- Bipolar Disorder
- Therapy Center
- When To See a Therapist
- Types of Therapy
- Best Online Therapy
- Best Couples Therapy
- Best Family Therapy
- Managing Stress
- Sleep and Dreaming
- Understanding Emotions
- Self-Improvement
- Healthy Relationships
- Student Resources
- Personality Types
- Verywell Mind Insights
- 2023 Verywell Mind 25
- Mental Health in the Classroom
- Editorial Process
- Meet Our Review Board
- Crisis Support
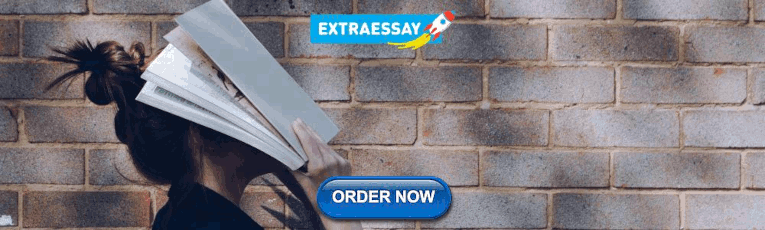
The Definition of Random Assignment According to Psychology
Kendra Cherry, MS, is a psychosocial rehabilitation specialist, psychology educator, and author of the "Everything Psychology Book."
:max_bytes(150000):strip_icc():format(webp)/IMG_9791-89504ab694d54b66bbd72cb84ffb860e.jpg)
Emily is a board-certified science editor who has worked with top digital publishing brands like Voices for Biodiversity, Study.com, GoodTherapy, Vox, and Verywell.
:max_bytes(150000):strip_icc():format(webp)/Emily-Swaim-1000-0f3197de18f74329aeffb690a177160c.jpg)
Materio / Getty Images
Random assignment refers to the use of chance procedures in psychology experiments to ensure that each participant has the same opportunity to be assigned to any given group in a study to eliminate any potential bias in the experiment at the outset. Participants are randomly assigned to different groups, such as the treatment group versus the control group. In clinical research, randomized clinical trials are known as the gold standard for meaningful results.
Simple random assignment techniques might involve tactics such as flipping a coin, drawing names out of a hat, rolling dice, or assigning random numbers to a list of participants. It is important to note that random assignment differs from random selection .
While random selection refers to how participants are randomly chosen from a target population as representatives of that population, random assignment refers to how those chosen participants are then assigned to experimental groups.
Random Assignment In Research
To determine if changes in one variable will cause changes in another variable, psychologists must perform an experiment. Random assignment is a critical part of the experimental design that helps ensure the reliability of the study outcomes.
Researchers often begin by forming a testable hypothesis predicting that one variable of interest will have some predictable impact on another variable.
The variable that the experimenters will manipulate in the experiment is known as the independent variable , while the variable that they will then measure for different outcomes is known as the dependent variable. While there are different ways to look at relationships between variables, an experiment is the best way to get a clear idea if there is a cause-and-effect relationship between two or more variables.
Once researchers have formulated a hypothesis, conducted background research, and chosen an experimental design, it is time to find participants for their experiment. How exactly do researchers decide who will be part of an experiment? As mentioned previously, this is often accomplished through something known as random selection.
Random Selection
In order to generalize the results of an experiment to a larger group, it is important to choose a sample that is representative of the qualities found in that population. For example, if the total population is 60% female and 40% male, then the sample should reflect those same percentages.
Choosing a representative sample is often accomplished by randomly picking people from the population to be participants in a study. Random selection means that everyone in the group stands an equal chance of being chosen to minimize any bias. Once a pool of participants has been selected, it is time to assign them to groups.
By randomly assigning the participants into groups, the experimenters can be fairly sure that each group will have the same characteristics before the independent variable is applied.
Participants might be randomly assigned to the control group , which does not receive the treatment in question. The control group may receive a placebo or receive the standard treatment. Participants may also be randomly assigned to the experimental group , which receives the treatment of interest. In larger studies, there can be multiple treatment groups for comparison.
There are simple methods of random assignment, like rolling the die. However, there are more complex techniques that involve random number generators to remove any human error.
There can also be random assignment to groups with pre-established rules or parameters. For example, if you want to have an equal number of men and women in each of your study groups, you might separate your sample into two groups (by sex) before randomly assigning each of those groups into the treatment group and control group.
Random assignment is essential because it increases the likelihood that the groups are the same at the outset. With all characteristics being equal between groups, other than the application of the independent variable, any differences found between group outcomes can be more confidently attributed to the effect of the intervention.
Example of Random Assignment
Imagine that a researcher is interested in learning whether or not drinking caffeinated beverages prior to an exam will improve test performance. After randomly selecting a pool of participants, each person is randomly assigned to either the control group or the experimental group.
The participants in the control group consume a placebo drink prior to the exam that does not contain any caffeine. Those in the experimental group, on the other hand, consume a caffeinated beverage before taking the test.
Participants in both groups then take the test, and the researcher compares the results to determine if the caffeinated beverage had any impact on test performance.
A Word From Verywell
Random assignment plays an important role in the psychology research process. Not only does this process help eliminate possible sources of bias, but it also makes it easier to generalize the results of a tested sample of participants to a larger population.
Random assignment helps ensure that members of each group in the experiment are the same, which means that the groups are also likely more representative of what is present in the larger population of interest. Through the use of this technique, psychology researchers are able to study complex phenomena and contribute to our understanding of the human mind and behavior.
Lin Y, Zhu M, Su Z. The pursuit of balance: An overview of covariate-adaptive randomization techniques in clinical trials . Contemp Clin Trials. 2015;45(Pt A):21-25. doi:10.1016/j.cct.2015.07.011
Sullivan L. Random assignment versus random selection . In: The SAGE Glossary of the Social and Behavioral Sciences. SAGE Publications, Inc.; 2009. doi:10.4135/9781412972024.n2108
Alferes VR. Methods of Randomization in Experimental Design . SAGE Publications, Inc.; 2012. doi:10.4135/9781452270012
Nestor PG, Schutt RK. Research Methods in Psychology: Investigating Human Behavior. (2nd Ed.). SAGE Publications, Inc.; 2015.
By Kendra Cherry, MSEd Kendra Cherry, MS, is a psychosocial rehabilitation specialist, psychology educator, and author of the "Everything Psychology Book."
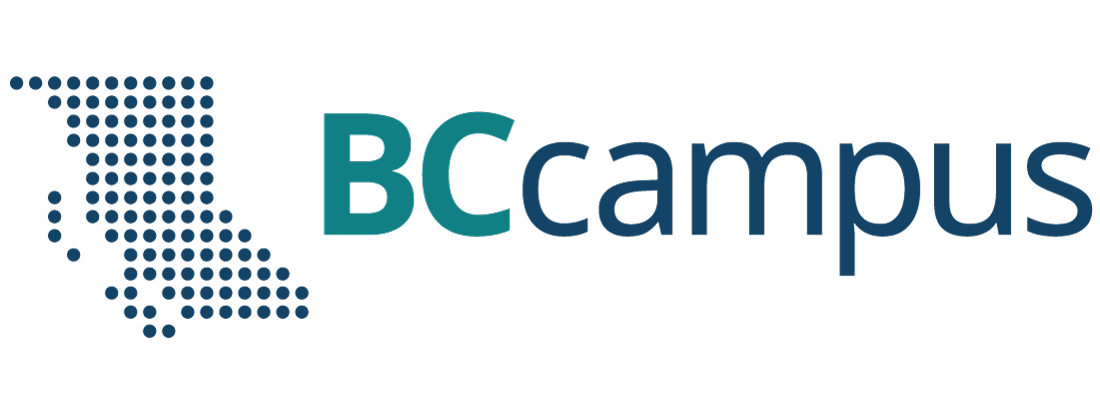
Want to create or adapt books like this? Learn more about how Pressbooks supports open publishing practices.
Chapter 6: Data Collection Strategies
6.1.1 Random Assignation
As previously mentioned, one of the characteristics of a true experiment is that researchers use a random process to decide which participants are tested under which conditions. Random assignation is a powerful research technique that addresses the assumption of pre-test equivalence – that the experimental and control group are equal in all respects before the administration of the independent variable (Palys & Atchison, 2014).
Random assignation is the primary way that researchers attempt to control extraneous variables across conditions. Random assignation is associated with experimental research methods. In its strictest sense, random assignment should meet two criteria. One is that each participant has an equal chance of being assigned to each condition (e.g., a 50% chance of being assigned to each of two conditions). The second is that each participant is assigned to a condition independently of other participants. Thus, one way to assign participants to two conditions would be to flip a coin for each one. If the coin lands on the heads side, the participant is assigned to Condition A, and if it lands on the tails side, the participant is assigned to Condition B. For three conditions, one could use a computer to generate a random integer from 1 to 3 for each participant. If the integer is 1, the participant is assigned to Condition A; if it is 2, the participant is assigned to Condition B; and, if it is 3, the participant is assigned to Condition C. In practice, a full sequence of conditions—one for each participant expected to be in the experiment—is usually created ahead of time, and each new participant is assigned to the next condition in the sequence as he or she is tested.
However, one problem with coin flipping and other strict procedures for random assignment is that they are likely to result in unequal sample sizes in the different conditions. Unequal sample sizes are generally not a serious problem, and you should never throw away data you have already collected to achieve equal sample sizes. However, for a fixed number of participants, it is statistically most efficient to divide them into equal-sized groups. It is standard practice, therefore, to use a kind of modified random assignment that keeps the number of participants in each group as similar as possible.
One approach is block randomization. In block randomization, all the conditions occur once in the sequence before any of them is repeated. Then they all occur again before any of them is repeated again. Within each of these “blocks,” the conditions occur in a random order. Again, the sequence of conditions is usually generated before any participants are tested, and each new participant is assigned to the next condition in the sequence. When the procedure is computerized, the computer program often handles the random assignment, which is obviously much easier. You can also find programs online to help you randomize your random assignation. For example, the Research Randomizer website will generate block randomization sequences for any number of participants and conditions ( Research Randomizer ).
Random assignation is not guaranteed to control all extraneous variables across conditions. It is always possible that, just by chance, the participants in one condition might turn out to be substantially older, less tired, more motivated, or less depressed on average than the participants in another condition. However, there are some reasons that this may not be a major concern. One is that random assignment works better than one might expect, especially for large samples. Another is that the inferential statistics that researchers use to decide whether a difference between groups reflects a difference in the population take the “fallibility” of random assignment into account. Yet another reason is that even if random assignment does result in a confounding variable and therefore produces misleading results, this confound is likely to be detected when the experiment is replicated. The upshot is that random assignment to conditions—although not infallible in terms of controlling extraneous variables—is always considered a strength of a research design. Note: Do not confuse random assignation with random sampling. Random sampling is a method for selecting a sample from a population; we will talk about this in Chapter 7.
Research Methods for the Social Sciences: An Introduction Copyright © 2020 by Valerie Sheppard is licensed under a Creative Commons Attribution-NonCommercial-ShareAlike 4.0 International License , except where otherwise noted.
Share This Book
If you're seeing this message, it means we're having trouble loading external resources on our website.
If you're behind a web filter, please make sure that the domains *.kastatic.org and *.kasandbox.org are unblocked.
To log in and use all the features of Khan Academy, please enable JavaScript in your browser.
AP®︎/College Statistics
Course: ap®︎/college statistics > unit 6.
- Statistical significance of experiment
Random sampling vs. random assignment (scope of inference)
- Conclusions in observational studies versus experiments
- Finding errors in study conclusions
- (Choice A) Just the residents involved in Hilary's study. A Just the residents involved in Hilary's study.
- (Choice B) All residents in Hilary's town. B All residents in Hilary's town.
- (Choice C) All residents in Hilary's country. C All residents in Hilary's country.
- (Choice A) Yes A Yes
- (Choice B) No B No
- (Choice A) Just the residents in Hilary's study. A Just the residents in Hilary's study.
Want to join the conversation?
- Upvote Button navigates to signup page
- Downvote Button navigates to signup page
- Flag Button navigates to signup page

Chapter 6: Experimental Research
6.2 experimental design, learning objectives.
- Explain the difference between between-subjects and within-subjects experiments, list some of the pros and cons of each approach, and decide which approach to use to answer a particular research question.
- Define random assignment, distinguish it from random sampling, explain its purpose in experimental research, and use some simple strategies to implement it.
- Define what a control condition is, explain its purpose in research on treatment effectiveness, and describe some alternative types of control conditions.
- Define several types of carryover effect, give examples of each, and explain how counterbalancing helps to deal with them.
In this section, we look at some different ways to design an experiment. The primary distinction we will make is between approaches in which each participant experiences one level of the independent variable and approaches in which each participant experiences all levels of the independent variable. The former are called between-subjects experiments and the latter are called within-subjects experiments.
Between-Subjects Experiments
In a between-subjects experiment , each participant is tested in only one condition. For example, a researcher with a sample of 100 college students might assign half of them to write about a traumatic event and the other half write about a neutral event. Or a researcher with a sample of 60 people with severe agoraphobia (fear of open spaces) might assign 20 of them to receive each of three different treatments for that disorder. It is essential in a between-subjects experiment that the researcher assign participants to conditions so that the different groups are, on average, highly similar to each other. Those in a trauma condition and a neutral condition, for example, should include a similar proportion of men and women, and they should have similar average intelligence quotients (IQs), similar average levels of motivation, similar average numbers of health problems, and so on. This is a matter of controlling these extraneous participant variables across conditions so that they do not become confounding variables.
Random Assignment
The primary way that researchers accomplish this kind of control of extraneous variables across conditions is called random assignment , which means using a random process to decide which participants are tested in which conditions. Do not confuse random assignment with random sampling. Random sampling is a method for selecting a sample from a population, and it is rarely used in psychological research. Random assignment is a method for assigning participants in a sample to the different conditions, and it is an important element of all experimental research in psychology and other fields too.
In its strictest sense, random assignment should meet two criteria. One is that each participant has an equal chance of being assigned to each condition (e.g., a 50% chance of being assigned to each of two conditions). The second is that each participant is assigned to a condition independently of other participants. Thus one way to assign participants to two conditions would be to flip a coin for each one. If the coin lands heads, the participant is assigned to Condition A, and if it lands tails, the participant is assigned to Condition B. For three conditions, one could use a computer to generate a random integer from 1 to 3 for each participant. If the integer is 1, the participant is assigned to Condition A; if it is 2, the participant is assigned to Condition B; and if it is 3, the participant is assigned to Condition C. In practice, a full sequence of conditions—one for each participant expected to be in the experiment—is usually created ahead of time, and each new participant is assigned to the next condition in the sequence as he or she is tested. When the procedure is computerized, the computer program often handles the random assignment.
One problem with coin flipping and other strict procedures for random assignment is that they are likely to result in unequal sample sizes in the different conditions. Unequal sample sizes are generally not a serious problem, and you should never throw away data you have already collected to achieve equal sample sizes. However, for a fixed number of participants, it is statistically most efficient to divide them into equal-sized groups. It is standard practice, therefore, to use a kind of modified random assignment that keeps the number of participants in each group as similar as possible. One approach is block randomization . In block randomization, all the conditions occur once in the sequence before any of them is repeated. Then they all occur again before any of them is repeated again. Within each of these “blocks,” the conditions occur in a random order. Again, the sequence of conditions is usually generated before any participants are tested, and each new participant is assigned to the next condition in the sequence. Table 6.2 “Block Randomization Sequence for Assigning Nine Participants to Three Conditions” shows such a sequence for assigning nine participants to three conditions. The Research Randomizer website ( http://www.randomizer.org ) will generate block randomization sequences for any number of participants and conditions. Again, when the procedure is computerized, the computer program often handles the block randomization.
Table 6.2 Block Randomization Sequence for Assigning Nine Participants to Three Conditions
Random assignment is not guaranteed to control all extraneous variables across conditions. It is always possible that just by chance, the participants in one condition might turn out to be substantially older, less tired, more motivated, or less depressed on average than the participants in another condition. However, there are some reasons that this is not a major concern. One is that random assignment works better than one might expect, especially for large samples. Another is that the inferential statistics that researchers use to decide whether a difference between groups reflects a difference in the population takes the “fallibility” of random assignment into account. Yet another reason is that even if random assignment does result in a confounding variable and therefore produces misleading results, this is likely to be detected when the experiment is replicated. The upshot is that random assignment to conditions—although not infallible in terms of controlling extraneous variables—is always considered a strength of a research design.
Treatment and Control Conditions
Between-subjects experiments are often used to determine whether a treatment works. In psychological research, a treatment is any intervention meant to change people’s behavior for the better. This includes psychotherapies and medical treatments for psychological disorders but also interventions designed to improve learning, promote conservation, reduce prejudice, and so on. To determine whether a treatment works, participants are randomly assigned to either a treatment condition , in which they receive the treatment, or a control condition , in which they do not receive the treatment. If participants in the treatment condition end up better off than participants in the control condition—for example, they are less depressed, learn faster, conserve more, express less prejudice—then the researcher can conclude that the treatment works. In research on the effectiveness of psychotherapies and medical treatments, this type of experiment is often called a randomized clinical trial .
There are different types of control conditions. In a no-treatment control condition , participants receive no treatment whatsoever. One problem with this approach, however, is the existence of placebo effects. A placebo is a simulated treatment that lacks any active ingredient or element that should make it effective, and a placebo effect is a positive effect of such a treatment. Many folk remedies that seem to work—such as eating chicken soup for a cold or placing soap under the bedsheets to stop nighttime leg cramps—are probably nothing more than placebos. Although placebo effects are not well understood, they are probably driven primarily by people’s expectations that they will improve. Having the expectation to improve can result in reduced stress, anxiety, and depression, which can alter perceptions and even improve immune system functioning (Price, Finniss, & Benedetti, 2008).
Placebo effects are interesting in their own right (see Note 6.28 “The Powerful Placebo” ), but they also pose a serious problem for researchers who want to determine whether a treatment works. Figure 6.2 “Hypothetical Results From a Study Including Treatment, No-Treatment, and Placebo Conditions” shows some hypothetical results in which participants in a treatment condition improved more on average than participants in a no-treatment control condition. If these conditions (the two leftmost bars in Figure 6.2 “Hypothetical Results From a Study Including Treatment, No-Treatment, and Placebo Conditions” ) were the only conditions in this experiment, however, one could not conclude that the treatment worked. It could be instead that participants in the treatment group improved more because they expected to improve, while those in the no-treatment control condition did not.
