Random Assignment in Psychology: Definition & Examples
Julia Simkus
Editor at Simply Psychology
BA (Hons) Psychology, Princeton University
Julia Simkus is a graduate of Princeton University with a Bachelor of Arts in Psychology. She is currently studying for a Master's Degree in Counseling for Mental Health and Wellness in September 2023. Julia's research has been published in peer reviewed journals.
Learn about our Editorial Process
Saul Mcleod, PhD
Editor-in-Chief for Simply Psychology
BSc (Hons) Psychology, MRes, PhD, University of Manchester
Saul Mcleod, PhD., is a qualified psychology teacher with over 18 years of experience in further and higher education. He has been published in peer-reviewed journals, including the Journal of Clinical Psychology.
Olivia Guy-Evans, MSc
Associate Editor for Simply Psychology
BSc (Hons) Psychology, MSc Psychology of Education
Olivia Guy-Evans is a writer and associate editor for Simply Psychology. She has previously worked in healthcare and educational sectors.
In psychology, random assignment refers to the practice of allocating participants to different experimental groups in a study in a completely unbiased way, ensuring each participant has an equal chance of being assigned to any group.
In experimental research, random assignment, or random placement, organizes participants from your sample into different groups using randomization.
Random assignment uses chance procedures to ensure that each participant has an equal opportunity of being assigned to either a control or experimental group.
The control group does not receive the treatment in question, whereas the experimental group does receive the treatment.
When using random assignment, neither the researcher nor the participant can choose the group to which the participant is assigned. This ensures that any differences between and within the groups are not systematic at the onset of the study.
In a study to test the success of a weight-loss program, investigators randomly assigned a pool of participants to one of two groups.
Group A participants participated in the weight-loss program for 10 weeks and took a class where they learned about the benefits of healthy eating and exercise.
Group B participants read a 200-page book that explains the benefits of weight loss. The investigator randomly assigned participants to one of the two groups.
The researchers found that those who participated in the program and took the class were more likely to lose weight than those in the other group that received only the book.
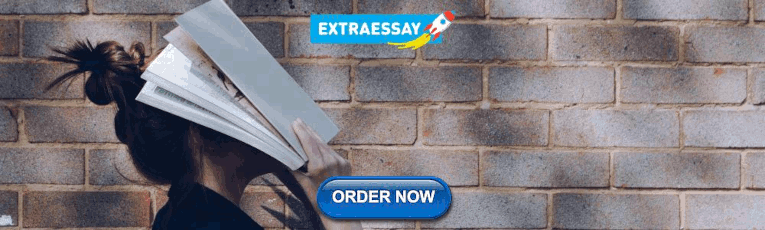
Importance
Random assignment ensures that each group in the experiment is identical before applying the independent variable.
In experiments , researchers will manipulate an independent variable to assess its effect on a dependent variable, while controlling for other variables. Random assignment increases the likelihood that the treatment groups are the same at the onset of a study.
Thus, any changes that result from the independent variable can be assumed to be a result of the treatment of interest. This is particularly important for eliminating sources of bias and strengthening the internal validity of an experiment.
Random assignment is the best method for inferring a causal relationship between a treatment and an outcome.
Random Selection vs. Random Assignment
Random selection (also called probability sampling or random sampling) is a way of randomly selecting members of a population to be included in your study.
On the other hand, random assignment is a way of sorting the sample participants into control and treatment groups.
Random selection ensures that everyone in the population has an equal chance of being selected for the study. Once the pool of participants has been chosen, experimenters use random assignment to assign participants into groups.
Random assignment is only used in between-subjects experimental designs, while random selection can be used in a variety of study designs.
Random Assignment vs Random Sampling
Random sampling refers to selecting participants from a population so that each individual has an equal chance of being chosen. This method enhances the representativeness of the sample.
Random assignment, on the other hand, is used in experimental designs once participants are selected. It involves allocating these participants to different experimental groups or conditions randomly.
This helps ensure that any differences in results across groups are due to manipulating the independent variable, not preexisting differences among participants.
When to Use Random Assignment
Random assignment is used in experiments with a between-groups or independent measures design.
In these research designs, researchers will manipulate an independent variable to assess its effect on a dependent variable, while controlling for other variables.
There is usually a control group and one or more experimental groups. Random assignment helps ensure that the groups are comparable at the onset of the study.
How to Use Random Assignment
There are a variety of ways to assign participants into study groups randomly. Here are a handful of popular methods:
- Random Number Generator : Give each member of the sample a unique number; use a computer program to randomly generate a number from the list for each group.
- Lottery : Give each member of the sample a unique number. Place all numbers in a hat or bucket and draw numbers at random for each group.
- Flipping a Coin : Flip a coin for each participant to decide if they will be in the control group or experimental group (this method can only be used when you have just two groups)
- Roll a Die : For each number on the list, roll a dice to decide which of the groups they will be in. For example, assume that rolling 1, 2, or 3 places them in a control group and rolling 3, 4, 5 lands them in an experimental group.
When is Random Assignment not used?
- When it is not ethically permissible: Randomization is only ethical if the researcher has no evidence that one treatment is superior to the other or that one treatment might have harmful side effects.
- When answering non-causal questions : If the researcher is just interested in predicting the probability of an event, the causal relationship between the variables is not important and observational designs would be more suitable than random assignment.
- When studying the effect of variables that cannot be manipulated: Some risk factors cannot be manipulated and so it would not make any sense to study them in a randomized trial. For example, we cannot randomly assign participants into categories based on age, gender, or genetic factors.
Drawbacks of Random Assignment
While randomization assures an unbiased assignment of participants to groups, it does not guarantee the equality of these groups. There could still be extraneous variables that differ between groups or group differences that arise from chance. Additionally, there is still an element of luck with random assignments.
Thus, researchers can not produce perfectly equal groups for each specific study. Differences between the treatment group and control group might still exist, and the results of a randomized trial may sometimes be wrong, but this is absolutely okay.
Scientific evidence is a long and continuous process, and the groups will tend to be equal in the long run when data is aggregated in a meta-analysis.
Additionally, external validity (i.e., the extent to which the researcher can use the results of the study to generalize to the larger population) is compromised with random assignment.
Random assignment is challenging to implement outside of controlled laboratory conditions and might not represent what would happen in the real world at the population level.
Random assignment can also be more costly than simple observational studies, where an investigator is just observing events without intervening with the population.
Randomization also can be time-consuming and challenging, especially when participants refuse to receive the assigned treatment or do not adhere to recommendations.
What is the difference between random sampling and random assignment?
Random sampling refers to randomly selecting a sample of participants from a population. Random assignment refers to randomly assigning participants to treatment groups from the selected sample.
Does random assignment increase internal validity?
Yes, random assignment ensures that there are no systematic differences between the participants in each group, enhancing the study’s internal validity .
Does random assignment reduce sampling error?
Yes, with random assignment, participants have an equal chance of being assigned to either a control group or an experimental group, resulting in a sample that is, in theory, representative of the population.
Random assignment does not completely eliminate sampling error because a sample only approximates the population from which it is drawn. However, random sampling is a way to minimize sampling errors.
When is random assignment not possible?
Random assignment is not possible when the experimenters cannot control the treatment or independent variable.
For example, if you want to compare how men and women perform on a test, you cannot randomly assign subjects to these groups.
Participants are not randomly assigned to different groups in this study, but instead assigned based on their characteristics.
Does random assignment eliminate confounding variables?
Yes, random assignment eliminates the influence of any confounding variables on the treatment because it distributes them at random among the study groups. Randomization invalidates any relationship between a confounding variable and the treatment.
Why is random assignment of participants to treatment conditions in an experiment used?
Random assignment is used to ensure that all groups are comparable at the start of a study. This allows researchers to conclude that the outcomes of the study can be attributed to the intervention at hand and to rule out alternative explanations for study results.
Further Reading
- Bogomolnaia, A., & Moulin, H. (2001). A new solution to the random assignment problem . Journal of Economic theory , 100 (2), 295-328.
- Krause, M. S., & Howard, K. I. (2003). What random assignment does and does not do . Journal of Clinical Psychology , 59 (7), 751-766.


An official website of the United States government
The .gov means it’s official. Federal government websites often end in .gov or .mil. Before sharing sensitive information, make sure you’re on a federal government site.
The site is secure. The https:// ensures that you are connecting to the official website and that any information you provide is encrypted and transmitted securely.
- Publications
- Account settings
Preview improvements coming to the PMC website in October 2024. Learn More or Try it out now .
- Advanced Search
- Journal List
- Gastroenterol Hepatol Bed Bench
- v.5(2); Spring 2012
How to control confounding effects by statistical analysis
Mohamad amin pourhoseingholi.
1 Department of Biostatistics, Shahid Beheshti University of Medical Sciences, Tehran, Iran
Ahmad Reza Baghestani
2 Department of Mathematic, Islamic Azad University - South Tehran Branch, Iran
Mohsen Vahedi
3 Department of Epidemiology and Biostatistics, School of Public Health, Tehran University of Medical Sciences, Tehran, Iran
A Confounder is a variable whose presence affects the variables being studied so that the results do not reflect the actual relationship. There are various ways to exclude or control confounding variables including Randomization, Restriction and Matching. But all these methods are applicable at the time of study design. When experimental designs are premature, impractical, or impossible, researchers must rely on statistical methods to adjust for potentially confounding effects. These Statistical models (especially regression models) are flexible to eliminate the effects of confounders.
Introduction
Confounding variables or confounders are often defined as the variables correlate (positively or negatively) with both the dependent variable and the independent variable ( 1 ). A Confounder is an extraneous variable whose presence affects the variables being studied so that the results do not reflect the actual relationship between the variables under study.
The aim of major epidemiological studies is to search for the causes of diseases, based on associations with various risk factors. There may be also other factors that are associated with the exposure and affect the risk of developing the disease and they will distort the observed association between the disease and exposure under study. A hypothetical example would be a study of relation between coffee drinking and lung cancer. If the person who entered in the study as a coffee drinker was also more likely to be cigarette smoker, and the study only measured coffee drinking but not smoking, the results may seem to show that coffee drinking increases the risk of lung cancer, which may not be true. However, if a confounding factor (in this example, smoking) is recognized, adjustments can be made in the study design or data analysis so that the effects of confounder would be removed from the final results. Simpson's paradox too is another classic example of confounding ( 2 ). Simpson's paradox refers to the reversal of the direction of an association when data from several groups are combined to form a single group.
The researchers therefore need to account for these variables - either through experimental design and before the data gathering, or through statistical analysis after the data gathering process. In this case the researchers are said to account for their effects to avoid a false positive (Type I) error (a false conclusion that the dependent variables are in a casual relationship with the independent variable). Thus, confounding is a major threat to the validity of inferences made about cause and effect (internal validity). There are various ways to modify a study design to actively exclude or control confounding variables ( 3 ) including Randomization, Restriction and Matching.
