ENOSUCHBLOG
Programming, philosophy, pedaling., understanding static single assignment forms, oct 23, 2020 tags: llvm , programming .
This post is at least a year old.
With thanks to Niki Carroll , winny, and kurufu for their invaluable proofreading and advice.
By popular demand , I’m doing another LLVM post. This time, it’s single static assignment (or SSA) form, a common feature in the intermediate representations of optimizing compilers.
Like the last one , SSA is a topic in compiler and IR design that I mostly understand but could benefit from some self-guided education on. So here we are.
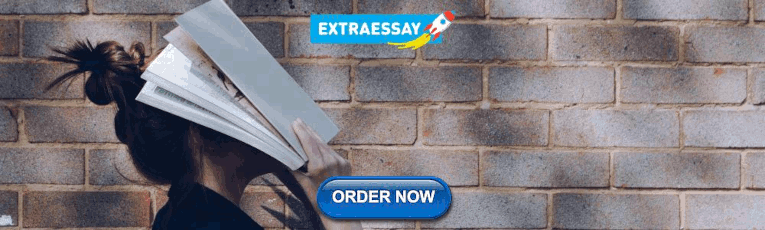
How to represent a program
At the highest level, a compiler’s job is singular: to turn some source language input into some machine language output . Internally, this breaks down into a sequence of clearly delineated 1 tasks:
- Lexing the source into a sequence of tokens
- Parsing the token stream into an abstract syntax tree , or AST 2
- Validating the AST (e.g., ensuring that all uses of identifiers are consistent with the source language’s scoping and definition rules) 3
- Translating the AST into machine code, with all of its complexities (instruction selection, register allocation, frame generation, &c)
In a single-pass compiler, (4) is monolithic: machine code is generated as the compiler walks the AST, with no revisiting of previously generated code. This is extremely fast (in terms of compiler performance) in exchange for some a few significant limitations:
Optimization potential: because machine code is generated in a single pass, it can’t be revisited for optimizations. Single-pass compilers tend to generate extremely slow and conservative machine code.
By way of example: the System V ABI (used by Linux and macOS) defines a special 128-byte region beyond the current stack pointer ( %rsp ) that can be used by leaf functions whose stack frames fit within it. This, in turn, saves a few stack management instructions in the function prologue and epilogue.
A single-pass compiler will struggle to take advantage of this ABI-supplied optimization: it needs to emit a stack slot for each automatic variable as they’re visited, and cannot revisit its function prologue for erasure if all variables fit within the red zone.
Language limitations: single-pass compilers struggle with common language design decisions, like allowing use of identifiers before their declaration or definition. For example, the following is valid C++:
C and C++ generally require pre-declaration and/or definition for identifiers, but member function bodies may reference the entire class scope. This will frustrate a single-pass compiler, which expects Rect::width and Rect::height to already exist in some symbol lookup table for call generation.
Consequently, (virtually) all modern compilers are multi-pass .
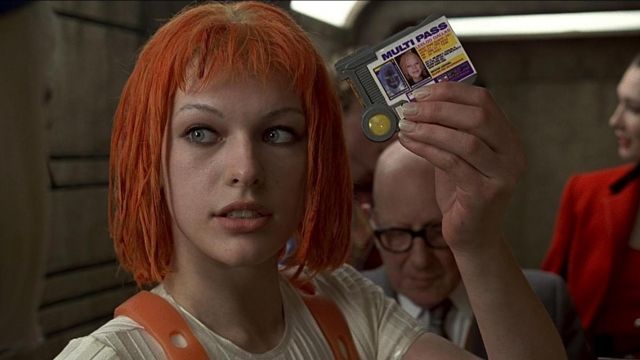
Multi-pass compilers break the translation phase down even more:
- The AST is lowered into an intermediate representation , or IR
- Analyses (or passes) are performed on the IR, refining it according to some optimization profile (code size, performance, &c)
- The IR is either translated to machine code or lowered to another IR, for further target specialization or optimization 4
So, we want an IR that’s easy to correctly transform and that’s amenable to optimization. Let’s talk about why IRs that have the static single assignment property fill that niche.
At its core, the SSA form of any program source program introduces only one new constraint: all variables are assigned (i.e., stored to) exactly once .
By way of example: the following (not actually very helpful) function is not in a valid SSA form with respect to the flags variable:
Why? Because flags is stored to twice: once for initialization, and (potentially) again inside the conditional body.
As programmers, we could rewrite helpful_open to only ever store once to each automatic variable:
But this is clumsy and repetitive: we essentially need to duplicate every chain of uses that follow any variable that is stored to more than once. That’s not great for readability, maintainability, or code size.
So, we do what we always do: make the compiler do the hard work for us. Fortunately there exists a transformation from every valid program into an equivalent SSA form, conditioned on two simple rules.
Rule #1: Whenever we see a store to an already-stored variable, we replace it with a brand new “version” of that variable.
Using rule #1 and the example above, we can rewrite flags using _N suffixes to indicate versions:
But wait a second: we’ve made a mistake!
- open(..., flags_1, ...) is incorrect: it unconditionally assigns O_CREAT , which wasn’t in the original function semantics.
- open(..., flags_0, ...) is also incorrect: it never assigns O_CREAT , and thus is wrong for the same reason.
So, what do we do? We use rule 2!
Rule #2: Whenever we need to choose a variable based on control flow, we use the Phi function (φ) to introduce a new variable based on our choice.
Using our example once more:
Our quandary is resolved: open always takes flags_2 , where flags_2 is a fresh SSA variable produced applying φ to flags_0 and flags_1 .
Observe, too, that φ is a symbolic function: compilers that use SSA forms internally do not emit real φ functions in generated code 5 . φ exists solely to reconcile rule #1 with the existence of control flow.
As such, it’s a little bit silly to talk about SSA forms with C examples (since C and other high-level languages are what we’re translating from in the first place). Let’s dive into how LLVM’s IR actually represents them.
SSA in LLVM
First of all, let’s see what happens when we run our very first helpful_open through clang with no optimizations:
(View it on Godbolt .)
So, we call open with %3 , which comes from…a load from an i32* named %flags ? Where’s the φ?
This is something that consistently slips me up when reading LLVM’s IR: only values , not memory, are in SSA form. Because we’ve compiled with optimizations disabled, %flags is just a stack slot that we can store into as many times as we please, and that’s exactly what LLVM has elected to do above.
As such, LLVM’s SSA-based optimizations aren’t all that useful when passed IR that makes direct use of stack slots. We want to maximize our use of SSA variables, whenever possible, to make future optimization passes as effective as possible.
This is where mem2reg comes in:
This file (optimization pass) promotes memory references to be register references. It promotes alloca instructions which only have loads and stores as uses. An alloca is transformed by using dominator frontiers to place phi nodes, then traversing the function in depth-first order to rewrite loads and stores as appropriate. This is just the standard SSA construction algorithm to construct “pruned” SSA form.
