If you're seeing this message, it means we're having trouble loading external resources on our website.
If you're behind a web filter, please make sure that the domains *.kastatic.org and *.kasandbox.org are unblocked.
To log in and use all the features of Khan Academy, please enable JavaScript in your browser.
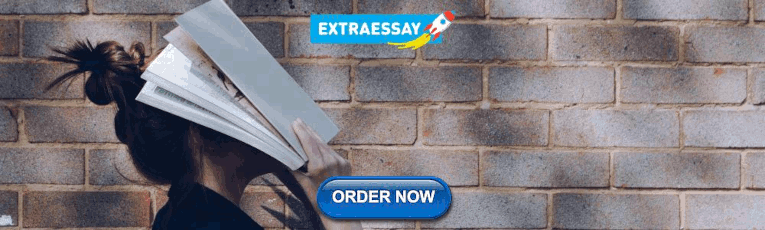
AP®︎/College Biology
Course: ap®︎/college biology > unit 7, evidence for evolution.
- Organelles in eukaryotic cells
- Intro to eukaryotic cells
- Cellular evidence of common ancestry
- Evolution of viruses
- Common ancestry and continuing evolution
Key points:
- Evidence for large-scale evolution ( macroevolution ) comes from anatomy and embryology, molecular biology, biogeography, and fossils.
- Similar anatomy found in different species may be homologous (shared due to ancestry) or analogous (shared due to similar selective pressures).
- Molecular similarities provide evidence for the shared ancestry of life. DNA sequence comparisons can show how different species are related.
- Biogeography , the study of the geographical distribution of organisms, provides information about how and when species may have evolved.
- Fossils provide evidence of long-term evolutionary changes, documenting the past existence of species that are now extinct.
Introduction
Evidence for evolution: tracing evolutionary histories.
- Anatomy and embryology. Anatomical features shared between organisms (including ones that are visible only during embryonic development) can indicate a shared evolutionary ancestry.
- Molecular biology. Similarities and differences between the "same" gene in different organisms (that is, a pair of homologous genes) can help us determine how closely related the organisms are.
- Biogeography. The geographical distribution of species can help us reconstruct their evolutionary histories.
- Fossils. The fossil record is not a complete record of evolutionary history, but it confirms the existence of now-extinct species and sometimes captures potential "in-between" forms on the path to present-day species.
Evidence for evolution: Anatomy and embryology
Homologous features, analogous features, determining relationships from similar features, evidence for evolution: molecular biology.
- The same genetic material (DNA)
- The same, or highly similar, genetic codes
- The same basic process of gene expression (transcription and translation)
Homologous genes
Evidence for evolution: biogeography, evidence for evolution: fossil record.
- Homologous physical features shared between species can provide evidence for common ancestry (but we have to be sure they are really homologous, and not the result of convergent evolution).
- Similarities and differences among biological molecules (e.g., in the DNA sequence of genes) can be used to determine species' relatedness.
- Biogeographical patterns provide clues about how species, both alive and extinct, are related to each other.
- The fossil record, though incomplete, provides valuable information about what species existed at particular times in Earth’s history.
Works cited:
- http://www.ck12.org/book/CK-12-Biology-Advanced-Concepts/section/10.22/
- Campbell, legs of snakes.
- Reece, J. B., Urry, L. A., Cain, M. L., Wasserman, S. A., Minorsky, P. V., and Jackson, R. B. (2011). Evolution is supported by an overwhelming amount of scientific evidence. In Campbell biology (10th ed., pp. 471-479). San Francisco, CA: Pearson.
Want to join the conversation?
- Upvote Button navigates to signup page
- Downvote Button navigates to signup page
- Flag Button navigates to signup page
- Translators
- Graphic Designers
Please enter the email address you used for your account. Your sign in information will be sent to your email address after it has been verified.
How To Write a Strong Research Hypothesis
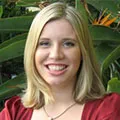
Are you looking to take your research project to the next level? Have you heard of the power of a hypothesis but need to figure out how to formulate one that will unlock potential discoveries? We can help!
So get ready; it's time to dive into unlocking the power of research! This blog post will explore what makes a well-crafted and powerful hypothesis - from identifying a research question to developing supporting evidence.
By learning how to craft a compelling hypothesis, you'll have more tremendous success in every step of your research project.
What are hypotheses, and why are they important?
A hypothesis is an educated guess or a proposition based on limited evidence as a starting point for further investigation. It provides a framework for research and allows researchers to refine their ideas, collect data, and draw conclusions. Hypotheses are essential to the process because they will enable us to organize our thoughts and test theories properly.
Hypotheses are used in many fields , from medicine to psychology to economics. In each area, developing hypotheses based on observations enable researchers to make predictions about their data and guide them toward finding meaningful results.
For example, in medicine, hypotheses can be used to predict which treatments will be most effective for particular conditions or which drugs may have adverse effects when taken together. This allows doctors to make better decisions when caring for patients.
In psychology, hypotheses are often used in experiments to determine whether certain variables influence behavior or mental processes. By testing different combinations of variables, psychologists can identify patterns and understand why people behave the way they do.
In economics, hypotheses provide economists with a framework for analyzing the relationship between economic variables such as wages and consumer spending habits. By understanding these relationships, economists can better understand how economic forces affect the economy.
Overall, hypotheses play an essential role in helping scientists develop new ideas and draw meaningful conclusions from the collected data. Without taking the step to create hypotheses, it would be difficult for researchers to make sense of the vast amounts of information available today and use it effectively in their investigations.
How to determine an effective research question to form your hypothesis
When conducting research, having a compelling research question is critical . Properly formulating this question will allow the researcher to develop their hypothesis. A research question provides a clear and focused goal for your research study and also gives direction on how to get there. A compelling research question should be specific, answerable in the context of your field of study, significant, novel (not already answered by previous studies), and timely – that is, relevant to current events or trends.
Before determining the best research question, you must first understand your topic. Think about the area of knowledge that interests you most and narrow it down to a single theme or concept within this topic. Focus on what interests you most within this theme, and make sure there is room for further exploration and analysis. Once you have chosen a specific topic and narrowed down your focus, you can begin formulating questions related to your project.
To ensure relevance and impact to your field of study, choose questions that address essential issues in the literature or suggest solutions to existing problems. Avoid overly broad topics with unclear objectives; instead, opt for focused questions to enable targeted data collection and analysis with concrete results.
Additionally, consider time frames when formulating questions. If the issue has been discussed extensively in the past but has not been revisited recently, then it's likely not worthy of a new investigation.
Once you have developed some potential questions related to your topic, review them carefully and decide which question best captures the essence of what you want to learn through researching this topic.
Ask yourself:
- Is this question answerable?
- Does it fit within my field of study?
- Is it significant enough?
- Would its findings be novel?
If so, then congratulations! You have identified a compelling research question.
Tips for crafting a well-crafted hypothesis
Once you have formulated the official research question, you may develop the formal hypothesis. When composing a hypothesis, it's essential to think carefully about the question you are trying to answer.
A solid hypothesis should be testable, meaning that it can be verified or disproved through research. It should also be specific and focused on one issue at a time. Here are some tips for crafting a well-crafted hypothesis:
- Consider the goal of your research: Think about what it is that you want to learn or determine from your experiment and make sure that your hypothesis reflects this goal.
- Create an educated guess as to why something is happening: Your hypothesis should explain why something is occurring based on what evidence you already have and direct further investigation into the matter. For example, if you hypothesize that increased carbon dioxide levels in the atmosphere will lead to global warming, your research should focus on examining this relationship further.
- Define any variables or parameters involved in the experiment: This includes things like temperature or chemical composition that could potentially affect the outcome of any experiments done in pursuit of testing your hypothesis.
- Use clear and precise language: Make sure your hypothesis is written with clear and precise language so that anyone reading it can understand exactly what you are attempting to investigate or explain. Avoid complex words and keep sentences short whenever possible.
Following these simple tips will help ensure that your hypothesis is well-crafted and ready for testing!
Examples of evidence that can support your hypothesis
When it comes to developing a hypothesis, supporting evidence is essential for making sure it holds up. This evidence helps strengthen the argument that is being driven by providing facts and logical reasoning that support the hypothesis.
Examples of evidence that can be used to back up a hypothesis include using data from experiments, case studies, and other research projects. Data from experiments can provide insight into how certain variables interact to form a particular outcome.
Case studies may offer greater depth in understanding a specific phenomenon's cause and effect; research projects may yield results that confirm or refute existing theories on a subject.
In addition to these traditional forms of evidence, personal experiences or observations can also help to support a hypothesis. For example, if someone's daily commute has been consistently faster since they changed routes, they could use their personal experience to argue that making this change resulted in shorter commutes.
Similarly, suppose someone has witnessed how two variables consistently coincide (i.e., when one goes up, another goes down). In that case, this could be used to support the notion that there is some correlation between these two aspects.
Overall, evidence to support your hypothesis is crucial for ensuring its validity and credibility. While conducting experiments or researching may seem like time-consuming processes, having solid supporting evidence will make it much easier to defend your ideas convincingly when challenged.
Therefore, it is crucial to take the time necessary to gather credible sources of information to provide the most substantial possible backing for your hypotheses.
Understanding the potential of hypotheses and how they can help your research project progress
The power of research lies in the ability to develop and test hypotheses. A hypothesis is a statement or an idea that can be tested to determine its validity.
Essentially, it is a form of educated guesswork that helps researchers form conclusions about their data. By developing a hypothesis for a research project, you are effectively setting up the framework for further exploration.