In randomization the random assignment of study subjects to exposure categories to breaking any links between exposure and confounders. This reduces potential for confounding by generating groups that are fairly comparable with respect to known and unknown confounding variables.
Restriction eliminates variation in the confounder (for example if an investigator only selects subjects of the same age or same sex then, the study will eliminate confounding by sex or age group). Matching which involves selection of a comparison group with respect to the distribution of one or more potential confounders.
Matching is commonly used in case-control studies (for example, if age and sex are the matching variables, then a 45 year old male case is matched to a male control with same age).
But all these methods mentioned above are applicable at the time of study design and before the process of data gathering. When experimental designs are premature, impractical, or impossible, researchers must rely on statistical methods to adjust for potentially confounding effects ( 4 ).
Statistical Analysis to eliminate confounding effects
Unlike selection or information bias, confounding is one type of bias that can be, adjusted after data gathering, using statistical models. To control for confounding in the analyses, investigators should measure the confounders in the study. Researchers usually do this by collecting data on all known, previously identified confounders. There are mostly two options to dealing with confounders in analysis stage; Stratification and Multivariate methods.
1. Stratification
Objective of stratification is to fix the level of the confounders and produce groups within which the confounder does not vary. Then evaluate the exposure-outcome association within each stratum of the confounder. So within each stratum, the confounder cannot confound because it does not vary across the exposure-outcome.
After stratification, Mantel-Haenszel (M-H) estimator can be employed to provide an adjusted result according to strata. If there is difference between Crude result and adjusted result (produced from strata) confounding is likely. But in the case that Crude result dose not differ from the adjusted result, then confounding is unlikely.
2. Multivariate Models
Stratified analysis works best in the way that there are not a lot of strata and if only 1 or 2 confounders have to be controlled. If the number of potential confounders or the level of their grouping is large, multivariate analysis offers the only solution.
Multivariate models can handle large numbers of covariates (and also confounders) simultaneously. For example in a study that aimed to measure the relation between body mass index and Dyspepsia, one could control for other covariates like as age, sex, smoking, alcohol, ethnicity, etc in the same model.
2.1. Logistic Regression
Logistic regression is a mathematical process that produces results that can be interpreted as an odds ratio, and it is easy to use by any statistical package. The special thing about logistic regression is that it can control for numerous confounders (if there is a large enough sample size). Thus logistic regression is a mathematical model that can give an odds ratio which is controlled for multiple confounders. This odds ratio is known as the adjusted odds ratio, because its value has been adjusted for the other covariates (including confounders).
2.2. Linear Regression
The linear regression analysis is another statistical model that can be used to examine the association between multiple covariates and a numeric outcome. This model can be employed as a multiple linear regression to see through confounding and isolate the relationship of interest ( 5 ). For example, in a research seeking for relationship between LDL cholesterol level and age, the multiple linear regression lets you answer the question, How does LDL level vary with age, after accounting for blood sugar and lipid (as the confounding factors)? In multiple linear regression (as mentioned for logistic regression), investigators can include many covariates at one time. The process of accounting for covariates is also called adjustment (similar to logistic regression model) and comparing the results of simple and multiple linear regressions can clarify that how much the confounders in the model distort the relationship between exposure and outcome.
2.3. Analysis of Covariance
The Analysis of Covariance (ANCOVA) is a type of Analysis of Variance (ANOVA) that is used to control for potential confounding variables. ANCOVA is a statistical linear model with a continuous outcome variable (quantitative, scaled) and two or more predictor variables where at least one is continuous (quantitative, scaled) and at least one is categorical (nominal, non-scaled). ANCOVA is a combination of ANOVA and linear regression. ANCOVA tests whether certain factors have an effect on the outcome variable after removing the variance for which quantitative covariates (confounders) account. The inclusion of this analysis can increase the statistical power.
The Analysis of Covariance (ANCOVA) is a type of Analysis of Variance (ANOVA) that is used to control for potential confounding variables . ANCOVA is a statistical linear model with a continuous outcome variable (quantitative, scaled) and two or more predictor variables where at least one is continuous (quantitative, scaled) and at least one is categorical (nominal, non-scaled). ANCOVA is a combination of ANOVA and linear regression. ANCOVA tests whether certain factors have an effect on the outcome variable after removing the variance for which quantitative covariates (confounders) account. The inclusion of this analysis can increase the statistical power.
Practical example
Suppose that, in a cross-sectional study, we are seeking for the relation between infection with Helicobacter. Pylori (HP) and Dyspepsia Symptoms. The study conducted on 550 persons with positive H.P and 440 persons without HP. The results are appeared in 2*2 crude table ( Table 1 ) that indicated that the relation between infection with H.P and Dyspepsia is a reverese association (OR = 0.60, 95% CI: 0.42-0.94). Now suppose that weight can be a potential confounder in this study. So we break the crude table down in two stratum according to the weight of subjects (normal weight or over weight) and then calculate OR's for each stratum again. If stratum-specific OR is similar to crude OR, there is no potential impact from confounding factors. In this example there are different OR for each stratum (for normal weight group OR= 0.80, 95% CI: 0.38-1.69 and for overweight group OR= 1.60, 95% CI: 0.79-3.27).
The crude contingency table of association between H.Pylori and Dyspepsia
The contingency table of association between H. Pylori and Dyspepsia for person who are in normal weight group
The contingency table of association between H. Pylori and Dyspepsia for person who are in over weight group
This shows that there is a potential confounding affects which is presented by weight in this study. This example is a type of Simpson's paradox, therefore the crude OR is not justified for this study. We calculated the Mantel-Haenszel (M-H) estimator as an alternative statistical analysis to remove the confounding effects (OR= 1.16, 95% CI: 0.71-1.90). Also logistic regression model (in which, weight is presented in multiple model) would be conducted to control the confounder, its result is similar as M-H estimator (OR= 1.15, 95% CI: 0.71-1.89).
The results of this example clearly indicated that if the impacts of confounders did not account in the analysis, the results can deceive the researchers with unjustified results.
Confounders are common causes of both treatment/exposure and of response/outcome. Confounding is better taken care of by randomization at the design stage of the research ( 6 ).
A successful randomization minimizes confounding by unmeasured as well as measured factors, whereas statistical control that addresses confounding by measurement and can introduce confounding through inappropriate control ( 7 – 9 ).
Confounding can persist, even after adjustment. In many studies, confounders are not adjusted because they were not measured during the process of data gathering. In some situation, confounder variables are measured with error or their categories are improperly defined (for example age categories were not well implied its confounding nature) ( 10 ). Also there is a possibility that the variables that are controlled as the confounders were actually not confounders.
Before applying a statistical correction method, one has to decide which factors are confounders. This sometimes is a complex issue ( 11 – 13 ). Common strategies to decide whether a variable is a confounder that should be adjusted or not, rely mostly on statistical criteria. The research strategy should be based on the knowledge of the field and on conceptual framework and causal model. So expertise' criteria should be involved for evaluating the confounders. Statistical models (especially regression models) are a flexible way of investigating the separate or joint effects of several risk factors for disease or ill health ( 14 ). But the researchers should notice that wrong assumptions about the form of the relationship between confounder and disease can lead to wrong conclusions about exposure effects too.
( Please cite as: Pourhoseingholi MA, Baghestani AR, Vahedi M. How to control confounding effects by statistical analysis. Gastroenterol Hepatol Bed Bench 2012;5(2):79-83.)
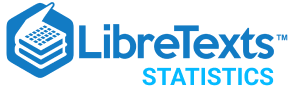
- school Campus Bookshelves
- menu_book Bookshelves
- perm_media Learning Objects
- login Login
- how_to_reg Request Instructor Account
- hub Instructor Commons
- Download Page (PDF)
- Download Full Book (PDF)
- Periodic Table
- Physics Constants
- Scientific Calculator
- Reference & Cite
- Tools expand_more
- Readability
selected template will load here
This action is not available.
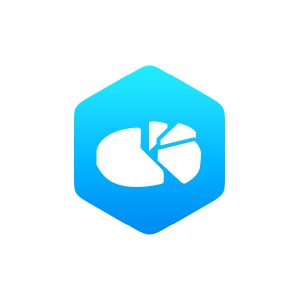
1.3: Threats to Internal Validity and Different Control Techniques
- Last updated
- Save as PDF
- Page ID 32915
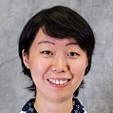
- Yang Lydia Yang
- Kansas State University
Internal validity is often the focus from a research design perspective. To understand the pros and cons of various designs and to be able to better judge specific designs, we identify specific threats to internal validity . Before we do so, it is important to note that the primary challenge to establishing internal validity in social sciences is the fact that most of the phenomena we care about have multiple causes and are often a result of some complex set of interactions. For example, X may be only a partial cause of Y or X may cause Y, but only when Z is present. Multiple causation and interactive effects make it very difficult to demonstrate causality. Turning now to more specific threats, Figure 1.3.1 below identifies common threats to internal validity.
Different Control Techniques
All of the common threats mentioned above can introduce extraneous variables into your research design, which will potentially confound your research findings. In other words, we won't be able to tell whether it is the independent variable (i.e., the treatment we give participants), or the extraneous variable, that causes the changes in the dependent variable. Controlling for extraneous variables reduces its threats on the research design and gives us a better chance to claim the independent variable causes the changes in the dependent variable, i.e., internal validity. There are different techniques we can use to control for extraneous variables.
Random assignment
Random assignment is the single most powerful control technique we can use to minimize the potential threats of the confounding variables in research design. As we have seen in Dunn and her colleagues' study earlier, participants are not allowed to self select into either conditions (spend $20 on self or spend on others). Instead, they are randomly assigned into either group by the researcher(s). By doing so, the two groups are likely to be similar on all other factors except the independent variable itself. One confounding variable mentioned earlier is whether individuals had a happy childhood to begin with. Using random assignment, those who had a happy childhood will likely end up in each condition group. Similarly, those who didn't have a happy childhood will likely end up in each condition group too. As a consequence, we can expect the two condition groups to be very similar on this confounding variable. Applying the same logic, we can use random assignment to minimize all potential confounding variables (assuming your sample size is large enough!). With that, the only difference between the two groups is the condition participants are assigned to, which is the independent variable, then we are confident to infer that the independent variable actually causes the differences in the dependent variables.
It is critical to emphasize that random assignment is the only control technique to control for both known and unknown confounding variables. With all other control techniques mentioned below, we must first know what the confounding variable is before controlling it. Random assignment does not. With the simple act of randomly assigning participants into different conditions, we take care both the confounding variables we know of and the ones we don't even know that could threat the internal validity of our studies. As the saying goes, "what you don't know will hurt you." Random assignment take cares of it.
