Psychological Experimental Design
- Living reference work entry
- First Online: 15 February 2024
- Cite this living reference work entry
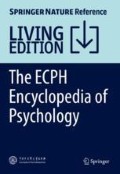
- Zhang Houcan 2 &
- He Dongjun 3
This is a preview of subscription content, log in via an institution to check access.
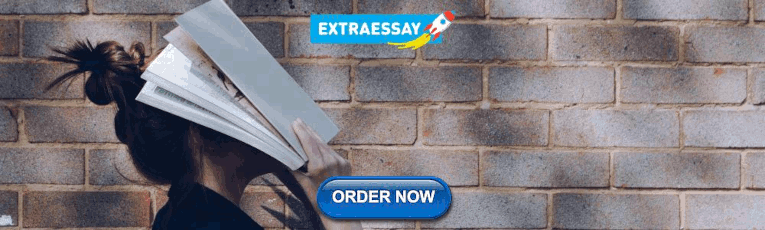
Access this chapter
Institutional subscriptions
Further Reading
Kantowitz BH, Roediger HL, Elmes DG (2015) Experimental psychology, 10th edn. Cengage Learning, Boston
Google Scholar
Zhang X-M, Hua S (2014) Experimental psychology. Beijing Normal University Publishing Group, Beijing
Download references
Author information
Authors and affiliations.
Faculty of Psychology, Beijing Normal University, Beijing, China
Zhang Houcan
School of Psychology, Chengdu Medical University, Chengdu, China
You can also search for this author in PubMed Google Scholar
Corresponding author
Correspondence to He Dongjun .
Rights and permissions
Reprints and permissions
Copyright information
© 2024 Encyclopedia of China Publishing House
About this entry
Cite this entry.
Houcan, Z., Dongjun, H. (2024). Psychological Experimental Design. In: The ECPH Encyclopedia of Psychology. Springer, Singapore. https://doi.org/10.1007/978-981-99-6000-2_490-1
Download citation
DOI : https://doi.org/10.1007/978-981-99-6000-2_490-1
Received : 04 January 2024
Accepted : 05 January 2024
Published : 15 February 2024
Publisher Name : Springer, Singapore
Print ISBN : 978-981-99-6000-2
Online ISBN : 978-981-99-6000-2
eBook Packages : Springer Reference Behavioral Science and Psychology Reference Module Humanities and Social Sciences Reference Module Business, Economics and Social Sciences
- Publish with us
Policies and ethics
- Find a journal
- Track your research

Experimental Psychology: 10 Examples & Definition
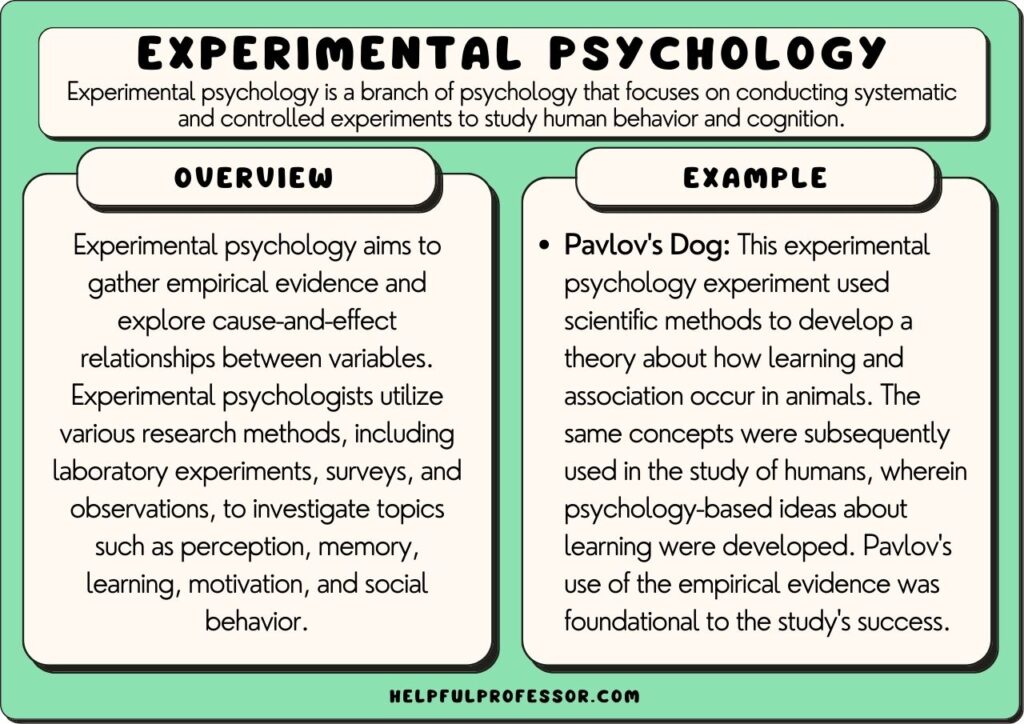
Experimental psychology refers to studying psychological phenomena using scientific methods. Originally, the primary scientific method involved manipulating one variable and observing systematic changes in another variable.
Today, psychologists utilize several types of scientific methodologies.
Experimental psychology examines a wide range of psychological phenomena, including: memory, sensation and perception, cognitive processes, motivation, emotion, developmental processes, in addition to the neurophysiological concomitants of each of these subjects.
Studies are conducted on both animal and human participants, and must comply with stringent requirements and controls regarding the ethical treatment of both.
Definition of Experimental Psychology
Experimental psychology is a branch of psychology that utilizes scientific methods to investigate the mind and behavior.
It involves the systematic and controlled study of human and animal behavior through observation and experimentation .
Experimental psychologists design and conduct experiments to understand cognitive processes, perception, learning, memory, emotion, and many other aspects of psychology. They often manipulate variables ( independent variables ) to see how this affects behavior or mental processes (dependent variables).
The findings from experimental psychology research are often used to better understand human behavior and can be applied in a range of contexts, such as education, health, business, and more.
Experimental Psychology Examples
1. The Puzzle Box Studies (Thorndike, 1898) Placing different cats in a box that can only be escaped by pulling a cord, and then taking detailed notes on how long it took for them to escape allowed Edward Thorndike to derive the Law of Effect: actions followed by positive consequences are more likely to occur again, and actions followed by negative consequences are less likely to occur again (Thorndike, 1898).
2. Reinforcement Schedules (Skinner, 1956) By placing rats in a Skinner Box and changing when and how often the rats are rewarded for pressing a lever, it is possible to identify how each schedule results in different behavior patterns (Skinner, 1956). This led to a wide range of theoretical ideas around how rewards and consequences can shape the behaviors of both animals and humans.
3. Observational Learning (Bandura, 1980) Some children watch a video of an adult punching and kicking a Bobo doll. Other children watch a video in which the adult plays nicely with the doll. By carefully observing the children’s behavior later when in a room with a Bobo doll, researchers can determine if television violence affects children’s behavior (Bandura, 1980).
4. The Fallibility of Memory (Loftus & Palmer, 1974) A group of participants watch the same video of two cars having an accident. Two weeks later, some are asked to estimate the rate of speed the cars were going when they “smashed” into each other. Some participants are asked to estimate the rate of speed the cars were going when they “bumped” into each other. Changing the phrasing of the question changes the memory of the eyewitness.
5. Intrinsic Motivation in the Classroom (Dweck, 1990) To investigate the role of autonomy on intrinsic motivation, half of the students are told they are “free to choose” which tasks to complete. The other half of the students are told they “must choose” some of the tasks. Researchers then carefully observe how long the students engage in the tasks and later ask them some questions about if they enjoyed doing the tasks or not.
6. Systematic Desensitization (Wolpe, 1958) A clinical psychologist carefully documents his treatment of a patient’s social phobia with progressive relaxation. At first, the patient is trained to monitor, tense, and relax various muscle groups while viewing photos of parties. Weeks later, they approach a stranger to ask for directions, initiate a conversation on a crowded bus, and attend a small social gathering. The therapist’s notes are transcribed into a scientific report and published in a peer-reviewed journal.
7. Study of Remembering (Bartlett, 1932) Bartlett’s work is a seminal study in the field of memory, where he used the concept of “schema” to describe an organized pattern of thought or behavior. He conducted a series of experiments using folk tales to show that memory recall is influenced by cultural schemas and personal experiences.
8. Study of Obedience (Milgram, 1963) This famous study explored the conflict between obedience to authority and personal conscience. Milgram found that a majority of participants were willing to administer what they believed were harmful electric shocks to a stranger when instructed by an authority figure, highlighting the power of authority and situational factors in driving behavior.
9. Pavlov’s Dog Study (Pavlov, 1927) Ivan Pavlov, a Russian physiologist, conducted a series of experiments that became a cornerstone in the field of experimental psychology. Pavlov noticed that dogs would salivate when they saw food. He then began to ring a bell each time he presented the food to the dogs. After a while, the dogs began to salivate merely at the sound of the bell. This experiment demonstrated the principle of “classical conditioning.”
10, Piaget’s Stages of Development (Piaget, 1958) Jean Piaget proposed a theory of cognitive development in children that consists of four distinct stages: the sensorimotor stage (birth to 2 years), where children learn about the world through their senses and motor activities, through to the the formal operational stage (12 years and beyond), where abstract reasoning and hypothetical thinking develop. Piaget’s theory is an example of experimental psychology as it was developed through systematic observation and experimentation on children’s problem-solving behaviors .
Types of Research Methodologies in Experimental Psychology
Researchers utilize several different types of research methodologies since the early days of Wundt (1832-1920).
1. The Experiment
The experiment involves the researcher manipulating the level of one variable, called the Independent Variable (IV), and then observing changes in another variable, called the Dependent Variable (DV).
The researcher is interested in determining if the IV causes changes in the DV. For example, does television violence make children more aggressive?
So, some children in the study, called research participants, will watch a show with TV violence, called the treatment group. Others will watch a show with no TV violence, called the control group.
So, there are two levels of the IV: violence and no violence. Next, children will be observed to see if they act more aggressively. This is the DV.
If TV violence makes children more aggressive, then the children that watched the violent show will me more aggressive than the children that watched the non-violent show.
A key requirement of the experiment is random assignment . Each research participant is assigned to one of the two groups in a way that makes it a completely random process. This means that each group will have a mix of children: different personality types, diverse family backgrounds, and range of intelligence levels.
2. The Longitudinal Study
A longitudinal study involves selecting a sample of participants and then following them for years, or decades, periodically collecting data on the variables of interest.
For example, a researcher might be interested in determining if parenting style affects academic performance of children. Parenting style is called the predictor variable , and academic performance is called the outcome variable .
Researchers will begin by randomly selecting a group of children to be in the study. Then, they will identify the type of parenting practices used when the children are 4 and 5 years old.
A few years later, perhaps when the children are 8 and 9, the researchers will collect data on their grades. This process can be repeated over the next 10 years, including through college.
If parenting style has an effect on academic performance, then the researchers will see a connection between the predictor variable and outcome variable.
Children raised with parenting style X will have higher grades than children raised with parenting style Y.
3. The Case Study
The case study is an in-depth study of one individual. This is a research methodology often used early in the examination of a psychological phenomenon or therapeutic treatment.
For example, in the early days of treating phobias, a clinical psychologist may try teaching one of their patients how to relax every time they see the object that creates so much fear and anxiety, such as a large spider.
The therapist would take very detailed notes on how the teaching process was implemented and the reactions of the patient. When the treatment had been completed, those notes would be written in a scientific form and submitted for publication in a scientific journal for other therapists to learn from.
There are several other types of methodologies available which vary different aspects of the three described above. The researcher will select a methodology that is most appropriate to the phenomenon they want to examine.
They also must take into account various practical considerations such as how much time and resources are needed to complete the study. Conducting research always costs money.
People and equipment are needed to carry-out every study, so researchers often try to obtain funding from their university or a government agency.
Origins and Key Developments in Experimental Psychology
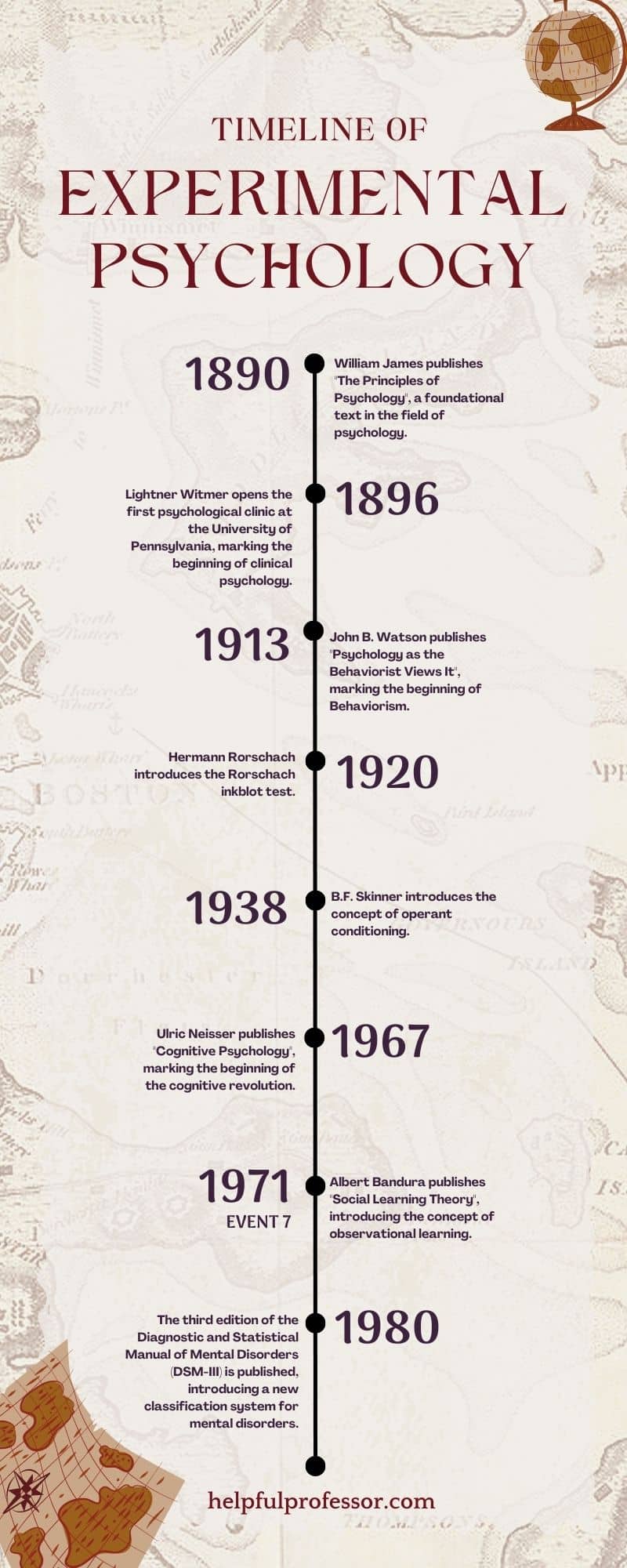
Wilhelm Maximilian Wundt (1832-1920) is considered one of the fathers of modern psychology. He was a physiologist and philosopher and helped establish psychology as a distinct discipline (Khaleefa, 1999).
In 1879 he established the world’s first psychology research lab at the University of Leipzig. This is considered a key milestone for establishing psychology as a scientific discipline. In addition to being the first person to use the term “psychologist,” to describe himself, he also founded the discipline’s first scientific journal Philosphische Studien in 1883.
Another notable figure in the development of experimental psychology is Ernest Weber . Trained as a physician, Weber studied sensation and perception and created the first quantitative law in psychology.
The equation denotes how judgments of sensory differences are relative to previous levels of sensation, referred to as the just-noticeable difference (jnd). This is known today as Weber’s Law (Hergenhahn, 2009).
Gustav Fechner , one of Weber’s students, published the first book on experimental psychology in 1860, titled Elemente der Psychophysik. His worked centered on the measurement of psychophysical facets of sensation and perception, with many of his methods still in use today.
The first American textbook on experimental psychology was Elements of Physiological Psychology, published in 1887 by George Trumball Ladd .
Ladd also established a psychology lab at Yale University, while Stanley Hall and Charles Sanders continued Wundt’s work at a lab at Johns Hopkins University.
In the late 1800s, Charles Pierce’s contribution to experimental psychology is especially noteworthy because he invented the concept of random assignment (Stigler, 1992; Dehue, 1997).
Go Deeper: 15 Random Assignment Examples
This procedure ensures that each participant has an equal chance of being placed in any of the experimental groups (e.g., treatment or control group). This eliminates the influence of confounding factors related to inherent characteristics of the participants.
Random assignment is a fundamental criterion for a study to be considered a valid experiment.
From there, experimental psychology flourished in the 20th century as a science and transformed into an approach utilized in cognitive psychology, developmental psychology, and social psychology .
Today, the term experimental psychology refers to the study of a wide range of phenomena and involves methodologies not limited to the manipulation of variables.
The Scientific Process and Experimental Psychology
The one thing that makes psychology a science and distinguishes it from its roots in philosophy is the reliance upon the scientific process to answer questions. This makes psychology a science was the main goal of its earliest founders such as Wilhelm Wundt.
There are numerous steps in the scientific process, outlined in the graphic below.
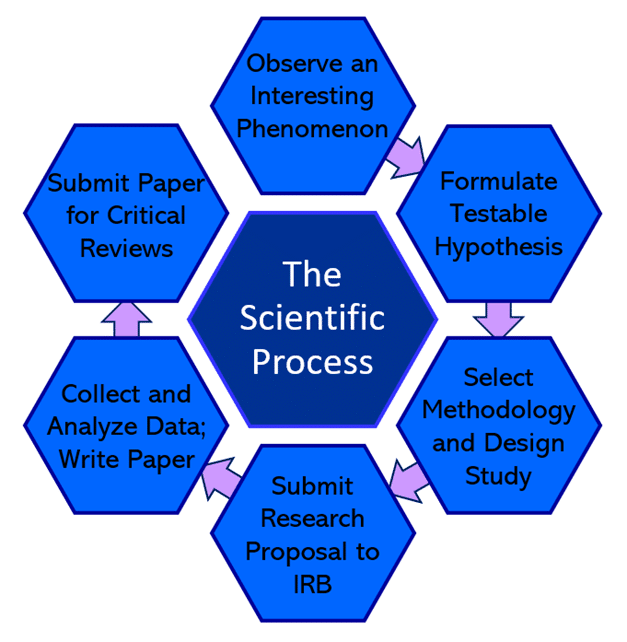
1. Observation
First, the scientist observes an interesting phenomenon that sparks a question. For example, are the memories of eyewitnesses really reliable, or are they subject to bias or unintentional manipulation?
2. Hypothesize
Next, this question is converted into a testable hypothesis. For instance: the words used to question a witness can influence what they think they remember.
3. Devise a Study
Then the researcher(s) select a methodology that will allow them to test that hypothesis. In this case, the researchers choose the experiment, which will involve randomly assigning some participants to different conditions.
In one condition, participants are asked a question that implies a certain memory (treatment group), while other participants are asked a question which is phrased neutrally and does not imply a certain memory (control group).
The researchers then write a proposal that describes in detail the procedures they want to use, how participants will be selected, and the safeguards they will employ to ensure the rights of the participants.
That proposal is submitted to an Institutional Review Board (IRB). The IRB is comprised of a panel of researchers, community representatives, and other professionals that are responsible for reviewing all studies involving human participants.
4. Conduct the Study
If the IRB accepts the proposal, then the researchers may begin collecting data. After the data has been collected, it is analyzed using a software program such as SPSS.
Those analyses will either support or reject the hypothesis. That is, either the participants’ memories were affected by the wording of the question, or not.
5. Publish the study
Finally, the researchers write a paper detailing their procedures and results of the statistical analyses. That paper is then submitted to a scientific journal.
The lead editor of that journal will then send copies of the paper to 3-5 experts in that subject. Each of those experts will read the paper and basically try to find as many things wrong with it as possible. Because they are experts, they are very good at this task.
After reading those critiques, most likely, the editor will send the paper back to the researchers and require that they respond to the criticisms, collect more data, or reject the paper outright.
In some cases, the study was so well-done that the criticisms were minimal and the editor accepts the paper. It then gets published in the scientific journal several months later.
That entire process can easily take 2 years, usually more. But, the findings of that study went through a very rigorous process. This means that we can have substantial confidence that the conclusions of the study are valid.
Experimental psychology refers to utilizing a scientific process to investigate psychological phenomenon.
There are a variety of methods employed today. They are used to study a wide range of subjects, including memory, cognitive processes, emotions and the neurophysiological basis of each.
The history of psychology as a science began in the 1800s primarily in Germany. As interest grew, the field expanded to the United States where several influential research labs were established.
As more methodologies were developed, the field of psychology as a science evolved into a prolific scientific discipline that has provided invaluable insights into human behavior.
Bartlett, F. C., & Bartlett, F. C. (1995). Remembering: A study in experimental and social psychology . Cambridge university press.
Dehue, T. (1997). Deception, efficiency, and random groups: Psychology and the gradual origination of the random group design. Isis , 88 (4), 653-673.
Ebbinghaus, H. (2013). Memory: A contribution to experimental psychology. Annals of neurosciences , 20 (4), 155.
Hergenhahn, B. R. (2009). An introduction to the history of psychology. Belmont. CA: Wadsworth Cengage Learning .
Khaleefa, O. (1999). Who is the founder of psychophysics and experimental psychology? American Journal of Islam and Society , 16 (2), 1-26.
Loftus, E. F., & Palmer, J. C. (1974). Reconstruction of auto-mobile destruction : An example of the interaction between language and memory. Journal of Verbal Learning and Verbal behavior , 13, 585-589.
Pavlov, I.P. (1927). Conditioned reflexes . Dover, New York.
Piaget, J. (1959). The language and thought of the child (Vol. 5). Psychology Press.
Piaget, J., Fraisse, P., & Reuchlin, M. (2014). Experimental psychology its scope and method: Volume I (Psychology Revivals): History and method . Psychology Press.
Skinner, B. F. (1956). A case history in scientlfic method. American Psychologist, 11 , 221-233
Stigler, S. M. (1992). A historical view of statistical concepts in psychology and educational research. American Journal of Education , 101 (1), 60-70.
Thorndike, E. L. (1898). Animal intelligence: An experimental study of the associative processes in animals. Psychological Review Monograph Supplement 2 .
Wolpe, J. (1958). Psychotherapy by reciprocal inhibition. Stanford, CA: Stanford University Press.
Appendix: Images reproduced as Text
Definition: Experimental psychology is a branch of psychology that focuses on conducting systematic and controlled experiments to study human behavior and cognition.
Overview: Experimental psychology aims to gather empirical evidence and explore cause-and-effect relationships between variables. Experimental psychologists utilize various research methods, including laboratory experiments, surveys, and observations, to investigate topics such as perception, memory, learning, motivation, and social behavior .
Example: The Pavlov’s Dog experimental psychology experiment used scientific methods to develop a theory about how learning and association occur in animals. The same concepts were subsequently used in the study of humans, wherein psychology-based ideas about learning were developed. Pavlov’s use of the empirical evidence was foundational to the study’s success.
Experimental Psychology Milestones:
1890: William James publishes “The Principles of Psychology”, a foundational text in the field of psychology.
1896: Lightner Witmer opens the first psychological clinic at the University of Pennsylvania, marking the beginning of clinical psychology.
1913: John B. Watson publishes “Psychology as the Behaviorist Views It”, marking the beginning of Behaviorism.
1920: Hermann Rorschach introduces the Rorschach inkblot test.
1938: B.F. Skinner introduces the concept of operant conditioning .
1967: Ulric Neisser publishes “Cognitive Psychology” , marking the beginning of the cognitive revolution.
1980: The third edition of the Diagnostic and Statistical Manual of Mental Disorders (DSM-III) is published, introducing a new classification system for mental disorders.
The Scientific Process
- Observe an interesting phenomenon
- Formulate testable hypothesis
- Select methodology and design study
- Submit research proposal to IRB
- Collect and analyzed data; write paper
- Submit paper for critical reviews
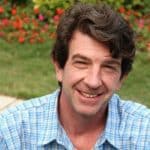
Dave Cornell (PhD)
Dr. Cornell has worked in education for more than 20 years. His work has involved designing teacher certification for Trinity College in London and in-service training for state governments in the United States. He has trained kindergarten teachers in 8 countries and helped businessmen and women open baby centers and kindergartens in 3 countries.
- Dave Cornell (PhD) https://helpfulprofessor.com/author/dave-cornell-phd/ 25 Positive Punishment Examples
- Dave Cornell (PhD) https://helpfulprofessor.com/author/dave-cornell-phd/ 25 Dissociation Examples (Psychology)
- Dave Cornell (PhD) https://helpfulprofessor.com/author/dave-cornell-phd/ 15 Zone of Proximal Development Examples
- Dave Cornell (PhD) https://helpfulprofessor.com/author/dave-cornell-phd/ Perception Checking: 15 Examples and Definition
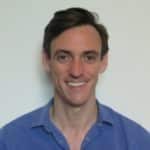
Chris Drew (PhD)
This article was peer-reviewed and edited by Chris Drew (PhD). The review process on Helpful Professor involves having a PhD level expert fact check, edit, and contribute to articles. Reviewers ensure all content reflects expert academic consensus and is backed up with reference to academic studies. Dr. Drew has published over 20 academic articles in scholarly journals. He is the former editor of the Journal of Learning Development in Higher Education and holds a PhD in Education from ACU.
- Chris Drew (PhD) #molongui-disabled-link 25 Positive Punishment Examples
- Chris Drew (PhD) #molongui-disabled-link 25 Dissociation Examples (Psychology)
- Chris Drew (PhD) #molongui-disabled-link 15 Zone of Proximal Development Examples
- Chris Drew (PhD) #molongui-disabled-link Perception Checking: 15 Examples and Definition
Leave a Comment Cancel Reply
Your email address will not be published. Required fields are marked *

Want to create or adapt books like this? Learn more about how Pressbooks supports open publishing practices.
6.2 Experimental Design
Learning objectives.
- Explain the difference between between-subjects and within-subjects experiments, list some of the pros and cons of each approach, and decide which approach to use to answer a particular research question.
- Define random assignment, distinguish it from random sampling, explain its purpose in experimental research, and use some simple strategies to implement it.
- Define what a control condition is, explain its purpose in research on treatment effectiveness, and describe some alternative types of control conditions.
- Define several types of carryover effect, give examples of each, and explain how counterbalancing helps to deal with them.
In this section, we look at some different ways to design an experiment. The primary distinction we will make is between approaches in which each participant experiences one level of the independent variable and approaches in which each participant experiences all levels of the independent variable. The former are called between-subjects experiments and the latter are called within-subjects experiments.
Between-Subjects Experiments
In a between-subjects experiment , each participant is tested in only one condition. For example, a researcher with a sample of 100 college students might assign half of them to write about a traumatic event and the other half write about a neutral event. Or a researcher with a sample of 60 people with severe agoraphobia (fear of open spaces) might assign 20 of them to receive each of three different treatments for that disorder. It is essential in a between-subjects experiment that the researcher assign participants to conditions so that the different groups are, on average, highly similar to each other. Those in a trauma condition and a neutral condition, for example, should include a similar proportion of men and women, and they should have similar average intelligence quotients (IQs), similar average levels of motivation, similar average numbers of health problems, and so on. This is a matter of controlling these extraneous participant variables across conditions so that they do not become confounding variables.
Random Assignment
The primary way that researchers accomplish this kind of control of extraneous variables across conditions is called random assignment , which means using a random process to decide which participants are tested in which conditions. Do not confuse random assignment with random sampling. Random sampling is a method for selecting a sample from a population, and it is rarely used in psychological research. Random assignment is a method for assigning participants in a sample to the different conditions, and it is an important element of all experimental research in psychology and other fields too.
In its strictest sense, random assignment should meet two criteria. One is that each participant has an equal chance of being assigned to each condition (e.g., a 50% chance of being assigned to each of two conditions). The second is that each participant is assigned to a condition independently of other participants. Thus one way to assign participants to two conditions would be to flip a coin for each one. If the coin lands heads, the participant is assigned to Condition A, and if it lands tails, the participant is assigned to Condition B. For three conditions, one could use a computer to generate a random integer from 1 to 3 for each participant. If the integer is 1, the participant is assigned to Condition A; if it is 2, the participant is assigned to Condition B; and if it is 3, the participant is assigned to Condition C. In practice, a full sequence of conditions—one for each participant expected to be in the experiment—is usually created ahead of time, and each new participant is assigned to the next condition in the sequence as he or she is tested. When the procedure is computerized, the computer program often handles the random assignment.
One problem with coin flipping and other strict procedures for random assignment is that they are likely to result in unequal sample sizes in the different conditions. Unequal sample sizes are generally not a serious problem, and you should never throw away data you have already collected to achieve equal sample sizes. However, for a fixed number of participants, it is statistically most efficient to divide them into equal-sized groups. It is standard practice, therefore, to use a kind of modified random assignment that keeps the number of participants in each group as similar as possible. One approach is block randomization . In block randomization, all the conditions occur once in the sequence before any of them is repeated. Then they all occur again before any of them is repeated again. Within each of these “blocks,” the conditions occur in a random order. Again, the sequence of conditions is usually generated before any participants are tested, and each new participant is assigned to the next condition in the sequence. Table 6.2 “Block Randomization Sequence for Assigning Nine Participants to Three Conditions” shows such a sequence for assigning nine participants to three conditions. The Research Randomizer website ( http://www.randomizer.org ) will generate block randomization sequences for any number of participants and conditions. Again, when the procedure is computerized, the computer program often handles the block randomization.