Figure 6.2 Hypothetical Results From a Study Including Treatment, No-Treatment, and Placebo Conditions
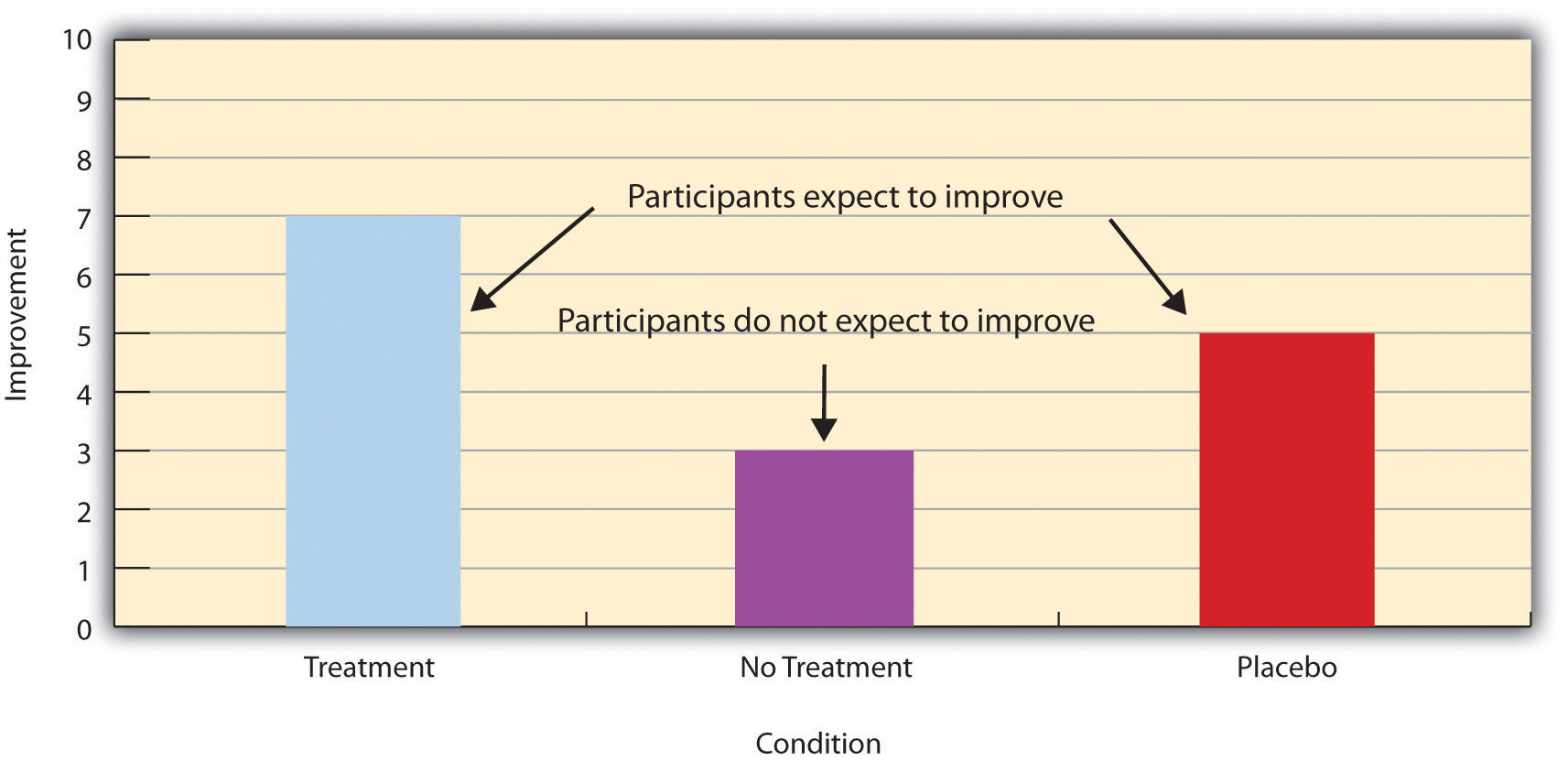
Fortunately, there are several solutions to this problem. One is to include a placebo control condition , in which participants receive a placebo that looks much like the treatment but lacks the active ingredient or element thought to be responsible for the treatment’s effectiveness. When participants in a treatment condition take a pill, for example, then those in a placebo control condition would take an identical-looking pill that lacks the active ingredient in the treatment (a “sugar pill”). In research on psychotherapy effectiveness, the placebo might involve going to a psychotherapist and talking in an unstructured way about one’s problems. The idea is that if participants in both the treatment and the placebo control groups expect to improve, then any improvement in the treatment group over and above that in the placebo control group must have been caused by the treatment and not by participants’ expectations. This is what is shown by a comparison of the two outer bars in Figure 6.2 “Hypothetical Results From a Study Including Treatment, No-Treatment, and Placebo Conditions” .
Of course, the principle of informed consent requires that participants be told that they will be assigned to either a treatment or a placebo control condition—even though they cannot be told which until the experiment ends. In many cases the participants who had been in the control condition are then offered an opportunity to have the real treatment. An alternative approach is to use a waitlist control condition , in which participants are told that they will receive the treatment but must wait until the participants in the treatment condition have already received it. This allows researchers to compare participants who have received the treatment with participants who are not currently receiving it but who still expect to improve (eventually). A final solution to the problem of placebo effects is to leave out the control condition completely and compare any new treatment with the best available alternative treatment. For example, a new treatment for simple phobia could be compared with standard exposure therapy. Because participants in both conditions receive a treatment, their expectations about improvement should be similar. This approach also makes sense because once there is an effective treatment, the interesting question about a new treatment is not simply “Does it work?” but “Does it work better than what is already available?”
The Powerful Placebo
Many people are not surprised that placebos can have a positive effect on disorders that seem fundamentally psychological, including depression, anxiety, and insomnia. However, placebos can also have a positive effect on disorders that most people think of as fundamentally physiological. These include asthma, ulcers, and warts (Shapiro & Shapiro, 1999). There is even evidence that placebo surgery—also called “sham surgery”—can be as effective as actual surgery.
Medical researcher J. Bruce Moseley and his colleagues conducted a study on the effectiveness of two arthroscopic surgery procedures for osteoarthritis of the knee (Moseley et al., 2002). The control participants in this study were prepped for surgery, received a tranquilizer, and even received three small incisions in their knees. But they did not receive the actual arthroscopic surgical procedure. The surprising result was that all participants improved in terms of both knee pain and function, and the sham surgery group improved just as much as the treatment groups. According to the researchers, “This study provides strong evidence that arthroscopic lavage with or without débridement [the surgical procedures used] is not better than and appears to be equivalent to a placebo procedure in improving knee pain and self-reported function” (p. 85).
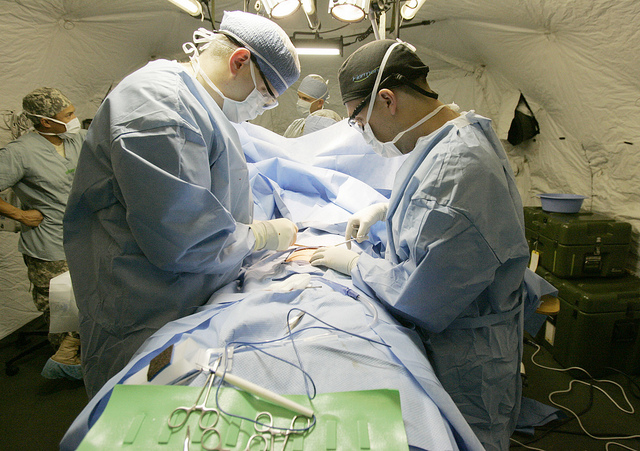
Research has shown that patients with osteoarthritis of the knee who receive a “sham surgery” experience reductions in pain and improvement in knee function similar to those of patients who receive a real surgery.
Army Medicine – Surgery – CC BY 2.0.
Within-Subjects Experiments
In a within-subjects experiment , each participant is tested under all conditions. Consider an experiment on the effect of a defendant’s physical attractiveness on judgments of his guilt. Again, in a between-subjects experiment, one group of participants would be shown an attractive defendant and asked to judge his guilt, and another group of participants would be shown an unattractive defendant and asked to judge his guilt. In a within-subjects experiment, however, the same group of participants would judge the guilt of both an attractive and an unattractive defendant.
The primary advantage of this approach is that it provides maximum control of extraneous participant variables. Participants in all conditions have the same mean IQ, same socioeconomic status, same number of siblings, and so on—because they are the very same people. Within-subjects experiments also make it possible to use statistical procedures that remove the effect of these extraneous participant variables on the dependent variable and therefore make the data less “noisy” and the effect of the independent variable easier to detect. We will look more closely at this idea later in the book.
Carryover Effects and Counterbalancing
The primary disadvantage of within-subjects designs is that they can result in carryover effects. A carryover effect is an effect of being tested in one condition on participants’ behavior in later conditions. One type of carryover effect is a practice effect , where participants perform a task better in later conditions because they have had a chance to practice it. Another type is a fatigue effect , where participants perform a task worse in later conditions because they become tired or bored. Being tested in one condition can also change how participants perceive stimuli or interpret their task in later conditions. This is called a context effect . For example, an average-looking defendant might be judged more harshly when participants have just judged an attractive defendant than when they have just judged an unattractive defendant. Within-subjects experiments also make it easier for participants to guess the hypothesis. For example, a participant who is asked to judge the guilt of an attractive defendant and then is asked to judge the guilt of an unattractive defendant is likely to guess that the hypothesis is that defendant attractiveness affects judgments of guilt. This could lead the participant to judge the unattractive defendant more harshly because he thinks this is what he is expected to do. Or it could make participants judge the two defendants similarly in an effort to be “fair.”
Carryover effects can be interesting in their own right. (Does the attractiveness of one person depend on the attractiveness of other people that we have seen recently?) But when they are not the focus of the research, carryover effects can be problematic. Imagine, for example, that participants judge the guilt of an attractive defendant and then judge the guilt of an unattractive defendant. If they judge the unattractive defendant more harshly, this might be because of his unattractiveness. But it could be instead that they judge him more harshly because they are becoming bored or tired. In other words, the order of the conditions is a confounding variable. The attractive condition is always the first condition and the unattractive condition the second. Thus any difference between the conditions in terms of the dependent variable could be caused by the order of the conditions and not the independent variable itself.
There is a solution to the problem of order effects, however, that can be used in many situations. It is counterbalancing , which means testing different participants in different orders. For example, some participants would be tested in the attractive defendant condition followed by the unattractive defendant condition, and others would be tested in the unattractive condition followed by the attractive condition. With three conditions, there would be six different orders (ABC, ACB, BAC, BCA, CAB, and CBA), so some participants would be tested in each of the six orders. With counterbalancing, participants are assigned to orders randomly, using the techniques we have already discussed. Thus random assignment plays an important role in within-subjects designs just as in between-subjects designs. Here, instead of randomly assigning to conditions, they are randomly assigned to different orders of conditions. In fact, it can safely be said that if a study does not involve random assignment in one form or another, it is not an experiment.
There are two ways to think about what counterbalancing accomplishes. One is that it controls the order of conditions so that it is no longer a confounding variable. Instead of the attractive condition always being first and the unattractive condition always being second, the attractive condition comes first for some participants and second for others. Likewise, the unattractive condition comes first for some participants and second for others. Thus any overall difference in the dependent variable between the two conditions cannot have been caused by the order of conditions. A second way to think about what counterbalancing accomplishes is that if there are carryover effects, it makes it possible to detect them. One can analyze the data separately for each order to see whether it had an effect.
When 9 Is “Larger” Than 221
Researcher Michael Birnbaum has argued that the lack of context provided by between-subjects designs is often a bigger problem than the context effects created by within-subjects designs. To demonstrate this, he asked one group of participants to rate how large the number 9 was on a 1-to-10 rating scale and another group to rate how large the number 221 was on the same 1-to-10 rating scale (Birnbaum, 1999). Participants in this between-subjects design gave the number 9 a mean rating of 5.13 and the number 221 a mean rating of 3.10. In other words, they rated 9 as larger than 221! According to Birnbaum, this is because participants spontaneously compared 9 with other one-digit numbers (in which case it is relatively large) and compared 221 with other three-digit numbers (in which case it is relatively small).
Simultaneous Within-Subjects Designs
So far, we have discussed an approach to within-subjects designs in which participants are tested in one condition at a time. There is another approach, however, that is often used when participants make multiple responses in each condition. Imagine, for example, that participants judge the guilt of 10 attractive defendants and 10 unattractive defendants. Instead of having people make judgments about all 10 defendants of one type followed by all 10 defendants of the other type, the researcher could present all 20 defendants in a sequence that mixed the two types. The researcher could then compute each participant’s mean rating for each type of defendant. Or imagine an experiment designed to see whether people with social anxiety disorder remember negative adjectives (e.g., “stupid,” “incompetent”) better than positive ones (e.g., “happy,” “productive”). The researcher could have participants study a single list that includes both kinds of words and then have them try to recall as many words as possible. The researcher could then count the number of each type of word that was recalled. There are many ways to determine the order in which the stimuli are presented, but one common way is to generate a different random order for each participant.
Between-Subjects or Within-Subjects?
Almost every experiment can be conducted using either a between-subjects design or a within-subjects design. This means that researchers must choose between the two approaches based on their relative merits for the particular situation.
Between-subjects experiments have the advantage of being conceptually simpler and requiring less testing time per participant. They also avoid carryover effects without the need for counterbalancing. Within-subjects experiments have the advantage of controlling extraneous participant variables, which generally reduces noise in the data and makes it easier to detect a relationship between the independent and dependent variables.
A good rule of thumb, then, is that if it is possible to conduct a within-subjects experiment (with proper counterbalancing) in the time that is available per participant—and you have no serious concerns about carryover effects—this is probably the best option. If a within-subjects design would be difficult or impossible to carry out, then you should consider a between-subjects design instead. For example, if you were testing participants in a doctor’s waiting room or shoppers in line at a grocery store, you might not have enough time to test each participant in all conditions and therefore would opt for a between-subjects design. Or imagine you were trying to reduce people’s level of prejudice by having them interact with someone of another race. A within-subjects design with counterbalancing would require testing some participants in the treatment condition first and then in a control condition. But if the treatment works and reduces people’s level of prejudice, then they would no longer be suitable for testing in the control condition. This is true for many designs that involve a treatment meant to produce long-term change in participants’ behavior (e.g., studies testing the effectiveness of psychotherapy). Clearly, a between-subjects design would be necessary here.
Remember also that using one type of design does not preclude using the other type in a different study. There is no reason that a researcher could not use both a between-subjects design and a within-subjects design to answer the same research question. In fact, professional researchers often do exactly this.
Key Takeaways
- Experiments can be conducted using either between-subjects or within-subjects designs. Deciding which to use in a particular situation requires careful consideration of the pros and cons of each approach.
- Random assignment to conditions in between-subjects experiments or to orders of conditions in within-subjects experiments is a fundamental element of experimental research. Its purpose is to control extraneous variables so that they do not become confounding variables.
- Experimental research on the effectiveness of a treatment requires both a treatment condition and a control condition, which can be a no-treatment control condition, a placebo control condition, or a waitlist control condition. Experimental treatments can also be compared with the best available alternative.
Discussion: For each of the following topics, list the pros and cons of a between-subjects and within-subjects design and decide which would be better.
- You want to test the relative effectiveness of two training programs for running a marathon.
- Using photographs of people as stimuli, you want to see if smiling people are perceived as more intelligent than people who are not smiling.
- In a field experiment, you want to see if the way a panhandler is dressed (neatly vs. sloppily) affects whether or not passersby give him any money.
- You want to see if concrete nouns (e.g., dog ) are recalled better than abstract nouns (e.g., truth ).
- Discussion: Imagine that an experiment shows that participants who receive psychodynamic therapy for a dog phobia improve more than participants in a no-treatment control group. Explain a fundamental problem with this research design and at least two ways that it might be corrected.
Birnbaum, M. H. (1999). How to show that 9 > 221: Collect judgments in a between-subjects design. Psychological Methods, 4 , 243–249.
Moseley, J. B., O’Malley, K., Petersen, N. J., Menke, T. J., Brody, B. A., Kuykendall, D. H., … Wray, N. P. (2002). A controlled trial of arthroscopic surgery for osteoarthritis of the knee. The New England Journal of Medicine, 347 , 81–88.
Price, D. D., Finniss, D. G., & Benedetti, F. (2008). A comprehensive review of the placebo effect: Recent advances and current thought. Annual Review of Psychology, 59 , 565–590.
Shapiro, A. K., & Shapiro, E. (1999). The powerful placebo: From ancient priest to modern physician . Baltimore, MD: Johns Hopkins University Press.
- Research Methods in Psychology. Provided by : University of Minnesota Libraries Publishing. Located at : http://open.lib.umn.edu/psychologyresearchmethods/ . License : CC BY-NC-SA: Attribution-NonCommercial-ShareAlike
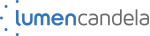
Privacy Policy
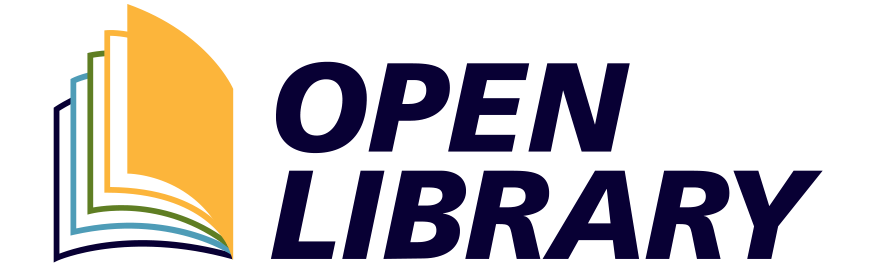
Want to create or adapt books like this? Learn more about how Pressbooks supports open publishing practices.
As previously mentioned, one of the characteristics of a true experiment is that researchers use a random process to decide which participants are tested under which conditions. Random assignation is a powerful research technique that addresses the assumption of pre-test equivalence – that the experimental and control group are equal in all respects before the administration of the independent variable (Palys & Atchison, 2014).
Random assignation is the primary way that researchers attempt to control extraneous variables across conditions. Random assignation is associated with experimental research methods. In its strictest sense, random assignment should meet two criteria. One is that each participant has an equal chance of being assigned to each condition (e.g., a 50% chance of being assigned to each of two conditions). The second is that each participant is assigned to a condition independently of other participants. Thus, one way to assign participants to two conditions would be to flip a coin for each one. If the coin lands on the heads side, the participant is assigned to Condition A, and if it lands on the tails side, the participant is assigned to Condition B. For three conditions, one could use a computer to generate a random integer from 1 to 3 for each participant. If the integer is 1, the participant is assigned to Condition A; if it is 2, the participant is assigned to Condition B; and, if it is 3, the participant is assigned to Condition C. In practice, a full sequence of conditions—one for each participant expected to be in the experiment—is usually created ahead of time, and each new participant is assigned to the next condition in the sequence as he or she is tested.