When developing a hypothesis, you must consider both the expected outcomes and possible alternative explanations. This will help you focus on testing the possible results without getting sidetracked by irrelevant information. Once you have established a concrete hypothesis, it can then be used as a basis for further research and experimentation.
The process of testing hypotheses is an integral part of the scientific method and can help researchers build confidence in their findings and conclusions. Through careful observation and experimentation, researchers can compare their results against what they initially hypothesized, allowing them to draw more accurate conclusions about their data. As such, hypotheses play an essential role in helping researchers connect the dots between different pieces of evidence and form meaningful conclusions.
Overall, understanding how hypotheses can be used in research projects can be immensely beneficial in helping progress towards reaching meaningful insights from their data. By setting up expectations ahead of time and then testing them against real-world conditions, researchers can gain valuable insights that could potentially change the way we understand our world – now that's something worth exploring!
Final thoughts
A hypothesis is a proposed explanation for an observable phenomenon. It's important to note that hypotheses are not the same thing as theories–a theory is a much broader and well-established frame of reference that explains multiple phenomena.
Generally, scientists form a research question and then narrow it down to a testable hypothesis. After making observations and conducting experiments to gather data, researchers can use evidence to support or reject the hypothesis.
By following these steps to formulate a solid hypothesis, you will be on your way to developing a successful research project. Happy researching!
Header image by Bnenin .
Related Posts
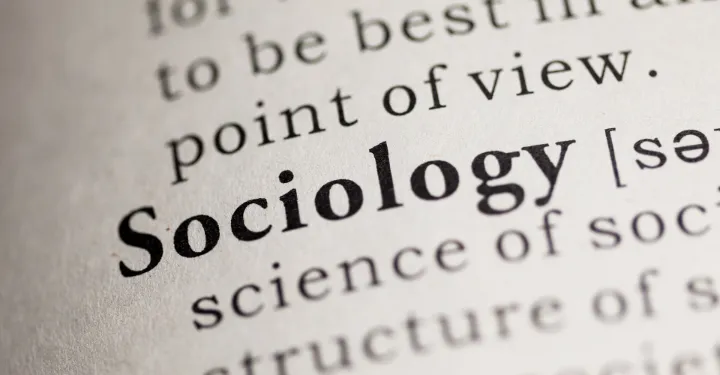
100 Sociology Research Topics You Can Use Right Now
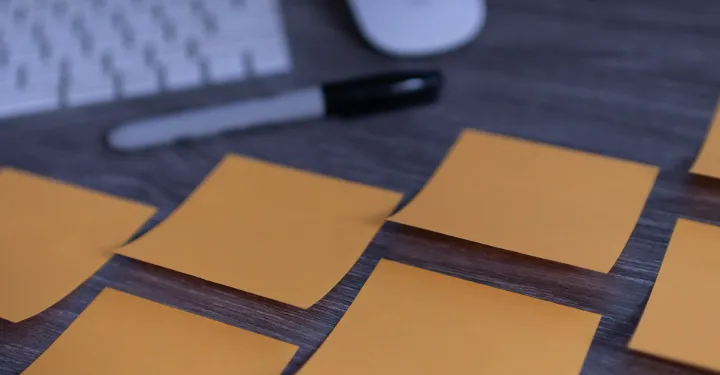
How to Write a Thematic Analysis
- Academic Writing Advice
- All Blog Posts
- Writing Advice
- Admissions Writing Advice
- Book Writing Advice
- Short Story Advice
- Employment Writing Advice
- Business Writing Advice
- Web Content Advice
- Article Writing Advice
- Magazine Writing Advice
- Grammar Advice
- Dialect Advice
- Editing Advice
- Freelance Advice
- Legal Writing Advice
- Poetry Advice
- Graphic Design Advice
- Logo Design Advice
- Translation Advice
- Blog Reviews
- Short Story Award Winners
- Scholarship Winners
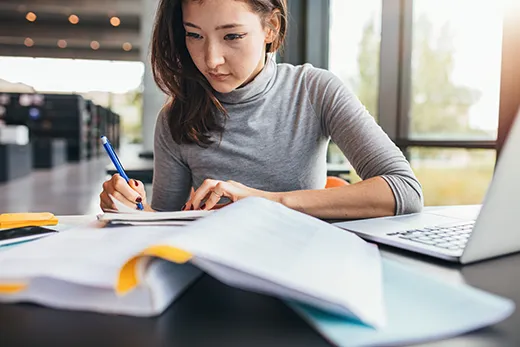
Need an academic editor before submitting your work?
- Skip to primary navigation
- Skip to main content
- Skip to primary sidebar
- Skip to footer
- Image & Use Policy
- Translations
UC MUSEUM OF PALEONTOLOGY
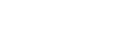
Understanding Evolution
Your one-stop source for information on evolution
Endosymbiosis
Evidence for endosymbiosis.
Biologist Lynn Margulis first made the case for endosymbiosis in the 1960s, but for many years other biologists were skeptical. Although Jeon watched his amoebae become infected with the x-bacteria and then evolve to depend upon them, no one was around over a billion years ago to observe the events of endosymbiosis. Why should we think that a mitochondrion used to be a free-living organism in its own right? It turns out that many lines of evidence support this idea. Most important are the many striking similarities between prokaryotes (like bacteria) and mitochondria:
- Membranes — Mitochondria have their own cell membranes, just like a prokaryotic cell does.
When you look at it this way, mitochondria really resemble tiny bacteria making their livings inside eukaryotic cells! Based on decades of accumulated evidence, the scientific community supports Margulis’s ideas: endosymbiosis is the best explanation for the evolution of the eukaryotic cell.
What’s more, the evidence for endosymbiosis applies not only to mitochondria, but to other cellular organelles as well. Chloroplasts are like tiny green factories within plant cells that help convert energy from sunlight into sugars, and they have many similarities to mitochondria. The evidence suggests that these chloroplast organelles were also once free-living bacteria.
The endosymbiotic event that generated mitochondria must have happened early in the history of eukaryotes, because all eukaryotes have them. Then, later, a similar event brought chloroplasts into some eukaryotic cells, creating the lineage that led to plants.
Despite their many similarities, mitochondria (and chloroplasts) aren’t free-living bacteria anymore. The first eukaryotic cell evolved more than a billion years ago. Since then, these organelles have become completely dependent on their host cells. For example, many of the key proteins needed by the mitochondrion are imported from the rest of the cell. Sometime during their long-standing relationship, the genes that code for these proteins were transferred from the mitochondrion to its host’s genome. Scientists consider this mixing of genomes to be the irreversible step at which the two independent organisms become a single individual.
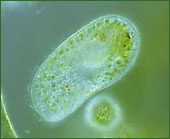
Paramecium bursaria , a single-celled eukaryote that swims around in pond water, may not have its own chloroplasts, but it does manage to “borrow” them in a rather unusual way. P. bursaria swallows photosynthetic green algae, but it stores them instead of digesting them. In fact, the normally clear paramecium can pack so many algae into its body that it even looks green! When P. bursaria swims into the light, the algae photosynthesize sugar, and both cells share lunch on the go. But P. bursaria doesn’t exploit its algae. Not only does the agile paramecium chauffeur its algae into well-lit areas, it also shares the food it finds with its algae if they are forced to live in the dark.
From prokaryotes to eukaryotes
Finding our roots
Subscribe to our newsletter
- Teaching resource database
- Correcting misconceptions
- Conceptual framework and NGSS alignment
- Image and use policy
- Evo in the News
- The Tree Room
- Browse learning resources
P-Value And Statistical Significance: What It Is & Why It Matters
Saul Mcleod, PhD
Editor-in-Chief for Simply Psychology
BSc (Hons) Psychology, MRes, PhD, University of Manchester
Saul Mcleod, PhD., is a qualified psychology teacher with over 18 years of experience in further and higher education. He has been published in peer-reviewed journals, including the Journal of Clinical Psychology.
Learn about our Editorial Process
Olivia Guy-Evans, MSc
Associate Editor for Simply Psychology
BSc (Hons) Psychology, MSc Psychology of Education
Olivia Guy-Evans is a writer and associate editor for Simply Psychology. She has previously worked in healthcare and educational sectors.
On This Page:
The p-value in statistics quantifies the evidence against a null hypothesis. A low p-value suggests data is inconsistent with the null, potentially favoring an alternative hypothesis. Common significance thresholds are 0.05 or 0.01.
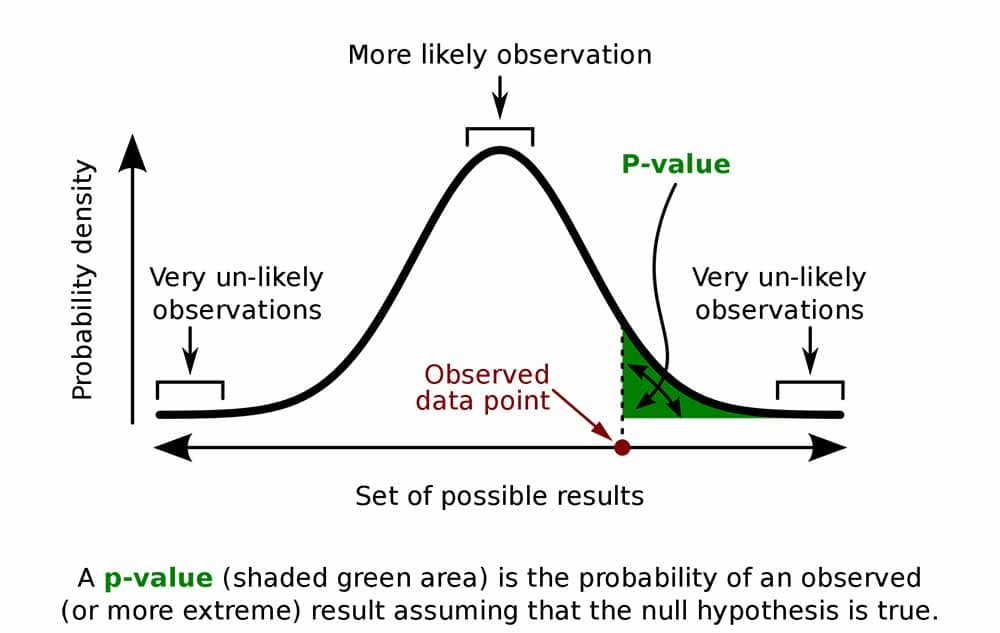
Hypothesis testing
When you perform a statistical test, a p-value helps you determine the significance of your results in relation to the null hypothesis.
