- UNC Libraries
- HSL Academic Process
- Systematic Reviews
- Step 3: Conduct Literature Searches
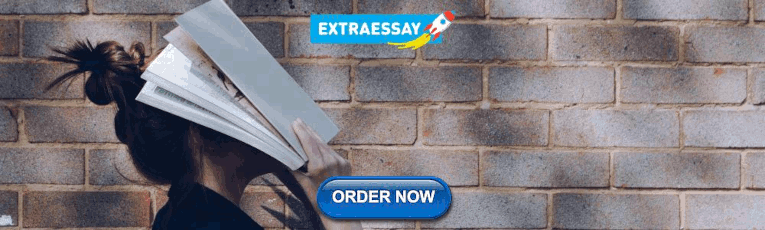
Systematic Reviews: Step 3: Conduct Literature Searches
Created by health science librarians.

- Step 1: Complete Pre-Review Tasks
- Step 2: Develop a Protocol
About Step 3: Conduct Literature Searches
Partner with a librarian, systematic searching process, choose a few databases, search with controlled vocabulary and keywords, acknowledge outdated or offensive terminology, helpful tip - building your search, use nesting, boolean operators, and field tags, build your search, translate to other databases and other searching methods, document the search, updating your review.
- Searching FAQs
- Step 4: Manage Citations
- Step 5: Screen Citations
- Step 6: Assess Quality of Included Studies
- Step 7: Extract Data from Included Studies
- Step 8: Write the Review
Check our FAQ's
Email us
Chat with us (during business hours)
Call (919) 962-0800
Make an appointment with a librarian
Request a systematic or scoping review consultation
Search the FAQs
In Step 3, you will design a search strategy to find all of the articles related to your research question. You will:
- Define the main concepts of your topic
- Choose which databases you want to search
- List terms to describe each concept
- Add terms from controlled vocabulary like MeSH
- Use field tags to tell the database where to search for terms
- Combine terms and concepts with Boolean operators AND and OR
- Translate your search strategy to match the format standards for each database
- Save a copy of your search strategy and details about your search
There are many factors to think about when building a strong search strategy for systematic reviews. Librarians are available to provide support with this step of the process.
Click an item below to see how it applies to Step 3: Conduct Literature Searches.
Reporting your review with PRISMA
For PRISMA, there are specific items you will want to report from your search. For this step, review the PRISMA-S checklist.
- PRISMA-S for Searching
- Specify all databases, registers, websites, organizations, reference lists, and other sources searched or consulted to identify studies. Specify the date when each source was last searched or consulted. Present the full search strategies for all databases, registers and websites, including any filters and limits used.
- For information on how to document database searches and other search methods on your PRISMA flow diagram, visit our FAQs "How do I document database searches on my PRISMA flow diagram?" and "How do I document a grey literature search for my PRISMA flow diagram?"
Managing your review with Covidence
For this step of the review, in Covidence you can:
- Document searches in Covidence review settings so all team members can view
- Add keywords from your search to be highlighted in green or red while your team screens articles in your review settings
How a librarian can help with Step 3
When designing and conducting literature searches, a librarian can advise you on :
- How to create a search strategy with Boolean operators, database-specific syntax, subject headings, and appropriate keywords
- How to apply previously published systematic review search strategies to your current search
- How to test your search strategy's performance
- How to translate a search strategy from one database's preferred structure to another
The goal of a systematic retrieve is to find all results that are relevant to your topic. Because systematic review searches can be quite extensive and retrieve large numbers of results, an important aspect of systematic searching is limiting the number of irrelevant results that need to be screened. Librarians are experts trained in literature searching and systematic review methodology. Ask us a question or partner with a librarian to save time and improve the quality of your review. Our comparison chart detailing two tiers of partnership provides more information on how librarians can collaborate with and contribute to systematic review teams.

Search Process
- Use controlled vocabulary, if applicable
- Include synonyms/keyword terms
- Choose databases, websites, and/or registries to search
- Translate to other databases
- Search using other methods (e.g. hand searching)
- Validate and peer review the search
Databases can be multidisciplinary or subject specific. Choose the best databases for your research question. Databases index various journals, so in order to be comprehensive, it is important to search multiple databases when conducting a systematic review. Consider searching databases with more diverse or global coverage (i.e., Global Index Medicus) when appropriate. A list of frequently used databases is provided below. You can access UNC Libraries' full listing of databases on the HSL website (arranged alphabetically or by subject ).
Generally speaking, when literature searching, you are not searching the full-text article. Instead, you are searching certain citation data fields, like title, abstract, keyword, controlled vocabulary terms, and more. When developing a literature search, a good place to start is to identify searchable concepts of the research question, and then expand by adding other terms to describe those concepts. Read below for more information and examples on how to develop a literature search, as well as find tips and tricks for developing more comprehensive searches.
Identify search concepts and terms for each
Start by identifying the main concepts of your research question. If unsure, try using a question framework to help identify the main searchable concepts. PICO is one example of a question framework and is used specifically for clinical questions. If your research question doesn't fit into the PICO model well, view other examples of question frameworks and try another!
View our example in PICO format
Question: for patients 65 years and older, does an influenza vaccine reduce the future risk of pneumonia, controlled vocabulary.
Controlled vocabulary is a set of terminology assigned to citations to describe the content of each reference. Searching with controlled vocabulary can improve the relevancy of search results. Many databases assign controlled vocabulary terms to citations, but their naming schema is often specific to each database. For example, the controlled vocabulary system searchable via PubMed is MeSH, or Medical Subject Headings. More information on searching MeSH can be found on the HSL PubMed Ten Tips Legacy Guide .
Note: Controlled vocabulary may be outdated, and some databases allow users to submit requests to update terminology.
View Controlled Vocabulary for our example PICO
As mentioned above, databases with controlled vocabulary often use their own unique system. A listing of controlled vocabulary systems by database is shown below.
Keyword Terms
Not all citations are indexed with controlled vocabulary terms, however, so it is important to combine controlled vocabulary searches with keyword, or text word, searches.
Authors often write about the same topic in varied ways and it is important to add these terms to your search in order to capture most of the literature. For example, consider these elements when developing a list of keyword terms for each concept:
- American versus British spelling
- hyphenated terms
- quality of life
- satisfaction
- vaccination
- influenza vaccination
There are several resources to consider when searching for synonyms. Scan the results of preliminary searches to identify additional terms. Look for synonyms, word variations, and other possibilities in Wikipedia, other encyclopedias or dictionaries, and databases. For example, PubChem lists additional drug names and chemical compounds.
Display Controlled Vocabulary and Keywords for our example PICO
Combining controlled vocabulary and text words in PubMed would look like this:
"Influenza Vaccines"[Mesh] OR "influenza vaccine" OR "influenza vaccines" OR "flu vaccine" OR "flu vaccines" OR "flu shot" OR "flu shots" OR "influenza virus vaccine" OR "influenza virus vaccines"
Social and cultural norms have been rapidly changing around the world. This has led to changes in the vocabulary used, such as when describing people or populations. Library and research terminology changes more slowly, and therefore can be considered outdated, unacceptable, or overly clinical for use in conversation or writing.
For our example with people 65 years and older, APA Style Guidelines recommend that researchers use terms like “older adults” and “older persons” and forgo terms like “senior citizens” and “elderly” that connote stereotypes. While these are current recommendations, researchers will recognize that terms like “elderly” have previously been used in the literature. Therefore, removing these terms from the search strategy may result in missed relevant articles.
Research teams need to discuss current and outdated terminology and decide which terms to include in the search to be as comprehensive as possible. The research team or a librarian can search for currently preferred terms in glossaries, dictionaries, published guidelines, and governmental or organizational websites. The University of Michigan Library provides suggested wording to use in the methods section when antiquated, non-standard, exclusionary, or potentially offensive terms are included in the search.
Check the methods sections or supplementary materials of published systematic reviews for search strategies to see what terminology they used. This can help inform your search strategy by using MeSH terms or keywords you may not have thought of. However, be aware that search strategies will differ in their comprehensiveness.
You can also run a preliminary search for your topic, sort the results by Relevance or Best Match, and skim through titles and abstracts to identify terminology from relevant articles that you should include in your search strategy.
Nesting is a term that describes organizing search terms inside parentheses. This is important because, just like their function in math, commands inside a set of parentheses occur first. Parentheses let the database know in which order terms should be combined.
Always combine terms for a single concept inside a parentheses set. For example:
( "Influenza Vaccines"[Mesh] OR "influenza vaccine" OR "influenza vaccines" OR "flu vaccine" OR "flu vaccines" OR "flu shot" OR "flu shots" OR "influenza virus vaccine" OR "influenza virus vaccines" )
Additionally, you may nest a subset of terms for a concept inside a larger parentheses set, as seen below. Pay careful attention to the number of parenthesis sets and ensure they are matched, meaning for every open parentheses you also have a closed one.
( "Influenza Vaccines"[Mesh] OR "influenza vaccine" OR "influenza vaccines" OR "flu vaccine" OR "flu vaccines" OR "flu shot" OR "flu shots" OR "influenza virus vaccine" OR "influenza virus vaccines" OR (( flu OR influenza ) AND ( vaccine OR vaccines OR vaccination OR immunization )))
Boolean operators
Boolean operators are used to combine terms in literature searches. Searches are typically organized using the Boolean operators OR or AND. OR is used to combine search terms for the same concept (i.e., influenza vaccine). AND is used to combine different concepts (i.e., influenza vaccine AND older adults AND pneumonia). An example of how Boolean operators can affect search retrieval is shown below. Using AND to combine the three concepts will only retrieve results where all are present. Using OR to combine the concepts will retrieve results that use all separately or together. It is important to note that, generally speaking, when you are performing a literature search you are only searching the title, abstract, keywords and other citation data. You are not searching the full-text of the articles.
The last major element to consider when building systematic literature searches are field tags. Field tags tell the database exactly where to search. For example, you can use a field tag to tell a database to search for a term in just the title, the title and abstract, and more. Just like with controlled vocabulary, field tag commands are different for every database.
If you do not manually apply field tags to your search, most databases will automatically search in a set of citation data points. Databases may also overwrite your search with algorithms if you do not apply field tags. For systematic review searching, best practice is to apply field tags to each term for reproducibility.
For example:
("Influenza Vaccines"[Mesh] OR "influenza vaccine"[tw] OR "influenza vaccines"[tw] OR "flu vaccine"[tw] OR "flu vaccines"[tw] OR "flu shot"[tw] OR "flu shots"[tw] OR "influenza virus vaccine"[tw] OR "influenza virus vaccines"[tw] OR ((flu[tw] OR influenza[tw]) AND (vaccine[tw] OR vaccines[tw] OR vaccination[tw] OR immunization[tw])))
View field tags for several health databases
For more information about how to use a variety of databases, check out our guides on searching.
- Searching PubMed guide Guide to searching Medline via the PubMed database
- Searching Embase guide Guide to searching Embase via embase.com
- Searching Scopus guide Guide to searching Scopus via scopus.com
- Searching EBSCO Databases guide Guide to searching CINAHL, PsycInfo, Global Health, & other databases via EBSCO
Combining search elements together
Organizational structure of literature searches is very important. Specifically, how terms are grouped (or nested) and combined with Boolean operators will drastically impact search results. These commands tell databases exactly how to combine terms together, and if done incorrectly or inefficiently, search results returned may be too broad or irrelevant.
For example, in PubMed:
(influenza OR flu) AND vaccine is a properly combined search and it produces around 50,000 results.
influenza OR flu AND vaccine is not properly combined. Databases may read it as everything about influenza OR everything about (flu AND vaccine), which would produce more results than needed.
We recommend one or more of the following:
- put all your synonyms together inside a set of parentheses, then put AND between the closing parenthesis of one set and the opening parenthesis of the next set
- use a separate search box for each set of synonyms
- run each set of synonyms as a separate search, and then combine all your searches
- ask a librarian if your search produces too many or too few results
View the proper way to combine MeSH terms and Keywords for our example PICO
Question: for patients 65 years and older, does an influenza vaccine reduce the future risk of pneumonia , translating search strategies to other databases.
Databases often use their own set of terminology and syntax. When searching multiple databases, you need to adjust the search slightly to retrieve comparable results. Our sections on Controlled Vocabulary and Field Tags have information on how to build searches in different databases. Resources to help with this process are listed below.
- Polyglot search A tool to translate a PubMed or Ovid search to other databases
- Search Translation Resources (Cornell) A listing of resources for search translation from Cornell University
- Advanced Searching Techniques (King's College London) A collection of advanced searching techniques from King's College London
Other searching methods
Hand searching.
Literature searches can be supplemented by hand searching. One of the most popular ways this is done with systematic reviews is by searching the reference list and citing articles of studies included in the review. Another method is manually browsing key journals in your field to make sure no relevant articles were missed. Other sources that may be considered for hand searching include: clinical trial registries, white papers and other reports, pharmaceutical or other corporate reports, conference proceedings, theses and dissertations, or professional association guidelines.
Searching grey literature
Grey literature typically refers to literature not published in a traditional manner and often not retrievable through large databases and other popular resources. Grey literature should be searched for inclusion in systematic reviews in order to reduce bias and increase thoroughness. There are several databases specific to grey literature that can be searched.
- Open Grey Grey literature for Europe
- OAIster A union catalog of millions of records representing open access resources from collections worldwide
- Grey Matters: a practical tool for searching health-related grey literature (CADTH) From CADTH, the Canadian Agency for Drugs and Technologies in Health, Grey Matters is a practical tool for searching health-related grey literature. The MS Word document covers a grey literature checklist, including national and international health technology assessment (HTA) web sites, drug and device regulatory agencies, clinical trial registries, health economics resources, Canadian health prevalence or incidence databases, and drug formulary web sites.
- Duke Medical Center Library: Searching for Grey Literature A good online compilation of resources by the Duke Medical Center Library.
Systematic review quality is highly dependent on the literature search(es) used to identify studies. To follow best practices for reporting search strategies, as well as increase reproducibility and transparency, document various elements of the literature search for your review. To make this process more clear, a statement and checklist for reporting literature searches has been developed and and can be found below.
- PRISMA-S: Reporting Literature Searches in Systematic Reviews
- Section 4.5 Cochrane Handbook - Documenting and reporting the search process
At a minimum, document and report certain elements, such as databases searched, including name (i.e., Scopus) and platform (i.e. Elsevier), websites, registries, and grey literature searched. In addition, this also may include citation searching and reaching out to experts in the field. Search strategies used in each database or source should be documented, along with any filters or limits, and dates searched. If a search has been updated or was built upon previous work, that should be noted as well. It is also helpful to document which search terms have been tested and decisions made for term inclusion or exclusion by the team. Last, any peer review process should be stated as well as the total number of records identified from each source and how deduplication was handled.
If you have a librarian on your team who is creating and running the searches, they will handle the search documentation.
You can document search strategies in word processing software you are familiar with like Microsoft Word or Excel, or Google Docs or Sheets. A template, and separate example file, is provided below for convenience.
- Search Strategy Documentation Template
- Search Strategy Documentation Example
*Some databases like PubMed are being continually updated with new technology and algorithms. This means that searches may retrieve different results than when originally run, even with the same filters, date limits, etc.
When you decide to update a systematic review search, there are two ways of identifying new articles:
1. rerun the original search strategy without any changes. .
Rerun the original search strategy without making any changes. Import the results into your citation manager, and remove all articles duplicated from the original set of search results.
2. Rerun the original search strategy and add an entry date filter.
Rerun the original search strategy and add a date filter for when the article was added to the database ( not the publication date). An entry date filter will find any articles added to the results since you last ran the search, unlike a publication date filter, which would only find more recent articles.
Some examples of entry date filters for articles entered since December 31, 2021 are:
- PubMed: AND ("2021/12/31"[EDAT] : "3000"[EDAT])
- Embase: AND [31-12-2021]/sd
- CINAHL: AND EM 20211231-20231231
- PsycInfo: AND RD 20211231-20231231
- Scopus: AND LOAD-DATE AFT 20211231
Your PRISMA flow diagram
For more information about updating the PRISMA flow diagram for your systematic review, see the information on filling out a PRISMA flow diagram for review updates on the Step 8: Write the Review page of the guide.
- << Previous: Step 2: Develop a Protocol
- Next: Step 4: Manage Citations >>
- Last Updated: Mar 28, 2024 9:43 AM
- URL: https://guides.lib.unc.edu/systematic-reviews
Search & Find
- E-Research by Discipline
- More Search & Find
Places & Spaces
- Places to Study
- Book a Study Room
- Printers, Scanners, & Computers
- More Places & Spaces
- Borrowing & Circulation
- Request a Title for Purchase
- Schedule Instruction Session
- More Services
Support & Guides
- Course Reserves
- Research Guides
- Citing & Writing
- More Support & Guides
- Mission Statement
- Diversity Statement
- Staff Directory
- Job Opportunities
- Give to the Libraries
- News & Exhibits
- Reckoning Initiative
- More About Us