Table 6.2 Block Randomization Sequence for Assigning Nine Participants to Three Conditions
Random assignment is not guaranteed to control all extraneous variables across conditions. It is always possible that just by chance, the participants in one condition might turn out to be substantially older, less tired, more motivated, or less depressed on average than the participants in another condition. However, there are some reasons that this is not a major concern. One is that random assignment works better than one might expect, especially for large samples. Another is that the inferential statistics that researchers use to decide whether a difference between groups reflects a difference in the population takes the “fallibility” of random assignment into account. Yet another reason is that even if random assignment does result in a confounding variable and therefore produces misleading results, this is likely to be detected when the experiment is replicated. The upshot is that random assignment to conditions—although not infallible in terms of controlling extraneous variables—is always considered a strength of a research design.
Treatment and Control Conditions
Between-subjects experiments are often used to determine whether a treatment works. In psychological research, a treatment is any intervention meant to change people’s behavior for the better. This includes psychotherapies and medical treatments for psychological disorders but also interventions designed to improve learning, promote conservation, reduce prejudice, and so on. To determine whether a treatment works, participants are randomly assigned to either a treatment condition , in which they receive the treatment, or a control condition , in which they do not receive the treatment. If participants in the treatment condition end up better off than participants in the control condition—for example, they are less depressed, learn faster, conserve more, express less prejudice—then the researcher can conclude that the treatment works. In research on the effectiveness of psychotherapies and medical treatments, this type of experiment is often called a randomized clinical trial .
There are different types of control conditions. In a no-treatment control condition , participants receive no treatment whatsoever. One problem with this approach, however, is the existence of placebo effects. A placebo is a simulated treatment that lacks any active ingredient or element that should make it effective, and a placebo effect is a positive effect of such a treatment. Many folk remedies that seem to work—such as eating chicken soup for a cold or placing soap under the bedsheets to stop nighttime leg cramps—are probably nothing more than placebos. Although placebo effects are not well understood, they are probably driven primarily by people’s expectations that they will improve. Having the expectation to improve can result in reduced stress, anxiety, and depression, which can alter perceptions and even improve immune system functioning (Price, Finniss, & Benedetti, 2008).
Placebo effects are interesting in their own right (see Note 6.28 “The Powerful Placebo” ), but they also pose a serious problem for researchers who want to determine whether a treatment works. Figure 6.2 “Hypothetical Results From a Study Including Treatment, No-Treatment, and Placebo Conditions” shows some hypothetical results in which participants in a treatment condition improved more on average than participants in a no-treatment control condition. If these conditions (the two leftmost bars in Figure 6.2 “Hypothetical Results From a Study Including Treatment, No-Treatment, and Placebo Conditions” ) were the only conditions in this experiment, however, one could not conclude that the treatment worked. It could be instead that participants in the treatment group improved more because they expected to improve, while those in the no-treatment control condition did not.
Figure 6.2 Hypothetical Results From a Study Including Treatment, No-Treatment, and Placebo Conditions

Fortunately, there are several solutions to this problem. One is to include a placebo control condition , in which participants receive a placebo that looks much like the treatment but lacks the active ingredient or element thought to be responsible for the treatment’s effectiveness. When participants in a treatment condition take a pill, for example, then those in a placebo control condition would take an identical-looking pill that lacks the active ingredient in the treatment (a “sugar pill”). In research on psychotherapy effectiveness, the placebo might involve going to a psychotherapist and talking in an unstructured way about one’s problems. The idea is that if participants in both the treatment and the placebo control groups expect to improve, then any improvement in the treatment group over and above that in the placebo control group must have been caused by the treatment and not by participants’ expectations. This is what is shown by a comparison of the two outer bars in Figure 6.2 “Hypothetical Results From a Study Including Treatment, No-Treatment, and Placebo Conditions” .
Of course, the principle of informed consent requires that participants be told that they will be assigned to either a treatment or a placebo control condition—even though they cannot be told which until the experiment ends. In many cases the participants who had been in the control condition are then offered an opportunity to have the real treatment. An alternative approach is to use a waitlist control condition , in which participants are told that they will receive the treatment but must wait until the participants in the treatment condition have already received it. This allows researchers to compare participants who have received the treatment with participants who are not currently receiving it but who still expect to improve (eventually). A final solution to the problem of placebo effects is to leave out the control condition completely and compare any new treatment with the best available alternative treatment. For example, a new treatment for simple phobia could be compared with standard exposure therapy. Because participants in both conditions receive a treatment, their expectations about improvement should be similar. This approach also makes sense because once there is an effective treatment, the interesting question about a new treatment is not simply “Does it work?” but “Does it work better than what is already available?”
The Powerful Placebo
Many people are not surprised that placebos can have a positive effect on disorders that seem fundamentally psychological, including depression, anxiety, and insomnia. However, placebos can also have a positive effect on disorders that most people think of as fundamentally physiological. These include asthma, ulcers, and warts (Shapiro & Shapiro, 1999). There is even evidence that placebo surgery—also called “sham surgery”—can be as effective as actual surgery.
Medical researcher J. Bruce Moseley and his colleagues conducted a study on the effectiveness of two arthroscopic surgery procedures for osteoarthritis of the knee (Moseley et al., 2002). The control participants in this study were prepped for surgery, received a tranquilizer, and even received three small incisions in their knees. But they did not receive the actual arthroscopic surgical procedure. The surprising result was that all participants improved in terms of both knee pain and function, and the sham surgery group improved just as much as the treatment groups. According to the researchers, “This study provides strong evidence that arthroscopic lavage with or without débridement [the surgical procedures used] is not better than and appears to be equivalent to a placebo procedure in improving knee pain and self-reported function” (p. 85).

Research has shown that patients with osteoarthritis of the knee who receive a “sham surgery” experience reductions in pain and improvement in knee function similar to those of patients who receive a real surgery.
Army Medicine – Surgery – CC BY 2.0.
Within-Subjects Experiments
In a within-subjects experiment , each participant is tested under all conditions. Consider an experiment on the effect of a defendant’s physical attractiveness on judgments of his guilt. Again, in a between-subjects experiment, one group of participants would be shown an attractive defendant and asked to judge his guilt, and another group of participants would be shown an unattractive defendant and asked to judge his guilt. In a within-subjects experiment, however, the same group of participants would judge the guilt of both an attractive and an unattractive defendant.
The primary advantage of this approach is that it provides maximum control of extraneous participant variables. Participants in all conditions have the same mean IQ, same socioeconomic status, same number of siblings, and so on—because they are the very same people. Within-subjects experiments also make it possible to use statistical procedures that remove the effect of these extraneous participant variables on the dependent variable and therefore make the data less “noisy” and the effect of the independent variable easier to detect. We will look more closely at this idea later in the book.
Carryover Effects and Counterbalancing
The primary disadvantage of within-subjects designs is that they can result in carryover effects. A carryover effect is an effect of being tested in one condition on participants’ behavior in later conditions. One type of carryover effect is a practice effect , where participants perform a task better in later conditions because they have had a chance to practice it. Another type is a fatigue effect , where participants perform a task worse in later conditions because they become tired or bored. Being tested in one condition can also change how participants perceive stimuli or interpret their task in later conditions. This is called a context effect . For example, an average-looking defendant might be judged more harshly when participants have just judged an attractive defendant than when they have just judged an unattractive defendant. Within-subjects experiments also make it easier for participants to guess the hypothesis. For example, a participant who is asked to judge the guilt of an attractive defendant and then is asked to judge the guilt of an unattractive defendant is likely to guess that the hypothesis is that defendant attractiveness affects judgments of guilt. This could lead the participant to judge the unattractive defendant more harshly because he thinks this is what he is expected to do. Or it could make participants judge the two defendants similarly in an effort to be “fair.”
Carryover effects can be interesting in their own right. (Does the attractiveness of one person depend on the attractiveness of other people that we have seen recently?) But when they are not the focus of the research, carryover effects can be problematic. Imagine, for example, that participants judge the guilt of an attractive defendant and then judge the guilt of an unattractive defendant. If they judge the unattractive defendant more harshly, this might be because of his unattractiveness. But it could be instead that they judge him more harshly because they are becoming bored or tired. In other words, the order of the conditions is a confounding variable. The attractive condition is always the first condition and the unattractive condition the second. Thus any difference between the conditions in terms of the dependent variable could be caused by the order of the conditions and not the independent variable itself.
There is a solution to the problem of order effects, however, that can be used in many situations. It is counterbalancing , which means testing different participants in different orders. For example, some participants would be tested in the attractive defendant condition followed by the unattractive defendant condition, and others would be tested in the unattractive condition followed by the attractive condition. With three conditions, there would be six different orders (ABC, ACB, BAC, BCA, CAB, and CBA), so some participants would be tested in each of the six orders. With counterbalancing, participants are assigned to orders randomly, using the techniques we have already discussed. Thus random assignment plays an important role in within-subjects designs just as in between-subjects designs. Here, instead of randomly assigning to conditions, they are randomly assigned to different orders of conditions. In fact, it can safely be said that if a study does not involve random assignment in one form or another, it is not an experiment.
There are two ways to think about what counterbalancing accomplishes. One is that it controls the order of conditions so that it is no longer a confounding variable. Instead of the attractive condition always being first and the unattractive condition always being second, the attractive condition comes first for some participants and second for others. Likewise, the unattractive condition comes first for some participants and second for others. Thus any overall difference in the dependent variable between the two conditions cannot have been caused by the order of conditions. A second way to think about what counterbalancing accomplishes is that if there are carryover effects, it makes it possible to detect them. One can analyze the data separately for each order to see whether it had an effect.
When 9 Is “Larger” Than 221
Researcher Michael Birnbaum has argued that the lack of context provided by between-subjects designs is often a bigger problem than the context effects created by within-subjects designs. To demonstrate this, he asked one group of participants to rate how large the number 9 was on a 1-to-10 rating scale and another group to rate how large the number 221 was on the same 1-to-10 rating scale (Birnbaum, 1999). Participants in this between-subjects design gave the number 9 a mean rating of 5.13 and the number 221 a mean rating of 3.10. In other words, they rated 9 as larger than 221! According to Birnbaum, this is because participants spontaneously compared 9 with other one-digit numbers (in which case it is relatively large) and compared 221 with other three-digit numbers (in which case it is relatively small).
Simultaneous Within-Subjects Designs
So far, we have discussed an approach to within-subjects designs in which participants are tested in one condition at a time. There is another approach, however, that is often used when participants make multiple responses in each condition. Imagine, for example, that participants judge the guilt of 10 attractive defendants and 10 unattractive defendants. Instead of having people make judgments about all 10 defendants of one type followed by all 10 defendants of the other type, the researcher could present all 20 defendants in a sequence that mixed the two types. The researcher could then compute each participant’s mean rating for each type of defendant. Or imagine an experiment designed to see whether people with social anxiety disorder remember negative adjectives (e.g., “stupid,” “incompetent”) better than positive ones (e.g., “happy,” “productive”). The researcher could have participants study a single list that includes both kinds of words and then have them try to recall as many words as possible. The researcher could then count the number of each type of word that was recalled. There are many ways to determine the order in which the stimuli are presented, but one common way is to generate a different random order for each participant.
Between-Subjects or Within-Subjects?
Almost every experiment can be conducted using either a between-subjects design or a within-subjects design. This means that researchers must choose between the two approaches based on their relative merits for the particular situation.
Between-subjects experiments have the advantage of being conceptually simpler and requiring less testing time per participant. They also avoid carryover effects without the need for counterbalancing. Within-subjects experiments have the advantage of controlling extraneous participant variables, which generally reduces noise in the data and makes it easier to detect a relationship between the independent and dependent variables.
A good rule of thumb, then, is that if it is possible to conduct a within-subjects experiment (with proper counterbalancing) in the time that is available per participant—and you have no serious concerns about carryover effects—this is probably the best option. If a within-subjects design would be difficult or impossible to carry out, then you should consider a between-subjects design instead. For example, if you were testing participants in a doctor’s waiting room or shoppers in line at a grocery store, you might not have enough time to test each participant in all conditions and therefore would opt for a between-subjects design. Or imagine you were trying to reduce people’s level of prejudice by having them interact with someone of another race. A within-subjects design with counterbalancing would require testing some participants in the treatment condition first and then in a control condition. But if the treatment works and reduces people’s level of prejudice, then they would no longer be suitable for testing in the control condition. This is true for many designs that involve a treatment meant to produce long-term change in participants’ behavior (e.g., studies testing the effectiveness of psychotherapy). Clearly, a between-subjects design would be necessary here.
Remember also that using one type of design does not preclude using the other type in a different study. There is no reason that a researcher could not use both a between-subjects design and a within-subjects design to answer the same research question. In fact, professional researchers often do exactly this.
Key Takeaways
- Experiments can be conducted using either between-subjects or within-subjects designs. Deciding which to use in a particular situation requires careful consideration of the pros and cons of each approach.
- Random assignment to conditions in between-subjects experiments or to orders of conditions in within-subjects experiments is a fundamental element of experimental research. Its purpose is to control extraneous variables so that they do not become confounding variables.
- Experimental research on the effectiveness of a treatment requires both a treatment condition and a control condition, which can be a no-treatment control condition, a placebo control condition, or a waitlist control condition. Experimental treatments can also be compared with the best available alternative.
Discussion: For each of the following topics, list the pros and cons of a between-subjects and within-subjects design and decide which would be better.
- You want to test the relative effectiveness of two training programs for running a marathon.
- Using photographs of people as stimuli, you want to see if smiling people are perceived as more intelligent than people who are not smiling.
- In a field experiment, you want to see if the way a panhandler is dressed (neatly vs. sloppily) affects whether or not passersby give him any money.
- You want to see if concrete nouns (e.g., dog ) are recalled better than abstract nouns (e.g., truth ).
- Discussion: Imagine that an experiment shows that participants who receive psychodynamic therapy for a dog phobia improve more than participants in a no-treatment control group. Explain a fundamental problem with this research design and at least two ways that it might be corrected.
Birnbaum, M. H. (1999). How to show that 9 > 221: Collect judgments in a between-subjects design. Psychological Methods, 4 , 243–249.
Moseley, J. B., O’Malley, K., Petersen, N. J., Menke, T. J., Brody, B. A., Kuykendall, D. H., … Wray, N. P. (2002). A controlled trial of arthroscopic surgery for osteoarthritis of the knee. The New England Journal of Medicine, 347 , 81–88.
Price, D. D., Finniss, D. G., & Benedetti, F. (2008). A comprehensive review of the placebo effect: Recent advances and current thought. Annual Review of Psychology, 59 , 565–590.
Shapiro, A. K., & Shapiro, E. (1999). The powerful placebo: From ancient priest to modern physician . Baltimore, MD: Johns Hopkins University Press.
Research Methods in Psychology Copyright © 2016 by University of Minnesota is licensed under a Creative Commons Attribution-NonCommercial-ShareAlike 4.0 International License , except where otherwise noted.
5.2 Experimental Design
Learning objectives.
- Explain the difference between between-subjects and within-subjects experiments, list some of the pros and cons of each approach, and decide which approach to use to answer a particular research question.
- Define random assignment, distinguish it from random sampling, explain its purpose in experimental research, and use some simple strategies to implement it
- Define several types of carryover effect, give examples of each, and explain how counterbalancing helps to deal with them.
In this section, we look at some different ways to design an experiment. The primary distinction we will make is between approaches in which each participant experiences one level of the independent variable and approaches in which each participant experiences all levels of the independent variable. The former are called between-subjects experiments and the latter are called within-subjects experiments.
Between-Subjects Experiments
In a between-subjects experiment , each participant is tested in only one condition. For example, a researcher with a sample of 100 university students might assign half of them to write about a traumatic event and the other half write about a neutral event. Or a researcher with a sample of 60 people with severe agoraphobia (fear of open spaces) might assign 20 of them to receive each of three different treatments for that disorder. It is essential in a between-subjects experiment that the researcher assigns participants to conditions so that the different groups are, on average, highly similar to each other. Those in a trauma condition and a neutral condition, for example, should include a similar proportion of men and women, and they should have similar average intelligence quotients (IQs), similar average levels of motivation, similar average numbers of health problems, and so on. This matching is a matter of controlling these extraneous participant variables across conditions so that they do not become confounding variables.
Random Assignment
The primary way that researchers accomplish this kind of control of extraneous variables across conditions is called random assignment , which means using a random process to decide which participants are tested in which conditions. Do not confuse random assignment with random sampling. Random sampling is a method for selecting a sample from a population, and it is rarely used in psychological research. Random assignment is a method for assigning participants in a sample to the different conditions, and it is an important element of all experimental research in psychology and other fields too.
In its strictest sense, random assignment should meet two criteria. One is that each participant has an equal chance of being assigned to each condition (e.g., a 50% chance of being assigned to each of two conditions). The second is that each participant is assigned to a condition independently of other participants. Thus one way to assign participants to two conditions would be to flip a coin for each one. If the coin lands heads, the participant is assigned to Condition A, and if it lands tails, the participant is assigned to Condition B. For three conditions, one could use a computer to generate a random integer from 1 to 3 for each participant. If the integer is 1, the participant is assigned to Condition A; if it is 2, the participant is assigned to Condition B; and if it is 3, the participant is assigned to Condition C. In practice, a full sequence of conditions—one for each participant expected to be in the experiment—is usually created ahead of time, and each new participant is assigned to the next condition in the sequence as he or she is tested. When the procedure is computerized, the computer program often handles the random assignment.
One problem with coin flipping and other strict procedures for random assignment is that they are likely to result in unequal sample sizes in the different conditions. Unequal sample sizes are generally not a serious problem, and you should never throw away data you have already collected to achieve equal sample sizes. However, for a fixed number of participants, it is statistically most efficient to divide them into equal-sized groups. It is standard practice, therefore, to use a kind of modified random assignment that keeps the number of participants in each group as similar as possible. One approach is block randomization . In block randomization, all the conditions occur once in the sequence before any of them is repeated. Then they all occur again before any of them is repeated again. Within each of these “blocks,” the conditions occur in a random order. Again, the sequence of conditions is usually generated before any participants are tested, and each new participant is assigned to the next condition in the sequence. Table 5.2 shows such a sequence for assigning nine participants to three conditions. The Research Randomizer website ( http://www.randomizer.org ) will generate block randomization sequences for any number of participants and conditions. Again, when the procedure is computerized, the computer program often handles the block randomization.
Random assignment is not guaranteed to control all extraneous variables across conditions. The process is random, so it is always possible that just by chance, the participants in one condition might turn out to be substantially older, less tired, more motivated, or less depressed on average than the participants in another condition. However, there are some reasons that this possibility is not a major concern. One is that random assignment works better than one might expect, especially for large samples. Another is that the inferential statistics that researchers use to decide whether a difference between groups reflects a difference in the population takes the “fallibility” of random assignment into account. Yet another reason is that even if random assignment does result in a confounding variable and therefore produces misleading results, this confound is likely to be detected when the experiment is replicated. The upshot is that random assignment to conditions—although not infallible in terms of controlling extraneous variables—is always considered a strength of a research design.
Matched Groups
An alternative to simple random assignment of participants to conditions is the use of a matched-groups design . Using this design, participants in the various conditions are matched on the dependent variable or on some extraneous variable(s) prior the manipulation of the independent variable. This guarantees that these variables will not be confounded across the experimental conditions. For instance, if we want to determine whether expressive writing affects people’s health then we could start by measuring various health-related variables in our prospective research participants. We could then use that information to rank-order participants according to how healthy or unhealthy they are. Next, the two healthiest participants would be randomly assigned to complete different conditions (one would be randomly assigned to the traumatic experiences writing condition and the other to the neutral writing condition). The next two healthiest participants would then be randomly assigned to complete different conditions, and so on until the two least healthy participants. This method would ensure that participants in the traumatic experiences writing condition are matched to participants in the neutral writing condition with respect to health at the beginning of the study. If at the end of the experiment, a difference in health was detected across the two conditions, then we would know that it is due to the writing manipulation and not to pre-existing differences in health.
Within-Subjects Experiments
In a within-subjects experiment , each participant is tested under all conditions. Consider an experiment on the effect of a defendant’s physical attractiveness on judgments of his guilt. Again, in a between-subjects experiment, one group of participants would be shown an attractive defendant and asked to judge his guilt, and another group of participants would be shown an unattractive defendant and asked to judge his guilt. In a within-subjects experiment, however, the same group of participants would judge the guilt of both an attractive and an unattractive defendant.
The primary advantage of this approach is that it provides maximum control of extraneous participant variables. Participants in all conditions have the same mean IQ, same socioeconomic status, same number of siblings, and so on—because they are the very same people. Within-subjects experiments also make it possible to use statistical procedures that remove the effect of these extraneous participant variables on the dependent variable and therefore make the data less “noisy” and the effect of the independent variable easier to detect. We will look more closely at this idea later in the book . However, not all experiments can use a within-subjects design nor would it be desirable to do so.
One disadvantage of within-subjects experiments is that they make it easier for participants to guess the hypothesis. For example, a participant who is asked to judge the guilt of an attractive defendant and then is asked to judge the guilt of an unattractive defendant is likely to guess that the hypothesis is that defendant attractiveness affects judgments of guilt. This knowledge could lead the participant to judge the unattractive defendant more harshly because he thinks this is what he is expected to do. Or it could make participants judge the two defendants similarly in an effort to be “fair.”
Carryover Effects and Counterbalancing
The primary disadvantage of within-subjects designs is that they can result in order effects. An order effect occurs when participants’ responses in the various conditions are affected by the order of conditions to which they were exposed. One type of order effect is a carryover effect. A carryover effect is an effect of being tested in one condition on participants’ behavior in later conditions. One type of carryover effect is a practice effect , where participants perform a task better in later conditions because they have had a chance to practice it. Another type is a fatigue effect , where participants perform a task worse in later conditions because they become tired or bored. Being tested in one condition can also change how participants perceive stimuli or interpret their task in later conditions. This type of effect is called a context effect (or contrast effect) . For example, an average-looking defendant might be judged more harshly when participants have just judged an attractive defendant than when they have just judged an unattractive defendant. Within-subjects experiments also make it easier for participants to guess the hypothesis. For example, a participant who is asked to judge the guilt of an attractive defendant and then is asked to judge the guilt of an unattractive defendant is likely to guess that the hypothesis is that defendant attractiveness affects judgments of guilt.
Carryover effects can be interesting in their own right. (Does the attractiveness of one person depend on the attractiveness of other people that we have seen recently?) But when they are not the focus of the research, carryover effects can be problematic. Imagine, for example, that participants judge the guilt of an attractive defendant and then judge the guilt of an unattractive defendant. If they judge the unattractive defendant more harshly, this might be because of his unattractiveness. But it could be instead that they judge him more harshly because they are becoming bored or tired. In other words, the order of the conditions is a confounding variable. The attractive condition is always the first condition and the unattractive condition the second. Thus any difference between the conditions in terms of the dependent variable could be caused by the order of the conditions and not the independent variable itself.
There is a solution to the problem of order effects, however, that can be used in many situations. It is counterbalancing , which means testing different participants in different orders. The best method of counterbalancing is complete counterbalancing in which an equal number of participants complete each possible order of conditions. For example, half of the participants would be tested in the attractive defendant condition followed by the unattractive defendant condition, and others half would be tested in the unattractive condition followed by the attractive condition. With three conditions, there would be six different orders (ABC, ACB, BAC, BCA, CAB, and CBA), so some participants would be tested in each of the six orders. With four conditions, there would be 24 different orders; with five conditions there would be 120 possible orders. With counterbalancing, participants are assigned to orders randomly, using the techniques we have already discussed. Thus, random assignment plays an important role in within-subjects designs just as in between-subjects designs. Here, instead of randomly assigning to conditions, they are randomly assigned to different orders of conditions. In fact, it can safely be said that if a study does not involve random assignment in one form or another, it is not an experiment.
A more efficient way of counterbalancing is through a Latin square design which randomizes through having equal rows and columns. For example, if you have four treatments, you must have four versions. Like a Sudoku puzzle, no treatment can repeat in a row or column. For four versions of four treatments, the Latin square design would look like:
You can see in the diagram above that the square has been constructed to ensure that each condition appears at each ordinal position (A appears first once, second once, third once, and fourth once) and each condition preceded and follows each other condition one time. A Latin square for an experiment with 6 conditions would by 6 x 6 in dimension, one for an experiment with 8 conditions would be 8 x 8 in dimension, and so on. So while complete counterbalancing of 6 conditions would require 720 orders, a Latin square would only require 6 orders.
Finally, when the number of conditions is large experiments can use random counterbalancing in which the order of the conditions is randomly determined for each participant. Using this technique every possible order of conditions is determined and then one of these orders is randomly selected for each participant. This is not as powerful a technique as complete counterbalancing or partial counterbalancing using a Latin squares design. Use of random counterbalancing will result in more random error, but if order effects are likely to be small and the number of conditions is large, this is an option available to researchers.
There are two ways to think about what counterbalancing accomplishes. One is that it controls the order of conditions so that it is no longer a confounding variable. Instead of the attractive condition always being first and the unattractive condition always being second, the attractive condition comes first for some participants and second for others. Likewise, the unattractive condition comes first for some participants and second for others. Thus any overall difference in the dependent variable between the two conditions cannot have been caused by the order of conditions. A second way to think about what counterbalancing accomplishes is that if there are carryover effects, it makes it possible to detect them. One can analyze the data separately for each order to see whether it had an effect.
When 9 Is “Larger” Than 221
Researcher Michael Birnbaum has argued that the lack of context provided by between-subjects designs is often a bigger problem than the context effects created by within-subjects designs. To demonstrate this problem, he asked participants to rate two numbers on how large they were on a scale of 1-to-10 where 1 was “very very small” and 10 was “very very large”. One group of participants were asked to rate the number 9 and another group was asked to rate the number 221 (Birnbaum, 1999) [1] . Participants in this between-subjects design gave the number 9 a mean rating of 5.13 and the number 221 a mean rating of 3.10. In other words, they rated 9 as larger than 221! According to Birnbaum, this difference is because participants spontaneously compared 9 with other one-digit numbers (in which case it is relatively large) and compared 221 with other three-digit numbers (in which case it is relatively small).
Simultaneous Within-Subjects Designs
So far, we have discussed an approach to within-subjects designs in which participants are tested in one condition at a time. There is another approach, however, that is often used when participants make multiple responses in each condition. Imagine, for example, that participants judge the guilt of 10 attractive defendants and 10 unattractive defendants. Instead of having people make judgments about all 10 defendants of one type followed by all 10 defendants of the other type, the researcher could present all 20 defendants in a sequence that mixed the two types. The researcher could then compute each participant’s mean rating for each type of defendant. Or imagine an experiment designed to see whether people with social anxiety disorder remember negative adjectives (e.g., “stupid,” “incompetent”) better than positive ones (e.g., “happy,” “productive”). The researcher could have participants study a single list that includes both kinds of words and then have them try to recall as many words as possible. The researcher could then count the number of each type of word that was recalled.
Between-Subjects or Within-Subjects?
Almost every experiment can be conducted using either a between-subjects design or a within-subjects design. This possibility means that researchers must choose between the two approaches based on their relative merits for the particular situation.
Between-subjects experiments have the advantage of being conceptually simpler and requiring less testing time per participant. They also avoid carryover effects without the need for counterbalancing. Within-subjects experiments have the advantage of controlling extraneous participant variables, which generally reduces noise in the data and makes it easier to detect a relationship between the independent and dependent variables.
A good rule of thumb, then, is that if it is possible to conduct a within-subjects experiment (with proper counterbalancing) in the time that is available per participant—and you have no serious concerns about carryover effects—this design is probably the best option. If a within-subjects design would be difficult or impossible to carry out, then you should consider a between-subjects design instead. For example, if you were testing participants in a doctor’s waiting room or shoppers in line at a grocery store, you might not have enough time to test each participant in all conditions and therefore would opt for a between-subjects design. Or imagine you were trying to reduce people’s level of prejudice by having them interact with someone of another race. A within-subjects design with counterbalancing would require testing some participants in the treatment condition first and then in a control condition. But if the treatment works and reduces people’s level of prejudice, then they would no longer be suitable for testing in the control condition. This difficulty is true for many designs that involve a treatment meant to produce long-term change in participants’ behavior (e.g., studies testing the effectiveness of psychotherapy). Clearly, a between-subjects design would be necessary here.
Remember also that using one type of design does not preclude using the other type in a different study. There is no reason that a researcher could not use both a between-subjects design and a within-subjects design to answer the same research question. In fact, professional researchers often take exactly this type of mixed methods approach.
Key Takeaways
- Experiments can be conducted using either between-subjects or within-subjects designs. Deciding which to use in a particular situation requires careful consideration of the pros and cons of each approach.
- Random assignment to conditions in between-subjects experiments or counterbalancing of orders of conditions in within-subjects experiments is a fundamental element of experimental research. The purpose of these techniques is to control extraneous variables so that they do not become confounding variables.
