Have a language expert improve your writing
Run a free plagiarism check in 10 minutes, generate accurate citations for free.
- Knowledge Base
- Research paper
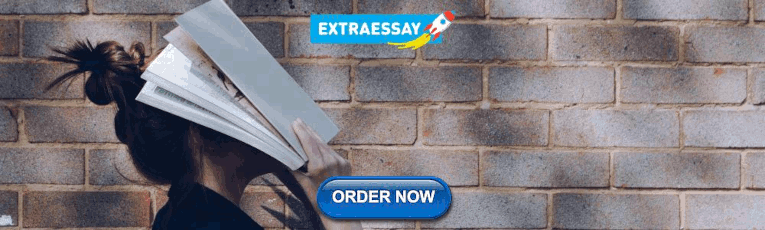
What Is a Theoretical Framework? | Guide to Organizing
Published on October 14, 2022 by Sarah Vinz . Revised on November 20, 2023 by Tegan George.
A theoretical framework is a foundational review of existing theories that serves as a roadmap for developing the arguments you will use in your own work.
Theories are developed by researchers to explain phenomena, draw connections, and make predictions. In a theoretical framework, you explain the existing theories that support your research, showing that your paper or dissertation topic is relevant and grounded in established ideas.
In other words, your theoretical framework justifies and contextualizes your later research, and it’s a crucial first step for your research paper , thesis , or dissertation . A well-rounded theoretical framework sets you up for success later on in your research and writing process.
Table of contents
Why do you need a theoretical framework, how to write a theoretical framework, structuring your theoretical framework, example of a theoretical framework, other interesting articles, frequently asked questions about theoretical frameworks.
Before you start your own research, it’s crucial to familiarize yourself with the theories and models that other researchers have already developed. Your theoretical framework is your opportunity to present and explain what you’ve learned, situated within your future research topic.
There’s a good chance that many different theories about your topic already exist, especially if the topic is broad. In your theoretical framework, you will evaluate, compare, and select the most relevant ones.
By “framing” your research within a clearly defined field, you make the reader aware of the assumptions that inform your approach, showing the rationale behind your choices for later sections, like methodology and discussion . This part of your dissertation lays the foundations that will support your analysis, helping you interpret your results and make broader generalizations .
- In literature , a scholar using postmodernist literary theory would analyze The Great Gatsby differently than a scholar using Marxist literary theory.
- In psychology , a behaviorist approach to depression would involve different research methods and assumptions than a psychoanalytic approach.
- In economics , wealth inequality would be explained and interpreted differently based on a classical economics approach than based on a Keynesian economics one.
To create your own theoretical framework, you can follow these three steps:
- Identifying your key concepts
- Evaluating and explaining relevant theories
- Showing how your research fits into existing research
1. Identify your key concepts
The first step is to pick out the key terms from your problem statement and research questions . Concepts often have multiple definitions, so your theoretical framework should also clearly define what you mean by each term.
To investigate this problem, you have identified and plan to focus on the following problem statement, objective, and research questions:
Problem : Many online customers do not return to make subsequent purchases.
Objective : To increase the quantity of return customers.
Research question : How can the satisfaction of company X’s online customers be improved in order to increase the quantity of return customers?
2. Evaluate and explain relevant theories
By conducting a thorough literature review , you can determine how other researchers have defined these key concepts and drawn connections between them. As you write your theoretical framework, your aim is to compare and critically evaluate the approaches that different authors have taken.
After discussing different models and theories, you can establish the definitions that best fit your research and justify why. You can even combine theories from different fields to build your own unique framework if this better suits your topic.
Make sure to at least briefly mention each of the most important theories related to your key concepts. If there is a well-established theory that you don’t want to apply to your own research, explain why it isn’t suitable for your purposes.
3. Show how your research fits into existing research
Apart from summarizing and discussing existing theories, your theoretical framework should show how your project will make use of these ideas and take them a step further.
You might aim to do one or more of the following:
- Test whether a theory holds in a specific, previously unexamined context
- Use an existing theory as a basis for interpreting your results
- Critique or challenge a theory
- Combine different theories in a new or unique way
A theoretical framework can sometimes be integrated into a literature review chapter , but it can also be included as its own chapter or section in your dissertation. As a rule of thumb, if your research involves dealing with a lot of complex theories, it’s a good idea to include a separate theoretical framework chapter.
There are no fixed rules for structuring your theoretical framework, but it’s best to double-check with your department or institution to make sure they don’t have any formatting guidelines. The most important thing is to create a clear, logical structure. There are a few ways to do this:
- Draw on your research questions, structuring each section around a question or key concept
- Organize by theory cluster
- Organize by date
It’s important that the information in your theoretical framework is clear for your reader. Make sure to ask a friend to read this section for you, or use a professional proofreading service .
As in all other parts of your research paper , thesis , or dissertation , make sure to properly cite your sources to avoid plagiarism .
To get a sense of what this part of your thesis or dissertation might look like, take a look at our full example .
If you want to know more about AI for academic writing, AI tools, or research bias, make sure to check out some of our other articles with explanations and examples or go directly to our tools!
Research bias
- Survivorship bias
- Self-serving bias
- Availability heuristic
- Halo effect
- Hindsight bias
- Deep learning
- Generative AI
- Machine learning
- Reinforcement learning
- Supervised vs. unsupervised learning
(AI) Tools
- Grammar Checker
- Paraphrasing Tool
- Text Summarizer
- AI Detector
- Plagiarism Checker
- Citation Generator
While a theoretical framework describes the theoretical underpinnings of your work based on existing research, a conceptual framework allows you to draw your own conclusions, mapping out the variables you may use in your study and the interplay between them.
A literature review and a theoretical framework are not the same thing and cannot be used interchangeably. While a theoretical framework describes the theoretical underpinnings of your work, a literature review critically evaluates existing research relating to your topic. You’ll likely need both in your dissertation .
A theoretical framework can sometimes be integrated into a literature review chapter , but it can also be included as its own chapter or section in your dissertation . As a rule of thumb, if your research involves dealing with a lot of complex theories, it’s a good idea to include a separate theoretical framework chapter.
Cite this Scribbr article
If you want to cite this source, you can copy and paste the citation or click the “Cite this Scribbr article” button to automatically add the citation to our free Citation Generator.
Vinz, S. (2023, November 20). What Is a Theoretical Framework? | Guide to Organizing. Scribbr. Retrieved April 1, 2024, from https://www.scribbr.com/dissertation/theoretical-framework/
Is this article helpful?
Sarah's academic background includes a Master of Arts in English, a Master of International Affairs degree, and a Bachelor of Arts in Political Science. She loves the challenge of finding the perfect formulation or wording and derives much satisfaction from helping students take their academic writing up a notch.
Other students also liked
What is a research methodology | steps & tips, how to write a literature review | guide, examples, & templates, what is a conceptual framework | tips & examples, unlimited academic ai-proofreading.
✔ Document error-free in 5minutes ✔ Unlimited document corrections ✔ Specialized in correcting academic texts
- No category
research design
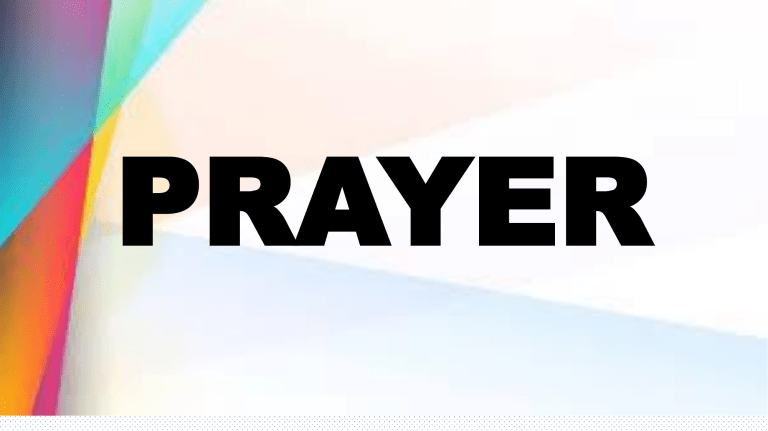
Related documents
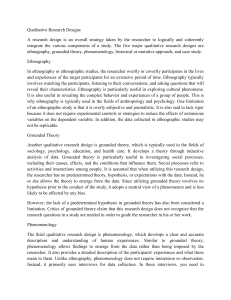
Add this document to collection(s)
You can add this document to your study collection(s)
Add this document to saved
You can add this document to your saved list
Suggest us how to improve StudyLib
(For complaints, use another form )
Input it if you want to receive answer
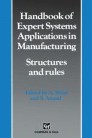
Handbook of Expert Systems Applications in Manufacturing Structures and rules pp 45–80 Cite as
A common skeletal framework for knowledge-based solutions to a representative set of manufacturing problems
- M. Marefat &
- P. Banerjee
130 Accesses
2 Citations
Part of the book series: Intelligent Manufacturing Series ((IMS))
Intelligent systems have been increasingly studied and used in the fields of flexible design and manufacture. Some recent applications include know-ledge-based systems for process planning, scheduling, facilities layout and intelligent design environments (Kumara et al , 1986; Kusiak and Chen, 1988; Steffan, 1986). The evolution of intelligent systems can be thought of as having passed through several phases. While logic and knowledge representation were the themes during the 1970s, the early 1980s were when expert systems gained popularity and the late 1980s acknowledged the development of many knowledge-based application programs as well as software tools. For the knowledge-based methods to advance, we need a deeper investigation of the fundamentals behind successful knowledge-based applications. In order to demonstrate that knowledge-based methods are not ad hoc , we need to study the common underlying themes, generalize particular solutions into problem solving methodologies, study the properties of the underlying solving structures and investigate the impact of representation. Such an investigation provides us with a framework and a deeper generic understanding of the solution methodology. This framework and understanding can be used in the new generation of intelligent systems to:
Evaluate the capabilities of certain classes of systems.
Enhance and improve the capabilities of existing and new systems through incorporation of those elements of the framework which are lacking in these systems.
Design new problem solving systems by applying the framework to particular problem domains and instantiating its key elements.
- Abstraction Level
- Hill Climbing
- Layout Problem
- Facility Layout
These keywords were added by machine and not by the authors. This process is experimental and the keywords may be updated as the learning algorithm improves.
This is a preview of subscription content, log in via an institution .
Buying options
- Available as PDF
- Read on any device
- Instant download
- Own it forever
- Compact, lightweight edition
- Dispatched in 3 to 5 business days
- Free shipping worldwide - see info
Tax calculation will be finalised at checkout
Purchases are for personal use only
Unable to display preview. Download preview PDF.
Banerjee, P. (1990) An automated reasoning architecture for a representative set of human designer tasks in manufacturing systems layout organization by designing default knowledge combining linear objective optimization and non-linear qualitative analysis, PhD Dissertation ,Industrial Engineering, Purdue University.
Google Scholar
Banerjee, P., Montreuil, B., Moodie, C.L. and Kashyap, R.L. (1990a) A qualitative reasoning-based interactive optimization methodology for layout design. HE Conference Proceedings ,230–5.
Chang, T.-C. and Wysk, R.A. (1985) An Introduction to Automated Process Planning Systems ,Prentice-Hall, Englewood Cliffs, NJ.
Choi, B.K., Barash, M.M. and Anderson, D.C. (1984) Automatic recognition of machined surfaces from a 3D solid modelor. Computer-Aided Design 16(2), 81–6.
Article Google Scholar
Davies, B.J. and Darbyshire, I.L. (1984) The use of expert systems in process planning. Annals of the CIRP ,33(1), 303–6.
Denardo, E.V. (1986) Dynamic Programming Theory and Applications ,Prentice-Hall, Englewood Cliffs, NJ.
Descotte, Y. and Latombe, J.C. (1981) GARI: A Problem Solver that Plans how to Machine Mechanical Parts . IJCAI-7, pp. 766–72.
Fisher, E.L. (1986) An Al-based methodology for factory design. AI Magazine ,7(4), 72–85.
Flemming, U., Coyne, R., Glavin, T. and Rychener, M. (1988) A generative expert system for the design of building layouts-version 2, in Artificial Intelligence in Engineering: Design ,(ed. J.S. Gero), Elsevier, Amsterdam, pp. 445–64.
Forbus, K.D. (1990) Qualitative physics: past, present, and future, in Qualitative Reasoning about Physical Systems (eds D.S. Weld and J. de Kleer), Morgan Kaufmann, San Francisco.
Foulds, L.R., Gibbons, P.B. and Gifrin, J.W. (1985) Facilities layout adjacency determination: an experimental comparison of three graph theoretic heuristics. Operations Research ,33(5), 1091–106.
Article MATH Google Scholar
Golany, B. and Rosenblatt, M.J. (1989) A heuristic algorithm for the quadratic assignment formulation to the plant layout problem. International Journal of Production Research ,27(2), 293–308.
Gossard, D.C., Zuffante, R.P. and Hiroshi, S. (1988) Representing dimensions, tolerances, and features in MCAE systems. IEEE Computer Graphics and Applications ,pp. 51–9.
Hassan, M.M.D. and Hogg, G.L. (1989) On converting a dual graph into a block layout. International Journal of Production Research ,27(7), 1149–60.
Heragu, S. and Kusiak, A. (1988) Knowledge based system for machine layout (KBML). HE Conference Proceedings ,pp. 159–64.
Hummel, K.E. (1989) Coupling rule-based and object-oriented programming for the classification of Mach. Computers in Engineering ,1, 409–18.
Ikeuchi, K. and Takeo, K. (1988) Automatic generation of object recognition programs. Proceedings of the IEEE ,76(8), 1016–35.
Jakiela, M.J. (1989) Design and implementation of a prototype ‘intelligent’ CAD system. Journal of Mechanics, Transfer and Automation in Design ,3, 252–8.
Karinthi, R.R. and Nau, D.S. (1989) Geometric Reasoning as a Guide to Process Planning . Proceedings of the ASME International Computers in Engineering Conference, July 30-August 3, pp. 609–16.
Knoll, T.F. and Jain, R.C. (1986) Recognizing partially visible objects using feature indexed hypotheses. IEEE Journal of Robotics and Automation ,RA-2(1), 3–13.
Korf, R.E. (1987) Planning as search: a quantitative approach. Artificial Intelligence , 33, 65–88.
Kumara, S.R.T., Joshi, S., Kashyap, R.L. et al . (1986) Expert systems in industrial engineering. International Journal Production Research ,24(5), 1107–25.
Kumara, S.R.T., Kashyap, R.L. and Moodie, C.L. (1988) Application of expert systems and pattern recognition methodologies to facilities layout planning. International Journal of Production Research ,26(5), 905-30. Kusiak, A. and Chen, M. (1988) Expert systems for planning and scheduling manufacturing systems. European Journal Operations Research ,34, 113–30.
Liu, C.R. and Srinivasan, R. (1984) Generative process planning using syntactic pattern recognition. Computers in Mechanical Engineering ,63–6.
Luby, S.C., Dixon, J.R. and Simmons, M.K. (1986) Design with features: creating and using a feature data base for evaluation of manufacturability of castings. Computers in Mechanical Engineering ,5(3), 25–33.
Malakooti, B. and Tsurushima, A. (1989) An expert system using priorities for solving multiple-criteria facility layout problems. International Journal of Pro-duction Research ,27(5), 793–808.
Marefat, M. and Kashyap, R.L. (1990) Geometric reasoning for recognition of three dimensional object features. IEEE Transactions on Pattern Analysis and Ma-chine Intelligence TPAMI-12(10)
Marefat, ML, Feghhi, S.J. and Kashyap, R.L. (1990) IDP: Automating the CAD/CAM Link by Reasoning about Shape . The Sixth Conference on Artificial Intelligence Applications, Santa Barbara, California.
Marefat, M., Timke, M. and Kashyap, R.L. (1990) A Framework for Image Interpre-tation in manufacturing applications . Proceedings of IEEE International Con-ference on Systems, Man, and Cybernetics (SMC), Los Angeles, California.