- Search This Site
- Privacy Policy
- Accessibility
- Give Us Your Feedback
- 208 Raleigh Street CB #3916
- Chapel Hill, NC 27515-8890
- 919-962-1053

- Hirsh Health Sciences
- Lilly Music
- Webster Veterinary
- Hirsh Health Sciences Library
NUTR 369: Systematic Reviews
- Controlled Vocabularies
- Search Strategies
- Databases & More
- Keyword Searching
- Citation Management
What is a Controlled Vocabulary?
“… a carefully selected list of words and phrases , which are used to tag units of information (document or work) so that they may be more easily retrieved by a search…Controlled vocabularies reduce ambiguity inherent in normal human languages where the same concept can be given different names and ensure consistency.” -Wikipedia
- Created and edited by a "governing body" to introduce new official terms, phase out old ones, and add new mapping for concepts
- Reduces the burden on researchers of a database by mapping human language synonyms to the official term
- Searching by a concept or idea, rather than by the presence of a string of letters
Other Controlled Vocabularies
There are thousands of thesauri and controlled vocabularies. Often there are multiple available for one general topic area. The following examples will give you a small idea of the variation and scope of controlled vocabularies.
- National Agriculture Library Thesaurus
- Getty Thesaurus of Geographic Names
- The Thesaurus of Graphic Materials
- Comprehensive Subject Index - EBSCO
- Thesaurus of ERIC Descriptors
- Art and Architecture Thesaurus
- Union List of Artist Names
- << Previous: Databases & More
- Next: Keyword Searching >>
Medical Subject Headings in PubMed
The Medical Subject Headings (MeSH) is one example of a controlled vocabulary. It can be used while searching the MEDLINE database via PubMed or OVID.
- Created and maintained by the National Library of Medicine
- Subject headings are applied to every article indexed in PubMed to identify key topics of the publication
- Reduces need to think of synonyms and natural language descriptions
- MeSH database allows you to search for the controlled term using keywords and build a search to use in PubMed
An Introduction to Medical Subject Headings from Tufts HHSL on Vimeo .
- Last Updated: Apr 11, 2023 2:17 PM
- URL: https://researchguides.library.tufts.edu/nutr369
- Locations and Hours
- UCLA Library
- Research Guides
- Biomedical Library Guides
Systematic Reviews
- Creating the Search
- Types of Literature Reviews
- Planning Your Systematic Review
- Database Searching
Before You Start
Step 1: structure your concepts, step 2: brainstorm keywords for each concept, step 3: determine appropriate controlled vocabulary terms, step 4: put it all together, step 5: refine your strategy.
- Search Filters and Hedges
- Grey Literature
- Managing and Appraising Results
- Further Resources
Explicitly state your research question, determine which databases you will search, and determine your inclusion/exclusion criteria for studies that you find. Here is some information on writing a protocol for your systematic review study . You might want to search PROSPERO , a database of protocols, to make sure that no one else is currently working on a review on the same topic. You can also submit your protocol to PROSPERO.
- Break down your research question into smaller concepts in order to make the next few steps manageable.
- Now we want to fill out the other columns on this table. The next step is to figure out any potential synonyms or conceptually equivalent ideas for each of our concepts. This will help us perform an exhaustive search using all possible terminology. If you have already identified any papers that you plan to include, it's helpful to scan their abstracts for any obvious keywords that should be included. Don't forget that there may be spelling variants (estrogen vs. oestrogen)!
- You can truncate words to retrieve multiple word endings. The truncation symbol is usually an asterisk (*) but can sometimes be another character - check the database's Help documentation to determine what it is for any particular database. For example, inflam* will retrieve the following keywords in the results: inflammation, inflammatory, inflamed, inflaming...etc. This can be helpful when searching for plurals.
- You can phrase search for exact phrases. For example, searching "post menopausal" in quotes will retrieve different results than searching post menopausal . Without quotes, most databases will look for the word post anywhere in the record and the word menopausal anywhere in the record, which may result in unwanted results. With quotes, you'll only see the exact phrase "post menopausal."

- At this point you'll need to decide which database you are going to search first and complete your search strategy using only that database's syntax. It can be a little confusing to try to switch back and forth between databases while still trying to finalize your search. For our example we will proceed using PubMed, but the techniques are the same in any database that offers controlled vocabulary searching.
- In PubMed, the controlled vocabulary is called MeSH. Here is a tutorial that explains how powerful and functional MeSH is . I recommend completing this tutorial to have a good understanding of the next few steps.
- Now that you've been introduced to MeSH, let's put it to work for our sample search. We're going to search for each of our concepts in the MeSH database to determine the appropriate MeSH heading for each of them. Now is also a good time to go back to any papers that you've already identified as relevant and see if there are any MeSH terms on those papers that you might want to use (to find the MeSH terms, search the papers in PubMed and look at the bottom of each article record).
- In the MeSH database, search for your concepts. Click on any relevant terms. From the MeSH database record, you can click "Add to Search Builder" to add the term into the PubMed search box. Then you can copy and paste it into your table.
- Note that this behavior of including narrower terms is usually called "explosion." MeSH explodes by default, but other controlled vocabularies might not. Look for the option to explode in other databases.
- For databases that do not use controlled vocabularies (such as Web of Science), you can search with only the keyword column.
- Now we are ready to construct a search using all the terms we've listed in the table. Since we put each concept on its own row, all the terms in the same row are conceptually equivalent, so we will combine them with the Boolean operator OR. You can do this in the PubMed Advanced search builder (or any database search builder if you're not in PubMed), or you can type it out. Even if the database allows you to save search strategies, it's good to document the searches in a file separately from the database.
- At this point you will want to determine which field you want to use to search the words you've listed in the Keywords column. This will differ depending on the database. For PubMed, you will probably want to use either the Title/Abstract field (which searches those two fields) or the Text Word field (which searches title, abstract, MeSH terms and subheadings, and chemical substance names). We'll proceed using Text Word for our keywords.
- ("post menopause"[Text Word] OR "post menopausal"[Text Word] OR postmenopaus*[Text Word] OR "Postmenopause"[Mesh]) AND ("hormone therapy"[Text Word] OR "hormone replacement therapy"[Text Word] OR estrogen[Text Word] OR oestrogen[Text Word] OR progesterone[Text Word] OR "Hormone Replacement Therapy"[Mesh]) AND (cardiovascular[Text Word] OR atherosclerosis[Text Word] OR hypertension[Text Word] OR "heart failure"[Text Word] OR arrythmia[Text Word] OR stroke[Text Word] OR "myocardial infarction"[Text Word] OR "heart attack"[Text Word] OR "Cardiovascular Diseases"[Mesh])
Here are some techniques you can use to improve your search results
- Go back to your protocol. Can you apply any of your inclusion and exclusion criteria to your search, for instance, English-only papers or a particular date limit?

- Sort your results by relevance and try to identify any particular relevant papers in the first few pages of results. If you find any good ones, scan their abstracts and MeSH terms to make sure you didn't miss anything important from your own search strategy.
- Sort your results by date of publication and try to identify any particularly irrelevant papers. If you are seeing thousands of results that are utterly off topic, click into them and see if you can figure out why they are coming up. Some common issues include: a broad MeSH term exploding to include very irrelevant terms, keywords that are too broad and used too commonly by authors, or unexpected results of truncation. In PubMed, you can always look at the "search details" box on the results page to see how PubMed translated your search commands.
- << Previous: Database Searching
- Next: Search Filters and Hedges >>
- Last Updated: Apr 17, 2024 2:02 PM
- URL: https://guides.library.ucla.edu/systematicreviews

Module 2: Formulating a Research Question and Searching for Sources
Related Keywords
Your search strategy can contain your main keywords , similar or related keywords and controlled terms (also called subject headings ).
Searching by a keyword will retrieve resources where the author(s) used that specific term. For this specific reason, you should also brainstorm similar or related keywords to incorporate into your search.
Methods for Identifying More Keywords
You can identify more keywords in multiple ways. Below are two methods.
Method 1: Use a Concept Model or Map
To use this method:
- Write your research topic or question, along with any ideas and concepts associated with it on a blank sheet of paper.
- Use themes to group your ideas, and connect related concepts using lines.
- Brand names and generic names
- Variation in spelling (e.g. “paediatric” or “pediatric”)
Please see Figure 2.1 below for an example where your research question is “How effective is cognitive behavioral therapy in improving mild-to-moderate depression in adolescents?”
Method 2: Use Your Main Keywords in a Database
- Locate an article on your topic.
- Scan the title, abstract, and author keywords to identify more keywords (see Figure 2.2 below) to use in your search.
Key Takeaways
Some databases like Ovid MEDLINE, PubMed and EBSCO CINAHL use controlled vocabularies like MeSH as well as keywords. The next section will explain what controlled vocabularies are and how to use them. If you know you are going to use a database with controlled vocabularies, please check out the next section.
Learning Activity
Brainstorm more concepts
Advanced Research Skills: Conducting Literature and Systematic Reviews Copyright © 2021 by Kelly Dermody; Cecile Farnum; Daniel Jakubek; Jo-Anne Petropoulos; Jane Schmidt; and Reece Steinberg is licensed under a Creative Commons Attribution 4.0 International License , except where otherwise noted.
Share This Book