(Parenthetical mine.)
mem2reg gets run at -O1 and higher, so let’s do exactly that:
Foiled again! Our stack slots are gone thanks to mem2reg , but LLVM has actually optimized too far : it figured out that our flags value is wholly dependent on the return value of our access call and erased the conditional entirely.
Instead of a φ node, we got this select :
which the LLVM Language Reference describes concisely:
The ‘select’ instruction is used to choose one value based on a condition, without IR-level branching.
So we need a better example. Let’s do something that LLVM can’t trivially optimize into a select (or sequence of select s), like adding an else if with a function that we’ve only provided the declaration for:
That’s more like it! Here’s our magical φ:
LLVM’s phi is slightly more complicated than the φ(flags_0, flags_1) that I made up before, but not by much: it takes a list of pairs (two, in this case), with each pair containing a possible value and that value’s originating basic block (which, by construction, is always a predecessor block in the context of the φ node).
The Language Reference backs us up:
The type of the incoming values is specified with the first type field. After this, the ‘phi’ instruction takes a list of pairs as arguments, with one pair for each predecessor basic block of the current block. Only values of first class type may be used as the value arguments to the PHI node. Only labels may be used as the label arguments. There must be no non-phi instructions between the start of a basic block and the PHI instructions: i.e. PHI instructions must be first in a basic block.
Observe, too, that LLVM is still being clever: one of our φ choices is a computed select ( %spec.select ), so LLVM still managed to partially erase the original control flow.
So that’s cool. But there’s a piece of control flow that we’ve conspicuously ignored.
What about loops?
Not one, not two, but three φs! In order of appearance:
Because we supply the loop bounds via count , LLVM has no way to ensure that we actually enter the loop body. Consequently, our very first φ selects between the initial %base and %add . LLVM’s phi syntax helpfully tells us that %base comes from the entry block and %add from the loop, just as we expect. I have no idea why LLVM selected such a hideous name for the resulting value ( %base.addr.0.lcssa ).
Our index variable is initialized once and then updated with each for iteration, so it also needs a φ. Our selections here are %inc (which each body computes from %i.07 ) and the 0 literal (i.e., our initialization value).
Finally, the heart of our loop body: we need to get base , where base is either the initial base value ( %base ) or the value computed as part of the prior loop ( %add ). One last φ gets us there.
The rest of the IR is bookkeeping: we need separate SSA variables to compute the addition ( %add ), increment ( %inc ), and exit check ( %exitcond.not ) with each loop iteration.
So now we know what an SSA form is , and how LLVM represents them 6 . Why should we care?
As I briefly alluded to early in the post, it comes down to optimization potential: the SSA forms of programs are particularly suited to a number of effective optimizations.
Let’s go through a select few of them.
Dead code elimination
One of the simplest things that an optimizing compiler can do is remove code that cannot possibly be executed . This makes the resulting binary smaller (and usually faster, since more of it can fit in the instruction cache).
“Dead” code falls into several categories 7 , but a common one is assignments that cannot affect program behavior, like redundant initialization:
Without an SSA form, an optimizing compiler would need to check whether any use of x reaches its original definition ( x = 100 ). Tedious. In SSA form, the impossibility of that is obvious:
And sure enough, LLVM eliminates the initial assignment of 100 entirely:
Constant propagation
Compilers can also optimize a program by substituting uses of a constant variable for the constant value itself. Let’s take a look at another blob of C:
As humans, we can see that y and z are trivially assigned and never modified 8 . For the compiler, however, this is a variant of the reaching definition problem from above: before it can replace y and z with 7 and 10 respectively, it needs to make sure that y and z are never assigned a different value.
Let’s do our SSA reduction:
This is virtually identical to our original form, but with one critical difference: the compiler can now see that every load of y and z is the original assignment. In other words, they’re all safe to replace!
So we’ve gotten rid of a few potential register operations, which is nice. But here’s the really critical part: we’ve set ourselves up for several other optimizations :
Now that we’ve propagated some of our constants, we can do some trivial constant folding : 7 + 10 becomes 17 , and so forth.
In SSA form, it’s trivial to observe that only x and a_{1..4} can affect the program’s behavior. So we can apply our dead code elimination from above and delete y and z entirely!
This is the real magic of an optimizing compiler: each individual optimization is simple and largely independent, but together they produce a virtuous cycle that can be repeated until gains diminish.
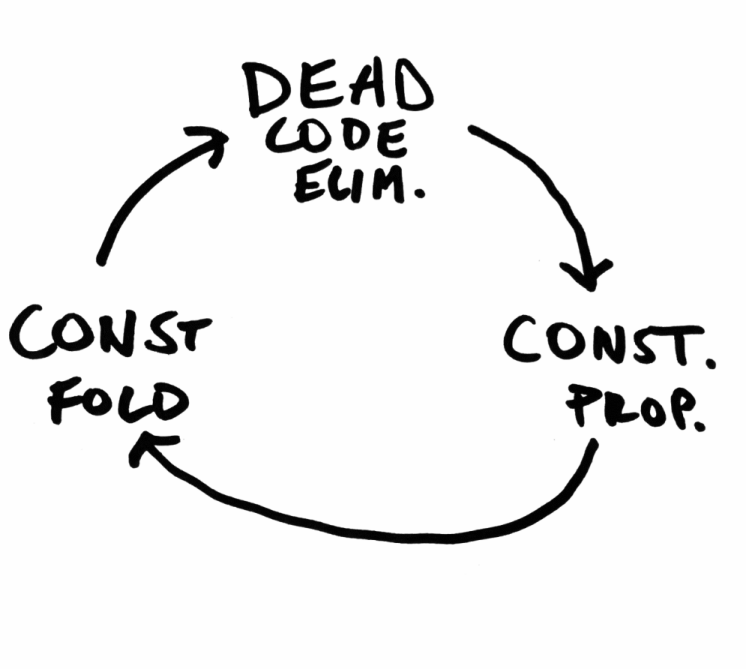
Register allocation
Register allocation (alternatively: register scheduling) is less of an optimization itself , and more of an unavoidable problem in compiler engineering: it’s fun to pretend to have access to an infinite number of addressable variables, but the compiler eventually insists that we boil our operations down to a small, fixed set of CPU registers .
The constraints and complexities of register allocation vary by architecture: x86 (prior to AMD64) is notoriously starved for registers 9 (only 8 full general purpose registers, of which 6 might be usable within a function’s scope 10 ), while RISC architectures typically employ larger numbers of registers to compensate for the lack of register-memory operations.
Just as above, reductions to SSA form have both indirect and direct advantages for the register allocator:
Indirectly: Eliminations of redundant loads and stores reduces the overall pressure on the register allocator, allowing it to avoid expensive spills (i.e., having to temporarily transfer a live register to main memory to accommodate another instruction).