Matching is another technique we can use to control for extraneous variables. We must first identify the extraneous variable that can potentially confound the research design. Then we want to rank order the participants on this extraneous variable or list the participants in a ascending or descending order. Participants who are similar on the extraneous variable will be placed into different treatment groups. In other words, they are "matched" on the extraneous variable. Then we can carry out the intervention/treatment as usual. If different treatment groups do show differences on the dependent variable, we would know it is not the extraneous variables because participants are "matched" or equivalent on the extraneous variable. Rather it is more likely to the independent variable (i.e., the treatments) that causes the changes in the dependent variable. Use the example above (self-spending vs. others-spending on happiness) with the same extraneous variable of whether individuals had a happy childhood to begin with. Once we identify this extraneous variable, we do need to first collect some kind of data from the participants to measure how happy their childhood was. Or sometimes, data on the extraneous variables we plan to use may be already available (for example, you want to examine the effect of different types of tutoring on students' performance in Calculus I course and you plan to match them on this extraneous variable: college entrance test scores, which is already collected by the Admissions Office). In either case, getting the data on the identified extraneous variable is a typical step we need to do before matching. So going back to whether individuals had a happy childhood to begin with. Once we have data, we'd sort it in a certain order, for example, from the highest score (meaning participants reporting the happiest childhood) to the lowest score (meaning participants reporting the least happy childhood). We will then identify/match participants with the highest levels of childhood happiness and place them into different treatment groups. Then we go down the scale and match participants with relative high levels of childhood happiness and place them into different treatment groups. We repeat on the descending order until we match participants with the lowest levels of childhood happiness and place them into different treatment groups. By now, each treatment group will have participants with a full range of levels on childhood happiness (which is a strength...thinking about the variation, the representativeness of the sample). The two treatment groups will be similar or equivalent on this extraneous variable. If the treatments, self-spending vs. other-spending, eventually shows the differences on individual happiness, then we know it's not due to how happy their childhood was. We will be more confident it is due to the independent variable.
You may be thinking, but wait we have only taken care of one extraneous variable. What about other extraneous variables? Good thinking.That's exactly correct. We mentioned a few extraneous variables but have only matched them on one. This is the main limitation of matching. You can match participants on more than one extraneous variables, but it's cumbersome, if not impossible, to match them on 10 or 20 extraneous variables. More importantly, the more variables we try to match participants on, the less likely we will have a similar match. In other words, it may be easy to find/match participants on one particular extraneous variable (similar level of childhood happiness), but it's much harder to find/match participants to be similar on 10 different extraneous variables at once.
Holding Extraneous Variable Constant
Holding extraneous variable constant control technique is self-explanatory. We will use participants at one level of extraneous variable only, in other words, holding the extraneous variable constant. Using the same example above, for example we only want to study participants with the low level of childhood happiness. We do need to go through the same steps as in Matching: identifying the extraneous variable that can potentially confound the research design and getting the data on the identified extraneous variable. Once we have the data on childhood happiness scores, we will only include participants on the lower end of childhood happiness scores, then place them into different treatment groups and carry out the study as before. If the condition groups, self-spending vs. other-spending, eventually shows the differences on individual happiness, then we know it's not due to how happy their childhood was (since we already picked those on the lower end of childhood happiness only). We will be more confident it is due to the independent variable.
Similarly to Matching, we have to do this one extraneous variable at a time. As we increase the number of extraneous variables to be held constant, the more difficult it gets. The other limitation is by holding extraneous variable constant, we are excluding a big chunk of participants, in this case, anyone who are NOT low on childhood happiness. This is a major weakness, as we reduce the variability on the spectrum of childhood happiness levels, we decreases the representativeness of the sample and generalizabiliy suffers.
Building Extraneous Variables into Design
The last control technique building extraneous variables into research design is widely used. Like the name suggests, we would identify the extraneous variable that can potentially confound the research design, and include it into the research design by treating it as an independent variable. This control technique takes care of the limitation the previous control technique, holding extraneous variable constant, has. We don't need to excluding participants based on where they stand on the extraneous variable(s). Instead we can include participants with a wide range of levels on the extraneous variable(s). You can include multiple extraneous variables into the design at once. However, the more variables you include in the design, the large the sample size it requires for statistical analyses, which may be difficult to obtain due to limitations of time, staff, cost, access, etc.
Purpose and Limitations of Random Assignment
In an experimental study, random assignment is a process by which participants are assigned, with the same chance, to either a treatment or a control group. The goal is to assure an unbiased assignment of participants to treatment options.
Random assignment is considered the gold standard for achieving comparability across study groups, and therefore is the best method for inferring a causal relationship between a treatment (or intervention or risk factor) and an outcome.
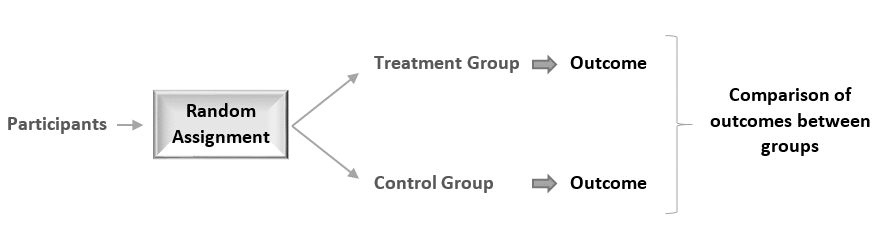
Random assignment of participants produces comparable groups regarding the participants’ initial characteristics, thereby any difference detected in the end between the treatment and the control group will be due to the effect of the treatment alone.
How does random assignment produce comparable groups?
1. random assignment prevents selection bias.
Randomization works by removing the researcher’s and the participant’s influence on the treatment allocation. So the allocation can no longer be biased since it is done at random, i.e. in a non-predictable way.
This is in contrast with the real world, where for example, the sickest people are more likely to receive the treatment.
2. Random assignment prevents confounding
A confounding variable is one that is associated with both the intervention and the outcome, and thus can affect the outcome in 2 ways:
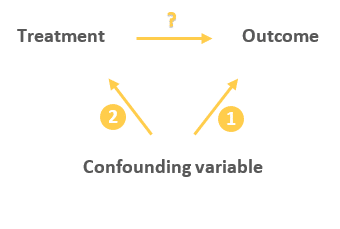
Either directly:
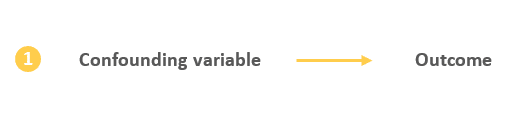
Or indirectly through the treatment:

This indirect relationship between the confounding variable and the outcome can cause the treatment to appear to have an influence on the outcome while in reality the treatment is just a mediator of that effect (as it happens to be on the causal pathway between the confounder and the outcome).
Random assignment eliminates the influence of the confounding variables on the treatment since it distributes them at random between the study groups, therefore, ruling out this alternative path or explanation of the outcome.
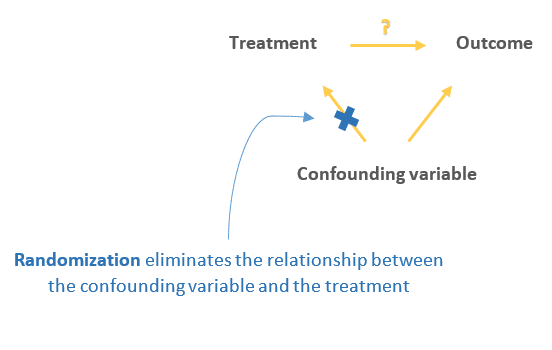
3. Random assignment also eliminates other threats to internal validity
By distributing all threats (known and unknown) at random between study groups, participants in both the treatment and the control group become equally subject to the effect of any threat to validity. Therefore, comparing the outcome between the 2 groups will bypass the effect of these threats and will only reflect the effect of the treatment on the outcome.
These threats include:
- History: This is any event that co-occurs with the treatment and can affect the outcome.
- Maturation: This is the effect of time on the study participants (e.g. participants becoming wiser, hungrier, or more stressed with time) which might influence the outcome.
- Regression to the mean: This happens when the participants’ outcome score is exceptionally good on a pre-treatment measurement, so the post-treatment measurement scores will naturally regress toward the mean — in simple terms, regression happens since an exceptional performance is hard to maintain. This effect can bias the study since it represents an alternative explanation of the outcome.
Note that randomization does not prevent these effects from happening, it just allows us to control them by reducing their risk of being associated with the treatment.
What if random assignment produced unequal groups?
Question: What should you do if after randomly assigning participants, it turned out that the 2 groups still differ in participants’ characteristics? More precisely, what if randomization accidentally did not balance risk factors that can be alternative explanations between the 2 groups? (For example, if one group includes more male participants, or sicker, or older people than the other group).
Short answer: This is perfectly normal, since randomization only assures an unbiased assignment of participants to groups, i.e. it produces comparable groups, but it does not guarantee the equality of these groups.
A more complete answer: Randomization will not and cannot create 2 equal groups regarding each and every characteristic. This is because when dealing with randomization there is still an element of luck. If you want 2 perfectly equal groups, you better match them manually as is done in a matched pairs design (for more information see my article on matched pairs design ).
This is similar to throwing a die: If you throw it 10 times, the chance of getting a specific outcome will not be 1/6. But it will approach 1/6 if you repeat the experiment a very large number of times and calculate the average number of times the specific outcome turned up.
So randomization will not produce perfectly equal groups for each specific study, especially if the study has a small sample size. But do not forget that scientific evidence is a long and continuous process, and the groups will tend to be equal in the long run when a meta-analysis aggregates the results of a large number of randomized studies.
So for each individual study, differences between the treatment and control group will exist and will influence the study results. This means that the results of a randomized trial will sometimes be wrong, and this is absolutely okay.
BOTTOM LINE:
Although the results of a particular randomized study are unbiased, they will still be affected by a sampling error due to chance. But the real benefit of random assignment will be when data is aggregated in a meta-analysis.
Limitations of random assignment
Randomized designs can suffer from:
1. Ethical issues:
Randomization is ethical only if the researcher has no evidence that one treatment is superior to the other.
Also, it would be unethical to randomly assign participants to harmful exposures such as smoking or dangerous chemicals.
2. Low external validity:
With random assignment, external validity (i.e. the generalizability of the study results) is compromised because the results of a study that uses random assignment represent what would happen under “ideal” experimental conditions, which is in general very different from what happens at the population level.
In the real world, people who take the treatment might be very different from those who don’t – so the assignment of participants is not a random event, but rather under the influence of all sort of external factors.