An official website of the United States government
The .gov means it’s official. Federal government websites often end in .gov or .mil. Before sharing sensitive information, make sure you’re on a federal government site.
The site is secure. The https:// ensures that you are connecting to the official website and that any information you provide is encrypted and transmitted securely.
- Publications
- Account settings
Preview improvements coming to the PMC website in October 2024. Learn More or Try it out now .
- Advanced Search
- Journal List
- J Med Libr Assoc
- v.110(1); 2022 Jan 1
MeSH and text-word search strategies: precision, recall, and their implications for library instruction
Michelle m. demars.
1 [email protected] , Health Sciences Librarian, California State University, Long Beach, Long Beach, CA
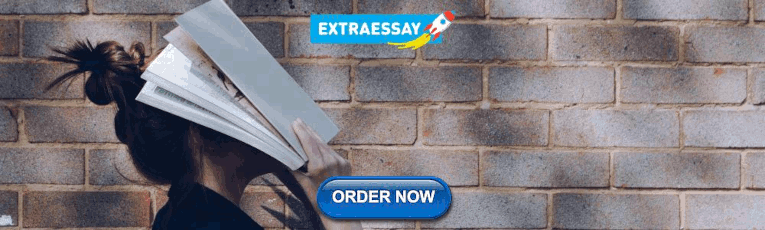
Carol Perruso
2 [email protected] , Associate Librarian, California State University, Long Beach, Long Beach, CA
Associated Data
Data associated with this article are available in ScholarWorks at http://hdl.handle.net/20.500.12680/kk91fr54x .
This study compared the recall and precision of MeSH-term versus text-word searching to better understand psychosocial MeSH terms and to provide guidance on whether to include both strategies in an information literacy session or how much time should be spent on teaching each search strategy.
Using the relevant recall method, a total of 3,162 resources were considered and evaluated to form a gold standard set of 1,521 relevant resources. We compared resources discussing psychosocial aspects of children and adolescents living with type 1 diabetes using two search strategies: text-word strategy versus MeSH-term strategy. The frequency of MeSH terms, the MeSH hierarchy, and elements of each search strategy were also examined.
Using the 1,521 relevant articles, we found that the text-word search strategy had 54% recall, while the MeSH-term strategy had 75% recall. Also, the precision of the text-word strategy was 34.4%, while the precision of the MeSH-term strategy was 47.7%. Therefore, the MeSH-term search strategy yielded both greater recall and greater precision. The MeSH strategy was also more complicated in design and usage than the text-word strategy.
Conclusions:
This study demonstrates the effectiveness of text-word and MeSH search strategies on precision and recall. The combination of text-word and MeSH strategies is recommended to achieve the most comprehensive results. These results support the idea that MeSH or a similar controlled vocabulary should be taught to experienced and knowledgeable students and practitioners who require a myriad of resources for their literature searches.
INTRODUCTION
For clinicians, health librarians, and students alike, conducting effective and efficient literature searches is an important part of evidence-based medicine (EBM). Sackett, who is considered one of the pioneers of evidence-based medicine, defines EBM as “the conscientious, explicit, and judicious use of current best evidence in making decisions about the care of individual patients” [ 1 ]. It is this desire to effectively and safely treat patients, using well-documented methods, that has health practitioners and health students in a continuous search for the best evidence and most relevant literature. EBM recommends consulting articles on randomized controlled trials or systematic reviews. This need for specific types of literature can influence a search strategy and adds to the skills needed to accomplish a successful search.
Effective searching to find relevant literature is a complex skill that is gradually learned and goes beyond many of the databases undergraduates are introduced to in their general education classes and well beyond Google or Google Scholar [ 2 ]. Nor is it something that can be mastered in a one-shot information literacy session. It requires more than a basic knowledge of common medical databases, each with different interfaces and controlled vocabularies. Information literacy competence for nursing students, as defined by the Association of College & Research Libraries, includes five standards with more than 130 outcomes and skills to be mastered. Addressing these standards, while dealing with the wide variety of skills students bring to the one or two sessions incorporated in their undergraduate studies, requires significant prioritizing. Librarians' hope is that students learn how, as practitioners, they can conduct effective searches as they pursue the goal of finding “as much information as is available on a specific topic and … as few articles as possible that are unrelated to the search topic” without becoming overwhelmed [ 3 ].
A health sciences librarian who teaches students to conduct literature searches frequently weighs whether to include both strategies or how much time should be spent teaching text-word searching versus subject heading searching. While working with two researchers, the question of the most effective search strategy surfaced, leading us to the present study comparing the precision and recall of Medical Subject Headings (MeSH) and text-word searching in PubMed and three other databases that use a similar controlled vocabulary, though the tree below each term may vary.
Even though many researchers have tackled this instructional challenge, with “evidence to suggest a positive relationship between library instruction and information literacy skill development” and multiple recommendations for “sustained training and support across year levels,” we could find no studies that specifically address teaching students the intricacies of selecting the best MeSH terms, a skill that taxes even experienced academic librarians and has generated a plethora of skills tutorials [ 4 – 13 ].
Yet nursing and other health care faculty naturally want students to learn the language and landscape of the medical literature, including MeSH. Such a request from a nursing professor, combined with helping two researchers with a scoping review, led to the current study. One of the aims of this study was to test whether MeSH or text-word searching (or a combination) was most effective in researching psychosocial phenomena in adolescents. The other goal, which involved converting text-words into comparable MeSH terms, was to observe and document our own process with an eye toward balancing the teaching of text words and MeSH or a similar controlled vocabulary in undergraduate and graduate information literacy instruction. For the first goal, we used recall and precision, two long-standing bibliometric measurements of the effectiveness of search strategies that continue to be used, even as advances in automated text retrieval have produced other evaluation methods [ 14 – 20 ].
Recall is defined “as the number of relevant citations retrieved by a search divided by the number of relevant citations” [ 21 ]. For example, if a search retrieves 100 documents, 75 of which are relevant to the research question, but misses another 25 relevant documents, then the recall of the search (75 retrieved relevant documents/125 total relevant documents) is 60%. Researchers strive to retrieve the most relevant articles possible without missing any important resources [ 22 ]. The benefit to higher recall is the breadth of coverage, while the challenge lies in the time required to examine each result. (Sensitivity can be an alternative term for recall when evaluating information retrieval.) The other factor, precision, is defined as “the number of relevant citations retrieved divided by the total number of citations retrieved” [ 21 ]. Using the same example, if the search retrieves 100 documents, 75 of which are relevant to the research question, the search's precision (75 relevant documents/100 retrieved documents) is 75%. This factor represents fewer, more focused results, with the goal to gather few articles that are unrelated to the topic [ 3 ]. The benefit to high precision is the exactitude of the results and the time saved in evaluating them, with the challenge being potentially missing relevant articles.
Many elements can affect the precision and recall of a literature search, and a researcher's search strategy is an important part of the equation. Other elements include the quality, quantity, and relevance of the articles in chosen databases. The two primary strategies commonly used by researchers are text-words or keywords and subject headings from controlled vocabulary. While we consider text-words and keywords to be interchangeable, the medical research literature uses text-words almost exclusively. However, this is different from the PubMed field Text Word [TW], which searches “all words and numbers in the title, abstract, other abstract, MeSH terms, MeSH Subheadings, Publication Types, Substance Names, Personal Name as Subject, Corporate Author, Secondary Source, Comment/Correction Notes, and Other Terms” [ 23 ]. With text-word or keyword searching, researchers generate their own search terms based on their topic and their knowledge of the vocabulary used by the discipline. Text-words are often used as a “substitute for a subject search when [the searcher does] not know the standard subject heading” [ 24 ]. They may be used to search the full text or portions of the record, such as the title and abstract of an article. (In addition to MEDLINE citations, PubMed includes in-process and “ahead of print” citations yet to be indexed with MeSH, out-of-scope general science and general chemistry journals, some author manuscripts, and NCBI books [ 25 ].) Subject searching uses controlled vocabulary “from a predetermined list of possible terms [assigned to] reflect the content of the item” [ 23 ]. MeSH terms are an example of a controlled vocabulary assigned by the National Library of Medicine to the article citations in MEDLINE and most PubMed content, and a similar controlled vocabulary is also used in some form in several other databases, such as CINAHL. Research indicates that a combination of strategies is the best approach [ 3 , 22 , 26 , 27 , 28 ].
Specifically, this study compares these two methods while researching psychosocial factors in children and adolescents with type 1 diabetes. This particular topic was chosen because of the collaboration with two researchers, Bell (an assistant professor at California State University, Long Beach) and Hazel (a clinical social worker in the Division of Endocrinology at Boston Children's Hospital), and because researching psychosocial factors in PubMed can present greater challenges than searching biomedical terms, for which PubMed has specific search tools. Because it is a broad topic, yet typical of one that health science students would undertake, we believed that it lent itself to an investigation of the pluses and minuses of MeSH (or similar controlled vocabulary) and text-word strategies.
There is no shortage of literature discussing various aspects of MeSH, including search strategy differences by type of user and the use of MeSH for literature searches. The comparison of text-word versus controlled vocabulary, such as MeSH, has also been discussed in the literature for many years, as have the challenges MeSH and similar controlled vocabulary present, especially to inexperienced searchers. While the prevalent thinking is that a combination approach is best [ 3 , 22 , 26 , 27 , 28 ], going back to the mid-1990s, Lowe and Barnett recognized that MeSH was not frequently utilized by health care professionals because of its complicated nature and lack of availability to those outside the library field [ 21 ].
Haynes and colleagues added to this early discussion with their study on developing search strategies with a focus on MEDLINE [ 26 ]. Their study outlined the challenges of balancing precision and recall while developing a search strategy, and their results showed that precision and recall were enhanced by combining MeSH and text-word searching.
The conversation continued nearly a decade later with studies on otolaryngology and sleep. Both Jenuwine and Floyd and Chang, Heskett, and Davidson found that text-word searching produced a higher number of results but did not exclude irrelevant articles very well [ 22 , 27 ]. Both studies concluded that thorough researchers should use a combination of strategies, especially when a comprehensive and broad search is required, such as for a systematic review.
Comparisons of the usage of MeSH and text-word search strategies open the door to a deeper conversation about how MeSH is constructed and if an understanding of this structure will lead to searches that are both precise and comprehensive. Gault, Shultz, and Davies sought to compare the mapping of MeSH across a variety of interfaces including the MeSH Browser and OVID [ 29 ]. Their study revealed inconsistencies in the results of the MeSH term associated with the search term, depending on the interface used. They also found that the interface selected could affect the search results even if each was mapping to MeSH. Richter and Austin contributed to the discussion with a report reviewing how MeSH and text-words are used to search for literature in PubMed and how text-words are mapped to a MeSH term [ 28 ]. By using example searches from the field of physical therapy, the authors searched PubMed for both search terms and acronyms to determine if the item entered mapped to MeSH terms. Slightly less than half of the terms mapped appropriately, and the remaining terms mapped inappropriately or not at all. This issue emphasizes the benefits of text-word searching as an alternative or additive to MeSH searching.
Given the evidence that both search strategies have their merits, librarians are faced with the question of how much of their limited instruction time should be dedicated to teaching each search strategy. The struggle to use valuable instruction time on MeSH (or similar controlled vocabulary) led to discussions between a health science librarian and nursing faculty about the perceptions of how nursing students were grasping text-word and MeSH search strategies as demonstrated in their coursework and assignments. There were commonalities among faculty observations, especially as they compared search strategies among undergraduates and early master's of science in nursing students to those of more experienced graduate students and doctor of nursing practice (DNP) students. Nursing faculty find that undergraduates will seek out the “path of least resistance” when it comes to their literature searches, often depending on text-word searching as that is what they are most familiar with [N. Cheffer, email to M. DeMars, July 6, 2021]. One faculty member noted, “Most [undergraduate] students use CINAHL which requires an initial first step to choose MeSH searches … and therefore students tend to settle too quickly for the keyword searches.” Comparatively, nursing faculty noticed that more experienced students, such as those in the DNP program, many of whom are already nurse practitioners, are more likely to grasp the concepts of MeSH and use it more frequently in both PubMed and CINAHL. Also noted by faculty was this population's awareness of the benefits of a more comprehensive search strategy in relation to preparing a manuscript for publication: “[DNP students] aspire to publish and know that identifying the MeSH terms they used is evidence of more professional literature searches” [AJ Jadalla, email to M. DeMars, July 7, 2021].
These nursing faculty observations highlight important distinctions between the two student populations. Inexperienced and undergraduate students are less likely to embrace MeSH, as they are still working to grasp clinical concepts and are less likely to need to justify their search process. Experienced and doctoral students are more likely to welcome MeSH search strategies and may already be using them for their work or practice. Additionally, their drive to publish a manuscript as part of their rigorous academic coursework may have this population more willing to learn the intricacies of MeSH for their assignments. The complicated hierarchy and tree structure of MeSH is often overshadowed by the popularity of text-word or keyword searching with its ease of use and the speed of finding results. MeSH terms are complicated in comparison, especially to inexperienced researchers, and therefore may be left out of an instructional session by health science librarians. Health practitioners also experience difficulties with MeSH, with search errors commonly related to the MeSH mapping structure [ 30 ]. These various complexities call into question if the additional results outweigh the time expended.
For practitioners, finding all relevant research is important, but rarely are they able to take the time-consuming steps necessary to create a “gold standard” list of sources “of known relevance to the concept … which when considered cumulatively, should ideally represent the full scope of that concept” [ 31 ]. Research shows that it is not unusual for this process to take in excess of 100 hours to gather and requires an expert searcher even more than a domain expert [ 32 , 33 ]. Such a complete list of relevant research is also a necessary first step to measuring recall. Completeness is never perfect, as it is limited not only by time but also by the sources searched, the quality of the search strategy, as well as any subjective bias in the search strategy or from evaluators. Researchers have used combinations of processes, including hand searching relevant journals, mining systematic reviews, searching multiple databases, searching grey literature, checking cited references, and expert or other qualitative evaluation [ 3 , 19 , 31 , 34 , 35 ]. When a hand search is not practical, some researchers use a method called relative recall [ 31 , 36 , 37 ]. Relative recall combines “multiple exhaustive and high-quality searches across a broad range of sources, as well as a rigorous screening process based on clear eligibility criteria … [to] minimise the potential for bias” [ 31 ]. In contrast to recall, precision is a relatively straightforward measure, with accuracy dependent on the comprehensiveness of the search strategy and the time needed to review results to determine the proportion that are relevant.
For this study comparing the recall and precision of MeSH terms (or similar controlled vocabulary) versus text-word searching, the relevant-recall method was used to form the gold standard set of resources. Building upon the resources Bell and Hazel [ 38 ] found using a text-word-only Boolean strategy in nine databases (Academic Search Premier, CINAHL, Dissertations & Theses, Embase, Global Health, LWW Nursing, PsycInfo, PubMed, and Web of Science) ( Appendix B ), we created parallel MeSH-only Boolean searches of PubMed, CINAHL, Embase, and PsycInfo ( Appendix A ), which are four databases that include the option of searching with MeSH terms or a similar controlled vocabulary. (See Appendix C for a comparison.) The MeSH search builder was used in the version of PubMed launched in spring 2020 to generate the search string, thereby bypassing nonindexed records. Both search strategies aimed to identify relevant research defined by Bell and Hazel as studies that included instruments measuring “individual and family factors … related to self-perception, interpersonal factors, and individual responses” of youth living with type 1 diabetes [ 38 ]. Given the nature of the MeSH tree structure and the hierarchy of terms, the MeSH terms used for the searches also included any terms that were categorized below them in the MeSH tree [ 39 ]. The MeSH terms in this default setting allow for the inclusion of the term, plus some that are related, resulting in broad results related to that term, a method known as MeSH explosion. In addition to their text-word searches, Bell and Hazel mined 14 systematic reviews and references in relevant resources for additional sources, which were added to the combined set [ 38 ]. Otherwise, we did not conduct hand searches, although Bell and Hazel conducted a few. The combination of these strategies yielded 3,162 sources, with 1,375 coming from the text-word search plus reference mining and 1,787 coming from the MeSH-term (or similar controlled vocabulary) search.
To refine this collection of resources and to document our process, we created a master spreadsheet showing which database and search method yielded each source. Prior to eliminating any articles, we noted MeSH terms for each, where available. We conducted multiple levels of evaluation to achieve the final list, first of titles, then of abstracts, and finally evaluation of the full text of the remaining sources. We then eliminated overlap between text-word and MeSH-term search results, resulting in 2,378 unique, English-language sources published from January 1, 2010, to July 7, 2020, from the two search strategies. We added ten sources found using cited references or mining systematic reviews for a total of 2,388 sources.
To further refine this set of resources to keep only sources of “known relevance to the concept” of psychosocial factors facing children and adolescents with type 1 diabetes, we employed three methods:
- Reviewing MeSH terms for the articles, building upon the expertise of the National Library of Medicine, whose indexers assign the terms;
- Reviewing titles and abstracts; and
- Reviewing the list of sources that Bell and Hazel ultimately selected for their narrower study, along with the reasons that items were excluded [ 38 ].
For this process, we divided the list of 2,388 articles in half, with each author evaluating items independently, conferring with each other or with Bell and Hazel as needed. This more independent approach aimed to emulate the text-word approach and was possible because of Bell and Hazel's expertise with the topic and because their search-result evaluation happened first, giving us a greater level of confidence [ 38 ]. Adding to the rigor of the review was one of the authors' expertise as a health sciences librarian and experience as an assistant clinical research coordinator at a major medical center.
We started by eliminating ninety-eight articles without the MeSH terms or subject headings of “Diabetes Mellitus” or “Diabetes Mellitus, Type 1” or that had only “Diabetes Mellitus, Type 2.” In addition to relying on MeSH terms, we evaluated article titles and/or abstracts. Only four of those eliminated came from the MeSH-only search strategy. Next, we eliminated seventy-two articles that did not have an “Adolescent” or “Child” assigned MeSH term. In addition, we reviewed article titles, abstracts, or the full text to confirm that the studies were about only adults. Studies about adults were kept if they also had “Adolescent” or “Child” MeSH terms or if the studies included participants in both age groups. Sources were only discarded if both authors agreed. We then evaluated the remaining 2,218 articles to determine whether they were relevant to psychosocial factors affecting children and adolescents with type 1 diabetes. Before doing this, we reviewed MeSH terms assigned to these articles to determine whether we needed to expand the number of psychosocial MeSH terms beyond the 23 terms used in our Boolean search. We added any MeSH terms with a subheading of “Psychology” (i.e., “/Psychology”) plus 13 terms that were assigned to relevant articles and that could have improved the original Boolean search strategy ( Table 1 ). For this step, 697 articles without at least one of the psychosocial MeSH terms on the list were eliminated. This left 1,521 articles and dissertations in the gold standard list. It also provided us with the relevant sources organized by database to measure the precision of two search strategies ( Figure 1 ).
MeSH terms used to evaluate source relevance