Directly: Compilers have historically lowered φs into copies before register allocation, meaning that register allocators traditionally haven’t benefited from the SSA form itself 11 . There is, however, (semi-)recent research on direct application of SSA forms to both linear and coloring allocators 12 13 .
A concrete example: modern JavaScript engines use JITs to accelerate program evaluation. These JITs frequently use linear register allocators for their acceptable tradeoff between register selection speed (linear, as the name suggests) and acceptable register scheduling. Converting out of SSA form is a timely operation of its own, so linear allocation on the SSA representation itself is appealing in JITs and other contexts where compile time is part of execution time.
There are many things about SSA that I didn’t cover in this post: dominance frontiers , tradeoffs between “pruned” and less optimal SSA forms, and feedback mechanisms between the SSA form of a program and the compiler’s decision to cease optimizing, among others. Each of these could be its own blog post, and maybe will be in the future!
In the sense that each task is conceptually isolated and has well-defined inputs and outputs. Individual compilers have some flexibility with respect to whether they combine or further split the tasks. ↩
The distinction between an AST and an intermediate representation is hazy: Rust converts their AST to HIR early in the compilation process, and languages can be designed to have ASTs that are amendable to analyses that would otherwise be best on an IR. ↩
This can be broken up into lexical validation (e.g. use of an undeclared identifier) and semantic validation (e.g. incorrect initialization of a type). ↩
This is what LLVM does: LLVM IR is lowered to MIR (not to be confused with Rust’s MIR ), which is subsequently lowered to machine code. ↩
Not because they can’t: the SSA form of a program can be executed by evaluating φ with concrete control flow. ↩
We haven’t talked at all about minimal or pruned SSAs, and I don’t plan on doing so in this post. The TL;DR of them: naïve SSA form generation can lead to lots of unnecessary φ nodes, impeding analyses. LLVM (and GCC, and anything else that uses SSAs probably) will attempt to translate any initial SSA form into one with a minimally viable number of φs. For LLVM, this tied directly to the rest of mem2reg . ↩
Including removing code that has undefined behavior in it, since “doesn’t run at all” is a valid consequence of invoking UB. ↩
And are also function scoped, meaning that another translation unit can’t address them. ↩
x86 makes up for this by not being a load-store architecture : many instructions can pay the price of a memory round-trip in exchange for saving a register. ↩
Assuming that %esp and %ebp are being used by the compiler to manage the function’s frame. ↩
LLVM, for example, lowers all φs as one of its very first preparations for register allocation. See this 2009 LLVM Developers’ Meeting talk . ↩
Wimmer 2010a: “Linear Scan Register Allocation on SSA Form” ( PDF ) ↩
Hack 2005: “Towards Register Allocation for Programs in SSA-form” ( PDF ) ↩
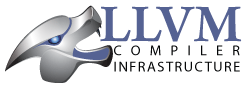
- LLVM Home |
- Documentation »
- LLVM Tutorial: Table of Contents »
3. Kaleidoscope: Code generation to LLVM IR ¶
- Chapter 3 Introduction
- Code Generation Setup
- Expression Code Generation
- Function Code Generation
- Driver Changes and Closing Thoughts
- Full Code Listing
3.1. Chapter 3 Introduction ¶
Welcome to Chapter 3 of the “ Implementing a language with LLVM ” tutorial. This chapter shows you how to transform the Abstract Syntax Tree , built in Chapter 2, into LLVM IR. This will teach you a little bit about how LLVM does things, as well as demonstrate how easy it is to use. It’s much more work to build a lexer and parser than it is to generate LLVM IR code. :)
Please note : the code in this chapter and later require LLVM 2.2 or later. LLVM 2.1 and before will not work with it. Also note that you need to use a version of this tutorial that matches your LLVM release: If you are using an official LLVM release, use the version of the documentation included with your release or on the llvm.org releases page .
3.2. Code Generation Setup ¶
In order to generate LLVM IR, we want some simple setup to get started. First we define virtual code generation (codegen) methods in each AST class:
The Codegen() method says to emit IR for that AST node along with all the things it depends on, and they all return an LLVM Value object. “Value” is the class used to represent a “ Static Single Assignment (SSA) register” or “SSA value” in LLVM. The most distinct aspect of SSA values is that their value is computed as the related instruction executes, and it does not get a new value until (and if) the instruction re-executes. In other words, there is no way to “change” an SSA value. For more information, please read up on Static Single Assignment - the concepts are really quite natural once you grok them.
Note that instead of adding virtual methods to the ExprAST class hierarchy, it could also make sense to use a visitor pattern or some other way to model this. Again, this tutorial won’t dwell on good software engineering practices: for our purposes, adding a virtual method is simplest.
The second thing we want is an “Error” method like we used for the parser, which will be used to report errors found during code generation (for example, use of an undeclared parameter):
The static variables will be used during code generation. TheModule is the LLVM construct that contains all of the functions and global variables in a chunk of code. In many ways, it is the top-level structure that the LLVM IR uses to contain code.
The Builder object is a helper object that makes it easy to generate LLVM instructions. Instances of the `IRBuilder < http://llvm.org/doxygen/IRBuilder_8h-source.html >`_ class template keep track of the current place to insert instructions and has methods to create new instructions.
The NamedValues map keeps track of which values are defined in the current scope and what their LLVM representation is. (In other words, it is a symbol table for the code). In this form of Kaleidoscope, the only things that can be referenced are function parameters. As such, function parameters will be in this map when generating code for their function body.
With these basics in place, we can start talking about how to generate code for each expression. Note that this assumes that the Builder has been set up to generate code into something. For now, we’ll assume that this has already been done, and we’ll just use it to emit code.
3.3. Expression Code Generation ¶
Generating LLVM code for expression nodes is very straightforward: less than 45 lines of commented code for all four of our expression nodes. First we’ll do numeric literals:
In the LLVM IR, numeric constants are represented with the ConstantFP class, which holds the numeric value in an APFloat internally ( APFloat has the capability of holding floating point constants of Arbitrary Precision). This code basically just creates and returns a ConstantFP . Note that in the LLVM IR that constants are all uniqued together and shared. For this reason, the API uses the “foo::get(...)” idiom instead of “new foo(..)” or “foo::Create(..)”.
References to variables are also quite simple using LLVM. In the simple version of Kaleidoscope, we assume that the variable has already been emitted somewhere and its value is available. In practice, the only values that can be in the NamedValues map are function arguments. This code simply checks to see that the specified name is in the map (if not, an unknown variable is being referenced) and returns the value for it. In future chapters, we’ll add support for loop induction variables in the symbol table, and for local variables .