Minsky, M. (1963) Steps toward artificial intelligence, in Computer and Thought ,(ed. Feigenbaum and Feldman), McGraw-Hill, New York, pp. 441–3.
Montreuil, B. and Ratliff, H.D. (1989) Utilizing cut trees as design skeletons for facility layout. HE Transactions ,21(2), 136–143.
Montreuil, B., Venkatadri, U. and Ratliff, H.D. (1989) Generating a layout from a design skeleton. Document 89-01 ,Department of Operations &Decision Systems, Laval University, Quebec, Canada (to appear in Management Science) .
Mortenson, M.E. (1985) Geometric Modeling ,John Wiley &Sons, New York.
Newell, A. and Simon, H.A. (1972) Human Problem Solving ,Prentice-Hall, Engle-wood Cliffs, NJ.
Picone, C.J. and Wilhelm, W.E. (1984) A perturbation scheme to improve Hillier’s solution to the facilities layout problem. Management Science ,30(10), 1238–49.
Pinilla, J.M., Finger, S. and Prinz, F.B. (1989) Shape feature description and recognition using an augmented topology graph grammar. NSF Engineering Design Reserach Conference, June 11-14, Amherst.
Requicha, A.A.G. (1980) Representations for rigid solids: theory, methods, and systems. IEEE Computer Graphics and Applications ,12(4), 45–60.
Requicha, A.A.G. and Chan, S. (1986) Representation of geometric features, toleran-ces, and attributes in solid modelers based on constructive geometry. IEEE Journal of Robotics and Automation ,RA-2(3), 156–66.
Sacerdoti, E.D. (1974) Planning in a hierarchy of abstraction spaces. Artificial Intelligence ,5, 115–35.
Schank, R.C. (1982) Dynamic Memory: A Theory of Reminding and Learning in Computers and People ,Cambridge University Press, Cambridge.
Scriabin, M. and Vergin, R.C. (1985) A cluster-analytic approach to facility layout. Management Science ,31(1), 33–49.
Shafer, G.A. (1976) A Mathematical Theory of Evidence ,Princeton University Press, Princeton, NJ.
MATH Google Scholar
Shah, J.J. and Rogers, M.T. (1988) Expert form feature modelling shell. Computer-Aided Design ,20(9), 515–24.
Steffan, M.S. (1986) A survey of artificial intelligence-based scheduling systems. II E Conference Proceedings ,395–405.
Stefik, M. (1981) Planning with constraints (MOLGEN: Part 1). Artificial Intelli-gence ,16, 111–40.
Tsatsoulis, C. and Kashyap, R.L. (1988a) A case-based system for process planning. International Journal of Robotics and Computer-Integrated Manufacturing ,4, 557–570.
Tsatsoulis, C. and Kashyap, R.L. (1988a) A system for knowledge-based process planning. Artificial Intelligence in Engineering ,3(2), 66–75.
Wang, H.-P. and Chang, H. (1987) Automated classification and coding based on extracted surface features in a CAD data base. International Journal of Advanced Manufacturing Technology ,2(1), 25–38.
Article MathSciNet Google Scholar
Woo, T.C. (1982) Feature Extraction by Volume Decomposition . Proceedings of the Conference on CAD/CAM Technology in Mechanical Engineering, MIT, Mass-achusetts, pp. 76–94.
You, I.C., Chu, C.N. and Kashyap, R.L. (1989) Expert system for castability evaluation using a fixed-features based approach. Robotics and Computer-Integrated Manufacturing ,6(3)
Zeigler, B.P. (1990) Object-Oriented Simulation with Hierarchical Modular Models ,Academic Press, New York.
Download references
You can also search for this author in PubMed Google Scholar
Editor information
Editors and affiliations.
Industrial Engineering, University of Cincinnati, Cincinnati, USA
A. Mital & S. Anand &
Rights and permissions
Reprints and permissions
Copyright information
© 1994 Springer Science+Business Media Dordrecht
About this chapter
Cite this chapter.
Marefat, M., Banerjee, P. (1994). A common skeletal framework for knowledge-based solutions to a representative set of manufacturing problems. In: Mital, A., Anand, S. (eds) Handbook of Expert Systems Applications in Manufacturing Structures and rules. Intelligent Manufacturing Series. Springer, Dordrecht. https://doi.org/10.1007/978-94-011-0703-7_3
Download citation
DOI : https://doi.org/10.1007/978-94-011-0703-7_3
Publisher Name : Springer, Dordrecht
Print ISBN : 978-94-010-4302-1
Online ISBN : 978-94-011-0703-7
eBook Packages : Springer Book Archive
Share this chapter
Anyone you share the following link with will be able to read this content:
Sorry, a shareable link is not currently available for this article.
Provided by the Springer Nature SharedIt content-sharing initiative
- Publish with us
Policies and ethics
- Find a journal
- Track your research

Peer Recognized
Make a name in academia
Research Paper Outline with Key Sentence Skeleton (+Paper Template)
A well-crafted research paper outline can make the process of wiring a journal paper smooth and efficient. The problem is that it is difficult to compose a realistic paper outline before starting to actually write the paper itself. Sure, the main headings in a typical scientific paper are largely the same, but to experience any real productivity benefits from a paper outline, you will need more than just top-level headings.
In this article, I will show you how to efficiently kick-start the research paper writing process in two steps:
- Use a key sentence skeleton to describe the main message of your research paper.
- Create a thorough research paper outline using the key sentences that you just developed.
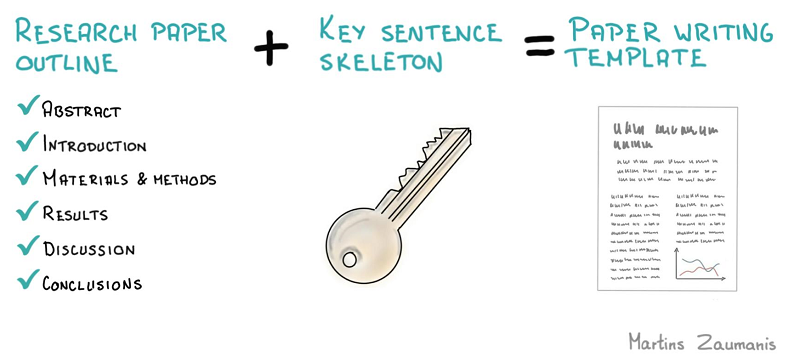
Together the research paper outline and the key sentence skeleton will serve as a blueprint for the process of writing the full research paper. Following this writing process should make writing more efficient and the finished paper – clearer.
Whenever I start to work on a new research paper, I open my writing template that includes the research paper outline and the key sentence skeleton. The template will also help with automated formatting.
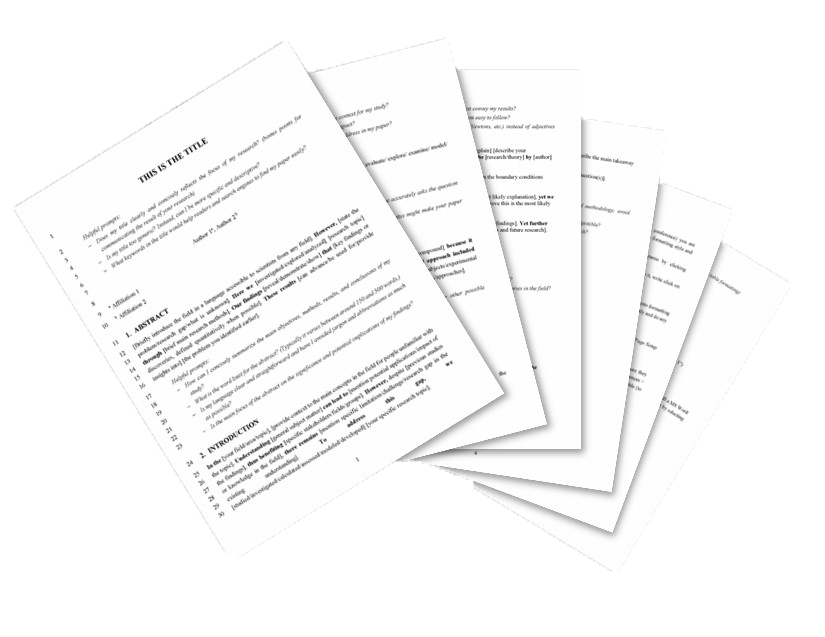
What exactly is a Research Paper Outline?
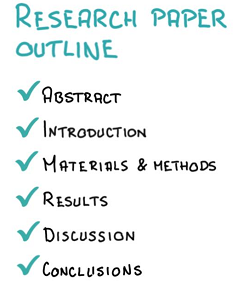
Picture yourself planning a cross-country road trip with your friends. What’s the first thing you do? You look at a map, right? Well, a research paper outline is your trusty map for writing a full research paper. A well written research paper outline will keep you on track and help you avoid getting lost.
The benefits of creating a research paper outline:
- The research paper outline will ensure that you know what to write in which section thus making the research paper writing process more efficient.
- A thorough paper outline will shave off some of those those tedious re-writes because your initial plan will enable you to write clearer right from the first draft.
- Agreeing on the paper outline among the co-authors (and supervisor) before starting to write will allow you to avoid any unnecessary quarrels and re-writes later on.
What exactly is a Key Sentence Skeleton?
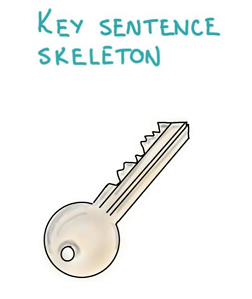
A good research paper should clearly communicate one central message. The paper’s key sentences help in defining this message. You will know that the sentences are complete if you are able to bind them together and use them as a short summary of your paper.
For each section of the research paper, I will provide you with a key sentence skeleton that holds filler words and square brackets which you can replace with your own text. Written well, the key sentences will hold the most essential information about your research.
The key sentences are a great way to kick off the writing process since you will no longer be staring at a blank screen. After finalizing the sentences, your next task will be to add more detail and evidence to substantiate them.
The key sentences help with another thing: they will force you to think about the message your want to convey with your research paper. This will allow you to further refine the research paper outline by including subheadings.
Research paper structure
The title of your research paper is the first thing readers encounter. They will use the title to decide whether they want to invest the time in reading your paper. Think of the title as the ultimate summary of your research paper. Spend enough time to come up with a clear, intriguing title that convinces that your research paper is worth reading.
To come up with a good title for your article, first create a list of keywords and short phrases that:
▪ Describe the problem you will address,
▪ Summarize the main idea of your research,
▪ Demonstrate the impact that your paper has.
Now, try mixing up different combinations of these words to come up with a title of no more than 10 words. Try to prepare a list of at least five potential titles ranging from descriptive to snappy and exciting. Then you can ask your peers to help you choose the winner.
Key questions to consider for the Title of a research paper:
- Does my title clearly and concisely reflects the focus of my research (bonus points for communicating the result of your research)?
- Is my title too generic? Can I be more specific and descriptive?
- What keywords in the title would help readers and search engines to find my paper easily?
The abstract is like the executive summary of your research paper – a concise overview that gives readers a taste of what’s to come. It helps people who are shopping for research papers decide whether your study aligns with their interests and warrants spending their time to read the entire paper.
To make sure the abstract accurately reflects the paper content, write it after completing your research paper.
Key questions to consider for an Abstract of a research paper:
- How can I concisely summarize the main objectives, methods, results, and conclusions of my study?
- What is the word limit for the abstract? (Typically it varies between around 150 and 500 words.)
- Is my language clear and straightforward and have I avoided jargon and abbreviations as much as possible?
- Is the main focus of the abstract on the significance and potential implications of my findings?
Key sentences for Abstract
[Briefly introduce the field in a language accessible to scientists from any field]. However, [state the problem/research gap/what is unknown]. Here we [investigated/explored/analyzed] [research topic] through [brief main research methods]. Our findings [reveal/demonstrate/show] that [key findings or discoveries, defined quantitatively when possible]. These results [can advance/be used for/provide insights into] [the problem you identified earlier].
Introduction
The introduction is one of the most challenging sections of the research paper to write. It should leave no doubt in the mind of the reader that what you are doing is important and that this work could push scientific knowledge forward. To demonstrate this convincingly, you will need to have a good understanding of the state-of-the-art in your field. Try to zoom out to see the bigger picture in order to demonstrate the potential impacts of your work.
Start the introduction with a brief context, followed by the motivation for the study. Next, explain the research gap you are going to bridge and what approach you will take to do it. You can also present the structure of the research paper.
Key questions to consider for the Introduction of a research paper:
- What background information is necessary to provide context for my study?
- Why is the topic significant and what is its potential impact?
- What is the main research problem or question that I address in my paper?
- How did I address this problem with my research?
Key sentences for Introduction
- In the [your field/area/topic], [provide context to the main concepts in the field for people unfamiliar with the topic]. Understanding [your subject matter] can lead to [mention potential applications/impact of the findings], thus benefiting [specific stakeholders/fields/groups]. However, despite [previous studies or knowledge in the field], there remains [mention specific limitation/challenge/research gap in the existing understanding]. To address this gap, we [studied/investigated/calculated/assessed/modeled/developed] [your specific research topic].
The objective statement defines the specific goals of your study, allowing the readers to understand what they can expect from your paper. Make it short and sweet: no long sentences or winding explanations. Just clearly state the main objective of the study, followed by sub-objectives if any.
Key questions to consider for Objective statement of a research paper:
- Can I list my research objective(s) as a concise statement (preferably one sentence)?
- Does the objective accurately present the question that is answered in the paper? (Do this after you have finished the paper.)
- Can I narrow down to one or two main objectives? (Many objectives might make your paper less focused and memorable.)
Key sentences for Objective
The main objective of this research is to [determine/assess/design/evaluate/explore/examine/model/apply/calculate] [describe the specific aim or purpose of the research].
In the methods section, describe your research design and methodology in enough details that would allow the readers to reproduce the study. This means that if you are using a novel method, you will have to describe it thoroughly. If, on the other hand, you applied a standardized method, or used an approach from another paper, it will be enough to briefly describe it and add a reference to the detailed original source.
Key questions to consider for Methods section of a research paper:
- Why did I select this research design to reach my study’s objectives over other possible approaches?
- Which data collection methods and instruments did I use for my research?
- What details are necessary to reproduce my results?
- What statistical or analytical techniques will I use to analyze my data?
- How did I address ethical considerations in my research (if any)?
- Can I create a methodology flowchart or a table that summarizes the steps taken?
Key sentences for Methods
To achieve the objective, we selected the [research design/method/approach/compound] because it [justify your motivation for choosing this over other approaches]. The selected approach included [describe specific steps/data collection methods/participants/subjects/experimental setup/population/surveys]. Data analyses were conducted using [specific techniques/approaches].
In most cases, it makes sense to combine the Results and Discussion sections because for most readers the results alone without an explanation will be meaningless. Here, however, I want to show you the distinction between these to sections and provide a unique key sentence for each, so I present these sections to you separately.
Organize and present your results in a sequence that makes it easy for the reader to follow the evolution of your logic. This is rarely the same chronological sequence in which you did the actual research.
In almost any research paper, you will be presenting the collected data or explaining certain scientific concepts. Most usually this is done using data charts or scientific visualizations. Creating these figures is a great place to start writing your results section. This will force you to think about the results and the ways you can best communicate them with your readers.
I wrote separate article that will show you tools you can use for creating data charts and another article that compares the different tools for scientific visualizations.
Once you have created the data charts, tables, and/or visualizations, describe what you see in them. Seek to answer the question: What have I found? Your statements should progress in a logical sequence and be backed by the visual information. Since, at this point, you are simply explaining what everyone should be able to see for themselves, you can use a declarative tone: The figure X demonstrates that…
Key questions to consider for Results section of a research paper:
- What data visualizations or scientific illustrations will best convey my results?