External validity can be also jeopardized in cases where not all participants are eligible or willing to accept the terms of the study.
3. Higher cost of implementation:
An experimental design with random assignment is typically more expensive than observational studies where the investigator’s role is just to observe events without intervening.
Experimental designs also typically take a lot of time to implement, and therefore are less practical when a quick answer is needed.
4. Impracticality when answering non-causal questions:
A randomized trial is our best bet when the question is to find the causal effect of a treatment or a risk factor.
Sometimes however, the researcher is just interested in predicting the probability of an event or a disease given some risk factors. In this case, the causal relationship between these variables is not important, making observational designs more suitable for such problems.
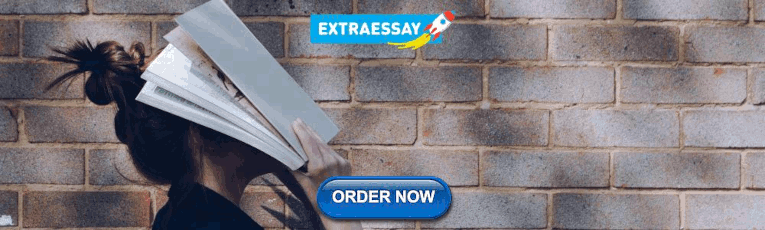
5. Impracticality when studying the effect of variables that cannot be manipulated:
The usual objective of studying the effects of risk factors is to propose recommendations that involve changing the level of exposure to these factors.
However, some risk factors cannot be manipulated, and so it does not make any sense to study them in a randomized trial. For example it would be impossible to randomly assign participants to age categories, gender, or genetic factors.
6. Difficulty to control participants:
These difficulties include:
- Participants refusing to receive the assigned treatment.
- Participants not adhering to recommendations.
- Differential loss to follow-up between those who receive the treatment and those who don’t.
All of these issues might occur in a randomized trial, but might not affect an observational study.
- Shadish WR, Cook TD, Campbell DT. Experimental and Quasi-Experimental Designs for Generalized Causal Inference . 2nd edition. Cengage Learning; 2001.
- Friedman LM, Furberg CD, DeMets DL, Reboussin DM, Granger CB. Fundamentals of Clinical Trials . 5th ed. 2015 edition. Springer; 2015.
Further reading
- Posttest-Only Control Group Design
- Pretest-Posttest Control Group Design
- Randomized Block Design
Statistical Thinking: A Simulation Approach to Modeling Uncertainty (UM STAT 216 edition)
3.6 causation and random assignment.
Medical researchers may be interested in showing that a drug helps improve people’s health (the cause of improvement is the drug), while educational researchers may be interested in showing a curricular innovation improves students’ learning (the curricular innovation causes improved learning).
To attribute a causal relationship, there are three criteria a researcher needs to establish:
- Association of the Cause and Effect: There needs to be a association between the cause and effect.
- Timing: The cause needs to happen BEFORE the effect.
- No Plausible Alternative Explanations: ALL other possible explanations for the effect need to be ruled out.
Please read more about each of these criteria at the Web Center for Social Research Methods .
The third criterion can be quite difficult to meet. To rule out ALL other possible explanations for the effect, we want to compare the world with the cause applied to the world without the cause. In practice, we do this by comparing two different groups: a “treatment” group that gets the cause applied to them, and a “control” group that does not. To rule out alternative explanations, the groups need to be “identical” with respect to every possible characteristic (aside from the treatment) that could explain differences. This way the only characteristic that will be different is that the treatment group gets the treatment and the control group doesn’t. If there are differences in the outcome, then it must be attributable to the treatment, because the other possible explanations are ruled out.
So, the key is to make the control and treatment groups “identical” when you are forming them. One thing that makes this task (slightly) easier is that they don’t have to be exactly identical, only probabilistically equivalent . This means, for example, that if you were matching groups on age that you don’t need the two groups to have identical age distributions; they would only need to have roughly the same AVERAGE age. Here roughly means “the average ages should be the same within what we expect because of sampling error.”
Now we just need to create the groups so that they have, on average, the same characteristics … for EVERY POSSIBLE CHARCTERISTIC that could explain differences in the outcome.
It turns out that creating probabilistically equivalent groups is a really difficult problem. One method that works pretty well for doing this is to randomly assign participants to the groups. This works best when you have large sample sizes, but even with small sample sizes random assignment has the advantage of at least removing the systematic bias between the two groups (any differences are due to chance and will probably even out between the groups). As Wikipedia’s page on random assignment points out,
Random assignment of participants helps to ensure that any differences between and within the groups are not systematic at the outset of the experiment. Thus, any differences between groups recorded at the end of the experiment can be more confidently attributed to the experimental procedures or treatment. … Random assignment does not guarantee that the groups are matched or equivalent. The groups may still differ on some preexisting attribute due to chance. The use of random assignment cannot eliminate this possibility, but it greatly reduces it.
We use the term internal validity to describe the degree to which cause-and-effect inferences are accurate and meaningful. Causal attribution is the goal for many researchers. Thus, by using random assignment we have a pretty high degree of evidence for internal validity; we have a much higher belief in causal inferences. Much like evidence used in a court of law, it is useful to think about validity evidence on a continuum. For example, a visualization of the internal validity evidence for a study that employed random assignment in the design might be:

The degree of internal validity evidence is high (in the upper-third). How high depends on other factors such as sample size.
To learn more about random assignment, you can read the following:
- The research report, Random Assignment Evaluation Studies: A Guide for Out-of-School Time Program Practitioners
3.6.1 Example: Does sleep deprivation cause an decrease in performance?
Let’s consider the criteria with respect to the sleep deprivation study we explored in class.
3.6.1.1 Association of cause and effect
First, we ask, Is there an association between the cause and the effect? In the sleep deprivation study, we would ask, “Is sleep deprivation associated with an decrease in performance?”
This is what a hypothesis test helps us answer! If the result is statistically significant , then we have an association between the cause and the effect. If the result is not statistically significant, then there is not sufficient evidence for an association between cause and effect.
In the case of the sleep deprivation experiment, the result was statistically significant, so we can say that sleep deprivation is associated with a decrease in performance.
3.6.1.2 Timing
Second, we ask, Did the cause come before the effect? In the sleep deprivation study, the answer is yes. The participants were sleep deprived before their performance was tested. It may seem like this is a silly question to ask, but as the link above describes, it is not always so clear to establish the timing. Thus, it is important to consider this question any time we are interested in establishing causality.
3.6.1.3 No plausible alternative explanations
Finally, we ask Are there any plausible alternative explanations for the observed effect? In the sleep deprivation study, we would ask, “Are there plausible alternative explanations for the observed difference between the groups, other than sleep deprivation?” Because this is a question about plausibility, human judgment comes into play. Researchers must make an argument about why there are no plausible alternatives. As described above, a strong study design can help to strengthen the argument.
At first, it may seem like there are a lot of plausible alternative explanations for the difference in performance. There are a lot of things that might affect someone’s performance on a visual task! Sleep deprivation is just one of them! For example, artists may be more adept at visual discrimination than other people. This is an example of a potential confounding variable. A confounding variable is a variable that might affect the results, other than the causal variable that we are interested in.
Here’s the thing though. We are not interested in figuring out why any particular person got the score that they did. Instead, we are interested in determining why one group was different from another group. In the sleep deprivation study, the participants were randomly assigned. This means that the there is no systematic difference between the groups, with respect to any confounding variables. Yes—artistic experience is a possible confounding variable, and it may be the reason why two people score differently. BUT: There is no systematic difference between the groups with respect to artistic experience, and so artistic experience is not a plausible explanation as to why the groups would be different. The same can be said for any possible confounding variable. Because the groups were randomly assigned, it is not plausible to say that the groups are different with respect to any confounding variable. Random assignment helps us rule out plausible alternatives.
3.6.1.4 Making a causal claim
Now, let’s see about make a causal claim for the sleep deprivation study:
- Association: There is a statistically significant result, so the cause is associated with the effect
- Timing: The participants were sleep deprived before their performance was measured, so the cause came before the effect
- Plausible alternative explanations: The participants were randomly assigned, so the groups are not systematically different on any confounding variable. The only systematic difference between the groups was sleep deprivation. Thus, there are no plausible alternative explanations for the difference between the groups, other than sleep deprivation
Thus, the internal validity evidence for this study is high, and we can make a causal claim. For the participants in this study, we can say that sleep deprivation caused a decrease in performance.
Key points: Causation and internal validity
To make a cause-and-effect inference, you need to consider three criteria:
- Association of the Cause and Effect: There needs to be a association between the cause and effect. This can be established by a hypothesis test.
Random assignment removes any systematic differences between the groups (other than the treatment), and thus helps to rule out plausible alternative explanations.
Internal validity describes the degree to which cause-and-effect inferences are accurate and meaningful.
Confounding variables are variables that might affect the results, other than the causal variable that we are interested in.
Probabilistic equivalence means that there is not a systematic difference between groups. The groups are the same on average.
How can we make "equivalent" experimental groups?
- Bipolar Disorder
- Therapy Center
- When To See a Therapist
- Types of Therapy
- Best Online Therapy
- Best Couples Therapy
- Best Family Therapy
- Managing Stress
- Sleep and Dreaming
- Understanding Emotions
- Self-Improvement
- Healthy Relationships
- Student Resources
- Personality Types
- Guided Meditations
- Verywell Mind Insights
- 2023 Verywell Mind 25
- Mental Health in the Classroom
- Editorial Process
- Meet Our Review Board
- Crisis Support
The Definition of Random Assignment According to Psychology
Kendra Cherry, MS, is a psychosocial rehabilitation specialist, psychology educator, and author of the "Everything Psychology Book."
:max_bytes(150000):strip_icc():format(webp)/IMG_9791-89504ab694d54b66bbd72cb84ffb860e.jpg)
Emily is a board-certified science editor who has worked with top digital publishing brands like Voices for Biodiversity, Study.com, GoodTherapy, Vox, and Verywell.
:max_bytes(150000):strip_icc():format(webp)/Emily-Swaim-1000-0f3197de18f74329aeffb690a177160c.jpg)
Materio / Getty Images
Random assignment refers to the use of chance procedures in psychology experiments to ensure that each participant has the same opportunity to be assigned to any given group in a study to eliminate any potential bias in the experiment at the outset. Participants are randomly assigned to different groups, such as the treatment group versus the control group. In clinical research, randomized clinical trials are known as the gold standard for meaningful results.
Simple random assignment techniques might involve tactics such as flipping a coin, drawing names out of a hat, rolling dice, or assigning random numbers to a list of participants. It is important to note that random assignment differs from random selection .
While random selection refers to how participants are randomly chosen from a target population as representatives of that population, random assignment refers to how those chosen participants are then assigned to experimental groups.
