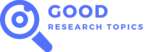
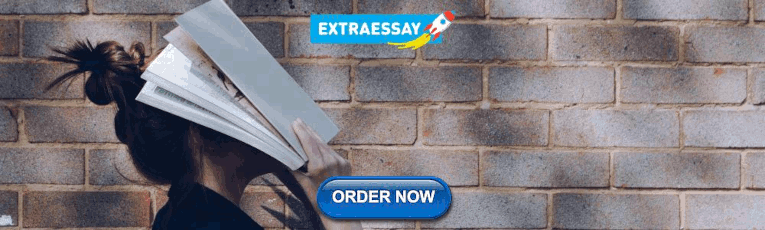
181+ Great PhD Research Topics In Artificial Intelligence [2024 Updated]
Are you curious about how smart machines learn and solve problems like humans? That’s what “PhD Research Topics in Artificial Intelligence” explore! Suppose teaching computers to think, learn, and make decisions just like us. These research topics are like treasure maps for scientists who want to discover new ways for machines to understand language, predict diseases, drive cars, and so much more!
In simple words, Artificial Intelligence (AI) is about making computers super smart. These PhD topics cover various fields like healthcare, finance, education, and even space! Scientists are like detectives, trying to find ways for AI to help people live better lives and solve big problems like predicting the weather or creating new medicines.
For example, robots help doctors, predict when accidents might happen on the road, or even explore space without humans! These PhD research topics are like a magical door to a world where machines can do incredible things to make our world safer, healthier, and more amazing. So, let’s take a peek into these topics and see the exciting possibilities AI offers to our future!
Also Like To Know: Top 10 Research Topics for Senior High School Students in 2024
Table of Contents
What Is PhD Research Topics In Artificial Intelligence?
PhD research topics in Artificial Intelligence (AI) refer to specific areas of study and investigation that scholars explore to advance the understanding and development of smart machines. These topics delve into various aspects of AI, aiming to solve complex problems and create innovative solutions using computer systems. Researchers in AI Ph.D. programs investigate diverse fields such as healthcare, finance, robotics, and more, seeking to improve how machines learn, reason, and make decisions.
These topics serve as pathways for scholars to conduct in-depth studies, discover new AI techniques, and apply them to real-world challenges. In essence, Ph.D. research topics in Artificial Intelligence represent the quests scientists undertake to expand AI capabilities, making machines smarter and more proficient in mimicking human intelligence to benefit society in diverse ways.
How Can I Choose Good PhD Research Topics In Artificial Intelligence?
Choosing a good PhD research topic in Artificial Intelligence (AI) involves careful consideration and planning. Here are five steps to help you navigate this process effectively:
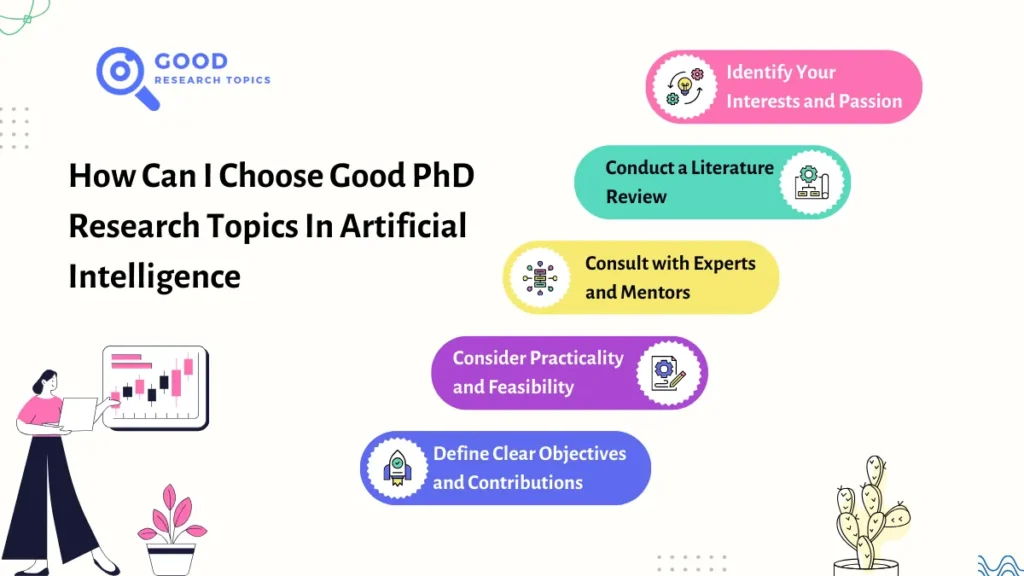
1. Identify Your Interests and Passion
Start by exploring your own interests within the broad field of AI. Reflect on the areas of AI that fascinate you the most—whether it’s machine learning, natural language processing, robotics, healthcare applications, ethics in AI, or any other niche. Choose a topic that aligns with your passion and strengths, as you’ll be dedicating several years to this research.
2. Conduct a Literature Review
Familiarize yourself with the existing research landscape in AI. Look into recent publications, conferences, and journals in the field. This step will help you understand the current trends, gaps, and potential research areas where you can make a meaningful contribution. Consider areas with emerging technologies or unresolved challenges that pique your interest.
3. Consult with Experts and Mentors
Engage with professors, AI professionals, or mentors in the field. Discuss your research interests and seek their guidance. They can provide valuable insights, suggest potential research directions, and help you refine your ideas. Their expertise can assist you in identifying relevant and impactful research topics.
4. Consider Practicality and Feasibility
Evaluate the feasibility of your research topics. Consider the availability of resources, data, and tools required for your proposed research. Ensure that your chosen topic is realistic within the timeframe and constraints of a PhD program. Assess whether you have access to necessary datasets, computing resources, and mentorship.
5. Define Clear Objectives and Contributions
Narrow down your topic and define clear research objectives. Your research should aim to contribute something novel to the field. Consider how your work could fill a gap in current knowledge, propose a solution to an existing problem, or introduce a new perspective. Ensure that your research questions are well-defined and achievable within the scope of a Ph.D.
List of 181+ Great PhD Research Topics In Artificial Intelligence
Here are the most interesting and great PhD Research Topics In Artificial Intelligenc.
Healthcare AI Research Topics
- Personalized Healthcare Plans Using AI-Based Predictive Models
- Ethical Considerations in AI-Based Medical Diagnosis and Treatment
- Robust and Interpretable AI Models for Medical Image Analysis
- Drug Repurposing and Discovery through AI-Driven Approaches
- AI-Powered Remote Patient Monitoring Systems
- Explainable AI for Clinical Decision Support Systems
- AI for Mental Health Diagnosis and Monitoring
- AI-Enhanced Prosthetics and Rehabilitation Systems
- Predictive Models for Epidemic Outbreaks using AI and Machine Learning
- AI in Genomic Medicine: Enhancing Precision Medicine Approaches
Finance Related PhD Research Topics In Artificial Intelligence
- Time Series Forecasting in Financial Markets using AI
- AI-Driven Algorithmic Trading Strategies
- Fraud Detection and Prevention using Machine Learning
- Explainable AI Models for Credit Scoring and Risk Assessment
- AI-Powered Regulatory Compliance in Financial Institutions
- Predictive Analytics for Asset Management and Portfolio Optimization
- AI-Based Robo-Advisors for Personal Finance Management
- Cryptocurrency Price Prediction using Machine Learning
- Reinforcement Learning Models for Financial Decision-Making
- Ethical Implications of AI in Finance: Bias and Fairness Issues
Education AI Research Topics
- Personalized Learning Paths through AI-Based Adaptive Educational Systems
- Automated Essay Scoring using Machine Learning Techniques
- Intelligent Tutoring Systems: AI-Driven Educational Assistance
- AI-Powered Content Creation for Educational Purposes
- AI-Enhanced Tools for Teachers to Improve Classroom Engagement
- Evaluating AI’s Impact on Learning Outcomes and Student Performance
- Gamification in Education: AI Techniques for Enhanced Learning
- Automated Plagiarism Detection and Prevention using AI
- AI-Enabled Accessibility Tools for Inclusive Education
- Ethical Considerations in AI-Driven Education: Data Privacy and Security
Environment and Sustainability PhD Research Topics In Artificial Intelligence
- Climate Change Prediction Models using AI and Big Data Analytics
- AI-Optimized Renewable Energy Systems
- AI-Driven Solutions for Wildlife Conservation and Monitoring
- Smart Grids and Energy Distribution Management using AI
- Environmental Impact Assessment with AI-Based Tools
- AI Applications for Sustainable Agriculture and Food Security
- AI-Based Natural Disaster Prediction and Response Systems
- Green Computing: AI for Energy-Efficient Systems
- Urban Planning and Management through AI and IoT Integration
- Ethical Use of AI in Environmental Conservation and Sustainability
Transportation PhD Research Topics In Artificial Intelligence
- Autonomous Vehicle Navigation and Decision-Making using AI
- Traffic Congestion Management through AI-Optimized Routing Systems
- AI-Based Predictive Maintenance for Transportation Infrastructure
- AI Solutions for Public Transportation Efficiency and Optimization
- Ethics of AI in Transportation: Safety and Liability Concerns
- Smart Traffic Lights and Intersection Management using AI
- Drone Technology and AI for Last-Mile Delivery Systems
- Human-Centric AI Systems for Transportation Accessibility
- AI in Aviation: Optimizing Air Traffic Control and Safety
- AI-Driven Solutions for Sustainable Transportation Planning
Robotics AI Research Topics
- Human-Robot Interaction: Developing Socially Intelligent Robots
- Autonomous Robot Swarms for Collaborative Tasks using AI
- AI-Powered Surgical Robotics: Precision and Safety in Operations
- AI-Enabled Industrial Robotics for Automation and Efficiency
- Explainable AI for Robotics: Transparency in Decision-Making
- Ethical Considerations in Autonomous Robotics: Morality and Responsibility
- AI-Driven Rehabilitation Robotics for Physical Therapy
- AI Applications in Soft Robotics and Biomechanics
- AI and Wearable Robotics: Enhancing Human Capabilities
- AI in Space Exploration: Robotics for Extraterrestrial Missions
Social Sciences PhD Research Topics In Artificial Intelligence
- Sentiment Analysis and Opinion Mining in Social Media using AI
- Predictive Models for Crime Prevention and Law Enforcement
- AI-Based Solutions for Mental Health Support and Counseling
- Cultural Heritage Preservation through AI and Digital Archives
- Ethical Implications of AI in Social Sciences: Bias and Discrimination
- AI-Powered Solutions for Disaster Relief and Humanitarian Aid
- AI and Behavioral Economics: Understanding Human Decision-Making
- AI for Social Network Analysis and Community Detection
- Personalized Social Services using AI: Challenges and Opportunities
- AI-Driven Solutions for Societal Equity and Inclusivity
Manufacturing and Industry AI Research Topics
- Predictive Maintenance and Quality Control in Manufacturing using AI
- AI-Enhanced Supply Chain Optimization and Inventory Management
- Robotics and AI Integration in Smart Factories (Industry 4.0)
- AI for Predictive Analytics in Equipment Failure Prevention
- Ethical Use of AI in Industrial Settings: Worker Safety and Rights
- AI-Powered Optimization of Production Processes
- Cybersecurity in AI-Driven Manufacturing Systems
- Human-Machine Collaboration in Manufacturing: Challenges and Benefits
- AI Applications for Waste Reduction and Sustainable Production
- AI and Additive Manufacturing: Innovations in 3D Printing
Entertainment and Media PhD Research Topics In Artificial Intelligence
- AI-Generated Content Creation in Entertainment Industry
- Recommendation Systems and Personalization in Media Streaming Platforms
- AI-Based Music Composition and Generation
- AI-Powered Gaming: Enhancing User Experience and Realism
- Sentiment Analysis in Movie Reviews and Entertainment Industry
- Ethical Concerns in AI-Generated Media: Authenticity and Ownership
- AI-Driven Storytelling and Narrative Generation
- Virtual Reality and AI: Immersive Media Experiences
- AI in Journalism: Automated News Writing and Fact-Checking
- AI-Enabled Creativity Tools for Content Producers
Law and Governance AI Research Topics
- Legal Document Analysis and Case Law Prediction using AI
- AI-Driven Contract Analysis and Management
- Predictive Models for Legal Decision-Making and Case Outcomes
- Ethics and Bias in AI-Based Judicial Systems
- AI Applications in Legislative Drafting and Policy Formulation
- AI-Enhanced Regulatory Compliance and Governance
- AI for Public Administration and Service Delivery
- Privacy and Security Concerns in AI-Driven Legal Systems
- AI and Human Rights: Ensuring Fairness and Justice
- AI and Electoral Systems: Transparency and Accountability
Agriculture Related PhD Research Topics In Artificial Intelligence
- Precision Agriculture using AI for Crop Monitoring and Yield Prediction
- AI-Based Pest and Disease Detection in Agriculture
- Autonomous Farming: AI-Driven Farm Equipment and Robotics
- Soil Quality Assessment and Management with AI
- AI for Sustainable Water Management in Agriculture
- Ethical Considerations in AI Applications in Agriculture
- AI-Optimized Greenhouse Farming and Controlled Environment Agriculture
- Climate Change Adaptation in Agriculture using AI
- AI-Driven Livestock Monitoring and Health Management
- Smart Farming: IoT and AI Integration in Agricultural Systems
Space and Astronomy AI Research Topics
- AI Applications in Space Exploration and Satellite Systems
- Autonomous Space Probes and Rovers: AI-Driven Missions
- AI for Astronomical Data Analysis and Discovery
- Space Debris Monitoring and Management with AI
- Ethics in AI-Assisted Space Exploration: Environmental Impact
- AI-Enhanced Space Communication and Navigation
- Predictive Models for Space Weather using AI
- AI-Driven Exoplanet Discovery and Characterization
- Autonomous Space Traffic Management with AI
- Robotic Assembly and Maintenance in Space using AI
Psychology and Cognitive Sciences Related PhD Research Topics In Artificial Intelligence
- AI-Driven Cognitive Models and Mental Health Diagnostics
- Ethical Implications of AI in Psychological Studies
- AI-Powered Therapeutic Interventions and Virtual Counseling
- Computational Models of Human Decision-Making with AI
- AI-Based Assistive Technologies for Cognitive Disabilities
- Neuroinformatics and AI: Analyzing Brain Imaging Data
- AI in Behavioral Psychology: Simulating Human Behavior
- Ethical Considerations in AI-Driven Behavioral Studies
- AI and Emotional Intelligence: Developing Emotionally Intelligent Systems
- Personalized Mental Health Care using AI-Driven Approaches
Linguistics and Language AI Research Topics
- Natural Language Understanding and Generation with AI
- AI-Driven Machine Translation and Multilingual Communication
- Sentiment Analysis in Multilingual Texts using AI
- Ethics of AI-Powered Language Models: Bias and Misinformation
- AI-Enhanced Language Learning and Teaching Systems
- AI-Based Phonetics and Speech Recognition Systems
- Cross-Linguistic Analysis and Typology with AI
- Language Evolution Simulation using AI Models
- AI-Enabled Computational Linguistics: Syntax and Semantics
- Multimodal Communication with AI: Text, Speech, and Images
Good PhD Research Topics In Artificial Intelligence In Quantum Computing
- Quantum Machine Learning Algorithms and Applications
- Hybrid Quantum-Classical Models in AI
- Quantum Computing for Optimization Problems in AI
- Quantum Neural Networks and Quantum Data Encoding
- Ethical Considerations in Quantum AI: Privacy and Security
- AI-Driven Quantum Error Correction and Noise Mitigation
- Quantum AI in Cryptography and Secure Communication
- Quantum Computing Simulations for AI Model Training
- Quantum-Inspired Algorithms for AI Applications
- Quantum-Safe AI Systems: Resistant to Future Attacks
Ethics and Philosophy AI Research Topics
- Ethical Frameworks and Guidelines for AI Development
- Explainable AI for Transparent and Interpretable Decision-Making
- Bias and Fairness in AI Algorithms and Decision Systems
- AI and Moral Reasoning: Developing Ethical Machines
- Accountability and Responsibility in AI: Legal and Ethical Perspectives
- AI and Human Rights: Ensuring Dignity and Equality
- Ethical Considerations in AI Research and Experimentation
- AI and Consciousness: Ethical Implications of Sentience
- Trustworthiness and Reliability of AI Systems
- Robustness and Safety in AI: Minimizing Risks and Harm
Cool Cybersecurity PhD Research Topics In Artificial Intelligence
- AI-Enhanced Threat Detection and Intrusion Prevention
- Ethical Hacking and Vulnerability Assessment using AI
- AI-Driven Cyberattack Prediction and Prevention
- Explainable AI for Security Analytics and Incident Response
- AI for Anomaly Detection in Network Traffic
- Secure AI Model Training and Data Privacy Preservation
- AI-Powered Authentication and Access Control Systems
- Adversarial Attacks and Defenses in AI Systems
- AI-Based Security Operations Center (SOC) Automation
- Quantum Computing Threats to AI Security: Mitigation Strategies
Human-Computer Interaction AI Research Topics
- AI-Enabled Personalization and User Modeling
- AI-Based Adaptive User Interfaces and Experiences
- Ethical Design of AI-Driven Human-Computer Interfaces
- AI in Augmented Reality (AR) and Virtual Reality (VR)
- Natural Language Interaction with AI Systems
- AI-Driven Emotion Recognition for User Engagement
- Accessibility and Inclusivity in AI-Enhanced Interfaces
- AI-Powered Recommender Systems for User Preferences
- Ethical Considerations in Persuasive AI Design
- Multimodal Interfaces: Integrating Speech, Vision, and Gesture with AI
General PhD Research Topics In Artificial Intelligence
- Continual Learning and Lifelong Adaptation in AI Systems
- Cognitive Architectures and Human-Like AI Models
- Multi-Agent Systems: Collaboration and Coordination among Intelligent Agents
- Federated Learning: Privacy-Preserving Machine Learning
- AI in Decision Support Systems and Autonomous Agents
- AI for Social Good: Addressing Societal Challenges
- AI-Driven Innovation and Creativity: Art, Music, and Literature Generation
- Quantum Machine Learning: AI Algorithms for Quantum Computing
- AI Governance and Regulation: Policy Frameworks and Standards
- Ethical AI Leadership and Responsible Innovation
Consequently, the world of Artificial Intelligence (AI) offers a vast array of exciting opportunities for PhD research. These PhD Research Topics In Artificial Intelligence serve as gateways to unlocking the potential of intelligent machines, spanning fields such as healthcare, finance, robotics, education, and more. As aspiring researchers delve into these areas, they embark on a journey to expand the boundaries of AI, seeking solutions to complex problems and envisioning innovative applications that can transform our lives.
Choosing a PhD research topic in AI involves a thoughtful process of discovery, where interests, expertise, feasibility, and impact converge. Researchers must strive to select topics that resonate with their passions, align with current trends and challenges, and have the potential to make meaningful contributions to the field.
By immersing themselves in the rich tapestry of AI research, scholars pave the way for advancements that can revolutionize industries, drive societal progress, and shape the future of technology. As they embark on this academic voyage, researchers in AI PhD programs become pioneers, pushing the boundaries of knowledge and innovation to create a world where intelligent machines work hand in hand with humanity, making remarkable strides toward a brighter, smarter, and more inclusive future.
Which Topic Is Best For Artificial Intelligence?
The best topic for Artificial Intelligence depends on your specific interests, expertise, and the potential for meaningful contributions in that particular area.
How Is AI Used In PhD Research?
AI is utilized in PhD research to enhance data analysis, model complex systems, automate tasks, and develop innovative solutions across various fields.
What Can You Do With A PhD In Artificial Intelligence?
With a PhD in artificial intelligence, you can lead groundbreaking AI research, develop innovative technologies, teach at universities, lead AI teams in industry, or contribute to policy-making and ethical guidelines in AI.
Related Posts
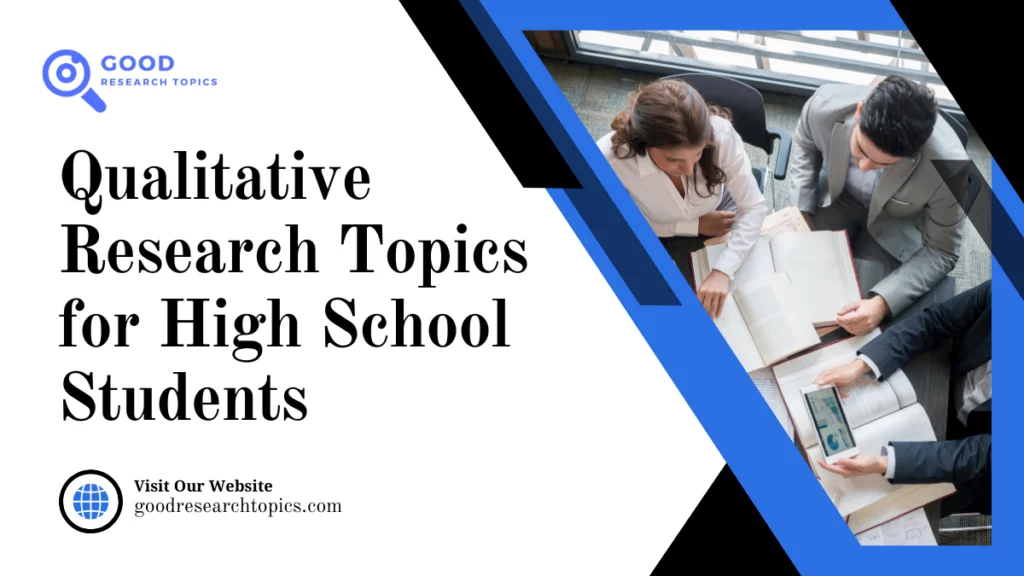
100+ Most Qualitative Research Topics For High School Students In 2024
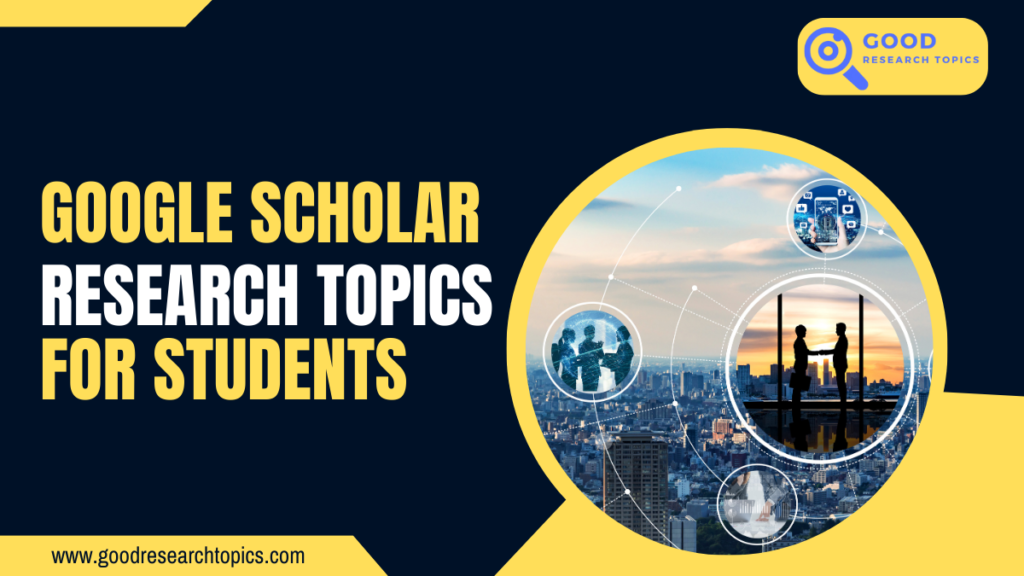
100+ Most Interesting Google Scholar Research Topics For Students [Updated 2024]
Leave a comment cancel reply.
Your email address will not be published. Required fields are marked *
Save my name, email, and website in this browser for the next time I comment.
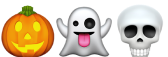
- Onsite training
3,000,000+ delegates
15,000+ clients
1,000+ locations
- KnowledgePass
- Log a ticket
01344203999 Available 24/7
12 Best Artificial Intelligence Topics for Research in 2024
Explore the "12 Best Artificial Intelligence Topics for Research in 2024." Dive into the top AI research areas, including Natural Language Processing, Computer Vision, Reinforcement Learning, Explainable AI (XAI), AI in Healthcare, Autonomous Vehicles, and AI Ethics and Bias. Stay ahead of the curve and make informed choices for your AI research endeavours.

Exclusive 40% OFF
Training Outcomes Within Your Budget!
We ensure quality, budget-alignment, and timely delivery by our expert instructors.
Share this Resource
- AI Tools in Performance Marketing Training
- Deep Learning Course
- Natural Language Processing (NLP) Fundamentals with Python
- Machine Learning Course
- Duet AI for Workspace Training
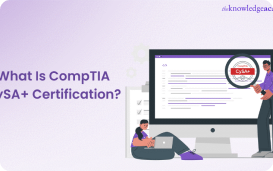
Table of Contents
1) Top Artificial Intelligence Topics for Research
a) Natural Language Processing
b) Computer vision
c) Reinforcement Learning
d) Explainable AI (XAI)
e) Generative Adversarial Networks (GANs)
f) Robotics and AI
g) AI in healthcare
h) AI for social good
i) Autonomous vehicles
j) AI ethics and bias
2) Conclusion
Top Artificial Intelligence Topics for Research
This section of the blog will expand on some of the best Artificial Intelligence Topics for research.
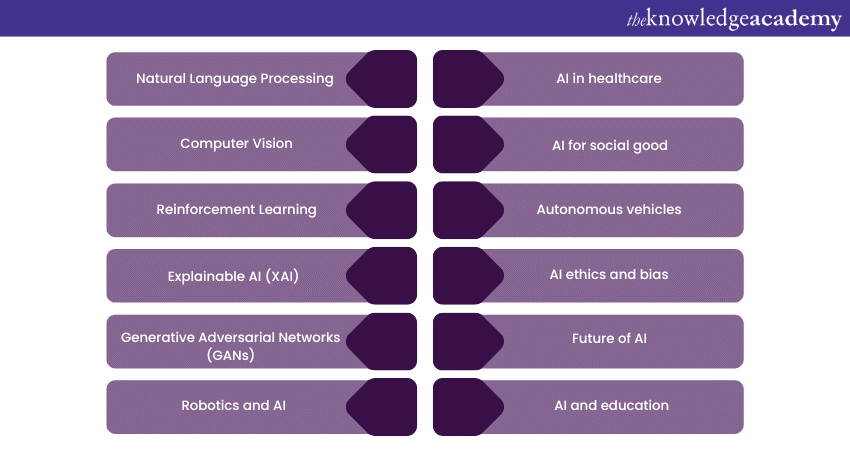
Natural Language Processing
Natural Language Processing (NLP) is centred around empowering machines to comprehend, interpret, and even generate human language. Within this domain, three distinctive research avenues beckon:
1) Sentiment analysis: This entails the study of methodologies to decipher and discern emotions encapsulated within textual content. Understanding sentiments is pivotal in applications ranging from brand perception analysis to social media insights.
2) Language generation: Generating coherent and contextually apt text is an ongoing pursuit. Investigating mechanisms that allow machines to produce human-like narratives and responses holds immense potential across sectors.
3) Question answering systems: Constructing systems that can grasp the nuances of natural language questions and provide accurate, coherent responses is a cornerstone of NLP research. This facet has implications for knowledge dissemination, customer support, and more.
Computer Vision
Computer Vision, a discipline that bestows machines with the ability to interpret visual data, is replete with intriguing avenues for research:
1) Object detection and tracking: The development of algorithms capable of identifying and tracking objects within images and videos finds relevance in surveillance, automotive safety, and beyond.
2) Image captioning: Bridging the gap between visual and textual comprehension, this research area focuses on generating descriptive captions for images, catering to visually impaired individuals and enhancing multimedia indexing.
3) Facial recognition: Advancements in facial recognition technology hold implications for security, personalisation, and accessibility, necessitating ongoing research into accuracy and ethical considerations.
Reinforcement Learning
Reinforcement Learning revolves around training agents to make sequential decisions in order to maximise rewards. Within this realm, three prominent Artificial Intelligence Topics emerge:
1) Autonomous agents: Crafting AI agents that exhibit decision-making prowess in dynamic environments paves the way for applications like autonomous robotics and adaptive systems.
2) Deep Q-Networks (DQN): Deep Q-Networks, a class of reinforcement learning algorithms, remain under active research for refining value-based decision-making in complex scenarios.
3) Policy gradient methods: These methods, aiming to optimise policies directly, play a crucial role in fine-tuning decision-making processes across domains like gaming, finance, and robotics.
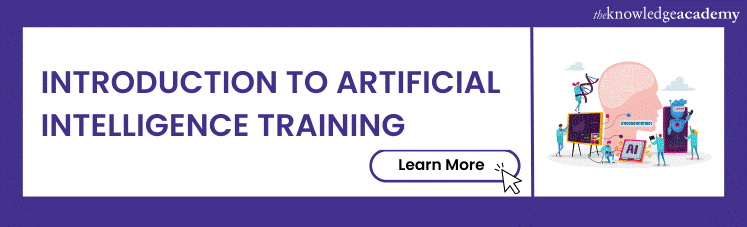
Explainable AI (XAI)
The pursuit of Explainable AI seeks to demystify the decision-making processes of AI systems. This area comprises Artificial Intelligence Topics such as:
1) Model interpretability: Unravelling the inner workings of complex models to elucidate the factors influencing their outputs, thus fostering transparency and accountability.
2) Visualising neural networks: Transforming abstract neural network structures into visual representations aids in comprehending their functionality and behaviour.
3) Rule-based systems: Augmenting AI decision-making with interpretable, rule-based systems holds promise in domains requiring logical explanations for actions taken.
Generative Adversarial Networks (GANs)
The captivating world of Generative Adversarial Networks (GANs) unfolds through the interplay of generator and discriminator networks, birthing remarkable research avenues:
1) Image generation: Crafting realistic images from random noise showcases the creative potential of GANs, with applications spanning art, design, and data augmentation.
2) Style transfer: Enabling the transfer of artistic styles between images, merging creativity and technology to yield visually captivating results.
3) Anomaly detection: GANs find utility in identifying anomalies within datasets, bolstering fraud detection, quality control, and anomaly-sensitive industries.
Robotics and AI
The synergy between Robotics and AI is a fertile ground for exploration, with Artificial Intelligence Topics such as:
1) Human-robot collaboration: Research in this arena strives to establish harmonious collaboration between humans and robots, augmenting industry productivity and efficiency.
2) Robot learning: By enabling robots to learn and adapt from their experiences, Researchers foster robots' autonomy and the ability to handle diverse tasks.
3) Ethical considerations: Delving into the ethical implications surrounding AI-powered robots helps establish responsible guidelines for their deployment.
AI in healthcare
AI presents a transformative potential within healthcare, spurring research into:
1) Medical diagnosis: AI aids in accurately diagnosing medical conditions, revolutionising early detection and patient care.
2) Drug discovery: Leveraging AI for drug discovery expedites the identification of potential candidates, accelerating the development of new treatments.
3) Personalised treatment: Tailoring medical interventions to individual patient profiles enhances treatment outcomes and patient well-being.
AI for social good
Harnessing the prowess of AI for Social Good entails addressing pressing global challenges:
1) Environmental monitoring: AI-powered solutions facilitate real-time monitoring of ecological changes, supporting conservation and sustainable practices.
2) Disaster response: Research in this area bolsters disaster response efforts by employing AI to analyse data and optimise resource allocation.
3) Poverty alleviation: Researchers contribute to humanitarian efforts and socioeconomic equality by devising AI solutions to tackle poverty.
Unlock the potential of Artificial Intelligence for effective Project Management with our Artificial Intelligence (AI) for Project Managers Course . Sign up now!
Autonomous vehicles
Autonomous Vehicles represent a realm brimming with potential and complexities, necessitating research in Artificial Intelligence Topics such as:
1) Sensor fusion: Integrating data from diverse sensors enhances perception accuracy, which is essential for safe autonomous navigation.
2) Path planning: Developing advanced algorithms for path planning ensures optimal routes while adhering to safety protocols.
3) Safety and ethics: Ethical considerations, such as programming vehicles to make difficult decisions in potential accident scenarios, require meticulous research and deliberation.
AI ethics and bias
Ethical underpinnings in AI drive research efforts in these directions:
1) Fairness in AI: Ensuring AI systems remain impartial and unbiased across diverse demographic groups.
2) Bias detection and mitigation: Identifying and rectifying biases present within AI models guarantees equitable outcomes.
3) Ethical decision-making: Developing frameworks that imbue AI with ethical decision-making capabilities aligns technology with societal values.
Future of AI
The vanguard of AI beckons Researchers to explore these horizons:
1) Artificial General Intelligence (AGI): Speculating on the potential emergence of AI systems capable of emulating human-like intelligence opens dialogues on the implications and challenges.
2) AI and creativity: Probing the interface between AI and creative domains, such as art and music, unveils the coalescence of human ingenuity and technological prowess.
3) Ethical and regulatory challenges: Researching the ethical dilemmas and regulatory frameworks underpinning AI's evolution fortifies responsible innovation.
AI and education
The intersection of AI and Education opens doors to innovative learning paradigms:
1) Personalised learning: Developing AI systems that adapt educational content to individual learning styles and paces.
2) Intelligent tutoring systems: Creating AI-driven tutoring systems that provide targeted support to students.
3) Educational data mining: Applying AI to analyse educational data for insights into learning patterns and trends.
Unleash the full potential of AI with our comprehensive Introduction to Artificial Intelligence Training . Join now!
Conclusion
The domain of AI is ever-expanding, rich with intriguing topics about Artificial Intelligence that beckon Researchers to explore, question, and innovate. Through the pursuit of these twelve diverse Artificial Intelligence Topics, we pave the way for not only technological advancement but also a deeper understanding of the societal impact of AI. By delving into these realms, Researchers stand poised to shape the trajectory of AI, ensuring it remains a force for progress, empowerment, and positive transformation in our world.
Unlock your full potential with our extensive Personal Development Training Courses. Join today!
Frequently Asked Questions
Upcoming data, analytics & ai resources batches & dates.
Fri 2nd Aug 2024
Fri 15th Nov 2024
Get A Quote
WHO WILL BE FUNDING THE COURSE?
My employer
By submitting your details you agree to be contacted in order to respond to your enquiry
- Business Analysis
- Lean Six Sigma Certification
Share this course
Our biggest spring sale.

