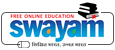
- Review Assignment
- Announcements
- About the Course
- Explore Courses
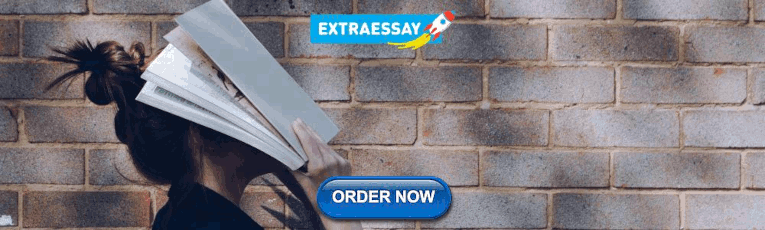
Python for Data Science : Result Published!!
- Log in to your account and navigate to the "Download E-certificate" section.
- Next to "Download E-certificate," you'll find another tab labeled "Share your experience." Click on it.
- Answer the provided questions about your experience.
- Optionally, you can add your picture or skip this step.
- An AI-assisted post will be generated for you. You can share this post on your social media platforms.
Python for Data Science : Final Feedback Form !!!
Dear students, We are glad that you have attended the NPTEL online certification course. We hope you found the NPTEL Online course useful and have started using NPTEL extensively. In this regard, we would like to have feedback from you regarding our course and whether there are any improvements, you would like to suggest. We are enclosing an online feedback form and would request you to spare some of your valuable time to input your observations. Your esteemed input will help us in serving you better. The link to give your feedback is: https://docs.google.com/forms/d/1WtZsDKHnfaYr8oGHL1Jqld7ydBJTnwCwRWezAXFJl50/viewform We thank you for your valuable time and feedback. Thanks & Regards, -NPTEL Team
March 2024 NPTEL Exams - Hall Tickets Released!
.jpg)
Exam Format - March, 2024!!
Dear Candidate, ****This is applicable only for the exam registered candidates**** Type of exam will be available in the list: Click Here You will have to appear at the allotted exam center and produce your Hall ticket and Government Photo Identification Card (Example: Driving License, Passport, PAN card, Voter ID, Aadhaar-ID with your Name, date of birth, photograph and signature) for verification and take the exam in person. You can find the final allotted exam center details in the hall ticket. The hall ticket is yet to be released. We will notify the same through email and SMS. Type of exam: Computer based exam (Please check in the above list corresponding to your course name) The questions will be on the computer and the answers will have to be entered on the computer; type of questions may include multiple choice questions, fill in the blanks, essay-type answers, etc. Type of exam: Paper and pen Exam (Please check in the above list corresponding to your course name) The questions will be on the computer. You will have to write your answers on sheets of paper and submit the answer sheets. Papers will be sent to the faculty for evaluation. On-Screen Calculator Demo Link: Kindly use the below link to get an idea of how the On-screen calculator will work during the exam. https://tcsion.com/ OnlineAssessment/ ScientificCalculator/ Calculator.html NOTE: Physical calculators are not allowed inside the exam hall. Thank you! -NPTEL Team
Python for Data Science : Solution for Assignment 4 released !!
Dear Learner, The Solution for Assignment 4 have been uploaded for the course "Python for Data Science". The solution set can be accessed using the following link: Assignment 4 solution: https://onlinecourses.nptel.ac.in/noc24_cs54/unit?unit=56&lesson=140 Please use the discussion forum if you have any queries. Thanks & Regards, NPTEL Team.
Reminder: NPTEL: Exam Registration is date is extended for Jan 2024 courses!
Dear Learner, The exam registration for the Jan 2024 NPTEL course certification exam is extended till February 20, 2024 - 05.00 P.M . CLICK HERE to register for the exam Choose from the Cities where exam will be conducted: Exam Cities Click here to view Timeline and Guideline : Guideline For further details on registration process please refer the previous announcement in the course page. -NPTEL Team
Python for Data Science : Solution for Assignment 3 released !!
Dear Learner, The Solution for Assignment 3 have been uploaded for the course "Python for Data Science". The solution set can be accessed using the following link: Assignment 3 solution: https://onlinecourses.nptel.ac.in/noc24_cs54/unit?unit=41&lesson=125 Please use the discussion forum if you have any queries. Thanks & Regards, NPTEL Team.
Week 4 Feedback Form: Python for Data Science!!
Dear Learners, Thank you for continuing with the course and hope you are enjoying it. We would like to know if the expectations with which you joined this course are being met and hence please do take 2 minutes to fill out our weekly feedback form. It would help us tremendously in gauging the learner experience. Here is the link to the form: https://docs.google.com/forms/d/1y81yHE1ipGTZiTk55_JHWnV2ByKbEB3foyHcpzE7diY/viewform Thank you -NPTEL team
Python for Data Science : Week 4 Supplementary Materials!!
Dear Learners, Please go through the lectures in "Supplementary material for week 4", as there will be questions asked in the assignment for week 4 as well as in the final exam. Have fun learning. Thanks & Regards NPTEL team
Python for Data Science : Solution for Assignment 1&2 released !!
Dear Learner, The Solution for Assignment 1&2 have been uploaded for the course "Python for Data Science". The solution set can be accessed using the following link: Assignment 1 solution: https://onlinecourses.nptel.ac.in/noc24_cs54/unit?unit=18&lesson=123 Assignment 2 solution: https://onlinecourses.nptel.ac.in/noc24_cs54/unit?unit=30&lesson=124 Please use the discussion forum if you have any queries. Thanks & Regards, NPTEL Team.
Python for Data Science : Assignment 4 is live now!!
Dear Learners, The lecture videos for Week 4 have been uploaded for the course "Python for Data Science" . The lectures can be accessed using the following link: Link: https://onlinecourses.nptel.ac.in/noc24_cs54/unit?unit=56&lesson=57 The other lectures in this week are accessible from the navigation bar to the left. Please remember to login into the website to view contents (if you aren't logged in already). Practice Assignment-4 for Week-4 is also released and can be accessed from the following link Link: https://onlinecourses.nptel.ac.in/noc24_cs54/unit?unit=56&assessment=134 Assignment-4 for Week-4 is also released and can be accessed from the following link Link: https://onlinecourses.nptel.ac.in/noc24_cs54/unit?unit=56&assessment=139 The assignment has to be submitted on or before Wednesday,[21/02/2024], 23:59 IST. As we have done so far, please use the discussion forums if you have any questions on this module. Note : Please check the due date of the assignments in the announcement and assignment page if you see any mismatch write to us immediately. Thanks and Regards, -NPTEL Team
Week 3 Feedback Form: Python for Data Science!!
Python for data science: reminder for assignment 1 & 2 deadline.
Dear Learners, The Deadline for Assignments 1 & 2 will close on Wednesday,[07/02/2024], 23:59 IST. Kindly submit the assignments before the deadline. Thanks and Regards, -NPTEL Team
Python for Data Science : Assignment 3 is live now!!
Dear Learners, The lecture videos for Week 3 have been uploaded for the course "Python for Data Science" . The lectures can be accessed using the following link: Link: https://onlinecourses.nptel.ac.in/noc24_cs54/unit?unit=41&lesson=42 The other lectures in this week are accessible from the navigation bar to the left. Please remember to login into the website to view contents (if you aren't logged in already). Practice Assignment-3 for Week-3 is also released and can be accessed from the following link Link: https://onlinecourses.nptel.ac.in/noc24_cs54/unit?unit=41&assessment=133 Assignment-3 for Week-3 is also released and can be accessed from the following link Link: https://onlinecourses.nptel.ac.in/noc24_cs54/unit?unit=41&assessment=137 The assignment has to be submitted on or before Wednesday,[14/02/2024], 23:59 IST. As we have done so far, please use the discussion forums if you have any questions on this module. Note : Please check the due date of the assignments in the announcement and assignment page if you see any mismatch write to us immediately. Thanks and Regards, -NPTEL Team
Week 2 Feedback Form: Python for Data Science!!
Reminder: nptel: exam registration is open now for jan 2024 courses.
Dear Learner,
Here is the much-awaited announcement on registering for the Jan 2024 NPTEL course certification exam.
1. The registration for the certification exam is open only to those learners who have enrolled in the course.
2. If you want to register for the exam for this course, login here using the same email id which you had used to enroll to the course in Swayam portal. Please note that Assignments submitted through the exam registered email id ALONE will be taken into consideration towards final consolidated score & certification.
3 . Date of exam: Mar 24, 2024
CLICK HERE to register for the exam.
Choose from the Cities where exam will be conducted: Exam Cities
4. Exam fees:
If you register for the exam and pay before Feb 12, 2024 - 5:00 PM, Exam fees will be Rs. 1000/- per exam .
5. 50% fee waiver for the following categories:
Students belonging to the SC/ST category: please select Yes for the SC/ST option and upload the correct Community certificate.
Students belonging to the PwD category with more than 40% disability: please select Yes for the option and upload the relevant Disability certificate.
6. Last date for exam registration: Feb 16, 2024 - 5:00 PM (Friday).
7. Between Feb 12, 2024 - 5:00 PM & Feb 16, 2024 - 5:00 PM late fee will be applicable.
8. Mode of payment: Online payment - debit card/credit card/net banking/UPI.
9. HALL TICKET:
The hall ticket will be available for download tentatively by 2 weeks prior to the exam date. We will confirm the same through an announcement once it is published.
10. FOR CANDIDATES WHO WOULD LIKE TO WRITE MORE THAN 1 COURSE EXAM:- you can add or delete courses and pay separately – till the date when the exam form closes. Same day of exam – you can write exams for 2 courses in the 2 sessions. Same exam center will be allocated for both the sessions.
11. Data changes:
Last date for data changes: Feb 16, 2024 - 5:00 PM :
We will charge an additional fee of Rs. 200 to make any changes related to name, DOB, photo, signature, SC/ST and PWD certificates after the last date of data changes.
The following 6 fields can be changed (until the form closes) ONLY when there are NO courses in the course cart. And you will be able to edit those fields only if you: -
REMOVE unpaid courses from the cart And/or - CANCEL paid courses
1. Do you come under the SC/ST category? *
2. SC/ST Proof
3. Are you a person with disabilities? *
4. Are you a person with disabilities above 40%?
5. Disabilities Proof
6. What is your role?
Note: Once you remove or cancel a course, you will be able to edit these fields immediately.
But, for cancelled courses, refund of fees will be initiated only after 2 weeks.
12. LAST DATE FOR CANCELLING EXAMS and getting a refund: Feb 16, 2024 - 5:00 PM
13. Click here to view Timeline and Guideline : Guideline
Domain Certification
Domain Certification helps learners to gain expertise in a specific Area/Domain. This can be helpful for learners who wish to work in a particular area as part of their job or research or for those appearing for some competitive exam or becoming job ready or specialising in an area of study.
Every domain will comprise Core courses and Elective courses. Once a learner completes the requisite courses as per the mentioned criteria, you will receive a Domain Certificate showcasing your scores and the domain of expertise. Kindly refer to the following link for the list of courses available under each domain: https://nptel.ac.in/domains
Outside India Candidates
Candidates who are residing outside India may also fill the exam form and pay the fees. Mode of exam and other details will be communicated to you separately.
Thanks & Regards,
Python for Data Science : Assignment 2 is live now!!
Dear Learners, The lecture videos for Week 2 have been uploaded for the course "Python for Data Science" . The lectures can be accessed using the following link: Link: https://onlinecourses.nptel.ac.in/noc24_cs54/unit?unit=30&lesson=31 The other lectures in this week are accessible from the navigation bar to the left. Please remember to login into the website to view contents (if you aren't logged in already). Practice Assignment-2 for Week-2 is also released and can be accessed from the following link Link: https://onlinecourses.nptel.ac.in/noc24_cs54/unit?unit=30&assessment=132 Assignment-2 for Week-2 is also released and can be accessed from the following link Link: https://onlinecourses.nptel.ac.in/noc24_cs54/unit?unit=30&assessment=136 The assignment has to be submitted on or before Wednesday,[07/02/2024], 23:59 IST. As we have done so far, please use the discussion forums if you have any questions on this module. Note : Please check the due date of the assignments in the announcement and assignment page if you see any mismatch write to us immediately. Thanks and Regards, -NPTEL Team
Week 1 Feedback Form: Python for Data Science!!
Python for data science : assignment 1 is live now.
Dear Learners, The lecture videos for Week 1 have been uploaded for the course "Python for Data Science" . The lectures can be accessed using the following link: Link: https://onlinecourses.nptel.ac.in/noc24_cs54/unit?unit=18&lesson=19 The other lectures in this week are accessible from the navigation bar to the left. Please remember to login into the website to view contents (if you aren't logged in already). Practice Assignment-1 for Week-1 is also released and can be accessed from the following link Link: https://onlinecourses.nptel.ac.in/noc24_cs54/unit?unit=18&assessment=131 Assignment-1 for Week-1 is also released and can be accessed from the following link Link: https://onlinecourses.nptel.ac.in/noc24_cs54/unit?unit=18&assessment=135 The assignment has to be submitted on or before Wednesday,[07/02/2024], 23:59 IST. As we have done so far, please use the discussion forums if you have any questions on this module. Note : Please check the due date of the assignments in the announcement and assignment page if you see any mismatch write to us immediately. Thanks and Regards, -NPTEL Team
Python for Data Science : Assignment 0 is live now!!
Dear Learners, We welcome you all to this course "Python for Data Science" . The assignment 0 has been released. This assignment is based on a prerequisite of the course. You can find the assignment in the link : https://onlinecourses.nptel.ac.in/noc24_cs54/unit?unit=16&assessment=130 Please note that this assignment is for practice and it will not be graded. Thanks & Regards -NPTEL Team
NPTEL: Exam Registration is open now for Jan 2024 courses!
Python for data science: welcome to nptel online course - jan 2024.
- Every week, about 2.5 to 4 hours of videos containing content by the Course instructor will be released along with an assignment based on this. Please watch the lectures, follow the course regularly and submit all assessments and assignments before the due date. Your regular participation is vital for learning and doing well in the course. This will be done week on week through the duration of the course.
- Please do the assignments yourself and even if you take help, kindly try to learn from it. These assignments will help you prepare for the final exams. Plagiarism and violating the Honor Code will be taken very seriously if detected during the submission of assignments.
- The announcement group - will only have messages from course instructors and teaching assistants - regarding the lessons, assignments, exam registration, hall tickets, etc.
- The discussion forum (Ask a question tab on the portal) - is for everyone to ask questions and interact. Anyone who knows the answers can reply to anyone's post and the course instructor/TA will also respond to your queries.
- Please make maximum use of this feature as this will help you learn much better.
- If you have any questions regarding the exam, registration, hall tickets, results, queries related to the technical content in the lectures, any doubts in the assignments, etc can be posted in the forum section
- The course is free to enroll and learn from. But if you want a certificate, you have to register and write the proctored exam conducted by us in person at any of the designated exam centres.
- The exam is optional for a fee of Rs 1000/- (Rupees one thousand only).
- Date and Time of Exams: March 24, 2024 Morning session 9am to 12 noon; Afternoon Session 2 pm to 5 pm.
- Registration URL: Announcements will be made when the registration form is open for registrations.
- The online registration form has to be filled and the certification exam fee needs to be paid. More details will be made available when the exam registration form is published. If there are any changes, it will be mentioned then.
- Please check the form for more details on the cities where the exams will be held, the conditions you agree to when you fill the form etc.
- Once again, thanks for your interest in our online courses and certification. Happy learning.
A project of

In association with

A Practical Guide to Python for Data Science

- data analysis
- data science
Working on real data science projects is a rewarding experience. But how do you get to the point where you can make a real contribution? What skills and experience do you need? What challenges might occur along the way? In this article, we’ll address all these questions.
Data has become ubiquitous in our modern world. It’s generated from many sources, including social media, IoT devices, business transactions, finance, government and public records, academic research, communication systems, and even satellites and remote sensing technology. Some estimates suggest that 90% of the world’s data has been generated in the previous two years alone , with over 300 million terabytes being created every day! How is it possible to understand and draw insights from all this data?
This is the job of a data scientist. In this article, we’ll give you an overview of how to become a data scientist, what a data scientist actually does, and what tools they use. Hint: It’s Python! Python has become an indispensable tool in the tech world for many reasons , and it’s particularly powerful for data science projects.
If you’re new to Python and are looking for some hands-on learning material, consider taking our Python Basics track; it combines three beginner-friendly courses to get you on your feet. For more in-depth material, the Learn Programming with Python track bundles together 5 interactive courses and includes 135 interactive coding challenges. There has never been a better time to learn Python than in 2024 .
A Brief History of Data Science
The roots of data science lie in the fields of statistics and computer science. In the 1960s and 1970s, statisticians and computer scientists began working on methods to analyze and interpret different kinds of datasets. However, it wasn't until the recent growth of digital data that the term "data science" emerged.
In the early 2000s, William S. Cleveland created an action plan to expand the field of statistics to incorporate data analysis. The report, titled Data Science: An Action Plan for Expanding the Technical Areas of the Field of Statistics is often credited with popularizing the term and emphasizing the interdisciplinary nature of the field.