- You want to test the relative effectiveness of two training programs for running a marathon.
- Using photographs of people as stimuli, you want to see if smiling people are perceived as more intelligent than people who are not smiling.
- In a field experiment, you want to see if the way a panhandler is dressed (neatly vs. sloppily) affects whether or not passersby give him any money.
- You want to see if concrete nouns (e.g., dog ) are recalled better than abstract nouns (e.g., truth).
- Birnbaum, M.H. (1999). How to show that 9>221: Collect judgments in a between-subjects design. Psychological Methods, 4 (3), 243-249. ↵

Share This Book
- Increase Font Size
Final dates! Join the tutor2u subject teams in London for a day of exam technique and revision at the cinema. Learn more →
Reference Library
Collections
- See what's new
- All Resources
- Student Resources
- Assessment Resources
- Teaching Resources
- CPD Courses
- Livestreams
Study notes, videos, interactive activities and more!
Psychology news, insights and enrichment
Currated collections of free resources
Browse resources by topic
- All Psychology Resources
Resource Selections
Currated lists of resources
Study Notes
Types of Experiment: Overview
Last updated 6 Sept 2022
- Share on Facebook
- Share on Twitter
- Share by Email
Different types of methods are used in research, which loosely fall into 1 of 2 categories.
Experimental (Laboratory, Field & Natural) & N on experimental ( correlations, observations, interviews, questionnaires and case studies).
All the three types of experiments have characteristics in common. They all have:
- an independent variable (I.V.) which is manipulated or a naturally occurring variable
- a dependent variable (D.V.) which is measured
- there will be at least two conditions in which participants produce data.
Note – natural and quasi experiments are often used synonymously but are not strictly the same, as with quasi experiments participants cannot be randomly assigned, so rather than there being a condition there is a condition.
Laboratory Experiments
These are conducted under controlled conditions, in which the researcher deliberately changes something (I.V.) to see the effect of this on something else (D.V.).
Control – lab experiments have a high degree of control over the environment & other extraneous variables which means that the researcher can accurately assess the effects of the I.V, so it has higher internal validity.
Replicable – due to the researcher’s high levels of control, research procedures can be repeated so that the reliability of results can be checked.
Limitations
Lacks ecological validity – due to the involvement of the researcher in manipulating and controlling variables, findings cannot be easily generalised to other (real life) settings, resulting in poor external validity.
Field Experiments
These are carried out in a natural setting, in which the researcher manipulates something (I.V.) to see the effect of this on something else (D.V.).
Validity – field experiments have some degree of control but also are conducted in a natural environment, so can be seen to have reasonable internal and external validity.
Less control than lab experiments and therefore extraneous variables are more likely to distort findings and so internal validity is likely to be lower.
Natural / Quasi Experiments
These are typically carried out in a natural setting, in which the researcher measures the effect of something which is to see the effect of this on something else (D.V.). Note that in this case there is no deliberate manipulation of a variable; this already naturally changing, which means the research is merely measuring the effect of something that is already happening.
High ecological validity – due to the lack of involvement of the researcher; variables are naturally occurring so findings can be easily generalised to other (real life) settings, resulting in high external validity.
Lack of control – natural experiments have no control over the environment & other extraneous variables which means that the researcher cannot always accurately assess the effects of the I.V, so it has low internal validity.
Not replicable – due to the researcher’s lack of control, research procedures cannot be repeated so that the reliability of results cannot be checked.
- Laboratory Experiment
- Field experiment
- Quasi Experiment
- Natural Experiment
- Field experiments
You might also like
Experimental design, field experiments, laboratory experiments, natural experiments, control of extraneous variables, independent and dependent variables, duration of long-term memory, our subjects.
- › Criminology
- › Economics
- › Geography
- › Health & Social Care
- › Psychology
- › Sociology
- › Teaching & learning resources
- › Student revision workshops
- › Online student courses
- › CPD for teachers
- › Livestreams
- › Teaching jobs
Boston House, 214 High Street, Boston Spa, West Yorkshire, LS23 6AD Tel: 01937 848885
- › Contact us
- › Terms of use
- › Privacy & cookies
© 2002-2024 Tutor2u Limited. Company Reg no: 04489574. VAT reg no 816865400.
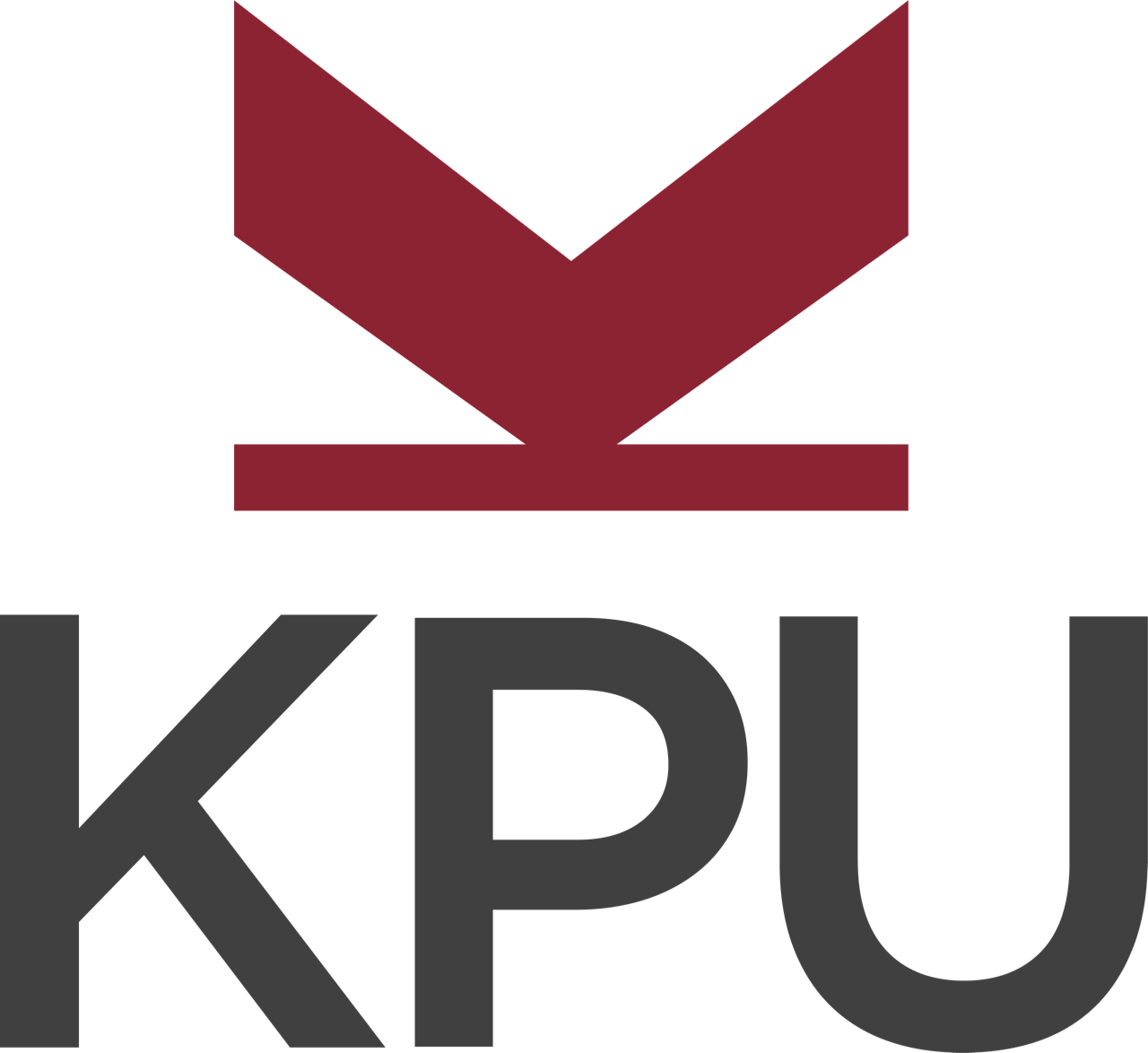
Want to create or adapt books like this? Learn more about how Pressbooks supports open publishing practices.
Experimental Research
In the late 1960s social psychologists John Darley and Bibb Latané proposed a counter-intuitive hypothesis. The more witnesses there are to an accident or a crime, the less likely any of them is to help the victim (Darley & Latané, 1968) [1] .
They also suggested the theory that this phenomenon occurs because each witness feels less responsible for helping—a process referred to as the “diffusion of responsibility.” Darley and Latané noted that their ideas were consistent with many real-world cases. For example, a New York woman named Catherine “Kitty” Genovese was assaulted and murdered while several witnesses evidently failed to help. But Darley and Latané also understood that such isolated cases did not provide convincing evidence for their hypothesized “bystander effect.” There was no way to know, for example, whether any of the witnesses to Kitty Genovese’s murder would have helped had there been fewer of them.
So to test their hypothesis, Darley and Latané created a simulated emergency situation in a laboratory. Each of their university student participants was isolated in a small room and told that they would be having a discussion about university life with other students via an intercom system. Early in the discussion, however, one of the students began having what seemed to be an epileptic seizure. Over the intercom came the following: “I could really-er-use some help so if somebody would-er-give me a little h-help-uh-er-er-er-er-er c-could somebody-er-er-help-er-uh-uh-uh (choking sounds)…I’m gonna die-er-er-I’m…gonna die-er-help-er-er-seizure-er- [chokes, then quiet]” (Darley & Latané, 1968, p. 379) .
In actuality, there were no other students. These comments had been prerecorded and were played back to create the appearance of a real emergency. The key to the study was that some participants were told that the discussion involved only one other student (the victim), others were told that it involved two other students, and still others were told that it included five other students. Because this was the only difference between these three groups of participants, any difference in their tendency to help the victim would have to have been caused by it. And sure enough, the likelihood that the participant left the room to seek help for the “victim” decreased from 85% to 62% to 31% as the number of “witnesses” increased.
The Parable of the 38 Witnesses
The story of Kitty Genovese has been told and retold in numerous psychology textbooks. The standard version is that there were 38 witnesses to the crime, that all of them watched (or listened) for an extended period of time, and that none of them did anything to help. However, recent scholarship suggests that the standard story is inaccurate in many ways (Manning, Levine, & Collins, 2007) [2] . For example, only six eyewitnesses testified at the trial, none of them was aware that they were witnessing a lethal assault, and there have been several reports of witnesses calling the police or even coming to the aid of Kitty Genovese. Although the standard story inspired a long line of research on the bystander effect and the diffusion of responsibility, it may also have directed researchers’ and students’ attention away from other equally interesting and important issues in the psychology of helping—including the conditions in which people do in fact respond collectively to emergency situations.
The research that Darley and Latané conducted was a particular kind of study called an experiment. Experiments are used to determine not only whether there is a meaningful relationship between two variables but also whether the relationship is a causal one that is supported by statistical analysis. For this reason, experiments are one of the most common and useful tools in the psychological researcher’s toolbox. In this chapter, we look at experiments in detail. We will first consider what sets experiments apart from other kinds of studies and why they support causal conclusions while other kinds of studies do not. We then look at two basic ways of designing an experiment—between-subjects designs and within-subjects designs—and discuss their pros and cons. Finally, we consider several important practical issues that arise when conducting experiments.
- Darley, J. M., & Latané, B. (1968). Bystander intervention in emergencies: Diffusion of responsibility. Journal of Personality and Social Psychology, 4 , 377–383. ↵
- Manning, R., Levine, M., & Collins, A. (2007). The Kitty Genovese murder and the social psychology of helping: The parable of the 38 witnesses. American Psychologist, 62 , 555–562. ↵
Research Methods in Psychology Copyright © 2019 by Rajiv S. Jhangiani, I-Chant A. Chiang, Carrie Cuttler, & Dana C. Leighton is licensed under a Creative Commons Attribution-NonCommercial-ShareAlike 4.0 International License , except where otherwise noted.
Share This Book
- Bipolar Disorder
- Therapy Center
- When To See a Therapist
- Types of Therapy
- Best Online Therapy
- Best Couples Therapy
- Best Family Therapy
- Managing Stress
- Sleep and Dreaming
- Understanding Emotions
- Self-Improvement
- Healthy Relationships
- Student Resources
- Personality Types
- Guided Meditations
- Verywell Mind Insights
- 2023 Verywell Mind 25
- Mental Health in the Classroom
- Editorial Process
- Meet Our Review Board
- Crisis Support
Understanding Methods for Research in Psychology
A Psychology Research Methods Study Guide
Kendra Cherry, MS, is a psychosocial rehabilitation specialist, psychology educator, and author of the "Everything Psychology Book."
:max_bytes(150000):strip_icc():format(webp)/IMG_9791-89504ab694d54b66bbd72cb84ffb860e.jpg)
Emily is a board-certified science editor who has worked with top digital publishing brands like Voices for Biodiversity, Study.com, GoodTherapy, Vox, and Verywell.
:max_bytes(150000):strip_icc():format(webp)/Emily-Swaim-1000-0f3197de18f74329aeffb690a177160c.jpg)
Types of Research in Psychology
- Cross-Sectional vs. Longitudinal Research
- Reliability and Validity
Glossary of Terms
Research in psychology focuses on a variety of topics , ranging from the development of infants to the behavior of social groups. Psychologists use the scientific method to investigate questions both systematically and empirically.
Research in psychology is important because it provides us with valuable information that helps to improve human lives. By learning more about the brain, cognition, behavior, and mental health conditions, researchers are able to solve real-world problems that affect our day-to-day lives.
At a Glance
Knowing more about how research in psychology is conducted can give you a better understanding of what those findings might mean to you. Psychology experiments can range from simple to complex, but there are some basic terms and concepts that all psychology students should understand.
Start your studies by learning more about the different types of research, the basics of experimental design, and the relationships between variables.
Research in Psychology: The Basics
The first step in your review should include a basic introduction to psychology research methods . Psychology research can have a variety of goals. What researchers learn can be used to describe, explain, predict, or change human behavior.
Psychologists use the scientific method to conduct studies and research in psychology. The basic process of conducting psychology research involves asking a question, designing a study, collecting data, analyzing results, reaching conclusions, and sharing the findings.
The Scientific Method in Psychology Research
The steps of the scientific method in psychology research are:
- Make an observation
- Ask a research question and make predictions about what you expect to find
- Test your hypothesis and gather data
- Examine the results and form conclusions
- Report your findings
Research in psychology can take several different forms. It can describe a phenomenon, explore the causes of a phenomenon, or look at relationships between one or more variables. Three of the main types of psychological research focus on:
Descriptive Studies
This type of research can tell us more about what is happening in a specific population. It relies on techniques such as observation, surveys, and case studies.
Correlational Studies
Correlational research is frequently used in psychology to look for relationships between variables. While research look at how variables are related, they do not manipulate any of the variables.
While correlational studies can suggest a relationship between two variables, finding a correlation does not prove that one variable causes a change in another. In other words, correlation does not equal causation.
Experimental Research Methods
Experiments are a research method that can look at whether changes in one variable cause changes in another. The simple experiment is one of the most basic methods of determining if there is a cause-and-effect relationship between two variables.
A simple experiment utilizes a control group of participants who receive no treatment and an experimental group of participants who receive the treatment.
Experimenters then compare the results of the two groups to determine if the treatment had an effect.
Cross-Sectional vs. Longitudinal Research in Psychology
Research in psychology can also involve collecting data at a single point in time, or gathering information at several points over a period of time.
Cross-Sectional Research
In a cross-sectional study , researchers collect data from participants at a single point in time. These are descriptive type of research and cannot be used to determine cause and effect because researchers do not manipulate the independent variables.
However, cross-sectional research does allow researchers to look at the characteristics of the population and explore relationships between different variables at a single point in time.
Longitudinal Research
A longitudinal study is a type of research in psychology that involves looking at the same group of participants over a period of time. Researchers start by collecting initial data that serves as a baseline, and then collect follow-up data at certain intervals. These studies can last days, months, or years.
The longest longitudinal study in psychology was started in 1921 and the study is planned to continue until the last participant dies or withdraws. As of 2003, more than 200 of the partipants were still alive.
The Reliability and Validity of Research in Psychology
Reliability and validity are two concepts that are also critical in psychology research. In order to trust the results, we need to know if the findings are consistent (reliability) and that we are actually measuring what we think we are measuring (validity).
Reliability
Reliability is a vital component of a valid psychological test. What is reliability? How do we measure it? Simply put, reliability refers to the consistency of a measure. A test is considered reliable if we get the same result repeatedly.
When determining the merits of a psychological test, validity is one of the most important factors to consider. What exactly is validity? One of the greatest concerns when creating a psychological test is whether or not it actually measures what we think it is measuring.
For example, a test might be designed to measure a stable personality trait but instead measures transitory emotions generated by situational or environmental conditions. A valid test ensures that the results accurately reflect the dimension undergoing assessment.
Review some of the key terms that you should know and understand about psychology research methods. Spend some time studying these terms and definitions before your exam. Some key terms that you should know include:
- Correlation
- Demand characteristic
- Dependent variable
- Hawthorne effect
- Independent variable
- Naturalistic observation
- Placebo effect
- Random assignment
- Replication
- Selective attrition
Erol A. How to conduct scientific research ? Noro Psikiyatr Ars . 2017;54(2):97-98. doi:10.5152/npa.2017.0120102
Aggarwal R, Ranganathan P. Study designs: Part 2 - Descriptive studies . Perspect Clin Res . 2019;10(1):34-36. doi:10.4103/picr.PICR_154_18
Curtis EA, Comiskey C, Dempsey O. Importance and use of correlational research . Nurse Res . 2016;23(6):20-25. doi:10.7748/nr.2016.e1382
Wang X, Cheng Z. Cross-sectional studies: Strengths, weaknesses, and recommendations . Chest . 2020;158(1S):S65-S71. doi:10.1016/j.chest.2020.03.012
Caruana EJ, Roman M, Hernández-Sánchez J, Solli P. Longitudinal studies . J Thorac Dis . 2015;7(11):E537-E540. doi:10.3978/j.issn.2072-1439.2015.10.63
Stanford Magazine. The vexing legacy of Lewis Terman .
By Kendra Cherry, MSEd Kendra Cherry, MS, is a psychosocial rehabilitation specialist, psychology educator, and author of the "Everything Psychology Book."
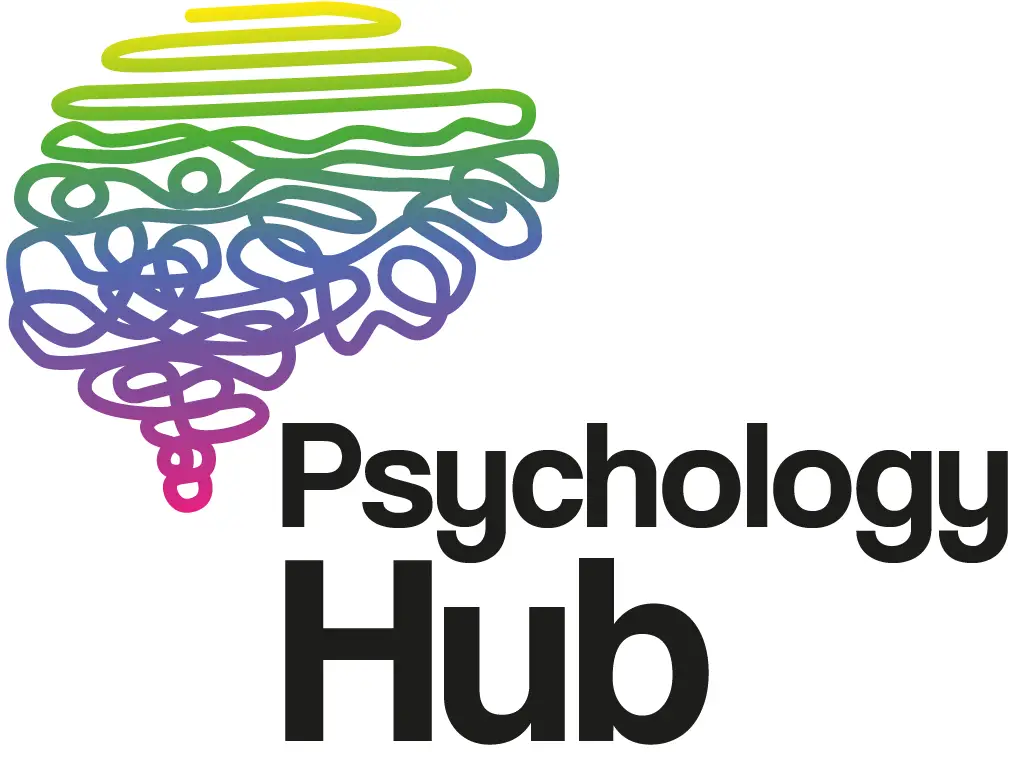
Experimental Methods In Psychology
March 7, 2021 - paper 2 psychology in context | research methods.
- Back to Paper 2 - Research Methods
There are three experimental methods in the field of psychology; Laboratory, Field and Natural Experiments. Each of the experimental methods holds different characteristics in relation to; the manipulation of the IV, the control of the EVs and the ability to accurately replicate the study in exactly the same way.
When conducting research, it is important to create an aim and a hypothesis, click here to learn more about the formation of aims and hypotheses.
- Psychopathology
- Social Psychology
- Approaches To Human Behaviour
- Biopsychology
- Research Methods
- Issues & Debates
- Teacher Hub
- Terms and Conditions
- Privacy Policy
- Cookie Policy
- [email protected]
- www.psychologyhub.co.uk
We're not around right now. But you can send us an email and we'll get back to you, asap.
Start typing and press Enter to search
Cookie Policy - Terms and Conditions - Privacy Policy
Want to create or adapt books like this? Learn more about how Pressbooks supports open publishing practices.
Ch 2: Psychological Research Methods
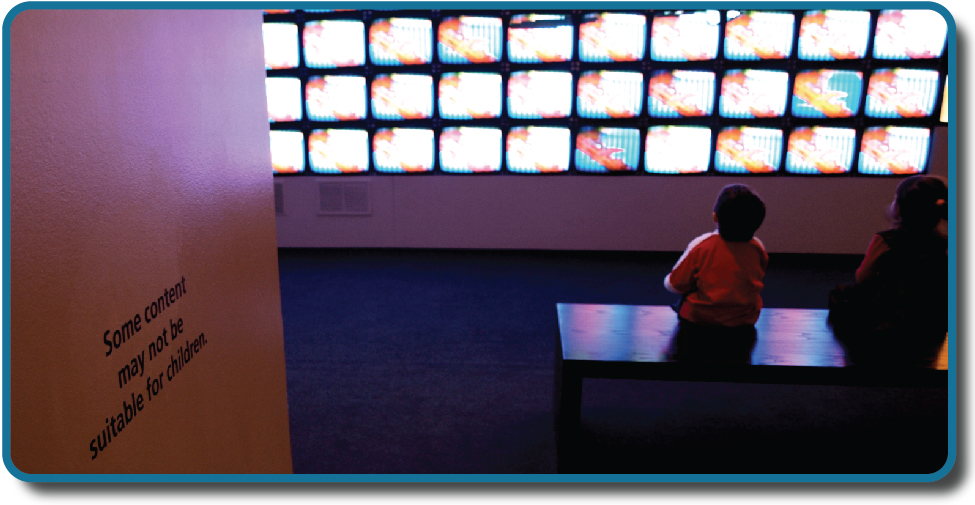
Have you ever wondered whether the violence you see on television affects your behavior? Are you more likely to behave aggressively in real life after watching people behave violently in dramatic situations on the screen? Or, could seeing fictional violence actually get aggression out of your system, causing you to be more peaceful? How are children influenced by the media they are exposed to? A psychologist interested in the relationship between behavior and exposure to violent images might ask these very questions.
The topic of violence in the media today is contentious. Since ancient times, humans have been concerned about the effects of new technologies on our behaviors and thinking processes. The Greek philosopher Socrates, for example, worried that writing—a new technology at that time—would diminish people’s ability to remember because they could rely on written records rather than committing information to memory. In our world of quickly changing technologies, questions about the effects of media continue to emerge. Is it okay to talk on a cell phone while driving? Are headphones good to use in a car? What impact does text messaging have on reaction time while driving? These are types of questions that psychologist David Strayer asks in his lab.
Watch this short video to see how Strayer utilizes the scientific method to reach important conclusions regarding technology and driving safety.
You can view the transcript for “Understanding driver distraction” here (opens in new window) .
How can we go about finding answers that are supported not by mere opinion, but by evidence that we can all agree on? The findings of psychological research can help us navigate issues like this.
Introduction to the Scientific Method
Learning objectives.
- Explain the steps of the scientific method
- Describe why the scientific method is important to psychology
- Summarize the processes of informed consent and debriefing
- Explain how research involving humans or animals is regulated
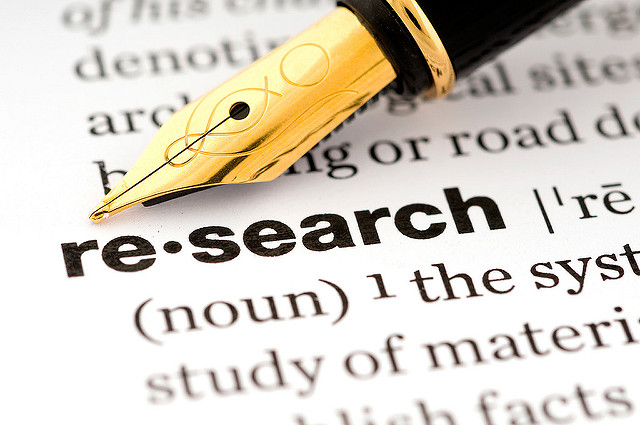
Scientists are engaged in explaining and understanding how the world around them works, and they are able to do so by coming up with theories that generate hypotheses that are testable and falsifiable. Theories that stand up to their tests are retained and refined, while those that do not are discarded or modified. In this way, research enables scientists to separate fact from simple opinion. Having good information generated from research aids in making wise decisions both in public policy and in our personal lives. In this section, you’ll see how psychologists use the scientific method to study and understand behavior.
The Scientific Process
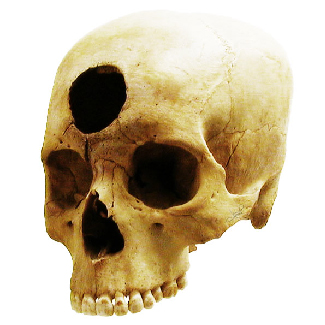
The goal of all scientists is to better understand the world around them. Psychologists focus their attention on understanding behavior, as well as the cognitive (mental) and physiological (body) processes that underlie behavior. In contrast to other methods that people use to understand the behavior of others, such as intuition and personal experience, the hallmark of scientific research is that there is evidence to support a claim. Scientific knowledge is empirical : It is grounded in objective, tangible evidence that can be observed time and time again, regardless of who is observing.
While behavior is observable, the mind is not. If someone is crying, we can see the behavior. However, the reason for the behavior is more difficult to determine. Is the person crying due to being sad, in pain, or happy? Sometimes we can learn the reason for someone’s behavior by simply asking a question, like “Why are you crying?” However, there are situations in which an individual is either uncomfortable or unwilling to answer the question honestly, or is incapable of answering. For example, infants would not be able to explain why they are crying. In such circumstances, the psychologist must be creative in finding ways to better understand behavior. This module explores how scientific knowledge is generated, and how important that knowledge is in forming decisions in our personal lives and in the public domain.
Process of Scientific Research
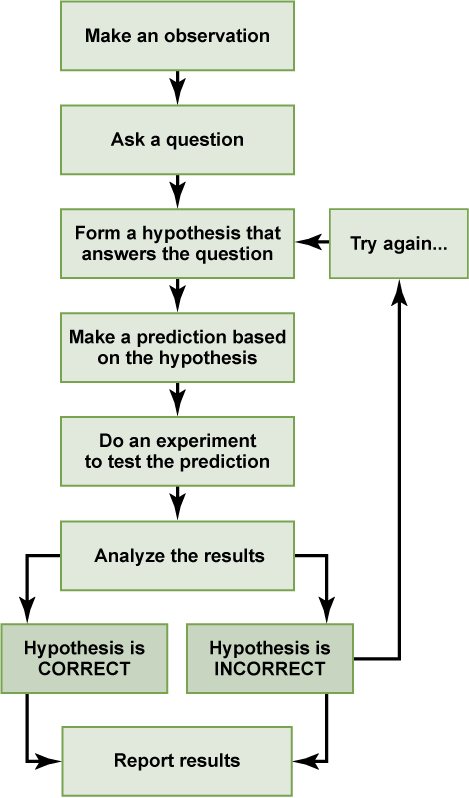
Scientific knowledge is advanced through a process known as the scientific method. Basically, ideas (in the form of theories and hypotheses) are tested against the real world (in the form of empirical observations), and those empirical observations lead to more ideas that are tested against the real world, and so on.