Random Assignment In Research
To determine if changes in one variable will cause changes in another variable, psychologists must perform an experiment. Random assignment is a critical part of the experimental design that helps ensure the reliability of the study outcomes.
Researchers often begin by forming a testable hypothesis predicting that one variable of interest will have some predictable impact on another variable.
The variable that the experimenters will manipulate in the experiment is known as the independent variable , while the variable that they will then measure for different outcomes is known as the dependent variable. While there are different ways to look at relationships between variables, an experiment is the best way to get a clear idea if there is a cause-and-effect relationship between two or more variables.
Once researchers have formulated a hypothesis, conducted background research, and chosen an experimental design, it is time to find participants for their experiment. How exactly do researchers decide who will be part of an experiment? As mentioned previously, this is often accomplished through something known as random selection.
Random Selection
In order to generalize the results of an experiment to a larger group, it is important to choose a sample that is representative of the qualities found in that population. For example, if the total population is 60% female and 40% male, then the sample should reflect those same percentages.
Choosing a representative sample is often accomplished by randomly picking people from the population to be participants in a study. Random selection means that everyone in the group stands an equal chance of being chosen to minimize any bias. Once a pool of participants has been selected, it is time to assign them to groups.
By randomly assigning the participants into groups, the experimenters can be fairly sure that each group will have the same characteristics before the independent variable is applied.
Participants might be randomly assigned to the control group , which does not receive the treatment in question. The control group may receive a placebo or receive the standard treatment. Participants may also be randomly assigned to the experimental group , which receives the treatment of interest. In larger studies, there can be multiple treatment groups for comparison.
There are simple methods of random assignment, like rolling the die. However, there are more complex techniques that involve random number generators to remove any human error.
There can also be random assignment to groups with pre-established rules or parameters. For example, if you want to have an equal number of men and women in each of your study groups, you might separate your sample into two groups (by sex) before randomly assigning each of those groups into the treatment group and control group.
Random assignment is essential because it increases the likelihood that the groups are the same at the outset. With all characteristics being equal between groups, other than the application of the independent variable, any differences found between group outcomes can be more confidently attributed to the effect of the intervention.
Example of Random Assignment
Imagine that a researcher is interested in learning whether or not drinking caffeinated beverages prior to an exam will improve test performance. After randomly selecting a pool of participants, each person is randomly assigned to either the control group or the experimental group.
The participants in the control group consume a placebo drink prior to the exam that does not contain any caffeine. Those in the experimental group, on the other hand, consume a caffeinated beverage before taking the test.
Participants in both groups then take the test, and the researcher compares the results to determine if the caffeinated beverage had any impact on test performance.
A Word From Verywell
Random assignment plays an important role in the psychology research process. Not only does this process help eliminate possible sources of bias, but it also makes it easier to generalize the results of a tested sample of participants to a larger population.
Random assignment helps ensure that members of each group in the experiment are the same, which means that the groups are also likely more representative of what is present in the larger population of interest. Through the use of this technique, psychology researchers are able to study complex phenomena and contribute to our understanding of the human mind and behavior.
Lin Y, Zhu M, Su Z. The pursuit of balance: An overview of covariate-adaptive randomization techniques in clinical trials . Contemp Clin Trials. 2015;45(Pt A):21-25. doi:10.1016/j.cct.2015.07.011
Sullivan L. Random assignment versus random selection . In: The SAGE Glossary of the Social and Behavioral Sciences. SAGE Publications, Inc.; 2009. doi:10.4135/9781412972024.n2108
Alferes VR. Methods of Randomization in Experimental Design . SAGE Publications, Inc.; 2012. doi:10.4135/9781452270012
Nestor PG, Schutt RK. Research Methods in Psychology: Investigating Human Behavior. (2nd Ed.). SAGE Publications, Inc.; 2015.
By Kendra Cherry, MSEd Kendra Cherry, MS, is a psychosocial rehabilitation specialist, psychology educator, and author of the "Everything Psychology Book."

Statistics Made Easy
What is a Confounding Variable? (Definition & Example)
In any experiment, there are two main variables:
The independent variable: the variable that an experimenter changes or controls so that they can observe the effects on the dependent variable.
The dependent variable: the variable being measured in an experiment that is “dependent” on the independent variable.

Researchers are often interested in understanding how changes in the independent variable affect the dependent variable.
However, sometimes there is a third variable that is not accounted for that can affect the relationship between the two variables under study.

This type of variable is known as a confounding variable and it can confound the results of a study and make it appear that there exists some type of cause-and-effect relationship between two variables that doesn’t actually exist.
Confounding variable: A variable that is not included in an experiment, yet affects the relationship between the two variables in an experiment. This type of variable can confound the results of an experiment and lead to unreliable findings.
For example, suppose a researcher collects data on ice cream sales and shark attacks and finds that the two variables are highly correlated. Does this mean that increased ice cream sales cause more shark attacks?
That’s unlikely. The more likely cause is the confounding variable temperature . When it is warmer outside, more people buy ice cream and more people go in the ocean.

Requirements for Confounding Variables
In order for a variable to be a confounding variable, it must meet the following requirements:
1. It must be correlated with the independent variable.
In the previous example, temperature was correlated with the independent variable of ice cream sales. In particular, warmer temperatures are associated with higher ice cream sales and cooler temperatures are associated with lower sales.
2. It must have a causal relationship with the dependent variable.
In the previous example, temperature had a direct causal effect on the number of shark attacks. In particular, warmer temperatures cause more people to go into the ocean which directly increases the probability of shark attacks occurring.
Why Are Confounding Variables Problematic?
Confounding variables are problematic for two reasons:
1. Confounding variables can make it seem that cause-and-effect relationships exist when they don’t.
In our previous example, the confounding variable of temperature made it seem like there existed a cause-and-effect relationship between ice cream sales and shark attacks.
However, we know that ice cream sales don’t cause shark attacks. The confounding variable of temperature just made it seem this way.
2. Confounding variables can mask the true cause-and-effect relationship between variables.
Suppose we’re studying the ability of exercise to reduce blood pressure. One potential confounding variable is starting weight, which is correlated with exercise and has a direct causal effect on blood pressure.

While increased exercise may lead to reduced blood pressure, an individual’s starting weight also has a big impact on the relationship between these two variables.
Confounding Variables & Internal Validity
In technical terms, confounding variables affect the internal validity of a study, which refers to how valid it is to attribute any changes in the dependent variable to changes in the independent variable.
When confounding variables are present, we can’t always say with complete confidence that the changes we observe in the dependent variable are a direct result of changes in the independent variable.
How to Reduce the Effect of Confounding Variables
There are several ways to reduce the effect of confounding variables, including the following methods:
1. Random Assignment
Random assignment refers to the process of randomly assigning individuals in a study to either a treatment group or a control group.
For example, suppose we want to study the effect of a new pill on blood pressure. If we recruit 100 individuals to participate in the study then we might use a random number generator to randomly assign 50 individuals to a control group (no pill) and 50 individuals to a treatment group (new pill).
By using random assignment, we increase the chances that the two groups will have roughly similar characteristics, which means that any difference we observe between the two groups can be attributed to the treatment.
This means the study should have internal validity – it’s valid to attribute any differences in blood pressure between the groups to the pill itself as opposed to differences between the individuals in the groups.
2. Blocking
Blocking refers to the practice of dividing individuals in a study into “blocks” based on some value of a confounding variable to eliminate the effect of the confounding variable.
For example, suppose researchers want to understand the effect that a new diet has on weight less. The independent variable is the new diet and the dependent variable is the amount of weight loss.
However, a confounding variable that will likely cause variation in weight loss is gender . It’s likely that the gender of an individual will effect the amount of weight they’ll lose, regardless of whether the new diet works or not.
One way to handle this problem is to place individuals into one of two blocks:
Then, within each block we would randomly assign individuals to one of two treatments:
- A standard diet
By doing this, the variation within each block would be much lower compared to the variation among all individuals and we would be able to gain a better understanding of how the new diet affects weight loss while controlling for gender.
3. Matching
A matched pairs design is a type of experimental design in which we “match” individuals based on values of potential confounding variables.
For example, suppose researchers want to know how a new diet affects weight loss compared to a standard diet. Two potential confounding variables in this situation are age and gender .
To account for this, researchers recruit 100 subjects, then group the subjects into 50 pairs based on their age and gender. For example:
- A 25-year-old male will be paired with another 25-year-old male, since they “match” in terms of age and gender.
- A 30-year-old female will be paired with another 30-year-old female since they also match on age and gender, and so on.
Then, within each pair, one subject will randomly be assigned to follow the new diet for 30 days and the other subject will be assigned to follow the standard diet for 30 days.
At the end of the 30 days, researchers will measure the total weight loss for each subject.

By using this type of design, researchers can be confident that any differences in weight loss can be attributed to the type of diet used rather than the confounding variables age and gender .
This type of design does have a few drawbacks, including:
1. Losing two subjects if one drops out. If one subject decides to drop out of the study, you actually lose two subjects since you no longer have a complete pair.
2. Time-consuming to find matches . It can be quite time-consuming to find subjects who match on certain variables, such as gender and age.
3. Impossible to match subjects perfectly . No matter how hard you try, there will always be some variation within the subjects in each pair.
However, if a study has the resources available to implement this design it can be highly effective at eliminating the effects of confounding variables.
Featured Posts

Hey there. My name is Zach Bobbitt. I have a Master of Science degree in Applied Statistics and I’ve worked on machine learning algorithms for professional businesses in both healthcare and retail. I’m passionate about statistics, machine learning, and data visualization and I created Statology to be a resource for both students and teachers alike. My goal with this site is to help you learn statistics through using simple terms, plenty of real-world examples, and helpful illustrations.