Today, data science encompasses a broad range of techniques and approaches to extract valuable insights from complex and vast datasets. Modern data scientists are responsible for collecting and cleaning data and then analyzing it to uncover patterns, trends, and actionable insights. They use a combination of statistical methods, machine learning algorithms, and domain knowledge to make informed decisions and predictions. Data scientists work across various industries – including finance, healthcare, technology, and more.
Key skills for a data scientist include proficiency in programming languages like Python, experience with data manipulation and visualization tools, a strong understanding of statistical concepts, and the ability to communicate findings effectively to both technical and non-technical people. The role of a data scientist continues to evolve with advancements in technology and the increasing importance of data-driven decision-making in various sectors.
The Path to Becoming a Data Scientist
The ways to become a data scientist are as varied as the datasets you can be expected to analyze. My personal journey began with a degree in mathematics and physics, where I discovered a love of research. This led to a PhD where I was required to learn programming with Python and was expected to start working with real-world data of atmospheric measurements. It was here I discovered a knack for using Python to do statistical analyses of different datasets . It was also my first exposure to machine learning – a powerful tool used to find hidden patterns in data. After a position as a postdoctoral researcher, I found my way into industry, where I worked as a professional data scientist.
However, there is not necessarily a typical path to becoming a data scientist. It usually involves a combination of educational background, specific subjects, and a diverse set of skills. Many data scientists hold a bachelor's degree or an advanced degree in fields like computer science, statistics, mathematics, or a related quantitative discipline. Relevant coursework may include statistics, machine learning, data analysis, and programming. Proficiency in programming languages like Python is crucial, and any subjects that expose you to data manipulation and visualization are valuable.
Strong analytical and problem-solving skills are essential, as data scientists need to extract meaningful insights from complex datasets. It’s not always clear which questions to ask, which techniques to use, and which tools to reach for. Additionally, effective communication skills are vital to convey findings to non-technical people. Continuous learning is also crucial in this dynamic field, as technologies are continuously evolving.
A great way to stand out among any group of people with diverse skills is by gaining hands-on experience through projects, internships, or online data science competitions, which can enhance practical skills. You can download your own dataset and start practicing data analysis in Python or take part in data science challenges . Certifications in data science and participation in the open-source community further provide experience working on real-world problems.
Python in Real-World Data Science
Data science isn’t just about writing Python code to handle data, develop predictive models, and produce nice visualizations. It has to have real-world impact. Data science matters because it empowers organizations to turn raw data into actionable insights, driving informed decision-making. In various domains – from healthcare and finance to marketing and technology – data science plays a crucial role in optimizing processes, predicting trends, and solving complex problems.
In healthcare, for example, data science has made a significant impact in the interpretation of medical images. Healthcare professionals rely on images from X-rays, MRIs, and CAT scans to get an idea of what’s happening inside a patient’s body. However, the interpretation of these images is done by humans, who could miss identifying microscopic features. Machine learning models can be trained on huge datasets of medical images and be used to automatically identify any areas of concern.
In manufacturing, data science contributes to improving product quality by analyzing data from production processes to identify factors influencing product defects and variability. Data might include physical measurements from sensors in the production process (such as temperature, pressure, and vibration) as well as quantitative or qualitative estimates of product quality. By leveraging techniques such as statistical analysis and anomaly detection, manufacturers can detect deviations from optimal operating conditions and take corrective actions in real time to ensure consistent product quality.
For numerous use cases like these, Python is an indispensable tool because of its versatility and readability. The number of open-source Python libraries – which contain extra functionality outside of the usual Python built-in functions and can be imported into your programs – makes Python incredibly useful in data science. For working with medical images, open-cv can be used to process and analyze many types of image files. For statistical analysis and anomaly detection, libraries such as pandas , NumPy , and scikit-learn are indispensable.
Python Libraries for Data Science
In the previous section, we mentioned some common Python libraries for data science. These have also appeared in our article Top 15 Python Libraries for Data Science . Libraries like pandas , NumPy , SciPy , Matplotlib , and scikit-learn form the backbone of Python-based data science projects. During the technical development of a project, these libraries are often used daily.
The pandas library offers powerful data structures and functions for data manipulation and analysis, making tasks like cleaning , filtering, and transforming datasets efficient and intuitive. And although it’s a standalone tool, SQL is also important when working with large datasets.
NumPy provides support for numerical computing with arrays, enabling fast and efficient operations on large datasets. SciPy complements NumPy by offering a wide range of scientific computing functions, including optimization, integration, and interpolation. Matplotlib facilitates the creation of high-quality visualizations, which are crucial for exploring and communicating insights from data. Lastly, scikit-learn offers a comprehensive suite of machine learning algorithms and tools for tasks such as classification, regression, clustering, and dimensionality reduction, allowing data scientists to build and deploy predictive models with ease.
Python's capability in data handling and manipulation can be invaluable in various data science projects. In a project involving the sentiment analysis of customer reviews, Python can be used to clean and preprocess text data, removing noise and extracting relevant features via libraries like pandas and NLTK (Natural Language Toolkit) . Exploratory data analysis (EDA) can be performed using Matplotlib and Seaborn . This allows data scientists to visualize patterns and trends in the data, aiding in the identification of different sentiments in text data and the key themes being described.
Data visualization plays an important role in data science. It’s a powerful tool for understanding complex datasets, communicating insights, and guiding decision-making. We already mentioned popular libraries like Matplotlib and Seaborn. These allow data scientists to effectively communicate their findings, facilitate collaboration across teams, and drive informed decision-making in various domains.
A Real-World Python for Data Science Example
For a real-world example of using Python for data science, consider a dataset of atmospheric soundings which we downloaded and prepared in the article 7 Datasets to Practice Data Analysis in Python . Follow the article link to download the data, then load the data into a pandas DataFrame called df. We’ll start from where we left off in that article.
Say we want to determine the height of the tropopause, where the temperature changes from decreasing with altitude to increasing with altitude. We first want to smooth the data to remove any small-scale variations. In the article How to Plot a Running Average in Python Using Matplotlib , we explain how to do this with pandas. Here’s the code:
Now, we want to determine the height at which the minimum temperature occurs. To get some inspiration on how to implement this, go to your favorite search engine and search for something like: ‘Python pandas find position of minimum’. After a little reading, you’ll find the built-in method argmin() . It returns the index of the minimum value in a series:
Be sure to plot the temperature profile to check that the results make sense:

From here, you could run this analysis for different seasons to see how the structure of the atmosphere changes over time.
Challenges of a Data Science Career
Depending on your path to becoming a data scientist, you’ll have different skills and experiences. Since the job is so multi-faceted, there will inevitably be gaps in your knowledge that you’ll have to fill. Coming from an academic background where everyone was an expert in a similar field, I was required to learn how to work effectively with people from a variety of backgrounds – many of whom were non-technical.
It’s also common for there to be organizational and communication hurdles, such as aligning what is technically possible with business objectives; management might want to optimize a process, but the available dataset might be insufficient to get there.
Managing expectations from others is important; some have the idea that machine learning can solve everything. To navigate these challenges, it’s important to prioritize clear and concise communication, focusing on storytelling techniques to convey the significance of data insights. Developing strong interdisciplinary collaboration, cultivating domain expertise, and actively engaging with other team members throughout the project lifecycle can help ensure alignment with organizational goals.
Besides developing the necessary soft skills, technical challenges can pose additional hurdles. One common challenge is debugging code, especially when dealing with complex algorithms or integrating multiple libraries and frameworks. To overcome this challenge, it’s necessary to adopt systematic debugging practices, such as using print() statements and the logging and debugging tools available in many integrated development environments (IDEs). We go into more detail on this in 4 Best Python IDE and Code Editors . Additionally, making use of online forums and community resources such as Stack Overflow can provide new perspectives into solving challenging technical issues.
Handling large datasets is another prevalent challenge in data science, particularly in terms of memory management and processing speed. To address this, techniques like data sampling, parallel processing, and distributed computing frameworks can be used. Optimizing code efficiency and minimizing memory usage is a critical factor in many applications, e.g. when processing large numbers of images or videos.
What’s Next in Your Python and Data Science Path?
Embarking on a journey in data science with Python opens doors to endless possibilities and opportunities for growth and innovation. Python's rich ecosystem of libraries, tools, and community support provides a solid foundation for data scientists to tackle complex challenges and make meaningful contributions across diverse domains. A good foundation in Python will make you not only proficient in working with data but also a solid Python developer .
As you continue your journey, remember to embrace curiosity and a growth mindset. Constantly seek new resources to get extra practice in Python and use courses and documentation to expand your knowledge and skills. There are some great books to help you learn . Dive into online communities, forums, and meetups to connect with fellow data enthusiasts, exchange ideas, and collaborate on projects. We discuss these topics in How to Master Python: A Guide for Beginners .
Don't hesitate to explore specialized areas within data science – such as machine learning, natural language processing, and deep learning – to deepen your expertise and stay at the forefront of innovation.
Whether you're a seasoned practitioner or just starting out, your journey in data science with Python will open the door to learning opportunities, impactful discoveries, and diverse career paths . So, keep coding and exploring what you can achieve with Python and data science.
You may also like
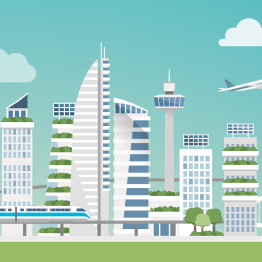
How Do You Write a SELECT Statement in SQL?
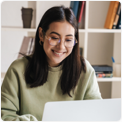
What Is a Foreign Key in SQL?

Enumerate and Explain All the Basic Elements of an SQL Query
- Top Courses
- Online Degrees
- Find your New Career
- Join for Free

Applied Data Science with Python Specialization
Gain new insights into your data . Learn to apply data science methods and techniques, and acquire analysis skills.
Taught in English
Some content may not be translated

Instructors: Christopher Brooks +3 more
Instructors

Financial aid available
405,352 already enrolled

Specialization - 5 course series
(25,941 reviews)
What you'll learn
Conduct an inferential statistical analysis
Discern whether a data visualization is good or bad
Enhance a data analysis with applied machine learning
Analyze the connectivity of a social network
Skills you'll gain
- Text Mining
- Python Programming
Details to know