We cannot process your enquiry without contacting you, please tick to confirm your consent to us for contacting you about your enquiry.
By submitting your details you agree to be contacted in order to respond to your enquiry.
We may not have the course you’re looking for. If you enquire or give us a call on 01344203999 and speak to our training experts, we may still be able to help with your training requirements.
Or select from our popular topics
- ITIL® Certification
- Scrum Certification
- Change Management Certification
- Business Analysis Courses
- Microsoft Azure Certification
- Microsoft Excel Courses
- Microsoft Project
- Explore more courses
Press esc to close
Fill out your contact details below and our training experts will be in touch.
Fill out your contact details below
Thank you for your enquiry!
One of our training experts will be in touch shortly to go over your training requirements.
Back to Course Information
Fill out your contact details below so we can get in touch with you regarding your training requirements.
* WHO WILL BE FUNDING THE COURSE?
Preferred Contact Method
No preference
Back to course information
Fill out your training details below
Fill out your training details below so we have a better idea of what your training requirements are.
HOW MANY DELEGATES NEED TRAINING?
HOW DO YOU WANT THE COURSE DELIVERED?
Online Instructor-led
Online Self-paced
WHEN WOULD YOU LIKE TO TAKE THIS COURSE?
Next 2 - 4 months
WHAT IS YOUR REASON FOR ENQUIRING?
Looking for some information
Looking for a discount
I want to book but have questions
One of our training experts will be in touch shortly to go overy your training requirements.
Your privacy & cookies!
Like many websites we use cookies. We care about your data and experience, so to give you the best possible experience using our site, we store a very limited amount of your data. Continuing to use this site or clicking “Accept & close” means that you agree to our use of cookies. Learn more about our privacy policy and cookie policy cookie policy .
We use cookies that are essential for our site to work. Please visit our cookie policy for more information. To accept all cookies click 'Accept & close'.

Research Topics & Ideas
Artifical Intelligence (AI) and Machine Learning (ML)
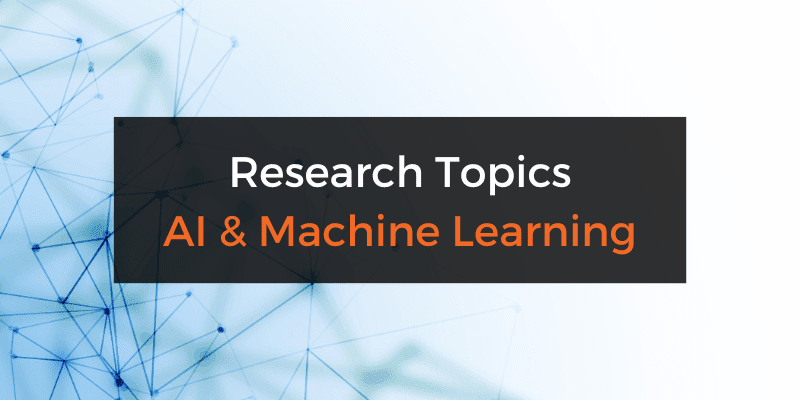
If you’re just starting out exploring AI-related research topics for your dissertation, thesis or research project, you’ve come to the right place. In this post, we’ll help kickstart your research topic ideation process by providing a hearty list of research topics and ideas , including examples from past studies.
PS – This is just the start…
We know it’s exciting to run through a list of research topics, but please keep in mind that this list is just a starting point . To develop a suitable research topic, you’ll need to identify a clear and convincing research gap , and a viable plan to fill that gap.
If this sounds foreign to you, check out our free research topic webinar that explores how to find and refine a high-quality research topic, from scratch. Alternatively, if you’d like hands-on help, consider our 1-on-1 coaching service .
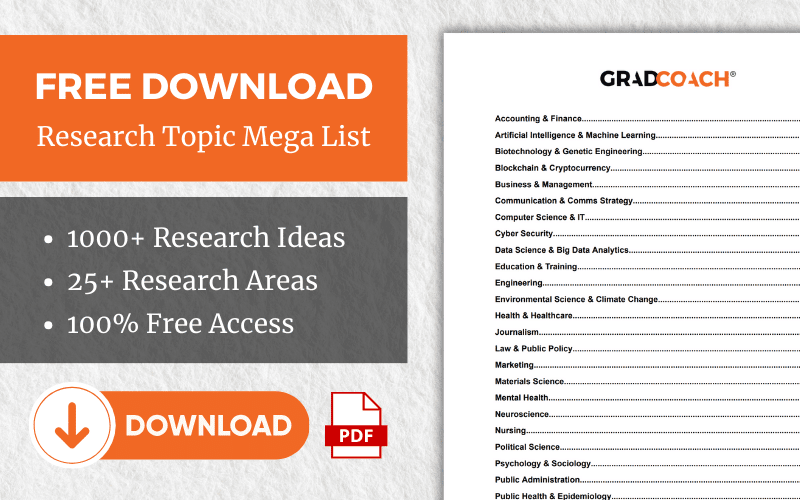
AI-Related Research Topics & Ideas
Below you’ll find a list of AI and machine learning-related research topics ideas. These are intentionally broad and generic , so keep in mind that you will need to refine them a little. Nevertheless, they should inspire some ideas for your project.
- Developing AI algorithms for early detection of chronic diseases using patient data.
- The use of deep learning in enhancing the accuracy of weather prediction models.
- Machine learning techniques for real-time language translation in social media platforms.
- AI-driven approaches to improve cybersecurity in financial transactions.
- The role of AI in optimizing supply chain logistics for e-commerce.
- Investigating the impact of machine learning in personalized education systems.
- The use of AI in predictive maintenance for industrial machinery.
- Developing ethical frameworks for AI decision-making in healthcare.
- The application of ML algorithms in autonomous vehicle navigation systems.
- AI in agricultural technology: Optimizing crop yield predictions.
- Machine learning techniques for enhancing image recognition in security systems.
- AI-powered chatbots: Improving customer service efficiency in retail.
- The impact of AI on enhancing energy efficiency in smart buildings.
- Deep learning in drug discovery and pharmaceutical research.
- The use of AI in detecting and combating online misinformation.
- Machine learning models for real-time traffic prediction and management.
- AI applications in facial recognition: Privacy and ethical considerations.
- The effectiveness of ML in financial market prediction and analysis.
- Developing AI tools for real-time monitoring of environmental pollution.
- Machine learning for automated content moderation on social platforms.
- The role of AI in enhancing the accuracy of medical diagnostics.
- AI in space exploration: Automated data analysis and interpretation.
- Machine learning techniques in identifying genetic markers for diseases.
- AI-driven personal finance management tools.
- The use of AI in developing adaptive learning technologies for disabled students.
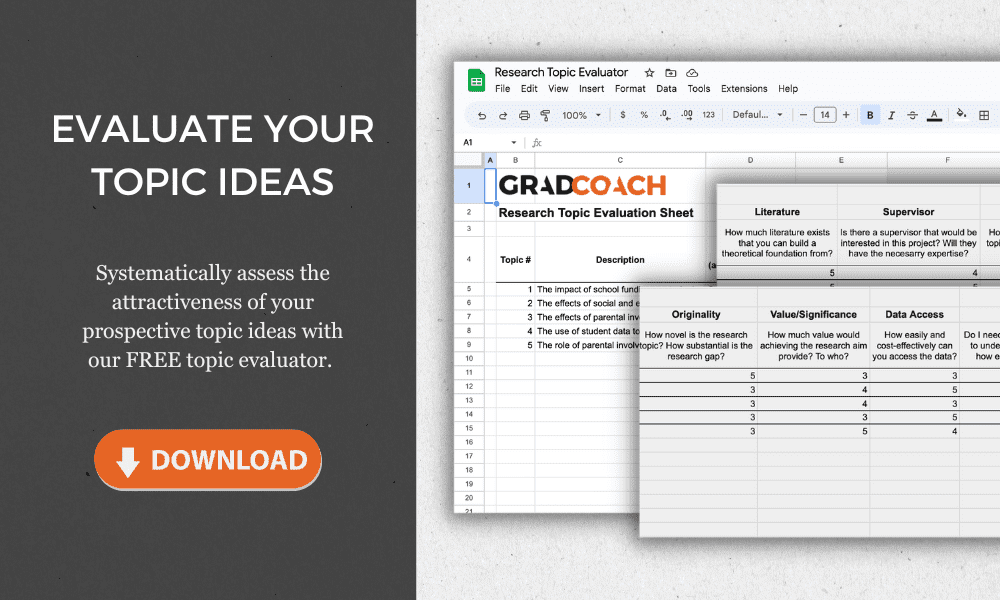
AI & ML Research Topic Ideas (Continued)
- Machine learning in cybersecurity threat detection and response.
- AI applications in virtual reality and augmented reality experiences.
- Developing ethical AI systems for recruitment and hiring processes.
- Machine learning for sentiment analysis in customer feedback.
- AI in sports analytics for performance enhancement and injury prevention.
- The role of AI in improving urban planning and smart city initiatives.
- Machine learning models for predicting consumer behaviour trends.
- AI and ML in artistic creation: Music, visual arts, and literature.
- The use of AI in automated drone navigation for delivery services.
- Developing AI algorithms for effective waste management and recycling.
- Machine learning in seismology for earthquake prediction.
- AI-powered tools for enhancing online privacy and data protection.
- The application of ML in enhancing speech recognition technologies.
- Investigating the role of AI in mental health assessment and therapy.
- Machine learning for optimization of renewable energy systems.
- AI in fashion: Predicting trends and personalizing customer experiences.
- The impact of AI on legal research and case analysis.
- Developing AI systems for real-time language interpretation for the deaf and hard of hearing.
- Machine learning in genomic data analysis for personalized medicine.
- AI-driven algorithms for credit scoring in microfinance.
- The use of AI in enhancing public safety and emergency response systems.
- Machine learning for improving water quality monitoring and management.
- AI applications in wildlife conservation and habitat monitoring.
- The role of AI in streamlining manufacturing processes.
- Investigating the use of AI in enhancing the accessibility of digital content for visually impaired users.
Recent AI & ML-Related Studies
While the ideas we’ve presented above are a decent starting point for finding a research topic in AI, they are fairly generic and non-specific. So, it helps to look at actual studies in the AI and machine learning space to see how this all comes together in practice.
Below, we’ve included a selection of AI-related studies to help refine your thinking. These are actual studies, so they can provide some useful insight as to what a research topic looks like in practice.
- An overview of artificial intelligence in diabetic retinopathy and other ocular diseases (Sheng et al., 2022)
- HOW DOES ARTIFICIAL INTELLIGENCE HELP ASTRONOMY? A REVIEW (Patel, 2022)
- Editorial: Artificial Intelligence in Bioinformatics and Drug Repurposing: Methods and Applications (Zheng et al., 2022)
- Review of Artificial Intelligence and Machine Learning Technologies: Classification, Restrictions, Opportunities, and Challenges (Mukhamediev et al., 2022)
- Will digitization, big data, and artificial intelligence – and deep learning–based algorithm govern the practice of medicine? (Goh, 2022)
- Flower Classifier Web App Using Ml & Flask Web Framework (Singh et al., 2022)
- Object-based Classification of Natural Scenes Using Machine Learning Methods (Jasim & Younis, 2023)
- Automated Training Data Construction using Measurements for High-Level Learning-Based FPGA Power Modeling (Richa et al., 2022)
- Artificial Intelligence (AI) and Internet of Medical Things (IoMT) Assisted Biomedical Systems for Intelligent Healthcare (Manickam et al., 2022)
- Critical Review of Air Quality Prediction using Machine Learning Techniques (Sharma et al., 2022)
- Artificial Intelligence: New Frontiers in Real–Time Inverse Scattering and Electromagnetic Imaging (Salucci et al., 2022)
- Machine learning alternative to systems biology should not solely depend on data (Yeo & Selvarajoo, 2022)
- Measurement-While-Drilling Based Estimation of Dynamic Penetrometer Values Using Decision Trees and Random Forests (García et al., 2022).
- Artificial Intelligence in the Diagnosis of Oral Diseases: Applications and Pitfalls (Patil et al., 2022).
- Automated Machine Learning on High Dimensional Big Data for Prediction Tasks (Jayanthi & Devi, 2022)
- Breakdown of Machine Learning Algorithms (Meena & Sehrawat, 2022)
- Technology-Enabled, Evidence-Driven, and Patient-Centered: The Way Forward for Regulating Software as a Medical Device (Carolan et al., 2021)
- Machine Learning in Tourism (Rugge, 2022)
- Towards a training data model for artificial intelligence in earth observation (Yue et al., 2022)
- Classification of Music Generality using ANN, CNN and RNN-LSTM (Tripathy & Patel, 2022)
As you can see, these research topics are a lot more focused than the generic topic ideas we presented earlier. So, in order for you to develop a high-quality research topic, you’ll need to get specific and laser-focused on a specific context with specific variables of interest. In the video below, we explore some other important things you’ll need to consider when crafting your research topic.
Get 1-On-1 Help
If you’re still unsure about how to find a quality research topic, check out our Research Topic Kickstarter service, which is the perfect starting point for developing a unique, well-justified research topic.
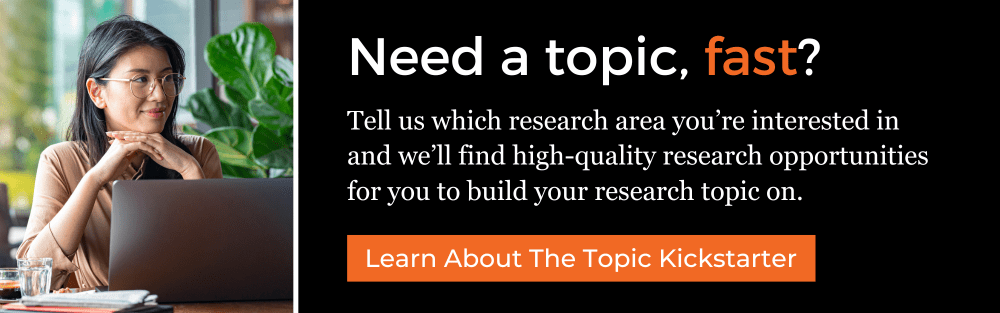
You Might Also Like:
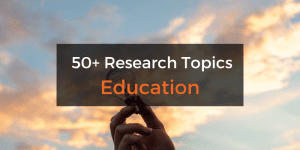
can one come up with their own tppic and get a search
can one come up with their own title and get a search
Submit a Comment Cancel reply
Your email address will not be published. Required fields are marked *
Save my name, email, and website in this browser for the next time I comment.
- Print Friendly
When you choose to publish with PLOS, your research makes an impact. Make your work accessible to all, without restrictions, and accelerate scientific discovery with options like preprints and published peer review that make your work more Open.
PLOS Biology
- PLOS Climate
- PLOS Complex Systems
PLOS Computational Biology
PLOS Digital Health
- PLOS Genetics
- PLOS Global Public Health
PLOS Medicine
- PLOS Mental Health
- PLOS Neglected Tropical Diseases
- PLOS Pathogens
- PLOS Sustainability and Transformation
- PLOS Collections
Artificial intelligence
Discover multidisciplinary research that explores the opportunities, applications, and risks of machine learning and artificial intelligence across a broad spectrum of disciplines.
Discover artificial intelligence research from PLOS
From exploring applications in healthcare and biomedicine to unraveling patterns and optimizing decision-making within complex systems, PLOS’ interdisciplinary artificial intelligence research aims to capture cutting-edge methodologies, advancements, and breakthroughs in machine learning, showcasing diverse perspectives, interdisciplinary approaches, and societal and ethical implications.
Given their increasing influence in our everyday lives, it is vital to ensure that artificial intelligence tools are both inclusive and reliable. Robust and trusted artificial intelligence research hinges on the foundation of Open Science practices and the meticulous curation of data which PLOS takes pride in highlighting and endorsing thanks to our rigorous standards and commitment to Openness.
Stay up-to-date on artificial intelligence research from PLOS
Research spotlights
As a leading publisher in the field, these articles showcase research that has influenced academia, industry and/or policy.

A performance comparison of supervised machine learning models for Covid-19 tweets sentiment analysis

CellProfiler 3.0: Next- generation image processing for biology

Predicting survival from colorectal cancer histology slides using deep learning: A retrospective multicenter study
Artificial intelligence research topics.
PLOS publishes research across a broad range of topics. Take a look at the latest work in your field.
AI and climate change
Deep learning
AI for healthcare
Artificial neural networks
Natural language processing (NLP)
Graph neural networks (GNNs)
AI ethics and fairness
Machine learning
AI and complex systems
Read the latest research developments in your field
Our commitment to Open Science means others can build on PLOS artificial intelligence research to advance the field. Discover selected popular artificial intelligence research below:
Artificial intelligence based writer identification generates new evidence for the unknown scribes of the Dead Sea Scrolls exemplified by the Great Isaiah Scroll (1QIsaa)
Artificial intelligence with temporal features outperforms machine learning in predicting diabetes
Bat detective—Deep learning tools for bat acoustic signal detection
Bias in artificial intelligence algorithms and recommendations for mitigation
Can machine-learning improve cardiovascular risk prediction using routine clinical data?
Convergence of mechanistic modeling and artificial intelligence in hydrologic science and engineering
Cyberbullying severity detection: A machine learning approach
Diverse patients’ attitudes towards Artificial Intelligence (AI) in diagnosis
Expansion of RiPP biosynthetic space through integration of pan-genomics and machine learning uncovers a novel class of lanthipeptides
Generalizable brain network markers of major depressive disorder across multiple imaging sites
Machine learning algorithm validation with a limited sample size
Neural spiking for causal inference and learning
Review of machine learning methods in soft robotics
Ten quick tips for harnessing the power of ChatGPT in computational biology
Ten simple rules for engaging with artificial intelligence in biomedicine
Browse the full PLOS portfolio of Open Access artificial intelligence articles
25,938 authors from 133 countries chose PLOS to publish their artificial intelligence research*
Reaching a global audience, this research has received over 5,502 news and blog mentions ^ , research in this field has been cited 117,554 times after authors published in a plos journal*, related plos research collections.
Covering a connected body of work and evaluated by leading experts in their respective fields, our Collections make it easier to delve deeper into specific research topics from across the breadth of the PLOS portfolio.
Check out our highlighted PLOS research Collections:

Machine Learning in Health and Biomedicine

Cities as Complex Systems

Open Quantum Computation and Simulation
Stay up-to-date on the latest artificial intelligence research from PLOS
Related journals in artificial intelligence
We provide a platform for artificial intelligence research across various PLOS journals, allowing interdisciplinary researchers to explore artificial intelligence research at all preclinical, translational and clinical research stages.
*Data source: Web of Science . © Copyright Clarivate 2024 | January 2004 – January 2024 ^Data source: Altmetric.com | January 2004 – January 2024
Breaking boundaries. Empowering researchers. Opening science.
PLOS is a nonprofit, Open Access publisher empowering researchers to accelerate progress in science and medicine by leading a transformation in research communication.
Open Access
All PLOS journals are fully Open Access, which means the latest published research is immediately available for all to learn from, share, and reuse with attribution. No subscription fees, no delays, no barriers.
Leading responsibly
PLOS is working to eliminate financial barriers to Open Access publishing, facilitate diversity and broad participation of voices in knowledge-sharing, and ensure inclusive policies shape our journals. We’re committed to openness and transparency, whether it’s peer review, our data policy, or sharing our annual financial statement with the community.
Breaking boundaries in Open Science
We push the boundaries of “Open” to create a more equitable system of scientific knowledge and understanding. All PLOS articles are backed by our Data Availability policy, and encourage the sharing of preprints, code, protocols, and peer review reports so that readers get more context.
Interdisciplinary
PLOS journals publish research from every discipline across science and medicine and related social sciences. Many of our journals are interdisciplinary in nature to facilitate an exchange of knowledge across disciplines, encourage global collaboration, and influence policy and decision-making at all levels
Community expertise
Our Editorial Boards represent the full diversity of the research and researchers in the field. They work in partnership with expert peer reviewers to evaluate each manuscript against the highest methodological and ethical standards in the field.
Rigorous peer review
Our rigorous editorial screening and assessment process is made up of several stages. All PLOS journals use anonymous peer review by default, but we also offer authors and reviewers options to make the peer review process more transparent.
- Who’s Teaching What
- Subject Updates
- MEng program
- Opportunities
- Minor in Computer Science
- Resources for Current Students
- Program objectives and accreditation
- Graduate program requirements
- Admission process
- Degree programs
- Graduate research
- EECS Graduate Funding
- Resources for current students
- Student profiles
- Instructors
- DEI data and documents
- Recruitment and outreach
- Community and resources
- Get involved / self-education
- Rising Stars in EECS
- Graduate Application Assistance Program (GAAP)
- MIT Summer Research Program (MSRP)
- Sloan-MIT University Center for Exemplary Mentoring (UCEM)
- Electrical Engineering
- Computer Science
- Artificial Intelligence + Decision-making
- AI and Society
- AI for Healthcare and Life Sciences
- Artificial Intelligence and Machine Learning
- Biological and Medical Devices and Systems
- Communications Systems
- Computational Biology
- Computational Fabrication and Manufacturing
- Computer Architecture
- Educational Technology
- Electronic, Magnetic, Optical and Quantum Materials and Devices
- Graphics and Vision
- Human-Computer Interaction
- Information Science and Systems
- Integrated Circuits and Systems
- Nanoscale Materials, Devices, and Systems
- Natural Language and Speech Processing
- Optics + Photonics
- Optimization and Game Theory
- Programming Languages and Software Engineering
- Quantum Computing, Communication, and Sensing
- Security and Cryptography
- Signal Processing
- Systems and Networking
- Systems Theory, Control, and Autonomy
- Theory of Computation
- Departmental History
- Departmental Organization
- Visiting Committee
- Explore all research areas
Our research covers a wide range of topics of this fast-evolving field, advancing how machines learn, predict, and control, while also making them secure, robust and trustworthy. Research covers both the theory and applications of ML. This broad area studies ML theory (algorithms, optimization, etc.); statistical learning (inference, graphical models, causal analysis, etc.); deep learning; reinforcement learning; symbolic reasoning ML systems; as well as diverse hardware implementations of ML.
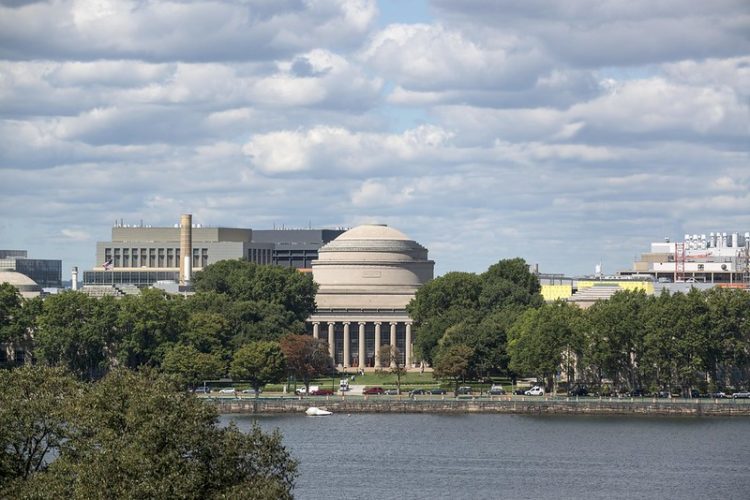
Latest news in artificial intelligence and machine learning
Creating bespoke programming languages for efficient visual ai systems.
Associate Professor Jonathan Ragan-Kelley optimizes how computer graphics and images are processed for the hardware of today and tomorrow.
QS World University Rankings rates MIT No. 1 in 11 subjects for 2024
The Institute also ranks second in five subject areas.
Three from MIT awarded 2024 Guggenheim Fellowships
MIT professors Roger Levy, Tracy Slatyer, and Martin Wainwright appointed to the 2024 class of “trail-blazing fellows.”
To build a better AI helper, start by modeling the irrational behavior of humans
A new technique can be used to predict the actions of human or AI agents who behave suboptimally while working toward unknown goals.
Priya Donti named AI2050 Early Career Fellow
Assistant Professor Priya Donti has been named an AI2050 Early Career Fellow by Schmidt Sciences, a philanthropic initiative from Eric and Wendy Schmidt aimed at helping to solve hard problems in AI.
Upcoming events
Eecs spring town hall.
Machine Learning - CMU
Phd program in machine learning.
Carnegie Mellon University's doctoral program in Machine Learning is designed to train students to become tomorrow's leaders through a combination of interdisciplinary coursework, hands-on applications, and cutting-edge research. Graduates of the Ph.D. program in Machine Learning will be uniquely positioned to pioneer new developments in the field, and to be leaders in both industry and academia.
Understanding the most effective ways of using the vast amounts of data that are now being stored is a significant challenge to society, and therefore to science and technology, as it seeks to obtain a return on the huge investment that is being made in computerization and data collection. Advances in the development of automated techniques for data analysis and decision making requires interdisciplinary work in areas such as machine learning algorithms and foundations, statistics, complexity theory, optimization, data mining, etc.
The Ph.D. Program in Machine Learning is for students who are interested in research in Machine Learning. For questions and concerns, please contact us .
The PhD program is a full-time in-person committment and is not offered on-line or part-time.
PhD Requirements
Requirements for the phd in machine learning.
- Completion of required courses , (6 Core Courses + 1 Elective)
- Mastery of proficiencies in Teaching and Presentation skills.
- Successful defense of a Ph.D. thesis.
Teaching Ph.D. students are required to serve as Teaching Assistants for two semesters in Machine Learning courses (10-xxx), beginning in their second year. This fulfills their Teaching Skills requirement.
Conference Presentation Skills During their second or third year, Ph.D. students must give a talk at least 30 minutes long, and invite members of the Speaking Skills committee to attend and evaluate it.
Research It is expected that all Ph.D. students engage in active research from their first semester. Moreover, advisor selection occurs in the first month of entering the Ph.D. program, with the option to change at a later time. Roughly half of a student's time should be allocated to research and lab work, and half to courses until these are completed.
Master of Science in Machine Learning Research - along the way to your PhD Degree.
Other Requirements In addition, students must follow all university policies and procedures .
Rules for the MLD PhD Thesis Committee (applicable to all ML PhDs): The committee should be assembled by the student and their advisor, and approved by the PhD Program Director(s). It must include:
- At least one MLD Core Faculty member
- At least one additional MLD Core or Affiliated Faculty member
- At least one External Member, usually meaning external to CMU
- A total of at least four members, including the advisor who is the committee chair
Financial Support
Application Information
For applicants applying in Fall 2023 for a start date of August 2024 in the Machine Learning PhD program, GRE Scores are REQUIRED. The committee uses GRE scores to gauge quantitative skills, and to a lesser extent, also verbal skills.
Proof of English Language Proficiency If you will be studying on an F-1 or J-1 visa, and English is not a native language for you (native language…meaning spoken at home and from birth), we are required to formally evaluate your English proficiency. We require applicants who will be studying on an F-1 or J-1 visa, and for whom English is not a native language, to demonstrate English proficiency via one of these standardized tests: TOEFL (preferred), IELTS, or Duolingo. We discourage the use of the "TOEFL ITP Plus for China," since speaking is not scored. We do not issue waivers for non-native speakers of English. In particular, we do not issue waivers based on previous study at a U.S. high school, college, or university. We also do not issue waivers based on previous study at an English-language high school, college, or university outside of the United States. No amount of educational experience in English, regardless of which country it occurred in, will result in a test waiver.
Submit valid, recent scores: If as described above you are required to submit proof of English proficiency, your TOEFL, IELTS or Duolingo test scores will be considered valid as follows: If you have not received a bachelor’s degree in the U.S., you will need to submit an English proficiency score no older than two years. (scores from exams taken before Sept. 1, 2021, will not be accepted.) If you are currently working on or have received a bachelor's and/or a master's degree in the U.S., you may submit an expired test score up to five years old. (scores from exams taken before Sept. 1, 2018, will not be accepted.)
Graduate Online Application
- Early Application Deadline – November 29, 2023 (3:00 p.m. EST)
- Final Application Deadline - December 13, 2023 (3:00 p.m. EST)
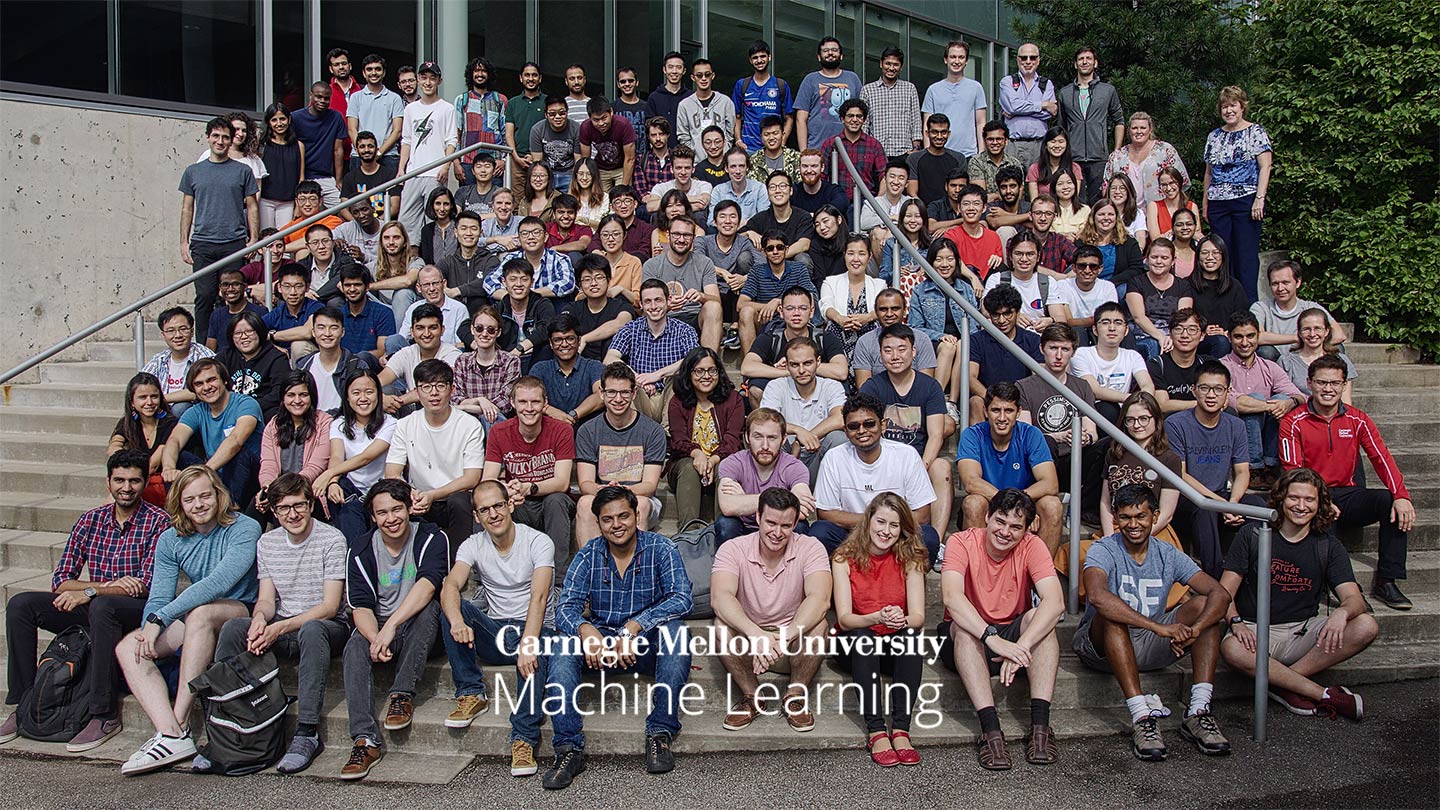
ARTIFICIAL INTELLIGENCE PHD RESEARCH TOPICS
Here in phdprojects.org we guide scholars for topic selection for your PhD and MS later we carry out research work as well. Our developers are furnished with advanced knowledge and research skills in the field of artificial intelligence (AI). We select the topics that would be original yet viable. Online guidance will be given for scholars. Contact us for further support.
Do you need a PhD or master’s degree to research in artificial intelligence?
- Nature of Research : Fundamental research includes deep understanding that comes with advanced study.
- Access to Resources : Generally, universities and research institutions have contact to resources which is vital for AI research.
- Peer Review : In spite of your educational background, a critical part of the research process is a peer review. Here we occupy with the broader research community for feedback.
Our service includes all aspects of research discussions. We assist tactically and suggest on modifications in the topic or propose our own topic, further we organise reference papers, explain the methodology and statistics that we are developing for research work as each path in AI research has its own limitations.
How helpful is Python programming for a PhD in computer science thesis work in artificial intelligence?
Pursuing a PhD in Python act as the ground breaking platform. Python programming are extremely used now a days due to its wide features as it has a rich set of libraries and tools for unique purposes, we carry out well by following latest techniques and applying proper algorithms.
Versatility and Applicability:
- Widespread Usage : Python language’s wide acceptance in academia makes it a go-to select for many projects as it is one of the most popular programming languages for AI research and development.
- Rich Libraries and Frameworks : Specialized libraries are offered by python and frameworks for AI, machine learning, data science, and natural language processing, such as TensorFlow, PyTorch, scikit-learn, NLTK.
- Data Manipulation : To operate data libraries like Pandas and NumPy offer sophisticated ways, which is critical for AI research.
- Visualization : An essential aspect of any research work is to make it easier to visualize data and results by using Matplotlib and Seaborn.
- Algorithm Implementation : To implement complex algorithms, that allows for easier debugging and maintenance Python’s ease and legibility acts as a good choice.
Research Prototyping:
- Quick Prototyping : For a quick development Python makes it easier to test hypotheses and repeat on your models.
- Interdisciplinary Work : Python’s pertinency will be given in other scientific disciplines as it eases interdisciplinary research involving AI.
Reproducibility and Collaboration:
- Code Sharing : For academic collaboration and code reproducibility Python’s readability and the accessibility of Jupyter Notebooks make it cooler to share code.
- Open Source : Python tools act as an open-source, by encouraging community contributions and by making it easier for researchers to customize tools as per specific needs.
Computational Efficiency:
- Interfacing with Low-Level Languages : Python interface with low-level languages like C/C++, we can optimize the performance-critical parts of our code as it is not a fast language.
- Cloud Integration : We can measure our experiments to more powerful machines or distribute tasks as it works well in cloud environments.
Skills Transferability:
- Industry Relevance : If we wish to change into industry roles post-PhD, as Python it is a major benefit as it is widely used in AI and data science projects.
- Broad Community Support : One can find a wealth of tutorials, forums and various resources under python as it helps you to overcome any trials you meet for your research.
Thus, python is a friendly language we switch on to tasks that are with easy patterns to implement your artificial intelligence projects in python. Python programming are well executed by our leading developers while the thesis work is done to the best level by our talented researchers, as many experts handle your paper the outcome will be at top level. Don’t worry your entire AI research workflow, right from data collection to publication are safely handled by us.
Tips for Writing an Academic Paper on Artificial Intelligence Topic
Writing an Academic Paper on AI is a vital process where one must have a detailed knowledge of the specified domain. Our services are steadfast as it is completely done by professionals. We help the scholars to understand that we aim to write a research paper that has not already been explored.
Some of the tips that we follow to write an academic paper have been listed out:
Preliminary Steps:
- Find a Research Gap : Here we will find out the existing literature to find unanswered questions or untouched areas which needs more study.
- Set Clear Objectives : The problem that we require to solve will be stated, the meaning and contributions of our work will be explained.
Literature Review:
- Conduct a Thorough Review : The relevant work, classified methodologies, and outline the theoretical framework will be mentioned at this step. We assure that how our work is different from the existing work.
- Use Academic Databases : Index records like PubMed, IEEE Xplore, and Google Scholar for high-quality sources.
Methodology:
- Be Rigorous and Detailed : We will briefly mention about the methods used in research so that no duplicates are made. Cover data sources, algorithms, and any calculations metrics.
- Validate Assumptions : We avoid criticisms during the review process so we clearly mention and validate assumptions.
Writing the Paper:
- Structured Approach : For Introduction, Literature Review, Methodology, Results, Discussion, Conclusion, and References we use traditional layout as it is global used, in case if you want a different format, we are willing to do it.
- Clarity and Simplicity : A clear and brief language is used.
- Use Figures and Tables : Here we coney data briefly as Visual aids like graphs, charts, and tables.
Validation and Results:
- Data Integrity : Our data is accurate and laid out correctly. Any manipulation or selection shall be justified.
- Discuss Limitations : The limitations of our research and the suggested areas where we work honest for our future work.
Peer Review and Revision:
- Feedback Loop : Your paper will be reviewed by our senior advisors, or experts in that specified field for productive feedback.
- Revise and Iterate : Multiple revisions and formatting take place at this stage.
Submission and Post-Submission:
- Follow Submission Guidelines : Our writers read and follow all the university guidelines provided by the journal or conference to which you are submitting.
- Prepare for Rejection or Revision : Don’t worry if your paper is rejected or requested for revision it is very common in the academic world. So that your paper can be improved further.
- Post-Publication Sharing : Through academic networks and social media your paper will be shared to gain visibility.
Extra Tips:
- Citations : to keep track of references we make use of a citation manager.
- Plagiarism : Our work will be original and all referred ideas will be properly quoted.
- Proofreading: We check for grammatical errors, and assure that the paper stick to the chosen style guide.
- Time Management : Our researchers allocate time for research, data collection, writing, and revision to manage time productively.
Hence forth we assure you that we present you the most compelling and impactful paper in the field of AI as we follow the above tips. Paper writing are done best by our writers due to our sincere commitment we always attain 100% success in our work.
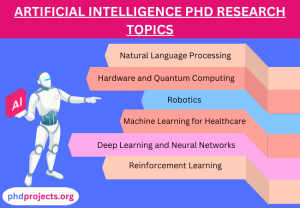
PhD research Topic Ideas in Artificial Intelligence
Dive into our page for more interesting topic ideas in the field of AI. Leading papers will be referred for your selecting topics so that customer satisfaction is key important to us. We have a multidisciplinary team of high qualified researchers and developers, working for research area in across domain.
- Transforming Education System through Artificial Intelligence and Machine Learning
- Investigating the Role of Artificial Intelligence in Building Smart Contact on Block-Chain
- Artificial Intelligence based Electronics Engineering Software Application System
- The Role of Artificial Intelligence in the Education Process of Political Science Field
- Artificial Intelligence applications addressing different aspects of the Covid-19 crisis and key technological solutions for future epidemics control
- Neuralink- An Elon Musk Start-up Achieve symbiosis with Artificial Intelligence
- Study of the Knowledge and Impact of Artificial Intelligence on an Academic Community
- Artificial Intelligence and Successful Factors for Selecting Product Innovation Development
- Artificial Intelligence Applied to Software Testing: A Literature Review
- Dependability Analysis and Verification Technology of Artificial Intelligence Software
- Effect of artificial-intelligence planning-procedures on system reliability
- 3 A 0.55V 1.1mW artificial-intelligence processor with PVT compensation for micro robots
- Explainable Artificial Intelligence for the Remaining Useful Life Prognosis of the Turbofan Engines
- Artificial Intelligence Based Student Learning Evaluation Tool
- Applying artificial intelligence techniques to human-computer interfaces
- Using artificial intelligence techniques for solving power systems problems at undergraduate level
- Developing a “Fundamentals of Artificial Intelligence” E-Learning Course
- Synthesizing Technical Analysis, Fundamental Analysis & Artificial Intelligence – An Applied Approach to Portfolio Optimisation & Performance Analysis of Stock Prices in India
- A Web-Based Patient Support System Using Artificial Intelligence to Improve Health Monitoring and Quality of Life
- An assessment of resource exploitation using artificial intelligence-based traffic control strategies
- PHD Guidance
- PHD PROJECTS UK
- PHD ASSISTANCE IN BANGALORE
- PHD Assistance
- PHD In 3 Months
- PHD Dissertation Help
- PHD IN JAVA PROGRAMMING
- PHD PROJECTS IN MATLAB
- PHD PROJECTS IN RTOOL
- PHD PROJECTS IN WEKA
- PhD projects in computer networking
- COMPUTER SCIENCE THESIS TOPICS FOR UNDERGRADUATES
- PHD PROJECTS AUSTRALIA
- PHD COMPANY
- PhD THESIS STRUCTURE
- PHD GUIDANCE HELP
- PHD PROJECTS IN HADOOP
- PHD PROJECTS IN OPENCV
- PHD PROJECTS IN SCILAB
- PHD PROJECTS IN WORDNET
- NETWORKING PROJECTS FOR PHD
- THESIS TOPICS FOR COMPUTER SCIENCE STUDENTS
- IEEE JOURNALS IN COMPUTER SCIENCE
- OPEN ACCESS JOURNALS IN COMPUTER SCIENCE
- SCIENCE CITATION INDEX COMPUTER SCIENCE JOURNALS
- SPRINGER JOURNALS IN COMPUTER SCIENCE
- ELSEVIER JOURNALS IN COMPUTER SCIENCE
- ACM JOURNALS IN COMPUTER SCIENCE
- INTERNATIONAL JOURNALS FOR COMPUTER SCIENCE AND ENGINEERING
- COMPUTER SCIENCE JOURNALS WITHOUT PUBLICATION FEE
- SCIENCE CITATION INDEX EXPANDED JOURNALS LIST
- THOMSON REUTERS INDEXED JOURNALS
- DOAJ COMPUTER SCIENCE JOURNALS
- SCOPUS INDEXED COMPUTER SCIENCE JOURNALS
- SCI INDEXED COMPUTER SCIENCE JOURNALS
- SPRINGER JOURNALS IN COMPUTER SCIENCE AND TECHNOLOGY
- ISI INDEXED JOURNALS IN COMPUTER SCIENCE
- PAID JOURNALS IN COMPUTER SCIENCE
- NATIONAL JOURNALS IN COMPUTER SCIENCE AND ENGINEERING
- MONTHLY JOURNALS IN COMPUTER SCIENCE
- SCIMAGO JOURNALS LIST
- THOMSON REUTERS INDEXED COMPUTER SCIENCE JOURNALS
- RESEARCH PAPER FOR SALE
- CHEAP PAPER WRITING SERVICE
- RESEARCH PAPER ASSISTANCE
- THESIS BUILDER
- WRITING YOUR JOURNAL ARTICLE IN 12 WEEKS
- WRITE MY PAPER FOR ME
- PHD PAPER WRITING SERVICE
- THESIS MAKER
- THESIS HELPER
- DISSERTATION HELP UK
- DISSERTATION WRITERS UK
- BUY DISSERTATION ONLINE
- PHD THESIS WRITING SERVICES
- DISSERTATION WRITING SERVICES UK
- DISSERTATION WRITING HELP
- PHD PROJECTS IN COMPUTER SCIENCE
- DISSERTATION ASSISTANCE
- Write my thesis
- Thesis writers
- Buy thesis papers
- Bachelor thesis
- Master's thesis
- Thesis editing services
- Thesis proofreading services
- Buy a thesis online
- Write my dissertation
- Dissertation proposal help
- Pay for dissertation
- Custom dissertation
- Dissertation help online
- Buy dissertation online
- Cheap dissertation
- Dissertation editing services
- Write my research paper
- Buy research paper online
- Pay for research paper
- Research paper help
- Order research paper
- Custom research paper
- Cheap research paper
- Research papers for sale
- Thesis subjects
- How It Works
177 Great Artificial Intelligence Research Paper Topics to Use
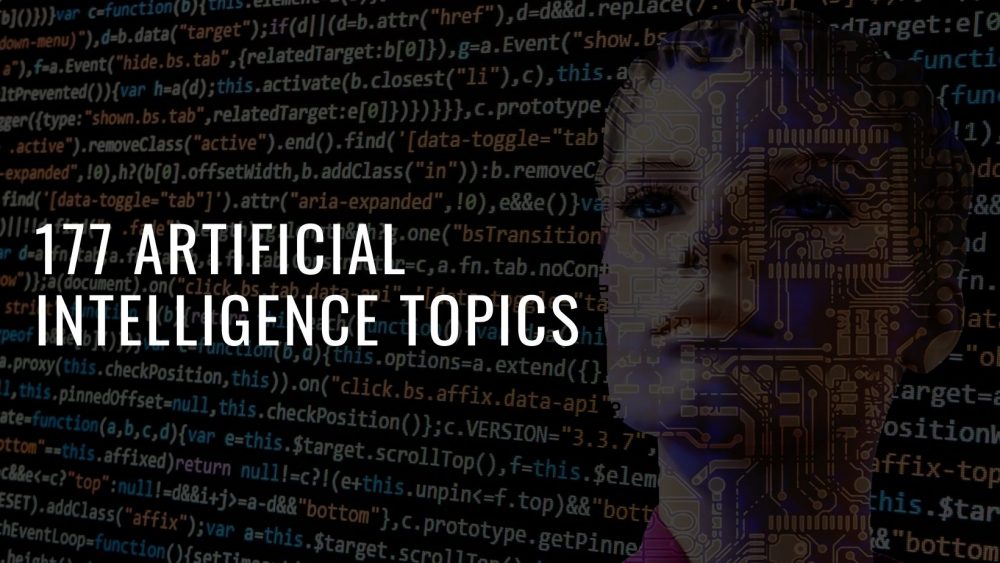
In this top-notch post, we will look at the definition of artificial intelligence, its applications, and writing tips on how to come up with AI topics. Finally, we shall lock at top artificial intelligence research topics for your inspiration.
What Is Artificial Intelligence?
It refers to intelligence as demonstrated by machines, unlike that which animals and humans display. The latter involves emotionality and consciousness. The field of AI has gained proliferation in recent days, with many scientists investing their time and effort in research.
How To Develop Topics in Artificial Intelligence
Developing AI topics is a critical thinking process that also incorporates a lot of creativity. Due to the ever-dynamic nature of the discipline, most students find it hard to develop impressive topics in artificial intelligence. However, here are some general rules to get you started:
Read widely on the subject of artificial intelligence Have an interest in news and other current updates about AI Consult your supervisor
Once you are ready with these steps, nothing is holding you from developing top-rated topics in artificial intelligence. Now let’s look at what the pros have in store for you.
Artificial Intelligence Research Paper Topics
- The role of artificial intelligence in evolving the workforce
- Are there tasks that require unique human abilities apart from machines?
- The transformative economic impact of artificial intelligence
- Managing a global autonomous arms race in the face of AI
- The legal and ethical boundaries of artificial intelligence
- Is the destructive role of AI more than its constructive role in society?
- How to build AI algorithms to achieve the far-reaching goals of humans
- How privacy gets compromised with the everyday collection of data
- How businesses and governments can suffer at the hands of AI
- Is it possible for AI to devolve into social oppression?
- Augmentation of the work humans do through artificial intelligence
- The role of AI in monitoring and diagnosing capabilities
Artificial Intelligence Topics For Presentation
- How AI helps to uncover criminal activity and solve serial crimes
- The place of facial recognition technologies in security systems
- How to use AI without crossing an individual’s privacy
- What are the disadvantages of using a computer-controlled robot in performing tasks?
- How to develop systems endowed with intellectual processes
- The challenge of programming computers to perform complex tasks
- Discuss some of the mathematical theorems for artificial intelligence systems
- The role of computer processing speed and memory capacity in AI
- Can computer machines achieve the performance levels of human experts?
- Discuss the application of artificial intelligence in handwriting recognition
- A case study of the key people involved in developing AI systems
- Computational aesthetics when developing artificial intelligence systems
Topics in AI For Tip-Top Grades
- Describe the necessities for artificial programming language
- The impact of American companies possessing about 2/3 of investments in AI
- The relationship between human neural networks and A.I
- The role of psychologists in developing human intelligence
- How to apply past experiences to analogous new situations
- How machine learning helps in achieving artificial intelligence
- The role of discernment and human intelligence in developing AI systems
- Discuss the various methods and goals in artificial intelligence
- What is the relationship between applied AI, strong AI, and cognitive simulation
- Discuss the implications of the first AI programs
- Logical reasoning and problem-solving in artificial intelligence
- Challenges involved in controlled learning environments
AI Research Topics For High School Students
- How quantum computing is affecting artificial intelligence
- The role of the Internet of Things in advancing artificial intelligence
- Using Artificial intelligence to enable machines to perform programming tasks
- Why do machines learn automatically without human hand holding
- Implementing decisions based on data processing in the human mind
- Describe the web-like structure of artificial neural networks
- Machine learning algorithms for optimal functions through trial and error
- A case study of Google’s AlphaGo computer program
- How robots solve problems in an intelligent manner
- Evaluate the significant role of M.I.T.’s artificial intelligence lab
- A case study of Robonaut developed by NASA to work with astronauts in space
- Discuss natural language processing where machines analyze language and speech
Argument Debate Topics on AI
- How chatbots use ML and N.L.P. to interact with the users
- How do computers use and understand images?
- The impact of genetic engineering on the life of man
- Why are micro-chips not recommended in human body systems?
- Can humans work alongside robots in a workplace system?
- Have computers contributed to the intrusion of privacy for many?
- Why artificial intelligence systems should not be made accessible to children
- How artificial intelligence systems are contributing to healthcare problems
- Does artificial intelligence alleviate human problems or add to them?
- Why governments should put more stringent measures for AI inventions
- How artificial intelligence is affecting the character traits of children born
- Is virtual reality taking people out of the real-world situation?
Quality AI Topics For Research Paper
- The use of recommender systems in choosing movies and series
- Collaborative filtering in designing systems
- How do developers arrive at a content-based recommendation
- Creation of systems that can emulate human tasks
- How IoT devices generate a lot of data
- Artificial intelligence algorithms convert data to useful, actionable results.
- How AI is progressing rapidly with the 5G technology
- How to develop robots with human-like characteristics
- Developing Google search algorithms
- The role of artificial intelligence in developing autonomous weapons
- Discuss the long-term goal of artificial intelligence
- Will artificial intelligence outperform humans at every cognitive task?
Computer Science AI Topics
- Computational intelligence magazine in computer science
- Swarm and evolutionary computation procedures for college students
- Discuss computational transactions on intelligent transportation systems
- The structure and function of knowledge-based systems
- A review of the artificial intelligence systems in developing systems
- Conduct a review of the expert systems with applications
- Critique the various foundations and trends in information retrieval
- The role of specialized systems in transactions on knowledge and data engineering
- An analysis of a journal on ambient intelligence and humanized computing
- Discuss the various computer transactions on cognitive communications and networking
- What is the role of artificial intelligence in medicine?
- Computer engineering applications of artificial intelligence
AI Ethics Topics
- How the automation of jobs is going to make many jobless
- Discuss inequality challenges in distributing wealth created by machines
- The impact of machines on human behavior and interactions
- How artificial intelligence is going to affect how we act accordingly
- The process of eliminating bias in Artificial intelligence: A case of racist robots
- Measures that can keep artificial intelligence safe from adversaries
- Protecting artificial intelligence discoveries from unintended consequences
- How a man can stay in control despite the complex, intelligent systems
- Robot rights: A case of how man is mistreating and misusing robots
- The balance between mitigating suffering and interfering with set ethics
- The role of artificial intelligence in negative outcomes: Is it worth it?
- How to ethically use artificial intelligence for bettering lives
Advanced AI Topics
- Discuss how long it will take until machines greatly supersede human intelligence
- Is it possible to achieve superhuman artificial intelligence in this century?
- The impact of techno-skeptic prediction on the performance of A.I
- The role of quarks and electrons in the human brain
- The impact of artificial intelligence safety research institutes
- Will robots be disastrous for humanity shortly?
- Robots: A concern about consciousness and evil
- Discuss whether a self-driving car has a subjective experience or not
- Should humans worry about machines turning evil in the end?
- Discuss how machines exhibit goal-oriented behavior in their functions
- Should man continue to develop lethal autonomous weapons?
- What is the implication of machine-produced wealth?
AI Essay Topics Technology
- Discuss the implication of the fourth technological revelation in cloud computing
- Big database technologies used in sensors
- The combination of technologies typical of the technological revolution
- Key determinants of the civilization process of industry 4.0
- Discuss some of the concepts of technological management
- Evaluate the creation of internet-based companies in the U.S.
- The most dominant scientific research in the field of artificial intelligence
- Discuss the application of artificial intelligence in the literature
- How enterprises use artificial intelligence in blockchain business operations
- Discuss the various immersive experiences as a result of digital AI
- Elaborate on various enterprise architects and technology innovations
- Mega-trends that are future impacts on business operations
Interesting Topics in AI
- The role of the industrial revolution of the 18 th century in A.I
- The electricity era of the late 19 th century and its contribution to the development of robots
- How the widespread use of the internet contributes to the AI revolution
- The short-term economic crisis as a result of artificial intelligence business technologies
- Designing and creating artificial intelligence production processes
- Analyzing large collections of information for technological solutions
- How biotechnology is transforming the field of agriculture
- Innovative business projects that work using artificial intelligence systems
- Process and marketing innovations in the 21 st century
- Medical intelligence in the era of smart cities
- Advanced data processing technologies in developed nations
- Discuss the development of stelliform technologies
Good Research Topics For AI
- Development of new technological solutions in I.T
- Innovative organizational solutions that develop machine learning
- How to develop branches of a knowledge-based economy
- Discuss the implications of advanced computerized neural network systems
- How to solve complex problems with the help of algorithms
- Why artificial intelligence systems are predominating over their creator
- How to determine artificial emotional intelligence
- Discuss the negative and positive aspects of technological advancement
- How internet technology companies like Facebook are managing large social media portals
- The application of analytical business intelligence systems
- How artificial intelligence improves business management systems
- Strategic and ongoing management of artificial intelligence systems
Graduate AI NLP Research Topics
- Morphological segmentation in artificial intelligence
- Sentiment analysis and breaking machine language
- Discuss input utterance for language interpretation
- Festival speech synthesis system for natural language processing
- Discuss the role of the Google language translator
- Evaluate the various analysis methodologies in N.L.P.
- Native language identification procedure for deep analytics
- Modular audio recognition framework
- Deep linguistic processing techniques
- Fact recognition and extraction techniques
- Dialogue and text-based applications
- Speaker verification and identification systems
Controversial Topics in AI
- Ethical implication of AI in movies: A case study of The Terminator
- Will machines take over the world and enslave humanity?
- Does human intelligence paint a dark future for humanity?
- Ethical and practical issues of artificial intelligence
- The impact of mimicking human cognitive functions
- Why the integration of AI technologies into society should be limited
- Should robots get paid hourly?
- What if AI is a mistake?
- Why did Microsoft shut down chatbots immediately?
- Should there be AI systems for killing?
- Should machines be created to do what they want?
- Is the computerized gun ethical?
Hot AI Topics
- Why predator drones should not exist
- Do the U.S. laws restrict meaningful innovations in AI
- Why did the campaign to stop killer robots fail in the end?
- Fully autonomous weapons and human safety
- How to deal with rogues artificial intelligence systems in the United States
- Is it okay to have a monopoly and control over artificial intelligence innovations?
- Should robots have human rights or citizenship?
- Biases when detecting people’s gender using Artificial intelligence
- Considerations for the adoption of a particular artificial intelligence technology
Are you a university student seeking research paper writing services or dissertation proposal help ? We offer custom help for college students in any field of artificial intelligence.
Leave a Reply Cancel reply
PhD Assistance
Artificial intelligence research topics for phd manuscripts 2021, introduction.
Imagine a world where knowledge isn’t limited to humans!!! A world in which computers will think and collaborate with humans to create a more exciting universe. Although this future is still a long way off, Artificial Intelligence has made significant progress in recent years. In almost every area of AI, such as quantum computing, healthcare, autonomous vehicles, the internet of things, robotics, and so on, there is a lot of research going on. So much so that the number of annual Published Research Papers on Artificial Intelligence has increased by 90% since 1996.
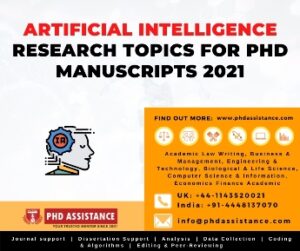
Keeping this in mind, there are several sub-topics on which you can concentrate if you want to study and write a thesis on Artificial Intelligence. This article covers a few of these subjects and provides a short overview. Here some of the recent Research Topics ,
- Artificial Intelligence and Machine learning – Recent Trands
- How AI and ML can aid healthcare systems in their response to COVID-19
- Machine learning and artificial intelligence in haematology
- Tackling the risk of stranded electricity assets with machine learning and artificial intelligence
Deep Learning
Deep Learning is a type of machine learning that learns by simulating the internal workings of the human brain in order to process data and make decisions.Deep Learning is a form of machine learning that employs artificial neural networks. These neural networks are linked in a web-like structure, similar to the human brain’s networks (basically a condensed version of our brain!).
Artificial neural networks have a web-like structure that allows them to process data in a nonlinear manner, which is a major advantage over conventional algorithms that can only process data in a linear manner. Rank Brain, one of the variables in the Google Search algorithm, is an example of a deep neural network.
Recent research topics
- Artificial intelligence & deep learning : PET and SPECT imaging
- Hierarchical Deep Learning Neural Network (HiDeNN): A computational science and engineering in AI architecture.
- AI for surgical safety: automatic assessment of the critical view of safety in laparoscopic cholecystectomy using Deep Learning
- Deep learning-enabled medical computer vision
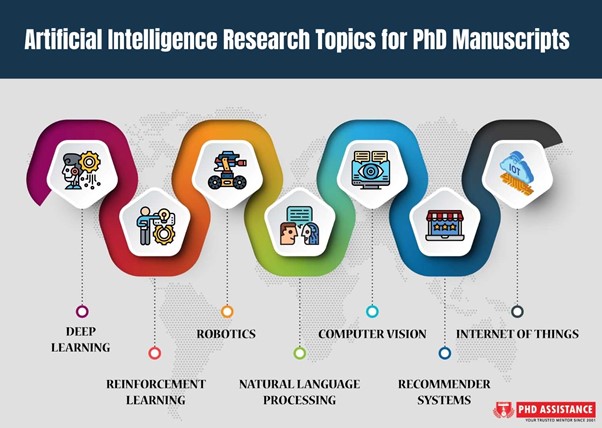
Reinforcement Learning
Reinforcing Learning is an aspect of Artificial Intelligence in which a computer learns something in the same way as humans do. Assume the computer is a student, for example. Over time, the hypothetical student learns from its errors. As a outcome of trial and error, Reinforcement Machine Learning Algorithms learn optimal behaviour.
This means that the algorithm determines the next way to proceed by learning behaviours based on its current state that will increase the reward in the future. This also works for robots, just as it does for humans!
Google’s AlphaGo Computer Programme , for example, used Reinforcement Learning to defeat the world champion in the game of Go (a human!) in 2017.
- Experimental quantum speed-up in reinforcement learning agents
- Potential-based multiobjective reinforcement learning approaches to low-impact agents for AI safety
Robotics is an area concerned with the creation of humanoid robots that can assist humans and perform several acts. In certain cases, robots can behave like humans, but can they think like humans as well?
Kismet, a social interaction robot developed at M.I.T.’s Artificial Intelligence Lab, is an example of this. It understands human body language as well as our voice and responds to them appropriately. Another example is NASA’s Robonaut, which was designed to assist astronauts in space.
- Regulating artificial intelligence and robotics: ethics by design in a digital society
- Regional anaesthesia :usages of artificial intelligence and robotics in
- Third Millennium Life Saving Smart Cyberspace Driven by AI and Robotics
Natural Language Processing
Humans can obviously communicate with each other by speech, but now machines can as well! This is known as Natural Language Processing, and it involves machines analysing and understanding language and expression as it is spoken (which means that if you speak to a computer, it might only respond!). Speech recognition, natural language production, natural language translation, and other aspects of NLP are all concerned with language. NLP is recently very important in customer service applications, particularly chatbots. These chatbots use machine learning and natural language processing to communicate with users in textual form and respond to their questions. As a result, you get a personal touch in your customer service experiences without actually speaking with a human.
Here are several research papers in the field of Natural Language Processing that have been published. You can look at them to get more ideas for research and thesis topics on this subject.
- Natural Language Processing–Based Virtual Cofacilitator for Online Cancer Support Groups: Protocol for an Algorithm Development and Validation Study
- Sympathetic the temporal evolution of COVID-19 Research Through machine learning and natural language processing
Computer Vision
The internet is full of images! This is the selfie age, and taking and posting a photo has never been easier. Each day, millions of images are uploaded to the internet and viewed. It’s important for computers to be able to see and understand images in order to make the most of the vast amount of images available online. And, while humans can do this without thinking about it, computers find it more difficult! This is where Computer Vision enters the image.
To extract information from images, Computer Vision utilizes Artificial Intelligence. This knowledge may include object detection in the image, image content recognition to group images together, and so on. Navigation for autonomous vehicles using images of the surroundings is one use of computer vision, such as AutoNav, which was used in the Spirit and Opportunity rovers that landed on Mars.
- Artificial intelligence for surgical safety: automatic assessment of the critical view of safety in laparoscopic cholecystectomy using deep learning
- An Open‐Source Computer Vision Tool for Automated Vocal Fold Tracking From Video endoscopy
Recommender Systems
Do you get movie and series recommendations from Netflix based on your previous choices or favourite genres? This is achieved by Recommender Systems, which offer you advice about what to do next from the vast array of options available online. Content-based Recommendation or even Collaborative Filtering may be used in a Recommender System.
The content of all the products is analysed in Content-Based Recommendation. For example, based on Natural Language Processing performed on the books, you might be recommended books that you may enjoy. Collaborative Filtering, on the other hand, analyses your past reading behaviour and then recommends books based on it.
- Artificial intelligence in recommender systems
- Deep Transfer Tensor Decomposition with Orthogonal Constraint for Recommender Systems.
- Recommender systems for configuration knowledge engineering
Internet Of Things
Artificial intelligence is concerned with the creation of systems that can learn to perform human-like tasks based on prior experience and without the need for human interaction. The Internet of Things, on the other hand, is a network of different devices linked to the internet and capable of collecting and exchanging data.
All of these IoT devices now generate a large amount of data, which must be collected and mined in order to produce actionable results. Artificial Intelligence enters the picture at this stage. The Internet of Things is used to collect and manage the massive amounts of data that Artificial Intelligence algorithms need. As a consequence, these algorithms transform the data into useful actionable results that IoT devices can use.
- Enhanced Medical Systems by using Artificial Intelligence and Internet of Things
- Artificial Intelligence and Internet of Things in Instrumentation and Control in Waste Biodegradation Plants: Recent Developments
- AIoT-Artificial Intelligence of Things
In this blog discussed the recent enhancement for artificial intelligences and their sub field. This will help to the PhD scholar who are interested to research in artificial intelligences domain.
- Shouval, R., Fein, J. A., Savani, B., Mohty, M., & Nagler, A. (2021). Machine learning and artificial intelligence in haematology. British journal of haematology, 192(2), 239-250.
- van der Schaar, M., Alaa, A. M., Floto, A., Gimson, A., Scholtes, S., Wood, A., … & Ercole, A. (2021). How artificial intelligence and machine learning can help healthcare systems respond to COVID-19. Machine Learning, 110(1), 1-14.
- Nyangon, J. (2021). Tackling the risk of stranded electricity assets with machine learning and artificial intelligence. In Sustainable Energy Investment-Technical, Market and Policy Innovations to Address Risk. IntechOpen.
- Saha, S., Gan, Z., Cheng, L., Gao, J., Kafka, O. L., Xie, X., … & Liu, W. K. (2021). Hierarchical Deep Learning Neural Network (HiDeNN): An artificial intelligence (AI) framework for computational science and engineering. Computer Methods in Applied Mechanics and Engineering, 373, 113452.
- Mascagni, P., Vardazaryan, A., Alapatt, D., Urade, T., Emre, T., Fiorillo, C., … & Padoy, N. (2021). Artificial intelligence for surgical safety: automatic assessment of the critical view of safety in laparoscopic cholecystectomy using deep learning. Annals of Surgery.
- Esteva, A., Chou, K., Yeung, S., Naik, N., Madani, A., Mottaghi, A., … & Socher, R. (2021). Deep learning-enabled medical computer vision. npj Digital Medicine, 4(1), 1-9.
- artificial intelligence phd topics
- artificial intelligence topics for research paper
- computer science research topics for phd
- PhD research topics in artificial intelligence
- recent phd research topics in artificial intelligence
- research topic for artificial intelligence
- research topics in computer science for phd
- research topics in computer vision