The basic steps in the scientific method are:
- Observe a natural phenomenon and define a question about it
- Make a hypothesis, or potential solution to the question
- Test the hypothesis
- If the hypothesis is true, find more evidence or find counter-evidence
- If the hypothesis is false, create a new hypothesis or try again
- Draw conclusions and repeat–the scientific method is never-ending, and no result is ever considered perfect
In order to ask an important question that may improve our understanding of the world, a researcher must first observe natural phenomena. By making observations, a researcher can define a useful question. After finding a question to answer, the researcher can then make a prediction (a hypothesis) about what he or she thinks the answer will be. This prediction is usually a statement about the relationship between two or more variables. After making a hypothesis, the researcher will then design an experiment to test his or her hypothesis and evaluate the data gathered. These data will either support or refute the hypothesis. Based on the conclusions drawn from the data, the researcher will then find more evidence to support the hypothesis, look for counter-evidence to further strengthen the hypothesis, revise the hypothesis and create a new experiment, or continue to incorporate the information gathered to answer the research question.
Basic Principles of the Scientific Method
Two key concepts in the scientific approach are theory and hypothesis. A theory is a well-developed set of ideas that propose an explanation for observed phenomena that can be used to make predictions about future observations. A hypothesis is a testable prediction that is arrived at logically from a theory. It is often worded as an if-then statement (e.g., if I study all night, I will get a passing grade on the test). The hypothesis is extremely important because it bridges the gap between the realm of ideas and the real world. As specific hypotheses are tested, theories are modified and refined to reflect and incorporate the result of these tests.
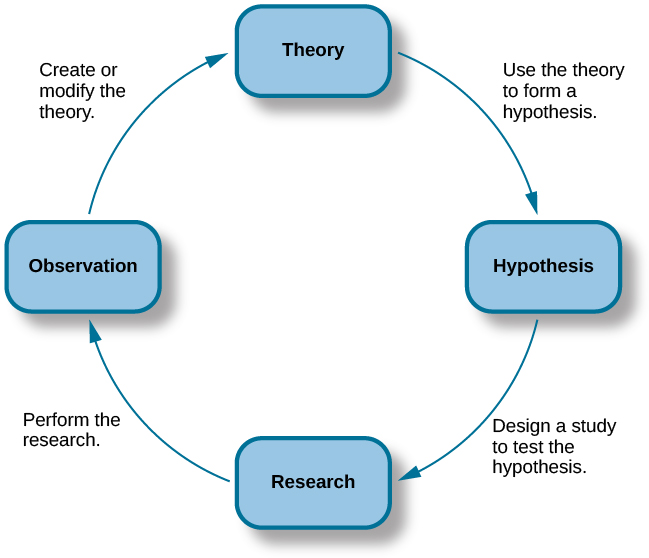
Other key components in following the scientific method include verifiability, predictability, falsifiability, and fairness. Verifiability means that an experiment must be replicable by another researcher. To achieve verifiability, researchers must make sure to document their methods and clearly explain how their experiment is structured and why it produces certain results.
Predictability in a scientific theory implies that the theory should enable us to make predictions about future events. The precision of these predictions is a measure of the strength of the theory.
Falsifiability refers to whether a hypothesis can be disproved. For a hypothesis to be falsifiable, it must be logically possible to make an observation or do a physical experiment that would show that there is no support for the hypothesis. Even when a hypothesis cannot be shown to be false, that does not necessarily mean it is not valid. Future testing may disprove the hypothesis. This does not mean that a hypothesis has to be shown to be false, just that it can be tested.
To determine whether a hypothesis is supported or not supported, psychological researchers must conduct hypothesis testing using statistics. Hypothesis testing is a type of statistics that determines the probability of a hypothesis being true or false. If hypothesis testing reveals that results were “statistically significant,” this means that there was support for the hypothesis and that the researchers can be reasonably confident that their result was not due to random chance. If the results are not statistically significant, this means that the researchers’ hypothesis was not supported.
Fairness implies that all data must be considered when evaluating a hypothesis. A researcher cannot pick and choose what data to keep and what to discard or focus specifically on data that support or do not support a particular hypothesis. All data must be accounted for, even if they invalidate the hypothesis.
Applying the Scientific Method
To see how this process works, let’s consider a specific theory and a hypothesis that might be generated from that theory. As you’ll learn in a later module, the James-Lange theory of emotion asserts that emotional experience relies on the physiological arousal associated with the emotional state. If you walked out of your home and discovered a very aggressive snake waiting on your doorstep, your heart would begin to race and your stomach churn. According to the James-Lange theory, these physiological changes would result in your feeling of fear. A hypothesis that could be derived from this theory might be that a person who is unaware of the physiological arousal that the sight of the snake elicits will not feel fear.
Remember that a good scientific hypothesis is falsifiable, or capable of being shown to be incorrect. Recall from the introductory module that Sigmund Freud had lots of interesting ideas to explain various human behaviors (Figure 5). However, a major criticism of Freud’s theories is that many of his ideas are not falsifiable; for example, it is impossible to imagine empirical observations that would disprove the existence of the id, the ego, and the superego—the three elements of personality described in Freud’s theories. Despite this, Freud’s theories are widely taught in introductory psychology texts because of their historical significance for personality psychology and psychotherapy, and these remain the root of all modern forms of therapy.
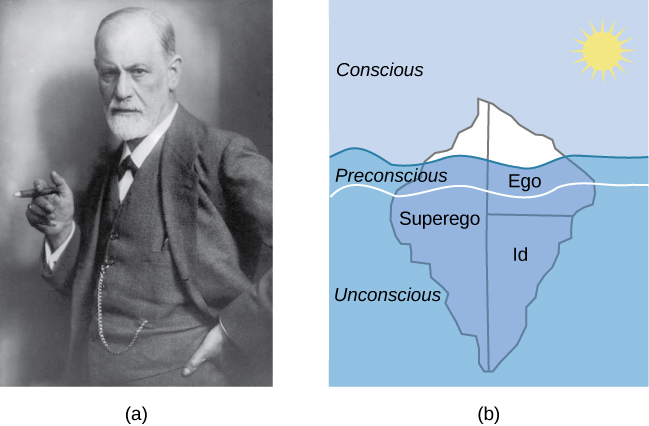
In contrast, the James-Lange theory does generate falsifiable hypotheses, such as the one described above. Some individuals who suffer significant injuries to their spinal columns are unable to feel the bodily changes that often accompany emotional experiences. Therefore, we could test the hypothesis by determining how emotional experiences differ between individuals who have the ability to detect these changes in their physiological arousal and those who do not. In fact, this research has been conducted and while the emotional experiences of people deprived of an awareness of their physiological arousal may be less intense, they still experience emotion (Chwalisz, Diener, & Gallagher, 1988).
Link to Learning
Why the scientific method is important for psychology.
The use of the scientific method is one of the main features that separates modern psychology from earlier philosophical inquiries about the mind. Compared to chemistry, physics, and other “natural sciences,” psychology has long been considered one of the “social sciences” because of the subjective nature of the things it seeks to study. Many of the concepts that psychologists are interested in—such as aspects of the human mind, behavior, and emotions—are subjective and cannot be directly measured. Psychologists often rely instead on behavioral observations and self-reported data, which are considered by some to be illegitimate or lacking in methodological rigor. Applying the scientific method to psychology, therefore, helps to standardize the approach to understanding its very different types of information.
The scientific method allows psychological data to be replicated and confirmed in many instances, under different circumstances, and by a variety of researchers. Through replication of experiments, new generations of psychologists can reduce errors and broaden the applicability of theories. It also allows theories to be tested and validated instead of simply being conjectures that could never be verified or falsified. All of this allows psychologists to gain a stronger understanding of how the human mind works.
Scientific articles published in journals and psychology papers written in the style of the American Psychological Association (i.e., in “APA style”) are structured around the scientific method. These papers include an Introduction, which introduces the background information and outlines the hypotheses; a Methods section, which outlines the specifics of how the experiment was conducted to test the hypothesis; a Results section, which includes the statistics that tested the hypothesis and state whether it was supported or not supported, and a Discussion and Conclusion, which state the implications of finding support for, or no support for, the hypothesis. Writing articles and papers that adhere to the scientific method makes it easy for future researchers to repeat the study and attempt to replicate the results.
Ethics in Research
Today, scientists agree that good research is ethical in nature and is guided by a basic respect for human dignity and safety. However, as you will read in the Tuskegee Syphilis Study, this has not always been the case. Modern researchers must demonstrate that the research they perform is ethically sound. This section presents how ethical considerations affect the design and implementation of research conducted today.
Research Involving Human Participants
Any experiment involving the participation of human subjects is governed by extensive, strict guidelines designed to ensure that the experiment does not result in harm. Any research institution that receives federal support for research involving human participants must have access to an institutional review board (IRB) . The IRB is a committee of individuals often made up of members of the institution’s administration, scientists, and community members (Figure 6). The purpose of the IRB is to review proposals for research that involves human participants. The IRB reviews these proposals with the principles mentioned above in mind, and generally, approval from the IRB is required in order for the experiment to proceed.
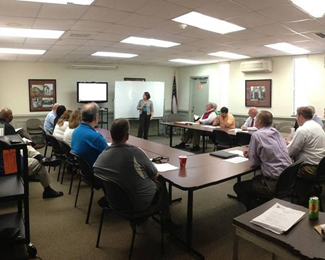
An institution’s IRB requires several components in any experiment it approves. For one, each participant must sign an informed consent form before they can participate in the experiment. An informed consent form provides a written description of what participants can expect during the experiment, including potential risks and implications of the research. It also lets participants know that their involvement is completely voluntary and can be discontinued without penalty at any time. Furthermore, the informed consent guarantees that any data collected in the experiment will remain completely confidential. In cases where research participants are under the age of 18, the parents or legal guardians are required to sign the informed consent form.
While the informed consent form should be as honest as possible in describing exactly what participants will be doing, sometimes deception is necessary to prevent participants’ knowledge of the exact research question from affecting the results of the study. Deception involves purposely misleading experiment participants in order to maintain the integrity of the experiment, but not to the point where the deception could be considered harmful. For example, if we are interested in how our opinion of someone is affected by their attire, we might use deception in describing the experiment to prevent that knowledge from affecting participants’ responses. In cases where deception is involved, participants must receive a full debriefing upon conclusion of the study—complete, honest information about the purpose of the experiment, how the data collected will be used, the reasons why deception was necessary, and information about how to obtain additional information about the study.
Dig Deeper: Ethics and the Tuskegee Syphilis Study
Unfortunately, the ethical guidelines that exist for research today were not always applied in the past. In 1932, poor, rural, black, male sharecroppers from Tuskegee, Alabama, were recruited to participate in an experiment conducted by the U.S. Public Health Service, with the aim of studying syphilis in black men (Figure 7). In exchange for free medical care, meals, and burial insurance, 600 men agreed to participate in the study. A little more than half of the men tested positive for syphilis, and they served as the experimental group (given that the researchers could not randomly assign participants to groups, this represents a quasi-experiment). The remaining syphilis-free individuals served as the control group. However, those individuals that tested positive for syphilis were never informed that they had the disease.
While there was no treatment for syphilis when the study began, by 1947 penicillin was recognized as an effective treatment for the disease. Despite this, no penicillin was administered to the participants in this study, and the participants were not allowed to seek treatment at any other facilities if they continued in the study. Over the course of 40 years, many of the participants unknowingly spread syphilis to their wives (and subsequently their children born from their wives) and eventually died because they never received treatment for the disease. This study was discontinued in 1972 when the experiment was discovered by the national press (Tuskegee University, n.d.). The resulting outrage over the experiment led directly to the National Research Act of 1974 and the strict ethical guidelines for research on humans described in this chapter. Why is this study unethical? How were the men who participated and their families harmed as a function of this research?
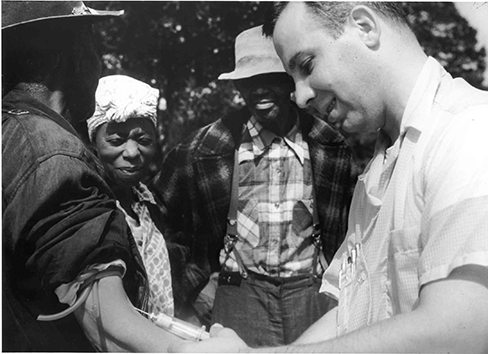
Learn more about the Tuskegee Syphilis Study on the CDC website .
Research Involving Animal Subjects
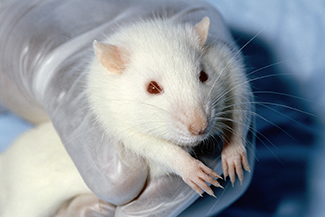
This does not mean that animal researchers are immune to ethical concerns. Indeed, the humane and ethical treatment of animal research subjects is a critical aspect of this type of research. Researchers must design their experiments to minimize any pain or distress experienced by animals serving as research subjects.
Whereas IRBs review research proposals that involve human participants, animal experimental proposals are reviewed by an Institutional Animal Care and Use Committee (IACUC) . An IACUC consists of institutional administrators, scientists, veterinarians, and community members. This committee is charged with ensuring that all experimental proposals require the humane treatment of animal research subjects. It also conducts semi-annual inspections of all animal facilities to ensure that the research protocols are being followed. No animal research project can proceed without the committee’s approval.
Introduction to Approaches to Research
- Differentiate between descriptive, correlational, and experimental research
- Explain the strengths and weaknesses of case studies, naturalistic observation, and surveys
- Describe the strength and weaknesses of archival research
- Compare longitudinal and cross-sectional approaches to research
- Explain what a correlation coefficient tells us about the relationship between variables
- Describe why correlation does not mean causation
- Describe the experimental process, including ways to control for bias
- Identify and differentiate between independent and dependent variables
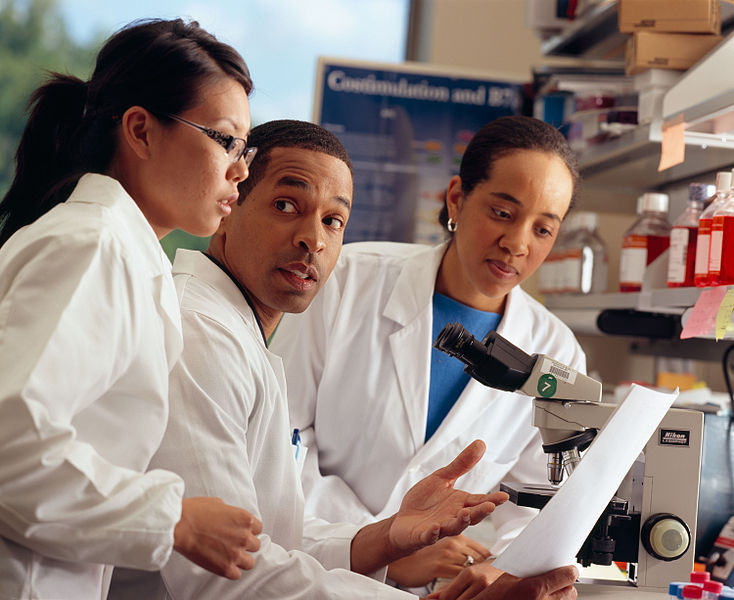
Psychologists use descriptive, experimental, and correlational methods to conduct research. Descriptive, or qualitative, methods include the case study, naturalistic observation, surveys, archival research, longitudinal research, and cross-sectional research.
Experiments are conducted in order to determine cause-and-effect relationships. In ideal experimental design, the only difference between the experimental and control groups is whether participants are exposed to the experimental manipulation. Each group goes through all phases of the experiment, but each group will experience a different level of the independent variable: the experimental group is exposed to the experimental manipulation, and the control group is not exposed to the experimental manipulation. The researcher then measures the changes that are produced in the dependent variable in each group. Once data is collected from both groups, it is analyzed statistically to determine if there are meaningful differences between the groups.
When scientists passively observe and measure phenomena it is called correlational research. Here, psychologists do not intervene and change behavior, as they do in experiments. In correlational research, they identify patterns of relationships, but usually cannot infer what causes what. Importantly, with correlational research, you can examine only two variables at a time, no more and no less.
Watch It: More on Research
If you enjoy learning through lectures and want an interesting and comprehensive summary of this section, then click on the Youtube link to watch a lecture given by MIT Professor John Gabrieli . Start at the 30:45 minute mark and watch through the end to hear examples of actual psychological studies and how they were analyzed. Listen for references to independent and dependent variables, experimenter bias, and double-blind studies. In the lecture, you’ll learn about breaking social norms, “WEIRD” research, why expectations matter, how a warm cup of coffee might make you nicer, why you should change your answer on a multiple choice test, and why praise for intelligence won’t make you any smarter.
You can view the transcript for “Lec 2 | MIT 9.00SC Introduction to Psychology, Spring 2011” here (opens in new window) .
Descriptive Research
There are many research methods available to psychologists in their efforts to understand, describe, and explain behavior and the cognitive and biological processes that underlie it. Some methods rely on observational techniques. Other approaches involve interactions between the researcher and the individuals who are being studied—ranging from a series of simple questions to extensive, in-depth interviews—to well-controlled experiments.
The three main categories of psychological research are descriptive, correlational, and experimental research. Research studies that do not test specific relationships between variables are called descriptive, or qualitative, studies . These studies are used to describe general or specific behaviors and attributes that are observed and measured. In the early stages of research it might be difficult to form a hypothesis, especially when there is not any existing literature in the area. In these situations designing an experiment would be premature, as the question of interest is not yet clearly defined as a hypothesis. Often a researcher will begin with a non-experimental approach, such as a descriptive study, to gather more information about the topic before designing an experiment or correlational study to address a specific hypothesis. Descriptive research is distinct from correlational research , in which psychologists formally test whether a relationship exists between two or more variables. Experimental research goes a step further beyond descriptive and correlational research and randomly assigns people to different conditions, using hypothesis testing to make inferences about how these conditions affect behavior. It aims to determine if one variable directly impacts and causes another. Correlational and experimental research both typically use hypothesis testing, whereas descriptive research does not.
Each of these research methods has unique strengths and weaknesses, and each method may only be appropriate for certain types of research questions. For example, studies that rely primarily on observation produce incredible amounts of information, but the ability to apply this information to the larger population is somewhat limited because of small sample sizes. Survey research, on the other hand, allows researchers to easily collect data from relatively large samples. While this allows for results to be generalized to the larger population more easily, the information that can be collected on any given survey is somewhat limited and subject to problems associated with any type of self-reported data. Some researchers conduct archival research by using existing records. While this can be a fairly inexpensive way to collect data that can provide insight into a number of research questions, researchers using this approach have no control on how or what kind of data was collected.
Correlational research can find a relationship between two variables, but the only way a researcher can claim that the relationship between the variables is cause and effect is to perform an experiment. In experimental research, which will be discussed later in the text, there is a tremendous amount of control over variables of interest. While this is a powerful approach, experiments are often conducted in very artificial settings. This calls into question the validity of experimental findings with regard to how they would apply in real-world settings. In addition, many of the questions that psychologists would like to answer cannot be pursued through experimental research because of ethical concerns.
The three main types of descriptive studies are, naturalistic observation, case studies, and surveys.
Naturalistic Observation
If you want to understand how behavior occurs, one of the best ways to gain information is to simply observe the behavior in its natural context. However, people might change their behavior in unexpected ways if they know they are being observed. How do researchers obtain accurate information when people tend to hide their natural behavior? As an example, imagine that your professor asks everyone in your class to raise their hand if they always wash their hands after using the restroom. Chances are that almost everyone in the classroom will raise their hand, but do you think hand washing after every trip to the restroom is really that universal?
This is very similar to the phenomenon mentioned earlier in this module: many individuals do not feel comfortable answering a question honestly. But if we are committed to finding out the facts about hand washing, we have other options available to us.
Suppose we send a classmate into the restroom to actually watch whether everyone washes their hands after using the restroom. Will our observer blend into the restroom environment by wearing a white lab coat, sitting with a clipboard, and staring at the sinks? We want our researcher to be inconspicuous—perhaps standing at one of the sinks pretending to put in contact lenses while secretly recording the relevant information. This type of observational study is called naturalistic observation : observing behavior in its natural setting. To better understand peer exclusion, Suzanne Fanger collaborated with colleagues at the University of Texas to observe the behavior of preschool children on a playground. How did the observers remain inconspicuous over the duration of the study? They equipped a few of the children with wireless microphones (which the children quickly forgot about) and observed while taking notes from a distance. Also, the children in that particular preschool (a “laboratory preschool”) were accustomed to having observers on the playground (Fanger, Frankel, & Hazen, 2012).
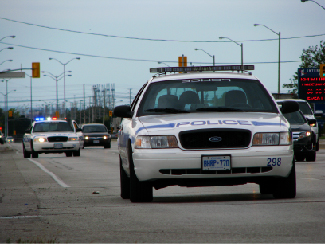
It is critical that the observer be as unobtrusive and as inconspicuous as possible: when people know they are being watched, they are less likely to behave naturally. If you have any doubt about this, ask yourself how your driving behavior might differ in two situations: In the first situation, you are driving down a deserted highway during the middle of the day; in the second situation, you are being followed by a police car down the same deserted highway (Figure 9).
It should be pointed out that naturalistic observation is not limited to research involving humans. Indeed, some of the best-known examples of naturalistic observation involve researchers going into the field to observe various kinds of animals in their own environments. As with human studies, the researchers maintain their distance and avoid interfering with the animal subjects so as not to influence their natural behaviors. Scientists have used this technique to study social hierarchies and interactions among animals ranging from ground squirrels to gorillas. The information provided by these studies is invaluable in understanding how those animals organize socially and communicate with one another. The anthropologist Jane Goodall, for example, spent nearly five decades observing the behavior of chimpanzees in Africa (Figure 10). As an illustration of the types of concerns that a researcher might encounter in naturalistic observation, some scientists criticized Goodall for giving the chimps names instead of referring to them by numbers—using names was thought to undermine the emotional detachment required for the objectivity of the study (McKie, 2010).
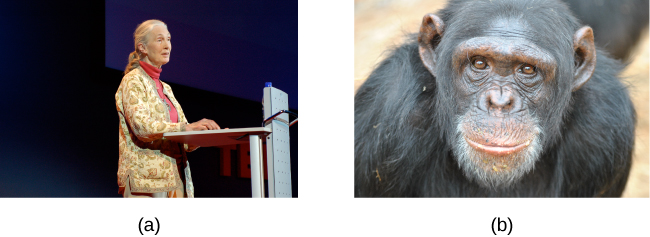
The greatest benefit of naturalistic observation is the validity, or accuracy, of information collected unobtrusively in a natural setting. Having individuals behave as they normally would in a given situation means that we have a higher degree of ecological validity, or realism, than we might achieve with other research approaches. Therefore, our ability to generalize the findings of the research to real-world situations is enhanced. If done correctly, we need not worry about people or animals modifying their behavior simply because they are being observed. Sometimes, people may assume that reality programs give us a glimpse into authentic human behavior. However, the principle of inconspicuous observation is violated as reality stars are followed by camera crews and are interviewed on camera for personal confessionals. Given that environment, we must doubt how natural and realistic their behaviors are.
The major downside of naturalistic observation is that they are often difficult to set up and control. In our restroom study, what if you stood in the restroom all day prepared to record people’s hand washing behavior and no one came in? Or, what if you have been closely observing a troop of gorillas for weeks only to find that they migrated to a new place while you were sleeping in your tent? The benefit of realistic data comes at a cost. As a researcher you have no control of when (or if) you have behavior to observe. In addition, this type of observational research often requires significant investments of time, money, and a good dose of luck.
Sometimes studies involve structured observation. In these cases, people are observed while engaging in set, specific tasks. An excellent example of structured observation comes from Strange Situation by Mary Ainsworth (you will read more about this in the module on lifespan development). The Strange Situation is a procedure used to evaluate attachment styles that exist between an infant and caregiver. In this scenario, caregivers bring their infants into a room filled with toys. The Strange Situation involves a number of phases, including a stranger coming into the room, the caregiver leaving the room, and the caregiver’s return to the room. The infant’s behavior is closely monitored at each phase, but it is the behavior of the infant upon being reunited with the caregiver that is most telling in terms of characterizing the infant’s attachment style with the caregiver.
Another potential problem in observational research is observer bias . Generally, people who act as observers are closely involved in the research project and may unconsciously skew their observations to fit their research goals or expectations. To protect against this type of bias, researchers should have clear criteria established for the types of behaviors recorded and how those behaviors should be classified. In addition, researchers often compare observations of the same event by multiple observers, in order to test inter-rater reliability : a measure of reliability that assesses the consistency of observations by different observers.
Case Studies
In 2011, the New York Times published a feature story on Krista and Tatiana Hogan, Canadian twin girls. These particular twins are unique because Krista and Tatiana are conjoined twins, connected at the head. There is evidence that the two girls are connected in a part of the brain called the thalamus, which is a major sensory relay center. Most incoming sensory information is sent through the thalamus before reaching higher regions of the cerebral cortex for processing.
The implications of this potential connection mean that it might be possible for one twin to experience the sensations of the other twin. For instance, if Krista is watching a particularly funny television program, Tatiana might smile or laugh even if she is not watching the program. This particular possibility has piqued the interest of many neuroscientists who seek to understand how the brain uses sensory information.
These twins represent an enormous resource in the study of the brain, and since their condition is very rare, it is likely that as long as their family agrees, scientists will follow these girls very closely throughout their lives to gain as much information as possible (Dominus, 2011).
In observational research, scientists are conducting a clinical or case study when they focus on one person or just a few individuals. Indeed, some scientists spend their entire careers studying just 10–20 individuals. Why would they do this? Obviously, when they focus their attention on a very small number of people, they can gain a tremendous amount of insight into those cases. The richness of information that is collected in clinical or case studies is unmatched by any other single research method. This allows the researcher to have a very deep understanding of the individuals and the particular phenomenon being studied.
If clinical or case studies provide so much information, why are they not more frequent among researchers? As it turns out, the major benefit of this particular approach is also a weakness. As mentioned earlier, this approach is often used when studying individuals who are interesting to researchers because they have a rare characteristic. Therefore, the individuals who serve as the focus of case studies are not like most other people. If scientists ultimately want to explain all behavior, focusing attention on such a special group of people can make it difficult to generalize any observations to the larger population as a whole. Generalizing refers to the ability to apply the findings of a particular research project to larger segments of society. Again, case studies provide enormous amounts of information, but since the cases are so specific, the potential to apply what’s learned to the average person may be very limited.
Often, psychologists develop surveys as a means of gathering data. Surveys are lists of questions to be answered by research participants, and can be delivered as paper-and-pencil questionnaires, administered electronically, or conducted verbally (Figure 11). Generally, the survey itself can be completed in a short time, and the ease of administering a survey makes it easy to collect data from a large number of people.
Surveys allow researchers to gather data from larger samples than may be afforded by other research methods . A sample is a subset of individuals selected from a population , which is the overall group of individuals that the researchers are interested in. Researchers study the sample and seek to generalize their findings to the population.
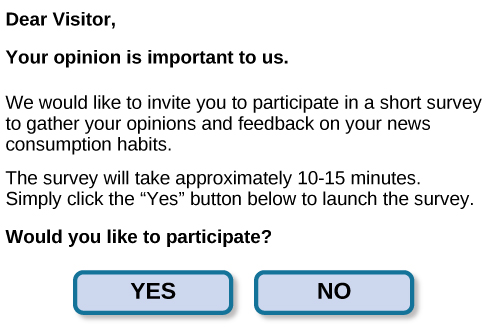
There is both strength and weakness of the survey in comparison to case studies. By using surveys, we can collect information from a larger sample of people. A larger sample is better able to reflect the actual diversity of the population, thus allowing better generalizability. Therefore, if our sample is sufficiently large and diverse, we can assume that the data we collect from the survey can be generalized to the larger population with more certainty than the information collected through a case study. However, given the greater number of people involved, we are not able to collect the same depth of information on each person that would be collected in a case study.
Another potential weakness of surveys is something we touched on earlier in this chapter: people don’t always give accurate responses. They may lie, misremember, or answer questions in a way that they think makes them look good. For example, people may report drinking less alcohol than is actually the case.
Any number of research questions can be answered through the use of surveys. One real-world example is the research conducted by Jenkins, Ruppel, Kizer, Yehl, and Griffin (2012) about the backlash against the US Arab-American community following the terrorist attacks of September 11, 2001. Jenkins and colleagues wanted to determine to what extent these negative attitudes toward Arab-Americans still existed nearly a decade after the attacks occurred. In one study, 140 research participants filled out a survey with 10 questions, including questions asking directly about the participant’s overt prejudicial attitudes toward people of various ethnicities. The survey also asked indirect questions about how likely the participant would be to interact with a person of a given ethnicity in a variety of settings (such as, “How likely do you think it is that you would introduce yourself to a person of Arab-American descent?”). The results of the research suggested that participants were unwilling to report prejudicial attitudes toward any ethnic group. However, there were significant differences between their pattern of responses to questions about social interaction with Arab-Americans compared to other ethnic groups: they indicated less willingness for social interaction with Arab-Americans compared to the other ethnic groups. This suggested that the participants harbored subtle forms of prejudice against Arab-Americans, despite their assertions that this was not the case (Jenkins et al., 2012).
Think It Over
Archival research.