Leave a Reply Cancel reply
Your email address will not be published. Required fields are marked *
- En español – ExME
- Em português – EME
Blinding: taking a better look at the blind side
Posted on 29th March 2018 by Neelam Khan
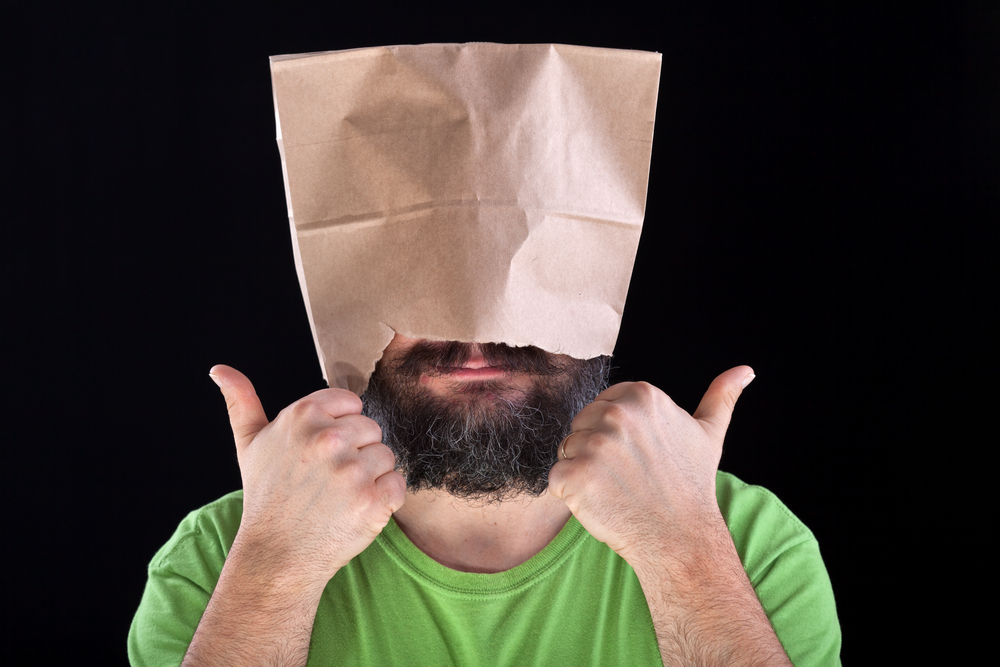
Blinding is a common element used in rigorously designed trials. Most people are familiar with the general concept but what is its purpose and what is the best way to perform it? This blog explores both of these questions and discusses ways to tackle situations where blinding cannot be done.
What is blinding?
In randomised controlled trials (RCTs), there may be 2 or more groups receiving different treatments (possibly including a placebo group). Blinding refers to the process of concealing participants’ group allocations. This may be in the form of single-blinding, where only the participants are unaware of which group they have been assigned to, or double-blinding, where neither the participants nor the researchers know which subjects are receiving the active treatment or placebo.
Why should blinding be used?
The purpose of blinding is to minimise bias. But is this not already achieved through randomisation?
Random assignment of participants to the different groups only helps to eliminate confounding variables present at the time of randomisation, thereby reducing selection bias. It does not, however, prevent differences from developing between the groups afterwards. This is where blinding plays a role.
First of all, blinding can help to preclude the occurrence of differential co-interventions. For example, if the researchers have not been blinded, they may (subconsciously) devote extra time, attention or even apply additional diagnostic and therapeutic procedures to the group receiving the active treatment. This will, in turn, impact the results of the study. Equally, non-blinded participants who are receiving a placebo may actively seek out other treatments for themselves, which may influence the study findings. Employing the use of blinding can prevent this from happening.
Another key reason for blinding is to prevent biased assessment of outcome. When evaluating subjective outcome measures, knowledge of participants’ group allocations may influence non-blinded researchers, thereby incorrectly producing results that are more favourable of the treatment in question. Likewise, participants who are aware of their group allocations may also influence the findings, especially self-reported outcome measures. For instance, participants may selectively report information or change their behaviour to match what they believe the researchers are expecting to see. This is an experimental artefact known as demand characteristics .
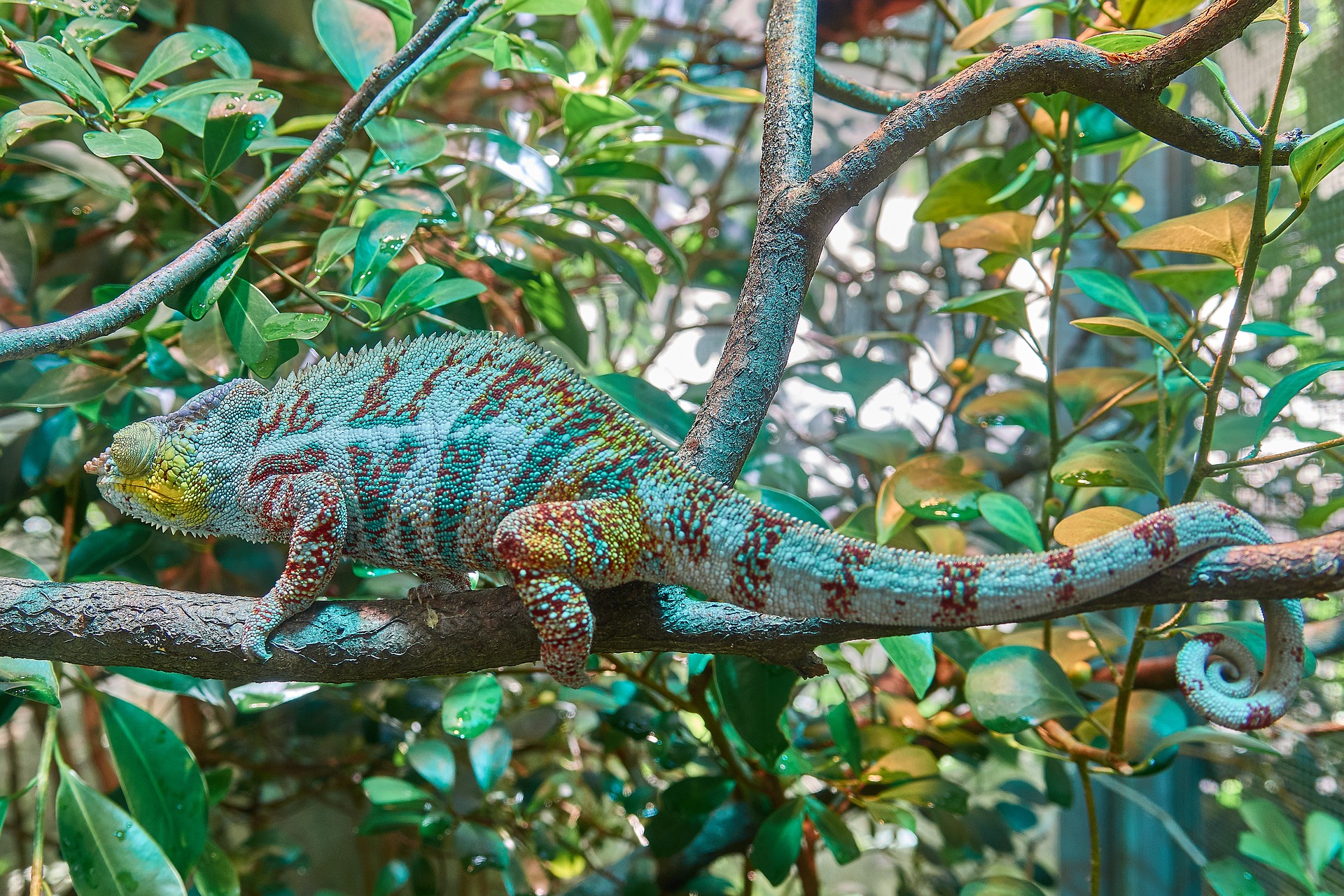
In research, demand characteristics refers to an experimental artefact where participants form an interpretation of the experiment’s purpose and unconsciously change their behaviour to fit that interpretation (Wikipedia definition)
Even if objective outcome measures are used, bias may still be introduced by non-blinded data analysts selectively using and reporting statistical tests that make the treatment appear more effective than it is. If blinding is utilised throughout the whole trial, the risk of such biases affecting the results is considerably lower.
Indeed, a systematic review of 250 RCTs in the peer-reviewed Journal of the American Medical Association provides empirical evidence to support the use of blinding in RCTs. Schulz et al. report that trials that were not double-blind produced larger estimates of effects (p=0.01), with odds ratios being exaggerated by 17%, compared to blinded trials. This highlights the risk of bias present in non-blinded trials and serves a caution to readers of trial reports to be wary of such design flaws in RCTs.
What is effective blinding?
As with anything, blinding must be applied properly if it is to be maximally effective. Hence, certain criteria should be fulfilled:
- Group allocation must be concealed successfully
- The ability to accurately measure outcomes must not be hampered
- Blinding should be applied to both participants and researchers – this includes clinicians, data collectors, outcome adjudicators and data analysts (sometimes multiple roles are performed by a single group of people)
What can be done when blinding is not possible?
Of course, we do not live in an ideal world so there are situations where blinding cannot be applied because it would be unethical, or it is simply not possible for practical reasons. For example, if the intervention being investigated is a form of surgery, it would unethical to perform ‘fake’ surgery on the control group and impossible to prevent the clinician from knowing which subjects will be undergoing surgery!
Nevertheless, certain measures can be taken to minimise any potential bias. If it is impossible to blind all of the aforementioned team members, then one should blind as many of them as possible. Returning to the example of a surgical intervention, although it is not possible to blind the operator, independent outcome assessors who are unaware of participants’ group allocations may be employed. The risk of bias would be further reduced if they assessed objective outcome measures and avoided subjective measures as much as possible. Moreover, blinding should be applied until after the statistical analyses are complete. One way to ensure this is to simply assign non-identifying labels to each group e.g. group X and Y.
Blinding is a vital design feature of RCTs and is as important as randomisation in terms of minimising bias. Notwithstanding all the alternative measures applied to make up for the lack of blinding, it is imperative that researchers acknowledge this particular limitation of their study and discuss the biases that may arise as a result in the published report.
Neelam Khan
Leave a reply cancel reply.
Your email address will not be published. Required fields are marked *
Save my name, email, and website in this browser for the next time I comment.
Subscribe to our newsletter
You will receive our monthly newsletter and free access to Trip Premium.
Related Articles
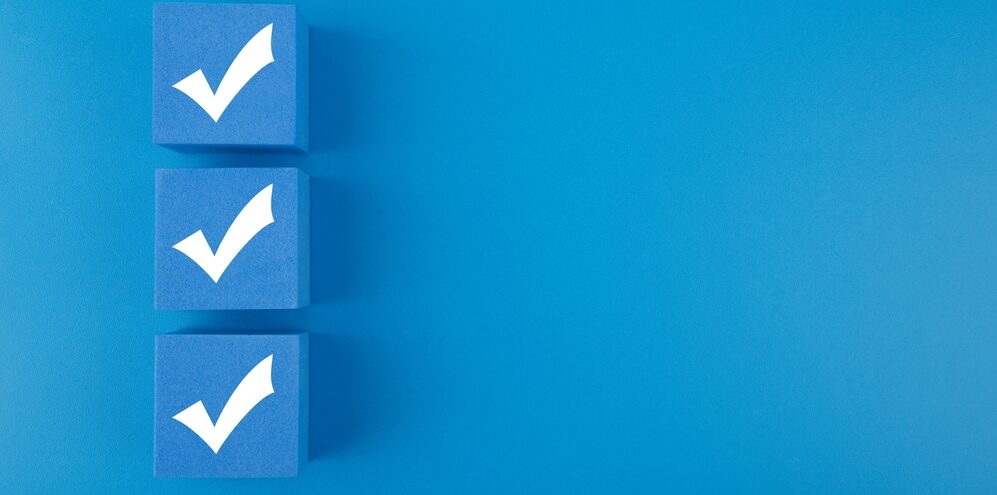
Why was the CONSORT Statement introduced?