Quick Contact
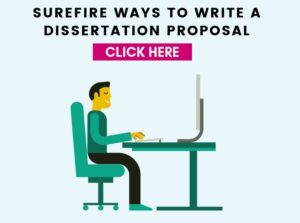
- Adversial Attacks
- Artificial Intelligence
- Artificial Intelligence (AI) and ML ( Machine Learning )
- Big Data Analysis
- Business and Management
- Categories of Research methodology – PhDAssistance
- Category of Research Proposal Services
- coding & algorithm
- Computer Data Science
- Category of Machine Learning – PhDassistance
- Computer Science/Research writing/Manuscript
- Course Work Service
- Data Analytics
- Data Processing
- Deep Networks
- Dissertation Statistics
- economics dissertation
- Editing Services
- Electrical Engineering Category
- Engineering & Technology
- finance dissertation writing
- Gap Identification
- Healthcare Dissertation Writing
- Intrusion-detection-system
- journals publishing
- Life Science Dissertation writing services
- literature review service
- Machine Learning
- medical thesis writing
- Peer review
- PhD Computer Programming
- PhD Dissertation
- Phd Journal Manuscript
- Annotated Bibliography
- PhD Publication Support
- Phd thesis writing services
- Phd Topic Selection
- Categories of PhdAssistance Dissertation
- Power Safety
- problem identification
- Quantitative Analysis
- quantitative research
- Recent Trends
- Referencing and Formatting
- Research Gap
- research journals
- Research Methodology
- research paper
- Research Proposal Service
- secondary Data collection
- Statistical Consulting Services
- Uncategorized
You're viewing this site as a domestic an international student
You're a domestic student if you are:
- a citizen of Australia or New Zealand,
- an Australian permanent resident, or
- a holder of an Australian permanent humanitarian visa.
You're an international student if you are:
- intending to study on a student visa,
- not a citizen of Australia or New Zealand,
- not an Australian permanent resident, or
- a temporary resident (visa status) of Australia.
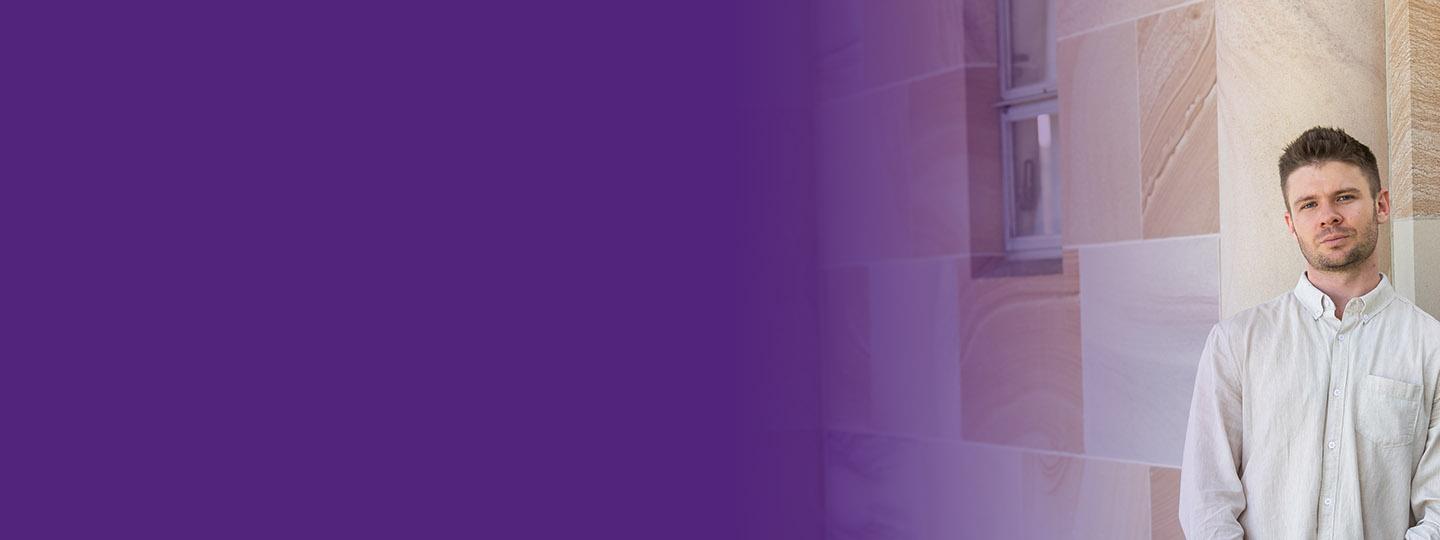
What's it like to do a PhD in artificial intelligence?
UQ people Published 5 Jan, 2023 · 4-minute read
Studying a PhD in artificial intelligence (AI) places you at the forefront of cutting-edge technology.
Artificial intelligence research continues to expand and evolve every day as we appreciate what our future could look like. This type of technology can help solve all kinds of problems, from small-scale, everyday complications to larger-than-life challenges.
An artificial intelligence PhD can kickstart your research career in this fascinating field and open many other doors as you explore new ways to problem-solve using technology.
We talked to UQ Postdoctoral Research Fellow Sam Hislop-Lynch about his experience studying a Doctor of Philosophy and what he hopes his research using artificial intelligence, and more specifically computer vision (CV), will achieve.
Learn more about studying a PhD at UQ .
PhD topics in artificial intelligence
PhD research topics in artificial intelligence can vary dramatically depending on your interests. Whether you choose your own PhD topic or join an existing PhD project , you could find yourself pursuing research in the areas of:
- data management and data science
- computer vision and multimedia computation
- machine learning and data mining
- optimisation and statistics
- human-centred AI.
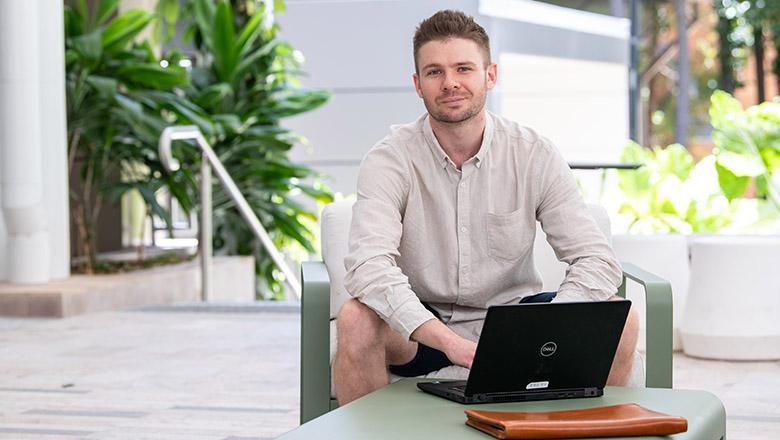
Sam landed on a computer vision PhD topic after working with the technology during his undergrad and postgrad studies. His research focuses on optimising traffic flow.
“Motorways often suffer from flow breakdown around ramps and weaving segments, and with our current technology, it's difficult to investigate this and propose solutions,” he says.
“I developed a suite of algorithms and software to allow researchers and practitioners to fly many drones above a motorway, stitch the imagery together and then extract vehicle trajectories from the footage.”
There are just some things that machines can achieve more efficiently and reliably than humans, and Sam’s research is testament to that.
“Ultimately, the research will reveal strategies that can be used to alleviate or prevent traffic flow breakdowns,” he says.
“This might take the form of better road design guidelines or even real-time strategies for mitigating congestion.”
It’s all about being able to see what’s working and what’s not, and it’s computer vision that allows us to do this.
These findings have a knock-on effect on the environment too. Less traffic congestion and stalling means a reduction in vehicle emissions.
At the end of the day, AI is helping us to better understand how we can use machines efficiently and responsibly, and the research undertaken during a PhD can help us to makes strides in this area.
Is a PhD in artificial intelligence worth it?
So, what are the perks of completing a PhD in AI? According to Sam, it teaches you invaluable skills in problem-solving, which can help open many other doors down the line and expand your professional network.
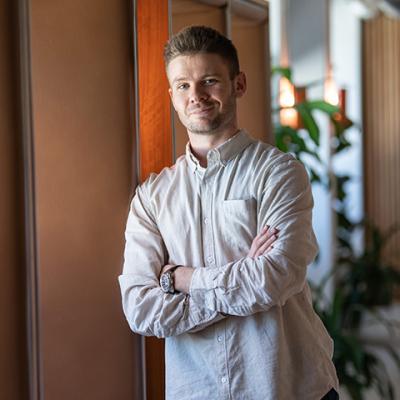
I began working with AI/CV technology because it allowed me to investigate problems that would otherwise be impossible to solve with human pattern recognition alone.
“AI/CV technology allows me to process large amounts of video data with only very little human input.”
Sam recognised that this type of technology was very valuable to several industries outside of transport engineering. The skills he has developed in artificial intelligence and computer vision can be applied in a range of settings and projects, to great effect.
“I’ve leveraged the knowledge that I obtained during my PhD to begin developing software for the mining industry.”
“I intend to pursue this further and expand the scope and number of products I offer,” he says.
Since the completion of his PhD, Sam has branched out into consultancy work while continuing his research. His experience is living proof that a PhD in AI is worth it, if you make the most of the professional opportunities available and think big when it comes to using the skills you’ve learned.
So, what should you consider when deciding whether a PhD in artificial intelligence is the right path for you? Sam encourages you to ask yourself the following:
- Are you interested in the topic?
- Does it align with your long-term goals?
- Where else can this knowledge be applied?
- Can the skills you will learn be used to solve other problems and leverage a better job?