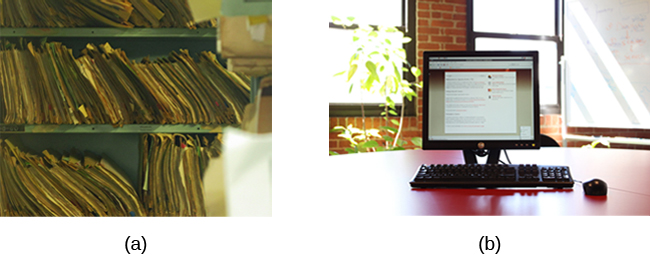
In comparing archival research to other research methods, there are several important distinctions. For one, the researcher employing archival research never directly interacts with research participants. Therefore, the investment of time and money to collect data is considerably less with archival research. Additionally, researchers have no control over what information was originally collected. Therefore, research questions have to be tailored so they can be answered within the structure of the existing data sets. There is also no guarantee of consistency between the records from one source to another, which might make comparing and contrasting different data sets problematic.
Longitudinal and Cross-Sectional Research
Sometimes we want to see how people change over time, as in studies of human development and lifespan. When we test the same group of individuals repeatedly over an extended period of time, we are conducting longitudinal research. Longitudinal research is a research design in which data-gathering is administered repeatedly over an extended period of time. For example, we may survey a group of individuals about their dietary habits at age 20, retest them a decade later at age 30, and then again at age 40.
Another approach is cross-sectional research . In cross-sectional research, a researcher compares multiple segments of the population at the same time. Using the dietary habits example above, the researcher might directly compare different groups of people by age. Instead of observing a group of people for 20 years to see how their dietary habits changed from decade to decade, the researcher would study a group of 20-year-old individuals and compare them to a group of 30-year-old individuals and a group of 40-year-old individuals. While cross-sectional research requires a shorter-term investment, it is also limited by differences that exist between the different generations (or cohorts) that have nothing to do with age per se, but rather reflect the social and cultural experiences of different generations of individuals make them different from one another.
To illustrate this concept, consider the following survey findings. In recent years there has been significant growth in the popular support of same-sex marriage. Many studies on this topic break down survey participants into different age groups. In general, younger people are more supportive of same-sex marriage than are those who are older (Jones, 2013). Does this mean that as we age we become less open to the idea of same-sex marriage, or does this mean that older individuals have different perspectives because of the social climates in which they grew up? Longitudinal research is a powerful approach because the same individuals are involved in the research project over time, which means that the researchers need to be less concerned with differences among cohorts affecting the results of their study.
Often longitudinal studies are employed when researching various diseases in an effort to understand particular risk factors. Such studies often involve tens of thousands of individuals who are followed for several decades. Given the enormous number of people involved in these studies, researchers can feel confident that their findings can be generalized to the larger population. The Cancer Prevention Study-3 (CPS-3) is one of a series of longitudinal studies sponsored by the American Cancer Society aimed at determining predictive risk factors associated with cancer. When participants enter the study, they complete a survey about their lives and family histories, providing information on factors that might cause or prevent the development of cancer. Then every few years the participants receive additional surveys to complete. In the end, hundreds of thousands of participants will be tracked over 20 years to determine which of them develop cancer and which do not.
Clearly, this type of research is important and potentially very informative. For instance, earlier longitudinal studies sponsored by the American Cancer Society provided some of the first scientific demonstrations of the now well-established links between increased rates of cancer and smoking (American Cancer Society, n.d.) (Figure 13).
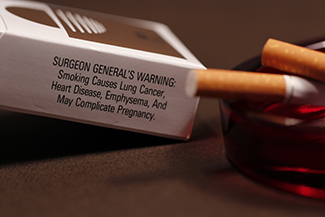
As with any research strategy, longitudinal research is not without limitations. For one, these studies require an incredible time investment by the researcher and research participants. Given that some longitudinal studies take years, if not decades, to complete, the results will not be known for a considerable period of time. In addition to the time demands, these studies also require a substantial financial investment. Many researchers are unable to commit the resources necessary to see a longitudinal project through to the end.
Research participants must also be willing to continue their participation for an extended period of time, and this can be problematic. People move, get married and take new names, get ill, and eventually die. Even without significant life changes, some people may simply choose to discontinue their participation in the project. As a result, the attrition rates, or reduction in the number of research participants due to dropouts, in longitudinal studies are quite high and increases over the course of a project. For this reason, researchers using this approach typically recruit many participants fully expecting that a substantial number will drop out before the end. As the study progresses, they continually check whether the sample still represents the larger population, and make adjustments as necessary.
Correlational Research
Did you know that as sales in ice cream increase, so does the overall rate of crime? Is it possible that indulging in your favorite flavor of ice cream could send you on a crime spree? Or, after committing crime do you think you might decide to treat yourself to a cone? There is no question that a relationship exists between ice cream and crime (e.g., Harper, 2013), but it would be pretty foolish to decide that one thing actually caused the other to occur.
It is much more likely that both ice cream sales and crime rates are related to the temperature outside. When the temperature is warm, there are lots of people out of their houses, interacting with each other, getting annoyed with one another, and sometimes committing crimes. Also, when it is warm outside, we are more likely to seek a cool treat like ice cream. How do we determine if there is indeed a relationship between two things? And when there is a relationship, how can we discern whether it is attributable to coincidence or causation?
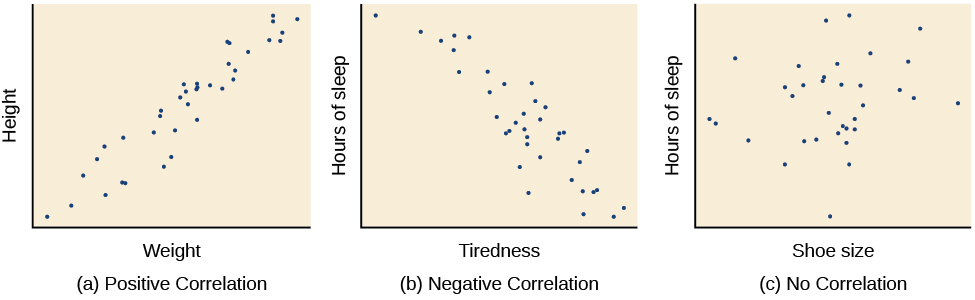
Correlation Does Not Indicate Causation
Correlational research is useful because it allows us to discover the strength and direction of relationships that exist between two variables. However, correlation is limited because establishing the existence of a relationship tells us little about cause and effect . While variables are sometimes correlated because one does cause the other, it could also be that some other factor, a confounding variable , is actually causing the systematic movement in our variables of interest. In the ice cream/crime rate example mentioned earlier, temperature is a confounding variable that could account for the relationship between the two variables.
Even when we cannot point to clear confounding variables, we should not assume that a correlation between two variables implies that one variable causes changes in another. This can be frustrating when a cause-and-effect relationship seems clear and intuitive. Think back to our discussion of the research done by the American Cancer Society and how their research projects were some of the first demonstrations of the link between smoking and cancer. It seems reasonable to assume that smoking causes cancer, but if we were limited to correlational research , we would be overstepping our bounds by making this assumption.
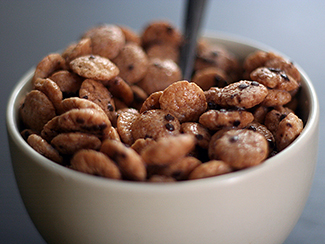
Unfortunately, people mistakenly make claims of causation as a function of correlations all the time. Such claims are especially common in advertisements and news stories. For example, recent research found that people who eat cereal on a regular basis achieve healthier weights than those who rarely eat cereal (Frantzen, Treviño, Echon, Garcia-Dominic, & DiMarco, 2013; Barton et al., 2005). Guess how the cereal companies report this finding. Does eating cereal really cause an individual to maintain a healthy weight, or are there other possible explanations, such as, someone at a healthy weight is more likely to regularly eat a healthy breakfast than someone who is obese or someone who avoids meals in an attempt to diet (Figure 15)? While correlational research is invaluable in identifying relationships among variables, a major limitation is the inability to establish causality. Psychologists want to make statements about cause and effect, but the only way to do that is to conduct an experiment to answer a research question. The next section describes how scientific experiments incorporate methods that eliminate, or control for, alternative explanations, which allow researchers to explore how changes in one variable cause changes in another variable.
Watch this clip from Freakonomics for an example of how correlation does not indicate causation.
You can view the transcript for “Correlation vs. Causality: Freakonomics Movie” here (opens in new window) .
Illusory Correlations
The temptation to make erroneous cause-and-effect statements based on correlational research is not the only way we tend to misinterpret data. We also tend to make the mistake of illusory correlations, especially with unsystematic observations. Illusory correlations , or false correlations, occur when people believe that relationships exist between two things when no such relationship exists. One well-known illusory correlation is the supposed effect that the moon’s phases have on human behavior. Many people passionately assert that human behavior is affected by the phase of the moon, and specifically, that people act strangely when the moon is full (Figure 16).
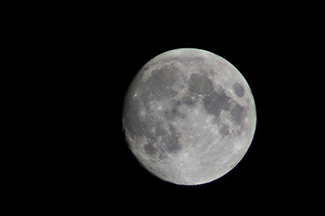
There is no denying that the moon exerts a powerful influence on our planet. The ebb and flow of the ocean’s tides are tightly tied to the gravitational forces of the moon. Many people believe, therefore, that it is logical that we are affected by the moon as well. After all, our bodies are largely made up of water. A meta-analysis of nearly 40 studies consistently demonstrated, however, that the relationship between the moon and our behavior does not exist (Rotton & Kelly, 1985). While we may pay more attention to odd behavior during the full phase of the moon, the rates of odd behavior remain constant throughout the lunar cycle.
Why are we so apt to believe in illusory correlations like this? Often we read or hear about them and simply accept the information as valid. Or, we have a hunch about how something works and then look for evidence to support that hunch, ignoring evidence that would tell us our hunch is false; this is known as confirmation bias . Other times, we find illusory correlations based on the information that comes most easily to mind, even if that information is severely limited. And while we may feel confident that we can use these relationships to better understand and predict the world around us, illusory correlations can have significant drawbacks. For example, research suggests that illusory correlations—in which certain behaviors are inaccurately attributed to certain groups—are involved in the formation of prejudicial attitudes that can ultimately lead to discriminatory behavior (Fiedler, 2004).
We all have a tendency to make illusory correlations from time to time. Try to think of an illusory correlation that is held by you, a family member, or a close friend. How do you think this illusory correlation came about and what can be done in the future to combat them?
Experiments
Causality: conducting experiments and using the data, experimental hypothesis.
In order to conduct an experiment, a researcher must have a specific hypothesis to be tested. As you’ve learned, hypotheses can be formulated either through direct observation of the real world or after careful review of previous research. For example, if you think that children should not be allowed to watch violent programming on television because doing so would cause them to behave more violently, then you have basically formulated a hypothesis—namely, that watching violent television programs causes children to behave more violently. How might you have arrived at this particular hypothesis? You may have younger relatives who watch cartoons featuring characters using martial arts to save the world from evildoers, with an impressive array of punching, kicking, and defensive postures. You notice that after watching these programs for a while, your young relatives mimic the fighting behavior of the characters portrayed in the cartoon (Figure 17).
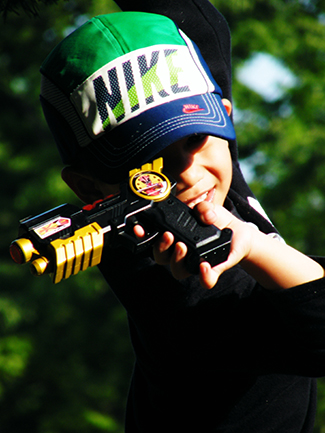
These sorts of personal observations are what often lead us to formulate a specific hypothesis, but we cannot use limited personal observations and anecdotal evidence to rigorously test our hypothesis. Instead, to find out if real-world data supports our hypothesis, we have to conduct an experiment.
Designing an Experiment
The most basic experimental design involves two groups: the experimental group and the control group. The two groups are designed to be the same except for one difference— experimental manipulation. The experimental group gets the experimental manipulation—that is, the treatment or variable being tested (in this case, violent TV images)—and the control group does not. Since experimental manipulation is the only difference between the experimental and control groups, we can be sure that any differences between the two are due to experimental manipulation rather than chance.
In our example of how violent television programming might affect violent behavior in children, we have the experimental group view violent television programming for a specified time and then measure their violent behavior. We measure the violent behavior in our control group after they watch nonviolent television programming for the same amount of time. It is important for the control group to be treated similarly to the experimental group, with the exception that the control group does not receive the experimental manipulation. Therefore, we have the control group watch non-violent television programming for the same amount of time as the experimental group.
We also need to precisely define, or operationalize, what is considered violent and nonviolent. An operational definition is a description of how we will measure our variables, and it is important in allowing others understand exactly how and what a researcher measures in a particular experiment. In operationalizing violent behavior, we might choose to count only physical acts like kicking or punching as instances of this behavior, or we also may choose to include angry verbal exchanges. Whatever we determine, it is important that we operationalize violent behavior in such a way that anyone who hears about our study for the first time knows exactly what we mean by violence. This aids peoples’ ability to interpret our data as well as their capacity to repeat our experiment should they choose to do so.
Once we have operationalized what is considered violent television programming and what is considered violent behavior from our experiment participants, we need to establish how we will run our experiment. In this case, we might have participants watch a 30-minute television program (either violent or nonviolent, depending on their group membership) before sending them out to a playground for an hour where their behavior is observed and the number and type of violent acts is recorded.
Ideally, the people who observe and record the children’s behavior are unaware of who was assigned to the experimental or control group, in order to control for experimenter bias. Experimenter bias refers to the possibility that a researcher’s expectations might skew the results of the study. Remember, conducting an experiment requires a lot of planning, and the people involved in the research project have a vested interest in supporting their hypotheses. If the observers knew which child was in which group, it might influence how much attention they paid to each child’s behavior as well as how they interpreted that behavior. By being blind to which child is in which group, we protect against those biases. This situation is a single-blind study , meaning that one of the groups (participants) are unaware as to which group they are in (experiment or control group) while the researcher who developed the experiment knows which participants are in each group.
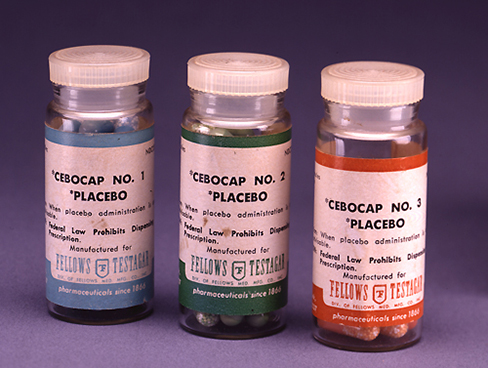
In a double-blind study , both the researchers and the participants are blind to group assignments. Why would a researcher want to run a study where no one knows who is in which group? Because by doing so, we can control for both experimenter and participant expectations. If you are familiar with the phrase placebo effect, you already have some idea as to why this is an important consideration. The placebo effect occurs when people’s expectations or beliefs influence or determine their experience in a given situation. In other words, simply expecting something to happen can actually make it happen.
The placebo effect is commonly described in terms of testing the effectiveness of a new medication. Imagine that you work in a pharmaceutical company, and you think you have a new drug that is effective in treating depression. To demonstrate that your medication is effective, you run an experiment with two groups: The experimental group receives the medication, and the control group does not. But you don’t want participants to know whether they received the drug or not.
Why is that? Imagine that you are a participant in this study, and you have just taken a pill that you think will improve your mood. Because you expect the pill to have an effect, you might feel better simply because you took the pill and not because of any drug actually contained in the pill—this is the placebo effect.
To make sure that any effects on mood are due to the drug and not due to expectations, the control group receives a placebo (in this case a sugar pill). Now everyone gets a pill, and once again neither the researcher nor the experimental participants know who got the drug and who got the sugar pill. Any differences in mood between the experimental and control groups can now be attributed to the drug itself rather than to experimenter bias or participant expectations (Figure 18).
Independent and Dependent Variables
In a research experiment, we strive to study whether changes in one thing cause changes in another. To achieve this, we must pay attention to two important variables, or things that can be changed, in any experimental study: the independent variable and the dependent variable. An independent variable is manipulated or controlled by the experimenter. In a well-designed experimental study, the independent variable is the only important difference between the experimental and control groups. In our example of how violent television programs affect children’s display of violent behavior, the independent variable is the type of program—violent or nonviolent—viewed by participants in the study (Figure 19). A dependent variable is what the researcher measures to see how much effect the independent variable had. In our example, the dependent variable is the number of violent acts displayed by the experimental participants.
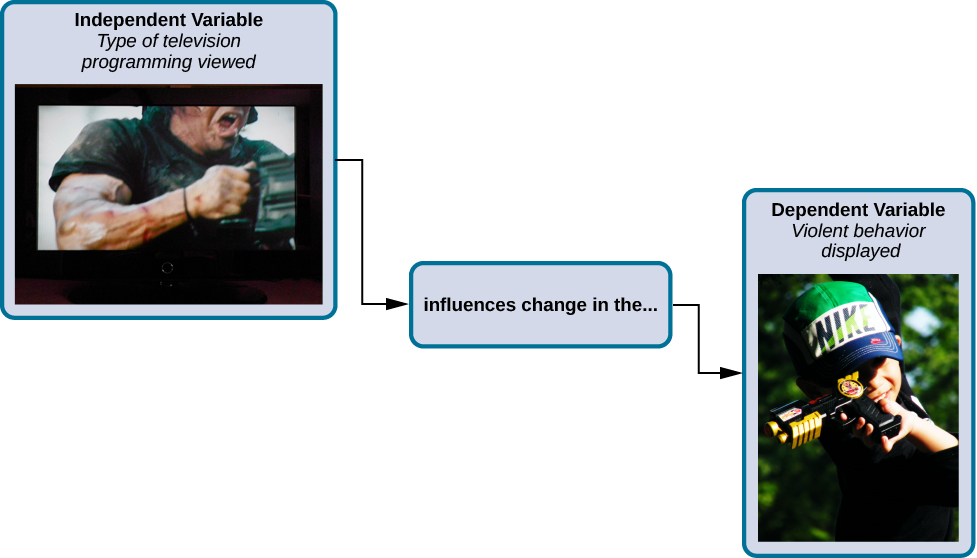
We expect that the dependent variable will change as a function of the independent variable. In other words, the dependent variable depends on the independent variable. A good way to think about the relationship between the independent and dependent variables is with this question: What effect does the independent variable have on the dependent variable? Returning to our example, what effect does watching a half hour of violent television programming or nonviolent television programming have on the number of incidents of physical aggression displayed on the playground?
Selecting and Assigning Experimental Participants
Now that our study is designed, we need to obtain a sample of individuals to include in our experiment. Our study involves human participants so we need to determine who to include. Participants are the subjects of psychological research, and as the name implies, individuals who are involved in psychological research actively participate in the process. Often, psychological research projects rely on college students to serve as participants. In fact, the vast majority of research in psychology subfields has historically involved students as research participants (Sears, 1986; Arnett, 2008). But are college students truly representative of the general population? College students tend to be younger, more educated, more liberal, and less diverse than the general population. Although using students as test subjects is an accepted practice, relying on such a limited pool of research participants can be problematic because it is difficult to generalize findings to the larger population.
Our hypothetical experiment involves children, and we must first generate a sample of child participants. Samples are used because populations are usually too large to reasonably involve every member in our particular experiment (Figure 20). If possible, we should use a random sample (there are other types of samples, but for the purposes of this section, we will focus on random samples). A random sample is a subset of a larger population in which every member of the population has an equal chance of being selected. Random samples are preferred because if the sample is large enough we can be reasonably sure that the participating individuals are representative of the larger population. This means that the percentages of characteristics in the sample—sex, ethnicity, socioeconomic level, and any other characteristics that might affect the results—are close to those percentages in the larger population.
In our example, let’s say we decide our population of interest is fourth graders. But all fourth graders is a very large population, so we need to be more specific; instead we might say our population of interest is all fourth graders in a particular city. We should include students from various income brackets, family situations, races, ethnicities, religions, and geographic areas of town. With this more manageable population, we can work with the local schools in selecting a random sample of around 200 fourth graders who we want to participate in our experiment.
In summary, because we cannot test all of the fourth graders in a city, we want to find a group of about 200 that reflects the composition of that city. With a representative group, we can generalize our findings to the larger population without fear of our sample being biased in some way.
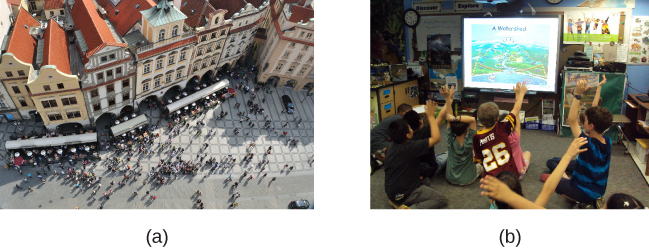
Now that we have a sample, the next step of the experimental process is to split the participants into experimental and control groups through random assignment. With random assignment , all participants have an equal chance of being assigned to either group. There is statistical software that will randomly assign each of the fourth graders in the sample to either the experimental or the control group.
Random assignment is critical for sound experimental design. With sufficiently large samples, random assignment makes it unlikely that there are systematic differences between the groups. So, for instance, it would be very unlikely that we would get one group composed entirely of males, a given ethnic identity, or a given religious ideology. This is important because if the groups were systematically different before the experiment began, we would not know the origin of any differences we find between the groups: Were the differences preexisting, or were they caused by manipulation of the independent variable? Random assignment allows us to assume that any differences observed between experimental and control groups result from the manipulation of the independent variable.
Issues to Consider
While experiments allow scientists to make cause-and-effect claims, they are not without problems. True experiments require the experimenter to manipulate an independent variable, and that can complicate many questions that psychologists might want to address. For instance, imagine that you want to know what effect sex (the independent variable) has on spatial memory (the dependent variable). Although you can certainly look for differences between males and females on a task that taps into spatial memory, you cannot directly control a person’s sex. We categorize this type of research approach as quasi-experimental and recognize that we cannot make cause-and-effect claims in these circumstances.
Experimenters are also limited by ethical constraints. For instance, you would not be able to conduct an experiment designed to determine if experiencing abuse as a child leads to lower levels of self-esteem among adults. To conduct such an experiment, you would need to randomly assign some experimental participants to a group that receives abuse, and that experiment would be unethical.
Introduction to Statistical Thinking
Psychologists use statistics to assist them in analyzing data, and also to give more precise measurements to describe whether something is statistically significant. Analyzing data using statistics enables researchers to find patterns, make claims, and share their results with others. In this section, you’ll learn about some of the tools that psychologists use in statistical analysis.
- Define reliability and validity
- Describe the importance of distributional thinking and the role of p-values in statistical inference
- Describe the role of random sampling and random assignment in drawing cause-and-effect conclusions
- Describe the basic structure of a psychological research article
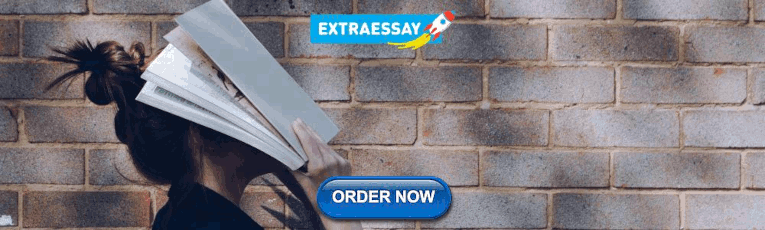
Interpreting Experimental Findings
Once data is collected from both the experimental and the control groups, a statistical analysis is conducted to find out if there are meaningful differences between the two groups. A statistical analysis determines how likely any difference found is due to chance (and thus not meaningful). In psychology, group differences are considered meaningful, or significant, if the odds that these differences occurred by chance alone are 5 percent or less. Stated another way, if we repeated this experiment 100 times, we would expect to find the same results at least 95 times out of 100.
The greatest strength of experiments is the ability to assert that any significant differences in the findings are caused by the independent variable. This occurs because random selection, random assignment, and a design that limits the effects of both experimenter bias and participant expectancy should create groups that are similar in composition and treatment. Therefore, any difference between the groups is attributable to the independent variable, and now we can finally make a causal statement. If we find that watching a violent television program results in more violent behavior than watching a nonviolent program, we can safely say that watching violent television programs causes an increase in the display of violent behavior.
Reporting Research
When psychologists complete a research project, they generally want to share their findings with other scientists. The American Psychological Association (APA) publishes a manual detailing how to write a paper for submission to scientific journals. Unlike an article that might be published in a magazine like Psychology Today, which targets a general audience with an interest in psychology, scientific journals generally publish peer-reviewed journal articles aimed at an audience of professionals and scholars who are actively involved in research themselves.
A peer-reviewed journal article is read by several other scientists (generally anonymously) with expertise in the subject matter. These peer reviewers provide feedback—to both the author and the journal editor—regarding the quality of the draft. Peer reviewers look for a strong rationale for the research being described, a clear description of how the research was conducted, and evidence that the research was conducted in an ethical manner. They also look for flaws in the study’s design, methods, and statistical analyses. They check that the conclusions drawn by the authors seem reasonable given the observations made during the research. Peer reviewers also comment on how valuable the research is in advancing the discipline’s knowledge. This helps prevent unnecessary duplication of research findings in the scientific literature and, to some extent, ensures that each research article provides new information. Ultimately, the journal editor will compile all of the peer reviewer feedback and determine whether the article will be published in its current state (a rare occurrence), published with revisions, or not accepted for publication.
Peer review provides some degree of quality control for psychological research. Poorly conceived or executed studies can be weeded out, and even well-designed research can be improved by the revisions suggested. Peer review also ensures that the research is described clearly enough to allow other scientists to replicate it, meaning they can repeat the experiment using different samples to determine reliability. Sometimes replications involve additional measures that expand on the original finding. In any case, each replication serves to provide more evidence to support the original research findings. Successful replications of published research make scientists more apt to adopt those findings, while repeated failures tend to cast doubt on the legitimacy of the original article and lead scientists to look elsewhere. For example, it would be a major advancement in the medical field if a published study indicated that taking a new drug helped individuals achieve a healthy weight without changing their diet. But if other scientists could not replicate the results, the original study’s claims would be questioned.
Dig Deeper: The Vaccine-Autism Myth and the Retraction of Published Studies
Some scientists have claimed that routine childhood vaccines cause some children to develop autism, and, in fact, several peer-reviewed publications published research making these claims. Since the initial reports, large-scale epidemiological research has suggested that vaccinations are not responsible for causing autism and that it is much safer to have your child vaccinated than not. Furthermore, several of the original studies making this claim have since been retracted.
A published piece of work can be rescinded when data is called into question because of falsification, fabrication, or serious research design problems. Once rescinded, the scientific community is informed that there are serious problems with the original publication. Retractions can be initiated by the researcher who led the study, by research collaborators, by the institution that employed the researcher, or by the editorial board of the journal in which the article was originally published. In the vaccine-autism case, the retraction was made because of a significant conflict of interest in which the leading researcher had a financial interest in establishing a link between childhood vaccines and autism (Offit, 2008). Unfortunately, the initial studies received so much media attention that many parents around the world became hesitant to have their children vaccinated (Figure 21). For more information about how the vaccine/autism story unfolded, as well as the repercussions of this story, take a look at Paul Offit’s book, Autism’s False Prophets: Bad Science, Risky Medicine, and the Search for a Cure.
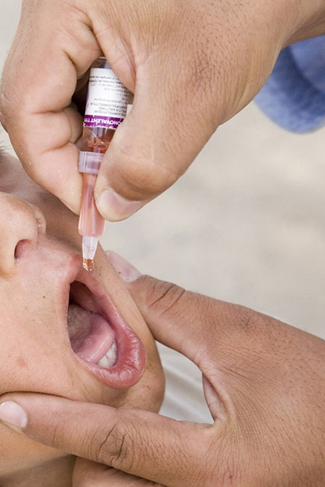
Reliability and Validity
Dig deeper: everyday connection: how valid is the sat.
Standardized tests like the SAT are supposed to measure an individual’s aptitude for a college education, but how reliable and valid are such tests? Research conducted by the College Board suggests that scores on the SAT have high predictive validity for first-year college students’ GPA (Kobrin, Patterson, Shaw, Mattern, & Barbuti, 2008). In this context, predictive validity refers to the test’s ability to effectively predict the GPA of college freshmen. Given that many institutions of higher education require the SAT for admission, this high degree of predictive validity might be comforting.
However, the emphasis placed on SAT scores in college admissions has generated some controversy on a number of fronts. For one, some researchers assert that the SAT is a biased test that places minority students at a disadvantage and unfairly reduces the likelihood of being admitted into a college (Santelices & Wilson, 2010). Additionally, some research has suggested that the predictive validity of the SAT is grossly exaggerated in how well it is able to predict the GPA of first-year college students. In fact, it has been suggested that the SAT’s predictive validity may be overestimated by as much as 150% (Rothstein, 2004). Many institutions of higher education are beginning to consider de-emphasizing the significance of SAT scores in making admission decisions (Rimer, 2008).
In 2014, College Board president David Coleman expressed his awareness of these problems, recognizing that college success is more accurately predicted by high school grades than by SAT scores. To address these concerns, he has called for significant changes to the SAT exam (Lewin, 2014).