The CONSORT statement aims at comprehensive and complete reporting of randomized controlled trials. This blog introduces you to the statement and why it is an important tool in the research world.
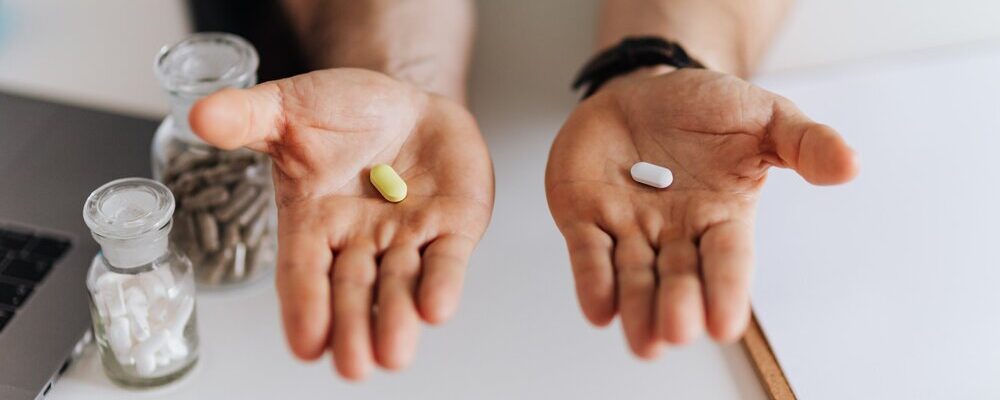
Crossover trials: what are they and what are their advantages and limitations?
This blog introduces you to crossover trials with a clear explanation and example, together with some advantages and limitations of this study design.
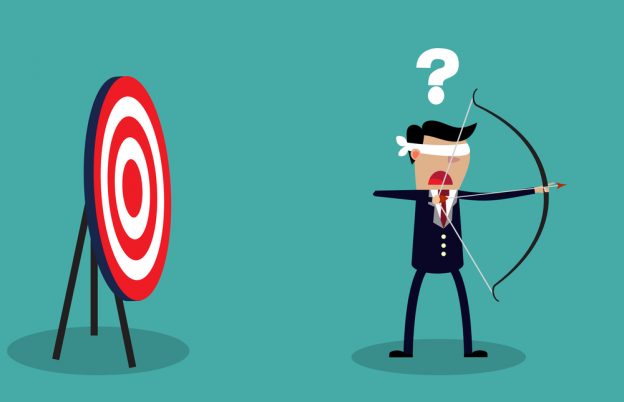
Blinding: A detailed guide for students
This blog provides a detailed overview of the concept of ‘blinding’ in randomised controlled trials (RCTs). It covers what blinding is, common methods of blinding, why blinding is important, and what researchers might do when blinding is not possible. It also explains the concept of allocation concealment.

Want to create or adapt books like this? Learn more about how Pressbooks supports open publishing practices.
7.3 Quasi-Experimental Research
Learning objectives.
- Explain what quasi-experimental research is and distinguish it clearly from both experimental and correlational research.
- Describe three different types of quasi-experimental research designs (nonequivalent groups, pretest-posttest, and interrupted time series) and identify examples of each one.
The prefix quasi means “resembling.” Thus quasi-experimental research is research that resembles experimental research but is not true experimental research. Although the independent variable is manipulated, participants are not randomly assigned to conditions or orders of conditions (Cook & Campbell, 1979). Because the independent variable is manipulated before the dependent variable is measured, quasi-experimental research eliminates the directionality problem. But because participants are not randomly assigned—making it likely that there are other differences between conditions—quasi-experimental research does not eliminate the problem of confounding variables. In terms of internal validity, therefore, quasi-experiments are generally somewhere between correlational studies and true experiments.
Quasi-experiments are most likely to be conducted in field settings in which random assignment is difficult or impossible. They are often conducted to evaluate the effectiveness of a treatment—perhaps a type of psychotherapy or an educational intervention. There are many different kinds of quasi-experiments, but we will discuss just a few of the most common ones here.
Nonequivalent Groups Design
Recall that when participants in a between-subjects experiment are randomly assigned to conditions, the resulting groups are likely to be quite similar. In fact, researchers consider them to be equivalent. When participants are not randomly assigned to conditions, however, the resulting groups are likely to be dissimilar in some ways. For this reason, researchers consider them to be nonequivalent. A nonequivalent groups design , then, is a between-subjects design in which participants have not been randomly assigned to conditions.
Imagine, for example, a researcher who wants to evaluate a new method of teaching fractions to third graders. One way would be to conduct a study with a treatment group consisting of one class of third-grade students and a control group consisting of another class of third-grade students. This would be a nonequivalent groups design because the students are not randomly assigned to classes by the researcher, which means there could be important differences between them. For example, the parents of higher achieving or more motivated students might have been more likely to request that their children be assigned to Ms. Williams’s class. Or the principal might have assigned the “troublemakers” to Mr. Jones’s class because he is a stronger disciplinarian. Of course, the teachers’ styles, and even the classroom environments, might be very different and might cause different levels of achievement or motivation among the students. If at the end of the study there was a difference in the two classes’ knowledge of fractions, it might have been caused by the difference between the teaching methods—but it might have been caused by any of these confounding variables.
Of course, researchers using a nonequivalent groups design can take steps to ensure that their groups are as similar as possible. In the present example, the researcher could try to select two classes at the same school, where the students in the two classes have similar scores on a standardized math test and the teachers are the same sex, are close in age, and have similar teaching styles. Taking such steps would increase the internal validity of the study because it would eliminate some of the most important confounding variables. But without true random assignment of the students to conditions, there remains the possibility of other important confounding variables that the researcher was not able to control.
Pretest-Posttest Design
In a pretest-posttest design , the dependent variable is measured once before the treatment is implemented and once after it is implemented. Imagine, for example, a researcher who is interested in the effectiveness of an antidrug education program on elementary school students’ attitudes toward illegal drugs. The researcher could measure the attitudes of students at a particular elementary school during one week, implement the antidrug program during the next week, and finally, measure their attitudes again the following week. The pretest-posttest design is much like a within-subjects experiment in which each participant is tested first under the control condition and then under the treatment condition. It is unlike a within-subjects experiment, however, in that the order of conditions is not counterbalanced because it typically is not possible for a participant to be tested in the treatment condition first and then in an “untreated” control condition.
If the average posttest score is better than the average pretest score, then it makes sense to conclude that the treatment might be responsible for the improvement. Unfortunately, one often cannot conclude this with a high degree of certainty because there may be other explanations for why the posttest scores are better. One category of alternative explanations goes under the name of history . Other things might have happened between the pretest and the posttest. Perhaps an antidrug program aired on television and many of the students watched it, or perhaps a celebrity died of a drug overdose and many of the students heard about it. Another category of alternative explanations goes under the name of maturation . Participants might have changed between the pretest and the posttest in ways that they were going to anyway because they are growing and learning. If it were a yearlong program, participants might become less impulsive or better reasoners and this might be responsible for the change.
Another alternative explanation for a change in the dependent variable in a pretest-posttest design is regression to the mean . This refers to the statistical fact that an individual who scores extremely on a variable on one occasion will tend to score less extremely on the next occasion. For example, a bowler with a long-term average of 150 who suddenly bowls a 220 will almost certainly score lower in the next game. Her score will “regress” toward her mean score of 150. Regression to the mean can be a problem when participants are selected for further study because of their extreme scores. Imagine, for example, that only students who scored especially low on a test of fractions are given a special training program and then retested. Regression to the mean all but guarantees that their scores will be higher even if the training program has no effect. A closely related concept—and an extremely important one in psychological research—is spontaneous remission . This is the tendency for many medical and psychological problems to improve over time without any form of treatment. The common cold is a good example. If one were to measure symptom severity in 100 common cold sufferers today, give them a bowl of chicken soup every day, and then measure their symptom severity again in a week, they would probably be much improved. This does not mean that the chicken soup was responsible for the improvement, however, because they would have been much improved without any treatment at all. The same is true of many psychological problems. A group of severely depressed people today is likely to be less depressed on average in 6 months. In reviewing the results of several studies of treatments for depression, researchers Michael Posternak and Ivan Miller found that participants in waitlist control conditions improved an average of 10 to 15% before they received any treatment at all (Posternak & Miller, 2001). Thus one must generally be very cautious about inferring causality from pretest-posttest designs.
Does Psychotherapy Work?
Early studies on the effectiveness of psychotherapy tended to use pretest-posttest designs. In a classic 1952 article, researcher Hans Eysenck summarized the results of 24 such studies showing that about two thirds of patients improved between the pretest and the posttest (Eysenck, 1952). But Eysenck also compared these results with archival data from state hospital and insurance company records showing that similar patients recovered at about the same rate without receiving psychotherapy. This suggested to Eysenck that the improvement that patients showed in the pretest-posttest studies might be no more than spontaneous remission. Note that Eysenck did not conclude that psychotherapy was ineffective. He merely concluded that there was no evidence that it was, and he wrote of “the necessity of properly planned and executed experimental studies into this important field” (p. 323). You can read the entire article here:
http://psychclassics.yorku.ca/Eysenck/psychotherapy.htm
Fortunately, many other researchers took up Eysenck’s challenge, and by 1980 hundreds of experiments had been conducted in which participants were randomly assigned to treatment and control conditions, and the results were summarized in a classic book by Mary Lee Smith, Gene Glass, and Thomas Miller (Smith, Glass, & Miller, 1980). They found that overall psychotherapy was quite effective, with about 80% of treatment participants improving more than the average control participant. Subsequent research has focused more on the conditions under which different types of psychotherapy are more or less effective.

In a classic 1952 article, researcher Hans Eysenck pointed out the shortcomings of the simple pretest-posttest design for evaluating the effectiveness of psychotherapy.
Wikimedia Commons – CC BY-SA 3.0.
Interrupted Time Series Design
A variant of the pretest-posttest design is the interrupted time-series design . A time series is a set of measurements taken at intervals over a period of time. For example, a manufacturing company might measure its workers’ productivity each week for a year. In an interrupted time series-design, a time series like this is “interrupted” by a treatment. In one classic example, the treatment was the reduction of the work shifts in a factory from 10 hours to 8 hours (Cook & Campbell, 1979). Because productivity increased rather quickly after the shortening of the work shifts, and because it remained elevated for many months afterward, the researcher concluded that the shortening of the shifts caused the increase in productivity. Notice that the interrupted time-series design is like a pretest-posttest design in that it includes measurements of the dependent variable both before and after the treatment. It is unlike the pretest-posttest design, however, in that it includes multiple pretest and posttest measurements.