Why study a PhD in artificial intelligence at UQ?
It isn’t just because we have a beautiful campus, state-of-the-art facilities, strong connections with industry leaders and a focus on professional development opportunities. Though all those things certainly help.
Read more about the benefits of studying your PhD at UQ .
Sam explains the specific benefits of studying an artificial intelligence PhD at UQ.
“Apart from the high academic standards and many knowledgeable staff to draw upon, UQ hosts the Wiener supercomputer,” he says.
“This is a purpose-built, bare-metal cluster full of graphical processing units (GPUs) which is well suited to computer vision and AI based research.”
Furthermore, UQ researchers are making strides in several disciplines with the use of AI , including health and medicine, business, society and government, mining, agriculture, defence and energy. Our people are using AI research to protect children , detect cancer and mitigate cyber-attacks .
You too could study a PhD in artificial intelligence at UQ to create positive and lasting change with your research.
Ready to follow your passion for research with a PhD in AI?
Learn more about studying a PhD at UQ
Share this Facebook Twitter LinkedIn Email
Related stories
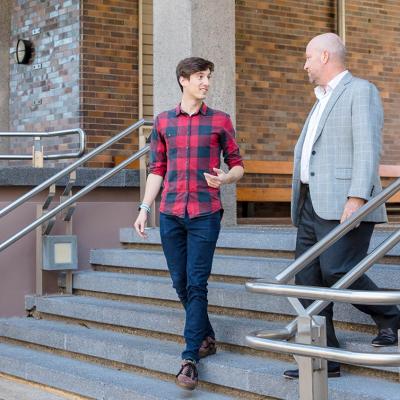
How to get a PhD
4-minute read
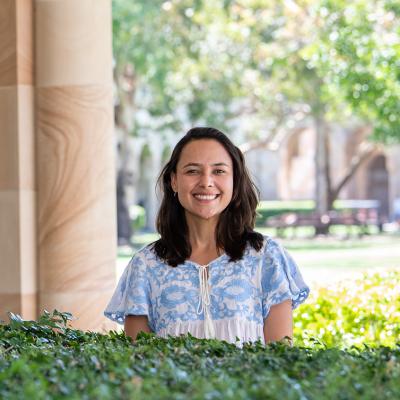
Why do a PhD at UQ?
7-minute read
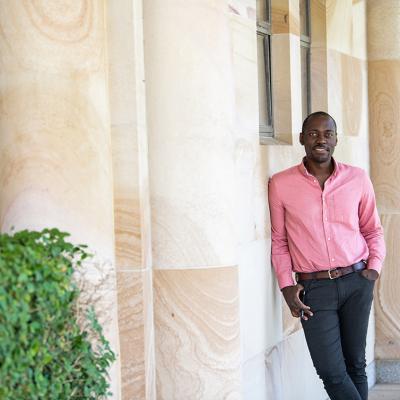
What makes a good PhD supervisor?
9-minute read
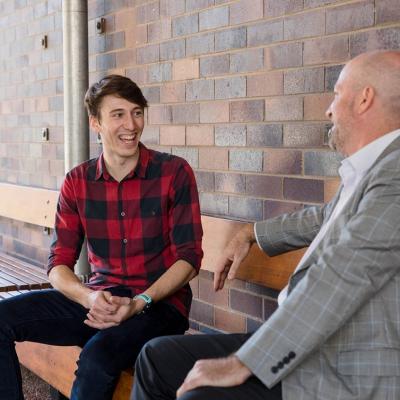
A PhD in renewable energy engineering
3-minute read
- Visits and Open Days
- Jobs and vacancies
- Undergraduate
- Postgraduate
- Accommodation
- Student Guide
- Student email
- Library and IT services
- Staff Guide
- Staff email
- Timetabling
Artificial Intelligence and Data Science Group
Featured story.
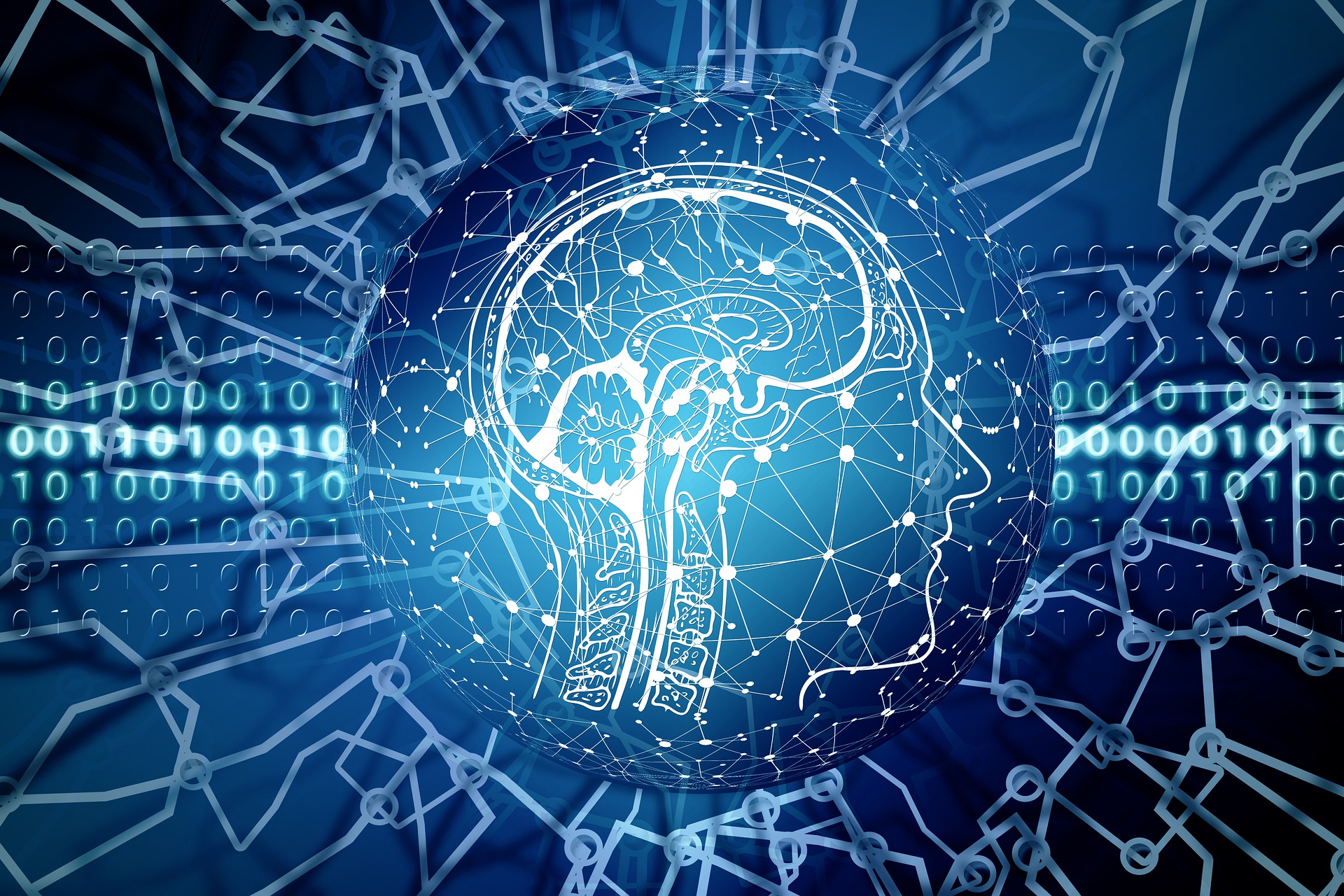
Suggested PhD Projects
Here are some suggested topics for PhD projects from our group members. These projects are merely suggestions and illustrate the broad interests of the research group. Potential research students are encouraged and welcome to produce their own suggestions in these research areas or other research areas in the field. All applicants are invited to contact the academic associated with the project when making an application.
Machine Learning for the Pharmacology of Ageing
Contact: alex freitas.
Recently, there has been a growing interest in ageing research, since the proportion of elderly people in the world’s population is expected to increase substantially in the next few decades. As people live longer, it becomes increasingly more common for a person to suffer from multiple age-related diseases. Since old age is the ultimate cause or the greatest risk factor for most of these diseases, progress in ageing research has the potential to lead to a more cost-effective treatment of many age-related diseases in a holistic fashion. In this context, researchers have collected a significant amount of data about ageing-related genes and medical drugs affecting an organism’s longevity – mainly about simpler model organisms, rather than humans. This data is often freely available on the web, which has facilitated the application of machine learning methods to the pharmacology or biomedicine of ageing, to try to discover some knowledge or patterns in such datasets. This project will focus on developing machine learning algorithms for analysing data about the pharmacology of ageing, i.e., data about medical drugs or chemical compounds that can be used as an intervention against ageing, mainly in model organisms. The broad type of machine learning method to be developed will be supervised machine learning (mainly classification), but the specific type of algorithm to be developed will be decided later, depending on the student’s interest and suitability to the target datasets. Note that, although this is an interdisciplinary project, this is a project for a PhD in Computer Science, so the student will be expected to develop a novel machine learning method. As examples of interdisciplinary papers on machine learning for ageing research, see e.g. (the first paper is particularly relevant for this project, whilst the second includes a broader discussion about machine learning for ageing research):
Relevant References:
D.G. Barardo, D. Newby, D. Thornton, T. Ghafourian, J.P. de Magalhaes and A.A. Freitas. Machine learning for predicting lifespan-extending chemical compounds. Aging (Albany NY), 9(7), 1721-1737, 2017.
Fabris, J.P. de Magalhaes, A.A. Freitas. A review of supervised machine learning applied to ageing research. Biogerontology, 18(2), 171-188, April 2017.
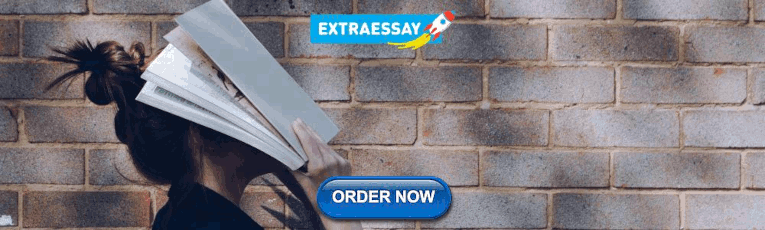
Machine Learning with Fairness-Aware Classification Algorithms
This project involves the classification task of machine learning, where an algorithm has to predict the class of an object (e.g. a customer or a patient) based on properties of that object (e.g. characteristics of a customer or patient). There are now many types of classification algorithms, and in general these algorithms were designed with the only (or main) goal of maximizing predictive performance. As a result, the application of such algorithms to real-world data about people often leads to predictions which have a good predictive accuracy but are unfair, in the sense of discriminating (being biased) against certain groups or types of people – characterized e.g. by values of attributes like gender or ethnicity. In the last few years, however, there has been a considerable amount of research on fairness-aware classification algorithms, which take into account the trade-off between achieving a high predictive accuracy and a high degree of fairness. The project will develop new classification algorithms to cope with this trade-off, focusing on classification algorithms that produce interpretable predictive models, rather than black box models.
[1] Friedler, A.A., Scheidegger, C., Venkatasubramanian, S., Choudhary, S., Hamilton, E.P. and Roth, D. A comparative study of fairness-enhancing interventions in machine learning. Proc. 2nd ACM Conf. on Fairness, Accountability and Transparency (FAT’19), 329-338. ACM Press, 2019.
[2] Mehrabi, N., Morstatter, F., Saxena, N., Lerman, K., & Galstyan, A. A survey on bias and fairness in machine learning. arXiv preprint: arXiv:1908.09635. 2019.
Cognition-enabled lifelong robot learning of behavioural and linguistic experience
Contact: ioanna giorgi.
Present-day cognitive robotics models draw on a hypothesised developmental paradigm of human cognitive functions to devise low-order skills in robots, such as perception, manipulation, navigation and motor coordination. These methods exploit embodied and situated cognition theories that are rooted in motor behaviour and the environment. In other words, the body of a physical artefact (e.g., a robot) and its interactions with the environment and other organisms in it contribute to the robot’s cognition. However, it is not clear how these models can explain or scale up to the high-level cognitive competence observed in human behaviour (e.g., reasoning, categorisation, abstraction and voluntary control). One approach to model robot learning of behavioural and cognitive skills is in incremental and developmental stages that resemble child development. According to child psychology and behaviour, conceptual development starts from perceptual clustering (e.g., prelinguistic infants grouping objects by colour) and progresses to nontrivial abstract thinking, which requires a fair amount of language . Thus, to solve the problem of modelling high-level cognitive skills in robots, language, in interaction with the robot’s body, becomes inseparable from cognition. This project is aimed at following a cognitive and developmental approach to robot learning that will allow robots to acquire behavioural and linguistic skills at a high level of cognitive competence and adaptation as humans. This learning should be lifelong : humans apply earlier-learned skills to make sense of continuous novel stimuli, which allows them to develop, grow and adjust to more complex practices. One such cognitive robot can be used across various themes: human-robot interaction using theory of mind (ToM) skills for robots, social robots and joint human-robot collaboration.
Note: The Cognitive Robotics and Autonomous Systems (CoRAS) laboratory at the School of Computing has access to several humanoids (NAO) and socially interactive robot platforms (Buddy Pro, Q.BO One, Amy A1), mobile robots (Turtlebot Waffle Pi, Burger), pet-like companion robots and gadgets like AR Epson glasses and Microsoft HoloLens.
Attention model for agent social learning during human-robot interaction.
Successful human-robot interaction requires that robots learn by observing and imitating human behaviour. The theory of learning behaviour through observation is referred to as social learning . Behavioural learning can also be enhanced by the environment itself and through reinforcement (i.e., establishing and encouraging a pattern of behaviour). One important component of such learning is cognitive attention , which deals with the degree to which we notice a behaviour. Cognitive attention renders some inputs more relevant while diminishing others, with the motivation that more focus is needed for the important stimuli in the context of social learning. Attention brings forth positive reinforcement (reward) or negative reinforcement (punishment). If the reward is greater than the punishment, behaviour is more likely to be imitated and reciprocated. In human-robot interaction, attention is crucial for two reasons: 1) to respond or reciprocate the behaviour appropriately during the interaction, and 2) to learn or imitate that behaviour for contingencies. This project is aimed at devising a cognitive attention model of a robot for social learning. The model will include memory, reasoning, language and multi-sensory data processing, i.e., “natural” stimuli during the interaction such as from vision, speech and sensorimotor experience. It can be based on a cognitive architecture approach or alternative computational approaches. The solution should ideally be encompassing multiple aspects of interaction (verbal and non-verbal), but it can also focus on such specific aspects (e.g., visual attention or intention reading).
How can a robot learn skills from a human tutor
Contact: giovanni masala.
The aim of this project is to enhance robot learning from a human tutor, similar to a child who learns from a human teacher. The agent will develop the ability to communicate through natural language from scratch, by interacting with a tutor, recognising their verbal and non-verbal inputs as well as emotions, and, finally, grounding the word meaning in the external environment. The project will start from an existing neuro-cognitive architecture under development [1], based on a Human-like approach to learning, progressively incrementing knowledge and language capabilities through experience and ample exposure, using a corpus based on early language lexicons (preschool literature). The robot will integrate with visuospatial information-processing mechanisms for embodied language acquisition, exploiting affective mechanisms of emotion detection for learning and cognition. The agent will be embodied into a humanoid robot as opposed to a computer or a virtual assistant, to enable real-world interactions with the humans and the external environment, to learn and refine its natural language understanding abilities guided or depending on the teacher’s emotions and visual input (object associations with the words, facial expression, and gestures). Emotions will influence the cognitive attention of the robotic agent, modulating the selectivity of attention on specific tasks, words, and objects, and motivating actions and behaviour.
[1] Golosio B, Cangelosi A, Gamotina O, MASALA GL, A Cognitive Neural Architecture Able to Learn and Communicate through Natural Language. PLoS ONE 10(11): e0140866, 2015.
Note: The Cognitive Robotics and Autonomous Systems (CoRAS) laboratory at the School of Computing has access to several humanoids (NAO) and socially interactive robot platforms (Buddy Pro, Q.BO One, Amy A1), mobile robots (Turtlebot Waffle Pi, Burger), pet-like companion robots and devices like AR Epson glasses and Microsoft HoloLens.
Explainability and Interpretability of Machine/Deep learning techniques in medical imaging
In medicine is very important the acceptance of Machine Learning systems not only in terms of performance but also considering the degree to which a human can understand the cause of a decision. Nowadays, the application of Computer Aided Detection Systems in radiology is often based on Deep Learning Systems thanks to their high performance. In general, more accurate models are less explainable and there is a scientific interest in the field of Explainable Artificial Intelligence, to develop new methods that explain and interpret ML models. There is not a concrete mathematical definition for interpretability or explainability, nor have they been measured by some metric; however, a number of attempts have been made in order to clarify not only these two terms but also related concepts such as comprehensibility. A possible target (but other medical diseases are allowed) of this research is a model to discover the severity of Breast Arterial Calcifications. Breast arterial calcification (BAC) is calcium deposition in peripheral arterioles. There is increasing evidence that BAC is a good indicator of the risk of cardiovascular disease. The accurate and automated detection of BACs in mammograms remains an unsolved task and the technology is far from clinical deployment. The challenging task is to develop an explainable model applicable to BAC detection, able to discriminate between severe and weak BACs in patients’ images.
Autonomous car makes me sick
Contact: palaniappan ramaswamy.
With the rapid advancements in autonomous car technology, we will soon see cars driving on their own on the roads. While some may dread this lack of control in fear of safety, generally it is much safe and the real issue lies elsewhere. Do you know that many of us will feel sick – motion sickness will become a huge problem and there is not much ongoing work to mitigate this situation. In this project, we will explore using transcutaneous auricular vagus nerve stimulation (taVNS) as an intervention technology. VNS is a medically approved technology for conditions such as epilepsy. But here we will study the non-invasive version of VNS in mitigating the effects of motion sickness. Functional near infra-red spectroscopy (fNIRS) will be utilised to assess the effect of the taVNS on motion sickness. Some prior signal processing knowledge will be required but knowledge on VNS and fNIRS can be gained from the project.
Stress management
The fundamental aspect of human experience is awareness. Combined with the ability to think, imagine and understand it results into the beautiful cosmic play we experience. However, with it comes along a multitude of problems, often illusory in nature – such as stress, anxiety, anger, negativity, etc. It isn’t hard to guess that in such states our behaviour is significantly altered, usually in harmful ways for both – us and the environment. There are techniques such as meditation, music, humour which can help us come back to our “real” senses and feel happier/peaceful again. So the fundamental enquiry would be about what sort of things do help us achieve a happier state, and moreover what’s their impact on both short term and long term brain functioning. This project will study this aim using EEG.
Information Visualisation Directed by Graph Data Mining
Contact: peter rodgers.
Data visualisation techniques are failing in the face of large data sets. This project attempts to increase the scale of graph data that can be visualised by developing data mining techniques to guide interactive visualisation. This sophisticated combining of information visualisation and data mining promises to greatly improve the size of data understandable by analysts, and will advance the state of the art in both disciplines. On successful completion, publications in high quality venues are envisaged. This project is algorithmically demanding, requiring good coding skills. The implementation language is negotiable, but Java, JavaScript or C++ are all reasonable target languages. Data will be derived from publicly available network intrusion or social network data sets. Tasks in this research project include: (1) implementing graph display software and interface. (2) developing project specific visualisation algorithms. (3) integrating graph pattern matching and other graph data mining systems into the visualisation algorithms.
Visual Analytics for Set Data
Visual Analytics is the process of gaining insights into data through combining AI and information visualization. At present, visual analytics for set based data is largely absent. There are a large number of sources for set based data, including social networks as well as medical and biological information. This project will look at producing set mining algorithms which can then be used to support set visualization methods such as Euler/Venn diagrams or Linear diagrams. Firstly, the use of existing data mining methods will produce useful information about sets and the data instances in them. After this effort, more complex algorithms for subset and set isomorphism will be developed to allow for pattern matching within set data. These set mining methods will be integrated into Euler diagram based exploratory set visualization techniques.
Using Soft Nanomembrane Electronics for Home-based Anxiety Monitoring
Contact: jim ang.
Sensor-enhanced virtual reality systems for mental health care and rehabilitation. New immersive technologies, such as virtual reality (VR) and augmented reality (AR) are playing an increasingly important role in the digital health revolution. Significant research has been carried out at University of Kent, in collaboration with medical scientists/practitioners, psychiatrists/psychologists, digital artists and material scientists (for novel sensor design and integration with VR). Such projects include designing VR for dementia care, eating disorder therapy, eye disorder therapy and VR-enabled brain-machine interactions. This PhD research can take on the following directions: (1) Co-design of VR for a specific healthcare domains, involving key stakeholders (e.g. patient representatives, clinicians, etc) to understand the design and deployment opportunities and challenges in realistic health contexts. (2) Deploy and evaluate VR prototypes to study the impact of the technologies in the target groups. (3) Design and evaluate machine learning algorithms to analyse behavioural and physiological signals for clinical meaningful information, e.g. classification of emotion, detection of eye movement, etc.
Relevant publications:
[1] M Mahmood, S Kwon, H Kim, Y Kim, P Siriaraya, J Choi, B Otkhmezuri, K Kang, KJ Yu, YC Jang, CS Ang, W Yeo (2021) Wireless Soft Scalp Electronics and Virtual Reality System for Motor Imagery ‐ Based Brain–Machine Interfaces. Advanced Science. 8(19).
[2] S Mishra, K Yu, Y Kim, Y Lee, M Mahmood, R Herbert, CS Ang, W Yeo, J Intarasirisawat, Y Kown, H Lim (2020). Soft, wireless periocular wearable electronics for real-time detection of eye vergence in a virtual reality toward mobile eye therapies. Science Advances. 6 (11), eaay1729.
[3] L Tabbaa, CS Ang, V Rose, P Siriaraya, I Stewart, KG Jenkins, M Matsangidou (2019) Bring the Outside In: Providing Accessible Experiences Through VR for People with Dementia in Locked Psychiatric Hospitals, Proceedings of the CHI 2019 Conference on Human Factors in Computing Systems.
[4] M Matsangidou, B Otkhmezuri, CS Ang, M Avraamides, G Riva, A Gaggioli, D Iosif, M Karekla (2020). “Now I can see me” designing a multi-user virtual reality remote psychotherapy for body weight and shape concerns. Human–Computer Interaction. 1-27.
Optimisation of Queries over Virtual Knowledge Graphs
Contact: elena botoeva.
Virtual Knowledge Graphs (also known as Ontology-Based Data Access) provide user-friendly access to Big Data stored in (possibly multiple) data sources, which can be traditional relational ones or more novel ones such as document and triple stores. In this framework an ontology is used as a conceptual representation of the data, and is connected to the data sources by the means of a mapping. User formulates queries over the ontology using a high-level query language like SPARQL; user queries are then automatically translated into queries over the underlying data sources, and the latter are executed by the database engines. Efficiency of the whole approach is highly dependent on optimality of the data source queries. While the technology is quite developed when the underlying data sources are relational, there are still many open problems when it comes to novel data sources, such as MongoDB, graph databases etc. The objective of this PhD project is to design novel techniques for optimising data source queries arising in the context of Virtual Knowledge Graphs.
Heuristics for Scalable Verification of Neural Networks
Due to the success of Deep Learning neural networks are now being employed in a variety of safety-critical applications such as autonomous driving cars and aircraft landing. Despite showing impressive results at various tasks, neural networks are known to be vulnerable (hence, not robust) to adversarial attacks: imperceptible to human eye perturbations to an input can lead to incorrect classification. Robustness verification of neural networks is currently a very hot topic both in academia and industry as neural networks. One of the main challenges in this field is deriving efficient techniques that can verify networks with hundred thousands / millions of neurons in reasonable time, which is not trivial given that exact verification is not tractable (NP- or coNP-complete for ReLU-based neural networks depending on the exact verification problem). Incomplete approaches generally offer better scalability but at the cost of completeness. The aim of the proposed PhD project will be to learn heuristics for efficient verification of neural networks.
Understanding Spiking Neural Networks
Contact: dominique chu.
Spiking Neural Networks (SNN) are brain-like neural networks. Unlike standard rate coding neural networks, signals are encoded in time. This makes them ideal for processing data that has a temporal component, such as time-series data, video or music. Another advantage of SNNs is that there exists neuromorphic hardware that can efficiently simulate SNNs. SNNs are generally thought to be “more powerful” than standard rate coding networks. However, it is not clear precisely in what sense they are more powerful, or what precisely it is that makes them more powerful. The idea of this project is to investigate this claim using a combination of mathematical and computational methods. As such the project will require an interdisciplinary research methodology at the interface between mathematics, computer science and neuroscience. The project would be suitable for a student who wishes to become and expert in an up-and-coming method in artificial intelligence. It has the scope for both theoretical investigations, but will also require implementing neural networks.
Training algorithms for spiking neural networks
Spiking neural networks encode information through the temporal order of the signals. They are more realistic models of the brain than standard artificial neural networks and they are also more efficient in encoding information. Spiking neural networks are therefore very popular in brain simulations. A disadvantage of spiking neural networks is that there are not many efficient training algorithms available. This project will be about finding novel training algorithms for spiking neural networks and to compare the trained networks with standard artificial neural networks on a number of benchmark AI tasks. An important part of this project will be not only to evaluate how well these spiking neural networks perform in relation to standard networks, but also to understand whether or not they are, as is often claimed, more efficient in the sense that they need smaller networks or fewer computing resources. The main approach of the model will be to gain inspiration from existing theories about how the how the human brain develops and learns. These existing theories will then be adapted so as to develop efficient training algorithms. This project will be primarily within AI, but it will also provide the opportunity to learn and apply techniques and ideas from computational neuroscience.
Machine learning systems to improve medical diagnosis
Contact: daniel soria.
Research shows that machine learning methods are extremely useful to discover or identify patterns that can help clinicians to tailor treatments. However, the implementation of those data mining procedures may be challenging because of high dimensional data sets, and the choice of proper machine learning methods may be tricky.
The aim of the research project will be to design and develop new intelligent machine learning systems with high degree of flexibility suitable for disease prediction/diagnosis, that are also easily understandable and explicable to non-experts in the field. Data will be sought from the UK Biobank, to examine whether the selected features are correlated with the occurrence of specific diseases (e.g., breast cancer), whether these relationships persist in the presence of covariates, and the potential role of comorbidities (e.g., obesity, diabetes and cardiovascular diseases) in the assessment of the developed models
How creative are crime-related texts and what does this tell us about cyber crime?
Contact: shujun li , anna jordanous.
The main aim of the PhD project is to investigate if crime-related texts can be evaluated in terms of creativity using automatic metrics. Such a study will help understand how crime-related texts are crafted (by criminals and by automated tools, possibly via a hybrid human-machine teaming approach), how they have evolved over time, how they are perceived by human receivers, and how new methods can be developed to educate people about tactics of cyber criminals. The four tasks of the PhD project will include the following: (1) collecting a large datasets of crime-related texts; (2) developing some objective (automatable) creativity metrics using supervised machine learning, targeted towards evaluating the creativity of crime-related texts (e.g., phishing emails, online hate speech, grooming, cyber bullying, etc.); (3) applying the creativity metrics to the collected data to see how malevolent creativity has evolved over years and for different crimes; (4) exploring the use of generative AI algorithms to create more creative therefore more deceptive crime-related texts.
Computational creativity and automated evaluation
Contact: anna jordanous.
In exploring how computers can perform creative tasks, computational creativity research has produced many systems that can generate creative products or creative activity. Evaluation, a critical part of the creative process, has not been employed to such a great extent within creative systems. Recent work has concentrated on evaluating the creativity of such computational systems, but there are two issues. Firstly, recent work in evaluation of computational creativity has consisted of the system(s) being evaluated by external evaluators, rather than by the creative system evaluating itself, or evaluation by other creative software agents that may interact with that system. Incorporation of self-evaluation into computational creativity systems *as part of guiding the creative process* is also under explored. In this project the candidate will experiment with incorporating evaluation methods into a creative system and analyse the results to explore how computational creativity systems can incorporate self-evaluation. The creative systems studied could be in the area of musical or linguistic creativity, or in a creative area of the student’s choosing. It is up to the student to decide whether to focus on evaluation methods for evaluating the quality of output from a creative system or the creativity of the system itself (or both). The PhD candidate would be required to propose how they would will explore the above scenarios, for a more specific project. Anna is happy to guide students in this and help them develop their research proposal.
Expressive musical performance software
Traditionally, when computational software performs music the performances can be criticised for being too unnatural, lacking interpretation and, in short, being too mechanical. However much progress has been made within the field of expressive musical performance and musical interpretation expression. Alongside these advances have been interesting findings in musical expectation (i.e. what people expect to hear when listening to a piece of music), as well as work on emotions that are present within music and on how information and meaning are conveyed in music. Each of these advances raises questions of how the relevant aspects could be interpreted by a musical performer. Potential application areas for computer systems that can perform music in an appropriately expressive manner include, for example, improving playback in music notation editors (like Sibelius), or the automated performance of music generated on-the-fly for ‘hold’ music (played when waiting on hold during phone calls). Practical work exploring this could involve writing software that performs existing pieces, or could be to write software that can improvise, interpreting incoming sound/music and generating an appropriate sonic/musical response to it in real time.
Brain-like Computer
Contact: frank wang.
The human brain consists of about one billion neurons. Each neuron forms about 1,000 connections to other neurons, amounting to more than a trillion connections. If each neuron could only help store a single memory, running out of space would be a problem. You might have only a few gigabytes of storage space, similar to the space in an iPod or a USB flash drive. Yet neurons combine so that each one helps with many memories at a time, exponentially increasing the brain’s memory storage capacity to something closer to around 2.5 petabytes (or a million gigabytes). The way our brain organizes data may help us manage continuously increasing data, especially in Cloud computing and Big Data. In this project, you are expected to simulate a brain-like computer. Such a computer should be categorised into the unconventional computer group, which is different from traditional Turing machine (with stored programmes) or Von Neumann computer (with an operating system).
My relevant papers: Adaptive Neuromorphic Architecture , Memristor Neural Networks , Grid-Oriented Storage (IEEE Transactions on Computers) .
My relevant keynote talk at Cambridge: Brain and Brain-Inspired Artificial Intelligence )
New Quantum Computer
Contact: frank wang.
Most recently, Frank Wang published an article on Quantum Information Processing (Springer Nature) to report a new quantum computer that can break Landauer’s Bound. Among a number of physical limits to computation, Landauer’s bound limits the minimum amount of energy for a computer to process a bit of information. In light of this study, we may have to presume the demise of this bound despite the many mysteries uncovered with it over the past 60 years.
My relevant papers: Breaking Landauer’s bound in a spin-encoded quantum computer (Springer Nature) , Can We Break the Landauer Bound in Spin-Based Electronics (IEEE Access) .
My relevant keynote talk at Cambridge: A New Quantum Computer Not Bound By Landauer’s Bound )
Thank you for visiting nature.com. You are using a browser version with limited support for CSS. To obtain the best experience, we recommend you use a more up to date browser (or turn off compatibility mode in Internet Explorer). In the meantime, to ensure continued support, we are displaying the site without styles and JavaScript.
- View all journals
- Explore content
- About the journal
- Publish with us
- Sign up for alerts
- NATURE INDEX
- 09 December 2020
Six researchers who are shaping the future of artificial intelligence
- Gemma Conroy ,
- Hepeng Jia ,
- Benjamin Plackett &
You can also search for this author in PubMed Google Scholar
Andy Tay is a science writer in Singapore.
As artificial intelligence (AI) becomes ubiquitous in fields such as medicine, education and security, there are significant ethical and technical challenges to overcome.
CYNTHIA BREAZEAL: Personal touch
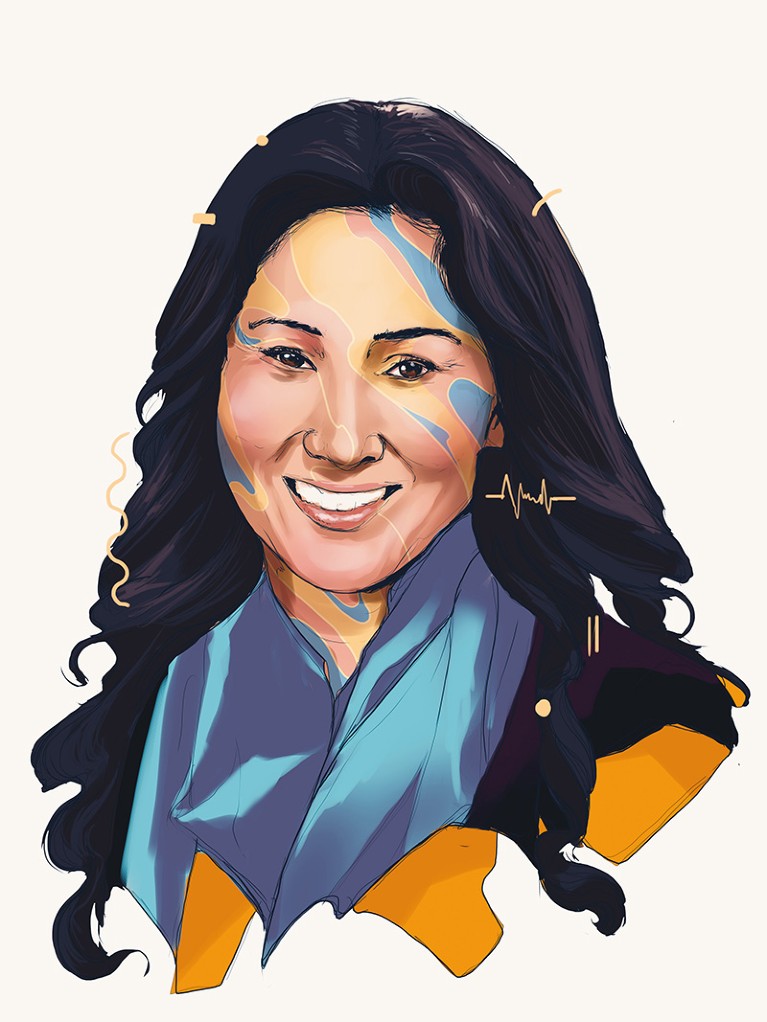
Credit: Taj Francis
While the credits to Star Wars drew to a close in a 1970s cinema, 10-year-old Cynthia Breazeal remained fixated on C-3PO, the anxious robot. “Typically, when you saw robots in science fiction, they were mindless, but in Star Wars they had rich personalities and could form friendships,” says Breazeal, associate director of the Massachusetts Institute of Technology (MIT) Media Lab in Cambridge, Massachusetts. “I assumed these robots would never exist in my lifetime.”
A pioneer of social robotics and human–robot interaction, Breazeal has made a career of conceptualizing and building robots with personality. As a master’s student at MIT’s Humanoid Robotics Group, she created her first robot, an insectile machine named Hannibal that was designed for autonomous planetary exploration and funded by NASA.
Some of the best-known robots Breazeal developed as a young researcher include Kismet, one of the first robots that could demonstrate social and emotional interactions with humans; Cog, a humanoid robot that could track faces and grasp objects; and Leonardo, described by the Institute of Electrical and Electronics Engineers in New Jersey as “one of the most sophisticated social robots ever built”.
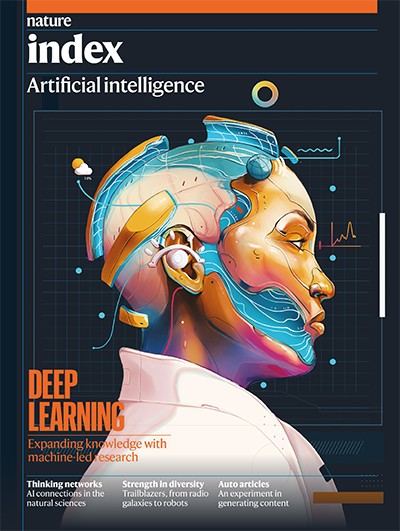
Nature Index 2020 Artificial intelligence
In 2014, Breazeal founded Jibo, a Boston-based company that launched her first consumer product, a household robot companion, also called Jibo. The company raised more than US$70 million and sold more than 6,000 units. In May 2020, NTT Disruption, a subsidiary of London-based telecommunications company, NTT, bought the Jibo technology, and plans to explore the robot’s applications in health care and education.
Breazeal returned to academia full time this year as director of the MIT Personal Robots Group. She is investigating whether robots such as Jibo can help to improve students’ mental health and wellbeing by providing companionship. In a preprint published in July, which has yet to be peer-reviewed, Breazeal’s team reports that daily interactions with Jibo significantly improved the mood of university students ( S. Jeong et al . Preprint at https://arxiv.org/abs/2009.03829; 2020 ). “It’s about finding ways to use robots to help support people,” she says.
In April 2020, Breazeal launched AI Education, a free online resource that teaches children how to design and use AI responsibly. “Our hope is to turn the hundreds of students we’ve started with into tens of thousands in a couple of years,” says Breazeal. — by Benjamin Plackett
CHEN HAO: Big picture
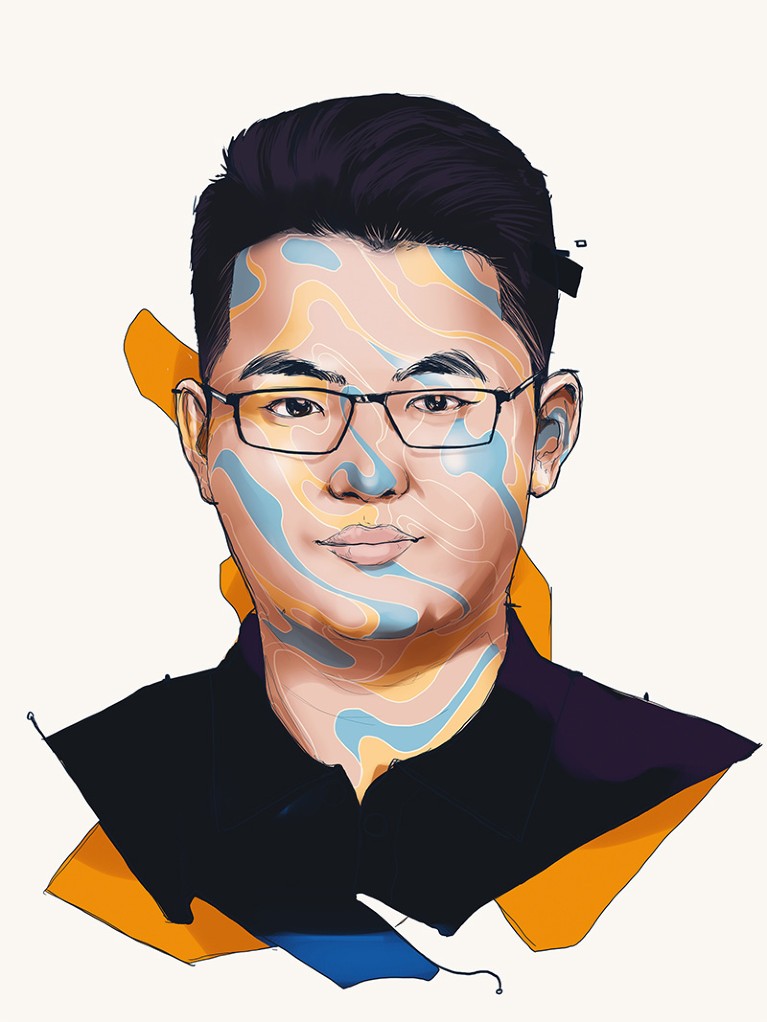
Analysing medical images is an intensive and technical task, and there is a shortage of pathologists and radiologists to meet demands. In a 2018 survey by the UK’s Royal College of Pathologists, just 3% of the National Health Service histopathology departments (which study diseases in tissues) said they had enough staff. A June 2020 report published by the Association of American Medical Colleges found that the United States’ shortage of physician specialists could climb to nearly 42,000 by 2033.
AI systems that can automate part of the process of medical imaging analysis could be the key to easing the burden on specialists. They can reduce tasks that usually take hours or days to seconds, says Chen Hao, founder of Imsight, an AI medical imaging start-up based in Shenzhen, China.
Launched in 2017, Imsight’s products include Lung-Sight, which can automatically detect and locate signs of disease in CT scans, and Breast-Sight, which identifies and measures the metastatic area in a tissue sample. “The analysis allows doctors to make a quick decision based on all of the information available,” says Chen.
Since the outbreak of COVID-19, two of Shenzhen’s largest hospitals have been using Imsight’s imaging technology to analyse subtle changes in patients’ lungs caused by treatment, which enables doctors to identify cases with severe side effects.
In 2019, Chen received the Young Scientist Impact Award from the Medical Image Computing and Computer-Assisted Intervention Society, a non-profit organization in Rochester, Minnesota. The award recognized a paper he led that proposed using a neural network to process fetal ultrasound images ( H. Chen et al. in Medical Image Computing and Computer-Assisted Intervention — MICCAI 2015 (eds N. Navab et al. ) 507–514; Springer, 2015 ). The technique, which has since been adopted in clinical practice in China, reduces the workload of the sonographer.
Despite the rapid advancement of AI’s role in health care, Chen rejects the idea that doctors can be easily replaced. “AI will not replace doctors,” he says. “But doctors who are better able to utilize AI will replace doctors who cannot.” — by Hepeng Jia
ANNA SCAIFE: Star sifting
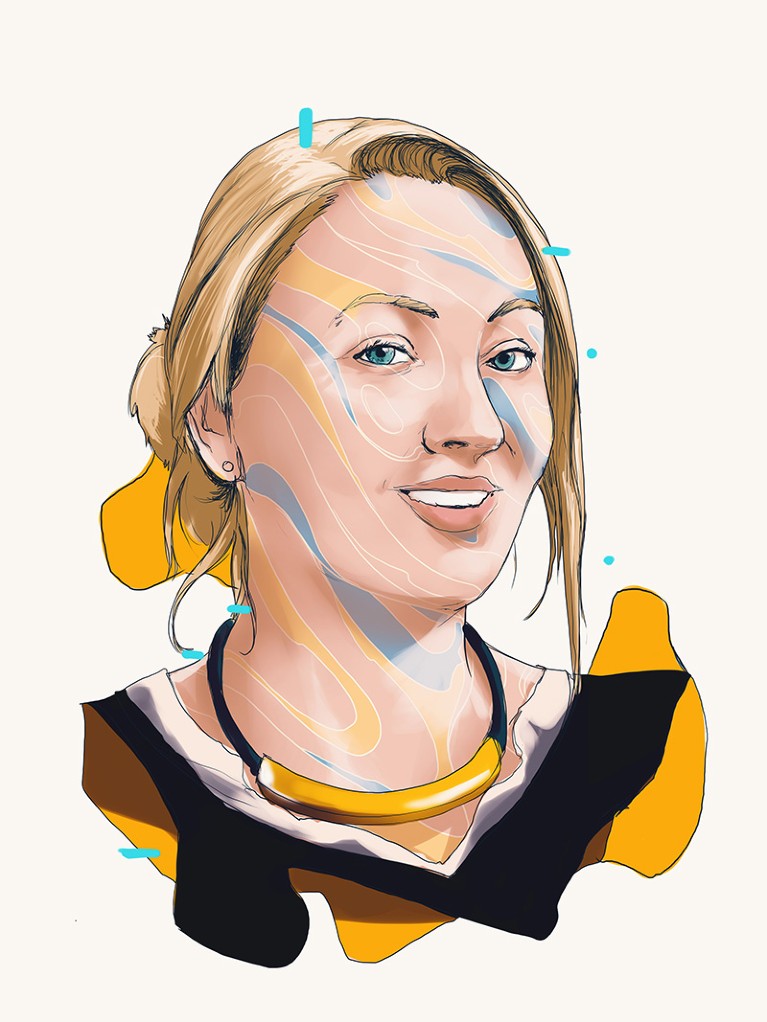
When construction of the Square Kilometre Array (SKA) is complete , it will be the world’s largest radio telescope. With roughly 200 radio dishes in South Africa and 130,000 antennas in Australia expected to be installed by the 2030s, it will produce an enormous amount of raw data, more than current systems can efficiently transmit and process.
Anna Scaife, professor of radio astronomy at the University of Manchester, UK, is building an AI system to automate radio astronomy data processing. Her aim is to reduce manual identification, classification and cataloguing of signals from astronomical objects such as radio galaxies, active galaxies that emit more light at radio wavelengths than at visible wavelengths.
In 2019, Scaife was the recipient of the Jackson-Gwilt Medal, one of the highest honours bestowed by the UK Royal Astronomical Society (RAS). The RAS recognized a study led by Scaife, which outlined data calibration models for Europe’s Low Frequency Array (LOFAR) telescope, the largest radio telescope operating at the lowest frequencies that can be observed from Earth ( A. M. M. Scaife and G. H. Heald Mon. Not. R. Astron. Soc. 423 , L30–L34; 2012 ). The techniques in Scaife’s paper underpin most low-frequency radio observations today.
“It’s a very peculiar feeling to win an RAS medal,” says Scaife. “It’s a mixture of excitement and disbelief, especially because you don’t even know that you were being considered, so you don’t have any opportunity to prepare yourself. Suddenly, your name is on a list that commemorates more than 100 years of astronomy history, and you’ve just got to deal with that.”
Scaife is the academic co-director of Policy@Manchester, the University of Manchester’s policy engagement institute, where she helps researchers to better communicate their findings to policymakers. She also runs a data science training network that involves South African and UK partner universities, with the aim to build a team of researchers to work with the SKA once it comes online. “I hope that the training programmes I have developed can equip young people with skills for the data science sector,” says Scaife. — by Andy Tay
TIMNIT GEBRU: Algorithmic bias
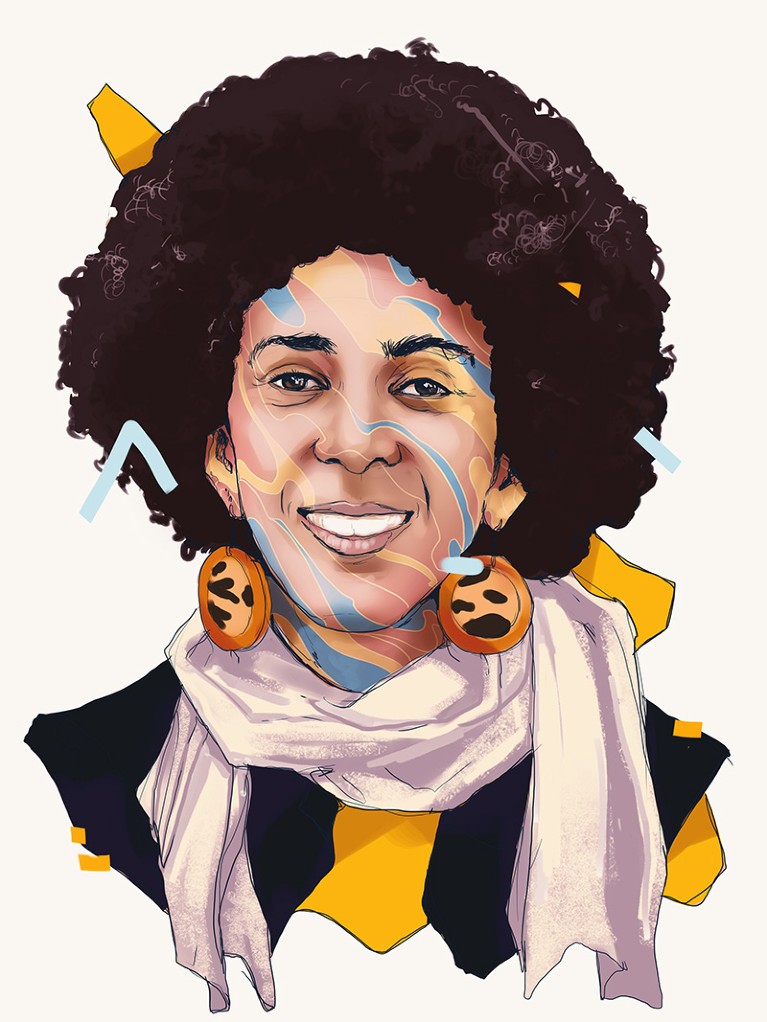
Computer vision is one of the most rapidly developing areas of AI. Algorithms trained to read and interpret images are the foundation of technologies such as self-driving cars, surveillance and augmented reality.
Timnit Gebru, a computer scientist and former co-lead of the Ethical AI Team at Google in Mountain View, California, recognizes the promise of such advances, but is concerned about how they could affect underrepresented communities, particularly people of colour . “My research is about trying to minimize and mitigate the negative impacts of AI,” she says.
In a 2018 study , Gebru and Joy Buolamwini, a computer scientist at the MIT Media Lab, concluded that three commonly used facial analysis algorithms drew overwhelmingly on data obtained from light-skinned people ( J. Buolamwini and T. Gebru. Proc. Mach. Learn. Res. 81 , 77–91; 2018 ). Error rates for dark-skinned females were found to be as high as 34.7% , due to a lack of data, whereas the maximum error rate for light-skinned males was 0.8%. This could result in people with darker skin getting inaccurate medical diagnoses, says Gebru. “If you’re using this technology to detect melanoma from skin photos, for example, then a lot of dark-skinned people could be misdiagnosed.”
Facial recognition used for government surveillance, such as during the Hong Kong protests in 2019, is also highly problematic , says Gebru, because the technology is more likely to misidentify a person with darker skin. “I’m working to have face surveillance banned,” she says. “Even if dark-skinned people were accurately identified, it’s the most marginalized groups that are most subject to surveillance.”
In 2017, as a PhD student at Stanford University in California under the supervision of Li Fei-Fei , Gebru co-founded the non-profit, Black in AI, with Rediet Abebe, a computer scientist at Cornell University in Ithaca, New York. The organization seeks to increase the presence of Black people in AI research by providing mentorship for researchers as they apply to graduate programmes, navigate graduate school, and enter and progress through the postgraduate job market. The organization is also advocating for structural changes within institutions to address bias in hiring and promotion decisions. Its annual workshop calls for papers with at least one Black researcher as the main author or co-author. — by Benjamin Plackett
YUTAKA MATSUO: Internet miner
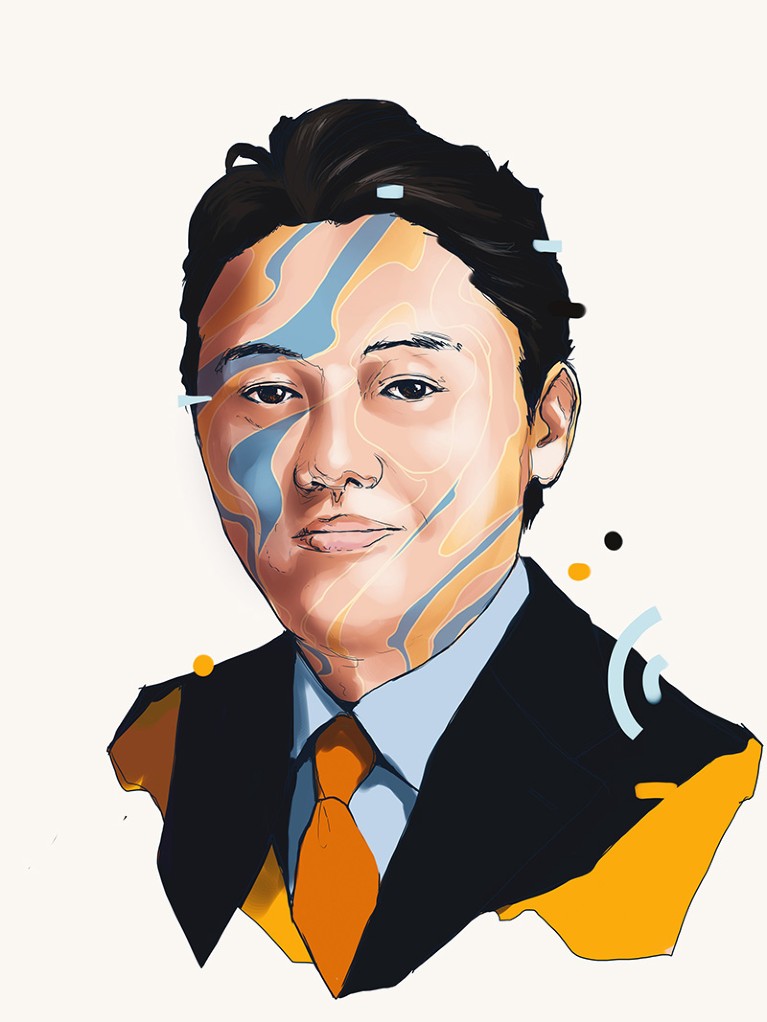
In 2010, Yutaka Matsuo created an algorithm that could detect the first signs of earthquakes by monitoring Twitter for mentions of tremors. His system not only detected 96% of the earthquakes that were registered by the Japan Meteorological Agency (JMA), it also sent e-mail alerts to registered users much faster than announcements could be broadcast by the JMA.
He applied a similar web-mining technique to the stock market. “We were able to classify news articles about companies as either positive or negative,” says Matsuo. “We combined that data to accurately predict profit growth and performance.”
Matsuo’s ability to extract valuable information from what people are saying online has contributed to his reputation as one of Japan’s leading AI researchers. He is a professor at the University of Tokyo’s Department of Technology Management and president of the Japan Deep Learning Association, a non-profit organization that fosters AI researchers and engineers by offering training and certification exams. In 2019, he was the first AI specialist added to the board of Japanese technology giant Softbank.
Over the past decade, Matsuo and his team have been supporting young entrepreneurs in launching internationally successful AI start-ups. “We want to create an ecosystem like Silicon Valley, which Japan just doesn’t have,” he says.
Among the start-ups supported by Matsuo is Neural Pocket, launched in 2018 by Roi Shigematsu, a University of Tokyo graduate. The company analyses photos and videos to provide insights into consumer behaviour.
Matsuo is also an adviser for ReadyFor, one of Japan’s earliest crowd-funding platforms. The company was launched in 2011 by Haruka Mera, who first collaborated with Matsuo as an undergraduate student at Keio University in Tokyo. The platform is raising funds for people affected by the COVID-19 pandemic, and reports that its total transaction value for donations rose by 4,400% between March and April 2020.
Matsuo encourages young researchers who are interested in launching AI start-ups to seek partnerships with industry. “Japanese society is quite conservative,” he says. “If you’re older, you’re more likely to get a large budget from public funds, but I’m 45, and that’s still considered too young.” — by Benjamin Plackett
DACHENG TAO: Machine visionary
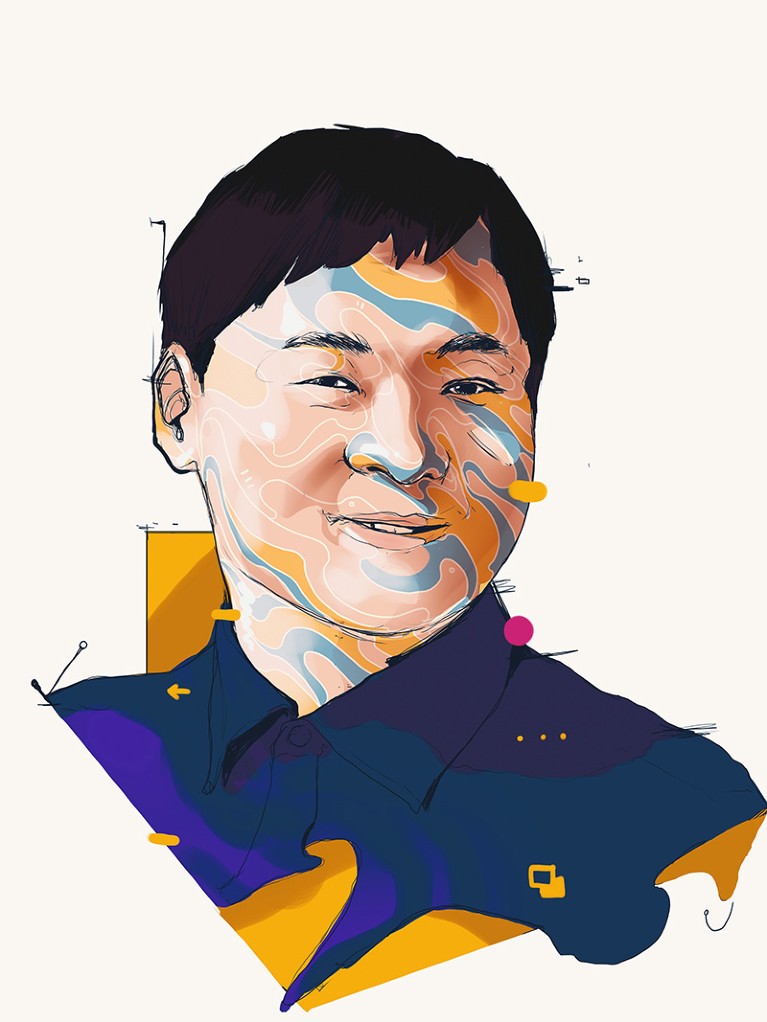
By 2030, an estimated one in ten cars globally will be self-driving. The key to getting these autonomous vehicles on the road is designing computer-vision systems that can identify obstacles to avoid accidents at least as effectively as a human driver .
Neural networks, sets of AI algorithms inspired by neurological processes that fire in the human cerebral cortex, form the ‘brains’ of self-driving cars. Dacheng Tao, a computer scientist at the University of Sydney, Australia, designs neural networks for computer-vision tasks. He is also building models and algorithms that can process videos captured by moving cameras, such as those in self-driving cars.
“Neural networks are very useful for modelling the world,” says Tao, director of the UBTECH Sydney Artificial Intelligence Centre, a partnership between the University of Sydney and global robotics company UBTECH.
In 2017, Tao was awarded an Australian Laureate Fellowship for a five-year project that uses deep-learning techniques to improve moving-camera computer vision in autonomous machines and vehicles. A subset of machine learning, deep learning uses neural networks to build systems that can ‘learn’ through their own data processing.
Since launching in 2018, Tao’s project has resulted in more than 40 journal publications and conference papers. He is among the most prolific researchers in AI research output from 2015 to 2019, as tracked by the Dimensions database, and is one of Australia’s most highly cited computer scientists. Since 2015, Tao’s papers have amassed more than 42,500 citations, as indexed by Google Scholar. In November 2020, he won the Eureka Prize for Excellence in Data Science, awarded by the Australian Museum.
In 2019, Tao and his team trained a neural network to construct 3D environments using a motion-blurred image, such as would be captured by a moving car. Details, including the motion, blurring effect and depth at which it was taken, helped the researchers to recover what they describe as “the 3D world hidden under the blurs”. The findings could help self-driving cars to better process their surroundings. — by Gemma Conroy
Nature 588 , S114-S117 (2020)
doi: https://doi.org/10.1038/d41586-020-03411-0
This article is part of Nature Index 2020 Artificial intelligence , an editorially independent supplement. Advertisers have no influence over the content.
Related Articles
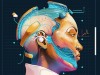
Partner content: Advancing precision medicine using AI and big data
Partner content: Using AI to accelerate drug discovery
Partner content: Using AI to make healthcare more human
Partner content: Strengthening links in the discovery chain
Partner content: LMU Munich harnesses AI to drive discovery
Partner content: Breaking AI out of the computer science bubble
Partner content: Discovering a theory to visualize the world
Partner content: Supporting the technology game-changers
Partner content: Data-driven AI illuminates the future
Partner content: The humans at the heart of AI
Partner content: New reach for computer imaging
Partner content: Building natural trust in artificial intelligence
Partner content: Raising a global centre for deep learning
Partner content: Japan’s new centre of gravity for clinical data science
Partner content: AI researchers wanted in Germany
- Computer science
- Institutions
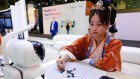
AI now beats humans at basic tasks — new benchmarks are needed, says major report
News 15 APR 24
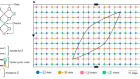
High-threshold and low-overhead fault-tolerant quantum memory
Article 27 MAR 24
Three reasons why AI doesn’t model human language
Correspondence 19 MAR 24
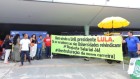
Dozens of Brazilian universities hit by strikes over academic wages
News 08 MAY 24
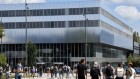
France’s research mega-campus faces leadership crisis
News 03 MAY 24
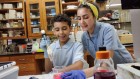
US National Academies report outlines barriers and solutions for scientist carers
Career News 02 MAY 24
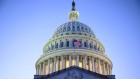
The US Congress is taking on AI —this computer scientist is helping
News Q&A 09 MAY 24
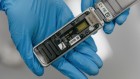
Powerful ‘nanopore’ DNA sequencing method tackles proteins too
Technology Feature 08 MAY 24
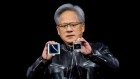
Who’s making chips for AI? Chinese manufacturers lag behind US tech giants
Clinician Researcher/Group Leader in Cancer Cell Therapies
An excellent opportunity is available for a Group Leader with expertise in cellular therapies to join the Cancer Research program at QIMR Berghofer.
Herston, Brisbane (AU)
QIMR Berghofer
Faculty Positions at the Center for Machine Learning Research (CMLR), Peking University
CMLR's goal is to advance machine learning-related research across a wide range of disciplines.
Beijing, China
Center for Machine Learning Research (CMLR), Peking University
Faculty Positions at SUSTech Department of Biomedical Engineering
We seek outstanding applicants for full-time tenure-track/tenured faculty positions. Positions are available for both junior and senior-level.
Shenzhen, Guangdong, China
Southern University of Science and Technology (Biomedical Engineering)
Southeast University Future Technology Institute Recruitment Notice
Professor openings in mechanical engineering, control science and engineering, and integrating emerging interdisciplinary majors
Nanjing, Jiangsu (CN)
Southeast University
Staff Scientist
A Staff Scientist position is available in the laboratory of Drs. Elliot and Glassberg to study translational aspects of lung injury, repair and fibro
Maywood, Illinois
Loyola University Chicago - Department of Medicine
Sign up for the Nature Briefing newsletter — what matters in science, free to your inbox daily.
Quick links
- Explore articles by subject
- Guide to authors
- Editorial policies
Library Research Guides - University of Wisconsin Ebling Library
Uw-madison libraries research guides.
- Course Guides
- Subject Guides
- University of Wisconsin-Madison
- Research Guides
- Artificial Intelligence (AI) in the Academic Health Sciences
Artificial Intelligence (AI) in the Academic Health Sciences : Overview
Introduction, ai limitations.
- Types of Generative AI
- Prompt Writing
- AI in Teaching and Learning
- AI in Research