The null hypothesis (H0) states no relationship exists between the two variables being studied (one variable does not affect the other). It states the results are due to chance and are not significant in supporting the idea being investigated. Thus, the null hypothesis assumes that whatever you try to prove did not happen.
The alternative hypothesis (Ha or H1) is the one you would believe if the null hypothesis is concluded to be untrue.
The alternative hypothesis states that the independent variable affected the dependent variable, and the results are significant in supporting the theory being investigated (i.e., the results are not due to random chance).
What a p-value tells you
A p-value, or probability value, is a number describing how likely it is that your data would have occurred by random chance (i.e., that the null hypothesis is true).
The level of statistical significance is often expressed as a p-value between 0 and 1.
The smaller the p -value, the less likely the results occurred by random chance, and the stronger the evidence that you should reject the null hypothesis.
Remember, a p-value doesn’t tell you if the null hypothesis is true or false. It just tells you how likely you’d see the data you observed (or more extreme data) if the null hypothesis was true. It’s a piece of evidence, not a definitive proof.
Example: Test Statistic and p-Value
Suppose you’re conducting a study to determine whether a new drug has an effect on pain relief compared to a placebo. If the new drug has no impact, your test statistic will be close to the one predicted by the null hypothesis (no difference between the drug and placebo groups), and the resulting p-value will be close to 1. It may not be precisely 1 because real-world variations may exist. Conversely, if the new drug indeed reduces pain significantly, your test statistic will diverge further from what’s expected under the null hypothesis, and the p-value will decrease. The p-value will never reach zero because there’s always a slim possibility, though highly improbable, that the observed results occurred by random chance.
P-value interpretation
The significance level (alpha) is a set probability threshold (often 0.05), while the p-value is the probability you calculate based on your study or analysis.
A p-value less than or equal to your significance level (typically ≤ 0.05) is statistically significant.
A p-value less than or equal to a predetermined significance level (often 0.05 or 0.01) indicates a statistically significant result, meaning the observed data provide strong evidence against the null hypothesis.
This suggests the effect under study likely represents a real relationship rather than just random chance.
For instance, if you set α = 0.05, you would reject the null hypothesis if your p -value ≤ 0.05.
It indicates strong evidence against the null hypothesis, as there is less than a 5% probability the null is correct (and the results are random).
Therefore, we reject the null hypothesis and accept the alternative hypothesis.
Example: Statistical Significance
Upon analyzing the pain relief effects of the new drug compared to the placebo, the computed p-value is less than 0.01, which falls well below the predetermined alpha value of 0.05. Consequently, you conclude that there is a statistically significant difference in pain relief between the new drug and the placebo.
What does a p-value of 0.001 mean?
A p-value of 0.001 is highly statistically significant beyond the commonly used 0.05 threshold. It indicates strong evidence of a real effect or difference, rather than just random variation.
Specifically, a p-value of 0.001 means there is only a 0.1% chance of obtaining a result at least as extreme as the one observed, assuming the null hypothesis is correct.
Such a small p-value provides strong evidence against the null hypothesis, leading to rejecting the null in favor of the alternative hypothesis.
A p-value more than the significance level (typically p > 0.05) is not statistically significant and indicates strong evidence for the null hypothesis.
This means we retain the null hypothesis and reject the alternative hypothesis. You should note that you cannot accept the null hypothesis; we can only reject it or fail to reject it.
Note : when the p-value is above your threshold of significance, it does not mean that there is a 95% probability that the alternative hypothesis is true.
One-Tailed Test
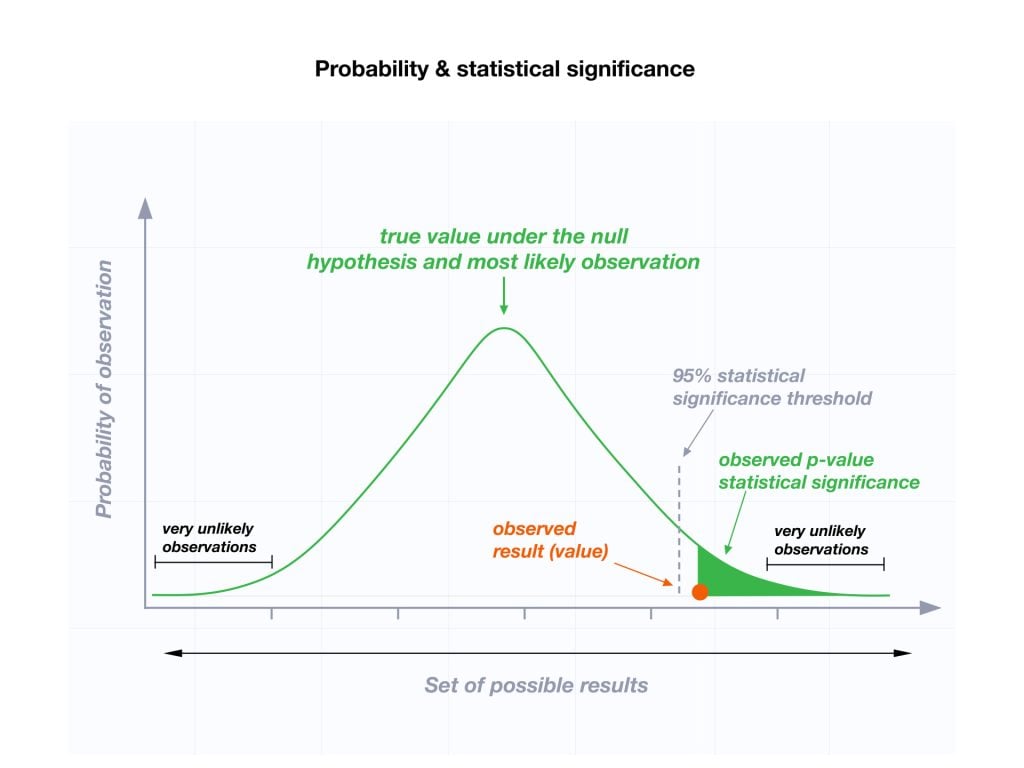
Two-Tailed Test
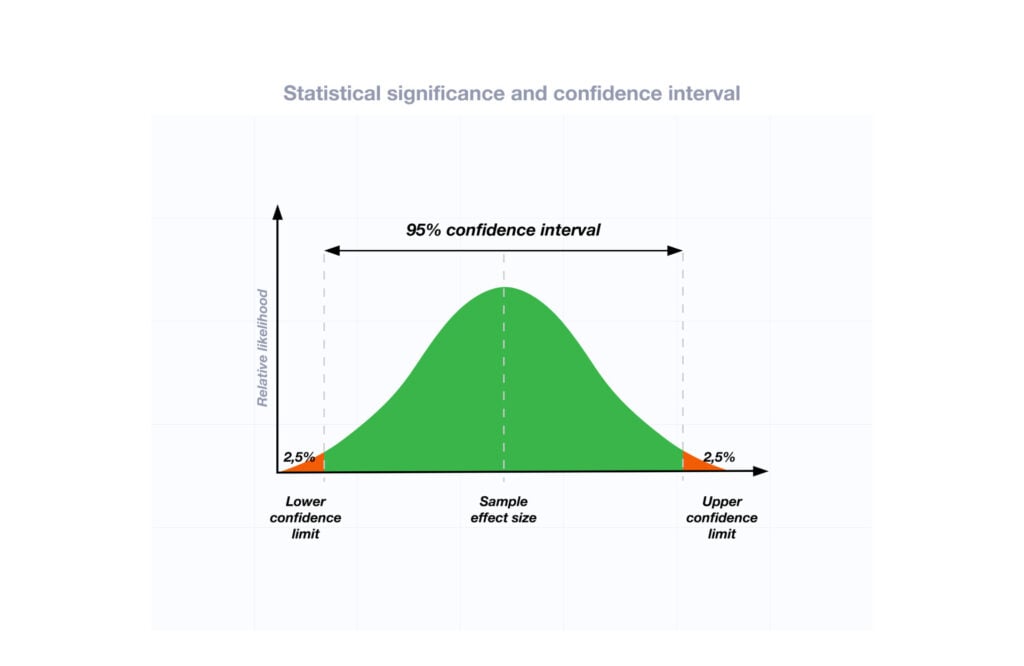
How do you calculate the p-value ?
Most statistical software packages like R, SPSS, and others automatically calculate your p-value. This is the easiest and most common way.
Online resources and tables are available to estimate the p-value based on your test statistic and degrees of freedom.
These tables help you understand how often you would expect to see your test statistic under the null hypothesis.