Statistical Significance
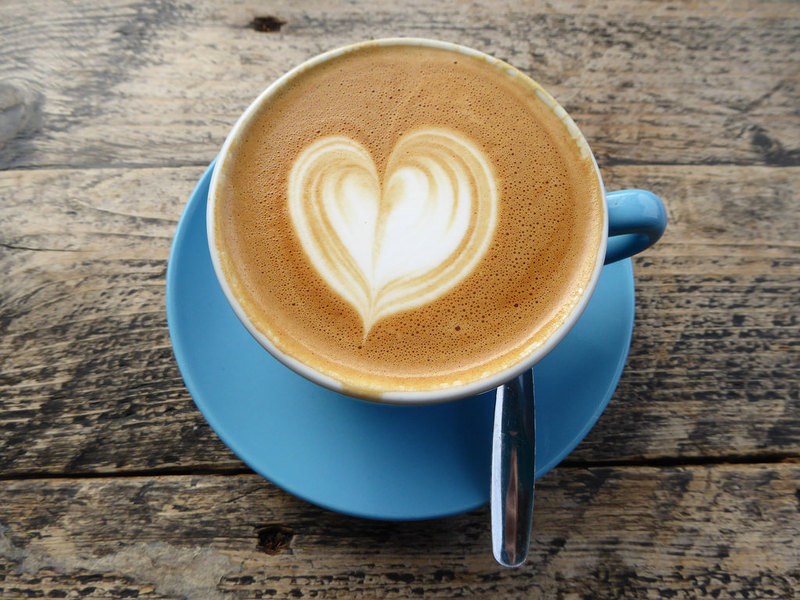
Does drinking coffee actually increase your life expectancy? A recent study (Freedman, Park, Abnet, Hollenbeck, & Sinha, 2012) found that men who drank at least six cups of coffee a day also had a 10% lower chance of dying (women’s chances were 15% lower) than those who drank none. Does this mean you should pick up or increase your own coffee habit? We will explore these results in more depth in the next section about drawing conclusions from statistics. Modern society has become awash in studies such as this; you can read about several such studies in the news every day.
Conducting such a study well, and interpreting the results of such studies requires understanding basic ideas of statistics , the science of gaining insight from data. Key components to a statistical investigation are:
- Planning the study: Start by asking a testable research question and deciding how to collect data. For example, how long was the study period of the coffee study? How many people were recruited for the study, how were they recruited, and from where? How old were they? What other variables were recorded about the individuals? Were changes made to the participants’ coffee habits during the course of the study?
- Examining the data: What are appropriate ways to examine the data? What graphs are relevant, and what do they reveal? What descriptive statistics can be calculated to summarize relevant aspects of the data, and what do they reveal? What patterns do you see in the data? Are there any individual observations that deviate from the overall pattern, and what do they reveal? For example, in the coffee study, did the proportions differ when we compared the smokers to the non-smokers?
- Inferring from the data: What are valid statistical methods for drawing inferences “beyond” the data you collected? In the coffee study, is the 10%–15% reduction in risk of death something that could have happened just by chance?
- Drawing conclusions: Based on what you learned from your data, what conclusions can you draw? Who do you think these conclusions apply to? (Were the people in the coffee study older? Healthy? Living in cities?) Can you draw a cause-and-effect conclusion about your treatments? (Are scientists now saying that the coffee drinking is the cause of the decreased risk of death?)
Notice that the numerical analysis (“crunching numbers” on the computer) comprises only a small part of overall statistical investigation. In this section, you will see how we can answer some of these questions and what questions you should be asking about any statistical investigation you read about.
Distributional Thinking
When data are collected to address a particular question, an important first step is to think of meaningful ways to organize and examine the data. Let’s take a look at an example.
Example 1 : Researchers investigated whether cancer pamphlets are written at an appropriate level to be read and understood by cancer patients (Short, Moriarty, & Cooley, 1995). Tests of reading ability were given to 63 patients. In addition, readability level was determined for a sample of 30 pamphlets, based on characteristics such as the lengths of words and sentences in the pamphlet. The results, reported in terms of grade levels, are displayed in Figure 23.

- Data vary . More specifically, values of a variable (such as reading level of a cancer patient or readability level of a cancer pamphlet) vary.
- Analyzing the pattern of variation, called the distribution of the variable, often reveals insights.
Addressing the research question of whether the cancer pamphlets are written at appropriate levels for the cancer patients requires comparing the two distributions. A naïve comparison might focus only on the centers of the distributions. Both medians turn out to be ninth grade, but considering only medians ignores the variability and the overall distributions of these data. A more illuminating approach is to compare the entire distributions, for example with a graph, as in Figure 24.
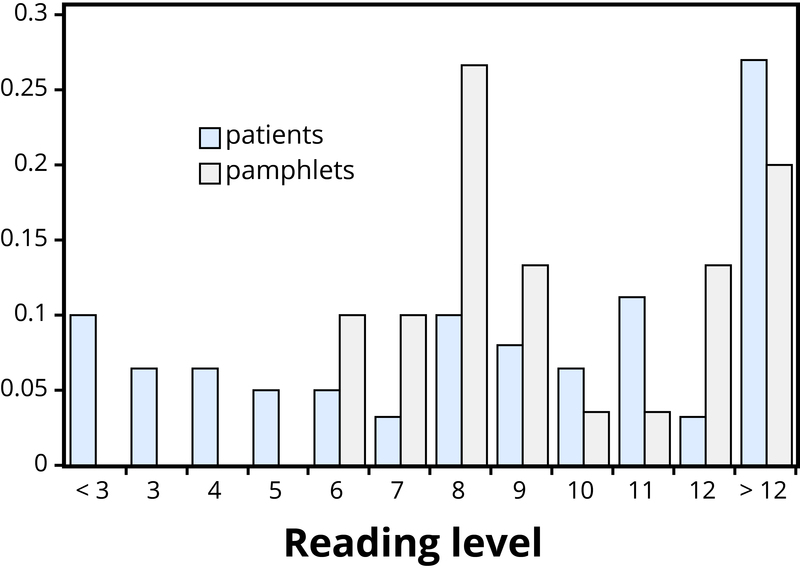
Figure 24 makes clear that the two distributions are not well aligned at all. The most glaring discrepancy is that many patients (17/63, or 27%, to be precise) have a reading level below that of the most readable pamphlet. These patients will need help to understand the information provided in the cancer pamphlets. Notice that this conclusion follows from considering the distributions as a whole, not simply measures of center or variability, and that the graph contrasts those distributions more immediately than the frequency tables.
Finding Significance in Data
Even when we find patterns in data, often there is still uncertainty in various aspects of the data. For example, there may be potential for measurement errors (even your own body temperature can fluctuate by almost 1°F over the course of the day). Or we may only have a “snapshot” of observations from a more long-term process or only a small subset of individuals from the population of interest. In such cases, how can we determine whether patterns we see in our small set of data is convincing evidence of a systematic phenomenon in the larger process or population? Let’s take a look at another example.
Example 2 : In a study reported in the November 2007 issue of Nature , researchers investigated whether pre-verbal infants take into account an individual’s actions toward others in evaluating that individual as appealing or aversive (Hamlin, Wynn, & Bloom, 2007). In one component of the study, 10-month-old infants were shown a “climber” character (a piece of wood with “googly” eyes glued onto it) that could not make it up a hill in two tries. Then the infants were shown two scenarios for the climber’s next try, one where the climber was pushed to the top of the hill by another character (“helper”), and one where the climber was pushed back down the hill by another character (“hinderer”). The infant was alternately shown these two scenarios several times. Then the infant was presented with two pieces of wood (representing the helper and the hinderer characters) and asked to pick one to play with.
The researchers found that of the 16 infants who made a clear choice, 14 chose to play with the helper toy. One possible explanation for this clear majority result is that the helping behavior of the one toy increases the infants’ likelihood of choosing that toy. But are there other possible explanations? What about the color of the toy? Well, prior to collecting the data, the researchers arranged so that each color and shape (red square and blue circle) would be seen by the same number of infants. Or maybe the infants had right-handed tendencies and so picked whichever toy was closer to their right hand?
Well, prior to collecting the data, the researchers arranged it so half the infants saw the helper toy on the right and half on the left. Or, maybe the shapes of these wooden characters (square, triangle, circle) had an effect? Perhaps, but again, the researchers controlled for this by rotating which shape was the helper toy, the hinderer toy, and the climber. When designing experiments, it is important to control for as many variables as might affect the responses as possible. It is beginning to appear that the researchers accounted for all the other plausible explanations. But there is one more important consideration that cannot be controlled—if we did the study again with these 16 infants, they might not make the same choices. In other words, there is some randomness inherent in their selection process.
Maybe each infant had no genuine preference at all, and it was simply “random luck” that led to 14 infants picking the helper toy. Although this random component cannot be controlled, we can apply a probability model to investigate the pattern of results that would occur in the long run if random chance were the only factor.
If the infants were equally likely to pick between the two toys, then each infant had a 50% chance of picking the helper toy. It’s like each infant tossed a coin, and if it landed heads, the infant picked the helper toy. So if we tossed a coin 16 times, could it land heads 14 times? Sure, it’s possible, but it turns out to be very unlikely. Getting 14 (or more) heads in 16 tosses is about as likely as tossing a coin and getting 9 heads in a row. This probability is referred to as a p-value . The p-value represents the likelihood that experimental results happened by chance. Within psychology, the most common standard for p-values is “p < .05”. What this means is that there is less than a 5% probability that the results happened just by random chance, and therefore a 95% probability that the results reflect a meaningful pattern in human psychology. We call this statistical significance .
So, in the study above, if we assume that each infant was choosing equally, then the probability that 14 or more out of 16 infants would choose the helper toy is found to be 0.0021. We have only two logical possibilities: either the infants have a genuine preference for the helper toy, or the infants have no preference (50/50) and an outcome that would occur only 2 times in 1,000 iterations happened in this study. Because this p-value of 0.0021 is quite small, we conclude that the study provides very strong evidence that these infants have a genuine preference for the helper toy.
If we compare the p-value to some cut-off value, like 0.05, we see that the p=value is smaller. Because the p-value is smaller than that cut-off value, then we reject the hypothesis that only random chance was at play here. In this case, these researchers would conclude that significantly more than half of the infants in the study chose the helper toy, giving strong evidence of a genuine preference for the toy with the helping behavior.
Drawing Conclusions from Statistics
Generalizability.
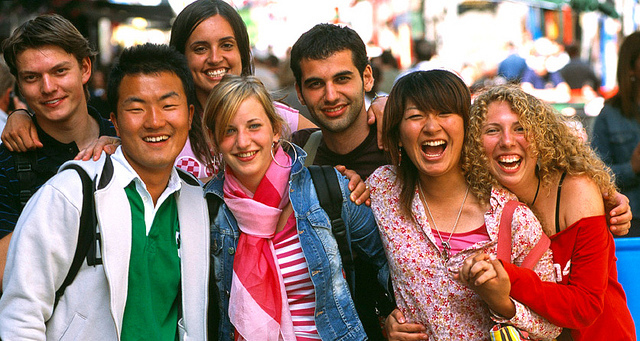
One limitation to the study mentioned previously about the babies choosing the “helper” toy is that the conclusion only applies to the 16 infants in the study. We don’t know much about how those 16 infants were selected. Suppose we want to select a subset of individuals (a sample ) from a much larger group of individuals (the population ) in such a way that conclusions from the sample can be generalized to the larger population. This is the question faced by pollsters every day.
Example 3 : The General Social Survey (GSS) is a survey on societal trends conducted every other year in the United States. Based on a sample of about 2,000 adult Americans, researchers make claims about what percentage of the U.S. population consider themselves to be “liberal,” what percentage consider themselves “happy,” what percentage feel “rushed” in their daily lives, and many other issues. The key to making these claims about the larger population of all American adults lies in how the sample is selected. The goal is to select a sample that is representative of the population, and a common way to achieve this goal is to select a r andom sample that gives every member of the population an equal chance of being selected for the sample. In its simplest form, random sampling involves numbering every member of the population and then using a computer to randomly select the subset to be surveyed. Most polls don’t operate exactly like this, but they do use probability-based sampling methods to select individuals from nationally representative panels.
In 2004, the GSS reported that 817 of 977 respondents (or 83.6%) indicated that they always or sometimes feel rushed. This is a clear majority, but we again need to consider variation due to random sampling . Fortunately, we can use the same probability model we did in the previous example to investigate the probable size of this error. (Note, we can use the coin-tossing model when the actual population size is much, much larger than the sample size, as then we can still consider the probability to be the same for every individual in the sample.) This probability model predicts that the sample result will be within 3 percentage points of the population value (roughly 1 over the square root of the sample size, the margin of error. A statistician would conclude, with 95% confidence, that between 80.6% and 86.6% of all adult Americans in 2004 would have responded that they sometimes or always feel rushed.
The key to the margin of error is that when we use a probability sampling method, we can make claims about how often (in the long run, with repeated random sampling) the sample result would fall within a certain distance from the unknown population value by chance (meaning by random sampling variation) alone. Conversely, non-random samples are often suspect to bias, meaning the sampling method systematically over-represents some segments of the population and under-represents others. We also still need to consider other sources of bias, such as individuals not responding honestly. These sources of error are not measured by the margin of error.
Cause and Effect
In many research studies, the primary question of interest concerns differences between groups. Then the question becomes how were the groups formed (e.g., selecting people who already drink coffee vs. those who don’t). In some studies, the researchers actively form the groups themselves. But then we have a similar question—could any differences we observe in the groups be an artifact of that group-formation process? Or maybe the difference we observe in the groups is so large that we can discount a “fluke” in the group-formation process as a reasonable explanation for what we find?
Example 4 : A psychology study investigated whether people tend to display more creativity when they are thinking about intrinsic (internal) or extrinsic (external) motivations (Ramsey & Schafer, 2002, based on a study by Amabile, 1985). The subjects were 47 people with extensive experience with creative writing. Subjects began by answering survey questions about either intrinsic motivations for writing (such as the pleasure of self-expression) or extrinsic motivations (such as public recognition). Then all subjects were instructed to write a haiku, and those poems were evaluated for creativity by a panel of judges. The researchers conjectured beforehand that subjects who were thinking about intrinsic motivations would display more creativity than subjects who were thinking about extrinsic motivations. The creativity scores from the 47 subjects in this study are displayed in Figure 26, where higher scores indicate more creativity.
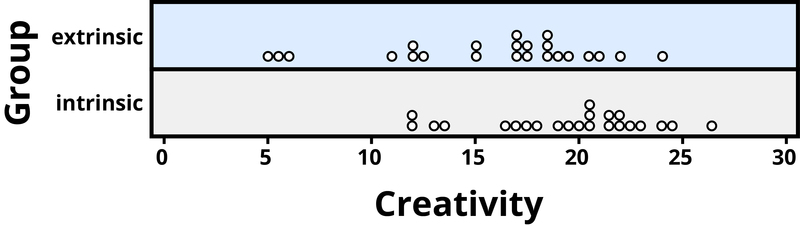
In this example, the key question is whether the type of motivation affects creativity scores. In particular, do subjects who were asked about intrinsic motivations tend to have higher creativity scores than subjects who were asked about extrinsic motivations?
Figure 26 reveals that both motivation groups saw considerable variability in creativity scores, and these scores have considerable overlap between the groups. In other words, it’s certainly not always the case that those with extrinsic motivations have higher creativity than those with intrinsic motivations, but there may still be a statistical tendency in this direction. (Psychologist Keith Stanovich (2013) refers to people’s difficulties with thinking about such probabilistic tendencies as “the Achilles heel of human cognition.”)
The mean creativity score is 19.88 for the intrinsic group, compared to 15.74 for the extrinsic group, which supports the researchers’ conjecture. Yet comparing only the means of the two groups fails to consider the variability of creativity scores in the groups. We can measure variability with statistics using, for instance, the standard deviation: 5.25 for the extrinsic group and 4.40 for the intrinsic group. The standard deviations tell us that most of the creativity scores are within about 5 points of the mean score in each group. We see that the mean score for the intrinsic group lies within one standard deviation of the mean score for extrinsic group. So, although there is a tendency for the creativity scores to be higher in the intrinsic group, on average, the difference is not extremely large.
We again want to consider possible explanations for this difference. The study only involved individuals with extensive creative writing experience. Although this limits the population to which we can generalize, it does not explain why the mean creativity score was a bit larger for the intrinsic group than for the extrinsic group. Maybe women tend to receive higher creativity scores? Here is where we need to focus on how the individuals were assigned to the motivation groups. If only women were in the intrinsic motivation group and only men in the extrinsic group, then this would present a problem because we wouldn’t know if the intrinsic group did better because of the different type of motivation or because they were women. However, the researchers guarded against such a problem by randomly assigning the individuals to the motivation groups. Like flipping a coin, each individual was just as likely to be assigned to either type of motivation. Why is this helpful? Because this random assignment tends to balance out all the variables related to creativity we can think of, and even those we don’t think of in advance, between the two groups. So we should have a similar male/female split between the two groups; we should have a similar age distribution between the two groups; we should have a similar distribution of educational background between the two groups; and so on. Random assignment should produce groups that are as similar as possible except for the type of motivation, which presumably eliminates all those other variables as possible explanations for the observed tendency for higher scores in the intrinsic group.
But does this always work? No, so by “luck of the draw” the groups may be a little different prior to answering the motivation survey. So then the question is, is it possible that an unlucky random assignment is responsible for the observed difference in creativity scores between the groups? In other words, suppose each individual’s poem was going to get the same creativity score no matter which group they were assigned to, that the type of motivation in no way impacted their score. Then how often would the random-assignment process alone lead to a difference in mean creativity scores as large (or larger) than 19.88 – 15.74 = 4.14 points?
We again want to apply to a probability model to approximate a p-value , but this time the model will be a bit different. Think of writing everyone’s creativity scores on an index card, shuffling up the index cards, and then dealing out 23 to the extrinsic motivation group and 24 to the intrinsic motivation group, and finding the difference in the group means. We (better yet, the computer) can repeat this process over and over to see how often, when the scores don’t change, random assignment leads to a difference in means at least as large as 4.41. Figure 27 shows the results from 1,000 such hypothetical random assignments for these scores.
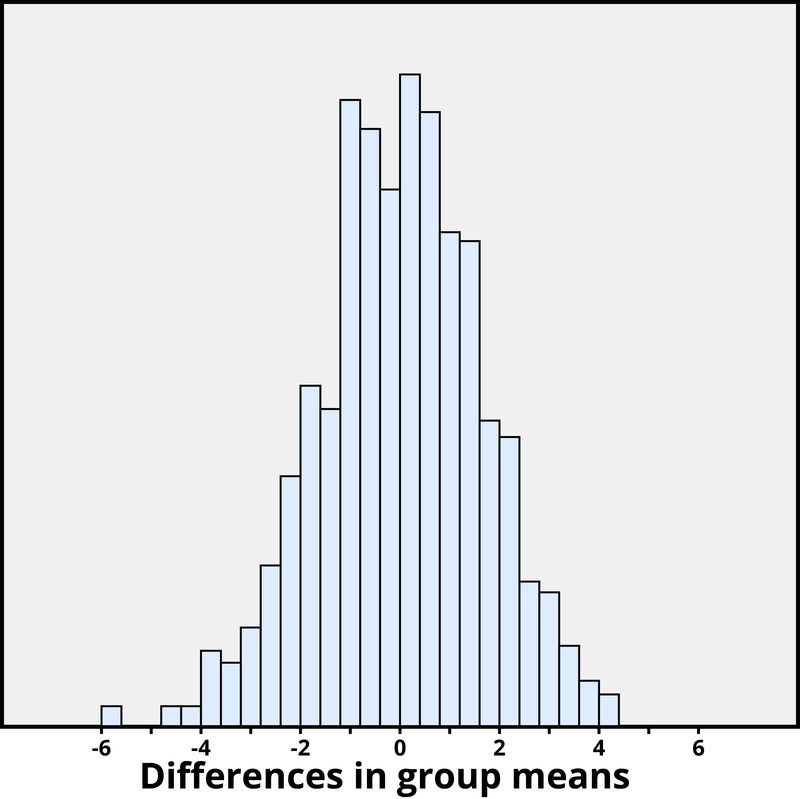
Only 2 of the 1,000 simulated random assignments produced a difference in group means of 4.41 or larger. In other words, the approximate p-value is 2/1000 = 0.002. This small p-value indicates that it would be very surprising for the random assignment process alone to produce such a large difference in group means. Therefore, as with Example 2, we have strong evidence that focusing on intrinsic motivations tends to increase creativity scores, as compared to thinking about extrinsic motivations.
Notice that the previous statement implies a cause-and-effect relationship between motivation and creativity score; is such a strong conclusion justified? Yes, because of the random assignment used in the study. That should have balanced out any other variables between the two groups, so now that the small p-value convinces us that the higher mean in the intrinsic group wasn’t just a coincidence, the only reasonable explanation left is the difference in the type of motivation. Can we generalize this conclusion to everyone? Not necessarily—we could cautiously generalize this conclusion to individuals with extensive experience in creative writing similar the individuals in this study, but we would still want to know more about how these individuals were selected to participate.
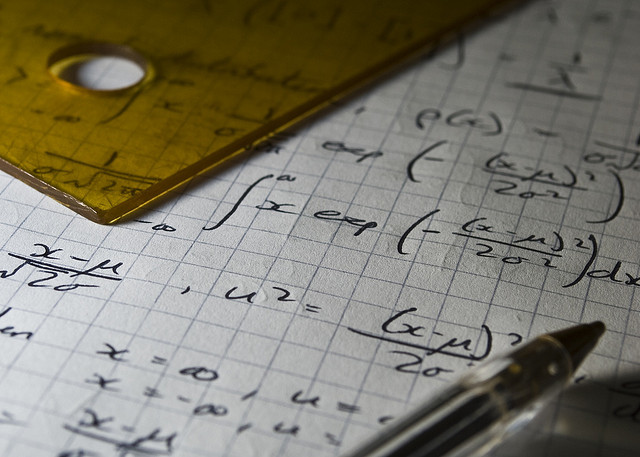
Statistical thinking involves the careful design of a study to collect meaningful data to answer a focused research question, detailed analysis of patterns in the data, and drawing conclusions that go beyond the observed data. Random sampling is paramount to generalizing results from our sample to a larger population, and random assignment is key to drawing cause-and-effect conclusions. With both kinds of randomness, probability models help us assess how much random variation we can expect in our results, in order to determine whether our results could happen by chance alone and to estimate a margin of error.
So where does this leave us with regard to the coffee study mentioned previously (the Freedman, Park, Abnet, Hollenbeck, & Sinha, 2012 found that men who drank at least six cups of coffee a day had a 10% lower chance of dying (women 15% lower) than those who drank none)? We can answer many of the questions:
- This was a 14-year study conducted by researchers at the National Cancer Institute.
- The results were published in the June issue of the New England Journal of Medicine , a respected, peer-reviewed journal.
- The study reviewed coffee habits of more than 402,000 people ages 50 to 71 from six states and two metropolitan areas. Those with cancer, heart disease, and stroke were excluded at the start of the study. Coffee consumption was assessed once at the start of the study.
- About 52,000 people died during the course of the study.
- People who drank between two and five cups of coffee daily showed a lower risk as well, but the amount of reduction increased for those drinking six or more cups.
- The sample sizes were fairly large and so the p-values are quite small, even though percent reduction in risk was not extremely large (dropping from a 12% chance to about 10%–11%).
- Whether coffee was caffeinated or decaffeinated did not appear to affect the results.
- This was an observational study, so no cause-and-effect conclusions can be drawn between coffee drinking and increased longevity, contrary to the impression conveyed by many news headlines about this study. In particular, it’s possible that those with chronic diseases don’t tend to drink coffee.
This study needs to be reviewed in the larger context of similar studies and consistency of results across studies, with the constant caution that this was not a randomized experiment. Whereas a statistical analysis can still “adjust” for other potential confounding variables, we are not yet convinced that researchers have identified them all or completely isolated why this decrease in death risk is evident. Researchers can now take the findings of this study and develop more focused studies that address new questions.
Explore these outside resources to learn more about applied statistics:
- Video about p-values: P-Value Extravaganza
- Interactive web applets for teaching and learning statistics
- Inter-university Consortium for Political and Social Research where you can find and analyze data.
- The Consortium for the Advancement of Undergraduate Statistics
- Find a recent research article in your field and answer the following: What was the primary research question? How were individuals selected to participate in the study? Were summary results provided? How strong is the evidence presented in favor or against the research question? Was random assignment used? Summarize the main conclusions from the study, addressing the issues of statistical significance, statistical confidence, generalizability, and cause and effect. Do you agree with the conclusions drawn from this study, based on the study design and the results presented?
- Is it reasonable to use a random sample of 1,000 individuals to draw conclusions about all U.S. adults? Explain why or why not.
How to Read Research
In this course and throughout your academic career, you’ll be reading journal articles (meaning they were published by experts in a peer-reviewed journal) and reports that explain psychological research. It’s important to understand the format of these articles so that you can read them strategically and understand the information presented. Scientific articles vary in content or structure, depending on the type of journal to which they will be submitted. Psychological articles and many papers in the social sciences follow the writing guidelines and format dictated by the American Psychological Association (APA). In general, the structure follows: abstract, introduction, methods, results, discussion, and references.
- Abstract : the abstract is the concise summary of the article. It summarizes the most important features of the manuscript, providing the reader with a global first impression on the article. It is generally just one paragraph that explains the experiment as well as a short synopsis of the results.
- Introduction : this section provides background information about the origin and purpose of performing the experiment or study. It reviews previous research and presents existing theories on the topic.
- Method : this section covers the methodologies used to investigate the research question, including the identification of participants , procedures , and materials as well as a description of the actual procedure . It should be sufficiently detailed to allow for replication.
- Results : the results section presents key findings of the research, including reference to indicators of statistical significance.
- Discussion : this section provides an interpretation of the findings, states their significance for current research, and derives implications for theory and practice. Alternative interpretations for findings are also provided, particularly when it is not possible to conclude for the directionality of the effects. In the discussion, authors also acknowledge the strengths and limitations/weaknesses of the study and offer concrete directions about for future research.
Watch this 3-minute video for an explanation on how to read scholarly articles. Look closely at the example article shared just before the two minute mark.
https://digitalcommons.coastal.edu/kimbel-library-instructional-videos/9/
Practice identifying these key components in the following experiment: Food-Induced Emotional Resonance Improves Emotion Recognition.
In this chapter, you learned to
- define and apply the scientific method to psychology
- describe the strengths and weaknesses of descriptive, experimental, and correlational research
- define the basic elements of a statistical investigation
Putting It Together: Psychological Research
Psychologists use the scientific method to examine human behavior and mental processes. Some of the methods you learned about include descriptive, experimental, and correlational research designs.
Watch the CrashCourse video to review the material you learned, then read through the following examples and see if you can come up with your own design for each type of study.
You can view the transcript for “Psychological Research: Crash Course Psychology #2” here (opens in new window).
Case Study: a detailed analysis of a particular person, group, business, event, etc. This approach is commonly used to to learn more about rare examples with the goal of describing that particular thing.
- Ted Bundy was one of America’s most notorious serial killers who murdered at least 30 women and was executed in 1989. Dr. Al Carlisle evaluated Bundy when he was first arrested and conducted a psychological analysis of Bundy’s development of his sexual fantasies merging into reality (Ramsland, 2012). Carlisle believes that there was a gradual evolution of three processes that guided his actions: fantasy, dissociation, and compartmentalization (Ramsland, 2012). Read Imagining Ted Bundy (http://goo.gl/rGqcUv) for more information on this case study.
Naturalistic Observation : a researcher unobtrusively collects information without the participant’s awareness.
- Drain and Engelhardt (2013) observed six nonverbal children with autism’s evoked and spontaneous communicative acts. Each of the children attended a school for children with autism and were in different classes. They were observed for 30 minutes of each school day. By observing these children without them knowing, they were able to see true communicative acts without any external influences.
Survey : participants are asked to provide information or responses to questions on a survey or structure assessment.
- Educational psychologists can ask students to report their grade point average and what, if anything, they eat for breakfast on an average day. A healthy breakfast has been associated with better academic performance (Digangi’s 1999).
- Anderson (1987) tried to find the relationship between uncomfortably hot temperatures and aggressive behavior, which was then looked at with two studies done on violent and nonviolent crime. Based on previous research that had been done by Anderson and Anderson (1984), it was predicted that violent crimes would be more prevalent during the hotter time of year and the years in which it was hotter weather in general. The study confirmed this prediction.
Longitudinal Study: researchers recruit a sample of participants and track them for an extended period of time.
- In a study of a representative sample of 856 children Eron and his colleagues (1972) found that a boy’s exposure to media violence at age eight was significantly related to his aggressive behavior ten years later, after he graduated from high school.
Cross-Sectional Study: researchers gather participants from different groups (commonly different ages) and look for differences between the groups.
- In 1996, Russell surveyed people of varying age groups and found that people in their 20s tend to report being more lonely than people in their 70s.
Correlational Design: two different variables are measured to determine whether there is a relationship between them.
- Thornhill et al. (2003) had people rate how physically attractive they found other people to be. They then had them separately smell t-shirts those people had worn (without knowing which clothes belonged to whom) and rate how good or bad their body oder was. They found that the more attractive someone was the more pleasant their body order was rated to be.