Email: [email protected] Phone: 608-262-2020

Below are some important terms for understanding generative AI:
- " Generative AI: Technology that creates content — including text, images, video and computer code — by identifying patterns in large quantities of training data, and then creating original material that has similar characteristics. Examples include ChatGPT for text and DALL-E and Midjourney for images."
- " Hallucination: A well-known phenomenon in large language models, in which the system provides an answer that is factually incorrect, irrelevant or nonsensical, because of limitations in its training data and architecture."
- " Large language model: A type of neural network that learns skills — including generating prose, conducting conversations and writing computer code — by analyzing vast amounts of text from across the internet. The basic function is to predict the next word in a sequence, but these models have surprised experts by learning new abilities."
- " Natural language processing: Techniques used by large language models to understand and generate human language, including text classification and sentiment analysis. These methods often use a combination of machine learning algorithms, statistical models and linguistic rules."
- " Neural network: A mathematical system, modeled on the human brain, that learns skills by finding statistical patterns in data. It consists of layers of artificial neurons: The first layer receives the input data, and the last layer outputs the results. Even the experts who create neural networks don’t always understand what happens in between."
All definitions from:
Pasick, A. (2023, March 27). Artificial Intelligence Glossary: Neural Networks and Other Terms Explained. The New York Times . https://www.nytimes.com/article/ai-artificial-intelligence-glossary.html
Generative AI is an emerging topic in academic health sciences research. It is important to understand some of the limitations and ethical considerations in using AI for this purpose. Here are a few examples of those limitations:
- Bias : The data used to train Generative AI models can contain bias that is then reflected or perpetuated in the outputs.
- Data Privacy: Data may be collected and used in ways that aren't transparent or disclosed to the user. This is why UW-Madison restricts institutional data from being entered into most generative AI products or services.
- Copyrigh t: The use of AI in research projects raises issues of authorship, in addition to intellectual property right concerns of publishers and creators of the content contained in the training data.
- Currency/Content: Generative AI is limited to the resources it has access to and how up to date that information is. Sources behind paywalls or firewalls are generally not accessible, thus impacting the quality of answers given by the AI model.
- Reproducibility: The same search prompt can yield differing results, depending on the user.
- Inaccuracy: Generative AI works on predicting what the user is looking for. This can lead to inaccurant answers and data hallucinations, including invented references and citations.
For information on ethics and generative AI, see the UW-Madison Generative AI guide .
- Generative AI guide (UW-Madison)
- Writing in the age of AI (UW-Madison)
- Artificial Intelligence (AI): an introduction to generative AI and its use in teaching, research, and publishing (Rutgers)
- AI Tools for Searching (St. Louis University Medical Center Library)
- Next: Types of Generative AI >>
- Last Updated: May 9, 2024 12:11 PM
- URL: https://researchguides.library.wisc.edu/AI-academic-health-sciences
- Data Science
- Data Analysis
- Data Visualization
- Machine Learning
- Deep Learning
- Computer Vision
- Artificial Intelligence
- AI ML DS Interview Series
- AI ML DS Projects series
- Data Engineering
- Web Scrapping
8 Best Topics for Research and Thesis in Artificial Intelligence
- Top 5 Artificial Intelligence(AI) Predictions in 2020
- Top 7 Artificial Intelligence Frameworks to Learn in 2022
- What Are The Ethical Problems in Artificial Intelligence?
- Top 7 Artificial Intelligence and Machine Learning Trends For 2022
- The State of Artificial Intelligence in India and How Far is Too Far?
- 5 Dangers of Artificial Intelligence in the Future
- Top Challenges for Artificial Intelligence in 2020
- Difference Between Machine Learning and Artificial Intelligence
- What is Artificial Intelligence as a Service (AIaaS) in the Tech Industry?
- 10 Best Artificial Intelligence Project Ideas To Kick-Start Your Career
- Artificial Intelligence (AI) Researcher Jobs in China
- Applied Artificial Intelligence in Estonia : A global springboard for startups
- Types of Reasoning in Artificial Intelligence
- Mapping Techniques in Artificial Intelligence and Robotics
- Top 15 Artificial Intelligence(AI) Tools List
- Artificial Intelligence in Robotics
- Top Data Science with Artificial Intelligence Colleges in India
- Difference Between Data Science and Artificial Intelligence
- Difference Between Artificial Intelligence and Human Intelligence
Imagine a future in which intelligence is not restricted to humans!!! A future where machines can think as well as humans and work with them to create an even more exciting universe. While this future is still far away, Artificial Intelligence has still made a lot of advancement in these times. There is a lot of research being conducted in almost all fields of AI like Quantum Computing, Healthcare, Autonomous Vehicles, Internet of Things , Robotics , etc. So much so that there is an increase of 90% in the number of annually published research papers on Artificial Intelligence since 1996. Keeping this in mind, if you want to research and write a thesis based on Artificial Intelligence, there are many sub-topics that you can focus on. Some of these topics along with a brief introduction are provided in this article. We have also mentioned some published research papers related to each of these topics so that you can better understand the research process.
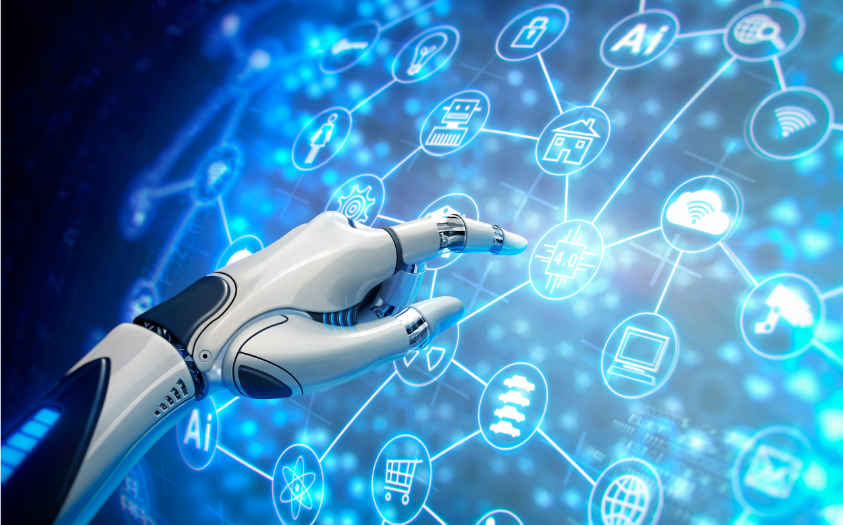
So without further ado, let’s see the different Topics for Research and Thesis in Artificial Intelligence!
1. Machine Learning
Machine Learning involves the use of Artificial Intelligence to enable machines to learn a task from experience without programming them specifically about that task. (In short, Machines learn automatically without human hand holding!!!) This process starts with feeding them good quality data and then training the machines by building various machine learning models using the data and different algorithms. The choice of algorithms depends on what type of data do we have and what kind of task we are trying to automate. However, generally speaking, Machine Learning Algorithms are divided into 3 types i.e. Supervised Machine Learning Algorithms, Unsupervised Machine Learning Algorithms , and Reinforcement Machine Learning Algorithms.
2. Deep Learning
Deep Learning is a subset of Machine Learning that learns by imitating the inner working of the human brain in order to process data and implement decisions based on that data. Basically, Deep Learning uses artificial neural networks to implement machine learning. These neural networks are connected in a web-like structure like the networks in the human brain (Basically a simplified version of our brain!). This web-like structure of artificial neural networks means that they are able to process data in a nonlinear approach which is a significant advantage over traditional algorithms that can only process data in a linear approach. An example of a deep neural network is RankBrain which is one of the factors in the Google Search algorithm.
3. Reinforcement Learning
Reinforcement Learning is a part of Artificial Intelligence in which the machine learns something in a way that is similar to how humans learn. As an example, assume that the machine is a student. Here the hypothetical student learns from its own mistakes over time (like we had to!!). So the Reinforcement Machine Learning Algorithms learn optimal actions through trial and error. This means that the algorithm decides the next action by learning behaviors that are based on its current state and that will maximize the reward in the future. And like humans, this works for machines as well! For example, Google’s AlphaGo computer program was able to beat the world champion in the game of Go (that’s a human!) in 2017 using Reinforcement Learning.
4. Robotics
Robotics is a field that deals with creating humanoid machines that can behave like humans and perform some actions like human beings. Now, robots can act like humans in certain situations but can they think like humans as well? This is where artificial intelligence comes in! AI allows robots to act intelligently in certain situations. These robots may be able to solve problems in a limited sphere or even learn in controlled environments. An example of this is Kismet , which is a social interaction robot developed at M.I.T’s Artificial Intelligence Lab. It recognizes the human body language and also our voice and interacts with humans accordingly. Another example is Robonaut , which was developed by NASA to work alongside the astronauts in space.
5. Natural Language Processing
It’s obvious that humans can converse with each other using speech but now machines can too! This is known as Natural Language Processing where machines analyze and understand language and speech as it is spoken (Now if you talk to a machine it may just talk back!). There are many subparts of NLP that deal with language such as speech recognition, natural language generation, natural language translation , etc. NLP is currently extremely popular for customer support applications, particularly the chatbot . These chatbots use ML and NLP to interact with the users in textual form and solve their queries. So you get the human touch in your customer support interactions without ever directly interacting with a human.
Some Research Papers published in the field of Natural Language Processing are provided here. You can study them to get more ideas about research and thesis on this topic.
6. Computer Vision
The internet is full of images! This is the selfie age, where taking an image and sharing it has never been easier. In fact, millions of images are uploaded and viewed every day on the internet. To make the most use of this huge amount of images online, it’s important that computers can see and understand images. And while humans can do this easily without a thought, it’s not so easy for computers! This is where Computer Vision comes in. Computer Vision uses Artificial Intelligence to extract information from images. This information can be object detection in the image, identification of image content to group various images together, etc. An application of computer vision is navigation for autonomous vehicles by analyzing images of surroundings such as AutoNav used in the Spirit and Opportunity rovers which landed on Mars.
7. Recommender Systems
When you are using Netflix, do you get a recommendation of movies and series based on your past choices or genres you like? This is done by Recommender Systems that provide you some guidance on what to choose next among the vast choices available online. A Recommender System can be based on Content-based Recommendation or even Collaborative Filtering. Content-Based Recommendation is done by analyzing the content of all the items. For example, you can be recommended books you might like based on Natural Language Processing done on the books. On the other hand, Collaborative Filtering is done by analyzing your past reading behavior and then recommending books based on that.
8. Internet of Things
Artificial Intelligence deals with the creation of systems that can learn to emulate human tasks using their prior experience and without any manual intervention. Internet of Things , on the other hand, is a network of various devices that are connected over the internet and they can collect and exchange data with each other. Now, all these IoT devices generate a lot of data that needs to be collected and mined for actionable results. This is where Artificial Intelligence comes into the picture. Internet of Things is used to collect and handle the huge amount of data that is required by the Artificial Intelligence algorithms. In turn, these algorithms convert the data into useful actionable results that can be implemented by the IoT devices.
Please Login to comment...
Similar reads.
- AI-ML-DS Blogs
Improve your Coding Skills with Practice
What kind of Experience do you want to share?
- Prospective students
- International students
- PhD candidates
- Professors and researchers
- Institutions and companies
- Academic Board
- Career opportunities
- Research topics
Foundational research topics in artificial intelligence
- Machine Learning: Developing algorithms and models that enable systems to learn from data, make predictions, and improve performance over time.
- Natural Language Processing (NLP): Analyzing, understanding, and generating human language to enable effective communication between humans and machines.
- Computer Vision: Enabling machines to interpret and understand visual information from images or videos, including object recognition, image classification, and scene understanding.
- Robotics and Autonomous Systems: Designing intelligent robots and systems capable of perceiving and interacting with their environment, making decisions, and performing tasks autonomously.
- Knowledge Representation and Reasoning: Developing methods to represent and organize knowledge in a structured form, allowing machines to reason, infer, and make intelligent decisions.
- Planning and Scheduling: Creating algorithms and techniques to generate optimal or near-optimal plans and schedules for complex tasks or processes.
- Expert Systems: Building AI systems that mimic human expertise in specific domains, enabling intelligent decision-making and problem-solving.
- Data Mining and Big Data Analytics: Extracting valuable insights, patterns, and knowledge from large and complex datasets, enabling informed decision-making.
- Intelligent Agents: Designing autonomous entities that can perceive their environment, learn from interactions, and make decisions to achieve specific goals.
- Cognitive Computing: Developing systems that simulate human thought processes, including perception, reasoning, learning, and problem-solving.
- Deep Learning: Advancing neural network architectures and algorithms to enable machines to learn and represent complex patterns and relationships in data.
- Reinforcement Learning: Training agents to make sequential decisions through interactions with an environment, optimizing long-term rewards.
- Ethical and Responsible AI: Investigating the societal impact of AI, ensuring fairness, transparency, privacy, and accountability in AI systems.
- Human-AI Interaction: Designing interfaces and interaction techniques that facilitate seamless collaboration and communication between humans and AI systems.
- Explainable AI: Development of AI systems and models that can provide transparent and interpretable explanations for their decisions and actions, enabling users to understand the underlying factors and logic behind AI-generated outcomes.
- Trustworthy AI: Design, development, and deployment of AI systems that are reliable, ethical, transparent, and accountable, ensuring that they operate in a manner that aligns with human values, respects privacy, and avoids biases and discrimination.
Our cookies
We use cookies for three reasons: to give you the best experience on PGS, to make sure the PGS ads you see on other sites are relevant , and to measure website usage. Some of these cookies are necessary to help the site work properly and can’t be switched off. Cookies also support us to provide our services for free, and by click on “Accept” below, you are agreeing to our use of cookies .You can manage your preferences now or at any time.
Privacy overview
We use cookies, which are small text files placed on your computer, to allow the site to work for you, improve your user experience, to provide us with information about how our site is used, and to deliver personalised ads which help fund our work and deliver our service to you for free.
The information does not usually directly identify you, but it can give you a more personalised web experience.
You can accept all, or else manage cookies individually. However, blocking some types of cookies may affect your experience of the site and the services we are able to offer.
You can change your cookies preference at any time by visiting our Cookies Notice page. Please remember to clear your browsing data and cookies when you change your cookies preferences. This will remove all cookies previously placed on your browser.
For more detailed information about the cookies we use, or how to clear your browser cookies data see our Cookies Notice
Manage consent preferences
Strictly necessary cookies
These cookies are necessary for the website to function and cannot be switched off in our systems.
They are essential for you to browse the website and use its features.
You can set your browser to block or alert you about these cookies, but some parts of the site will not then work. We can’t identify you from these cookies.
Functional cookies
These help us personalise our sites for you by remembering your preferences and settings. They may be set by us or by third party providers, whose services we have added to our pages. If you do not allow these cookies, then these services may not function properly.
Performance cookies
These cookies allow us to count visits and see where our traffic comes from, so we can measure and improve the performance of our site. They help us to know which pages are popular and see how visitors move around the site. The cookies cannot directly identify any individual users.
If you do not allow these cookies we will not know when you have visited our site and will not be able to improve its performance for you.
Marketing cookies
These cookies may be set through our site by social media services or our advertising partners. Social media cookies enable you to share our content with your friends and networks. They can track your browser across other sites and build up a profile of your interests. If you do not allow these cookies you may not be able to see or use the content sharing tools.
Advertising cookies may be used to build a profile of your interests and show you relevant adverts on other sites. They do not store directly personal information, but work by uniquely identifying your browser and internet device. If you do not allow these cookies, you will still see ads, but they won’t be tailored to your interests.
Course type
Qualification, university name, phd degrees in artificial intelligence (ai).
16 degrees at 13 universities in the UK.
Customise your search
Select the start date, qualification, and how you want to study
About Postgraduate Artificial Intelligence (AI)
Artificial Intelligence (AI) is a branch of computer science focused on creating machines that can perform tasks with a simulated human intelligence. AI builds computers which can learn, reason, problem-solve and understand natural language. It is a relatively new and rapidly advancing field with a huge range of practical applications from speech recognition and image processing to autonomous vehicles and virtual assistants.
Currently there are 15 artificial intelligencePhD programmes offered at UK universities and entry requirements typically include a strong background in computer science, software engineering or a related field, along with a well-constructed research proposal, which should address an important or underdeveloped aspect of AI and will form the basis for your PhD studies.
The PhD course itself will have a duration of around three to six years and will primarily be centered around your research proposal which you’ll develop under the supervision of an academic tutor.
What to Expect
For a PhD, you can expect to be doing a lot of self-driven study. You may be part of a research team or a member of a laboratory or engineering workshop, but a significant amount of your time will still be spent researching material for your thesis and developing your project. AI is a highly versatile field and you might find yourself working in machine learning, robotics, natural language processing, computational intelligence, or even the ethics behind striving to create intelligent machines and what effects this might have on human society.
You’ll present your research periodically; however, the main assessment is your PhD dissertation, which after submitting, you will be required to defend orally in front of a panel of academics. Once this is complete, you’ll be qualified as a Doctor of Philosophy in artificial intelligence and will be ready for roles in AI research, data science, industry or academia.