Add to your LinkedIn profile
See how employees at top companies are mastering in-demand skills
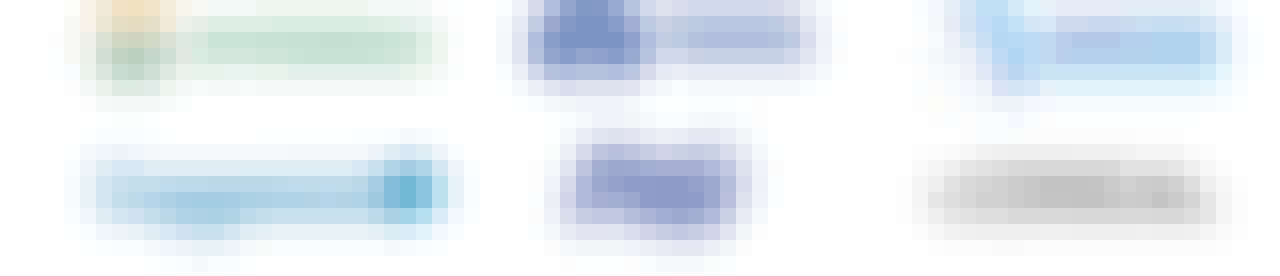
Advance your subject-matter expertise
- Learn in-demand skills from university and industry experts
- Master a subject or tool with hands-on projects
- Develop a deep understanding of key concepts
- Earn a career certificate from University of Michigan
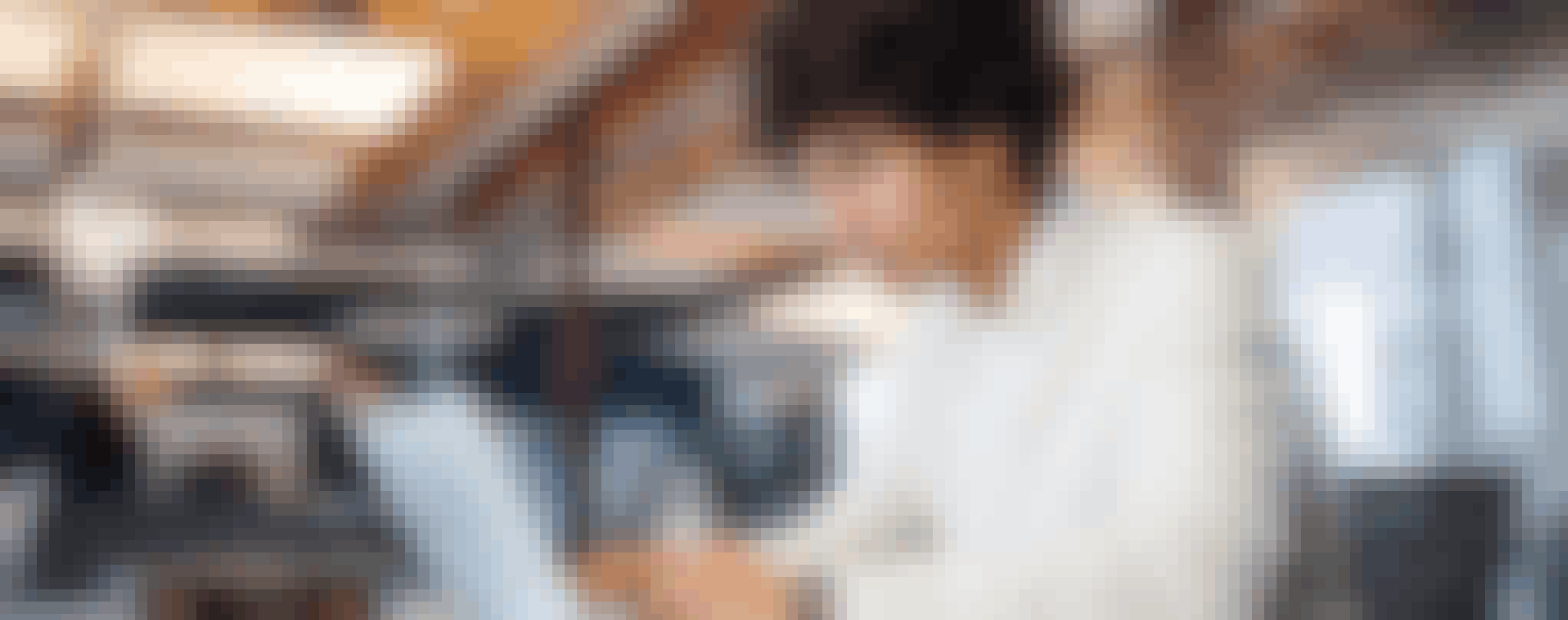
Earn a career certificate
Add this credential to your LinkedIn profile, resume, or CV
Share it on social media and in your performance review
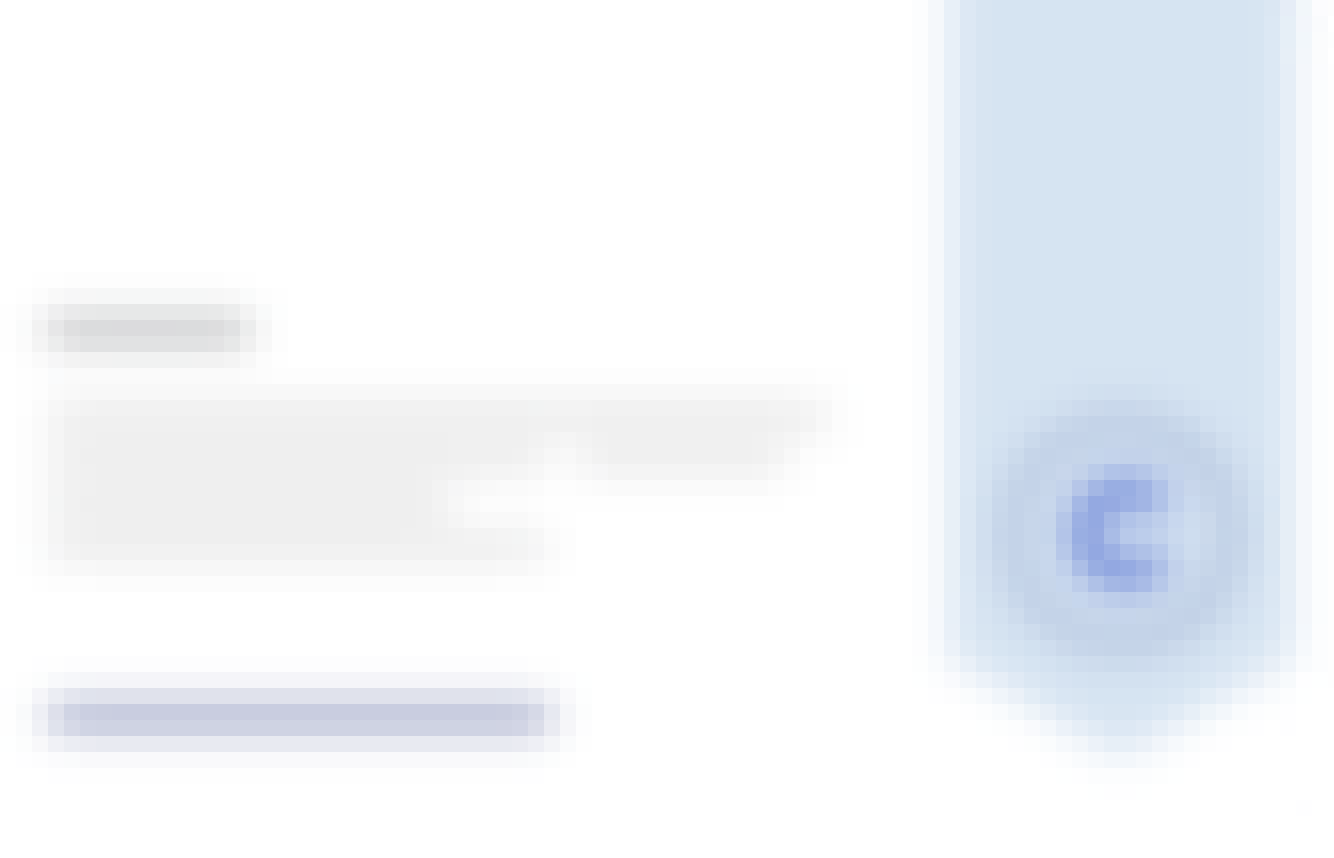
The 5 courses in this University of Michigan specialization introduce learners to data science through the python programming language. This skills-based specialization is intended for learners who have a basic python or programming background, and want to apply statistical, machine learning, information visualization, text analysis, and social network analysis techniques through popular python toolkits such as pandas, matplotlib, scikit-learn, nltk, and networkx to gain insight into their data.
Introduction to Data Science in Python (course 1), Applied Plotting, Charting & Data Representation in Python (course 2), and Applied Machine Learning in Python (course 3) should be taken in order and prior to any other course in the specialization. After completing those, courses 4 and 5 can be taken in any order. All 5 are required to earn a certificate.
Introduction to Data Science in Python
Understand techniques such as lambdas and manipulating csv files
Describe common Python functionality and features used for data science
Query DataFrame structures for cleaning and processing
Explain distributions, sampling, and t-tests
Applied Plotting, Charting & Data Representation in Python
Describe what makes a good or bad visualization
Understand best practices for creating basic charts
Identify the functions that are best for particular problems
Create a visualization using matplotlb
Applied Machine Learning in Python
Describe how machine learning is different than descriptive statistics
Create and evaluate data clusters
Explain different approaches for creating predictive models
Build features that meet analysis needs
Applied Text Mining in Python
Understand how text is handled in Python
Apply basic natural language processing methods
Write code that groups documents by topic
Describe the nltk framework for manipulating text
Applied Social Network Analysis in Python
Represent and manipulate networked data using the NetworkX library
Analyze the connectivity of a network
Measure the importance or centrality of a node in a network
Predict the evolution of networks over time

The mission of the University of Michigan is to serve the people of Michigan and the world through preeminence in creating, communicating, preserving and applying knowledge, art, and academic values, and in developing leaders and citizens who will challenge the present and enrich the future.
Prepare for a degree
Taking this Specialization by University of Michigan may provide you with a preview of the topics, materials and instructors in a related degree program which can help you decide if the topic or university is right for you.
University of Michigan
Master of Applied Data Science
Degree · 1 – 3 years
Why people choose Coursera for their career

New to Data Analysis? Start here.

Open new doors with Coursera Plus
Unlimited access to 7,000+ world-class courses, hands-on projects, and job-ready certificate programs - all included in your subscription
Advance your career with an online degree
Earn a degree from world-class universities - 100% online
Join over 3,400 global companies that choose Coursera for Business
Upskill your employees to excel in the digital economy
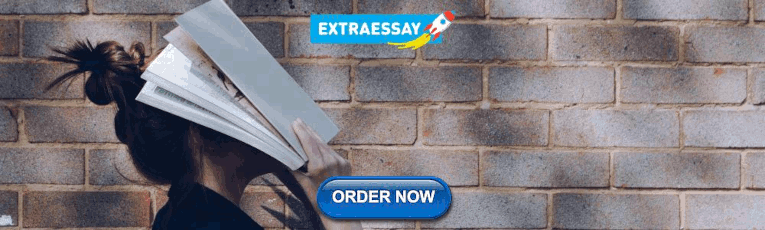
Frequently asked questions
Is this course really 100% online do i need to attend any classes in person.
This course is completely online, so there’s no need to show up to a classroom in person. You can access your lectures, readings and assignments anytime and anywhere via the web or your mobile device.
What is the refund policy?
If you subscribed, you get a 7-day free trial during which you can cancel at no penalty. After that, we don’t give refunds, but you can cancel your subscription at any time. See our full refund policy Opens in a new tab .
Can I just enroll in a single course?
Yes! To get started, click the course card that interests you and enroll. You can enroll and complete the course to earn a shareable certificate, or you can audit it to view the course materials for free. When you subscribe to a course that is part of a Specialization, you’re automatically subscribed to the full Specialization. Visit your learner dashboard to track your progress.
Is financial aid available?
Yes. In select learning programs, you can apply for financial aid or a scholarship if you can’t afford the enrollment fee. If fin aid or scholarship is available for your learning program selection, you’ll find a link to apply on the description page.
Can I take the course for free?
When you enroll in the course, you get access to all of the courses in the Specialization, and you earn a certificate when you complete the work. If you only want to read and view the course content, you can audit the course for free. If you cannot afford the fee, you can apply for financial aid Opens in a new tab .
Will I earn university credit for completing the Specialization?
This Specialization doesn't carry university credit, but some universities may choose to accept Specialization Certificates for credit. Check with your institution to learn more.
More questions
Python for Data Science NPTEL | Week 4
Session: JAN-APR 2024/ JULY-DEC 2023
Course name: Python For Data Science
Course Link: Click Here
These are NPTEL Python for Data Science Assignment 4 Answers
Q1. Which of the following are regression problems? Assume that appropriate data is given. Predicting the house price. Predicting whether it will rain or not on a given day. Predicting the maximum temperature on a given day. Predicting the sales of the ice-creams.
Answer: a, c, d
Q2. Which of the followings are binary classification problems? Predicting whether a patient is diagnosed with cancer or not. Predicting whether a team will win a tournament or not. Predicting the price of a second-hand car. Classify web text into one of the following categories: Sports, Entertainment, or Technology.
Answer: a, b
Q3. If a linear regression model achieves zero training error, can we say that all the data points lie on a hyperplane in the (d+1)-dimensional space? Here, d is the number of features. Yes No
Answer: Yes
Q4. Which of the following machine learning techniques would NOT be appropriate to solve the problem given in the problem statement? kNN Random Forest Logistic Regression Linear regression
Answer: Linear regression
Q5. After applying logistic regression, what is/are the correct observations from the resultant confusion matrix? True Positive = 29, True Negative = 94 True Positive = 94, True Negative = 29 False Positive = 5, True Negative = 94 None of the above
Answer: a, c
Q6. The logistic regression model built between the input and output variables is checked for its prediction accuracy of the test data. What is the accuracy range (in %) of the predictions made over test data? 60 – 79 90 – 95 30 – 59 80 – 89
Answer: 90 – 95
Q7. How are categorical variables preprocessed before model building? Standardization Dummy variables Correlation None of the above
Answer: Dummy variables
Q8. A multiple linear regression model is built on the Global Happiness Index dataset ‘GHI_Report.csv’. What is the RMSE of the baseline model? 2.00 0.50 1.06 0.75
Answer: 1.06
Q9. A regression model with the following function y=60+5.2x was built to understand the impact of humidity (x) on rainfall (y). The humidity this week is 30 more than the previous week. What is the predicted difference in rainfall? 156 mm 15.6 mm -156 mm None of the above
Answer: 156 mm
Q10. X nd Y are two variables that have a strong linear relationship. Which of the following statements are incorrect? There cannot be a negative relationship between the two variables. The relationship between the two variables is purely causal. One variable may or may not cause a change in the other variable. The variables can be positively or negatively correlated with each other.
More Weeks of Python for Data Science: Click here
More Nptel Courses: Click here
Session: JAN-APR 2023
Course Name: Python for Data Science
Q1. Which of the following are regression problems? Assume that appropriate data is given. a. Predicting the house price. b. Predicting whether it will rain or not on a given day. c. Predicting the maximum temperature on a given day. d. Predicting the sales of the ice-creams.
Q2. Which of the followings are binary classification problems? a. Predicting whether a patient is diagnosed with cancer or not. b. Predicting whether a team will win a tournament or not. c. Predicting the price of a second-hand car. d. Classify web text into one of the following categories: Sports, Entertainment, or Technology.
Q3. If a linear regression model achieves zero training error, can we say that all the data points lie on a hyperplane in the (d+1)-dimensional space? Here, d is the number of features. a. Yes b. No
Answer: a. Yes
Read the information given below and answer the questions from 4 to 6: Data Description: An automotive service chain is launching its new grand service station this weekend.They offer to service a wide variety of cars. The current capacity of the station is to check 315 cars thoroughly per day. As an inaugural offer, they claim to freely check all cars that arrive on their launch day, and report whether they need servicing or not! Unexpectedly, they get 450 cars. The servicemen will not work longer than the working hours, but the data analysts have to!
Can you save the day for the new service station? How can a data scientist save the day for them? He has been given a data set, ‘ServiceTrain.csv’ that contains some attributes of the car that can be easily measured and a conclusion that if a service is needed or not. Now for the cars they cannot check in detail, they measure those attributes and store them in ‘ ServiceTest.csv ’ Problem Statement: Use machine learning techniques to identify whether the cars require service or not Read the given datasets ‘ ServiceTrain.csv ’ and ‘ ServiceTest.csv ’ as train data and test data respectively and import all the required packages for analysis.
Q4. Which of the following machine learning techniques would NOT be appropriate to solve the problem given in the problem statement? a. kNN b. Random Forest c. Logistic Regression d. Linear regression
Answer: d. Linear regression
Prepare the data by following the steps given below, and answer questions 6 and 7.
- Encode categorical variable, Service – Yes as 1 and No as 0 for both the train and test datasets.
- Split the set of independent features and the dependent feature on both the train and test datasets.
- Set random_state for the instance of the logistic regression class as 0.
Q5. After applying logistic regression, what is/are the correct observations from the resultant confusion matrix? a. True Positive = 29, True Negative = 94 b. True Positive = 94, True Negative = 29 c. False Positive = 5, True Negative = 94 d. None of the above
Q6. The logistic regression model built between the input and output variables is checked for its prediction accuracy of the test data. What is the accuracy range (in %) of the predictions made over test data? a. 60 – 79 b. 90 – 95 c. 30 – 59 d. 80 – 89
Answer: b. 90 – 95
Q7. How are categorical variables preprocessed before model building? a. Standardization b. Dummy variables c. Correlation d. None of the above
Answer: b. Dummy variables
The Global Happiness Index report contains the Happiness Score data with multiple features (namely the Economy, Family, Health, and Freedom) that could affect the target variable value. Prepare the data by following the steps given below, and answer question 8
- Split the set of independent features and the dependent feature on the given dataset
- Create training and testing data from the set of independent features and dependent feature by splitting the original data in the ratio 3:1 respectively, and set the value for random_state of the training/test split method’s instance as 1
Q8. A multiple linear regression model is built on the Global Happiness Index dataset “GHI Report.csv”. What is the RMSE of the baseline model? a. 2.00 b. 0.50 c. 1.06 d. 0.75
Answer: c. 1.06
Q9. A regression model with the following function y = 60 + 5.2x was built to understand the impact of humidity (x) on rainfall (y). The humidity this week is 30 more than the previous week. What is the predicted difference in rainfall? a. 156 mm b. 15.6 mm c. -156 mm d. None of the above
Answer: a. 156 mm
Q10. X and Y are two variables that have a strong linear relationship. Which of the following statements are incorrect? a. There cannot be a negative relationship between the two variables. b. The relationship between the two variables is purely causal. c. One variable may or may not cause a change in the other variable. d. The variables can be positively or negatively correlated with each other.
More Weeks of Python for Data Science NPTEL: Click here
More NPTEL courses: https://progiez.com/nptel
Session: JULY-DEC 2022
Course name: Python for Data Science
Link to Enroll: Click Here
Q1. The power consumption of an individual house in a residential complex has been recorded for the previous year. This data is analysed to predict the power consumption for the next year. Under which type of machine learning problem does this fall under? a. Classification b. Regression c. Reinforcement Learning d. None of the above
Answer: b. Regression
Q2. A dataset contains data collected by the Tamil Nadu Pollution Control Board on environmental conditions (154 variables) from one of their monitoring stations. This data is further analyzed to understand the most significant factors that affect the Air Quality Index. The predictive algorithm that can be used in this situation is __________. a. Logistic Regression b. Simple Linear Regression c. Multiple Linear Regression d. None of the above
Answer: c. Multiple Linear Regression
Q3. A regression model with the following function y = 60 + 5.2x was built to understand the impact of humidity (x) on rainfall (y). The humidity this week is 30 more than the previous week. What is the predicted difference in rainfall? a. 156 mm b. 15.6 mm c. -156 mm d. None of the above
5. The plot shown below denotes the percentage distribution of the target column values within the train_data dataframe. Which of the following options are correct?