However, one problem with coin flipping and other strict procedures for random assignment is that they are likely to result in unequal sample sizes in the different conditions. Unequal sample sizes are generally not a serious problem, and you should never throw away data you have already collected to achieve equal sample sizes. However, for a fixed number of participants, it is statistically most efficient to divide them into equal-sized groups. It is standard practice, therefore, to use a kind of modified random assignment that keeps the number of participants in each group as similar as possible.
One approach is block randomization. In block randomization, all the conditions occur once in the sequence before any of them is repeated. Then they all occur again before any of them is repeated again. Within each of these “blocks,” the conditions occur in a random order. Again, the sequence of conditions is usually generated before any participants are tested, and each new participant is assigned to the next condition in the sequence. When the procedure is computerized, the computer program often handles the random assignment, which is obviously much easier. You can also find programs online to help you randomize your random assignation. For example, the Research Randomizer website will generate block randomization sequences for any number of participants and conditions ( Research Randomizer ).
Random assignation is not guaranteed to control all extraneous variables across conditions. It is always possible that, just by chance, the participants in one condition might turn out to be substantially older, less tired, more motivated, or less depressed on average than the participants in another condition. However, there are some reasons that this may not be a major concern. One is that random assignment works better than one might expect, especially for large samples. Another is that the inferential statistics that researchers use to decide whether a difference between groups reflects a difference in the population take the “fallibility” of random assignment into account. Yet another reason is that even if random assignment does result in a confounding variable and therefore produces misleading results, this confound is likely to be detected when the experiment is replicated. The upshot is that random assignment to conditions—although not infallible in terms of controlling extraneous variables—is always considered a strength of a research design. Note: Do not confuse random assignation with random sampling. Random sampling is a method for selecting a sample from a population; we will talk about this in Chapter 7.
Research Methods, Data Collection and Ethics Copyright © 2020 by Valerie Sheppard is licensed under a Creative Commons Attribution-NonCommercial-ShareAlike 4.0 International License , except where otherwise noted.
Share This Book
Impact evaluation using Difference-in-Differences
RAUSP Management Journal
ISSN : 2531-0488
Article publication date: 30 September 2019
Issue publication date: 9 December 2019
This paper aims to present the Difference-in-Differences (DiD) method in an accessible language to a broad research audience from a variety of management-related fields.
Design/methodology/approach
The paper describes the DiD method, starting with an intuitive explanation, goes through the main assumptions and the regression specification and covers the use of several robustness methods. Recurrent examples from the literature are used to illustrate the different concepts.
By providing an overview of the method, the authors cover the main issues involved when conducting DiD studies, including the fundamentals as well as some recent developments.
Originality/value
The paper can hopefully be of value to a broad range of management scholars interested in applying impact evaluation methods.
- Impact evaluation
- Policy evaluation
- Causal effects
- Difference-in-Differences
- Parallel trends assumption
Fredriksson, A. and Oliveira, G.M.d. (2019), "Impact evaluation using Difference-in-Differences", RAUSP Management Journal , Vol. 54 No. 4, pp. 519-532. https://doi.org/10.1108/RAUSP-05-2019-0112
Emerald Publishing Limited
Copyright © 2019, Anders Fredriksson and Gustavo Magalhães de Oliveira.
Published in RAUSP Management Journal . Published by Emerald Publishing Limited. This article is published under the Creative Commons Attribution (CC BY 4.0) licence. Anyone may reproduce, distribute, translate and create derivative works of this article (for both commercial and non-commercial purposes), subject to full attribution to the original publication and authors. The full terms of this licence may be seen at http://creativecommons.org/licences/by/4.0/legalcode
1. Introduction
Difference-in-Differences (DiD) is one of the most frequently used methods in impact evaluation studies. Based on a combination of before-after and treatment-control group comparisons, the method has an intuitive appeal and has been widely used in economics, public policy, health research, management and other fields. After the introductory section, this paper outlines the method, discusses its main assumptions, then provides further details and discusses potential pitfalls. Examples of typical DiD evaluations are referred to throughout the text, and a separate section discusses a few papers from the broader management literature. Conclusions are also presented.
Differently from the case of randomized experiments that allow for a simple comparison of treatment and control groups, DiD is an evaluation method used in non-experimental settings. Other members of this “family” are matching, synthetic control and regression discontinuity. The goal of these methods is to estimate the causal effects of a program when treatment assignment is non-random; hence, there is no obvious control group[ 1 ]. Although random assignment of treatment is prevalent in medical studies and has become more common also in the social sciences, through e.g. pilot studies of policy interventions, most real-life situations involve non-random assignment. Examples include the introduction of new laws, government policies and regulation[ 2 ]. When discussing different aspects of the DiD method, a much researched 2006 healthcare reform in Massachusetts, that aimed to give nearly all residents healthcare coverage, will be used as an example of a typical DiD study object. In order to estimate the causal impact of this and other policies, a key challenge is to find a proper control group.
In the Massachusetts example, one could use as control a state that did not implement the reform. A DiD estimate of reform impact can then be constructed, which in its simplest form is equivalent to calculating the after-before difference in outcomes in the treatment group, and subtracting from this difference the after-before difference in the control group. This double difference can be calculated whenever treatment and control group data on the outcomes of interest exist before and after the policy intervention. Having such data is thus a prerequisite to apply DiD. As will be detailed below, however, fulfilling this criterion does not imply that the method is always appropriate or that it will give an unbiased estimate of the causal effect.
Labor economists were among the first to apply DiD methods[ 3 ]. Ashenfelter (1978) studied the effect of training programs on earnings and Card (1990) studied labor market effects in Miami after a (non-anticipated) influx of Cuban migrants. As a control group, Card used other US cities, similar to Miami along some characteristics, but without the migration influx. Card & Krueger (1994) studied the impact of a New Jersey rise in the minimum wage on employment in fast-food restaurants. Neighboring Pennsylvania maintained its minimum wage and was used as control. Many other studies followed.
Although the basic method has not changed, several issues have been brought forward in the literature, and academic studies have evolved along with these developments. Two non-technical references covering DiD are Gertler, Martinez, Premand, Rawlings, and Vermeersch (2016) and White & Raitzer (2017) , whereas Angrist & Pischke (2009 , chapter 5) and Wooldridge (2012 , chapter 13) are textbook references. In chronological order, Angrist and Krueger (1999) , Bertrand, Duflo, and Mullainathan (2004) , Blundell & Costa Dias (2000 , 2009 ), Imbens & Wooldridge (2009) , Lechner (2011) , Athey & Imbens (2017) , Abadie & Cattaneo (2018) and Wing, Simon, and Bello-Gomez (2018) also review the method, including more technical content. The main issues brought forward in these works and in other references are discussed below.
2. The Difference-in-Differences method
The DiD method combines insights from cross-sectional treatment-control comparisons and before-after studies for a more robust identification. First consider an evaluation that seeks to estimate the effect of a (non-randomly implemented) policy (“treatment”) by comparing outcomes in the treatment group to a control group, with data from after the policy implementation. Assume there is a difference in outcomes. In the Massachusetts health reform example, perhaps health is better in the treatment group. This difference may be due to the policy, but also because there are key characteristics that differ between the groups and that are determinants of the outcomes studied, e.g. income in the health reform example: Massachusetts is relatively rich, and wealthier people on average have better health. A remedy for this situation is to evaluate the impact of the policy after controlling for the factors that differ between the two groups. This is only possible for observable characteristics, however. Perhaps important socioeconomic and other characteristics that determine outcomes are not in the dataset, or even fundamentally unobservable. And even if it would be possible to collect additional data for certain important characteristics, the knowledge about which are all the relevant variables is imperfect. Controlling for all treatment-control group differences is thus difficult.
Consider instead a before-after study, with data from the treatment group. The policy under study is implemented between the before and after periods. Assume a change over time is observed in the outcome variables of interest, such as better health. In this case, the change may have been caused by the policy, but may also be due to other changes that occurred at the same time as the policy was implemented. Perhaps there were other relevant government programs during the time of the study, or the general health status is changing over time. With treatment group data only, the change in the outcome variables may be incorrectly attributed to the intervention under study.
Now consider combining the after-before approach and the treatment-control group comparison. If the after-before difference in the control group is deducted from the same difference in the treatment group, two things are achieved. First, if other changes that occur over time are also present in the control group, then these factors are controlled for when the control group after-before difference is netted out from the impact estimate. Second, if there are important characteristics that are determinants of outcomes and that differ between the treatment and control groups, then, as long as these treatment-control group differences are constant over time, their influence is eliminated by studying changes over time. Importantly, this latter point applies also to treatment-control group differences in time-invariant unobservable characteristics (as they are netted out). It is thus possible to get around the problem, present in cross-sectional studies, that one cannot control for unobservable factors (further discussed below).
To formalize some of what has been said above, the basic DiD study has data from two groups and two time periods, and the data is typically at the individual level, that is, at a lower level than the treatment intervention itself. The data can be repeated cross-sectional samples of the population concerned (ideally random draws) or a panel. Wooldridge (2012 , chapter 13) gives examples of DiD studies using the two types of data structures and discusses the potential advantages of having a panel rather than repeated cross sections (also refer to Angrist & Pischke, 2009 , chapter 5; and Lechner, 2011 ).
With two groups and two periods, and with a sample of data from the population of interest, the DiD estimate of policy impact can be written as follows: (1) D i D = ( y ¯ s = T r e a t m e n t , t = A f t e r − y ¯ s = T r e a t m e n t , t = B e f o r e ) − ( y ¯ s = C o n t r o l , t = A f t e r − y ¯ s = C o n t r o l , t = B e f o r e ) where y is the outcome variable, the bar represents the average value (averaged over individuals, typically indexed by i ), the group is indexed by s (because in many studies, policies are implemented at the state level) and t is time. With before and after data for treatment and control, the data is thus divided into the four groups and the above double difference is calculated. The information is typically presented in a 2 × 2 table, then a third row and a third column are added in order to calculate the after-before and treatment-control differences and the DiD impact measure. Figure 1 illustrates how the DiD estimate is constructed.
The above calculation and illustration say nothing about the significance level of the DiD estimate, hence regression analysis is used. In an OLS framework, the DiD estimate is obtained as the β -coefficient in the following regression, in which A s are treatment/control group fixed effects, B t before/after fixed effects, I st is a dummy equaling 1 for treatment observations in the after period (otherwise it is zero) and ε ist the error term[ 4 ]: (2) y i s t = A s + B t + β I s t + ε i s t
In order to verify that the estimate of β will recover the DiD estimate in (1), use (2) to get E ( y i s t | s = C o n t r o l , t = B e f o r e ) = A C o n t r o l + B B e f o r e E ( y i s t | s = C o n t r o l , t = A f t e r ) = A C o n t r o l + B A f t e r E ( y i s t | s = T r e a t m e n t , t = B e f o r e ) = A T r e a t m e n t + B B e f o r e E ( y i s t | s = T r e a t m e n t , t = A f t e r ) = A T r e a t m e n t + B A f t e r + β
In these expressions, E ( y ist | s , t ) is the expected value of y ist in population subgroup ( s , t ), which is estimated by the sample average y ̄ s,t . Estimating (2) and plugging in the sample counterpart of the above expressions into (1), with the hat notation representing coefficient estimates, gives DiD =β̂[ 5 ].
The DiD model is not limited to the 2 × 2 case, and expression 2 is written in a more general form than what was needed so far. For models with several treatment- and/or control groups, A s stands for fixed effects for each of the different groups. Similarly, with several before- and/or after periods, each period has its own fixed effect, represented by B t . If the reform is implemented in all treatment groups/states at the same time, I st switches from zero to one in all such locations at the same time. In the general case, however, the reform is staggered and hence implemented in different treatment groups/states s at different times t . I st then switches from 0 to 1 accordingly. All these cases are covered by expression 2[ 6 ].
Individual-level control variables X ist can also be added to the regression, which becomes:
(3A) y i s t = A s + B t + c X i s t + β I s t + ε i s t
An important aspect of DiD estimation concerns the data used. Although it cannot be done with a 2 × 2 specification (as there would be four observations only), models with many time periods and treatment/control groups can also be analyzed with state-level (rather than individual-level) data (e.g. US or Brazilian data, with 50 and 27 states, respectively). There would then be no i -index in regression 3 A. Perhaps the relevant data is at the state level (e.g. unemployment rates from statistical institutes). Individual-level observations can also be aggregated. An advantage of the latter approach is that one avoids the problem (discussed in Section 4) that the within group-period (e.g. state-year) error terms tend to be correlated across individuals, hence standard errors should be corrected. With either type of data, also state-level control variables, Z st , may be included in expression 3 A[ 7 ]. A more general form of the regression specification, with individual-level data, becomes:
(3B) y i s t = A s + B t + c X i s t + d Z s t + β I s t + ε i s t
3. Parallel trends and other assumptions
Estimation of DiD models hinges upon several assumptions, which are discussed in detail by Lechner (2011) . The following paragraphs are mainly dedicated to the “parallel trends” assumption, the discussion of which is a requirement for any DiD paper (“no pre-treatment effects” and “common support” are also discussed below). Another important assumption is the Stable Unit Treatment Value Assumption, which implies that there should be no spillover effects between the treatment and control groups, as the treatment effect would then not be identified ( Duflo, Glennerster, & Kremer, 2008 ). Furthermore, the control variables X ist and Z st should be exogenous, unaffected by the treatment. Otherwise, β̂ will be biased. A typical approach is to use covariates that predate the intervention itself, although this does not fully rule out endogeneity concerns, as there may be anticipation effects. In some DiD studies and data sets, the controls may be available for each time period (as suggested by the t -index on X ist and Z st ), which is fine as long as they are not affected by the treatment. Implied by the assumptions is that there should be no compositional changes over time. An example would be if individuals with poor health move to Massachusetts (from a control state to the treatment state). The health reform impact would then likely be underestimated.
Identification based on DiD relies on the parallel trends assumption, which states that the treatment group, absent the reform, would have followed the same time trend as the control group (for the outcome variable of interest). Observable and unobservable factors may cause the level of the outcome variable to differ between treatment and control, but this difference (absent the reform in the treatment group) must be constant over time. Because the treatment group is only observed as treated, the assumption is fundamentally untestable. One can lend support to the assumption, however, through the use of several periods of pre-reform data, showing that the treatment and control groups exhibit a similar pattern in pre-reform periods. If such is the case, the conclusion that the impact estimated comes from the treatment itself, and not from a combination of other sources (including those causing the different pre-trends), becomes more credible. Pre-trends cannot be checked in a dataset with one before-period only, however ( Figure 1 ). In general, such studies are therefore less robust. A certain number of pre-reform periods is highly desirable and certainly a recommended “best practice” in DiD studies.
The papers on the New Jersey minimum wage increase by Card & Krueger (1994 , 2000 ) (the first referred to in Section 1) illustrate this contention and its relevance. The 1994 paper uses a two-period dataset, February 1992 (before) and November 1992 (after). By using DiD, the paper implicitly assumes parallel trends. The authors conclude that the minimum wage increase had no negative effect on fast-food restaurant employment. In the 2000 paper, the authors have access to additional data, from 1991 to 1997. In a graph of employment over time, there is little visual support for the parallel trends assumption. The extended dataset suggests that employment variation may be due to other time-varying factors than the minimum wage policy itself (for further discussion, refer to Angrist & Pischke, 2009 , chapter 5).
Figure 2(a) exemplifies, from Galiani, Gertler, and Schargrodsky (2005) and Gertler et al. (2016) , how visual support for the parallel trends assumption is typically verified in empirical work. The authors study the impact of privatizing water services on child mortality in Argentina. Using a decade of mortality data and comparing areas with privatized- (treatment) and non-privatized water companies (control), similar pre-reform (pre-1995) trends are observed. In this case also the levels are almost identical, but this is not a requirement. The authors go on to find a statistically significant reduction in child mortality in areas with privatized water services. Figure 2(b) provides another example, with data on a health variable before (and after) the 2006 Massachusetts reform, as illustrated by Courtemanche & Zapata, 2014 .
A more formal approach to provide support for the parallel trends assumption is to conduct placebo regressions, which apply the DiD method to the pre-reform data itself. There should then be no significant “treatment effect”. When running such placebo regressions, one option is to exclude all post-treatment observations and analyze the pre-reform periods only (if there is enough data available). In line with this approach, Schnabl (2012) , who studies the effects of the 1998 Russian financial crisis on bank lending, uses two years of pre-crisis data for a placebo test. An alternative is to use all data, and add to the regression specification interaction terms between each pre-treatment period and the treatment group indicator(s). The latter method is used by Courtemanche & Zapata (2014) , studying the Massachusetts health reform. A further robustness test of the DiD method is to add specific time trend-terms for the treatment and control groups, respectively, in expression 3B, and then check that the difference in trends is not significant ( Wing et al. , 2018 , p. 459)[ 8 ].
The above discussion concerns the “raw” outcome variable itself. Lechner (2011) formulates the parallel trends assumption conditional on control variables (which should be exogenous). One study using a conditional parallel trends assumption is the paper on mining and local economic activity in Peru by Aragón & Rud (2013) , especially their Figure 3. Another issue, which can be inspected in graphs such as Figure 2 , is that there should be no effect from the reform before its implementation. Finally, “common support” is needed. If the treatment group includes only high values of a control variable and the control group only low values, one is, in fact, comparing incomparable entities. There must instead be overlap in the distribution of the control variables between the different groups and time periods.
It should be noted that the parallel trends assumption is scale dependent, which is an undesirable feature of the DiD method. Unless the outcome variable is constant during the pre-reform periods, in both treatment and control, it matters if the variable is used “as is” or if it is transformed (e.g. wages vs log wages). One approach to this issue is to use the data in the form corresponding to the parameter one wants to estimate ( Lechner, 2011 ), rather than adapting the data to a format that happens to fit the parallel trends assumption.
A closing remark in this section is that it is worth spending time when planning the empirical project, before the actual analysis, carefully considering all possible data sources, if first-hand data needs to be collected, etc. Perhaps data limitations are such that a robust DiD study – including a parallel trend check – is not feasible. On the other hand, in the process of learning about the institutional details of the intervention studied, new data sources may appear.
4. Further details and considerations for the use of Difference-in-Differences
4.1 using control variables for a more robust identification.
With a non-random assignment to treatment, there is always the concern that the treatment states would have followed a different trend than the control states, even absent the reform. If, however, one can control for the factors that differ between the groups and that would lead to differences in time trends (and if these factors are exogenous), then the true effect from the treatment can be estimated[ 9 ]. In the above regression framework (expression 3B), one should thus control for the variables that differ between treatment and control and that would cause time trends in outcomes to differ. With treatment assignment at the state level, this is primarily a concern for state-level control variables ( Z st ). The main reason for including also individual-level controls ( X ist ) is instead to decrease the variance of the regression coefficient estimates ( Angrist & Pischke, 2009 , chapters 2 and 5; Wooldridge, 2012 , chapters 6 and 13).