Related subjects:
- PhD Artificial Intelligence (AI)
- PhD Animation Software
- PhD Bioinformatics
- PhD Business Information Systems
- PhD Computer Animation
- PhD Computer Architectures
- PhD Computer Communications and Networking
- PhD Computer Cybernetics
- PhD Computer Games Design
- PhD Computer Graphics
- PhD Computer Network Components
- PhD Computer Science and Information Technology
- PhD Computer Security Systems
- PhD Computer Systems
- PhD Computing Methodologies
- PhD Data Science
- PhD Expert Systems
- PhD Geographical Information Systems Software
- PhD Graphics And Multimedia Software
- PhD Health Informatics
- PhD Human Computer Interface Development
- PhD Image Processing
- PhD Informatics
- PhD Information Management
- PhD Information Security
- PhD Information Systems
- PhD Information Technology
- PhD Information Work and Information Use
- PhD Internet Security Systems
- PhD Internet Systems
- PhD Knowledge Management Systems
- PhD Librarianship and Library Management
- PhD Libraries and Librarianship
- PhD Mobile Computing
- PhD Modelling and Simulation Systems
- PhD Multimedia
- PhD Network Systems Management
- PhD Network Systems Management Software
- PhD Pattern Recognition
- PhD Software Development
- PhD Software Engineering
- PhD Software Testing
- PhD Software for Specific Subjects and Industries
- PhD Systems Analysis and Design
- PhD Using Software

- Course title (A-Z)
- Course title (Z-A)
- Price: high - low
- Price: low - high
Artificial Intelligence and Intelligent Agents PhD
Bangor university.
Research topics include knowledge-based systems, logic, multi-agent systems, distributed systems, machine learning, data mining, Read more...
- 3 years Full time degree: £4,712 per year (UK)
Computer Science PhD, MPhil - Knowledge Discovery and Machine Learning
University of leicester.
Computing at Leicester offers supervision for the degrees of Doctor of Philosophy (PhD) - full-time and part-time Master of Philosophy Read more...
- 3 years Full time degree: £4,786 per year (UK)
- 6 years Part time degree: £2,393 per year (UK)
PhD Robotics and Systems Engineering
University of salford.
INTRODUCTION Automation for the Food Industry Research The food industry is very labour intensive and as a result is under threat from Read more...
- 3 years Full time degree: £4,780 per year (UK)
- 5 years Part time degree: £2,390 per year (UK)
Robotics and Autonomous Systems PhD
University of surrey.
Why choose this programme On our Robotics and Autonomous Systems PhD, you’ll study, design and build novel solutions and behaviours for Read more...
- 4 years Full time degree: £4,712 per year (UK)
- 8 years Part time degree: £2,356 per year (UK)
Artificial Intelligence Enabled Healthcare MRes and MPhil/PhD
Ucl (university college london).
The CDT programme consists of a 1 year MRes followed by a 3 year PhD. Throughout this period the CDT will continue to closely monitor the Read more...
- 1 year Full time degree: £6,035 per year (UK)
- 2 years Part time degree: £2,930 per year (UK)
PhD Artificial Intelligence and Music
Queen mary university of london.
The UKRI Centre for Doctoral Training in Artificial Intelligence and Music (AIM) is a leading PhD research programme aimed at the Read more...
PhD with Integrated Study Machine Intelligence for Nano-electronic Devices (MINDS)
University of southampton.
This four-year iPhD is designed to develop and nurture the next generation of technology pioneers who will have the skills, assets and Read more...
- 4 years Full time degree
PhD Robotics
Sheffield hallam university.
Course summary Undertake extensive, supervised studies in the Centre for Automation and Robotics Research Specialise in pertinent Read more...
- 7 years Part time degree: £2,356 per year (UK)
Artificial Intelligence Machine Learning and Advanced Computing PhD
Three fully-funded 4-year PhD scholarships are available to start in October 2021 in the area of Artificial Intelligence machine learning Read more...
DPhil in Autonomous Intelligent Machines and Systems (EPSRC Centre for Doctoral Training)
University of oxford.
The Autonomous Intelligent Machines and Systems (AIMS) Centre for Doctoral Training (CDT) provides graduates with the opportunity to Read more...
- 4 years Full time degree: £9,500 per year (UK)
- 8 years Part time degree: £4,750 per year (UK)
Text and Data Mining (PhD/MPhil)
Cardiff university.
Focus your studies on text and data mining through our Computer Science and Informatics research programmes (MPhil, PhD). Studying for a Read more...
- 3 years Full time degree
- 5 years Part time degree
Practice-Oriented Artificial Intelligence PhD
University of bristol.
Practice-oriented artificial intelligence is about bridging the gap between complex problem domains such as those found in science and Read more...
- 4 years Full time degree: £4,758 per year (UK)
Informatics: ANC: Machine Learning, Computational Neuroscience, Computational Biology PhD
The university of edinburgh.
The Institute for Adaptive and Neural Computation (IANC) is a world-leading institute dedicated to the theoretical and empirical study of Read more...
- 6 years Part time degree
PhD Intelligent Systems
Ulster university.
The vision is to develop a bio-inspired computational basis for Artificial Intelligence to power future cognitive technologies. Our mission Read more...
- 6 years Part time degree: £2,360 per year (UK)
Statistics and Machine Learning (DPhil)
The Modern Statistics and Statistical Machine Learning CDT is a four-year DPhil research programme (or eight years if studying Read more...
Informatics: AIAI: Foundations and Applications of Artificial Intelligence, Automated Reasoning, Agents, Data Intensive Research PhD
At the Artificial Intelligence and its Applications Institute, we enable computer systems to reproduce and complement human abilities, work Read more...
Course type:
- Full time PhD
- Part time PhD
Qualification:
Related subjects:.
Explore your training options in 10 minutes Get Started
- Graduate Stories
- Partner Spotlights
- Bootcamp Prep
- Bootcamp Admissions
- University Bootcamps
- Coding Tools
- Software Engineering
- Web Development
- Data Science
- Tech Guides
- Tech Resources
- Career Advice
- Online Learning
- Internships
- Apprenticeships
- Tech Salaries
- Associate Degree
- Bachelor's Degree
- Master's Degree
- University Admissions
- Best Schools
- Certifications
- Bootcamp Financing
- Higher Ed Financing
- Scholarships
- Financial Aid
- Best Coding Bootcamps
- Best Online Bootcamps
- Best Web Design Bootcamps
- Best Data Science Bootcamps
- Best Technology Sales Bootcamps
- Best Data Analytics Bootcamps
- Best Cybersecurity Bootcamps
- Best Digital Marketing Bootcamps
- Los Angeles
- San Francisco
- Browse All Locations
- Digital Marketing
- Machine Learning
- See All Subjects
- Bootcamps 101
- Full-Stack Development
- Career Changes
- View all Career Discussions
- Mobile App Development
- Cybersecurity
- Product Management
- UX/UI Design
- What is a Coding Bootcamp?
- Are Coding Bootcamps Worth It?
- How to Choose a Coding Bootcamp
- Best Online Coding Bootcamps and Courses
- Best Free Bootcamps and Coding Training
- Coding Bootcamp vs. Community College
- Coding Bootcamp vs. Self-Learning
- Bootcamps vs. Certifications: Compared
- What Is a Coding Bootcamp Job Guarantee?
- How to Pay for Coding Bootcamp
- Ultimate Guide to Coding Bootcamp Loans
- Best Coding Bootcamp Scholarships and Grants
- Education Stipends for Coding Bootcamps
- Get Your Coding Bootcamp Sponsored by Your Employer
- GI Bill and Coding Bootcamps
- Tech Intevriews
- Our Enterprise Solution
- Connect With Us
- Publication
- Reskill America
- Partner With Us
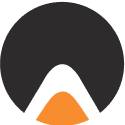
- Resource Center
- Bachelor’s Degree
- Master’s Degree
Best Doctorates in Artificial Intelligence: Top PhD Programs, Career Paths, and Salaries
The growing application of advanced technology in our daily lives has led to a high demand for professionals with qualifications in the field of artificial intelligence. The best PhDs in Artificial Intelligence offer tech professionals an opportunity to help meet this increased demand and land the highest-paying artificial intelligence jobs.
A careful look at some important factors for a PhD in Artificial Intelligence, such as program cost, length, and location, will help in determining the right artificial intelligence PhD program for you. This article also discusses some of the best artificial intelligence jobs and what you can expect to earn as a PhD in Artificial Intelligence salary.
Find your bootcamp match
What is a phd in artificial intelligence.
A PhD in Artificial Intelligence is a doctorate program with an artificial intelligence research focus. Students are required to complete original research in various areas of applied artificial intelligence. These areas may include machine learning, artificial neural networks, speech recognition, and processing. PhD students will be assigned an academic advisor who will guide the student throughout their research.
How to Get Into an Artificial Intelligence PhD Program: Admission Requirements
The requirements to get into an artificial intelligence PhD program are a minimum of a Bachelor’s Degree in Computer Science or a related field, such as computer engineering, data science, or statistics. Students will also need a strong background in programming and system analysis, and fluency in several computer languages. There may also be course prerequisites in areas such as English, writing, deep learning, and neural cognitive modeling.
Further requirements may include transcripts of your undergraduate or graduate coursework and standardized tests scores such as the GRE. You may also be required to submit a statement of purpose that includes a description, proposal, or preliminary idea of your research areas of interest for your doctoral thesis.
PhD in Artificial Intelligence Admission Requirements
- Bachelor’s or master’s degree
- Transcripts
- Letters of recommendation
- Statement of purpose
- Standardized test scores
- Strong background in programming languages such as Python and Java
- Knowledge of artificial intelligence subjects such as deep learning, neural and cognitive modeling, or computing
Artificial Intelligence PhD Acceptance Rates: How Hard Is It to Get Into a PhD Program in Artificial Intelligence?
It can be hard to get into a PhD program in Artificial Intelligence. Some programs are highly selective, and acceptance is not always based on merit alone. However, acceptance into some PhD programs is relatively easy if you meet all of the admissions requirements.
How to Get Into the Best Universities
[query_class_embed] how-to-get-into-*school
Best PhDs in Artificial Intelligence: In Brief
Best universities for artificial intelligence phds: where to get a phd in artificial intelligence.
The best universities for artificial intelligence PhDs are Arizona State University, Syracuse University, and Drexel University. They have some of the best artificial intelligence laboratories, high acceptance rates, and the right networks of people. Below is a detailed list of the best schools to get a PhD in Artificial Intelligence.
Arizona State University was founded on March 12, 1885. It is a public research institution that offers various programs at the graduate level including business administration, economics, and computer science. The graduate programs of Arizona State University are well known for their first-class facilities as well as renowned researchers.
PhD in Computer Science
This PhD program at Arizona State University requires 84 credit hours, a written comprehensive exam, an oral comprehensive exam, a prospectus, and a dissertation. The university has an artificial intelligence laboratory where students can conduct research. Some of the topic areas include artificial intelligence, big data, statistical modeling, cloud computing, social computing, data mining, and machine learning.
PhD in Computer Science Overview
- Program Length: Approx. 5 years
- Acceptance Rate: N/A
- Tuition: $11,720/year (in state); $23,544/year (out of state)
- PhD Funding Opportunities: Awards and fellowships, graduate appointments, distinguished wards
PhD in Computer Science Admission Requirements
- Minimum GPA of 3.5 in last 60 hours of bachelor’s degree or in master’s degree overall
- 3 letters of recommendation
- Curriculum vitae or resume
- Proof of English proficiency
Capitol Technology University is a private research institution founded on June 1, 1927. It is best known for its proven academic excellence, as well as expert guidance in doctoral research. Capitol Technology University offers graduate programs in areas such as aeronautical science, cyber security, business analytics, data science, and computer science.
PhD in Artificial Intelligence
About 60 credits of coursework are required for the PhD in Artificial Intelligence at Capitol Technology University. The program emphasizes the principles of autonomous systems and expounds on how computers operate to match the human-like operation of computers for decision-making and problem-solving processes. The program is available both online and in person.
PhD in Artificial Intelligence Overview
- Program Length: Approx. 3 years
- Tuition: $933/credit
- PhD Funding Opportunities: Hometown Heroes discounts, EdAssist partner discounts, loans
PhD in Artificial Intelligence Admission Requirements
- Master’s degree in a relevant field
- 5 years of work experience
- Application letter
- Application fee: $100
- Official transcripts
- 2 letters of recommendation
Cornell University's main campus in Ithaca was founded in 1865 by Ezra Cornell and Andrew Dickson White. It is divided into seven undergraduate campuses and seven graduate divisions. Its computer science PhD program is ranked in the top six of such programs in the nation by US News & World Report, and it performs studious and academically-tasking research.
This program is designed for students with a particular interest in the general components of computing processes. Some study areas students can choose to specialize in include artificial intelligence, machine learning, data structures, robotics, natural language processing, quantitative analysis, programming languages and methodology, robotics, and theory of computation.
- Program Length: 4 - 6 years
- Tuition and Fees: $29,500/year
- PhD Funding Opportunities: Teaching assistantships, research assistantships, fellowships, loans
- Application fee of $105
- Undergraduate degree
- Official transcripts
- English language proficiency (for international applicants)
Drexel University offers research and professional degree programs in the arts and sciences, biomedical engineering, education, science and health systems, business, computing, and informatics. Drexel University graduate programs are well known for their flexibility.
Students in this program carry out in-depth and creative research. They may choose from areas such as artificial intelligence, computer vision, human-computer interaction, information assurance, and security research areas. Whatever the path they choose to specialize in, they must meet specified course requirements including 18 credits in computer science courses.
- Program Length: Within 7 years
- Tuition: $1,342/credit
- PhD Funding Opportunities: Private scholarships, student loans, Drexel Dean’s Fellowship, and research, teaching, and graduate assistantships
PhD in Computer and Information Science Admission Requirements
- Official final transcripts from all colleges/universities attended
- Official GRE scores
- Essay/statement of purpose
- Current resume
- Official TOEFL scores (international applicants)
Founded in 1868, Oregon State University is the largest public research university in the state with students from over 100 countries. It offers more than 80 graduate programs in fields such as AI, bioengineering, mechanical engineering, and biological and ecological engineering. It is well known for its abundant learning resources that aid students' research.
PhD in Artificial Intelligence and Robotics
This artificial intelligence and robotics PhD program teaches the theories, algorithms, and systems for making intelligent decisions in complex and uncertain environments. Students can explore research areas like perception and interpretation of sensor data, automated planning and reasoning, and human-machine interaction.
PhD in Artificial Intelligence and Robotics Overview
- Tuition: $557/credit (in state); $1,105/credit (out of state)
- PhD Funding Opportunities: Graduate, teaching, and research assistantships, graduate diversity recruitment bonus program
PhD in Artificial Intelligence and Robotics Admission Requirements
- Application fee
- Statement of objectives
- Graduate/undergraduate transcripts
- GPA of 3.00
Pace University was founded in 1906 as a business school by St. Clair Pace and Charles A. Pace. It has a main campus in New York City and secondary campuses in Westchester County, New York. It offers PhD programs in clinical psychology, computer science, mental health counseling, nursing, and school psychology.
Only students with demonstrated field experience are accepted into this extremely selective program. Students are closely involved in vital, strategic advanced research projects and make authentic advances in the field in areas such as pattern recognition in medical image segmentation, artificial intelligence, and intelligent systems. Each student is expected to pass three qualification exams.
- Program Length: 3 years
- Tuition: $1,420/credit
- PhD Funding Opportunities : Graduate assistantships, federal work-study, student loans
- Master’s Degree in Computer Science or a related field
- Research presentation
- $70 application fee
- Personal statement
- All official post-secondary transcripts
Syracuse University was founded on March 24, 1870. It offers over 200 graduate programs in fields such as data and research, computer science, and engineering, among others. It is well known for its professional programs, investment in research and innovation, and solid reputation.
PhD in Computer and Information Science and Engineering
The PhD in Computer and Information Science and Engineering program offered at Syracuse University is a well-structured program. Graduate students must complete a minimum of 48 credits in technical graduate courses, as well as other research courses.
In addition, each student must complete at least four credits of professional development courses. A proposal and a dissertation must also be completed and defended by the students.
PhD in Computer and Information Science and Engineering Overview
- Program Length: Within 5 years
- Acceptance Rate: 14.3%
- Tuition: $1,802/credit
- PhD Funding Opportunities: Teaching, research, and graduate assistantships, scholarships, university fellowships, research excellence doctoral funding programs
PhD in Computer and Information Science and Engineering Admission Requirements
- Bachelor’s or Master’s Degree in Computer Engineering, Computer and Information Science, or a related field
- TOEFL score (international applicants)
The University of Colorado is a public research institution located in Boulder, Colorado. It offers over 124 graduate and professional degrees in the arts and sciences, business, education, engineering, law, and music. The university allows students to collaborate with esteemed faculty and researchers and gain world-class expertise in their chosen fields.
This PhD in Computer Science at the University of Colorado requires 30 hours of graduate-level coursework, as well as 30 hours of thesis work. It also requires the completion of a preliminary exam, comprehensive exam, and dissertation defense, typically within six years of beginning your coursework.
- Program Length: Within 6 years
- Tuition: $780/credit (in state); $847/credit (out of state)
- PhD Funding Opportunities: Assistantships, fellowships, faculty research grants
- At least 3 courses in computer science beyond the introductory level
- Research experience
- Resume with research and publication details
- Copy of transcripts for the required undergraduate degree or master's degree
- Proof of financial support and a funding plan
Founded on August 26, 1817, the University of Michigan is known as one of the first public universities in the nation. It is ranked as the ninth best school for artificial intelligence by US News & World Report. The school offers master’s and PhD programs in areas like aerospace engineering, architecture, urban planning, and dentistry.
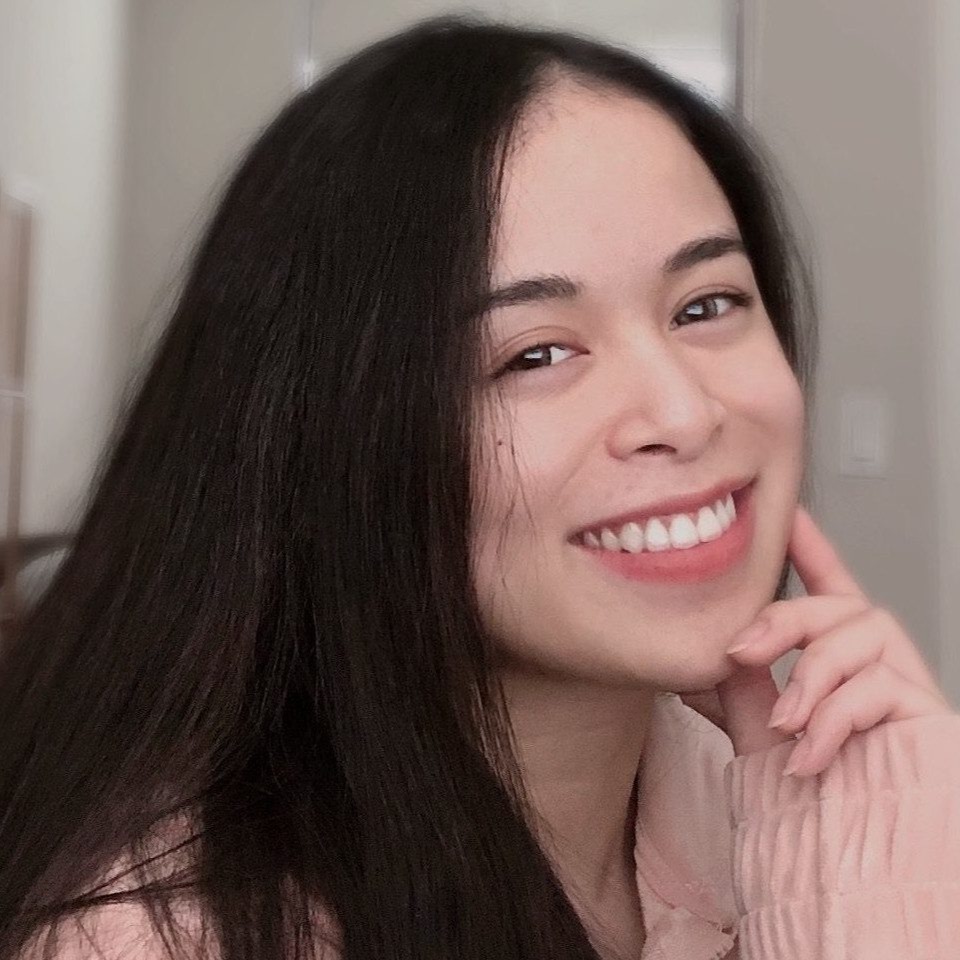
"Career Karma entered my life when I needed it most and quickly helped me match with a bootcamp. Two months after graduating, I found my dream job that aligned with my values and goals in life!"
Venus, Software Engineer at Rockbot
PhD in Computer Science and Engineering
Intended for students who wish to pursue research or careers as postsecondary teachers, this PhD program is highly recognized for its offerings in relatively broad fields of knowledge. It is known for imparting a demonstrated ability in its graduates to carry out independent research, yielding significant original results.
PhD in Computer Science and Engineering Overview
- Program Length: 4 - 5 years
- Acceptance Rate: 1%
- Tuition and Fees: $14,558/full term (resident); $27,023/full term (nonresident)
- PhD Funding Opportunities: Graduate research assistantships on research grants and contracts, teaching assistantships
PhD in Computer Sciences and Engineering Admission Requirements
- Master's or bachelor's degree in any related field
- GPA of at least 3.5
- 3 strong letters of recommendation
- Proof of English proficiency (international students)
With over 11,000 graduate students enrolled, the University of Texas ranks among the 10 best public universities in the country, by US News & World Report. It offers graduate programs in various fields such as accounting, business, engineering, education and development, and computer science.
This program provides cutting-edge research experience as well as expertise in advanced computer science subjects. Through this program, students can specialize in the artificial intelligence track and conduct their research work on topics like information security, computer architecture, assistive technology, computer networks, and deep learning.
- Program Length: Within 8 years
- Tuition and Fees: $347.53/credit (in state); $1,344.63/credit (out of state)
- PhD Funding Opportunities: Grants and loans; department, institutional, and external fellowships and scholarships; research, teaching, and graduate assistantships
PhD in Computer Science Admission Requirements
- Transcripts of an ABA, BS, or MS degree in Computer Science/related area
- Completed graduate school application
- Resume/curriculum vitae
Can You Get a PhD in Artificial Intelligence Online?
Yes, you can get a PhD in Artificial Intelligence online. Some universities in the United States offer online artificial intelligence doctorate programs, like Capitol Technology University. The amount of time required to complete the online artificial intelligence program is about the same as the in-person program, depending on the institution.
Best Online PhD Programs in Artificial Intelligence
How long does it take to get a phd in artificial intelligence.
It takes about three to five years to get a PhD in Artificial Intelligence. That duration may be longer for people with particularly lengthy or complex research work or for those working part-time toward their PhD.
The reason for the longer duration could be a result of the institution’s requirements, the amount of research required before a thesis can be submitted, a student’s cooperation with their academic supervisor, or the program’s format, that is, whether it is full-time or part-time.
Is a PhD in Artificial Intelligence Hard?
No, a PhD in Artificial Intelligence is not that hard. Getting a PhD in computer-related programs can indeed be difficult, but when compared with other fields of theoretical study in computer science, a PhD in Artificial Intelligence is relatively easier.
PhD programs are very research-focused. What makes the PhD in Artificial Intelligence easier is that more emphasis is placed on empirical evaluation than on performance. That is, you have to prove that your method makes sense intuitively, not that it actually works. For your AI PhD, you can even show how an already established method works in a new area.
How Much Does It Cost to Get a PhD in Artificial Intelligence?
It costs about $19,792 per year to get a PhD in Artificial Intelligence , according to the National Center for Education Statistics. This average varies depending on the type of university involved. For public institutions, annual tuition averages about $12,410, while private universities charge about $26,597 per year. These rates are for in-state students only.
It is important to note that additional fees and costs also apply, such as application fees and department fees. However, most PhD programs have guaranteed financial support, although it doesn’t usually cover the entire cost of the degree.
How to Pay for a PhD in Artificial Intelligence: PhD Funding Options
Some of the PhD funding options available for students include research assistantships, where PhD students assist a member of faculty with their research. Students can also participate in a teaching assistantships, where they assist a professor in various academic-related areas. Instead of being paid for their work, students receive partial or full tuition or a stipend.
Another option for PhD funding is tuition waivers, which are awarded based on things like merit, race, or occupation. There also may be financial scholarships or loans available to help with costs.
Best Online Master’s Degrees
[query_class_embed] online-*subject-masters-degrees
What Is the Difference between an Artificial Intelligence Master’s Degree and PhD?
One difference between an artificial intelligence master’s degree and a PhD is that a master’s degree program consists mostly of classwork and projects, with little intensive research work, while a PhD in Artificial Intelligence predominantly involves intensive research work aimed at finding answers and solutions to existing questions and problems.
It is harder to get a PhD than a master’s degree . In the field of artificial intelligence, master’s students will focus on multiple areas of learning, such as building multi-agent systems, economic systems, or artificial agents. PhD students will be more focused on solving a specific issue, requiring intense focus and strong critical-thinking and problem-solving skills, and making advancements in the field.
Master’s vs PhD in Artificial Intelligence Job Outlook
There is a difference in the job outlook for AI master’s degree holders and AI PhD holders. Those with a PhD in Artificial Intelligence have more job opportunities overall and can expect a higher salary than those with a Master’s Degree in Artificial Intelligence.
Some examples of jobs in artificial intelligence that require a PhD are big data engineer and architect, research scientist, natural language processing engineer, software architect, senior software engineer, and machine learning engineer. Those requiring a master’s degree include computer and information research scientist, data analyst, and artificial intelligence developer.
Difference in Salary for Artificial Intelligence Master’s vs PhD
A PhD in Artificial Intelligence is a terminal degree that brings with it higher salaries than a Master’s in Artificial Intelligence does. Someone with a PhD in the field of artificial intelligence earns about $115,000 per year, according to PayScale.
On the other hand, an artificial intelligence master’s degree holder earns $102,000 per year on average. This means that those with an AI PhD can expect to make about $13,000 more every year than those with an AI master’s degree.
Related Artificial Intelligence Degrees
[query_class_embed] https://careerkarma.com/blog/business-intelligence-degree-online-a-guide/ https://careerkarma.com/blog/best-artificial-intelligence-bachelors-degrees/ https://careerkarma.com/blog/best-online-artificial-intelligence-and-machine-learning-bachelors-degrees/
Why You Should Get a PhD in Artificial Intelligence
You should get a PhD in Artificial Intelligence because it opens the door to more employment opportunities and higher salaries. If you’re already employed, you may be able to keep your job for part or even all of your PhD program. Artificial intelligence is a growing and in-demand field, so obtaining the highest level of education will set you up for continuous opportunities.
Reasons for Getting a PhD in Artificial Intelligence
- More employment opportunities. With a PhD in Artificial Intelligence, landing a good job shouldn’t be a problem. The degree opens the door to more and better career opportunities.
- High-paying jobs. A Doctorate in Artificial Intelligence brings with it not only more career opportunities but also better salaries. PayScale shows that the average salary of a PhD degree holder in the field of artificial intelligence is about $115,000. However, the earning potential with this degree is considerably higher, as much as $200,000 per year for positions like principal scientist.
- Expertise in a versatile field. A doctorate degree in artificial intelligence will provide you with a deep understanding of AI theories and strong technical skills, which you can use to help solve future problems in a wide variety of fields.
- Plentiful research opportunities. With a PhD in Artificial Intelligence, you have the opportunity to engage in numerous research projects, which will increase your overall knowledge in the AI field and imbue you with the ability to find solutions to a wide variety of problems.
Getting a PhD in Artificial Intelligence: Artificial Intelligence PhD Coursework
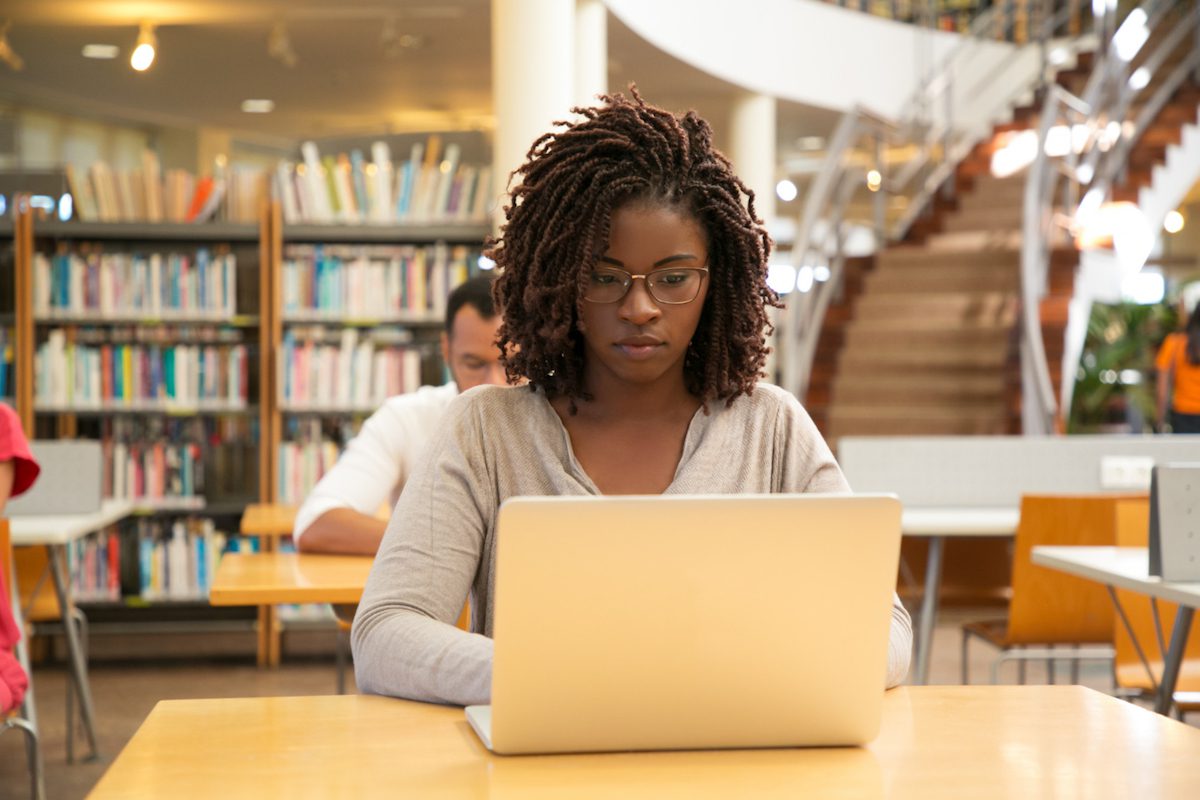
Getting a PhD in Artificial Intelligence requires significant coursework in addition to the research element of the degree. Some common course topics include advanced data structures and algorithms, natural language learning, intermediate statistics, machine learning in practice, and regression analysis.
Foundations of Machine Learning
This course aims to teach students the founding principles of machine learning, its theory, and the techniques involved. The course includes different categories of machine learning including supervised learning, unsupervised learning, and reinforcement learning.
Advanced Data Structures and Algorithms
Through this course, students learn the branches of data science and how data is managed and organized to make it easily accessible. The knowledge gained from this course will equip the student with the skills needed for developing effective and useful software designs and algorithms.
Matrix Algebra
This course teaches students how to use matrix algebra in experimental design. Students learn how to analyze complex data and use linear algebra to explore theories that involve two or more matrices. This skill is very useful when conducting research.
Probability and Random Variables
The course on probability and random variables in the field of artificial intelligence aims to teach PhD students how to reduce uncertainty when there is a margin of error as a result of the inadequacy of perfect information.
Machine Learning in Practice
In this course, students will put all that they have learned in the machine learning field into practice. They will apply their skills and knowledge in the areas of mathematics, computing, engineering, and data analysis to real-world datasets and examples.
Best Master’s Degrees
[query_class_embed] *subject-masters-degrees
How to Get a PhD in Artificial Intelligence: Doctoral Program Requirements
To get a PhD in Artificial Intelligence, students must meet specific criteria that vary with each school and program. Some of the more common ones include a competency requirement, a project requirement, a breadth requirement, a teaching requirement, and approval of the thesis. Students also need to pass the necessary examinations before a PhD in Artificial Intelligence can be awarded.
Students are expected to demonstrate competency in various branches of artificial intelligence and all the theories taught in the program. They are also required to perform well on their examinations, usually with a grade of B+ or higher.
PhD students are required to take a specific number of upper-level courses for their degree. These courses need to encompass the different areas of artificial intelligence as well as the research problems and techniques associated with them.
As a way of showcasing the skills they have gained in their years of doctorate studies, PhD students must create and complete a project that’s in harmony with the project requirements of their particular program. This project will be based in a specialized field of artificial intelligence and must be approved by the project supervisors before this requirement can be met.
It is a requirement for PhD students to serve in the position of teaching assistant for at least two semesters or teach a course in their field for at least a semester. These roles allow PhD students to interact with students, sharpening their communication skills and preparing them for a possible career in academics.
Before a PhD in the field of artificial intelligence can be awarded, thesis research will be required of the PhD student. The thesis usually focuses on answering industry-wide problems. Once the thesis has been approved by the school supervising committee, then a doctoral degree in artificial intelligence can be given.
Potential Careers with an Artificial Intelligence Degree
[query_class_embed] how-to-become-a-*profession
PhD in Artificial Intelligence Salary and Job Outlook
The job outlook for artificial intelligence PhD holders is very good, as we are living in a time of rapid technological advancements. The US Bureau of Labor Statistics (BLS) predicts a growth of 22 percent in jobs like computer and research scientist through 2030. With an average salary of $115,000 for AI PhD holders, as stated above, you can expect to earn a great salary with your degree.
What Can You Do With a PhD in Artificial Intelligence?
With a PhD in Artificial Intelligence, you can get employed by top tech companies and work on a broad array of AI applications, programs, and systems. These include facial-recognition software, self-driving cars and drones, digital personal assistants, and more. AI students gain employment as computer scientists, AI experts, machine learning engineers, computational linguists, and robotics engineers after graduation. Here are some of the best jobs for artificial intelligence PhD holders.
Best Jobs with a PhD in Artificial Intelligence
- Big Data Engineer/Architect
- Software Architect
- Machine Learning Engineer
- Software Engineer
- Data Scientist
What Is the Average Salary for a PhD in Artificial Intelligence?
The average salary for a graduate with a PhD in Artificial Intelligence is $115,000 , according to PayScale. The exact salary you can expect will depend on a wide range of factors, which include your job title and responsibilities, the industry in which you are employed, your specific experience and knowledge, and the company’s geographic region.
Highest-Paying Artificial Intelligence Jobs for PhD Grads
Best artificial intelligence jobs with a doctorate.
The best artificial intelligence jobs with a doctorate degree are software architect, big data engineer/architect, machine learning engineer, data scientist, and software engineer. According to BLS, AI is taking a bigger role in our security , which will lead to even more job prospects in the field.
An artificial intelligence researcher conducts research to create and design new and better ways of solving problems. The models they create are used by data scientists to solve the real-world problems they were designed to solve.
- Salary with an Artificial Intelligence PhD: $131,490
- Job Outlook: 22% job growth from 2020 to 2030
- Number of Jobs: 33,000
- Highest-Paying States: Oregon, Arizona, Texas
Using their experience and research in artificial intelligence and software development, software engineers are capable of leading major software development projects. They can work for big IT companies such as IBM, Intel, Microsoft, and Alphabet.
- Salary with an Artificial Intelligence PhD: $126,668
- Number of Jobs: 1,847,900
- Highest-Paying States: Washington, California, New York
Engineers who work in machine learning are at the crossroads of software design and data science. They use big data technologies and programming structures to build production-ready, flexible, scalable models that can manage terabytes of actual data.
- Salary with an Artificial Intelligence PhD: $112,567
A robotics engineer is in charge of designing robots and robotic systems. With the implementation of AI, they will focus on designing complex robotic communication systems software and hardware components.
- Salary with an Artificial Intelligence PhD: $95,300
- Job Outlook: 7% job growth from 2020 to 2030
- Number of Jobs: 299,200
- Highest-Paying States: New Mexico, Louisiana, District of Columbia
Computational linguists’ job is to make communication possible between humans and machines by teaching computers how to understand us. They have a complex understanding of various programming languages and perform product-specific research in computational linguistics.
- Salary with an Artificial Intelligence PhD: $83,335
Is a PhD in Artificial Intelligence Worth It?
Yes, a PhD in Artificial Intelligence is worth it. Artificial intelligence and cognitive technology are expected to be the catalyst for the next scientific revolution. In a data-rich world, creating AI technology that can learn via developing and drawing conclusions based on human behavior could change the future and offer limitless industrial, social, and scientific possibilities.
As the world becomes more dependent on advancing technologies and intelligent machines, there is an ever-growing market for PhD holders in the field of artificial intelligence. A PhD in Artificial Intelligence will provide you with expertise in the field and qualify you for high demand careers in the field.
Additional Reading About Artificial Intelligence
[query_class_embed] https://careerkarma.com/blog/artificial-intelligence/ https://careerkarma.com/blog/best-ai-blogs/ https://careerkarma.com/blog/online-artificial-intelligence-courses/
PhD in Artificial Intelligence FAQ
A PhD in Artificial Intelligence offers numerous lucrative career opportunities such as robotics engineer, senior software engineer, and machine learning expert. You will also be qualified to teach in a postsecondary institution.
The best places to pursue a PhD in Artificial Intelligence are accredited universities and colleges. Some of the top such institutions in the US include Arizona State University, Drexel University, and Syracuse University.
For students interested in a career in artificial intelligence, a computer science doctoral degree is a popular option. Many colleges and universities offer computer science doctorate programs with a focus on artificial intelligence or machine learning.
Because artificial intelligence is still a relatively new field, it’s difficult to know exactly how profitable the AI sector is. The value of artificial intelligence to the global economy will be $13 trillion by 2030, according to a report by the European Parliament. The world’s growing dependency on technological innovations and intelligent machines indicates that more growth is coming in this field.
About us: Career Karma is a platform designed to help job seekers find, research, and connect with job training programs to advance their careers. Learn about the CK publication .
What's Next?