Understanding the Statistical Test:
Different statistical tests are designed to answer specific research questions or hypotheses. Each test has its own underlying assumptions and characteristics.
For example, you might use a t-test to compare means, a chi-squared test for categorical data, or a correlation test to measure the strength of a relationship between variables.
Be aware that the number of independent variables you include in your analysis can influence the magnitude of the test statistic needed to produce the same p-value.
This factor is particularly important to consider when comparing results across different analyses.
Example: Choosing a Statistical Test
If you’re comparing the effectiveness of just two different drugs in pain relief, a two-sample t-test is a suitable choice for comparing these two groups. However, when you’re examining the impact of three or more drugs, it’s more appropriate to employ an Analysis of Variance ( ANOVA) . Utilizing multiple pairwise comparisons in such cases can lead to artificially low p-values and an overestimation of the significance of differences between the drug groups.
How to report
A statistically significant result cannot prove that a research hypothesis is correct (which implies 100% certainty).
Instead, we may state our results “provide support for” or “give evidence for” our research hypothesis (as there is still a slight probability that the results occurred by chance and the null hypothesis was correct – e.g., less than 5%).
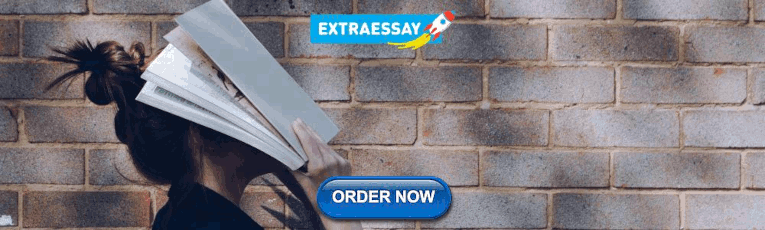
Example: Reporting the results
In our comparison of the pain relief effects of the new drug and the placebo, we observed that participants in the drug group experienced a significant reduction in pain ( M = 3.5; SD = 0.8) compared to those in the placebo group ( M = 5.2; SD = 0.7), resulting in an average difference of 1.7 points on the pain scale (t(98) = -9.36; p < 0.001).
The 6th edition of the APA style manual (American Psychological Association, 2010) states the following on the topic of reporting p-values:
“When reporting p values, report exact p values (e.g., p = .031) to two or three decimal places. However, report p values less than .001 as p < .001.
The tradition of reporting p values in the form p < .10, p < .05, p < .01, and so forth, was appropriate in a time when only limited tables of critical values were available.” (p. 114)
- Do not use 0 before the decimal point for the statistical value p as it cannot equal 1. In other words, write p = .001 instead of p = 0.001.
- Please pay attention to issues of italics ( p is always italicized) and spacing (either side of the = sign).
- p = .000 (as outputted by some statistical packages such as SPSS) is impossible and should be written as p < .001.
- The opposite of significant is “nonsignificant,” not “insignificant.”
Why is the p -value not enough?
A lower p-value is sometimes interpreted as meaning there is a stronger relationship between two variables.
However, statistical significance means that it is unlikely that the null hypothesis is true (less than 5%).
To understand the strength of the difference between the two groups (control vs. experimental) a researcher needs to calculate the effect size .
When do you reject the null hypothesis?
In statistical hypothesis testing, you reject the null hypothesis when the p-value is less than or equal to the significance level (α) you set before conducting your test. The significance level is the probability of rejecting the null hypothesis when it is true. Commonly used significance levels are 0.01, 0.05, and 0.10.
Remember, rejecting the null hypothesis doesn’t prove the alternative hypothesis; it just suggests that the alternative hypothesis may be plausible given the observed data.
The p -value is conditional upon the null hypothesis being true but is unrelated to the truth or falsity of the alternative hypothesis.
What does p-value of 0.05 mean?
If your p-value is less than or equal to 0.05 (the significance level), you would conclude that your result is statistically significant. This means the evidence is strong enough to reject the null hypothesis in favor of the alternative hypothesis.
Are all p-values below 0.05 considered statistically significant?
No, not all p-values below 0.05 are considered statistically significant. The threshold of 0.05 is commonly used, but it’s just a convention. Statistical significance depends on factors like the study design, sample size, and the magnitude of the observed effect.
A p-value below 0.05 means there is evidence against the null hypothesis, suggesting a real effect. However, it’s essential to consider the context and other factors when interpreting results.
Researchers also look at effect size and confidence intervals to determine the practical significance and reliability of findings.
How does sample size affect the interpretation of p-values?
Sample size can impact the interpretation of p-values. A larger sample size provides more reliable and precise estimates of the population, leading to narrower confidence intervals.
With a larger sample, even small differences between groups or effects can become statistically significant, yielding lower p-values. In contrast, smaller sample sizes may not have enough statistical power to detect smaller effects, resulting in higher p-values.
Therefore, a larger sample size increases the chances of finding statistically significant results when there is a genuine effect, making the findings more trustworthy and robust.
Can a non-significant p-value indicate that there is no effect or difference in the data?
No, a non-significant p-value does not necessarily indicate that there is no effect or difference in the data. It means that the observed data do not provide strong enough evidence to reject the null hypothesis.
There could still be a real effect or difference, but it might be smaller or more variable than the study was able to detect.
Other factors like sample size, study design, and measurement precision can influence the p-value. It’s important to consider the entire body of evidence and not rely solely on p-values when interpreting research findings.
Can P values be exactly zero?
While a p-value can be extremely small, it cannot technically be absolute zero. When a p-value is reported as p = 0.000, the actual p-value is too small for the software to display. This is often interpreted as strong evidence against the null hypothesis. For p values less than 0.001, report as p < .001
Further Information
- P-values and significance tests (Kahn Academy)
- Hypothesis testing and p-values (Kahn Academy)
- Wasserstein, R. L., Schirm, A. L., & Lazar, N. A. (2019). Moving to a world beyond “ p “< 0.05”.
- Criticism of using the “ p “< 0.05”.
- Publication manual of the American Psychological Association
- Statistics for Psychology Book Download
Bland, J. M., & Altman, D. G. (1994). One and two sided tests of significance: Authors’ reply. BMJ: British Medical Journal , 309 (6958), 874.
Goodman, S. N., & Royall, R. (1988). Evidence and scientific research. American Journal of Public Health , 78 (12), 1568-1574.
Goodman, S. (2008, July). A dirty dozen: twelve p-value misconceptions . In Seminars in hematology (Vol. 45, No. 3, pp. 135-140). WB Saunders.
Lang, J. M., Rothman, K. J., & Cann, C. I. (1998). That confounded P-value. Epidemiology (Cambridge, Mass.) , 9 (1), 7-8.

Hypothesis Testing (cont...)
Hypothesis testing, the null and alternative hypothesis.
In order to undertake hypothesis testing you need to express your research hypothesis as a null and alternative hypothesis. The null hypothesis and alternative hypothesis are statements regarding the differences or effects that occur in the population. You will use your sample to test which statement (i.e., the null hypothesis or alternative hypothesis) is most likely (although technically, you test the evidence against the null hypothesis). So, with respect to our teaching example, the null and alternative hypothesis will reflect statements about all statistics students on graduate management courses.
The null hypothesis is essentially the "devil's advocate" position. That is, it assumes that whatever you are trying to prove did not happen ( hint: it usually states that something equals zero). For example, the two different teaching methods did not result in different exam performances (i.e., zero difference). Another example might be that there is no relationship between anxiety and athletic performance (i.e., the slope is zero). The alternative hypothesis states the opposite and is usually the hypothesis you are trying to prove (e.g., the two different teaching methods did result in different exam performances). Initially, you can state these hypotheses in more general terms (e.g., using terms like "effect", "relationship", etc.), as shown below for the teaching methods example:
Depending on how you want to "summarize" the exam performances will determine how you might want to write a more specific null and alternative hypothesis. For example, you could compare the mean exam performance of each group (i.e., the "seminar" group and the "lectures-only" group). This is what we will demonstrate here, but other options include comparing the distributions , medians , amongst other things. As such, we can state:
Now that you have identified the null and alternative hypotheses, you need to find evidence and develop a strategy for declaring your "support" for either the null or alternative hypothesis. We can do this using some statistical theory and some arbitrary cut-off points. Both these issues are dealt with next.
Significance levels
The level of statistical significance is often expressed as the so-called p -value . Depending on the statistical test you have chosen, you will calculate a probability (i.e., the p -value) of observing your sample results (or more extreme) given that the null hypothesis is true . Another way of phrasing this is to consider the probability that a difference in a mean score (or other statistic) could have arisen based on the assumption that there really is no difference. Let us consider this statement with respect to our example where we are interested in the difference in mean exam performance between two different teaching methods. If there really is no difference between the two teaching methods in the population (i.e., given that the null hypothesis is true), how likely would it be to see a difference in the mean exam performance between the two teaching methods as large as (or larger than) that which has been observed in your sample?
So, you might get a p -value such as 0.03 (i.e., p = .03). This means that there is a 3% chance of finding a difference as large as (or larger than) the one in your study given that the null hypothesis is true. However, you want to know whether this is "statistically significant". Typically, if there was a 5% or less chance (5 times in 100 or less) that the difference in the mean exam performance between the two teaching methods (or whatever statistic you are using) is as different as observed given the null hypothesis is true, you would reject the null hypothesis and accept the alternative hypothesis. Alternately, if the chance was greater than 5% (5 times in 100 or more), you would fail to reject the null hypothesis and would not accept the alternative hypothesis. As such, in this example where p = .03, we would reject the null hypothesis and accept the alternative hypothesis. We reject it because at a significance level of 0.03 (i.e., less than a 5% chance), the result we obtained could happen too frequently for us to be confident that it was the two teaching methods that had an effect on exam performance.