Matching is another way to use control variables to make DiD more robust. As suggested by the name, treatment and control group observations are matched, which should reduce bias. First, think of a cross-sectional study with one dichotomous state-level variable that is relevant for treatment assignment and outcomes (e.g. Democrat/Republican state). Also assume that, even if states of one category/type are more likely to be treated, there are still treatment and control states of both types (“common support”). In this case, separate treatment effects would first be estimated for each category. The average treatment effect is then obtained by weighting with the number of treated states in each category. When the number of control variables grows and/or take on many different values (or are continuous), such exact matching is typically not possible. One alternative is to instead use the multidimensional space of covariates Z s and calculate the distance between observations in this space. Each treatment observation is matched to one or several control observations (through e.g. Mahalanobis matching, n -nearest neighbor matching), then an averaging is done over the treatment observations. Coarsening is another option. The multidimensional Z s -space is divided into different bins, observations are matched within bins and the average treatment effect is obtained by weighting over bins. Yet an option is the propensity score, P ( Z s ). This one-dimensional measure represents the probability, given Z s , that a state belongs to the treatment group. In practice, P ( Z s ) is the predicted probability from a logit or probit model of the treatment indicator regressed on Z s . The method thus matches observations based on the propensity score, again using n -nearest neighbor matching, etc[ 10 ].
When implementing matching in DiD studies, treatment and control observations are matched with methods similar to the above, e.g. coarsening or propensity score. In the case of a 2 × 2 study, a double difference similar to (1) is calculated, but the control group observations are weighted according to the results of the matching procedure[ 11 ]. An example of a DiD+matching study of the Massachusetts reform is Sommers, Long, and Baicker (2014) . Based on county-level data, the authors use the propensity score to find a comparison group to Massachusetts counties.
A third approach using control variables is the synthetic control method. Similar to DiD, it aims at balancing pre-intervention trends in the outcome variables. In the original reference, Abadie & Gardeazabal (2003) construct a counterfactual Basque Country by using data from other Spanish regions. Inspired by matching, the method minimizes the (multidimensional) distance between the values of the covariates in the treatment and control groups, by choosing different weights for the different control regions. The distance measure also depends, however, on a weight factor for each individual covariate. This second set of weights is chosen such that the pre-intervention trend in the control group, for the outcome of interest, is as close as possible to the pre-intervention trend for the treatment group. As described by Abadie & Cattaneo (2018) , the synthetic control method aims at providing a “data-driven” control group selection (and is typically implemented in econometrics software packages).
The Massachusetts health study of Courtemanche & Zapata (2014) illustrates a practice for how a DiD study may go about in selecting a control group. In the main specification, the authors use the rest of the United States as control (except a few states), and pre-reform trends are checked (including placebo tests). The control group is thereafter restricted, respectively, to the ten states with the most similar pre-reform health outcomes, to the ten states with the most similar pre-reform health trends and to other New England states only. Synthetic controls are also used. The DiD estimate is similar across specifications.
Related to the discussion of control variables is the threat to identification from compositional changes, briefly mentioned in Section 3. Assume a certain state implements a health reform. Compare with a neighboring state. If the policy induces control group individuals with poor health to move to the treatment state, the treatment outcome will then be composed also of these movers. In this case, the ideal is to have data on (and control for) individuals’ “migration status”. In practice, such data may not be available and controls X ist and Z st are instead used. This is potentially not enough, however, as there may be changes also in unobserved factors and/or spillovers and complementarities related to the changes in e.g. socioeconomic variables. One practice used to lend credibility to a DiD analysis is to search for treatment-induced compositional changes by using each covariate as a dependent variable in an expression 2-style regression. Any significant effect (the β -coefficient) would indicate a potentially troublesome compositional change ( Aragón & Rud, 2013 ).
4.2 Difference-in-Difference-in-Differences
Difference-in-Difference-in-Differences (DiDiD) is an extension of the DiD concept ( Angrist & Pischke, 2009 ), briefly mentioned through an example. Long, Yemane, & Stockley (2010) study the effects of the special provisions for young people in the Massachusetts health reform. The authors use data on both young adults and slightly older adults. Through the DiDiD method, they compare the change over time in health outcomes for young adults in Massachusetts to young adults in a comparison state and to slightly older adults in Massachusetts and construct a triple difference, to also control for other changes that occur in the treatment state.
4.3 Standard errors[ 12 ]
In the basic OLS framework, observations are assumed to be independent and standard errors homoscedastic. The standard errors of the regression coefficients then take a particularly simple form. Such errors are typically “corrected”, however, to allow for heteroscedasticity (Ecker-Huber-White heteroscedasticity-robust standard errors). The second “standard” correction is to allow for clustering. Think of individual-level data from different regions, where some regions are treated; others are not. Within a region (“cluster”), the individuals are likely to share many characteristics: perhaps they go to the same schools, work at the same firms, have access to the same media outlets, are exposed to similar weather, etc. Factors such as these make observations within clusters correlated. In effect, there is less variation than if the data had been independent random draws from the population at large. Standard errors need to be corrected accordingly, typically implying that the significance levels of the regression coefficients are reduced[ 13 ].
For correct inference with DiD, a third adjustment needs to be done. With many time periods, the data can exhibit serial correlation. This holds for many typical dependent variables in DiD studies, such as health outcomes, and, in particular, the treatment variable itself. The observations within each of the treatment and control groups can thus be correlated over time. Failing to correct for this fact can largely overstate significance levels, which was the topic of the much influential paper by Bertrand et al. (2004) .
One way of handling the within-group clustering issue is to collapse the individual data to state-level averages. Similarly, the serial correlation problem can be handled by collapsing all pre-treatment periods to one before-period, and all post-treatment periods to one after-period. Having checked the parallel trends assumption, one thus works with two periods of data, at the state level (which requires many treatment and control states). A drawback, however, is that the sample size is greatly reduced. The option to instead continue with the individual-level data and calculate standard errors that are robust to heteroscedasticity, within-group effects and serial correlation, are provided by many econometric software packages.
5. Examples of Difference-in-Differences studies in the broader management literature
The DiD method is increasingly applied in management studies. A growing number of scholars use the method in areas such as innovation ( Aggarwal & Hsu, 2014 ; Flammer & Kacperczyk, 2016 ; Singh & Agrawal, 2011 ), board of directors composition ( Berger, Kick, & Schaeck, 2014 ), lean production ( Distelhorst, Hainmueller, & Locke, 2016 ), organizational goals management ( Holm, 2018 ), CEO remuneration ( Conyon, Hass, Peck, Sadler, & Zhang, 2019 ), regulatory certification ( Bruno, Cornaggia, & Cornaggia, 2016 ), social media ( Kumar, Bezawada, Rishika, Janakiraman, & Kannan (2016) , employee monitoring ( Pierce, Snow, & McAfee, 2015 ) and environmental policy ( He & Zhang, 2018 ).
Different sources of exogenous variation have been used for econometric identification in DiD papers in the management literature. A few examples are given here. Chen, Crossland, & Huang (2014) study the effects of female board representation on mergers and acquisitions. In a robustness test to their main analysis, further addressing the issue that board composition may be endogenous, the authors exploit the fact that female board representation increases exogenously if a male board director dies. A small sample of 24 such firms are identified and matched to 24 control firms, and a basic two-group two-period DiD regression is run on this sample.
Younge, Tong, and Fleming (2014) instead use DiD as the main method and study how constraints on employee mobility affect the acquisition likelihood. The authors use as a source of identification a 1985 change in the Michigan antitrust law that had as an effect that employers could prohibit workers from leaving for a competitor. Ten US states, where no changes allegedly occurred around 1985, are used as the control group. The authors also use (coarsened exact) matching on firm characteristics to select the control group firms most similar to the Michigan firms. In addition, graphs of pre-treatment trends are presented.
Hosken, Olson, and Smith (2018) study the effect of mergers on competition. The authors do not have an exogenous source of variation, which is discussed at length. They compare grocery retail prices in geographical areas where horizontal mergers have taken place (treatment), to areas without such mergers. Several different control groups are constructed, and a test with pre-treatment price data only is conducted, to assure there is no difference in price trends. Synthetic controls are also used.
Another study is Flammer (2015) , who investigates whether product market competition affects investments in corporate social responsibility. Flammer (2015) uses import tariff reductions as the source of variation in the competitive environment and compares affected sectors (treatment) to non-affected sectors (control) over time. A matching procedure is used to increase comparability between the groups, and a robustness check restricts the sample to treatment sectors where the tariff reductions are likely to be de facto exogenous. The author also uses control variables in the DiD regression, but as pointed out in the paper, these variables have already been used in the matching procedure, and their inclusion does not alter the results.
Lemmon & Roberts (2010) study regulatory changes in the insurance industry as an exogenous contraction in the supply of below-investment-grade credit. Using Compustat data, they undertake a DiD analysis complemented by propensity score matching and explicitly analyze the parallel trends assumption. Iyer, Peydró, da-Rocha-Lopes, and Schoar (2013) examine how banks react in terms of lending when facing a negative liquidity shock. Based on Portuguese corporate loan-level data, they undertake a DiD analysis, with an identification strategy that exploits the unexpected shock to the interbank markets in August 2007. Other papers that have used DiD to study the effect of shocks to credit supply are Schnabl (2012) , referenced above, and Khwaja & Mian (2008) .
In addition to these topics, several DiD papers published in management journals relate to public policy and health, an area reviewed by Wing et al. (2018) . The above referenced Aragón & Rud (2013) and Courtemanche & Zapata (2014) are two of many papers that apply several parts of the DiD toolbox.
6. Discussion and conclusion
The paper presents an overview of the DiD method, summarized here in terms of some practical recommendations. Researchers wishing to apply the method should carefully plan their research design and think about what the source of (preferably exogenous) variation is, and how it can identify causal effects. The control group should be comparable to the treatment group and have the same data availability. Matching and other methods can refine the control group selection. Enough time periods should be available to credibly motivate the parallel trends assumption and, in case not fulfilled, it is likely that DiD is not an appropriate method. The robustness of the analysis can be enhanced by using exogenous control variables, either directly in the regression and/or through a matching procedure. Standard errors should be robust and clustered in order to account for heteroscedasticity, within-group correlation and serial correlation. Details may differ, however, including what the relevant cluster is, which depends on the study at hand, and researchers are encouraged to delve further into this topic ( Bertrand et al. , 2004 ; Cameron & Miller, 2015 ). Yet other methods, such as DiDiD and synthetic controls were discussed, while a discussion of e.g. time-varying treatment effects and another quasi-experimental technique, regression discontinuity, were left out. Several methodological DiD papers were cited above, the reading of which is encouraged, perhaps together with texts covering other non-experimental methods.
The choice of research method will vary according to many circumstances. DiD has the potential to be a feasible design in many subfields of management studies and scholars interested in the topic hopefully find this text of interest. The wide range of surveys and databases – Economatica, Capital IQ and Compustat are a few examples – enables the application of DiD in distinct contexts and to different research questions. Beyond data, the above-cited studies also demonstrate innovative ways of getting an exogenous source of variation for a credible identification strategy.
Illustration of the two-group two-period DiD estimate. The assumed treatment group counterfactual equals the treatment group pre-reform value plus the after-before difference from the control group
Graphs used to visually check the parallel trends assumption. (a) (left) Child mortality rates, different areas of Buenos Aires, Argentina, 1990-1999 (reproduced from Galiani et al ., 2005 ); (b) (right) Days per year not in good physical health, 2001-2009, Massachusetts and control states (from Courtemanche & Zapata, 2014 )
The reader is assumed to have basic knowledge about regression analysis (e.g. Wooldridge, 2012 ) and also about the core concepts in impact evaluation, e.g. identification strategy, causal inference, counterfactuals, randomization and treatment effects (e.g. Gertler, Martinez, Premand, Rawlings, & Vermeersch, 2016 , chapters 3-4; White & Raitzer, 2017 , chapters 3-4).
In this text, the terms policy, program, reform, law, regulation, intervention, shock or treatment are used interchangeably, when referring to the object being evaluated, i.e. the treatment.
Lechner (2011 ) provides a historical account, including Snow’s study of cholera in London in the 1850s.
The variable denominations are similar to those in Bertrand et al. (2004 ). An alternative way to specify regression 2, in the 2 × 2 case, is to use an intercept, treatment- and after dummies and a dummy equaling the interaction between the treatment and after dummies (e.g. Wooldridge, 2012 , chapter 13). The regression results are identical.
Angrist & Pischke (2009 ), Blundell & Costa Dias (2009 ), Lechner (2011 ) and Wing et al. (2018 ) are examples of references that provide additional details on the correspondence between the “potential outcomes framework”, the informal/intuitive/graphical derivation of the DiD measure and the regression specification, as well as a discussion of population vs. sample properties.
Note that the interpretation of β changes somewhat if the reform is staggered ( Goodman-Bacon, 2018 ). An even more general case, not covered in this text, is when I st switches on and off. A particular group/state can then go back and forth between being treated and untreated (e.g. Bertrand et al ., 2004 ). Again different is the case where I st is continuous (e.g. Aragón & Rud, 2013 ).
Note that X ist and Z st are both vectors of variables. The X -variables could be e.g. gender, age and income, i.e. three variables, each with individual level observations. Z st can be e.g. state unemployment, variables representing racial composition, number of hospital beds, etc., depending on the study. The regression coefficients c and d are (row) vectors.
See also Wing et al. (2018 , pp. 460-461) for a discussion of the related concept of event studies. Their set-up can also be used to study short- and long term reform effects. A slightly different type of placebo test is to use control states only, to study if there is an effect where there should be none ( Bertrand et al. , 2004 ).
In relation to this discussion, note that the Difference-in-Differences method estimates the Average Treatment Effect on the Treated , not on the population (e.g. Blundell & Costa Dias, 2009 ; Lechner, 2011 ; White & Raitzer, 2017 , chapter 5).
Matching (also referred to as “selection on observables”) hinges upon the Conditional Independence Assumption (CIA) (or “unconfoundedness”), which says that, conditional on the control variables, treatment and control would have the same expected outcome, in either treatment state (treated/untreated). Hence the treatment group, if untreated, would have the same expected outcome as the control group, and the selection bias disappears (e.g. Angrist & Pischke, 2009 , chapter 3). Rosenbaum & Rubin (1983 ) showed that if the CIA holds for a set of variables Z s , then it also holds for the propensity score P ( Z s ).
Such a method is used for panel data. When the data are repeated cross sections, each of the three groups treatment-before, control-before and control-after needs to be matched to the treatment-after observations ( Blundell & Costa Dias, 2000 ; Smith & Todd, 2005 ).
For a general discussion, refer to Angrist & Pischke (2009 ) and Wooldridge (2012 ). Abadie, Athey, Imbens, and Wooldridge (2017 ), Bertrand et al. (2004 ) and Cameron & Miller (2015 ) provide more details.
When there are group effects, it is important to have a large enough number of group-period cells, in order to apply DiD, an issue further discussed in Bertrand et al. (2004 ).
Abadie , A. , & Cattaneo , M. D. ( 2018 ). Econometric methods for program evaluation . Annual Review of Economics , 10 , 465 – 503 .
Abadie , A. , & Gardeazabal , J. ( 2003 ). The economic costs of conflict: A case study of the Basque Country . American Economic Review , 93 , 113 – 132 .
Abadie , A. , Athey , S. , Imbens , G. W. , & Wooldridge , J. ( 2017 ). When should you adjust standard errors for clustering? . (No. Working Paper 24003) . National Bureau of Economic Research (NBER) .
Aggarwal , V. A. , & Hsu , D. H. ( 2014 ). Entrepreneurial exits and innovation . Management Science , 60 , 867 – 887 .
Angrist , J. D. , & Krueger , A. B. ( 1999 ). Empirical strategies in labor economics . In Ashenfelter , O. , & Card , D. (Eds), Handbook of labor economics (Vol. 3 , pp. 1277 – 1366 ). Amsterdam, The Netherlands : Elsevier .
Angrist , J. D. , & Pischke , J. S. ( 2009 ). Mostly harmless econometrics: An empiricist's companion , Princeton, NJ : Princeton University Press .
Aragón , F. M. , & Rud , J. P. ( 2013 ). Natural resources and local communities: Evidence from a peruvian gold mine . American Economic Journal: Economic Policy , 5 , 1 – 25 .
Ashenfelter , O. ( 1978 ). Estimating the effect of training programs on earnings . The Review of Economics and Statistics , 60 , 47 – 57 .
Athey , S. , & Imbens , G. W. ( 2017 ). The state of applied econometrics: Causality and policy evaluation . Journal of Economic Perspectives , 31 , 3 – 32 .
Berger , A. N. , Kick , T. , & Schaeck , K. ( 2014 ). Executive board composition and bank risk taking . Journal of Corporate Finance , 28 , 48 – 65 .
Bertrand , M. , Duflo , E. , & Mullainathan , S. ( 2004 ). How much should we trust differences-in-differences estimates? The Quarterly Journal of Economics , 119 , 249 – 275 .
Blundell , R. , & Costa Dias , M. ( 2000 ). Evaluation methods for non‐experimental data . Fiscal Studies , 21 , 427 – 468 .
Blundell , R. , & Costa Dias , M. ( 2009 ). Alternative approaches to evaluation in empirical microeconomics . Journal of Human Resources , 44 , 565 – 640 .
Bruno , V. , Cornaggia , J. , & Cornaggia , J. K. ( 2016 ). Does regulatory certification affect the information content of credit ratings? . Management Science , 62 , 1578 – 1597 .
Cameron , A. C. , & Miller , D. L. ( 2015 ). A practitioner’s guide to cluster-robust inference . Journal of Human Resources , 50 , 317 – 372 .
Card , D. ( 1990 ). The impact of the Mariel boatlift on the Miami labor market . ILR Review , 43 , 245 – 257 .
Card , D. , & Krueger , A. B. ( 1994 ). Wages and employment: A case study of the fast-food industry in New Jersey and Pennsylvania . American Economic Review , 84 , 772 – 793 .
Card , D. , & Krueger , A. B. ( 2000 ). Minimum wages and employment: A case study of the fast-food industry in New Jersey and Pennsylvania: reply . American Economic Review , 90 , 1397 – 1420 .
Chen , G. , Crossland , C. , & Huang , S. ( 2014 ). Female board representation and corporate acquisition intensity . Strategic Management Journal , 37 , 303 – 313 .
Conyon , M. J. , Hass , L. H. , Peck , S. I. , Sadler , G. V. , & Zhang , Z. ( 2019 ). Do compensation consultants drive up CEO pay? Evidence from UK public firms . British Journal of Management , 30 , 10 – 29 .
Courtemanche , C. J. , & Zapata , D. ( 2014 ). Does universal coverage improve health? The Massachusetts experience . Journal of Policy Analysis and Management , 33 , 36 – 69 .
