 3 ).
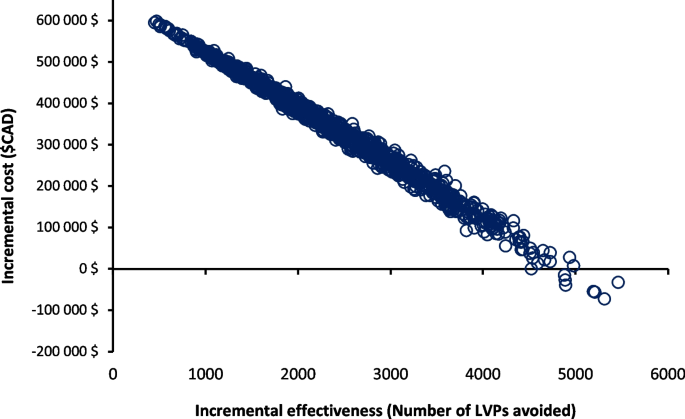
Probabilistic sensitivity analysis comparing A&F module and status quo scenario (no A&F module targeting LVPs). The x-axis represents the incremental effectiveness, number of LVPs avoided. The y-axis represents the incremental costs between A&F module and status quo scenario. Each circle represents a single simulation for a total of 10,000 simulations
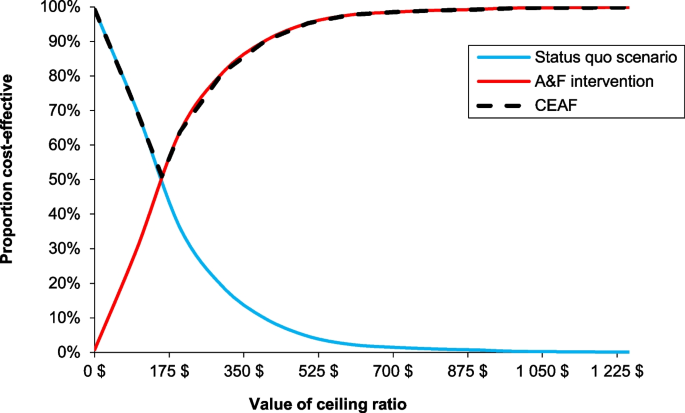
Cost-effectiveness acceptability curve (CEAC) between A&F module and status quo scenario and cost-effectiveness acceptability frontier (CEAF). The x-axis represents the willingness-to-pay (WTP) for each LVP avoided. The y-axis represents the percentage of simulations in which the A&F module is cost-effectiveness relative to the status quo scenario at different WTP threshold. The switch point where the A&F module became a cost-effective intervention corresponds to $160 per LVP avoided, equal to the ICER estimate. A&F module became 100% cost-effective at a WTP of $1000 per LVP avoided. The A&F module had the highest expected net benefit, for all values of WTP greater than the ICER. At our ICER estimate, 50% of the distribution of ICERs were cost-effective
Adding a virtual facilitation visit to the A&F module (one visit per A&F cycle) would reduce the estimated ICER (improve its cost-effectiveness profile compared to the base case scenario) to $108 per LVP avoided (Table 4 ). More frequent feedback (monthly) is associated with a slight improvement in its cost-effectiveness profile ($154 per LVP avoided). The A&F module is more cost-effective ($48 per LVP avoided) when only high-volume trauma centers are considered for the implementation of the module. Similarly, an increase in the costs of LVPs by 100% and a longer time horizon would lead to a reduction in the ICER to $10 and $106 per LVP avoided, respectively. On the other hand, a discount rate of 5% increases the ICER to $199 per LVP avoided (Table 4 ).
The results of this early economic evaluation suggest that the addition of an A&F module targeting LVPs to a provincial trauma quality assurance program over a time horizon of 6 years is associated with an ICER of $160 per LVP avoided. In analyses that simultaneously accounted for uncertainty in all key model parameters, 50% of simulations were cost-effective at a WTP of $160 per LVP avoided. The A&F module is more cost-effective with the addition of facilitation visits, frequent evaluation and if restricted to high-volume trauma centers.