- Clinical psychologists can test a new pharmaceutical treatment for depression by giving some patients the new pill and others an already-tested one to see which is the more effective treatment.
American Cancer Society. (n.d.). History of the cancer prevention studies. Retrieved from http://www.cancer.org/research/researchtopreventcancer/history-cancer-prevention-study
American Psychological Association. (2009). Publication Manual of the American Psychological Association (6th ed.). Washington, DC: Author.
American Psychological Association. (n.d.). Research with animals in psychology. Retrieved from https://www.apa.org/research/responsible/research-animals.pdf
Arnett, J. (2008). The neglected 95%: Why American psychology needs to become less American. American Psychologist, 63(7), 602–614.
Barton, B. A., Eldridge, A. L., Thompson, D., Affenito, S. G., Striegel-Moore, R. H., Franko, D. L., . . . Crockett, S. J. (2005). The relationship of breakfast and cereal consumption to nutrient intake and body mass index: The national heart, lung, and blood institute growth and health study. Journal of the American Dietetic Association, 105(9), 1383–1389. Retrieved from http://dx.doi.org/10.1016/j.jada.2005.06.003
Chwalisz, K., Diener, E., & Gallagher, D. (1988). Autonomic arousal feedback and emotional experience: Evidence from the spinal cord injured. Journal of Personality and Social Psychology, 54, 820–828.
Dominus, S. (2011, May 25). Could conjoined twins share a mind? New York Times Sunday Magazine. Retrieved from http://www.nytimes.com/2011/05/29/magazine/could-conjoined-twins-share-a-mind.html?_r=5&hp&
Fanger, S. M., Frankel, L. A., & Hazen, N. (2012). Peer exclusion in preschool children’s play: Naturalistic observations in a playground setting. Merrill-Palmer Quarterly, 58, 224–254.
Fiedler, K. (2004). Illusory correlation. In R. F. Pohl (Ed.), Cognitive illusions: A handbook on fallacies and biases in thinking, judgment and memory (pp. 97–114). New York, NY: Psychology Press.
Frantzen, L. B., Treviño, R. P., Echon, R. M., Garcia-Dominic, O., & DiMarco, N. (2013). Association between frequency of ready-to-eat cereal consumption, nutrient intakes, and body mass index in fourth- to sixth-grade low-income minority children. Journal of the Academy of Nutrition and Dietetics, 113(4), 511–519.
Harper, J. (2013, July 5). Ice cream and crime: Where cold cuisine and hot disputes intersect. The Times-Picaune. Retrieved from http://www.nola.com/crime/index.ssf/2013/07/ice_cream_and_crime_where_hot.html
Jenkins, W. J., Ruppel, S. E., Kizer, J. B., Yehl, J. L., & Griffin, J. L. (2012). An examination of post 9-11 attitudes towards Arab Americans. North American Journal of Psychology, 14, 77–84.
Jones, J. M. (2013, May 13). Same-sex marriage support solidifies above 50% in U.S. Gallup Politics. Retrieved from http://www.gallup.com/poll/162398/sex-marriage-support-solidifies-above.aspx
Kobrin, J. L., Patterson, B. F., Shaw, E. J., Mattern, K. D., & Barbuti, S. M. (2008). Validity of the SAT for predicting first-year college grade point average (Research Report No. 2008-5). Retrieved from https://research.collegeboard.org/sites/default/files/publications/2012/7/researchreport-2008-5-validity-sat-predicting-first-year-college-grade-point-average.pdf
Lewin, T. (2014, March 5). A new SAT aims to realign with schoolwork. New York Times. Retreived from http://www.nytimes.com/2014/03/06/education/major-changes-in-sat-announced-by-college-board.html.
Lowry, M., Dean, K., & Manders, K. (2010). The link between sleep quantity and academic performance for the college student. Sentience: The University of Minnesota Undergraduate Journal of Psychology, 3(Spring), 16–19. Retrieved from http://www.psych.umn.edu/sentience/files/SENTIENCE_Vol3.pdf
McKie, R. (2010, June 26). Chimps with everything: Jane Goodall’s 50 years in the jungle. The Guardian. Retrieved from http://www.theguardian.com/science/2010/jun/27/jane-goodall-chimps-africa-interview
Offit, P. (2008). Autism’s false prophets: Bad science, risky medicine, and the search for a cure. New York: Columbia University Press.
Perkins, H. W., Haines, M. P., & Rice, R. (2005). Misperceiving the college drinking norm and related problems: A nationwide study of exposure to prevention information, perceived norms and student alcohol misuse. J. Stud. Alcohol, 66(4), 470–478.
Rimer, S. (2008, September 21). College panel calls for less focus on SATs. The New York Times. Retrieved from http://www.nytimes.com/2008/09/22/education/22admissions.html?_r=0
Rothstein, J. M. (2004). College performance predictions and the SAT. Journal of Econometrics, 121, 297–317.
Rotton, J., & Kelly, I. W. (1985). Much ado about the full moon: A meta-analysis of lunar-lunacy research. Psychological Bulletin, 97(2), 286–306. doi:10.1037/0033-2909.97.2.286
Santelices, M. V., & Wilson, M. (2010). Unfair treatment? The case of Freedle, the SAT, and the standardization approach to differential item functioning. Harvard Education Review, 80, 106–134.
Sears, D. O. (1986). College sophomores in the laboratory: Influences of a narrow data base on social psychology’s view of human nature. Journal of Personality and Social Psychology, 51, 515–530.
Tuskegee University. (n.d.). About the USPHS Syphilis Study. Retrieved from http://www.tuskegee.edu/about_us/centers_of_excellence/bioethics_center/about_the_usphs_syphilis_study.aspx.
CC licensed content, Original
- Psychological Research Methods. Provided by : Karenna Malavanti. License : CC BY-SA: Attribution ShareAlike
CC licensed content, Shared previously
- Psychological Research. Provided by : OpenStax College. License : CC BY: Attribution . License Terms : Download for free at https://openstax.org/books/psychology-2e/pages/1-introduction. Located at : https://openstax.org/books/psychology-2e/pages/2-introduction .
- Why It Matters: Psychological Research. Provided by : Lumen Learning. License : CC BY: Attribution Located at: https://pressbooks.online.ucf.edu/lumenpsychology/chapter/introduction-15/
- Introduction to The Scientific Method. Provided by : Lumen Learning. License : CC BY: Attribution Located at: https://pressbooks.online.ucf.edu/lumenpsychology/chapter/outcome-the-scientific-method/
- Research picture. Authored by : Mediterranean Center of Medical Sciences. Provided by : Flickr. License : CC BY: Attribution Located at : https://www.flickr.com/photos/mcmscience/17664002728 .
- The Scientific Process. Provided by : Lumen Learning. License : CC BY-SA: Attribution ShareAlike Located at: https://pressbooks.online.ucf.edu/lumenpsychology/chapter/reading-the-scientific-process/
- Ethics in Research. Provided by : Lumen Learning. License : CC BY: Attribution Located at: https://pressbooks.online.ucf.edu/lumenpsychology/chapter/ethics/
- Ethics. Authored by : OpenStax College. Located at : https://openstax.org/books/psychology-2e/pages/2-4-ethics . License : CC BY: Attribution . License Terms : Download for free at https://openstax.org/books/psychology-2e/pages/1-introduction .
- Introduction to Approaches to Research. Provided by : Lumen Learning. License : CC BY-NC-SA: Attribution NonCommercial ShareAlike Located at: https://pressbooks.online.ucf.edu/lumenpsychology/chapter/outcome-approaches-to-research/
- Lec 2 | MIT 9.00SC Introduction to Psychology, Spring 2011. Authored by : John Gabrieli. Provided by : MIT OpenCourseWare. License : CC BY-NC-SA: Attribution-NonCommercial-ShareAlike Located at : https://www.youtube.com/watch?v=syXplPKQb_o .
- Paragraph on correlation. Authored by : Christie Napa Scollon. Provided by : Singapore Management University. License : CC BY-NC-SA: Attribution-NonCommercial-ShareAlike Located at : http://nobaproject.com/modules/research-designs?r=MTc0ODYsMjMzNjQ%3D . Project : The Noba Project.
- Descriptive Research. Provided by : Lumen Learning. License : CC BY-SA: Attribution ShareAlike Located at: https://pressbooks.online.ucf.edu/lumenpsychology/chapter/reading-clinical-or-case-studies/
- Approaches to Research. Authored by : OpenStax College. License : CC BY: Attribution . License Terms : Download for free at https://openstax.org/books/psychology-2e/pages/1-introduction. Located at : https://openstax.org/books/psychology-2e/pages/2-2-approaches-to-research
- Analyzing Findings. Authored by : OpenStax College. Located at : https://openstax.org/books/psychology-2e/pages/2-3-analyzing-findings . License : CC BY: Attribution . License Terms : Download for free at https://openstax.org/books/psychology-2e/pages/1-introduction.
- Experiments. Provided by : Lumen Learning. License : CC BY: Attribution Located at: https://pressbooks.online.ucf.edu/lumenpsychology/chapter/reading-conducting-experiments/
- Research Review. Authored by : Jessica Traylor for Lumen Learning. License : CC BY: Attribution Located at: https://pressbooks.online.ucf.edu/lumenpsychology/chapter/reading-conducting-experiments/
- Introduction to Statistics. Provided by : Lumen Learning. License : CC BY: Attribution Located at: https://pressbooks.online.ucf.edu/lumenpsychology/chapter/outcome-statistical-thinking/
- histogram. Authored by : Fisher’s Iris flower data set. Provided by : Wikipedia.
- License : CC BY-SA: Attribution-ShareAlike Located at : https://en.wikipedia.org/wiki/Wikipedia:Meetup/DC/Statistics_Edit-a-thon#/media/File:Fisher_iris_versicolor_sepalwidth.svg .
- Statistical Thinking. Authored by : Beth Chance and Allan Rossman . Provided by : California Polytechnic State University, San Luis Obispo.
- License : CC BY-NC-SA: Attribution-NonCommerci al-S hareAlike . License Terms : http://nobaproject.com/license-agreement Located at : http://nobaproject.com/modules/statistical-thinking . Project : The Noba Project.
- Drawing Conclusions from Statistics. Authored by: Pat Carroll and Lumen Learning. Provided by : Lumen Learning. License : CC BY: Attribution Located at: https://pressbooks.online.ucf.edu/lumenpsychology/chapter/reading-drawing-conclusions-from-statistics/
- Statistical Thinking. Authored by : Beth Chance and Allan Rossman, California Polytechnic State University, San Luis Obispo. Provided by : Noba. License: CC BY-NC-SA: Attribution-NonCommercial-ShareAlike Located at : http://nobaproject.com/modules/statistical-thinking .
- The Replication Crisis. Authored by : Colin Thomas William. Provided by : Ivy Tech Community College. License: CC BY: Attribution
- How to Read Research. Provided by : Lumen Learning. License : CC BY: Attribution Located at: https://pressbooks.online.ucf.edu/lumenpsychology/chapter/how-to-read-research/
- What is a Scholarly Article? Kimbel Library First Year Experience Instructional Videos. 9. Authored by: Joshua Vossler, John Watts, and Tim Hodge. Provided by : Coastal Carolina University License : CC BY NC ND: Attribution-NonCommercial-NoDerivatives Located at : https://digitalcommons.coastal.edu/kimbel-library-instructional-videos/9/
- Putting It Together: Psychological Research. Provided by : Lumen Learning. License : CC BY: Attribution Located at: https://pressbooks.online.ucf.edu/lumenpsychology/chapter/putting-it-together-psychological-research/
- Research. Provided by : Lumen Learning. License : CC BY: Attribution Located at:
All rights reserved content
- Understanding Driver Distraction. Provided by : American Psychological Association. License : Other. License Terms: Standard YouTube License Located at : https://www.youtube.com/watch?v=XToWVxS_9lA&list=PLxf85IzktYWJ9MrXwt5GGX3W-16XgrwPW&index=9 .
- Correlation vs. Causality: Freakonomics Movie. License : Other. License Terms : Standard YouTube License Located at : https://www.youtube.com/watch?v=lbODqslc4Tg.
- Psychological Research – Crash Course Psychology #2. Authored by : Hank Green. Provided by : Crash Course. License : Other. License Terms : Standard YouTube License Located at : https://www.youtube.com/watch?v=hFV71QPvX2I .
Public domain content
- Researchers review documents. Authored by : National Cancer Institute. Provided by : Wikimedia. Located at : https://commons.wikimedia.org/wiki/File:Researchers_review_documents.jpg . License : Public Domain: No Known Copyright
grounded in objective, tangible evidence that can be observed time and time again, regardless of who is observing
well-developed set of ideas that propose an explanation for observed phenomena
(plural: hypotheses) tentative and testable statement about the relationship between two or more variables
an experiment must be replicable by another researcher
implies that a theory should enable us to make predictions about future events
able to be disproven by experimental results
implies that all data must be considered when evaluating a hypothesis
committee of administrators, scientists, and community members that reviews proposals for research involving human participants
process of informing a research participant about what to expect during an experiment, any risks involved, and the implications of the research, and then obtaining the person’s consent to participate
purposely misleading experiment participants in order to maintain the integrity of the experiment
when an experiment involved deception, participants are told complete and truthful information about the experiment at its conclusion
committee of administrators, scientists, veterinarians, and community members that reviews proposals for research involving non-human animals
research studies that do not test specific relationships between variables
research investigating the relationship between two or more variables
research method that uses hypothesis testing to make inferences about how one variable impacts and causes another
observation of behavior in its natural setting
inferring that the results for a sample apply to the larger population
when observations may be skewed to align with observer expectations
measure of agreement among observers on how they record and classify a particular event
observational research study focusing on one or a few people
list of questions to be answered by research participants—given as paper-and-pencil questionnaires, administered electronically, or conducted verbally—allowing researchers to collect data from a large number of people
subset of individuals selected from the larger population
overall group of individuals that the researchers are interested in
method of research using past records or data sets to answer various research questions, or to search for interesting patterns or relationships
studies in which the same group of individuals is surveyed or measured repeatedly over an extended period of time
compares multiple segments of a population at a single time
reduction in number of research participants as some drop out of the study over time
relationship between two or more variables; when two variables are correlated, one variable changes as the other does
number from -1 to +1, indicating the strength and direction of the relationship between variables, and usually represented by r
two variables change in the same direction, both becoming either larger or smaller
two variables change in different directions, with one becoming larger as the other becomes smaller; a negative correlation is not the same thing as no correlation
changes in one variable cause the changes in the other variable; can be determined only through an experimental research design
unanticipated outside factor that affects both variables of interest, often giving the false impression that changes in one variable causes changes in the other variable, when, in actuality, the outside factor causes changes in both variables
seeing relationships between two things when in reality no such relationship exists
tendency to ignore evidence that disproves ideas or beliefs
group designed to answer the research question; experimental manipulation is the only difference between the experimental and control groups, so any differences between the two are due to experimental manipulation rather than chance
serves as a basis for comparison and controls for chance factors that might influence the results of the study—by holding such factors constant across groups so that the experimental manipulation is the only difference between groups
description of what actions and operations will be used to measure the dependent variables and manipulate the independent variables
researcher expectations skew the results of the study
experiment in which the researcher knows which participants are in the experimental group and which are in the control group
experiment in which both the researchers and the participants are blind to group assignments
people's expectations or beliefs influencing or determining their experience in a given situation
variable that is influenced or controlled by the experimenter; in a sound experimental study, the independent variable is the only important difference between the experimental and control group
variable that the researcher measures to see how much effect the independent variable had
subjects of psychological research
subset of a larger population in which every member of the population has an equal chance of being selected
method of experimental group assignment in which all participants have an equal chance of being assigned to either group
consistency and reproducibility of a given result
accuracy of a given result in measuring what it is designed to measure
determines how likely any difference between experimental groups is due to chance
statistical probability that represents the likelihood that experimental results happened by chance
Psychological Science is the scientific study of mind, brain, and behavior. We will explore what it means to be human in this class. It has never been more important for us to understand what makes people tick, how to evaluate information critically, and the importance of history. Psychology can also help you in your future career; indeed, there are very little jobs out there with no human interaction!
Because psychology is a science, we analyze human behavior through the scientific method. There are several ways to investigate human phenomena, such as observation, experiments, and more. We will discuss the basics, pros and cons of each! We will also dig deeper into the important ethical guidelines that psychologists must follow in order to do research. Lastly, we will briefly introduce ourselves to statistics, the language of scientific research. While reading the content in these chapters, try to find examples of material that can fit with the themes of the course.
To get us started:
- The study of the mind moved away Introspection to reaction time studies as we learned more about empiricism
- Psychologists work in careers outside of the typical "clinician" role. We advise in human factors, education, policy, and more!
- While completing an observation study, psychologists will work to aggregate common themes to explain the behavior of the group (sample) as a whole. In doing so, we still allow for normal variation from the group!
- The IRB and IACUC are important in ensuring ethics are maintained for both human and animal subjects
Psychological Science: Understanding Human Behavior Copyright © by Karenna Malavanti is licensed under a Creative Commons Attribution-NonCommercial-ShareAlike 4.0 International License , except where otherwise noted.
Share This Book
Using Science to Inform Educational Practices
Experimental Research
As you’ve learned, the only way to establish that there is a cause-and-effect relationship between two variables is to conduct a scientific experiment. Experiment has a different meaning in the scientific context than in everyday life. In everyday conversation, we often use it to describe trying something for the first time, such as experimenting with a new hairstyle or new food. However, in the scientific context, an experiment has precise requirements for design and implementation.
Video 2.8.1. Experimental Research Design provides explanation and examples for correlational research. A closed-captioned version of this video is available here .
The Experimental Hypothesis
In order to conduct an experiment, a researcher must have a specific hypothesis to be tested. As you’ve learned, hypotheses can be formulated either through direct observation of the real world or after careful review of previous research. For example, if you think that children should not be allowed to watch violent programming on television because doing so would cause them to behave more violently, then you have basically formulated a hypothesis—namely, that watching violent television programs causes children to behave more violently. How might you have arrived at this particular hypothesis? You may have younger relatives who watch cartoons featuring characters using martial arts to save the world from evildoers, with an impressive array of punching, kicking, and defensive postures. You notice that after watching these programs for a while, your young relatives mimic the fighting behavior of the characters portrayed in the cartoon. Seeing behavior like this right after a child watches violent television programming might lead you to hypothesize that viewing violent television programming leads to an increase in the display of violent behaviors. These sorts of personal observations are what often lead us to formulate a specific hypothesis, but we cannot use limited personal observations and anecdotal evidence to test our hypothesis rigorously. Instead, to find out if real-world data supports our hypothesis, we have to conduct an experiment.
Designing an Experiment
The most basic experimental design involves two groups: the experimental group and the control group. The two groups are designed to be the same except for one difference— experimental manipulation. The experimental group gets the experimental manipulation—that is, the treatment or variable being tested (in this case, violent TV images)—and the control group does not. Since experimental manipulation is the only difference between the experimental and control groups, we can be sure that any differences between the two are due to experimental manipulation rather than chance.
In our example of how violent television programming might affect violent behavior in children, we have the experimental group view violent television programming for a specified time and then measure their violent behavior. We measure the violent behavior in our control group after they watch nonviolent television programming for the same amount of time. It is important for the control group to be treated similarly to the experimental group, with the exception that the control group does not receive the experimental manipulation. Therefore, we have the control group watch non-violent television programming for the same amount of time as the experimental group.
We also need to define precisely, or operationalize, what is considered violent and nonviolent. An operational definition is a description of how we will measure our variables, and it is important in allowing others to understand exactly how and what a researcher measures in a particular experiment. In operationalizing violent behavior, we might choose to count only physical acts like kicking or punching as instances of this behavior, or we also may choose to include angry verbal exchanges. Whatever we determine, it is important that we operationalize violent behavior in such a way that anyone who hears about our study for the first time knows exactly what we mean by violence. This aids peoples’ ability to interpret our data as well as their capacity to repeat our experiment should they choose to do so.
Once we have operationalized what is considered violent television programming and what is considered violent behavior from our experiment participants, we need to establish how we will run our experiment. In this case, we might have participants watch a 30-minute television program (either violent or nonviolent, depending on their group membership) before sending them out to a playground for an hour where their behavior is observed and the number and type of violent acts are recorded.
Ideally, the people who observe and record the children’s behavior are unaware of who was assigned to the experimental or control group, in order to control for experimenter bias. Experimenter bias refers to the possibility that a researcher’s expectations might skew the results of the study. Remember, conducting an experiment requires a lot of planning, and the people involved in the research project have a vested interest in supporting their hypotheses. If the observers knew which child was in which group, it might influence how much attention they paid to each child’s behavior as well as how they interpreted that behavior. By being blind to which child is in which group, we protect against those biases. This situation is a single-blind study , meaning that the participants are unaware as to which group they are in (experiment or control group) while the researcher knows which participants are in each group.
In a double-blind study , both the researchers and the participants are blind to group assignments. Why would a researcher want to run a study where no one knows who is in which group? Because by doing so, we can control for both experimenter and participant expectations. If you are familiar with the phrase placebo effect , you already have some idea as to why this is an important consideration. The placebo effect occurs when people’s expectations or beliefs influence or determine their experience in a given situation. In other words, simply expecting something to happen can actually make it happen.
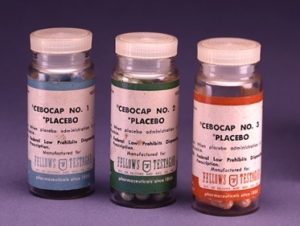
Why is that? Imagine that you are a participant in this study, and you have just taken a pill that you think will improve your mood. Because you expect the pill to have an effect, you might feel better simply because you took the pill and not because of any drug actually contained in the pill—this is the placebo effect.
To make sure that any effects on mood are due to the drug and not due to expectations, the control group receives a placebo (in this case, a sugar pill). Now everyone gets a pill, and once again, neither the researcher nor the experimental participants know who got the drug and who got the sugar pill. Any differences in mood between the experimental and control groups can now be attributed to the drug itself rather than to experimenter bias or participant expectations.
Video 2.8.2. Introduction to Experimental Design introduces fundamental elements for experimental research design.
Independent and Dependent Variables
In a research experiment, we strive to study whether changes in one thing cause changes in another. To achieve this, we must pay attention to two important variables, or things that can be changed, in any experimental study: the independent variable and the dependent variable. An independent variable is manipulated or controlled by the experimenter. In a well-designed experimental study, the independent variable is the only important difference between the experimental and control groups. In our example of how violent television programs affect children’s display of violent behavior, the independent variable is the type of program—violent or nonviolent—viewed by participants in the study (Figure 2.3). A dependent variable is what the researcher measures to see how much effect the independent variable had. In our example, the dependent variable is the number of violent acts displayed by the experimental participants.
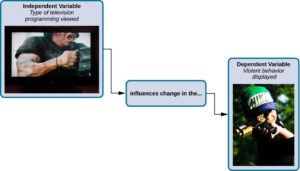
Figure 2.8.1. In an experiment, manipulations of the independent variable are expected to result in changes in the dependent variable.
We expect that the dependent variable will change as a function of the independent variable. In other words, the dependent variable depends on the independent variable. A good way to think about the relationship between the independent and dependent variables is with this question: What effect does the independent variable have on the dependent variable? Returning to our example, what effect does watching a half-hour of violent television programming or nonviolent television programming have on the number of incidents of physical aggression displayed on the playground?
Selecting and Assigning Experimental Participants
Now that our study is designed, we need to obtain a sample of individuals to include in our experiment. Our study involves human participants, so we need to determine who to include. Participants are the subjects of psychological research, and as the name implies, individuals who are involved in psychological research actively participate in the process. Often, psychological research projects rely on college students to serve as participants. In fact, the vast majority of research in psychology subfields has historically involved students as research participants (Sears, 1986; Arnett, 2008). But are college students truly representative of the general population? College students tend to be younger, more educated, more liberal, and less diverse than the general population. Although using students as test subjects is an accepted practice, relying on such a limited pool of research participants can be problematic because it is difficult to generalize findings to the larger population.
Our hypothetical experiment involves children, and we must first generate a sample of child participants. Samples are used because populations are usually too large to reasonably involve every member in our particular experiment (Figure 2.4). If possible, we should use a random sample (there are other types of samples, but for the purposes of this chapter, we will focus on random samples). A random sample is a subset of a larger population in which every member of the population has an equal chance of being selected. Random samples are preferred because if the sample is large enough we can be reasonably sure that the participating individuals are representative of the larger population. This means that the percentages of characteristics in the sample—sex, ethnicity, socioeconomic level, and any other characteristics that might affect the results—are close to those percentages in the larger population.
In our example, let’s say we decide our population of interest is fourth graders. But all fourth graders is a very large population, so we need to be more specific; instead, we might say our population of interest is all fourth graders in a particular city. We should include students from various income brackets, family situations, races, ethnicities, religions, and geographic areas of town. With this more manageable population, we can work with the local schools in selecting a random sample of around 200 fourth-graders that we want to participate in our experiment.
In summary, because we cannot test all of the fourth graders in a city, we want to find a group of about 200 that reflects the composition of that city. With a representative group, we can generalize our findings to the larger population without fear of our sample being biased in some way.
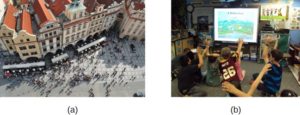
Figure 2.8.2. Researchers may work with (a) a large population or (b) a sample group that is a subset of the larger population.
Now that we have a sample, the next step of the experimental process is to split the participants into experimental and control groups through random assignment. With random assignment , all participants have an equal chance of being assigned to either group. There is statistical software that will randomly assign each of the fourth graders in the sample to either the experimental or the control group.
Random assignment is critical for sound experimental design. With sufficiently large samples, random assignment makes it unlikely that there are systematic differences between the groups. So, for instance, it would be improbable that we would get one group composed entirely of males, a given ethnic identity, or a given religious ideology. This is important because if the groups were systematically different before the experiment began, we would not know the origin of any differences we find between the groups: Were the differences preexisting, or were they caused by manipulation of the independent variable? Random assignment allows us to assume that any differences observed between experimental and control groups result from the manipulation of the independent variable.
Exercise 2.2 Randomization in Sampling and Assignment
Use this online tool to generate randomized numbers instantly and to learn more about random sampling and assignments.
Issues to Consider
While experiments allow scientists to make cause-and-effect claims, they are not without problems. True experiments require the experimenter to manipulate an independent variable, and that can complicate many questions that psychologists might want to address. For instance, imagine that you want to know what effect sex (the independent variable) has on spatial memory (the dependent variable). Although you can certainly look for differences between males and females on a task that taps into spatial memory, you cannot directly control a person’s sex. We categorize this type of research approach as quasi-experimental and recognize that we cannot make cause-and-effect claims in these circumstances.
Experimenters are also limited by ethical constraints. For instance, you would not be able to conduct an experiment designed to determine if experiencing abuse as a child leads to lower levels of self-esteem among adults. To conduct such an experiment, you would need to randomly assign some experimental participants to a group that receives abuse, and that experiment would be unethical.
Interpreting Experimental Findings
Once data is collected from both the experimental and the control groups, a statistical analysis is conducted to find out if there are meaningful differences between the two groups. The statistical analysis determines how likely any difference found is due to chance (and thus not meaningful). In psychology, group differences are considered meaningful, or significant, if the odds that these differences occurred by chance alone are 5 percent or less. Stated another way, if we repeated this experiment 100 times, we would expect to find the same results at least 95 times out of 100.
The greatest strength of experiments is the ability to assert that any significant differences in the findings are caused by the independent variable. This occurs because of random selection, random assignment, and a design that limits the effects of both experimenter bias and participant expectancy should create groups that are similar in composition and treatment. Therefore, any difference between the groups is attributable to the independent variable, and now we can finally make a causal statement. If we find that watching a violent television program results in more violent behavior than watching a nonviolent program, we can safely say that watching violent television programs causes an increase in the display of violent behavior.
Candela Citations
- Experimental Research. Authored by : Nicole Arduini-Van Hoose. Provided by : Hudson Valley Community College. Retrieved from : https://courses.lumenlearning.com/edpsy/chapter/experimental-research/. License : CC BY-NC-SA: Attribution-NonCommercial-ShareAlike
- Experimental Research. Authored by : Nicole Arduini-Van Hoose. Provided by : Hudson Valley Community College. Retrieved from : https://courses.lumenlearning.com/adolescent/chapter/experimental-research/. Project : https://courses.lumenlearning.com/adolescent/chapter/experimental-research/. License : CC BY-NC-SA: Attribution-NonCommercial-ShareAlike
Educational Psychology Copyright © 2020 by Nicole Arduini-Van Hoose is licensed under a Creative Commons Attribution-NonCommercial-ShareAlike 4.0 International License , except where otherwise noted.
Share This Book

Want to create or adapt books like this? Learn more about how Pressbooks supports open publishing practices.
Experimental Research
Learning Objectives
- Explain the difference between between-subjects and within-subjects experiments, list some of the pros and cons of each approach, and decide which approach to use to answer a particular research question.
- Define random assignment, distinguish it from random sampling, explain its purpose in experimental research, and use some simple strategies to implement it
- Define several types of carryover effect, give examples of each, and explain how counterbalancing helps to deal with them.