Distelhorst , G. , Hainmueller , J. , & Locke , R. M. ( 2016 ). Does lean improve labor standards? Management and social performance in the Nike supply chain . Management Science , 63 , 707 – 728 .
Duflo , E. , Glennerster , R. , & Kremer , M. ( 2008 ). Using randomization in development economics research: A toolkit . In P. Schultz , & J. Strauss , (Eds.), Handbook of development economics (Vol. 4 ). Amsterdam, The Netherlands and Oxford, UK : Elsevier; North-Holland , 3895 – 3962 .
Flammer , C. ( 2015 ). Does product market competition foster corporate social responsibility? . Strategic Management Journal , 38 , 163 – 183 .
Flammer , C. , & Kacperczyk , A. ( 2016 ). The impact of stakeholder orientation on innovation: Evidence from a natural experiment . Management Science , 62 , 1982 – 2001 .
Galiani , S. , Gertler , P. , & Schargrodsky , E. ( 2005 ). Water for life: The impact of the privatization of water services on child mortality . Journal of Political Economy , 113 , 83 – 120 .
Gertler , P. J. , Martinez , S. , Premand , P. , Rawlings , L. B. , & Vermeersch , C. M. ( 2016 ). Impact evaluation in practice , Washington, DC : The World Bank .
Goodman-Bacon , A. ( 2018 ). Difference-in-Differences with variation in treatment timing . NBER Working Paper No. 25018 . NBER .
He , P. , & Zhang , B. ( 2018 ). Environmental tax, polluting plants’ strategies and effectiveness: Evidence from China . Journal of Policy Analysis and Management , 37 , 493 – 520 .
Holm , J. M. ( 2018 ). Successful problem solvers? Managerial performance information use to improve low organizational performance . Journal of Public Administration Research and Theory , 28 , 303 – 320 .
Hosken , D. S. , Olson , L. M. , & Smith , L. K. ( 2018 ). Do retail mergers affect competition? Evidence from grocery retailing . Journal of Economics & Management Strategy , 27 , 3 – 22 .
Imbens , G. W. , & Wooldridge , J. M. ( 2009 ). Recent developments in the econometrics of program evaluation . Journal of Economic Literature , 47 , 5 – 86 .
Iyer , R. , Peydró , J. L. , da-Rocha-Lopes , S. , & Schoar , A. ( 2013 ). Interbank liquidity crunch and the firm credit crunch: Evidence from the 2007-2009 crisis . Review of Financial Studies , 27 , 347 – 372 .
Khwaja , A. I. , & Mian , A. ( 2008 ). Tracing the impact of bank liquidity shocks: Evidence from an emerging market . American Economic Review , 98 , 1413 – 1442 .
Kumar , A. , Bezawada , R. , Rishika , R. , Janakiraman , R. , & Kannan , P. K. ( 2016 ). From social to sale: The effects of firm-generated content in social media on customer behavior . Journal of Marketing , 80 , 7 – 25 .
Lechner , M. ( 2011 ). The estimation of causal effects by difference-in-difference methods . Foundations and Trends® in Econometrics , 4 , 165 – 224 .
Lemmon , M. , & Roberts , M. R. ( 2010 ). The response of corporate financing and investment to changes in the supply of credit . Journal of Financial and Quantitative Analysis , 45 , 555 – 587 .
Long , S. K. , Yemane , A. , & Stockley , K. ( 2010 ). Disentangling the effects of health reform in Massachusetts: How important are the special provisions for young adults? . American Economic Review , 100 , 297 – 302 .
Pierce , L. , Snow , D. C. , & McAfee , A. ( 2015 ). cleaning house: The impact of information technology monitoring on employee theft and productivity . Management Science , 61 , 2299 – 2319 .
Rosenbaum , P. R. , & Rubin , D. B. ( 1983 ). The Central role of the propensity score in observational studies for causal effects . Biometrika , 70 , 41 – 55 .
Schnabl , P. ( 2012 ). The international transmission of bank liquidity shocks: Evidence from an emerging market . The Journal of Finance , 67 , 897 – 932 .
Singh , J. , & Agrawal , A. ( 2011 ). Recruiting for ideas: How firms exploit the prior inventions of new hires . Management Science , 57 :, 129 – 150 .
Smith , J. A. , & Todd , P. E. ( 2005 ). Does matching overcome LaLonde's critique of nonexperimental estimators? Journal of Econometrics , 125 , 305 – 353 .
Sommers , B. D. , Long , S. K. , & Baicker , K. ( 2014 ). Changes in mortality after Massachusetts health care reform: A quasi-experimental study . Annals of Internal Medicine , 160 , 585 – 594 .
White , H. , & Raitzer , D. A. ( 2017 ). Impact evaluation of development interventions: A practical guide , Mandaluyong, Philippines : Asian Development Bank .
Wing , C. , Simon , K. , & Bello-Gomez , R. A. ( 2018 ). Designing difference in difference studies: Best practices for public health policy research . Annual Review of Public Health , 39 , 453 – 469 .
Wooldridge , J. M. ( 2012 ). Introductory econometrics: a modern approach ( 5th ed .). Mason, OH : South-Western College Publisher .
Younge , K. A. , Tong , T. W. , & Fleming , L. ( 2014 ). How anticipated employee mobility affects acquisition likelihood: Evidence from a natural experiment . Strategic Management Journal , 36 , 686 – 708 .
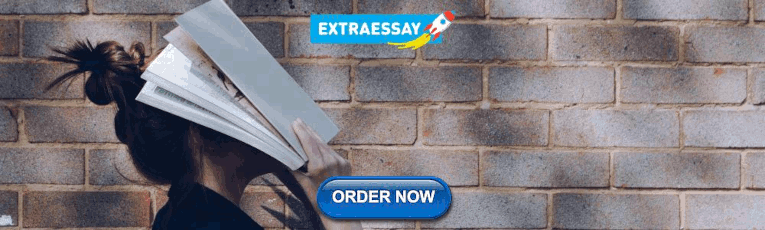
Acknowledgements
Anders Fredriksson and Gustavo Magalhães de Oliveira contributed equally to this paper.
The authors thank the editor, two anonymous referees and Pamela Campa, Maria Perrotta Berlin and Carolina Segovia for feedback that improved the paper. Any errors are our own.
Corresponding author
Related articles, we’re listening — tell us what you think, something didn’t work….
Report bugs here
All feedback is valuable
Please share your general feedback
Join us on our journey
Platform update page.
Visit emeraldpublishing.com/platformupdate to discover the latest news and updates
Questions & More Information
Answers to the most commonly asked questions here

Statistics Made Easy
Random Selection vs. Random Assignment
Random selection and random assignment are two techniques in statistics that are commonly used, but are commonly confused.
Random selection refers to the process of randomly selecting individuals from a population to be involved in a study.
Random assignment refers to the process of randomly assigning the individuals in a study to either a treatment group or a control group.
You can think of random selection as the process you use to “get” the individuals in a study and you can think of random assignment as what you “do” with those individuals once they’re selected to be part of the study.
The Importance of Random Selection and Random Assignment
When a study uses random selection , it selects individuals from a population using some random process. For example, if some population has 1,000 individuals then we might use a computer to randomly select 100 of those individuals from a database. This means that each individual is equally likely to be selected to be part of the study, which increases the chances that we will obtain a representative sample – a sample that has similar characteristics to the overall population.
By using a representative sample in our study, we’re able to generalize the findings of our study to the population. In statistical terms, this is referred to as having external validity – it’s valid to externalize our findings to the overall population.
When a study uses random assignment , it randomly assigns individuals to either a treatment group or a control group. For example, if we have 100 individuals in a study then we might use a random number generator to randomly assign 50 individuals to a control group and 50 individuals to a treatment group.
By using random assignment, we increase the chances that the two groups will have roughly similar characteristics, which means that any difference we observe between the two groups can be attributed to the treatment. This means the study has internal validity – it’s valid to attribute any differences between the groups to the treatment itself as opposed to differences between the individuals in the groups.
Examples of Random Selection and Random Assignment
It’s possible for a study to use both random selection and random assignment, or just one of these techniques, or neither technique. A strong study is one that uses both techniques.
The following examples show how a study could use both, one, or neither of these techniques, along with the effects of doing so.
Example 1: Using both Random Selection and Random Assignment
Study: Researchers want to know whether a new diet leads to more weight loss than a standard diet in a certain community of 10,000 people. They recruit 100 individuals to be in the study by using a computer to randomly select 100 names from a database. Once they have the 100 individuals, they once again use a computer to randomly assign 50 of the individuals to a control group (e.g. stick with their standard diet) and 50 individuals to a treatment group (e.g. follow the new diet). They record the total weight loss of each individual after one month.

Results: The researchers used random selection to obtain their sample and random assignment when putting individuals in either a treatment or control group. By doing so, they’re able to generalize the findings from the study to the overall population and they’re able to attribute any differences in average weight loss between the two groups to the new diet.
Example 2: Using only Random Selection
Study: Researchers want to know whether a new diet leads to more weight loss than a standard diet in a certain community of 10,000 people. They recruit 100 individuals to be in the study by using a computer to randomly select 100 names from a database. However, they decide to assign individuals to groups based solely on gender. Females are assigned to the control group and males are assigned to the treatment group. They record the total weight loss of each individual after one month.

Results: The researchers used random selection to obtain their sample, but they did not use random assignment when putting individuals in either a treatment or control group. Instead, they used a specific factor – gender – to decide which group to assign individuals to. By doing this, they’re able to generalize the findings from the study to the overall population but they are not able to attribute any differences in average weight loss between the two groups to the new diet. The internal validity of the study has been compromised because the difference in weight loss could actually just be due to gender, rather than the new diet.
Example 3: Using only Random Assignment
Study: Researchers want to know whether a new diet leads to more weight loss than a standard diet in a certain community of 10,000 people. They recruit 100 males athletes to be in the study. Then, they use a computer program to randomly assign 50 of the male athletes to a control group and 50 to the treatment group. They record the total weight loss of each individual after one month.

Results: The researchers did not use random selection to obtain their sample since they specifically chose 100 male athletes. Because of this, their sample is not representative of the overall population so their external validity is compromised – they will not be able to generalize the findings from the study to the overall population. However, they did use random assignment, which means they can attribute any difference in weight loss to the new diet.
Example 4: Using Neither Technique
Study: Researchers want to know whether a new diet leads to more weight loss than a standard diet in a certain community of 10,000 people. They recruit 50 males athletes and 50 female athletes to be in the study. Then, they assign all of the female athletes to the control group and all of the male athletes to the treatment group. They record the total weight loss of each individual after one month.

Results: The researchers did not use random selection to obtain their sample since they specifically chose 100 athletes. Because of this, their sample is not representative of the overall population so their external validity is compromised – they will not be able to generalize the findings from the study to the overall population. Also, they split individuals into groups based on gender rather than using random assignment, which means their internal validity is also compromised – differences in weight loss might be due to gender rather than the diet.

Published by Zach
Leave a reply cancel reply.
Your email address will not be published. Required fields are marked *
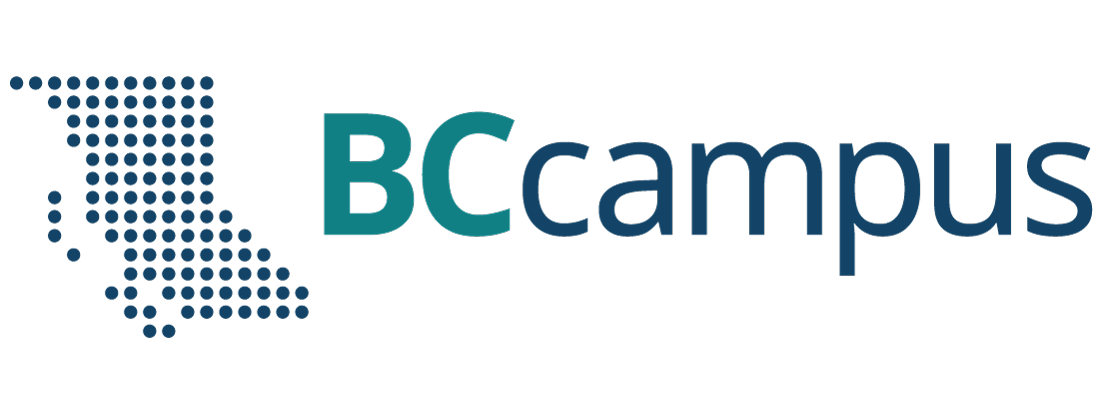
Want to create or adapt books like this? Learn more about how Pressbooks supports open publishing practices.
Chapter 6: Experimental Research
Experimental Design
Learning Objectives
- Explain the difference between between-subjects and within-subjects experiments, list some of the pros and cons of each approach, and decide which approach to use to answer a particular research question.
- Define random assignment, distinguish it from random sampling, explain its purpose in experimental research, and use some simple strategies to implement it.
- Define what a control condition is, explain its purpose in research on treatment effectiveness, and describe some alternative types of control conditions.
- Define several types of carryover effect, give examples of each, and explain how counterbalancing helps to deal with them.
In this section, we look at some different ways to design an experiment. The primary distinction we will make is between approaches in which each participant experiences one level of the independent variable and approaches in which each participant experiences all levels of the independent variable. The former are called between-subjects experiments and the latter are called within-subjects experiments.
Between-Subjects Experiments
In a between-subjects experiment , each participant is tested in only one condition. For example, a researcher with a sample of 100 university students might assign half of them to write about a traumatic event and the other half write about a neutral event. Or a researcher with a sample of 60 people with severe agoraphobia (fear of open spaces) might assign 20 of them to receive each of three different treatments for that disorder. It is essential in a between-subjects experiment that the researcher assign participants to conditions so that the different groups are, on average, highly similar to each other. Those in a trauma condition and a neutral condition, for example, should include a similar proportion of men and women, and they should have similar average intelligence quotients (IQs), similar average levels of motivation, similar average numbers of health problems, and so on. This matching is a matter of controlling these extraneous participant variables across conditions so that they do not become confounding variables.
Random Assignment
The primary way that researchers accomplish this kind of control of extraneous variables across conditions is called random assignment , which means using a random process to decide which participants are tested in which conditions. Do not confuse random assignment with random sampling. Random sampling is a method for selecting a sample from a population, and it is rarely used in psychological research. Random assignment is a method for assigning participants in a sample to the different conditions, and it is an important element of all experimental research in psychology and other fields too.
In its strictest sense, random assignment should meet two criteria. One is that each participant has an equal chance of being assigned to each condition (e.g., a 50% chance of being assigned to each of two conditions). The second is that each participant is assigned to a condition independently of other participants. Thus one way to assign participants to two conditions would be to flip a coin for each one. If the coin lands heads, the participant is assigned to Condition A, and if it lands tails, the participant is assigned to Condition B. For three conditions, one could use a computer to generate a random integer from 1 to 3 for each participant. If the integer is 1, the participant is assigned to Condition A; if it is 2, the participant is assigned to Condition B; and if it is 3, the participant is assigned to Condition C. In practice, a full sequence of conditions—one for each participant expected to be in the experiment—is usually created ahead of time, and each new participant is assigned to the next condition in the sequence as he or she is tested. When the procedure is computerized, the computer program often handles the random assignment.
One problem with coin flipping and other strict procedures for random assignment is that they are likely to result in unequal sample sizes in the different conditions. Unequal sample sizes are generally not a serious problem, and you should never throw away data you have already collected to achieve equal sample sizes. However, for a fixed number of participants, it is statistically most efficient to divide them into equal-sized groups. It is standard practice, therefore, to use a kind of modified random assignment that keeps the number of participants in each group as similar as possible. One approach is block randomization . In block randomization, all the conditions occur once in the sequence before any of them is repeated. Then they all occur again before any of them is repeated again. Within each of these “blocks,” the conditions occur in a random order. Again, the sequence of conditions is usually generated before any participants are tested, and each new participant is assigned to the next condition in the sequence. Table 6.2 shows such a sequence for assigning nine participants to three conditions. The Research Randomizer website will generate block randomization sequences for any number of participants and conditions. Again, when the procedure is computerized, the computer program often handles the block randomization.
Random assignment is not guaranteed to control all extraneous variables across conditions. It is always possible that just by chance, the participants in one condition might turn out to be substantially older, less tired, more motivated, or less depressed on average than the participants in another condition. However, there are some reasons that this possibility is not a major concern. One is that random assignment works better than one might expect, especially for large samples. Another is that the inferential statistics that researchers use to decide whether a difference between groups reflects a difference in the population takes the “fallibility” of random assignment into account. Yet another reason is that even if random assignment does result in a confounding variable and therefore produces misleading results, this confound is likely to be detected when the experiment is replicated. The upshot is that random assignment to conditions—although not infallible in terms of controlling extraneous variables—is always considered a strength of a research design.
Treatment and Control Conditions
Between-subjects experiments are often used to determine whether a treatment works. In psychological research, a treatment is any intervention meant to change people’s behaviour for the better. This intervention includes psychotherapies and medical treatments for psychological disorders but also interventions designed to improve learning, promote conservation, reduce prejudice, and so on. To determine whether a treatment works, participants are randomly assigned to either a treatment condition , in which they receive the treatment, or a control condition , in which they do not receive the treatment. If participants in the treatment condition end up better off than participants in the control condition—for example, they are less depressed, learn faster, conserve more, express less prejudice—then the researcher can conclude that the treatment works. In research on the effectiveness of psychotherapies and medical treatments, this type of experiment is often called a randomized clinical trial .
There are different types of control conditions. In a no-treatment control condition , participants receive no treatment whatsoever. One problem with this approach, however, is the existence of placebo effects. A placebo is a simulated treatment that lacks any active ingredient or element that should make it effective, and a placebo effect is a positive effect of such a treatment. Many folk remedies that seem to work—such as eating chicken soup for a cold or placing soap under the bedsheets to stop nighttime leg cramps—are probably nothing more than placebos. Although placebo effects are not well understood, they are probably driven primarily by people’s expectations that they will improve. Having the expectation to improve can result in reduced stress, anxiety, and depression, which can alter perceptions and even improve immune system functioning (Price, Finniss, & Benedetti, 2008) [1] .
Placebo effects are interesting in their own right (see Note “The Powerful Placebo” ), but they also pose a serious problem for researchers who want to determine whether a treatment works. Figure 6.2 shows some hypothetical results in which participants in a treatment condition improved more on average than participants in a no-treatment control condition. If these conditions (the two leftmost bars in Figure 6.2 ) were the only conditions in this experiment, however, one could not conclude that the treatment worked. It could be instead that participants in the treatment group improved more because they expected to improve, while those in the no-treatment control condition did not.