Flowchart of evaluation process
The amount of time this process took would weigh heavily on practitioners and students. Similar to the amount of time for such searching found by others, the two authors (as well as Bell and Hazel) each spent in excess of 100 hours before reaching their final lists. Even with a well-constructed search combining the two methods, evaluating results would have consumed well more than 100 hours [ 33 ].
Of the 1,521 relevant articles and dissertations, 372 were found only using the text-word search strategy, 692 were found only using the MeSH-term strategy, and 450 were found with both strategies. An additional seven results were found only by mining citations ( Figure 2 ). Using the 1,521 relevant articles and dissertations as the denominator for the recall formula used by Ting [ 40 ], we found that the text-word search strategy had 54% recall (822 retrieved/1,521 relevant sources), while the MeSH-term strategy had 75% recall (1,139 retrieved/1,521 relevant sources).

Relationship of sources found by each method
To measure the precision of each method, we used Ting's method and divided the number of sources retrieved by each method by the total number of sources retrieved by the two search methods combined [ 40 ]. For this step, we removed eight sources found manually. Thus, the precision of the text-word strategy was 34.4% (822 relevant sources retrieved/2,388 total unique sources), while the precision of the MeSH-term strategy was 47.7% (1,139 relevant sources retrieved/2,388 unique sources). Therefore, the MeSH-term search strategy yielded both greater recall and greater precision.
The disparity widened when we compared the two search strategies for the 1,367 articles that appeared in the only freely available database, PubMed. The text-word strategy yielded 49.8% of the articles, while the MeSH-term strategy produced 81.4%. The greater recall and greater precision may have been influenced by the automatic explosion of the MeSH terms.
However, despite higher recall and precision for the MeSH-term strategy, there were 236 sources in the gold standard set (15.5%) that had no MeSH terms assigned or were not indexed in PubMed and were only found using the MeSH-term strategy in another database. All but twenty-two of those could be discovered only using the text-word strategy or through reference mining.
Furthermore, we calculated the recall of each search strategy for the four databases. The MeSH-term strategy yielded:
- 60.2% recall in PubMed (916 of 1,521 relevant sources)
- 24% recall in Embase (365 sources)
- 17.8% recall in CINAHL (269 sources)
- 11.6% recall in PsycInfo (177 sources)
The text-word search strategy yielded:
- 34.2% recall in Embase (520 of 1,521 relevant sources)
- 32.8% recall in PubMed (499 sources)
- 28.5% recall in CINAHL (433 sources)
- 19.9% recall in PsycInfo (303 sources)
- 8.9% recall in Academic Search Premier (136 sources)
The MeSH term strategy was most effective in PubMed, while Embase and PubMed were nearly tied in the text-word strategy. For PsycInfo and CINAHL, the text-word strategy was more effective than the MeSH-term strategy. Also of note:
- Eighty-five sources appeared in PubMed that neither search strategy located but were found in other databases.
- The MeSH-term Boolean strategy in PubMed missed 135 sources with appropriate MeSH terms. Of these, all but nineteen were found using the text-word strategy.
- The MeSH-term Boolean strategy yielded 287 sources in PubMed that had none of the MeSH terms used in the strategy. Of these, the text-word strategy missed 229.
Finally, we examined the most frequent MeSH terms and concepts to aid in future search strategies. For this, we grouped a few similar terms. “Diabetes Mellitus, Type 1/Psychology” was overwhelmingly the most frequent, assigned to 821 of the 1,281 articles with MeSH terms in PubMed. (“Diabetes Mellitus, Type 1” was included in the MeSH-term strategy, but this count is only for those with the subheading “Psychology.”) Among the top 40 concepts, several frequently assigned terms of note were not part of the MeSH-term search strategy ( Figure 3 ).

Frequency of assigned MeSH terms
The results of this study reaffirm that the MeSH-term search strategy yields both greater recall and greater precision. Therefore, even though the difficulty level of using MeSH (or similar controlled vocabulary) to search for literature may be higher, there is a substantial benefit to using MeSH as part of an effective search strategy. The benefit is especially pronounced when a researcher is conducting an exhaustive literature search, as would be the case for a systematic review. The search results that MeSH provides can support the needs of health practitioners looking to find high-quality evidence as part of their EBM practices and students seeking publication. This study also supports the prevalent thinking that using a combination of MeSH and text-word strategies is beneficial. Although the MeSH strategy provided high precision and recall, it missed a substantial number of resources in PubMed (15.5% in this study) as not all sources were indexed using MeSH terms, either because they were not selected for indexing or because of the time needed for indexing [ 41 ]. Additionally, we found that for at least two important databases (CINAHL and PsycInfo), text-word searching was more effective. Therefore, the best practice would include a text-word search to catch any resources that would otherwise be missed because of their lack of indexing and to add a search of non-MeSH databases to catch any additional resources. Many combinations can accomplish gathering both indexed and nonindexed literature. One strategy is the combination of MeSH and text-words in a singular search [ 35 ], and another is performing two separate searches—one MeSH and one text-word—and then combining the results. If researchers are performing a quick search or only require a singular article for an assignment, then integrating MeSH may not be as important. However, if they are doing extensive and comprehensive searches such as systematic reviews, adding MeSH will provide greater coverage.
Even with the demonstrated benefits of MeSH (or similar controlled vocabulary) and the recommendations of a combined MeSH and text-word approach, a medical or health sciences librarian should consider the needs of their student population when including basic MeSH strategies in their curriculum. Librarians should consider the experience level of the students they will be teaching and the publishing goals of the students. Those who are considering publication may be drawn to MeSH as a search strategy to strengthen their manuscript. As highlighted by the observations of nursing faculty, for beginners and undergraduate students, learning the complexities of MeSH may prove to be too time-consuming and may even hit some resistance from this population, especially considering the likelihood that their assignments would not require more than a few relevant articles. One strategy tested with some success in a Canadian nursing program ramped up information literacy instruction over three years by teaching basic CINAHL searching and popular and scholarly literature differences in year one, advanced CINAHL searching and critical website evaluation in year two, and formulating a research question and searching PubMed using both MeSH and clinical queries in year three [ 4 ].
For doctoral and experienced graduate students, however, a librarian may consider MeSH to be an essential component of their research curriculum. By focusing on the basics of MeSH terms and search strategies, a medical or health sciences librarian can provide students and current and future practitioners with a beneficial edge to their research strategies [ 42 ]. It is our recommendation that the basics should include not only skills-based information but also an introduction to the structure, mapping, and functionality of MeSH, as the intricacies of MeSH hierarchies can impact their effectiveness as a search strategy. For example, if a text-word term is searched in PubMed, one can explore how the database interprets and maps that term by exploring the history and search details sections. This area of the interface allows researchers to evaluate the MeSH terms associated with their text-word terms without directly interacting with the MeSH search tool, helping students understand the structure of MeSH terms in a way that is familiar. From a teaching perspective, this strategy is quick to demonstrate and easy to integrate into the librarian's curriculum, making it an appropriate introduction to the strategy. By providing experienced searchers with instruction in MeSH, librarians can provide them with a more comprehensive search approach that will support assignments and future manuscript publication opportunities. Instructors should also advise graduate students of the importance of combining text-word and MeSH-term strategies, as research repeatedly recommends.
Overall, this study demonstrates the impact of text-word and MeSH search strategies on the precision and recall of search results and hence their importance to instruction. The combination of text-word and MeSH strategies provides the most comprehensive results, and the use of MeSH provides the most precise results. However, the complexities of MeSH and skills needed to master it may only be needed by experienced and knowledgeable students and practitioners who require a myriad of resources for their research. Additionally, by exploring diabetes, a topic that many health sciences students choose to write about, and one for which we could find no previous study of recall and precision, the hope was to assist future researchers in this important field of adolescent health. The recent welcome addition of two MeSH terms, “Psychosocial Intervention” and “Psychosocial Functioning” should make future research much easier.
Several limitations should be considered regarding this study. It should be noted that our research was biased toward the Bell and Hazel approach as it was their desire to generate a scoping review that aided in propelling this study forward. This may have influenced the fact that only fourteen of the sources found using the MeSH-only strategy were ultimately added to the Bell and Hazel study. This study was also limited because very little hand searching was used. Additionally, the vast majority of results for the gold standard list were limited to articles currently indexed using MeSH within each database (fifty-seven sources not assigned MeSH terms in any of the databases were found using text words only). We recognize that some vendors have adapted the MeSH controlled vocabulary for their products. The results were also skewed to those published in the English language as we are fluent only in English. The success of both the text-word and MeSH-term strategies was also limited by the quality of the search strategies, as evidenced by the fact the MeSH term “Adaptation, Psychological” was assigned to 144 sources but was not included in the search strategy.
Future research regarding MeSH and information literacy instruction should explore how librarians and researchers can effectively use and teach MeSH terms. An additional study surveying the practices and trends of health science and medical librarians teaching MeSH would expand on the relationship between MeSH and information literacy instruction and could shed light on how many librarians are teaching MeSH search strategies and to what level of student. An additional study building off our current research could compare current search results and those from a MeSH-term subject heading combination, “Diabetes Mellitus, Type 1/Psychology.” The concept behind this potential future study was inspired by the surprising number of results that included this pairing. This comparison may provide insight into effective strategies that are easy enough for beginning researchers but effective enough to utilize MeSH to its fullest. Additional future research could be developed to gain a better understanding of why there were results that seemed out of place or results that should have been found but were missed.
ACKNOWLEDGMENTS
The idea for this study was inspired by discussions with Dr. Trevor Bell and Elizabeth Hazel as they sought librarian input for their work to generate a scoping review focused on psychosocial aspects of type 1 diabetes in children and adolescents. We thank them for their support and input as we dove into the world of diabetes research. Additionally, we thank Katherine Majewski from the Office of Engagement and Training at the US National Library of Medicine for her valuable and thoughtful feedback.
DATA AVAILABILITY STATEMENT
Supplemental files.
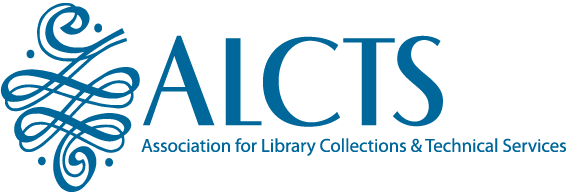
ALA User Menu
- Contact ALCTS
Breadcrumb navigation
- Member How-To
ALCTS is now part of Core: Leadership, Infrastructure, Futures! Visit us on our new Core website .
SKOS: A Guide for Information Professionals
- Share This Page
A Guide to Representing Structured Controlled Vocabularies in the Simple Knowledge Organization System
Priscilla jane frazier.
Simple Knowledge Organization System (SKOS) and associated web technologies aim to enable preexisting controlled vocabularies to be consumed on the web and to allow vocabulary creators to publish born-digital vocabularies on the web.
This guide allows catalogers, librarians, and other information professionals to understand and use SKOS, a World Wide Web Consortium (W3C) standard designed for the representation of controlled vocabularies, to be consumed within the web environment.
The guide includes a brief history of classification technologies, the history of SKOS, and an examination of SKOS within the context of related technology standards like eXtensible Markup Language (XML) and Resource Description Framework (RDF). Following a discussion of the elements and syntax of the SKOS vocabulary, the guide covers various integrity conditions that are used as a guideline for best practices in constructing SKOS vocabularies. The guide then examines past literature, highlighting the conversion, validation, improvement, and automatic generation of SKOS vocabularies. The final section discusses future directions that SKOS development might take.
Article Contents: SKOS Defined | Elements of SKOS | SKOS Integrity Conditions | Literature Review | References | Appendix

Throughout history, classification systems have been widely used in the library community. Knowledge organization systems, and more specifically controlled structured vocabularies, are a growing area within the field of classification systems. Within a web context, formats have been proposed for representing controlled vocabularies in a structured way using the web standards XML and RDF.
An important distinction needs to be made between controlled vocabularies that have been published to the web and those that have been published in a structured way specifically for the web. Natural-language vocabularies, such as simple subject heading lists, thesauri and back-of-the-book indexes are extremely useful for humans, but the meaning that machines can derive from them is very limited. Linked open data and linked open vocabularies are both Semantic Web technologies that enable the publishing of controlled vocabularies for the web in such a way that both humans and machines can recognize meaning (Kaltenböck and Bauer 2012). The creation of linked open vocabularies allows for increased and more meaningful points of access and discovery, and greater effectiveness in information retrieval.
A controlled vocabulary allows for organization of some content, or knowledge, such that it can be easily retrieved at a later time. These vocabularies are “controlled” in that they make use of authorized identification of the content they contain. These groupings of concepts are carefully selected and described so that the information they contain can be retrieved in the most intelligent ways possible. Take, for example, the very small collection of terms called the veggie vocab, found in figure 1. This group of terms about vegetables is listed in alphabetical order. At a glance, one can recognize that the list is comprised of the names of foods (in English) and the names of species (in Latin). However, the same information can be displayed in a different way that makes it much easier for people to understand the relationships between the different terms.

