- Memberships
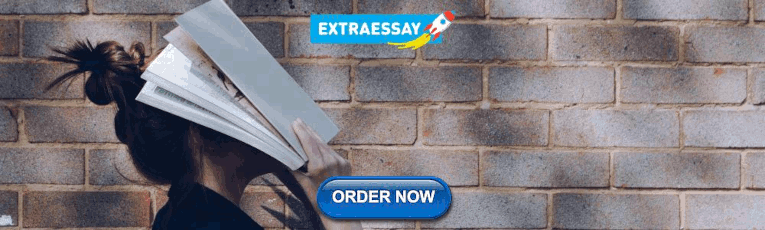
Means End Analysis: the basics and example
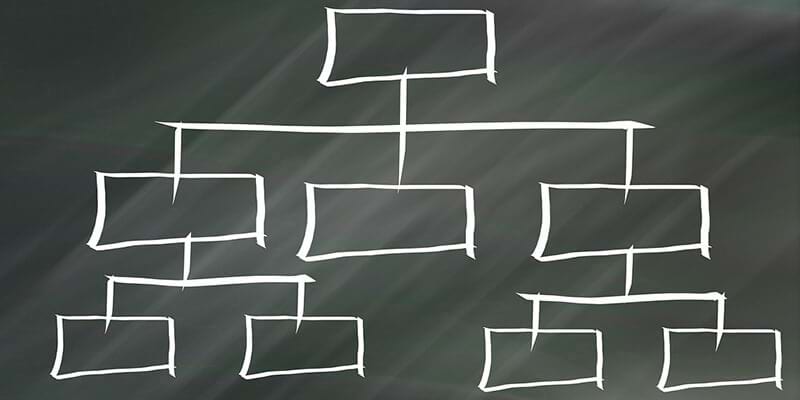
Means End Analysis (MEA): this article explains the concept of Means End Analysis or MEA in a practical way. This article contains the general definition of the technique, and the steps involved in the process, including a means end analysis example. After reading it, you will understand the basics of this Problem Solving tool. Enjoy reading!
What is a Means End Analysis (MEA)?
Means End Analysis (MEA) is a problem-solving technique that has been used since the fifties of the last century to stimulate creativity .
Means End Analysis is also a way of looking at the organisational planning , and helps in achieving the end-goals .

With Means End Analysis, it is possible to control the entire process of problem solving. It starts from a predetermined goal, in which actions are chosen that lead to that goal.
Each action that is executed leads to the next action; everything is connected together in order to reach the end-goal. In the meantime however, problems may arise. It is often hard to determine where exactly the crux is.
With the help of Means End Analysis, both forward and backward research can be done to determine where the stagnation is occurring. This enables the larger parts of a problem to be solved first, to subsequently return to the smaller problems afterwards.
Intermediate steps
In order for Means End Analysis to be effective, it is advisable to get all relevant actions and intermediate steps leading to the goal in the picture, making them detectable.
Additionally, it is handy to be capable of tracking (small) changes, and to measure the differences between the actual and desired state of the individual actions.
If this doesn’t happen, there is a significant risk that a mistake or change will have further consequences across the series of actions following it, making it harder and harder to intervene.
Every organisation works with goals that need to be met.
Depending on the goal a short term (a week or a month), mid-long term (a year), and a long term (muliple years) are determined. It is nice both for the organisation and for the employees when these goals are successfully met.
By making an analysis of the means and the intermediate actions with the help of Means End Analysis beforehand, it is easier to focus and not lose your way. It is a fact that goals don’t just achieve themselves. Based on careful planning , action should be undertaken.
Without planning there’s a significant chance for the organisation to head in the wrong direction, deviating from its pre-determined goal.
Means End Analysis example
To successfully execute Means End Analysis it is advisable to think from large to small; the eventual goal needs to be split into smaller sub-goals, making it overseeable for all parties that are going to work towards on achieving it.
When a commercial electronic business has the end-goal to reach a turnover of 15 million euro’s within a year, that is a noble thought. It means that all actions in that year will be geared towards meeting that 15 million euro limit.
However, it will only work when it becomes clear what has to be done to meet that turnover of 15 million. With the help of Means End Analysis, the end goal is split into a few smaller goals, which will contribute to the 15 million turnover:
- A specific product, for example the newest smartphone, needs to be sold aggressively;
- A minimal selling price is set, which dealers also must comply with;
- Aside from the newest smartphone, there are some related products that will be go to market as well.
Means End Analysis : Executable steps
Regardless of the splitting into smaller sub-goals, it will still not be possible for the organisation to achieve a turnover of 15 million. The search for even smaller, more specific steps, aids in them to achieving the end-goal.
These sub-sub-goals are translated into executable steps that are deployed by the organisation and used to achieve the original goal of a turnover of 15 million. In case there is stagnation of a problem somewhere, it becomes much easier to find the problem and fix that part of the process. Prior sub-goals are elaborated upon below:
- A specific marketing plan is developed for the smartphone to give publicity to the new product, especially via social media;
- New applications will be developped by the electronic business to be sold as a by-product;
- A special discount is offered to students when they can prove that they are, in fact, registered at an institute of higher education;
- An advertisement will be placed in door-to-door newspapers, whereby a coupon can be used to obtain a substantial trade-in discount for the old mobile phone.
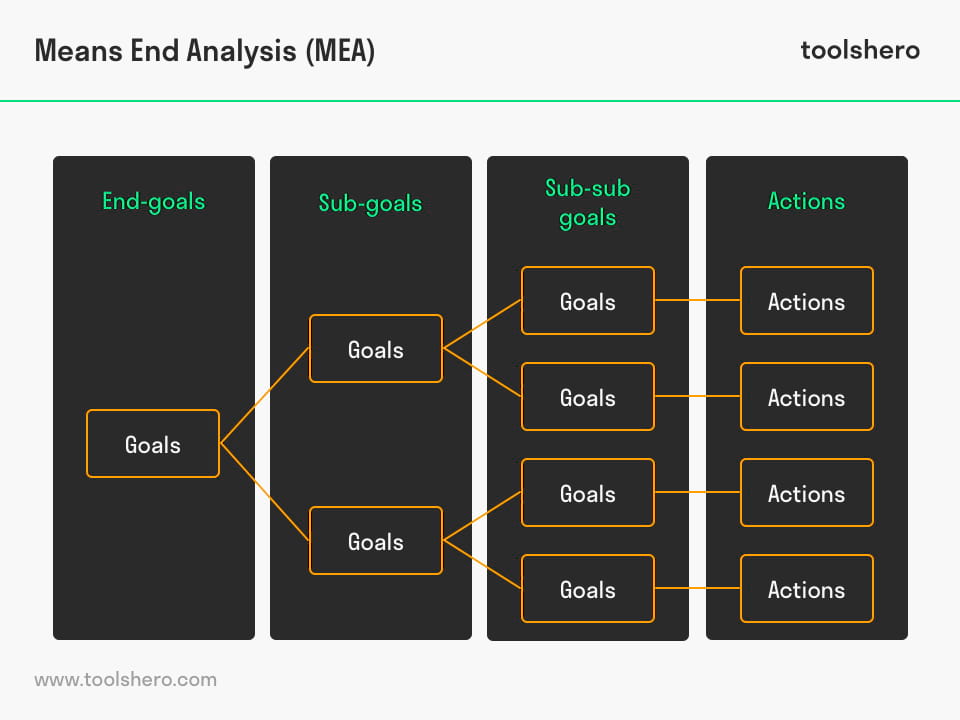
Figure 1 – an example overview of a Means End Analysis
Means End Analysis is about thoroughly thinking through which steps are needed in order to reach the end-goal.
Additionally, everyone within the organisation gets a reality check, because it shows that even the smallest steps have an impact on the overall goal that has been set .

It’s Your Turn
What do you think? Is Means End Analysis applicable in your personal or professional environment? Do you recognize the practical explanation or do you have more suggestions? What are your success factors for achieving end-goals??
Share your experience and knowledge in the comments box below.
More information
- Fikes, R. E., & Nilsson, N. J. (1971). STRIPS: A new approach to the application of theorem proving to problem solving . Artificial intelligence, 2(3-4), 189-208.
- Johnson, A. P. (2005). A short guide to action research . Boston: Pearson/ Allyn and Bacon .
- Sweller, J. (1988). Cognitive load during problem solving: Effects on learning . Cognitive science, 12(2), 257-285.
How to cite this article: Mulder, P. (2018). Means End Analysis (MEA) . Retrieved [insert date] from Toolshero: https://www.toolshero.com/problem-solving/means-end-analysis-mea/
Original publication date: 04/19/2018 | Last update: 01/25/2024
Add a link to this page on your website: <a href=”https://www.toolshero.com/problem-solving/means-end-analysis-mea/”> Toolshero: Means End Analysis (MEA)</a>
Did you find this article interesting?
Your rating is more than welcome or share this article via Social media!
Average rating 4.5 / 5. Vote count: 13
No votes so far! Be the first to rate this post.
We are sorry that this post was not useful for you!
Let us improve this post!
Tell us how we can improve this post?

Patty Mulder
Patty Mulder is an Dutch expert on Management Skills, Personal Effectiveness and Business Communication. She is also a Content writer, Business Coach and Company Trainer and lives in the Netherlands (Europe). Note: all her articles are written in Dutch and we translated her articles to English!
Related ARTICLES

Convergent Thinking: the Definition and Theory
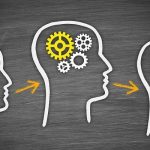
CATWOE Analysis: theory and example
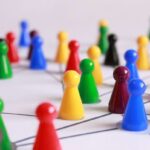
Systems Thinking: Theory and Definition
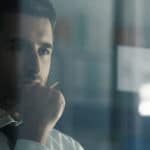
5 Whys Root Cause Analysis (Toyoda)
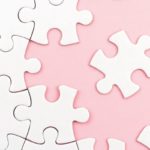
Positive Deviance (PD) explained
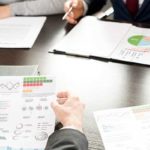
Root Cause Analysis (RCA)
Also interesting.

Crowdsourcing: the meaning, definition and some examples
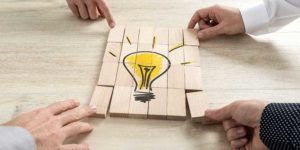
Systematic Inventive Thinking (SIT)
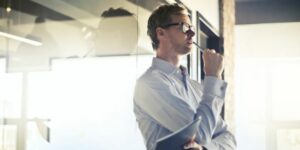
Linear Thinking by Edward De Bono explained
Leave a reply cancel reply.
You must be logged in to post a comment.
BOOST YOUR SKILLS
Toolshero supports people worldwide ( 10+ million visitors from 100+ countries ) to empower themselves through an easily accessible and high-quality learning platform for personal and professional development.
By making access to scientific knowledge simple and affordable, self-development becomes attainable for everyone, including you! Join our learning platform and boost your skills with Toolshero.

POPULAR TOPICS
- Change Management
- Marketing Theories
- Problem Solving Theories
- Psychology Theories
ABOUT TOOLSHERO
- Free Toolshero e-book
- Memberships & Pricing

How it works
For Business
Join Mind Tools
Article • 4 min read
Means-End Analysis
Identifying the steps needed to solve a problem.
By the Mind Tools Content Team
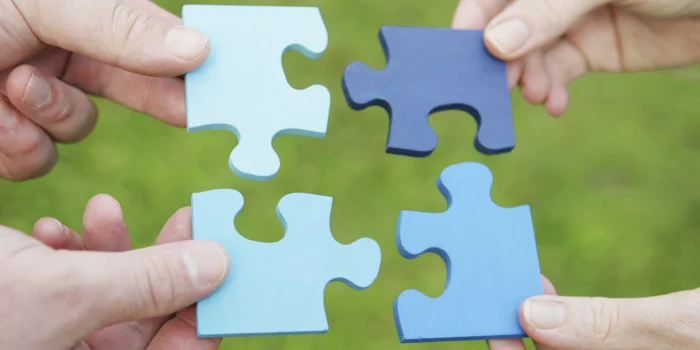
Means-End Analysis is a simple tool that helps you to identify the practical steps needed to solve a problem or to reach a desired state.
In this article we'll explore Means-End Analysis, and we'll look at how you can apply it.
About the Tool
Means-End Analysis is essentially an early form of Gap Analysis . It was created by researchers Allen Newell and Herbert Simon in the late 1950s, and it was then published their 1972 book, "Human Problem Solving."
Newell and Simon were creating an effective problem-solving program for early computers, and Means-End Analysis was a direct result of this research. They named the tool "Means-End" because it helps you define the means needed to reach a desired end.
Means-End Analysis might seem quite simplistic at first glance. However, when you begin using it, you'll find that it's a practical and useful method for solving simple problems.
How to Use the Tool
Step 1: define your initial state (problem).
Start by defining the problem you're trying to solve. It might help to write the problem down on a piece of paper, or even draw out a diagram.
It's important to you make sure you're trying to solve the right problem, and not just a symptom of a deeper issue. If you're struggling to do this, you can use tools such as Root Cause Analysis , Cause and Effect Analysis , CATWOE , and the 5 Whys to help define your problem, and to make sure that you're addressing the real issue.
Step 2: Visualize Your Goal State
Next, picture the ideal state you'd like to be in. This is the outcome you'd like to see, once the problem is solved.
Again, it might be helpful to write this out on paper.
Step 3: List the Differences Between States
Now, look at the differences between your initial state and your goal state. And then explore the obstacles that are stopping you from reaching this state. Make a list of these differences and obstacles.
If the obstacles seem overwhelmingly large, consider using Drill Down to break them down into easy-to-understand chunks.
Step 4: Create Sub-Goals
Once you have a list of the differences and obstacles that you need to overcome, you need to create sub-goals that will help you address each of these.
Think of these sub-goals as steps that will ultimately lead you to your desired goal state – look at each obstacle you've listed, and then create the plans you need to overcome them.
Step 5: Take Action
The last step is to take action on your analysis.
If you're dealing with a simple problem, you'll be able to identify all of the actions that you need to take to solve your problem quickly. ( Action Plans are useful here.)
However, if you're solving a difficult problem, or planning a new project, you'll likely have to do further analysis and planning. The Simplex Process is useful for solving complex problems, while our project management section will help you plan more complex projects.
Means-End Analysis is a simple problem-solving tool that you can use to solve well-defined problems, and to kick-start the planning stage of a new project.
To use the tool, first look at your initial state (the state you're in right now). Then, picture your desired goal state – this is the state you want to be in once you've solved the problem or completed the project.
Next, make a list of the obstacles that are standing in the way of your goal state, and create sub-goals that will guide you in overcoming all those obstacles.
Finally, take action on your analysis.
You've accessed 1 of your 2 free resources.
Get unlimited access
Discover more content
Matrix management.
An Outline of Approaches to Matrix Management With Guidance Around the Issues Involved
Book Insights
Carrots and Sticks: Unlock the Power of Incentives to Get Things Done
Add comment
Comments (0)
Be the first to comment!
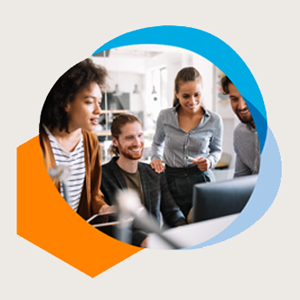
Try Mind Tools for FREE
Get unlimited access to all our career-boosting content and member benefits with our 7-day free trial.
Sign-up to our newsletter
Subscribing to the Mind Tools newsletter will keep you up-to-date with our latest updates and newest resources.
Subscribe now
Business Skills
Personal Development
Leadership and Management
Member Extras
Most Popular
Newest Releases
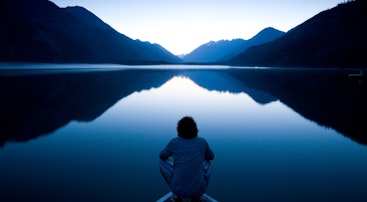
What Is Gibbs' Reflective Cycle?
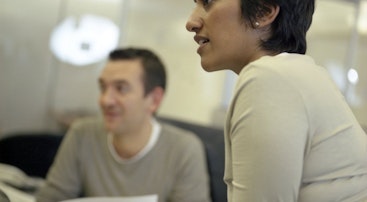
Team Briefings
Mind Tools Store
About Mind Tools Content
Discover something new today
Onboarding with steps.
Helping New Employees to Thrive
NEW! Pain Points Podcast - Perfectionism
Why Am I Such a Perfectionist?
How Emotionally Intelligent Are You?
Boosting Your People Skills
Self-Assessment
What's Your Leadership Style?
Learn About the Strengths and Weaknesses of the Way You Like to Lead
Recommended for you
Design a better business: new tools, skills and mindset for strategy and innovation.
Patrick van der Pijl, Justin Lokitz, and Lisa Kay Solomon
Business Operations and Process Management
Strategy Tools
Customer Service
Business Ethics and Values
Handling Information and Data
Project Management
Knowledge Management
Self-Development and Goal Setting
Time Management
Presentation Skills
Learning Skills
Career Skills
Communication Skills
Negotiation, Persuasion and Influence
Working With Others
Difficult Conversations
Creativity Tools
Self-Management
Work-Life Balance
Stress Management and Wellbeing
Coaching and Mentoring
Change Management
Team Management
Managing Conflict
Delegation and Empowerment
Performance Management
Leadership Skills
Developing Your Team
Talent Management
Problem Solving
Decision Making
Member Podcast
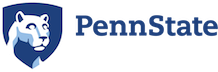
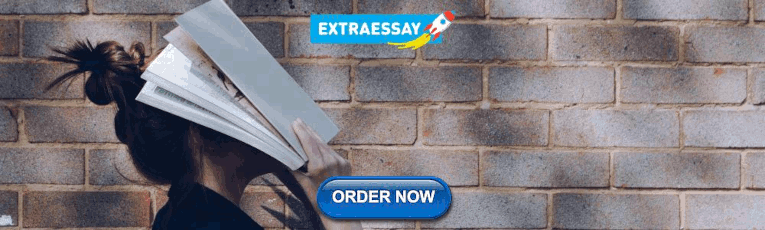
Psych 256: Cognitive Psychology SP15
Making connections between theory and reality, problem solving using means-end analysis.
Everyone has had a long list of to dos or personal things to take care of. Looking at the whole it can seem a little overwhelming and most of us get lost, depressed, or even frustrated. Using a means-end analysis is basically looking at a goal, starting point, and the best way to get from point A to point B by breaking down the list or problems to make them more doable in our lives (Newell & Simon, 1972). For this blog, I will pass on some tips on how to manage your homework load that you have each semester to lessen the stress. Keeping in mind, that these tips have worked for me but can be modified to individual needs, because let’s face it, each of us have different lifestyles and means-end analysis will not necessarily work for everyone problem.
Each of us when we start a new semester, whether it is at a new school or the current one, can feel overwhelmed by the work load that looms ahead of us. What I do to lessen the stress is I take each class syllabus and course calendar and a full size desk top calendar, the ones with the large squares for the dates, and I write down the class and what is due each week including test and quizzes. This way I can see clearly what I have to complete. This is the process of setting subgoals which helps to move toward the overall goal of course completion (Goldstein, 2011). Once classes are taken care of I will then insert any social or work related items. With all of this in place I have a black and white picture of my life each month. Once this is in place I can set up a realistic schedule to complete the semester and how it is broken down weekly, allows me to manage it properly without over stressing out. I take one day at a time. As each day passes you can cross out what has been completed and feel a sense of accomplishment.
Now we move into supplies to fulfill the semester. I will look at each class and see if any will need something other than the usual, such as art supplies, software, etc. Take a list to your local store and remember it is ok to over buy on paper, printer ink, and writing tools because you never can estimate what you might use up. I find that having all the items for each class available will help keep stress levels down by not having to run to the store at the last minute. Being well stocked will help prepare for the semester.
Next, let’s look at textbooks. Most of us will buy them and throw them in the back of our cars. The best thing to do is to look them over, read the index, and maybe a few chapters to get acclimated to the class. I use colored tabs to mark out each chapter, so I can easily flip to it. Being familiar with the classes you are taking and what is required in readings, online work, extra supplies, etc. will help you plan your weeks to come plus lower your stress levels.
Now the important thing is your study area. Some people will study in their bedroom, but this is too tempting of a place to take a nap or simply fall asleep and then your day is over. If all you have is your bedroom then I would suggest going to the school library or a quiet area in a coffee shop or 24 hour restaurant. I have a desk in a room with little to no distractions. I have everything I need in this room to complete my homework, readings, and school projects. I have set times to do my school work according to my calendar that I discussed earlier. Another thing that might help is turn off cell phones and any other communication or social media devices. Let people know that you will be studying at set times so that they will not text or call to disturb you. These types of disruptions can derail you from what you are reading or writing. If you have children, you can do what a friend of mine does and put a sign on the door letting your children know that it is mommy/daddy study time. Of course this doesn’t always work because children are children and that just doesn’t happen. In that case, perhaps work it out with your partner that they handle the kids while you are studying or have a friend or relative watch the children for that bit of time. Having a place to work just for you will increase productivity and or course lower some stress.
With some of the tips I have given, you can lower stress levels of the new semester by setting smaller goals so you can reach the finish line at the end of the semester. This is all made possible by using a means-end analysis solution. Keep in mind this may not work for you or you may have to modify the ideas that I have given to make it work in your life. Whatever will work by planning out your semester so you can achieve the grades is great. I hope you will be able to add some of or all of my tips to get you to your semester goals by breaking down the whole to more manageable pieces.
Goldstein, E. (2011). Cognitive psychology: Connecting mind, research, and everyday experience (3rd ed.). Australia: Wadsworth Cengage Learning.
Newell, A., & Simon, H. A. (1972). Human problem solving (Vol. 104, No. 9). Englewood Cliffs, NJ: Prentice-Hall.
Leave a Reply
You must be logged in to post a comment.
7.3 Problem-Solving
Learning objectives.
By the end of this section, you will be able to:
- Describe problem solving strategies
- Define algorithm and heuristic
- Explain some common roadblocks to effective problem solving
People face problems every day—usually, multiple problems throughout the day. Sometimes these problems are straightforward: To double a recipe for pizza dough, for example, all that is required is that each ingredient in the recipe be doubled. Sometimes, however, the problems we encounter are more complex. For example, say you have a work deadline, and you must mail a printed copy of a report to your supervisor by the end of the business day. The report is time-sensitive and must be sent overnight. You finished the report last night, but your printer will not work today. What should you do? First, you need to identify the problem and then apply a strategy for solving the problem.
The study of human and animal problem solving processes has provided much insight toward the understanding of our conscious experience and led to advancements in computer science and artificial intelligence. Essentially much of cognitive science today represents studies of how we consciously and unconsciously make decisions and solve problems. For instance, when encountered with a large amount of information, how do we go about making decisions about the most efficient way of sorting and analyzing all the information in order to find what you are looking for as in visual search paradigms in cognitive psychology. Or in a situation where a piece of machinery is not working properly, how do we go about organizing how to address the issue and understand what the cause of the problem might be. How do we sort the procedures that will be needed and focus attention on what is important in order to solve problems efficiently. Within this section we will discuss some of these issues and examine processes related to human, animal and computer problem solving.
PROBLEM-SOLVING STRATEGIES
When people are presented with a problem—whether it is a complex mathematical problem or a broken printer, how do you solve it? Before finding a solution to the problem, the problem must first be clearly identified. After that, one of many problem solving strategies can be applied, hopefully resulting in a solution.
Problems themselves can be classified into two different categories known as ill-defined and well-defined problems (Schacter, 2009). Ill-defined problems represent issues that do not have clear goals, solution paths, or expected solutions whereas well-defined problems have specific goals, clearly defined solutions, and clear expected solutions. Problem solving often incorporates pragmatics (logical reasoning) and semantics (interpretation of meanings behind the problem), and also in many cases require abstract thinking and creativity in order to find novel solutions. Within psychology, problem solving refers to a motivational drive for reading a definite “goal” from a present situation or condition that is either not moving toward that goal, is distant from it, or requires more complex logical analysis for finding a missing description of conditions or steps toward that goal. Processes relating to problem solving include problem finding also known as problem analysis, problem shaping where the organization of the problem occurs, generating alternative strategies, implementation of attempted solutions, and verification of the selected solution. Various methods of studying problem solving exist within the field of psychology including introspection, behavior analysis and behaviorism, simulation, computer modeling, and experimentation.
A problem-solving strategy is a plan of action used to find a solution. Different strategies have different action plans associated with them (table below). For example, a well-known strategy is trial and error. The old adage, “If at first you don’t succeed, try, try again” describes trial and error. In terms of your broken printer, you could try checking the ink levels, and if that doesn’t work, you could check to make sure the paper tray isn’t jammed. Or maybe the printer isn’t actually connected to your laptop. When using trial and error, you would continue to try different solutions until you solved your problem. Although trial and error is not typically one of the most time-efficient strategies, it is a commonly used one.
Another type of strategy is an algorithm. An algorithm is a problem-solving formula that provides you with step-by-step instructions used to achieve a desired outcome (Kahneman, 2011). You can think of an algorithm as a recipe with highly detailed instructions that produce the same result every time they are performed. Algorithms are used frequently in our everyday lives, especially in computer science. When you run a search on the Internet, search engines like Google use algorithms to decide which entries will appear first in your list of results. Facebook also uses algorithms to decide which posts to display on your newsfeed. Can you identify other situations in which algorithms are used?
A heuristic is another type of problem solving strategy. While an algorithm must be followed exactly to produce a correct result, a heuristic is a general problem-solving framework (Tversky & Kahneman, 1974). You can think of these as mental shortcuts that are used to solve problems. A “rule of thumb” is an example of a heuristic. Such a rule saves the person time and energy when making a decision, but despite its time-saving characteristics, it is not always the best method for making a rational decision. Different types of heuristics are used in different types of situations, but the impulse to use a heuristic occurs when one of five conditions is met (Pratkanis, 1989):
- When one is faced with too much information
- When the time to make a decision is limited
- When the decision to be made is unimportant
- When there is access to very little information to use in making the decision
- When an appropriate heuristic happens to come to mind in the same moment
Working backwards is a useful heuristic in which you begin solving the problem by focusing on the end result. Consider this example: You live in Washington, D.C. and have been invited to a wedding at 4 PM on Saturday in Philadelphia. Knowing that Interstate 95 tends to back up any day of the week, you need to plan your route and time your departure accordingly. If you want to be at the wedding service by 3:30 PM, and it takes 2.5 hours to get to Philadelphia without traffic, what time should you leave your house? You use the working backwards heuristic to plan the events of your day on a regular basis, probably without even thinking about it.
Another useful heuristic is the practice of accomplishing a large goal or task by breaking it into a series of smaller steps. Students often use this common method to complete a large research project or long essay for school. For example, students typically brainstorm, develop a thesis or main topic, research the chosen topic, organize their information into an outline, write a rough draft, revise and edit the rough draft, develop a final draft, organize the references list, and proofread their work before turning in the project. The large task becomes less overwhelming when it is broken down into a series of small steps.
Further problem solving strategies have been identified (listed below) that incorporate flexible and creative thinking in order to reach solutions efficiently.
Additional Problem Solving Strategies :
- Abstraction – refers to solving the problem within a model of the situation before applying it to reality.
- Analogy – is using a solution that solves a similar problem.
- Brainstorming – refers to collecting an analyzing a large amount of solutions, especially within a group of people, to combine the solutions and developing them until an optimal solution is reached.
- Divide and conquer – breaking down large complex problems into smaller more manageable problems.
- Hypothesis testing – method used in experimentation where an assumption about what would happen in response to manipulating an independent variable is made, and analysis of the affects of the manipulation are made and compared to the original hypothesis.
- Lateral thinking – approaching problems indirectly and creatively by viewing the problem in a new and unusual light.
- Means-ends analysis – choosing and analyzing an action at a series of smaller steps to move closer to the goal.
- Method of focal objects – putting seemingly non-matching characteristics of different procedures together to make something new that will get you closer to the goal.
- Morphological analysis – analyzing the outputs of and interactions of many pieces that together make up a whole system.
- Proof – trying to prove that a problem cannot be solved. Where the proof fails becomes the starting point or solving the problem.
- Reduction – adapting the problem to be as similar problems where a solution exists.
- Research – using existing knowledge or solutions to similar problems to solve the problem.
- Root cause analysis – trying to identify the cause of the problem.
The strategies listed above outline a short summary of methods we use in working toward solutions and also demonstrate how the mind works when being faced with barriers preventing goals to be reached.
One example of means-end analysis can be found by using the Tower of Hanoi paradigm . This paradigm can be modeled as a word problems as demonstrated by the Missionary-Cannibal Problem :
Missionary-Cannibal Problem
Three missionaries and three cannibals are on one side of a river and need to cross to the other side. The only means of crossing is a boat, and the boat can only hold two people at a time. Your goal is to devise a set of moves that will transport all six of the people across the river, being in mind the following constraint: The number of cannibals can never exceed the number of missionaries in any location. Remember that someone will have to also row that boat back across each time.
Hint : At one point in your solution, you will have to send more people back to the original side than you just sent to the destination.
The actual Tower of Hanoi problem consists of three rods sitting vertically on a base with a number of disks of different sizes that can slide onto any rod. The puzzle starts with the disks in a neat stack in ascending order of size on one rod, the smallest at the top making a conical shape. The objective of the puzzle is to move the entire stack to another rod obeying the following rules:
- 1. Only one disk can be moved at a time.
- 2. Each move consists of taking the upper disk from one of the stacks and placing it on top of another stack or on an empty rod.
- 3. No disc may be placed on top of a smaller disk.
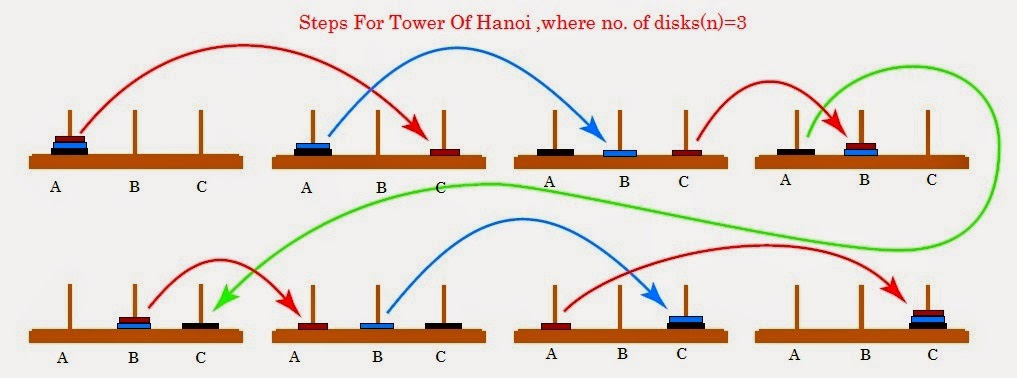
Figure 7.02. Steps for solving the Tower of Hanoi in the minimum number of moves when there are 3 disks.
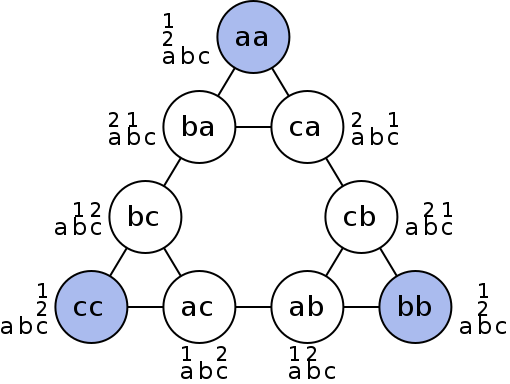
Figure 7.03. Graphical representation of nodes (circles) and moves (lines) of Tower of Hanoi.
The Tower of Hanoi is a frequently used psychological technique to study problem solving and procedure analysis. A variation of the Tower of Hanoi known as the Tower of London has been developed which has been an important tool in the neuropsychological diagnosis of executive function disorders and their treatment.
GESTALT PSYCHOLOGY AND PROBLEM SOLVING
As you may recall from the sensation and perception chapter, Gestalt psychology describes whole patterns, forms and configurations of perception and cognition such as closure, good continuation, and figure-ground. In addition to patterns of perception, Wolfgang Kohler, a German Gestalt psychologist traveled to the Spanish island of Tenerife in order to study animals behavior and problem solving in the anthropoid ape.
As an interesting side note to Kohler’s studies of chimp problem solving, Dr. Ronald Ley, professor of psychology at State University of New York provides evidence in his book A Whisper of Espionage (1990) suggesting that while collecting data for what would later be his book The Mentality of Apes (1925) on Tenerife in the Canary Islands between 1914 and 1920, Kohler was additionally an active spy for the German government alerting Germany to ships that were sailing around the Canary Islands. Ley suggests his investigations in England, Germany and elsewhere in Europe confirm that Kohler had served in the German military by building, maintaining and operating a concealed radio that contributed to Germany’s war effort acting as a strategic outpost in the Canary Islands that could monitor naval military activity approaching the north African coast.
While trapped on the island over the course of World War 1, Kohler applied Gestalt principles to animal perception in order to understand how they solve problems. He recognized that the apes on the islands also perceive relations between stimuli and the environment in Gestalt patterns and understand these patterns as wholes as opposed to pieces that make up a whole. Kohler based his theories of animal intelligence on the ability to understand relations between stimuli, and spent much of his time while trapped on the island investigation what he described as insight , the sudden perception of useful or proper relations. In order to study insight in animals, Kohler would present problems to chimpanzee’s by hanging some banana’s or some kind of food so it was suspended higher than the apes could reach. Within the room, Kohler would arrange a variety of boxes, sticks or other tools the chimpanzees could use by combining in patterns or organizing in a way that would allow them to obtain the food (Kohler & Winter, 1925).
While viewing the chimpanzee’s, Kohler noticed one chimp that was more efficient at solving problems than some of the others. The chimp, named Sultan, was able to use long poles to reach through bars and organize objects in specific patterns to obtain food or other desirables that were originally out of reach. In order to study insight within these chimps, Kohler would remove objects from the room to systematically make the food more difficult to obtain. As the story goes, after removing many of the objects Sultan was used to using to obtain the food, he sat down ad sulked for a while, and then suddenly got up going over to two poles lying on the ground. Without hesitation Sultan put one pole inside the end of the other creating a longer pole that he could use to obtain the food demonstrating an ideal example of what Kohler described as insight. In another situation, Sultan discovered how to stand on a box to reach a banana that was suspended from the rafters illustrating Sultan’s perception of relations and the importance of insight in problem solving.
Grande (another chimp in the group studied by Kohler) builds a three-box structure to reach the bananas, while Sultan watches from the ground. Insight , sometimes referred to as an “Ah-ha” experience, was the term Kohler used for the sudden perception of useful relations among objects during problem solving (Kohler, 1927; Radvansky & Ashcraft, 2013).
Solving puzzles.
Problem-solving abilities can improve with practice. Many people challenge themselves every day with puzzles and other mental exercises to sharpen their problem-solving skills. Sudoku puzzles appear daily in most newspapers. Typically, a sudoku puzzle is a 9×9 grid. The simple sudoku below (see figure) is a 4×4 grid. To solve the puzzle, fill in the empty boxes with a single digit: 1, 2, 3, or 4. Here are the rules: The numbers must total 10 in each bolded box, each row, and each column; however, each digit can only appear once in a bolded box, row, and column. Time yourself as you solve this puzzle and compare your time with a classmate.
How long did it take you to solve this sudoku puzzle? (You can see the answer at the end of this section.)
Here is another popular type of puzzle (figure below) that challenges your spatial reasoning skills. Connect all nine dots with four connecting straight lines without lifting your pencil from the paper:
Did you figure it out? (The answer is at the end of this section.) Once you understand how to crack this puzzle, you won’t forget.
Take a look at the “Puzzling Scales” logic puzzle below (figure below). Sam Loyd, a well-known puzzle master, created and refined countless puzzles throughout his lifetime (Cyclopedia of Puzzles, n.d.).

What steps did you take to solve this puzzle? You can read the solution at the end of this section.
Pitfalls to problem solving.
Not all problems are successfully solved, however. What challenges stop us from successfully solving a problem? Albert Einstein once said, “Insanity is doing the same thing over and over again and expecting a different result.” Imagine a person in a room that has four doorways. One doorway that has always been open in the past is now locked. The person, accustomed to exiting the room by that particular doorway, keeps trying to get out through the same doorway even though the other three doorways are open. The person is stuck—but she just needs to go to another doorway, instead of trying to get out through the locked doorway. A mental set is where you persist in approaching a problem in a way that has worked in the past but is clearly not working now.
Functional fixedness is a type of mental set where you cannot perceive an object being used for something other than what it was designed for. During the Apollo 13 mission to the moon, NASA engineers at Mission Control had to overcome functional fixedness to save the lives of the astronauts aboard the spacecraft. An explosion in a module of the spacecraft damaged multiple systems. The astronauts were in danger of being poisoned by rising levels of carbon dioxide because of problems with the carbon dioxide filters. The engineers found a way for the astronauts to use spare plastic bags, tape, and air hoses to create a makeshift air filter, which saved the lives of the astronauts.
Researchers have investigated whether functional fixedness is affected by culture. In one experiment, individuals from the Shuar group in Ecuador were asked to use an object for a purpose other than that for which the object was originally intended. For example, the participants were told a story about a bear and a rabbit that were separated by a river and asked to select among various objects, including a spoon, a cup, erasers, and so on, to help the animals. The spoon was the only object long enough to span the imaginary river, but if the spoon was presented in a way that reflected its normal usage, it took participants longer to choose the spoon to solve the problem. (German & Barrett, 2005). The researchers wanted to know if exposure to highly specialized tools, as occurs with individuals in industrialized nations, affects their ability to transcend functional fixedness. It was determined that functional fixedness is experienced in both industrialized and nonindustrialized cultures (German & Barrett, 2005).
In order to make good decisions, we use our knowledge and our reasoning. Often, this knowledge and reasoning is sound and solid. Sometimes, however, we are swayed by biases or by others manipulating a situation. For example, let’s say you and three friends wanted to rent a house and had a combined target budget of $1,600. The realtor shows you only very run-down houses for $1,600 and then shows you a very nice house for $2,000. Might you ask each person to pay more in rent to get the $2,000 home? Why would the realtor show you the run-down houses and the nice house? The realtor may be challenging your anchoring bias. An anchoring bias occurs when you focus on one piece of information when making a decision or solving a problem. In this case, you’re so focused on the amount of money you are willing to spend that you may not recognize what kinds of houses are available at that price point.
The confirmation bias is the tendency to focus on information that confirms your existing beliefs. For example, if you think that your professor is not very nice, you notice all of the instances of rude behavior exhibited by the professor while ignoring the countless pleasant interactions he is involved in on a daily basis. Hindsight bias leads you to believe that the event you just experienced was predictable, even though it really wasn’t. In other words, you knew all along that things would turn out the way they did. Representative bias describes a faulty way of thinking, in which you unintentionally stereotype someone or something; for example, you may assume that your professors spend their free time reading books and engaging in intellectual conversation, because the idea of them spending their time playing volleyball or visiting an amusement park does not fit in with your stereotypes of professors.
Finally, the availability heuristic is a heuristic in which you make a decision based on an example, information, or recent experience that is that readily available to you, even though it may not be the best example to inform your decision . Biases tend to “preserve that which is already established—to maintain our preexisting knowledge, beliefs, attitudes, and hypotheses” (Aronson, 1995; Kahneman, 2011). These biases are summarized in the table below.
Were you able to determine how many marbles are needed to balance the scales in the figure below? You need nine. Were you able to solve the problems in the figures above? Here are the answers.

Many different strategies exist for solving problems. Typical strategies include trial and error, applying algorithms, and using heuristics. To solve a large, complicated problem, it often helps to break the problem into smaller steps that can be accomplished individually, leading to an overall solution. Roadblocks to problem solving include a mental set, functional fixedness, and various biases that can cloud decision making skills.
References:
Openstax Psychology text by Kathryn Dumper, William Jenkins, Arlene Lacombe, Marilyn Lovett and Marion Perlmutter licensed under CC BY v4.0. https://openstax.org/details/books/psychology
Review Questions:
1. A specific formula for solving a problem is called ________.
a. an algorithm
b. a heuristic
c. a mental set
d. trial and error
2. Solving the Tower of Hanoi problem tends to utilize a ________ strategy of problem solving.
a. divide and conquer
b. means-end analysis
d. experiment
3. A mental shortcut in the form of a general problem-solving framework is called ________.
4. Which type of bias involves becoming fixated on a single trait of a problem?
a. anchoring bias
b. confirmation bias
c. representative bias
d. availability bias
5. Which type of bias involves relying on a false stereotype to make a decision?
6. Wolfgang Kohler analyzed behavior of chimpanzees by applying Gestalt principles to describe ________.
a. social adjustment
b. student load payment options
c. emotional learning
d. insight learning
7. ________ is a type of mental set where you cannot perceive an object being used for something other than what it was designed for.
a. functional fixedness
c. working memory
Critical Thinking Questions:
1. What is functional fixedness and how can overcoming it help you solve problems?
2. How does an algorithm save you time and energy when solving a problem?
Personal Application Question:
1. Which type of bias do you recognize in your own decision making processes? How has this bias affected how you’ve made decisions in the past and how can you use your awareness of it to improve your decisions making skills in the future?
anchoring bias
availability heuristic
confirmation bias
functional fixedness
hindsight bias
problem-solving strategy
representative bias
trial and error
working backwards
Answers to Exercises
algorithm: problem-solving strategy characterized by a specific set of instructions
anchoring bias: faulty heuristic in which you fixate on a single aspect of a problem to find a solution
availability heuristic: faulty heuristic in which you make a decision based on information readily available to you
confirmation bias: faulty heuristic in which you focus on information that confirms your beliefs
functional fixedness: inability to see an object as useful for any other use other than the one for which it was intended
heuristic: mental shortcut that saves time when solving a problem
hindsight bias: belief that the event just experienced was predictable, even though it really wasn’t
mental set: continually using an old solution to a problem without results
problem-solving strategy: method for solving problems
representative bias: faulty heuristic in which you stereotype someone or something without a valid basis for your judgment
trial and error: problem-solving strategy in which multiple solutions are attempted until the correct one is found
working backwards: heuristic in which you begin to solve a problem by focusing on the end result

Share This Book
- Increase Font Size

An official website of the United States government
The .gov means it’s official. Federal government websites often end in .gov or .mil. Before sharing sensitive information, make sure you’re on a federal government site.
The site is secure. The https:// ensures that you are connecting to the official website and that any information you provide is encrypted and transmitted securely.
- Publications
- Account settings
Preview improvements coming to the PMC website in October 2024. Learn More or Try it out now .
- Advanced Search
- Journal List
- HHS Author Manuscripts

Remembering the past and imagining the future: Identifying and enhancing the contribution of episodic memory
Recent studies have shown that imagining or simulating future events relies on many of the same cognitive and neural processes as remembering past events. According to the constructive episodic simulation hypothesis ( Schacter and Addis, 2007 ), such overlap indicates that both remembered past and imagined future events rely heavily on episodic memory: future simulations are built on retrieved details of specific past experiences that are recombined into novel events. An alternative possibility is that commonalities between remembering and imagining reflect the influence of more general, non-episodic factors such as narrative style or communicative goals that shape the expression of both memory and imagination. We consider recent studies that distinguish the contributions of episodic and non-episodic processes in remembering the past and imagining the future by using an episodic specificity induction – brief training in recollecting the details of a past experience – and also extend this approach to the domains of problem solving and creative thinking. We conclude by suggesting that the specificity induction may target a process of scene construction that contributes to episodic memory as well as to imagination, problem solving, and creative thinking.
Decades of research in psychology and neuroscience have revealed much about how memory connects individuals to the past, but researchers have increasingly realized that memory is also important for connecting the present with the future ( Szpunar et al., 2014 ). This realization has been fueled in part by demonstrations of striking similarities in the cognitive and neural processes involved in remembering the past and imagining or simulating possible future experiences (for recent reviews, see Klein, 2013 ; Schacter et al., 2012 ; Szpunar, 2010 ). These similarities include phenomenal characteristics (e.g. similar sensory and contextual features; D’Argembeau and Van der Linden, 2004 ; Szpunar and McDermott, 2008 ), a common tendency to reflect major concerns of the self (e.g. Rathbone et al., 2011 ), and reliance on a common core network of brain regions (e.g. Benoit and Schacter, 2015 ; Buckner and Carroll, 2007 ; Schacter et al., 2007 ; Spreng et al., 2009 ). Some differences between remembering the past and imagining the future have also been documented (for discussion, see MacLeod, 2016 ; Schacter et al., 2012 ), but the similarities are striking nonetheless.
A number of researchers have argued that these similarities arise, at least in part, because remembering past experiences and imagining future experiences both rely heavily on a particular form of memory known as episodic memory. According to Tulving (2002) , episodic memory supports our ability to recollect specific personal experiences – events that happened to us at particular times and places in the past. Episodic memory allows the remembering self to engage in “mental time travel” and to consciously re-experience a past happening. Episodic memory is thus distinguished from other kinds of memory, such as semantic memory , which supports general knowledge and retrieval of factual information, and procedural memory , which supports the acquisition of skills, as well as related forms of nonconscious or implicit memory (e.g. Schacter and Tulving, 1994 ).
Evidence that episodic memory plays an important role in imagining future experiences comes from various sources. For example, amnesic patients, who have severe deficits in episodic memory as a consequence of damage to brain regions including the hippocampus, also have difficulties imagining their personal futures or novel scenes (e.g. Conway et al., 2016 ; Hassabis et al., 2007 ; Klein et al., 2002 ; Race et al., 2011 ; but see also, Squire et al., 2010 ). A striking example comes from the profoundly amnesic patient KC: he could not remember a single specific episode from his past, nor could he imagine a single specific episode that might occur in his personal future ( Rosenbaum et al., 2005 ; Tulving, 1985 ). Similarly, other patient populations that exhibit reduced recall of episodic details about their personal pasts also imagine relatively few episodic details about their personal futures, including patients with temporary amnesia ( Juskenaite et al., 2014 ), depression ( Williams et al., 1996 ), schizophrenia ( D’Argembeau et al., 2008 ), post-traumatic stress disorder ( Brown et al., 2014 ), and Alzheimer’s disease ( Addis et al., 2009 ).
These and related observations led Schacter and Addis (2007) to put forth the constructive episodic simulation hypothesis : a critical function of episodic memory is to support the construction of imagined future events by allowing the retrieval of information about past experiences, and the flexible recombination of elements of past experiences, into simulations of possible future scenarios. This flexibility of episodic memory makes it well suited to supporting simulations of different ways that future experiences might unfold, but according to the constructive episodic simulation hypothesis, this very flexibility can also result in memory errors from miscombining elements of past experiences or confusing imagined and actual events ( Schacter and Addis, 2007 ; for recent evidence, see Devitt et al., 2016 , and for related ideas, see Dudai and Carruthers, 2005 ; Dudai and Edelson, 2016 ; Schacter, et al., 2011 ; Suddendorf and Busby, 2003 ).
It is important to note that while the constructive episodic simulation hypothesis focuses on the contribution of episodic memory to imagining future experiences, it is clear that general knowledge or semantic memory also contributes importantly to future thinking (e.g. Irish et al., 2012 ). While a discussion of the contribution of semantic memory to future thinking is beyond the scope of the present article, several recent articles summarize and discuss relevant evidence ( Klein, 2013 ; MacLeod, 2016 ; Merck et al., 2016 ; Schacter et al., 2012 ; Szpunar et al., 2014 ).
A Challenge to the Constructive Episodic Simulation Hypothesis
In line with the evidence discussed above, several studies have shown that healthy older adults provide fewer episodic details than young adults both when they remember the past and imagine the future (see Schacter et al., 2013 , for a recent review). This pattern was first reported in a study from our laboratory by Addis et al. (2008) where young and older adults performed an adapted version of the Autobiographical Interview (AI; Levine et al., 2002 ), which distinguishes between the “internal” and “external” details that comprise autobiographical memories. Internal details reflect episodic information: what happened during an experience, who was there, and when and where the event occurred. By contrast, external details are mainly comprised of semantic information: related facts, reflections on and inferences about the meaning of what happened, or references to other events. Addis et al. (2008) reported that older adults provided significantly fewer internal/episodic details and more external/semantic details about both remembered past events and imagined future events compared with young adults. These and subsequent similar findings (e.g. Addis et al., 2010 ; Cole et al., 2013 ; Rendell et al., 2012 ) suggest that age-related differences in remembering the past and imagining the future may be primarily attributable to changes in episodic memory mechanisms, and thus support the constructive episodic simulation hypothesis.
However, this conclusion was challenged by further research in our laboratory showing that when older adults simply describe a picture of a complex scene, a task that should not recruit episodic memory mechanisms, they also exhibit reduced internal details (i.e. details referring to elements present in the picture) and increased external details (i.e. commentary and inferences about the picture; Gaesser et al., 2011 ). These findings raise the possibility that age-related changes in remembering the past and imagining the future reflect primarily the operation of non-episodic mechanisms, such as changes in narrative style or communicative goals that occur with aging and could affect performance similarly on memory, imagination, and picture description tasks. For example, some studies suggest that older adults employ a more general narrative style and have different communicative goals than young adults (e.g. Adams et al., 1997 ; Labouvie-Vief and Blanchard-Fields, 1982 ). If older adults communicate in a more general way than do young adults, then they may report fewer internal details and more external details than young adults when asked to remember the past and imagine the future not because of age-related differences in episodic memory mechanisms, but because of differences in descriptive ability, narrative style, or other non-episodic processes that produce similar patterns on a picture description task (for general discussion of narrative and memory, see Fivush and Merrill, 2016 ).
These findings also raise broader questions about interpreting similarities between remembering the past and imagining the future, even in studies that are not focused on aging. Contrary to the central idea of the constructive episodic simulation hypothesis that these similarities reflect mainly the operation of episodic memory processes, such similarities might reflect primarily or entirely the operation of more general, non-episodic processes, such as communicative goals or narrative style, that similarly influence remembering the past, imagining the future, and describing a picture in the present. Observations of cultural effects on remembering the past and imagining the future (see Wang, 2016 ) also suggest a role for general influences. These observations raise an important question: Can we identify the contribution of episodic memory to performance on a particular cognitive task and distinguish it from the influences of non-episodic processes?
Distinguishing Episodic from Non-Episodic Processes: An Episodic Specificity Induction
We ( Madore et al., 2014 ) recently developed an experimental approach to distinguish episodic from non-episodic influences that relies on what we call an episodic specificity induction : brief training in recollecting details of a recent experience (cf. Maestas and Rude, 2012 ; Neshat-Doost et al., 2012 ; Raes et al., 2009 ). The logic of our approach is straightforward: if a cognitive task relies at least in part on episodic memory, then performance on that task should be affected by a prior episodic specificity induction. By contrast, if performance on a cognitive task does not rely on episodic memory, then task performance should not be influenced by a prior episodic specificity induction. Thus, we use the specificity induction as an experimental tool for targeting episodic memory.
Our episodic specificity induction is based on a well-established procedure known as the Cognitive Interview (CI; Fisher and Geiselman, 1992 ), a protocol that encourages people to focus on specific details of past experiences during retrieval attempts, and that is useful for increasing detailed episodic recall in eyewitnesses (for review, see Memon et al., 2010 ). In our first study using this induction ( Madore et al., 2014 ), both young and older participants viewed a video of people interacting in a kitchen. For the specificity induction, participants were then guided to recall the video in specific episodic detail with procedures adapted from the CI: generating a mental picture and reporting everything they remember about the scene in as much detail as possible, including what people looked like and did, how objects were arranged, and so forth. For a control induction, the same participants watched a video similar to the one shown before the specificity induction and were then instructed to provide their general impressions of the video, but were not asked to recall specific details. Shortly after each induction, participants viewed pictures of everyday scenes, and either remembered past experiences or imagined future experiences related to each picture, or simply described the contents of the pictures, as in the earlier work from our laboratory ( Gaesser et al., 2011 ).
Compared with the control induction, the episodic specificity induction produced an increase in the number of internal (episodic) details that young and older participants provided when they remembered past experiences and imagined future experiences. In sharp contrast, however, the specificity induction had no effect on the number of external (semantic) details that participants produced on these memory and imagination tasks. Critically, the specificity induction had no effect on the number of internal or external details that participants produced on the picture description task. We replicated this pattern of results in a second experiment using a different control induction ( Madore et al., 2014 ).
These findings suggest that the specificity induction selectively targets and enhances episodic retrieval, dissociating it from both semantic retrieval and narrative description. We therefore argued that, in line with the constructive episodic simulation hypothesis, these data provide strong evidence that remembering the past and imagining the future (but not describing a picture) depend heavily on episodic memory. However, another interpretation is that whereas the memory and imagination tasks require participants to generate details that are not present in the picture cues, describing a picture is more directly constrained by the properties of the presented picture and does not require such generative retrieval. Perhaps the specificity induction impacts any task that requires generative retrieval, whether or not that task involves episodic memory in particular (for discussion of generative retrieval, see Addis et al., 2012 ; Conway and Pleydell-Pearce, 2000 ). Therefore, it is important to determine whether an episodic specificity induction still selectively affects memory and imagination tasks compared with a non-episodic control task that also requires generative retrieval.
To address the issue, Madore and Schacter (2016) conducted an experiment that is similar to the one described above with two important differences. First, we used words instead of pictures as cues for memory and imagination. Second, for the non-episodic task we used a word comparison task in which participants receive a word cue, generate a size sentence that included the word cue as well as related words (e.g. for the cue word “Apple”, a size sentence would be “Tree is larger than Pie is larger than Apple”), and then generate definitions for the three nouns. For this task, the critical measure is the amount of detail in the definitions that participants generate.
The results showed again that the episodic specificity induction, compared with control inductions, boosted the number of internal details that people provide when remembering past experiences and imagining future experiences. Critically, the specificity induction had no effect on the word comparison task: the definitions that participants generated contained similar amounts of detail following the episodic specificity induction and the control inductions.
These findings are important because 1) they indicate that the specificity induction selectively impacts episodic retrieval during remembering and imagining even when the non-episodic task (i.e. word comparison) requires generative search and retrieval; and 2) along with the results of our previous experiments, these data show that the specificity induction increases the amount of detail contained in episodic simulations of future experiences. In light of the many adaptive functions supported by episodic simulation ( Schacter, 2012 ), specificity inductions may be useful for enhancing performance on tasks that benefit from detailed episodic simulations (e.g. Beaman et al., 2007 ; Gaesser and Schacter, 2014 ; MacLeod, 2016 ; Sheldon et al., 2011 ). We elaborate on this point in the next section.
Using the Specificity Induction to Identify Contributions of Episodic Memory to Means-End Problem Solving and Divergent Creative Thinking
The foregoing results suggest that we can use the specificity induction to identify and enhance possible contributions of episodic memory to other cognitive tasks that do not require episodic memory, but may nonetheless be influenced by it. One such task is Means-End Problem Solving (MEPS; Platt and Spivack, 1975 ), where people are presented with hypothetical social problems, such as difficulties with friends or handling a situation at work, and are asked to generate steps or means that lead to problem solutions. For example, a step that is relevant to solving a problem involving difficulties with friends would be to ask a friend about what is wrong. Several studies suggest that episodic memory may contribute to performance on the MEPS task in depressed and anxious individuals (e.g. Raes et al., 2005 ), as well as in healthy young and older adults (e.g. Beaman et al., 2007 ; Sheldon et al., 2011 ).
In light of these results and our previous specificity induction studies, we predicted that our episodic specificity induction would enhance performance on the MEPS task. We tested the prediction in an experiment where young and older adults received either an episodic specificity induction or a control induction, and then performed the MEPS task ( Madore and Schacter, 2014 ). Consistent with our prediction, participants in both groups generated a greater number of steps relevant to solving MEPS problems following the episodic specificity induction than following a control induction, and their solution steps contained more episodic details after the specificity induction. By contrast, type of induction had no effect on the number of irrelevant steps that participants generated, indicating again that the effects of the specificity induction are selective.
Recent evidence also suggests a link between episodic memory and a component of creativity known as divergent thinking or the ability to generate creative ideas by combining diverse types of information ( Guilford, 1967 ). For example, amnesic patients with episodic memory deficits also exhibit impairments in divergent thinking ( Duff et al., 2013 ) and brain regions associated with episodic memory become active during a divergent thinking task (e.g. Benedek et al., 2014 ). Moreover, a study from our laboratory showed that performance on a divergent thinking task is positively correlated with the amount of episodic detail that young and older adults generate when they imagine future experiences ( Addis et al., 2016 ).
These findings led us to predict that performance on a divergent thinking task would be enhanced by a prior episodic specificity induction. We tested this hypothesis on the Alternate Uses Task, where participants attempt to generate unusual and creative uses of common objects (e.g. try to think of different ways in which a newspaper could be used; Guilford, 1967 ). In line with our hypothesis, participants generated significantly more unusual uses on this task after an episodic specificity induction than after a control induction ( Madore et al., 2015 ). Once again, the effects of the specificity induction were selective: type of induction had no effect on performance on an object association task in which participants were given the names of common objects and attempted to think of related objects. The object association task is thought to involve little divergent thinking or episodic imagery ( Abraham et al., 2012 ), and thus we expected that performance on this task would not benefit from a prior specificity induction, in line with observed results.
Concluding Comments
The findings reviewed in this article support two broad conclusions. First, the specificity induction can help to distinguish episodic retrieval processes that are important for memory and imagination tasks from non-episodic processes that are important for tasks such as picture description and word comparison. These findings support the constructive episodic simulation hypothesis ( Schacter and Addis, 2007 ) by specifically linking episodic memory with imagining future experiences. Second, the specificity induction can be used to identify and enhance the contribution of episodic retrieval to a range of cognitive tasks, including imagining the future, solving means-end problems, and divergent creative thinking.
An important challenge for future research is to characterize more precisely what processes common to memory, imagination, problem solving, and divergent thinking tasks are impacted by the specificity induction. We think that the specificity induction biases the way in which participants approach cognitive tasks by encouraging them to focus on episodic details related to places, people, or actions, which in turn impacts subsequent performance on tasks that involve creating mental events containing details like those emphasized during the specificity induction. Thus, the specificity induction may impact primarily what Hassabis and Maguire (2007) have called scene construction : assembling and maintaining a coherent mental scene or event. Scene construction is closely related to episodic memory. As Hassabis and Maguire (2007) observed, “A rich recollective experience is a key feature of episodic memory recall…Here, we have put forward the case for scene construction as a well-defined and key component process in supporting that recollective experience. Scene construction provides the stage on which the remembered event is played…” We agree with this assessment, and further hypothesize that some degree of scene construction is involved when people remember a past experience, imagine a future experience, simulate ways to solve everyday means-end problems, or generate alternative uses of objects. The induction, by biasing an episodic retrieval orientation toward specificity, may facilitate subsequent scene construction by encouraging people to focus their retrieval attempts on details of the key elements that comprise a mental scene (i.e. people, objects, or settings). By contrast, we suggest that scene construction plays little or no role in tasks such as picture description, word comparison, or object association, which are not impacted by the specificity induction and should not require mental scene building. While it will be important for future studies to test this hypothesis experimentally, note that research on scene construction has focused especially on the spatial coherence of constructed scenes; indeed, one of the key measures of scene construction, the spatial coherence index, assesses the spatial integrity of a constructed scene ( Hassabis et al., 2007 ). We do not claim that the specificity induction selectively impacts the spatial coherence of a constructed scene. Instead, we suggest that the induction could potentially impact the details associated with both elements of a scene and their relations, including spatial relations. Thus, we use the term “scene construction” in a broad sense that is roughly similar to the notion of “event construction” discussed by others (e.g. Romero and Moscovitch, 2012 ).
If the specificity induction does indeed impact primarily a scene or event construction process, questions arise concerning whether the induction selectively or exclusively impacts episodic memory processes associated with such constructions. As noted earlier, scene construction and episodic retrieval are closely related, and therefore it makes good sense that an episodic specificity induction would impact scene construction. But according to Hassabis and Maguire (2007) , scene construction is also involved in imagining scenes that are not, strictly speaking, “episodic” in the sense that they do not refer to a particular personally experienced episode from the past or to a possible personal future episode (e.g. “imagine a jungle”; for discussion, see Hassabis and Maguire, 2007 ). Although episodic memories may contribute to such seemingly generic scenes (e.g. when imagining a jungle I may remember a jungle scene from a recently seen movie), it remains to be determined whether the effects of the specificity induction observed in our studies indicate that heightened focus on episodic details during the induction enhances subsequent scene construction during memory, imagination, problem solving, and divergent thinking tasks, or whether the induction increases focus on both episodic and more generic scene details that selectively impact performance on these and related tasks. Experiments that address such issues will allow us to determine how to best characterize the nature and effects of specificity inductions on subsequent tasks.
Finally, we suggest that disentangling the contributions of episodic and non-episodic processes to memory, imagination, and related functions should also provide a stronger foundation for understanding how these processes contribute to related constructs, such as psychological well-being (e.g. MacLeod, 2016 ; MacLeod and Conway, 2005 ). For example, several studies have linked reduced specificity of autobiographical memory and future thinking with reduced psychological well-being (e.g. Brown et al., 2014 ; Williams et al., 1996 , 2007 ) and some evidence indicates that training aimed at increasing autobiographical memory specificity can enhance psychological well-being (e.g. Neshat-Doost et al., 2013; Raes et al., 2009 ). Yet little is known about which features of specificity training contribute to observed improvements in psychological well-being (e.g. episodic retrieval processes, non-episodic narrative processes, or both). Future research aimed at pinpointing the processes that support training effects should enhance our understanding of how remembering the past and imagining the future support a variety of psychological functions.
Acknowledgments
The author(s) disclosed receipt of the following financial support for the research, authorship, and/or publication of this article: Preparation of this article was supported by grants from the National Institute of Mental Health (MH060941) and National Institute on Aging (AG08441) to DLS.
Biographies
Daniel L Schacter received his PhD from the University of Toronto and is currently William R Kenan, Jr. Professor of Psychology at Harvard University. His research has explored numerous aspects of human memory and imagination, and he is the author of several books, including Searching for Memory (1996) and The Seven Sins of Memory (2001). Schacter has received a number of awards, including the American Psychological Association’s Award for Distinguished Scientific Contributions, and has been elected to the American Academy of Arts and Science and the National Academy of Sciences.
Kevin P Madore is a PhD student in Psychology at Harvard University. He studies the processes and uses of episodic memory. He received his BA in Psychology and History from Middlebury College.
Declaration of Conflicting Interests
The author(s) declared no potential conflicts of interest with respect to the research, authorship, and/or publication of this article.
- Abraham A, Pieritz K, Thybusch K, et al. Creativity and the brain: Uncovering the neural signature of conceptual expansion. Neuropsychologia. 2012; 50 :1906–1917. doi: 10.1016/j.neuropsychologia.2012.04.015. [ PubMed ] [ CrossRef ] [ Google Scholar ]
- Adams C, Smith MC, Nyquist L, et al. Adult age-group differences in recall for the literal and interpretive meanings of narrative text. Journal of Gerontology. 1997; 52 :187–195. doi: 10.1093/geronb/52B.4.P187. [ PubMed ] [ CrossRef ] [ Google Scholar ]
- Addis DR, Knapp K, Roberts RP, et al. Routes to the past: Neural substrates of direct and generative autobiographical memory retrieval. NeuroImage. 2012; 59 :2908–2922. doi: 10.1016/j.neuroimage.2011.09.066. [ PMC free article ] [ PubMed ] [ CrossRef ] [ Google Scholar ]
- Addis DR, Musicaro R, Pan L, et al. Episodic simulation of past and future events in older adults: Evidence from an experimental recombination task. Psychology and Aging. 2010; 25 :369–376. doi: 10.1037/a0017280. [ PMC free article ] [ PubMed ] [ CrossRef ] [ Google Scholar ]
- Addis DR, Pan L, Musicaro R, et al. Divergent thinking and constructing episodic simulations. Memory. 2016; 24 :89–97. doi: 10.1080/09658211.2014.985591. [ PMC free article ] [ PubMed ] [ CrossRef ] [ Google Scholar ]
- Addis DR, Sacchetti DC, Ally BA, et al. Episodic simulation of future events is impaired in mild Alzheimer’s disease. Neuropsychologia. 2009; 47 :2660–2671. doi: 10.1016/j.neuropsychologia.2009.05.018. [ PMC free article ] [ PubMed ] [ CrossRef ] [ Google Scholar ]
- Addis DR, Wong AT, Schacter DL. Age-related changes in the episodic simulation of future events. Psychological Science. 2008; 19 :33–41. doi: 10.1111/j.1467-9280.2008.02043.x. [ PubMed ] [ CrossRef ] [ Google Scholar ]
- Beaman A, Pushkar P, Etezadi S, et al. Autobiographical memory specificity predicts social problem solving ability in old and young adults. The Quarterly Journal of Experimental Psychology. 2007; 60 :1275–1288. doi: 10.1080/17470210600943450. [ PubMed ] [ CrossRef ] [ Google Scholar ]
- Benedek M, Jauk E, Fink A, et al. To create or to recall? Neural mechanisms underlying the generation of creative new ideas. NeuroImage. 2014; 88 :125–133. doi: 10.1016/j.neuroimage.2013.11.021. [ PMC free article ] [ PubMed ] [ CrossRef ] [ Google Scholar ]
- Benoit RG, Schacter DL. Specifying the core network supporting episodic simulation and episodic memory by activation likelihood estimation. Neuropsychologia. 2015; 75 :450–457. doi: 10.1016/j.neuropsychologia.2015.06.034. [ PMC free article ] [ PubMed ] [ CrossRef ] [ Google Scholar ]
- Brown AD, Addis DR, Romano TA, et al. Episodic and semantic components of autobiographical memories and imagined future events in posttraumatic stress disorder. Memory. 2014; 22 :595–604. doi: 10.1080/09658211.2013.807842. [ PubMed ] [ CrossRef ] [ Google Scholar ]
- Buckner RL, Carroll DC. Self-projection and the brain. Trends in Cognitive Sciences. 2007; 11 :49–57. doi: 10.1016/j.tics.2006.11.004. [ PubMed ] [ CrossRef ] [ Google Scholar ]
- Cole SN, Morrison CM, Conway MA. Episodic future thinking: Linking neuropsychological performance with episodic detail in young and old adults. The Quarterly Journal of Experimental Psychology. 2013; 66 :1687–1706. doi: 10.1080/17470218.2012.758157. [ PubMed ] [ CrossRef ] [ Google Scholar ]
- Conway MA, Loveday C, Cole SN. The remembering-imagining system. Memory Studies. 2016; 9 :256–265. [ Google Scholar ]
- Conway MA, Pleydell-Pearce CW. The construction of autobiographical memories in the self-memory system. Psychological Review. 2000; 107 :261–288. doi: 10.1037//0033-295X.107.2.261. [ PubMed ] [ CrossRef ] [ Google Scholar ]
- D’Argembeau A, Raffard S, Van der Linden M. Remembering the past and imagining the future in schizophrenia. Journal of Abnormal Psychology. 2008; 117 :247–251. doi: 10.1037/0021-843X.117.1.247. [ PubMed ] [ CrossRef ] [ Google Scholar ]
- D’Argembeau A, Van der Linden M. Phenomenal characteristics associated with projecting oneself back into the past and forward into the future: Influence of valence and temporal distance. Consciousness and Cognition. 2004; 13 :844–858. doi: 10.1016/j.concog.2004.07.007. [ PubMed ] [ CrossRef ] [ Google Scholar ]
- Devitt AL, Monk-Fromont E, Schacter DL, et al. Factors that influence the generation of autobiographical memory conjunction errors. Memory. 2016; 24 :204–222. doi: 10.1080/09658211.2014.998680. [ PMC free article ] [ PubMed ] [ CrossRef ] [ Google Scholar ]
- Dudai Y, Carruthers M. The Janus face of Mnemosyne. Nature. 2005; 434 :567. doi: 10.1038/434567a. [ PubMed ] [ CrossRef ] [ Google Scholar ]
- Dudai Y, Edelson M. Personal memory: Is it personal, is it memory? Memory Studies. 2016; 9 :275–283. [ Google Scholar ]
- Duff MC, Kurzcek J, Rubin R, et al. Hippocampal amnesia disrupts creative thinking. Hippocampus. 2013; 23 :1143–1149. doi: 10.1002/hipo.22208. [ PMC free article ] [ PubMed ] [ CrossRef ] [ Google Scholar ]
- Fisher RP, Geiselman RE. Memory-enhancing techniques for investigative interviewing: The cognitive interview. Springfield, IL: Charles C. Thomas Books; 1992. [ Google Scholar ]
- Fivush R, Merrill N. An ecological systems approach to family narratives. Memory Studies. 2016; 9 :305–314. [ Google Scholar ]
- Gaesser B, Sacchetti DC, Addis DR, et al. Characterizing age-related changes in remembering the past and imagining the future. Psychology and Aging. 2011; 26 :80–84. doi: 10.1037/a0021054. [ PMC free article ] [ PubMed ] [ CrossRef ] [ Google Scholar ]
- Gaesser B, Schacter DL. Episodic simulation and episodic memory can increase intentions to help others. Proceedings of the National Academy of Sciences USA. 2014; 111 :4415–4420. doi: 10.1073/pnas.1402461111. [ PMC free article ] [ PubMed ] [ CrossRef ] [ Google Scholar ]
- Guilford JP. The Nature of Human Intelligence. New York: McGraw Hill; 1967. [ Google Scholar ]
- Hassabis D, Kumaran D, Vann DS, et al. Patients with hippocampal amnesia cannot imagine new experiences. Proceedings of the National Academy of Sciences USA. 2007; 1014 :1726–1731. doi: 10.1073/pnas.0610561104. [ PMC free article ] [ PubMed ] [ CrossRef ] [ Google Scholar ]
- Hassabis D, Maguire EA. Deconstructing episodic memory with construction. Trends in Cognitive Sciences. 2007; 11 :299–306. doi: 10.1016/j.tics.2007.05.001. [ PubMed ] [ CrossRef ] [ Google Scholar ]
- Irish M, Addis DR, Hodges JR, et al. Considering the role of semantic memory in episodic future thinking: Evidence from semantic dementia. Brain. 2012; 135 :2178–2191. doi: 10.1093/brain/aws119. [ PubMed ] [ CrossRef ] [ Google Scholar ]
- Juskenaite A, Quinette P, Desgranges B, et al. Mental simulations of future scenarios in transient global amnesia. Neuropsychologia. 2014; 63 :1–9. doi: 10.1016/j.neuropsychologia.2014.08.002. [ PubMed ] [ CrossRef ] [ Google Scholar ]
- Klein SB. The complex act of projecting oneself into the future. Wiley Interdisciplinary Reviews - Cognitive Science. 2013; 4 :63–79. doi: 10.1002/wcs.1210. [ PubMed ] [ CrossRef ] [ Google Scholar ]
- Klein SB, Loftus J, Kihlstrom JF. Memory and temporal experience: The effects of episodic memory loss on an amnesic patient’s ability to remember the past and imagine the future. Social Cognition. 2002; 20 :353–379. doi: 10.1521/soco.20.5.353.21125. [ CrossRef ] [ Google Scholar ]
- Labouvie-Vief G, Blanchard-Fields F. Cognitive ageing and psychological growth. Ageing and Society. 1982; 2 :183–209. doi: 10.1017/S0144686X00009429. [ CrossRef ] [ Google Scholar ]
- Levine B, Svoboda E, Hay JF, et al. Aging and autobiographical memory: Dissociating episodic from semantic retrieval. Psychology and Aging. 2002; 17 :677–689. doi: 10.1037/0882-7974.17.4.677. [ PubMed ] [ CrossRef ] [ Google Scholar ]
- MacLeod AK. Prospection, well-being and memory. Memory Studies. 2016; 9 :266–274. [ Google Scholar ]
- MacLeod AK, Conway C. Well-being and the anticipation of future positive experiences: The role of income, social networks, and planning ability. Cognition and Emotion. 2005; 19 :357–374. doi: 10.1080/02699930441000247. [ PubMed ] [ CrossRef ] [ Google Scholar ]
- Madore KP, Addis DR, Schacter DL. Creativity and memory: Effects of an episodic specificity induction on divergent thinking. Psychological Science. 2015 doi: 10.1177/0956797615591863. [ PMC free article ] [ PubMed ] [ CrossRef ] [ Google Scholar ]
- Madore KP, Gaesser B, Schacter DL. Constructive episodic simulation: Dissociable effects of a specificity induction on remembering, imagining, and describing in young and older adults. Journal of Experimental Psychology: Learning, Memory, and Cognition. 2014; 40 :609–622. doi: 10.1037/a0034885. [ PMC free article ] [ PubMed ] [ CrossRef ] [ Google Scholar ]
- Madore KP, Schacter DL. An episodic specificity induction enhances means-end problem solving in young and older adults. Psychology and Aging. 2014; 29 :913–924. doi: 10.1037/a0038209. [ PMC free article ] [ PubMed ] [ CrossRef ] [ Google Scholar ]
- Madore KP, Schacter DL. Remembering the past and imagining the future: Selective effects of an episodic specificity induction on detail generation. The Quarterly Journal of Experimental Psychology. 2016; 69 :285–298. doi: 10.1080/17470218.2014.999097. [ PMC free article ] [ PubMed ] [ CrossRef ] [ Google Scholar ]
- Maestas KL, Rude SS. The benefits of expressive writing on autobiographical memory specificity: A randomized controlled trial. Cognitive Therapy and Research. 2012; 36 :234–246. doi: 10.1007/s10608-011-9358-y. [ CrossRef ] [ Google Scholar ]
- Memon A, Meissner CA, Fraser J. The cognitive interview: A meta-analytic review and study space analysis of the past 25 years. Psychology, Public Policy, and Law. 2010; 16 :340–372. doi: 10.1037/a0020518. [ CrossRef ] [ Google Scholar ]
- Merck C, Topcu MN, Hirst W. Collective mental time travel: Creating a shared future through our shared past. Memory Studies. 2016; 9 :284–294. [ Google Scholar ]
- Neshat-Doost HT, Dalgleish T, Yule W, et al. Enhancing autobiographical memory specificity through cognitive training: An intervention for depression translated from basic science. Clinical Psychological Science. 2012; 1 :84–92. doi: 10.1177/2167702612454613. [ CrossRef ] [ Google Scholar ]
- Platt J, Spivack G. Manual for the means-end problem solving test (MEPS): A measure of interpersonal problem solving skill. Philadelphia: Hahnemann Medical College and Hospital; 1975. [ Google Scholar ]
- Race E, Keane MM, Verfaellie M. Medial temporal lobe damage causes deficits in episodic memory and episodic future thinking not attributable to deficits in narrative construction. Journal of Neuroscience. 2011; 31 :10262–10269. doi: 10.1523/JNEUROSCI.1145-11.2011. [ PMC free article ] [ PubMed ] [ CrossRef ] [ Google Scholar ]
- Raes F, Hermans D, Williams JMG, et al. Reduced specificity of autobiographical memory: A mediator between rumination and ineffective social problem-solving in major depression? Journal of Affective Disorders. 2005; 87 :331–335. doi: 10.1016/j.jad.2005.05.004. [ PubMed ] [ CrossRef ] [ Google Scholar ]
- Raes F, Williams JMG, Hermans D. Reducing cognitive vulnerability to depression: A preliminary investigation of MEmory Specificity Training (MEST) in inpatients with depressive symptomatology. Journal of Behavior Therapy and Experimental Psychiatry. 2009; 40 :24–38. doi: 10.1016/j.jbtep.2008.03.001. [ PubMed ] [ CrossRef ] [ Google Scholar ]
- Rathbone CJ, Conway MA, Moulin CJA. Remembering and imagining: The role of the self. Consciousness and Cognition. 2011; 20 :1175–1182. doi: 10.1016/j.concog.2011.02.013. [ PubMed ] [ CrossRef ] [ Google Scholar ]
- Rendell PG, Bailey PE, Henry JD, et al. Older adults have greater difficulty imagining future rather than atemporal experiences. Psychology and Aging. 2012; 27 :1089–1098. doi: 10.1037/a0029748. [ PubMed ] [ CrossRef ] [ Google Scholar ]
- Romero K, Moscovitch M. Episodic memory and event construction in aging and amnesia. Journal of Memory and Language. 2012; 67 :270–284. doi: 10.1016/j.jml.2012.05.002. [ CrossRef ] [ Google Scholar ]
- Rosenbaum RS, Kohler S, Schacter DL, et al. The case of K.C.: Contributions of a memory-impaired person to memory theory. Neuropsychologia. 2005; 43 :98–1021. doi: 10.1016/j.neuropsychologia.2004.10.007. [ PubMed ] [ CrossRef ] [ Google Scholar ]
- Schacter DL. Adaptive constructive processes and the future of memory. American Psychologist. 2012; 67 :603–613. doi: 10.1037/a0029869. [ PMC free article ] [ PubMed ] [ CrossRef ] [ Google Scholar ]
- Schacter DL, Addis DR. The cognitive neuroscience of constructive memory: Remembering the past and imagining the future. Philosophical Transactions of the Royal Society of London (B) 2007; 362 :773–786. doi: 10.1098/rstb.2007.2087. [ PMC free article ] [ PubMed ] [ CrossRef ] [ Google Scholar ]
- Schacter DL, Addis DR, Buckner RL. Remembering the past to imagine the future: The prospective brain. Nature Reviews Neuroscience. 2007; 8 :657–661. doi: 10.1038/nrn2213. [ PubMed ] [ CrossRef ] [ Google Scholar ]
- Schacter DL, Addis DR, Hassabis D, et al. The future of memory: Remembering, imagining, and the brain. Neuron. 2012; 76 :677–694. doi: 10.1016/j.neuron.2012.11.001. [ PMC free article ] [ PubMed ] [ CrossRef ] [ Google Scholar ]
- Schacter DL, Gaesser B, Addis DR. Remembering the past and imagining the future in the elderly. Gerontology. 2013; 59 :143–151. doi: 10.1159/000342198. [ PMC free article ] [ PubMed ] [ CrossRef ] [ Google Scholar ]
- Schacter DL, Guerin SA, St Jacques PL. Memory distortion: An adaptive perspective. Trends in Cognitive Sciences. 2011; 15 :467–474. doi: 10.1016/j.tics.2011.08.004. [ PMC free article ] [ PubMed ] [ CrossRef ] [ Google Scholar ]
- Schacter DL, Tulving E. Memory Systems 1994. Cambridge, MA: MIT Press; 1994. [ Google Scholar ]
- Sheldon S, McAndrews MP, Moscovitch M. Episodic memory processes mediated by the medial temporal lobes contribute to open-ended problem solving. Neuropsychologia. 2011; 49 :2439–2447. doi: 10.1016/j.neuropsychologia.2011.04.021. [ PubMed ] [ CrossRef ] [ Google Scholar ]
- Spreng RN, Mar RA, Kim AS. The common neural basis of autobiographical memory, prospection, navigation, theory of mind, and the default mode: A quantitative meta-analysis. Journal of Cognitive Neuroscience. 2009; 21 :489–510. doi: 10.1162/jocn.2008.21029. [ PubMed ] [ CrossRef ] [ Google Scholar ]
- Squire LR, van der Horst AS, McDuff SGR, et al. Role of the hippocampus in remembering the past and imagining the future. Proceedings of the National Academy of Sciences USA. 2010; 107 :19044–19048. doi: 10.1073/pnas.1014391107. [ PMC free article ] [ PubMed ] [ CrossRef ] [ Google Scholar ]
- Suddendorf T, Busby J. Mental time travel in animals? Trends in Cognitive Sciences. 2003; 7 :391–396. doi: 10.1016/S1364-6613(03)00187-6. [ PubMed ] [ CrossRef ] [ Google Scholar ]
- Szpunar KK. Episodic future thought: An emerging concept. Perspectives on Psychological Science. 2010; 5 :142–162. doi: 10.1177/1745691610362350. [ PubMed ] [ CrossRef ] [ Google Scholar ]
- Szpunar KK, McDermott KB. Episodic future thought and its relation to remembering: Evidence from ratings of subjective experience. Consciousness and Cognition. 2008; 17 :330–334. doi: 10.1016/j.concog.2007.04.006. [ PubMed ] [ CrossRef ] [ Google Scholar ]
- Szpunar KK, Spreng RN, Schacter DL. A taxonomy of prospection: Introducing an organizational framework for future-oriented cognition. Proceedings of the National Academy of Sciences USA. 2014; 111 :18414–18421. doi: 10.1073/pnas.1417144111. [ PMC free article ] [ PubMed ] [ CrossRef ] [ Google Scholar ]
- Tulving E. Memory and consciousness. Canadian Psychologist. 1985; 26 :1–12. doi: 10.1037/h0080017. [ CrossRef ] [ Google Scholar ]
- Tulving E. Episodic memory: From mind to brain. Annual Review of Psychology. 2002; 53 :1–25. doi: 10.1146/annurev.psych.53.100901.135114. [ PubMed ] [ CrossRef ] [ Google Scholar ]
- Wang Q. Remembering the self in cultural contexts: A cultural dynamic theory of autobiographical memory. Memory Studies. 2016; 9 :295–304. [ Google Scholar ]
- Williams JMG, Barnhofer T, Crane C, et al. Autobiographical memory specificity and emotional disorder. Psychological Bulletin. 2007; 133 :122–148. doi: 10.1037/0033-2909.133.1.122. [ PMC free article ] [ PubMed ] [ CrossRef ] [ Google Scholar ]
- Williams JMG, Ellis NC, Tyers C, et al. The specificity of autobiographical memory and imageability of the future. Memory & Cognition. 1996; 24 :116–125. doi: 10.3758/BF03197278. [ PubMed ] [ CrossRef ] [ Google Scholar ]
Means-Ends Analysis
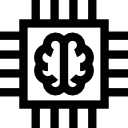
Means-Ends analysis is a method of solving problems. This method is useful for well-formed problems, less so for less-formed problems.
State Space [ edit ]
Problem solving occurs in a state space . Imagine first an initial state and then a goal state . We want to get from the initial state to the goal state . There might be many different paths from the initial state to the goal state .
We can talk about how to solve this problem in terms of differences between different states and the goal (end) state . I want to deduce the difference between two different states.
So I can look at my current problem, and then my end- state . I will then ask "how many differences are there between my current state and my end state ".
Imagine we start at step one, our initial state . The next step in this process is to create every possible permutation from my initial state . The next step is to calculate the difference in the states I just made and my end state .
There is an obvious method here: if I generate 6 different possible states from my initial state , I can then calculate the difference between each of those states and the end state . I would look for the option that had the least number of differences to produce the most optimal solution.
Universal method of problem solving [ edit ]
A means-ends analysis is considered a universal method of solving problems. However, there is no guarantee of success.
References [ edit ]
- ↑ http://www.flaticon.com/
Give a specific name, value or other brief answer without explanation or calculation.
Reach a conclusion from the information given.
Obtain a numerical answer showing the relevant stages in the working.
A unit of abstract mathematical system subject to the laws of arithmetic.
Means-Ends Planning: An Example Soar System
Cite this chapter.
- Aladin Akyürek 3
Part of the book series: Studies in Cognitive Systems ((COGS,volume 10))
161 Accesses
3 Citations
Although Soar is intended to cover the full range of weak problem-solving methods-often hypothesized in cognitive science as basic for all intelligent agents, earlier attempts to add means-ends analysis to Soar’s repertoire of methods have not been particularly successful or convincing. Considering its psychological significance, stipulated by Newell and Simon (1972), it seems essential that Soar, when taken as a general cognitive architecture, should allow the means-ends analysis to arise naturally from its own structure. This paper presents a planner program that interleaves a difference reduction process with Soar’s default mechanism for operator subgoaling that it modifies in order to map meansends analysis onto Soar. The scheme advanced is shown to produce “macro-operators” of a novel kind, called macrochunks , which may have important implications for explaining routine behavior. The approach taken and the problems that had to be dealt with in implementing this planner are treated in detail. Also, SoarL —a language used for state representations- is reviewed with respect to the frame problem.
This is a preview of subscription content, log in via an institution to check access.
Access this chapter
- Available as PDF
- Read on any device
- Instant download
- Own it forever
- Available as EPUB and PDF
- Compact, lightweight edition
- Dispatched in 3 to 5 business days
- Free shipping worldwide - see info
Tax calculation will be finalised at checkout
Purchases are for personal use only
Institutional subscriptions
Unable to display preview. Download preview PDF.
Akyürek, A. (1992). On a computational model of human planning. In J. A. Michon & A. Akyürek (Eds.), Soar: A cognitive architecture in perspective (pp. 81–108). Dordrecht, The Netherlands: Kluwer.
Chapter Google Scholar
Anderson, J. R. (1986). Knowledge compilation: The general learning mechanism. In R. S. Michalski. J. G. Carbonell. & T. M. Mitchell (Eds.). Machine learning: An artificial intelligence approach (Vol. II. pp. 289–310). Los Altos. CA: Morgan Kaufma
Google Scholar
Atwood, M. E. & Polson. P. G. (1976). A process model for water jug problems. Cognitive Psychology , 8 ,191–216.
Article Google Scholar
Carbonell, J. G. (1983). Learning by analogy: Formulating and generalizing plans from past experience, In R. S. Michalski. J. G. Carbonell, & T. M. Mitchell (Eds.). Machine learning: An artificial intelligence approach (pp. 137–161). Los Altos. CA: Morgan Kaufmann.
Dawson, C., & Siklóssy, L. (1977). The role of preprocessing in problem solving systems. In Proceedings of the Fifth International Joint Conference on Artificial Intelligence (pp. 465–471). San Mateo. CA: Morgan Kaufmann.
DeJong, G., & Mooney. R. (1986). Explanation-based learning: An alternative view. Machine Learning , 1 , 145–176.
Ernst, G. W. (1969). Sufficient conditions for the success of GPS. Journal of the Association for Computing Machinery , 16 , 517–533.
Fikes, R. E., Hart, P.E., & Nilsson, N. J. (1972). Learning and executing generalized robot plans. Artificial Intelligence , 3 , 251–288.
Fikes, R. E., & Nilsson, N. J. (1971). STRIPS: A new approach to the application of theorem proving to problem solving. Artificial Intelligence , 2 , 189–208.
Hayes. P. J. (1973). The frame problem and related problems in artificial intelligence. In A. Elithorn & D. Jones (Eds.). Artificial and human thinking (pp. 45–59). Amsterdam: Elsevier.
Jeffries, R., Polson, P. G., & Razran, L. (1977). A process model for missionaries-cannibals and other river-crossing problems. Cognitive Psychology , 9 ,412–440.
Korf, R. E. (1985). Learning to solve problems by searching for macro-operators . Boston, MA: Pitman.
Knoblock, C. A. (1989). Learning hierarchies of abstraction spaces. In Proceedings of the Sixth International Workshop on Machine Learning (pp. 241–245). San Mateo. CA: Morgan Kaufmann.
Laird, J. E. (1984). Universal subgoaling (Tech. Rep. CMU-CS-84-129). Pittsburgh. PA: Carnegie Mellon University, Department of Computer Science. [Also available as part of Laird, I., Rosenbloom, P., & Newell, A. (1986). Universal subgoaling and chunking: The automatic generation and learning of goal hierarchies (pp. 1–131). Boston. MA: Kluwer.)
Laird, J. E., Congdon, C. B., Altmann, E., & Swedlow, K. (1990). Soar user’s manual: Version 5.2 (Tech. Rep. CMU-CS-90-179). Pittsburgh, PA: Carnegie Mellon University, School of Computer Science.
Laird, J., & Newell, A. (1983). A universal weak method ([ech. Rep. CMU-CS-83-141). Pittsburgh, PA: Carnegie Mellon University, Department of Computer Science.
Laird, J. E., & Rosenbloom, P. S. (1990). Integrating execution, planning, and learning in Soar for external environments. In Proceedings of the Eighth National Conference on Artificial Intelligence (pp. 1022–1029). San Mateo, CA: Morgan Kaufmann.
Laird, J. E., Rosenbloom, P. S., & Newell, A. (1984). Towards chunking as a general learning mechanism. In Proceedings of the Fourth National Conference on Artificial Intelligence (pp. 188–192). San Mateo, CA: Morgan Kaufmann.
Laird, J. E., Rosenbloom, P. S., & Newell, A. (1986). Chunking in Soar: The anatomy of a general learning mechanism. Machine Learning , 1 , 11–46.
Laird, J., Swedlow, K., Altmann, E., Congdon, C. B., & Wiesmeyer, M. (1989). Soar user’s manual: Version 4.5 . Pittsburgh, PA: Carnegie Mellon University, School of Computer Science.
McDermott, D. (1987). AI, logic, and the frame problem. In F. M. Brown (Ed.), The frame problem in artificial intelligence (pp. 105–118). Los Altos, CA: Morgan Kaufmann.
McDermott, D. (1978). Planning and acting. Cognitive Science , 2 , 71–109).
Minton, S. (1988). Learning search control knowledge: An explanation-based approach . Boston, MA: Kluwer.
Book Google Scholar
Minton, S., Knoblock, C. A., Kuokka, D. R., Gil, Y., Joseph, R. L., & Carbonell, J. G. (1989). PRODIGY 2.0: The manual and tutorial (Tech. Rep. CMU-CS-89-146). Pittsburgh, PA: Carnegie-Mellon University, School of Computer Science.
Mitchell, T. M., Keller, R. M., & Kedar-Cabelli, S. T. (1986). Explanation-based generalization: A unifying view. Machine Learning , 1 ,47–80.
Newell, A. (1980). Reasoning, problem solving, and decision processes: The problem space as a fundamental category. In R. S. Nickerson (Ed.), Attention and performance (Vol. 8, pp. 693–718). Hillsdale, NJ: Erlbaum.
Newell, A. (1990). Unified theories of cognition . Cambridge, MA: Harvard University Press.
Newell, A. (1992). Unified theories of cognition and the role of Soar. In J. A. Michon & A. Akyürek (Eds.), Soar: A cognitive architecture in perspective (pp. 25–79). Dordrecht, The Netherlands: Kluwer.
Newell, A., Rosenbloom, P. S., & Laird, J. E. (1989). Symbolic architectures for cognition. In M. I. Posner (Ed.), Foundations of cognitive science (pp. 93–131). Cambridge, MA: MIT Press.
Newell, A., Shaw, J. C., & Simon, H. A. (1962). The processes of creative thinking. In H. E. Gruber, G. Terrell, & M. Wertheimer (Eds.), Contemporary approaches to creative thinking (pp. 63–119). New York: Atherton Press.
Newell, A., & Simon, H. A. (1963). GPS, a program that simulates human thought. In E. A. Feigenbaum & J. Feldman (Eds.), Computers and thought (pp. 279–293). New York: McGraw-Hill. (Original work published 1961)
Newell, A., & Simon, H. A. (1972). Human problem solving . Englewood Cliffs. NJ: PrenticeHall.
Newell, A., Yost, G., Laird, J. E., Rosenbloom, P. S., & Altmann, E. (1990). Formulating the problem space computational model . Paper presented at the 25th Anniversary Symposium. School of Computer Science, Carnegie Mellon University, Pittsburgh, PA.
Nilsson, N. J.(1980). Principles of artificial intelligence . Los Altos, CA: Morgan Kaufmann.
Russell, S. J. (1989). Execution architectures and compilation. In Proceedings of the Eleventh International Joint Conference on Artificial Intelligence (pp. 15–20). San Mateo, CA: Morgan Kaufmann.
Sacerdoti, E. D. (1974). Planning in a hierarchy of abstraction spaces. Artificial Intelligence , 5 ,115–135.
Sandewall, E. J. (1969). A planning problem solver based on look-ahead in stochastic game trees. Journal of the Association for Computing Machinery , 16 , 364–382.
Schubert, L. (1990). Monotonic solution of the frame problem in the situation calculus: An efficient method for worlds with fully specified actions. In H. E. Kyburg, R. P. Loui, & G. N. Carlson (Eds.), Knowledge representation and defeasible reasoning (pp. 23–67). Dordrecht, The Netherlands: Kluwer.
Sussman, G. J. (1975). A computer model of skill acquisition . New York: American Elsevier.
Tambe, M., Newell, A., & Rosenbloom, P. S. (1990). The problem of expensive chunks and its solution by restricting expressiveness. Machine Learning , 5 ,299–348.
Tenenberg, J. D. (1988). Abstraction in planning (Tech. Rep. No. 250). Rochester, NY: University of Rochester. Computer Science Department.
Unruh, A., & Rosenbloom, P. S. (1989). Abstraction in problem solving and learning. In Proceedings of the Eleventh International Joint Conference on Artificial Intelligence (pp. 681–687). San Mateo. CA: Morgan Kaufmann.
Unruh, A., & Rosenbloom, P. S. (1990). Two new weak method increments for abstraction . Manuscript submitted for publication.
Wilkins, D. E. (1988). Practical planning: Extending the classical AI planning paradigm . San Mateo,CA: Morgan Kaufmann.
Woods, W. A. (1975). What’s in a link: Foundations for semantic networks. In D. G. Bobrow & A. Collins (Eds.). Representation and understanding: Studies in cognitive science (pp. 35–82). Orlando, FL: Academic Press.
Download references
Author information
Authors and affiliations.
Department of Psychology, University of Groningen, Groningen, The Netherlands
Aladin Akyürek
You can also search for this author in PubMed Google Scholar
Editor information
Editors and affiliations.
John A. Michon & Aladin Akyürek &
Rights and permissions
Reprints and permissions
Copyright information
© 1992 Springer Science+Business Media Dordrecht
About this chapter
Akyürek, A. (1992). Means-Ends Planning: An Example Soar System. In: Michon, J.A., Akyürek, A. (eds) Soar: A Cognitive Architecture in Perspective. Studies in Cognitive Systems, vol 10. Springer, Dordrecht. https://doi.org/10.1007/978-94-011-2426-3_5
Download citation
DOI : https://doi.org/10.1007/978-94-011-2426-3_5
Publisher Name : Springer, Dordrecht
Print ISBN : 978-94-010-5070-8
Online ISBN : 978-94-011-2426-3
eBook Packages : Springer Book Archive
Share this chapter
Anyone you share the following link with will be able to read this content:
Sorry, a shareable link is not currently available for this article.
Provided by the Springer Nature SharedIt content-sharing initiative
- Publish with us
Policies and ethics
- Find a journal
- Track your research
What is means-ends analysis?
Get Started With Data Science
Learn the fundamentals of Data Science with this free course. Future-proof your career by adding Data Science skills to your toolkit — or prepare to land a job in AI, Machine Learning, or Data Analysis.
In today's fast-paced and complex world, problem-solving skills are crucial for success in various domains. Means-ends analysis (MEA) is an effective problem-solving approach that involves dividing a problem into smaller goals and identifying the means that stand in the way of the current state and the desired goal.
The means-end analysis utilizes the following steps to achieve goals.
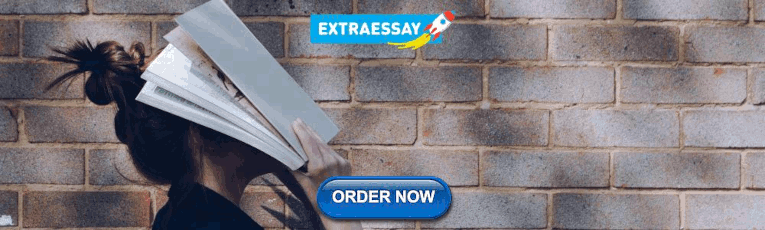
IMAGES
VIDEO
COMMENTS
Means End Analysis (MEA) is a problem-solving technique that has been used since the fifties of the last century to stimulate creativity. Means End Analysis is also a way of looking at the organisational planning, and helps in achieving the end-goals. With Means End Analysis, it is possible to control the entire process of problem solving.
Means-End Analysis is essentially an early form of Gap Analysis. It was created by researchers Allen Newell and Herbert Simon in the late 1950s, and it was then published their 1972 book, "Human Problem Solving." ... Means-End Analysis is a simple problem-solving tool that you can use to solve well-defined problems, and to kick-start the ...
6.3: Means -Ends Analysis. In Means-End Analysis you try to reduce the difference between initial state and goal state by creating sub goals until a sub goal can be reached directly (probably you know several examples of recursion which works on the basis of this). An example for a problem that can be solved by Means-End Analysis are the ...
Means end analysis problem solving drives creative solutions. Learn to apply means end analysis problem solving to tackle even the most complex scenarios. ... For our means end analysis example, let's begin with slide #27 in the deck. This one is arranged as a process map. Notice the series of linear steps connected with an arrow.
Means-ends analysis, heuristic, or trial-and-error, problem-solving strategy in which an end goal is identified and then fulfilled via the generation of subgoals and action plans that help overcome obstacles encountered along the way. Solving a problem with means-ends analysis typically begins by.
In insight problem-solving, the cognitive processes that help you solve a problem happen outside your conscious awareness. 4. Working backward. Working backward is a problem-solving approach often ...
Means-End Analysis is a problem-solving technique that identifies the current state, defines the end goal, and determines the modular action plan to reach the end state. Split the End Goals into sub-goals and sub-sub goals, and then draw action plans to achieve sub-goals first and then progress towards achieving the main goal. ...
Means-ends analysis is a problem solving strategy that arose from the work on problem solving of Newell and Simon (1972). In means-ends analysis, one solves a problem by considering the obstacles that stand between the initial problem state and the goal state. The elimination of these obstacles (and, recursively, the obstacles in the way of ...
Means-end problem solving may also require cognitive abilities to distinguish between the means and end object, ... Moreover, infants' daily experiences may impact their ability to succeed in the two means-end tasks. For example, current results suggested that at the age of 6 months, pulling the towel occurred 30% of the observation time ...
Means-ends analysis. Means-ends analysis [1] ( MEA) is a problem solving technique used commonly in artificial intelligence (AI) for limiting search in AI programs. It is also a technique used at least since the 1950s as a creativity tool, most frequently mentioned in engineering books on design methods. MEA is also related to means-ends ...
However, despite the recent impressive findings involving apparent understanding of means-end relations found in various animals, the pivotal question remains: to what degree do states of cognition characterized by terms like "means-end reasoning", "insight", "understanding", and "problem solving" contribute to an animal's behaviors as opposed to "simpler" mechanisms ...
Using a means-end analysis is basically looking at a goal, starting point, and the best way to get from point A to point B by breaking down the list or problems to make them more doable in our lives (Newell & Simon, 1972). For this blog, I will pass on some tips on how to manage your homework load that you have each semester to lessen the stress.
Problem-solving skills are essential in our daily lives. The video explains different problem-solving methods, including trial and error, algorithm strategy, and heuristics. It also discusses concepts like means-end analysis, working backwards, fixation, and insight. These techniques help us tackle both well-defined and ill-defined problems ...
Working backwards is a useful heuristic in which you begin solving the problem by focusing on the end result. Consider this example: You live in Washington, D.C. and have been invited to a wedding at 4 PM on Saturday in Philadelphia. ... One example of means-end analysis can be found by using the Tower of Hanoi paradigm.
Means-End Approach. Means-End Approaches. •Goal: In means-ends analysis, the problem solver begins by envisioning the end, or ultimate goal, and then determines the best strategy for attaining the goal in his current situation. The goal should be realistic and attainable. Realistic means that the individual can produce the result and ...
INTRODUCTION. Means-end problem-solving (MEPS) involves the execution of an intentional sequence of actions performed on a "means" object to achieve a goal related to an "end" object. 1-3 Means-end tasks range in complexity from early developmental tasks like pulling a towel to retrieve a distant object through using tools (ie, using a spoon to eat food, a rake to gather toys, or a ...
One such task is Means-End Problem Solving (MEPS; Platt and Spivack, 1975), where people are presented with hypothetical social problems, such as difficulties with friends or handling a situation at work, and are asked to generate steps or means that lead to problem solutions. For example, a step that is relevant to solving a problem involving ...
Means-Ends analysis is a method of solving problems. This method is useful for well-formed problems, less so for less-formed problems. State Space []. Problem solving occurs in a state space.Imagine first an initial state and then a goal state.We want to get from the initial state to the goal state.There might be many different paths from the initial state to the goal state.
in artificial intelligence, a technique to solve problems that sets up subgoals as means to achieve the goals (ends) and compares subgoals and goals using a recursive goal-reduction search procedure.See General Problem Solver.; more generally, any problem-solving strategy that assesses the difference between the current state and a desired end state and attempts to discover means to reduce ...
Abstract. Although Soar is intended to cover the full range of weak problem-solving methods-often hypothesized in cognitive science as basic for all intelligent agents, earlier attempts to add means-ends analysis to Soar's repertoire of methods have not been particularly successful or convincing. Considering its psychological significance ...
Associations between MEPSAT outcomes (means-end learning and level of means-end performance) and Bayley-III. Reliability Quadratic Weighted Dependent Kappas for Means-End Learning Sample Inter-Rater Reliability Intra-Rater Reliability Typical .869 (.785, .952) .936 (.854, >.999) Motor delay .979 (.965, .993) .857 (.730, .985) Intraclass ...
By applying means−end analysis, we can establish a writing schedule, set daily word count targets, seek feedback and revisions, and finalize the manuscript. This approach keeps track of the progress to accomplish the goal within the specified time frame. Advantages. Problem-solving: Ensures a systematic approach by providing a structured ...
Means Ends Analysis Search Technique Solved Example Artificial Intelligence by Dr. Mahesh HuddarIn this video, I will discuss how to apply Means Ends Analysi...
The Cause and Effect Diagram is a versatile tool that can be used in various problem-solving and improvement initiatives, including quality management, process improvement, root cause analysis, and project management. It encourages collaboration, brainstorming, and structured analysis, enabling teams to gain deeper insights into complex ...