Get matched with top bootcamps
Ask a question to our community, take our careers quiz.
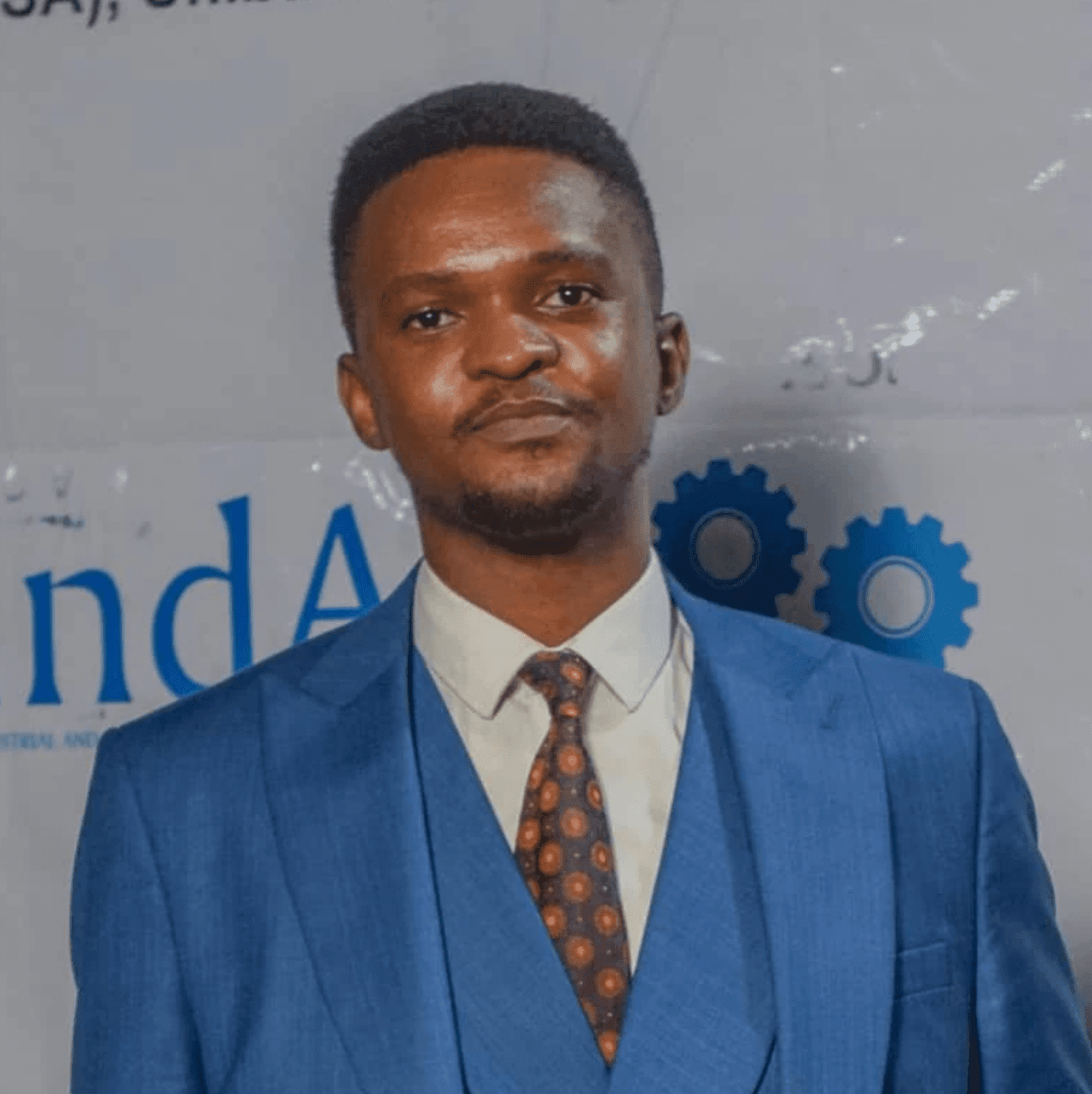
Leave a Reply Cancel reply
Your email address will not be published. Required fields are marked *
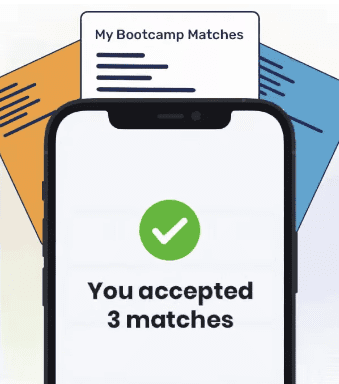
DeepMind is Google's AI research hub. Here's what it does, where it's located, and how it differs from OpenAI.
- DeepMind is Google's AI research hub focused on building artificial general intelligence.
- DeepMind has been applied to real-world problems in healthcare, science, and engineering.
- DeepMind has a number of competitors, including OpenAI, though Google's model is for profit.
In the last few years, artificial intelligence has stepped out of the pages of science fiction and into everyday life.
Today, we're surrounded by AI systems like Gemini, ChatGPT, Dall-E, CoPilot, and countless others, but Google DeepMind is somewhat different.
Launched back in 2010, DeepMind is a company with the goal of developing an artificial general intelligence , often referred to as AGI.
What does Google's DeepMind do?
While many AI systems in use today are very good at completing specific kinds of tasks for which they were trained, the goal of AGI is to build a human-like intelligence that can learn, reason, and problem-solve a wide range of topics and tasks across a plethora of domains.
In other words, it's designed to mimic human intelligence.
This is different from systems like ChatGPT and Google Gemini , which are narrow AI systems that are very good at the specific task of understanding natural language well enough to deliver useful information through human-like interactions.
Of course, DeepMind has not yet achieved AGI, but has made impressive achievements nonetheless. In practice, DeepMind has been applied to solving real-world problems in healthcare, science and engineering. It's perhaps most famous, though, for its mastery of enormously challenging games.
In 2015, for example, DeepMind's AlphaGo became the first computer program to ever defeat a human opponent at Go (a game considered far more complex than chess). Less than two years later, AlphaGo went on to defeat the top-ranked Go player in the world.
Who runs Google's DeepMind?
DeepMind was created in 2010 by a trio of computer engineers from the Gatsby Computational Neuroscience Unit at University College London, and early research focused on getting AI systems to learn to play games without any instruction — the software would learn games like Breakout, Pong and Space Invaders through trial and error, eventually mastering the rules and becoming an expert at the games.
Google acquired DeepMind in 2014 for a price somewhere between $400 million and $650 million. Today, the company remains a part of Google's Alphabet portfolio of businesses where Demis Hassabis , one of DeepMinds three original founders, continues to lead the development of AGI as CEO.
In April 2023, Google CEO Sundar Pichai announced that Google would merge DeepMind with the Brain team from Google Research to create a single AI unit — named Google DeepMind — to "help us build more capable systems more safely and responsibly."
Google DeepMind remains based in London primarily, but also has researchers in Montreal, Canada, and at the Googleplex corporate headquarters in Mountain View, California.
What's the difference between DeepMind and OpenAI?
Of course, DeepMind is hardly alone in its AI research and development; it has a number of competitors, including the headline-making OpenAI.
These two companies take a very different approach to AI development, though. DeepMind is a for-profit part of Google's Alphabet, Inc., for example, while OpenAI was originally established as a non-profit, before transitioning to a "capped-profit" model .
The two companies have developed AI models and applications in ways that have contributed to AI research in sometimes complementary ways. While DeepMind mastered Go with AlphaGo, for example, OpenAI developed Generative Pre-trained Transformer language models (for example, ChatGPT) that allow machines to better understand natural language, for more interactive and immersive experiences.
Do you need a PhD to work at DeepMind?
Given the deep complexity of what DeepMind is developing, one might assume that prospective employees might all require a PhD. In reality, though, that's not true. Google hires a large number of researchers and computer engineers with lesser degrees to help advance the state of the art in artificial intelligence.
If you enjoyed this story, be sure to follow Business Insider on Microsoft Start.
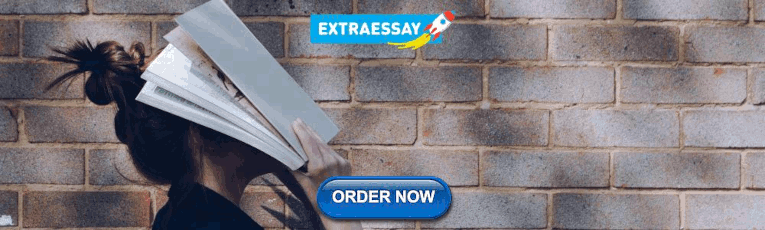
IMAGES
VIDEO
COMMENTS
These PhD Research Topics In Artificial Intelligence serve as gateways to unlocking the potential of intelligent machines, spanning fields such as healthcare, finance, robotics, education, and more. As aspiring researchers delve into these areas, they embark on a journey to expand the boundaries of AI, seeking solutions to complex problems and ...
In this blog, we embark on a journey to delve into 12 Artificial Intelligence Topics that stand as promising avenues for thorough research and exploration. Table of Contents. 1) Top Artificial Intelligence Topics for Research. a) Natural Language Processing. b) Computer vision. c) Reinforcement Learning. d) Explainable AI (XAI)
A comprehensive list of research topics ideas in the AI and machine learning area. Includes access to a free webinar and topic evaluator. About Us; Services. 1-On-1 Coaching. ... An overview of artificial intelligence in diabetic retinopathy and other ocular diseases (Sheng et al., 2022) HOW DOES ARTIFICIAL INTELLIGENCE HELP ASTRONOMY? A REVIEW ...
Robust and trusted artificial intelligence research hinges on the foundation of Open Science practices and the meticulous curation of data which PLOS takes pride in highlighting and endorsing thanks to our rigorous standards and commitment to Openness. As a leading publisher in the field, these articles showcase research that has influenced ...
The 2023 PhD topics in Artificial Intelligence highlight the dynamic field's growth and promise for revolutionizing industries and improving quality of life. The research emphasizes ethical AI, addressing bias, fairness, and transparency. Advancements in natural language processing make AI more accessible and intuitive.
Artificial Intelligence and Machine Learning. Our research covers a wide range of topics of this fast-evolving field, advancing how machines learn, predict, and control, while also making them secure, robust and trustworthy. Research covers both the theory and applications of ML. This broad area studies ML theory (algorithms, optimization, etc ...
Advancing Knowledge-Based Economies and Societies through AI and Optimization: Innovations, Challenges, and Implications. Erfan Babaee Tirkolaee. Gerhard-Wilhelm Weber. 321 views. A nexus for research in core and applied AI areas, this journal focuses on the enormous expansion of AI into aspects of modern life such as finance, law, medicine ...
The Machine Learning (ML) Ph.D. program is a fully-funded doctoral program in machine learning (ML), designed to train students to become tomorrow's leaders through a combination of interdisciplinary coursework, and cutting-edge research. Graduates of the Ph.D. program in machine learning are uniquely positioned to pioneer new developments in the field, and to be leaders in both industry and ...
ARTIFICIAL INTELLIGENCE PHD RESEARCH TOPICS. Here in phdprojects.org we guide scholars for topic selection for your PhD and MS later we carry out research work as well. Our developers are furnished with advanced knowledge and research skills in the field of artificial intelligence (AI). We select the topics that would be original yet viable.
We have 475 Artificial Intelligence PhD Research Projects PhD Projects, Programmes & Scholarships. Show more Show all . ... working on the topic of multi-modal AI for healthcare. Read more Supervisor: Dr C Chen. 10 June 2024 PhD Research Project Funded PhD Project (Students Worldwide)
AI Ethics Topics. How the automation of jobs is going to make many jobless. Discuss inequality challenges in distributing wealth created by machines. The impact of machines on human behavior and interactions. How artificial intelligence is going to affect how we act accordingly.
Here some of the recent Research Topics, Artificial Intelligence and Machine learning - Recent Trands. How AI and ML can aid healthcare systems in their response to COVID-19. Machine learning and artificial intelligence in haematology. Tackling the risk of stranded electricity assets with machine learning and artificial intelligence.
PhD research topics in artificial intelligence can vary dramatically depending on your interests. Whether you choose your own PhD topic or join an existing PhD project, you could find yourself pursuing research in the areas of: data management and data science. computer vision and multimedia computation. machine learning and data mining.
Interactive Artificial Intelligence. 31,777 USD / year. 4 years. Our goal in the Interactive Artificial Intelligence programme from University of Bristol is to train you to become an innovator and research leader in responsible, data-driven, and knowledge-intensive human-in-the-loop AI systems. Ph.D. / Full-time, Part-time / On Campus.
Here are some suggested topics for PhD projects from our group members. These projects are merely suggestions and illustrate the broad interests of the research group. ... more accurate models are less explainable and there is a scientific interest in the field of Explainable Artificial Intelligence, to develop new methods that explain and ...
Gemma Conroy, Hepeng Jia, Benjamin Plackett &. Andy Tay. As artificial intelligence (AI) becomes ubiquitous in fields such as medicine, education and security, there are significant ethical and ...
The research topics described in the table below are investigated by faculty and researchers of the three University partners (i.e. Pisa, Florence and Sienna) and of CNR Pisa (i.e. ISTI and IIT). All of these investigations are supported by national and international projects at academic or industrial level. Algorithm Design
Artificial Intelligence (AI) focuses on creating and training machines that find solutions to problems which normally require human intelligence. Artificial Intelligence systems can be powered by machine learning, deep learning, or operating rules. Being a relatively new field, AI comes with both benefits and potential risks.
This guide provides information and resources on the applications of Artificial Intelligence (AI) in the academic health sciences. This includes the use of AI in teaching, research, and publishing. The primary types of AI used in these areas are Generative AI, including AI chatbots, and Deep Learning technology.
So without further ado, let's see the different Topics for Research and Thesis in Artificial Intelligence!. 1. Machine Learning. Machine Learning involves the use of Artificial Intelligence to enable machines to learn a task from experience without programming them specifically about that task. (In short, Machines learn automatically without human hand holding!!!)
The Artificial Intelligence in Medicine (AIM) PhD track, newly developed by the Department of Biomedical Informatics (DBMI) at Harvard Medical School, will enable future academic, clinical, industry, and government leaders to rapidly transform patient care, improve health equity and outcomes, and accelerate precision medicine by creating new AI technologies that reason across massive-scale ...
Banking Bot. Banking bot is one of the excellent research and thesis topics for AI projects in 2022. This AI project involves building a banking bot that uses artificial intelligence algorithms that analyze user queries to understand their message and accordingly perform the appropriate action. It is a specially designed application for banks ...
The rise of artificial intelligence (AI) and deep learning has been transforming humans ability to execute complex inference tasks using computers. Read more. Supervisor: Dr L Su. 31 May 2024 PhD Research Project Funded PhD Project (UK Students Only) More Details. Last chance to apply.
Foundational research topics in artificial intelligence. Machine Learning: Developing algorithms and models that enable systems to learn from data, make predictions, and improve performance over time. Natural Language Processing (NLP): Analyzing, understanding, and generating human language to enable effective communication between humans and ...
The UKRI Centre for Doctoral Training in Artificial Intelligence and Music (AIM) is a leading PhD research programme aimed at the Read more... 3 years Full time degree: £4,786 per year (UK) 6 years Part time degree: £2,393 per year (UK) Apply now Visit website Request info Book event. View 8 additional courses. Compare.
PhD in Artificial Intelligence Salary and Job Outlook. The job outlook for artificial intelligence PhD holders is very good, as we are living in a time of rapid technological advancements. The US Bureau of Labor Statistics (BLS) predicts a growth of 22 percent in jobs like computer and research scientist through 2030. With an average salary of ...
Higher ed moving beyond initial artificial intelligence (AI) fears to focus on practical and specific opportunities for the technology was a recurring theme at the Digital Universities U.S. conference that concluded on Wednesday in St. Louis.. The conference, co-hosted this week by Inside Higher Ed and Times Higher Education in collaboration with Washington University in St. Louis, brought ...
BY Ahtisham Younas, PhD, RN. Published research needs to be used in real-world practice for making a meaningful difference in lives of patients, families, health care professionals, and other individuals whom the research affects. We know from research that it could take about 15 to 17 years for the published research to make it into real-world ...
DeepMind is Google's AI research hub focused on building artificial general intelligence. DeepMind has been applied to real-world problems in healthcare, science, and engineering.
The scholarship covers full tuition and required fees for one year and a stipend of $36,084. Xie is a fourth year PhD student. She is expected to graduate April 2025. Xie's research explores the relationship between artificial intelligence and humans and explores opportunities for innovation. Her dissertation advisor is UMSI professor Qiaozhu ...