
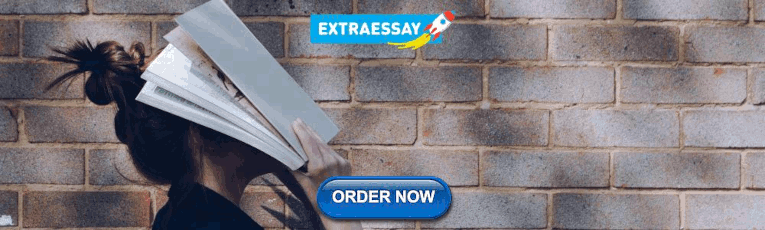
Perceptions, Challenges and Effectiveness of Modular Distance Learning Approach to The Academic Performance of Humanities and Social Sciences (HUMSS) Students of Botolan National High School
##plugins.themes.academic_pro.article.sidebar##, main article content.
The COVID-19 pandemic has necessitated a significant shift towards modular distance learning in education systems worldwide. In the Philippines, the Department of Education has developed Self-Learning Modules (SLMs) to ensure quality primary education for all learners during the pandemic. This research study aims to identify the challenges and effectiveness of the modular distance learning approach on the academic performance of Grade 12 Humanities and Social Sciences students at Botolan National High School. Employing a descriptive research design, data were collected through a validated questionnaire and analyzed using weighted arithmetic mean, frequency, percentage distribution, and Pearson-r correlation. The study found that the majority of student-respondents were female, aged 17.31 years old, and achieved very satisfactory academic grades. The students perceived the modular distance learning approach as agreeable, with self-motivation being the most challenging aspect. The ability to express ideas was the most effective aspect, while the ability to answer without pressure was the least effective. The study found no significant relationship between student's perceptions of the modular distance learning approach and their academic performance. Based on the findings, the study recommends using the modular distance learning approach and developing policies to address students' mental health issues. Furthermore, further research is needed to investigate teacher readiness and technology competency, as well as other variables not covered in this study, to improve the effectiveness of the modular distance learning approach. The study demonstrates that despite the limitations caused by the pandemic, modular distance learning can be an efficient alternative to traditional face-to-face education.
Article Details


An official website of the United States government
The .gov means it’s official. Federal government websites often end in .gov or .mil. Before sharing sensitive information, make sure you’re on a federal government site.
The site is secure. The https:// ensures that you are connecting to the official website and that any information you provide is encrypted and transmitted securely.
- Publications
- Account settings
Preview improvements coming to the PMC website in October 2024. Learn More or Try it out now .
- Advanced Search
- Journal List
- HHS Author Manuscripts

On the effectiveness of self-paced learning
Metacognitive monitoring and control must be accurate and efficient in order to allow self-guided learners to improve their performance. Yet few examples exist in which allowing learners to control learning produces higher levels of performance than restricting learners’ control. Here we investigate the consequences of allowing learners to self-pace study of a list of words on later recognition, and show that learners with control of study-time allocation significantly outperformed subjects with no control, even when the total study time was equated between groups (Experiments 1 and 2). The self-pacing group also outperformed a group for which study time was automatically allocated as a function of normative item difficulty (Experiment 2). The advantage of self-pacing was apparent only in subjects who utilized a discrepancy reduction strategy—that is, who allocated more study time to normatively difficult items. Self-pacing can improve memory performance, but only when appropriate allocation strategies are used.
Being an effective student requires the ability to guide one's own learning activities effectively (e.g., Finley, Tullis, & Benjamin, 2009 ; Metcalfe, 2009 ). Students choose what to study, how to study, when to study, and how long to study; these self-regulated aspects of learning have important implications for the effectiveness of their learning efforts and achievement in education ( Dunlosky & Theide, 1998 ). Recent research on the effectiveness of self-controlled study has revealed beneficial effects of allowing subjects choice about which items to re-study ( Kornell & Metcalfe, 2006 ; Nelson, Dunlosky, Graf, & Narens, 1994 ) and how to schedule re-study ( Benjamin & Bird, 2006 ; Son, 2004 ; Toppino, Cohen, Davis, & Moors, 2009 ). Here we consider the effectiveness of allowing learners control over the allocation of study time across a heterogeneously difficult set of items. In addition, we investigate how individual differences in allocation policy affect the benefits of according control to learners.
In the experiments presented here, we directly compare the effects of self-control over study time with control conditions in which total study time is equated and items are either allocated equivalent study time (Experiments 1 and 2) or allocated study time based on their normative difficulty (Experiment 2). As we review shortly, the extant literature on control over study time provides no clear answer on whether such control is beneficial and even lesser guidance on the control strategies that affect those potential benefits.
The effect of control of study time allocation is related to two metacognitive skills that have been studied in the metacognition literature. First, learners must be able to apply an effective learning strategy to a heterogeneously difficult set of items. Second, monitoring of the items must be reasonably accurate—that is, subjects must be able to discern which items will be easy and which will be difficult for them to learn. We treat these issues in turn in the next two sections.
Strategies in allocation of study time
One of the most fundamental aspects of self-regulated learning is deciding how to allocate study time across items. Learners usually devote more time to items they judge to be difficult, at least when no time constraints exist. A meta-analysis by Son and Metcalfe (2000) revealed that, out of 46 published conditions examining the relationship between difficulty and study time, 35 revealed a preference to devote more study time to material judged to be difficult. Individual differences in the degree to which subjects modulate their study time across items have also been noted. For example, both younger and older adults spend more time studying difficult items, but younger adults modulate their study time based upon item difficulty to a greater extent than do older adults ( Dunlosky & Connor, 1997 ).
Two general theoretical approaches explain how subjects allocate study time by outlining the decision process used to cease study of an item. The discrepancy reduction theory suggests that people stop studying an item once that item's judged level of learning meets a pre-set criterion ( Dunlosky & Theide, 1998 ), while the region of proximal learning theory ( Metcalfe & Kornell, 2003 ) suggests that people stop studying an item once the “rate of return” (amount of mnemonic benefit per time unit of study) falls below a criterion rate. Although they rely upon different cessation-of-study mechanisms, discrepancy reduction and the region of proximal learning theories often predict the same self-paced study behavior: people study the difficult items longer than the easy ones.
Memory Monitoring
Both theoretical approaches assert that learners base self-regulation directly on metacognitive monitoring, a view that is supported by evidence that judgments of learning (JOLs) are dissociable from recall (e.g., Benjamin, 2003 ; Benjamin, Bjork, & Schwartz, 1998 ; Metcalfe, Schwartz, & Joaquim, 1993 ) but nonetheless correlated with later study choices ( Metcalfe & Finn, 2008 ). Because study choices appear to be directly based upon introspective monitoring of memory ( Nelson & Narens, 1994 ; Metcalfe & Finn, 2008 ), the effectiveness of self-regulation faces a major bottleneck in the accuracy of memory monitoring. Giving learners control over study allows biases and inaccuracies in metacognitive monitoring to influence performance negatively by leading learners to make suboptimal or counterproductive decisions while controlling their study.
Memory assessments are prone to inaccuracies, sometimes dramatically so. For example, poor students overestimate performance by up to an astonishing 30% of their actual test score ( Dunning, Johnson, Ehrlinger, & Kruger, 2003 ; Hacker, Bol, Horgan, & Rakow, 2000 ). An insidious problem is ineffective monitoring across materials within a task. Learners who exhibit poor relative accuracy (or resolution ) are not able to distinguish between materials that will be relatively easy to learn and ones that will be challenging. Judgments concerning levels of learning and states of knowledge exhibit poor resolution under many conditions ( Dunlosky & Nelson, 1992 ; Dunlosky & Nelson, 1994; Shaughnessy, 1981 ), and often do not appropriately reflect the influence of variables that affect performance considerably ( Benjamin, 2005 ; Koriat, Bjork, Sheffer, & Bar, 2004 ; Karpicke & Roediger, 2008 ; Zechmeister & Shaughnessy, 1980 , Roediger & Karpicke, 2006 ).
The sophistication of learners’ mental models and memory monitoring ability determines the potential effectiveness of self-guided learning. Whether the opportunity for control plays out in a net gain for self-controlled processing may depend on individual differences in metacognitive skill and the difficulty of the metacognitive aspects of the task. For example, learners who are afforded the opportunity for accurate metacognitive monitoring (by delaying the occasion of their JOL until well after the initial exposure) choose to re-study less well learned materials than learners who have no such diagnostic monitoring opportunity. The differences in accuracy of metacognitive monitoring lead to differences in re-study choices, which lead to differences in performance ( Thiede, Anderson, & Therriault, 2003 ).
Efficiency of self-paced study
Although there is much research concerning how subjects allocate study time across different materials ( Baker & Anderson, 1982 ; Maki & Serra, 1992 ), with different criterion tasks ( Le Ny, Denheire, & Taillanter, 1972 ; Zacks, 1969 ; Belmont & Butterfield, 1971 ), and even with varying subject populations ( Dufresne & Kobasigawa, 1988 , 1989 ; Kobasigawa & Metcalf-Haggert,1993 , Dunlosky & Connor, 1997 ), the basic question of whether people are effective at distributing their own study time compared to a group that does not control their own time distribution remains unresolved. Metcalfe and Kornell (2005) noted that, although there are ample data on how learners allocate study across items, “we still do not know whether what they do enhances their learning, or is in any way optimal.”
Only two studies ( Mazzoni & Cornoldi, 1993 ; Koriat, Ma'ayan, & Nussinson, 2006 ) have addressed whether people can effectively allocate their study time. Mazzoni and Cornoldi (1993) compared a group that was allowed to self-pace study with a control group that viewed words presented at a constant rate. The constant rate for subjects in that control group was determined by the average rate with which those subjects chose to study items in a previous list. The self-paced group recalled more words than the control subjects; however, Koriat, Ma'ayan, and Nussinson (2006) did not replicate this result when they compared a self-pacing group to a control group that was equated on total study time. In their experiment, each control subject was yoked to a self-pacing subject in terms of word order and total study time, but the study time was divided evenly between the words. With these differing results, it is difficult to conclude that allowing learners to self-pace actually benefits their overall performance. In this paper, we evaluate whether self-pacing is efficacious even when a strict total-study-time control is implemented.
Given the many conditions in which metacognitive monitoring is inaccurate, and the numerous opportunities for subjects to implement an ineffective control policy, it is not obvious that subjects can effectively allocate study time to improve their memory. In addition, it has been theorized that spending more time on difficult items may be unwise, since there are conditions under which those items provide a low rate of return ( Metcalfe & Kornell, 2003 ). By spending more time on the difficult items, self-pacing subjects will necessarily devote a smaller proportion of time to the easy- and medium-difficulty items, and this choice could adversely impact overall performance. In Experiment 1, we compare the memory of subjects who choose how to allocate their study time with the memory of subjects who spend the same total time as the self-pacers, but have no control over their study schedule. Control subjects viewed the same words as self-paced subjects, but viewed them for the average amount of time taken per word by their self-paced partner.
Experiment 1
We use a procedure similar to that of Koriat et al. (2006) , in which control subjects were yoked to self-paced subjects in total study time. Whereas previous studies have utilized free recall or cued recall to measure memory, we utilized recognition. There are numerous advantages to using recognition. First, because recognition testing affords fewer opportunities for test strategies to differ across subjects ( Benjamin, 2008 ), it allows us to more precisely focus on the effects of strategies employed during encoding. Second, because recognition tasks are metacognitively deceptive for learners—that is, because the modal expectation of a memory task appears to conform more to the demands of recall than recognition testing ( Benjamin, 2003 ; Glanzer & Bowles, 1976 ; Guttentag & Carroll, 1998 )—finding evidence for the effectiveness of metacognitive control on recognition meets a somewhat higher standard and should generalize to other memory tasks. Finally, motivated by the inconsistent prior findings using free recall, we sought a test paradigm with high power and in which decision strategies can be effectively corrected for. Recognition meets these dual constraints because many more items can be used than in recall paradigms, and because we can bring the Theory of Signal Detection ( Green & Swets, 1966 ; Macmillan & Creelman, 2005 ) to bear on eliminating individual differences in the decision component of the recognition task (but see Benjamin, Diaz, & Wee, 2009 ).
Participants
One-hundred and forty eight introductory-level psychology students from the University of Illinois participated in exchange for course credit.
One-hundred and sixty words were selected from the MRC Psycholinguistic Database ( Wilson, 1988 ), and ranged on measures of familiarity (range 214-657, mean = 495.00, SD = 111.52), concreteness (range 186-645, mean=444.83, SD = 163.18), and imageability (range: 210-667, mean= 467.48, SD = 142.15). For each pair of subjects, eighty of these words were randomly selected to comprise the study list. The selection and order of the presented words were randomized and the presentation list included four additional primacy and four recency buffer words that were excluded from analysis. All 160 words comprised the test list.
Design and Procedure
Subjects were run individually on desktop computers in individual rooms. The first subject in a given computer room was assigned to the self-paced condition. The next subject on that computer arrived after the first subject completed the experiment and was assigned to the fixed-rate condition. Thereafter, subjects were alternately assigned to self-paced and fixed-rate conditions. The first subject in each pair studied each word for as long as they chose and proceeded to the next word by pressing the space bar. The fixed-rate subjects viewed the same list of words in the same order as their yoked partners, but each word they viewed was presented for a constant amount of time, determined by calculating the average amount of time per word taken by the yoked subject. Words were presented individually in the middle of a white computer screen in black, Times New Roman, 60 point font. All subjects were instructed to “do your best to remember the words for a later memory test.”