Binary operators start to get more interesting. The basic idea here is that we recursively emit code for the left-hand side of the expression, then the right-hand side, then we compute the result of the binary expression. In this code, we do a simple switch on the opcode to create the right LLVM instruction.
In the example above, the LLVM builder class is starting to show its value. IRBuilder knows where to insert the newly created instruction, all you have to do is specify what instruction to create (e.g. with CreateFAdd ), which operands to use ( L and R here) and optionally provide a name for the generated instruction.
One nice thing about LLVM is that the name is just a hint. For instance, if the code above emits multiple “addtmp” variables, LLVM will automatically provide each one with an increasing, unique numeric suffix. Local value names for instructions are purely optional, but it makes it much easier to read the IR dumps.
LLVM instructions are constrained by strict rules: for example, the Left and Right operators of an add instruction must have the same type, and the result type of the add must match the operand types. Because all values in Kaleidoscope are doubles, this makes for very simple code for add, sub and mul.
On the other hand, LLVM specifies that the fcmp instruction always returns an ‘i1’ value (a one bit integer). The problem with this is that Kaleidoscope wants the value to be a 0.0 or 1.0 value. In order to get these semantics, we combine the fcmp instruction with a uitofp instruction . This instruction converts its input integer into a floating point value by treating the input as an unsigned value. In contrast, if we used the sitofp instruction , the Kaleidoscope ‘<’ operator would return 0.0 and -1.0, depending on the input value.
Code generation for function calls is quite straightforward with LLVM. The code above initially does a function name lookup in the LLVM Module’s symbol table. Recall that the LLVM Module is the container that holds all of the functions we are JIT’ing. By giving each function the same name as what the user specifies, we can use the LLVM symbol table to resolve function names for us.
Once we have the function to call, we recursively codegen each argument that is to be passed in, and create an LLVM call instruction . Note that LLVM uses the native C calling conventions by default, allowing these calls to also call into standard library functions like “sin” and “cos”, with no additional effort.
This wraps up our handling of the four basic expressions that we have so far in Kaleidoscope. Feel free to go in and add some more. For example, by browsing the LLVM language reference you’ll find several other interesting instructions that are really easy to plug into our basic framework.
3.4. Function Code Generation ¶
Code generation for prototypes and functions must handle a number of details, which make their code less beautiful than expression code generation, but allows us to illustrate some important points. First, lets talk about code generation for prototypes: they are used both for function bodies and external function declarations. The code starts with:
This code packs a lot of power into a few lines. Note first that this function returns a “Function*” instead of a “Value*”. Because a “prototype” really talks about the external interface for a function (not the value computed by an expression), it makes sense for it to return the LLVM Function it corresponds to when codegen’d.
The call to FunctionType::get creates the FunctionType that should be used for a given Prototype. Since all function arguments in Kaleidoscope are of type double, the first line creates a vector of “N” LLVM double types. It then uses the Functiontype::get method to create a function type that takes “N” doubles as arguments, returns one double as a result, and that is not vararg (the false parameter indicates this). Note that Types in LLVM are uniqued just like Constants are, so you don’t “new” a type, you “get” it.
The final line above actually creates the function that the prototype will correspond to. This indicates the type, linkage and name to use, as well as which module to insert into. “ external linkage ” means that the function may be defined outside the current module and/or that it is callable by functions outside the module. The Name passed in is the name the user specified: since “ TheModule ” is specified, this name is registered in “ TheModule “s symbol table, which is used by the function call code above.
The Module symbol table works just like the Function symbol table when it comes to name conflicts: if a new function is created with a name that was previously added to the symbol table, the new function will get implicitly renamed when added to the Module. The code above exploits this fact to determine if there was a previous definition of this function.
In Kaleidoscope, I choose to allow redefinitions of functions in two cases: first, we want to allow ‘extern’ing a function more than once, as long as the prototypes for the externs match (since all arguments have the same type, we just have to check that the number of arguments match). Second, we want to allow ‘extern’ing a function and then defining a body for it. This is useful when defining mutually recursive functions.
In order to implement this, the code above first checks to see if there is a collision on the name of the function. If so, it deletes the function we just created (by calling eraseFromParent ) and then calling getFunction to get the existing function with the specified name. Note that many APIs in LLVM have “erase” forms and “remove” forms. The “remove” form unlinks the object from its parent (e.g. a Function from a Module) and returns it. The “erase” form unlinks the object and then deletes it.
In order to verify the logic above, we first check to see if the pre-existing function is “empty”. In this case, empty means that it has no basic blocks in it, which means it has no body. If it has no body, it is a forward declaration. Since we don’t allow anything after a full definition of the function, the code rejects this case. If the previous reference to a function was an ‘extern’, we simply verify that the number of arguments for that definition and this one match up. If not, we emit an error.
The last bit of code for prototypes loops over all of the arguments in the function, setting the name of the LLVM Argument objects to match, and registering the arguments in the NamedValues map for future use by the VariableExprAST AST node. Once this is set up, it returns the Function object to the caller. Note that we don’t check for conflicting argument names here (e.g. “extern foo(a b a)”). Doing so would be very straight-forward with the mechanics we have already used above.
Code generation for function definitions starts out simply enough: we just codegen the prototype (Proto) and verify that it is ok. We then clear out the NamedValues map to make sure that there isn’t anything in it from the last function we compiled. Code generation of the prototype ensures that there is an LLVM Function object that is ready to go for us.
Now we get to the point where the Builder is set up. The first line creates a new basic block (named “entry”), which is inserted into TheFunction . The second line then tells the builder that new instructions should be inserted into the end of the new basic block. Basic blocks in LLVM are an important part of functions that define the Control Flow Graph . Since we don’t have any control flow, our functions will only contain one block at this point. We’ll fix this in Chapter 5 :).
Once the insertion point is set up, we call the CodeGen() method for the root expression of the function. If no error happens, this emits code to compute the expression into the entry block and returns the value that was computed. Assuming no error, we then create an LLVM ret instruction , which completes the function. Once the function is built, we call verifyFunction , which is provided by LLVM. This function does a variety of consistency checks on the generated code, to determine if our compiler is doing everything right. Using this is important: it can catch a lot of bugs. Once the function is finished and validated, we return it.
The only piece left here is handling of the error case. For simplicity, we handle this by merely deleting the function we produced with the eraseFromParent method. This allows the user to redefine a function that they incorrectly typed in before: if we didn’t delete it, it would live in the symbol table, with a body, preventing future redefinition.