It is easy to see, from the visualization in figure 2, that the vocabulary is about types of vegetables, Vegetables being the “top” term in the vocabulary. The terms Bean , Root , and Gourd are directly below Vegetables . These terms describe varieties of vegetables. Below this second level of terms is a group of terms of actual vegetables, like Potato and Pumpkin . The relationships between terms are hierarchical—in other words, are broader or narrower in scope with relation to one another. The two types of hierarchical relationships in controlled vocabularies are broader term (BT) and narrower term (NT). For example, Root is a narrower term in relation to Vegetables, but a broader term in relation to Parsnip .

Another way to visualize the vocabulary is as a hierarchical report. Figure 3 shows the hierarchy of terms and terms to which they are related. This figure also includes the term Veggie with the label UF. An equivalency relationship, such as use for (UF) or use (USE), allows the vocabulary to make connections between synonyms and near-synonyms. In this particular vocabulary, Vegetable is an authorized or preferred term, and Veggie is an unauthorized or non-preferred term for the same concept.
The final type of relationship that can be reflected in a vocabulary is an associative relationship. This type of relationship allows the vocabulary to make connections between related terms (RT), or terms that have neither hierarchical nor equivalency relationships. For example, Fruit might be a related term to Vegetable .
SKOS Defined
SKOS is a common data model for knowledge organization systems such as thesauri, classification schemes, subject heading systems, and taxonomies. Using SKOS, a knowledge organization system or controlled vocabulary can be expressed as machine-readable data. Once expressed in SKOS, data can then be exchanged between computer applications and published in a machine-readable format on the web.
The SKOS-Core 1.0 Guide was first introduced in 2001 by the W3C Semantic Web Deployment Working Group (SWDWG) in order to develop SKOS as a W3C standardized classification system. The W3C SWDWG currently maintains several pieces of documentation on SKOS that are freely available on the web. First, the SKOS Reference document, which is currently at the final W3C recommendation or standard stage, defines SKOS. Second, the SKOS Primer document provides a guide for users of the system. Third, the SKOS Use Cases and Requirements document presents a list of representative use cases and a set of requirements derived from these use cases. The SWDWG also maintains an open mailing list and a wiki through which the public may contribute to the development of SKOS.
According to the SKOS Reference document, the aims of the system are:
- “to provide a bridge between different communities of practice within the library and information sciences involved in the design and application of knowledge organization systems.”
- “to provide a bridge between these communities and the Semantic Web by transferring existing models of knowledge organization to the Semantic Web technology context, and by providing a low-cost migration path for porting existing knowledge organization systems to RDF.”
SKOS is a data-sharing standard that was built upon several preexisting Semantic Web standards for formal logic and structure. These technologies provide ways of expressing meaning that are amenable to computation and that complement and give structure to information already existing on the web. SKOS was built on RDF, and thus SKOS data are represented as RDF triples. RDF and other related web technologies are defined in the appendix.
The Elements of SKOS
The skos vocabulary.
The vocabulary of SKOS includes various elements that work together to represent knowledge organization systems. These elements include concepts, labels, relationships, mapping properties, collections, and notes.
skos:Concept (instance of owl:Class ). Web Ontology Language (OWL) and Resource Description Framework Schema (RDFS) syntactical equivalents to SKOS elements are provided for reference.
A SKOS concept is any unit of thought: an idea, an object, an event. These concepts are the building blocks of many knowledge organization systems. Because concepts are abstract ideas that exist in the mind, they are independent of the terms used to describe them. Let’s take carrots for example. The English word “carrot” that we use to describe the orange vegetable that rabbits like to eat is actually independent of the concept of a carrot. The idea of a concept and its descriptor (or label) being two separate entities is vital to the SKOS model because it allows machines to identify concepts via their identifier and humans to identify concepts via their label. The SKOS concept element allows vocabulary builders to describe and distinguish concepts and their descriptors (labels). A SKOS concept can be created in two steps:
- Create or reuse a uniform resource identifier (URI) to uniquely identify a concept.
- Assert (make a statement) in RDF, using the property rdf:type , that the resource identified by this URI is of type skos:Concept
In SKOS, a label is the element that is the descriptor of a concept. The three SKOS label elements are sub-properties of the RDFS element rdfs:label . The purpose of these three elements is to link a skos:Concept to an RDF plain literal, or character string.
skos:prefLabel (instance of owl: AnnotationProperty and sub-property of rdfs:label )
Preferred Label is a SKOS element that makes it possible to assign an authorized name to a concept. The two following examples show that the preferred label for the concept of a vegetable is the word “vegetable” in English and “légume” in French. Example:
For information retrieval and information organization purposes, no two concepts in the same knowledge organization system should be given the same preferred label for any given language tag.
skos:altLabel (instance of owl:AnnotationProperty and sub-property of rdfs:label )
Alternate Label makes it possible to assign an unauthorized name to a concept. This label allows multiple same-language descriptors for a concept to be stored. The following example shows that the preferred label for the concept fava_bean is the term “fava bean” and an alternate label is the term “broad bean.” Example:
skos:hiddenLabel (instance of owl:AnnotationProperty and sub-property of rdfs:label )
Hidden Label is a label for a resource that a knowledge organization system designer would like to be accessible to applications performing text-based indexing and search operations, but not visible otherwise. The following example shows that the preferred label for the concept “potato” is the term “potato,” and two hidden labels for the concept are “tater” and “spud.” Hidden label may be used for nicknames or colloquialisms, which may be used to refer to the concept in question, but are not appropriate for official documentation. Example:
Relationships
skos:broader and skos:narrower (instances of owl:ObjectProperty )
These two SKOS labels assert hierarchical relationships between concepts, i.e., that one concept is broader or narrower in meaning than another. Example:
skos:related (instance of owl:ObjectProperty )
This SKOS label allows a designer to assert an associative relationship between two concepts.
In the SKOS data model, skos:related is not defined as a transitive property, and the transitive closure of skos:broader must be disjoint from skos:related . If the concepts Vegetable and Fruit are related via skos:related , there must not be a chain of skos:broader relationships from Vegetable to Fruit . In other words, there must not be simultaneous instances of hierarchical and associative relationships between concepts.
Semantic Relationships
skos:semanticRelation (instance of owl:ObjectProperty )
SKOS semantic relations are connections between SKOS concepts. This type of relation occurs when a link between two concepts is inherent in the meaning of the linked concepts. Each of the SKOS labels skos:broader , skos:narrower , skos:broaderTransitive , skos:narrowerTransitive , and skos:related are sub-properties of skos:semanticRelation .
skos:broaderTransitive and skos:narrowerTransitive (instances of owl:TransitiveProperty )
Like skos:broader and skos:narrower , these two SKOS labels assert hierarchical relationships between concepts, i.e., one concept is broader or narrower in meaning than another. The transitive nature of these two labels signifies that statements such as: "if vegetables is broader than gourd and gourd is broader than pumpkin , then vegetables is assumed to be broader than pumpkin " can be expressed in the SKOS data model.
Mapping Properties
skos:mappingRelation (instance of owl:ObjectProperty )
The SKOS mapping labels are used to state mapping (or alignment) connections between SKOS concepts existing in different concept schemes, for example, Library of Congress Subject Headings (LCSH), Medical Subject Headings (MeSH), and Thesaurus for Graphic Materials (TGM).
skos:closeMatch (instance of owl:ObjectProperty and owl:SymmetricProperty )
skos:exactMatch (instance of owl:ObjectProperty , owl:SymmetricProperty and owl:TransitiveProperty )
skos:exactMatch is a sub-property of skos:closeMatch .
skos:broadMatch and skos:narrowmatch (instances of owl:ObjectProperty )
skos:broadMatch and skos:narrowMatch are used to specify a hierarchical link between concepts. These two properties are inversely related to one another. skos:broadMatch is a sub-property of skos:broader and skos:narrowMatch is a sub-property of skos:narrower .
skos:relatedMatch (instance of owl:ObjectProperty and owl:SymmetricProperty )
Collections of Concepts
skos:Collection (instance of owl:Class )
The SKOS concept collections labels are used to describe labeled or ordered groups of SKOS concepts. For example, the veggie vocab can be considered a collection of SKOS concepts because it is a group of concepts that have something in common.
skos:OrderedCollection (instance of owl:Class )
The SKOS concept ordered collection is used to capture a list of items that have been put into some type of order, i.e., chronology or alphabetization.
skos:member (instance of owl:ObjectProperty )
The SKOS concept member is used to define multiple members of a collection.
skos:memberList (instance of owl:ObjectProperty and owl:FunctionalProperty )
The SKOS concept member is used to define multiple members of a collection in a list format.
This SKOS label was created for general documentation purposes. There is a hierarchical link between skos:note and its different specializations that allows multiple notes to be captured separately and appropriately and for additional information associated with a concept to be retrieved in a straightforward way.
skos:scopeNote (instance of owl:AnnotationProperty )
This label supplies some information about the intended meaning of a concept. It is usually used as an indication of how the use of a concept is limited in indexing practice.
skos:definition (instance of owl:AnnotationProperty )
This label supplies a complete explanation of the intended meaning of a concept.
skos:example (instance of owl:AnnotationProperty )
This label supplies an example of the use of a concept.
skos:historyNote (instance of owl:AnnotationProperty )
This label describes significant changes that have been made to the meaning or form of a concept.
skos:editorialNote (instance of owl:AnnotationProperty )
This label supplies information that is an administrative aid, such as reminders of editorial work still to be done, notifications that future editorial changes might be made, etc.
skos:changeNote (instance of owl:AnnotationProperty )
This label documents fine-grained changes to a concept for the purposes of administration and maintenance.
It should also be mentioned that it is possible to use non-SKOS properties to document concepts (i.e. Dublin Core’s dct:creator ).
In this example, the dct:creator property is further defined by the use of the Friend-of-a-Friend system (FOAF) in order to state that the identity of the “creator” of this concept (Priscilla Jane Frazier) is connected to other individuals via the FOAF community .
SKOS Integrity Conditions
The SKOS Reference document includes several integrity conditions. Integrity conditions are statements that help determine whether or not given data (for example, a vocabulary) are consistent with respect to the SKOS data model. The purpose of SKOS integrity conditions is to encourage the construction of well-formed and consistent data and to promote interoperability between data represented in SKOS.
skos:ConceptScheme is disjoint with skos:Concept
This condition states that SKOS concept schemes, or groups of SKOS concepts, must not be on the same hierarchical level as SKOS concepts and vice versa. For example, in the veggie vocab, Vegetables is a skos:ConceptScheme . This means that Vegetables must not also be a skos:Concept and one of the concepts, like Lima Bean, may not be a skos:ConceptScheme .
skos:prefLabel , skos:altLabel and skos:hiddenLabel are pairwise disjoint properties
This condition states that no SKOS concept may be a member of more than one preferred label, alternate label, and hidden label.
A resource has no more than one value of skos:prefLabel per language tag
This condition states that no SKOS concept may have more than one preferred label for each language tag. For example, the concept summer_squash has the preferred label of “Summer Squash” in English and of “Cucurbita pepo” in Latin, and there may not be any other preferred labels in English or Latin.
skos:related is disjoint with the property skos:broaderTransitive
This condition states that no two SKOS concepts may be connected by both related and broader transitive relationships.
skos:Collection is disjoint with each of skos:Concept and skos:ConceptScheme
This condition states that SKOS collections, or labeled or ordered groups of SKOS concepts, must not be on the same hierarchical level as SKOS concepts and vice versa. For example, in the veggie vocab, Vegetables is a skos:Collection . This means that Vegetables must not also be a skos:Concept and one of the concepts, like Lima Bean, may not be a skos:Collection .
skos:exactMatch is disjoint with each of the properties skos:broadMatch and skos:relatedMatch
This condition states that no two SKOS concepts may be related by more than one of exact match, broader match, and related match.
Literature Review
This literature review covers known SKOS conversion and validation techniques. Also included are numerous SKOS custom expansions and improvement techniques that have been implemented in the past. Finally, the literature review will discuss the new technique of vocabulary creation using SKOS and the state of the field as it stands today.
In the information profession, research and development efforts on the conversion of controlled vocabularies to the SKOS format are being pursued, and a number of technologies and methods have been proposed. Although a manual conversion of a controlled vocabulary by a person or group of people is possible, it is time-consuming and likely prone to error. Applications have been developed to automatically convert vocabularies into the SKOS format, to validate the quality of the formatting of a vocabulary in SKOS format (e.g., PoolParty online SKOS Consistency Checker ), and most recently, to improve the quality and validity of SKOS vocabularies (e.g., the Skosify tool can be used to convert and improve vocabularies expressed as RDFS and OWL into SKOS format). This literature review covers SKOS conversion and validation techniques. Numerous SKOS custom expansions and improvement techniques that have been implemented in the past are also documented. Finally, the literature review will discuss new techniques for vocabulary creation using SKOS, and the state of the field as it stands today.
Conversion Techniques
In 2001, the Semantic Web Advanced Development for Europe (SWAD-E) published Migrating Thesauri to the Semantic Web: Guidelines and Case Studies for Generating RDF Encodings of Existing Thesauri , a thesaurus research prototype that presents guidelines and methods for migrating traditional thesaurus systems to RDF based thesaurus systems. The process described in this document consists of three stages. First, an RDF encoding of the thesaurus is generated. Second, the encoding is taken through error checking and validation processes. Third, the encoding is published on the web. During the first step, a traditional, or term-oriented, view of a thesaurus is converted to a concept-oriented view. Thus, each “preferred term” in a thesaurus becomes a “preferred label” for a “concept.” Each “preferred label” is given a tag of skos:prefLabel , and each concept is given a tag of skos:concept . Each “concept” in the thesaurus is assigned a unique URI that can be linked through the web to URIs for related concepts. The technique of designating unique and persistent URIs for all concepts in a vocabulary allows machines to understand the relationships between concepts similar to the way humans understand these types of relationships intrinsically.
Assem, et al.(2006) expand on the technique used in step one of the SWAD-E document. Their paper suggests three activities that will effectively link the term-oriented view of a thesaurus to its concept-oriented view. First, the digital format and the documentation of the thesaurus are analyzed to determine the features of the thesaurus and how it is encoded. Second, a mapping between the thesaurus data items and the SKOS RDF is defined. Third, a transformation program, or algorithm, is created. The authors mention a sub-activity in which pre-existing URIs, if present, are identified. If no URIs exist, in the term-oriented view of a thesaurus, the authors suggest creating randomly generated unique identifiers or using the name of unique preferred terms to generate URIs.
Assem, et al. apply their new technique to three existing thesauri: Integrated Public Sector Vocabulary (IPSV), Common Thesaurus for Audiovisual Archives (GTAA), and Medical Subject Headings (MeSH). These particular thesauri are chosen because of their popularity and range in complexity. The authors find that conversion of the largest and most complex thesaurus, MeSH, is problematic but those difficulties assist in identifying the boundaries of the applicability of their technique. The fact that MeSH contains textual notes that combine several types of knowledge, or compound concepts, leads the authors to conclude that some thesauri have complex structures for which no direct SKOS counterpart exists. Additionally, IPSV and MeSH contain management information about their terms, which cannot be represented within the SKOS standard. The authors also mention that at the time of their study validation of SKOS RDF is difficult, due to the lack of validation technologies.
In 2008 Summers, et al. presented a technique for converting Library of Congress Subject Headings (LCSH) to SKOS in their paper “LCSH, SKOS and Linked Data.” In their research, the authors use content in MARC bibliographic records to harvest LCSH terms and map them to corresponding SKOS concepts. For example, Library of Congress Control Numbers are given to every LCSH, are mapped onto skos:Concept and are used to create unique URIs for each concept. Pre-coordinated LCSH terms, or LCSH terms that have been previously combined together in anticipation of a search on that combination of terms, which have the potential to create problems in SKOS because they represent more than one term or concept, are simply flattened into one concept in this technique. To accomplish the conversion, the authors write code using the Python programming language and use open-source MARCXML and RDF processing tools to create an object-oriented streaming interface to mint URIs and link them together. Additionally, the authors suggest that an extension of SKOS would allow the full meaning of LCSH terms to be captured in SKOS form and that the integration of other Semantic Web vocabularies such as Dublin Core could allow SKOS vocabularies to be even more meaningful.
The earlier conversion methods of SWAD-E were adapted by Neubert in his 2009 paper “Bringing the ‘Thesaurus for Economics’ on to the Web of Linked Data.” Neubert found that SKOS’s built-in multilingual features are useful, given that the Thesaurus for Economics, or STW, is made up of both English and German terms. The author states that the conversion of STW into SKOS was straightforward. However, at the time of his research, several new SKOS classes, including skos:notation , had been introduced by W3C. Neubert was able to take advantage of the fact that SKOS could now accommodate internal management information notation, something that Assem, et al. had observed SKOS was lacking in 2006.