Our study fills a major knowledge gap on the potential cost-effectiveness of A&F interventions to de-implement low-value care. Comparison of our results with the literature on acute trauma care is difficult, because there are no studies that have assessed the cost-effectiveness profile of A&F interventions in the context of acute injury care. However, a 2022 systematic review on the economic value of A&F interventions in various health areas summarized results of 35 studies that compared different A&F strategies targeting health professionals compliance with desire practices or patient health outcomes [ 15 ]. The results of this systematic review mirror our findings. Of 14 cost-effectiveness analyses based on changes in compliance to desired practice from the public healthcare payer perspective, 12 (86%) studies found that the A&F interventions were more costly but more effective than the comparator [ 15 ]. From studies assessing de-implementation of LVPs [ 34 , 35 ], A&F interventions were associated with a reduction in the overuse of LVPs and had the potential to be cost-effective [ 34 , 35 ]. Four (28%) studies included in the review conducted simulations to assess the influence of A&F characteristics on cost-effectiveness in scenario analyses [ 34 , 35 , 36 , 37 ]. Despite having different comparator groups (do-nothing scenario), these studies also observed improved cost-effectiveness when facilitation visits are added to A&F intervention [ 35 ] and the time horizon of the intervention is increased to 9 months (4 to 9 months) [ 34 ]. In addition, our study provides evidence that cost-effectiveness of A&F interventions may be improved by increasing frequency and restricting the intervention to high volume hospitals.
Strengths and limitations
Our study is based on effectiveness parameters from a meta-analysis on over 140 RCTs on the effectiveness of A&F interventions in different healthcare settings [ 11 ], on observed data on the frequency of LVPs [ 24 ], and on costs based on a mature, province-wide quality assurance program ( https://www.donneesquebec.ca/recherche/dataset/as-471-rapports-financiers-annuels-des-etablissements ). In addition, we conducted extensive sensitivity analyses and a range of scenario analyses to evaluate the robustness of our results and to assess the influence of key A&F characteristics on its cost-effectiveness profile. Despite these strengths, our results should be interpreted within the context of the study’s limitations. First, our evaluation is based on estimates of effectiveness from a meta-analysis published in 2012. While this study represents the most up-to-date evidence synthesis available (the Cochrane review is yet to be updated [ 38 ] and systematic reviews published more recently have not included meta-analyses) [ 39 ], it does not include the most recent evidence. Furthermore, while we used estimates specific to deimplementation interventions from the review, none of the studies were specific to trauma, none evaluated an intervention delivered in the context of accreditation, and none compared a deimplementation module in a system with an A&F intervention already in place. Furthermore, risk of bias was low for only 31% of included studies. The estimate used may therefore represent an underestimate or overestimate of the true effectiveness. Second, we conducted an early economic evaluation to assess if a hypothetical A&F module could be cost-effective and, if so, under which conditions. As such, the results of our economic evaluation provide encouragement that the true ICER of the intervention were it to be designed and implemented in the Québec trauma system might be promising as well. We used a broad range of scenarios and parameter values within our probabilistic sensitivity analyses but attributed the same weight to all scenarios analyzed. The base-case scenario will not necessarily be the one that will be implemented. However, intervention costs were based on resources currently used in the Québec trauma care continuum and opportunity costs related to LVPs avoided were based on observed baseline frequencies. We plan to conduct an economic evaluation after our cluster randomized trial (funded and currently underway) to assess the true (observed) cost-effectiveness of the intervention in a pragmatic setting. Third, opportunity costs of LVPs avoided did not fully account for physician fees and were based on unit costs that are known to underestimate their true costs. In addition, we did not account for potential resource repercussions of LVPs, for example, re-imaging due to uncertain findings or treatment of clinically nonsignificant incidental findings. Our scenario analysis where costs associated with LVPs were increased by 100% probably better reflects the Québec Ministry of Health perspective; the large decrease in the ICER ($160 to $10) suggest that opportunity costs related to LVPs are an important determinant of the cost-effectiveness of an A&F module targeting