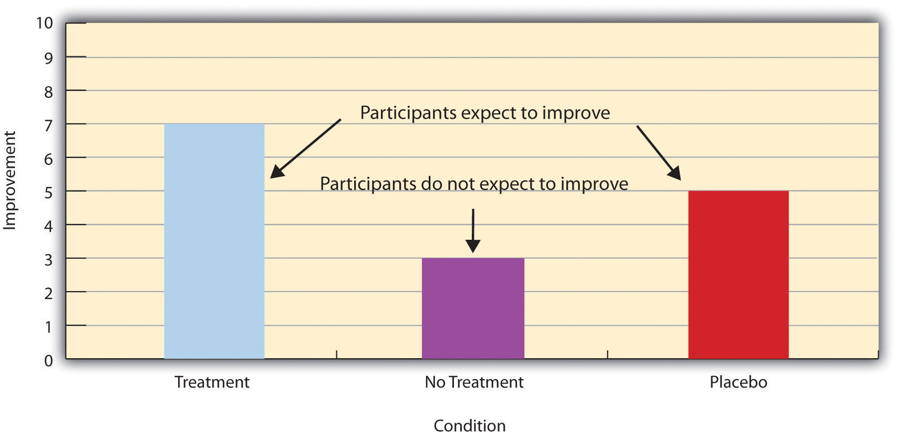
Fortunately, there are several solutions to this problem. One is to include a placebo control condition , in which participants receive a placebo that looks much like the treatment but lacks the active ingredient or element thought to be responsible for the treatment’s effectiveness. When participants in a treatment condition take a pill, for example, then those in a placebo control condition would take an identical-looking pill that lacks the active ingredient in the treatment (a “sugar pill”). In research on psychotherapy effectiveness, the placebo might involve going to a psychotherapist and talking in an unstructured way about one’s problems. The idea is that if participants in both the treatment and the placebo control groups expect to improve, then any improvement in the treatment group over and above that in the placebo control group must have been caused by the treatment and not by participants’ expectations. This difference is what is shown by a comparison of the two outer bars in Figure 6.2 .
Of course, the principle of informed consent requires that participants be told that they will be assigned to either a treatment or a placebo control condition—even though they cannot be told which until the experiment ends. In many cases the participants who had been in the control condition are then offered an opportunity to have the real treatment. An alternative approach is to use a waitlist control condition , in which participants are told that they will receive the treatment but must wait until the participants in the treatment condition have already received it. This disclosure allows researchers to compare participants who have received the treatment with participants who are not currently receiving it but who still expect to improve (eventually). A final solution to the problem of placebo effects is to leave out the control condition completely and compare any new treatment with the best available alternative treatment. For example, a new treatment for simple phobia could be compared with standard exposure therapy. Because participants in both conditions receive a treatment, their expectations about improvement should be similar. This approach also makes sense because once there is an effective treatment, the interesting question about a new treatment is not simply “Does it work?” but “Does it work better than what is already available?
The Powerful Placebo
Many people are not surprised that placebos can have a positive effect on disorders that seem fundamentally psychological, including depression, anxiety, and insomnia. However, placebos can also have a positive effect on disorders that most people think of as fundamentally physiological. These include asthma, ulcers, and warts (Shapiro & Shapiro, 1999) [2] . There is even evidence that placebo surgery—also called “sham surgery”—can be as effective as actual surgery.
Medical researcher J. Bruce Moseley and his colleagues conducted a study on the effectiveness of two arthroscopic surgery procedures for osteoarthritis of the knee (Moseley et al., 2002) [3] . The control participants in this study were prepped for surgery, received a tranquilizer, and even received three small incisions in their knees. But they did not receive the actual arthroscopic surgical procedure. The surprising result was that all participants improved in terms of both knee pain and function, and the sham surgery group improved just as much as the treatment groups. According to the researchers, “This study provides strong evidence that arthroscopic lavage with or without débridement [the surgical procedures used] is not better than and appears to be equivalent to a placebo procedure in improving knee pain and self-reported function” (p. 85).
Within-Subjects Experiments
In a within-subjects experiment , each participant is tested under all conditions. Consider an experiment on the effect of a defendant’s physical attractiveness on judgments of his guilt. Again, in a between-subjects experiment, one group of participants would be shown an attractive defendant and asked to judge his guilt, and another group of participants would be shown an unattractive defendant and asked to judge his guilt. In a within-subjects experiment, however, the same group of participants would judge the guilt of both an attractive and an unattractive defendant.
The primary advantage of this approach is that it provides maximum control of extraneous participant variables. Participants in all conditions have the same mean IQ, same socioeconomic status, same number of siblings, and so on—because they are the very same people. Within-subjects experiments also make it possible to use statistical procedures that remove the effect of these extraneous participant variables on the dependent variable and therefore make the data less “noisy” and the effect of the independent variable easier to detect. We will look more closely at this idea later in the book. However, not all experiments can use a within-subjects design nor would it be desirable to.
Carryover Effects and Counterbalancing
The primary disad vantage of within-subjects designs is that they can result in carryover effects. A carryover effect is an effect of being tested in one condition on participants’ behaviour in later conditions. One type of carryover effect is a practice effect , where participants perform a task better in later conditions because they have had a chance to practice it. Another type is a fatigue effect , where participants perform a task worse in later conditions because they become tired or bored. Being tested in one condition can also change how participants perceive stimuli or interpret their task in later conditions. This type of effect is called a context effect . For example, an average-looking defendant might be judged more harshly when participants have just judged an attractive defendant than when they have just judged an unattractive defendant. Within-subjects experiments also make it easier for participants to guess the hypothesis. For example, a participant who is asked to judge the guilt of an attractive defendant and then is asked to judge the guilt of an unattractive defendant is likely to guess that the hypothesis is that defendant attractiveness affects judgments of guilt. This knowledge could lead the participant to judge the unattractive defendant more harshly because he thinks this is what he is expected to do. Or it could make participants judge the two defendants similarly in an effort to be “fair.”
Carryover effects can be interesting in their own right. (Does the attractiveness of one person depend on the attractiveness of other people that we have seen recently?) But when they are not the focus of the research, carryover effects can be problematic. Imagine, for example, that participants judge the guilt of an attractive defendant and then judge the guilt of an unattractive defendant. If they judge the unattractive defendant more harshly, this might be because of his unattractiveness. But it could be instead that they judge him more harshly because they are becoming bored or tired. In other words, the order of the conditions is a confounding variable. The attractive condition is always the first condition and the unattractive condition the second. Thus any difference between the conditions in terms of the dependent variable could be caused by the order of the conditions and not the independent variable itself.
There is a solution to the problem of order effects, however, that can be used in many situations. It is counterbalancing , which means testing different participants in different orders. For example, some participants would be tested in the attractive defendant condition followed by the unattractive defendant condition, and others would be tested in the unattractive condition followed by the attractive condition. With three conditions, there would be six different orders (ABC, ACB, BAC, BCA, CAB, and CBA), so some participants would be tested in each of the six orders. With counterbalancing, participants are assigned to orders randomly, using the techniques we have already discussed. Thus random assignment plays an important role in within-subjects designs just as in between-subjects designs. Here, instead of randomly assigning to conditions, they are randomly assigned to different orders of conditions. In fact, it can safely be said that if a study does not involve random assignment in one form or another, it is not an experiment.
An efficient way of counterbalancing is through a Latin square design which randomizes through having equal rows and columns. For example, if you have four treatments, you must have four versions. Like a Sudoku puzzle, no treatment can repeat in a row or column. For four versions of four treatments, the Latin square design would look like:
There are two ways to think about what counterbalancing accomplishes. One is that it controls the order of conditions so that it is no longer a confounding variable. Instead of the attractive condition always being first and the unattractive condition always being second, the attractive condition comes first for some participants and second for others. Likewise, the unattractive condition comes first for some participants and second for others. Thus any overall difference in the dependent variable between the two conditions cannot have been caused by the order of conditions. A second way to think about what counterbalancing accomplishes is that if there are carryover effects, it makes it possible to detect them. One can analyze the data separately for each order to see whether it had an effect.
When 9 is “larger” than 221
Researcher Michael Birnbaum has argued that the lack of context provided by between-subjects designs is often a bigger problem than the context effects created by within-subjects designs. To demonstrate this problem, he asked participants to rate two numbers on how large they were on a scale of 1-to-10 where 1 was “very very small” and 10 was “very very large”. One group of participants were asked to rate the number 9 and another group was asked to rate the number 221 (Birnbaum, 1999) [4] . Participants in this between-subjects design gave the number 9 a mean rating of 5.13 and the number 221 a mean rating of 3.10. In other words, they rated 9 as larger than 221! According to Birnbaum, this difference is because participants spontaneously compared 9 with other one-digit numbers (in which case it is relatively large) and compared 221 with other three-digit numbers (in which case it is relatively small) .
Simultaneous Within-Subjects Designs
So far, we have discussed an approach to within-subjects designs in which participants are tested in one condition at a time. There is another approach, however, that is often used when participants make multiple responses in each condition. Imagine, for example, that participants judge the guilt of 10 attractive defendants and 10 unattractive defendants. Instead of having people make judgments about all 10 defendants of one type followed by all 10 defendants of the other type, the researcher could present all 20 defendants in a sequence that mixed the two types. The researcher could then compute each participant’s mean rating for each type of defendant. Or imagine an experiment designed to see whether people with social anxiety disorder remember negative adjectives (e.g., “stupid,” “incompetent”) better than positive ones (e.g., “happy,” “productive”). The researcher could have participants study a single list that includes both kinds of words and then have them try to recall as many words as possible. The researcher could then count the number of each type of word that was recalled. There are many ways to determine the order in which the stimuli are presented, but one common way is to generate a different random order for each participant.
Between-Subjects or Within-Subjects?
Almost every experiment can be conducted using either a between-subjects design or a within-subjects design. This possibility means that researchers must choose between the two approaches based on their relative merits for the particular situation.
Between-subjects experiments have the advantage of being conceptually simpler and requiring less testing time per participant. They also avoid carryover effects without the need for counterbalancing. Within-subjects experiments have the advantage of controlling extraneous participant variables, which generally reduces noise in the data and makes it easier to detect a relationship between the independent and dependent variables.
A good rule of thumb, then, is that if it is possible to conduct a within-subjects experiment (with proper counterbalancing) in the time that is available per participant—and you have no serious concerns about carryover effects—this design is probably the best option. If a within-subjects design would be difficult or impossible to carry out, then you should consider a between-subjects design instead. For example, if you were testing participants in a doctor’s waiting room or shoppers in line at a grocery store, you might not have enough time to test each participant in all conditions and therefore would opt for a between-subjects design. Or imagine you were trying to reduce people’s level of prejudice by having them interact with someone of another race. A within-subjects design with counterbalancing would require testing some participants in the treatment condition first and then in a control condition. But if the treatment works and reduces people’s level of prejudice, then they would no longer be suitable for testing in the control condition. This difficulty is true for many designs that involve a treatment meant to produce long-term change in participants’ behaviour (e.g., studies testing the effectiveness of psychotherapy). Clearly, a between-subjects design would be necessary here.
Remember also that using one type of design does not preclude using the other type in a different study. There is no reason that a researcher could not use both a between-subjects design and a within-subjects design to answer the same research question. In fact, professional researchers often take exactly this type of mixed methods approach.
Key Takeaways
- Experiments can be conducted using either between-subjects or within-subjects designs. Deciding which to use in a particular situation requires careful consideration of the pros and cons of each approach.
- Random assignment to conditions in between-subjects experiments or to orders of conditions in within-subjects experiments is a fundamental element of experimental research. Its purpose is to control extraneous variables so that they do not become confounding variables.
- Experimental research on the effectiveness of a treatment requires both a treatment condition and a control condition, which can be a no-treatment control condition, a placebo control condition, or a waitlist control condition. Experimental treatments can also be compared with the best available alternative.
- You want to test the relative effectiveness of two training programs for running a marathon.
- Using photographs of people as stimuli, you want to see if smiling people are perceived as more intelligent than people who are not smiling.
- In a field experiment, you want to see if the way a panhandler is dressed (neatly vs. sloppily) affects whether or not passersby give him any money.
- You want to see if concrete nouns (e.g., dog ) are recalled better than abstract nouns (e.g., truth ).
- Discussion: Imagine that an experiment shows that participants who receive psychodynamic therapy for a dog phobia improve more than participants in a no-treatment control group. Explain a fundamental problem with this research design and at least two ways that it might be corrected.
- Price, D. D., Finniss, D. G., & Benedetti, F. (2008). A comprehensive review of the placebo effect: Recent advances and current thought. Annual Review of Psychology, 59 , 565–590. ↵
- Shapiro, A. K., & Shapiro, E. (1999). The powerful placebo: From ancient priest to modern physician . Baltimore, MD: Johns Hopkins University Press. ↵
- Moseley, J. B., O’Malley, K., Petersen, N. J., Menke, T. J., Brody, B. A., Kuykendall, D. H., … Wray, N. P. (2002). A controlled trial of arthroscopic surgery for osteoarthritis of the knee. The New England Journal of Medicine, 347 , 81–88. ↵
- Birnbaum, M.H. (1999). How to show that 9>221: Collect judgments in a between-subjects design. Psychological Methods, 4(3), 243-249. ↵
An experiment in which each participant is only tested in one condition.
A method of controlling extraneous variables across conditions by using a random process to decide which participants will be tested in the different conditions.
All the conditions of an experiment occur once in the sequence before any of them is repeated.
Any intervention meant to change people’s behaviour for the better.
A condition in a study where participants receive treatment.
A condition in a study that the other condition is compared to. This group does not receive the treatment or intervention that the other conditions do.
A type of experiment to research the effectiveness of psychotherapies and medical treatments.
A type of control condition in which participants receive no treatment.
A simulated treatment that lacks any active ingredient or element that should make it effective.
A positive effect of a treatment that lacks any active ingredient or element to make it effective.
Participants receive a placebo that looks like the treatment but lacks the active ingredient or element thought to be responsible for the treatment’s effectiveness.
Participants are told that they will receive the treatment but must wait until the participants in the treatment condition have already received it.
Each participant is tested under all conditions.
An effect of being tested in one condition on participants’ behaviour in later conditions.
Participants perform a task better in later conditions because they have had a chance to practice it.
Participants perform a task worse in later conditions because they become tired or bored.
Being tested in one condition can also change how participants perceive stimuli or interpret their task in later conditions.
Testing different participants in different orders.
Research Methods in Psychology - 2nd Canadian Edition Copyright © 2015 by Paul C. Price, Rajiv Jhangiani, & I-Chant A. Chiang is licensed under a Creative Commons Attribution-NonCommercial-ShareAlike 4.0 International License , except where otherwise noted.
Share This Book

An official website of the United States government
The .gov means it’s official. Federal government websites often end in .gov or .mil. Before sharing sensitive information, make sure you’re on a federal government site.
The site is secure. The https:// ensures that you are connecting to the official website and that any information you provide is encrypted and transmitted securely.
- Publications
- Account settings
Preview improvements coming to the PMC website in October 2024. Learn More or Try it out now .
- Advanced Search
- Journal List

When randomisation is not good enough: Matching groups in intervention studies
Francesco sella.
1 Centre for Mathematical Cognition, Loughborough University, Loughborough, UK
2 Department of Experimental Psychology, University of Oxford, Oxford, UK
Roi Cohen Kadosh
Associated data.
Randomised assignment of individuals to treatment and controls groups is often considered the gold standard to draw valid conclusions about the efficacy of an intervention. In practice, randomisation can lead to accidental differences due to chance. Researchers have offered alternatives to reduce such differences, but these methods are not used frequently due to the requirement of advanced statistical methods. Here, we recommend a simple assignment procedure based on variance minimisation (VM), which assigns incoming participants automatically to the condition that minimises differences between groups in relevant measures. As an example of its application in the research context, we simulated an intervention study whereby a researcher used the VM procedure on a covariate to assign participants to a control and intervention group rather than controlling for the covariate at the analysis stage. Among other features of the simulated study, such as effect size and sample size, we manipulated the correlation between the matching covariate and the outcome variable and the presence of imbalance between groups in the covariate. Our results highlighted the advantages of VM over prevalent random assignment procedure in terms of reducing the Type I error rate and providing accurate estimates of the effect of the group on the outcome variable. The VM procedure is valuable in situations whereby the intervention to an individual begins before the recruitment of the entire sample size is completed. We provide an Excel spreadsheet, as well as scripts in R, MATLAB, and Python to ease and foster the implementation of the VM procedure.
Supplementary Information
The online version contains supplementary material available at 10.3758/s13423-021-01970-5.
Introduction
Randomisation in controlled trials.
A common problem in intervention studies is comparing the effect of intervention while minimising the influence of confounding factors. In the pre-treatment assessment, a researcher usually measures the characteristics that the treatment aims to modify (i.e., outcome measures) as well as other variables that can exert an influence on the treatment (i.e., covariates). Then, the researcher will randomly assign individuals to the treatment and the control condition. In the ideal scenario, the control condition matches the treatment condition except for that specific feature of the treatment that the researcher considers to be crucial for causing a change in the outcome measures (e.g., placebo vs the active molecule in pharmacological studies). If the treatment is effective, the treatment group should improve in the outcome measures compared to the control group.
In the case of randomisation with large sample size, the statistical test for a difference at baseline or in other covariates becomes irrelevant as occurring significant differences reflect Type I error (de Boer et al., 2015 ; Roberts & Torgerson, 1999 ), which more likely arises when several covariates are considered (Austin et al., 2010 ). However, large sample sizes are difficult to achieve. Many researchers, especially in the clinical sciences, rely on small naturally occurring samples composed of individuals who voluntarily join the study when they wish to. In this scenario, the sampling is suboptimal as participants are not randomly sampled from the population, but they take part in the study based on convenience and opportunity. Although the assignment to different treatment conditions can be random, differences at baseline are more likely to emerge in small compared to large trials (Bruhn & Mckenzie, 2009 ; Chia, 2000 ; Nguyen & Collins, 2017 ; Saint-mont, 2015 ). Unfortunately, there is no statistical way to control for these differences between groups at pre-test (Miller & Chapman, 2001 ; Van Breukelen, 2006 ). Therefore, the imbalance in the pre-treatment scores can compromise the evaluation of the treatment efficacy, and seriously harm the interpretability of the results. To correct for this, the researcher may choose to allocate individuals to a condition based on previously collected pre-treatment scores and match the groups on these scores. However, this procedure requires the researcher to complete the pre-treatment assessment of all participants before the beginning of the treatment. The whole process may take several months, increase the attrition rate before the treatment begins and cannot account for unwanted changes in the measures of interest. Furthermore, the immediate implementation of the treatment is frequently necessary, especially in a clinical setting, where the treatment must begin in a critical phase of the patients’ clinical condition.