Validation Techniques
Neubert (2009) also discusses the use of SPARQL queries to check SKOS vocabularies for inconsistencies. The author describes the process of loading SKOS vocabularies into a SPARQL server and running inconsistency checks, where queries that check for illogical links between concepts would bring these inconsistencies to the attention of the implementer. Neubert also mentions that this process could potentially improve thesaurus maintenance if inconsistency checks could be performed routinely. The concept of automatic validation of SKOS vocabularies was new at the time of his research. One drawback to this validation process is that an implementer inputting the SPARQL queries would only be able to locate inconsistencies that had previously been anticipated. Inconsistencies that are not queried would not be identified by the SPARQL server.
In their 2012 paper “Improving the Quality of SKOS Vocabularies with Skosify,” Suominen and Hyvönen propose a tool with the ability to check the quality of a SKOS vocabulary. The authors cite a lack of quality and validity of existing SKOS vocabularies as the reason for the development of this new tool, named the PoolParty Online SKOS Consistency Checker, or PoolParty . The researchers created a list of eleven validation criteria by which SKOS vocabularies are checked. The criteria were gathered from the W3C SKOS Reference document and from the authors’ examination of fourteen freely available SKOS vocabularies. The authors found that all of the medium- and large-sized vocabularies failed at least one validation check, meaning that they did not meet some of the SKOS integrity constraints. Only one of the fourteen vocabularies passed all eleven validation criteria.
Custom Expansions
As seen in Assem, et al. and Neubert, controlled vocabularies often contain constructs that have no direct counterpart in SKOS. As stated in the SKOS Primer , SKOS is designed for simple extension of language constructs for the specialization of a particular vocabulary. This is made possible by expounding upon a SKOS construct using the extension rdfs:subClassOf . Neubert proposes two SKOS extensions for use with the STW thesaurus. First, he splits skos:Concept into two “subclasses.” This change is chosen because along with the standard terms, or descriptors, STW includes a taxonomy of approximately 500 classes that are used to aid user information retrieval. Neubert splits skos:Concept into two parts: zbwext:Descriptor rdfs:subClassOf skos:Concept for concepts and zbwext:Thsys rdfs:subClassOf skos:Concept for classes. This approach allows for well-defined semantics in terms of broader and narrower relationships between concepts and allows users to search for both concepts and classes. Additionally, Neubert extended the newly introduced skos:note using the following notation: zbwext:useInsteadNote rdfs:subClassOf skos:note for notes that guide users to designated preferred labels rather than other preferred labels in certain circumstances.
Improvement Techniques
Along with their validation tool PoolParty, Suominen and Hyvönen (2012) introduce Skosify , a tool created to improve the quality and validity of SKOS vocabularies. Skosify is a command line tool capable of reading one or more SKOS files and outputting a file in which errors and problems have not only been identified, but corrected. Skosify is able to address nine of the eleven validation criteria mentioned earlier in their paper. After being tested on fourteen vocabularies, Skosify corrects problems in all nine of these categories. The tool has the ability to correct missing language tags if a default language is provided, to detect unlabeled concept schemes and add specified labels and to remove unnecessary white space surrounding property values. Skosify can recognize and correct more sophisticated problems having to do with the relationships between concepts in vocabularies. The tool is able to identify top level concepts and add hasTopConcept and topConceptOf relationships to that concept scheme, recognize the designation of more than one prefLabel for a single concept and correct the error, and identify as well as correct concepts that have been mislabeled as collections. Additionally, the tool recognizes when a concept is linked to a label using two different label properties and removes the less important property, recognizes when concepts are linked together in a way that is disjointed and removes the related relationship assertion without disabling the broader hierarchy, and recognizes cycles or concepts that have a broader relationship with themselves and removes the offending relationship.
Vocabulary Creation with SKOS
In 2010, Gerbé and Kerhervé proposed a new approach to vocabulary creation with their paper “A Model-Driven Approach to SKOS Implementation.” Their technique involves viewing the SKOS conceptual model as a metamodel for structured controlled vocabularies. Using a model management and model engineering approach, the authors state that flexible and extensible vocabularies can be managed and created, which may be useful for nontraditional or highly complex vocabularies. Model operators, or prompts used in model management, such as map, match, merge, and compose, can be used to map term-oriented vocabularies onto a concept-oriented platform. Gerbé and Kerhervé introduced a metamodel and SKOS engine for use in the development of SKOS vocabularies. The metamodel is expressed in much the same way as a database schema, and is supported by the MySQL database system. The SKOS engine is built as a database with an interface for use in populating and visualizing the vocabulary content. The authors state that the tool is also capable of importing, exporting, and merging vocabularies. This model-driven database approach to SKOS vocabulary creation takes the capabilities of tools for working with SKOS vocabularies to a new level of sophistication by offering an advanced data storage system and a graphical user interface.
State of the Art
In 2012, Manaf, Bechhofer, and Stevens presented a survey of the current state of SKOS vocabularies on the web. A total of 478 vocabularies were identified as complying with the given definition of a SKOS vocabulary. Analyses of those vocabularies include investigation of the use of SKOS constructs, the use of SKOS semantic relations and lexical labels, and the structure in terms of the hierarchical and associative relations, branching factors, and depth of the vocabularies.
The researchers collect the following data on each SKOS vocabulary: number of SKOS concepts; depth of each SKOS concept and depth of the concept hierarchy; number of links for skos:broader , skos:narrower , and skos:related properties; total number of concepts not connected to any other concepts; total number of concepts with skos:narrower relations but no skos:broader relations; and maximum number of skos:broader properties. According to the researchers, SKOS concepts and concept labeling is core to SKOS vocabularies, but not all SKOS vocabularies in the study use SKOS lexical labels for their concepts. Approximately one-third of the SKOS vocabularies studied fall into the category of term lists, with no use of any SKOS semantic relations. The researchers find that not all published SKOS vocabularies explicitly declare SKOS concepts present in the vocabularies. The survey results can serve to provide a better understanding of the modeling styles of the SKOS vocabularies published on the web, especially when considering the creation of applications that utilize these vocabularies.
English abstract
The present study is a literature review, based on the comparative study between controlled vocabularies and social tags from various perspectives. Critical comments of experts on similarities, co-relationship, uses, trends between controlled vocabularies and social tags have found their manifestation in this literature review. Authors made an effort to portray the overall picture of previous research done on these areas.
Bogers, T. & Petras, V. (2015). Tagging vs. Controlled Vocabulary: Which is More Helpful for Book Search? In Proceedings of iconference 2015.
Bogers, T. & Petras, V. (2017). An In-Depth Analysis of Tags and Controlled Metadata for Book Search. In Proceedings of iconference 2017.
Bogers, T. & Petras, V. (2017). Supporting Book Search: A Comprehensive Comparison of Tags vs. Controlled Vocabulary Metadata. Data and Information Management, 2017; 1(1): 17–34
Cattuto, C., Christoph, S., Andrea, B., Vito DP S., Vittorio, L., Andreas, H., Miranda, G., & Gerd, S. (2007). Network Properties of Folksonomies. AI Communications, 20, 245-262.
Dash, C. G. J. (2015). A matter of context: An investigation into the representation of bias in social tags and Library of Congress Subject Headings. Department of Information Studies, AberystwythUniversity, Retrieved from
https://pdfs.semanticscholar.org/c16a/5609399930a2643258e69b46437678a0132a.pdf
Doctorow, C. (2001). Metacrap: Putting the Torch to Seven Straw-Men of the Meta-Utopia. Retrieved from https://people.well.com/user/doctorow/metacrap.htm
Feinberg, M. (2006). An Examination of Authority in Social Classification Systems. Advances in Classification Research Online, 17(1), 1-11.
Furner, J. (2010). Folksonomies. Encyclopedia of Library and Information Sciences. New York: Taylor and Francis, 1858-1866.
Golder S. & Huberman, B. A. (2006). Usage patterns of collaborative tagging systems, Journal of Information Science, 32(2), 198–208.
Kipp, M. E. I. (2011). Controlled vocabularies and tags: An analysis of research methods.North American Symposium on Knowledge Organization (NASKO), Toronto, June 15-16, 2011.
Koolen, M. (2014). User Reviews in the Search Index? That’ll Never Work!. In ECIR ’14: Proceedings of the 36th European Conference on Information Retrieval. 323–334.
Kroski, E. (2005). The Hive Mind: Folksonomies and User-Based Tagging. Info Tangle Blog, December, 2005
Lee, D. H. & Schleyer, T. (2010). A Comparison of MeSH Terms and CiteULike Social Tags As Metadata for the Same Items. In IHI ’10: Proceedings of the 1st ACM International Health Informatics Symposium. ACM, New York, NY, USA, 445–448.
Lu, C., Park, J.-R. & Hu, X. (2010). User Tags versus Expert assigned Subject Terms: A Comparison of LibraryThing Tags and Library of Congress Subject Headings. Journal of Information Science, 36(6), 763–779.
Ma, X. & Cahier, J. P. (2012). Visual Distinctive Language: Using a Hypertopic based Iconic Tagging System for Knowledge Sharing. In Enabling Technologies: Infrastructure for Collaborative Enterprises (WETICE) 2012 IEEE 21st International Workshop. IEEE. 456-461.
Martinez-Avila, D. (2015). Knowledge Organization in the Intersection with Information Technologies. Knowledge Organization, 42(7), 486–498, Retrieved from
http://search.ebscohost.com/login.aspx?direct=true&db=iih&AN=111299953&lang=pt-br&site=ehostlive.
Mathes, A. (2004). Folksonomies - Cooperative Classification and Communication Through Shared Metadata. Retrieved from http://www.adammathes.com/academic/computer�mediated-communication/folksonomies.html
Matthews, B., Jones, C., Puzon, B., Moon, J., Tudhope, D. et al. (2010). An evaluation of enhancing social tagging with a knowledge organization system. ASLIB Proceedings, 62(4/5), 447-465
Noruzi, A. (2006). Folksonomies: (Un) Controlled Vocabulary? Knowledge Organization, 33 (4), 199-203.
O'Reilly, T. (2005). What Is Web 2.0?: Design Patterns and Business Models for the Next Generation of Software". Retrieved from http://www.oreillynet.com/pub/a/oreilly/tim/news/2005/09/30/what-is- web-20.html.
Rafferty, P. M. (2017). Tagging. Knowledge Organization, 45(6), 500-516.
Rorissa, A. (2008). User-generated descriptions of individual images versus labels of groups and image: A comparison using basic level theory. Information Processing & Management. 44.5: 1741-1753.
Shiri A.A, Revie C, & Chowdhury G. (2002). Thesaurus-assisted search term selection and query expansion: a review of user-centered studies. Knowledge Organization, 29(1), 1–19.
Trant, J. (2009). Studying Social Tagging and Folksonomy: A Review and Framework. Journal of Digital Information 10(1), 1-44
Vaidya, P. & Harinarayan, N. S. (2016). The role of social tags in web resource discovery: an evaluation of user-generated keywords. Annals of Library and Information Studies, 63(Dec.), 289-297.
Wal, T. V. (2007). Folksonomy coinage and definition, Retrieved from http://vanderwal.net/folksonomy.html.
Zauder, K. Jadranka, L. L., & Mihaela, B. Z. (2007). Collaborative Tagging Supported Knowledge Discovery. Information Technology Interfaces 2007. ITI 2007. 29th International Conference on, IEEE. 437-442
Downloads per month over past year
View more statistics
Actions (login required)
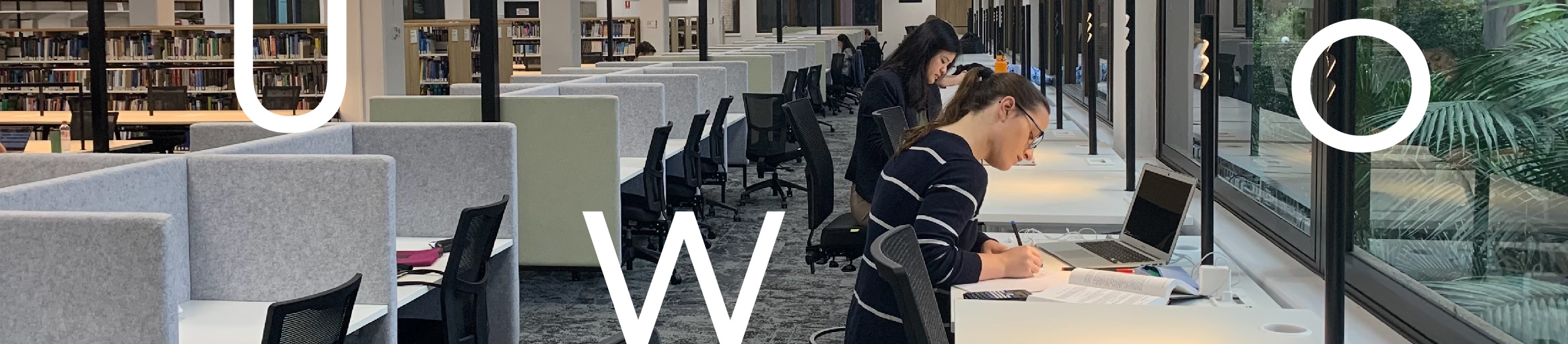
Help us improve our Library guides with this 5 minute survey . We appreciate your feedback!
- UOW Library
- Key guides for researchers
Systematic Review
- Controlled vocabularies (MeSH)
- What is a systematic review?
- Five other types of systematic review
- How is a literature review different?
- Search tips for systematic reviews
- Controlled vocabularies
- Grey literature
- Transferring your search
- Documenting your results
- Support & contact
- Last Updated: Feb 22, 2024 12:34 PM
- URL: https://uow.libguides.com/systematic-review
Insert research help text here
LIBRARY RESOURCES
Library homepage
Library SEARCH
A-Z Databases
STUDY SUPPORT
Academic Skills Centre
Referencing and citing
Digital Skills Hub
MORE UOW SERVICES
UOW homepage
Student support and wellbeing
IT Services
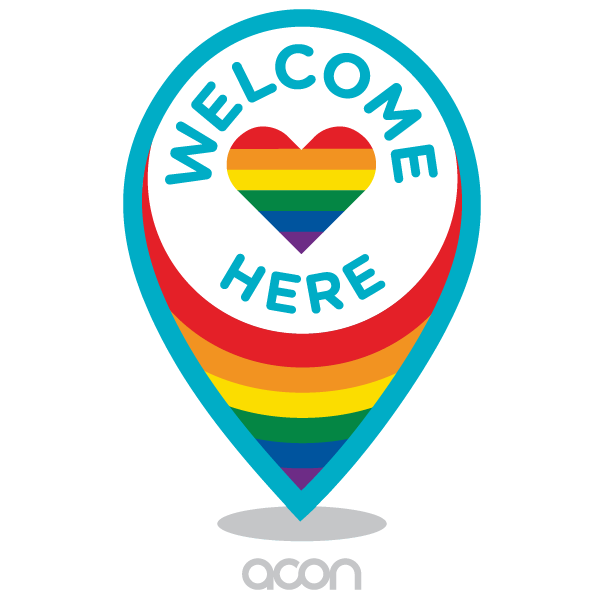
On the lands that we study, we walk, and we live, we acknowledge and respect the traditional custodians and cultural knowledge holders of these lands.
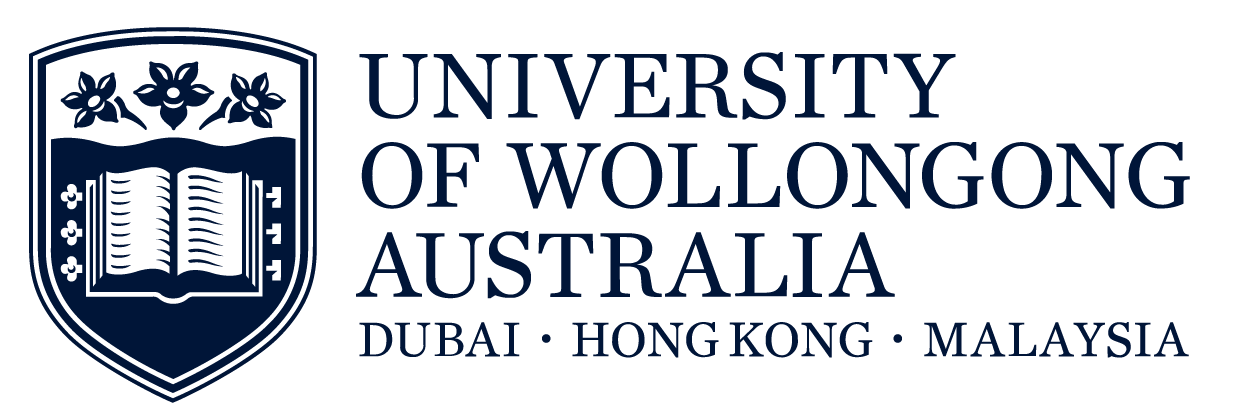
Copyright & disclaimer | Privacy & cookie usage
University of Tasmania, Australia
Systematic reviews for health: 7. boolean operators.
- Handbooks / Guidelines for Systematic Reviews
- Standards for Reporting
- Registering a Protocol
- Tools for Systematic Review
- Online Tutorials & Courses
- Books and Articles about Systematic Reviews
- Finding Systematic Reviews
- Critical Appraisal
- Library Help
- Bibliographic Databases
- Grey Literature
- Handsearching
- Citation Tracking
- 1. Formulate the Research Question
- 2. Identify the Key Concepts
- 3. Develop Search Terms - Free-Text
- 4. Develop Search Terms - Controlled Vocabulary
- 5. Search Fields
- 6. Phrase Searching, Wildcards and Proximity Operators
- 7. Boolean Operators
- 8. Search Limits
- 9. Pilot Search Strategy & Monitor Its Development
- 10. Final Search Strategy
- 11. Adapt Search Syntax
- Documenting Search Strategies
- Handling Results & Storing Papers
Step 7. Boolean Operators
Once all free-text terms and controlled vocabulary terms have been identified, you can start the proper searching process. It is recommended to search for each identified search term individually, then use the correct Boolean operators to combine the terms. This will help prevent any human errors. It also allows you to see which search terms add value to the search and if a particular search term produces too many irrelevant results.
Boolean Operators Explained
An OR search will find information which includes either search term. All free text and controlled vocabulary terms identified for a concept are to be combined with an OR. This is to broaden the search and to capture all articles on a topic regardless of which term is used in the article.
An AND search will find results with information common to both search terms. Once all relevant information for each concept has been found, the concepts are joined with AND. This is to narrow the search and to only capture articles in which all concepts appear.
A NOT search will exclude words from your search results. This is to narrow your search, telling the database to ignore concepts that may be implied by your search terms. If you are interested in e.g. only finding human studies you may be tempted to type NOT animals . This means that articles that include the word animals are excluded, including studies on animals as well as humans, which potentially are relevant. As a NOT search has the potential to exclude relevant articles, it is not normally recommended for a systematic review.
NOTE! All databases support these Boolean operators. The syntax for the NOT operator may vary slightly. For more information visit the Search Help menu within the relevant database or see
Example - In General
Combine all terms within a concept with OR. Then, join the searches for each concept with AND .
Example - In Detail
- Medline via Ovid
- Medline via PubMed
This is how you could approach the example in Medline via Ovid :
1. Carry out separate searches for each free-text term and controlled vocabulary term in Concept 1 of Concept Table.
To search for MeSH terms, use Search Tools section to Map Term . To search for free-text terms, use Search Fields section and tick relevant fields, e.g. Title, Abstract, Keyword Heading Word.
1 Dementia.ab,kf,ti. 2 Alzheimer.ab,kf,ti. 3 "Huntington*".ab,kf,ti. 4 Kluver.ab,kf,ti. 5 Lewy.ab,kf,ti. 6 exp Dementia/
NOTE! In Ovid you need to tick a box if you want to explode a MeSH term; the default is non-explode. This is different to PubMed.
2. Combine all individual searches for Concept 1 with OR:
7 1 or 2 or 3 or 4 or 5 or 6
Either type as above, or select searches 1 to 6 and click Combine with OR .
3. Repeat steps 1 and 2 for all other concepts.
4. Combine the OR searches for each concept with AND .
44 7 and 22 and 31 and 43
Either type as above, or select searches 7, 22, 31 and 43 and click Combine with AND .
See source of example
This is how you could approach the example in Medline via PubMed :
In PubMed Advanced Search, change the drop-down menu to Title/Abstract or MeSH Terms accordingly, then enter each search term and Add to History (change Search drop-down)
#1 dementia [tiab] #2 alzheimer [tiab] #3 huntington* [tiab] #4 kluver [tiab] #5 lewy [tiab] #6 dementia [mh]
NOTE! There is no option for non-exploded MeSH term in the drop-down menu. If you want to not explode a MeSH term, keep the drop-down default of All Fields and use this syntax in the search box: Dementia[MeSH:NoExp] or dementia[mh:noexp]
#7 #1 OR #2 OR #3 OR #4 OR #5 OR #6
Either type as above, or use the Add Query / Add with OR link in the History under Actions to bring the individual searches back up into the Query Box . This will give you search #7
(((((Dementia [tiab]) OR Alzheimer [tiab]) OR Huntington* [tiab]) OR Kluver [tiab]) OR Lewy [tiab]) OR Dementia [mh]
#49 #7 AND #24 AND #34 AND #48
Either type as above, or use the Add Query / Add with OR link in the History to bring the individual searches back up into the Query Box . This will give you search #49 :
(((((((((Dementia [tiab]) OR Alzheimer [tiab]) OR Huntington* [tiab]) OR Kluver [tiab]) OR Lewy [tiab]) OR Dementia [mh])) AND ((((((((((((((((Animal-assisted therapy [tiab]) OR Animal-assisted activit* [tiab]) OR Animal-assisted intervention* [tiab]) OR Animal therapy [tiab]) OR Pet therapy [tiab]) OR Dog therapy [tiab]) OR Dog-assisted therapy [tiab]) OR Canine-assisted therapy [tiab]) OR Aquarium [tiab]) OR Animal Assisted Therapy [mh:noExp]) OR Pets [mh]) OR Dogs [mh]) OR Cats [mh]) OR Birds [mh:noexp]) OR Bonding, Human-Pet [mh]) OR Animals, Domestic [mh:noExp])) AND (((((((((Music therapy [tiab]) OR Music* [tiab]) OR Singing [tiab]) OR Sing [tiab]) OR Auditory stimulat* [tiab]) OR Music [mh]) OR Music Therapy [mh]) OR Acoustic Stimulation [mh]) OR Singing [mh])) AND ((((((((((((Aggression [tiab]) OR Neuropsychiatric [tiab]) OR Apathy inventory [tiab]) OR Cornell scale [tiab]) OR Cohen Mansfield [tiab]) OR BEHAVE-AD [tiab]) OR CERAD-BRSD [tiab]) OR Behavior* [tiab]) OR Behaviour* [tiab]) OR Aggression [mh]) OR Personality inventory [mh]) OR Psychomotor agitation [mh])
Need More Help? Book a consultation with a Learning and Research Librarian or contact [email protected] .
- << Previous: 6. Phrase Searching, Wildcards and Proximity Operators
- Next: 8. Search Limits >>
- Last Updated: Apr 4, 2024 10:17 AM
- URL: https://utas.libguides.com/SystematicReviews