de-implementation. Furthermore, we only considered direct healthcare costs associated with the two competing strategies and did not factor in the effects of indirect costs (e.g., time off work for patients) from LVPs, which would also have led to an underestimation of the intervention’s cost-effectiveness. Fourth, our study is based on the single healthcare payer model, and it is uncertain if our findings would be applicable to other jurisdictions with alternate payer systems. Also, physicians in Canada receive payments based on fee for service that is periodically negotiated [ 40 ], so our results are dependent on current unit costs in our system and may not apply well in non-universal health systems or other jurisdictions with different structures. Fifth, in the absence of evidence indicating otherwise, our base case scenario was based on the strong assumption that effectiveness was the same for all 6 indicators. However, in probabilistic sensitivity analyses, we allowed the effectiveness of LVP to vary independently. Sixth, we were unable to take account of the uncertainty of the cost estimates of implementing the A&F module, derived from expert consultation, which we anticipate may have been underestimated. Finally, we deliberately focused on adherence to desired practice (LVPs avoided) rather than health outcomes (e.g., adverse events) due to lack of available data associated with utility/disutility of LVPs for trauma patients. Nevertheless, a strong argument can be made for focus on the measurement of LVPs avoided for assessment of the quality of our A&F intervention, as they relate most closely to actions that are within the control of healthcare professionals. Indeed, economic evaluations of similar A&F interventions have obtained more meaningful results with similar intermediate outcomes than with Quality-Adjusted Life Years (QALYs) [ 41 ]. Studies have also demonstrated that reducing LVPs will reduce physical harms and adverse events [ 42 , 43 , 44 ]. However, this probably led to an underestimation of the true cost-effectiveness profile of our A&F module as health outcomes or negative health consequences of LVPs are not considered in the measure of effectiveness [ 15 ].
Potential impact
The outcome parameter used in decision model (LVPs avoided) is unique and does not have an explicit cost-effectiveness threshold associated with it. Therefore, the decision to invest in the intervention will be based on the decision-makers willingness-to-pay, i.e., would they be prepared to invest 160$ per LVP avoided? However, the decision should also be based on other considerations, e.g., opportunity costs are likely to be greater than those estimated, cost-effectiveness may be increased if virtual facilitation visits are added, if the frequency of evaluations are increased, and if the intervention is restricted to high-volume trauma centers (level I and II). The intervention has the potential to lead to a global awareness of healthcare overuse and therefore a decrease in other LVPs [ 24 ].
Our economic evaluation suggests that an A&F module targeting de-implementation, integrated into a provincial quality-assurance program, has a high potential to reduce LVPs while increasing total healthcare costs, with an ICER of $160 per LVP avoided. Results suggest that virtual facilitation visits, frequent evaluation and implementing the intervention only in high-volume centers increase cost-effectiveness. However, its economic potential is likely underestimated in this study due to opportunity costs that were underestimated (costs of LVPs) or not accounted for (indirect costs, health outcomes, and long-term consequences). The findings of the present study may inform the development of A&F interventions targeting de-implementation and they demonstrate the feasibility of conducting early economic evaluations to inform optimal A&F intervention design.
Availability of data and materials
Quebec Trauma Registry is subject to a third-party restriction (Quebec Ministry of Health and Social Services).
Abbreviations
Low-value practices
Audit and Feedback
Randomized Controlled Trial
Willingness-to-pay
Computed tomography
Interquartile Range
Cost-effectiveness acceptability curve
Cost-effectiveness acceptability frontier
Probability sensitivity analysis
Choosing Wisely. Home page. Accessed February 21, 2022. https://www.choosingwisely.org/ .
Brownlee S, Chalkidou K, Doust J, et al. Evidence for overuse of medical services around the world. Lancet. 2017;390(10090):156–68.
Article PubMed PubMed Central Google Scholar
Canadian Institute for Health Information. CIHI. unnecessary care in Canada: technical report. Ottawa, ON: CIHI, 2017.
Lown Institute. Low-value care. https://lowninstitute.org/lown-issues/low-value-care/ . Accessed 20 Dec 2022.
Berwick DM, Hackbarth AD. Eliminating waste in US health care. JAMA. 2012;307(14):1513–6.
Article CAS PubMed Google Scholar
Boat TF, Chao SM, O’Neill PH. From waste to value in health care. JAMA. 2008;299(5):568–71.