a. Yes > 20, No > 60 b. No > 70, Yes > 20 c. Yes > 30, No > 70 d. Yes > 70, No > 30
Answer: b. No > 70, Yes > 20
Q6. After applying logistic regression, what is/are the correct observations from the resultant confusion matrix? a. True Positive = 29, True Negative = 94 b. True Positive = 94, True Negative = 29 c. False Positive = 5, True Negative = 94 d. None of the above
Answer: b. True Positive = 94, True Negative = 29
Q7. The logistic regression model built between the input and output variables is checked for its prediction accuracy of the test data. What is the accuracy range (in %) of the predictions made over test data? a. 60 – 79 b. 90 – 95 c. 30 – 59 d. 80 – 89
Answer: b. 90 – 95
Q8. How are categorical variables preprocessed before model building? a. Standardization b. Dummy variables c. Correlation d. None of the above
Q9. A multiple linear regression model is built on the Global Happiness Index dataset “GHI_Report.csv”. What is the RMSE of the baseline model? a. 2.00 b. 0.50 c. 1.06 d. 0.75
10. X and Y are two variables that have a strong linear relationship. Which of the following statements are incorrect? a. There cannot be a negative relationship between the two variables. b. The relationship between the two variables is purely causal. c. One variable may or may not cause a change in the other variable. d. The variables can be positively or negatively correlated with each other.
Python for Data Science NPTEL All weeks: https://progies.in/answers/nptel/python-for-data-science
More NPTEL course answers: https://progies.in/answers/nptel