- In what sequence should I organize my results to make them easy to follow?
- Can I use quantitative descriptions (how many percent/ Newtons, etc.) instead of adjectives (high, little, poor)?
Key sentences for Results
The [figure/table/result] demonstrates that [describe key results shown in the table/chart/graphic/visualization].
The discussion rather than the results are the heart of any research paper. To demonstrate why your results are worthy of publishing you must now answer the all-important So what? question . How well you do so will ultimately define the success of your paper.
In the discussion you interpret the results and show their significance; you make sense of the results by showing relationships, patterns, correlations; you show how your research aligns (or doesn’t) with previous research and the scientific theory. In other words, you guide your readers through the maze of the results to present the explanation that links your results back to the objective of the paper.
It is not, by any means, easy to come up with a meaningful discussion. Sometimes you will be confronted by results that do not agree with your initial hypothesis and are difficult to explain. But don’t give up. Just make sure that you put in the time and effort to analyze the results thoroughly.
To help the reader follow the discussion, take advantage of subheadings to group related paragraphs together.
Key questions to consider for Discussion of a research paper:
- What do my results mean?
- Are my claims backed by the results?
- Was the objective of my study achieved?
- How do my results fill the research gaps identified in the literature search?
- How do my findings align with or contradict previous studies or existing theories in the field?
- What are the potential impacts of my findings?
- Could there be an alternative explanation for my results?
- What limitations of my study should I acknowledge?
- Are there any unexpected or surprising results that require further exploration?
- What conclusions can be drawn from my results?
Key sentences for Discussion
The results [suggest/indicate/demonstrate/show/provide insight into/explain] [describe your interpretation of the results]. This finding [aligns with/contrasts with] the [research/theory] by [author] in that [explain the similarities/differences].
The provided result interpretation is [constrained by/limited to] [explain the boundary conditions beyond which your results should not be extrapolated].
Another possible explanation of the results is that [lat out the second most likely explanation], yet we believe this interpretation is less likely because [explain why you don’t believe this is the most likely interpretation].
Our results could be used to [explain the possible impacts/significance of the findings]. Yet further research is necessary to [determine/conclude] if [discuss unanswered questions and future research].
Conclusions tend to be easy to write because, for the most part, they just summarize the findings from the discussion . Either paragraph style or bullet-point style conclusions can be used. I prefer the bullet-point style because it clearly separates the different conclusions and provides an easy-to-digest overview for the casual browser. It also forces me to be more succinct.
In the first few sentences of conclusions, briefly re-state the problem and the methodology. This helps since many readers directly scroll to conclusions before reading any other section.
After the introductory sentences, summarize your findings and provide the main takeaway from your research paper. At the end of the conclusion section, consider including a paragraph reiterating the central message of the paper. You could also note just how your work contributes to filling the knowledge gap you identified in the introduction. In other words, show how the work advances the field from its present state of knowledge.
Key questions to consider for conclusion of a research paper:
- How can I succinctly summarize the main findings of my study?
- Can I make the conclusions standalone (e.g. restate the problem and methodology, avoid abbreviations)?
- Can I use bullet point to make the conclusions more succinct and easily digestible?
- What are the broader implications and potential applications of my research?
- Have I answered the question that was posed in the paper’s objectives?
- Can I explain what gaps in existing knowledge have I filled?
- What are the practical recommendations and impact of my research?
Key sentences for Conclusion
In this paper we [researched/addressed/tackled]. Based on the results reported in this paper, the following conclusions are drawn:
- [describe conclusion No.1]
- [describe conclusion No.2]
- [describe conclusion No.3]
In summary, the results [demonstrate/prove/showcase/explain/highlight] [describe the main takeaway message].
Future research should [determine/answer/include] [describe the unanswered question(s)].
Appendices include supplementary data, charts, graphs, or any information that might interest readers but doesn’t belong in the main body of your paper.
Key question to consider for appendices:
- What detailed data, technical details, or lengthy calculations should I include in the appendicies?
- Can I upload my results into a data repository rather than submitting as an appendix?
- Have I referred to the appendices within the main body of my research paper where appropriate?
To sum it all up
Writing a research paper is not nearly as difficult if you start with a strong paper outline, a key sentence skeleton, and writing prompts. In this article, I provided you with these tools in a single research paper writing template .
As a reminder, here is a research paper writing workflow that will allow you to come up with a solid research paper:
In this article I provided you with a typical research paper outline that includes: Title, Abstract, Introduction, Objective, Methods, Results, Discussion, Conclusions, Appendices.
For each paper section, I gave you a key sentence skeleton. Writing these sentences before starting to fully dig into writing each individual section will help you to refine the paper outline and define the main message of your paper.
To help you fully write each research paper section, I provided you with helpful prompts. They will get you thinking about how to better formulate each section. Read this article to see how to write a research paper most efficiently.
Have fun writing your next research paper!
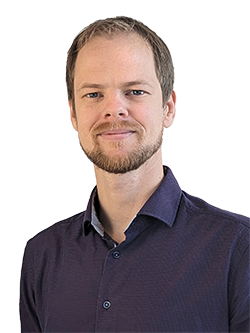
Hey! My name is Martins Zaumanis and I am a materials scientist in Switzerland ( Google Scholar ). As the first person in my family with a PhD, I have first-hand experience of the challenges starting scientists face in academia. With this blog, I want to help young researchers succeed in academia. I call the blog “Peer Recognized”, because peer recognition is what lifts academic careers and pushes science forward.
Besides this blog, I have written the Peer Recognized book series and created the Peer Recognized Academy offering interactive online courses.
Related articles:
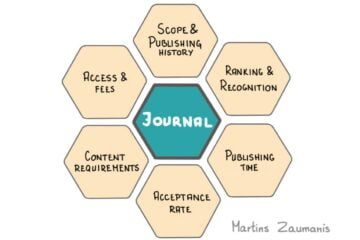
One comment
- Pingback: Download a research paper cover letter template - Peer Recognized
Leave a Reply Cancel reply
Your email address will not be published. Required fields are marked *
I want to join the Peer Recognized newsletter!
This site uses Akismet to reduce spam. Learn how your comment data is processed .
Privacy Overview
Copyright © 2024 Martins Zaumanis
Contacts: [email protected]
Privacy Policy
- --> Publisher Home

EXPLORING EMERGING TRENDS IN NURSING DURING COVID-19: A SKELETAL FRAMEWORK ANALYSIS.
Main article content.
Covid-19, Technology Era, Trends, Nursing, Patient Care, Robotics, AI.
Our skeletal framework research reveals important themes and difficulties experienced by nursing practitioners as it examines developing patterns in nursing during the COVID-19 pandemic. The quick uptake of telemedicine, the increased emphasis on infection control, and the growing recognition of the mental health needs of nurses highlight how dynamic nursing practice is in this crisis. Moreover, the pandemic has highlighted the need for a more equitable approach to healthcare delivery by bringing to light already-existing health inequities. The role of nurses in overcoming these issues has been crucial, as seen by their adoption of resilience-building tactics, advocacy for underrepresented groups, and flexibility in providing virtual care. In other words, nursing is facing multiple and numerous technological advancement such as robotics, Artificial intelligence (AI), health mobile applications, personalized patient medicines, and other. Nursing professionals may ensure the continuous progress of the nursing profession despite the COVID-19 epidemic by addressing these developing trends and improving patient care and outcomes in the face of unprecedented circumstances.
Article Sidebar
Article details.

This work is licensed under a Creative Commons Attribution-NonCommercial 4.0 International License .
All articles published in JPTCP are licensed under Copyright Creative Commons Attribution-NonCommercial 4.0 International License.
Alsahli, Norah Hassan Mohammed
KSA, Ministry of Health
Alzahrani ,Naif Hassan Mohammed
Almutairi ,hamad hadi, almutairi ,malik awadhalla, almutairi,abdulaziz dakhilullah, almutairi ,fahad dakhilullah, aldawsari ,fahad mushi dhafer, alegaily, bahjat aseme a., almutairi,sultan razqan, almekbel, latifah saad, alhawsawi,bashaer hashim, shatarah, hanaa baker, modal header.

An official website of the United States government
The .gov means it’s official. Federal government websites often end in .gov or .mil. Before sharing sensitive information, make sure you’re on a federal government site.
The site is secure. The https:// ensures that you are connecting to the official website and that any information you provide is encrypted and transmitted securely.
- Publications
- Account settings
Preview improvements coming to the PMC website in October 2024. Learn More or Try it out now .
- Advanced Search
- Journal List
- Int J Environ Res Public Health

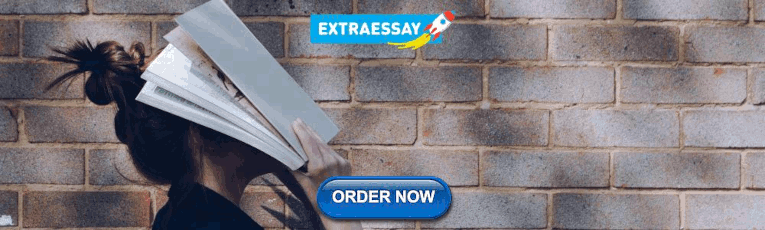
The Effectiveness of Workplace Musculoskeletal Injury Risk Factor Screening Tools for Reducing Injury: A Systematic Review
Richard roberts.
1 School of Public Health, University of Alberta, Edmonton, AB T6G 1C9, Canada
2 Faculty of Rehabilitation Medicine, University of Alberta, Edmonton, AB T6G 2G4, Canada
Don Voaklander
Sebastian straube.
3 Division of Preventive Medicine, Department of Medicine, University of Alberta, Edmonton, AB T6G 2R3, Canada
Liz Dennett
4 John W. Scott Health Sciences Library, University of Alberta, Edmonton, AB T6G 2R7, Canada
Carol Cancelliere
5 Faculty of Health Sciences, Ontario Tech University, Oshawa, ON L1H 7K4, Canada
Christine Guptill
6 School of Rehabilitation Sciences, University of Ottawa, Ottawa, ON K1H 8M5, Canada
Linda Miller
7 EWI Works, Edmonton, AB T6E 3N8, Canada
Danielle Lemay
8 Occupational Hygiene & Product Safety, Suncor Energy, Calgary, AB T2P 3E3, Canada
Melnard De Leon
9 Department of Physical Therapy, University of Alberta, Edmonton, AB T6G 2G4, Canada
Douglas P. Gross
Associated data.
Data available upon request from the authors.
Introduction: Musculoskeletal injury (MSI) contributes to global health burdens. Effective MSI prevention is necessary. MSI risk factor screening tools can be used by employers to identify and mitigate occupational hazards. Rigorous synthesis of the effectiveness of these tools has not taken place. We synthesized literature on effectiveness of MSI risk factor screening tools for reducing injury through informing prevention interventions. Materials and Methods: A literature search of Medline, Embase, Cochrane Library (Trials), CINAHL, Scopus and PsycINFO databases was performed. Included studies required an analytic design, used an MSI risk factor screening tool to inform an intervention in a working-age population and reported an outcome of MSI development, injury or compensation/work absence. Data extraction and study quality rating (Downs and Black criteria) were completed. Studies were sub-categorized as having used a single MSI screening tool (single-tool) to inform an injury prevention intervention or involving multiple simultaneous screening tools (multiple-tool). Study outcomes were synthesized when possible. Results: Eighteen articles representing fourteen studies met our inclusion criteria. No high-quality studies were identified (maximum Downs and Black score of 19). Studies did not employ previously validated tools but instead, typically, those purpose-built for a single use. The results were inconsistent both when using tools alone and in combination with other tools. Outcome measure heterogeneity precluded meaningful meta-analysis. Conclusions: There is limited evidence regarding use of MSI risk factor screening tools for preventing injury. Rigorous studies that utilize previously validated tools are needed.
1. Introduction
Musculoskeletal injuries (MSI) are among the largest contributors to the global burden of pain, disability and work loss [ 1 ]. The prevalence of MSI is increasing worldwide, most notably among low- and middle-income countries [ 2 ]. We lack a unified international-level strategy to prioritize their treatment, as exists more generally for communicable diseases [ 2 ]. Given these substantial burdens and alongside current treatment barriers, there is a definitive need for strategies that mitigate MSI symptoms or prevent incident MSI (primary prevention) [ 3 ]. The latter strategy is especially important and can be enacted through targeted and effective interventions in populations that are most at risk of MSI. Workers exposed to physical loading in the workplace are a key population for these targeted approaches. Since 2000, occupational exposure causing neck and back pain has alone contributed nearly 14% of all occupational disability-adjusted life years globally [ 4 ]. Occupational health and safety regulations often have employers identify, assess and control or reduce occupational risk factors associated with MSI. Various MSI risk factor screening tools exist and are aimed at risk identification. These tools include, but are not limited to, questionnaires as well as observational criteria to identify types of workload risk—including intensity, frequency or duration of tasks [ 5 ]. A recent scoping review identified 19 different risk assessment tools, concluding that this was a “large number of observational assessment tools” [ 5 ]. In a North American context, MSI screening tools most commonly used by occupational health professionals across job sectors include the NIOSH Lifting Equation, Rapid Upper Limb Assessment (RULA) and Rapid Entire Body Assessment (REBA) [ 6 ].
Throughout this review, MSI risk factor screening tools are viewed in the context of informing interventions to prevent MSI and its effects. Previous research has reviewed the measurement properties of MSI risk factor screening tools, with varying reliability and validity reported [ 7 ]. Reliability appears to vary across items within individual tools and depends on rater experience [ 8 ]. However, the primary goal of using these tools is to reduce the risk and consequences of MSI in the workplace. To reduce risk of reported MSI, these tools typically inform use of specific interventions that directly address and mitigate the risks identified by the tool. In fact, several tools recommend application of specific interventions that are contingent on risk levels or scores identified by the tool. For example, the RSI QuickScan questionnaire is an MSI risk factor screening tool that establishes a risk profile for arm, shoulder and neck symptoms [ 9 ]. This information then informs tailored interventions based on the individual risk profile, with 16 possible interventions implemented based on a decision tree algorithm. The recommendations are thus an integral part of the tools’ scoring. Therefore, in the context of informing interventions, the effectiveness of an MSI screening tool depends on how accurately the tool identifies risk factors as well as how effectively it informs the implementation of (a) targeted prevention intervention(s). Despite the apparent breadth of MSI risk factor screening tools, some researchers have raised concerns about their utility and effectiveness [ 10 , 11 ]. MSI risk factor screening tools are typically developed using biomechanical, laboratory or consensus studies rather than through methodologically rigorous trials in actual work environments. Furthermore, rationales for adoption of industrial standards and threshold limits for workload exposures have been criticized as lacking rigor or transparency. Armstrong et al. recommend a solution: formal evaluation of these risk assessment procedures using the same techniques required for medical or public health standards [ 11 ].
Research is needed to assess the current scientific literature involving evaluation of the effectiveness of MSI risk factor screening tools for informing injury prevention interventions through a rigorous epidemiological lens. This will provide critically important information regarding whether these tools can successfully be used to prevent MSI and its consequences. Therefore, our research question was: “in working age adults, what is the effectiveness of MSI risk factor screening tools for preventing onset and consequences (i.e., pain, disability, quality of life, work loss and reduced productivity) of work-related MSI?”
2. Materials and Methods
This review followed Preferred Reporting Items for Systematic Reviews and Meta-Analysis guidelines [ 12 ]. The review protocol was registered with the International Prospective Register of Systematic Reviews (CRD42021232747).