Minimising group differences
One solution is the use of covariate-adaptive randomisation procedures (Chen & Lee, 2011 ; Dragalin et al., 2003 ; Endo et al., 2006 ; Scott et al., 2002 ), which allocate participants to the different conditions as they join the study and, at the same time, reduce the difference between groups on predefined critical variables. There are three commonly used types of covariate-adaptive randomisation methods: stratified randomisation, dynamic hierarchical randomisation, and minimisation (Lin et al., 2015 ). Differences at baseline can be reduced by using stratified randomisation, whereby specific (prognostic) variables are divided into strata and participants are randomly selected from each stratum. However, stratified randomisation becomes difficult to implement as the factors to control for increase (Therneau, 1993 ). In dynamic hierarchical randomisation, covariates are ranked in order of importance and participants are assigned to conditions via biased coin allocation when thresholds of imbalance are exceeded in selected covariates (Signorini et al., 1993 ). A minimisation procedure, the focus of this paper, calculates the level of imbalance in covariates that assigning a participant to each condition would cause, then allocates with high probability (to maintain a degree of randomness) the current participant to the condition that minimises the imbalance.
In this vein, the use of covariate-adaptive randomisation procedures not only matches groups on covariates, but also implicitly forces researchers to state in advance those critical covariates related to the treatment rather than controlling for their effect at a later stage, when running statistical analyses (Simmons et al., 2011 ). A covariate-adaptive randomisation procedure attempts to reduce the unwanted differences at baseline that inadvertently emerge from a random assignment. However, it is worth highlighting that the covariate-adaptive randomisation procedures aim to solve the imbalances at pre-test that might emerge from the random assignment of participants, rather than issues related to non-random selection of participants from naturally occurring samples.
Despite a variety of covariate-adaptive randomisation procedures at disposal, researchers conducting training/treatment studies, including randomised control trials (RCTs), seldom implement these methods (Ciolino et al., 2019 ; Lin et al., 2015 ; Taves, 2010 ). The lack of popularity of these procedures might be due to multiple factors. Researchers may feel more comfortable in implementing more traditional and easier to understand stratified/block randomisation. In this vein, an efficient implementation of covariate-adaptive procedures would require the consultancy of an expert statistician for the entire duration of the trial; an extra cost that principal investigators may prefer to avoid (Ciolino et al., 2019 ). Finally, the lack of free, easy-to-use, computerised functions to automatically implement covariate-adaptive procedures may have contributed to their still limited dissemination (Treasure & Farewell, 2012 ; Treasure & MacRae, 1998 ).
Here, we provide a procedure based on variance minimisation (VM; Frane, 1998 ; Pocock & Simon, 1975 ; Scott et al., 2002 ; Treasure & MacRae, 1998 ), which assigns the next incoming participant to the condition that minimises differences between groups in the chosen measures. Our procedure brings the benefit of using multiple covariates without creating strata in advance, as done in the stratified randomisation, and it is relatively easy to implement compared with the more complex dynamic hierarchical randomisation. The logic and the calculation behind the procedure are simple and easy-to-grasp also from an audience of non-experts. We provided ready-to-use code to implement the procedure using different (also free) software along with step-by-step written instructions, thereby reducing any costs associated with product licenses or consultancy from expert statisticians.
Description of the VM procedure
The goal of the VM procedure is to find the best group assignment for participants prior to an intervention, such that the groups are matched in terms of the scores that the researcher suspects might cause random differences in post-intervention outcomes. The VM procedure requires the researcher to define the number of groups to which participants can be assigned and to collect individual scores for each variable on which groups are matched. These variables can be continuous or binary, where nominal variables with more than two categories can be transformed into multiple dummy variables (as in regression analysis) before being passed to the VM procedure (see section Using VM Procedure on Non-Dichotomous Nominal Variables, in the Supplementary Materials ). The procedure particularly suits those studies in which proper matching is essential, but the assignment to groups needs to occur while the recruitment is still ongoing. It works as follows.
The first participants joining the study are sequentially assigned one to each group. For example, in case of three different groups (i.e., A, B, C), the first participant is assigned to Group A, the second participant to Group B, and the third participant to Group C. Then the fourth participant is added temporarily to each group, and for each temporary group assignment, the algorithm checks which group assignment for this participant would minimize the between-group variance (i.e., V in Fig. Fig.1) 1 ) of the measures of interest and assigns the participant to that group. The next (fifth) participant undergoes the same procedure, but the algorithm will not assign the present participant to the group of the previous participant in order to ensure a balanced distribution of participants in each condition. The same procedure goes on until there is only one group remaining, which in the case of three groups would be for the sixth participant. The sixth participant would be automatically assigned to the remaining group, such that each group would now have two participants assigned to them. Then, the entire procedure starts again with the possibility for the next participant to be assigned to all available groups (for a formal description of the variance minimisation procedure, see section Details of the Minimisation Procedure, in the Supplementary Materials ).

Comparison of assignment to groups using ( a ) variance minimisation and ( b ) random assignment. When a new participant joins a study, variance minimisation assigns the participant to the group that minimises the variance between groups along with the pre-defined variables (i.e., V ); in this case intelligence (IQ), executive functions (EFs), attentional performance (AP), and gender, while keeping the number of participants in each group balanced. Random assignment, on the other hand, assigns the participant to every group with equal probability and does not match the groups
To avoid predictable group assignments due to this shrinking set of available groups, the user can also specify a small probability of random assignment over the VM procedure (see section Discontinuous Implementation of the VM Procedure: The Parameter pRand, in the Supplementary Materials ). This random component makes the assignment unpredictable even if the researcher has access to previous group allocations.
Simulations
We present multiple simulations to illustrate how the VM procedure can be implemented in different scenarios and the advantages it provides.
In the first simulation, we implemented the VM procedure to assign participants to three experimental groups based on three continuous and one dichotomous variable. We compared the matching obtained from the VM procedure with random assignment. In the second simulation, we showed that the VM procedure better detects group differences and provides better estimates of effects compared with the attempt to control for the effect of covariates. In the supplementary materials , we demonstrate how to incorporate a random component in the VM procedure to ensure a non-deterministic assignment of participants to conditions (section Discontinuous Implementation of the VM Procedure: The Parameter pRand ) and how the VM can match participants also on non-dichotomous nominal variables (section Using VM Procedure on Non-Dichotomous Nominal Variables ). We briefly discuss the results of these two additional simulations in the Discussion section.
The functions to implement the VM procedure in Excel, MATLAB, Python, and R along with tutorials, as well as the R code of the simulation, can be found at the Open Science Framework ( https://osf.io/6jfvk/?view_only=8d405f7b794d4e3bbff7e345e6ef4eed ).
VM procedure outperforms random assignment in matching groups on continuous and dichotomous variables
In the first fictional example, a researcher wants to evaluate whether the combination of cognitive training of executive functions and brain stimulation improves the clinical symptoms of ADHD. The study design comprises three groups: the first group receives brain stimulation and the executive functions training; the second group receives sham stimulation and the training; the third group receives neither training nor stimulation (passive control group). The researcher aims to match the three groups on intelligence, executive functions performance, attentional performance, and gender. Figure Figure1 1 illustrates how VM assigns incoming participants compared with a traditional random assignment.
We simulated 1,000 data sets whereby we randomly drew the scores for IQ, executive functions, and attentional performance from a normal distribution, with a mean of 100 and a standard deviation of 15. Participants’ gender came from a binomial distribution with the same probability for a participant to be male or female. The simulated values for the matching variables were randomly generated, therefore there were no real differences between groups. We varied the sample size to be very small ( n = 36), small ( n = 66), medium ( n = 159), and large ( n = 969), reflecting the researcher’s intention to evaluate the possible presence of an extremely large ( f = 0.55), large ( f = 0.40), medium ( f = 0.25), and small ( f = 0.10) effect size, respectively, while keeping the alpha at .05 and power at 80% (Faul et al., 2009 ). We assigned participants to the three groups randomly or by using the VM procedure.
We ran univariate analyses of variance (ANOVAs) with IQ, executive functions, and attentional performance as dependent variables and group as factor whereas differences in gender distribution across groups were analysed using χ 2 tests. In Fig. Fig.2, 2 , we show the distributions of F , p , and η 2 values from ANOVAs on IQ, executive functions, and attentional performance (top panel), whereas in the case of gender, we presented the distribution and χ 2 , p , and Cramer’s V values (bottom panel) separately for the random assignment and the VM procedure across different sample sizes. Compared with random assignment, the VM procedure yielded smaller F , η 2 , χ 2 , and Cramer’s V values and the distribution of p -values was skewed toward 1, rather than uniform. The VM procedure demonstrated an efficient matching between groups starting from a very small sample size while keeping the number of participants in each group balanced. Moreover, both the VM procedure and the random assignment violated ANOVA assumptions on the normality of residuals and homogeneity of variance between groups with a similar rate (see Supplementary Materials, Fig. S1 ).

A comparison of the VM procedure and random assignment based on simulated data. Top panel: Distributions of F -values, p -values, and η 2 values from ANOVAs comparing groups on intelligence (IQ), executive functions (EFs), and attentional performance (AP) separately for the VM procedure (orange boxplots) and the random assignment (blue boxplots). Bottom panel: Distributions of χ 2 , p -values, and Cramer’s V values comparing groups on gender separately for the VM procedure (orange boxplots) and the random assignment (blue boxplots). The boxplots represent the quartiles whereas the whiskers represent the 95% limits of the distribution. (Colour figure online)
Matching groups on a covariate versus controlling for a covariate with imbalance
We simulated an intervention study to display the advantages that the minimisation procedure provides in terms of detecting group differences and better estimates of effects compared with the attempt to control for the effect of covariates in the statistical analysis after the intervention was completed. A researcher evaluates the effect of an intervention on a dependent variable Y while controlling for the possible confounding effect of a covariate A, which positively correlates with Y, and a covariate B that correlates with covariate A (i.e., pattern correlation 1), or Y (i.e., pattern correlation 2), or neither of them (i.e., pattern correlation 3). In this vein, the covariate A represents a variable that the researchers ought to control for, given its known relation with the dependent variable Y, whereas the covariate B represents a non-matching variable that is still inserted into the model as it might have a real or spurious correlation with the covariate A and the dependent variable Y. We simulated a small, medium, and large effect of the intervention (i.e., Cohen’s d = 0.2; d = 0.5; d = 0.8) and, accordingly, we varied the total sample size to be 788, 128, and 52 to achieve a power of 80% while keeping the alpha at .05 (Faul et al., 2009 ). For comparison, we used the same sample sizes, 788, 128, and 52, when simulating the absence of an intervention effect (i.e., Cohen’s d = 0). Crucially, we compared the scenario whereby the researcher matches participants on the covariate A (i.e., VM on CovA) before implementing the intervention or randomly assigns participants to the control and training group and then attempts to control for the effect of covariate after the intervention (i.e., Control for CovA). The subsequent inclusion of the covariate A in the analysis, especially in the case of imbalance between groups in the covariate A, would bias the effect of the group on Y when the difference between groups in the covariate A is larger in the direction of the intervention effect. Conversely, the minimisation procedure reduces the difference between groups on the covariate A and the inclusion of the covariate A into the analysis (i.e., analysis of covariance; ANCOVA) would not cause biases in the estimation of the effect of the group on Y.
In the case of the control for covariate approach, we generated the scores of the covariate A by taking them from a standard normal distribution ( M = 0, SD = 1) and we randomly assigned participants to the control and training group. We generated an imbalance in the covariate A by calculating the standard error of the mean and multiplying it for the standard normal deviates ±1.28, ±1.64, ±1.96 corresponding to the 20%, 10%, and 5% probabilities respectively of the standard normal distribution. The use of the standard error allowed to keep the imbalance proportionate to the sample size. The obtained imbalance was added to the scores of the covariate A only for the training group, thereby generating a difference in covariate A that went in the same or in the opposite direction with respect to the intervention effect (i.e., larger scores on the dependent variable only for the training group; Egbewale et al., 2014 ). We also included the case of absent imbalance for reference. In the case of the VM procedure, we took the previously generated scores of the covariate A with the imbalance, and we assigned participants to the control or training group using the VM procedure. Then, we generated the scores of Y that were correlated with the covariate A according to four correlations, that were, 0, 0.5, 0.7, and 0.9. Finally, we added 0, 0.2, 0.5, 0.8 to the Y scores of the training group to simulate an absent, small, medium, and large effect of the intervention.
In both the random assignment and the VM procedure, the covariate B was generated to alternatively have a correlation of 0.5 ( SD = 0.1) with the covariate A (i.e., Pattern 1), Y (i.e., Pattern 2), or no correlation with these two variables (i.e., Pattern 3). We randomly selected the correlation from a normal distribution with an average 0.5 and standard deviation of 0.1 to add some noise to the correlation while maintaining it positive and centred on 0.5.
Overall, we varied multiple experimental conditions in 504 scenarios (for a similar approach, see Egbewale et al., 2014 ):
- seven imbalances on the covariate A: −1.96, −1.64, −1.28, 0, 1.28, 1.64, 1.96;
- four correlations between covariates A and Y: 0, 0.5, 0.7, 0.9;
- six treatment effects: 0 (×3 as the absence of the effect was tested with three sample sizes, that were, 52, 128, 788), 0.2, 0.5, 0.8;
- three patterns of correlation between the covariate B, covariate A, and Y.
We simulated each scenario 1,000 times.
As expected, the correlations between the covariate B and the other two variables varied according to the pre-specified patterns of correlations, which were practically identical in the VM and control for covariate approach (see Table S1 in the Supplementary Materials).
We ran a series of ANCOVAs with Y as the dependent variable, the covariates A and B, and group [Training, Control] as independent variables. We used a regression approach as the variable group was converted to a dichotomous numerical variable (i.e., control = 0, training = 1) to directly use the regression coefficients as estimates for the effect of each variable on Y. Both the VM procedure and the control for the covariate approach display a similar rate in violating ANCOVA assumptions of the normality of residuals and homogeneity of variance between groups (see Supplementary Materials; Fig. S2 ).
In this fictitious scenario, the researcher would be interested in evaluating the effect of the group on Y while controlling for covariates. Therefore, we reported the proportion of significant results ( p < .05; Fig. Fig.3) 3 ) and the estimated effect (i.e., coefficient of the regression; Fig. Fig.4) 4 ) for the effect of group on Y depending on the imbalance in the covariate A, the effect size of the intervention, and the degree of correlation between the covariate A and Y. For simplicity, in Figs. Figs.3 3 and and4, 4 , we reported only the simulation with a large sample size (i.e., n = 788) when the effect of the intervention was absent (i.e., d =0). The pattern of results remained stable across the patterns of correlations of the covariate B. Therefore, we reported the proportion of significant results and estimated effects for the group, covariate A, and covariate B across the patterns correlation of the covariate B in the Supplementary Materials (Figs. S5 – S22 ).

Proportion of significant results ( y -axis) for the effect of group in the ANCOVA (Y ~ CovA + CovB + Group) separately for the VM procedure (orange lines) and control for CovA approach (blue lines) across imbalances of the covariate A ( x -axis) when the sample size varied according to the effect size to be detected (rows; absent = 0, n = 788; small = 0.2, n = 788; medium = 0.5, n = 128; large = 0.8, n = 52) and the correlation between the covariate A and the dependent variable Y ranged between 0 and 0.9 (columns). The black dotted line represents alpha (i.e., 0.05) and the dashed black line represents the expected power (i.e., 0.8). (Colour figure online)

Median of estimates ( y -axis; regression coefficients) for the effect of group in the ANCOVA (Y ~ CovA + CovB + Group) separately for the VM procedure (orange lines) and control for CovA approach (blue lines) across imbalances of the covariate A ( x -axis) when the sample size varied according to the effect size to be detected (rows; absent = 0, n = 788; small = 0.2, n = 788; medium = 0.5, n = 128; large = 0.8, n = 52) and the correlation between the covariate A and the dependent variable Y ranged between 0 and 0.9 (columns). The black dotted line represents the expected regression coefficients (i.e., 0, 0.2, 0.5, 0.8). (Colour figure online)
When the effect of the intervention was present (second to fourth rows in Fig. Fig.3), 3 ), the VM procedure showed a more stable detection of significant results also in the presence of serious imbalances in the covariate A. This stability became clearer as the correlation between the covariate A and Y increased. When the effect of the intervention was absent (first row in Fig. Fig.3), 3 ), the VM procedure always kept the Type I error around 0.05 while the control covariate approach inflated Type I error rate in the case of strong imbalance in the covariate A when it was highly correlated (i.e., 0.7, 0.9) with the outcome variable Y.
A similar pattern of results emerged when we compared the estimates of the effect of the group (i.e., regression coefficients) yielded by the VM procedure and the control for covariate approach. The VM procedure always provided accurate estimates of the effect of the group. Conversely, the control for covariate approach returned biased estimates with large imbalances in the covariate A and when its correlation with the outcome variable Y was high (i.e., 0.7, 0.9; Fig. Fig.4 4 ).
In treatment studies, groups should be as similar as possible in all the variables of interest before the beginning of the treatment. An optimal matching can ensure that the effect of the treatment is not related to the pre-treatment characteristics of the groups and can, therefore, be extended to the general population. In contrast, the random assignment can yield relevant, and even statistically significant, differences between the groups before the treatment (Treasure & MacRae, 1998 ).
The proposed VM procedure constitutes a quick and useful tool to match groups before treatment on both continuous and categorical covariates (Pocock & Simon, 1975 ; Scott et al., 2002 ; Treasure & MacRae, 1998 ). The latter, though, need to be transformed into dummy variables to be passed to the minimisation algorithm (for a minimisation procedure that directly handles nominal covariates see Colavincenzo, 2013 ). We simulated an intervention study whereby a researcher used the VM procedure on a covariate to assign participants to a control and intervention group rather than controlling for the covariate at the analysis stage. Among other features of the simulated study, we manipulated the correlation between the matching covariate and the outcome variable and the presence of imbalance between groups in the covariate. Controlling for covariates post hoc inflated Type I error rate and yielded biased estimates of the effect of the group on the outcome variable when the imbalance between groups in the covariate increased and the correlation between the covariate and the outcome variable was high. Conversely, the use of VM on the covariate did not inflate Type I error rate and provided accurate estimates of the effect of the group on the outcome variable.
The progressive shrinking of available conditions when using the VM procedure ensures a perfect balance in the number of participants across conditions while still minimising covariate imbalance. However, some participants will be forcefully assigned to a given condition irrespective of their scores in the covariates. Therefore, in some instances, the researcher will know in advance the condition the participants will be assigned to and not all participants will have the chance to be assigned to each of the available conditions. This restriction might be relevant for clinical trials where one of the conditions is potentially beneficial (i.e., the treatment group). In this case, the researcher can insert a random component into the VM procedure by defining the probability to implement a random assignment. The random component prevents the researcher from being sure about the condition some participants will be assigned to and gives all participants the possibility, in principle, to be assigned to one of the conditions. Using a small amount of randomness (e.g., pRand = 0.1) provides a good balance between matching groups on covariates while avoiding predictable allocation (see section Discontinuous Implementation of the VM Procedure: The Parameter pRand, in the Supplementary Materials ).