- Python for Data Science
- Data Analysis
- Machine Learning
- Deep Learning
- Deep Learning Interview Questions
- ML Projects
- ML Interview Questions
Data Science Interview Questions and Answers
What is data science, basic data science interview questions for fresher, q.1 what is marginal probability, q.2 what are the probability axioms, q.3 what is conditional probability.
- Q.4 What is Bayes Theorem and when is it used in data science?
Q.5 Define variance and conditional variance.
Q.6 explain the concepts of mean, median, mode, and standard deviation., q.7 what is the normal distribution and standard normal distribution.
- Q.8 What is SQL, and what does it stand for?
Q.9 Explain the differences between SQL and NoSQL databases.
Q.10 what are the primary sql database management systems (dbms), q.11 what is the er model in sql, q.12 what is data transformation, q.13 what are the main components of a sql query, q.14 what is a primary key, q.15 what is the purpose of the group by clause, and how is it used, q.16 what is the where clause used for, and how is it used to filter data, q.17 how do you retrieve distinct values from a column in sql, q.18 what is the having clause, q.19 how do you handle missing or null values in a database table, q.20 what is the difference between supervised and unsupervised machine learning, q.21 what is linear regression, and what are the different assumptions of linear regression algorithms, q.22 logistic regression is a classification technique, why its name is regressions, not logistic classifications, q.23 what is the logistic function (sigmoid function) in logistic regression, q.24 what is overfitting and how can be overcome this, q.25 what is a support vector machine (svm), and what are its key components, q.26 explain the k-nearest neighbors (knn) algorithm..
- Q.27 What is the Naive Bayes algorithm, what are the different assumptions of Naive Bayes?
Q.28 What are decision trees, and how do they work?
Q.29 explain the concepts of entropy and information gain in decision trees., q.30 what is the difference between the bagging and boosting model, q.31 describe random forests and their advantages over single-decision trees., q.32 what is k-means, and how will it work, q.33 what is a confusion matrix explain with an example., q.34 what is a classification report and explain the parameters used to interpret the result of classification tasks with an example., intermediate data science interview questions, q.35 explain the uniform distribution., q.36 describe the bernoulli distribution., q.37 what is the binomial distribution.
- Q.38 Explain the exponential distribution and where it's commonly used.
Q.39 Describe the Poisson distribution and its characteristics.
Q40. explain the t-distribution and its relationship with the normal distribution., q.41 describe the chi-squared distribution., q.42 what is the difference between z-test, f-test, and t-test, q.43 what is the central limit theorem, and why is it significant in statistics, q.44 describe the process of hypothesis testing, including null and alternative hypotheses., q.45 how do you calculate a confidence interval, and what does it represent, q.46 what is a p-value in statistics, q.47 explain type i and type ii errors in hypothesis testing., q.48 what is the significance level (alpha) in hypothesis testing, q.49 how can you calculate the correlation coefficient between two variables, q.50 what is covariance, and how is it related to correlation, q.51 explain how to perform a hypothesis test for comparing two population means., q.52 explain the concept of normalization in database design..
- Q.53 What is database normalization?
Q.54 Define different types of SQL functions.
Q.55 explain the difference between inner join and left join., q.56 what is a subquery, and how can it be used in sql, q.57 how do you perform mathematical calculations in sql queries, q.58 what is the purpose of the case statement in sql, q.59 what is the difference between a database and a data warehouse, q.60 what is regularization in machine learning, state the differences between l1 and l2 regularization, q.61 explain the concepts of bias-variance trade-off in machine learning., q.62 how do we choose the appropriate kernel function in svm.
- Q.63 How does Naive Bayes handle categorical and continuous features?
- Q.64 What is Laplace smoothing (add-one smoothing) and why is it used in Naive Bayes?
Q.65 What are imbalanced datasets and how can we handle them?
Q.66 what are outliers in the dataset and how can we detect and remove them, q.67 what is the curse of dimensionality and how can we overcome this, q.68 how does the random forest algorithm handle feature selection, q.69 what is feature engineering explain the different feature engineering methods..
- Q.70 How will we deal with the categorical text values in machine learning?
- Q.71 What is DBSCAN and How will we use it?
Q.72 How does the EM (Expectation-Maximization) algorithm work in clustering?
Q.73 explain the concept of silhouette score in clustering evaluation., q.74 what is the relationship between eigenvalues and eigenvectors in pca, q.75 what is the cross-validation technique in machine learning, q.76 what are the roc and auc, explain its significance in binary classification..
- Q.77 Describe gradient descent and its role in optimizing machine learning models
Q.78 Describe batch gradient descent, stochastic gradient descent, and mini-batch gradient descent.
- Q.79 Explain the Apriori - Association Rule Mining
Data Science Interview Questions for Experienced
Q.80 explain multivariate distribution in data science., q.81 describe the concept of conditional probability density function (pdf)., q.82 what is the cumulative distribution function (cdf), and how is it related to pdf, q.83 what is anova what are the different ways to perform anova tests, q.84 how can you prevent gradient descent from getting stuck in local minima, q.85 explain the gradient boosting algorithms in machine learning..
- Q.86 Explain convolutions operations of CNN architecture?
- Q.87 What is feed forward network and how it is different from recurrent neural network?
Q.88 Explain the difference between generative and discriminative models?
- Q.89 What is the forward and backward propagations in deep learning?
Q.90 Describe the use of Markov models in sequential data analysis?
Q.91 what is generative ai.
- Q.92 What are different neural network architectures used to generate artificial data in deep learning?
Q.93 What is deep reinforcement learning technique?
Q.94 what is transfer learning, and how is it applied in deep learning.
- Q.95 What is the difference between object detection and image segmentation.
Q.96 Explain the concept of word embeddings in natural language processing (NLP).
Q.97 what is seq2seq model.
- Q.98 What is artificial neural networks.
Q.99 What is marginal probability?
Q.100 what are the probability axioms.
Data Science Interview Questions – Explore the Data Science Interview Questions and Answers for beginners and experienced professionals looking for new opportunities in data science.
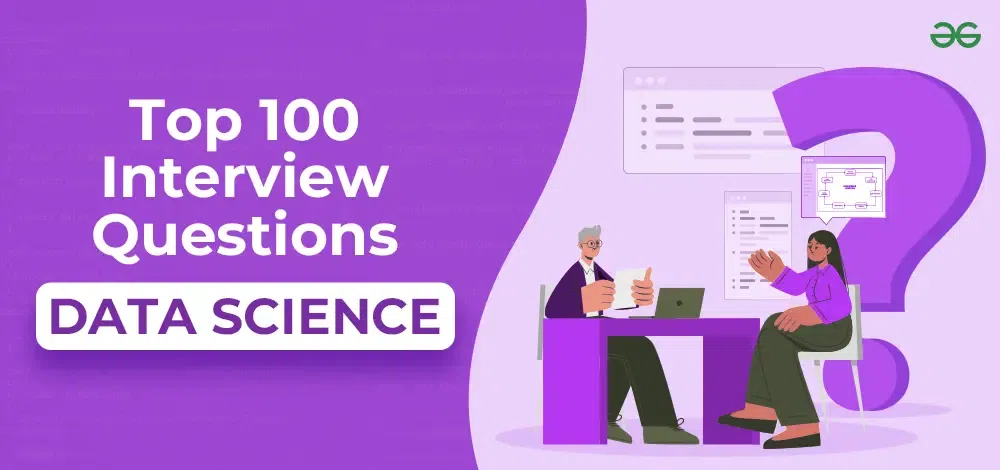
We all know that data science is a field where data scientists mine raw data, analyze it, and extract useful insights from it. The article outlines the frequently asked questionas during the data science interview. Practising all the below questions will help you to explore your career as a data scientist .
Table of Content
Data science is a field that extracts knowledge and insights from structured and unstructured data by using scientific methods, algorithms, processes, and systems. It combines expertise from various domains, such as statistics, computer science, machine learning, data engineering, and domain-specific knowledge, to analyze and interpret complex data sets.
Furthermore, data scientists use a combination of multiple languages, such as Python and R . They are also frequent users of data analysis tools like pandas, NumPy, and scikit-learn, as well as machine learning libraries.
After exploring the brief of data science, let’s dig into the data science interview questions and answers.
A key idea in statistics and probability theory is marginal probability, which is also known as marginal distribution. With reference to a certain variable of interest, it is the likelihood that an event will occur, without taking into account the results of other variables. Basically, it treats the other variables as if they were “marginal” or irrelevant and concentrates on one.
Marginal probabilities are essential in many statistical analyses, including estimating anticipated values, computing conditional probabilities, and drawing conclusions about certain variables of interest while taking other variables’ influences into account.
The fundamental rules that control the behaviour and characteristics of probabilities in probability theory and statistics are referred to as the probability axioms, sometimes known as the probability laws or probability principles.
There are three fundamental axioms of probability:
- Non-Negativity Axiom
- Normalization Axiom
- Additivity Axiom
The event or outcome occurring based on the existence of a prior event or outcome is known as conditional probability. It is determined by multiplying the probability of the earlier occurrence by the increased lprobability of the later, or conditional, event.
Q.4 What is Bayes’ Theorem and when is it used in data science?
The Bayes theorem predicts the probability that an event connected to any condition would occur. It is also taken into account in the situation of conditional probability. The probability of “causes” formula is another name for the Bayes theorem.
In data science, Bayes’ Theorem is used primarily in:
- Bayesian Inference
- Text Classification
- Medical Diagnosis
- Predictive Modeling
When working with ambiguous or sparse data, Bayes’ Theorem is very helpful since it enables data scientists to continually revise their assumptions and come to more sensible conclusions.
A statistical concept known as variance quantifies the spread or dispersion of a group of data points within a dataset. It sheds light on how widely individual data points depart from the dataset’s mean (average). It assesses the variability or “scatter” of data.
Conditional Variance
A measure of the dispersion or variability of a random variable under certain circumstances or in the presence of a particular event, as the name implies. It reflects a random variable’s variance that is dependent on the knowledge of another random variable’s variance.
Mean: The mean, often referred to as the average, is calculated by summing up all the values in a dataset and then dividing by the total number of values.
Median: When data are sorted in either ascending or descending order, the median is the value in the middle of the dataset. The median is the average of the two middle values when the number of data points is even. In comparison to the mean, the median is less impacted by extreme numbers, making it a more reliable indicator of central tendency.
Mode: The value that appears most frequently in a dataset is the mode. One mode (unimodal), several modes (multimodal), or no mode (if all values occur with the same frequency) can all exist in a dataset.
Standard deviation : The spread or dispersion of data points in a dataset is measured by the standard deviation. It quantifies the variance between different data points.
The normal distribution, also known as the Gaussian distribution or bell curve, is a continuous probability distribution that is characterized by its symmetric bell-shaped curve. The normal distribution is defined by two parameters: the mean ( μ ) and the standard deviation ( σ ). The mean determines the center of the distribution, and the standard deviation determines the spread or dispersion of the distribution. The distribution is symmetric around its mean, and the bell curve is centered at the mean. The probabilities for values that are further from the mean taper off equally in both directions. Similar rarity applies to extreme values in the two tails of the distribution. Not all symmetrical distributions are normal, even though the normal distribution is symmetrical.
The standard normal distribution, also known as the Z distribution, is a special case of the normal distribution where the mean ( μ ) is 0 and the standard deviation ( σ ) is 1. It is a standardized form of the normal distribution, allowing for easy comparison of scores or observations from different normal distributions.
Q.8 What is SQL, and what does it stand for?
SQL stands for Structured Query Language.It is a specialized programming language used for managing and manipulating relational databases. It is designed for tasks related to database management, data retrieval, data manipulation, and data definition.
Both SQL (Structured Query Language) and NoSQL (Not Only SQL) databases, differ in their data structures, schema, query languages, and use cases. The following are the main variations between SQL and NoSQL databases.
Relational database systems, both open source and commercial, are the main SQL (Structured Query Language) database management systems (DBMS), which are widely used for managing and processing structured data. Some of the most popular SQL database management systems are listed below:
- Microsoft SQL Server
- Oracle Database
The structure and relationships between the data entities in a database are represented by the Entity-Relationship (ER) model, a conceptual framework used in database architecture. The ER model is frequently used in conjunction with SQL for creating the structure of relational databases even though it is not a component of the SQL language itself.
The process of transforming data from one structure, format, or representation into another is referred to as data transformation. In order to make the data more suited for a given goal, such as analysis, visualisation, reporting, or storage, this procedure may involve a variety of actions and changes to the data. Data integration, cleansing, and analysis depend heavily on data transformation, which is a common stage in data preparation and processing pipelines.
A relational database’s data can be retrieved, modified, or managed via a SQL (Structured Query Language) query. The operation of a SQL query is defined by a number of essential components, each of which serves a different function.
A relational database table’s main key, also known as a primary keyword, is a column that is unique for each record. It is a distinctive identifier.The primary key of a relational database must be unique. Every row of data must have a primary key value and none of the rows can be null.
In SQL, the GROUP BY clause is used to create summary rows out of rows that have the same values in a set of specified columns. In order to do computations on groups of rows as opposed to individual rows, it is frequently used in conjunction with aggregate functions like SUM, COUNT, AVG, MAX, or MIN. we may produce summary reports and perform more in-depth data analysis using the GROUP BY clause.
In SQL, the WHERE clause is used to filter rows from a table or result set according to predetermined criteria. It enables us to pick only the rows that satisfy particular requirements or follow a pattern. A key element of SQL queries, the WHERE clause is frequently used for data retrieval and manipulation.
Using the DISTINCT keyword in combination with the SELECT command, we can extract distinct values from a column in SQL. By filtering out duplicate values and returning only unique values from the specified column, the DISTINCT keyword is used.
To filter query results depending on the output of aggregation functions, the HAVING clause, a SQL clause, is used along with the GROUP BY clause. The HAVING clause filters groups of rows after they have been grouped by one or more columns, in contrast to the WHERE clause, which filters rows before they are grouped.
Missing or NULL values can arise due to various reasons, such as incomplete data entry, optional fields, or data extraction processes.
- Replace NULL with Placeholder Values
- Handle NULL Values in Queries
- Use Default Values
The difference between Supervised Learning and Unsupervised Learning are as follow:
Linear Regression – It is type of Supervised Learning where we compute a linear relationship between the predictor and response variable. It is based on the linear equation concept given by:
[Tex]\hat{y} = \beta_1x+\beta_o [/Tex] , where
- [Tex]\hat{y} [/Tex] = response / dependent variable
- [Tex]\beta_1 [/Tex] = slope of the linear regression
- [Tex]\beta_o [/Tex] = intercept for linear regression
- [Tex]x [/Tex] = predictor / independent variable(s)
There are 4 assumptions we make about a Linear regression problem:
- Linear relationship : This assumes that there is a linear relationship between predictor and response variable. This means that, which changing values of predictor variable, the response variable changes linearly (either increases or decreases).
- Normality : This assumes that the dataset is normally distributed, i.e., the data is symmetric about the mean of the dataset.
- Independence : The features are independent of each other, there is no correlation among the features/predictor variables of the dataset.
- Homoscedasticity : This assumes that the dataset has equal variance for all the predictor variables. This means that the amount of independent variables have no effect on the variance of data.
While logistic regression is used for classification, it still maintains a regression structure underneath. The key idea is to model the probability of an event occurring (e.g., class 1 in binary classification) using a linear combination of features, and then apply a logistic (Sigmoid) function to transform this linear combination into a probability between 0 and 1. This transformation is what makes it suitable for classification tasks.
In essence, while logistic regression is indeed used for classification, it retains the mathematical and structural characteristics of a regression model, hence the name.
Sigmoid Function: It is a mathematical function which is characterized by its S- shape curve. Sigmoid functions have the tendency to squash a data point to lie within 0 and 1. This is why it is also called Squashing function, which is given as:
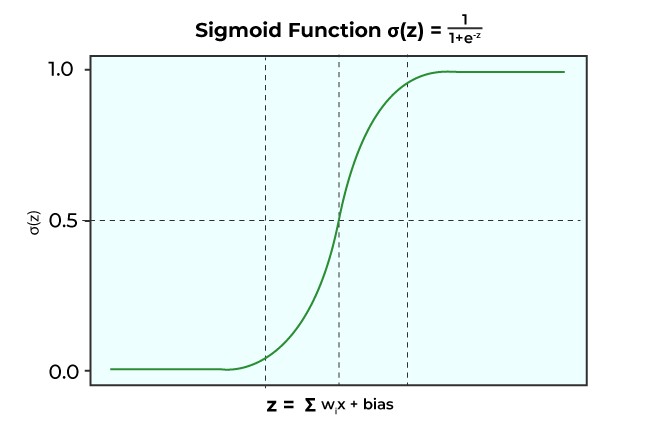
Some of the properties of Sigmoid function is:
- Range: [0,1]
Overfitting refers to the result of analysis of a dataset which fits so closely with training data that it fails to generalize with unseen/future data. This happens when the model is trained with noisy data which causes it to learn the noisy features from the training as well.
To avoid Overfitting and overcome this problem in machine learning, one can follow the following rules:
- Feature selection : Sometimes the training data has too many features which might not be necessary for our problem statement. In that case, we use only the necessary features that serve our purpose
- Cross Validation : This technique is a very powerful method to overcome overfitting. In this, the training dataset is divided into a set of mini training batches, which are used to tune the model.
- Regularization : Regularization is the technique to supplement the loss with a penalty term so as to reduce overfitting. This penalty term regulates the overall loss function, thus creating a well trained model.
- Ensemble models : These models learn the features and combine the results from different training models into a single prediction.
Support Vector machines are a type of Supervised algorithm which can be used for both Regression and Classification problems. In SVMs, the main goal is to find a hyperplane which will be used to segregate different data points into classes. Any new data point will be classified based on this defined hyperplane.
Support Vector machines are highly effective when dealing with high dimensionality space and can handle non linear data very well. But if the number of features are greater than number of data samples, it is susceptible to overfitting.
The key components of SVM are:
- Kernels Function : It is a mapping function used for data points to convert it into high dimensionality feature space.
- Hyperplane : It is the decision boundary which is used to differentiate between the classes of data points.
- Margin : It is the distance between Support Vector and Hyperplane
- C: It is a regularization parameter which is used for margin maximization and misclassification minimization.
The k-Nearest Neighbors (KNN) algorithm is a simple and versatile supervised machine learning algorithm used for both classification and regression tasks. KNN makes predictions by memorizing the data points rather than building a model about it. This is why it is also called “ lazy learner ” or “ memory based ” model too.
KNN relies on the principle that similar data points tend to belong to the same class or have similar target values. This means that, In the training phase, KNN stores the entire dataset consisting of feature vectors and their corresponding class labels (for classification) or target values (for regression). It then calculates the distances between that point and all the points in the training dataset. (commonly used distance metrics are Euclidean distance and Manhattan distance).
(Note : Choosing an appropriate value for k is crucial. A small k may result in noisy predictions, while a large k can smooth out the decision boundaries. The choice of distance metric and feature scaling also impact KNN’s performance.)
Q.27 What is the Naïve Bayes algorithm, what are the different assumptions of Naïve Bayes?
The Naïve Bayes algorithm is a probabilistic classification algorithm based on Bayes’ theorem with a “naïve” assumption of feature independence within each class. It is commonly used for both binary and multi-class classification tasks, particularly in situations where simplicity, speed, and efficiency are essential.
The main assumptions that Naïve Bayes theorem makes are:
- Feature independence – It assumes that the features involved in Naïve Bayes algorithm are conditionally independent, i.e., the presence/ absence of one feature does not affect any other feature
- Equality – This assumes that the features are equal in terms of importance (or weight).
- Normality – It assumes that the feature distribution is Normal in nature, i.e., the data is distributed equally around its mean.
Decision trees are a popular machine learning algorithm used for both classification and regression tasks. They work by creating a tree-like structure of decisions based on input features to make predictions or decisions. Lets dive into its core concepts and how they work briefly:
- Decision trees consist of nodes and edges.
- The tree starts with a root node and branches into internal nodes that represent features or attributes.
- These nodes contain decision rules that split the data into subsets.
- Edges connect nodes and indicate the possible decisions or outcomes.
- Leaf nodes represent the final predictions or decisions.