A systematic literature search was carried out by a health sciences librarian (L.D.) in Medline via Ovid (1946–18 March 2021), Embase via Ovid (1974–18 March 2021), Scopus (searched 19 March 2021), CINAHL Plus with Full Text (via EBSCOhost) (1937–19 March 2021), Wiley Cochrane Central Register of Controlled Trials (CENTRAL) (searched 19 March 2021) and APA PsycINFO (1806–November Week 3, 2021) databases. Team members collaborated to develop a sensitive search strategy that utilized two approaches: (1) searching by the names of specific tools identified in a preliminary literature review or provided by stakeholders ([L.M., D.L.]), and (2) searching generically with combinations of subject headings and keywords pertaining to MSI, occupational settings and screening tools. The results of both approaches were limited to quantitative primary research studies only. The grey literature was not searched, which is a change from our protocol. After preliminary searching of the voluminous grey literature, it was determined that this searching would not result in rigorous evaluations, which was the focus of this study. Our definition of MSI was adapted from WorkSafeBC’s definition that encompasses injuries and disorders of muscles, tendons, ligaments, joints and soft tissues (nerve and vascular injury) [ 13 ]. For this study, we did not include generic search terms for vascular, nerve or vibration-induced injuries but did include specific search terms for carpal tunnel syndrome. The full search strategy is available (see Supplementary Material S1 ).
A PICOS (Population, Intervention, Comparison, Outcome, Study Design) framework was used for development of article inclusion criteria. Articles were required to have an English-language title and abstract and were eligible if they assessed:
P—a working-age population in a working environment (exclusion of pre-employment screening and studies in military populations);
I—applied an MSI risk factor screening tool using individual or workplace-related risk factors to prevent MSI injury and its related consequences (exclusion of studies reporting only measurement properties, such as predictive validity);
C—compared to other MSI prevention strategies that were not informed by an MSI risk factor screening tool;
O—reported on at least one primary outcome related to MSI development, injury or compensation/insurance claims (a variety of secondary outcomes were also examined, including workplace MSI risks and behaviors, as well as any self-reported MSI outcomes, such as pain, disability, discomfort, etc.);
S—utilized an analytic study design (i.e., randomized clinical trial; cohort, case-control study, quasi-experimental studies).
We expected that MSI risk factor screening tools would affect injury outcomes by conditionally applying a specific prevention intervention based on risk levels or scores identified by the screening tool. Tool results inform implementation of a tailored intervention aimed at mitigating any identified risk factors. For example, if the tool identifies excessive physical loading as a risk, the recommendation may be to modify work tasks or activities to reduce risk or have workers undergo a fitness or strengthening program to increase their manual handling abilities. A variety of risk factor/intervention combinations are possible for both physical and mental risks at the workplace. This process is conceptualized in a logic map in Figure 1 .

Logic map showing how musculoskeletal injury (MSI) risk factor screening tools inform interventions to affect MSI-associated outcomes.
We made modifications to our review protocol prior to our analysis. Specifically, to capture all potentially relevant articles, studies did not require a minimum sample size to be included. Additionally, we clarified that eligible study populations must not have been identified as injured prior to study enrolment; thus, eligible outcomes became incident MSI, compensation claims or insurance claims.
Following completion of the database search, article titles and abstracts were added to online review manager Covidence [ 14 ] and de-duplicated. Titles and abstracts were then independently screened for initial inclusion by research team members. If two research team members concluded that an article potentially met inclusion criteria, or that eligibility could not be ascertained from title and abstract alone, the full-text article was obtained. Disagreements at abstract stage were resolved first by consensus and then by a senior research team member if any remained unresolved.
Full-text articles were independently assessed for eligibility by a smaller subset of the research team. Articles had to be deemed eligible by two team members, and disagreements at full-text stage were resolved by consensus prior to or after consulting the third team member. Articles for which consensus was not reached at the full-text stage were provided to the entire research team for discussion. Additional articles were identified directly for full-text eligibility screen through citation searching of included articles and systematic reviews identified during screening. One article was identified as a subsequent analysis of a study population from an article included at full-text stage and was retrieved for full-text eligibility screening.
A standardized spreadsheet was used for data extraction of included articles. One reviewer performed the initial data extraction, with verification by a second reviewer. Extracted article data included study design, study setting and context, participant characteristics, MSI screening tool descriptions and alternative treatments of study arms, outcome measure descriptions and reported outcome results. Effect estimates were presented where possible.
Included articles were synthesized depending on their method of MSI risk factor screening tool application. The first category of “single-tool” articles contains studies that, in at least one study arm, applied a single MSI screening tool to inform an intervention in isolation from any other additional screening tools, assessments or interventions. These study designs provide the most direct assessments of MSI screening tool effect. The second category of “multiple-tool” articles applied one or more MSI risk factor screening tools in combination with other assessments and interventions (that may or may not have been informed by the screening tool of interest). For this latter group of articles, it was deemed that the causal effect of any single MSI screening tool use could not be meaningfully isolated from the causal effect of distinctly separate but concurrently applied assessments and associated interventions. Consultation with community partners indicated that prevention interventions within industry contexts are most often pragmatically applied in “multiple-tool” situations.
The Downs and Black (D&B) quality assessment checklist was used to assess included article quality [ 15 ]. The quality assessment checklist contains 27 questions assessing quality of reported material, internal validity stemming from selection bias, information bias and confounding as well as external validity and study power [ 15 ]. The checklist is appropriate for quasi-experimental, cohort and randomized control trial (RCT) study designs, allowing simple comparison between a plurality of study methodologies. The Downs and Black score was assigned out of a total possible 28 points for each article. Score interpretation has previously used quality bands of excellent (>25), good (20–25), fair (15–19) and poor (≤14) article quality [ 16 ].
This review follows principles of best evidence synthesis and incorporates components of Synthesis Without Meta-analysis reporting guidelines, the latter of which is intended to complement PRISMA reporting guidelines [ 17 , 18 ]. All included articles of medium quality or higher (D&B of fair or better) were retained for narrative synthesis. Study outcome categorizations were adapted from the original protocol and included musculoskeletal discomfort, work absence, health resource utilization, changes to workplace behaviour, self-assessed health status, workplace-related MSI and claims cost. Outcome metrics were standardized using effect direction, as recommended by Boon and Thomson (direction reported if >70% of categorized study outcomes had similar direction of effect), with consistency of evidence for these outcomes assessed using an effect direction plot adapted from the same authors [ 19 ]. A sign test was not performable for assessment of outcome heterogeneity due to too few articles. An algorithm for evidence level (strong to insufficient) was adapted from the Institute for Work and Health [ 20 ] ( Table 1 ). Any materials used in the review are available from the authors.
Decision algorithm for level of evidence. Adapted from the Best Evidence Synthesis Guidelines used by Kennedy et. al [ 20 ].
The initial database search yielded 12,207 results, and 4025 duplicates were removed; 8182 articles were screened for potential eligibility, of which 79 full-text articles were reviewed for inclusion. Percent agreement during abstract screening ranged from 88% to 100%, and all discrepancies were resolved through consensus. Fourteen articles met the inclusion criteria following full-text review and were included for analysis. Citation searching from the included articles, key systematic reviews and incidental related articles yielded 15 articles that were retrieved for full-text analysis. Four articles were retained from this second identification group. In total, 18 articles were included for quality assessment and data extraction. Most articles excluded at the full-text stage did not evaluate the effect of an MSI risk factor screening tool (see Figure 2 ).

PRISMA 2020 flow diagram for new systematic reviews which included searches of databases, registers and other sources [ 12 ].
Article quality appraisal was applied using the Downs and Black criteria ( Supplementary Table S2 ). Articles of at least medium quality (Downs and Black score band of fair; see Table 1 ) were retained for narrative synthesis. No high-quality articles were identified.
3.1. Characteristics of Single-Tool Articles
Five single-tool articles representing four studies were identified and retained following quality assessment. All five articles were scored as medium-quality, meeting at least half of the methodological criteria [ 9 , 21 , 22 , 23 , 24 ]. Positives included reporting of most necessary information, real-world study environments, reasonable intervention compliance, low likelihood of influence from participants lost to follow-up and typically adequate power. Negatives included poor reporting of potential adverse events or characteristics of participants lost to follow-up, poor generalizability from participant selection and sampling methodology, mixed accuracy of outcome measures and some incomplete adjustment for potential confounders.
Supplementary Table S3 (upper half) summarizes the characteristics of the retained single-tool studies, all of which are RCTs. One study, reported in two articles, assessed an MSI screening tool and tool-guided interventions based on occupational health [ 9 ] and economic [ 24 ] outcomes. The study participants were either computer users [ 22 , 23 ] or part of a general working population [ 9 , 21 , 24 ]. Participants were followed anywhere from 2 weeks to 2 years [ 21 , 22 ] following tool use, and screening tool arm sample sizes ranged from 35 to 1374 participants [ 21 , 23 ]. MSI risk factor screening tools were used in these studies to inform a variety of work modifications, including administrative controls and physical hazard elimination [ 21 ], ergonomic workplace adjustment [ 22 , 23 ] and a multicomponent intervention program [ 9 , 24 ]. Data sources for outcome measures included self-report questionnaires [ 9 , 22 , 23 , 24 ], daily symptom diaries [ 23 ] and company-provided occupational data [ 9 , 21 , 24 ]. Other comparator arms included tool-assisted risk assessment but withholding tool recommendations until completion of follow-up [ 9 , 22 , 24 ] and providing a variety of general [ 23 ] or specific [ 21 ] occupational health information to participants. The computer-user studies focused heavily on measurements of musculoskeletal discomfort [ 22 , 23 ] while also including some behavioural change measures. The general working population studies more frequently reported measures of work absence [ 9 , 21 , 24 ], and one included resource utilization measures [ 24 ].
3.2. Characteristics of Multiple-Tool Articles
Thirteen multiple-tool articles were identified [ 25 , 26 , 27 , 28 , 29 , 30 , 31 , 32 , 33 , 34 , 35 , 36 , 37 ], and seven, representing five studies, were retained following quality assessment [ 26 , 27 , 28 , 30 , 35 , 36 , 37 ]. All seven retained articles were scored as medium-quality. Compared to the single-tool articles, the multiple-tool articles described confounding variables and patients lost to follow-up less frequently and did not provide a priori indicators of follow-up articles for related same-study articles. The multiple-tool study populations did, however, have higher representativeness of their source populations.
Supplementary Table S3 (lower half) summarizes the characteristics of the retained multiple-tool studies. One study encompassed three follow-up articles [ 26 , 27 , 28 ] published from 2002 to 2005, with an original 2001 article not retained due to poor article quality [ 25 ]. Study design variety was larger in these studies, with three quasi-experimental study designs [ 26 , 27 , 28 , 30 , 36 ] and two RCTs [ 35 , 37 ]. Participants in the studies included health workers from Canada [ 30 ] and Australia [ 26 , 27 , 28 ], construction workers from the Netherlands [ 35 ], foundry workers from Italy [ 36 ] and farmers from the United States [ 37 ]. Follow-up was typically longer than included study counterparts—12 months at minimum. The range of sample sizes was comparable with the included studies. Data sources for the retained multiple-tool studies included workplace-associated records [ 26 , 27 , 28 , 35 , 36 ], insurance compensation documents [ 26 , 27 , 28 ], regional occupational health records [ 30 ] as well as self-report forms [ 35 , 37 ] and standardized phone calls [ 37 ]. Five studies reported count or rate outcomes of workplace-associated MSI [ 26 , 27 , 28 , 30 , 36 , 37 ], all but one reported a measure of work absence [ 26 , 27 , 28 , 35 , 36 , 37 ], one reported a measure of musculoskeletal discomfort [ 35 ] and three reported a measure of claims cost [ 26 , 27 , 28 , 37 ]. One study reported on measures of other healthcare utilization [ 37 ] and another reported on self-assessed health status [ 35 ].
Six articles were scored as poor quality and are not characterized in this paper beyond their quality appraisals [ 25 , 29 , 31 , 32 , 33 , 34 ]. Compared to the retained articles, these poor-quality articles less frequently reported on study characteristics, were significantly less representative of their source populations, did not necessarily recruit comparable groups for screening tool use and control groups, did not adequately adjust for differing participant follow-up time or confounding by other means and used less valid outcome measurement instruments.
3.3. Synthesis of Included Study Results
Table 2 presents the effect direction plot showing consistency of outcomes for the included studies. In total, seven outcome categories were provided from the included studies—musculoskeletal discomfort, work absence, health resource utilization, work behavior modification, workplace-associated MSI, claims cost and self-rated health status. The results according to these outcomes are shown below.
Effect direction plot for retained included studies.
In this plot, upward arrow ▲ indicates positive health effect, downward arrow ▼ indicates negative health effect and sideways arrow ◄► indicates no change/mixed/conflicting findings. Final sample size in intervention group: large arrow▲ indicates >300, medium arrow ▲ indicates 50–300 and small arrow ▲ indicates <50. Study quality (Downs and Black) for all included studies was medium.
No high-quality studies are present in the analysis, and each study utilizes a different MSI risk factor screening tool. Therefore, there is insufficient evidence to determine the effect of any specific MSI risk factor screening tool on any of the previously identified outcome categories.
3.4. Effects on Musculoskeletal Discomfort
Three medium-quality single-tool studies show either conflicting evidence [ 22 , 23 ] or no change [ 9 , 24 ] in musculoskeletal discomfort measures following their respective MSI-risk-factor-screening-tool-guided interventions. One medium-quality multiple-tool study shows no change [ 35 ] in musculoskeletal discomfort measures following use of an MSI risk factor screening tool as an intervention component. Therefore, there is limited evidence that MSI risk factor screening tools either do not affect or inconsistently affect musculoskeletal discomfort when used by themselves and insufficient evidence of their effect on musculoskeletal discomfort when used in combination with other interventions.
3.5. Effects on Work Absence
Two medium-quality single-tool studies show no change [ 9 , 21 , 24 ] in work absence measures following their respective MSI-risk-factor-screening-tool-guided interventions. Three medium-quality multiple-tool studies show no change in work absence measures [ 35 , 36 , 37 ] and one medium-quality study shows a decrease in work absence measures [ 26 , 27 , 28 ] following use of an MSI risk factor screening tool as an intervention component. Therefore, there is limited evidence that MSI risk factor screening tools either do not affect or inconsistently affect work absence, both when used by themselves or in combination with other interventions.
3.6. Effects on Health Resource Utilization
One medium-quality study shows no change [ 9 , 24 ] in measures of health resource utilization following an MSI-risk-factor-screening-tool-guided intervention. No included multiple-tool studies assessed health resource utilization outcomes following use of an MSI risk factor screening tool as an intervention component. Therefore, there is insufficient evidence regarding the effect of MSI risk factor screening tools on health resource utilization, both when used by themselves or in combination with other interventions.
3.7. Effects on Workplace Behaviour
One medium-quality study shows conflicting evidence [ 22 ] in measures of workplace behavior modification following an MSI-risk-factor-screening-tool-guided intervention. No included multiple-tool studies assessed workplace behavior modification outcomes following use of an MSI risk factor screening tool as an intervention component. There is insufficient evidence regarding the effect of MSI risk factor screening tools on work behavior modification, both when used by themselves or in combination with other interventions.
3.8. Effects on Workplace-Associated MSI
No included single-tool studies assessed workplace-associated MSI outcomes following an MSI-risk-factor-screening-tool-guided intervention. Two medium-quality multiple-tool studies show decreases in workplace-associated MSI [ 26 , 27 , 28 , 36 ], another shows an increase in workplace-associated MSI [ 30 ] and another shows no change [ 37 ] following use of an MSI risk factor screening tool as an intervention component. Therefore, there is insufficient evidence regarding the effect of MSI risk factor screening tools on workplace-associated MSI when used by themselves and mixed evidence when used in combination with other interventions.
3.9. Effects on Claims Costs
No included single-tool studies assessed measures of claims cost following an MSI-risk-factor-screening-tool-guided intervention. One medium-quality multiple-tool study shows decreases in claims cost [ 26 , 27 , 28 ] and another medium-quality multiple-tool study shows no change in claims cost [ 37 ] following use of an MSI risk factor screening tool as an intervention component. Therefore, there is insufficient evidence regarding the effect of MSI risk factor screening tools on claims costs when used by themselves and mixed evidence when used in combination with other interventions.