Whilst there is relatively little justification why a significance level of 0.05 is used rather than 0.01 or 0.10, for example, it is widely used in academic research. However, if you want to be particularly confident in your results, you can set a more stringent level of 0.01 (a 1% chance or less; 1 in 100 chance or less).
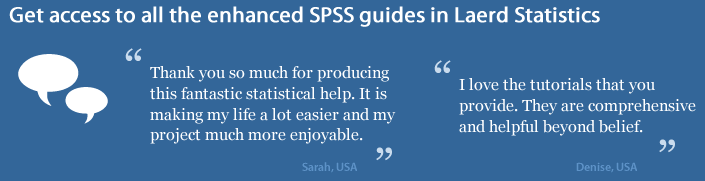
One- and two-tailed predictions
When considering whether we reject the null hypothesis and accept the alternative hypothesis, we need to consider the direction of the alternative hypothesis statement. For example, the alternative hypothesis that was stated earlier is:
The alternative hypothesis tells us two things. First, what predictions did we make about the effect of the independent variable(s) on the dependent variable(s)? Second, what was the predicted direction of this effect? Let's use our example to highlight these two points.
Sarah predicted that her teaching method (independent variable: teaching method), whereby she not only required her students to attend lectures, but also seminars, would have a positive effect (that is, increased) students' performance (dependent variable: exam marks). If an alternative hypothesis has a direction (and this is how you want to test it), the hypothesis is one-tailed. That is, it predicts direction of the effect. If the alternative hypothesis has stated that the effect was expected to be negative, this is also a one-tailed hypothesis.
Alternatively, a two-tailed prediction means that we do not make a choice over the direction that the effect of the experiment takes. Rather, it simply implies that the effect could be negative or positive. If Sarah had made a two-tailed prediction, the alternative hypothesis might have been:
In other words, we simply take out the word "positive", which implies the direction of our effect. In our example, making a two-tailed prediction may seem strange. After all, it would be logical to expect that "extra" tuition (going to seminar classes as well as lectures) would either have a positive effect on students' performance or no effect at all, but certainly not a negative effect. However, this is just our opinion (and hope) and certainly does not mean that we will get the effect we expect. Generally speaking, making a one-tail prediction (i.e., and testing for it this way) is frowned upon as it usually reflects the hope of a researcher rather than any certainty that it will happen. Notable exceptions to this rule are when there is only one possible way in which a change could occur. This can happen, for example, when biological activity/presence in measured. That is, a protein might be "dormant" and the stimulus you are using can only possibly "wake it up" (i.e., it cannot possibly reduce the activity of a "dormant" protein). In addition, for some statistical tests, one-tailed tests are not possible.
Rejecting or failing to reject the null hypothesis
Let's return finally to the question of whether we reject or fail to reject the null hypothesis.
If our statistical analysis shows that the significance level is below the cut-off value we have set (e.g., either 0.05 or 0.01), we reject the null hypothesis and accept the alternative hypothesis. Alternatively, if the significance level is above the cut-off value, we fail to reject the null hypothesis and cannot accept the alternative hypothesis. You should note that you cannot accept the null hypothesis, but only find evidence against it.
Have a language expert improve your writing
Run a free plagiarism check in 10 minutes, generate accurate citations for free.
- Knowledge Base
- Null and Alternative Hypotheses | Definitions & Examples
Null & Alternative Hypotheses | Definitions, Templates & Examples
Published on May 6, 2022 by Shaun Turney . Revised on June 22, 2023.
The null and alternative hypotheses are two competing claims that researchers weigh evidence for and against using a statistical test :
- Null hypothesis ( H 0 ): There’s no effect in the population .
- Alternative hypothesis ( H a or H 1 ) : There’s an effect in the population.
Table of contents
Answering your research question with hypotheses, what is a null hypothesis, what is an alternative hypothesis, similarities and differences between null and alternative hypotheses, how to write null and alternative hypotheses, other interesting articles, frequently asked questions.
The null and alternative hypotheses offer competing answers to your research question . When the research question asks “Does the independent variable affect the dependent variable?”:
- The null hypothesis ( H 0 ) answers “No, there’s no effect in the population.”
- The alternative hypothesis ( H a ) answers “Yes, there is an effect in the population.”
The null and alternative are always claims about the population. That’s because the goal of hypothesis testing is to make inferences about a population based on a sample . Often, we infer whether there’s an effect in the population by looking at differences between groups or relationships between variables in the sample. It’s critical for your research to write strong hypotheses .
You can use a statistical test to decide whether the evidence favors the null or alternative hypothesis. Each type of statistical test comes with a specific way of phrasing the null and alternative hypothesis. However, the hypotheses can also be phrased in a general way that applies to any test.
Receive feedback on language, structure, and formatting
Professional editors proofread and edit your paper by focusing on:
- Academic style
- Vague sentences
- Style consistency
See an example
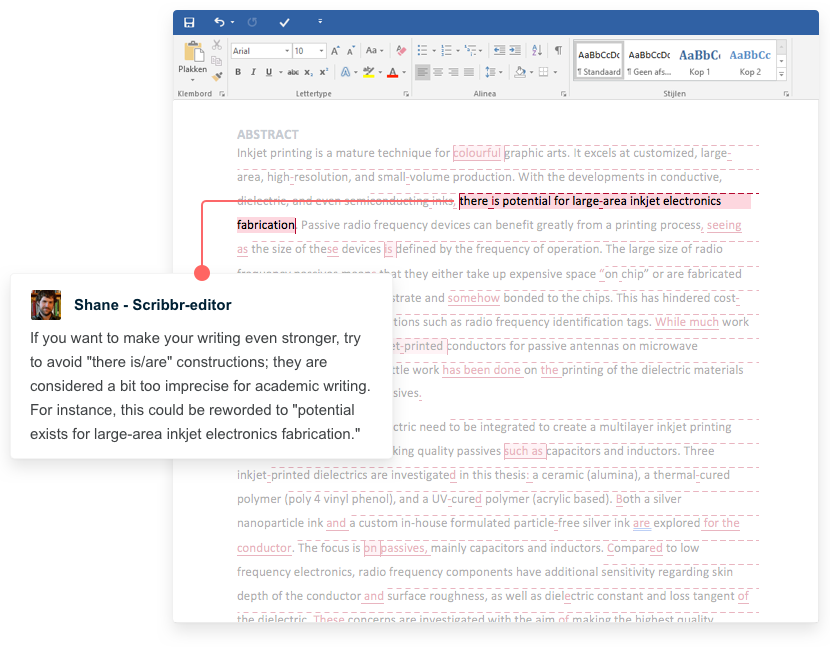
The null hypothesis is the claim that there’s no effect in the population.
If the sample provides enough evidence against the claim that there’s no effect in the population ( p ≤ α), then we can reject the null hypothesis . Otherwise, we fail to reject the null hypothesis.
Although “fail to reject” may sound awkward, it’s the only wording that statisticians accept . Be careful not to say you “prove” or “accept” the null hypothesis.
Null hypotheses often include phrases such as “no effect,” “no difference,” or “no relationship.” When written in mathematical terms, they always include an equality (usually =, but sometimes ≥ or ≤).
You can never know with complete certainty whether there is an effect in the population. Some percentage of the time, your inference about the population will be incorrect. When you incorrectly reject the null hypothesis, it’s called a type I error . When you incorrectly fail to reject it, it’s a type II error.
Examples of null hypotheses
The table below gives examples of research questions and null hypotheses. There’s always more than one way to answer a research question, but these null hypotheses can help you get started.
*Note that some researchers prefer to always write the null hypothesis in terms of “no effect” and “=”. It would be fine to say that daily meditation has no effect on the incidence of depression and p 1 = p 2 .
The alternative hypothesis ( H a ) is the other answer to your research question . It claims that there’s an effect in the population.
Often, your alternative hypothesis is the same as your research hypothesis. In other words, it’s the claim that you expect or hope will be true.
The alternative hypothesis is the complement to the null hypothesis. Null and alternative hypotheses are exhaustive, meaning that together they cover every possible outcome. They are also mutually exclusive, meaning that only one can be true at a time.
Alternative hypotheses often include phrases such as “an effect,” “a difference,” or “a relationship.” When alternative hypotheses are written in mathematical terms, they always include an inequality (usually ≠, but sometimes < or >). As with null hypotheses, there are many acceptable ways to phrase an alternative hypothesis.
Examples of alternative hypotheses
The table below gives examples of research questions and alternative hypotheses to help you get started with formulating your own.
Null and alternative hypotheses are similar in some ways:
- They’re both answers to the research question.
- They both make claims about the population.
- They’re both evaluated by statistical tests.
However, there are important differences between the two types of hypotheses, summarized in the following table.
Here's why students love Scribbr's proofreading services
Discover proofreading & editing
To help you write your hypotheses, you can use the template sentences below. If you know which statistical test you’re going to use, you can use the test-specific template sentences. Otherwise, you can use the general template sentences.
General template sentences
The only thing you need to know to use these general template sentences are your dependent and independent variables. To write your research question, null hypothesis, and alternative hypothesis, fill in the following sentences with your variables:
Does independent variable affect dependent variable ?
- Null hypothesis ( H 0 ): Independent variable does not affect dependent variable.
- Alternative hypothesis ( H a ): Independent variable affects dependent variable.
Test-specific template sentences
Once you know the statistical test you’ll be using, you can write your hypotheses in a more precise and mathematical way specific to the test you chose. The table below provides template sentences for common statistical tests.