Despite the benefits of the minimisation procedure, limitations must be carefully considered. First, the application of the VM procedure on small sample sizes does not prevent the treatment effect from being influenced by the unequal distribution of unobserved confounding variables, whose equal distribution is most likely achieved with large sample sizes. This limitation related to small sample sizes affects both the VM procedure and random assignment. Nevertheless, the selection of matching covariates for the minimisation procedure encourages researchers to carefully think in advance about possible confounding variables and match participants on them. Secondly, we showed that the VM is beneficial in simple ANOVA/ANCOVA simulations. In the case of more complex models (e.g., with an interaction), the researcher should carefully consider whether the minimisation procedure constitutes an advantage to the design. We recommend running simulations tailored to specific research designs to ensure that the VM procedure adequately matches participants across conditions.
Third, the minimisation procedure considers all covariates equally important without giving the user the possibility to allow more imbalance in some covariates compared to others (for a minimisation procedure that allows weighting see Saghaei, 2011 ). It is therefore paramount that the researchers will carefully consider the covariates they wish to match the groups on.
Overall, our minimisation procedure, even after considering the above-mentioned limitations, provides important advantages over the randomisation procedure that is used frequently. Its relative simplicity encourages researchers to use covariate-adaptive matching procedures (Ciolino et al., 2019 ; Lin et al., 2015 ). To allow the requested shift from the randomisation procedure, we provide scripts, written using popular software (i.e., R, Python, MATLAB, and Excel), which allow a fast and easy implementation of the VM procedure and integration with other stimulus presentation and analysis scripts. In this light, the treatment can start in the same session in which pre-treatment measures are acquired, thereby reducing the total number of sessions and, consequently, the overall costs. The immediate application of the treatment also excludes the possibility that pre-treatment measures change between the period of the initial recruitment and the actual implementation of the treatment. We strongly recommend using the VM procedure in these studies to yield more effective and valid RCTs.
(DOCX 2855 kb)
Acknowledgements
This study was supported by the European Research Council (Learning&Achievement 338065).
Open practices statement
The R code of the analyses is available at https://osf.io/6jfvk/?view_only=8d405f7b794d4e3bbff7e345e6ef4eed
Publisher’s note
Springer Nature remains neutral with regard to jurisdictional claims in published maps and institutional affiliations.
- Austin PC, Manca A, Zwarenstein M, Juurlink DN, Stanbrook MB. Baseline comparisons in randomized controlled trials. Journal of Clinical Epidemiology. 2010; 63 (8):940–942. doi: 10.1016/j.jclinepi.2010.03.009. [ PubMed ] [ CrossRef ] [ Google Scholar ]
- Bruhn M, Mckenzie D. In pursuit of balance: Randomization in practice in development field experiments. American Economic Journal: Applied Economics. 2009; 4 (1):200–232. [ Google Scholar ]
- Chen LH, Lee WC. Two-way minimization: A novel treatment allocation method for small trials. PLOS ONE. 2011; 6 (12):1–8. doi: 10.1371/journal.pone.0028604. [ PMC free article ] [ PubMed ] [ CrossRef ] [ Google Scholar ]
- Chia KS. Randomisation: Magical cure for bias? Annals of the Academy of Medicine . Singapore. 2000; 29 (5):563–564. [ PubMed ] [ Google Scholar ]
- Ciolino, J. D., Palac, H. L., Yang, A., Vaca, M., & Belli, H. M. (2019). Ideal vs. real: A systematic review on handling covariates in randomized controlled trials. BMC Medical Research Methodology, 19 (1), 136. 10.1186/s12874-019-0787-8 [ PMC free article ] [ PubMed ]
- Colavincenzo, J. (2013). Doctoring your clinical trial with adaptive randomization: SAS® Macros to perform adaptive randomization. Proceedings of the SAS® Global Forum 2013 Conference [Internet]. Cary (NC): SAS Institute Inc. https://support.sas.com/resources/papers/proceedings13/181-2013.pdf
- de Boer MR, Waterlander WE, Kuijper LDJ, Steenhuis IHM, Twisk JWR. Testing for baseline differences in randomized controlled trials: An unhealthy research behavior that is hard to eradicate. International Journal of Behavioral Nutrition and Physical Activity. 2015; 12 (1):1–8. doi: 10.1186/s12966-015-0162-z. [ PMC free article ] [ PubMed ] [ CrossRef ] [ Google Scholar ]
- Dragalin V, Fedorov V, Patterson S, Jones B. Kullback-Leibler divergence for evaluating bioequivalence. Statistics in Medicine. 2003; 22 (6):913–930. doi: 10.1002/sim.1451. [ PubMed ] [ CrossRef ] [ Google Scholar ]
- Egbewale, B. E., Lewis, M. & Sim, J. (2014). Bias, precision and statistical power of analysis of covariance in the analysis of randomized trials with baseline imbalance: a simulation study. BMC Med Res Methodol, 14 , 49. 10.1186/1471-2288-14-49 [ PMC free article ] [ PubMed ]
- Endo A, Nagatani F, Hamada C, Yoshimura I. Minimization method for balancing continuous prognostic variables between treatment and control groups using Kullback-Leibler divergence. Contemporary Clinical Trials. 2006; 27 (5):420–431. doi: 10.1016/j.cct.2006.05.002. [ PubMed ] [ CrossRef ] [ Google Scholar ]
- Faul F, Erdfelder E, Buchner A, Lang A-G. Statistical power analyses using G*Power 3.1: tests for correlation and regression analyses. Behavior Research Methods. 2009; 41 (4):1149–1160. doi: 10.3758/BRM.41.4.1149. [ PubMed ] [ CrossRef ] [ Google Scholar ]
- Frane JW. A method of biased coin randomisation, its implementation and its validation. Drug Information Journal. 1998; 32 :423–432. doi: 10.1177/009286159803200213. [ CrossRef ] [ Google Scholar ]
- Lin Y, Zhu M, Su Z. The pursuit of balance: An overview of covariate-adaptive randomization techniques in clinical trials. Contemporary Clinical Trials. 2015; 45 :21–25. doi: 10.1016/j.cct.2015.07.011. [ PubMed ] [ CrossRef ] [ Google Scholar ]
- Miller GA, Chapman JP. Misunderstanding analysis of covariance. Journal of Abnormal Psychology. 2001; 110 (1):40–48. doi: 10.1037/0021-843X.110.1.40. [ PubMed ] [ CrossRef ] [ Google Scholar ]
- Nguyen, T., & Collins, G. S. (2017). Simple randomization did not protect against bias in smaller trials, 84 , 105–113. 10.1016/j.jclinepi.2017.02.010 [ PubMed ]
- Pocock SJ, Simon R. Sequential treatment assignment with balancing for prognostic factors in the controlled clinical trial. Biometrics. 1975; 31 (1):103. doi: 10.2307/2529712. [ PubMed ] [ CrossRef ] [ Google Scholar ]
- Roberts C, Torgerson DJ. Understanding controlled trials: Baseline imbalance in randomised controlled trials. BMJ. 1999; 319 (7203):185–185. doi: 10.1136/bmj.319.7203.185. [ PMC free article ] [ PubMed ] [ CrossRef ] [ Google Scholar ]
- Saghaei M. An overview of randomization and minimization programs for randomized clinical trials. Journal of Medical Signals and Sensors. 2011; 1 (1):55. doi: 10.4103/2228-7477.83520. [ PMC free article ] [ PubMed ] [ CrossRef ] [ Google Scholar ]
- Saint-mont, U. (2015). Randomization does not help much, comparability does. PLOS ONE, 10 (7), Article e0132102. 10.1371/journal.pone.0132102 [ PMC free article ] [ PubMed ]
- Scott NW, McPherson GC, Ramsay CR, Campbell MK. The method of minimization for allocation to clinical trials: A review. Controlled Clinical Trials. 2002; 23 (6):662–674. doi: 10.1016/S0197-2456(02)00242-8. [ PubMed ] [ CrossRef ] [ Google Scholar ]
- Signorini DF, Leung O, Simes RJ, Beller E, Gebski VJ, Callaghan T. Dynamic balanced randomization for clinical trials. Statistics in Medicine. 1993; 12 (24):2343–2350. doi: 10.1002/sim.4780122410. [ PubMed ] [ CrossRef ] [ Google Scholar ]
- Simmons JP, Nelson LD, Simonsohn U. False-positive psychology: Undisclosed flexibility in data collection and analysis allows presenting anything as significant. Psychological Science. 2011; 22 (11):1359–1366. doi: 10.1177/0956797611417632. [ PubMed ] [ CrossRef ] [ Google Scholar ]
- Taves DR. The use of minimization in clinical trials. Contemporary Clinical Trials. 2010; 31 (2):180–184. doi: 10.1016/j.cct.2009.12.005. [ PubMed ] [ CrossRef ] [ Google Scholar ]
- Therneau TM. How many stratification factors are “too many” to use in a randomization plan? Controlled Clinical Trials. 1993; 14 (2):98–108. doi: 10.1016/0197-2456(93)90013-4. [ PubMed ] [ CrossRef ] [ Google Scholar ]
- Treasure T, Farewell V. Minimization in interventional trials : great value but residual vulnerability. Journal of Clinical Epidemiology. 2012; 65 (1):7–9. doi: 10.1016/j.jclinepi.2011.07.005. [ PubMed ] [ CrossRef ] [ Google Scholar ]
- Treasure T, MacRae KD. Minimisation: The platinum standard for trials? BMJ (Clinical Research Ed.) 1998; 317 (7155):362–363. doi: 10.1136/bmj.317.7155.362. [ PMC free article ] [ PubMed ] [ CrossRef ] [ Google Scholar ]
- Van Breukelen GJP. ANCOVA versus change from baseline had more power in randomized studies and more bias in nonrandomized studies. Journal of Clinical Epidemiology. 2006; 59 (9):920–925. doi: 10.1016/j.jclinepi.2006.02.007. [ PubMed ] [ CrossRef ] [ Google Scholar ]
Skip to main navigation
- Email Updates
- Federal Court Finder
Conference Acts to Promote Random Case Assignment
Published on March 12, 2024
The Judicial Conference of the United States has strengthened the policy governing random case assignment, limiting the ability of litigants to effectively choose judges in certain cases by where they file a lawsuit.
The policy addresses all civil actions that seek to bar or mandate state or federal actions, “whether by declaratory judgment and/or any form of injunctive relief.” In such cases, judges would be assigned through a district-wide random selection process.
“Since 1995, the Judicial Conference has strongly supported the random assignment of cases and the notion that all district judges remain generalists,” said Judge Robert J. Conrad, Jr., secretary of the Conference. “The random case-assignment policy deters judge-shopping and the assignment of cases based on the perceived merits or abilities of a particular judge. It promotes the impartiality of proceedings and bolsters public confidence in the federal Judiciary.”
In most of the nation’s 94 federal district courts, local case assignment plans facilitate the random selection of judges. Some plans assign cases to a judge in the division of the court where the case is filed. In divisions where only a single judge sits, these rules have made it possible for a litigant to pre-select that judge by filing in that division.
In a November 2021 letter, Senator Thom Tillis (R-N.C.), and Patrick Leahy, a Vermont senator who since has retired, raised concerns about a concentration of patent cases filed in single-judge divisions.
Chief Justice John G. Roberts, Jr., referenced this letter in his 2021 Year-End Report on the Federal Judiciary , calling for a study of judicial assignment practices in patent cases.
“Senators from both sides of the aisle have expressed concern that case assignment procedures … might, in effect, enable the plaintiff to select a particular judge to hear a case,” Roberts said. During the patent-case study, the Court Administration and Case Management Committee (CACM) determined that similar issues might occur in bankruptcy and other types of civil litigation. Public debate grew when several highly controversial lawsuits, seeking nationwide injunctions against federal government policies, were filed in single-judge court divisions.
In submitting the proposed policy to the Judicial Conference, the CACM Committee said that some local case assignment plans risked creating an appearance of “judge shopping.” The committee also noted that the value of trying a civil case in the nearest court division becomes less important when the impact of a ruling might be felt statewide or even nationally.
The amended policy applies to cases involving state or federal laws, rules, regulations, policies, or executive branch orders. District courts may continue to assign cases to a single-judge division when they do not seek to bar or mandate state or federal actions, whether by declaratory judgment and/or any form of injunctive relief.
In addition to the Judiciary policy, the CACM committee will disseminate guidance to all district courts regarding civil case assignment.
The 26-member Judicial Conference is the policy-making body for the federal court system. By statute, the Chief Justice of the United States serves as its presiding officer and its members are the chief judges of the 13 courts of appeals, a district judge from each of the 12 geographic circuits, and the chief judge of the Court of International Trade.
The Conference convenes twice a year to consider administrative and policy issues affecting the court system.
Related Topics: Judicial Conference of the United States
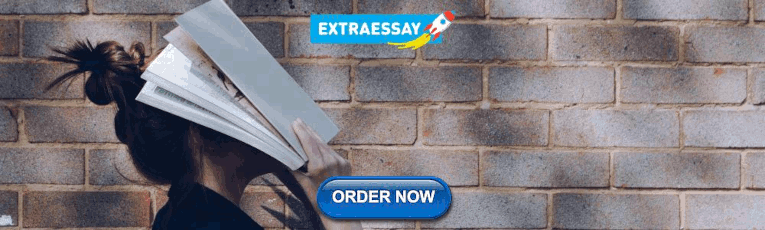
IMAGES
VIDEO
COMMENTS
Random sampling and random assignment are both important concepts in research, but it's important to understand the difference between them. Random sampling (also called probability sampling or random selection) is a way of selecting members of a population to be included in your study.
Random assignment helps you separation causation from correlation and rule out confounding variables. As a critical component of the scientific method, experiments typically set up contrasts between a control group and one or more treatment groups. The idea is to determine whether the effect, which is the difference between a treatment group ...
Random selection (also called probability sampling or random sampling) is a way of randomly selecting members of a population to be included in your study. On the other hand, random assignment is a way of sorting the sample participants into control and treatment groups. Random selection ensures that everyone in the population has an equal ...
Random assignment is essential because it increases the likelihood that the groups are the same at the outset. With all characteristics being equal between groups, other than the application of the independent variable, any differences found between group outcomes can be more confidently attributed to the effect of the intervention.
One is that random assignment works better than one might expect, especially for large samples. Another is that the inferential statistics that researchers use to decide whether a difference between groups reflects a difference in the population take the "fallibility" of random assignment into account.
Random assignment or random placement is an experimental technique for assigning human participants or animal subjects to different groups in an experiment (e.g., a treatment group versus a control group) using randomization, such as by a chance procedure (e.g., flipping a coin) or a random number generator. This ensures that each participant or subject has an equal chance of being placed in ...
Random sampling vs. random assignment (scope of inference) Hilary wants to determine if any relationship exists between Vitamin D and blood pressure. She is considering using one of a few different designs for her study. Determine what type of conclusions can be drawn from each study design.
Random assignment is a method for assigning participants in a sample to the different conditions, and it is an important element of all experimental research in psychology and other fields too. In its strictest sense, random assignment should meet two criteria. One is that each participant has an equal chance of being assigned to each condition ...
One is that random assignment works better than one might expect, especially for large samples. Another is that the inferential statistics that researchers use to decide whether a difference between groups reflects a difference in the population take the "fallibility" of random assignment into account.
A hidden hero in this adventure of discovery is a method called random assignment, a cornerstone in psychological research that helps scientists uncover the truths about the human mind and behavior. ... This similarity between groups allows researchers to attribute any differences observed in the outcomes directly to the independent variable ...
4. Further details and considerations for the use of Difference-in-Differences 4.1 Using control variables for a more robust identification. With a non-random assignment to treatment, there is always the concern that the treatment states would have followed a different trend than the control states, even absent the reform.
One outstanding question is whether "random" individual differences in the causal effect (i.e. individual differences that may not be accounted for by measured covariates) can be estimated from the data produced from the simple between-subjects design, and if so, under what assumptions. ... First, random assignment, the sine qua non of ...
Random selection, or random sampling, is a way of selecting members of a population for your study's sample. In contrast, random assignment is a way of sorting the sample into control and experimental groups. Random sampling enhances the external validity or generalizability of your results, while random assignment improves the internal ...
Random selection and random assignment are two techniques in statistics that are commonly used, but are commonly confused. Random selection refers to the process of randomly selecting individuals from a population to be involved in a study. Random assignment refers to the process of randomly assigning the individuals in a study to either a ...
Random sampling allows us to obtain a sample representative of the population. Therefore, results of the study can be generalized to the population. Random assignment allows us to make sure that the only difference between the various treatment groups is what we are studying. For example, in the serif/sans serif example, random assignment helps ...
Random assignment is a fundamental part of a "true" experiment because it helps ensure that any differences found between the groups are attributable to the treatment, rather than a confounding variable. So, to summarize, random sampling refers to how you select individuals from the population to participate in your study.
Random assignment is a method for assigning participants in a sample to the different conditions, and it is an important element of all experimental research in psychology and other fields too. In its strictest sense, random assignment should meet two criteria. One is that each participant has an equal chance of being assigned to each condition ...
Difference between Random Selection and Random Assignment. Random selection and random assignment are commonly confused or used interchangeably, though the terms refer to entirely different processes. Random selection refers to how sample members (study participants) are selected from the population for inclusion in the study.
Comparison of assignment to groups using (a) variance minimisation and (b) random assignment.When a new participant joins a study, variance minimisation assigns the participant to the group that minimises the variance between groups along with the pre-defined variables (i.e., V); in this case intelligence (IQ), executive functions (EFs), attentional performance (AP), and gender, while keeping ...
1. I have a question about Difference-in-Difference estimation. It is well-known that the key identification assumption in Diff-in-Diff is the parallel trends assumption that says that in the absence of treatment, the evolution of the outcomes in the treated group would move in parallel to the control group. Of course this is a counterfactual ...
Random assignment in psychology involves each participant having an equal chance of being chosen for any of the groups, including the control and experimental groups. It helps control for potential confounding variables, reducing the likelihood of pre-existing differences between groups. This method enhances the internal validity of experiments ...
Random assignment can be thought of as equivalent to stage 1 and the randomization inference procedure as equivalent to stage 2. Before treatment is assigned, the P values from the experiment (across all possible treatment assignments) are uniformly distributed between 0 and 1.
The Judicial Conference of the United States has strengthened the policy governing random case assignment, limiting the ability of litigants to effectively choose judges in certain cases by where they file a lawsuit. The policy addresses all civil actions that seek to bar or mandate state or federal actions, "whether by declaratory judgment ...