In this section, we look at some different ways to design an experiment. The primary distinction we will make is between approaches in which each participant experiences one level of the independent variable and approaches in which each participant experiences all levels of the independent variable. The former are called between-subjects experiments and the latter are called within-subjects experiments.
Between-Subjects Experiments
In a between-subjects experiment , each participant is tested in only one condition. For example, a researcher with a sample of 100 university students might assign half of them to write about a traumatic event and the other half write about a neutral event. Or a researcher with a sample of 60 people with severe agoraphobia (fear of open spaces) might assign 20 of them to receive each of three different treatments for that disorder. It is essential in a between-subjects experiment that the researcher assigns participants to conditions so that the different groups are, on average, highly similar to each other. Those in a trauma condition and a neutral condition, for example, should include a similar proportion of men and women, and they should have similar average IQs, similar average levels of motivation, similar average numbers of health problems, and so on. This matching is a matter of controlling these extraneous participant variables across conditions so that they do not become confounding variables.
Random Assignment
The primary way that researchers accomplish this kind of control of extraneous variables across conditions is called random assignment , which means using a random process to decide which participants are tested in which conditions. Do not confuse random assignment with random sampling. Random sampling is a method for selecting a sample from a population, and it is rarely used in psychological research. Random assignment is a method for assigning participants in a sample to the different conditions, and it is an important element of all experimental research in psychology and other fields too.
In its strictest sense, random assignment should meet two criteria. One is that each participant has an equal chance of being assigned to each condition (e.g., a 50% chance of being assigned to each of two conditions). The second is that each participant is assigned to a condition independently of other participants. Thus one way to assign participants to two conditions would be to flip a coin for each one. If the coin lands heads, the participant is assigned to Condition A, and if it lands tails, the participant is assigned to Condition B. For three conditions, one could use a computer to generate a random integer from 1 to 3 for each participant. If the integer is 1, the participant is assigned to Condition A; if it is 2, the participant is assigned to Condition B; and if it is 3, the participant is assigned to Condition C. In practice, a full sequence of conditions—one for each participant expected to be in the experiment—is usually created ahead of time, and each new participant is assigned to the next condition in the sequence as they are tested. When the procedure is computerized, the computer program often handles the random assignment.
One problem with coin flipping and other strict procedures for random assignment is that they are likely to result in unequal sample sizes in the different conditions. Unequal sample sizes are generally not a serious problem, and you should never throw away data you have already collected to achieve equal sample sizes. However, for a fixed number of participants, it is statistically most efficient to divide them into equal-sized groups. It is standard practice, therefore, to use a kind of modified random assignment that keeps the number of participants in each group as similar as possible. One approach is block randomization . In block randomization, all the conditions occur once in the sequence before any of them is repeated. Then they all occur again before any of them is repeated again. Within each of these “blocks,” the conditions occur in a random order. Again, the sequence of conditions is usually generated before any participants are tested, and each new participant is assigned to the next condition in the sequence. Table 5.2 shows such a sequence for assigning nine participants to three conditions. The Research Randomizer website ( http://www.randomizer.org ) will generate block randomization sequences for any number of participants and conditions. Again, when the procedure is computerized, the computer program often handles the block randomization.
Random assignment is not guaranteed to control all extraneous variables across conditions. The process is random, so it is always possible that just by chance, the participants in one condition might turn out to be substantially older, less tired, more motivated, or less depressed on average than the participants in another condition. However, there are some reasons that this possibility is not a major concern. One is that random assignment works better than one might expect, especially for large samples. Another is that the inferential statistics that researchers use to decide whether a difference between groups reflects a difference in the population takes the “fallibility” of random assignment into account. Yet another reason is that even if random assignment does result in a confounding variable and therefore produces misleading results, this confound is likely to be detected when the experiment is replicated. The upshot is that random assignment to conditions—although not infallible in terms of controlling extraneous variables—is always considered a strength of a research design.
Matched Groups
An alternative to simple random assignment of participants to conditions is the use of a matched-groups design . Using this design, participants in the various conditions are matched on the dependent variable or on some extraneous variable(s) prior the manipulation of the independent variable. This guarantees that these variables will not be confounded across the experimental conditions. For instance, if we want to determine whether expressive writing affects people’s health then we could start by measuring various health-related variables in our prospective research participants. We could then use that information to rank-order participants according to how healthy or unhealthy they are. Next, the two healthiest participants would be randomly assigned to complete different conditions (one would be randomly assigned to the traumatic experiences writing condition and the other to the neutral writing condition). The next two healthiest participants would then be randomly assigned to complete different conditions, and so on until the two least healthy participants. This method would ensure that participants in the traumatic experiences writing condition are matched to participants in the neutral writing condition with respect to health at the beginning of the study. If at the end of the experiment, a difference in health was detected across the two conditions, then we would know that it is due to the writing manipulation and not to pre-existing differences in health.
Within-Subjects Experiments
In a within-subjects experiment , each participant is tested under all conditions. Consider an experiment on the effect of a defendant’s physical attractiveness on judgments of his guilt. Again, in a between-subjects experiment, one group of participants would be shown an attractive defendant and asked to judge his guilt, and another group of participants would be shown an unattractive defendant and asked to judge his guilt. In a within-subjects experiment, however, the same group of participants would judge the guilt of both an attractive and an unattractive defendant.
The primary advantage of this approach is that it provides maximum control of extraneous participant variables. Participants in all conditions have the same mean IQ, same socioeconomic status, same number of siblings, and so on—because they are the very same people. Within-subjects experiments also make it possible to use statistical procedures that remove the effect of these extraneous participant variables on the dependent variable and therefore make the data less “noisy” and the effect of the independent variable easier to detect. We will look more closely at this idea later in the book . However, not all experiments can use a within-subjects design nor would it be desirable to do so.
Carryover Effects and Counterbalancing
The primary disadvantage of within-subjects designs is that they can result in order effects. An order effect occurs when participants’ responses in the various conditions are affected by the order of conditions to which they were exposed. One type of order effect is a carryover effect. A carryover effect is an effect of being tested in one condition on participants’ behavior in later conditions. One type of carryover effect is a practice effect , where participants perform a task better in later conditions because they have had a chance to practice it. Another type is a fatigue effect , where participants perform a task worse in later conditions because they become tired or bored. Being tested in one condition can also change how participants perceive stimuli or interpret their task in later conditions. This type of effect is called a context effect (or contrast effect) . For example, an average-looking defendant might be judged more harshly when participants have just judged an attractive defendant than when they have just judged an unattractive defendant. Within-subjects experiments also make it easier for participants to guess the hypothesis. For example, a participant who is asked to judge the guilt of an attractive defendant and then is asked to judge the guilt of an unattractive defendant is likely to guess that the hypothesis is that defendant attractiveness affects judgments of guilt. This knowledge could lead the participant to judge the unattractive defendant more harshly because he thinks this is what he is expected to do. Or it could make participants judge the two defendants similarly in an effort to be “fair.”
Carryover effects can be interesting in their own right. (Does the attractiveness of one person depend on the attractiveness of other people that we have seen recently?) But when they are not the focus of the research, carryover effects can be problematic. Imagine, for example, that participants judge the guilt of an attractive defendant and then judge the guilt of an unattractive defendant. If they judge the unattractive defendant more harshly, this might be because of his unattractiveness. But it could be instead that they judge him more harshly because they are becoming bored or tired. In other words, the order of the conditions is a confounding variable. The attractive condition is always the first condition and the unattractive condition the second. Thus any difference between the conditions in terms of the dependent variable could be caused by the order of the conditions and not the independent variable itself.
There is a solution to the problem of order effects, however, that can be used in many situations. It is counterbalancing , which means testing different participants in different orders. The best method of counterbalancing is complete counterbalancing in which an equal number of participants complete each possible order of conditions. For example, half of the participants would be tested in the attractive defendant condition followed by the unattractive defendant condition, and others half would be tested in the unattractive condition followed by the attractive condition. With three conditions, there would be six different orders (ABC, ACB, BAC, BCA, CAB, and CBA), so some participants would be tested in each of the six orders. With four conditions, there would be 24 different orders; with five conditions there would be 120 possible orders. With counterbalancing, participants are assigned to orders randomly, using the techniques we have already discussed. Thus, random assignment plays an important role in within-subjects designs just as in between-subjects designs. Here, instead of randomly assigning to conditions, they are randomly assigned to different orders of conditions. In fact, it can safely be said that if a study does not involve random assignment in one form or another, it is not an experiment.
A more efficient way of counterbalancing is through a Latin square design which randomizes through having equal rows and columns. For example, if you have four treatments, you must have four versions. Like a Sudoku puzzle, no treatment can repeat in a row or column. For four versions of four treatments, the Latin square design would look like:
You can see in the diagram above that the square has been constructed to ensure that each condition appears at each ordinal position (A appears first once, second once, third once, and fourth once) and each condition precedes and follows each other condition one time. A Latin square for an experiment with 6 conditions would by 6 x 6 in dimension, one for an experiment with 8 conditions would be 8 x 8 in dimension, and so on. So while complete counterbalancing of 6 conditions would require 720 orders, a Latin square would only require 6 orders.
Finally, when the number of conditions is large experiments can use random counterbalancing in which the order of the conditions is randomly determined for each participant. Using this technique every possible order of conditions is determined and then one of these orders is randomly selected for each participant. This is not as powerful a technique as complete counterbalancing or partial counterbalancing using a Latin squares design. Use of random counterbalancing will result in more random error, but if order effects are likely to be small and the number of conditions is large, this is an option available to researchers.
There are two ways to think about what counterbalancing accomplishes. One is that it controls the order of conditions so that it is no longer a confounding variable. Instead of the attractive condition always being first and the unattractive condition always being second, the attractive condition comes first for some participants and second for others. Likewise, the unattractive condition comes first for some participants and second for others. Thus any overall difference in the dependent variable between the two conditions cannot have been caused by the order of conditions. A second way to think about what counterbalancing accomplishes is that if there are carryover effects, it makes it possible to detect them. One can analyze the data separately for each order to see whether it had an effect.
When 9 Is “Larger” Than 221
Researcher Michael Birnbaum has argued that the lack of context provided by between-subjects designs is often a bigger problem than the context effects created by within-subjects designs. To demonstrate this problem, he asked participants to rate two numbers on how large they were on a scale of 1-to-10 where 1 was “very very small” and 10 was “very very large”. One group of participants were asked to rate the number 9 and another group was asked to rate the number 221 (Birnbaum, 1999) [1] . Participants in this between-subjects design gave the number 9 a mean rating of 5.13 and the number 221 a mean rating of 3.10. In other words, they rated 9 as larger than 221! According to Birnbaum, this difference is because participants spontaneously compared 9 with other one-digit numbers (in which case it is relatively large) and compared 221 with other three-digit numbers (in which case it is relatively small).
Simultaneous Within-Subjects Designs
So far, we have discussed an approach to within-subjects designs in which participants are tested in one condition at a time. There is another approach, however, that is often used when participants make multiple responses in each condition. Imagine, for example, that participants judge the guilt of 10 attractive defendants and 10 unattractive defendants. Instead of having people make judgments about all 10 defendants of one type followed by all 10 defendants of the other type, the researcher could present all 20 defendants in a sequence that mixed the two types. The researcher could then compute each participant’s mean rating for each type of defendant. Or imagine an experiment designed to see whether people with social anxiety disorder remember negative adjectives (e.g., “stupid,” “incompetent”) better than positive ones (e.g., “happy,” “productive”). The researcher could have participants study a single list that includes both kinds of words and then have them try to recall as many words as possible. The researcher could then count the number of each type of word that was recalled.
Between-Subjects or Within-Subjects?
Almost every experiment can be conducted using either a between-subjects design or a within-subjects design. This possibility means that researchers must choose between the two approaches based on their relative merits for the particular situation.
Between-subjects experiments have the advantage of being conceptually simpler and requiring less testing time per participant. They also avoid carryover effects without the need for counterbalancing. Within-subjects experiments have the advantage of controlling extraneous participant variables, which generally reduces noise in the data and makes it easier to detect any effect of the independent variable upon the dependent variable. Within-subjects experiments also require fewer participants than between-subjects experiments to detect an effect of the same size.
A good rule of thumb, then, is that if it is possible to conduct a within-subjects experiment (with proper counterbalancing) in the time that is available per participant—and you have no serious concerns about carryover effects—this design is probably the best option. If a within-subjects design would be difficult or impossible to carry out, then you should consider a between-subjects design instead. For example, if you were testing participants in a doctor’s waiting room or shoppers in line at a grocery store, you might not have enough time to test each participant in all conditions and therefore would opt for a between-subjects design. Or imagine you were trying to reduce people’s level of prejudice by having them interact with someone of another race. A within-subjects design with counterbalancing would require testing some participants in the treatment condition first and then in a control condition. But if the treatment works and reduces people’s level of prejudice, then they would no longer be suitable for testing in the control condition. This difficulty is true for many designs that involve a treatment meant to produce long-term change in participants’ behavior (e.g., studies testing the effectiveness of psychotherapy). Clearly, a between-subjects design would be necessary here.
Remember also that using one type of design does not preclude using the other type in a different study. There is no reason that a researcher could not use both a between-subjects design and a within-subjects design to answer the same research question. In fact, professional researchers often take exactly this type of mixed methods approach.
- Birnbaum, M.H. (1999). How to show that 9>221: Collect judgments in a between-subjects design. Psychological Methods, 4 (3), 243-249. ↵
An experiment in which each participant is tested in only one condition.
Means using a random process to decide which participants are tested in which conditions.
All the conditions occur once in the sequence before any of them is repeated.
An experiment design in which the participants in the various conditions are matched on the dependent variable or on some extraneous variable(s) prior the manipulation of the independent variable.
An experiment in which each participant is tested under all conditions.
An effect that occurs when participants' responses in the various conditions are affected by the order of conditions to which they were exposed.
An effect of being tested in one condition on participants’ behavior in later conditions.
An effect where participants perform a task better in later conditions because they have had a chance to practice it.
An effect where participants perform a task worse in later conditions because they become tired or bored.
Unintended influences on respondents’ answers because they are not related to the content of the item but to the context in which the item appears.
Varying the order of the conditions in which participants are tested, to help solve the problem of order effects in within-subjects experiments.
A method in which an equal number of participants complete each possible order of conditions.
A method in which the order of the conditions is randomly determined for each participant.
Research Methods in Psychology Copyright © 2019 by Rajiv S. Jhangiani, I-Chant A. Chiang, Carrie Cuttler, & Dana C. Leighton is licensed under a Creative Commons Attribution-NonCommercial-ShareAlike 4.0 International License , except where otherwise noted.
Share This Book
Research Hypothesis In Psychology: Types, & Examples
Saul Mcleod, PhD
Editor-in-Chief for Simply Psychology
BSc (Hons) Psychology, MRes, PhD, University of Manchester
Saul Mcleod, PhD., is a qualified psychology teacher with over 18 years of experience in further and higher education. He has been published in peer-reviewed journals, including the Journal of Clinical Psychology.
Learn about our Editorial Process
Olivia Guy-Evans, MSc
Associate Editor for Simply Psychology
BSc (Hons) Psychology, MSc Psychology of Education
Olivia Guy-Evans is a writer and associate editor for Simply Psychology. She has previously worked in healthcare and educational sectors.
On This Page:
A research hypothesis, in its plural form “hypotheses,” is a specific, testable prediction about the anticipated results of a study, established at its outset. It is a key component of the scientific method .
Hypotheses connect theory to data and guide the research process towards expanding scientific understanding
Some key points about hypotheses:
- A hypothesis expresses an expected pattern or relationship. It connects the variables under investigation.
- It is stated in clear, precise terms before any data collection or analysis occurs. This makes the hypothesis testable.
- A hypothesis must be falsifiable. It should be possible, even if unlikely in practice, to collect data that disconfirms rather than supports the hypothesis.
- Hypotheses guide research. Scientists design studies to explicitly evaluate hypotheses about how nature works.
- For a hypothesis to be valid, it must be testable against empirical evidence. The evidence can then confirm or disprove the testable predictions.
- Hypotheses are informed by background knowledge and observation, but go beyond what is already known to propose an explanation of how or why something occurs.
Predictions typically arise from a thorough knowledge of the research literature, curiosity about real-world problems or implications, and integrating this to advance theory. They build on existing literature while providing new insight.
Types of Research Hypotheses
Alternative hypothesis.
The research hypothesis is often called the alternative or experimental hypothesis in experimental research.
It typically suggests a potential relationship between two key variables: the independent variable, which the researcher manipulates, and the dependent variable, which is measured based on those changes.
The alternative hypothesis states a relationship exists between the two variables being studied (one variable affects the other).
A hypothesis is a testable statement or prediction about the relationship between two or more variables. It is a key component of the scientific method. Some key points about hypotheses:
- Important hypotheses lead to predictions that can be tested empirically. The evidence can then confirm or disprove the testable predictions.
In summary, a hypothesis is a precise, testable statement of what researchers expect to happen in a study and why. Hypotheses connect theory to data and guide the research process towards expanding scientific understanding.
An experimental hypothesis predicts what change(s) will occur in the dependent variable when the independent variable is manipulated.
It states that the results are not due to chance and are significant in supporting the theory being investigated.
The alternative hypothesis can be directional, indicating a specific direction of the effect, or non-directional, suggesting a difference without specifying its nature. It’s what researchers aim to support or demonstrate through their study.
Null Hypothesis
The null hypothesis states no relationship exists between the two variables being studied (one variable does not affect the other). There will be no changes in the dependent variable due to manipulating the independent variable.
It states results are due to chance and are not significant in supporting the idea being investigated.
The null hypothesis, positing no effect or relationship, is a foundational contrast to the research hypothesis in scientific inquiry. It establishes a baseline for statistical testing, promoting objectivity by initiating research from a neutral stance.
Many statistical methods are tailored to test the null hypothesis, determining the likelihood of observed results if no true effect exists.
This dual-hypothesis approach provides clarity, ensuring that research intentions are explicit, and fosters consistency across scientific studies, enhancing the standardization and interpretability of research outcomes.
Nondirectional Hypothesis
A non-directional hypothesis, also known as a two-tailed hypothesis, predicts that there is a difference or relationship between two variables but does not specify the direction of this relationship.
It merely indicates that a change or effect will occur without predicting which group will have higher or lower values.
For example, “There is a difference in performance between Group A and Group B” is a non-directional hypothesis.
Directional Hypothesis
A directional (one-tailed) hypothesis predicts the nature of the effect of the independent variable on the dependent variable. It predicts in which direction the change will take place. (i.e., greater, smaller, less, more)
It specifies whether one variable is greater, lesser, or different from another, rather than just indicating that there’s a difference without specifying its nature.
For example, “Exercise increases weight loss” is a directional hypothesis.
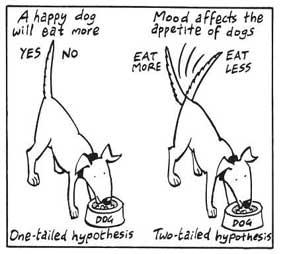
Falsifiability
The Falsification Principle, proposed by Karl Popper , is a way of demarcating science from non-science. It suggests that for a theory or hypothesis to be considered scientific, it must be testable and irrefutable.
Falsifiability emphasizes that scientific claims shouldn’t just be confirmable but should also have the potential to be proven wrong.
It means that there should exist some potential evidence or experiment that could prove the proposition false.
However many confirming instances exist for a theory, it only takes one counter observation to falsify it. For example, the hypothesis that “all swans are white,” can be falsified by observing a black swan.
For Popper, science should attempt to disprove a theory rather than attempt to continually provide evidence to support a research hypothesis.
Can a Hypothesis be Proven?
Hypotheses make probabilistic predictions. They state the expected outcome if a particular relationship exists. However, a study result supporting a hypothesis does not definitively prove it is true.
All studies have limitations. There may be unknown confounding factors or issues that limit the certainty of conclusions. Additional studies may yield different results.
In science, hypotheses can realistically only be supported with some degree of confidence, not proven. The process of science is to incrementally accumulate evidence for and against hypothesized relationships in an ongoing pursuit of better models and explanations that best fit the empirical data. But hypotheses remain open to revision and rejection if that is where the evidence leads.
- Disproving a hypothesis is definitive. Solid disconfirmatory evidence will falsify a hypothesis and require altering or discarding it based on the evidence.
- However, confirming evidence is always open to revision. Other explanations may account for the same results, and additional or contradictory evidence may emerge over time.
We can never 100% prove the alternative hypothesis. Instead, we see if we can disprove, or reject the null hypothesis.
If we reject the null hypothesis, this doesn’t mean that our alternative hypothesis is correct but does support the alternative/experimental hypothesis.
Upon analysis of the results, an alternative hypothesis can be rejected or supported, but it can never be proven to be correct. We must avoid any reference to results proving a theory as this implies 100% certainty, and there is always a chance that evidence may exist which could refute a theory.
How to Write a Hypothesis
- Identify variables . The researcher manipulates the independent variable and the dependent variable is the measured outcome.
- Operationalized the variables being investigated . Operationalization of a hypothesis refers to the process of making the variables physically measurable or testable, e.g. if you are about to study aggression, you might count the number of punches given by participants.
- Decide on a direction for your prediction . If there is evidence in the literature to support a specific effect of the independent variable on the dependent variable, write a directional (one-tailed) hypothesis. If there are limited or ambiguous findings in the literature regarding the effect of the independent variable on the dependent variable, write a non-directional (two-tailed) hypothesis.
- Make it Testable : Ensure your hypothesis can be tested through experimentation or observation. It should be possible to prove it false (principle of falsifiability).
- Clear & concise language . A strong hypothesis is concise (typically one to two sentences long), and formulated using clear and straightforward language, ensuring it’s easily understood and testable.
Consider a hypothesis many teachers might subscribe to: students work better on Monday morning than on Friday afternoon (IV=Day, DV= Standard of work).
Now, if we decide to study this by giving the same group of students a lesson on a Monday morning and a Friday afternoon and then measuring their immediate recall of the material covered in each session, we would end up with the following:
- The alternative hypothesis states that students will recall significantly more information on a Monday morning than on a Friday afternoon.
- The null hypothesis states that there will be no significant difference in the amount recalled on a Monday morning compared to a Friday afternoon. Any difference will be due to chance or confounding factors.
More Examples
- Memory : Participants exposed to classical music during study sessions will recall more items from a list than those who studied in silence.
- Social Psychology : Individuals who frequently engage in social media use will report higher levels of perceived social isolation compared to those who use it infrequently.
- Developmental Psychology : Children who engage in regular imaginative play have better problem-solving skills than those who don’t.
- Clinical Psychology : Cognitive-behavioral therapy will be more effective in reducing symptoms of anxiety over a 6-month period compared to traditional talk therapy.
- Cognitive Psychology : Individuals who multitask between various electronic devices will have shorter attention spans on focused tasks than those who single-task.
- Health Psychology : Patients who practice mindfulness meditation will experience lower levels of chronic pain compared to those who don’t meditate.
- Organizational Psychology : Employees in open-plan offices will report higher levels of stress than those in private offices.
- Behavioral Psychology : Rats rewarded with food after pressing a lever will press it more frequently than rats who receive no reward.

- MSc/MRes Psychological Research Methods
Psychological Research Methods MRes / MSc
- Level(s) of Study: Postgraduate taught / Postgraduate research
- Start Date(s): September 2025
- Duration: One year full-time / two years part-time
- Study Mode(s): Full-time / Part-time
- Campus: City Campus
- Entry Requirements: More information
Find us on:
- Connect through Twitter
Introduction:
Our Psychological Research Methods Masters degree provides extensive training if you are looking to develop a broad range of transferable skills relevant to independent research and beyond. The course has been designed specifically to prepare graduates in Psychology or related disciplines for:
- a PhD degree
- research related careers in academic or applied psychological disciplines
- careers in which data handling and analytical skills are of relevance.
You might also complete the course as a means of improving your research skills in preparation for further training as a professional psychologist (such as a clinical or educational psychologist).
Both awards are equivalent, although the MSc has a larger taught component whilst the MRes places greater emphasis on the conduct of independent research. You may wish to apply to the MSc in Psychological Research Methods in the first instance and then you can make a choice of award later, in conjunction with the Course Leader and teaching team.
What you’ll study
*We are currently reviewing the content of our courses to ensure that they remain relevant and current to out students’ future ambitions and society. Please continue to check this course webpage for the latest developments.
Choosing your route
The MSc emphasises a taught focus across a range of methods and will be suitable for students keen to develop a broad profile of independent research skills.
The MRes places a greater emphasis on independent study and is suitable for students who are already engaged in psychological research and wish to focus on a particular area.
The innovative course structure means you don't need to decide which award you want to focus on until the end of Term One.
In Term One, ALL full-time MSc and MRes students complete the same core modules. Part-time students take the same module in Term One but spread them across two years. Current modules include:
Advanced Statistics One
This module examines the theoretical and philosophical underpinnings of statistics used in experimental research (e.g., statistical inference, power and effect size). The framework for the module is a regression / GLM approach to statistics that focuses on the relationship between multiple linear regression, ANOVA and ANCOVA. The module also covers the application of these concepts in widely available computer software such as R. These include the relationship between different experimental designs (e.g., factorial designs, multi-stage sampling, RCTs, cross-sectional designs, longitudinal designs or single-case studies) and statistical issues such as power and generalizability. Practical issues such as dealing with violations of statistical assumptions or missing data are also considered.
Psychometrics One: Developing Psychometric Scales in Research and Practice
This module will provide you with a basic knowledge of psychometric theory and how this theory can be applied to the different stages of test development. More specifically, the module aims to demonstrate the process of test development through practical application of theory, whereby you will work to produce your own psychometric scale.
Qualitative Research Design and Analysis One
Qualitative research is concerned with the subjective world and aims to investigate human experience in order to understand peoples’ opinions, motivations and feelings. As you explore this fascinating topic, qualitative research is not just one method but a constellation of designs and methods, each offering a unique perspective on a chosen research topic. You will learn how to evaluate qualitative research, as well as how to manage, handle, report and present the qualitative data you are working with.
Observational Methods
This module equips you with a knowledge of, and practical skills in, observational methods. You will work with other students on a collective observational analysis of video-taped material to build up your skills so that you will graduate from the module competent to use structured observational methods in your own research studies.
Psychological Experiments: Tools and Methodologies
The module provides you with a better understanding of experimental design and methodology in Psychology. You will gain practical skills in building experiments using specialist software to run them and collect data. You will also gain a better understanding and practical experience of the how to generate or manipulate stimuli for your experimental needs. You will also explore open science in Psychology and issues of replicability. Many of these skills will be practical and transferable in other areas.
In Term Two, students pursuing the MSc award will complete five more taught modules and an empirical research project (worth 60 credits), under the supervision of a research active member of staff. MRes students complete either two 10 credits taught modules of their choice, or one 20 credit taught module of their choice, allowing them to concentrate exclusively on quantitative, psychometric methods or qualitative methods. MRes students complete a larger empirical research project (worth 100 credits) under the supervision of a research active member of staff.
Advanced Statistics Two
Advanced Statistics Two uses the regression framework adopted in Advanced Statistics One and introduces additional advanced statistical topics such as logistic regression, Poisson regression, meta-analysis and multilevel modelling. The module builds on practical topics introduced in Advanced Experimentation and Statistics (One) such as dealing with violations of assumptions and the limitations of standard research designs for real world data (e.g., handling unbalanced or missing data in repeated measures analyses).The module is taught using R.
Psychometrics Two: Using Psychometric Scales in Research and Practice
Psychometrics Two builds on the understanding and skills you developed in Psychometrics One, advancing them to considers how scales are used in research and practice. It also considers other forms of psychometrics beyond just surveys and questionnaires and you will tackle a range of problem based scenarios drawn from real world situations.
Qualitative Research Design and Analysis Two
This module will provide you with both the theoretical underpinnings and analytic practice of conversation analysis (CA), membership categorisation (MCA), and discursive psychology (DP). The module includes lectures on theory / method combined with seminars looking at data and perform analysis.
Structural Equation Modelling (SEM)
On this module, you will be introduced to the theoretical and philosophical underpinnings of structural equation modelling (SEM) and equipped with the skills, and understanding, to appropriately construct, analyse, and interpret theoretical path analytic CFA, and SEM models.
Technological Tools in Psychology
In this module, you'll be introduced to some of the technologically informed tools (e.g., EEG, bio-packs, eye-tracking, TMS) and associated methods of contemporary psychology. This module will provide you with practical hands-on experience of a range of cutting-edge psychological research tools and the data they produce. You'll also learn about and critically consider a range of issues in and around the theory, methodology, and application of these tools and approaches.
Psychology Research Project
Your project is an independent piece of work that is supervised by a member of staff who will guide and support you as you work towards producing a final report for assessment. This will be the main opportunity for you to carry out an extensive piece of independent research of your own choosing and for you to demonstrate that you have the skills to devise, conduct, analyse, present and report an empirical study to postgraduate level.
Don’t just take our word for it, hear from our students themselves
Student profiles, harriet broadbent.
The support offered by NTU is second to none in my opinion and the responses from previous postgraduate students were what really stood out when deciding to stay at NTU.
No results were found
Video Gallery