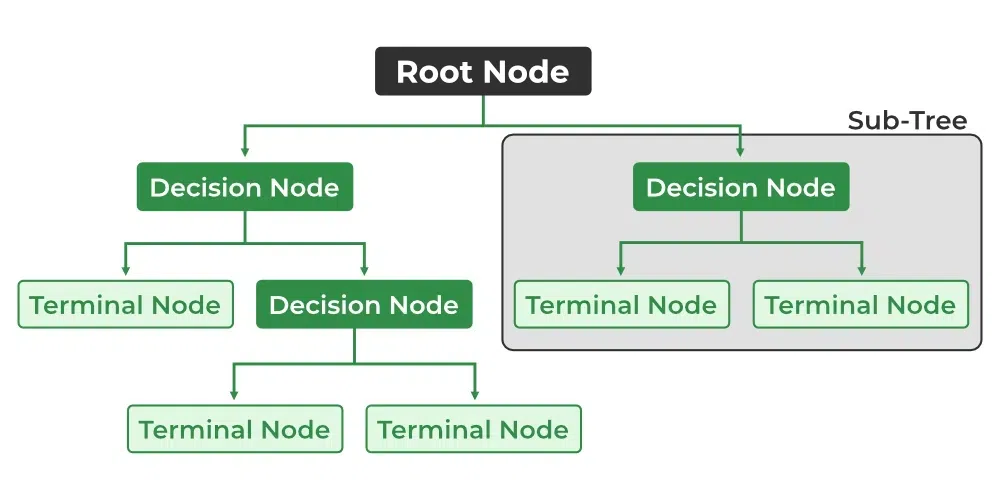
The objective is to increase data homogeneity, which is often measured using standards like mean squared error (for regression) or Gini impurity (for classification). Decision trees can handle a variety of attributes and can effectively capture complex data relationships. They can, however, overfit, especially when deep or complex. To reduce overfitting, strategies like pruning and restricting tree depth are applied.
Entropy : Entropy is the measure of randomness. In terms of Machine learning, Entropy can be defined as the measure of randomness or impurity in our dataset. It is given as:
Information gain: It is defined as the change in the entropy of a feature given that there’s an additional information about that feature. If there are more than one features involved in Decision tree split, then the weighted average of entropies of the additional features is taken.
E = Entropy
Random Forests are an ensemble learning technique that combines multiple decision trees to improve predictive accuracy and reduce overfitting. The advantages it has over single decision trees are:
- Improved Generalization : Single decision trees are prone to overfitting, especially when they become deep and complex. Random Forests mitigate this issue by averaging predictions from multiple trees, resulting in a more generalized model that performs better on unseen data
- Better Handling of High-Dimensional Data : Random Forests are effective at handling datasets with a large number of features. They select a random subset of features for each tree, which can improve the performance when there are many irrelevant or noisy features
- Robustness to Outliers: Random Forests are more robust to outliers because they combine predictions from multiple trees, which can better handle extreme cases
K-Means is an unsupervised machine learning algorithm used for clustering or grouping similar data points together. It aims to partition a dataset into K clusters, where each cluster represents a group of data points that are close to each other in terms of some similarity measure. The working of K-means is as follow:
- Choose the number of clusters K
- For each data point in the dataset, calculate its distance to each of the K centroids and then assign each data point to the cluster whose centroid is closest to it
- Recalculate the centroids of the K clusters based on the current assignment of data points.
- Repeat the above steps until a group of clusters are formed.
Confusion matrix is a table used to evaluate the performance of a classification model by presenting a comprehensive view of the model’s predictions compared to the actual class labels. It provides valuable information for assessing the model’s accuracy, precision, recall, and other performance metrics in a binary or multi-class classification problem.
A famous example demonstration would be Cancer Confusion matrix:
- TP (True Positive) = The number of instances correctly predicted as the positive class
- TN (True Negative) = The number of instances correctly predicted as the negative class
- FP (False Positive) = The number of instances incorrectly predicted as the positive class
- FN (False Negative) = The number of instances incorrectly predicted as the negative class
A classification report is a summary of the performance of a classification model, providing various metrics that help assess the quality of the model’s predictions on a classification task.
The parameters used in a classification report typically include:
- Precision : Precision is the ratio of true positive predictions to the total predicted positives. It measures the accuracy of positive predictions made by the model.
Precision = TP/(TP+FP)
- Recall (Sensitivity or True Positive Rate) : Recall is the ratio of true positive predictions to the total actual positives. It measures the model’s ability to identify all positive instances correctly.
Recall = TP / (TP + FN)
- Accuracy : Accuracy is the ratio of correctly predicted instances (both true positives and true negatives) to the total number of instances. It measures the overall correctness of the model’s predictions.
Accuracy = (TP + TN) / (TP + TN + FP + FN)
- F1-Score : The F1-Score is the harmonic mean of precision and recall. It provides a balanced measure of both precision and recall and is particularly useful when dealing with imbalanced datasets.
F1-Score = 2 * (Precision * Recall) / (Precision + Recall)
- TP = True Positive
- TN = True Negative
- FP = False Positive
- FN = False Negative
A fundamental probability distribution in statistics is the uniform distribution, commonly referred to as the rectangle distribution. A constant probability density function (PDF) across a limited range characterises it. In simpler terms, in a uniform distribution, every value within a specified range has an equal chance of occurring.
A discrete probability distribution, the Bernoulli distribution is focused on discrete random variables. The number of heads you obtain while tossing three coins at once or the number of pupils in a class are examples of discrete random variables that have a finite or countable number of potential values.
The binomial distribution is a discrete probability distribution that describes the number of successes in a fixed number of independent Bernoulli trials, where each trial has only two possible outcomes: success or failure. The outcomes are often referred to as “success” and “failure,” but they can represent any dichotomous outcome, such as heads or tails, yes or no, or defective or non-defective.
The fundamental presumptions of a binomial distribution are that each trial has exactly one possible outcome, each trial has an equal chance of success, and each trial is either independent of the others or mutually exclusive.
Q.38 Explain the exponential distribution and where it’s commonly used.
The probability distribution of the amount of time between events in the Poisson point process is known as the exponential distribution. The gamma distribution is thought of as a particular instance of the exponential distribution. Additionally, the geometric distribution’s continuous analogue is the exponential distribution.
Common applications of the exponential distribution include:
- Reliability Engineering
- Queueing Theory
- Telecommunications
- Natural Phenomena
- Survival Analysis
The Poisson distribution is a probability distribution that describes the number of events that occur within a fixed interval of time or space when the events happen at a constant mean rate and are independent of the time since the last event.
Key characteristics of the Poisson distribution include:
- Discreteness: The Poisson distribution is used to model the number of discrete events that occur within a fixed interval.
- Constant Mean Rate: The events occur at a constant mean rate per unit of time or space.
- Independence: The occurrences of events are assumed to be independent of each other. The probability of multiple events occurring in a given interval is calculated based on the assumption of independence.
The t-distribution, also known as the Student’s t-distribution, is used in statistics for inferences about population means when the sample size is small and the population standard deviation is unknown. The shape of the t-distribution is similar to the normal distribution, but it has heavier tails.
Relationship between T-Distribution and Normal Distribution: The t-distribution converges to the normal distribution as the degrees of freedom increase. In fact, when the degrees of freedom become very large, the t-distribution approaches the standard normal distribution (normal distribution with mean 0 and standard deviation 1). This is a result of the Central Limit Theorem.
The chi-squared distribution is a continuous probability distribution that arises in statistics and probability theory. It is commonly denoted as χ 2 (chi-squared) and is associated with degrees of freedom. The chi-squared distribution is particularly used to model the distribution of the sum of squared independent standard normal random variables.It is also used to determine if data series are independent, the goodness of fit of a data distribution, and the level of confidence in the variance and standard deviation of a random variable with a normal distribution.
The z-test, t-test, and F-test are all statistical hypothesis tests used in different situations and for different purposes. Here’s a overview of each test and the key differences between them.
In summary, the choice between a z-test, t-test, or F-test depends on the specific research question and the characteristics of the data.
The Central Limit Theorem states that, regardless of the shape of the population distribution, the distribution of the sample means approaches a normal distribution as the sample size increases.This is true even if the population distribution is not normal. The larger the sample size, the closer the sampling distribution of the sample mean will be to a normal distribution.
Hypothesis testing is a statistical method used to make inferences about population parameters based on sample data.It is a systematic way of evaluating statements or hypotheses about a population using observed sample data.To identify which statement is best supported by the sample data, it compares two statements about a population that are mutually exclusive.
- Null hypothesis(H0): The null hypothesis (H0) in statistics is the default assumption or assertion that there is no association between any two measured cases or any two groups. In other words, it is a fundamental assumption or one that is founded on knowledge of the problem.
- Alternative hypothesis(H1) : The alternative hypothesis, or H1, is the null-hypothesis-rejecting hypothesis that is utilised in hypothesis testing.
A confidence interval (CI) is a statistical range or interval estimate for a population parameter, such as the population mean or population proportion, based on sample data. to calculate confidence interval these are the following steps.
- Collect Sample Data
- Choose a Confidence Level
- Select the Appropriate Statistical Method
- Calculate the Margin of Error (MOE)
- Calculate the Confidence Interval
- Interpret the Confidence Interval
Confidence interval represents a range of values within which we believe, with a specified level of confidence (e.g., 95%), that the true population parameter lies.
The term “p-value,” which stands for “probability value,” is a key one in statistics and hypothesis testing. It measures the evidence contradicting a null hypothesis and aids in determining whether a statistical test’s findings are statistically significant. Here is a definition of a p-value and how it is used in hypothesis testing.
Rejecting a null hypothesis that is actually true in the population results in a type I error (false-positive); failing to reject a null hypothesis that is actually untrue in the population results in a type II error (false-negative).
type I and type II mistakes cannot be completely avoided, the investigator can lessen their risk by increasing the sample size (the less likely it is that the sample will significantly differ from the population).
A crucial metric in hypothesis testing that establishes the bar for judging whether the outcomes of a statistical test are statistically significant is the significance level, which is sometimes indicated as (alpha). It reflects the greatest possible chance of committing a Type I error, or mistakenly rejecting a valid null hypothesis.
The significance level in hypothesis testing.
- Setting the Significance Level
- Interpreting the Significance Level
- Hypothesis Testing Using Significance Level
- Choice of Significance Level
The degree and direction of the linear link between two variables are quantified by the correlation coefficient. The Pearson correlation coefficient is the most widely used method for determining the correlation coefficient. The Pearson correlation coefficient can be calculated as follows.
- Collect Data
- Calculate the Means
- Calculate the Covariance
- Calculate the Standard Deviations
- Calculate the Pearson Correlation Coefficient (r)
- Interpret the Correlation Coefficient.
Both covariance and correlation are statistical metrics that show how two variables are related to one another.However, they serve slightly different purposes and have different interpretations.
- Covariance :Covariance measures the degree to which two variables change together. It expresses how much the values of one variable tend to rise or fall in relation to changes in the other variable.
- Correlation : A standardised method for measuring the strength and direction of a linear relationship between two variables is correlation. It multiplies the standard deviations of the two variables to scale the covariance.
When comparing two population means, a hypothesis test is used to determine whether there is sufficient statistical support to claim that the means of the two distinct populations differ significantly. Tests we can commonly use for include “paired t-test” or “two -sample t test” . The general procedures for carrying out such a test are as follows.
- Formulate Hypotheses
- Choose the Significance Level
- Define Test Statistic
- Draw a Conclusion
- Final Results
By minimising data duplication and enhancing data integrity, normalisation is a method in database architecture that aids in the effective organisation of data. It include dividing a big, complicated table into smaller, associated tables while making sure that connections between data elements are preserved. The basic objective of normalisation is to reduce data anomalies, which can happen when data is stored in an unorganised way and include insertion, update, and deletion anomalies.
Q.53 What is database normalization?
Database denormalization is the process of intentionally introducing redundancy into a relational database by merging tables or incorporating redundant data to enhance query performance. Unlike normalization, which minimizes data redundancy for consistency, denormalization prioritizes query speed. By reducing the number of joins required, denormalization can improve read performance for complex queries. However, it may lead to data inconsistencies and increased maintenance complexity. Denormalization is often employed in scenarios where read-intensive operations outweigh the importance of maintaining a fully normalized database structure. Careful consideration and trade-offs are essential to strike a balance between performance and data integrity.
SQL functions can be categorized into several types based on their functionality.
- Scalar Functions
- Aggregate Functions
- Window Functions
- Table-Valued Functions
- System Functions
- User-Defined Functions
- Conversion Functions
- Conditional Functions
INNER JOIN and LEFT JOIN are two types of SQL JOIN operations used to combine data from multiple tables in a relational database. Here are the some main differences between them.
A subquery is a query that is nested within another SQL query, also referred to as an inner query or nested query. On the basis of the outcomes of another query, we can use it to get data from one or more tables. SQL’s subqueries capability is employed for a variety of tasks, including data retrieval, computations, and filtering.
In SQL, we can perform mathematical calculations in queries using arithmetic operators and functions. Here are some common methods for performing mathematical calculations.
- Arithmetic Operators
- Mathematical Functions
- Custom Expressions
The SQL CASE statement is a flexible conditional expression that may be used to implement conditional logic inside of a query. we can specify various actions or values based on predetermined criteria.
Database: Consistency and real-time data processing are prioritised, and they are optimised for storing, retrieving, and managing structured data. Databases are frequently used for administrative functions like order processing, inventory control, and customer interactions.
Data Warehouse: Data warehouses are made for processing analytical data. They are designed to facilitate sophisticated querying and reporting by storing and processing massive amounts of historical data from various sources. Business intelligence, data analysis, and decision-making all employ data warehouses.
Regularization : Regularization is the technique to restrict the model overfitting during training by inducing a penalty to the loss. The penalty imposed on the loss function is added so that the complexity of the model can be controlled, thus overcoming the issue of overfitting in the model.
The following are the differences between L1 and L2 regularization:
When creating predictive models, the bias-variance trade-off is a key concept in machine learning that deals with finding the right balance between two sources of error, bias and variance. It plays a crucial role in model selection and understanding the generalization performance of a machine learning algorithm. Here’s an explanation of these concepts:
- Bias :Bias is simply described as the model’s inability to forecast the real value due of some difference or inaccuracy. These differences between actual or expected values and the predicted values are known as error or bias error or error due to bias.
- Variance : Variance is a measure of data dispersion from its mean location. In machine learning, variance is the amount by which a predictive model’s performance differs when trained on different subsets of the training data. More specifically, variance is the model’s variability in terms of how sensitive it is to another subset of the training dataset, i.e. how much it can adapt on the new subset of the training dataset.
As a Data Science Professional, Our focus should be to achieve the the best fit model i.e Low Bias and Low Variance. A model with low bias and low variance suggests that it can capture the underlying patterns in the data (low bias) and is not overly sensitive to changes in the training data (low variance). This is the perfect circumstance for a machine learning model, since it can generalize effectively to new, previously unknown data and deliver consistent and accurate predictions. However, in practice, this is not achievable.
.png)
If the algorithm is too simplified (hypothesis with linear equation), it may be subject to high bias and low variance, making it error-prone. If algorithms fit too complicated a hypothesis (hypothesis with a high degree equation), it may have a large variance and a low bias. In the latter case, the new entries will underperform. There is, however, something in between these two situations called as a Trade-off or Bias Variance Trade-off . So, that An algorithm can’t be more complex and less complex at the same time.
A kernel function is responsible for converting the original data points into a high dimensionality feature space. Choosing the appropriate kernel function in a Support Vector Machine is a crucial step, as it determines how well the SVM can capture the underlying patterns in your data. Below mentioned are some of the ways to choose the suitable kernel function:
- If the dataset exhibits linear relationship
In this case, we should use Linear Kernel function. It is simple, computationally efficient and less prone to overfitting. For example, text classification, sentiment analysis, etc.
- If the dataset requires probabilistic approach
The sigmoid kernel is suitable when the data resembles a sigmoid function or when you have prior knowledge suggesting this shape. For example, Risk assessment, Financial applications, etc.
- If the dataset is Simple Non Linear in nature
In this case, use a Polynomial Kernel Function. Polynomial functions are useful when we are trying to capture moderate level of non linearity. For example, Image and Speech Recognition, etc.
- If the dataset is Highly Non-Linear in Nature/ we do not know about the underlying relationship
In that case, a Radial basis function is the best choice. RBF kernel can handle highly complex dataset and is useful when you’re unsure about the data’s underlying distribution. For example, Financial forecasting, bioinformatics, etc.
Q.63 How does Naïve Bayes handle categorical and continuous features?
Naive Bayes is probabilistic approach which assumes that the features are independent of each other. It calculates probabilities associated with each class label based on the observed frequencies of feature values within each class in the training data. This is done by finding the conditional probability of Feature given a class. (i.e., P(feature | class)). To make predictions on categorical data, Naive Bayes calculates the posterior probability of each class given the observed feature values and selects the class with the highest probability as the predicted class label. This is called as “maximum likelihood” estimation.
Q.64 What is Laplace smoothing (add-one smoothing) and why is it used in Naïve Bayes?
In Naïve Bayes, the conditional probability of an event given a class label is determined as P(event| class). When using this in a classification problem (let’s say a text classification), there could a word which did not appear in the particular class. In those cases, the probability of feature given a class label will be zero. This could create a big problem when getting predictions out of the training data.
To overcome this problem, we use Laplace smoothing. Laplace smoothing addresses the zero probability problem by adding a small constant (usually 1) to the count of each feature in each class and to the total count of features in each class. Without smoothing, if any feature is missing in a class, the probability of that class given the features becomes zero, making the classifier overly confident and potentially leading to incorrect classifications
Imbalanced datasets are datasets in which the distribution of class labels (or target values) is heavily skewed, meaning that one class has significantly more instances than any other class. Imbalanced datasets pose challenges because models trained on such data can have a bias toward the majority class, leading to poor performance on the minority class, which is often of greater interest. This will lead to the model not generalizing well on the unseen data.
To handle imbalanced datasets, we can approach the following methods:
- Up-sampling : In this case, we can increase the classes for minority by either sampling without replacement or generating synthetic examples. Some of the popular examples are SMOTE (Synthetic Minority Over-sampling Technique), etc.
- Down-sampling : Another case would be to randomly cut down the majority class such that it is comparable to minority class.
- Bagging : Techniques like Random Forests, which can mitigate the impact of class imbalance by constructing multiple decision trees from bootstrapped samples
- Boosting : Algorithms like AdaBoost and XGBoost can give more importance to misclassified minority class examples in each iteration, improving their representation in the final model