Note: The template sentences above assume that you’re performing one-tailed tests . One-tailed tests are appropriate for most studies.
If you want to know more about statistics , methodology , or research bias , make sure to check out some of our other articles with explanations and examples.
- Normal distribution
- Descriptive statistics
- Measures of central tendency
- Correlation coefficient
Methodology
- Cluster sampling
- Stratified sampling
- Types of interviews
- Cohort study
- Thematic analysis
Research bias
- Implicit bias
- Cognitive bias
- Survivorship bias
- Availability heuristic
- Nonresponse bias
- Regression to the mean
Hypothesis testing is a formal procedure for investigating our ideas about the world using statistics. It is used by scientists to test specific predictions, called hypotheses , by calculating how likely it is that a pattern or relationship between variables could have arisen by chance.
Null and alternative hypotheses are used in statistical hypothesis testing . The null hypothesis of a test always predicts no effect or no relationship between variables, while the alternative hypothesis states your research prediction of an effect or relationship.
The null hypothesis is often abbreviated as H 0 . When the null hypothesis is written using mathematical symbols, it always includes an equality symbol (usually =, but sometimes ≥ or ≤).
The alternative hypothesis is often abbreviated as H a or H 1 . When the alternative hypothesis is written using mathematical symbols, it always includes an inequality symbol (usually ≠, but sometimes < or >).
A research hypothesis is your proposed answer to your research question. The research hypothesis usually includes an explanation (“ x affects y because …”).
A statistical hypothesis, on the other hand, is a mathematical statement about a population parameter. Statistical hypotheses always come in pairs: the null and alternative hypotheses . In a well-designed study , the statistical hypotheses correspond logically to the research hypothesis.
Cite this Scribbr article
If you want to cite this source, you can copy and paste the citation or click the “Cite this Scribbr article” button to automatically add the citation to our free Citation Generator.
Turney, S. (2023, June 22). Null & Alternative Hypotheses | Definitions, Templates & Examples. Scribbr. Retrieved April 17, 2024, from https://www.scribbr.com/statistics/null-and-alternative-hypotheses/
Is this article helpful?
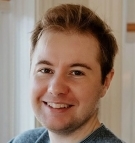
Shaun Turney
Other students also liked, inferential statistics | an easy introduction & examples, hypothesis testing | a step-by-step guide with easy examples, type i & type ii errors | differences, examples, visualizations, what is your plagiarism score.
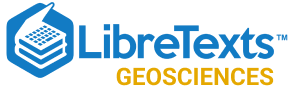
- school Campus Bookshelves
- menu_book Bookshelves
- perm_media Learning Objects
- login Login
- how_to_reg Request Instructor Account
- hub Instructor Commons
- Download Page (PDF)
- Download Full Book (PDF)
- Periodic Table
- Physics Constants
- Scientific Calculator
- Reference & Cite
- Tools expand_more
- Readability
selected template will load here
This action is not available.
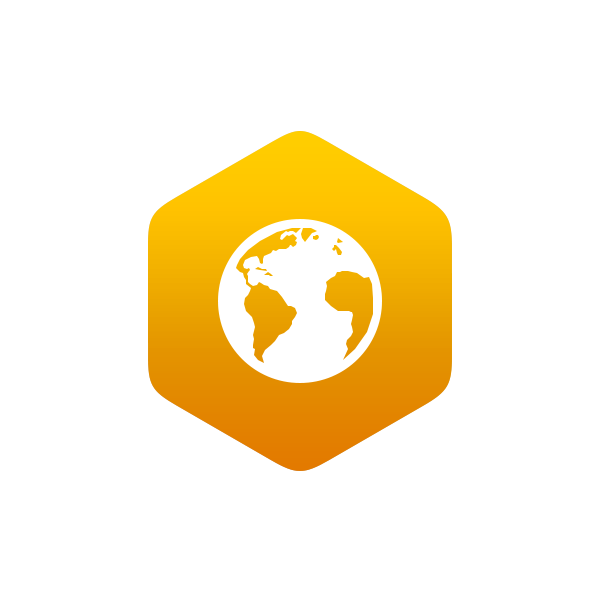
2.1: Alfred Wegener’s Continental Drift Hypothesis
- Last updated
- Save as PDF
- Page ID 11199
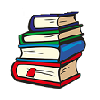
- Chris Johnson, Matthew D. Affolter, Paul Inkenbrandt, & Cam Mosher
- Salt Lake Community College via OpenGeology
Alfred Wegener (1880-1930) was a German scientist who specialized in meteorology and climatology. His knack for questioning accepted ideas started in 1910 when he disagreed with the explanation that the Bering Land Bridge was formed by isostasy and that similar land bridges once connected the continents [ 1 ]. After reviewing the scientific literature, he published a hypothesis stating the continents were originally connected and then drifted apart. While he did not have the precise mechanism worked out, his hypothesis was backed up by a long list of evidence.
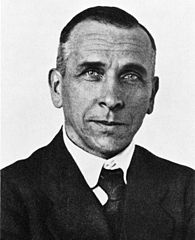
Early Evidence for Continental Drift Hypothesis
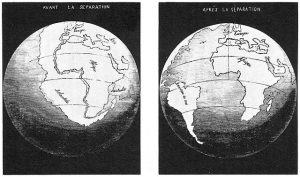
Wegener’s first piece of evidence was that the coastlines of some continents fit together like pieces of a jigsaw puzzle. People noticed the similarities in the coastlines of South America and Africa on the first world maps, and some suggested the continents had been ripped apart [ 3 ]. Antonio Snider-Pellegrini did preliminary work on continental separation and matching fossils in 1858.
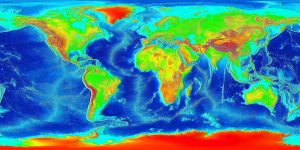
What Wegener did differently was synthesizing a large amount of data in one place. He used the true edges of the continents, based on the shapes of the continental shelves [ 4 ]. This resulted in a better fit than previous efforts that traced the existing coastlines [ 5 ].
Wegener also compiled evidence by comparing similar rocks, mountains, fossils, and glacial formations across oceans. For example, the fossils of the primitive aquatic reptile Mesosaurus were found on the separate coastlines of Africa and South America. Fossils of another reptile, Lystrosaurus, were found in Africa, India, and Antarctica. He pointed out these were land-dwelling creatures could not have swum across an entire ocean.
Opponents of continental drift insisted trans-oceanic land bridges allowed animals and plants to move between continents [ 6 ]. The land bridges eventually eroded away, leaving the continents permanently separated. The problem with this hypothesis is the improbability of a land bridge being tall and long enough to stretch across a broad, deep ocean.
More support for continental drift came from the puzzling evidence that glaciers once existed in normally very warm areas in southern Africa, India, Australia, and Arabia. These climate anomalies could not be explained by land bridges. Wegener found similar evidence when he discovered tropical plant fossils in the frozen region of the Arctic Circle. As Wegener collected more data, he realized the explanation that best fit all the climate, rock, and fossil observations involved moving continents.
Proposed Mechanism for Continental Drift
Figure \(\PageIndex{5}\): [Click to Animate] Animation of the basic idea of convection: an uneven heat source in a fluid causes rising material next to the heat and sinking material far from the heat.
Wegener’s work was considered a fringe science theory for his entire life. One of the biggest flaws in his hypothesis was the inability to provide a mechanism for how the continents moved. Obviously, the continents did not appear to move, and changing the conservative minds of the scientific community would require exceptional evidence that supported a credible mechanism. Other pro-continental drift followers used expansion, contraction, or even the moon’s origin to explain how the continents moved. Wegener used centrifugal forces and precession, but this model was proven wrong [ 7 ]. He also speculated about seafloor spreading, with hints of convection, but could not substantiate these proposals [ 8 ]. As it turns out, current scientific knowledge reveals convection is the major force in driving plate movements.
Development of Plate Tectonic Theory
Wegener died in 1930 on an expedition in Greenland. Poorly respected in his lifetime, Wegener and his ideas about moving continents seemed destined to be lost in history as fringe science. However, in the 1950s, evidence started to trickle in that made continental drift a more viable idea. By the 1960s, scientists had amassed enough evidence to support the missing mechanism—namely, seafloor spreading—for Wegener’s hypothesis of continental drift to be accepted as the theory of plate tectonics. Ongoing GPS and earthquake data analyses continue to support this theory. The next section provides the pieces of evidence that helped transform one man’s wild notion into a scientific theory.