After viewing the target list of 80 words, subjects engaged in a recognition task that included the 80 studied items and 80 previously unstudied items. The order of the 160 words was randomly determined and each word remained on the screen until subjects provided a recognition judgment on a scale of 1 to 4. This scale ranged from “I am certain I have not seen that word” (1) to “I think I have not seen that word” (2) to “I think I have seen that word” (3) to “I am certain I have seen that word” (4). Each yoked pair of subjects received the test words in the same order. Confidence ratings were used to generate a measure of discrimination (d a ) between old and new items based on unequal-variance signal-detection theory ( Green & Swets, 1966 ; for a discussion of the advantages of such a measure in recognition, see Matzen & Benjamin, 2009 )
All statistics reported here are significant at an α < .05 level. Mean discriminability (d a ) is shown in the left panels of Figure 1 (subject analysis) and Figure 2 (item analysis), and hit and false alarm rates are shown in Table 1 . Self-paced subjects revealed significantly higher performance than their paired controls (d a[selfpaced] =1.73 [SEM=0.07], d a[fixed-rate] =1.51 [SEM=0.07]; t [73] 1 = 2.20) despite being equated on total study time, revealing that the individual control of study time is beneficial for learning. A similar result is obtained following an item analysis, in which discrimination was calculated for each word once from the combined responses of the entire group of self-paced subjects and once from the combined responses of the entire group of control subjects. Item discriminability calculated from the self-paced subjects was significantly greater than that computed from the control subjects (d a =1.58 [SEM=0.06], d a =1.43 [SEM=0.05]; t [159]=4.25).

Left panel: Item discriminability as a function of condition based on a subject analysis. Right panels: Discriminability for discrepancy reducers (top) and discrepancy increasers (bottom).

Left panel: Item discriminability as a function of condition based on an item analysis. Right panels: Discriminability for items as calculated by discrepancy reducers (top) and discrepancy increasers (bottom).
Percent hits and false alarms (and standard deviations) for Experiments 1 & 2.
Benefits of self-pacing as a function of individual differences in control strategy
We mentioned previously that individual differences in how study time is allocated are likely to affect the outcome of experiments in which strategic control is experimentally manipulated. Here we consider study time allocation as a function of item difficulty and classify learners into two general categories. Learners who spent more time studying the more normatively difficult items were considered discrepancy reducers ( Dunlosky & Thiede, 1998 ), and learners who allocated more time to easier items were considered to be discrepancy increasers .
To evaluate an individual subject's allocation policy, we calculated the correlation across items between allotted study time and normative item difficulty, d a , as assessed by mean performance on that item by the fixed-rate control group. The average correlation between study time and normative word difficulty for all subjects in the self-paced condition was not significantly different than zero (r = 0.02, SD= 0.13). Thirty-five subjects exhibited negative correlations, classifying them as discrepancy reducers, and 39 subjects revealed positive correlations, classifying them as discrepancy increasers.
The performance of each of these subsets of subjects is compared to their yoked controls, and the mean levels are shown in the right panels of Figures 1 and and2. 2 . Discrepancy reducers exhibited reliably higher levels of discriminability than their yoked controls (d a [reducers] =1.77 [SEM=0.10], d a [yoked to reducers] =1.42 [SEM=0.10]; t[34] = 2.41), but discrepancy increasers did not (d a [increasers] =1.69 [SEM=0.11], d a [yoked to incressers] =1.59 [SEM=0.10]; t[38] = 0.75). An item analysis based on the same breakdown of subjects is shown in Figure 2 , and replicates this finding (d a[reducers] =1.62 [SEM=0.05], d a[yoked to reducers] =1.30 [SEM = 0.05]; t[159] = 6.04; d a[increasers] =1.49 [SEM=0.05], da [yoked to increasers] =1.47 [SEM=0.05]; t[159] = 0.38, respectively). These results hint that allocation strategy modulates the benefits that self-pacing provides. A 2-way ANOVA on self-pacing condition (self-pacing or yoked) and allocation strategy (reducers or increasers) showed only a significant effect of self-pacing condition (F(1,72)=5.20) indicating that self-pacing benefits memory performance. Given that the ANOVA did not reveal a significant interaction between condition and strategy, the modulating effect of self-paced strategy is unclear; we will further investigate the impact of differing allocation strategies on performance in Experiment 2.
Self-paced learning significantly improves memory performance compared to a control condition in which the same overall study time was used but time was not differentially allocated across items. By choosing how to distribute study time, subjects outperformed others who spent the same total time studying the material. The improvement seemed to be driven principally by the subjects who devoted more time to difficult words than to easy words.
Metacognition can be used effectively to improve cognition. However, only some learners utilize metacognition effectively as the strategy utilized during self-paced learning modulates the benefits that self-pacing provides. The act of self-pacing in and of itself does not improve memory; rather, self-pacing yields improvements only under certain circumstances. Some learners utilize suboptimal self-regulation techniques and perform no better than those who do not regulate their learning. Suboptimal metacognition may result from a lack of motivation or of knowledge; self-pacers may not have the motivation to monitor and control their learning or may not have the requisite knowledge to do so effectively. This motivation hypothesis will be contradicted, however, by evidence in the general discussion.
One concern in this experiment is that the difference between the yoked control groups is as large as the difference between the discrepancy reducers and increasers. Because subjects were not randomly assigned to the allocation variable, and consequently neither were the yoked controls, such an effect can reflect uncontrolled differences in study-list composition. One goal of Experiment 2 is to replicate Experiment 1 with sufficient power to directly compare discrepancy reducers and discrepancy increasers, thus evading concerns over the role of the control group in undergirding this individual-difference effect.
In addition, we evaluate whether the advantage of self-pacing arises only from the fact that normatively more difficult items are studied for greater amounts of time, or whether idiosyncratic differences in difficulty are also important. To test these hypotheses, Experiment 2 included a condition in which study times are automatically distributed based on normative item difficulty.
This contrast is relevant to educational and technological decisions concerning the best way of implementing study regimens. If it is the case that normative difficulty is an effective proxy for idiosyncratic difficulty, then automated study routines can bypass self-control—which may be unduly influenced by individual differences in motivation or other factors—in developing an effective study regimen. However, if intraindividual differences in item difficulty are high compared to intraitem differences in normative difficulty, then such a short-cut will not prove effective.
Experiment 2
It is unclear whether the opportunity to self-regulate in a word-learning task affords any advantages over a regimen in which normatively difficult items are allotted greater study time. There are strong reasons to suspect that such normative allotment of study time may be superior. First, the tacit individual assessments of item difficulty that precede study-time allocation are prone to the many biases and inadequacies discussed earlier, whereas normative data on item memorability are not susceptible to such sources of error. Second, control over self-pacing allows for individual differences in metacognitive sophistication to play a major role; when study times are allocated automatically, individual differences in allocation policy are irrelevant, and any beneficial effects will likely be evident for a larger subset of subjects. On the other hand, there is also one major reason to predict that normative allocation of study time will be inferior to self control. If idiosyncratic individual differences in item difficulty are larger than intra-list variance in normative item difficulty, normative assessments of that difficulty will be inadequate for a large subset of subjects.
We will evaluate these hypotheses in this experiment by forcing subjects in one condition to adopt a discrepancy reduction strategy based upon normative difficulty. We are interested in whether self-pacers’ performance can be improved by tying their study time to item difficulty, such that everyone in this condition becomes a perfect discrepancy reducer. The materials and procedure for the second experiment were the same as those used in the first experiment with an additional condition in which presentation times were determined based on an algorithm relating normative discriminability (from the control group in Experiment 1) to study time.
Two hundred and thirty-four introductory-level psychology students from the University of Illinois participated in exchange for course credit.
Subjects were assigned to groups of three based upon the order of their arrival; the first subject completing the experiment in each individual room was assigned to the self-paced condition, the second to the fixed-rate condition, and the third to the normative allotment condition. The latter two subjects were given the same total study time as taken by the self-paced subject in that group. The self-paced and fixed-rated conditions were the same as in Experiment 1, and the third condition provided differential study times based on normative difficulty. The presentation times were scaled in this group such that words of normatively lower discriminability were displayed for longer times than words of higher discriminability. We calculated the presentation times with the following formula:
in which ST i is the study time for item i, TT is the total study time allotted to this subject, and d a is the detection-based measure of memory discriminability for that item (based on performance by the control group in Experiment 1). The parameter v was manipulated slightly (v= 2.5 in the high variability group and v= 1.5 in the low variability group) across two different subgroups of subjects. The former value was chosen for half of the subjects in order to match the standard deviation of presentation times for the normative-allotment subjects to the standard deviation of study times that resulted from self-paced subjects in Experiment 1. However, when the large disadvantage in performance in this condition became evident, the value of v was reduced in order to decrease the variability in presentation times across items. It was a concern that the unpredictability in study time per item might be so jarring that performance would suffer, so we reduced the variability of presentation times for half of the subjects.
Within the normative-allotment group, subjects’ overall discriminability did not differ between the high and low variability groups (d a[high] =0.93 [SEM=0.07], d a[low] =1.06 [SEM=0.08]; t[76] = 1.12), so subjects were combined across the levels of this variable. Performance in all three conditions is shown in Figures 1 and and2. 2 . A one-way ANOVA revealed a significant effect of condition (F(2,75)=11.16) . Post-hoc comparisons revealed that self-paced subjects showed higher discriminability than the control group (d a[self-paced] =1.48, d a[fixed-rate] =1.27, [SEM=0.11]; t[77] = 1.99; p=0.05), as in Experiment 1, and subjects in the normative-allotment condition showed lower discriminability (d a =0.99) than both the self-paced ([SEM=0.11]; t[77]=4.41) and control ([SEM=0.10]; t[77] = 2.76) subjects. As shown in Figure 2 , an item analysis obtained similar results, in which words were significantly easier to discriminate for self-paced subjects than for control (d a[self-paced] =1.39 , d a[fixed-rate] =1.21 [SEM=0.03]; t[159] = 4.21) or the normative-allotment (d a =0.93, [SEM]=0.03; t[159]=10.59) subjects. Words were also significantly easier to discriminate for control subjects than for the normative-allotment subjects ([SEM=0.03], t[159]=8.49).
Self-paced subjects were again divided into two groups based on the correlation between study time and item difficulty as measured in the control group. In Experiment 2, the average correlation between item difficulty and study time allocation was again not different than zero (mean r = 0.03, SD = 0.12, t(77)=1.78). Thirty out of 78 self-paced subjects exhibited a negative correlation between discriminability and self-paced study time and were therefore classified as discrepancy reducers. As shown in the right panels of Figures 1 and and2, 2 , and replicating what was seen in Experiment 1, only the discrepancy reducers exhibited better memory performance than their control subjects (discrepancy reducers: (d a [self-paced] =1.77, d a[fixed-rate] =1.17 [SEM=0.17], t[37]=3.47; discrepancy increasers: (d a [self-paced] =1.30 d a [fixed-rate] =1.33 [SEM=0.13]; t[47]=0.22). In contrast to Experiment 1, a direct comparison between self-paced discrepancy reducers and self-paced discrepancy increasers showed a significant advantage for discrepancy reducers (t(77)=2.30). Importantly, neither the control nor normative-allotment subjects yoked to the discrepancy reducers differed from those yoked to discrepancy increasers in performance (t[77] = 1.01; t[77] = 0.72 respectively), indicating that differences in total study time and idiosyncratic item selection did not play a role in producing the advantage for subjects exerting self-control over study.
The results of the Experiment 2 replicate those of Experiment 1: self-pacers exhibited superior recognition to control subjects, and this effect was driven by the subset of self-pacers who were discrepancy reducers. Because the benefits of discrepancy reducers are entirely correlational, however, it is impossible to conclude with any certainty that the strategy itself—rather than any correlated intellectual or motivational variables—fostered the performance advantage. These third variables will be considered more closely in the General Discussion. Experiment 2 also shows that subjects in the normative-allotment condition performed significantly worse than those in the other two conditions. This surprising result indicates that an allocation policy based on normative difficulty is not superior to one completely controlled by subjects and based upon their idiosyncratic metacognitive judgments.
General Discussion
In both Experiments 1 and 2, recognition performance for learners who controlled their own study time was compared to learners who spent the same amount of overall study time but viewed the items for a standard amount of time. Learners with control of study-time allocation significantly outperformed subjects with no control, even when the total study time was equated between groups. This effect was driven only by those subjects who allocated their time in a manner consistent with a discrepancy-reduction strategy. In the second experiment, subjects in the third condition (normative-allotment subjects) viewed each item for a length of time determined by that item's objective difficulty. These learners performed significantly worse than both self-pacers and control subjects.
When data from the two experiments are combined, self-paced discrepancy reducers showed higher discriminability than self-paced discrepancy increasers (d a[reducrers] =1.77, d a[increasers] =1.53 t(153)=2.14), but the control subjects yoked to the reducers did not perform differently than control subjects yoked to the increasers (d a[yoked to reducers]= 1.32, d a[yoked to increasers] =1.45 t(153)=1.32). Further, a 2-way ANOVA on self-pacing condition (self-paced or yoked) and allocation strategy (reducer or increaser) from the data combined across both experiments reveals both a benefit of self-pacing (F(1,150)=11.88) and an interaction between self-pacing condition and allocation strategy (F(1,150)=9.30). This result indicates that the strategy utilized during self-paced learning modulates the benefit of having control and allays our concern over the reliability of the individual-difference effect that was questionable in the Experiment 1 data alone.
Giving learners more control over their study behavior resulted in better memory performance, even without increasing total study time. Evidence presented here supports a growing trend to trust the metacognitive capabilities of learners and accordingly allow more self-regulation during learning ( Finley, Tullis, & Benjamin, 2009 ; Kornell & Bjork, 2007 ; Kornell & Metcalfe, 2006 ). Although they must devote resources to the act of self-regulation, subjects with the opportunity to regulate study time still outperformed their yoked counterparts.
Control of one's own study, however, proves not inherently advantageous; specific strategies mediate the effectiveness of control. In these experiments, only discrepancy reducers outperformed their yoked partners and discrepancy reducers made up less than half of all the self-paced subjects tested. In addition to strategy utilized during learning, other individual differences exist in the effectiveness of implementing control. The degree to which the metacognitive strategy is implemented and the level of modulation utilized by the learner may influence the effectiveness of control. For example, older adults are shown to modulate their self-paced study time to a lesser extent than younger adults, and some researchers have suggested that older adults’ deficient utilization of control during study contributes to their deficits in memory performance ( Dunlosky & Connor, 1997 ).