3.10. Effects on Self-Rated Health Status
No included single-tool studies assessed measures of self-rated health status following an MSI-risk-factor-screening-tool-guided intervention. One medium-quality multiple-tool study shows no change [ 35 ] in measures of self-rated health status following use of an MSI risk factor screening tool as an intervention component. Therefore, there is insufficient evidence for use of MSI risk factor screening tools on self-rated health status both when used by themselves or in combination with other interventions.
4. Discussion
The current evidence is insufficient to characterize the effect of MSI risk factor screening tool use on relevant MSI outcomes when used by themselves. The available evidence demonstrates an inconsistent effect of screening tool use on musculoskeletal discomfort and work absence. When used in combination with other tools and interventions in the context of a broader injury prevention program, there is mixed evidence for the effect of MSI risk factor screening tools on workplace-associated MSI and claims costs. For more certain conclusions on the utility and real-world effectiveness of MSI risk factor screening tools, high-quality randomized controlled trials should be conducted examining the impact of the currently available tools on MSI injury and related outcomes. If used in workplace settings, MSI risk factor screening tools should be one component of a broader MSI risk mitigation strategy.
This study utilized rigorous epidemiological data synthesis methods to assess the current state of the scientific literature regarding the effect of using MSI risk factor screening tools to inform injury prevention interventions on important outcomes, such as MSI and related claims and resource utilization. In total, 18 articles representing 14 studies met the article inclusion criteria dictated in the final protocol. Of these 18 articles, only 12 met the minimum quality criteria for retention in the literature synthesis. Of these twelve articles, only five—representing four studies and containing no overlap in screening tools used—used an MSI risk factor screening tool to guide an intervention in a manner that enabled meaningful isolation of the effect of the tool as compared to the effect of other distinctly separate but concurrent tools and interventions. Despite plausible isolation of the effects of these remaining screening tools, outcome measures were too heterogeneous to allow effect size data pooling; rather, the highest level of evidence that could be gleaned from the current literature is, overall, whether screening tools were or were not associated with a positive health effect for specified outcome measure categories.
There are numerous supplementary findings from this systematic review. First, none of the named tools from the preliminary database search that were identified as commonly used (e.g., NIOSH lifting equation, RULA, REBA) were found to have been evaluated rigorously beyond their own validation studies. This literature shows that, instead, MSI risk factor screening tools are, in practice, typically purpose-built or adopted from local occupational health centres. Occupational health and safety professionals designing these novel tools would see minimal examples supporting use of specific screening tools in the literature and instead may base their designs on international standards for biomechanical risk factors, which themselves are not definitively robust [ 11 ]. Any documentation of a high-quality, targeted and real-world application using a previously validated tool would significantly strengthen the state of the current MSI risk factor screening tool literature, especially if such studies also employ clearly defined, replicable outcome measures. In time, tool use resulting in more consistent positive health effects could be identified, adopted and refined.
Second, there is a distinct difference between the characteristics of single-tool and multiple-tool studies, the former group requiring that the effect of a single tool be identifiable. Notably, the selected study sample in multiple-tool studies was more consistently representative of its source population. These studies used a more pragmatic approach to screening and intervention and may better reflect actual practice, where, often, numerous assessment tools and potential interventions are simultaneously introduced in an attempt to improve some aspect of MSI. One conclusion from this finding is that MSI risk factor screening tool use is commonly only one component of a broader MSI risk mitigation strategy. It remains unclear how the effect of MSI screening tools changes with different types of concurrent interventions. This is an additional research avenue made clear from the results of the current systematic review.
This study provides, to the authors’ knowledge, the first systematic review specifically assessing the effects of MSI risk factor screening tools in actual work environments for informing MSI prevention programs. The strengths of the study included use of a robust database search strategy created through collaboration with an experienced health sciences librarian, use of up-to-date guidelines on systematic review structure and reporting and involvement of multiple stakeholder groups to provide guidance on practical needs of the occupational health and safety industry. Previous research synthesis has focused instead on the variety of available MSI risk factor screening tools [ 5 ], the effect of overall occupational health and safety interventions on preventing similar categories of MSI outcomes [ 20 ] and on use of clinical decision support tools to identify useful interventions for already injured patients with disabling musculoskeletal disorders [ 38 ]. However, the conclusions from this review show similarities to those from the occupational health and safety intervention review: both identify significant areas of evidence limited in certainty by a lack of high-quality literature, albeit the latter involving a substantially larger sample of 36 studies [ 20 ]. Considering the wide array of available MSI risk factor screening tools, this lack of data may point to the possibility of missed MSI screening tool use in the grey literature, which was not searched. This constitutes a limitation to our methods, yet we are confident that we located the highest-quality peer-reviewed articles in this research area. Another limitation of the current study was that we did not evaluate the large body of research examining the measurement properties (i.e., reliability and validity) of the MSI screening tools. However, this has been examined in previous reviews [ 7 , 8 ] and our focus on trials examining the real-world impact of MSI screening tools is novel.
5. Conclusions
Overall, there is a small quantity of insufficient research and limited evidence regarding use of MSI risk factor screening tools for informing injury prevention interventions. For more certain conclusions on the utility and effectiveness of MSI risk factor screening tools, high-quality research on the currently available tools is necessary.
Acknowledgments
A version of this work was presented at the 2022 International Congress on Occupational Health (6–11 February 2022) and an associated abstract was published within the Journal of Safety and Health at Work (SH@W). The authors wish to acknowledge Craig Hrynchuk of the Alberta Municipal Health & Safety Association, Mike Roberts of the British Columbia Municipal Safety Association and Ali Golabchi of EWI Works for their support and guidance as stakeholder partners during the review process.
Supplementary Materials
The following supporting information can be downloaded at: https://www.mdpi.com/article/10.3390/ijerph20032762/s1 , Supplementary Material S1: Search Strategy, Table S2: Downs and Black study quality assessment scoring for eligible articles, prior to removal due to poor quality, Table S3: Characteristics of included studies: Characteristics included design, setting, description of MSI screening tool, study arm description, outcome measurements, and results.
Funding Statement
This research is supported by a grant from WorkSafeBC (grant RS2020-SP10). The authors declare that this study received funding from WorkSafeBC. The funder was not involved in the study design, collection, analysis, interpretation of data, the writing of this article or the decision to submit it for publication.
Author Contributions
D.V., S.S., C.C., C.G., L.M., D.L. and D.P.G. assisted with planning and conceptualizing the study, conducting the review and writing the article. L.D. assisted with planning and undertaking the literature search and writing the article. R.R., T.S. and M.D.L. assisted with undertaking the literature review, article screening and writing the article. All authors have read and agreed to the published version of the manuscript.
Institutional Review Board Statement
Formal ethical approval was not required since this was a systematic review of previously published studies.
Informed Consent Statement
Not applicable.
Data Availability Statement
Conflicts of interest.
This research is supported with a grant from WorkSafeBC (grant RS2020-SP10). Richard Roberts and Teri Slade report financial support provided from this grant. Article publishing charges were provided from this grant. Carol Cancelliere is supported by the Canadian Chiropractic Research Foundation as a Research Chair in Knowledge Translation in the Faculty of Health Sciences at Ontario Tech University. Author L.M. is employed by EWI Works and author D.L. is employed by Suncor Energy. The remaining authors declare that the research was conducted in the absence of any commercial or financial relationships that could be construed as a potential conflict of interest.
Disclaimer/Publisher’s Note: The statements, opinions and data contained in all publications are solely those of the individual author(s) and contributor(s) and not of MDPI and/or the editor(s). MDPI and/or the editor(s) disclaim responsibility for any injury to people or property resulting from any ideas, methods, instructions or products referred to in the content.
Thank you for visiting nature.com. You are using a browser version with limited support for CSS. To obtain the best experience, we recommend you use a more up to date browser (or turn off compatibility mode in Internet Explorer). In the meantime, to ensure continued support, we are displaying the site without styles and JavaScript.
- View all journals
- My Account Login
- Explore content
- About the journal
- Publish with us
- Sign up for alerts
- Open access
- Published: 01 April 2024
Spatial-temporal graph neural ODE networks for skeleton-based action recognition
- Longji Pan 1 ,
- Jianguang Lu 1 &
- Xianghong Tang 1
Scientific Reports volume 14 , Article number: 7629 ( 2024 ) Cite this article
Metrics details
- Computational science
- Computer science
- Information technology
In the field of skeleton-based action recognition, accurately recognizing human actions is crucial for applications such as virtual reality and motion analysis. However, this task faces challenges such intraindividual action differences and long-term temporal dependencies. To address these challenges, we propose an innovative model called spatial-temporal graph neural ordinary differential equations (STG-NODE). First, in the data preprocessing stage, the dynamic time warping (DTW) algorithm is used to normalize and calculate 3D skeleton data to facilitate the derivation of customized adjacency matrices for improving the influence of intraindividual action differences. Secondly, a custom ordinary differential equation (ODE) integrator is applied based on the initial conditions of the temporal features, producing a solution function that simulates the dynamic evolution trend of the events of interest. Finally, the outstanding ODE solver is used to numerically solve the time features based on the solution function to increase the influence of long-term dependencies on the recognition accuracy of the model and provide it with a more powerful temporal modeling ability. Through extensive experiments conducted on the NTU RGB+D 60 and Kinetics Skeleton 400 benchmark datasets, we demonstrate the superior performance of STG-NODE in the action recognition domain. The success of the STG-NODE model also provides new ideas and methods for the future development of the action recognition field.
Introduction
Rapid advancements within the field of computer vision have had profound and far-reaching impacts across various domains 1 , 2 , 3 . Within this realm, action recognition stands as a pivotal branch, that is dedicated to the comprehension and analysis of human actions in images and videos. However, to further improve the robustness and practicality of recognition, the field of skeleton-based action recognition is emerging. Within this subfield, traditional methods rely on red-green-blue (RGB) data 4 , 5 ; in contrast, skeletal data encompass time series that encapsulate the 2D or 3D positional coordinates of multiple human joints. These data can be directly captured by sensor devices or extracted from images using pose estimation techniques. Compared with conventional RGB video recognition approaches, action recognition based on skeleton data demonstrates reduced sensitivity to disruptive factors such as changes in lighting, environmental backgrounds, and occlusions that occur during the recognition process. This resilience to environmental variations enhances the robustness and practicality of action recognition systems, making them more reliable across a range of real-world scenarios. Notably, skeleton-based action recognition technology offers potent solutions in various applications, encompassing video surveillance, human-computer interaction, and video comprehension, among others 6 , 7 . It is an efficient, noninvasive 8 and robust recognition method and is indispensable in the field of computer vision.
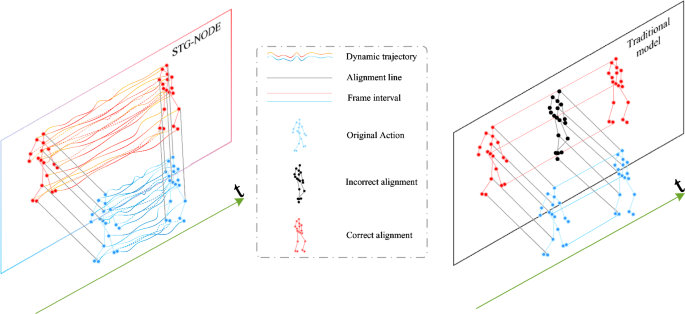
The main idea of this work. Most traditional methods address static images based on frame intervals and suffer from reduced accuracy because they ignore the diversity of different individual motions. In contrast, STG-NODE produces ODE-based dynamic graphs and properly aligns the key points of actions.
The development of skeleton-based action recognition methods has gone through three different stages.
1) Traditional feature engineering stage: In this early stage, researchers mainly relied on hand-designed feature extraction methods for processing skeleton data and performing action recognition. These methods often require expertise and experience to select and design features. The focus of this research concerns how to extract meaningful information from skeleton data. With the emergence of the machine learning era, researchers have begun to manually design skeleton data and shape them into pseudoimages 9 , 10 , 11 or coordinate vector sequences 12 , 13 . The methods developed in this stage cannot fully express the complex information contained in skeleton data, and they have difficulty coping with the diversity and complexity of different actions. Therefore, the attained recognition accuracy is greatly limited, making it difficult for skeleton-based action recognition algorithms to be promoted or employed in wider application fields.
2) RNN and CNN stage: With the rise of deep learning technology, skeleton-based action recognition has undergone a revolutionary change. Deep learning models such as recurrent neural networks (RNNs) and convolutional neural networks (CNNs) are beginning to be employed for processing skeleton data. The focus of an RNN is to model the time series information contained in skeleton data to capture the time series characteristics of actions 14 , 15 , 16 . RNN models, such as long short-term memory (LSTM), can handle long-term dependencies, automatically extract key features, and map them to action categories. This capability enables them to achieve action recognition 17 , 18 , 19 . In contrast, the main goal of CNNs is to extract local features from skeleton data through convolution operations to identify key skeleton patterns. A CNN exhibits spatial invariance and can ignore the specific positions of joints. It gradually extracts hierarchical features through multilayer convolution and is finally combined with a classifier to achieve action recognition 20 , 21 , 22 . Nonetheless, the effectiveness of the abovementioned RNN and CNN methods in terms of recognizing actions from skeletal data is still limited. This limitation stems from the inability of these methods to openly represent the spatial relationships between joints, preventing neural networks from directly and proficiently capturing the collaborative spatial interactions between joints.
3) GCN stage: In recent years, the concept of graph convolutional networks (GCNs), as an extension of the convolution paradigm from images to graphs, has notably penetrated various fields 23 , 24 , 25 , 26 . The intrinsic graph structure of a non-Euclidean space is seamlessly coordinated with the intrinsic configuration of the human skeleton and is essentially a graph-like structure. Joints act as vertices and are connected to each other through edges that reflect the connections between bones in the human body. This complex architecture enables the description of dependencies in interconnected joints. The pioneering work 27 concerning spatial-temporal graph convolutional networks (ST-GCNs), which encapsulate human skeleton data within graph frameworks, is particularly important. In this approach, a GCN is used for skeleton-based action recognition. This impetus has pushed GCN-based methods to the forefront of recognition tasks, cleverly capturing the subtleties of space and time by constructing spatiotemporal graphs. It is worth noting that this type of method demonstrates not only significant robustness but also commendable computational efficiency 14 , 28 .
Specifically, the AS-GCN model proposed by Li et al. 29 successfully captures richer dependencies and more detailed patterns in actions. The model proposed by Peng et al. 30 uses a neural architecture search (NAS) to explore the spatiotemporal correlations between nodes and employs multiple dynamic graph modules to construct a search space. However, most GCN variants, including the abovementioned models, ignore the issue of “intraindividual action differences”. For example, the same action performed by the same person at different times or locations will produce different action attributes and differences, and these attributes and differences can seriously affect the recognition accuracy of the utilized model. The AGC-LSTM model proposed by Si et al. 31 successfully captures discriminative spatial configuration features and temporal dynamics and successfully explores the co-occurrence relationship between the spatial and temporal domains. Subsequently, Shi et al. 32 proposed the MS-AAGCN model that uses a data-driven approach to increase its flexibility and generalization capabilities; the authors confirmed that the adaptive learning graph topology is more suitable for action recognition tasks than human-based graphs. The above approaches are all valid spatiotemporal network models, but they mainly consider short-range connections. However, the MST-GCN model proposed by Chen et al. 33 proved that long-range dependencies are also important for action recognition. Compared with traditional deep neural networks, an ordinary GCN or a spatiotemporal GCN model significantly improves the resulting recognition accuracy. However, according to our analysis, the current mainstream models face at least the following two challenges. 1) The intraindividual differences among actions are neglected. The current GCN-based models often ignore the impact of intraindividual action differences on the accuracy achieved in skeleton-based action recognition tasks. 2) The susceptibility of the existing graph neural networks (GNNs) to oversmoothing reflects an inherent limitation of these networks. As the network layers deepen, all node representations tend to converge to a uniform value, which greatly affects the ability of the employed model to capture long-term dependencies, especially long-term temporal dependencies.