Reilly BM, Evans AT. Much ado about (doing) nothing. Ann Intern Med. 2009;150(4):270–1.
Article PubMed Google Scholar
Hauser CJ, Adams CA Jr, Eachempati SR. Surgical Infection Society guideline: prophylactic antibiotic use in open fractures: an evidence-based guideline. Surg infect. 2006;7(4):379–405.
Article Google Scholar
Niven DJ, Mrklas KJ, Holodinsky JK, et al. Towards understanding the de-adoption of low-value clinical practices: a scoping review. BMC Med. 2015;13:255.
World Health Organization. Using audit and feedback to health professionals to improve the quality and safety of health care. https://apps.who.int/iris/handle/10665/332014 . Accessed 20 Dec 2022.
Noah I. Optimizing Audit and Feedback Interventions to Improve Quality in Primary Care. 2014.
Flottorp S, Jamtvedt G, Gibis B, McKee M. Using audit and feedback to health professionals to improve the quality and safety of health care. http://www.euro.who.int/__data/assets/pdf_file/0003/124419/e94296.pdf . Published 2010. Accessed.
Soleymani F, Rashidian A, Dinarvand R, Kebriaeezade A, Hosseini M, Abdollahi M. Assessing the effectiveness and cost-effectiveness of audit and feedback on physician’s prescribing indicators: study protocol of a randomized controlled trial with economic evaluation. Daru. 2012;20(1):88–88.
Avery AJ, Rodgers S, Cantrill JA, et al. A pharmacist-led information technology intervention for medication errors (PINCER): a multicentre, cluster randomised, controlled trial and cost-effectiveness analysis. Lancet. 2012;379(9823):1310–9.
Moore L, Guertin JR, Tardif P-A, et al. Economic evaluations of audit and feedback interventions: a systematic review. BMJ Qual Saf. 2022;31(10):754.
Squires JE, Sullivan K, Eccles MP, Worswick J, Grimshaw JM. Are multifaceted interventions more effective than single-component interventions in changing health-care professionals’ behaviours? An overview of systematic reviews. Implement Sci. 2014;9(1):152.
Fitch K, Bernstein JS, Aguilar MD, et al. The RAND/UCLA Appropriateness Method User's Manual. https://www.rand.org/pubs/monograph_reports/MR1269.html . Published 2001. Accessed 17 Sept 2018.
Heyworth J. Trauma services in a district general hospital. BMJ (Clinical research ed). 1990;300(6728):876–7.
CADTH. Guidelines for the economic evaluation of health technologies: Canada. 4th edition. Ottawa: CADTH; 2017.
Husereau D, Drummond M, Augustovski F, et al. Consolidated Health Economic Evaluation Reporting Standards 2022 (CHEERS 2022) statement: updated reporting guidance for health economic evaluations. BMJ (Clinical research ed). 2022;376:e067975.
PubMed Google Scholar
Ministère de la Santé et des Services sociaux. Trajectoire - Traumatologie. https://www.msss.gouv.qc.ca/professionnels/soins-et-services/guide-urgences-trajectoire-traumatologie/ . Published 2022. Accessed 13 Sept 2022.
Developing a framework and research agenda for overuse and appropriateness measures. Agency for Healthcare Research and Quality Appropriateness Small Conference. Published 2009. Accessed 17 Sept 2018.
Moore L, Bérubé M, Tardif PA, et al. Quality Indicators targeting low-value clinical practices in trauma care. JAMA Surg. 2022;157(6):507–14.
Moore L, Bérubé M, Tardif PA, et al. Validation of quality indicators targeting low-value trauma care. JAMA Surg. 2022;157(11):1008–16.
Stelfox HT, Straus SE. Measuring quality of care: considering measurement frameworks and needs assessment to guide quality indicator development. J Clin Epidemiol. 2013;66(12):1320–7.
Ivers N, Jamtvedt G, Flottorp S, et al. Audit and feedback: effects on professional practice and healthcare outcomes. Cochrane Datab Syst Rev. 2012;6:Cd000259.
Google Scholar
Hozo SP, Djulbegovic B, Hozo I. Estimating the mean and variance from the median, range, and the size of a sample. BMC Med Res Methodol. 2005;5(1):13.
Wan X, Wang W, Liu J, Tong T. Estimating the sample mean and standard deviation from the sample size, median, range and/or interquartile range. BMC Med Res Methodol. 2014;14(1):135.
Conombo B, Guertin JR, Hoch JS, et al. Potential avoidable costs of low-value clinical practices in acute injury care in an integrated canadian provincial trauma system. JAMA Surg. 2023;158(9):977–9.
Canby JBT. Applying activity-based costing to healthcare settings. Healthc Financ Manag. 1995;49(2):50–2 54-56.
Chan YC. Improving hospital cost accounting with activity-based costing. Health Care Manage Rev. 1993;18(1):71–7.
Guyatt GH, Oxman AD, Kunz R, et al. Incorporating considerations of resources use into grading recommendations. BMJ. 2008;336(7654):1170–3.
Fenwick E, Claxton K, Sculpher M. Representing uncertainty: the role of cost-effectiveness acceptability curves. Health Econ. 2001;10(8):779–87.
Ling R, Giles M, Searles A. Administration of indwelling urinary catheters in four Australian Hospitals: cost-effectiveness analysis of a multifaceted nurse-led intervention. BMC Health Serv Res. 2021;21(1):897.
Gandjour A, Lauterbach KW. When is it worth reducing overuse of health care services? The example of prescribing expensive antihypertensives. Med Klin (Munich). 2005;100(9):535–41.
Barasa EW, Ayieko P, Cleary S, English M. A multifaceted intervention to improve the quality of care of children in district hospitals in Kenya: a cost-effectiveness analysis. PLoS Med. 2012;9(6):e1001238.
Huis A, Schoonhoven L, Grol R, Donders R, Hulscher M, van Achterberg T. Impact of a team and leaders-directed strategy to improve nurses’ adherence to hand hygiene guidelines: a cluster randomised trial. Int J Nurs Stud. 2013;50(4):464–74.
Crawshaw J, Meyer C, Antonopoulou V, et al. Identifying behaviour change techniques in 287 randomized controlled trials of audit and feedback interventions targeting practice change among healthcare professionals. Implement Sci. 2023;18(1):63.
Dunsmore J, Duncan E, MacLennan S, N’Dow J, MacLennan S. Effectiveness of de-implementation strategies for low-value prescribing in secondary care: a systematic review. Implement Sci Commun. 2023;4(1):115.
Ridic G, Gleason S, Ridic O. Comparisons of health care systems in the United States. Germany Canada Mater Sociomed. 2012;24(2):112–20.
Elliott RA, Putman Kd Fau - Franklin M, Franklin M Fau - Annemans L, et al. Cost effectiveness of a pharmacist-led information technology intervention for reducing rates of clinically important errors in medicines management in general practices (PINCER). (1179–2027 (Electronic)).
Elliott RA, Putman KD, Franklin M, et al. Cost effectiveness of a pharmacist-led information technology intervention for reducing rates of clinically important errors in medicines management in general practices (PINCER). Pharmacoeconomics. 2014;32(6):573–90.
Johri M, Ng ESW, Bermudez-Tamayo C, Hoch JS, Ducruet T, Chaillet N. A cluster-randomized trial to reduce caesarean delivery rates in Quebec: cost-effectiveness analysis. BMC Med. 2017;15(1):96.
Rodriguez-Martinez CE, Sossa-Briceño MP, Castro-Rodriguez JA. Cost-effectiveness of the utilization of “good practice” or the lack thereof according to a bronchiolitis evidence-based clinical practice guideline. J Eval Clin Pract. 2019;25(4):682–8.
Download references
Acknowledgements
We thank Natalie Yanchar, Éric Mercier, Jérôme Paquet, Tarek Razek, Martin Lesieur, Paule Lessard Boneaventure and Christine Rizzo for their role as members of the advisory committee.
Role of funder/sponsor
The funders had no role in developing this manuscript.
This research was supported by the Canadian Institutes of Health Research (Foundation grant, #353374).
Author information
Authors and affiliations.
Department of Social and Preventative Medicine, Université Laval, Québec City, Québec, Canada
Blanchard Conombo, Jason R. Guertin & Lynne Moore
Population Health and Optimal Health Practices Research Unit, Trauma – Emergency – Critical Care Medicine, Quebec University Hospital, Centre de Recherche du CHU de Québec-Université Laval, 18E Rue, Local H-012a, Québec City, Québec, 1401G1J 1Z4, Canada
Blanchard Conombo, Mélanie Bérubé, Simon Berthelot, Alexis F. Turgeon, Amina Belcaid & Lynne Moore
Division of Health Policy and Management, Department of Public Health Sciences, University of California at Davis, Davis, CA, USA
Jeffrey S. Hoch
Department of Medicine, University of Ottawa, Ottawa, ON, Canada
Jeremy Grimshaw
Faculty of Nursing, Université Laval, Québec City, Québec, Canada
Mélanie Bérubé & Christian Malo
Department of Family Medicine and Emergency Medicine, Université Laval, Québec City, Québec, Canada
Simon Berthelot & Patrick Archambault
Centre de Recherche Intégrée Pour Un Système Apprenant en Santé Et Services Sociaux, Centre Intégré de Santé Et de Services Sociaux de Chaudière-Appalaches, Lévis, Québec, Canada
Simon Berthelot
Department of Anesthesiology and Critical Care Medicine, Division of Critical Care Medicine, Université Laval, Québec City, Québec, Canada
François Lauzier & Alexis F. Turgeon
Department of Critical Care Medicine, Medicine and Community Health Sciences, O’Brien Institute for Public Health, University of Calgary, Calgary, AB, Canada
Henry T. Stelfox
VITAM-Centre de Recherche en Santé Durable, Québec City, Québec, Canada
Patrick Archambault
You can also search for this author in PubMed Google Scholar
Contributions
BC, LM, JRG, JSH, HTS, PA, SB, FL, AFT, JG, MB, CM and AB developed the original research concept and developed the study design. BC, LM, FL, AFT, JSH, HTS, AB, MB and JRG have contributed to the data acquisition, analysis, or interpretation. JH, HTS and SB oversaw the analysis and provided feedback. BC and LM developed the draft manuscript. All authors made substantial contributions to the manuscript development, critical revision and approved the final version of the manuscript.
Corresponding author
Correspondence to Lynne Moore .
Ethics declarations
Ethics approval and consent to participate.
This study was approved by the CHU-de Québec – Université Laval research ethics committee and patient consent is not required.
Consent for publication
Not applicable.
Competing interests
The authors declare no competing interests.
Additional information
Publisher’s note.
Springer Nature remains neutral with regard to jurisdictional claims in published maps and institutional affiliations.
Supplementary Information
Supplementary material 1., rights and permissions.
Open Access This article is licensed under a Creative Commons Attribution 4.0 International License, which permits use, sharing, adaptation, distribution and reproduction in any medium or format, as long as you give appropriate credit to the original author(s) and the source, provide a link to the Creative Commons licence, and indicate if changes were made. The images or other third party material in this article are included in the article's Creative Commons licence, unless indicated otherwise in a credit line to the material. If material is not included in the article's Creative Commons licence and your intended use is not permitted by statutory regulation or exceeds the permitted use, you will need to obtain permission directly from the copyright holder. To view a copy of this licence, visit http://creativecommons.org/licenses/by/4.0/ . The Creative Commons Public Domain Dedication waiver ( http://creativecommons.org/publicdomain/zero/1.0/ ) applies to the data made available in this article, unless otherwise stated in a credit line to the data.
Reprints and permissions
About this article
Cite this article.
Conombo, B., Guertin, J.R., Hoch, J.S. et al. Implementation of an audit and feedback module targeting low-value clinical practices in a provincial trauma quality assurance program: a cost-effectiveness study. BMC Health Serv Res 24 , 479 (2024). https://doi.org/10.1186/s12913-024-10969-2
Download citation
Received : 09 October 2023
Accepted : 09 April 2024
Published : 18 April 2024
DOI : https://doi.org/10.1186/s12913-024-10969-2
Share this article
Anyone you share the following link with will be able to read this content:
Sorry, a shareable link is not currently available for this article.
Provided by the Springer Nature SharedIt content-sharing initiative
- Audit and feedback
- Cost-effectiveness analysis
- Low-value care
BMC Health Services Research
ISSN: 1472-6963
- General enquiries: [email protected]
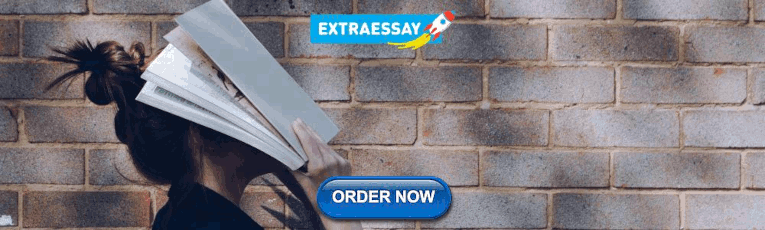
IMAGES
VIDEO
COMMENTS
1 Of course economics in principle and in most cases is pursued as a positive science, is concerned with the description and . ... 3.1 Socioeconomic Research in the Field of Economics: ...
2.2. Demographic and socioeconomic factors. Yusuf et al. (Citation 2014) define a demographic factor as the population characteristics, comprising of sex, marital status, living arrangements and household composition, language, ethnic background, health and disability, education and training, employment status and occupation, income and household consumption, and population densities and urban ...
The individual- and context-specific nature of poverty also influences the poverty analysis process. It helps poverty analysts to capture variations of the nature and severity of poverty according to age and gender as well as social, cultural, economic, political, environmental and spatial contexts. 3.4.
1 Of course economics in principle and in most cases is pursued as a positive science, is concerned with the description and explanation of economic phenomena, and "is in principle independent of any particular ethical position or normative judgments" (Friedman, Citation 1953).But positive economics can be used to derive normative implications. Most economists still identify normativity ...
Socio-economic status (SES) is conceptualized as the social and economic standing or class of an. individual or family. Socio-economic status of farmers is an important variable in agricultural ...
However, the characteristics of the socioeconomic context should not be overlooked. Specifically, this research was conducted in southeastern Spain. This region's overall socioeconomic reality, even some time after the economic crisis period, remains unfavorable.
Economic Characteristics and Subjective Well-Being. The primary objective of this study. is to examine the relationship between economic characteristics and w ell-being as one of the. components ...
The following are the most common demographic and socioeconomic background variables in cross-national and cross-cultural comparative surveys: Sex. Traditionally, this variable is collected as the biological sex of the respondent. "Male" and "female" are sex categories, whereas "masculine" and "feminine" are gender categories ...
However, the scope of the current research is the investigation of the existing differentiation in the socio-economic characteristics and the lifestyle of adults in Greece, in different geographical areas (urban, semi-urban, rural), the degree of treatment of these differentiated characteristics by geographical area, and the exploration of the possibility of more easily addressing these ...
Research during the past decade shows that social class or socioeconomic status (SES) is related to satisfaction and stability in romantic unions, the quality of parent-child relationships, and a range of developmental outcomes for adults and children. ... Personal characteristics and resilience to economic hardship and its consequences ...
However, research by Yang et al. (2015) suggests that a shrinking family size is associated with increased carbon emissions. Furthermore, studies by Yang et al. ... We examined household social-economic characteristics and assessed their influence on household carbon emissions. It was observed that households within the same economic group ...
Capturing essential economic characteristics of the population in the census is recommended as a core topic for the 2020 Round of Population and Housing Censuses. To support these efforts, this guide updates international census recommendations aligned with the latest ICLS standards, and develops practical tools and training to support census planners in adapting these recommendations to the ...
The overall trend in economic research during this period is the application of modern economic theories to China's economic life, as the country was increasingly integrated into the global economy and the socialist market economy system with Chinese characteristics and its theory continued to improve.
Elementary and Secondary Education (K-12) Children of unauthorized immigrants are 6.8% of students enrolled in kindergarten through grade 12. That share has grown from 5.4% in 2003. Students with U.S.-born parents account for 78% of school-age children; those with legal immigrant parents account for the remaining 15%.
The economic wellbeing of the millennial generation, which entered its working-age years around the time of the 2007-09 recession, has received considerable attention from economists and the popular press. This chapter compares the socioeconomic and demographic characteristics of millennials with those of earlier
The people's responses toward the prolonged practices of healthcare access are an essential component to the socioeconomic status. It is vital to delineate the social groups as per the requirement of the public health research that can easily formulate the behavioral pattern about the necessities of the access of healthcare physicians.
Policy implications. We find that the economic damages resulting from climate change until 2049 are those to which the world economy is already committed and that these greatly outweigh the costs ...
Founded in 1920, the NBER is a private, non-profit, non-partisan organization dedicated to conducting economic research and to disseminating research findings among academics, public policy makers, and business professionals.
Source: Pew Research Center tabulations of the 2000 census (5% IPUMS) and the 2010 and 2021 American Community Surveys (1% IPUMS). PEW RESEARCH CENTER. ... Economic characteristics of the U.S. Hispanic population, 2021 Among U.S. Hispanics All U.S. born Foreign born; MEDIAN ANNUAL HOUSEHOLD INCOME: $59,000:
With the increase of the requirement for the economy of vehicles and the strengthening of the concept of environmental protection, the development of future vehicles will develop in the direction of high efficiency and cleanliness, and the current power system of vehicles based on traditional fossil fuels will gradually transition to hybrid power. As an essential technological direction for ...
Economic characteristics of electricity. by Devin Hartman. Aug 10, 2016. Download PDFPrint. The physical fundamentals of energy inform the economic fundamentals of electricity. Power plants that generate electricity face both fixed and variable costs. Fixed costs do not vary with the level of output; they primarily include capital costs and ...
Based on advanced adiabatic compressed air energy storage, a combined cooling, heating and power system is constructed. The thermodynamic and economic characteristics under 4 different combinations of different operation conditions and gas storage chambers are compared. The sensitivity analysis of three key parameters is carried out.
Households are classified by the race or detailed Asian group of the head. "Full-time, year-round workers" are defined as people ages 16 and older who usually worked at least 35 hours per week and at least 48 weeks in the past year. The share of the population ages 16 and older who are not employed differs from the unemployment rate because ...
They found that socio-economic characteristics significantly impact shopping cost sensitivity and highlight the potential of online shopping services for some products depending on the relation between the value of time and delivery time savings. Both research efforts utilized integrated choice and latent variable (ICLV) models.
The economy of a developing country may possess characteristics that distinguish it from an industrialized economy, and markets often function differently in developing countries. These ...
At present, capacity configuration optimization research focuses on cost minimization as a single objective, or multi-objectives such as cost, reliability, and carbon emission cost, to configure the capacity of electrolysis and energy storage equipment. ... reflecting the optimal economic characteristics of the system. (2)
We turn to the analysis of the impact of various title characteristics on citations in economic papers. In particular, we examine the effect of the number of words Footnote 1 and the presence of non-alphanumeric characters (nonalpha), while controlling for variables that previous studies found to have an effect on the citation count.These include the length of the article in pages, the number ...
Overall, growth is moderating and is expected to continue to trend downward through 2024. With the third estimate of Real GDP, the Bureau of Economic Analysis also releases the Gross Domestic Income (GDI) data. Real GDI increased 4.8% in Q4 compared to 1.9% in Q3 2023. The average of GDP and GDI is often considered a supplemental measure of U.S ...
Our economic evaluation compared a hypothetical scenario in which the A&F module targeting LVPs is implemented in a Canadian provincial trauma quality assurance program to a status quo scenario in which the A&F module is not implemented. In scenarios analyses we assessed the impact of A&F characteristics on its cost-effectiveness.
The Global Roadmap for an inclusive Circular Economy initiative will host this side event as part of the World Circular Economy Forum (WCEF) 2024. The aim is to provide a comprehensive update on the initiative and advance the dialogue with stakeholders on the pivotal topic of the global circular economy transition and international development.