Mapping of the Ocean Floors
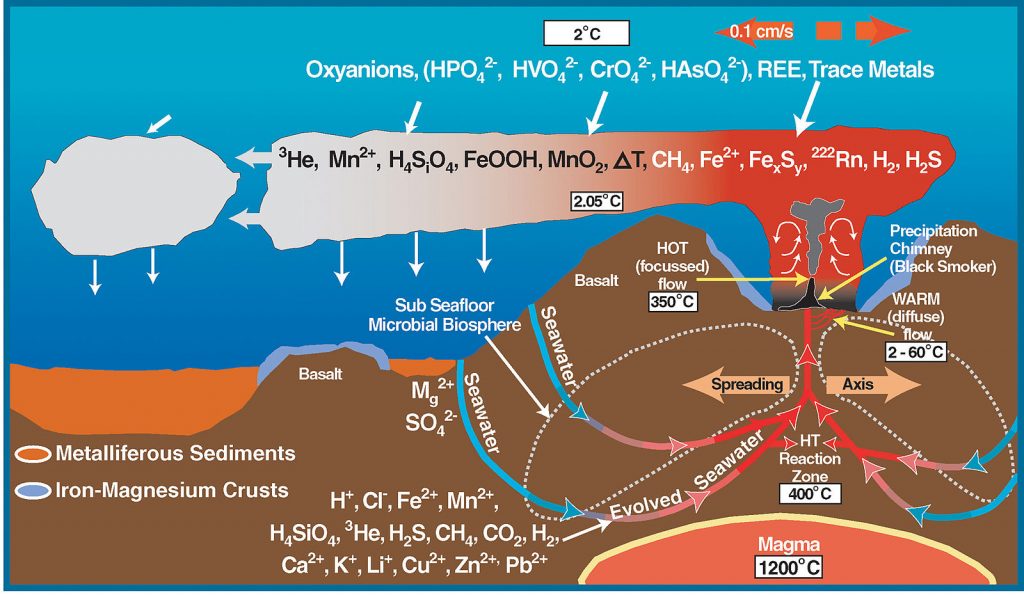
In 1947 researchers started using an adaptation of SONAR to map a region in the middle of the Atlantic Ocean with poorly-understood topographic and thermal properties [ 9 ]. Using this information, Bruce Heezen and Marie Tharp created the first detailed map of the ocean floor to reveal the Mid-Atlantic Ridge [ 10 ], a basaltic mountain range that spanned the length of the Atlantic Ocean, with rock chemistry and dimensions unlike the mountains found on the continents. Initially, scientists thought the ridge was part of a mechanism that explained the expanding Earth or ocean-basin growth hypotheses [ 11 ; 12 ]. In 1959, Harry Hess proposed the hypothesis of seafloor spreading – that the mid-ocean ridges represented tectonic plate factories, where a new oceanic plate was issuing from these long volcanic ridges. Scientists later included transform faults perpendicular to the ridges to better account for varying rates of movement between the newly formed plates [ 13 ]. When earthquake epicenters were discovered along the ridges, the idea that earthquakes were linked to plate movement took hold [ 14 ].
Seafloor sediment, measured by dredging and drilling, provided another clue. Scientists once believed sediment accumulated on the ocean floors over a very long time in a static environment. When some studies showed less sediment than expected, these results were initially used to argue against the continental movement [ 15 ; 16 ]. With more time, researchers discovered these thinner sediment layers were located close to mid-ocean ridges, indicating the ridges were younger than the surrounding ocean floor. This finding supported the idea that the seafloor was not fixed in one place [ 17 ].
Paleomagnetism
The seafloor was also mapped magnetically. Scientists had long known of strange magnetic anomalies that formed a striped pattern of symmetrical rows on both sides of mid-oceanic ridges. What made these features unusual was the north and south magnetic poles within each stripe was reversed in alternating rows [ 18 ]. By 1963, Harry Hess and other scientists used these magnetic reversal patterns to support their model for seafloor spreading [ 19 ] (see also Lawrence W. Morley [ 20 ]).
Paleomagnetism is the study of magnetic fields frozen within rocks, basically a fossilized compass. In fact, the first hard evidence to support plate motion came from paleomagnetism.
Igneous rocks containing magnetic minerals like magnetite typically provide the most useful data. In their liquid state as magma or lava, the magnetic poles of the minerals align themselves with the Earth’s magnetic field. When the rock cools and solidifies, this alignment is frozen into place, creating a permanent paleomagnetic record that includes magnetic inclination related to global latitude, and declination related to magnetic north.
Scientists had noticed for some time the alignment of magnetic north in many rocks was nowhere close to the earth’s current magnetic north. Some explained this as part of the normal movement of earth magnetic north pole. Eventually, scientists realized adding the idea of continental movement explained the data better than the pole movement alone [ 21 ].
Wadati-Benioff Zones
Around the same time mid-ocean ridges were being investigated, other scientists linked the creation of ocean trenches and island arcs to seismic activity and tectonic plate movement [ 22 ]. Several independent research groups recognized earthquake epicenters traced the shapes of oceanic plates sinking into the mantle. These deep earthquake zones congregated in planes that started near the surface around ocean trenches and angled beneath the continents and island arcs [ 23 ]. Today these earthquake zones called Wadati-Benioff zones.
Based on the mounting evidence, the theory plate tectonics continued to take shape. J. Tuzo Wilson was the first scientist to put the entire picture together by proposing that the opening and closing of the ocean basins [ 24 ]. Before long, scientists proposed other models showing plates moving with respect to each other, with clear boundaries between them [ 25 ]. Others started piecing together complicated histories of tectonic plate movement [ 26 ]. The plate tectonic revolution had taken hold.
- 1. Fluegel, von H. W. Wegener-Ampferer-Schwinner. Ein Beitrag zur Geschichte der Geologie in Österreich. Mitt. Oesterr. Geol. Ges. 73 , 237–254 (1980).
- 3. Bacon, F. & Montagu, B. The Works of Francis Bacon, Lord Chancellor of England: With a Life of the Author . (Parry & McMillan, 1848).
- 4. Drake, E. T. Alfred Wegener’s reconstruction of Pangea. Geology 4 , 41–44 (1976).
- 5. Mantovani, R. Les fractures de l’écorce terrestre et la théorie de Laplace. Bull. Soc. Sc. et Arts Réunion 41–53 (1889).
- 6. Wells, H. G., Huxley, J. & Wells, G. P. The Science of Life. Philosophy 6 , 506–507 (1931).
- 7. Scheidegger, A. E. Examination of the physics of theories of orogenesis. Geol. Soc. Am. Bull. 64 , 127–150 (1953).
- 8. Jacoby, W. R. Modern concepts of Earth dynamics anticipated by Alfred Wegener in 1912. Geology 9 , 25–27 (1981).
- 9. Tolstoy, I. & Ewing, M. North Atlantic hydrography and the Mid-Atlantic Ridge. Geol. Soc. Am. Bull. 60 , 1527–1540 (1949).
- 10. Heezen, B. C., Tharp, M. & Ewing, M. The Floors of the Oceans I. The North Atlantic. Geological Society of America Special Papers 65 , 1–126 (1959).
- 11. Heezen, B. C. The Rift in the Ocean Floor. Sci. Am. 203 , 98–110 (1960).
- 12. Dietz, R. S. Continent and ocean basin evolution by spreading of the seafloor. Nature 190 , 854–857 (1961).
- 13. Wilson, J. T. A new class of faults and their bearing on continental drift. Nature (1965).
- 14. Heezen, B. C. & Tharp, M. Tectonic Fabric of the Atlantic and Indian Oceans and Continental Drift. Philosophical Transactions of the Royal Society of London A: Mathematical, Physical and Engineering Sciences 258 , 90–106 (1965).
- 15. Ewing, M., Ewing, J. I. & Talwani, M. Sediment distribution in the oceans: The Mid-Atlantic Ridge. Geol. Soc. Am. Bull. 75 , 17–36 (1964).
- 16. Saito, T., Ewing, M. & Burckle, L. H. Tertiary sediment from the mid-atlantic ridge. Science 151 , 1075–1079 (1966).
- 17. Ewing, M., Houtz, R. & Ewing, J. South Pacific sediment distribution. J. Geophys. Res. 74 , 2477–2493 (1969).
- 18. Mason, R. G. A magnetic survey off the west coast of the United-States between latitudes 32-degrees-N and 36-degrees-N longitudes 121-degrees-W and 128-degrees-W. Geophysical Journal of the Royal Astronomical Society 1 , 320 (1958).
- 19. Vine, F. J. & Matthews, D. H. Magnetic anomalies over oceanic ridges. Nature 199 , 947–949 (1963).
- 20. Frankel, H. The Development, Reception, and Acceptance of the Vine-Matthews-Morley Hypothesis. Hist. Stud. Phys. Biol. Sci. 13 , 1–39 (1982).
- 21. Irving, E. Palaeomagnetic and palaeoclimatological aspects of polar wandering. Geofis. pura appl. 33 , 23–41 (1956).
- 22. Coats, R. R. Magma type and crustal structure in the Aleutian Arc. in The Crust of the Pacific Basin 92–109 (American Geophysical Union, 1962). doi:10.1029/GM006p0092
- 23. Wadati, K. On the activity of deep-focus earthquakes in the Japan Islands and neighbourhoods. Geophys. Mag. 8 , 305–325 (1935).
- 24. Wilson, J. T. Did the Atlantic close and then re-open? (Nature, 1966).
- 25. McKenzie, D. P. & Parker, R. L. The North Pacific: an Example of Tectonics on a Sphere. Nature 216 , 1276–1280 (1967).
- 26. Atwater, T. Implications of Plate Tectonics for the Cenozoic Tectonic Evolution of Western North America. Geol. Soc. Am. Bull. 81 , 3513–3536 (1970).
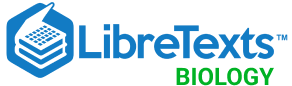
- school Campus Bookshelves
- menu_book Bookshelves
- perm_media Learning Objects
- login Login
- how_to_reg Request Instructor Account
- hub Instructor Commons
- Download Page (PDF)
- Download Full Book (PDF)
- Periodic Table
- Physics Constants
- Scientific Calculator
- Reference & Cite
- Tools expand_more
- Readability
selected template will load here
This action is not available.
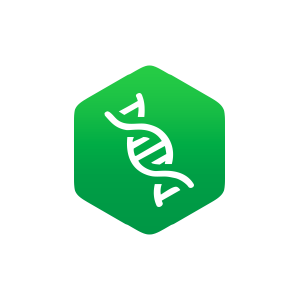
7.8: The Endosymbiotic Theory
- Last updated
- Save as PDF
- Page ID 3220
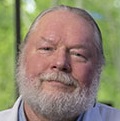
- Gary Kaiser
- Community College of Baltimore Country (Cantonsville)
Learning Objectives
- Briefly describe what is meant by the endosymbiotic theory.
- Give some evidence supporting the theory that mitochondria and chloroplasts may have arisen from prokaryotic organisms.
It is thought that life arose on earth around four billion years ago. The endosymbiotic theory states that some of the organelles in today's eukaryotic cells were once prokaryotic microbes. In this theory, the first eukaryotic cell was probably an amoeba-like cell that got nutrients by phagocytosis and contained a nucleus that formed when a piece of the cytoplasmic membrane pinched off around the chromosomes. Some of these amoeba-like organisms ingested prokaryotic cells that then survived within the organism and developed a symbiotic relationship. Mitochondria formed when bacteria capable of aerobic respiration were ingested; chloroplasts formed when photosynthetic bacteria were ingested. They eventually lost their cell wall and much of their DNA because they were not of benefit within the host cell. Mitochondria and chloroplasts cannot grow outside their host cell.
Evidence for this is based on the following:
- Chloroplasts are the same size as prokaryotic cells, divide by binary fission, and, like bacteria, have Fts proteins at their division plane. The mitochondria are the same size as prokaryotic cells, divide by binary fission, and the mitochondria of some protists have Fts homologs at their division plane.
- Mitochondria and chloroplasts have their own DNA that is circular, not linear.
- Mitochondria and chloroplasts have their own ribosomes that have 30S and 50S subunits, not 40S and 60S.
- Several more primitive eukaryotic microbes, such as Giardia and Trichomonas have a nuclear membrane but no mitochondria.
Although evidence is less convincing, it is also possible that flagella and cilia may have come from spirochetes.
Example \(\PageIndex{1}\)
- Give three points of evidence supporting the theory that mitochondria and chloroplasts may have arisen from prokaryotic organisms.
- The endosymbiotic theory states that some of the organelles in eukaryotic cells were once prokaryotic microbes.
- Mitochondria and chloroplasts are the same size as prokaryotic cells and divide by binary fission.
- Mitochondria and chloroplasts have their own DNA which is circular, not linear.
- Mitochondria and chloroplasts have their own ribosomes which have 30S and 50S subunits, not 40S and 60S.
The endosymbiotic theory states that mitochondria and chlopoplasts in today's eukaryotic cells were once separate prokaryotic microbes.
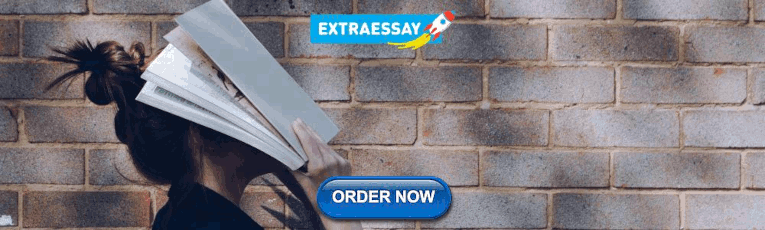
IMAGES
VIDEO
COMMENTS
In this article, we'll examine the evidence for evolution on both macro and micro scales. First, we'll look at several types of evidence (including physical and molecular features, geographical information, and fossils) that provide evidence for, and can allow us to reconstruct, macroevolutionary events. At the end of the article, we'll finish ...
The evidence for evolution is compelling and extensive. Looking at every level of organization in living systems, biologists see the signature of past and present evolution. Darwin dedicated a large portion of his book, On the Origin of Species, to identifying patterns in nature that were consistent with evolution, and since Darwin, our ...
In this section, we will explore the lines of evidence that are used to reconstruct this story. These lines of evidence include: Fossil evidence and transitional features. Distribution in time and space. Homologies. Developmental biology. Nested hierarchies. Observations of evolution in the wild. Experiments.
The theory of gravity and quantum mechanics are not proven but but you do not question them being taught. Evolution is a scientific theory and has a bunch of supporting verifiable evidence. It is a theory that accurately describes observations and has made predictions that we have been verified. Gaps in the fossil record do not disprove evolution.
Scientific evidence is evidence that serves to either support or counter a scientific theory or hypothesis, although scientists also use evidence in other ways, such as when applying theories to practical problems. Such evidence is expected to be empirical evidence and interpretable in accordance with the scientific method.Standards for scientific evidence vary according to the field of ...
This evidence helps strengthen the argument that is being driven by providing facts and logical reasoning that support the hypothesis. Examples of evidence that can be used to back up a hypothesis include using data from experiments, case studies, and other research projects. Data from experiments can provide insight into how certain variables ...
Anatomy and Embryology. Another type of evidence for evolution is the presence of structures in organisms that share the same basic form. For example, the bones in the appendages of a human, dog, bird, and whale all share the same overall construction (Figure 21.5.2 21.5. 2 ). That similarity results from their origin in the appendages of a ...
scientific hypothesis, an idea that proposes a tentative explanation about a phenomenon or a narrow set of phenomena observed in the natural world.The two primary features of a scientific hypothesis are falsifiability and testability, which are reflected in an "If…then" statement summarizing the idea and in the ability to be supported or refuted through observation and experimentation.
Evidence for endosymbiosis. Biologist Lynn Margulis first made the case for endosymbiosis in the 1960s, but for many years other biologists were skeptical. Although Jeon watched his amoebae become infected with the x-bacteria and then evolve to depend upon them, no one was around over a billion years ago to observe the events of endosymbiosis.
Like extant organisms, fossils vary in size from microscopic, like single-celled bacteria, to gigantic, like dinosaurs and trees. Figure 18.5A. 1 18.5 A. 1: "Sue" T-rex skeleton: The bones of this Tyrannosaurus rex were preserved through the process of permineralization, which suggests that this organism was covered by sediment soon after ...
Solid disconfirmatory evidence will falsify a hypothesis and require altering or discarding it based on the evidence. However, confirming evidence is always open to revision. ... If there is evidence in the literature to support a specific effect of the independent variable on the dependent variable, write a directional (one-tailed) hypothesis. ...
5. Phrase your hypothesis in three ways. To identify the variables, you can write a simple prediction in if…then form. The first part of the sentence states the independent variable and the second part states the dependent variable. If a first-year student starts attending more lectures, then their exam scores will improve.
It indicates strong evidence against the null hypothesis, as there is less than a 5% probability the null is correct (and the results are random). Therefore, we reject the null hypothesis and accept the alternative hypothesis. ... Instead, we may state our results "provide support for" or "give evidence for" our research hypothesis (as ...
Now that you have identified the null and alternative hypotheses, you need to find evidence and develop a strategy for declaring your "support" for either the null or alternative hypothesis. We can do this using some statistical theory and some arbitrary cut-off points. Both these issues are dealt with next. Hypothesis Testing Significance levels
The null and alternative hypotheses are two competing claims that researchers weigh evidence for and against using a statistical test: Null hypothesis (H 0): There's no effect in the population. Alternative hypothesis (H a or H 1): There's an effect in the population. The effect is usually the effect of the independent variable on the ...
A veritable legion of evidence supports the seafloor spreading hypothesis. Studies conducted with thermal probes, for example, indicate that the heat flow through bottom sediments is generally comparable to that through the continents except over the mid-ocean ridges, where at some sites the heat flow measures three to four times the normal value. The anomalously high values are considered to ...
A hypothesis is an essential part of the scientific method and helps to guide the research process by providing a clear focus for investigation. It enables scientists to design experiments or studies to gather evidence and data that can support or refute the proposed explanation or prediction.
By the 1960s, scientists had amassed enough evidence to support the missing mechanism—namely, seafloor spreading—for Wegener's hypothesis of continental drift to be accepted as the theory of plate tectonics. Ongoing GPS and earthquake data analyses continue to support this theory. The next section provides the pieces of evidence that ...
Hypothesis as evidence for the validity of that theory. Evidence That Matthew and Luke Used Mark as a Source The significant overlap of material between Mark and the other two Synoptic Gospels suggests either (1) Mark used Matthew and/or Luke as a source, or (2) both Matthew and Luke used Mark. The latter seems more likely for these reasons:
Briefly describe what is meant by the endosymbiotic theory. Give three points of evidence supporting the theory that mitochondria and chloroplasts may have arisen from prokaryotic organisms. ... We also acknowledge previous National Science Foundation support under grant numbers 1246120, 1525057, and 1413739. Legal.
Study with Quizlet and memorize flashcards containing terms like The experiments of Stanley Miller and Harold Urey tested whether_____could trigger the formation of simple organic compounds from _____, Which of the following provides support for the RNA world hypothesis?, Which of the following are proposed stages in the evolution of cells? and more.
1. Evidence 2. Desire 3. Support. To go through these briefly: 1. I'm using the term "evidence" in a general sense to include things you directly observe and also convincing arguments of some sort or another. Evidence can be ambiguous and, much to people's confusion, it doesn't always point in the same direction.
Incorporate what we already know about the conditions to support use. Identify whether we can facilitate evidence use in existing systems. In one of the first blog posts in this series on evidence use, we discussed some of the research on the organizational conditions that support evidence use. The five key dimensions of ACF's evidence ...
For example, TMEM72/Tmem72 is estimated to be positively selected by iKa/Ks (1.13) but negatively selected by the conventional Ka/Ks ratio (0.21). Further evidence showed its rapid evolution, which further support the power of the new algorithm. ### Competing Interest Statement The authors have declared no competing interest.