This code does have a bug, though. Since the PrototypeAST::Codegen can return a previously defined forward declaration, our code can actually delete a forward declaration. There are a number of ways to fix this bug, see what you can come up with! Here is a testcase:
3.5. Driver Changes and Closing Thoughts ¶
For now, code generation to LLVM doesn’t really get us much, except that we can look at the pretty IR calls. The sample code inserts calls to Codegen into the “ HandleDefinition ”, “ HandleExtern ” etc functions, and then dumps out the LLVM IR. This gives a nice way to look at the LLVM IR for simple functions. For example:
Note how the parser turns the top-level expression into anonymous functions for us. This will be handy when we add JIT support in the next chapter. Also note that the code is very literally transcribed, no optimizations are being performed except simple constant folding done by IRBuilder. We will add optimizations explicitly in the next chapter.
This shows some simple arithmetic. Notice the striking similarity to the LLVM builder calls that we use to create the instructions.
This shows some function calls. Note that this function will take a long time to execute if you call it. In the future we’ll add conditional control flow to actually make recursion useful :).
This shows an extern for the libm “cos” function, and a call to it.
When you quit the current demo, it dumps out the IR for the entire module generated. Here you can see the big picture with all the functions referencing each other.
This wraps up the third chapter of the Kaleidoscope tutorial. Up next, we’ll describe how to add JIT codegen and optimizer support to this so we can actually start running code!
3.6. Full Code Listing ¶
Here is the complete code listing for our running example, enhanced with the LLVM code generator. Because this uses the LLVM libraries, we need to link them in. To do this, we use the llvm-config tool to inform our makefile/command line about which options to use:
Here is the code:
Next: Adding JIT and Optimizer Support
Lesson 5: Global Analysis & SSA
- global analysis & optimization
- static single assignment
- SSA slides from Todd Mowry at CMU another presentation of the pseudocode for various algorithms herein
- Revisiting Out-of-SSA Translation for Correctness, Code Quality, and Efficiency by Boissinot on more sophisticated was to translate out of SSA form
- tasks due October 7
Lots of definitions!
- Reminders: Successors & predecessors. Paths in CFGs.
- A dominates B iff all paths from the entry to B include A .
- The dominator tree is a convenient data structure for storing the dominance relationships in an entire function. The recursive children of a given node in a tree are the nodes that that node dominates.
- A strictly dominates B iff A dominates B and A ≠ B . (Dominance is reflexive, so "strict" dominance just takes that part away.)
- A immediately dominates B iff A dominates B but A does not strictly dominate any other node that strictly dominates B . (In which case A is B 's direct parent in the dominator tree.)
- A dominance frontier is the set of nodes that are just "one edge away" from being dominated by a given node. Put differently, A 's dominance frontier contains B iff A does not strictly dominate B , but A does dominate some predecessor of B .
- Post-dominance is the reverse of dominance. A post-dominates B iff all paths from B to the exit include A . (You can extend the strict version, the immediate version, trees, etc. to post-dominance.)
An algorithm for finding dominators:
The dom relation will, in the end, map each block to its set of dominators. We initialize it as the "complete" relation, i.e., mapping every block to the set of all blocks. The loop pares down the sets by iterating to convergence.
The running time is O(n²) in the worst case. But there's a trick: if you iterate over the CFG in reverse post-order , and the CFG is well behaved (reducible), it runs in linear time—the outer loop runs a constant number of times.
Natural Loops
Some things about loops:
- Natural loops are strongly connected components in the CFG with a single entry.
- Natural loops are formed around backedges , which are edges from A to B where B dominates A .
- A natural loop is the smallest set of vertices L including A and B such that, for every v in L , either all the predecessors of v are in L or v = B .
- A language that only has for , while , if , break , continue , etc. can only generate reducible CFGs. You need goto or something to generate irreducible CFGs.
Loop-Invariant Code Motion (LICM)
And finally, loop-invariant code motion (LICM) is an optimization that works on natural loops. It moves code from inside a loop to before the loop, if the computation always does the same thing on every iteration of the loop.
A loop's preheader is its header's unique predecessor. LICM moves code to the preheader. But while natural loops need to have a unique header, the header does not necessarily have a unique predecessor. So it's often convenient to invent an empty preheader block that jumps directly to the header, and then move all the in-edges to the header to point there instead.
LICM needs two ingredients: identifying loop-invariant instructions in the loop body, and deciding when it's safe to move one from the body to the preheader.
To identify loop-invariant instructions:
(This determination requires that you already calculated reaching definitions! Presumably using data flow.)
It's safe to move a loop-invariant instruction to the preheader iff:
- The definition dominates all of its uses, and
- No other definitions of the same variable exist in the loop, and
- The instruction dominates all loop exits.
The last criterion is somewhat tricky: it ensures that the computation would have been computed eventually anyway, so it's safe to just do it earlier. But it's not true of loops that may execute zero times, which, when you think about it, rules out most for loops! It's possible to relax this condition if:
- The assigned-to variable is dead after the loop, and
- The instruction can't have side effects, including exceptions—generally ruling out division because it might divide by zero. (A thing that you generally need to be careful of in such speculative optimizations that do computations that might not actually be necessary.)
Static Single Assignment (SSA)
You have undoubtedly noticed by now that many of the annoying problems in implementing analyses & optimizations stem from variable name conflicts. Wouldn't it be nice if every assignment in a program used a unique variable name? Of course, people don't write programs that way, so we're out of luck. Right?
Wrong! Many compilers convert programs into static single assignment (SSA) form, which does exactly what it says: it ensures that, globally, every variable has exactly one static assignment location. (Of course, that statement might be executed multiple times, which is why it's not dynamic single assignment.) In Bril terms, we convert a program like this:
Into a program like this, by renaming all the variables:
Of course, things will get a little more complicated when there is control flow. And because real machines are not SSA, using separate variables (i.e., memory locations and registers) for everything is bound to be inefficient. The idea in SSA is to convert general programs into SSA form, do all our optimization there, and then convert back to a standard mutating form before we generate backend code.
Just renaming assignments willy-nilly will quickly run into problems. Consider this program:
If we start renaming all the occurrences of a , everything goes fine until we try to write that last print a . Which "version" of a should it use?
To match the expressiveness of unrestricted programs, SSA adds a new kind of instruction: a ϕ-node . ϕ-nodes are flow-sensitive copy instructions: they get a value from one of several variables, depending on which incoming CFG edge was most recently taken to get to them.
In Bril, a ϕ-node appears as a phi instruction:
The phi instruction chooses between any number of variables, and it picks between them based on labels. If the program most recently executed a basic block with the given label, then the phi instruction takes its value from the corresponding variable.
You can write the above program in SSA like this:
It can also be useful to see how ϕ-nodes crop up in loops.
(An aside: some recent SSA-form IRs, such as MLIR and Swift's IR , use an alternative to ϕ-nodes called basic block arguments . Instead of making ϕ-nodes look like weird instructions, these IRs bake the need for ϕ-like conditional copies into the structure of the CFG. Basic blocks have named parameters, and whenever you jump to a block, you must provide arguments for those parameters. With ϕ-nodes, a basic block enumerates all the possible sources for a given variable, one for each in-edge in the CFG; with basic block arguments, the sources are distributed to the "other end" of the CFG edge. Basic block arguments are a nice alternative for "SSA-native" IRs because they avoid messy problems that arise when needing to treat ϕ-nodes differently from every other kind of instruction.)
Bril in SSA
Bril has an SSA extension . It adds support for a phi instruction. Beyond that, SSA form is just a restriction on the normal expressiveness of Bril—if you solemnly promise never to assign statically to the same variable twice, you are writing "SSA Bril."
The reference interpreter has built-in support for phi , so you can execute your SSA-form Bril programs without fuss.
The SSA Philosophy
In addition to a language form, SSA is also a philosophy! It can fundamentally change the way you think about programs. In the SSA philosophy:
- definitions == variables
- instructions == values
- arguments == data flow graph edges
In LLVM, for example, instructions do not refer to argument variables by name—an argument is a pointer to defining instruction.
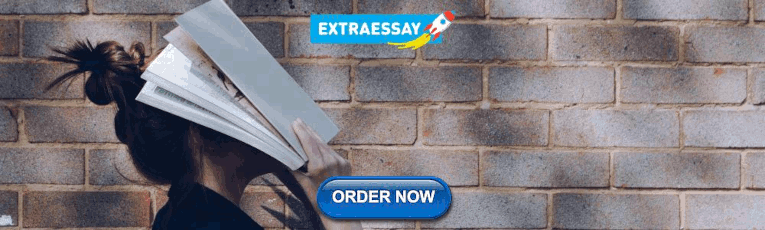
Converting to SSA
To convert to SSA, we want to insert ϕ-nodes whenever there are distinct paths containing distinct definitions of a variable. We don't need ϕ-nodes in places that are dominated by a definition of the variable. So what's a way to know when control reachable from a definition is not dominated by that definition? The dominance frontier!
We do it in two steps. First, insert ϕ-nodes:
Then, rename variables:
Converting from SSA
Eventually, we need to convert out of SSA form to generate efficient code for real machines that don't have phi -nodes and do have finite space for variable storage.
The basic algorithm is pretty straightforward. If you have a ϕ-node:
Then there must be assignments to x and y (recursively) preceding this statement in the CFG. The paths from x to the phi -containing block and from y to the same block must "converge" at that block. So insert code into the phi -containing block's immediate predecessors along each of those two paths: one that does v = id x and one that does v = id y . Then you can delete the phi instruction.
This basic approach can introduce some redundant copying. (Take a look at the code it generates after you implement it!) Non-SSA copy propagation optimization can work well as a post-processing step. For a more extensive take on how to translate out of SSA efficiently, see “Revisiting Out-of-SSA Translation for Correctness, Code Quality, and Efficiency” by Boissinot et al.
- Find dominators for a function.
- Construct the dominance tree.
- Compute the dominance frontier.
- One thing to watch out for: a tricky part of the translation from the pseudocode to the real world is dealing with variables that are undefined along some paths.
- You will want to make sure the output of your "to SSA" pass is actually in SSA form. There's a really simple is_ssa.py script that can check that for you.
- You'll also want to make sure that programs do the same thing when converted to SSA form and back again. Fortunately, brili supports the phi instruction, so you can interpret your SSA-form programs if you want to check the midpoint of that round trip.
- For bonus "points," implement global value numbering for SSA-form Bril code.
Verified Transformation of Continuation-Passing Style into Static Single Assignment Form
- Conference paper
- First Online: 27 June 2023
- Cite this conference paper
- Siyu Liu ORCID: orcid.org/0009-0002-1136-6633 9 &
- Yuting Wang ORCID: orcid.org/0000-0003-3990-2418 9
Part of the book series: Lecture Notes in Computer Science ((LNCS,volume 13931))
Included in the following conference series:
- International Symposium on Theoretical Aspects of Software Engineering
365 Accesses
Static Single Assignment form (SSA) is widely adopted as an intermediate representation (IR) of production compilers as it enables aggressive compiler optimizations based on data-flow analysis. We are concerned with developing verified compilers for functional programming languages that exploit the benefits of SSA. The most obvious approach to achieving our goal is to verify the transformation from Continuation-Passing Style (CPS)—an intermediate representation widely adopted by both production and verified functional compilers—to SSA. In this paper, we show how to verify the translation from CPS to SSA and how to apply the verified translation towards building verified functional compilers. Concretely, we develop and verify a transformation algorithm from PCF programs in CPS to SSA. By extending the transformation with a verified CPS transformation from PCF, we get a verified compilation chain from PCF to SSA. We have also connected this chain with LLVM at the target level to provide a foundation for building more sophisticated verified functional compilers targeting SSA IRs.
This is a preview of subscription content, log in via an institution to check access.
Access this chapter
- Available as PDF
- Read on any device
- Instant download
- Own it forever
- Available as EPUB and PDF
- Compact, lightweight edition
- Dispatched in 3 to 5 business days
- Free shipping worldwide - see info
Tax calculation will be finalised at checkout
Purchases are for personal use only
Institutional subscriptions
The complete artifact in Coq is at https://doi.org/10.5281/zenodo.7882331 .
Appel, A.W.: SSA is functional programming. ACM SIGPLAN Notices 33 (4), 17–20 (1998). https://doi.org/10.1145/278283.278285
Article Google Scholar
Balasubramanian, A., Baranowski, M.S., Burtsev, A., Panda, A., Rakamarić, Z., Ryzhyk, L.: System programming in rust: Beyond safety. In: Proceedings of the 16th Workshop on Hot Topics in Operating Systems, pp. 156–161 (2017). https://doi.org/10.1145/3139645.3139660
Barthe, G., Demange, D., Pichardie, D.: Formal verification of an SSA-based middle-end for CompCert. ACM Trans. Program. Lang. Syst. 36 (1) (mar 2014). https://doi.org/10.1145/2579080
Bélanger, O.S., Monnier, S., Pientka, B.: Programming type-safe transformations using higher-order abstract syntax. In: Gonthier, G., Norrish, M. (eds.) Certified Programs and Proofs - Third International Conference, CPP 2013, Melbourne, VIC, Australia, December 11–13, 2013, Proceedings. Lecture Notes in Computer Science, vol. 8307, pp. 243–258. Springer (2013). https://doi.org/10.1007/978-3-319-03545-1_16
Bélanger, O.S., Weaver, M.Z., Appel, A.W.: Certified code generation from CPS to C. preparation. (2019), https://www.cs.princeton.edu/~appel/papers/CPStoC.pdf
Danvy, O., Millikin, K., Nielsen, L.R.: On one-pass CPS transformations. BRICS Report Series 14 (6) (2007)
Google Scholar
Dargaye, Z.: Vérification formelle d’un compilateur optimisant pour langages fonctionnels. Ph.D. thesis, Université Paris-Diderot-Paris VII (2009)
Demange, D., Pichardie, D., Stefanesco, L.: Verifying fast and sparse SSA-based optimizations in Coq. In: Franke, B. (ed.) Compiler Construction, pp. 233–252. Springer, Berlin Heidelberg, Berlin, Heidelberg (2015). https://doi.org/10.1007/978-3-662-46663-6_12
Dowek, G., Lévy, J.J.: Introduction to the theory of programming languages. Springer Science & Business Media (2010)
Farvardin, K., Reppy, J.: A new backend for Standard ML of New Jersey. In: Proceedings of the 32nd Symposium on Implementation and Application of Functional Languages,pp. 55–66 (2020). https://doi.org/10.1145/3462172.3462191
Herklotz, Y., Demange, D., Blazy, S.: Mechanised semantics for gated static single assignment. In: Krebbers, R., Traytel, D., Pientka, B., Zdancewic, S. (eds.) Proceedings of the 12th ACM SIGPLAN International Conference on Certified Programs and Proofs, CPP 2023, Boston, MA, USA, January 16–17, 2023, pp. 182–196. ACM (2023). https://doi.org/10.1145/3573105.3575681
Kelsey, R.A.: A correspondence between continuation passing style and static single assignment form. ACM SIGPLAN Notices 30 (3), 13–22 (1995). https://doi.org/10.1145/202530.202532
Kennedy, A.: Compiling with continuations, continued. In: Proceedings of the 12th ACM SIGPLAN International Conference on Functional Programming, pp. 177–190 (2007). https://doi.org/10.1145/1291151.1291179
L. Beringer, J.S., Rastello, F.: Static Single Assignment Book. Springer Science & Business Media (2018)
Lattner, C.: Introduction to the LLVM compiler infrastructure. In: Itanium conference and expo (2006)
Leroy, X.: A formally verified compiler back-end. J. Autom. Reason. 43 (4), 363–446 (2009). https://doi.org/10.1007/s10817-009-9155-4
Article MathSciNet MATH Google Scholar
Paraskevopoulou, Z., Appel, A.W.: Closure conversion is safe for space. Proceedings of the ACM on Programming Languages 3(ICFP), pp. 1–29 (2019). https://doi.org/10.1145/3341687
Paraskevopoulou, Z., Grover, A.: Compiling with continuations, correctly. Proc. ACM Program. Lang. 5 (OOPSLA) (oct 2021). https://doi.org/10.1145/3485491
Paraskevopoulou, Z., Li, J.M., Appel, A.W.: Compositional optimizations for CertiCoq. Proc. ACM Program. Lang. 5 (ICFP) (aug 2021). https://doi.org/10.1145/3473591
Plotkin, G.D.: Call-by-name, call-by-value and the \(\lambda \) -calculus. Theoret. Comput. Sci. 1 (2), 125–159 (1975). https://doi.org/10.1016/0304-3975(75)90017-1
Plotkin, G.D.: LCF considered as a programming language. Theoret. Comput. Sci. 5 (3), 223–255 (1977)
Smith, J.B.: Ocamllex and Ocamlyacc. Practical OCaml, pp. 193–211 (2007)
Wang, Y., Nadathur, G.: A higher-order abstract syntax approach to verified transformations on functional programs. In: Thiemann, P. (ed.) Programming Languages and Systems, pp. 752–779. Springer, Berlin Heidelberg, Berlin, Heidelberg (2016). https://doi.org/10.1007/978-3-662-49498-1_29
Zakowski, Y., Beck, C., Yoon, I., Zaichuk, I., Zaliva, V., Zdancewic, S.: Modular, compositional, and executable formal semantics for LLVM IR. Proceedings of the ACM on Programming Languages 5 (ICFP), 1–30 (2021). https://doi.org/10.1145/3473572
Zhang, Y., Yang, M., Zhou, B., Yang, Z., Zhang, W., Zang, B.: Swift: a register-based JIT compiler for embedded JVMs. In: Proceedings of the 8th ACM SIGPLAN/SIGOPS conference on Virtual Execution Environments, pp. 63–74 (2012). https://doi.org/10.1145/2365864.2151035
Zhao, J., Nagarakatte, S., Martin, M.M., Zdancewic, S.: Formalizing the LLVM intermediate representation for verified program transformations. In: Proceedings of the 39th annual ACM SIGPLAN-SIGACT symposium on Principles of programming languages, pp. 427–440 (2012). https://doi.org/10.1145/2103621.2103709
Download references
Acknowledgments
We would like to thank the anonymous referees for their helpful feedback which improved this paper significantly. This work was supported by the National Natural Science Foundation of China (NSFC) under Grant No. 62002217.
Author information
Authors and affiliations.
John Hopcroft Center for Computer Science, School of Electronic Information and Electrical Engineering, Shanghai Jiao Tong University, Shanghai, 200240, China
Siyu Liu & Yuting Wang
You can also search for this author in PubMed Google Scholar
Corresponding author
Correspondence to Yuting Wang .
Editor information
Editors and affiliations.
University of Bristol, Bristol, UK
Cristina David
Peking University, Beijing, China
Rights and permissions
Reprints and permissions
Copyright information
© 2023 The Author(s), under exclusive license to Springer Nature Switzerland AG
About this paper
Cite this paper.
Liu, S., Wang, Y. (2023). Verified Transformation of Continuation-Passing Style into Static Single Assignment Form. In: David, C., Sun, M. (eds) Theoretical Aspects of Software Engineering. TASE 2023. Lecture Notes in Computer Science, vol 13931. Springer, Cham. https://doi.org/10.1007/978-3-031-35257-7_2
Download citation
DOI : https://doi.org/10.1007/978-3-031-35257-7_2
Published : 27 June 2023
Publisher Name : Springer, Cham
Print ISBN : 978-3-031-35256-0
Online ISBN : 978-3-031-35257-7
eBook Packages : Computer Science Computer Science (R0)
Share this paper
Anyone you share the following link with will be able to read this content:
Sorry, a shareable link is not currently available for this article.
Provided by the Springer Nature SharedIt content-sharing initiative
- Publish with us
Policies and ethics
- Find a journal
- Track your research
Navigation Menu
Search code, repositories, users, issues, pull requests..., provide feedback.
We read every piece of feedback, and take your input very seriously.
Saved searches
Use saved searches to filter your results more quickly.
To see all available qualifiers, see our documentation .
- Notifications
Have a question about this project? Sign up for a free GitHub account to open an issue and contact its maintainers and the community.
By clicking “Sign up for GitHub”, you agree to our terms of service and privacy statement . We’ll occasionally send you account related emails.
Already on GitHub? Sign in to your account
Single Static Assignment - MLIR and SMTLIB. #1
chadbrewbaker commented Dec 18, 2023
statusfailed commented Dec 19, 2023
Sorry, something went wrong.
No branches or pull requests
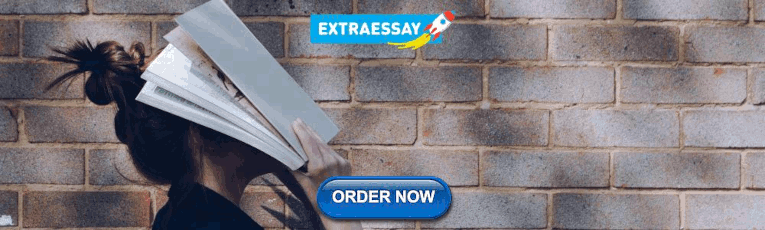
COMMENTS
In compiler design, static single assignment form (often abbreviated as SSA form or simply SSA) is a property of an intermediate representation ... The LLVM Compiler Infrastructure uses SSA form for all scalar register values (everything except memory) in its primary code representation. SSA form is only eliminated once register allocation ...
By popular demand, I'm doing another LLVM post. This time, it's single static assignment (or SSA) form, a common feature in the intermediate representations of optimizing compilers. Like the last one, SSA is a topic in compiler and IR design that I mostly understand but could benefit from some self-guided education on.
•Static Single Assignment (SSA) •CFGs but with immutable variables •Plus a slight "hack" to make graphs work out •Now widely used (e.g., LLVM) •Intra-procedural representation only •An SSA representation for whole program is possible (i.e., each global variable and memory location has static single
LLVM is a Static Single Assignment (SSA) based representation that provides type safety, low-level operations, flexibility, and the capability of representing 'all' high-level languages cleanly. It is the common code representation used throughout all phases of the LLVM compilation strategy.
In LLVM you have to manually specify the name of the value and the previous basic block. end: %retval = phi i32 [%a, %btrue], [%b, %bfalse] Here we instruct the phi instruction to choose %a if the previous basic block was %btrue. If the previous basic block was %bfalse, then %b will be used. The value is then assigned to a new variable %retval.
SSA Form. SSA form. Static single-assignment form arranges for every value computed by a program to have a unique assignment (aka, "definition") A procedure is in SSA form if every variable has (statically) exactly one definition SSA form simplifies several important optimizations, including various forms of redundancy elimination. Example.
Since SSA has two requirements: Every variable is assigned exactly once Every variable is defined before it is used Phi function of a loop may be like this: %indvars.iv = phi i64 [ 0, %entry ], [ %indvars.iv.next, %for.body ] In this phi function, %indvars.iv.next appears before it is generated. Does it violate SSA form? In my opinion, it only appears before it is defined, but it is not used ...
"Value" is the class used to represent a "Static Single Assignment (SSA) register" or "SSA value" in LLVM. The most distinct aspect of SSA values is that their value is computed as the related instruction executes, and it does not get a new value until (and if) the instruction re-executes.
Many compilers convert programs into static single assignment (SSA) form, which does exactly what it says: it ensures that, globally, every variable has exactly one static assignment location. (Of course, that statement might be executed multiple times, ... In LLVM, for example, ...
Keywords LLVM, Coq, single static assignment 1. Introduction Compiler bugs can manifest as crashes during compilation, or, much worse, result in the silent generation of incorrect programs. Such mis-compilations can introduce subtle errors that are difficult to diagnose and generally puzzling to software developers. A re-
LLVM uses static single assignment (SSA) form for its IR, meaning that every Value has a single definition point. So what is the easiest (and most generic) way to find "the" definition point of a Value without having to examine each use and determine how our Value is being used? In the code below, I am interested in definition points of Values used as function arguments.
- Must satisfy the single static assignment invariant • Each %uid appears on the left-hand side of an assignment only once in the entire control flow graph. - %The value of a uid remains unchanged throughout its lifetime - Analogous to "let %uid = e in …" in OCaml • Intended to be an abstract version of machine registers.
3.4K subscribers in the LLVM community. A place to discuss or ask questions relating to the LLVM Compiler Infrastructure. Skip to main content. ... Hacker News: Understanding static single assignment forms blog. upvote r/ProgrammingLanguages. r/ProgrammingLanguages. This subreddit is dedicated to the theory, design and implementation of ...
Abstract. Modern compilers, such as LLVM and GCC, use a static single assignment (SSA) intermediate representation (IR) to simplify and enable many advanced optimizations. However, formally verifying the correctness of SSA-based optimizations is challenging because SSA properties depend on a function's entire control-flow graph.
Static Single Assignment form (SSA) is widely adopted as an intermediate representation (IR) of production compilers as it enables aggressive compiler optimizations based on data-flow analysis. ... LLVM and GCC) which adopt Static Single Assignment form (SSA) as their IR. SSA becomes prevalent in compiler infrastructures as it allows for ...
static single assignment in clang. Clang Frontend. Mohammad_Adil November 15, 2012, 7:45pm 1. Hi, During which part of compilation does clang generate the SSA form? ... During lowering to LLVM IR. (well, I suspect Clang emits lots of things/everything as memory operands & then LLVM's mem2reg cleans it all up into more SSA variable usage)
The Gated Static Single Assignment (GSA) form was proposed by Ottenstein et al. in 1990, as an intermediate representation for implementing advanced static analyses and optimisation passes in compilers. ... Evaluating Value-Graph Translation Validation for LLVM. In Proceedings of the 32nd ACM SIGPLAN Conference on Programming Language Design ...
chadbrewbaker commented on Dec 18, 2023. Any way you could output in Single Static Assignment for LLVM/MLIR and SSA SMTLIB lisp for solvers like Microsoft Z3/CVC5? You could then shell out to LLVM and Z3/CVC5 to make optimization passes, and give you an easy compile target for WASM with a little IO boilerplate.
The first llvm.dbg.assign refers to the alloca through !DIAssignID!13, and the second refers to the store through !DIAssignID!16.. Store-like instructions¶. In the absence of a linked llvm.dbg.assign, a store to an address that is known to be the backing storage for a variable is considered to represent an assignment to that variable.. This gives us a safe fall-back in cases where llvm.dbg ...
An if-then-else therefore involves several blocks: Optionally, the block after the then and else blocks (if then and else don't return or branch elsewhere). To insert an if-then-else, the original Basic Block must be split into (1) and (4). The condition checking and conditional branching go into (1), and (2) and (3) finish with a branch to (4).
Clang Static Analyzer has two separate documentation folders: clang/docs/analyzer and clang/www/analyzer and there is some overlap between their contents. For example, the checkers are listed in both folders: checkers.rst in clang/docs/analyzer available_checks.html and alpha_checks.html in clang/www/analyzer …with the very important difference that the lists in clang/www/analyzer are ...