Academia.edu no longer supports Internet Explorer.
To browse Academia.edu and the wider internet faster and more securely, please take a few seconds to upgrade your browser .
Enter the email address you signed up with and we'll email you a reset link.
- We're Hiring!
- Help Center
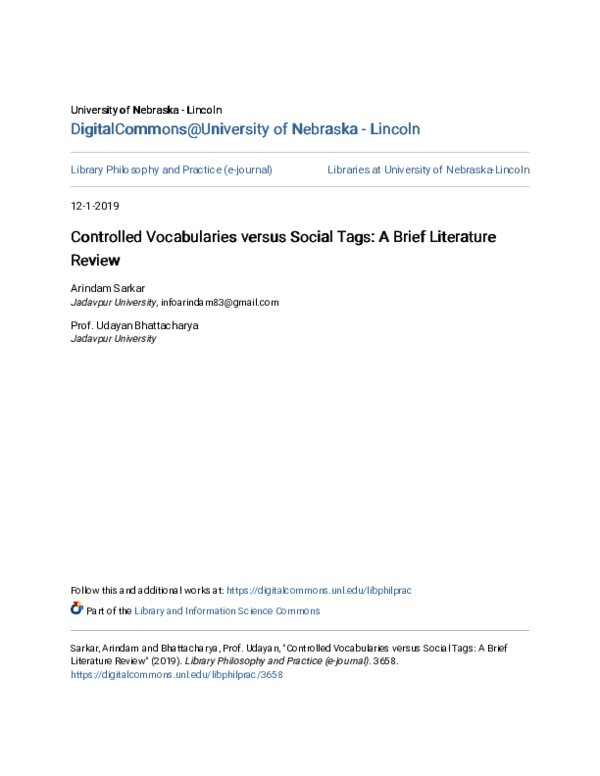
Controlled Vocabularies versus Social Tags: A Brief Literature Review