To this end, the spatial-temporal graph neural ODE network (STG-NODE) proposed herein integrates well-designed components to overcome these challenges. As shown in Figure 1 , compared with the traditional methods, STG-NODE has excellent advantages in terms of accurately identifying key actions. First, its discretization layer with residual connections, inspired by 34 , can be viewed as a discretization of ODEs. Subsequently, a continuous graph neural network (CGNN) 35 is derived to alleviate the oversmoothing problem. Taking advantage of this strategy, an ODE-temporal convolutional network (TCN) module is developed to enhance the temporal modeling ability of the model so that it can simulate long-term temporal dependencies. It is worth mentioning that the dynamics introduced by the ODE-TCN module improve the interpretability of the model in this task domain. Second, STG-NODE designs a semantic-based adjacency matrix for skeleton-based action recognition. This innovation is based on an elaborate data preprocessing pipeline, which includes skeleton alignment, semantic feature extraction, category labeling, and dynamic time warping (DTW)-based similarity computation steps, resulting in a semantic adjacency matrix. This unique approach significantly improves the flexibility of the STG-NODE model by effectively mitigating the impact of intraindividual action differences on the accuracy of the skeleton-based action recognition process, ultimately improving its performance in terms of recognizing complex human motions from skeletal data. To verify the superiority of our proposed model (i.e., STG-NODE), we conduct extensive experiments on two large datasets: the NTU RGB+D 60 dataset 13 and the Kinetics Skeleton 400 dataset 36 . Our model achieves superior performance to that of the competing methods on both datasets.
The main contributions of our work are as follows.
The main contribution of STG-NODE is the introduction of tensor-based ordinary differential equations for conducting skeleton-based action recognition. Specifically, this study designs an ODE-TCN module to enhance the temporal modeling capabilities of the model. This enhancement enables the model to effectively model long-term temporal dependencies, thereby improving its suitability for tasks involving complex temporal patterns. Notably, the dynamics introduced by the ODE-TCN module enhance the interpretability of the resulting model in the context of skeleton-based action recognition.
A semantic adjacency matrix customized for skeleton-based action recognition is proposed. This innovation is based on a customized data preprocessing pipeline and a similarity calculation with DTW, ultimately creating a semantic-based semantic adjacency matrix. This unique approach enhances the flexibility of the model and, ultimately, its performance with respect to recognizing complex human motions from skeletal data.
To validate the effectiveness of our proposed STG-NODE model, we conduct extensive experiments on two large datasets: NTU RGB+D 60 and Kinetics Skeleton 400. Our model consistently achieves superior performance on both datasets, demonstrating its ability to address the challenges encountered in skeleton-based action recognition tasks.
The remainder of this paper is organized as follows. Section “ The proposed approach ” first briefly describes the motivation of this paper, followed by detailed descriptions of the key components in the proposed STG-NODE model. Section “ Experiments ” verifies the effectiveness of our method through comparative and ablation experiments and analyses. Finally, we conclude the paper in Section “ Conclusion ”.
The proposed approach
Repeatedly performing the same action introduces diversity in skeletal data due to variations in physiological characteristics and action execution conditions. This phenomenon, which is known as intraindividual action differences, affects recognition accuracy. Furthermore, the importance of long-term temporal dependencies is evident in action recognition tasks since actions are coherent and sequential in nature. Neglecting temporal dependencies may lead to information losses and limited recognition accuracy.
These challenges drive our innovative approach. First, the motivation behind the introduction of tensor-based ODEs stems from the need to capture and model the long-term temporal dependencies inherent in skeleton data. Theoretically, the dynamics resulting from ODEs fit the inherent coherence properties of skeleton-based action recognition tasks. To this end, the ODE-TCN module is introduced to enhance the temporal modeling capabilities of our model and simultaneously inject dynamics to better simulate the temporal attributes of real actions, allowing the model to more accurately capture the dynamic changes exhibited by action sequences. Second, the motivation behind introducing semantic-based category similarity matrices is to address the intraindividual action differences encountered in skeleton-based action recognition scenarios. Theoretically, we plan to obtain semantic action features through specific operations and then calculate the target matrix. To this end, by carefully designing a data preprocessing strategy, including alignment, semantic feature extraction, category labeling, and DTW-based similarity calculations, this study creates a novel semantic adjacency matrix. This matrix is expected to enhance the adaptability of the developed model by effectively mitigating the effect of intraindividual action differences, thereby improving the accuracy of skeleton-based action recognition. These efforts ultimately lead to the development of the STG-NODE model.
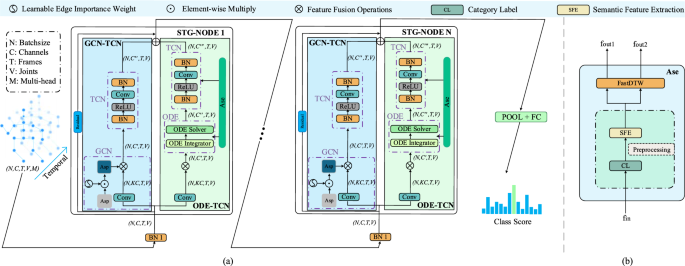
( a ) Basic network framework. The POOL-FC layer and the final class score component form the output module. Asp represents the spatial adjacency matrix; Ase represents the semantic adjacency matrix. ( b ) The details of Ase. fin denotes the original data; fout1 and fout2 are the category similarity matrices.
Model framework
Figure 2 (a) shows the basic framework of our proposed STG-NODE model. It mainly consists of three parts: an ordinary differential equation-temporal convolutional network (ODE-TCN) module, a graph convolutional network-temporal convolutional network (GCN-TCN) module and an output module. The ODE-TCN module is composed of an integrator, a solver and a temporal convolutional network connected in series. The integrator is implemented with an integral function to generate a solution function, which is obtained by integrating the input data in the temporal dimension. The solver is implemented with an ordinary differential equation solver based on the numerical solution with respect to the time characteristics of the solution function so that the model can effectively model long-term time dependencies. The GCN-TCN module is composed of a graph convolutional network and a temporal convolutional network in series; this module empowers the model by ensuring that it comprehensively considers and leverages the joint relationships and spatial structures contained within skeleton data. This leads to an improved understanding and analysis of the spatial features and relationships in human body movements, ultimately resulting in enhanced model performance. Functionality of the Output Module: This module meticulously consolidates and summarizes the features acquired from skeleton data. This process enables the amalgamation of skeleton features into higher-level representations, effectively capturing the abstract characteristics of various actions. Subsequently, these refined features are mapped to various potential action categories, yielding probability distributions for each category. Furthermore, both the ODE-TCN and GCN-TCN modules conduct feature extraction in parallel across different layers. Following feature fusion, these modules seamlessly feed the features into the subsequent parallel structure. Ultimately, the amalgamated features are fed into the output module, enabling the model to perform action classification and accurately determine the action category to which the input data belong. To better present the details of the ODE-TCN module, we show the Ase submodule separately in Figure 2 (b).
Adjacency matrix construction
In our model, we use two types of adjacency matrices. Drawing inspiration from the ST-GCN 27 , the spatial adjacency matrix is formulated as follows:
A is an adjacency matrix, D is a diagonal matrix whose diagonal elements are node degrees, \(A^{hop}\) is the shortest number of hops between nodes, and inf means that the corresponding nodes are not reachable.
In addition, it is crucial to consider the impact of intraindividual action differences on the accuracy of skeleton-based action recognition tasks. For example, the same person performing the same action at different times or locations produces different action properties and differences that cannot be revealed in a spatial graph. To capture the above semantic variability, we use the FastDTW 37 algorithm to calculate the joint nodes of a human body action based on the node characteristics (all node coordinates within a period of time), thereby constructing a semantic adjacency matrix. This semantic adjacency matrix quantifies the degrees and strengths of the correlations between joint points in human movements through an algorithm, thereby extracting semantic information. By providing semantic information concerning action execution, the semantic adjacency matrix enables the model to better understand the intrinsic correlations and meanings of actions. As shown in Figure 3 , with the FastDTW algorithm, point a of a series M is correlated with point z of another series N but not with point y of series N. Specifically, given two time series \(M = (m_{1},\ m_{2},\cdots ,\ m_{k})\) and \(N = (n_{1}, n_{2},\cdots , n_{l})\) , FastDTW is a dynamic programming algorithm that is defined as follows:
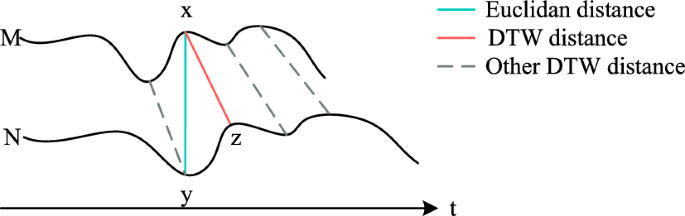
An example of the difference between the Euclidean distance and the FastDTW distance.
Where \(D(i,\ j)\) denotes the shortest distance between subseries \(M=(m_{1},\ m_{2},\cdots ,\ m_{i})\) and \(N=(n_{1},\ n_{2},\cdots ,\ n_{j})\) , with \(dist(m_{i},\ n_{j})\) representing a distance metric such as the absolute distance measure. Consequently, \(FastDTW(M,\ N)=D(k,\ l)\) signifies the ultimate distance between M and N , providing a more accurate assessment of the similarity between two time series than that provided by the Euclidean distance. The value of the multiplier \(\epsilon\) ranges from 0 \(< \epsilon \leqslant\) 1. By adjusting the value of \(\epsilon\) , the approximation degree of the FastDTW algorithm can be controlled, which reduces its computational complexity to a certain extent and improves the computational speed of the model.
Accordingly, we define the semantic adjacency matrix through the FastDTW distance as follows:
where \(X^{i}\) represents the time series of node i , and \(\epsilon\) controls the sparsity level of the adjacency matrix.
Customized ODE integrator and solver
GCNs update node embeddings by aggregating features derived from both the nodes themselves and their neighbors using a graph convolution operation. The conventional form of this convolution operation can be expressed as follows:
where \(f_{in}\in {\mathbb {R}}^{N\times C}\) denotes the input of the previous graph convolution layer, \({\hat{A}}\in {\mathbb {R}}^{N\times N}\) is the normalized adjacency matrix, and \(W\in {\mathbb {R}}^{C\times {C}'}\) is a learnable parameter matrix that models the interactions among different features. However, such GCNs have been shown to suffer from the issue of oversmoothing as the networks become deeper 38 , 39 , which significantly restricts their ability to model long-range dependencies. In response to this limitation, we introduce our novel STG-NODE block.
To allow interactions between the adjacency matrices and modules, we are inspired by the success of the CGNN 35 and consider a more powerful discrete dynamic function:
where \(f_{in}\in {\mathbb {R}}^{N\times T\times F}\) is a space-time tensor that represents the hidden embedding of the examined node in the previous layer, \(\times _{i}\) denotes the tensor matrix multiplication operation executed on mode i , \({\hat{A}}\) is the regularized adjacency matrix, Z is the temporal transformation matrix, W is the feature transformation matrix, and \(h_{0}\) denotes the initial input of the GCN, which can be acquired through another neural network. Motivated by the CGNN, a restart distribution \(H_{0}\) is used to alleviate the oversmoothing problem.
Although the residual structure shown in Eq. 5 is powerful, training it can be challenging due to the large number of parameters involved. Therefore, our goal is to extend the discrete formulation to a continuous expression in the skeleton-based action recognition domain. To effectively convert the residual structure into an ODE structure, we follow the successful practices of previous researchers, such as the series of practices adopted in 40 .
Specifically, the continuous expression of Eq. 5 is shown as follows:
Finally, we draw inspiration from neural ODEs 34 and introduce our STG-NODE framework. The continuous form of the hidden representation is as follows:
Runge-Kutta solvers are generally more stable than Euler solvers, which is critical for accurately capturing the subtle changes and characteristics of action sequences. In addition, a Runge-Kutta solver has higher accuracy when processing action sequences with nonlinear characteristics and rapid changes and can more accurately capture details and important features from actions. Based on these considerations, we choose the Runge-Kutta method as the ODE solver in our model.
STG-NODE module and performance analysis
The preceding sections have provided a detailed exposition of the key components contained within STG-NODE. This section operates from a macro perspective, delineating the holistic STG-NODE module. As illustrated in Figure 1, the model adopts a serial-parallel structure comprising an ODE-TCN block and a GCN-TCN block. This inventive architecture not only facilitates the seamless amalgamation of spatiotemporal information but also harnesses the inherent strengths of ODEs, thereby enhancing the precision achieved in skeleton-based action recognition tasks.
On the one hand, STG-NODE presents a plethora of advantages over traditional GCNs and TCNs, significantly bolstering the foundational aspects of skeletal action recognition models. ODEs fundamentally capture dynamic behaviors by modeling state evolution trends over consecutive time intervals. This property impeccably aligns with the nuanced nature of human motion, enabling the model to discern the subtle temporal intricacies contained within skeletal data. Furthermore, the ODE-based framework exhibits superior generalization capabilities to those of the traditional methods and adeptly handles irregularly sampled or missing data points within the input skeleton sequence. Its rich feature reservoir and adept module combinations substantially augment the ability of the model to unravel intricate the correlations inherent in skeletal actions. However, compared to models that lack DTW integration (e.g., 27 , 41 ), the ODE-TCN module utilizes FastDTW to compute the semantic adjacency matrix. This matrix enables the ODE-TCN module to focus more on nodes with greater relevance when propagating information in the temporal dimension during the training process, thereby capturing key frames in actions with varying lengths. This allows the model to better adapt to situations where the execution speed is faster or slower than the action features are learned. Ultimately, this mechanism allows STG-NODE to mitigate the impact of intraindividual action differences.
In summary, the STG-NODE model has the advantages of ODEs and DTW enhancement. In theory, this comprehensive advantage makes the STG-NODE model significantly better than the traditional models. This enhancement is reflected in its ability to effectively capture the spatiotemporal complexity of skeletal actions, resulting in significant performance advantages in action recognition tasks.
Training loss expression
The cross-entropy loss is chosen as the loss function, as it is well suited for addressing multiclassification tasks and exhibits strong sensitivity to variations in predicted probability distributions. This property encourages the model to prioritize the correct category. The formulation of the cross-entropy loss is outlined below:
where C is the number of categories, \(t_{c}\) is the value of the c -th category in the real labels, \(y_{c}\) is the predicted probability output by the model for the c -th category, y is the output of the model, indicating the probability predicted by the model for each category, t is the real label, only one element is 1, and the others are 0.
The goal of the loss function is to guide the optimization process of the model parameters by minimizing the difference between the predicted probability of each category and the one-hot encoding of the actual corresponding label, thereby enabling the model to more accurately predict action categories.
Experiments
In this section, an extensive performance evaluation of the devised STG-NODE model is implemented across two expansive datasets: NTU RGB+D 60 13 and Kinetics Skeleton 400 36 . Given the relatively modest size of the NTU RGB+D 60 dataset, a meticulous ablation study is conducted to ascertain the efficacy of the enhancements incorporated into the model. Subsequently, a comprehensive comparative analysis is performed, benchmarking our STG-NODE model against other approaches. This multifaceted evaluation, spanning two datasets (two completely different benchmarks plus two different indicator scales), serves to corroborate both the broad applicability and the precision of our proposed framework in terms of achieving definitive recognition outcomes.
The NTU RGB+D 60 dataset, which contains an extensive collection of 56,000 action clips classified into 60 different action categories, is of crucial importance in the field of 3D human action recognition. This comprehensive range covers a variety of action types, from the nuances of everyday behaviors to health-related routines and complex two-person interactions. These captured segments were recorded from the perspective of three synchronized camera angles within the controlled confines of a laboratory environment. These visual narratives reveal the complex spatial coordinates of joints (X, Y, Z) in 3D with the help of the discriminative capabilities provided by the Kinect depth sensor.
The evaluation paradigm for this dataset is carefully constructed around two strong paradigms: cross-subject (X-Sub) and cross-view (X-View) protocols. Under the X-Sub benchmark, the partitioning strategy is based on individual IDs and ultimately allocates 40,320 samples for fine-grained training and an additional 16,560 samples for rigorous testing. At the same time, the X-View framework utilizes perspectives derived from different camera angles to form similar partitioning patterns. In this configuration, a selective subset of 18,960 samples from camera 1 is reserved for exhaustive testing purposes, while a large repository of 37,920 samples acquired from cameras 2 and 3 strongly supports the comprehensive training scheme.
The Kinetics Skeleton 400 dataset is a comprehensive compilation of approximately 300,000 video clips that were carefully curated from various sources on YouTube. This massive dataset contains 400 different human action categories, covering a wide range of scenarios from everyday activities to dynamic motion scenarios and complex interactions with complex actions. Notably, each video clip in the Kinetics Skeleton 400 dataset maintains a consistent temporal structure with an average duration of approximately 10 seconds. The clips were captured at a standard frame rate of 30 frames/second, yielding a total of 300 frames.
Each frame in these clips is meticulously analyzed, during which up to two joints with the highest average confidence levels are selected. This rigorous process culminates in the precise definition of 18 joints for each bone structure, each of which characterized by its 2D coordinates and corresponding confidence. The resulting skeletal representation provides the basis for a comprehensive action analysis.
Furthermore, in the context of the Kinetics Skeleton 400 experiment, we evaluate the achieved recognition performance according to the top-1 and top-5 classification accuracy metrics, which evaluate the determinism and robustness of the tested model. The estimated 2D joint positions generated by the OpenPose pose estimation framework 42 provided by the ST-GCN are used as inputs. This choice ensures that the experiments are performed on a consistent and reputable basis, allowing for a robust and accurate analysis of dataset-rich human behavioral dynamics.
Experimental settings
The experiments are conducted on a Linux server equipped with an Intel(R) Xeon(R) Silver 4316 CPU running at 2.3 GHz and four NVIDIA TESLA A100 GPUs. Adhering to the foundational framework of the ST-GCN, STG-NODE follows a similar structural setup. The architecture encompasses 10 STG-NODE blocks within the STG-NODE model, with each block consisting of one ODE-TCN and one GCN-TCN. For the semantic adjacency matrix, precise calibration is achieved by setting the thresholds \(\sigma\) and \(\epsilon\) to 0.1 and 0.6, respectively.
Stochastic gradient descent (SGD) with Nesterov momentum (0.9) is applied as the optimization strategy with a learning rate of 0.15, and it is accompanied by a decay ratio of 0.0001. The cross-entropy loss function is chosen for gradient backpropagation. This process is executed using a batch size of 64, spanning a training period that extends to 80 iterations. Notably, the experimental setup applies several supplementary preprocessing strategies to each dataset. Initially, the coordinate information of the samples is extracted through the utilization of a dedicated skeleton sequence visualization tool, as provided in 27 . Subsequently, the extracted data are carefully processed using the DTW algorithm, and finally, a customized semantic adjacency matrix is derived. This strategic preprocessing procedure augments the discernment capabilities of the model and contributes to the overall efficacy of the experimental analysis.
To evaluate the performance of STG-NODE, we compare our proposed STG-NODE model with other skeleton-based action recognition methods on the Kinetics Skeleton 400 dataset and the NTU RGB+D 60 dataset. The comparison results are shown in Table 1 , which shows that our model has strong performance advantages on both datasets.
Specifically, our model produces remarkable recognition results on the Kinetics Skeleton 400 and NTU RGB+D 60 datasets, achieving the best performance. However, when delving into the nuances of the Kinetics dataset, the recognition accuracy improvement achieved over the method of 41 is not sufficiently large. A plausible explanation for this phenomenon can be attributed to the composition of the Kinetics Skeleton 400 test set. This dataset contains individuals whose behavioral styles or unique characteristics are not fully represented within the scope of the training set. The emergence of this new individual variability poses urgent challenges and may require the inclusion of additional data to enhance the generalizability of the model. Thus, it becomes evident that our model yearns for a more substantial sample size to adeptly assimilate and adapt to the diverse intricacies of different individuals, thereby elevating its performance on the Kinetics dataset.
Furthermore, notably, it performs well on the NTU RGB+D dataset, surpassing the benchmarks set by models such as that of 41 . This serves as a compelling testament to the exceptional prowess of our model with respect to addressing action recognition tasks from varying viewpoints. The undeniable significance of this capacity becomes even more pronounced in real-world applications.
To more clearly highlight the advantages of STG-NODE over the existing models, we select some action categories with obvious coherence differences for comparison. Generally, people have a better memory and understanding of common action sequences and therefore perform more naturally and coherently when performing such actions. We call these action categories “strong-coherence action categories”, as shown in the red box in Figure 4 , including drinking water (label: 1), sitting down (label: 8), standing (label: 9), etc. Conversely, some actions may feel confusing or unnatural, with less coherence. We call these action categories “weakly coherent action categories”, as shown in the green box in Figure 4 , including typing on a keyboard (label: 30), experiencing back pain (label: 46) and vomiting (label: 48), etc. In Figure 4 , we clearly observe that in the weakly coherent action category, STG-NODE has a smaller performance improvement over the ST-GCN (dark areas remain in the green box). However, in the strongly coherent action category, STG-NODE yields significantly improved performance over that of the ST-GCN model (the dark areas in the red box have basically disappeared).
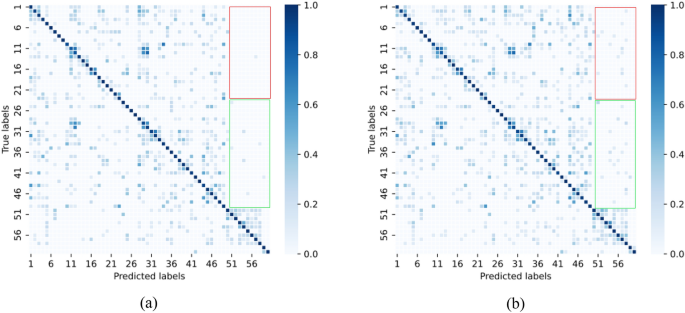
Confusion matrices produced for the NTU RGB+D 60 dataset. ( a ) shows the experimental results of STG-NODE; ( b ) presents the experimental result of the ST-GCN.
The two datasets used in the experiments exhibit distinctly disparate properties. While the Kinetic dataset employs a 2D skeleton detected by a deep neural network as the input, the NTU RGB+D 60 dataset employs data from a Kinect depth sensor. Further differentiating the two datasets, NTU RGB+D 60 employs a stationary camera, whereas the Kinetics dataset often captures videos using handheld devices, thus introducing significant camera motion. The noteworthy efficacy exhibited by the proposed STG-NODE model across both datasets underscores the prowess of our spatiotemporal dependency modeling approach. This accomplishment can be attributed to two key factors.
Leveraging the tensor-based ODE framework significantly augments the temporal modeling ability of the model. Simultaneously, the dynamics introduced by the ODE can be construed as the evolutionary journey of an action sequence, thereby providing insights into the rationale used by the model for action recognition.
Employing a strategic approach, the DTW algorithm serves as a conduit that introduces the semantic adjacency matrix. This augmentation bolsters the semantic acumen of the model and adeptly mitigates the influence of individual intraindividual action discrepancies on the precision achieved in skeleton-based action recognition tasks.
Ablation study
Ablation experiments concerning action recognition are performed on the NTU RGB+D 60 dataset and the Kinetics Skeleton 400 dataset to examine the effectiveness of the proposed components in the above STG-NODE model. Then, different learning rates are set for a verification implemented on the X-Sub benchmark and X-View benchmark to achieve the best recognition accuracy.
Evaluation of the effectiveness of each STG-NODE module: To determine the necessity and effectiveness of the individual modules in the STG-NODE model, a system analysis is performed by iteratively omitting certain modules from the original architecture and subsequently comparing the performances of the ablated versions. Two different variants of the STG-NODE model are designed for this purpose.
1. STG-Semantic: In this model, a semantic adjacency matrix is constructed based on the semantic similarity of the target skeleton. However, the ODE solver is replaced by a regular GCN to verify the effectiveness of the ODE structure in terms of capturing long-range dependencies.
2. STG-ODE*: This model contains ODE modules but does not involve the creation of specialized adjacency matrices. This omission is intended to identify the necessity of introducing a semantic adjacency matrix.
The results are shown in Table 2 , which shows that the accuracy and efficiency of a model adding any module exceed those of the baseline model. It is worth noting that the best performance is achieved when all modules are integrated. This synergy leads to significant improvements in the accuracies achieved on the X-View and X-Sub benchmarks of the NTU RGB+D 60 dataset (2.1% and 3.2%, respectively), while improving the top-1 and top-5 accuracies attained on the Kinetics Skeleton 400 dataset by up to 1.8% and 1.9%, respectively.
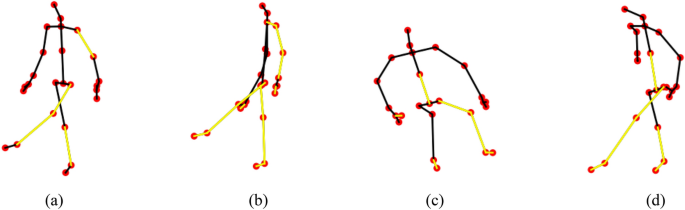
Visualization of kicking actions. ( a ) is the first action of person No. 1, ( b ) is the second action of person No. 1, ( c ) is the first action of person No. 2, and ( d ) is the second action of person No. 2. The two actions occur at different angles, heights and distances.
These results underscore the significant performance improvement provided by our STG-NODE architecture due to its innovative temporal modeling approach and specialized semantic adjacency matrix. In addition, to more clearly demonstrate the effect of integrating the semantic adjacency matrix into the ODE-TCN module, we draw action visualization diagrams of different individuals performing the same action (such as kicking something). As shown in Figure 5 , the yellow highlighted part represents the edge composed of joint points with higher correlations when executing the action. When the model encounters different individuals performing the same action at different speeds, it focuses on these parts to help mitigate the impact of intraindividual action differences on the accuracy of skeleton-based action recognition.
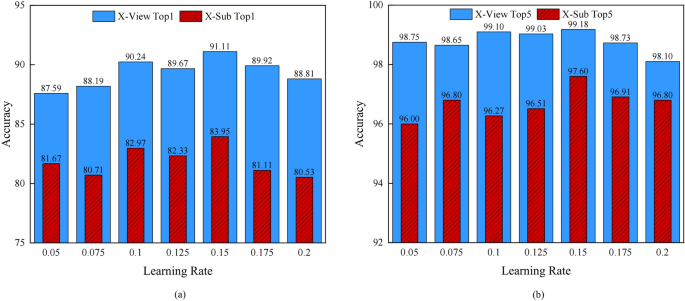
Recognition accuracy fluctuations observed in two accuracy evaluations conducted on the NTU RGB+D dataset with different learning rates.
Selection of the Optimal Learning Rate for STG-NODE: We conduct a comprehensive evaluation of the accuracy achieved by STG-NODE across 7 distinct learning rates. Figure 6 (b) compares the top-5 accuracy results obtained on the two benchmarks, and Figure 6 (a) compares the top-1 accuracy results obtained on the two benchmarks. Notably, as depicted in the figure, the experimental accuracy peaks when the learning rate is set to 0.15.
Many efforts have been made to address the complex challenge of action recognition. However, little attention has been given to solving the difficult problem of extracting long-range dependencies without succumbing to the oversmoothing problem that is inherent in GCN-related architectures. This paper presents a groundbreaking ODE-based spatiotemporal forecasting model called STG-NODE. To the best of our knowledge, this is the first attempt to link continuous differential equations to node representations for developing skeleton-based action recognition networks, and STG-NODE provides the ability to shape deeper architectures and exploit a wider range of dependencies than can other methods. Furthermore, the incorporation of a customized semantic adjacency matrix greatly improves the efficiency of the model. The performance achieved by STG-NODE in four challenging tests (two benchmarks plus two metrics) is better than that of many existing methods. In future research, we will delve into the extraction of complex local features from skeletons and consider further exploiting a graph structure to capture the relationships between different parts of an input sequence, such as the interaction dependencies between different body parts in human activity recognition tasks.
Data availability
All data included in this study are available upon request by contact with the corresponding author.
Xu, C. et al. Uncertainty-aware multiview deep learning for internet of things applications. IEEE Trans. Industr. Inf. 19 , 1456–1466 (2022).
Article Google Scholar
Zhao, W. et al. Telecomnet: Tag-based weakly-supervised modally cooperative hashing network for image retrieval. IEEE Trans. Pattern Anal. Mach. Intell. 44 , 7940–7954 (2021).
Xu, C. et al. Reliable conflictive multi-view learning. arXiv preprint arXiv:2402.16897 (2024).
Carreira, J. & Zisserman, A. Quo vadis, action recognition? a new model and the kinetics dataset. In proceedings of the IEEE Conference on Computer Vision and Pattern Recognition , 6299–6308 (2017).
Duan, H., Zhao, Y., Xiong, Y., Liu, W. & Lin, D. Omni-sourced webly-supervised learning for video recognition. In European Conference on Computer Vision , 670–688 (Springer, 2020).
Aggarwal, J. K. & Ryoo, M. S. Human activity analysis: A review. Acm Comput. Surv. (Csur) 43 , 1–43 (2011).
Hu, W., Tan, T., Wang, L. & Maybank, S. A survey on visual surveillance of object motion and behaviors. IEEE Transactions on Systems, Man, and Cybernetics, Part C (Applications and Reviews) 34 , 334–352 (2004).
Su, Y., Zhu, H., Tan, Y., An, S. & Xing, M. Prime: privacy-preserving video anomaly detection via motion exemplar guidance. Knowl.-Based Syst. 278 , 110872 (2023).
Li, B. et al. Skeleton based action recognition using translation-scale invariant image mapping and multi-scale deep cnn. In 2017 IEEE International Conference on Multimedia & Expo Workshops (ICMEW) , 601–604 (IEEE, 2017).
Liu, M., Liu, H. & Chen, C. Enhanced skeleton visualization for view invariant human action recognition. Pattern Recogn. 68 , 346–362 (2017).
Article ADS Google Scholar
Soo Kim, T. & Reiter, A. Interpretable 3d human action analysis with temporal convolutional networks. In Proceedings of the IEEE conference on computer vision and pattern recognition workshops , 20–28 (2017).
Liu, J., Shahroudy, A., Xu, D. & Wang, G. Spatio-temporal lstm with trust gates for 3d human action recognition. In Computer Vision–ECCV 2016: 14th European Conference, Amsterdam, The Netherlands, October 11-14, 2016, Proceedings, Part III 14 , 816–833 (Springer, 2016).
Shahroudy, A., Liu, J., Ng, T.-T. & Wang, G. Ntu rgb+ d: A large scale dataset for 3d human activity analysis. In Proceedings of the IEEE conference on computer vision and pattern recognition , 1010–1019 (2016).
Du, Y., Wang, W. & Wang, L. Hierarchical recurrent neural network for skeleton based action recognition. In Proceedings of the IEEE conference on computer vision and pattern recognition , 1110–1118 (2015).
Li, S., Li, W., Cook, C., Zhu, C. & Gao, Y. Independently recurrent neural network (indrnn): Building a longer and deeper rnn. In Proceedings of the IEEE conference on computer vision and pattern recognition , 5457–5466 (2018).
Si, C., Jing, Y., Wang, W., Wang, L. & Tan, T. Skeleton-based action recognition with spatial reasoning and temporal stack learning. In Proceedings of the European conference on computer vision (ECCV) , 103–118 (2018).
Song, S., Lan, C., Xing, J., Zeng, W. & Liu, J. An end-to-end spatio-temporal attention model for human action recognition from skeleton data. In Proceedings of the AAAI conference on artificial intelligence , vol. 31 (2017).
Zhang, P. et al. View adaptive recurrent neural networks for high performance human action recognition from skeleton data. In Proceedings of the IEEE international conference on computer vision , 2117–2126 (2017).
Zhu, W. et al. Co-occurrence feature learning for skeleton based action recognition using regularized deep lstm networks. In Proceedings of the AAAI conference on artificial intelligence , vol. 30 (2016).
Li, C., Hou, Y., Wang, P. & Li, W. Multiview-based 3-d action recognition using deep networks. IEEE Trans. Hum.-Mach. Syst. 49 , 95–104 (2018).
Article CAS Google Scholar
Ke, Q., Bennamoun, M., An, S., Sohel, F. & Boussaid, F. A new representation of skeleton sequences for 3d action recognition. In Proceedings of the IEEE conference on computer vision and pattern recognition , 3288–3297 (2017).
Cao, C. et al. Skeleton-based action recognition with gated convolutional neural networks. IEEE Trans. Circuits Syst. Video Technol. 29 , 3247–3257 (2018).
Atwood, J. & Towsley, D. Diffusion-convolutional neural networks. Advances in neural information processing systems 29 (2016).
Duvenaud, D. K. et al. Convolutional networks on graphs for learning molecular fingerprints. Advances in neural information processing systems 28 (2015).
Hamilton, W., Ying, Z. & Leskovec, J. Inductive representation learning on large graphs. Adv. Neural Inf. Proc. syst. 30 (2017).
Su, Y., Xing, M., An, S., Peng, W. & Feng, Z. Vdarn: video disentangling attentive relation network for few-shot and zero-shot action recognition. Ad Hoc Netw. 113 , 102380 (2021).
Yan, S., Xiong, Y. & Lin, D. Spatial temporal graph convolutional networks for skeleton-based action recognition. In Proceedings of the AAAI conference on artificial intelligence , vol. 32 (2018).
Vemulapalli, R., Arrate, F. & Chellappa, R. Human action recognition by representing 3d skeletons as points in a lie group. In Proceedings of the IEEE conference on computer vision and pattern recognition , 588–595 (2014).
Li, M. et al. Actional-structural graph convolutional networks for skeleton-based action recognition. In Proceedings of the IEEE/CVF conference on computer vision and pattern recognition , 3595–3603 (2019).
Peng, W., Hong, X., Chen, H. & Zhao, G. Learning graph convolutional network for skeleton-based human action recognition by neural searching. In Proc. AAAI Conf. Artif. Intell. 34 , 2669–2676 (2020).
Google Scholar
Si, C., Chen, W., Wang, W., Wang, L. & Tan, T. An attention enhanced graph convolutional lstm network for skeleton-based action recognition. In Proceedings of the IEEE/CVF conference on computer vision and pattern recognition , 1227–1236 (2019).
Shi, L., Zhang, Y., Cheng, J. & Lu, H. Skeleton-based action recognition with multi-stream adaptive graph convolutional networks. IEEE Trans. Image Process. 29 , 9532–9545 (2020).
Chen, Z., Li, S., Yang, B., Li, Q. & Liu, H. Multi-scale spatial temporal graph convolutional network for skeleton-based action recognition. In Proc. AAAI Conf. Artif. Intell. 35 , 1113–1122 (2021).
Chen, R. T., Rubanova, Y., Bettencourt, J. & Duvenaud, D. K. Neural ordinary differential equations. Adv. Neural Inf. Process. Syst. 31 (2018).
Xhonneux, L.-P., Qu, M. & Tang, J. Continuous graph neural networks. In International Conference on Machine Learning , 10432–10441 (PMLR, 2020).
Kay, W. et al. The kinetics human action video dataset. arXiv preprint arXiv:1705.06950 (2017).
Salvador, S. & Chan, P. Toward accurate dynamic time warping in linear time and space. Intell. Data Anal. 11 , 561–580 (2007).
Li, Q., Han, Z. & Wu, X.-M. Deeper insights into graph convolutional networks for semi-supervised learning. In Proceedings of the AAAI conference on artificial intelligence , vol. 32 (2018).
Zhou, J. et al. Graph neural networks: A review of methods and applications. AI open 1 , 57–81 (2020).
Fang, Z., Long, Q., Song, G. & Xie, K. Spatial-temporal graph ode networks for traffic flow forecasting. In Proceedings of the 27th ACM SIGKDD conference on knowledge discovery & data mining , 364–373 (2021).
Lovanshi, M. & Tiwari, V. Human skeleton pose and spatio-temporal feature-based activity recognition using st-gcn. Multimedia Tools Appl. 1–26 (2023).
Cao, Z., Simon, T., Wei, S.-E. & Sheikh, Y. Realtime multi-person 2d pose estimation using part affinity fields. In Proceedings of the IEEE conference on computer vision and pattern recognition , 7291–7299 (2017).
Jiang, X., Xu, K. & Sun, T. Action recognition scheme based on skeleton representation with ds-lstm network. IEEE Trans. Circuits Syst. Video Technol. 30 , 2129–2140 (2019).
Dhiman, C. & Vishwakarma, D. K. View-invariant deep architecture for human action recognition using two-stream motion and shape temporal dynamics. IEEE Trans. Image Process. 29 , 3835–3844 (2020).
Liu, H., Zhang, L., Guan, L. & Liu, M. Gfnet: A lightweight group frame network for efficient human action recognition. In ICASSP 2020-2020 IEEE International Conference on Acoustics, Speech and Signal Processing (ICASSP) , 2583–2587 (IEEE, 2020).
Ding, C., Liu, K., Cheng, F. & Belyaev, E. Spatio-temporal attention on manifold space for 3d human action recognition. Appl. Intell. 51 , 560–570 (2021).
Shao, Z., Li, Y. & Zhang, H. Learning representations from skeletal self-similarities for cross-view action recognition. IEEE Trans. Circuits Syst. Video Technol. 31 , 160–174 (2020).
Huynh-The, T., Hua, C.-H., Ngo, T.-T. & Kim, D.-S. Image representation of pose-transition feature for 3d skeleton-based action recognition. Inf. Sci. 513 , 112–126 (2020).
Vishwakarma, D. K. & Jain, K. Three-dimensional human activity recognition by forming a movement polygon using posture skeletal data from depth sensor. ETRI J. 44 , 286–299 (2022).
Download references
Acknowledgements
This work is supported by The Science and Technology Foundation of Guizhou Province (QKHJC-ZK(2021)YB015) and Guizhou Provincial Key Technology R &D Program (QKHZC(2022)YB074).
Author information
Authors and affiliations.
Guizhou University, State Key Laboratory of Public Big Data, Guiyang, 550025, China
Longji Pan, Jianguang Lu & Xianghong Tang
You can also search for this author in PubMed Google Scholar
Contributions
J.L. and X.T. conceived the experiment(s), L.P. conducted the experiment(s), L.P. analysed the results. All authors reviewed the manuscript.
Corresponding author
Correspondence to Jianguang Lu .
Ethics declarations
Competing interests.
The authors declare no competing interests.
Additional information
Publisher's note.
Springer Nature remains neutral with regard to jurisdictional claims in published maps and institutional affiliations.
Rights and permissions
Open Access This article is licensed under a Creative Commons Attribution 4.0 International License, which permits use, sharing, adaptation, distribution and reproduction in any medium or format, as long as you give appropriate credit to the original author(s) and the source, provide a link to the Creative Commons licence, and indicate if changes were made. The images or other third party material in this article are included in the article’s Creative Commons licence, unless indicated otherwise in a credit line to the material. If material is not included in the article’s Creative Commons licence and your intended use is not permitted by statutory regulation or exceeds the permitted use, you will need to obtain permission directly from the copyright holder. To view a copy of this licence, visit http://creativecommons.org/licenses/by/4.0/ .
Reprints and permissions
About this article
Cite this article.
Pan, L., Lu, J. & Tang, X. Spatial-temporal graph neural ODE networks for skeleton-based action recognition. Sci Rep 14 , 7629 (2024). https://doi.org/10.1038/s41598-024-58190-9
Download citation
Received : 12 January 2024
Accepted : 26 March 2024
Published : 01 April 2024
DOI : https://doi.org/10.1038/s41598-024-58190-9
Share this article
Anyone you share the following link with will be able to read this content:
Sorry, a shareable link is not currently available for this article.
Provided by the Springer Nature SharedIt content-sharing initiative
By submitting a comment you agree to abide by our Terms and Community Guidelines . If you find something abusive or that does not comply with our terms or guidelines please flag it as inappropriate.
Quick links
- Explore articles by subject
- Guide to authors
- Editorial policies
Sign up for the Nature Briefing newsletter — what matters in science, free to your inbox daily.

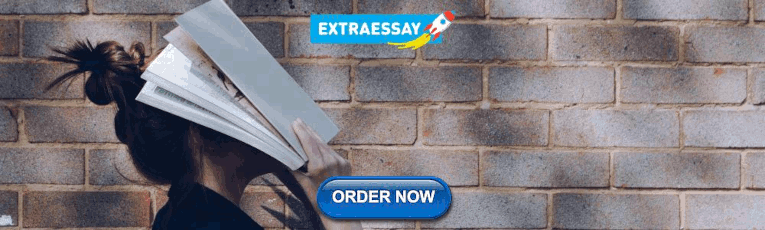
IMAGES
VIDEO
COMMENTS
skeletal framework, which is defined as characteristics identified from previous inquiry that provide an internal structure that ... research method aimed at the discovery of theory from systematically obtained data (Glaser & Strauss, 1967; Strauss, 1987) and "an inductive, theory discovery methodology" (Martin & ...
A theoretical framework guides the research process like a roadmap for the study, so you need to get this right. Theoretical framework 1,2 is the structure that supports and describes a theory. A theory is a set of interrelated concepts and definitions that present a systematic view of phenomena by describing the relationship among the variables for explaining these phenomena.
For instance, a quick search for theoretical or conceptual frameworks in the abstracts of articles in Educational Research Complete (a common database for educational research) in STEM fields demonstrates a dramatic change over the last 20 years: from only 778 articles published between 2000 and 2010 to 5703 articles published between 2010 and ...
A theoretical framework is a foundational review of existing theories that serves as a roadmap for developing the arguments you will use in your own work. Theories are developed by researchers to explain phenomena, draw connections, and make predictions. In a theoretical framework, you explain the existing theories that support your research ...
Download scientific diagram | Skeletal outline of the research framework. from publication: Keeping sites in sight: Conversations with teachers about the design of toolkits peculiar to a ...
In research, the concepts and theories serve as building blocks or skeleton for the foundation or bases of the study.CONCEPTUAL FRAMEWORK covers the explana...
1. Introduction. Human skeletal muscle is the motor of the locomotion function of the human body. This specific living tissue has complex multiscale and hierarchical architecture (i.e., from fibers to myofibrils, sarcomeres, and contractile proteins (actin and myosin)) and function (e.g., voluntary contraction control) [1-4].Hierarchical bundles of assembled fibers and fibrils, which are ...
Bone forms the skeletal framework of all vertebrates. It is a composite tissue consisting of organic matrix, inorganic minerals, cells, and water. ... Control of the growth plate is also an area of intense research. Indian hedgehog (Ihh), a protein of the same family as sonic hedgehog, regulates the rate of hypertrophic chondrocyte ...
International Journal of Oral Science (2023) The tissue-resident skeletal stem cells (SSCs), which are self-renewal and multipotent, continuously provide cells (including chondrocytes, bone cells ...
The human skeletal form underlies bipedalism, but the genetic basis of skeletal proportions (SPs) is not well characterized. ... We used BOLT-LMM to regress variants on each skeletal measure using a linear mixed-model association framework. After generating summary statistics for each ... O.S. and B.I.F. were supported by NSF Graduate Research ...
Description. Skeletal Research, An Experimental Approach discusses experimental studies on bone cell and tissue biochemistry, biomechanics, isolation, and methods of analysis. Divided into four parts encompassing 21 chapters, this book describes the advantages and limitations of approaches and options available for bone laboratories.
1. Introduction. The human skeletal system is a marvel of biological engineering. From the explanation of the main functions of bones and their composition to the precise mechanisms of bone formation and remodeling, we seek to unravel and shed light on the pivotal roles that bones play beyond mere structural support with a focus on research and insights from the fields of physiology, anatomy ...
The resulting skeletal frameworks allow sponges to grow upwards and facilitate water exchange with minimal metabolic cost. Several studies on sponge skeletogenesis have been published. We are beginning to understand the mechanisms of spicule secretion and the role of spicules and skeletal frameworks in the biology, ecology, and evolution of ...
The Steps in Writing a Review of Related Literature RESEARCH DESIGN Design in the field of research serves as a blueprint or a skeletal framework of your research study. It includes many related aspects of your research work. Your research design is realized by any of these types of qualitative research that has its data collection technique. 1.
The skeletal framework presented in Figure 12.2 is based on prior work by Vosniadou regarding conceptual change in physical science (e.g., Vosniadou, 1994Vosniadou, , 2003, and also takes into ...
human skeleton, the internal skeleton that serves as a framework for the body. This framework consists of many individual bones and cartilages.There also are bands of fibrous connective tissue—the ligaments and the tendons—in intimate relationship with the parts of the skeleton. This article is concerned primarily with the gross structure and the function of the skeleton of the normal ...
Skeleton articles from across Nature Portfolio. The skeleton is a rigid framework of bones that forms the supporting structure of the musculoskeletal system, and also functions in movement ...
International Journal Production Research,24(5), 1107-25. ... Marefat, M., Banerjee, P. (1994). A common skeletal framework for knowledge-based solutions to a representative set of manufacturing problems. In: Mital, A., Anand, S. (eds) Handbook of Expert Systems Applications in Manufacturing Structures and rules. Intelligent Manufacturing Series.
Step 1: Structure the main headings of your research paper. In this article I provided you with a typical research paper outline that includes: Title, Abstract, Introduction, Objective, Methods, Results, Discussion, Conclusions, Appendices. Step 2: Write the key sentences. For each paper section, I gave you a key sentence skeleton.
Our skeletal framework research reveals important themes and difficulties experienced by nursing practitioners as it examines developing patterns in nursing during the COVID-19 pandemic. The quick uptake of telemedicine, the increased emphasis on infection control, and the growing recognition of the mental health needs of nurses highlight how dynamic nursing practice is in this crisis.
Abstract and Figures. This paper describes the stapl Skeleton Framework, a high-level skeletal approach for parallel programming. This framework abstracts the underlying details of data ...
Terms in this set (33) Research Design. -A plan or something that is conceptualized by the mind. - a research design serves as the blueprint or skeletal framework of your study. Ethnography. -Involves a study of a certain cultural group or organization in which the researcher immerse in the participants' natural setting.
Research is needed to assess the current scientific literature involving evaluation of the effectiveness of MSI risk factor screening tools for informing injury prevention interventions through a rigorous epidemiological lens. ... Intervention, Comparison, Outcome, Study Design) framework was used for development of article inclusion criteria ...
Compared with RGB data, skeletal data is more robust to complex background information. And it is easier to process and more computationally efficient. This can significantly improve the recognition accuracy of human action classification. Skeleton-based human action recognition has gradually become a popular research area.
The focus of this research concerns how to extract meaningful information from skeleton data. ... (ST-GCNs), which encapsulate human skeleton data within graph frameworks, is particularly ...