Benefits of self-pacing
In order to more closely examine the advantages evidenced by self-paced subjects, we conducted an analysis of the relationship between normative item difficulty (estimated from subjects in the control condition of Experiments 1 and 2) and the difficulty experienced by self-paced subjects across both experiments. We have plotted item difficulty as calculated from self-paced subjects against item difficulty as calculated from control subjects in Figure 3 . If self-pacing is advantageous for items across the difficulty spectrum, then the function relating item difficulty between the groups will lie parallel to the major diagonal but have a higher intercept. If self-pacing affects item difficulty differently for easy than for difficult items, the slope of the line will significantly differ from the diagonal. Several important effects are evident in Figure 3 . First, the intercept of this line is higher than 0, indicating that self-pacing reduced the overall difficulty of the items (intercept = 0.34; SE = 0.08; t(157)=4.46). Further, the slope of the line for self-paced subjects is shallower than the diagonal (slope=0.88; SE=0.05; t(157)=2.14), revealing that self-pacing reduced the heterogeneity of item difficulties. Self-pacing improved performance on difficult items to a greater extent than on the easier items. The position of this function reveals that self-pacers outperformed yoked, fixed-rate subjects by increasing performance for the normatively difficult items without sacrificing performance on the easier items.

Item discriminability as computed from self-paced subjects plotted against item discriminability as computed from control (fixed-rate) subjects.
Selection of allocation policy
Across both experiments, less than half of the self-pacers engaged in a discrepancy-reduction strategy. The failure to choose the more beneficial strategy could be caused by several things. Subjects may lack the motivation or knowledge to implement a good strategy, have low performance goals, or attempt to implement a discrepancy reduction strategy but fail to accurately monitor item difficulty.
Two pieces of evidence suggest that the differences between discrepancy increasers and reducers are not motivational. A comparison of the standard deviations of the presentation times that result from discrepancy reducers’ and increasers’ allocation behavior reveals no significant differences between the extent to which increasers and reducers regulate their learning (2.59 vs. 2.22; t[151]=0.83). If one assumes that lower motivation would translate into less variability in study time across items, then this result suggests that neither group was more motivated than the other. This result is difficult to interpret however, since it is a null result, and the power to detect a medium-sized effect (Cohen, 1988) is only 0.86. In addition, the time spent per word did not differ significantly between discrepancy increasers and reducers (t [increaser] =3.1 s vs. t [reducer] =3.8 s, t[151]=1.68, p = 0.10). Discrepancy reducers, then, do not appear to exhibit greater levels of motivation during study, as they spend similar amounts of time studying and modulate their learning to roughly the same degree as discrepancy increasers.
Previous research shows that subjects overwhelmingly choose to devote more time to items that they judge to be difficult ( Son & Metcalfe, 2000 ), which suggests that the failure of subjects to follow a discrepancy reduction strategy may result from a lack of awareness of which items will be difficult at the time of the test or from low performance goals (Dunlosky & Thiede, 2004). Dunlosky and Thiede (2004) showed that when given significant time constraints or very low performance goals, subjects shift their study strategies from spending more time on difficult items to studying only the easy-to-master subset of materials. Discrepancy increasers in the current study may be subjects who adopted low performance goals and therefore devoted time disproportionately to the easier items. This line of reasoning suggests that discrepancy increasers would likely spend less time overall studying. The analysis presented previously does not support this claim, though the effect is numerically in the predicted direction and not trivial in magnitude. On the basis of the current evidence, both the motivational and low performance explanations seem unlikely; the most viable explanations remaining are that subjects are discrepancy increasers because they cannot predict individual items’ test difficulty during study or because they employ a bad metacognitive strategy.
Benefits of self-regulation of study time
Learning can be enhanced through successful implementation of self-guided study time allocation. But, as Metcalfe (2009) argues, there are two necessary components for control of study to be helpful: monitoring must be accurate, and appropriate choices must be implemented during study. Without accurate monitoring, appropriate choices can neither be made nor implemented. In this study, less than half of all subjects implemented an effective study strategy. Improvements in metacognition could result in more successful and efficient learning, both in and out of the classroom. Flavell (1979) offers a broad, hopeful vision for the usefulness of metacognitive instruction, suggesting that metacognitive ideas “could be parlayed into a method of teaching children (and adults) to make wise and thoughtful life decisions as well as to comprehend and learn better in formal educational settings” (p.910). For individuals who have deficits in their ability to monitor or control study, direct instruction or experience in metacognitive monitoring and allocation strategies can improve student's choices and learning (see Finley et. al., 2009 ). Information technology also plays a promising role in training metacognitive monitoring and control, with the hope that student learning will be enhanced (for examples see Finley et al., 2009 ). In these experiments, allowing learners control over their own learning boosted performance, and the control strategy utilized during self-regulated learning modulated this boost. This research thus links metacognitive control with performance, showing that differences in how control is utilized correspond to differences in memory. Overall, trusting learners with control over their own learning has the potential to improve their learning.
Acknowledgments
This research was funded in part by grant R01 AG026263 from the National Institutes of Health. We thank the members of the Human Memory and Cognition Laboratory at the University of Illinois, Urbana-Champaign for their helpful insights, comments, and criticism.
Publisher's Disclaimer: This is a PDF file of an unedited manuscript that has been accepted for publication. As a service to our customers we are providing this early version of the manuscript. The manuscript will undergo copyediting, typesetting, and review of the resulting proof before it is published in its final citable form. Please note that during the production process errors may be discovered which could affect the content, and all legal disclaimers that apply to the journal pertain.
1 In a design like this one, one pair of yoked subjects is treated as a single unit of analysis. A traditional between-subjects comparison would not account for the pairwise yoking in the design.
- Baker L, Anderson RI. Effects of inconsistent information on text processing: Evidence for comprehension monitoring. Reading Research Quarterly. 1982; 17 :281–294. [ Google Scholar ]
- Belmont JM, Butterfield EC. Learning strategies as determinants of memory deficiencies. Cognitive Psychology. 1971; 2 :411–420. [ Google Scholar ]
- Benjamin AS. Predicting and postdicting the effects of word frequency on memory. Memory & Cognition. 2003; 31 :297–305. [ PubMed ] [ Google Scholar ]
- Benjamin AS. Response speeding mediates the contribution of cue familiarity and target retrievability to metamnemonic judgments. Psychonomic Bulletin & Review. 2005; 12 :874–879. [ PubMed ] [ Google Scholar ]
- Benjamin AS. Memory is more than just remembering: Strategic control of encoding, accessing memory, and making decisions. In: Benjamin AS, Ross BH, editors. The Psychology of Learning and Motivation: Skill and Strategy in Memory Use. Vol. 48. Academic Press; London: 2008. pp. 175–223. [ Google Scholar ]
- Benjamin AS, Bird RD. Metacognitive control of the spacing of study repetitions. Journal of Memory and Language. 2006; 55 :126–137. [ Google Scholar ]
- Benjamin AS, Bjork RA, Schwarz BL. The mismeasure of memory: When retrieval fluency is misleading as a metamnemonic index. Journal of Experimental Psychology: General. 1998; 127 :55–68. [ PubMed ] [ Google Scholar ]
- Benjamin AS, Diaz ML, Wee S. Signal detection with criterion noise: Applications to recognition memory. Psychological Review. 2009; 116 :84–114. [ PMC free article ] [ PubMed ] [ Google Scholar ]
- Dunlosky J, Connor LT. Age differences in the allocation of study time account for age differences in memory performance. Memory & Cognition. 1997; 25 :691–700. [ PubMed ] [ Google Scholar ]
- Dufresne A, Kobasigawa A. Developmental differences in children's allocation of study time. The Journal of Genetic Psychology. 1988; 149 :87–92. [ Google Scholar ]
- Dufresne A, Kobasigawa A. Children's spontaneous allocation of study time: Differential and sufficient aspects. Journal of Experimental Child Psychology. 1989; 47 :274–296. [ Google Scholar ]
- Dunlosky J, Nelson TO. Importance of the kind of cue for judgements of learning (JOLs) and the delayed JOL effect. Memory & Cognition. 1992; 20 :374–380. [ PubMed ] [ Google Scholar ]
- Dunlosky J, Theide KW. What makes people study more? An evaluation of factors that affect self-paced study. Acta Psychologica. 1998; 98 :37–56. [ PubMed ] [ Google Scholar ]
- Dunning D, Johnson K, Ehrlinger J, Kruger J. Why people fail to recognize their own incompetence. Current Directions in Psychological Science. 2003; 12 :83–87. [ Google Scholar ]
- Finley JR, Tullis JG, Benjamin AS. Metacognitive control of learning and remembering. In: Khine MS, Saleh IM, editors. New Science of Learning: Cognition, Computers and Collaboration in Education. Springer Science & Business Media; New York: 2009. [ Google Scholar ]
- Flavell JH. Metacognition and cognitive monitoring: A new area of cognitive-development theory. American Psychologist. 1979; 34 :906–911. [ Google Scholar ]
- Glanzer M, Bowles N. Analysis of the word frequency effect in recognition memory. Journal of Experimental Psychology: Human Learning & Memory. 1976; 2 :21–31. [ Google Scholar ]
- Green D, Swets J. Signal detection theory and psychophysics. John Wiley and Sons; New York: 1966. [ Google Scholar ]
- Guttentag R, Carroll D. Memorability judgments for high- and low-frequency words. Memory & Cognition. 1998; 26 :951–958. [ PubMed ] [ Google Scholar ]
- Hacker DJ, Bol L, Horgan D, Rakow E. Test prediction and performance in a classroom context. Journal of Educational Psychology. 2000; 92 :160–170. [ Google Scholar ]
- Karpicke JD, Roediger HL. The critical importance of retrieval for learning. Science. 2008; 319 :966–968. [ PubMed ] [ Google Scholar ]
- Kobasigawa A, Metcalf-Heggart A. Spontaneous allocation of study-time by first- and third- grade children on a simple memory task. The Journal of Genetic Psychology. 1993; 154 :223–235. [ Google Scholar ]
- Koriat A, Bjork RA, Sheffer L, Bar SK. Predicting one's own forgetting: The role of experience-based and theory-based processes. Journal of Experimental Psychology: General. 2004; 143 :643–656. [ PubMed ] [ Google Scholar ]
- Koriat A, Ma'ayan H, Nussinson R. The intricate relationships between monitoring and control in metacognition: Lessons for the cause-and-effect relation between subjective experience and behavior. Journal of Experimental Psychology: General. 2006; 135 :36–60. [ PubMed ] [ Google Scholar ]
- Kornell N, Bjork RA. The promises and perils of self-regulated study. Psychonomic Bulletin and Review. 2007; 14 :219–224. [ PubMed ] [ Google Scholar ]
- Kornell N, Metcalfe J. Study efficacy and the region of proximal learning framework. Journal of Experimental Psychology: Learning, Memory, & Cognition. 2006; 32 :609–622. [ PubMed ] [ Google Scholar ]
- Le Ny JF, Denheire G, Taillanter D. Regulation of study-time and interstimulus similarity in self-paced learning conditions. Acta Psychologica. 1972; 36 :280–289. [ Google Scholar ]
- Macmillan NA, Creelman CD. Detection Theory. 2nd ed. Lawrence Erlbaum; Mahwah, New Jersey: 2005. [ Google Scholar ]
- Maki RH, Serra M. The basis of test predictions for text material. The Journal of Experimental Psychology: Learning, Memory, and Cognition. 1992; 18 :116–126. [ Google Scholar ]
- Mazzoni G, Cornoldi C. Strategies in Study Time Allocation: Why is study time sometimes not effective? Journal of Experimental Psychology: General. 1993; 122 :47–60. [ Google Scholar ]
- Matzen LE, Benjamin AS. Remembering words not presented in sentences: How study context changes patterns of false memories. Memory & Cognition. 2009; 37 :52–64. [ PMC free article ] [ PubMed ] [ Google Scholar ]
- Metcalfe J. Metacognitive judgments and control of study. Current Directions in Psychological Science. 2009; 18 :159–163. [ PMC free article ] [ PubMed ] [ Google Scholar ]
- Metcalfe J, Finn B. Evidence that judgments of learning are causally related to study choice. Psychonomic Bulletin & Review. 2008; 15 :174–179. [ PubMed ] [ Google Scholar ]
- Metcalfe J, Kornell N. The dynamics of learning and the allocation of study time to a region of proximal learning. Journal of Experimental Psychology: General. 2003; 132 :530–542. [ PubMed ] [ Google Scholar ]
- Metcalfe J, Kornell N. A region of proximal learning model of study time allocation. Journal of Memory and Language. 2005; 52 :463–477. [ Google Scholar ]
- Metcalfe J, Schwartz BL, Joaquim SG. The cue-familiarity heuristic in metacognition. Journal of Experimental Psychology: Learning, Memory, & Cognition. 1993:851–861. [ PubMed ] [ Google Scholar ]
- Nelson TO, Dunlosky J, Graf A, Narens L. Utilization of metacognitive judgments in the allocation of study during multitrial learning. Psychological Science. 1994; 5 :207–213. [ Google Scholar ]
- Nelson TO, Narens L. Why investigate metacognition? In: Metcalfe J, Shimamura AP, editors. Metacognition: Knowing about knowing. MIT Press; Cambridge, MA: 1994. pp. 195–206. [ Google Scholar ]
- Roediger HL, Karpicke JD. Test-enhanced learning: Taking memory tests improves long term retention. Psychological Science. 2006; 17 :249–255. [ PubMed ] [ Google Scholar ]
- Shaughnessy JJ. Memory monitoring accuracy and modification of rehearsal strategies. Journal of Verbal Learning and Verbal Behavior. 1981; 20 :216–230. [ Google Scholar ]
- Son LK. Spacing one's study: Evidence for a metacognitive control strategy. Journal of Experimental Psychology: Learning, Memory, and Cognition. 2004; 30 :601–604. [ PubMed ] [ Google Scholar ]
- Son LK, Metcalfe J. Metacognitive and control strategies in study-time allocation. Journal of Experiment Psychology: Learning, Memory, and Cognition. 2000; 26 :204–221. [ PubMed ] [ Google Scholar ]
- Thiede KW, Anderson MCM, Therriault D. Accuracy of metacognitive monitoring affects learning of texts. Journal of Educational Psychology. 2003; 95 (1):66–73. [ Google Scholar ]
- Toppino TC, Cohen MS, Davis ML, Moors AC. Metacognitive control over the distribution of practice: When is spacing preferred? Journal of Experimental Psychology: Learning, Memory, and Cognition. 2009; 35 :1352–1358. [ PubMed ] [ Google Scholar ]
- Wilson MD. The MRC Psycholinguistic Database: Machine Readable Dictionary, Version 2. Behavioural Research Methods, Instruments and Computers. 1988; 20 :6–11. [ Google Scholar ]
- Zacks RT. Invariance of total learning time under different conditions of practice. Journal of Experimental Psychology. 1969; 32 :441–447. [ Google Scholar ]
- Zechmeister EB, Shaughnessy JJ. When you know that you know and when you think that you know but you don't. Bulletin of the Psychonomic Society. 1980; 15 :41–44. [ Google Scholar ]
You are using an outdated browser. Please upgrade your browser to improve your experience.
EFFECTIVENESS OF SELF LEARNING MODULES (SLM) IN TEACHING MATHEMATICS 3
- Sandra M. Benito 1
- Johhny S. Bantulo 1
- Fortunata S. Haudar 1
- 1. Ramon Magsaysay Memorial Colleges- Graduate School General Santos City, Philippines
Description
Abstract: This study aimed to find out whether the use of self-learning modules was effective in improving the academic performance among learners of grade 3. The subjects of the study were thirty-four (35) learners of Mamangos Maulana Kandog Elementary school for the school year 2020-2021 at Kiamba, Sarangani Province, Philippines. The study used the experimental design. Utilizing the frequency counts, and t-test for correlation of big size sample. The findings of the study revealed that the most of the grade 3 learners improved after the use of learners in Mathematics subject.
Keywords: educational management, effectiveness, self-learning module, Mathematics 3, Philippines.
Title: EFFECTIVENESS OF SELF LEARNING MODULES (SLM) IN TEACHING MATHEMATICS 3
Author: Sandra M. Benito, Johhny S. Bantulo, Fortunata S. Haudar
International Journal of Recent Research in Thesis and Dissertation (IJRRTD)
Vol. 3, Issue 1, January 2022 - June 2022
Page No: 33-45
Paper Publications (Publisher)
Website: www.paperpublications.org
Published date: 27-April-2022
DOI: https://doi.org/10.5281/zenodo.6497186
Paper Download Link (Source): https://www.paperpublications.org/upload/book/EFFECTIVENESS%20OF%20SELF-27042022-4.pdf
EFFECTIVENESS OF SELF-27042022-4.pdf
Files (925.7 kb), additional details, related works.
- [1] Abiri, E., Darabi, A., & Salem, S. (2018). Design of multiple-valued logic gates using gate-diffusion input for image processing applications. Computers & Electrical Engineering, 69, 142- 157.
- [2] Ackerman, R., & Thompson, V. (2017). Meta-reasoning: Shedding meta-cognitive light on reasoning research. The Routledge international handbook of thinking and reasoning, 1-15.
- [3] Aikenhead, G. (2021). A 21st century culture-based mathematics for the majority of students. Philosophy of Mathematics Education Journal, (37).
- [4] Agaton, C., and Cueto, L. (2021). Learning at home: Parents lived experiences on distance learning during Covid-19 pandemic in the Philippines. Learning, 10(3).
- [5] Alea, L., Fabrea, M., Roldan, R.,& Farooqi, A. (2020). Teachers' Covid-19 awareness, distance learning education experiences and perceptions towards institutional readiness and challenges. International Journal of Learning, Teaching and Educational Research, 19(6), 127-144.
- [6] Almeida, D., Byrne, A., Smith, R., & Ruiz, S. (2021). How relevant is grit? The importance of social capital in first-generation college students' academic success. Journal of College Student Retention: Research, Theory & Practice, 23(3), 539-559.
- [7] Ambayon, E. &Millenes, C. (2020). Modular-based approach and students' achievement in literature. Available at SSRN 3723644.
- [8] Amirali, M. (2015). Students, concepts of the nature of mathematics and attitude towardsmathematics learning. Journal of Research and Reflection in Education. 4(1), 27.
- [9] Anderson, L. (2021). Schooling interrupted: Educating children and youth in the COVID-19 Era. Center for Educational Policy Studies Journal, 11 (Sp. Issue), 17-38.
- [10] Aquino, J. (2020). Modular Approach in Teaching With The End-In-Review Of Proposing A Module In Organic Chemistry. Journal of Critical Reviews, 7(17), 2114-2126.
- [11] Armstrong, P. (2015). Bloom's Taxonomy. Vanderbilt University Center for Teaching. Retrieved from https://cft . vanderbilt.edu/guides-sub pages/blooms-taxonomy/.
- [12] Atmojo, A. &Nugroho, A. (2020). EFL classes must go online! Teaching activities and challenges during COVID-19 pandemic in Indonesia. Register Journal, 13(1), 49-76.
- [13] Asgar, A., & Satyanarayana, R. (2021). An evaluation of faculty development programme on the design and development of self-learning materials for open distance learning. Asian Association of Open Universities Journal.
- [14] Babar, S. (2015). Relationship between methods of mathematics teaching at B.ed teacher training programme and its applications in the actual classrooms (Un-Published Thesis). Islamabad: AIOU
- [15] Bai, X., & González, O. (2019). A comparative study of teachers' and students' beliefs towards teacher-centered and learner-centered approaches in grade 12 English as a foreign language class at one governmental senior secondary school in Shaan'xi Province, China. Scholar: Human Sciences, 11(1), 37-37.
- [16] Ballane, G. (2019). Understanding of Self-Confidence in High School Students (Doctoral dissertation, Walden University).
- [17] Baturay, M. &Toker, S. (2019). Internet addiction among college students: Some causes and effects. Education and Information Technologies, 24(5),2863-2885.
- [18] Berlinghoff, W. &Gouvêa, F. (2021). Math through the Ages: A Gentle History for Teachers and Others Expanded Second Edition (Vol. 32). American Mathematical Soc.
- [19] Bishara, S. (2018). Active and traditional teaching, self-image, and motivation in learning math among pupils with learning disabilities. Cogent Education, 5(1), 1436123
- [20] Bledsoe, T. & Baskin, J. (2014). Recognizing student fear: The elephant in the classroom. College Teaching, 62(1), 32-41.
This site uses cookies. Find out more on how we use cookies
- Open access
- Published: 16 October 2021
Mathematics self-concept and challenges of learners in an online learning environment during COVID-19 pandemic
- Rex Bringula ORCID: orcid.org/0000-0002-1789-9601 1 ,
- Jon Jester Reguyal 1 ,
- Don Dominic Tan 1 &
- Saida Ulfa 2
Smart Learning Environments volume 8 , Article number: 22 ( 2021 ) Cite this article
171k Accesses
23 Citations
1 Altmetric
Metrics details
In this mixed-methods research, the relationship between four factors of individual online learners and their mathematics self-concept was explored. In addition, the challenges the students faced in learning mathematics online during the Coronavirus disease (COVID-19) pandemic were determined. The participant students were from two mathematics classes offered online during the summer of 2020. Pure online classes were first offered during this period because face-to-face learning sessions were suspended due to the COVID-19 pandemic. It was found that students owned the devices they were using for online classes. Internet connection and power interruption were the most problematic aspects of online learning. Students had positive as well as negative mathematics online learning self-concepts. Individual factors were partly related to mathematics self-concept. Qualitative data shows that students faced technological, personal, domestic, assessment, pedagogical, consultation, and test anxiety challenges. Implications and recommendations for teaching mathematics in an online environment are offered.
Introduction
Academic self-concept (ASC) is one's academic self-perceptions of one's general ability in school (Shavelson et al., 1976 ). It also refers to the interests of the students towards a particular course (Joyce & Yates, 2007 ). This idea has been widely investigated in the field of mathematics that eventually forms a field of study named mathematics self-concept (Lee & Kung, 2018 ; Pajares & Miller, 1994 ; Reyes, 1984 ). Mathematics self-concept has “to do with how sure a person is of being able to learn new topics in mathematics, perform well in mathematics class, and do well on mathematics tests” (p. 560, Reyes, 1984 ). It also refers to an individual's perception of his/her abilities related to mathematics as compared to others (Bong & Skaalvik, 2003 ). It is related to mathematics achievement (Lee & Kung, 2018 ). Thus, it is important to understand how students perceived their mathematics learning abilities.
However, these studies (e.g., Bong & Skaalvik, 2003 ; Lee & Kung, 2018 ; Pajares & Miller, 1994 ; Reyes, 1984 ) were investigated in the context of face-to-face learning where students can seek immediate learning interventions from teachers or their classmates. Moreover, while prior works (e.g., Baticulon et al., 2021 ; Fabito et al., 2021 ) outlined the barriers to online learning in the context of the COVID-19 pandemic, the mathematics self-concept in the context of online learning is not yet investigated. The sudden shift from face-to-face to online learning platforms posed challenges to students. Shifting to an online learning platform requires access to a device, Internet, and physical learning space, and a strong habit of learner’s autonomy. Due to the digital divide, device ownership is a persistent challenge in the effective implementation of online learning. For developing countries, intermittent Internet connection remains a barrier to effective online learning (Salac & Kim, 2016 ).
The shift from a classroom environment to a home learning environment raises another concern for students. Students that have no access to a personal physical online learning environment could be disrupted by noise and other distractions (Baticulon et al., 2021 ; Bringula et al., 2021 ). The pedagogical style also changed. In particular, there are parts of the content of the syllabus that students have to learn on their own (i.e., asynchronous session)—tasks that may not be a practice in a face-to-face setup. The ability of the students to learn and study course material during asynchronous sessions poses difficulties to online learners. These barriers are found to hinder the effective implementation of online learning. However, it is unclear whether these variables have a significant relationship with the students’ mathematics online learning self-concept (i.e., perceived abilities of the students to learn online mathematics online courses).
In other words, while technological, personal, institutional, and community barriers are identified in online learning in this time of the COVID-19 pandemic (Baticulon et al., 2021 ; Fabito et al., 2021 ), the relationship of these constraints to the mathematics self-concept of the students in a fully online class is still unknown. In addition, mathematics self-concept in an online learning setup is not considered in the existing guidelines on improving mathematics online education (e.g., Lee & Kung, 2018 ). Understanding the mathematics self-concept of the students at the early stages of the implementation of online learning could inform educators to improve or diversify their mathematics online teaching strategies. Hence, it is imperative to understand the relationship of these variables with the mathematics self-concept of the students in an online learning environment.
The findings of this study will benefit the teachers, the students, and the schools. Teachers may apply the recommended approach derived from the findings of the study in improving their online pedagogies. Schools may utilize the findings of the study in providing institutionalized strategies in addressing students’ online learning mathematics self-concept. In turn, students' online mathematics self-concept may be improved and students' mathematics learning may be achieved. Consequently, students' attrition to mathematics online learning courses may be lessened.
This study attempted to contribute to the existing threads of discussion of mathematics self-concept in the context of a fully online learning environment. The study investigated the mathematics self-concept of two classes of computing students during the summer of 2020. The results of the study served as a basis in the formulation of a checklist of suggestions for online mathematics teachers. Specifically, the study aims to answer the following research questions (RQ). (RQ) (1) What are the online learners-related factors in terms of device ownership, perceived Internet speed, personal physical learning space access, and mathematics learning autonomy? (2) What is the mathematics self-concept of online learners in terms of mathematics ability, mathematics interest, and perceived mathematics performance? (3) Is there a significant relationship between learner-related factors and mathematics self-concept? (4) Is there a significant difference in the mathematics self-concept of online learners between those with personal learning space and those without personal learning space?, and (5) What are the experiences of the respondents in terms of challenges in online learning?
Literature review
Online mathematics education.
Berge et al. ( 2000 ) set forth the following recommendations for teachers to ensure effective implementation of online learning courses: (1) state the hardware and software requirements of the course, (2) be available for consultation, (3) be creative in interacting with students online, (4) provide course performance feedback, (5) listen to student concerns and encourage class participation, (6) establish clear policies, goals, course objectives, and course expectations, (7) be knowledgeable with the online learning software, (8) use different pedagogical styles, (9) encourage collaboration among students, and (10) be proactive and solve problems to avoid escalation. Meanwhile, Herrington et al. ( 2004 ) suggested that online activities should be composed of relevant, ill-defined, varied, and complex tasks that could be integrated across different subject areas. Moreover, teachers may also provide activities supporting opportunities for collaboration and reflection.
Different studies reported the factors that influenced the achievement of online mathematics education students. The results of these studies served as focal points on improving online mathematics teaching. The study of Wadsworth et al. ( 2007 ) disclosed that four learning strategies (motivation, concentration, information processing, and self-testing) and self-efficacy predicted online mathematics grade achievement. They suggested that online mathematics educators provided real-world examples and conduct meetings with students regarding learning strategies. In a similar study, Glass and Sue ( 2008 ) showed that assignments were the most preferred learning object and had the most impact on learning. Thus, adequate practice drills and timely feedback were necessary for online mathematics education. The findings of Wadsworth et al. ( 2007 ) and Glass and Sue ( 2008 ) are consistent with the guidelines of Herrington et al. ( 2004 ).
Güzeller and Akin ( 2012 ) compared the mathematics achievement, attitudes, anxiety, and self-efficacy of students in the web-based mathematics instructions (WBMI) and traditional mathematics instructions (TMI). It was found that there was a significant difference between the WBMI and TMI in terms of achievement, attitudes, anxiety, and self-efficacy, having more favorable results on the WBMI. Students were advised to take the WBMI to familiarize themselves with this platform. Kim et al. ( 2014 ) extended the study of Güzeller and Akin (2012) by investigating the impact of anxiety and other forms of academic emotions (anger, shame, boredom, enjoyment, and pride) on online mathematics achievement. Kim et al. ( 2014 ) further hypothesized that motivation, self-efficacy, and cognitive processes (e.g., cognitive strategy, self-regulated learning) influenced online mathematics achievement. Academic emotions were accounted for 37% of the variation in student achievement.
Finally, Cho and Heron ( 2015 ) determined the impact of self-regulated learning (SRL), learning strategies, and emotions on satisfaction with online learning and online mathematics achievement. The study revealed that motivation influenced achievement, and both motivation and emotion were related to satisfaction. Based on these findings, the study formulated the following recommendations for teachers: enhance students' self-efficacy, design supporting tools in online courseware, provide course orientation, provide SRL support through social media, and restructure the format of the course.
Academic self-concept, academic self-efficacy, and mathematics self-concept
Arens et al. ( 2020 ) discussed the similarity and the difference between academic self-concept and academic self-efficacy. In general, both academic self-concept and academic self-efficacy address students' competence (Bong & Skaalvik, 2003 cited in Arens et al., 2020 ). However, academic self-concept is related to the self-perceived competence of a student in an academic domain in general (e.g., math; Marsh & Craven, 2006 ). Meanwhile, academic self-efficacy is self-perceived confidence to perform successfully a given task in a specific domain (e.g., Bandura, 2001 ; Zimmerman, 2000 ). In other words, self-concept is a domain-specific construct while self-efficacy is a domain- and task-specific construct (Arens et al., 2020 ).
Self-concept is investigated in the field of mathematics. Mathematics self-concept is attributed to students’ aspirations to pursue degree programs in STEM (science, technology, engineering, and mathematics) (Sax et al., 2015 ). Students with positive mathematics self-concept stay and finish their chosen degree program which, in turn, contributes to improving the school's student retention (Ackerman et al., 2013 ). This can be explained by the findings that mathematics self-concept is positively related to mathematics achievement (Kung, 2009 ; Lee & Kung, 2018 ). Moreover, mathematics self-concept and mathematics self-efficacy (i.e., a belief of student’s ability in solving mathematical problems or tasks related to mathematics; Masitoh & Firtriyani, 2018 ) predicted mathematics achievement (Kung, 2009 ). Thus, it is necessary to understand the mathematics self-concept of the students since it serves as a basis to cultivate the students’ learning interests in the program.
The measurement of mathematics self-concept is primarily based on the prior works of self-concept (e.g., Marsh & O'Neill, 1984 ; Marsh et al., 1983 , 2005 ). The questionnaire was composed of different areas including mathematics, verbal abilities, academic capabilities, problem-solving/creativity, physical abilities/sports, physical appearance, relations with same-sex peers, relations with opposite-sex peers, relations with parents, religion/spirituality, honesty/reliability, emotional stability/security, and general self-concept. The mathematics factor was composed of items such as “I find many mathematical problems interesting and challenging”, “Mathematics makes me feel inadequate”, “I am quite good at mathematics”, “I have trouble understanding anything that is based upon mathematics”, and “I have always done well in mathematics classes”. Recent studies on mathematics self-concept based their works on self-concept questionnaire developed by Marsh and colleagues (Marsh & O'Neill, 1984 ; Marsh et al., 1983 , 2005 ). For example, Lee and Kung ( 2018 ) investigated the mathematics self-concept and mathematics achievement of junior high school students in Taiwan. The authors devised a 13-item questionnaire measuring competence in mathematics, affection towards mathematics, and comparison of mathematics abilities.
Barriers to online learning
Cavanaugh et al. ( 2009 ) reported the barriers in the existing literature to online learning implementation as well as the benefits and challenges of online learning. Higher levels of motivation, expanding educational access, providing high-quality learning opportunities, improving student outcomes and skills, allowing educational choice, and administrative efficiency are notable benefits of online learning. Meanwhile, the high cost of start-ups, digital divide issues, governmental approval, and student readiness are the challenges raised in the implementation of online learning.
In a recent study, Binti Abd Aziz et al. ( 2020 ) investigated the barriers to online learning. According to the authors, addressing these barriers could lead to effective online learning practices. They identified the barriers in terms of attitudes, interruptions, technology skills, and personal skills. Attitudes towards online learning refer to the feelings of the people towards online learning. Computer, online, and computer application literacy skills are the components of technology skills. Interruptions to online learning are defined as the limits to technological access because students may be living in rural areas, being part of a minority group, having disabilities, or due to mature age. Personal skills are skills relating to prior experience of using online learning. Path analysis disclosed that attitudes toward online learning and technology skills are the main barriers to online learning.
The inability of the students to study at their own pace has also posed a barrier to students' online learning. Learner autonomy is the ability of learners to assume control or to take charge of their learning (Benson, 2001 ). Autonomous learners were able to understand the online learning process (Fotiadou et al., 2017 ), which enabled them to achieve high grades in online learning classes (Yen & Liu, 2009 ). In other words, mathematics learner autonomy is the ability of learners to understand and assume control learning of the online materials with little supervision (Benson, 2001 ; Fotiadou et al., 2017 ).
In the Philippines, Pena-Bandalaria ( 2009 ) reported that personal concerns (e.g., difficulty to interact and contact teachers, difficulty to seek help, difficulty in understanding the topics), technical concerns (e.g., problems accessing the course site), and the digital divide were barriers to online learning. This finding is consistent with the results of the study of Gledhill et al. ( 2017 ). Gledhill et al. ( 2017 ) also revealed that limited or poor access to the Internet, technology, and networks were the constraints of e-learning in less developed countries. Perceived Internet speed is the subjective evaluation of the speed of the Internet in supporting online learning sessions (Gledhill et al., 2017 ). Natividad ( 2021 ), and Salac and Kim ( 2016 ) explained the slow Internet connection in the Philippines. They agreed that Internet connection in the Philippines is slow due to limited Internet infrastructure which is brought by outdated laws and heavy bureaucratic processes for the development of Internet infrastructure. Furthermore, intermittent power supply was a major problem that hinders e-learning implementation in less developed countries (Bhuasiri et al., 2012 ).
In this time of COVID-19 pandemic, Philippine higher education institutions also implemented emergency online learning programs (Murphy, 2020 ). The emergency implementation may caught students unprepared (Aguilera-Hermida, 2020 ; Daniel, 2020 ). Baticulon et al. ( 2021 ) reported the major barriers of Filipino medical students to adopt online learning. These barriers can be classified as technological (lack of devices, issues with the online platform, Internet connectivity), individual (students’ learning style, physical and mental health), domestic (concerns at home, financial distress), institutional (school curriculum), and community barriers (lockdown restrictions, infrastructure challenges, and sociopolitical issues). Students found it difficult to understand the learning materials on their own. It was also reported that students had difficulty studying at home because of noise, distractions, and small space. Personal physical learning space refers to the space dedicated to online learning that is free from distraction or noise (Baticulon et al., 2021 ).
In a similar study of Fabito et al. ( 2021 ), they found that difficulty of clarifying topics or discussions with the professors, lack of study or working area dedicated for online activities, and lack of good Internet connection were the top three barriers and challenges that the 300 computing students (Computer Science and Information Technology) experienced. The study concluded that students and teachers were both not prepared to undergo full online learning. In a similar study, Bringula et al. ( 2021 ) found that the number of owned devices had a positive influence on the perceived academic online learning performance of computing students. Device ownership refers to the number of devices students own in accessing the LMS (Bringula et al., 2021 ). It was shown that students that own multiple devices are more likely to have positive dispositions towards their academic online learning performance than those students who have difficulty access to online learning devices.
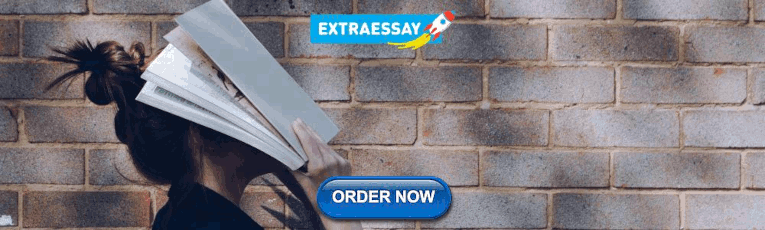
Synthesis of literature review
There is a wealth of studies proposing the effective delivery of mathematics online education. These recommendations did not consider students' mathematic online self-concept. Moreover, the recommended teaching strategies are not set forth in the context of COVID-19 pandemic where students faced physical and psychological challenges (Baticulon et al., 2021 ; Bringula et al., 2021 ; Gledhill et al., 2017 ; Pena-Bandalaria, 2009 ). In particular, the continuous lockdowns in the Philippines exacerbate the existing phenomenon of digital divide, e.g., students cannot utilize public pay-for-access of computers and Internet in computer shops (Baticulon et al., 2021 ). Students may experience difficulty on engaging to mathematics online learning due to limited access to basic online resources. In turn, students may feel less capable of learning the mathematics online materials at their own pace. Determining the possible connection between barriers to online learning and students’ mathematics self-concept may help teachers, parents, and educational institutions to formulate pedagogical interventions to achieve desired online mathematics achievement.
Methodology
Research design, research setting, participants, sample, and data gathering procedure.
This mixed-method study was conducted in one department of a university in Manila. In the quantitative part, the participants of the study were second-year college students (subsequently referred to as online learners) enrolled in two classes in Numerical Analysis. There were 69 online learners enrolled in the said course. This was the only mathematics course offered at the time the study was conducted. The study was conducted after the first week of full implementation of pure online learning through a learning management system (LMS). Learning materials and lecture sessions were both delivered in synchronous and asynchronous methods, but mostly delivered in asynchronous methods (36 h out of 54 h). Online learners were informed at the beginning of the online class sessions about this setup. The survey form was distributed in the LMS. An online survey form was distributed to all online learners but only 54 students participated in the study. Online learners in the study are composed of male (59%) and female (41%) students with an average age of 20 years old.
Research instrument
The study utilized a content-validated survey form that served as a research instrument. The survey form consisted of two parts. The first part gathered information about the online learners profiles such as device ownership, perceived Internet speed, personal physical learning space ownership, and mathematics learning autonomy. They were also asked whether they have personal/private physical space for online learning (Baticulon et al., 2021 ). Perceived Internet speed can be answered using the responses “Very slow”, “Slow”, “Sometimes fast, sometimes slow”, “Fast”, and “Very fast”.
The mathematics learning autonomy intends to determine the level of independence to learn mathematics. It was measured using a 5-point scale in which the most negative response (i.e., total dependence to teachers or classmates) had an assigned value of 1 while the most positive response had a value of 5 (i.e., can independently learn the course content). These variables were selected because these were deemed relevant to the participants of the study and these were believed to influence engagement in online learning in the context of the COVID-19 pandemic (Pynos, 2016 ).
The second part solicited data on the mathematics self-concept of online learners. Mathematics self-concept consisted of mathematics ability (12 items), interest (2 items), and perceived mathematics performance (1 item). Online learners were asked about their perceived abilities and interest in mathematics learning when the course is delivered in an online setting. All items of ability and interest were preceded by the phrase “Considering that the course is delivered online,…”. The items could be answered using the responses “very untrue to me”, “untrue to me”, “unsure”, “true to me”, and “very true to me”. These verbal responses had assigned values from -2 to 2, where the most negative response has a value of -2 while the most positive response has a value of 2. Students were asked to complete the sentence “Considering that the course is delivered online, your mathematics grade will be…” to measure their perceived mathematics performance. This question could be answered using the responses “higher than face-to-face”, “about the same with face-to-face”, “lower than face-to-face”, and “not sure/cannot tell”.
The definition of academic self-concept relating to ability (Shavelson et al., 1976 ) and interest (Joyce & Yates, 2007 ) served as the basis in the construction of the research instrument. The items of the research instrument were adapted from Joyce and Yates ( 2007 ), Marsh et al. ( 1983 ), and Lee and Kung ( 2018 ). Only the ability component in the questionnaire of Lee and Kung ( 2018 ) was adopted in this study. The items applicable to online learning were retained. The adapted research instrument was then pilot-tested to 50 students who were not part of the study. Factor and Cronbach’s alpha analyses revealed that all items were found valid (factor loading ≥ 0.50) and reliable (Cronbach’s alpha α ≥ 0.70).
Statistical treatment of data
Frequency counts, means, and cross-tabulation were used to describe the data. Spearman Rank correlation was employed to determine the relationship between the profile of the online learners and mathematics self-concept. Mann–Whitney U test was used to determine whether there is a significant difference between the mathematics self-concept of online learners when categorized by personal physical learning space access. A 0.05 level of significance was used to determine the significance of the results.
Interview sessions, selection of interview participants, and participants
In the qualitative part, a series of separate interviews were conducted with three Information Technology (IT) students to further explain the findings of the study. The participants were selected based on their mathematics abilities and access to personal learning space. The authors sought the recommendations of the teachers who handled the course to identify the possible participants. The teachers identified and categorized the mathematics abilities of the students. These classifications are reliable because teachers know their students’ capabilities (Cheong et al., 2004 ; Lambert, 2002 ; Reeve, 2006 ). The participants consisted of 3 male, third-year students with an average age of 20 years old and they had varying degrees of mathematics abilities (i.e., struggling, average, and high performing). One of the informants (i.e., respondents) has no personal space dedicated to online learning. Two female participants were invited but they refused to participate in the study.
The interviews were conducted through Google Meet. Informants were interviewed one-by-one on different occasions to protect their identities. Students were asked about their challenges in online learning. The students were asked about their study practices in a face-to-face class (e.g., attending classes, review preparations for quizzes and exams, practicing solving math problems, reading materials, and taking lecture notes), challenges experienced in an online learning class, their perceived abilities in a face-to-face and online learning class, and their recommendations relating to the improvement of online pedagogy.
Qualitative data analysis
The interviews were transcribed and were tabulated in a word processor. The tabulated results were then presented for validation. The purpose of the validation process was to determine whether other students had the same experiences. The validation of interview results was done through a presentation to another set of students (i.e., the validators) with the same level of mathematics abilities and also enrolled in the mathematics class during the summer period. Throughout these procedures, all identities of the participants were kept confidential. The validators were asked whether they agree or disagree with the responses collected from the interviews. The validators were composed of IT students (3 males with an average age of 20 years old; 2 third-year students and 1 s-year student; one of the respondents has no personal learning space). After the validation process, the tabulated responses were analyzed through qualitative content analysis (Hsieh & Shannon, 2005 ; Mayring, 2014 ).
The responses were coded and categorized based on Baticulon et al.'s ( 2021 ) classifications of barriers to online learning. The codes were keywords or phrases that represented the challenges of learning mathematics in an online environment or their recommendations to their teachers about teaching mathematics in an online setup. The authors also made their classifications if an item did not fit on the Baticulon et al.'s ( 2021 ) classification. The codes were then assigned to themes (i.e., challenges of online learning and the recommendations to improve online learning). One of the authors coded the responses. When coding the text, the coder was guided by the themes. The process was repeated until all keywords and phrases were assigned to the themes. Afterward, the research team deliberated whether they agree (or disagree) with the themes. In case of disagreement, the deliberation process was repeated until a consensus was reached (Bringula et al., 2019 ).
Both the quantitative and qualitative results of the study were presented during the department general faculty meeting to elicit feedback and inputs on how mathematics online teaching practices be improved and to validate the themes proposed in this study. The attendees of the meeting served as the external validators. The external validators involved two mathematics teachers (both female with at least 25 years of teaching experience), one LMS administrator (female with 20 years of work experience; conducts LMS training and develops University-wide online course materials), and one academic administrator. All external validators agreed with the themes and recommendations of the study.
RQ1: Learners-related factors
It is found that 98% of the online learners own 1 or 2 devices (see Table 1 ). More than half of the respondents do not have personal learning space during online learning sessions. Seventy percent reported that intermittent Internet connection is the most problematic aspect of online learning. They perceived that mathematics delivered in an online platform is harder to learn than in a face-to-face setup. A large percentage of online learners (89%) mainly rely on lectures from teachers or from the help of their classmates to understand mathematical concepts. Fifty-six percent of the students rely on their teacher’s or classmates’ consultation to understand the lessons.
RQ2: Mathematics self-concept in an online learning setup
Online learners have negative notions about their capabilities in terms of understanding the lessons, solving problems easily, finishing the course, performing better relative to their classmates’ abilities, and performing better relative to their schoolmates’ abilities (Table 2 ). They feel it is more enjoyable to learn in a face-to-face setting than online. In terms of mathematics performance, 43% of the students feel that they will get lower grades in the online course than when it is done in a face-to-face session. Another 43% reported that they are unsure of what grade they will get at the end of the semester (Fig. 1 ). This perceived academic performance could be attributed to the unfamiliarity of the new learning environment. Students feel unprepared for online learning (Daniel, 2020 ; Fabito et al., 2021 ; Murphy, 2020 ) and they may feel it undesirable (Aguilera-Hermida, 2020 ).
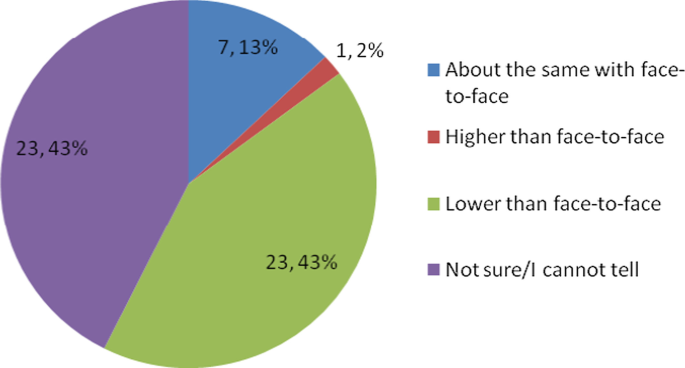
Perceived mathematics performance in an online learning setup
Despite these negative notions, students have positive outlooks in terms of achieving good grades, doing well in the course, attending classes, doing assignments, helping their classmates in their assignments, recalling the lessons, and passing the course. They perceive that learning through the LMS is interesting. These findings suggest that they believe that their abilities can still meet the demands of the course. They are confident that they can still perform well despite the challenges and uncertainties they are facing.
RQ3: Relationship between learners-related factors and mathematics self-concept
It was also determined the relationship between the profile of online learners and their mathematics self-concept (Table 3 ). The number of devices they can use has positive relationship with understanding ( r = 0.27, p < 0.05) and recalling ( r = 0.34, p < 0.05) the lesson. Perceived Internet speed is positively related to the ease of attending class ( r = 0.29, p < 0.05). Statistical analyses found that correlations exist between mathematics learning autonomy and mathematics self-concept. Out of the 15 correlation analyses, six variables have significant correlations with mathematics learning autonomy. Mathematics learning autonomy have positive relationship with the abilities to get good grades ( r = 0.42), solve problems easily ( r = 0.40), do assignments easily ( r = 0.31), recall the lessons ( r = 0.34), and perform better than their classmates ( r = 0.40) or schoolmates ( r = 0.39).
RQ4: Difference in the mathematics self-concept of online learners between with and without personal learning spaces
Further analysis was conducted to determine if mathematics self-concept differs between online learners with and without personal learning spaces. Both online learners (with personal learning space, 30%; without learning space, 33%) agreed that mathematics learning in an online environment is harder than face-to-face. However, they have different opinions in terms of their mathematics grades. Online learners with no learning space perceived that they might have a lower grade than in a face-to-face course (30%) while those who have personal learning spaces are unsure of what grades they will get at the end of the semester (28%). The test of the difference between the means of self-concept of learners explains these results.
Mann Whitney U test revealed that the mathematics self-concept of the two groups of learners differ significantly in terms of achieving good grades ( U (52) = 252.0), solving problems easily ( U (52) = 243.5), doing well in the course ( U (52) = 249.5), answering assignments ( U (52) = 256.0), and recalling the lessons ( U (52) = 225.0) (Table 4 ). The results are unlikely to have arisen from sampling error ( p < 0.05). Online learners with no personal learning space had lower mathematics concepts than privileged online learners.
RQ5: Challenges on online learning
Table 5 shows the challenges that the informants faced in learning mathematics in an online environment. All validators agreed that they experienced these challenges. All the informants and validators alike agreed that technological challenges are the most pressing concern in online learning. Only one of the informants reported power interruption. This informant is in the province and his province has been experiencing regular power interruptions. This statement confirms the study of Bhuasiri et al. ( 2012 ). The other informants and validators may not experience this because they are all in the National Capital Region where power interruption is rare.
The second challenge involves a problem that can only be solved by the students themselves. One of the informants said:
It is hard to focus on my studies. Unlike in a classroom setup, the environment is conducive to learning. All you need to do is to listen to the teacher. When you are at school, your mind is conditioned to study. When you are at home, you are in a comfort zone. I tend to do other things and delay doing my assignments. I admit: I become less productive and do not manage my time well when I am at home.
However, it must be noted that one of the validators did not agree that he procrastinates. This is the only item that the validators disagree with. Domestic challenges contribute to the distraction of students' online learning. Even while during class sessions, students were asked to run for errands or do simple household chores. Some situations are beyond the control of the students and their families (e.g., visitors). Noise and distractions contribute to domestic challenges. This finding confirms the study of Baticulon et al. ( 2021 ) and Fabito et al. ( 2021 ). One informant commented:
I have other responsibilities at home. Sometimes, I feel guilty because they are all busy doing household chores while I am on just on my computer throughout the day. Sometimes, I have to run errands. There are times that I have to respond to our neighbors' calls who are looking for my parents.
One validator agreed and said:
I agree with this. I want to also add that I only used the living room for my online learning sessions. Sometimes, the people in the house forgot that I am having an online learning session. They play music while walking around the living room. There was even an incident that they look at my laptop thinking that I was only watching movies.
Informants and validators reported that they experienced assessment challenges. Assessment challenges involve few practice drills, design of the online examination, clarity of instructions, and assessment feedback. One informant commented that teachers only provide about three questions and let them study and solve the other problems. This practice is construed as ineffective because, according to the informant, practice is an important activity that builds up their mathematics skills. The informant explained that this could be attributed to the desire of the teacher to cover the whole content of the course syllabus. When he was asked whether he prefers quality over quantity of the content, he chose the former. The validator stated: “There is no point in covering the whole syllabus when you did not understand any of them.”
The design of the examination refers to the way the questions are presented on an online platform. These include questions that require answers with long inputs of formula that are susceptible to typographical errors, multiple types of questions that are prone to guessing, time allotment, unclear instructions, and familiarity with the system itself. Informants and validators pointed out those problems that need to input their solutions in a text box entail a lot of time. They explained that they solve the problems on a piece of paper and then transfer them to the online submission system. They also raised their concerns on submission deadlines as they also have other courses with the same course requirements to satisfy. They also requested feedback on their activities and quiz results so that they would not commit the same mistakes.
The informants and validators further pointed out that they need more time as they familiarized themselves using the system. This is consistent with the study of Binti Abd Aziz et al. ( 2020 ). One informant said: “In an online exam, you are not only concerned with the correctness of your answers. You are also concerned about how you will input your answers correctly in the system. Teachers have to take into account that we also need time to familiarize ourselves with the system.”
The informants and validators also raised a pedagogical issue. The study of Baticulon et al. ( 2021 ) classified understanding the content of the course as a personal barrier. However, this is not the case based on the interviews with the informants and validators. According to informants and validators, it is difficult to understand the topics in online learning because of its delivery. When it is delivered appropriately, they can understand the topics and have better chances of passing the quizzes and exams. They prefer a combination of content delivery strategies including discussion of PowerPoint slides with step-by-step solutions through online meeting apps (e.g., Google Meet), and recorded videos of step-by-step solutions. One of the informants emphasized this comment: “Please do not let us study mathematics on our own. You do not just give the materials to us and let us understand the content.”
Before online learning, informants and validators seek the assistance of their teachers, classmates, or friends. The informants and validators understand that it is difficult to seek consultation because it is difficult to find a common time for consultation. Teachers and students have other responsibilities to attend to after online classroom sessions.
Students developed test anxiety because of the aforementioned challenges. According to the statement of one informant,
There is always a nervous factor when taking the quizzes or examinations since these are time-based activities. I am anxious since my Internet connection could suddenly become unstable or there might be a power interruption. Some teachers do not allow returning to the questions. Once you skipped the question, it will be given a zero mark. Unlike in a paper-based test, you can skip the questions and go back working on it if there is still time. I understand my teachers. They are thinking that a time-limited quiz/exam is a way to deter cheating.
This study investigated the classroom experience of online learners in a mathematics class during the summer of 2020. Toward this goal, the study attempted to determine the relationship between the online learners-related factors and their mathematics self-concept. Moreover, interviews were conducted to determine the challenges they faced in learning mathematics delivered on an online platform. The online-related factors in terms of device ownership revealed that they own 1 or 2 devices. Access to the device is not a problem to this set of participants relative to the general student population that may experience the digital divide (Cavanaugh et al., 2009 ; Pena-Bandalaria, 2009 ). This can be explained by the fact that the participants of this study are IT students, where learning activities, even before the pandemic, are highly dependent on devices.
The quantitative result shows that Internet connection is the most problematic aspect of online learning. An intermittent Internet connection can greatly affect the attendance of the students in online classes. This finding is consistent with the interview results in terms of technological challenges. This is a national problem since the Philippines has slow Internet connectivity (Chiu et al., 2017 ). According to Natividad ( 2021 ), and Salac and Kim ( 2016 ), the Philippines has a slow Internet connection because of the outdated Philippine law and red tape that hinders the quick installations of cell towers. This result confirms the findings of Bhuasiri et al. ( 2012 ) and Baticulon et al. ( 2021 ). Although only one of the informants reported an issue of power interruption, his concern is valid. His concern might not be similar to other informants or validators simply because the other informants and validators are all living in Metro Manila.
Almost half of the participants have no personal learning space during online learning sessions. Online learners with no personal learning space had lower mathematics concepts than privileged online learners. The lack of personal learning space during online sessions puts online learners in a disadvantaged position to attain an optimal learning experience. This is consistent with the findings of Baticulon et al. ( 2021 ), and Fabito et al. ( 2021 ). As explained in the interviews, students who lack personal learning space are more susceptible to distractions during, and even after online learning sessions. Noise and running errands are the most common forms of distraction. The interview results show that other members of the family may simply forget the students are in an online class. In short, as one student commented, access to personal physical learning space can create an environment conducive to online learning.
Online learners disclosed that they understood the content through lectures and constant consultation with teachers. This is consistent with the interview results that students dislike studying the course content on their own. This mathematics learning autonomy is the exact opposite of the nature of asynchronous learning. In asynchronous learning sessions, students have to study a lecture on their own. In other words, learners who are consultation-dependent will resist this educational shift.
As shown in Table 3 , students with low learning autonomy are expected to have lower dispositions of their mathematics abilities. Students who feel inferior about their mathematical abilities tend to have lower mathematics performance (Lee & Kung, 2018 ). In students’ point of view, asynchronous session activities (e.g., reading materials, assignments, practice drills, and quizzes) are challenging in the aspects of assessment, pedagogy, and consultation. These challenges explain why students experience test anxiety. Consequently, these difficulties contributed to their feeling of uncertain or low grade perceptions. The quantitative results provide insights to address this issue, i.e., students have to be gradually introduced into the concept of learners’ autonomy. Furthermore, family members may dedicate a place in the house that will serve as an online learning space.
The shift to an educational setting had a negative impact on the mathematics self-concept of learners. More than 80% of the respondents perceived that they will have a lower grade in mathematics. They also have negative notions of their mathematics self-concept in terms of understanding the lesson, solving problems, finishing the course, performing better relative to their classmates or schoolmates, and enjoying the online class. These negative notions on their capabilities and interest in online learning can be explained by the fact that full online learning is just implemented recently. While online learners have experience using the LMS before the COVID-19 pandemic, they are not yet fully familiar with a fully online learning setup. This is evident in one of the narratives of the informants. On one hand, the positive mathematics self-concept indicates that they are hopeful in the aspects of achieving good grades, attending classes, doing assignments, helping their classmates in their assignments, recalling lectures, passing the course, having the interest to learn, and doing well overall in the course. Teachers have to sustain these positive outlooks to achieve the course outcomes.
Device ownership has a positive relationship with understanding and recalling the lecture. Multiple devices such as laptops and mobile devices are dependable for students' online learning (Muyinda et al., 2010 ). Multiple device ownership allows online learners to view multiple screens and to store multiple copies of learning materials (Pynos, 2016 ). Multiple device usage in learning also creates seamless connectivity that enables the continuity of the learning experience (Milrad et al., 2013 ). This practice allows easy access to information that is useful for solving problems. For example, an online learner may be looking at his/her laptop screen for the given problem while he/she is looking into another device (e.g., cellphone) that displays the formula and the sample solved problems. Furthermore, multiple devices can address accessibility or installation issues.
Perceived Internet speed is positively related to the ease of attending class. This finding is expected. What is more interesting is that perceived Internet speed does not relate to the other items of mathematics self-concept. The results imply that a fast Internet connection is only necessary to attend the class but not necessarily related to the online learners' perceptions about their mathematics abilities. Their perceptions about their abilities and interest in mathematics are not related to the speed of Internet access. In other words, there is no link between the confidence of online learners in their mathematics abilities and their speed of Internet access.
Meanwhile, mathematics learning autonomy is correlated with most of the mathematics self-concept. This vivid finding denotes that mathematics self-concept is mostly related to the perceived abilities of online learners to study at their own pace, i.e., as students become more independent learners, they tend to have a higher mathematics self-concept. Teachers have to emphasize to the online learners that online learning is different from face-to-face where teachers can intervene when confusion or challenges arise in understanding the lessons. Teachers, at the onset of the course, are encouraged to orient online learners that they are expected to be independent learners. Problem sets and learning materials may be given in advance to develop the habit of independent learning.
Consistent with the literature, the respondents of this study experienced technological, personal, and domestic challenges. There is a challenge that students can be addressed by themselves (e.g., procrastination) but most are beyond their control. Domestic challenges require the support and understanding of family members. The students and their family members must have open communication. They should set house rules in terms of household chores and running errands.
The study of Baticulon et al. ( 2021 ) categorized the inability to understand the content of the course as a personal barrier to online learning. In this study, it was shown that this is a pedagogical challenge than a personal problem. Furthermore, it was disclosed that teachers have direct responsibilities on four out of the seven identified challenges. Challenges in the teaching and assessment had the most number of concerns. These results guide teachers to devise creative teaching and fair assessment strategies that could address these concerns. For teaching and learning activities, teachers are advised to provide ample time for lectures and deliver the contents through different forms of multimedia. At the end of each lecture, teachers may elicit feedback from students to assess if the students understood the lessons. It is advisable to gather feedback from struggling as well as high-performing students to understand the challenges of the students with diverse mathematical abilities. Group learning activities may be conducted using the Group Discussion function of the LMS. Teachers may provide practice drills that are not yet included for grade computation. Provide 2–3 days to allow students to do their assignments. The asynchronous sessions may also be utilized as consultation time. Teachers may use the randomized function of the LMS to pick random questions from its databank. Teachers may also request students to show their computer windows during the quiz (see “ Appendix A ”).
Another important role of the teachers is to sustain the positive and counter the negative mathematics self-concept of the students. Teachers at the onset of the course, the questionnaire here may be utilized to determine the mathematics self-concept of the students. Students should be oriented about the course expectations. Teachers should introduce independent learning gradually (“ Appendix A ”).
Conclusions, recommendations, limitations, and implications
This study investigated the profile of online learners and its influence on their mathematics self-concept. It is revealed that online learners in this study have access to devices. Physical learning space is one important aspect of an online learning environment. However, some online learners have physical learning space limitations which make online learning inconvenient. This limitation contributed to their low academic self-concept.
The majority had reported an intermittent Internet connection. Online learners have mixed notions about their mathematics capabilities and interest in learning mathematics in an online environment. They expressed uncertainties about the possible grades they will get at the end of the semester. The ability of online learners to study mathematics at their own pace is the most desired skill for online learners. Moreover, online learners with limited learning space are more likely to experience a lower mathematics self-concept because they cannot focus on the course. Thus, it can be concluded that the profile of online learners partly influences their mathematics self-concept.
Teachers play a significant role in improving and sustaining the mathematics self-concept of online learners. At the beginning of the class, teachers must inform online learners that having a habit of self-paced learning is a highly desirable discipline. Teachers have to sustain the positive mathematics self-concepts of online learners. They may assure online learners that online consultations are available when needed. Timely feedback on the works of online learners is highly encouraged to sustain their positive outlook about their capabilities. Individualized feedback can be provided to inform online learners that they are performing well (or not performing well) relative to his/her classmates.
The negative mathematics self-concepts of online learners serve as a basis for teachers to find ways to address these negative notions. Teachers have to be creative in delivering the content of the course (O’Doherty et al., 2018 ). For instance, PowerPoint slides with a voice recording or a previous video recording of the lesson may be utilized for lecture sessions. These materials may be accessed anytime and students with a slow Internet connection can still follow the phase of the course. Teachers may conduct synchronous learning sessions to answer questions or clarifications. An unwavering teacher’s dedication and understanding are suggested to assist online learners to finish the course.
The study is limited in terms of the participants and sample size. These limitations existed because of the timing of the shift of mode instructions in the university. There were only limited courses offered and a small number of students were enrolled when the study was conducted. Thus, the findings of the study may not be widely applicable beyond this population. Despite these limitations, this study provides clear insights into the students' mathematics self-concept and the challenges they faced in an online learning environment. The realities discovered in this study cannot be denied and deserves the attention of mathematics teachers. Nevertheless, a university-wide investigation of mathematics self-concept may be initiated to improve further the findings of the study.
There are issues raised in the study that cannot be solved by teachers. The members of the family must understand that online learners need physical learning space and minimal disruptions. To address this concern, teachers, or schools may send letters to parents about online learning to observe the online learning schedule of their children. Cooperation and understanding from family members are necessary for providing an environment conducive to online learning. It is strongly recommended that family members dedicate a physical learning space for online learners.
Educational institutions have to select an LMS that can support the demands of the course. The institution needs to understand the online learning requirements of the different degree programs. It is imperative to understand the strengths and limitations of the different LMS. A selection criteria committee may be instituted to select an LMS and to review its effectiveness relative to the needs of the students and faculty. Usability testing of the LMS may be done after the implementation. This will identify the ease of use and satisfaction of use of the LMS. The evaluation process may also evaluate whether the LMS supported the pedagogical requirements of the faculty (Pipan et al., 2008 ).
Another challenge the institution facing is the possibility of students that might be left behind because of inadequate access to devices. The formation of a technical support group is also desirable. Educational institutions may extend their help to online learners by lending laptops, tablets, and mobile Wi-Fi. Local government units may also offer assistance to underprivileged students. For example, a city local government unit in the Philippines provided online learning devices (e.g., laptops or tablets) to students (Casinas, 2020 ) and installed Internet centers to support online learning (Kabagani, 2020 ).
Lastly, the government may reinforce fast Internet connections through legislation. One of the possible legislations is to shorten the application of business process applications of constructing Internet facilities (Natividad, 2021 ; Salac & Kim, 2016 ). The government may allocate funds for the development of Internet infrastructures. These funds may be directed to rural areas. A government-private partnership may also be initiated. With this partnership, lengthy bureaucratic procedures will be avoided. Finally, the government may promote a market of competitiveness through the inclusions of other Internet providers (Salac & Kim, 2016 ).
Availability of data and materials
Data cannot be shared because of existing laws in the country of the authors.
Abbreviations
Academic self-concept
Coronavirus disease
Information technology
- Learning management system
Self-regulated learning
Traditional mathematics instructions
Mann–Whitney U test
Web-based mathematics instructions
Ackerman, P. L., Kanfer, R., & Beier, M. E. (2013). Trait complex, cognitive ability, and domain knowledge predictors of baccalaureate success, STEM Persistence, and Gender Differences. Journal of Educational Psychology, 105 (3), 911–927.
Article Google Scholar
Aguilera-Hermida, A. P. (2020). College students’ use and acceptance of emergency online learning due to COVID-19. International Journal of Educational Research Open, 1 , 100011.
Arens, A. K., Frenzel, A. C., & Goetz, T. (2020). Self-concept and self-efficacy in math: Longitudinal interrelations and reciprocal linkages with achievement. The Journal of Experimental Education . https://doi.org/10.1080/00220973.2020.1786347
Bandura, A. (2001). Social cognitive theory: An agentic perspective. Annual Review of Psychology, 52 , 1–26.
Baticulon, R. E., Sy, J. J., Alberto, N. R. I., Baron, M. B. C., Mabulay, R. E. C., Rizada, L. G. T., Tiu, C. J. S., Clarion, C. A., & Reyes, J. C. B. (2021). Barriers to online learning in the time of COVID-19: A national survey of medical students in the Philippines. Medical Science Educator, 31 , 615–626.
Benson, P. (2001). Teaching and researching autonomy in language learning . Longman/Pearson Education.
Google Scholar
Berge, Z. L., Collins, M., & Dougherty, K. (2000). Design guidelines for web-based courses. In B. Abbey (Ed.), Instructional and cognitive impacts of web-based education (pp. 32–40). IGI Global.
Chapter Google Scholar
Bhuasiri, W., Xaymoungkhoun, O., Zo, H., Rho, J. J., & Ciganek, A. P. (2012). Critical success factors for e-learning in developing countries: A comparative analysis between ICT experts and faculty. Computers & Education, 58 (2), 843–855.
Binti Abd Aziz, N. A., Bin Musa, M. H., & Binti Abd Aziz, N. N. (2020). A study on barriers contributing to an effective online learning among undergraduates students. Open Journal of Science and Technology, 3 (1), 17–23.
Bong, M., & Skaalvik, E. M. (2003). Academic self-concept and self-efficacy: How different are they really? Educational Psychology Review, 15 (1), 1–39.
Bringula, R., Elon, R., Melosantos, L., & Tarrosa, J. R. (2019). Teaching agile methodology through role-playing: What to expect and what to watch out. In Proceedings of the 2019 3rd international conference on education and multimedia technology (pp. 355–359). New York, NY: Association for Computing Machinery.
Bringula, R. P., Batalla, M. Y. C., & Borebor, M. T. F. (2021). Modeling computing students’ perceived academic performance in an online learning environment [Paper presentation]. ACM-SIGITE'21, SnowBird, UT, USA. https://doi.org/10.1145/3450329.3476856.
Casinas, J. A. (2020). 'One is to one': Pasig City to provide 138,000 learning devices to public school students. Manila Bulletin . Retrieved from https://mb.com.ph/2020/07/02/one-is-to-one-pasig-city-to-provide-138000-learning-devices-to-public-school-students/ .
Cavanaugh, C. S., Barbour, M. K., & Clark, T. (2009). Research and practice in K-12 online learning: A review of open access literature. The International Review of Research in Open and Distributed Learning, 10 (1), 1–22.
Cheong, Y. F., Parajes, F., & Oberman, P. S. (2004). Motivation and academic help-seeking in high school computer science. Computer Science Education, 14 (1), 3–19.
Chiu, J. L., Bool, N. C., & Chiu, C. L. (2017). Challenges and factors influencing initial trust and behavioral intention to use mobile banking services in the Philippines. Asia Pacific Journal of Innovation and Entrepreneurship . https://doi.org/10.1108/APJIE-08-2017-029
Cho, M. H., & Heron, M. L. (2015). Self-regulated learning: The role of motivation, emotion, and use of learning strategies in students’ learning experiences in a self-paced online mathematics course. Distance Education, 36 (1), 80–99.
Daniel, J. (2020). Education and the COVID-19 pandemic. Prospects, 49 (1), 91–96.
Fabito, B. S., Trillanes, A. O., & Sarmiento, J. R. (2021). Barriers and challenges of computing students in an online learning environment: Insights from one private university in the Philippines. International Journal of Computing Sciences Research, 5 (1), 441–458. https://doi.org/10.25147/ijcsr.2017.001.1.51
Fotiadou, A., Angelaki, C., & Mavroidis, I. (2017). Learner autonomy as a factor of the learning process in distance education. European Journal of Open, Distance and E-Learning, 20 (1), 95–110.
Glass, J., & Sue, V. (2008). Student preferences, satisfaction, and perceived learning in an online mathematics class. MERLOT Journal of Online Learning and Teaching, 4 (3), 325–338.
Gledhill, L., Dale, V. H., Powney, S., Gaitskell-Phillips, G. H., & Short, N. R. (2017). An international survey of veterinary students to assess their use of online learning resources. Journal of Veterinary Medical Education, 44 (4), 692–703.
Güzeller, C. O., & Akın, A. (2012). The effect of web-based mathematics instruction on mathematics achievement, attitudes, anxiety, and self-efficacy of 6th grade students. International Journal of Academic Research in Progressive Education and Development, 1 (2), 42–54.
Herrington, J., Reeves, T. C., Oliver, R., & Woo, Y. (2004). Designing authentic activities in web-based courses. Journal of Computing in Higher Education, 16 (1), 3–29.
Hsieh, H. F., & Shannon, S. E. (2005). Three approaches to qualitative content analysis. Qualitative Health Research, 15 (9), 1277–1288.
Joyce, T. B. Y., & Yates, S. M. (2007). A Rasch analysis of the academic self-concept questionnaire. International Education Journal, 8 (2), 470–484.
Kabagani, L. J. (2020). Pasig installs Internet centers for distance learning. Philippine News Agency . Retrieved from https://www.pna.gov.ph/articles/1113118 .
Kim, C., Park, S. W., & Cozart, J. (2014). Affective and motivational factors of learning in online mathematics courses. British Journal of Educational Technology, 45 (1), 171–185.
Kung, H. Y. (2009). Perception or confidence? Self-concept, self-efficacy, and achievement in mathematics: A longitudinal study. Policy Futures in Education, 7 (4), 387–398.
Lambert, L. (2002). A framework for shared leadership. Educational Leadership, 59 (8), 37–40.
Lee, C.-Y., & Kung, H.-Y. (2018). Math self-concept and mathematics achievement: Examining gender variation and reciprocal relations among junior high school students in Taiwan. EURASIA Journal of Mathematics, Science and Technology Education, 14 (4), 1239–1252. https://doi.org/10.29333/ejmste/82535
Marsh, H. W., & Craven, R. G. (2006). Reciprocal effects of self-concept and performance from a multidimensional perspective: Beyond seductive pleasure and unidimensional perspectives. Perspectives on Psychological Science: A Journal of the Association for Psychological Science, 1 (2), 133–163.
Marsh, H. W., Ellis, L. A., Parada, R. H., Richards, G., & Heubeck, B. G. (2005). A short version of the Self Description Questionnaire II: Operationalizing criteria for short-form evaluation with new applications of confirmatory factor analyses. Psychological Assessment, 17 (1), 81–102.
Marsh, H. W., & O’Neill, R. (1984). Self-description questionnaire III: The construct validity of multidimensional self-concept ratings by late adolescents. Journal of Educational Measurement, 21 (2), 153–174.
Marsh, H. W., Smith, I. D., & Barnes, J. (1983). Multitrait-multimethod analyses of the self-description questionnaire: Student-teacher agreement on multidimensional ratings of student self-concept. American Educational Research Journal, 20 (3), 333–357.
Masitoh, L. F., & Fitriyani, H. (2018). Improving students’ mathematics self-efficacy through problem-based learning. Malikussaleh Journal of Mathematics Learning, 1 (1), 26–30.
Mayring, P. (2014). Qualitative content analysis: Theoretical foundation, basic procedures, and software solution . Beltz.
Milrad, M., Wong, L.-H., Sharples, M., Hwang, G.-J., Looi, C.-K., & Ogata, H. (2013). Seamless learning: An international perspective on next generation technology enhanced learning. In Z. L. Berge & L. Y. Muilenburg (Eds.), Handbook of mobile learning (pp. 95–108). Routledge.
Murphy, M. P. (2020). COVID-19 and emergency eLearning: Consequences of the securitization of higher education for post-pandemic pedagogy. Contemporary Security Policy, 41 (3), 492–505. https://doi.org/10.1080/13523260.2020.1761749
Muyinda, P. B., Lubega, J. T., & Lynch, K. (2010). Mobile learning objects deployment and utilization in developing countries. International Journal of Computing and ICT Research, 4 (1), 37–46.
Natividad, N. (2021). Why Internet speeds in the Philippines are so slow . Retrieved from https://www.vice.com/en/article/n7vy3m/why-internet-speeds-philippines-slow-laws
O’Doherty, D., Dromey, M., Lougheed, J., Hannigan, A., Last, J., & McGrath, D. (2018). Barriers and solutions to online learning in medical education–an integrative review. BMC Medical Education, 18 (1), 1–11.
Pajares, F., & Miller, M. D. (1994). Role of self-efficacy and self-concept beliefs in mathematical problem solving: A path analysis. Journal of Educational Psychology, 86 (2), 193–203.
Pena-Bandalaria, M. M. D. (2009). E-learning in the Philippines: Trends, directions, and challenges. International Journal on E-Learning, 8 (4), 495–510.
Pipan, M., Arh, T., & Blazic, B. J. (2008). Evaluation and selection of the most applicable Learning Management System. WSEAS Transactions on Advances in Engineering Education, 5 (3), 129–136.
Pynos, R. (2016). Student engagement and its relationship to mobile device ownership and the role of technology in student learning (Doctoral dissertation). Duquesne University, USA. Retrieved from https://dsc.duq.edu/etd/104
Reeve, J. (2006). Teachers as facilitators: What autonomy-supportive teachers do and why their students benefit. The Elementary Journal, 106 (3), 225–236.
Reyes, L. H. (1984). Affective variables and mathematics education. The Elementary School Journal, 84 , 558–581.
Salac, R. A., & Kim, Y. S. (2016). A study on the internet connectivity in the Philippines. Asia Pacific Journal of Business Review, 1 (1), 67–88.
Sax, L. J., Kanny, M. A., Riggers-Piehl, T. A., Whang, H., & Paulson, L. N. (2015). “But I’m not good at math”: The changing salience of mathematical self-concept in shaping women’s and men’s STEM aspirations. Research in Higher Education, 56 (8), 813–842.
Shavelson, R. J., Hubner, J. J., & Stanton, G. C. (1976). Self-concept: Validation of construct interpretations. Review of Educational Research, 14 , 159–168.
Wadsworth, L. M., Husman, J., Duggan, M. A., & Pennington, M. N. (2007). Online mathematics achievement: Effects of learning strategies and self-efficacy. Journal of Developmental Education, 30 (3), 6–14.
Yen, C., & Liu, S. (2009). Learner autonomy as a predictor of course success and final grades in community college online courses. Journal of Educational Computing Research, 41 , 347–367. https://doi.org/10.2190/EC.41.3.e
Zimmerman, B. J. (2000). Self-efficacy: An essential motive to learn. Contemporary Educational Psychology, 25 (1), 82–91.
Download references
Acknowledgements
We would like to thank the participants of the study and those whom all helped us distribute the survey form. This paper is partly funded by the institutions of the authors.
This paper is partially funded by the institutions of the authors.
Author information
Authors and affiliations.
University of the East, CM Recto Avenue, 2219, Sampaloc, Manila, Philippines
Rex Bringula, Jon Jester Reguyal & Don Dominic Tan
Educational Technology Department, Faculty of Education, Universitas Negeri Malang, Malang, Indonesia
You can also search for this author in PubMed Google Scholar
Contributions
The authors of this study have equal contributions in the paper. All authors read and approved the final manuscript.
Corresponding author
Correspondence to Rex Bringula .
Ethics declarations
Competing interests.
The authors declare no conflicting interest in this study.
Additional information
Publisher's note.
Springer Nature remains neutral with regard to jurisdictional claims in published maps and institutional affiliations.
Appendix A: Checklist of suggestions for online mathematics teaching
Mathematics Self-concept in an Online Setting
Determine the mathematics self-concept of the students at the start of the class.
Inform the students of the setup of the course.
Introduce learners to the concept of independent learning.
Assist students in re-enforcing positive self-concepts.
Teaching Learning Activities
Provide ample time for lectures.
Immediately elicit feedback after the lecture.
Gather feedback from high-performing and struggling students about the phase and clarity of the lecture.
Use the combination of PowerPoint slides, online meetings, and videos for course content delivery.
Make the due dates reasonable (2–3 days).
Use the Group Discussion function of the LMS to encourage group study among students.
Assessment and Consultation
Provide individualized feedback on students’ activities.
Inform students about their class performance.
Dedicate synchronous sessions intended for consultation or feedback.
Provide more seatwork and practice drills.
Provide assessment activities that are not recorded.
Balance the types of the assessment – e.g., minimize multiple-choice, more on problems showing solutions, and discourage providing answers that are format-sensitive.
Balance the difficulty levels of the assessment.
Use the randomized function of the LMS to generate quizzes and exam questions.
Rights and permissions
Open Access This article is licensed under a Creative Commons Attribution 4.0 International License, which permits use, sharing, adaptation, distribution and reproduction in any medium or format, as long as you give appropriate credit to the original author(s) and the source, provide a link to the Creative Commons licence, and indicate if changes were made. The images or other third party material in this article are included in the article's Creative Commons licence, unless indicated otherwise in a credit line to the material. If material is not included in the article's Creative Commons licence and your intended use is not permitted by statutory regulation or exceeds the permitted use, you will need to obtain permission directly from the copyright holder. To view a copy of this licence, visit http://creativecommons.org/licenses/by/4.0/ .
Reprints and permissions
About this article
Cite this article.
Bringula, R., Reguyal, J.J., Tan, D.D. et al. Mathematics self-concept and challenges of learners in an online learning environment during COVID-19 pandemic. Smart Learn. Environ. 8 , 22 (2021). https://doi.org/10.1186/s40561-021-00168-5
Download citation
Received : 13 June 2021
Accepted : 05 October 2021
Published : 16 October 2021
DOI : https://doi.org/10.1186/s40561-021-00168-5
Share this article
Anyone you share the following link with will be able to read this content:
Sorry, a shareable link is not currently available for this article.
Provided by the Springer Nature SharedIt content-sharing initiative
- Mathematics
- Physical learning space
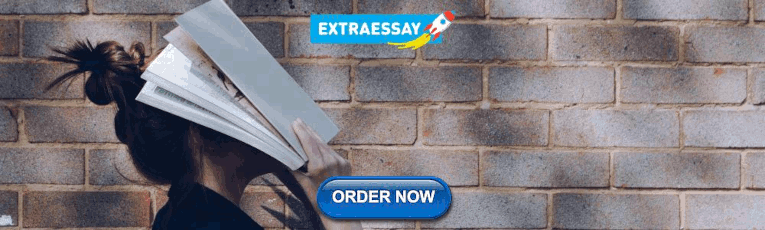
IMAGES
VIDEO
COMMENTS
The COVID-19 pandemic shifts the traditional classroom or face-to-face teaching and learning into distance learning. The district of Sto. Domingo implemented Modular Distance Learning(MDL) in which a self-learning module(SLM) is the primary tool in the teaching and learning process.
The second part is the. acceptability of the self-learning module in terms of objective, content and clarit y, activity, and presentation. E ach criteria has 5. statements or questions answerable ...
Abstract. In this paper, a tool to assess the quality of self-learning modules (SLMs) was developed using the Best Worst Method (BWM) by Rezaei (2015). Four major quality criteria namely Content (weight = 0.603), Instruction (weight = 160), Technicality (weight = 0.097), and Ethical and Cultural Considerations (weight = 0.140) were established ...
The term "modular approach" refers to learning that takes the form of individualized instruction and allows students to use Self-Learning Modules (SLMs) in the print or advanced format/electronic ...
My purpose here is to reflect upon the ways that existing theories and research can be applied to designing a MOOC-like learning experience to support learner self-regulation. I begin with descriptions of the learning experience, MOOCs, and Zimmerman's sociocognitive model of self-regulation (Zimmerman, 2000 ).
Abstract. During the pandemic, the Department of Education used Self-Learning Modules (SLMs) for modular distance learning. The descriptive-evaluative design was used to assess the effectiveness ...
Individualisation of instructions is the need of th e time. Effective learning requires individual guid ance, personal attention and overall individual efforts of the lea rners. Self Learning Modules may prove to be a boon for students due to their enriched features. They perform the ro le of an efficient teacher. Students become indepen dent thinkers. They gain the freedom to learn without ...
Self-directed learning and self-design became unexpectedly popular and common during the COVID-19 era. Learners are encouraged to take charge of their learning and, often the opportunity to independently design their learning experience. This research illustrates the use of technology in teaching and learning technology with a central theme of promoting self-directed learning with engaging ...
Children's engagement in self-learning modules (SLMs) amid the pandemic: a predictive analysis on the role of internet access, household food security, and parental involvement to modular classes ... Register to receive personalised research and resources by email. Sign me up. Taylor and Francis Group Facebook page. Taylor and Francis Group X ...
At the same time, the neutral results of the self-learning module experience significantly impacted the GWA and rejected the null hypothesis. Thus, for a better result for this study, the researchers recommended that a standardized test be used to get a high positive correlation of modular class experiences to the learners' academic performance.
This study used qualitative and quantitative approaches to evaluate the effectiveness of self-learning modules (SLMs) developed to facilitate and individualize students' learning of basic medical sciences. Twenty physiology and nineteen microanatomy SLMs were designed with interactive images, animations, narrations, and self-assessments.
The COVID-19 pandemic has necessitated a significant shift towards modular distance learning in education systems worldwide. In the Philippines, the Department of Education has developed Self-Learning Modules (SLMs) to ensure quality primary education for all learners during the pandemic. This research study aims to identify the challenges and effectiveness of the modular distance learning ...
The teaching and learning of self-study research have received increased attention in recent years, although there is still limited research about the learning of self-study. In this article, we share results from a self-study community of practice that describes how one group of novice teacher-educator-researchers learned self-study in a ...
The current study was conducted with an aim to see the relative effectiveness of self learning modules and traditional method of teaching on acquisition of process skills in science among class IX students in relation to their study habits. The present investigation was experimental in nature following quasi experimental method with pre-test and post-test non equivalent group design. The study ...
Abstract. Metacognitive monitoring and control must be accurate and efficient in order to allow self-guided learners to improve their performance. Yet few examples exist in which allowing learners to control learning produces higher levels of performance than restricting learners' control. Here we investigate the consequences of allowing ...
International Journal of Computer Engineering in Research Trends Multidisciplinary, Open Access, Peer-Reviewed and fully refereed Research Paper Volume-8, Issue-5, 2021 Regular Edition E-ISSN: 2349-7084 ... Self-learning modules will keep the students at their own pace and boosts their interest and confidence in learning amidst pandemic and ...
Abstract: This study aimed to find out whether the use of self-learning modules was effective in improving the academic performance among learners of grade 3. The subjects of the study were thirty-four (35) learners of Mamangos Maulana Kandog Elementary school for the school year 2020-2021 at Kiamba, Sarangani Province, Philippines. The study used the experimental design. Utilizing the ...
In this mixed-methods research, the relationship between four factors of individual online learners and their mathematics self-concept was explored. In addition, the challenges the students faced in learning mathematics online during the Coronavirus disease (COVID-19) pandemic were determined. The participant students were from two mathematics classes offered online during the summer of 2020 ...
Individuals born 1981-1999, reflecting the age range of OUWB students for whom this module was made, have been shown to prefer self-paced, interactive learning environments with immediate feedback ...
In this theory, the learner watches the behavior of others and what results from that behavior. However, learning does not involve a behavior change in that learner, but learning comes about as a result of observation. Therefore, Barrett considers the humanistic theory as a basic source of self- learning concept.
A self-learning module is an orderly set of instructions designed to facilitate learners' mastery of a body of knowledge or a procedure. When combined with other modules, learners can master a vast body of knowledge or a complex process. ... The research and development method produced a particular product and tests its effectiveness. Research ...
Self Learning Modules is in the form of individualized instruction that allows learners to use self-learning modules in print or digital format, whichever is applicable in the context of the learner and other learning resources like learner's materials, textbooks, activity sheets, study guides and other study materials.
ABSTRACT. Education in the new normal is a challenging task in the Philippines in an attempt to push through education amidst. the deadly pandemic caused by covid-19. The Department of Education ...