Related Papers
Margaret Kipp
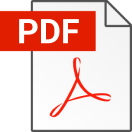
Annals of Library and Information Studies (ALIS)
Kalyan Sundar Samanta
Proceedings of The Asist Annual Meeting
Emma Tonkin
The panel will bring together an intentional variety of perspectives on the process and outcomes of tagging, within and without social networking. In particular, how the context is apparent in the vocabulary, language or classifications used in communication. At the individual or conceptual level, tags are seen as a means to avoid some of the issues associated within more formal frameworks, such as fossilized terms of meanings, but at the context, language or ontology level a concept must be expressed via a relatively impoverished vocabulary of jointly shared terms and ideas. One such misunderstanding, one that drives much of the debate on tagging, is the widespread definition of ontology as “a hierarchical structure to describe conceptual structures that are closed, inflexible and restrictive”. However, a lexicon of tags – terms – can be tailored to improve and optimize communication accuracy. The panel will attempt to show how tagging is applied to indicate or derive an appropriate semantics, given the user's understanding of the information's context. Then discussing how this process fits with established theory in knowledge management, KM, linguistics and classification research amongst others, and investigate wider implications.
Lala Hajibayova
A. I. M. Jakaria Rahman
The purpose of the study was to investigate social tagging practice in science book context. In addition, it identified the usefulness of social tags as supplementary of controlled vocabulary to enhance the use of library resources. More specifically, this study examined to know to what extent the social tags match with controlled vocabulary, and whether or not any additional perception is provided by social tags to improve the accessibility and information retrieval in a digital environment. In both cases, the social tags were considered with respect to the appropriateness to the specific book. For the successful implementation of social tagging in library systems, there is a need to understand how users assign social tags to library collections, what vocabularies they use and how far the social tag relates to controlled vocabulary. This understanding can help libraries to decide on how to implement and review the social tagging. It is found that there is a clear difference between assigning expert created subject terms and social tagging practice to library books. Cataloguers assigned relatively few terms per book through the use of restricted and established vocabulary following firm rules, whereas, the end users enjoyed liberty with unlimited terms. The social tagging represented other aspects that could not be either covered within the strict subject headings assigned rules or cataloguing rules. Such diverse impressions can be seen as an access point to the same library collections according to users’ interest and opinions. This study revealed that as a standalone tool neither the controlled vocabulary nor the social tagging practice can work like a satisfactory information retrieval tool. A hybrid catalogue with combining both LCSHs and social tags would give its patrons the best of both worlds in terms of access to materials.
DESIDOC Journal of Library & Information Technology
Social tagging allows users to assign any free-form keywords as tags to any digital resources through a decentralised way. Many information scientists find that there are similarities through their studies between usergenerated social tags and the librarian-generated subject headings for the libraries. The present study was conducted to identify the similarity and dissimilarity between user-generated social tags and librarian-generated subject terms of 1000 books in the domain of History. The study also conducted to identify whether social tags can replace controlled vocabularies. The study finds that only a small portion of terms overlaps with each other (3.54 % of social tags & 56.07 % of SLSH terms) and Spearman’s rank correlation proves that there is a good association between overlapping terms. Jaccard similarity coefficient highlights that users and the librarian use different terminologies (as J = 0.13, 0.12 & 0.11). Individual title wise comparison also defines that 90 per c...
Valerie Durieux
Aslib Proceedings
Koraljka Golub
David Millen
The panel will discuss tagging of documents where a particular vocabulary, language or classification is used for communication. At the individual or conceptual level, tags avoids some of the issues of fossilized terms or meanings, but at the context, language or category level, the meaning must be of a more community or social network nature. There is even a 'tag'to 'tag'relationship where the “to” object may either be a user or information. Therefore, tags as a language can be tailored to improve communication accuracy for the object.
Abstract Social tagging is one of the major phenomena transforming the World Wide Web from a static platform into an actively shared information space.
RELATED PAPERS
International Journal of Cancer
Prof. Anjali Karande
nguyễn võ thế sang
HTS Teologiese Studies / Theological Studies
Graham Duncan
Annals of Emergency Medicine
Daniela Joselin Delgado Valenzuela
JaeRyong Shim
redmine.roda.dgarq.gov.pt
José Carlos Ramalho
Unterstützung – Kooperation – Kontrolle
Judith Hangartner
Revista Cubana de …
Dagmar Paredes Lopez
2000 10th European Signal Processing Conference
Fernando Mondragón Nava
IEEE Wireless Communications Letters
priyabrata parida
Reproduction, Fertility and Development
Evelyn Telfer
Manisa Hür Işık Gazetesi
Necdet Cura
Journal of Evolution of medical and Dental Sciences
Siddharth Dubey
Bezmialem Science
Deniz Borcak
El Universal
José Octavio Islas Carmona
Pain Medicine
Results in Physics
ΔΗΜΗΤΡΗΣ ΤΣΕΛΕΣ
Indonesian Journal of Electrical Engineering and Informatics (IJEEI)
Jereesha Mary S J
Crop Protection
Vinton Omaleki
Revista médica de Chile
Fernando Valenzuela
hjjhgj kjghtrg
Internal Medicine Journal
Snjezana Dotlic
Juan Garcia-valero
Revista Mexicana De Biodiversidad
Eduardo Balart
RELATED TOPICS
- We're Hiring!
- Help Center
- Find new research papers in:
- Health Sciences
- Earth Sciences
- Cognitive Science
- Mathematics
- Computer Science
- Academia ©2024
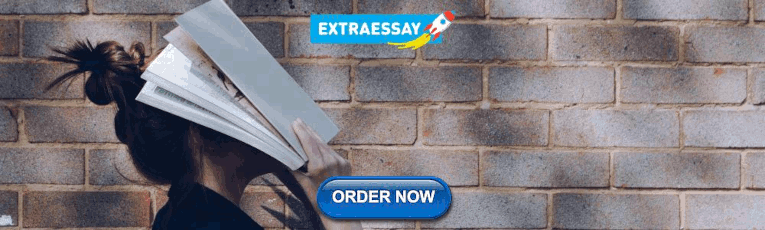
IMAGES
VIDEO
COMMENTS
The Medical Subject Headings (MeSH) is one example of a controlled vocabulary. It can be used while searching the MEDLINE database via PubMed or OVID. Created and maintained by the National Library of Medicine. Subject headings are applied to every article indexed in PubMed to identify key topics of the publication.
A controlled vocabulary is an organised set of phrases or words used to index content in a database so that it can be efficiently retrieved. These are sometimes referred to as subject terms, subject headings, thesaurus terms, or index terms. Examples of controlled vocabularies include Medical Subject Headings (MeSH), APA Thesaurus of ...
Controlled vocabulary is a set of terminology assigned to citations to describe the content of each reference. Searching with controlled vocabulary can improve the relevancy of search results. ... document various elements of the literature search for your review. To make this process more clear, a statement and checklist for reporting ...
LITERATURE REVIEW. Controlled vocabularies are tree-structured knowledge organization systems (KOS) that provide conceptual relationships among entities and properties of concepts (Baker et al., 2013). They are knowledge structures that allow users to organize and find information, and understand the scope of a discipline or domain being ...
Controlled Vocabularies. Controlled vocabularies are a standardized set of terms used to describe the content of a resource in a database. This is known as. indexing. Using controlled vocabulary terms will usually generate fewer and more relevant results. However, you must know the exact term/vocabulary to use. An example is provided below.
In addition, controlled vocabulary also will facilitate comparison of organizational structures and procedures both nationally and internationally. ... A systematic literature review, Academic Emergency Medicine, 23 (2016) 503-510. [Google Scholar] Other Formats. PDF (790K) Actions.
What is a Controlled Vocabulary? "… a carefully selected list of words and phrases, which are used to tag units of information (document or work) so that they may be more easily retrieved by a search…Controlled vocabularies reduce ambiguity inherent in normal human languages where the same concept can be given different names and ensure ...
Step 1: Structure Your Concepts. Break down your research question into smaller concepts in order to make the next few steps manageable. You may find it helpful to document the next few steps using a table in Word or Excel. For example, if your research question is PICO-formatted, you might start a table that looks like this:
Related Keywords - Advanced Research Skills: Conducting Literature and Systematic Reviews. Module 2: Formulating a Research Question and Searching for Sources. Your search strategy can contain your main keywords, similar or related keywords and controlled terms (also called subject headings ). Searching by a keyword will retrieve resources ...
Develop Search Terms. The Cochrane Handbook, 4.4.4 suggests searches should comprise a combination of subject terms selected from the controlled vocabulary or thesaurus ('exploded' where appropriate) with a wide range of free-text terms (see Step 3) in order to identify as many relevant records as possible searches.. If you use keywords only, you could miss articles that do not use your ...
A review of the literature since then shows that numerous studies, in various disciplines, have found that a quarter to a third of records returned in a keyword search would be lost without controlled vocabulary. Other writers, though, have continued to suggest that controlled vocabulary be discontinued. Addressing criticisms of the Gross ...
The results of this study reaffirm that the MeSH-term search strategy yields both greater recall and greater precision. Therefore, even though the difficulty level of using MeSH (or similar controlled vocabulary) to search for literature may be higher, there is a substantial benefit to using MeSH as part of an effective search strategy.
A Guide to Representing Structured Controlled Vocabularies in the Simple Knowledge Organization System Priscilla Jane Frazier March 2015 Simple Knowledge Organization System ... Finally, the literature review will discuss new techniques for vocabulary creation using SKOS, and the state of the field as it stands today. Conversion Techniques.
The use of controlled vocabularies in requirements engineering activities: a protocol for a systematic literature review. José L. Barros-Justo1 Samuel Sepúlveda2 Nelson Martínez-Araujo1 Alejandro González-García1 1 School of Informatics (ESEI), University of Vigo, 32004 Ourense, Spain 2 Departamento de Ciencias de la Computación e Informática (DCI), Centro de Estudios en Ingeniería de
The present study is a literature review, based on the comparative study between controlled vocabularies and social tags from various perspectives. Critical comments of experts on similarities, co-relationship, uses, trends between controlled vocabularies and social tags have found their manifestation in this literature review. Authors made an effort to portray the overall picture of previous ...
Abstract: The present study is a literature review, based on the comparative study between. controlled vocabularies and social tags from various perspectives. Critical comments of. experts on ...
Abstract: The present study is a literature review, based on the comparative study between controlled vocabularies and social tags from various perspectives. Critical comments of experts on similarities, co-relationship, uses, trends between controlled vocabularies and social tags have found their manifestation in this literature review.
What is a systematic review? Five other types of systematic review ; How is a literature review different? Systematic review research process. Search tips for systematic reviews ; Controlled vocabularies ; Grey literature ; Transferring your search ; Documenting your results ; Tools and frameworks. Tools ; Frameworks ; Support & contact
Boolean Operators - Systematic Reviews for Health - Subject Guides at University of Tasmania. Step 7. Boolean Operators. Once all free-text terms and controlled vocabulary terms have been identified, you can start the proper searching process. It is recommended to search for each identified search term individually, then use the correct Boolean ...
The following literature review covers relevant research on controlled vocabularies, including research addressing the benefits of using controlled vocabularies as low-level semantic ontologies. Then, we discuss the research objectives, methods, and results. Lastly, we present a
Through this study an effort has been made to portray the overall picture of previous research regarding this topic. Keywords: Controlled vocabularies; Social tags; Literature review; Co-relationship Introduction The controlled vocabulary is a set of preselected terms made by experts in controlled way for assigning in various applications.
First released in 2014, the Library of Congress Medium of Performance Thesaurus for Music (LCMPT) is a tool for describing the instruments, voices, etc., used in the performance of musical works. LCMPT is available through Classification Web, which is updated daily; subscriptions may be purchased through the Cataloging Distribution Service.
Controlled vocabularies for scientific data Conducting a literature review on the application of controlled vocabularies for scientific data is challenging, given the wide diversity and scope of items that qualify as data. Another challenge is that there are various scientific communities—each demonstrating different levels of