Figure 7.5 “A Hypothetical Interrupted Time-Series Design” shows data from a hypothetical interrupted time-series study. The dependent variable is the number of student absences per week in a research methods course. The treatment is that the instructor begins publicly taking attendance each day so that students know that the instructor is aware of who is present and who is absent. The top panel of Figure 7.5 “A Hypothetical Interrupted Time-Series Design” shows how the data might look if this treatment worked. There is a consistently high number of absences before the treatment, and there is an immediate and sustained drop in absences after the treatment. The bottom panel of Figure 7.5 “A Hypothetical Interrupted Time-Series Design” shows how the data might look if this treatment did not work. On average, the number of absences after the treatment is about the same as the number before. This figure also illustrates an advantage of the interrupted time-series design over a simpler pretest-posttest design. If there had been only one measurement of absences before the treatment at Week 7 and one afterward at Week 8, then it would have looked as though the treatment were responsible for the reduction. The multiple measurements both before and after the treatment suggest that the reduction between Weeks 7 and 8 is nothing more than normal week-to-week variation.
Figure 7.5 A Hypothetical Interrupted Time-Series Design

The top panel shows data that suggest that the treatment caused a reduction in absences. The bottom panel shows data that suggest that it did not.
Combination Designs
A type of quasi-experimental design that is generally better than either the nonequivalent groups design or the pretest-posttest design is one that combines elements of both. There is a treatment group that is given a pretest, receives a treatment, and then is given a posttest. But at the same time there is a control group that is given a pretest, does not receive the treatment, and then is given a posttest. The question, then, is not simply whether participants who receive the treatment improve but whether they improve more than participants who do not receive the treatment.
Imagine, for example, that students in one school are given a pretest on their attitudes toward drugs, then are exposed to an antidrug program, and finally are given a posttest. Students in a similar school are given the pretest, not exposed to an antidrug program, and finally are given a posttest. Again, if students in the treatment condition become more negative toward drugs, this could be an effect of the treatment, but it could also be a matter of history or maturation. If it really is an effect of the treatment, then students in the treatment condition should become more negative than students in the control condition. But if it is a matter of history (e.g., news of a celebrity drug overdose) or maturation (e.g., improved reasoning), then students in the two conditions would be likely to show similar amounts of change. This type of design does not completely eliminate the possibility of confounding variables, however. Something could occur at one of the schools but not the other (e.g., a student drug overdose), so students at the first school would be affected by it while students at the other school would not.
Finally, if participants in this kind of design are randomly assigned to conditions, it becomes a true experiment rather than a quasi experiment. In fact, it is the kind of experiment that Eysenck called for—and that has now been conducted many times—to demonstrate the effectiveness of psychotherapy.
Key Takeaways
- Quasi-experimental research involves the manipulation of an independent variable without the random assignment of participants to conditions or orders of conditions. Among the important types are nonequivalent groups designs, pretest-posttest, and interrupted time-series designs.
- Quasi-experimental research eliminates the directionality problem because it involves the manipulation of the independent variable. It does not eliminate the problem of confounding variables, however, because it does not involve random assignment to conditions. For these reasons, quasi-experimental research is generally higher in internal validity than correlational studies but lower than true experiments.
- Practice: Imagine that two college professors decide to test the effect of giving daily quizzes on student performance in a statistics course. They decide that Professor A will give quizzes but Professor B will not. They will then compare the performance of students in their two sections on a common final exam. List five other variables that might differ between the two sections that could affect the results.
Discussion: Imagine that a group of obese children is recruited for a study in which their weight is measured, then they participate for 3 months in a program that encourages them to be more active, and finally their weight is measured again. Explain how each of the following might affect the results:
- regression to the mean
- spontaneous remission
Cook, T. D., & Campbell, D. T. (1979). Quasi-experimentation: Design & analysis issues in field settings . Boston, MA: Houghton Mifflin.
Eysenck, H. J. (1952). The effects of psychotherapy: An evaluation. Journal of Consulting Psychology, 16 , 319–324.
Posternak, M. A., & Miller, I. (2001). Untreated short-term course of major depression: A meta-analysis of studies using outcomes from studies using wait-list control groups. Journal of Affective Disorders, 66 , 139–146.
Smith, M. L., Glass, G. V., & Miller, T. I. (1980). The benefits of psychotherapy . Baltimore, MD: Johns Hopkins University Press.
Research Methods in Psychology Copyright © 2016 by University of Minnesota is licensed under a Creative Commons Attribution-NonCommercial-ShareAlike 4.0 International License , except where otherwise noted.
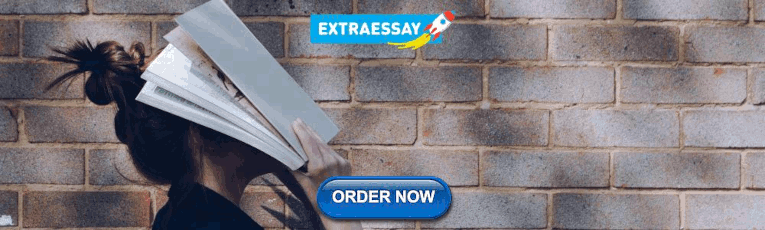
IMAGES
VIDEO
COMMENTS
Does random assignment eliminate confounding variables? Yes, random assignment eliminates the influence of any confounding variables on the treatment because it distributes them at random among the study groups. Randomization invalidates any relationship between a confounding variable and the treatment.
Abstract. A Confounder is a variable whose presence affects the variables being studied so that the results do not reflect the actual relationship. There are various ways to exclude or control confounding variables including Randomization, Restriction and Matching. But all these methods are applicable at the time of study design.
Correlation, Causation, and Confounding Variables. Random assignment helps you separation causation from correlation and rule out confounding variables. As a critical component of the scientific method, experiments typically set up contrasts between a control group and one or more treatment groups. The idea is to determine whether the effect, which is the difference between a treatment group ...
Why does random assignment matter? Random assignment is an important part of control in experimental research, because it helps strengthen the internal validity of an experiment and avoid biases.. In experiments, researchers manipulate an independent variable to assess its effect on a dependent variable, while controlling for other variables. To do so, they often use different levels of an ...
Confounding variables (a.k.a. confounders or confounding factors) are a type of extraneous variable that are related to a study's independent and dependent variables. A variable must meet two conditions to be a confounder: It must be correlated with the independent variable. This may be a causal relationship, but it does not have to be.
Random assignment controls for confounding due to both measurable and unmeasurable causes. So it is especially useful when confounding variables are unknown or cannot be measured. It also controls for time-varying confounders, that is when the exposure and the confounders are measured repeatedly in studies where participants are followed over ...
Confounding variables or confounders are often defined as the variables correlate (positively or negatively) with both the dependent variable and the independent variable. ... In randomization the random assignment of study subjects to exposure categories to breaking any links between exposure and confounders. This reduces potential for ...
Random assignment. Random assignment is the single most powerful control technique we can use to minimize the potential threats of the confounding variables in research design. As we have seen in Dunn and her colleagues' study earlier, participants are not allowed to self select into either conditions (spend $20 on self or spend on others).
Random assignment eliminates the influence of the confounding variables on the treatment since it distributes them at random between the study groups, therefore, ruling out this alternative path or explanation of the outcome. 3. Random assignment also eliminates other threats to internal validity. By distributing all threats (known and unknown ...
The use of random assignment cannot eliminate this possibility, but it greatly reduces it. ... Because the groups were randomly assigned, it is not plausible to say that the groups are different with respect to any confounding variable. Random assignment helps us rule out plausible alternatives. 3.6.1.4 Making a causal claim.
The Definition of Random Assignment According to Psychology. Random assignment refers to the use of chance procedures in psychology experiments to ensure that each participant has the same opportunity to be assigned to any given group in a study to eliminate any potential bias in the experiment at the outset. Participants are randomly assigned ...
Random assignment produces groups that are, in theory, equal at the start of the experiment. This controls for confounding variables that may skew results. Random assignment in psychology produces ...
How to Reduce the Effect of Confounding Variables. There are several ways to reduce the effect of confounding variables, including the following methods: 1. Random Assignment. Random assignment refers to the process of randomly assigning individuals in a study to either a treatment group or a control group.
A confounding variable is related to both the supposed cause and the supposed effect of the study. It can be difficult to separate the true effect of the independent variable from the effect of the confounding variable. In your research design, it's important to identify potential confounding variables and plan how you will reduce their impact.
that random assignment does a good job of distributing participants who share a particular quality relatively equally between groups. After a short experiment, the teacher discusses confounding variables with the class. Random assignment can eliminate these potential confounds and using a
Random assignment of participants to the different groups only helps to eliminate confounding variables present at the time of randomisation, thereby reducing selection bias. It does not, however, prevent differences from developing between the groups afterwards. This is where blinding plays a role.
Random assignment of patients to comparison groups stochastically tends, with increasing sample size or number of experiment replications, to minimize the confounding of treatment outcome differences by the effects of differences among these groups in unknown/unmeasured patient characteristics. To what degree such confounding is actually avoided we cannot know unless we have validly measured ...
Random assignment or random placement is an experimental technique for assigning human participants or animal subjects to different groups in an experiment (e.g., a treatment group versus a control group) using randomization, such as by a chance procedure (e.g., flipping a coin) or a random number generator. This ensures that each participant or subject has an equal chance of being placed in ...
It does not eliminate the problem of confounding variables, however, because it does not involve random assignment to conditions. For these reasons, quasi-experimental research is generally higher in internal validity than correlational studies but lower than true experiments.
However, it does not eliminate the problem of confounding variables, because it does not involve random assignment to conditions or counterbalancing. For these reasons, quasi-experimental research is generally higher in internal validity than non-experimental studies but lower than true experiments.
Random assignment fails to fulfill its function of balancing potential confounders when, after randomization, experimental conditions non-trivially differ on one or more confounding variables—which would raise the concern that this inequality explains observed differences between experimental conditions.
4. Random effects are confounding variables if the variables they represent cause selection into the exposure and variation in the outcome. For example, if what school a student attends both affects their propensity to be suspended and their academic success, then a random effect varying by school may account for this confounding relationship.
None of the other statements are true, (D) Random assignment should eliminate subject-relevant confounding variables (e.g., conscientiousness, IQ, hair color). ... Random assignment does not relate to sampling or validity and has no impact on the need for statistics, (A) Charlotte and Tamar's way of measuring friendliness is not reliable ...