An Outlier is a data point that is significantly different from other data points. Usually, Outliers are present in the extremes of the distribution and stand out as compared to their out data point counterparts.
For detecting Outliers we can use the following approaches:
- Visual inspection: This is the easiest way which involves plotting the data points into scatter plot/box plot, etc.
- statistics : By using measure of central tendency, we can determine if a data point falls significantly far from its mean, median, etc. making it a potential outlier.
- Z-score: if a data point has very high Z-score, it can be identified as Outlier
For removing the outliers, we can use the following:
- Removal of outliers manually
- Doing transformations like applying logarithmic transformation or square rooting the outlier
- Performing imputations wherein the outliers are replaced with different values like mean, median, mode, etc.
When dealing with a dataset that has high dimensionality (high number of features), we are often encountered with various issues and problems. Some of the issues faced while dealing with dimensionality dataset are listed below:
- Computational expense : The biggest problem with handling a dataset with vast number of features is that it takes a long time to process and train the model on it. This can lead to wastage of both time and monetary resources.
- Data sparsity : Many times data points are far from each other (high sparsity). This makes it harder to find the underlying patterns between features and can be a hinderance in proper analysis
- Visualising issues and overfitting : It is rather easy to visualize 2d and 3d data. But beyond this order, it is difficult to properly visualize our data. Furthermore, more data features can be correlated and provide misleading information to the model training and cause overfitting.
These issues are what are generally termed as “Curse of Dimensionality”.
To overcome this, we can follow different approaches – some of which are mentioned below:
- Feature Selection : Many a times, not all the features are necessary. It is the user’s job to select out the features that would be necessary in solving a given problem statement.
- Feature engineering : Sometimes, we may need a feature that is the combination of many other features. This method can, in general, reduces the features count in the dataset.
- Dimensionality Reduction techniques : These techniques reduce the number of features in a dataset while preserving as much useful information as possible. Some of the famous Dimensionality reduction techniques are: Principle component analysis (PCA), t-Distributed Stochastic Neighbor Embedding (t-SNE), etc.
- Regularization: Some regularization techniques like L1 and L2 regularizations are useful when deciding the impact each feature has on the model training.
Mentioned below is how Random forest handles feature selection
- When creating individual trees in the Random Forest ensemble, a subset of features is assigned to each tree which is called Feature Bagging. Feature Bagging introduces randomness and diversity among the trees.
- After the training, the features are assigned a “importance score” based on how well those features performed by reducing the error of the model. Features that consistently contribute to improving the model’s accuracy across multiple trees are deemed more important
- Then the features are ranked based on their importance scores. Features with higher importance scores are considered more influential in making predictions.
Feature Engineering : It can be defined as a method of preprocessing of data for better analysis purpose which involves different steps like selection, transformation, deletion of features to suit our problem at hand. Feature Engineering is a useful tool which can be used for:
- Improving the model’s performance and Data interpretability
- Reduce computational costs
- Include hidden patterns for elevated Analysis results.
Some of the different methods of doing feature engineering are mentioned below:
- Principle Component Analysis (PCA) : It identifies orthogonal axes (principal components) in the data that capture the maximum variance, thereby reducing the data features.
- One-Hot Encoding – When we need to encode Nominal Categorical Data
- Label Encoding – When we need to encode Ordinal Categorical Data
- Feature Transformation : Sometimes, we can create new columns essential for better modelling just by combining or modifying one or more columns.
Q.70 How we will deal with the categorical text values in machine learning?
Often times, we are encountered with data that has Categorical text values. For example, male/female, first-class/second-class/third-class, etc. These Categorical text values can be divided into two types and based on that we deal with them as follows:
- If it is Categorical Nominal Data: If the data does not have any hidden order associated with it (e.g., male/female), we perform One-Hot encoding on the data to convert it into binary sequence of digits
- If it is Categorical Ordinal Data : When there is a pattern associated with the text data, we use Label encoding. In this, the numerical conversion is done based on the order of the text data. (e.g., Elementary/ Middle/ High/ Graduate,etc.)
Q.71 What is DBSCAN and How we will use it?
Density-Based Spatial Clustering of Applications with Noise (DBSCAN), is a density-based clustering algorithm used for grouping together data points that are close to each other in high-density regions and labeling data points in low-density regions as outliers or noise. Here is how it works:
- For each data point in the dataset, DBSCAN calculates the distance between that point and all other data points
- DBSCAN identifies dense regions by connecting core points that are within each other’s predefined threshold (eps) neighborhood.
- DBSCAN forms clusters by grouping together data points that are density-reachable from one another.
The Expectation-Maximization (EM) algorithm is a probabilistic approach used for clustering data when dealing with mixture models. EM is commonly used when the true cluster assignments are not known and when there is uncertainty about which cluster a data point belongs to. Here is how it works:
- First, the number of clusters K to be formed is specified.
- Then, for each data point, the likelihood of it belonging to each of the K clusters is calculated. This is called the Expectation (E) step
- Based on the previous step, the model parameters are updated. This is called Maximization (M) step.
- Together it is used to check for convergence by comparing the change in log-likelihood or the parameter values between iterations.
- If it converges, then we have achieved our purpose. If not, then the E-step and M-step are repeated until we reach convergence.
Silhouette score is a metric used to evaluate the quality of clusters produced by a clustering algorithm. Here is how it works:
- the average distance between the data point and all other data points in the same cluster is first calculated. Let us call this as (a)
- Then for the same data point, the average distance (b) between the data point and all data points in the nearest neighboring cluster (i.e., the cluster to which it is not assigned)
- if -1<S<0, it signifies that data point is closer to a neighboring cluster than to its own cluster.
- if S is close to zero, data point is on or very close to the decision boundary between two neighboring clusters.
- if 0<S<1, data point is well within its own cluster and far from neighboring clusters.
In Principal Component Analysis (PCA), eigenvalues and eigenvectors play a crucial role in the transformation of the original data into a new coordinate system. Let us first define the essential terms:
- Eigen Values : Eigenvalues are associated with each eigenvector and represent the magnitude of the variance (spread or extent) of the data along the corresponding eigenvector
- Eigen Vectors : Eigenvectors are the directions or axes in the original feature space along which the data varies the most or exhibits the most variance
The relationship between them is given as:
[Tex]AV = \lambda{V} [/Tex] , where
A = Feature matrix
V = eigen vector
[Tex]\lambda [/Tex] = Eigen value.
A larger eigenvalue implies that the corresponding eigenvector captures more of the variance in the data.The sum of all eigenvalues equals the total variance in the original data. Therefore, the proportion of total variance explained by each principal component can be calculated by dividing its eigenvalue by the sum of all eigenvalues
Cross-validation is a resampling technique used in machine learning to assess and validate the performance of a predictive model. It helps in estimating how well a model is likely to perform on unseen data, making it a crucial step in model evaluation and selection. Cross validation is usually helpful when avoiding overfitting the model. Some of the widely known cross validation techniques are:
- K-Fold Cross-Validation : In this, the data is divided into K subsets, and K iterations of training and testing are performed.
- Stratified K-Fold Cross-Validation : This technique ensures that each fold has approximately the same proportion of classes as the original dataset (helpful in handling data imbalance)
- Shuffle-Split Cross-Validation : It randomly shuffles the data and splits it into training and testing sets.
Receiver Operating Characteristic (ROC) is a graphical representation of a binary classifier’s performance. It plots the true positive rate (TPR) vs the false positive rate (FPR) at different classification thresholds.
True positive rate (TPR) : It is the ratio of true positive predictions to the total actual positives.
False positive rate (FPR) : It is the ratio of False positive predictions to the total actual positives.
FPR= FP / (TP + FN)
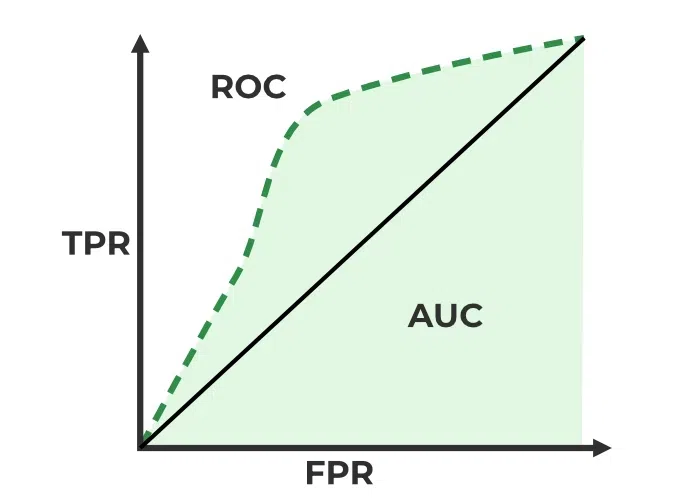
Area Under the Curve (AUC) as the name suggests is the area under the ROC curve. The AUC is a scalar value that quantifies the overall performance of a binary classification model and ranges from 0 to 1, where a model with an AUC of 0.5 indicates random guessing, and an AUC of 1 represents a perfect classifier.
Q.77 Describe gradient descent and its role in optimizing machine learning models.
Gradient descent is a fundamental optimization algorithm used to minimize a cost or loss function in machine learning and deep learning. Its primary role is to iteratively adjust the parameters of a machine learning model to find the values that minimize the cost function, thereby improving the model’s predictive performance. Here’s how Gradient descent help in optimizing Machine learning models:
- Minimizing Cost functions : The primary goal of gradient descent is to find parameter values that result in the lowest possible loss on the training data.
- Convergence : The algorithm continues to iterate and update the parameters until it meets a predefined convergence criterion, which can be a maximum number of iterations or achieving a desired level of accuracy.
- Generalization : Gradient descent ensure that the optimized model generalizes well to new, unseen data.
Batch Gradient Descent: In Batch Gradient Descent, the entire training dataset is used to compute the gradient of the cost function with respect to the model parameters (weights and biases) in each iteration. This means that all training examples are processed before a single parameter update is made. It converges to a more accurate minimum of the cost function but can be slow, especially in a high dimensionality space.
Stochastic Gradient Descent: In Stochastic Gradient Descent, only one randomly selected training example is used to compute the gradient and update the parameters in each iteration. The selection of examples is done independently for each iteration. This is capable of faster updates and can handle large datasets because it processes one example at a time but high variance can cause it to converge slower.
Mini-Batch Gradient Descent: Mini-Batch Gradient Descent strikes a balance between BGD and SGD. It divides the training dataset into small, equally-sized subsets called mini-batches. In each iteration, a mini-batch is randomly sampled, and the gradient is computed based on this mini-batch. It utilizes parallelism well and takes advantage of modern hardware like GPUs but can still exhibits some level of variance in updates compared to Batch Gradient Descent.
Q.79 Explain the Apriori — Association Rule Mining
Association Rule mining is an algorithm to find relation between two or more different objects. Apriori association is one of the most frequently used and most simple association technique. Apriori Association uses prior knowledge of frequent objects properties. It is based on Apriori property which states that:
“All non-empty subsets of a frequent itemset must also be frequent”
A vector with several normally distributed variables is said to have a multivariate normal distribution if any linear combination of the variables likewise has a normal distribution. The multivariate normal distribution is used to approximatively represent the features of specific characteristics in machine learning, but it is also important in extending the central limit theorem to several variables.
In probability theory and statistics, the conditional probability density function (PDF) is a notion that represents the probability distribution of a random variable within a certain condition or constraint. It measures the probability of a random variable having a given set of values given a set of circumstances or events.
The probability that a continuous random variable will take on particular values within a range is described by the Probability Density Function (PDF), whereas the Cumulative Distribution Function (CDF) provides the cumulative probability that the random variable will fall below a given value. Both of these concepts are used in probability theory and statistics to describe and analyse probability distributions. The PDF is the CDF’s derivative, and they are related by integration and differentiation.
The statistical method known as ANOVA, or Analysis of Variance, is used to examine the variation in a dataset and determine whether there are statistically significant variations between group averages. When comparing the means of several groups or treatments to find out if there are any notable differences, this method is frequently used.
There are several different ways to perform ANOVA tests, each suited for different types of experimental designs and data structures:
- One-Way ANOVA
- Two-Way ANOVA
- Three-Way ANOVA
When conducting ANOVA tests we typically calculate an F-statistic and compare it to a critical value or use it to calculate a p-value.
Ans: The local minima problem occurs when the optimization algorithm converges a solution that is minimum within a small neighbourhood of the current point but may not be the global minimum for the objective function.
To mitigate local minimal problems, we can use the following technique:
- Use initialization techniques like Xavier/Glorot and He to model trainable parameters. This will help to set appropriate initial weights for the optimization process.
- Set Adam or RMSProp as optimizer, these adaptive learning rate algorithms can adapt the learning rates for individual parameters based on historical gradients.
- Introduce stochasticity in the optimization process using mini-batches, which can help the optimizer to escape local minima by adding noise to the gradient estimates.
- Adding more layers or neurons can create a more complex loss landscape with fewer local minima.
- Hyperparameter tuning using random search cv and grid search cv helps to explore the parameter space more thoroughly suggesting right hyperparameters for training and reducing the risk of getting stuck in local minima.
Gradient boosting techniques like XGBoost, and CatBoost are used for regression and classification problems. It is a boosting algorithm that combines the predictions of weak learners to create a strong model. The key steps involved in gradient boosting are:
- Initialize the model with weak learners, such as a decision tree.
- Calculate the difference between the target value and predicted value made by the current model.
- Add a new weak learner to calculate residuals and capture the errors made by the current ensemble.
- Update the model by adding fraction of the new weak learner’s predictions. This updating process can be controlled by learning rate.
- Repeat the process from step 2 to 4, with each iteration focusing on correcting the errors made by the previous model.
Q.86 Explain convolutions operations of CNN architecture?
In a CNN architecture, convolution operations involve applying small filters (also called kernels) to input data to extract features. These filters slide over the input image covering one small part of the input at a time, computing dot products at each position creating a feature map. This operation captures the similarity between the filter’s pattern and the local features in the input. Strides determine how much the filter moves between positions. The resulting feature maps capture patterns, such as edges, textures, or shapes, and are essential for image recognition tasks. Convolution operations help reduce the spatial dimensions of the data and make the network translation-invariant, allowing it to recognize features in different parts of an image. Pooling layers are often used after convolutions to further reduce dimensions and retain important information.
Q.87 What is feed forward network and how it is different from recurrent neural network?
Deep learning designs that are basic are feedforward neural networks and recurrent neural networks. They are both employed for different tasks, but their structure and how they handle sequential data differ.
Feed Forward Neural Network
- In FFNN, the information flows in one direction, from input to output, with no loops
- It consists of multiple layers of neurons, typically organized into an input layer, one or more hidden layers, and an output layer.
- Each neuron in a layer is connected to every neuron in the subsequent layer through weighted connections.
- FNNs are primarily used for tasks such as classification and regression, where they take a fixed-size input and produce a corresponding output
Recurrent Neural Network
- A recurrent neural network is designed to handle sequential data, where the order of input elements matters. Unlike FNNs, RNNs have connections that loop back on themselves, allowing them to maintain a hidden state that carries information from previous time steps.
- This hidden state enables RNNs to capture temporal dependencies and context in sequential data, making them well-suited for tasks like natural language processing, time series analysis, and sequence generation.
- However, standard RNNs have limitations in capturing long-range dependencies due to the vanishing gradient problem.
Generative models focus on generating new data samples, while discriminative models concentrate on classification and prediction tasks based on input data.
Generative Models:
- Objective: Model the joint probability distribution P(X, Y) of input X and target Y.
- Use: Generate new data, often for tasks like image and text generation.
- Examples: Variational Autoencoders (VAEs), Generative Adversarial Networks (GANs).
Discriminative Models:
- Objective: Model the conditional probability distribution P(Y | X) of target Y given input X.
- Use: Classify or make predictions based on input data.
- Examples: Logistic Regression, Support Vector Machines, Convolutional Neural Networks (CNNs) for image classification.
Q.89 What is the forward and backward propogations in deep learning?
Forward and backward propagations are key processes that occur during neural network training in deep learning. They are essential for optimizing network parameters and learning meaningful representations from input.
The process by which input data is passed through the neural network to generate predictions or outputs is known as forward propagation. The procedure begins at the input layer, where data is fed into the network. Each neuron in a layer calculates the weighted total of its inputs, applies an activation function, and sends the result to the next layer. This process continues through the hidden layers until the final output layer produces predictions or scores for the given input data.
The technique of computing gradients of the loss function with regard to the network’s parameters is known as backward propagation. It is utilized to adjust the neural network parameters during training using optimization methods such as gradient descent.
The process starts with the computation of the loss, which measures the difference between the network’s predictions and the actual target values. Gradients are then computed by using the chain rule of calculus to propagate this loss backward through the network. This entails figuring out how much each parameter contributed to the error. The computed gradients are used to adjust the network’s weights and biases, reducing the error in subsequent forward passes.
Markov models are effective methods for capturing and modeling dependencies between successive data points or states in a sequence. They are especially useful when the current condition is dependent on earlier states. The Markov property, which asserts that the future state or observation depends on the current state and is independent of all prior states. There are two types of Markov models used in sequential data analysis:
- Markov chains are the simplest form of Markov models, consisting of a set of states and transition probabilities between these states. Each state represents a possible condition or observation, and the transition probabilities describe the likelihood of moving from one state to another.
- Hidden Markov Models extend the concept of Markov chains by introducing a hidden layer of states and observable emissions associated with each hidden state. The true state of the system (hidden state) is not directly observable, but the emissions are observable.
Applications:
- HMMs are used to model phonemes and words in speech recognition systems, allowing for accurate transcription of spoken language
- HMMs are applied in genomics for gene prediction and sequence alignment tasks. They can identify genes within DNA sequences and align sequences for evolutionary analysis.
- Markov models are used in modeling financial time series data, such as stock prices, to capture the dependencies between consecutive observations and make predictions.
Generative AI is an abbreviation for Generative Artificial Intelligence, which refers to a class of artificial intelligence systems and algorithms that are designed to generate new, unique data or material that is comparable to, or indistinguishable from, human-created data. It is a subset of artificial intelligence that focuses on the creative component of AI, allowing machines to develop innovative outputs such as writing, graphics, audio, and more. There are several generative AI models and methodologies, each adapted to different sorts of data and applications such as:
- Generative AI models such as GPT (Generative Pretrained Transformer) can generate human-like text.” Natural language synthesis, automated content production, and chatbot responses are all common uses for these models.
- Images are generated using generative adversarial networks (GANs).” GANs are made up of a generator network that generates images and a discriminator network that determines the authenticity of the generated images. Because of the struggle between the generator and discriminator, high-quality, realistic images are produced.
- Generative AI can also create audio content, such as speech synthesis and music composition.” Audio content is generated using models such as WaveGAN and Magenta.
Q.92 What are different neural network architecture used to generate artificial data in deep learning?
Various neural networks are used to generate artificial data. Here are some of the neural network architectures used for generating artificial data:
- GANs consist of two components – generator and discriminator, which are trained simultaneously through adversarial training. They are used to generating high-quality images, such as photorealistic faces, artwork, and even entire scenes.
- VAEs are generative models that learn a probabilistic mapping from the data space to a latent space. They also consist of encoder and decoder. They are used for generating images, reconstructing missing parts of images, and generating new data samples. They are also applied in generating text and audio.
- RNNs are a class of neural networks with recurrent connections that can generate sequences of data. They are often used for sequence-to-sequence tasks. They are used in text generation, speech synthesis, music composition.
- Transformers are a type of neural network architecture that has gained popularity for sequence-to-sequence tasks. They use self-attention mechanisms to capture dependencies between different positions in the input data. They are used in natural language processing tasks like machine translation, text summarization, and language generation.
- Autoencoders are neural networks that are trained to reconstruct their input data. Variants like denoising autoencoders and contractive autoencoders can be used for data generation. They are used for image denoising, data inpainting, and generating new data samples.
Deep Reinforcement Learning (DRL) is a cutting-edge machine learning technique that combines the principles of reinforcement learning with the capability of deep neural networks. Its ability to enable machines to learn difficult tasks independently by interacting with their environments, similar to how people learn via trial and error, has garnered significant attention.
DRL is made up of three fundamental components:
- The agent interacts with the environment and takes decision.
- The environment is the outside world with which the agent interacts and receives feedback.
- The reward signal is a scalar value provided by the environment after each action, guiding the agent toward maximizing cumulative rewards over time.
- In robotics, DRL is used to control robots, manipulation and navigation.
- DRL plays a role in self-driving cars and vehicle control
- Can also be used for customized recommendations
Transfer learning is a strong machine learning and deep learning technique that allows models to apply knowledge obtained from one task or domain to a new, but related. It is motivated by the notion that what we learn in one setting can be applied to a new, but comparable, challenge.
Benefits of Transfer Learning:
- We may utilize knowledge from a large dataset by starting with a pretrained model, making it easier to adapt to a new task with data.
- Training a deep neural network from scratch can be time-consuming and costly in terms of compute. Transfer learning enables us to bypass the earliest phases of training, saving both time and resources.
- Pretrained models frequently learn rich data representations. Models that use these representations can generalize better, even when the target task has a smaller dataset.
Transfer Learning Process:
- It’s a foundation step in transfer learning. The pretrained data is already trained on large and diverse dataset for a related task.
- To leverage the knowlege, output layers of the pretrained model are removed leaving the layers responsible for feature extraction. The target data is passed through these layers to extract feature information.
- using these extracted features, the model captures patterns and representations from the data.
- After the feature extraction process, the model is fine-tuned for the specific target task.
- Output layers are added to the model and these layer are designed to produce the desired output for the target task.
- Backpropagation is used to iteratively update the model’s weights during fine-tuning. This method allows the model to tailor its representations and decision boundaries to the specifics of the target task.
- Even as the model focuses in the target task, the knowledge and features learned from the pre-trained layers continue to contribute to its understanding. This dual learning process improves the model’s performance and enables it to thrive in tasks that require little data or resources.
Q.95 What is difference between object detections and image segmentations.
Object detection and Image segmentation are both computer vision tasks that entail evaluating and comprehending image content, but they serve different functions and give different sorts of information.
Object Detection:
- goal of object detection is to identify and locate objects and represent the object in bounding boxes with their respective labels.
- used in applications like autonomous driving for detecting pedestrians and vehicle
Image Segmentation:
- focuses on partitioning an image into multiple regions, where each segment corresponding to a coherent part of the image.
- provide pixel level labeling of the entire image
- used in applications that require pixel level understanding such as medical image analysis for organ and tumor delineation.
In NLP, the concept of word embedding is use to capture semantic and contextual information. Word embeddings are dense representations of words or phrases in continuous-valued vectors in a high-dimensional space. Each word is mapped to a vector with the real numbers, these vectors are learned from large corpora of text data.
Word embeddings are based on the Distributional Hypothesis, which suggests that words that appear in similar context have similar meanings. This idea is used by word embedding models to generate vector representations that reflect the semantic links between words depending on how frequently they co-occur with other words in the text.
The most common word embeddings techniques are-
- Bag of Words (BOW)
- Glove: Global Vector for word representation
- Term frequency-inverse document frequency (TF-IDF)
A neural network architecture called a Sequence-to-Sequence (Seq2Seq) model is made to cope with data sequences, making it particularly helpful for jobs involving variable-length input and output sequences. Machine translation, text summarization, question answering, and other tasks all benefit from its extensive use in natural language processing.
The Seq2Seq consists of two main components: encoder and decoder. The encoder takes input sequence and converts into fixed length vector . The vector captures features and context of the sequence. The decoder takes the vector as input and generated output sequence. This autoregressive technique frequently entails influencing the subsequent prediction using the preceding one.
Q.98 What is artificial neural networks.
Artificial neural networks take inspiration from structure and functioning of human brain. The computational units in ANN are called neurons and these neurons are responsible to process and pass the information to the next layer.
ANN has three main components:
- Input Layer : where the network receives input features.
- Hidden Layer: one or more layers of interconnected neurons responsible for learning patterns in the data
- Output Layer : provides final output on processed information.
A key idea in statistics and probability theory is marginal probability, which is also known as marginal distribution. With reference to a certain variable of interest, it is the likelihood that an event will occur, without taking into account the results of other variables. Basically, it treats the other variables as if they were “marginal” or irrelevant and concentrates on one.
Marginal probabilities are essential in many statistical analyses, including estimating anticipated values, computing conditional probabilities, and drawing conclusions about certain variables of interest while taking other variables’ influences into account.
We all know that data science is growing career and if you are looking a future in data science, then explore this detailed article on data science interview questions.
Please Login to comment...
Similar reads.
- AI-ML-DS With Python
- interview-questions
- Data Science
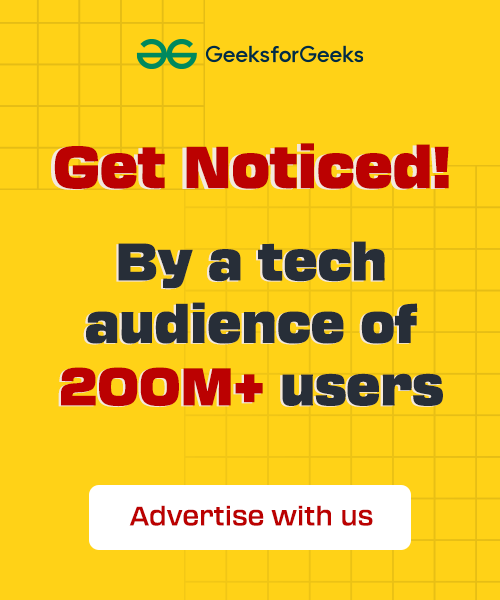
Improve your Coding Skills with Practice
What kind of Experience do you want to share?
- Monday, April 22, 2024
NPTEL Programming, Data Structures And Algorithms Using Python Week4 Assignment
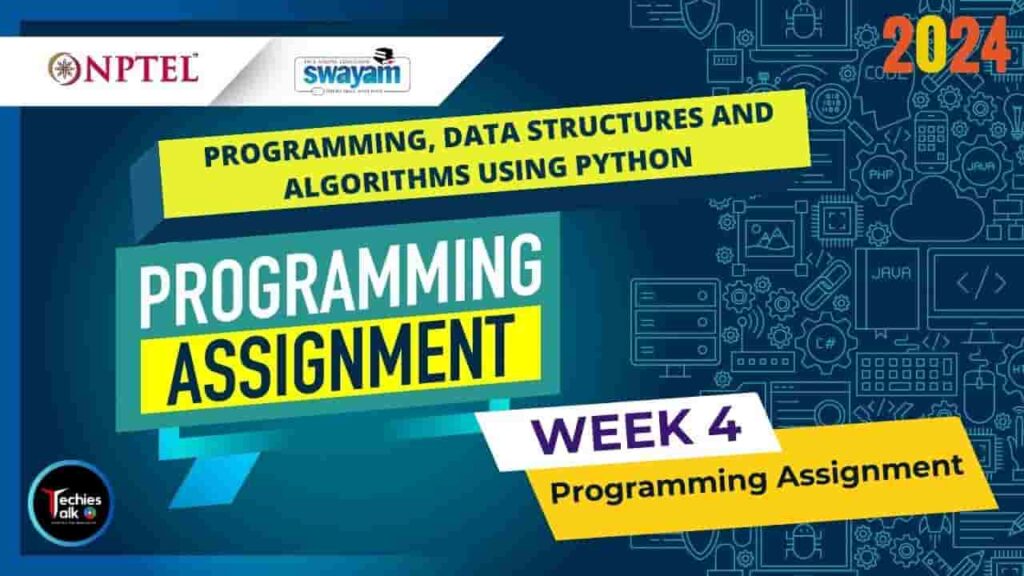
NPTEL Course is an introduction to programming and problem solving in Python. It does not assume any prior knowledge of programming. Using some motivating examples, the course quickly builds up basic concepts such as conditionals, loops, functions, lists, strings and tuples. It goes on to cover searching and sorting algorithms, dynamic programming and backtracking, as well as topics such as exception handling and using files. As far as data structures are concerned, the course covers Python dictionaries as well as classes and objects for defining user defined datatypes such as linked lists and binary search trees.
Programming, Data Structures And Algorithms Using Python Week4 Assignment Jan 2024
INTENDED AUDIENCE : Students in any branch of mathematics/science/engineering, 1st year PREREQUISITES : School level mathematics INDUSTRY SUPPORT : This course should be of value to any company requiring programming skills.
Course Layout
Week 1: Informal introduction to programming, algorithms and data structures via GCD Downloading and installing Python GCD in Python: variables, operations, control flow – assignments, conditionals, loops, functions Week 2: Python: types, expressions, strings, lists, tuples Python memory model: names, mutable and immutable values List operations: slices etc Binary search Inductive function definitions: numerical and structural induction Elementary inductive sorting: selection and insertion sort In-place sorting Week 3: Basic algorithmic analysis: input size, asymptotic complexity, O() notation Arrays vs lists Merge sort Quicksort Stable sorting Week 4: Dictionaries More on Python functions: optional arguments, default values Passing functions as arguments Higher order functions on lists: map, lter, list comprehension Week 5: Exception handling Basic input/output Handling files String processing Week 6: Backtracking: N Queens, recording all solutions Scope in Python: local, global, non-local names Nested functions Data structures: stack, queue Heaps Week 7: Abstract data-types Classes and objects in Python “Linked” lists: find, insert, delete Binary search trees: find, insert, delete Height-balanced binary search trees Week 8: Efficient evaluation of recursive definitions: memorization Dynamic programming: examples Other programming languages: C and manual memory management Other programming paradigms: functional programming
Programming Assignment 1
Write Python functions as specified below. Paste the text for all functions together into the submission window.
- You may define additional auxiliary functions as needed.
- In all cases you may assume that the value passed to the function is of the expected type, so your function does not have to check for malformed inputs.
- For each function, there are some public test cases and some (hidden) private test cases.
- “Compile and run” will evaluate your submission against the public test cases.
- “Submit” will evaluate your submission against the hidden private test cases and report a score on 100. There are 10 private testcases in all, each with equal weightage. You will get feedback about which private test cases pass or fail, though you cannot see the actual test cases.
- Ignore warnings about “Presentation errors”.
We represent scores of batsmen across a sequence of matches in a two level dictionary as follows:
Each match is identified by a string, as is each player. The scores are all integers. The names associated with the matches are not fixed (here they are 'match1' , 'match2' , 'match3' ), nor are the names of the players. A player need not have a score recorded in all matches.
Define a Python function orangecap(d) that reads a dictionary d of this form and identifies the player with the highest total score. Your function should return a pair (playername,topscore) where playername is a string, the name of the player with the highest score, and topscore is an integer, the total score of playername.
The input will be such that there are never any ties for highest total score.
For instance:
Let us consider polynomials in a single variable x with integer coefficients. For instance:
Each term of the polynomial can be represented as a pair of integers (coefficient,exponent). The polynomial itself is then a list of such pairs.
We have the following constraints to guarantee that each polynomial has a unique representation:
- Terms are sorted in descending order of exponent
- No term has a zero cofficient
- No two terms have the same exponent
- Exponents are always nonnegative
For example, the polynomial introduced earlier is represented as:
The zero polynomial, 0, is represented as the empty list [] , since it has no terms with nonzero coefficients.
Write Python functions for the following operations:
that add and multiply two polynomials, respectively.
You may assume that the inputs to these functions follow the representation given above. Correspondingly, the outputs from these functions should also obey the same constraints.
You can write auxiliary functions to “clean up” polynomials – e.g., remove zero coefficient terms, combine like terms, sort by exponent etc. Build a library of functions that can be combined to achieve the desired format.
You may also want to convert the list representation to a dictionary representation and manipulate the dictionary representation, and then convert back.
Some examples:
Search code, repositories, users, issues, pull requests...
Provide feedback.
We read every piece of feedback, and take your input very seriously.
Saved searches
Use saved searches to filter your results more quickly.
To see all available qualifiers, see our documentation .
- Notifications
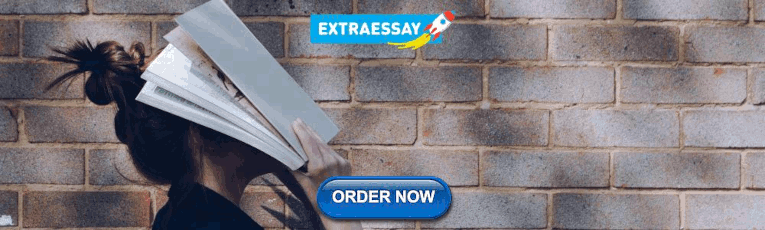
IMAGES
VIDEO
COMMENTS
#pythonfordatascience #nptel #swayam #python #datascience Python for Data Science All week Assignment Solution - https://www.youtube.com/playlist?list=PL__28...
Python for Data Science: Reminder for Assignment 1 & 2 deadline!! Dear Learners, The Deadline for Assignments 1 & 2 will close on Wednesday, [07/02/2024], 23:59 IST. Kindly submit the assignments before the deadline. Thanks and Regards, -NPTEL Team.
Python for Data Science Week 4: Assignment 4 Solutions || 2023#nptel #nptel2023
This course will introduce the learner to the basics of the python programming environment, including fundamental python programming techniques such as lambdas, reading and manipulating csv files, and the numpy library. The course will introduce data manipulation and cleaning techniques using the popular python pandas data science library and ...
Saved searches Use saved searches to filter your results more quickly
These free exercises are nothing but Python assignments for the practice where you need to solve different programs and challenges. All exercises are tested on Python 3. Each exercise has 10-20 Questions. The solution is provided for every question. These Python programming exercises are suitable for all Python developers.
A Real-World Python for Data Science Example. For a real-world example of using Python for data science, consider a dataset of atmospheric soundings which we downloaded and prepared in the article 7 Datasets to Practice Data Analysis in Python. Follow the article link to download the data, then load the data into a pandas DataFrame called df.
The course will introduce data manipulation and cleaning techniques using the popular python pandas data science library and introduce the abstraction of the Series and DataFrame as the central data structures for data analysis, along with tutorials on how to use functions such as groupby, merge, and pivot tables effectively. ... Assignment 4 ...
Photo by BRUNO EMMANUELLE on Unsplash Format 3 — Onsite Case study/ Take home Assignment. This can be an Onsite (nowadays Online) short duration (1-3 hours) case study, or a take home assignment of 3-7 days where a candidate is given a sample dataset (which is fairly similar to a real dataset in size and complexity) and asked to solve for a business objective in 90 mins.
The 5 courses in this University of Michigan specialization introduce learners to data science through the python programming language. This skills-based specialization is intended for learners who have a basic python or programming background, and want to apply statistical, machine learning, information visualization, text analysis, and social network analysis techniques through popular ...
Python supports numbers, strings, sets, lists, tuples, and dictionaries. These are the standard data types. I will explain each of them in detail. Declare And Assign Value To Variable. Assignment sets a value to a variable. To assign variable a value, use the equals sign (=) myFirstVariable = 1 mySecondVariable = 2 myFirstVariable = "Hello You"
Answer: d. Linear regression. These are NPTEL Python for Data Science Assignment 4 Answers. Prepare the data by following the steps given below, and answer questions 6 and 7. Encode categorical variable, Service - Yes as 1 and No as 0 for both the train and test datasets.
#nptel #programming #software #nptelcourseanswers #knowledge #learn #python #python for data science#datascience #data #science
View Data-Science-Python-Coursera-MICHIGAN-_Assignment4.pdf from CSC 1380 at St. John's University. 1/31/2021 Data-Science-Python-Coursera-MICHIGAN-/Assignment+4 ...
This problem has been solved! You'll get a detailed solution from a subject matter expert that helps you learn core concepts. Question: Course - Coursera - Applied machine learning by Python - module 4 - Assignment 4 - Predicting and understanding viewer engagement with educational videos. About the prediction problem One critical property of a ...
This article will guide you to accomplish the final assignment of Data Visualization with Python, a course created by IBM and offered by Coursera.Nevertheless, this tutorial is for anyone— enrolled in the course or not — who wants to learn how to code an interactive dashboard in Python using Plotly's Dash library.
Python 101. Data Analysis with Python. Data visualization is the graphical representation of data in order to interactively and efficiently convey insights to clients, customers, and stakeholders in general. It is a way to summarize your findings and display it in a form that facilitates interpretation and can help in identifying.
Introduction to Data Science with Python - 13 Assignment 3 (Pandas)
Data Scientist II at Big Analytixs | Book Author - Big Data Science & Analytics | 4xAzure Certified | 3xHackathon winner | R | Python | Big data | Data Science | ML ...
Q.26 Explain the k-nearest neighbors (KNN) algorithm. The k-Nearest Neighbors (KNN) algorithm is a simple and versatile supervised machine learning algorithm used for both classification and regression tasks. KNN makes predictions by memorizing the data points rather than building a model about it.
Note: Coursera courses are available for free to audit, and if that option is not available, you can complete the courses during the trial period or ask for financial aid. 1. Python . Python is a necessary programming language for data science. You will learn it for data manipulation, analysis, visualization, and machine learning.
This line returns the overall dataset, converted from the binary file into a Python list. data returns gridded data separated into each day of the study period. This dataset can now be analyzed. Returning the data for a particular cell in the study area grid for the entire study period
NPTEL Programming, Data Structures And Algorithms Using Python Week4 Assignment. February 21, 2024. Faheem Ahmad. NPTEL Course is an introduction to programming and problem solving in Python. It does not assume any prior knowledge of programming. Using some motivating examples, the course quickly builds up basic concepts such as conditionals ...
RMIT University School of Computing Technologies Practical Data Science with Python Assignment 1: Data Cleaning and Summarising Due: 23:59 on the 21st of April, 2024 This assignment is worth 25% of your overall mark. Introduction In this assignment, you will examine a (set of) data file(s) and carry out the first steps of the data science process, including the cleaning and exploring of data.
Contribute to kaz2es/Final-Assignment-of-IBM-Python-Project-for-Data-Science development by creating an account on GitHub. ... kaz2es / Final-Assignment-of-IBM-Python-Project-for-Data-Science Public. Notifications Fork 0; Star 0. Code; Issues 0; Pull requests 0; Actions; Projects 0; Security; Insights Footer ...
NiceGUI is a great choice if you want to make user interfaces quickly and easily with Python. It will help you build powerful python apps where you retain full control of the internal state and that you can test and deploy easily. Hopefully, it can allow you to express your creativity in your data science projects without being limited by tools.
Prompt engineering for LLMs involves carefully crafting prompts to maximize the quality and relevance of the model's output. This process is both an art and a science, as it requires an understanding of how the model interprets and processes language. Articles often feature titles like "Top 10 Prompts for ChatGPT".
Multiplicative decomposition. Image by author.. where y — is a value from a time series, S — seasonal components, T — trend component and n — noise.. To produce decomposition, besides selecting the decomposition type, you need to set a seasonal period (e.g. p=1 for annual, p=4 for quarterly, p=12 for monthly data etc.).
In my example, I am using llama-cpp-python LLM, which is a python wrapper around llama.cpp. We can serve GGUF models with llama-cpp-python, which are lightweight and available in different quantized versions. You can find a more detailed explanation of how to use and serve a GGUF model with llama-cpp-python in one of my previous posts here: