Please wait...
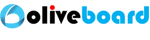
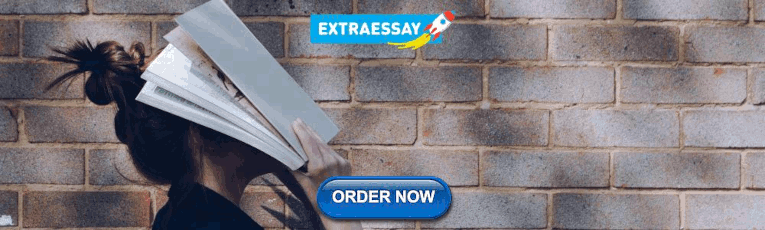
Essay, Precis & Comprehension: English Language Preparation
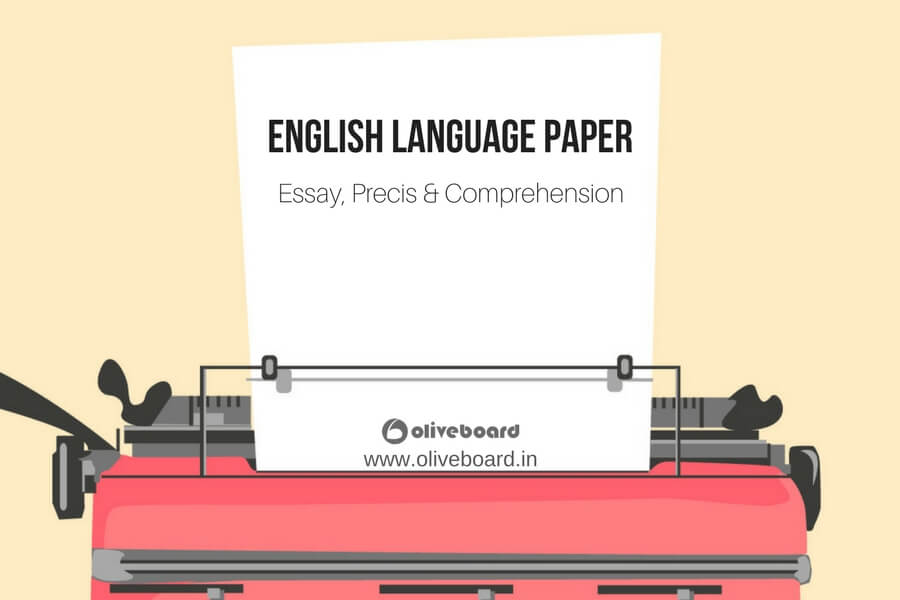
The English language Paper is a scoring section which is a major deciding factor in various Bank & Government examinations. It tests your writing & reading skills, grammar & vocabulary.
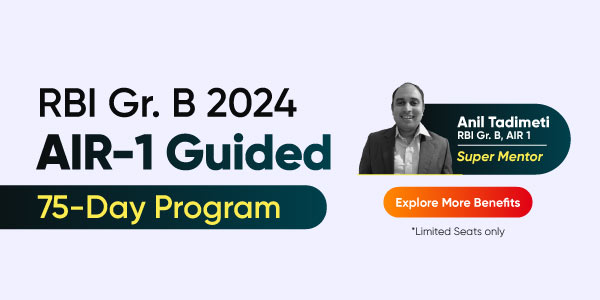
This English Paper comprises of: Essay, Precis and Comprehension in most exams like the RBI Grade B. However, the comprehension part is common among the SBI, IBPS and SSC CGL examinations. In the following article, we have provided a few tips to help you score better in your English paper of Bank & Government exams.
English Comprehension
- Your need to have a good reading speed to master comprehension
Learn how to improve your reading speed: Improve Reading Speed for English Paper
- Understand the context of the passage as sometimes the questions may not be direct
- Read newspapers, and editorials on trending topics. Bank Exam English paper ’s comprehensions may consist passages based on topics like Economy, Banking, Finance, etc.
- Master your vocabulary. Though this comprehension won’t test you on vocabulary, you should be able to understand the meaning of the words through the context
- Read up idioms and phrases and understand their meaning. You can expect questions on idioms and phrases

What should an Essay comprise of?
Essay is a short piece of writing on a particular topic. Essays are error free in terms or grammar and spelling and also follow a structure and have a flow of ideas. Following is a basic ideal essay structure:
Introduction:
Should contain brief introduction of the topic and background. Mention your view before elaborating it in the body of the essay.
Used to present views on the subject in detailed manner. Restrict this to 2 or 3 paragraphs. Detail out examples to support your view. Put forth your strongest argument first followed by second strongest and so on. Each paragraph can contain one idea and sentences supporting it.
Conclusion:
Summarize your main argument using strongest evidences that support it. Don’t introduce a new idea here. Restate your main view, but do not use the same words used in the body.
It’s important that you plan your essay before you type it out. Spend a few minutes on planning and think about what you’re going to write instead of starting immediately. Suppose you have 15 minutes, spend 5 minutes planning, 8 minutes writing and 2 minutes revising/editing.
Precis ( pronounced Preisi ) is a concise summary of a passage. It includes all the essential points, mood and tone of the authors and main idea of the original passage.
- Go through sample precis and practice
- Follow rules for precis writing
- Stick to the word limit provided
Step 1: Read the given passage, highlight and underline the important points and note down the keywords in the same order as in the original passage.
Step 2: Note down the central theme/gist of the passage and tone of the author.
Step 3: Re-read the passage and compare it with the notes you made to check if you missed any crucial information.
Step 4: Provide an appropriate topic to your precis.
Step 5: Draft a precis based on the notes.
For a more detailed guide on how to write a perfect precis, click here: Comprehensive Guide to Write a Perfect Precis for English Paper .
General tips
- Ensure correct usage of grammar, spelling & punctuation
- Stick to the word limit
- Avoid using fancy words. Use simple language
- Solve previous year English language papers
- Read newspaper editorials daily. Topics are mostly based on what has been in news for the past 1 year. Refer to current affairs capsules and pick topics from there, read them and write short essays on them
- Make a note of important facts and figures
- Don’t take the time for granted. In most Bank & Government exam’s descriptive section you will have to type on the keyboard, so those of you who are slow at typing need to practice typing precis and essays on computers. The keyboard will be of old type and hence may be slow. So a faster typing speed will give you an edge over others in the exam
Click to take free sectional English paper mock tests for RBI Grade B Phase 2!
We hope these last minute tips on English language paper of Bank and Government Exams help you prepare better. Download and use this article as a handy guide during your preparation to improve your score in English paper.
We wish you all the very best for your exam.
Further reading:
RBI Grade B Phase 2 Economic & Social Issues Preparation Guide
Rbi grade b phase 2 finance & management preparation guide.

The most comprehensive online preparation portal for MBA, Banking and Government exams. Explore a range of mock tests and study material at www.oliveboard.in
Oliveboard Live Courses & Mock Test Series
- Download 500+ Free Ebooks for Govt. Exams Preparations
- Attempt Free SSC CGL Mock Test 2024
- Attempt Free IBPS Mock Test 2024
- Attempt Free SSC CHSL Mock Test 2024
- Download Oliveboard App
- Follow Us on Google News for Latest Update
- Join Telegram Group for Latest Govt Jobs Update

Leave a comment Cancel reply
Download 500+ Free Ebooks (Limited Offer)👉👉
Thank You 🙌

Essay vs Composition: Difference and Comparison
Some students make a mistake, thinking an essay and composition are synonymous. These terms are not contrary on the one side, and on the other side, there is a significant distinction between them.
Key Takeaways Essay and composition are both forms of academic writing that require critical thinking, analysis, and effective communication; essay is a more specific term that refers to a piece of writing that presents a thesis statement and supports it with evidence and analysis. The composition can encompass various types of writing, including essays, narratives, and descriptive pieces; an essay is a specific type of composition with a more structured format. An essay includes an introduction, body paragraphs, and a conclusion, while composition may not have a specific structure or format.
Essay vs. Composition
Essays are about the writer’s opinion on a particular topic. They are structured and follow patterns, including an introduction, a body paragraph, and a conclusion. The composition can be about any topic, and it is not structured. It is not about any specific opinion or argument.
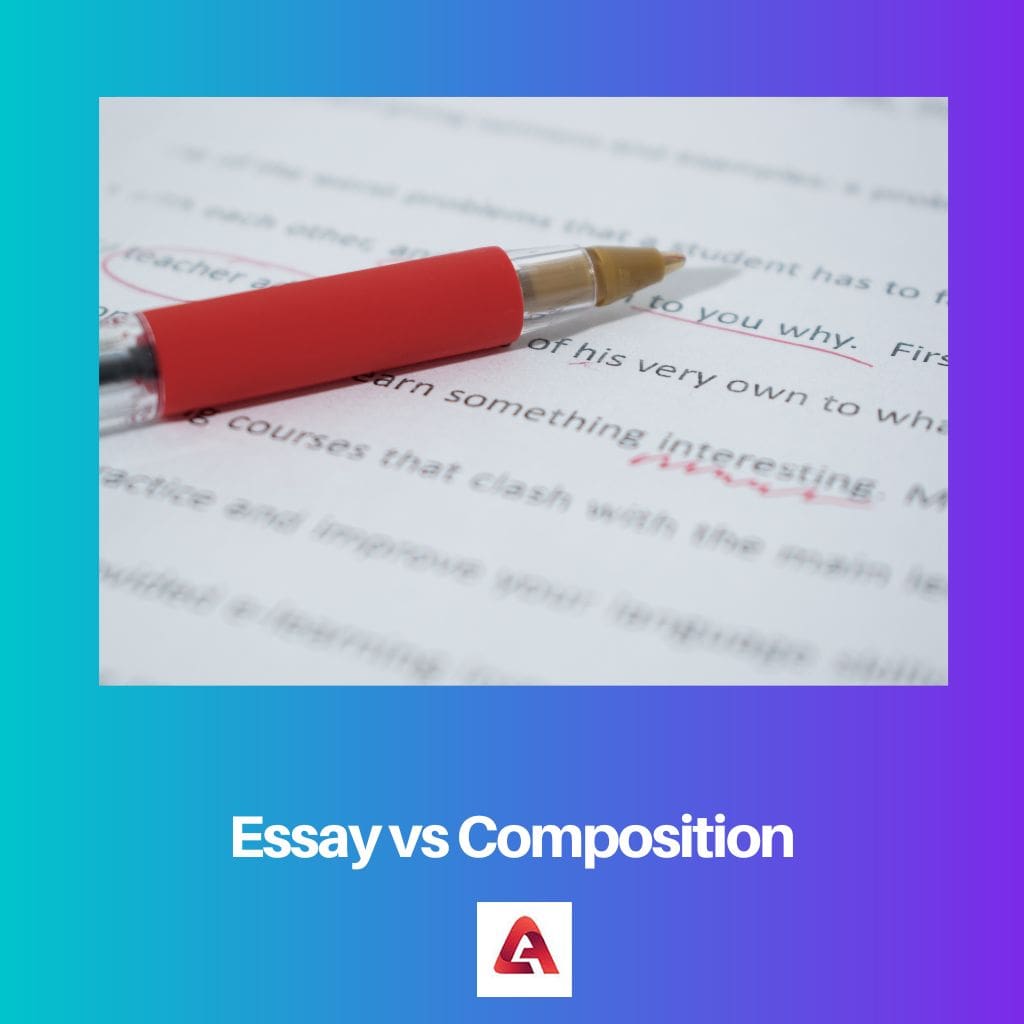
As such, essay and composition are not interchangeable terms. They also have different writing purposes. An essay aims to push readership to develop their position on a topic. A composition explains the topic and compares phenomena without declaring the author’s position.
An essay is a text of a small volume (sometimes a college essay can be up to 7-10 pages long, but usually, the required volume is not more than 2-3 pages). The essay is written in a prosaic style. In an essay, the author states his personal opinion on a topic.
The author can express his vision in a free form. In an essay, the author is speaking on a particular phenomenon, event, or opinion that is reasoning with his view. The essay requires not only gathering specific relevant information but also adding it to your thoughts and arguments.
Similar Reads
- Composition vs Inheritance: Difference and Comparison
- Aggregation vs Composition: Difference and Comparison
- Blog vs Essay: Difference and Comparison
- The an Essay vs Creative Writing: Difference and Comparison
This is not a one-day job for most students. That is why they apply to paper writing services for help from skilled professional writers. These services aim to teach students how to explain their thoughts and structure their essays correctly.
The work created with the help of writing services is a completed essay that can be added to the student’s impressions. The composition is a creative paper presenting the author’s thoughts and feelings on the topic without explaining his opinion.
For example, the composition topic about the Great Depression is “Franklin D. Roosevelt’s role during the Great Depression.” The essay topic about the Great Depression will be: “Did the New Deal solve the problem of the Great Depression?”
Comparison Table
What is essay.
This genre has recently become popular, but its roots date back to the 16 th century. Today, the essay is offered as a college and university assignment. An essay is a type of work built around a central topic.
The main purpose of writing an essay is to provoke the reader into reflection . Writing an essay allows learning to formulate your thoughts, structure information, find arguments, express the individual impression, and formulate your position.
The characteristics of an essay are a small volume, a specific topic, and free composition. The author must build a trusting relationship with the reader; therefore, writing an essay is much more difficult than writing a composition.
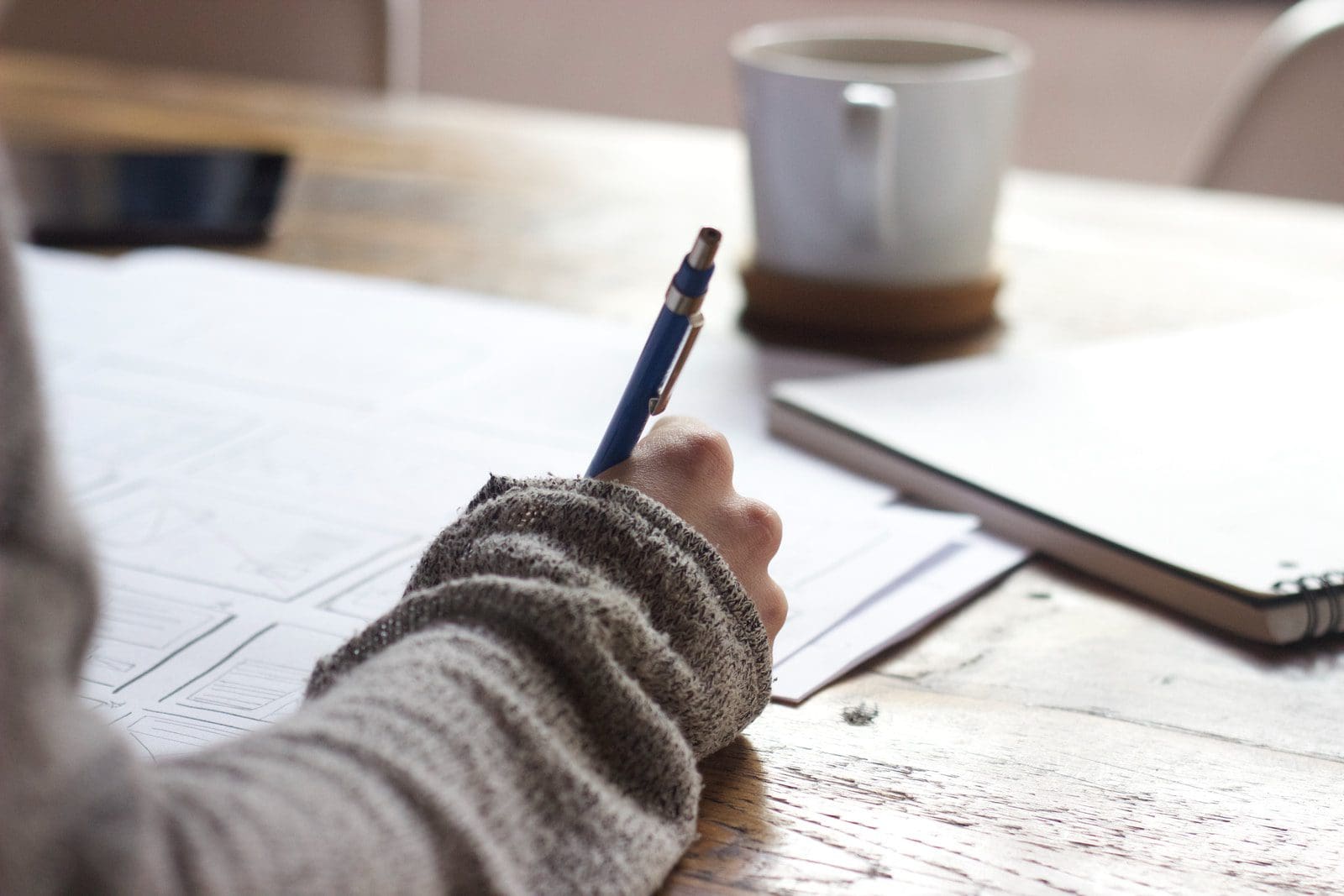
What is Composition?
A composition is a creative work, on a prescribed topic. It has a clear presentation structure.
In the composition, you can agree or disagree with the opinion of other authors, express your thoughts about what you read, compare works of different authors, and analyze their vision. A composition is expected to provide full disclosure of the topic.
To provide it, the paper must follow a set structure: an introduction that outlines the essential problem of the topic. This body explains and reveals the main idea of the composition and a logical conclusion. Therefore, a composition has a larger volume than an essay.
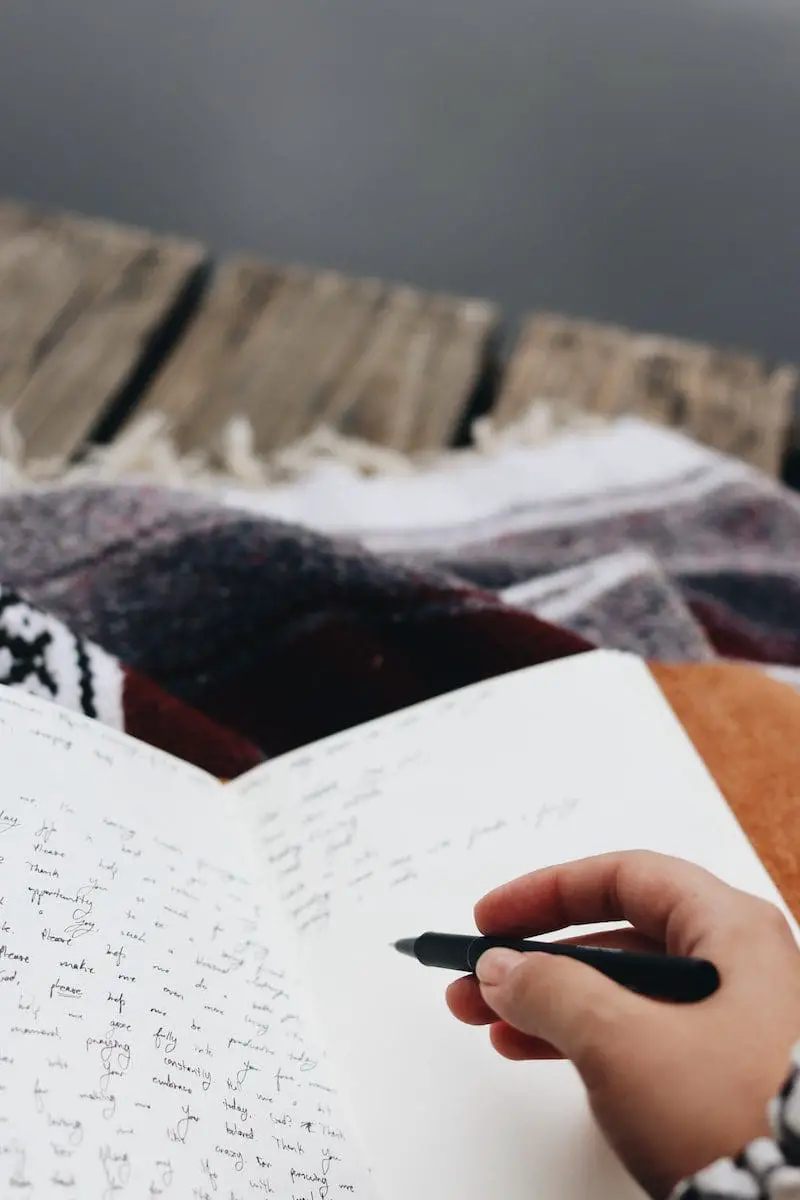
Main Differences Between An Essay And Composition
- There is a significant difference in style. A composition mainly contains the analysis of the topic. At the same time, the author’s position is clearly expressed in the essay.
- Compositions and essays vary in length. The essay, most often, has a small volume because the author’s thoughts must be clearly stated. The composition has a prescribed structure and a larger volume.
- An essay allows the author to express creativity and show his vision and attitude toward a specific phenomenon. A composition explains the topic according to its concept and doesn’t have to be supplemented with unusual thoughts.
- To write an essay, finding an original idea or developing an out-of-the-box view of a situation is significant. At the same time, writing a composition requires reading about the topic and talking about it.
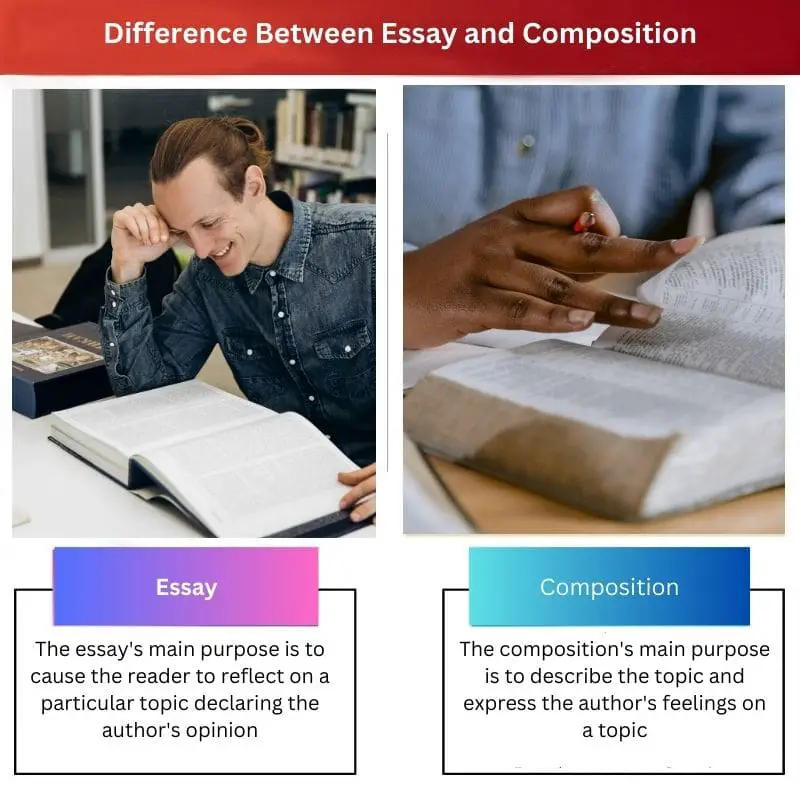
Last Updated : 11 June, 2023

I’ve put so much effort writing this blog post to provide value to you. It’ll be very helpful for me, if you consider sharing it on social media or with your friends/family. SHARING IS ♥️
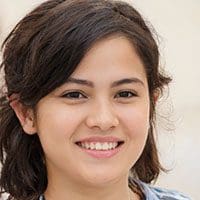
Emma Smith holds an MA degree in English from Irvine Valley College. She has been a Journalist since 2002, writing articles on the English language, Sports, and Law. Read more about me on her bio page .
Share this post!
24 thoughts on “essay vs composition: difference and comparison”.
Informative and thought-provoking! This article serves as a valuable resource for students and teachers, offering a clear understanding of the differences between essays and compositions.
The article’s comprehensive breakdown of the differences between essays and compositions is enlightening. It’s a valuable resource that could greatly benefit students and writers aiming to enhance their academic writing skills.
Definitely agree! This article provides a solid understanding of these academic writing forms.
The depth of the analysis in this post provides significant value, particularly in helping writers develop greater clarity on the requirements of essays and compositions.
The article offers a comprehensive analysis of the differences between essays and compositions, emphasizing the importance of understanding their distinct characteristics. It’s a great resource for students, teachers, and anyone interested in academic writing.
Absolutely agree! This is a valuable resource for anyone looking to improve their writing skills.
The article provides an excellent breakdown of essays and compositions, offering valuable insights into their unique characteristics and purposes. It is a highly informative read for both students and writers.
The article’s depth of analysis is impressive, making it a valuable guide for understanding the distinctions between essays and compositions.
Absolutely! This article serves as a detailed and comprehensive resource for grasping the nuances of academic writing.
This article effectively highlights the distinctions between essays and compositions, serving as an insightful resource for students and educators alike. The detailed comparison table is particularly helpful in understanding the differences.
Absolutely, the comparison table is a fantastic visual aid for grasping the disparities between essays and compositions.
The wealth of information provided in this article is incredibly enlightening, offering a thorough understanding of the differences between essays and compositions. It’s an invaluable read for students and aspiring writers.
I completely agree! This article is a comprehensive and informative resource for anyone looking to enhance their academic writing skills.
Absolutely! The distinctions laid out here provide a clear understanding of these academic writing forms, serving as a valuable resource for students and educators.
The post presents a well-structured and detailed comparison of essays and compositions, providing an insightful guide for students and writers. It offers a wealth of information on these academic writing forms.
I completely agree! This article delivers significant value in clarifying the distinctions between essays and compositions.
Absolutely, the depth and precision in the comparisons is commendable and highly beneficial for aspiring authors.
The post offers insightful comparisons between essays and compositions, providing a clear understanding of their respective purposes and structures. It’s a valuable read for students and writers seeking a deeper understanding of academic writing forms.
Absolutely, the precision in drawing the distinctions makes this article a must-read for students and academic writers.
The comparisons and detailed explanations are highly informative and beneficial for aspiring authors and students alike.
This article presents a detailed and well-structured comparison of essays and compositions, offering valuable insights into their unique characteristics and purposes. It’s a significant resource for students and writers alike.
Absolutely! The article delivers crucial information for developing a profound understanding of essays and compositions, providing an essential guide for aspiring authors and students.
The article’s clarifications make it clear that essays and compositions are not interchangeable terms, and provide a detailed description of their unique characteristics. Writers and educators will likely find this information incredibly helpful.
Definitely! The distinctions highlighted here are essential for understanding the nuances of academic writing.
Leave a Comment Cancel reply
Save my name, email, and website in this browser for the next time I comment.
Want to save this article for later? Click the heart in the bottom right corner to save to your own articles box!
British Council Malaysia
- English schools
- myClass Login
- Show search Search Search Close search
- English courses
- Kids and teens
- Learning resources for parents
Tips on how to prepare for comprehension and composition

Comprehension and composition are topics that our children learn in school. They are part of the learning process that will help them to eventually become better readers, writers and thinkers. Some students experience difficulty in this area especially when it comes to the comprehension and composition exercises.
Here are some helpful tips to guide us, both parents and children, in overcoming the hurdles related to this subject matter:
How important is background knowledge?
Over the past two decades, research into how we read and write has shown that the biggest factor affecting comprehension and composition is how much we know about the topic we are reading or writing about.
Your Pokémon Go obsessed child with a C average would likely be scoring an A in a comprehension paper if the text was about Pokémon Go. Student with good background knowledge can open up a comprehension paper on any range of topics and quickly understand what the writer is talking about. In their composition paper, they can easily pull out interesting and well-developed ideas for any question they are asked. Unfortunately, Pokémon Go is unlikely to be the sole topic of exams.
In order for your child to produce the relevant, interesting, and wide ranging essay required to get top marks, knowledge of the following topic areas is useful:
- Choice is a new issue in many countries. For most of human history, the concept of choosing clothes, schools, or even spouses was an alien idea.
- Even now, not all countries or societies have the same amount of choices available.
- Some countries choose to restrict their citizen’s choice based on economic or ideological reasons.
Without knowing this, students can only write about their own limited experience of choice – perhaps the difference between a hawker centre and a mall, which won’t lead to failure but also won’t bring in the higher grades.
What does your child need to do?
In the comprehension paper, the question types that students struggle with most – inference, authorial intention, and summary – are all based on their understanding of the passage.
An encyclopaedic knowledge of all 151 Pokémon may help your child ‘catch ‘em all’ but because of the huge variety of topics that comprehension and composition tests could include, this is not enough to succeed academically. For example, topics may include food, geography, consumerism and technology, science, nature and pollution.
Bearing this in mind, how can your child build their background knowledge to do better in this kind of exam?
The short term
In the short term, teens often possess a fair amount of background knowledge gained from the variety of subjects they study and their personal interests (sports, travel, Pokémon Go!).
In the lead up to the exam, try drawing these strands together. For example, getting your child to make a timeline charting when scientific discoveries were made, historical events happened or works of literature were written. Perhaps they can also try placing on a map the countries they have read about in social studies, English, or geography. Research into cognitive processes suggests that by drawing these kinds of links in our knowledge, it becomes more meaningful and memorable.
The long term
Building up a general knowledge bank over time will give your child a huge advantage. Our curriculum at the British Council supports this by focusing on essay writing and non-fiction texts filled with information.
From Secondary 1, our students study a topic for five weeks and regularly review what they’ve learned through quizzes and assessment tasks throughout the year. This leads to long-term learning and retention: absolutely essential for those mid-year and end-of-year exams!
That doesn’t mean that your child’s passion for Pokémon should be squashed. Research is clear that the more students read about a topic, whatever it’s about, the better they do in school exams.
But in addition to this, your child needs to build a broad general knowledge to be able to quickly comprehend exam questions on a whole range of topics. Who knows, perhaps a well-chosen example of how Pokémon Go has affected sedentary lifestyles might make a difference!

Home » Education » Difference Between Essay and Composition
Difference Between Essay and Composition
Main difference – essay vs composition.
Many students think that the two words Essay and Composition mean the same and can be used interchangeably. While it is true that essay is an essay a type of composition, not all compositions are essays. Let us first look at the meaning of composition. A composition can refer to any creative work, be it a short story, poem, essay, research paper or a piece of music. Therefore, the main difference between essay and composition is that essay is a type of composition whereas composition refers to any creative work .
What is an Essay
An essay is a literary composition that describes, analyzes, and evaluates a certain topic or an issue . It typically contains a combination of facts and figures and personal opinions, ideas of the writer. Essays are a type of commonly used academic writing in the field of education. In fact, the essay can be introduced as the main type of literary composition written in school level.
An essay typically consists of a brief introduction, a body that consists of supporting paragraphs, and a conclusion. However, the structure, content and the purpose of an essay can depend on the type of the essay. An essay can be classified into various types depending on the given essay title, or the style of the essay writer. Narrative , Descriptive , Argumentative , Expository , Persuasive , etc. are some of these essay types. The content , structure and style of the essay also depend on the nature of the essay. The complexity of the essay also depends on the type of the essay. For example, narrative and descriptive essays can be written even by primary school students whereas argumentative and persuasive essays are usually being written by older students.

What is a Composition
The term composition can refer to any creative work . A composition can be a piece of music, art of literature. For example, Symphony No. 40 in G minor is a composition by Mozart.
The term literary composition can refer a poem, short story, essay, drama , novel or even a research paper. It refers to an original and creative literary work.

Essay is a relatively short piece of writing on a particular topic.
Composition is a creative work.
Interconnection
Essay is a type of composition.
Not all compositions are essays.
Essay can be categorized as narrative, descriptive, persuasive, argumentative, expository, etc.
A composition can be a short story, novel, poem, essay, drama, painting, piece of music, etc.
Prose vs verse
Essay is always written in prose.

About the Author: admin
you may also like these.

An official website of the United States government
The .gov means it’s official. Federal government websites often end in .gov or .mil. Before sharing sensitive information, make sure you’re on a federal government site.
The site is secure. The https:// ensures that you are connecting to the official website and that any information you provide is encrypted and transmitted securely.
- Publications
- Account settings
Preview improvements coming to the PMC website in October 2024. Learn More or Try it out now .
- Advanced Search
- Journal List
- HHS Author Manuscripts

Relations between Reading and Writing: A Longitudinal Examination from Grades 3 to 6
Young-suk grace kim.
University of California Irvine
Yaacov Petscher
Florida Center for Reading Research
Jeanne Wanzek
Vanderbilt University
Stephanie Al Otaiba
Southern Methodist University
We investigated developmental trajectories of and the relation between reading and writing (word reading, reading comprehension, spelling, and written composition), using longitudinal data from students in Grades 3 to 6 in the US. Results revealed that word reading and spelling were best described as having linear growth trajectories whereas reading comprehension and written composition showed nonlinear growth trajectories with a quadratic function during the examined developmental period. Word reading and spelling were consistently strongly related (.73 ≤ r s ≤ .80) whereas reading comprehension and written composition were weakly related (.21 ≤ r s ≤ .37). Initial status and linear slope were negatively and moderately related for word reading (−.44) whereas they were strongly and positively related for spelling (.73). Initial status of word reading predicted initial status and growth rate of spelling; and growth rate of word reading predicted growth rate of spelling. In contrast, spelling did not predict word reading. When it comes to reading comprehension and writing, initial status of reading comprehension predicted initial status (.69), but not linear growth rate, of written comprehension. These results indicate that reading-writing relations are stronger at the lexical level than at the discourse level and may be a unidirectional one from reading to writing at least between Grades 3 and 6. Results are discussed in light of the interactive dynamic literacy model of reading-writing relations, and component skills of reading and writing development.
Reading and writing are the foundational skills for academic achievement and civic life. Many tasks, including those in school, require both reading and writing (e.g., taking notes or summarizing a chapter). Although reading and writing have been considered separately in much of the previous research in terms of theoretical models and curriculum ( Shanahan, 2006 ), their relations have been recognized (see Fitzgerald & Shanahan, 2000 ; Langer & Flihan, 2000 ; Shanahan, 2006 for review). In the present study, our goal was to expand our understanding of developmental trajectories of reading and writing (word reading, reading comprehension, spelling, and written composition), and to examine developmental relations between reading and writing at the lexical level (word reading and spelling) and discourse level (reading comprehension and written composition), using longitudinal data from upper-elementary grades (Grades 3 to 6).
Successful reading comprehension entails construction of an accurate situation model based on the given written text ( Kintsch, 1988 ). Therefore, decoding or reading words is a necessary skill (Hoover & Gough, 1990). The other necessary skill is comprehension, which involves parsing and analysis of linguistic information of the given text. This requires working memory and attention to hold and access linguistic information (Daneman & Merikle, 1996; Kim, 2017 ) as well as oral language skills such as vocabulary and grammatical knowledge ( Cromley & Azevedo, 2007 ; Elleman, Lindo, Morphy, & Compton, 2009 ; Kim, 2015 , 2017 ; National Institute of Child Health and Human Development, 2000 ; Vellutino, Tunmer, Jaccard, & Chen, 2007 ). In addition, construction of an accurate situation model requires making inferences and integrating propositions across the text and with one’s background knowledge to establish global coherence. These inference and integration processes draw on higher order cognitive skills such as inference, perspective taking, and comprehension monitoring ( Cain & Oakhill, 1999 ; Cain, Oakhill, & Bryant, 2004 ; Cromley & Azevedo, 2007 ; Kim, 2015 , 2017 ; Kim & Phillips, 2014 ; Oakhill & Cain, 2012 ; Pressley & Ghatala, 1990 ).
In writing (written composition), one has to generate content in print. As a production task, transcription skills (spelling, handwriting or keyboarding fluency) are necessary (e.g., Berninger & Amtmann, 2002; Graham, Berninger, Abbott, Abbott, & Whitaker, 1997 ; Juel, Griffith, & Gough, 1986 ). Generated ideas undergo translation into oral language in order to express ideas and propositions with accurate words and sentence structures; and thus, writing draws on oral language skills ( Berninger & Abbott, 2010 ; Kim et al., 2011 , 2013 , 2015a ; Olinghouse, 2008 ). Of course, quality writing is not a sum of words and sentences, but requires local and global coherence ( Kim & Schatschneider, 2017 ; Bamberg, 1983 ). Coherence is achieved when propositions are logically and tightly presented and organized, and meet the needs of the audience. This draws on higher order cognitive skills such as inference, perspective taking ( Kim & Schatschneider, 2017 ; Kim & Graham, 2018 ), and self-regulation and monitoring (Berninger & Amtmann, 2002; Kim & Graham, 2018 ; Limpo & Alves, 2013 ). Coordinating these multiple processes of generating, translating, and transcribing ideas relies on working memory to access short term and long term memory (Berninger & Amtmann, 2002; Hayes & Chenoweth, 2007 ; Kellogg, 1999 ; Kim & Schatschneider, 2017 ) as well as sustained attention ( Berninger & Winn, 2006 ).
What is apparent in this brief review is similarities of component skills of reading and writing skills (see Kim & Graham, 2018 ; Fitzgerald & Shanahan, 2000 ). Then, what is the nature of reading and writing relations 1 ? According to the interactive and dynamic literacy model ( Kim & Graham, 2018 ), reading and writing are hypothesized to co-develop and influence each other during development (interactive), but the relations change as a function of grain size and developmental phase (dynamic). The interactive nature of the relation is expected for two reasons. First, if reading and writing share language and cognitive resources to a large extent, then, development of those skills would influence both reading and writing. Second, the functional and experiential aspect of reading and writing facilitates co-development ( Fitzgerald & Shanahan, 2000 ). The majority of reading and writing tasks occur together (e.g., writing in response to written source materials; note taking after reading); and this functional aspect would facilitate and reinforce learning key knowledge and meta-awareness about print and text attributes (e.g., text structures) in the context of reading and writing.
Reading-writing relations are also expected to be dynamic or to change as a function of various factors such as grain size ( Kim & Graham, 2018 ). When the grain size is relatively small (i.e., word reading and spelling), reading-writing relations are expected to be stronger because these draw on a more or less confined set of skills such as orthography, phonology, and semantics ( Adams, 1990 ; Carlisle & Katz, 2006 ; Deacon & Bryant, 2005 ; Kim, Apel, & Al Otaiba, 2013 ; Nagy, Berninger, & Abbott, 2006 ; Ehri, 2000 ; Treiman, 1993 ). In contrast, when the grain size is larger (i.e., discourse-level skills such as reading comprehension and written composition), the relation is hypothesized to be weaker because discourse literacy skills draw on a more highly complex set of component skills, which entails more ways to be divergent (see Kim & Graham, 2018 ). Extant evidence provides support for different magnitudes of relations as a function of grain size (i.e., lexical versus discourse level literacy skills). Moderate to strong correlations have been reported for lexical-level literacy skills (i.e., word reading and spelling; .50 ≤ r s ≤ .84; Ahmed, Wagner, & Lopez, 2014 ; Berninger & Swanson, 1994 ; Ehri, 2000 ; Juel et al., 1986 ; Kim, 2011 ; Kim, Al Otaiba, Wanzek, & Gatlin, 2015a ) whereas a weaker relation has been reported for reading comprehension and written composition (.01 ≤ r s ≤ .59; Abbott & Berninger, 1993 ; Ahmed et al., 2014 ; Berninger & Abbott, 2010 ; Berninger et al., 1993; Juel et al., 1986 ; Kim et al., 2015a ).
Although previous work on reading-writing relations has been informative, empirical investigations of developmental relations between reading and writing using longitudinal data were limited. In fact, little is known about developmental patterns of writing skills (for reading development, see, for example, Kieffer, 2011 ; McCoach, O’Connell, Reis, & Levitt, 2006 ; Morgan, Farkas, & Wu, 2011 ), let alone developmental relations between reading and writing. In other words, our understanding is limited about a) the functional form or shape of development – whether writing skills, including both spelling and written composition, develop linearly or non-linearly; and b) the nature of growth in terms of the relation between initial status and the other growth parameters (linear slope and/or quadratic function) – a positive relation between initial status and linear growth would indicate that students with more advanced skills at initial status would growth faster, similar to the Matthew Effect ( Stanovich, 1986 ), whereas a negative relation would indicate a mastery relation where students with advanced initial status showing less growth.
Relatively few studies have investigated developmental trajectories for either spelling or writing. In spelling, a nonlinear developmental trajectory was reported for Norwegian-speaking children in the first three years of schooling ( Lervag & Hulme, 2010 ). Nonlinear developmental trajectories in spelling were also found for Korean-speaking children and developmental trajectories differed as a function of word characteristics ( Kim, Petscher, & Park, 2016 ). In written composition, only a couple of studies have investigated development trajectories. Kim, Puranik, and Al Otaiba (2015b) investigated growth trajectories of writing within Grade 1 (beginning to end) for three groups of English-speaking children: typically developing children, children with language impairment, and those with speech impairment. They found that although there were differences in initial status among the three groups, the linear developmental rate in writing did not differ among the three groups of children. This study was limited, however, because it examined development within a relatively short period (Grade 1), and the functional form of the growth trajectory was limited to a linear model because only three waves of data were available. Another longitudinal study, conducted by Ahmed and her colleagues ( 2014 ), followed English-speaking children from Grades 1 to 4, but growth trajectories over time were not examined because their focus was the relation between reading and writing, using changes in scores between grades.
The vast majority of previous studies on reading-writing relations have been cross-sectional investigations, and they have reported somewhat mixed findings. Some reported a unidirectional relation of reading to writing ( Kim, 2011 ; Kim et al., 2015a ); some reported a direction from writing to reading ( Berninger, Abbott, Abbott, Graham, & Richards, 2002 ; see also Graham & Hebert’s [2010] meta-analysis); and others reported bidirectional relations ( Berninger & Abbott, 2010 ; Kim & Graham, 2018 ; Shanahan & Lomax, 1986; Shanahan & Lomax, 1988). Results from limited extant longitudinal studies are also mixed. Lerkkanen, Rasku-Puttonen, Aunola, and Nurmi (2004) , using longitudinal data (4 time points across the year) from Finnish first grade children, reported a bidirectional relation between reading (composed of word reading and reading comprehension) and spelling during the initial phase of development, but not during the later phase. As for the relation between written composition and reading (composed of word reading and reading comprehension), the direction was from writing to reading, but not the other way around. Ahmed et al. (2014) examined reading-writing relations at the lexical, sentence, and discourse levels using longitudinal data from Grades 1 to 4, and found different patterns at different grain sizes. They reported a unidirectional relation from reading to writing at the lexical (word reading-spelling) and discourse levels (reading comprehension and written composition), but a bidirectional relation at the sentence level.
Findings from these studies suggest that reading and writing are related, but the developmental nature of relations still remains unclear. Building on these previous studies, the primary goal of the present study was to expand our understanding of the development of reading and writing, and their interrelations. To this end, we examined growth trajectories and developmental relations of reading and writing at the lexical and discourse-levels. Although previous studies did reveal relations between reading and writing, the number of studies which explicitly examined developmental relations at the same grain size of language (i.e., lexical level and discourse level) using longitudinal data is extremely limited, with the above noted Ahmed et al.’s (2014) study as an exception. We examined the reading-writing relations at the lexical-level and discourse-level, respectively. This is because theory and evidence clearly indicate that the component skills of reading and writing differ for lexical literacy skills (e.g., Adams, 1990 ; Treiman, 1993 ) versus discourse literacy skills (e.g., Berninger & Winn, 2006 ; Hoover & Gough, 1990; Perfetti & Stafura, 2014; Kim, 2017 ).
With the overarching goal of examining developmental relations between reading and writing at the lexical and discourse-levels, we had the following two research questions:
- What are the patterns of development of reading (word reading and reading comprehension) and writing (spelling and written composition) from Grades 3 to 6?
How are growth trajectories in reading and writing interrelated over time from Grades 3 to 6?
With regard to the first research question, we expected nonlinear growth trajectories for word reading, spelling, and reading comprehension where linear development is followed by a slowing down (or plateau). Due to lack of prior evidence in the grades we examined (i.e., Grades 3 to 6), we did not have a specific hypothesis about the functional form of growth trajectories for written composition. In terms of reading-writing relations, we hypothesized a stronger relation between word reading and spelling than that for reading comprehension and written composition. We also hypothesized a bidirectional relation particularly between word reading and spelling based on fairly strong bivariate relations reviewed above. For reading comprehension and written composition, we expected a weaker relation, and did not have a specific hypothesis about bidirectionality, given lack of empirical data in upper elementary grades.
Participants
Data from the present study are from a longitudinal study of students’ reading and writing development in the South Eastern region of the US. Cross sectional results on predictors of writing in Grades 1 to 3 have been reported previously ( Kim et al., 2014 , 2015a ). However, longitudinal data from Grades 3 (mean age = 8.25, SD =.39) to 6, the focal grades in the present study, have not been reported. The longitudinal study was composed of two cohorts of children in the same district. In other words, the sample sizes in each grade (see Table 1 ) were the sum of two cohorts of children.
Descriptive statistics for outcome measures
Note. WJ = Woodcock Johnson; LWID = Letter Word Identification Task; PC = Passage Comprehension; WIAT = Wechsler Individual Achievement Test; One day = One day prompt; TDTO = Thematic Development and Text Organization
As shown in Table 1 , total sample size in each grade varied across years and each measure 2 . For instance, in spelling, data from a total of 359 children were available in Grade 3 whereas in Grade 6, data were available for 278 children. An empirical test of whether missingness is completely at random (MCAR; Little, 1988 ) or not revealed that all data in grades 3–6 were MCAR, χ 2 (492) = 530.13, p = .114, with the exception of the grade 6 writing data, χ 2 (4) = 21.46, p < .001. However, a review of the data suggested that the data were not non-ignorable missing and the patterns of missing were unrelated to the variables themselves. As such, full-information maximum likelihood was the appropriate method for estimating coefficients in the presence of missing data ( Enders, 2010 ).
The sample was composed of 53% male students who were predominantly African-Americans (59%), followed by White (29%), Multi-racial (9%), Other (2%), and Native American or Asian (1%). We noted a pattern of more attrition related to free and reduced lunch price status. In grade 3, 51% of students were eligible for free or reduced price lunch compared to 49% in grade 4, 39% in grade 5, and 29% in grade 6. Further, 10% of students in grade 3 were identified with a primary exceptionality, 7% in grade 4, 6% in grade 5, and 6% in grade 6. No students were identified as having limited English proficiency.
Word reading
Children’s word reading was assessed by the Letter Word Identification task of the Woodcock Johnson-III (WJ-III; Woodcock, McGrew, & Mather, 2001 ). In this task, the child is asked read aloud words of increasing difficulty. This task assesses children’s decoding skill and knowledge of word specific spellings in English. Cronbach’s alpha estimates across grades 3–6 ranged from .90 to .91 according the test manual. The Letter Word Identification task of WJ-III has been widely used in previous studies and has been shown to be strongly related to other word reading tasks (e.g., r = .92; Kim et al., 2015a ; Kim & Wagner, 2015 ).
Reading comprehension
The Passage Comprehension task of WJ-III was used. This is a cloze task where the child is asked to read sentences and short passages and to fill in the blanks. Cronbach’s alpha estimates across the grades ranged from .76 to .84. This has also been widely used as a measure of reading comprehension with strong correlations with other well-established measures of reading comprehension (e.g., .70 ≤ r s ≤ .82; Keenan et al., 2008 ; Kim & Wagner, 2015 ).
The Spelling task of WJ-III was used. This is a dictation task where the child hears the word in isolation, in a sentence, and in isolation again, and is asked to spell it. Cronbach’s alpha estimates across the grades ranged from .90 to .91. The WJ-III has been reported to be strongly related to word reading skills (.76 ≤ r s ≤ .83; Kim et al., 2015a ; McGrew, Schrank, & Woodcock, 2007 ).
Written composition
Written composition was measured by two tasks: The Essay Composition task of the Wechsler Individual Achievement Test-3 rd (WIAT-3; Wechsler, 2009 ) and an experimental task that were used in previous studies ( Kim et al., 2014 , 2015a ; McMaster, Du, & Pétursdôttir, 2009 ; also see Abbott & Berninger, 1993 for a similar prompt). In the WIAT task, the child was asked to write about her favorite game and provide three reasons. The WIAT task has been widely used in previous studies (e.g., Berninger & Abbott, 2010 ) and was related to other writing prompts (.38 ≤ r s ≤ .45; Kim et al., 2015a ). In the experimental task, the child was asked to write about something interesting that happened after they got home from school one day (One day prompt hereafter). The One day prompt has been shown to be related to the WIAT writing task ( r = .45; Kim et al., 2015a ) and was related to other indicators of writing proficiency such as writing productivity and fluency ( McMaster et al., 2009 ). Children were given 15 minutes to complete each of their writing tasks.
Students’ written compositions were evaluated on the quality of ideas on a scale of 0 (unscorable) to 7, which was modified from the widely used 6+1 Trait approach ( Northwest Regional Educational Laboratory, 2011 ). A similar approach has been widely used in previous studies ( Graham, Berninger, & Fan, 2007 ; Hooper, Swartz, Wakely, de Kruif, & Montgomery, 2002 ; Kim et al., 2014 ; , 2015a ; Olinghouse, 2008 ). Compositions that had rich and clear ideas with details received higher scores. In addition to the idea quality, the WIAT Essay Composition task was also evaluated on thematic development and text organization (TDTO hereafter) following the examiner’s manual. Coders were rigorously trained to achieve high reliability within each year as well as across the years. For the present study, we established inter-rater reliability using 40–50 written compositions per prompt per year; Cohen’s Kappa ranged from .78 to .97.
Children were assessed in the spring by carefully trained assessors in a quiet space in each school. Assessment consisted of two sessions of individual assessment and two sessions of small group sessions. Research assistants were trained for two hours prior to each assessment session were required to pass a fidelity check before administering assessments to the participants in order to ensure accuracy in administration and scoring. The reading tasks and the spelling task were individually administered whereas the written compositions were administered in a small group setting (3–4 children).
Data Analytic Approach
We employed a combination of latent individual growth curve modeling and structural equation modeling in this study. An important aspect of evaluating the structural cross-construct relations is first understanding the underlying functional form of growth for each of the four outcome types. To this end, four specific latent variable models were tested for each outcome: a linear growth model, a non-linear growth model with non-linearity defined through a quadratic term, a linear free-loading growth model, and a linear latent change score (or dual change score) model. Each of these models reflect an alternative consideration of how growth is shaped ( Petscher, Quinn, & Wagner, 2016 ). The linear latent growth model describes a strictly linear relation over time regardless of the number of time points in the model; thus, even though there are four observed waves of data, the linear model forces a linear growth curve. The non-linear growth model extends the linear model by allowing multiple non-linear terms to be added above the linear slope; and the different alternative nonlinear models were evaluated for precise estimation of non-linearity. In the present data, four available time points permitted specification of a quadratic parameter to be estimated to determine the rate of celeration that one grows (i.e., acceleration or deceleration). The freed loading growth curve model is eponymous such that the loadings on the slope factor in the growth model are freely estimated rather than fixed at particular time intervals. In this way, the shape of the curve is defined by the estimated loadings, not a priori determined values. For example, in a linear growth model the loadings may be coded as 0, 1, 2, 3 for four time points and the equal interval coding points to the assumption of equal interval change over time. A freed loading growth model may code the loading structure as 0, *, *, 1 where 0 and 1 denote the beginning and end of change and * denotes freely estimated proportional change that may occur between times 1 and 4. The dual change score model ( McArdle, 2009 ) may be viewed as a hybrid of direct and/or indirect models with individual growth curve analysis. Dual change models include two types of change parameters, an average slope factor, such as in the linear model, and a proportional change parameter that reflects the relation between a prior time point and the change between two time points.
For the word reading, spelling, and reading comprehension outcomes, the latent growth models were fit directly to the observed measures. However, for written composition, with multiple measures of writing data at each time point, multiple indicator growth models ( Meredith & Tisak, 1990 ) were specified for each of the four general model types described above. The inclusion of the multiple indicators necessitate additional model testing steps to evaluate levels of longitudinal invariance for the loadings, intercepts, and variances. The level(s) of measurement invariance serves to ensure that the latent variables are measured on the same metric over time so that differences in the latent means and variances are due to individual differences in the latent scores and not due to biases that are consequential to a lack of measurement invariance. Loading invariance was first tested, followed by various iterations of freeing model constraints on the basis of modification indices. Once a decision was made regarding measurement invariance, the multiple indicator growth models were specified.
Following the growth model evaluations, two structural equation models were specified for pairs of constructs. First, the latent intercept and slope factors from the word reading growth model were used as predictors of factors in the spelling growth model, as well as the latent intercept from the spelling growth model as a predictor of growth in word reading. Second, the latent intercept and slope factors from the reading comprehension growth model were used as predictors of factors in the writing growth model. Fit for all latent variables was evaluated using the comparative fit index (CFI; Bentler, 1990 ), Tucker-Lewis index (TLI; Bentler & Bonett, 1980 ), and the root mean square error of approximation (RMSEA; Browne & Cudeck, 1992 ). CFI and TLI values greater than .90 are considered to be minimally sufficient criteria for acceptable model fit ( Hooper, Coughlan, & Mullen, 2008 ) and RMSEA values <.10 are desirable. The Bayes Information Criteria (BIC) was used as another index for comparing model fit with model difference of at least 5 suggesting practically important differences (Raftery, 1995).
Descriptive Statistics
Table 1 provides the descriptive statistics (W scores for the WJ measures & standard scores when available) across all measures and time points. Mean standard scores in the standardized and normed tasks were in the average range across the years (93–111). Average W scores in WJ-III Letter-Word Identification scores increased from grade 3 ( M = 499.40, SD = 19.34) to grade 6 ( M = 519.48, SD = 17.98), as did the WJ-III Spelling scores (grade 3: M = 498.85, SD = 17.08; grade 6: M = 515.91, SD = 16.16), and the WJ-III Passage Comprehension scores (grade 3: M = 491.34, SD = 11.26; grade 6: M = 501.61, SD = 11.49).
For writing measures, raw scores showed increases from grades 3 to 6 on both the WIAT TDTO (grade 3: M = 6.67, SD = 2.88; grade 6: M = 9.62, SD = 4.01) and the WIAT idea quality (grade 3: M = 3.80, SD = 0.88; grade 6: M = 4.33, SD = 1.15). The mean WIAT TDTO standard scores were in the average range (106–111). In contrast, mean scores for the One Day idea quality measure did not show a similar pattern of growth, but decreased slightly (grade 3: M = 4.40, SD = 1.07; grade 6: M = 4.25, SD = 0.99). Although this may appear surprising, a slight dip or no growth in a particular year in writing quality has been previously reported ( Ahmed et al., 2014 ).
Correlations among the measures across grades are reported in Table 2 . The relations between reading and writing in each grade varied: Word reading and spelling were strongly related (.73 ≤ r s ≤ .80) whereas reading comprehension and writing were somewhat weakly related (.21 ≤ r s ≤ .37). Correlation matrices within tasks across grades show that word reading tasks (.75 ≤ r s ≤ .86) and spelling (.83 ≤ r s ≤ .89) were strongly correlated across grades. Reading comprehension was also fairly strongly related across the grades (.60 ≤ r s ≤ .69) In contrast, correlations in writing scores across grades were weakly to moderately related (.15 ≤ r s ≤ .50).
Correlations among variables
G = Grade; LWID = Letter Word Identification Task; PC = Passage Comprehension; WT = WIAT Essay task; One day = One day prompt; TDTO = Thematic Development and Text Organization
Research Question 1
What are the patterns of development in reading (word reading and reading comprehension) and writing (spelling and written composition) from Grades 3 to 6?
Prior to the specification of the growth models for all outcomes, the longitudinal invariance of the writing measures was evaluated with Mplus v7.0 ( Muthén & Muthén, 1998–2013 ). The first phase of the model building was to identify the extent to which a single factor best represented the measurement-level covariances among the three measured writing variables at each of the grade levels. However, because the model was just-identified (i.e., 0 degrees of freedom), fit indices were not available for the grade-based models. The baseline model for longitudinal invariance specified longitudinal constraints on the loadings, intercepts, and residual variances and the model fit was poor: χ 2 (75) = 480.97, RMSEA = .107, (90% RMSEA CI = .098, .116), CFI = .68, TLI = .72. Through a series of model revisions a final model was specified that included invariant loadings and intercepts, partially invariant residual variances (i.e., grade 3 WIAT TDTO was freely estimated), and the addition of three residual covariances among writing measures, χ 2 (62) = 115.40, RMSEA = .043 (90% RMSEA CI = .030, .055), CFI = .96, TLI = .96, and the fit of this final model was significantly better than the fully invariant model (Δχ 2 = 365.57, Δdf = 13, p < .001).
As noted above, four alternative growth models were examined and compared for each of the outcomes, word reading, spelling, reading comprehension, and writing. Model fit results are reported in Table 3 . Generally, each model configuration fit well to the outcomes. For example, the word reading models all maintained acceptable CFI and TLI (>.95) as well as RMSEA (<.10). When using the BIC to compare relative model fit, both the dual change score model (BIC = 9,720) and freed loading model (BIC = 9,719) were lower by at least 5 points from the linear latent growth (BIC = 9,735) and quadratic growth (BIC = 9,729) models but only differed by 1 point from each other. Based on the χ 2 /df ratio and the measurement simplicity, the freed loading model was selected for word reading. The results from the freed loading model indicated that 45% of the total growth in word reading having occurred between grades 3 and 4, 26% of growth occurring between grades 4 and 5, and 29% of growth occurring between grades 5 and 6. The comparison of the spelling growth models showed an advantage for the dual change score model over the freed loading and non-linear growth models by 11 points on the BIC, as well as a 41 point difference with the linear latent growth model.
Developmental model fit for word reading, spelling, reading comprehension, and writing
Note . BIC = Bayes Information Criteria, df = degrees of freedom, RMSEA = root mean square error of approximation, LB = 90% RMSEA lower bound, UB = 90% RMSEA upper bound, CFI = comparative fit index, TLI = Tucker-Lewis index.
For reading comprehension, the quadratic growth and dual change scores models fit better to the other two alternatives, and similar to the word reading model selection, the χ 2 /df ratio and the measurement parsimony led to the selection of the quadratic growth model. Finally, the quadratic growth model was selected for the writing outcome based on its relative fit to the dual change models (i.e., ΔBIC = 30), and its superior fit to the linear latent growth model (Δχ 2 = 14.74, Δdf = 4, p < .01) and the freed loading model (Δχ 2 = 8.74, Δdf = 2, p < .05).
Randomly selected individual growth curves ( n = 25) for each of the four outcomes are presented in Figure 1 . The word reading curves reflect the linear relation over time with slight individual differences in the amount of change occurring. Similarly, though spelling change over time appear non-linear, the variance in the linear and quadratic slope functions were minimal and resulted in relatively parallel development. Both the reading comprehension and latent writing trajectories demonstrated individual differences in change with large differences observed in the latent writing development.

Randomly selected estimated individual curves from n =25 for word reading, spelling, reading comprehension, and latent writing across grades 3–6 (Times 1–4).
Research Question 2
The first structural analysis tested the relation between word reading intercept (centered in grade 3) and slope in predicting spelling intercept (centered in grade 3) and slope, χ 2 (22) = 27.92, RMSEA = .024, 90% RMSEA CI = .000, .047, CFI = .99, TLI = .99. Standardized path coefficients are presented in Figure 2 (Unstandardized model coefficients are reported in Appendix A1 ). Word reading intercept (initial status) and slope were moderately and negatively related (−.44), indicating that children who had higher word reading in Grade 3 had a slower growth rate in word reading. In contrast, spelling intercept and slope had a strong and positive relation (.73), indicating that children who had a higher spelling skill showed a faster growth rate over time. In terms of the relation between word reading and spelling, Grade 3 word reading scores significantly predicted Grade 3 spelling scores (.86) as well as the average spelling growth trajectory (.96). Word reading growth also uniquely predicted the average spelling growth trajectory (.22). In contrast, Grade 3 spelling scores did not significantly predict growth in word reading (.16, p > .50). A model including bi-directional paths from word reading slope to spelling slope did not converge; a final model included a covariance between word reading and spelling slopes with the correlation estimated as .08 ( p > .50). The inclusion of the word reading predictors resulted in 75% of the variance in Grade 3 spelling explained along with 84% of the variance in spelling growth.

Latent word reading development predicting latent spelling development. LWID = Letter Word Identification
Standardized path coefficients for the predictive model of reading comprehension development to writing development are shown in Figure 3 : χ 2 (110) = 218.45, RMSEA = .045, 90% RMSEA CI = .037, .054, CFI = .95, TLI = .94 (Unstandardized model coefficients are reported in Appendix A2 ). Although our goal was to examine how growth trajectories (initial status, linear slope, and quadratic terms) in reading comprehension and writing are related to one another, this was not permitted due to zero variance in the linear slope and quadratic terms in reading comprehension as well as the quadratic term in writing. As shown in Figure 3 , Grade 3 reading comprehension significantly predicted Grade 3 writing (.69) 3 , but did not significantly explain differences in the linear writing slope (.10, p = .29). Grade 3 reading comprehension explained 48% of the variance in Grade 3 writing and 1% of the variance in the linear writing slope. Furthermore, intercept and linear slope in writing were not related (.09, p = .74); and the relation between intercept and linear slope in reading comprehension was not estimated due to lack of variance in the slope of reading comprehension.

Latent reading comprehension development predicting latent writing development. PC = Passage Comprehension; W = Writing quality
Two overarching questions guided the present study: a) what are the growth trajectories or growth patterns in reading and writing across Grades 3 to 6, and b) what is the developmental relation between reading and writing for children in these grades. We focused on development from Grades 3 to 6 when children are expected to have developed foundational literacy skills, but continue to develop reading and writing skills. Colloquially, they have moved from a learning to read to a reading to learn phase (Chall, 1983).
We found that different growth models described best the four different reading and writing outcomes. Overall, alternative models for the four literacy outcomes fit the data well. Unlike our hypothesis of nonlinear trajectories, for lexical-level literacy skills, word reading and spelling, linear models (freed loading and dual change models) described data best, at least from Grades 3 to 6. For word reading, results from the freed loading growth curve fit the data best and showed the amount of growth in word reading varied as function of development time points. The largest amount of growth (45% of total growth) occurred between grades 3 and 4 with less growth between grades 4 and 5 (26%) and between grades 5 and 6 (29%). This is convergent with a previous study, which found that growth in reading skills was larger in lower grades than upper grades ( Kieffer, 2011 ). For spelling, again the dual change score model described the data best. The dual change score model does not differ from the traditional growth model in terms of shapes of growth pattern. The dual change score model, however, adds nuances because it captures proportional (i.e., auto-regressive) growth parameters (changes between two time points) in addition to average growth parameters in traditional growth models (changes across all the time points).
Interestingly, however, for reading comprehension and written composition, nonlinear trajectories with a quadratic function described the data best. In other words, developmental trajectories were characterized by an initial linear development followed by slowing down (or plateau). This nonlinear trajectory in reading comprehension is convergent with previous work (e.g., Kieffer, 2011 ). The present study is the first one to describe any growth pattern over time in written composition beyond a single academic year and/or from Grades 3 to 6. Taken together with the limited extant work, it appears that reading comprehension and written composition develop in nonlinear trajectories, characterized by initial strong growth and followed by a pattern of deceleration, or slowing down, at least from Grades 3 to 6.
Another interesting finding about growth trajectories in reading and writing was the relation between initial status and linear growth rate. For word reading, children’s status in Grade 3 was negatively related to rate of growth (−.44) such that those who had advanced word reading in Grade 3 had a slower growth rate through the grades. Spelling, on the other hand, showed a different pattern with a strong positive relation between initial status and growth rate (.73), indicating that students with a higher spelling skill at Grade 3 developed at a faster rate from Grades 3 to 6. Although there might be several explanations, we speculate that these results are attributed, at least partially, to the fact that children in these grades are in different developmental phases in word reading versus spelling. In word reading, many children have reached high levels of proficiency by Grade 3, and therefore, their subsequent learning rate is slower as their learning approaches a ceiling. In spelling, however, students’ overall development in Grade 3 did not quite reach as high because spelling requires greater accuracy and precision in orthographic representations than reading ( Ehri, 2000 ). Therefore, there is sufficient room for further growth for the majority of learners, and those with more advanced spelling in Grade 3 continue to grow at a fast rate in subsequent grades, presumably because they have more solid foundations in component skills of spelling. Another possible explanation may relate to instruction; that is by third grade, relatively little reading instructional focus is at the word level, because students are expected to have mastered learning to read whereas spelling instruction may continue, particularly for more complex word patterns. This speculation, however, requires future studies.
Results for discourse-level literacy skills were less clear. Unfortunately, the relation between initial status and growth rates was not estimable for reading comprehension due to the lack of variance in the linear slope and quadratic parameters. In written composition, although there was variation in the linear slope, the relation between initial status and linear slope was not statistically significant. This finding suggests that initial student writing levels do not necessarily predict future growth in writing. However, given that this was the first study to explicitly examine the relations between initial status and growth trajectories in writing, our findings cannot be compared to any previous research, and so will require replication in future studies.
Turning to the relation between reading and writing, we hypothesized a dynamic relation between reading and writing as a function of grain size – differential relations for the lexical-level skills versus discourse-level skills, hypothesizing a stronger relation between word reading and spelling than between reading comprehension and written composition. This hypothesis was supported such that bivariate correlations between word reading and spelling were strong across grades (.73 ≤ r s ≤ .80). The strong correlation between word reading and spelling is convergent with theoretical explanations and empirical evidence that word reading and spelling rely on a limited number of highly similar skills such as phonological awareness, orthographic awareness (letter knowledge and letter patterns), and morphological awareness ( Apel, Wilson-Fowler, Brimo, & Perrin, 2012 ; Berninger et al., 1998 ; Ehri, Satlow, & Gaskins, 2009 ; Kim, 2011 ; Kim et al., 2013 ;Treiman, 1998).
When reading-writing relations were examined at the discourse level, the relation was weak (.21 ≤ r s ≤ .37), convergent with previous evidence ( Ahmed et al., 2014 ; Berninger & Swanson, 1994 ; Berninger & Abbott, 2010 ; Kim et al., 2015a ). The overall weak relation indicates that reading comprehension and written composition have shared variance, but are unique and independent to a large extent, at least during the relatively early phase of development examined in the present study (Grades 3 – 6). Reading comprehension and written comprehension draw on complex, similar sets of skills and knowledge such as oral language, lexical-level literacy skills, higher-order cognitive skills, background knowledge, and self-regulatory processes (e.g., Berninger & Abbott, 2010 ; Berninger et al., 2002 ; Conners, 2009 ; Cain, Oakhill, & Bryant, 2004 ; Compton, Miller, Elleman, & Steacy, 2014 ; Cromley & Azevedo, 2007 ; Graham et al., 2002; Graham et al., 2007 ; Kim, 2015 , 2017 ; Kim & Schatschneider, 2017 ; Kim & Schatschneider, 2018; Vellutino et al., 2007 ). However, as noted earlier, higher order skills such as reading comprehension and written comprehension which draw on a number of knowledge, skills, and factors are likely to be divergent as a construct. Furthermore, demands for reading comprehension and written composition differ. As a production task that involves multiple processes of planning (including generating and organizing ideas), goal setting, translating, monitoring, reviewing, evaluation, and revising (Hayes, 2012; Hayes & Flower, 1980), skilled writing requires regulating one’s attention, decisions, and behaviors throughout these process ( Berninger & Winn, 2006 ; Hayes & Flower, 1980; Hayes, 2012). Therefore, although reading comprehension and written composition draw on a similar set of knowledge and skills (e.g., oral language, self-regulation), the extent to which component skills are required for reading comprehension versus writing tasks might vary, resulting in a weaker relation ( Kim & Graham, 2018 ).
The hypothesis about the interactive nature of relations between reading and writing was not supported in the present study. Instead, our findings indicate a unidirectional relation from reading to writing both at the lexical and discourse levels. Initial status in word reading strongly predicted initial status (.86) and linear growth rate of spelling (.96). In other words, children who had higher word reading in Grade 3 also had higher spelling in Grade 3 and experienced a faster growth rate in spelling. Growth rate in word reading also predicted growth rate in spelling (.22) after accounting for the contribution of initial status in word reading, indicating that children who had faster growth in word reading also had faster growth in spelling. When the contribution of spelling to word reading was examined, initial status in spelling was positively related to word reading slope, but was not statistically significant. When growth rate in spelling was hypothesized to predict growth rate in word reading, the model did not converge. Although the causes of model non-convergence is unclear, overall the present findings indicate that development of word reading facilitates development of spelling skills but not the other way around at least from Grades 3 to 6. The unidirectional relation from word reading to spelling is convergent with a previous longitudinal study from Grades 1 to 4 ( Ahmed et al., 2014 ), but divergent with a meta-analysis reporting a large effect of spelling instruction on word reading (average effect size = .68; Graham & Hebert, 2010).
Furthermore, reading comprehension in Grade 3 fairly strongly predicted writing in Grade 3 (.69). However, neither initial status in reading comprehension (in Grade 3) nor in written composition predicted linear growth in written composition. The relation from reading comprehension to writing is convergent with an earlier study by Ahmed et al. (2014) with younger children, and suggests that knowledge of and experiences with reading comprehension are likely to contribute to written composition, but not the other way around, at least during Grades 3 to 6. This appears to contradict previous findings on the effect of writing instruction on reading (Graham & Hebert, 2010) or the positive effects on reading and writing when instruction explicitly targets both reading and writing ( Graham et al., in press ). These discrepancies might suggest that for writing to transfer to reading at the discourse level, explicit and targeted instruction might be necessary. Although writing acquisition and experiences may help children to think about and to reflect on how information is presented in written texts, which promotes awareness of text structure and text meaning, and, consequently, reading comprehension ( Graham & Harris, 2017 ; Langer & Flihan, 2000 ), these might be beneficial for children who have highly developed meta-cognition or might require instruction that explicitly identifies these aspects to promote transfer of skills between writing and reading comprehension. Future studies are warranted for this speculation.
Limitations and Conclusion
Results of the present findings should be interpreted with the following limitations in mind. First, there was a lack of variance in the linear parameter of reading comprehension as well as in the quadratic parameter of reading comprehension and written composition. These indicate that children in Grades 3 to 6 did not vary in linear growth rate in reading comprehension and quadratic function in reading comprehension and written composition. While these are potentially important findings themselves, these limited the scope of relations that could be estimated in the present study. Measuring a construct (e.g., reading comprehension) using multiple tasks would be beneficial in several aspects, including reduction of measurement error and addressing the issue of zero variance in future studies. Furthermore, previous studies have shown that reading comprehension measures vary in the extent to which they tap into component skills ( Cutting & Scarborough, 2006 ; Keenan, Betjemann, & Olson, 2008 ). Therefore, the extent to which our present findings are influenced by the use of a particular reading comprehension task (i.e., WJ Passage Comprehension) is an open question and requires future work. Second, the foci of the present study were developmental trajectories and reading-writing relations; and thus, an investigation of component skills and their relations to growth trajectories of reading and writing was beyond the scope of the present study. Such an investigation would shed light on shared and unique aspects of reading and writing development (see Kim & Graham, 2018 ). Third, we did not observe the amount or quality of instruction in reading or writing; future research might explore how instruction and interventions mediate growth trajectories. Moreover, variation across classrooms across the grades was not accounted for in the statistical model for its complexity. Finally, our findings should be replicated with different samples of students in terms of both ethnicity, English language proficiency, and free and reduced lunch price status.
In conclusion, we found that linear developmental trajectories describe development of lexical-level literacy skills whereas a nonlinear function describes development of discourse-level literacy skills from Grades 3 to 6. We also found that reading-writing relations are more likely to be from reading to writing at lexical- and discourse levels, at least during these grades. Future longitudinal and experimental investigations are needed to replicate and extend the present study to further reveal similarities and uniqueness of reading versus writing, and the nature of their relations.
Acknowledgments
This research was supported by [masked for blind review]. The authors appreciate participating children, their parents, and teachers and school personnel.
Unstandardized model coefficients for passage comprehension and writing structural equation model
Note . WG3= grade 3 writing, WG4 = grade 4 writing, WG5 = grade 5 writing, WG6 = grade 6 writing; PC = passage comprehension; Int. = intercept; Var./Res. Var. = model variances and residual variances. p -values of 999 are indicative of model coefficients that were assigned a fixed value.
Unstandardized model coefficients for word reading and spelling structural equation model
Note . SG3= grade 3 spelling, SG4 = grade 4 spelling, SG5 = grade 5 spelling, SG6 = grade 6 spelling; SG34 = grade 3–4 latent change score, SG45 = grade 4–5 latent change score, SG56 = grade 5–6; latent change score, WR = word reading; LWID = letter word identification; Int. = intercept; Var./Res. Var. = model variances and residual variances. p -values of 999 are indicative of model coefficients that were assigned a fixed value.
1 The similarities that reading and writing draw on do not indicate that reading and writing are the same or a single construct ( Kim & Graham, 2018 ). Instead, reading and writing differ in demands and thus, in the extent to which they draw on resources. Spelling places greater demands on memory for accurate recall of word specific spelling patterns than does word reading, and word reading and spelling are not likely the same constructs (see Ehri, 2000 for a review; but see Kent et al., 2015; Mehta, Foorman, Branum-Martin, & Taylor, 2005 ). Written composition is also a more self-directed process than reading comprehension, and thus, is likely to draw on self-regulation to a greater extent than for reading comprehension ( Kim & Graham, 2018 ).
2 There is a dip in sample size in Grade 4. This was primarily because a few schools’ decision not to participate in the study during that year with changes in the leadership.
3 An alternative model tested a covariance between reading comprehension and written composition initial status, resulting in a .67 correlation between the constructs.
Contributor Information
Young-Suk Grace Kim, University of California Irvine.
Yaacov Petscher, Florida Center for Reading Research.
Jeanne Wanzek, Vanderbilt University.
Stephanie Al Otaiba, Southern Methodist University.
- Abbott RD, Berninger VW. Structural equation modeling of relationships among developmental skills and writing skills in primary- and intermediate-grade writers. Journal of Educational Psychology. 1993; 85 :478–508. [ Google Scholar ]
- Adams MJ. Beginning to reading: Thinking and learning about print. Cambridge, MA: MIT Press; 1990. [ Google Scholar ]
- Ahmed Y, Wagner RK, Lopez D. Developmental relations between reading and writing at the word, sentence, and text levels: A latent change score analysis. Journal of Educational Psychology. 2014; 106 :419–434. [ PMC free article ] [ PubMed ] [ Google Scholar ]
- Apel K, Wilson-Fowler EB, Brimo D, Perrin NA. Metalinguistic contributions to reading and spelling in second and third grade students. Reading and Writing: An Interdisciplinary Journal. 2012; 25 :1283–1305. [ Google Scholar ]
- Bamberg B. What makes a text coherent? College Composition and Communication. 1983; 34 :417–429. [ Google Scholar ]
- Bentler PM. Comparative fit indexes in structural models. Psychological Bulletin. 1990; 107 :238–246. [ PubMed ] [ Google Scholar ]
- Bentler PM, Bonett DG. Significance tests and goodness of fit in the analysis of covariance structures. Psychological Bulletin. 1980; 88 :588. [ Google Scholar ]
- Berninger VW, Abbott RD. Listening comprehension, oral expression, reading comprehension, and written expression: Related yet unique language systems in Grades 1, 3, 5, and 7. Journal of Educational Psychology. 2010; 102 :635–651. doi: 10.1037/a0019319. [ PMC free article ] [ PubMed ] [ CrossRef ] [ Google Scholar ]
- Berninger VW, Abbott RD, Abbott SP, Graham S, Richards T. Writing and reading: Connections between language by hand and language by eye. Journal of Learning Disabilities. 2002; 35 :39–56. doi: 10.1177/002221940203500104. [ PubMed ] [ CrossRef ] [ Google Scholar ]
- Berninger VW, Abbott RD, Rogan L, Reed E, Abbott S, Brooks A, … Graham S. Teaching spelling to children with specific learning disabilities: The mind’s ear and eye beat the computer or pencil. Learning Disability Quarterly. 1998; 21 :106–122. [ Google Scholar ]
- Berninger V, Amtmann D. Preventing written expression disabilities through early and continuing assessment and intervention for handwriting and/or spelling problems: Research into practice. In: Swanson H, Harris K, Graham S, editors. Handbook of Learning Disabilities. New York: The Guilford Press; 2003. pp. 323–344. [ Google Scholar ]
- Berninger VW, Swanson HL. Children’s writing; toward a process theory of the development of skilled writing. In: Butterfield E, editor. Children’s writing: Toward a process theory of development of skilled writing. Greenwich, CT: JAI Press; 1994. pp. 57–81. Reproduced in The Learning and Teaching of Reading and Writing (by R. Stainthorp). Wiley, 2006. [ Google Scholar ]
- Berninger VW, Winn WD. Implications of advancements in brain research and technology for writing development, writing instruction, and educational evolution. In: MacArthur C, Graham S, Fitzgerald J, editors. Handbook of writing research. New York, NY: Guilford Press; 2006. pp. 96–114. [ Google Scholar ]
- Bourassa D, Treiman R. Linguistic foundations of spelling development. In: Wyse D, Andrews R, Hoffman J, editors. Routledge international handbook of English, language and literacy teaching. London, UK: Routledge; 2009. pp. 182–192. [ Google Scholar ]
- Bourassa DC, Treiman R, Kessler B. Use of morphology in spelling by children with dyslexia and typically developing children. Memory & Cognition. 2006; 34 :703–714. [ PubMed ] [ Google Scholar ]
- Browne MW, Cudeck R. Alternative ways of assessing model fit. Sociological Methods & Research. 1992; 21 :230–258. [ Google Scholar ]
- Cain K, Oakhill JV. Inference ability and its relation to comprehension failure in young children. Reading and Writing. 1999; 11 :489–503. [ Google Scholar ]
- Cain K, Oakhill J, Bryant P. Children’s reading comprehension ability: Concurrent prediction by working memory, verbal ability, and component skills. Journal of Educational Psychology. 2004; 96 :31–42. [ Google Scholar ]
- Carlisle JF, Katz LA. Effects of word and morpheme familiarity on reading of derived words. Reading and Writing: An Interdisciplinary Journal. 2006; 19 :669–694. [ Google Scholar ]
- Compton DL, Miller AC, Elleman AM, Steacy LM. Have we forsaken reading theory in the name of “quick fix” interventions for children with reading disability? Scientific Studies of Reading. 2014; 18 :55–73. [ Google Scholar ]
- Conners FA. Attentional control and the simple view of reading. Reading and Writing: An Interdisciplinary Journal. 2009; 22 :591–613. [ Google Scholar ]
- Cromley J, Azevedo R. Testing and refining the direct and inferential mediation model of reading comprehension. Journal of Educational Psychology. 2007; 99 :311–325. [ Google Scholar ]
- Cutting LE, Scarborough HS. Prediction of reading comprehension: Relative contributions of word recognition, language proficiency, and other cognitive skills can depend o how comprehension is measured. Scientific Studies of Reading. 2006; 10 :277, 299. doi: 10.1207/s1532799xssr1003_5. [ CrossRef ] [ Google Scholar ]
- Daneman M, Carpenter PA. Individual differences in working memory and reading. Journal of verbal learning and verbal behavior. 1980; 19 :450–466. [ Google Scholar ]
- Deacon SH, Bryant PE. What young children do and do not know about the spelling of inflections and derivations. Developmental Science. 2005; 8 :583–594. [ PubMed ] [ Google Scholar ]
- Ehri LC. Learning to read and learning to spell: Two sides of a coin. Topics in Language Disorders. 2000; 20 (3):19–36. [ Google Scholar ]
- Ehri LC, Satlow E, Gaskins I. Grapho-phonemic enrichment strengthens keyword analysis instruction for struggling young readers. Reading & Writing Quarterly. 2009; 25 :162–191. [ Google Scholar ]
- Elleman AM, Lindo EJ, Morphy P, Compton DL. The impact of vocabulary instruction on passage-level comprehension of school-age children: A meta-analysis. Journal of Research on Educational Effectiveness. 2009; 2 :1–44. [ Google Scholar ]
- Enders CK. Applied missing data analysis. New York, NY: Guilford Press; 2010. [ Google Scholar ]
- Fitzgerald J, Shanahan T. Reading and writing relations and their development. Educational Psychologist. 2000; 35 :39–50. [ Google Scholar ]
- Graham S, Berninger VW, Abbott RD, Abbott SP, Whitaker D. Role of mechanics in composing of elementary school students: A new methodological approach. Journal of Educational Psychology. 1997; 89 :170–182. [ Google Scholar ]
- Graham S, Harris KR. Reading and writing connections: How writing can build better readers (and vice versa) In: Ng C, Bartlett B, editors. Improving reading and reading engagement in the 21 st century. Singapore: Springer; 2017. pp. 333–350. [ Google Scholar ]
- Graham S, Berninger VW, Fan W. The structural relationship between writing attitude and writing achievement in first and third grade students. Contemporary Educational Psychology. 2007; 32 :516–536. doi: 10.1016/j.cedpsych.2007.01.002. [ CrossRef ] [ Google Scholar ]
- Graham S, Liu X, Aitken A, Ng C, Bartlett B, Harris KR, Holzapfel J. Effectiveness of literacy programs balancing reading and writing instruction: A meta-analysis. Reading Research Quarterly in press. [ Google Scholar ]
- Hayes JR, Chenoweth NA. Working memory in an editing task. Written communication. 2007; 24 :283–294. [ Google Scholar ]
- Hooper D, Coughlan J, Mullen M. Structural equation modelling: Guidelines for determining model fit. Electronic Journal of Business Research Methods. 2008; 6 :53–60. [ Google Scholar ]
- Hooper SR, Swartz CW, Wakely MB, de Kruif REL, Montgomery JW. Executive functions in elementary school children with and without problems in written expression. Journal of Learning Disabilities. 2002; 35 :57–68. doi: 10.1177/002221940203500105. [ PubMed ] [ CrossRef ] [ Google Scholar ]
- Juel C, Griffith PL, Gough PB. Acquisition of literacy: A longitudinal study of children in first and second grade. Journal of Educational Psychology. 1986; 78 :243–255. [ Google Scholar ]
- Keenan JM, Betjemann RS, Olson RK. Reading comprehension tests vary in the skills they assess: Differential dependence on decoding and oral comprehension. Scientific Studies of Reading. 2008; 12 :281–300. doi: 10.1080/10888430802132279. [ CrossRef ] [ Google Scholar ]
- Kellogg RT. A model of working memory in writing. In: Levy CM, Randell S, editors. The science of writing: Theories of, methods, individual differences, and applications. Mahwah, NJ: Erlbaum; 1999. pp. 57–71. [ Google Scholar ]
- Kent S, Wanzek J, Petscher Y, Al Otaiba S, Kim YS. Writing fluency and quality in kindergarten and first grade: The role of attention, reading, transcription, and oral language. Reading and Writing: An Interdisciplinary Journal. 2014; 27 :1163–1188. doi: 10.1007/s11145-013-9480-1. [ PMC free article ] [ PubMed ] [ CrossRef ] [ Google Scholar ]
- Kieffer M. Converging trajectories: Reading growth in language minority learners and their classmates, kindergarten to grade 8. American Educational Research Journal. 2011; 48 :1187–1225. doi: 10.3102/0002831211419490. [ CrossRef ] [ Google Scholar ]
- Kim YS. Considering linguistic and orthographic features in early literacy acquisition: Evidence from Korean. Contemporary Educational Psychology. 2011; 36 :177–189. doi: 10.1016/j.cedpsych.2010.06.003. [ CrossRef ] [ Google Scholar ]
- Kim YS. Language and cognitive predictors of text comprehension: Evidence from multivariate analysis. Child Development. 2015; 86 :128–144. doi: 10.1111/cdev.12293. [ PubMed ] [ CrossRef ] [ Google Scholar ]
- Kim YSG. Why the simple view of reading is not simplistic: Unpacking the simple view of reading using a direct and indirect effect model of reading (DIER) Scientific Studies of Reading. 2017; 21 :310–333. doi: 10.1080/10888438.2017.1291643. [ CrossRef ] [ Google Scholar ]
- Kim YS, Al Otaiba S, Puranik C, Folsom JS, Greulich L, Wagner RK. Componential skills of beginning writing: An exploratory study. Learning and Individual Differences. 2011; 21 :517–525. doi: 10.1016/j.lindif.2011.06.004. [ PMC free article ] [ PubMed ] [ CrossRef ] [ Google Scholar ]
- Kim YS, Al Otaiba S, Puranik C, Folsom JS, Greulich L. The contributions of vocabulary and letter writing automaticity to word reading and spelling for kindergartners. Reading and Writing: An Interdisciplinary Journal. 2014; 27 :237–253. doi: 10.1007/s11145-013-9440-9. [ PMC free article ] [ PubMed ] [ CrossRef ] [ Google Scholar ]
- Kim YS, Al Otaiba S, Wanzek J, Gatlin B. Towards an understanding of dimension, predictors, and gender gaps in written composition. Journal of Educational Psychology. 2015; 107 :79–95. doi: 10.1037/a0037210. [ PMC free article ] [ PubMed ] [ CrossRef ] [ Google Scholar ]
- Kim YS, Apel K, Al Otaiba S. The relation of linguistic awareness and vocabulary to word reading and spelling for first-grade students participating in response to instruction. Language, Speech, and Hearing Services in Schools. 2013; 44 :1–11. doi: 10.1044/0161-1461(2013/12-0013). [ PMC free article ] [ PubMed ] [ CrossRef ] [ Google Scholar ]
- Kim Y-SG, Graham S. Integrating reading and writing: Interactive dynamic literacy model. 2018 Manuscript submitted for publication. [ Google Scholar ]
- Kim YSG, Petscher Y, Park Y. Examining word factors and child factors for acquisition of conditional sound-spelling consistencies: A longitudinal study. Scientific Studies of Reading. 2016; 20 :265–282. doi: 10.1080/10888438.2016.1162794. [ CrossRef ] [ Google Scholar ]
- Kim YS, Phillips B. Cognitive correlates of listening comprehension. Reading Research Quarterly. 2014; 49 :269–281. doi: 10.1002/rrq.74. [ CrossRef ] [ Google Scholar ]
- Kim YS, Puranik C, Al Otaiba S. Developmental trajectories of writing skills in first grade: Examining the effects of SES and language and/or speech impairments. Elementary School Journal. 2015; 115 :593– 613. doi:0013-5984/2015/11504-0008. [ PMC free article ] [ PubMed ] [ Google Scholar ]
- Kim YSG, Schatschneider C. Expanding the developmental models of writing: A direct and indirect effects model of developmental writing (DIEW) Journal of Educational Psychology. 2017; 109 :35–50. doi: 10.1037/edu0000129. [ PMC free article ] [ PubMed ] [ CrossRef ] [ Google Scholar ]
- Kim YSG, Wagner RK. Text (Oral) reading fluency as a construct in reading development: An investigation of its mediating role for children from Grades 1 to 4. Scientific Studies of Reading. 2015; 19 :224–242. doi: 10.1080/10888438.2015.1007375. [ PMC free article ] [ PubMed ] [ CrossRef ] [ Google Scholar ]
- Kintsch W. The use of knowledge in discourse processing: A construction-integration model. Psychological Review. 1988; 95 :163–182. doi: 10.1037/0033-295X.95.2.163. [ PubMed ] [ CrossRef ] [ Google Scholar ]
- Langer JA, Flihan S. Writing and reading relationships: Constructive tasks. In: Indrisano R, Squire JR, editors. Writing and Research/Theory/Practice. Newark, DE: International Reading Association; 2000. pp. 112–139. [ Google Scholar ]
- Lerkkanen M, Rasku-Puttonen H, Aunola K, Nurmi J. The developmental dynamics of literacy skills during the first grade. Educational Psychology. 2004; 24 :793–810. [ Google Scholar ]
- Lervag A, Hulme C. Predicting the growth of early spelling skills: Are there heterogeneous developmental trajectories? Scientific Studies of Reading. 2010; 14 :485–513. [ Google Scholar ]
- Limpo T, Alves RA. Modelling writing development: Contribution of transcription and self-regulation to Portuguese students’ text generation quality. Journal of Educational Psychology. 2013; 105 :401–413. [ Google Scholar ]
- Little RJ. A test of missing completely at random for multivariate data with missing values. Journal of the American Statistical Association. 1988; 83 :1198–1202. [ Google Scholar ]
- McArdle JJ. Latent variable modeling of differences and changes with longitudinal data. Annual Review of Psychology. 2009; 60 :577–605. [ PubMed ] [ Google Scholar ]
- McCoach DB, O’Connell AA, Reis SM, Levitt HA. Growing readers: A hierarchical linear model of children’s reading growth during the first 2 years of school. Journal of Educational Psychology. 2006; 98 :14–28. [ Google Scholar ]
- McGrew KS, Schrank FA, Woodcock RW. Technical manual: Woodcock–Johnson III Normative Update. Rolling Meadows, IL: Riverside; 2007. [ Google Scholar ]
- McMaster KL, Du X, Pétursdôttir AL. Technical features of curriculum-based measures for beginning writers. Journal of Learning Disabilities. 2009; 42 :41–60. doi: 10.1177/0022219408326212. [ PubMed ] [ CrossRef ] [ Google Scholar ]
- Mehta PD, Foorman BR, Branum-Martin L, Taylor WP. Literacy as a Unidimensional Multilevel Construct: Validation, Sources of Influence, and Implications in a Longitudinal Study in Grades 1 to 4. Scientific Studies of Reading. 2005; 9 :85–116. doi: 10.1207/s1532. [ CrossRef ] [ Google Scholar ]
- Meredith W, Tisak J. Latent curve analysis. Psychometrika. 1990; 55 :107–122. [ Google Scholar ]
- Morgan PL, Farkas G, Wu Q. Kindergarten children’s growth trajectories in reading and mathematics: Who falls increasingly behind? Journal of Learning Disabilities. 2011; 44 :472–488. [ PMC free article ] [ PubMed ] [ Google Scholar ]
- Muthén B, Muthén L. Mplus user’s guide. 7. Los Angeles, CA: 1998–2013. [ Google Scholar ]
- Nagy W, Berninger V, Abbott R. Contributions of morphology beyond phonology to literacy outcomes of upper elementary and middle school students. Journal of Educational Psychology. 2006; 98 :134–147. [ Google Scholar ]
- National Institute of Child Health and Human Development. Report of the National Reading Panel. Teaching children to read: An evidence-based assessment of the scientific research literature on reading and its implications for reading instruction. Washington, DC: U.S. Government Printing Office; 2000. (NIH Publication No. 00-4769) [ Google Scholar ]
- Northwest Regional Educational Laboratory. 6 + 1 trait writing. 2011 Retrieved from http://educationnorthwest.org/traits .
- Oakhill J, Cain K. The precursors of reading comprehension and word reading in young readers: Evidence from a four-year longitudinal study. Scientific Studies of Reading. 2012; 16 :91–121. [ Google Scholar ]
- Olinghouse NG. Student- and instruction-level predictors of narrative writing in third-grade students. Reading and Writing: An Interdisciplinary Journal. 2008; 21 :3–26. doi: 10.1007/s11145-007-9062-1. [ CrossRef ] [ Google Scholar ]
- Petscher Y, Quinn JM, Wagner RK. Modeling the co-development of correlated processes with longitudinal and cross-construct effects. Developmental Psychology. 2016; 52 :1690. [ PMC free article ] [ PubMed ] [ Google Scholar ]
- Pressley M, Ghatala ES. Self-regulated learning: Monitoring learning from text. Educational Psychologist. 1990; 25 :19–33. [ Google Scholar ]
- Shanahan T. Relations among oral language, reading, and writing development. In: MacArthur CA, Graham S, Fitzgerald J, editors. Handbook of writing research. New York, NY: Guilford Press; 2006. pp. 171–183. [ Google Scholar ]
- Stanovich Keith E. Matthew effects in reading: Some consequences of individual differences in the acquisition of literacy. Reading Research Quarterly. 1986; 22 :360–407. [ Google Scholar ]
- Treiman R. Beginning to spell: A study of first-grade children. New York: Oxford University Press; 1993. [ Google Scholar ]
- Vellutino FR, Tunmer WE, Jaccard JJ, Chen R. Components of reading ability: Multivariate evidence for a convergent skills model of reading development. Scientific Studies of Reading. 2007; 11 :3–32. doi: 10.1080/10888430709336632. [ CrossRef ] [ Google Scholar ]
- Wechsler D. Wechsler Individual Achievement Test. 3. San Antonio, TX: Pearson; 2009. [ Google Scholar ]
- Woodcock RW, McGrew KS, Mather N. Woodcock–Johnson III Tests of Achievement. Itasca, IL: Riverside; 2001. [ Google Scholar ]
Thank you for visiting nature.com. You are using a browser version with limited support for CSS. To obtain the best experience, we recommend you use a more up to date browser (or turn off compatibility mode in Internet Explorer). In the meantime, to ensure continued support, we are displaying the site without styles and JavaScript.
- View all journals
- My Account Login
- Explore content
- About the journal
- Publish with us
- Sign up for alerts
- Open access
- Published: 30 October 2023
A large-scale comparison of human-written versus ChatGPT-generated essays
- Steffen Herbold 1 ,
- Annette Hautli-Janisz 1 ,
- Ute Heuer 1 ,
- Zlata Kikteva 1 &
- Alexander Trautsch 1
Scientific Reports volume 13 , Article number: 18617 ( 2023 ) Cite this article
17k Accesses
12 Citations
94 Altmetric
Metrics details
- Computer science
- Information technology
ChatGPT and similar generative AI models have attracted hundreds of millions of users and have become part of the public discourse. Many believe that such models will disrupt society and lead to significant changes in the education system and information generation. So far, this belief is based on either colloquial evidence or benchmarks from the owners of the models—both lack scientific rigor. We systematically assess the quality of AI-generated content through a large-scale study comparing human-written versus ChatGPT-generated argumentative student essays. We use essays that were rated by a large number of human experts (teachers). We augment the analysis by considering a set of linguistic characteristics of the generated essays. Our results demonstrate that ChatGPT generates essays that are rated higher regarding quality than human-written essays. The writing style of the AI models exhibits linguistic characteristics that are different from those of the human-written essays. Since the technology is readily available, we believe that educators must act immediately. We must re-invent homework and develop teaching concepts that utilize these AI models in the same way as math utilizes the calculator: teach the general concepts first and then use AI tools to free up time for other learning objectives.
Similar content being viewed by others
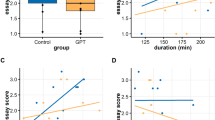
ChatGPT-3.5 as writing assistance in students’ essays
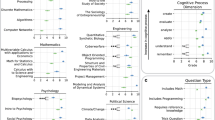
Perception, performance, and detectability of conversational artificial intelligence across 32 university courses
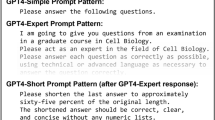
The model student: GPT-4 performance on graduate biomedical science exams
Introduction.
The massive uptake in the development and deployment of large-scale Natural Language Generation (NLG) systems in recent months has yielded an almost unprecedented worldwide discussion of the future of society. The ChatGPT service which serves as Web front-end to GPT-3.5 1 and GPT-4 was the fastest-growing service in history to break the 100 million user milestone in January and had 1 billion visits by February 2023 2 .
Driven by the upheaval that is particularly anticipated for education 3 and knowledge transfer for future generations, we conduct the first independent, systematic study of AI-generated language content that is typically dealt with in high-school education: argumentative essays, i.e. essays in which students discuss a position on a controversial topic by collecting and reflecting on evidence (e.g. ‘Should students be taught to cooperate or compete?’). Learning to write such essays is a crucial aspect of education, as students learn to systematically assess and reflect on a problem from different perspectives. Understanding the capability of generative AI to perform this task increases our understanding of the skills of the models, as well as of the challenges educators face when it comes to teaching this crucial skill. While there is a multitude of individual examples and anecdotal evidence for the quality of AI-generated content in this genre (e.g. 4 ) this paper is the first to systematically assess the quality of human-written and AI-generated argumentative texts across different versions of ChatGPT 5 . We use a fine-grained essay quality scoring rubric based on content and language mastery and employ a significant pool of domain experts, i.e. high school teachers across disciplines, to perform the evaluation. Using computational linguistic methods and rigorous statistical analysis, we arrive at several key findings:
AI models generate significantly higher-quality argumentative essays than the users of an essay-writing online forum frequented by German high-school students across all criteria in our scoring rubric.
ChatGPT-4 (ChatGPT web interface with the GPT-4 model) significantly outperforms ChatGPT-3 (ChatGPT web interface with the GPT-3.5 default model) with respect to logical structure, language complexity, vocabulary richness and text linking.
Writing styles between humans and generative AI models differ significantly: for instance, the GPT models use more nominalizations and have higher sentence complexity (signaling more complex, ‘scientific’, language), whereas the students make more use of modal and epistemic constructions (which tend to convey speaker attitude).
The linguistic diversity of the NLG models seems to be improving over time: while ChatGPT-3 still has a significantly lower linguistic diversity than humans, ChatGPT-4 has a significantly higher diversity than the students.
Our work goes significantly beyond existing benchmarks. While OpenAI’s technical report on GPT-4 6 presents some benchmarks, their evaluation lacks scientific rigor: it fails to provide vital information like the agreement between raters, does not report on details regarding the criteria for assessment or to what extent and how a statistical analysis was conducted for a larger sample of essays. In contrast, our benchmark provides the first (statistically) rigorous and systematic study of essay quality, paired with a computational linguistic analysis of the language employed by humans and two different versions of ChatGPT, offering a glance at how these NLG models develop over time. While our work is focused on argumentative essays in education, the genre is also relevant beyond education. In general, studying argumentative essays is one important aspect to understand how good generative AI models are at conveying arguments and, consequently, persuasive writing in general.
Related work
Natural language generation.
The recent interest in generative AI models can be largely attributed to the public release of ChatGPT, a public interface in the form of an interactive chat based on the InstructGPT 1 model, more commonly referred to as GPT-3.5. In comparison to the original GPT-3 7 and other similar generative large language models based on the transformer architecture like GPT-J 8 , this model was not trained in a purely self-supervised manner (e.g. through masked language modeling). Instead, a pipeline that involved human-written content was used to fine-tune the model and improve the quality of the outputs to both mitigate biases and safety issues, as well as make the generated text more similar to text written by humans. Such models are referred to as Fine-tuned LAnguage Nets (FLANs). For details on their training, we refer to the literature 9 . Notably, this process was recently reproduced with publicly available models such as Alpaca 10 and Dolly (i.e. the complete models can be downloaded and not just accessed through an API). However, we can only assume that a similar process was used for the training of GPT-4 since the paper by OpenAI does not include any details on model training.
Testing of the language competency of large-scale NLG systems has only recently started. Cai et al. 11 show that ChatGPT reuses sentence structure, accesses the intended meaning of an ambiguous word, and identifies the thematic structure of a verb and its arguments, replicating human language use. Mahowald 12 compares ChatGPT’s acceptability judgments to human judgments on the Article + Adjective + Numeral + Noun construction in English. Dentella et al. 13 show that ChatGPT-3 fails to understand low-frequent grammatical constructions like complex nested hierarchies and self-embeddings. In another recent line of research, the structure of automatically generated language is evaluated. Guo et al. 14 show that in question-answer scenarios, ChatGPT-3 uses different linguistic devices than humans. Zhao et al. 15 show that ChatGPT generates longer and more diverse responses when the user is in an apparently negative emotional state.
Given that we aim to identify certain linguistic characteristics of human-written versus AI-generated content, we also draw on related work in the field of linguistic fingerprinting, which assumes that each human has a unique way of using language to express themselves, i.e. the linguistic means that are employed to communicate thoughts, opinions and ideas differ between humans. That these properties can be identified with computational linguistic means has been showcased across different tasks: the computation of a linguistic fingerprint allows to distinguish authors of literary works 16 , the identification of speaker profiles in large public debates 17 , 18 , 19 , 20 and the provision of data for forensic voice comparison in broadcast debates 21 , 22 . For educational purposes, linguistic features are used to measure essay readability 23 , essay cohesion 24 and language performance scores for essay grading 25 . Integrating linguistic fingerprints also yields performance advantages for classification tasks, for instance in predicting user opinion 26 , 27 and identifying individual users 28 .
Limitations of OpenAIs ChatGPT evaluations
OpenAI published a discussion of the model’s performance of several tasks, including Advanced Placement (AP) classes within the US educational system 6 . The subjects used in performance evaluation are diverse and include arts, history, English literature, calculus, statistics, physics, chemistry, economics, and US politics. While the models achieved good or very good marks in most subjects, they did not perform well in English literature. GPT-3.5 also experienced problems with chemistry, macroeconomics, physics, and statistics. While the overall results are impressive, there are several significant issues: firstly, the conflict of interest of the model’s owners poses a problem for the performance interpretation. Secondly, there are issues with the soundness of the assessment beyond the conflict of interest, which make the generalizability of the results hard to assess with respect to the models’ capability to write essays. Notably, the AP exams combine multiple-choice questions with free-text answers. Only the aggregated scores are publicly available. To the best of our knowledge, neither the generated free-text answers, their overall assessment, nor their assessment given specific criteria from the used judgment rubric are published. Thirdly, while the paper states that 1–2 qualified third-party contractors participated in the rating of the free-text answers, it is unclear how often multiple ratings were generated for the same answer and what was the agreement between them. This lack of information hinders a scientifically sound judgement regarding the capabilities of these models in general, but also specifically for essays. Lastly, the owners of the model conducted their study in a few-shot prompt setting, where they gave the models a very structured template as well as an example of a human-written high-quality essay to guide the generation of the answers. This further fine-tuning of what the models generate could have also influenced the output. The results published by the owners go beyond the AP courses which are directly comparable to our work and also consider other student assessments like Graduate Record Examinations (GREs). However, these evaluations suffer from the same problems with the scientific rigor as the AP classes.
Scientific assessment of ChatGPT
Researchers across the globe are currently assessing the individual capabilities of these models with greater scientific rigor. We note that due to the recency and speed of these developments, the hereafter discussed literature has mostly only been published as pre-prints and has not yet been peer-reviewed. In addition to the above issues concretely related to the assessment of the capabilities to generate student essays, it is also worth noting that there are likely large problems with the trustworthiness of evaluations, because of data contamination, i.e. because the benchmark tasks are part of the training of the model, which enables memorization. For example, Aiyappa et al. 29 find evidence that this is likely the case for benchmark results regarding NLP tasks. This complicates the effort by researchers to assess the capabilities of the models beyond memorization.
Nevertheless, the first assessment results are already available – though mostly focused on ChatGPT-3 and not yet ChatGPT-4. Closest to our work is a study by Yeadon et al. 30 , who also investigate ChatGPT-3 performance when writing essays. They grade essays generated by ChatGPT-3 for five physics questions based on criteria that cover academic content, appreciation of the underlying physics, grasp of subject material, addressing the topic, and writing style. For each question, ten essays were generated and rated independently by five researchers. While the sample size precludes a statistical assessment, the results demonstrate that the AI model is capable of writing high-quality physics essays, but that the quality varies in a manner similar to human-written essays.
Guo et al. 14 create a set of free-text question answering tasks based on data they collected from the internet, e.g. question answering from Reddit. The authors then sample thirty triplets of a question, a human answer, and a ChatGPT-3 generated answer and ask human raters to assess if they can detect which was written by a human, and which was written by an AI. While this approach does not directly assess the quality of the output, it serves as a Turing test 31 designed to evaluate whether humans can distinguish between human- and AI-produced output. The results indicate that humans are in fact able to distinguish between the outputs when presented with a pair of answers. Humans familiar with ChatGPT are also able to identify over 80% of AI-generated answers without seeing a human answer in comparison. However, humans who are not yet familiar with ChatGPT-3 are not capable of identifying AI-written answers about 50% of the time. Moreover, the authors also find that the AI-generated outputs are deemed to be more helpful than the human answers in slightly more than half of the cases. This suggests that the strong results from OpenAI’s own benchmarks regarding the capabilities to generate free-text answers generalize beyond the benchmarks.
There are, however, some indicators that the benchmarks may be overly optimistic in their assessment of the model’s capabilities. For example, Kortemeyer 32 conducts a case study to assess how well ChatGPT-3 would perform in a physics class, simulating the tasks that students need to complete as part of the course: answer multiple-choice questions, do homework assignments, ask questions during a lesson, complete programming exercises, and write exams with free-text questions. Notably, ChatGPT-3 was allowed to interact with the instructor for many of the tasks, allowing for multiple attempts as well as feedback on preliminary solutions. The experiment shows that ChatGPT-3’s performance is in many aspects similar to that of the beginning learners and that the model makes similar mistakes, such as omitting units or simply plugging in results from equations. Overall, the AI would have passed the course with a low score of 1.5 out of 4.0. Similarly, Kung et al. 33 study the performance of ChatGPT-3 in the United States Medical Licensing Exam (USMLE) and find that the model performs at or near the passing threshold. Their assessment is a bit more optimistic than Kortemeyer’s as they state that this level of performance, comprehensible reasoning and valid clinical insights suggest that models such as ChatGPT may potentially assist human learning in clinical decision making.
Frieder et al. 34 evaluate the capabilities of ChatGPT-3 in solving graduate-level mathematical tasks. They find that while ChatGPT-3 seems to have some mathematical understanding, its level is well below that of an average student and in most cases is not sufficient to pass exams. Yuan et al. 35 consider the arithmetic abilities of language models, including ChatGPT-3 and ChatGPT-4. They find that they exhibit the best performance among other currently available language models (incl. Llama 36 , FLAN-T5 37 , and Bloom 38 ). However, the accuracy of basic arithmetic tasks is still only at 83% when considering correctness to the degree of \(10^{-3}\) , i.e. such models are still not capable of functioning reliably as calculators. In a slightly satiric, yet insightful take, Spencer et al. 39 assess how a scientific paper on gamma-ray astrophysics would look like, if it were written largely with the assistance of ChatGPT-3. They find that while the language capabilities are good and the model is capable of generating equations, the arguments are often flawed and the references to scientific literature are full of hallucinations.
The general reasoning skills of the models may also not be at the level expected from the benchmarks. For example, Cherian et al. 40 evaluate how well ChatGPT-3 performs on eleven puzzles that second graders should be able to solve and find that ChatGPT is only able to solve them on average in 36.4% of attempts, whereas the second graders achieve a mean of 60.4%. However, their sample size is very small and the problem was posed as a multiple-choice question answering problem, which cannot be directly compared to the NLG we consider.
Research gap
Within this article, we address an important part of the current research gap regarding the capabilities of ChatGPT (and similar technologies), guided by the following research questions:
RQ1: How good is ChatGPT based on GPT-3 and GPT-4 at writing argumentative student essays?
RQ2: How do AI-generated essays compare to essays written by students?
RQ3: What are linguistic devices that are characteristic of student versus AI-generated content?
We study these aspects with the help of a large group of teaching professionals who systematically assess a large corpus of student essays. To the best of our knowledge, this is the first large-scale, independent scientific assessment of ChatGPT (or similar models) of this kind. Answering these questions is crucial to understanding the impact of ChatGPT on the future of education.
Materials and methods
The essay topics originate from a corpus of argumentative essays in the field of argument mining 41 . Argumentative essays require students to think critically about a topic and use evidence to establish a position on the topic in a concise manner. The corpus features essays for 90 topics from Essay Forum 42 , an active community for providing writing feedback on different kinds of text and is frequented by high-school students to get feedback from native speakers on their essay-writing capabilities. Information about the age of the writers is not available, but the topics indicate that the essays were written in grades 11–13, indicating that the authors were likely at least 16. Topics range from ‘Should students be taught to cooperate or to compete?’ to ‘Will newspapers become a thing of the past?’. In the corpus, each topic features one human-written essay uploaded and discussed in the forum. The students who wrote the essays are not native speakers. The average length of these essays is 19 sentences with 388 tokens (an average of 2.089 characters) and will be termed ‘student essays’ in the remainder of the paper.
For the present study, we use the topics from Stab and Gurevych 41 and prompt ChatGPT with ‘Write an essay with about 200 words on “[ topic ]”’ to receive automatically-generated essays from the ChatGPT-3 and ChatGPT-4 versions from 22 March 2023 (‘ChatGPT-3 essays’, ‘ChatGPT-4 essays’). No additional prompts for getting the responses were used, i.e. the data was created with a basic prompt in a zero-shot scenario. This is in contrast to the benchmarks by OpenAI, who used an engineered prompt in a few-shot scenario to guide the generation of essays. We note that we decided to ask for 200 words because we noticed a tendency to generate essays that are longer than the desired length by ChatGPT. A prompt asking for 300 words typically yielded essays with more than 400 words. Thus, using the shorter length of 200, we prevent a potential advantage for ChatGPT through longer essays, and instead err on the side of brevity. Similar to the evaluations of free-text answers by OpenAI, we did not consider multiple configurations of the model due to the effort required to obtain human judgments. For the same reason, our data is restricted to ChatGPT and does not include other models available at that time, e.g. Alpaca. We use the browser versions of the tools because we consider this to be a more realistic scenario than using the API. Table 1 below shows the core statistics of the resulting dataset. Supplemental material S1 shows examples for essays from the data set.
Annotation study
Study participants.
The participants had registered for a two-hour online training entitled ‘ChatGPT – Challenges and Opportunities’ conducted by the authors of this paper as a means to provide teachers with some of the technological background of NLG systems in general and ChatGPT in particular. Only teachers permanently employed at secondary schools were allowed to register for this training. Focusing on these experts alone allows us to receive meaningful results as those participants have a wide range of experience in assessing students’ writing. A total of 139 teachers registered for the training, 129 of them teach at grammar schools, and only 10 teachers hold a position at other secondary schools. About half of the registered teachers (68 teachers) have been in service for many years and have successfully applied for promotion. For data protection reasons, we do not know the subject combinations of the registered teachers. We only know that a variety of subjects are represented, including languages (English, French and German), religion/ethics, and science. Supplemental material S5 provides some general information regarding German teacher qualifications.
The training began with an online lecture followed by a discussion phase. Teachers were given an overview of language models and basic information on how ChatGPT was developed. After about 45 minutes, the teachers received a both written and oral explanation of the questionnaire at the core of our study (see Supplementary material S3 ) and were informed that they had 30 minutes to finish the study tasks. The explanation included information on how the data was obtained, why we collect the self-assessment, and how we chose the criteria for the rating of the essays, the overall goal of our research, and a walk-through of the questionnaire. Participation in the questionnaire was voluntary and did not affect the awarding of a training certificate. We further informed participants that all data was collected anonymously and that we would have no way of identifying who participated in the questionnaire. We orally informed participants that they consent to the use of the provided ratings for our research by participating in the survey.
Once these instructions were provided orally and in writing, the link to the online form was given to the participants. The online form was running on a local server that did not log any information that could identify the participants (e.g. IP address) to ensure anonymity. As per instructions, consent for participation was given by using the online form. Due to the full anonymity, we could by definition not document who exactly provided the consent. This was implemented as further insurance that non-participation could not possibly affect being awarded the training certificate.
About 20% of the training participants did not take part in the questionnaire study, the remaining participants consented based on the information provided and participated in the rating of essays. After the questionnaire, we continued with an online lecture on the opportunities of using ChatGPT for teaching as well as AI beyond chatbots. The study protocol was reviewed and approved by the Research Ethics Committee of the University of Passau. We further confirm that our study protocol is in accordance with all relevant guidelines.
Questionnaire
The questionnaire consists of three parts: first, a brief self-assessment regarding the English skills of the participants which is based on the Common European Framework of Reference for Languages (CEFR) 43 . We have six levels ranging from ‘comparable to a native speaker’ to ‘some basic skills’ (see supplementary material S3 ). Then each participant was shown six essays. The participants were only shown the generated text and were not provided with information on whether the text was human-written or AI-generated.
The questionnaire covers the seven categories relevant for essay assessment shown below (for details see supplementary material S3 ):
Topic and completeness
Logic and composition
Expressiveness and comprehensiveness
Language mastery
Vocabulary and text linking
Language constructs
These categories are used as guidelines for essay assessment 44 established by the Ministry for Education of Lower Saxony, Germany. For each criterion, a seven-point Likert scale with scores from zero to six is defined, where zero is the worst score (e.g. no relation to the topic) and six is the best score (e.g. addressed the topic to a special degree). The questionnaire included a written description as guidance for the scoring.
After rating each essay, the participants were also asked to self-assess their confidence in the ratings. We used a five-point Likert scale based on the criteria for the self-assessment of peer-review scores from the Association for Computational Linguistics (ACL). Once a participant finished rating the six essays, they were shown a summary of their ratings, as well as the individual ratings for each of their essays and the information on how the essay was generated.
Computational linguistic analysis
In order to further explore and compare the quality of the essays written by students and ChatGPT, we consider the six following linguistic characteristics: lexical diversity, sentence complexity, nominalization, presence of modals, epistemic and discourse markers. Those are motivated by previous work: Weiss et al. 25 observe the correlation between measures of lexical, syntactic and discourse complexities to the essay gradings of German high-school examinations while McNamara et al. 45 explore cohesion (indicated, among other things, by connectives), syntactic complexity and lexical diversity in relation to the essay scoring.
Lexical diversity
We identify vocabulary richness by using a well-established measure of textual, lexical diversity (MTLD) 46 which is often used in the field of automated essay grading 25 , 45 , 47 . It takes into account the number of unique words but unlike the best-known measure of lexical diversity, the type-token ratio (TTR), it is not as sensitive to the difference in the length of the texts. In fact, Koizumi and In’nami 48 find it to be least affected by the differences in the length of the texts compared to some other measures of lexical diversity. This is relevant to us due to the difference in average length between the human-written and ChatGPT-generated essays.
Syntactic complexity
We use two measures in order to evaluate the syntactic complexity of the essays. One is based on the maximum depth of the sentence dependency tree which is produced using the spaCy 3.4.2 dependency parser 49 (‘Syntactic complexity (depth)’). For the second measure, we adopt an approach similar in nature to the one by Weiss et al. 25 who use clause structure to evaluate syntactic complexity. In our case, we count the number of conjuncts, clausal modifiers of nouns, adverbial clause modifiers, clausal complements, clausal subjects, and parataxes (‘Syntactic complexity (clauses)’). The supplementary material in S2 shows the difference between sentence complexity based on two examples from the data.
Nominalization is a common feature of a more scientific style of writing 50 and is used as an additional measure for syntactic complexity. In order to explore this feature, we count occurrences of nouns with suffixes such as ‘-ion’, ‘-ment’, ‘-ance’ and a few others which are known to transform verbs into nouns.
Semantic properties
Both modals and epistemic markers signal the commitment of the writer to their statement. We identify modals using the POS-tagging module provided by spaCy as well as a list of epistemic expressions of modality, such as ‘definitely’ and ‘potentially’, also used in other approaches to identifying semantic properties 51 . For epistemic markers we adopt an empirically-driven approach and utilize the epistemic markers identified in a corpus of dialogical argumentation by Hautli-Janisz et al. 52 . We consider expressions such as ‘I think’, ‘it is believed’ and ‘in my opinion’ to be epistemic.
Discourse properties
Discourse markers can be used to measure the coherence quality of a text. This has been explored by Somasundaran et al. 53 who use discourse markers to evaluate the story-telling aspect of student writing while Nadeem et al. 54 incorporated them in their deep learning-based approach to automated essay scoring. In the present paper, we employ the PDTB list of discourse markers 55 which we adjust to exclude words that are often used for purposes other than indicating discourse relations, such as ‘like’, ‘for’, ‘in’ etc.
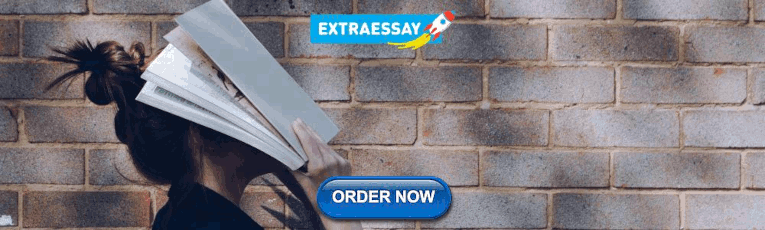
Statistical methods
We use a within-subjects design for our study. Each participant was shown six randomly selected essays. Results were submitted to the survey system after each essay was completed, in case participants ran out of time and did not finish scoring all six essays. Cronbach’s \(\alpha\) 56 allows us to determine the inter-rater reliability for the rating criterion and data source (human, ChatGPT-3, ChatGPT-4) in order to understand the reliability of our data not only overall, but also for each data source and rating criterion. We use two-sided Wilcoxon-rank-sum tests 57 to confirm the significance of the differences between the data sources for each criterion. We use the same tests to determine the significance of the linguistic characteristics. This results in three comparisons (human vs. ChatGPT-3, human vs. ChatGPT-4, ChatGPT-3 vs. ChatGPT-4) for each of the seven rating criteria and each of the seven linguistic characteristics, i.e. 42 tests. We use the Holm-Bonferroni method 58 for the correction for multiple tests to achieve a family-wise error rate of 0.05. We report the effect size using Cohen’s d 59 . While our data is not perfectly normal, it also does not have severe outliers, so we prefer the clear interpretation of Cohen’s d over the slightly more appropriate, but less accessible non-parametric effect size measures. We report point plots with estimates of the mean scores for each data source and criterion, incl. the 95% confidence interval of these mean values. The confidence intervals are estimated in a non-parametric manner based on bootstrap sampling. We further visualize the distribution for each criterion using violin plots to provide a visual indicator of the spread of the data (see Supplementary material S4 ).
Further, we use the self-assessment of the English skills and confidence in the essay ratings as confounding variables. Through this, we determine if ratings are affected by the language skills or confidence, instead of the actual quality of the essays. We control for the impact of these by measuring Pearson’s correlation coefficient r 60 between the self-assessments and the ratings. We also determine whether the linguistic features are correlated with the ratings as expected. The sentence complexity (both tree depth and dependency clauses), as well as the nominalization, are indicators of the complexity of the language. Similarly, the use of discourse markers should signal a proper logical structure. Finally, a large lexical diversity should be correlated with the ratings for the vocabulary. Same as above, we measure Pearson’s r . We use a two-sided test for the significance based on a \(\beta\) -distribution that models the expected correlations as implemented by scipy 61 . Same as above, we use the Holm-Bonferroni method to account for multiple tests. However, we note that it is likely that all—even tiny—correlations are significant given our amount of data. Consequently, our interpretation of these results focuses on the strength of the correlations.
Our statistical analysis of the data is implemented in Python. We use pandas 1.5.3 and numpy 1.24.2 for the processing of data, pingouin 0.5.3 for the calculation of Cronbach’s \(\alpha\) , scipy 1.10.1 for the Wilcoxon-rank-sum tests Pearson’s r , and seaborn 0.12.2 for the generation of plots, incl. the calculation of error bars that visualize the confidence intervals.
Out of the 111 teachers who completed the questionnaire, 108 rated all six essays, one rated five essays, one rated two essays, and one rated only one essay. This results in 658 ratings for 270 essays (90 topics for each essay type: human-, ChatGPT-3-, ChatGPT-4-generated), with three ratings for 121 essays, two ratings for 144 essays, and one rating for five essays. The inter-rater agreement is consistently excellent ( \(\alpha >0.9\) ), with the exception of language mastery where we have good agreement ( \(\alpha =0.89\) , see Table 2 ). Further, the correlation analysis depicted in supplementary material S4 shows weak positive correlations ( \(r \in 0.11, 0.28]\) ) between the self-assessment for the English skills, respectively the self-assessment for the confidence in ratings and the actual ratings. Overall, this indicates that our ratings are reliable estimates of the actual quality of the essays with a potential small tendency that confidence in ratings and language skills yields better ratings, independent of the data source.
Table 2 and supplementary material S4 characterize the distribution of the ratings for the essays, grouped by the data source. We observe that for all criteria, we have a clear order of the mean values, with students having the worst ratings, ChatGPT-3 in the middle rank, and ChatGPT-4 with the best performance. We further observe that the standard deviations are fairly consistent and slightly larger than one, i.e. the spread is similar for all ratings and essays. This is further supported by the visual analysis of the violin plots.
The statistical analysis of the ratings reported in Table 4 shows that differences between the human-written essays and the ones generated by both ChatGPT models are significant. The effect sizes for human versus ChatGPT-3 essays are between 0.52 and 1.15, i.e. a medium ( \(d \in [0.5,0.8)\) ) to large ( \(d \in [0.8, 1.2)\) ) effect. On the one hand, the smallest effects are observed for the expressiveness and complexity, i.e. when it comes to the overall comprehensiveness and complexity of the sentence structures, the differences between the humans and the ChatGPT-3 model are smallest. On the other hand, the difference in language mastery is larger than all other differences, which indicates that humans are more prone to making mistakes when writing than the NLG models. The magnitude of differences between humans and ChatGPT-4 is larger with effect sizes between 0.88 and 1.43, i.e., a large to very large ( \(d \in [1.2, 2)\) ) effect. Same as for ChatGPT-3, the differences are smallest for expressiveness and complexity and largest for language mastery. Please note that the difference in language mastery between humans and both GPT models does not mean that the humans have low scores for language mastery (M=3.90), but rather that the NLG models have exceptionally high scores (M=5.03 for ChatGPT-3, M=5.25 for ChatGPT-4).
When we consider the differences between the two GPT models, we observe that while ChatGPT-4 has consistently higher mean values for all criteria, only the differences for logic and composition, vocabulary and text linking, and complexity are significant. The effect sizes are between 0.45 and 0.5, i.e. small ( \(d \in [0.2, 0.5)\) ) and medium. Thus, while GPT-4 seems to be an improvement over GPT-3.5 in general, the only clear indicator of this is a better and clearer logical composition and more complex writing with a more diverse vocabulary.
We also observe significant differences in the distribution of linguistic characteristics between all three groups (see Table 3 ). Sentence complexity (depth) is the only category without a significant difference between humans and ChatGPT-3, as well as ChatGPT-3 and ChatGPT-4. There is also no significant difference in the category of discourse markers between humans and ChatGPT-3. The magnitude of the effects varies a lot and is between 0.39 and 1.93, i.e., between small ( \(d \in [0.2, 0.5)\) ) and very large. However, in comparison to the ratings, there is no clear tendency regarding the direction of the differences. For instance, while the ChatGPT models write more complex sentences and use more nominalizations, humans tend to use more modals and epistemic markers instead. The lexical diversity of humans is higher than that of ChatGPT-3 but lower than that of ChatGPT-4. While there is no difference in the use of discourse markers between humans and ChatGPT-3, ChatGPT-4 uses significantly fewer discourse markers.
We detect the expected positive correlations between the complexity ratings and the linguistic markers for sentence complexity ( \(r=0.16\) for depth, \(r=0.19\) for clauses) and nominalizations ( \(r=0.22\) ). However, we observe a negative correlation between the logic ratings and the discourse markers ( \(r=-0.14\) ), which counters our intuition that more frequent use of discourse indicators makes a text more logically coherent. However, this is in line with previous work: McNamara et al. 45 also find no indication that the use of cohesion indices such as discourse connectives correlates with high- and low-proficiency essays. Finally, we observe the expected positive correlation between the ratings for the vocabulary and the lexical diversity ( \(r=0.12\) ). All observed correlations are significant. However, we note that the strength of all these correlations is weak and that the significance itself should not be over-interpreted due to the large sample size.
Our results provide clear answers to the first two research questions that consider the quality of the generated essays: ChatGPT performs well at writing argumentative student essays and outperforms the quality of the human-written essays significantly. The ChatGPT-4 model has (at least) a large effect and is on average about one point better than humans on a seven-point Likert scale.
Regarding the third research question, we find that there are significant linguistic differences between humans and AI-generated content. The AI-generated essays are highly structured, which for instance is reflected by the identical beginnings of the concluding sections of all ChatGPT essays (‘In conclusion, [...]’). The initial sentences of each essay are also very similar starting with a general statement using the main concepts of the essay topics. Although this corresponds to the general structure that is sought after for argumentative essays, it is striking to see that the ChatGPT models are so rigid in realizing this, whereas the human-written essays are looser in representing the guideline on the linguistic surface. Moreover, the linguistic fingerprint has the counter-intuitive property that the use of discourse markers is negatively correlated with logical coherence. We believe that this might be due to the rigid structure of the generated essays: instead of using discourse markers, the AI models provide a clear logical structure by separating the different arguments into paragraphs, thereby reducing the need for discourse markers.
Our data also shows that hallucinations are not a problem in the setting of argumentative essay writing: the essay topics are not really about factual correctness, but rather about argumentation and critical reflection on general concepts which seem to be contained within the knowledge of the AI model. The stochastic nature of the language generation is well-suited for this kind of task, as different plausible arguments can be seen as a sampling from all available arguments for a topic. Nevertheless, we need to perform a more systematic study of the argumentative structures in order to better understand the difference in argumentation between human-written and ChatGPT-generated essay content. Moreover, we also cannot rule out that subtle hallucinations may have been overlooked during the ratings. There are also essays with a low rating for the criteria related to factual correctness, indicating that there might be cases where the AI models still have problems, even if they are, on average, better than the students.
One of the issues with evaluations of the recent large-language models is not accounting for the impact of tainted data when benchmarking such models. While it is certainly possible that the essays that were sourced by Stab and Gurevych 41 from the internet were part of the training data of the GPT models, the proprietary nature of the model training means that we cannot confirm this. However, we note that the generated essays did not resemble the corpus of human essays at all. Moreover, the topics of the essays are general in the sense that any human should be able to reason and write about these topics, just by understanding concepts like ‘cooperation’. Consequently, a taint on these general topics, i.e. the fact that they might be present in the data, is not only possible but is actually expected and unproblematic, as it relates to the capability of the models to learn about concepts, rather than the memorization of specific task solutions.
While we did everything to ensure a sound construct and a high validity of our study, there are still certain issues that may affect our conclusions. Most importantly, neither the writers of the essays, nor their raters, were English native speakers. However, the students purposefully used a forum for English writing frequented by native speakers to ensure the language and content quality of their essays. This indicates that the resulting essays are likely above average for non-native speakers, as they went through at least one round of revisions with the help of native speakers. The teachers were informed that part of the training would be in English to prevent registrations from people without English language skills. Moreover, the self-assessment of the language skills was only weakly correlated with the ratings, indicating that the threat to the soundness of our results is low. While we cannot definitively rule out that our results would not be reproducible with other human raters, the high inter-rater agreement indicates that this is unlikely.
However, our reliance on essays written by non-native speakers affects the external validity and the generalizability of our results. It is certainly possible that native speaking students would perform better in the criteria related to language skills, though it is unclear by how much. However, the language skills were particular strengths of the AI models, meaning that while the difference might be smaller, it is still reasonable to conclude that the AI models would have at least comparable performance to humans, but possibly still better performance, just with a smaller gap. While we cannot rule out a difference for the content-related criteria, we also see no strong argument why native speakers should have better arguments than non-native speakers. Thus, while our results might not fully translate to native speakers, we see no reason why aspects regarding the content should not be similar. Further, our results were obtained based on high-school-level essays. Native and non-native speakers with higher education degrees or experts in fields would likely also achieve a better performance, such that the difference in performance between the AI models and humans would likely also be smaller in such a setting.
We further note that the essay topics may not be an unbiased sample. While Stab and Gurevych 41 randomly sampled the essays from the writing feedback section of an essay forum, it is unclear whether the essays posted there are representative of the general population of essay topics. Nevertheless, we believe that the threat is fairly low because our results are consistent and do not seem to be influenced by certain topics. Further, we cannot with certainty conclude how our results generalize beyond ChatGPT-3 and ChatGPT-4 to similar models like Bard ( https://bard.google.com/?hl=en ) Alpaca, and Dolly. Especially the results for linguistic characteristics are hard to predict. However, since—to the best of our knowledge and given the proprietary nature of some of these models—the general approach to how these models work is similar and the trends for essay quality should hold for models with comparable size and training procedures.
Finally, we want to note that the current speed of progress with generative AI is extremely fast and we are studying moving targets: ChatGPT 3.5 and 4 today are already not the same as the models we studied. Due to a lack of transparency regarding the specific incremental changes, we cannot know or predict how this might affect our results.
Our results provide a strong indication that the fear many teaching professionals have is warranted: the way students do homework and teachers assess it needs to change in a world of generative AI models. For non-native speakers, our results show that when students want to maximize their essay grades, they could easily do so by relying on results from AI models like ChatGPT. The very strong performance of the AI models indicates that this might also be the case for native speakers, though the difference in language skills is probably smaller. However, this is not and cannot be the goal of education. Consequently, educators need to change how they approach homework. Instead of just assigning and grading essays, we need to reflect more on the output of AI tools regarding their reasoning and correctness. AI models need to be seen as an integral part of education, but one which requires careful reflection and training of critical thinking skills.
Furthermore, teachers need to adapt strategies for teaching writing skills: as with the use of calculators, it is necessary to critically reflect with the students on when and how to use those tools. For instance, constructivists 62 argue that learning is enhanced by the active design and creation of unique artifacts by students themselves. In the present case this means that, in the long term, educational objectives may need to be adjusted. This is analogous to teaching good arithmetic skills to younger students and then allowing and encouraging students to use calculators freely in later stages of education. Similarly, once a sound level of literacy has been achieved, strongly integrating AI models in lesson plans may no longer run counter to reasonable learning goals.
In terms of shedding light on the quality and structure of AI-generated essays, this paper makes an important contribution by offering an independent, large-scale and statistically sound account of essay quality, comparing human-written and AI-generated texts. By comparing different versions of ChatGPT, we also offer a glance into the development of these models over time in terms of their linguistic properties and the quality they exhibit. Our results show that while the language generated by ChatGPT is considered very good by humans, there are also notable structural differences, e.g. in the use of discourse markers. This demonstrates that an in-depth consideration not only of the capabilities of generative AI models is required (i.e. which tasks can they be used for), but also of the language they generate. For example, if we read many AI-generated texts that use fewer discourse markers, it raises the question if and how this would affect our human use of discourse markers. Understanding how AI-generated texts differ from human-written enables us to look for these differences, to reason about their potential impact, and to study and possibly mitigate this impact.
Data availability
The datasets generated during and/or analysed during the current study are available in the Zenodo repository, https://doi.org/10.5281/zenodo.8343644
Code availability
All materials are available online in form of a replication package that contains the data and the analysis code, https://doi.org/10.5281/zenodo.8343644 .
Ouyang, L. et al. Training language models to follow instructions with human feedback (2022). arXiv:2203.02155 .
Ruby, D. 30+ detailed chatgpt statistics–users & facts (sep 2023). https://www.demandsage.com/chatgpt-statistics/ (2023). Accessed 09 June 2023.
Leahy, S. & Mishra, P. TPACK and the Cambrian explosion of AI. In Society for Information Technology & Teacher Education International Conference , (ed. Langran, E.) 2465–2469 (Association for the Advancement of Computing in Education (AACE), 2023).
Ortiz, S. Need an ai essay writer? here’s how chatgpt (and other chatbots) can help. https://www.zdnet.com/article/how-to-use-chatgpt-to-write-an-essay/ (2023). Accessed 09 June 2023.
Openai chat interface. https://chat.openai.com/ . Accessed 09 June 2023.
OpenAI. Gpt-4 technical report (2023). arXiv:2303.08774 .
Brown, T. B. et al. Language models are few-shot learners (2020). arXiv:2005.14165 .
Wang, B. Mesh-Transformer-JAX: Model-Parallel Implementation of Transformer Language Model with JAX. https://github.com/kingoflolz/mesh-transformer-jax (2021).
Wei, J. et al. Finetuned language models are zero-shot learners. In International Conference on Learning Representations (2022).
Taori, R. et al. Stanford alpaca: An instruction-following llama model. https://github.com/tatsu-lab/stanford_alpaca (2023).
Cai, Z. G., Haslett, D. A., Duan, X., Wang, S. & Pickering, M. J. Does chatgpt resemble humans in language use? (2023). arXiv:2303.08014 .
Mahowald, K. A discerning several thousand judgments: Gpt-3 rates the article + adjective + numeral + noun construction (2023). arXiv:2301.12564 .
Dentella, V., Murphy, E., Marcus, G. & Leivada, E. Testing ai performance on less frequent aspects of language reveals insensitivity to underlying meaning (2023). arXiv:2302.12313 .
Guo, B. et al. How close is chatgpt to human experts? comparison corpus, evaluation, and detection (2023). arXiv:2301.07597 .
Zhao, W. et al. Is chatgpt equipped with emotional dialogue capabilities? (2023). arXiv:2304.09582 .
Keim, D. A. & Oelke, D. Literature fingerprinting : A new method for visual literary analysis. In 2007 IEEE Symposium on Visual Analytics Science and Technology , 115–122, https://doi.org/10.1109/VAST.2007.4389004 (IEEE, 2007).
El-Assady, M. et al. Interactive visual analysis of transcribed multi-party discourse. In Proceedings of ACL 2017, System Demonstrations , 49–54 (Association for Computational Linguistics, Vancouver, Canada, 2017).
Mennatallah El-Assady, A. H.-J. & Butt, M. Discourse maps - feature encoding for the analysis of verbatim conversation transcripts. In Visual Analytics for Linguistics , vol. CSLI Lecture Notes, Number 220, 115–147 (Stanford: CSLI Publications, 2020).
Matt Foulis, J. V. & Reed, C. Dialogical fingerprinting of debaters. In Proceedings of COMMA 2020 , 465–466, https://doi.org/10.3233/FAIA200536 (Amsterdam: IOS Press, 2020).
Matt Foulis, J. V. & Reed, C. Interactive visualisation of debater identification and characteristics. In Proceedings of the COMMA workshop on Argument Visualisation, COMMA , 1–7 (2020).
Chatzipanagiotidis, S., Giagkou, M. & Meurers, D. Broad linguistic complexity analysis for Greek readability classification. In Proceedings of the 16th Workshop on Innovative Use of NLP for Building Educational Applications , 48–58 (Association for Computational Linguistics, Online, 2021).
Ajili, M., Bonastre, J.-F., Kahn, J., Rossato, S. & Bernard, G. FABIOLE, a speech database for forensic speaker comparison. In Proceedings of the Tenth International Conference on Language Resources and Evaluation (LREC’16) , 726–733 (European Language Resources Association (ELRA), Portorož, Slovenia, 2016).
Deutsch, T., Jasbi, M. & Shieber, S. Linguistic features for readability assessment. In Proceedings of the Fifteenth Workshop on Innovative Use of NLP for Building Educational Applications , 1–17, https://doi.org/10.18653/v1/2020.bea-1.1 (Association for Computational Linguistics, Seattle, WA, USA \(\rightarrow\) Online, 2020).
Fiacco, J., Jiang, S., Adamson, D. & Rosé, C. Toward automatic discourse parsing of student writing motivated by neural interpretation. In Proceedings of the 17th Workshop on Innovative Use of NLP for Building Educational Applications (BEA 2022) , 204–215, https://doi.org/10.18653/v1/2022.bea-1.25 (Association for Computational Linguistics, Seattle, Washington, 2022).
Weiss, Z., Riemenschneider, A., Schröter, P. & Meurers, D. Computationally modeling the impact of task-appropriate language complexity and accuracy on human grading of German essays. In Proceedings of the Fourteenth Workshop on Innovative Use of NLP for Building Educational Applications , 30–45, https://doi.org/10.18653/v1/W19-4404 (Association for Computational Linguistics, Florence, Italy, 2019).
Yang, F., Dragut, E. & Mukherjee, A. Predicting personal opinion on future events with fingerprints. In Proceedings of the 28th International Conference on Computational Linguistics , 1802–1807, https://doi.org/10.18653/v1/2020.coling-main.162 (International Committee on Computational Linguistics, Barcelona, Spain (Online), 2020).
Tumarada, K. et al. Opinion prediction with user fingerprinting. In Proceedings of the International Conference on Recent Advances in Natural Language Processing (RANLP 2021) , 1423–1431 (INCOMA Ltd., Held Online, 2021).
Rocca, R. & Yarkoni, T. Language as a fingerprint: Self-supervised learning of user encodings using transformers. In Findings of the Association for Computational Linguistics: EMNLP . 1701–1714 (Association for Computational Linguistics, Abu Dhabi, United Arab Emirates, 2022).
Aiyappa, R., An, J., Kwak, H. & Ahn, Y.-Y. Can we trust the evaluation on chatgpt? (2023). arXiv:2303.12767 .
Yeadon, W., Inyang, O.-O., Mizouri, A., Peach, A. & Testrow, C. The death of the short-form physics essay in the coming ai revolution (2022). arXiv:2212.11661 .
TURING, A. M. I.-COMPUTING MACHINERY AND INTELLIGENCE. Mind LIX , 433–460, https://doi.org/10.1093/mind/LIX.236.433 (1950). https://academic.oup.com/mind/article-pdf/LIX/236/433/30123314/lix-236-433.pdf .
Kortemeyer, G. Could an artificial-intelligence agent pass an introductory physics course? (2023). arXiv:2301.12127 .
Kung, T. H. et al. Performance of chatgpt on usmle: Potential for ai-assisted medical education using large language models. PLOS Digital Health 2 , 1–12. https://doi.org/10.1371/journal.pdig.0000198 (2023).
Article Google Scholar
Frieder, S. et al. Mathematical capabilities of chatgpt (2023). arXiv:2301.13867 .
Yuan, Z., Yuan, H., Tan, C., Wang, W. & Huang, S. How well do large language models perform in arithmetic tasks? (2023). arXiv:2304.02015 .
Touvron, H. et al. Llama: Open and efficient foundation language models (2023). arXiv:2302.13971 .
Chung, H. W. et al. Scaling instruction-finetuned language models (2022). arXiv:2210.11416 .
Workshop, B. et al. Bloom: A 176b-parameter open-access multilingual language model (2023). arXiv:2211.05100 .
Spencer, S. T., Joshi, V. & Mitchell, A. M. W. Can ai put gamma-ray astrophysicists out of a job? (2023). arXiv:2303.17853 .
Cherian, A., Peng, K.-C., Lohit, S., Smith, K. & Tenenbaum, J. B. Are deep neural networks smarter than second graders? (2023). arXiv:2212.09993 .
Stab, C. & Gurevych, I. Annotating argument components and relations in persuasive essays. In Proceedings of COLING 2014, the 25th International Conference on Computational Linguistics: Technical Papers , 1501–1510 (Dublin City University and Association for Computational Linguistics, Dublin, Ireland, 2014).
Essay forum. https://essayforum.com/ . Last-accessed: 2023-09-07.
Common european framework of reference for languages (cefr). https://www.coe.int/en/web/common-european-framework-reference-languages . Accessed 09 July 2023.
Kmk guidelines for essay assessment. http://www.kmk-format.de/material/Fremdsprachen/5-3-2_Bewertungsskalen_Schreiben.pdf . Accessed 09 July 2023.
McNamara, D. S., Crossley, S. A. & McCarthy, P. M. Linguistic features of writing quality. Writ. Commun. 27 , 57–86 (2010).
McCarthy, P. M. & Jarvis, S. Mtld, vocd-d, and hd-d: A validation study of sophisticated approaches to lexical diversity assessment. Behav. Res. Methods 42 , 381–392 (2010).
Article PubMed Google Scholar
Dasgupta, T., Naskar, A., Dey, L. & Saha, R. Augmenting textual qualitative features in deep convolution recurrent neural network for automatic essay scoring. In Proceedings of the 5th Workshop on Natural Language Processing Techniques for Educational Applications , 93–102 (2018).
Koizumi, R. & In’nami, Y. Effects of text length on lexical diversity measures: Using short texts with less than 200 tokens. System 40 , 554–564 (2012).
spacy industrial-strength natural language processing in python. https://spacy.io/ .
Siskou, W., Friedrich, L., Eckhard, S., Espinoza, I. & Hautli-Janisz, A. Measuring plain language in public service encounters. In Proceedings of the 2nd Workshop on Computational Linguistics for Political Text Analysis (CPSS-2022) (Potsdam, Germany, 2022).
El-Assady, M. & Hautli-Janisz, A. Discourse Maps - Feature Encoding for the Analysis of Verbatim Conversation Transcripts (CSLI lecture notes (CSLI Publications, Center for the Study of Language and Information, 2019).
Hautli-Janisz, A. et al. QT30: A corpus of argument and conflict in broadcast debate. In Proceedings of the Thirteenth Language Resources and Evaluation Conference , 3291–3300 (European Language Resources Association, Marseille, France, 2022).
Somasundaran, S. et al. Towards evaluating narrative quality in student writing. Trans. Assoc. Comput. Linguist. 6 , 91–106 (2018).
Nadeem, F., Nguyen, H., Liu, Y. & Ostendorf, M. Automated essay scoring with discourse-aware neural models. In Proceedings of the Fourteenth Workshop on Innovative Use of NLP for Building Educational Applications , 484–493, https://doi.org/10.18653/v1/W19-4450 (Association for Computational Linguistics, Florence, Italy, 2019).
Prasad, R. et al. The Penn Discourse TreeBank 2.0. In Proceedings of the Sixth International Conference on Language Resources and Evaluation (LREC’08) (European Language Resources Association (ELRA), Marrakech, Morocco, 2008).
Cronbach, L. J. Coefficient alpha and the internal structure of tests. Psychometrika 16 , 297–334. https://doi.org/10.1007/bf02310555 (1951).
Article MATH Google Scholar
Wilcoxon, F. Individual comparisons by ranking methods. Biom. Bull. 1 , 80–83 (1945).
Holm, S. A simple sequentially rejective multiple test procedure. Scand. J. Stat. 6 , 65–70 (1979).
MathSciNet MATH Google Scholar
Cohen, J. Statistical power analysis for the behavioral sciences (Academic press, 2013).
Freedman, D., Pisani, R. & Purves, R. Statistics (international student edition). Pisani, R. Purves, 4th edn. WW Norton & Company, New York (2007).
Scipy documentation. https://docs.scipy.org/doc/scipy/reference/generated/scipy.stats.pearsonr.html . Accessed 09 June 2023.
Windschitl, M. Framing constructivism in practice as the negotiation of dilemmas: An analysis of the conceptual, pedagogical, cultural, and political challenges facing teachers. Rev. Educ. Res. 72 , 131–175 (2002).
Download references
Open Access funding enabled and organized by Projekt DEAL.
Author information
Authors and affiliations.
Faculty of Computer Science and Mathematics, University of Passau, Passau, Germany
Steffen Herbold, Annette Hautli-Janisz, Ute Heuer, Zlata Kikteva & Alexander Trautsch
You can also search for this author in PubMed Google Scholar
Contributions
S.H., A.HJ., and U.H. conceived the experiment; S.H., A.HJ, and Z.K. collected the essays from ChatGPT; U.H. recruited the study participants; S.H., A.HJ., U.H. and A.T. conducted the training session and questionnaire; all authors contributed to the analysis of the results, the writing of the manuscript, and review of the manuscript.
Corresponding author
Correspondence to Steffen Herbold .
Ethics declarations
Competing interests.
The authors declare no competing interests.
Additional information
Publisher's note.
Springer Nature remains neutral with regard to jurisdictional claims in published maps and institutional affiliations.
Supplementary Information
Supplementary information 1., supplementary information 2., supplementary information 3., supplementary tables., supplementary figures., rights and permissions.
Open Access This article is licensed under a Creative Commons Attribution 4.0 International License, which permits use, sharing, adaptation, distribution and reproduction in any medium or format, as long as you give appropriate credit to the original author(s) and the source, provide a link to the Creative Commons licence, and indicate if changes were made. The images or other third party material in this article are included in the article's Creative Commons licence, unless indicated otherwise in a credit line to the material. If material is not included in the article's Creative Commons licence and your intended use is not permitted by statutory regulation or exceeds the permitted use, you will need to obtain permission directly from the copyright holder. To view a copy of this licence, visit http://creativecommons.org/licenses/by/4.0/ .
Reprints and permissions
About this article
Cite this article.
Herbold, S., Hautli-Janisz, A., Heuer, U. et al. A large-scale comparison of human-written versus ChatGPT-generated essays. Sci Rep 13 , 18617 (2023). https://doi.org/10.1038/s41598-023-45644-9
Download citation
Received : 01 June 2023
Accepted : 22 October 2023
Published : 30 October 2023
DOI : https://doi.org/10.1038/s41598-023-45644-9
Share this article
Anyone you share the following link with will be able to read this content:
Sorry, a shareable link is not currently available for this article.
Provided by the Springer Nature SharedIt content-sharing initiative
This article is cited by
Defense against adversarial attacks: robust and efficient compressed optimized neural networks.
- Insaf Kraidia
- Afifa Ghenai
- Samir Brahim Belhaouari
Scientific Reports (2024)
AI-driven translations for kidney transplant equity in Hispanic populations
- Oscar A. Garcia Valencia
- Charat Thongprayoon
- Wisit Cheungpasitporn
How will the state think with ChatGPT? The challenges of generative artificial intelligence for public administrations
- Thomas Cantens
AI & SOCIETY (2024)
By submitting a comment you agree to abide by our Terms and Community Guidelines . If you find something abusive or that does not comply with our terms or guidelines please flag it as inappropriate.
Quick links
- Explore articles by subject
- Guide to authors
- Editorial policies
Sign up for the Nature Briefing: AI and Robotics newsletter — what matters in AI and robotics research, free to your inbox weekly.

Writing a synthesis versus reading: strategies involved and impact on comprehension
- Open access
- Published: 20 August 2022
- Volume 36 , pages 849–880, ( 2023 )
Cite this article
You have full access to this open access article
- Núria Castells ORCID: orcid.org/0000-0002-4784-9672 1 ,
- Marta Minguela ORCID: orcid.org/0000-0001-6216-253X 1 &
- Esther Nadal ORCID: orcid.org/0000-0001-9508-7732 1
4284 Accesses
5 Citations
Explore all metrics
Little evidence is available regarding the differential impact of reading versus reading and writing on multiple source comprehension. The present study aims to: (1) compare the inferential comprehension performance of students in reading versus reading/synthesis conditions; (2) explore the impact of performing the tasks on paper versus on screen with Read&Answer (R&A) software; and (3) explore the extent to which rereading, notetaking, and the quality of the written synthesis can explain student’s comprehension scores. For the students in the synthesis condition, we also examined the relationship between the quality of the synthesis they produced and the comprehension they achieved. 155 psychology undergraduates were randomly assigned either to the reading (n = 78) or to the reading/synthesis condition (n = 77). From this sample, 79 participants carried out the task with the Read&Answer software, and 76 solved the task on paper. All the students took a prior knowledge questionnaire, and read three complementary texts about the conception of intelligence. Students in the reading condition answered an inferential comprehension test, whereas students in the synthesis condition were asked to write a synthesis before taking the same test. Results show no differences in comprehension between students in the four conditions (task and media). There was no significant association between rereading and task condition. However, students in the synthesis condition were more likely to take notes. We found that two of the categories for the quality of the synthesis, textual organization and accuracy of content had an impact on inferential comprehension for the participants who wrote it. The quality of the synthesis mediated between student’s prior knowledge and inferential comprehension.
Similar content being viewed by others
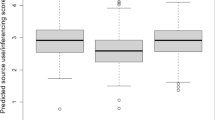
Source inclusion in synthesis writing: an NLP approach to understanding argumentation, sourcing, and essay quality
Scott Crossley, Qian Wan, … Danielle McNamara
The use of source-related strategies in evaluating multiple psychology texts: a student–scientist comparison
Sarah von der Mühlen, Tobias Richter, … Kirsten Berthold
Multiple Document Comprehension of University Students
Avoid common mistakes on your manuscript.
Introduction
When students are doing course work and taking exams, they typically face the challenge of dealing with multiple sources that contain information that may be divergent or even partly contradictory. The ability to access information transmitted in multiple texts, the capacity to process it, integrate it, and adopt a critical perspective towards it constitutes a learning process that cannot be taken as a given when a student enters university. Several studies (Mateos & Solé, 2009 ; Miras et al., 2013 ; Spivey, 1997 ) stress the difficulty of learning from multiple sources, an activity typical of the university experience. Since students may approach learning from multiple texts in diverse ways, more research is needed to examine the different strategies they use and how these strategies help them to achieve this aim.
Previous research has shown that asking students to write using multiple sources enabled them to elaborate and organize the information they read in greater depth and enhanced their reading comprehension and final learning (Wiley & Voss, 1999 ). However, it is not clear how specific writing tasks and the strategies involved in them affect comprehension outcomes (Hebert et al., 2013 ). The present paper explores this issue by comparing the inferential comprehension outcomes of students who read several sources and students who also wrote a text after reading the sources, and by analyzing the strategies they used to deal with these two tasks. The use of the Read&Answer software allowed us to keep track of the strategies students applied.
Multiple sources comprehension
Comprehending multiple texts in depth is not an easy task (Britt et al., 2017 ; List & Alexander, 2019 ). Following on from Kintsch ( 1998 ), when dealing with a single text, students must progress towards different levels of representation: surface level (decode and understand the words and sentences), text-based (representation of the ideas explicitly mentioned in the text) and situation model (integrating information with prior knowledge and generating inferences). Inference is essential to establish the local or overall coherence of a text, through connecting several units of information (Basaraba et al., 2013 ). Additionally, when dealing with multiple documents, students must process and integrate information across texts to form a documents model (Britt et al., 1999 ), which is an integrated cognitive representation of the main content of the texts (called the Integrated Model), as well as a representation of source information (i.e., authorship, form, rhetorical goals…) together with the construction of links between texts as consistent or contradictory with one another (called the Intertext Model). For Barzilai et al. ( 2018 ), integration is central in multiple text comprehension because it involves articulating information from different sources in order to achieve aims such as understanding an issue or writing a synthesis. The capacity to infer information from the sources and across the sources seems to be crucial for integrating and achieving a comprehension of multiple texts (List & Alexander, 2019 ). The cross-textual linking, which may be either low-level (referring to explicit content introduced in the sources) or high-level (categories or functional aspects of the source texts that are not always explicit and should be inferred), might be facilitated by using writing in diverse forms (e.g., note-taking, graphic organizers, summarizing, synthesizing—List & Alexander, 2019 ).
Synthesis writing
The idea that writing has a positive impact on learning has a long tradition, doubtlessly linked to the acknowledgement of the potentially epistemic nature of the activity (Galbraith & Baaijen, 2018 ). In specific circumstances, writing enables one to incorporate information, restructure knowledge and make conceptual changes (Scardamalia & Bereiter, 1987 ; Schumacher & Nash, 1991 ). Indeed, according to the functional view of reading-writing connections (Fitzgerald & Shanahan, 2020 ), comprehension can be enhanced by writing about texts while performing actions of different types (Applebee, 1984 ; Graham & Hebert, 2011 ; Hebert et al., 2013 ). First, writers must select the most relevant information from the texts, focusing on the content. Secondly, when writing, students need to organize ideas from the texts coherently, building connections between them. Thirdly, since the written document is permanent, it allows for reflection, in the sense that the writer can review, connect and construct new versions of the ideas in the text. Fourthly, the process requires the writer to take active decisions about the text’s content and/or structure. Finally, since writers need to put text ideas into their own words, their understanding of these ideas may be increased.
At undergraduate level, students are habitually required to integrate information from multiple sources into a written text or document (i.e., dissertations, research work, essays, etc.). Despite differences in the types of documents students are assigned, from a cognitive perspective they share some basic aspects that make the task challenging. For Barzilai et al. ( 2018 ), and based on empirical studies, when people read-to-write from sources (Nelson & King, 2022 ), they deal with the following issues: understanding the content of the source texts and the aims of the authors; understanding the relations/connections that can be established between them; selecting the information relevant to achieving the aim; identifying a thread that helps to organize the selected information; taking decisions about the structure; linking the ideas in writing and revising during the process and at the end.
This explanation highlights the three main operations of synthesis writing: selecting, organizing and connecting the information (Barzilai et al., 2018 ; Spivey & King, 1989 ). Therefore, when they synthesize, students may perform various roles: e.g., as readers of source texts, writers of notes, readers of notes, writers of drafts and readers of their own text (Mateos & Solé, 2009 ). Several researchers have hypothesized that, since reading leads to learning and writing has epistemic potential (Nelson & King, 2022 ), the transitions between sources and the students’ own text when students assume these changing roles may explain the transformation of their knowledge and their enhanced comprehension of the source texts (Moran & Billen, 2014 ; Nelson & King, 2022 ).
Writing and reading multiple sources and comprehension
Several meta-analyses have pointed to the fact that writing interventions which involve writing about a single text had an impact on comprehension outcomes (Bangert-Drowns et al., 2004 ; Graham & Hebert, 2011 ; Hebert et al., 2013 ). However, would reading-to-write from sources have the same impact on comprehension?
Research on the impact of synthesis writing on comprehension has not achieved conclusive results. Before revising the most relevant results from research, two precautions should be mentioned. First, the writing from sources tasks assigned to students are not always clearly conceptualized; that is, despite sometimes being called “synthesis”, they may involve not just selecting, organizing and connecting information, but also providing one’s own opinion on, or criticizing, a subject. Secondly, the tasks aimed at assessing comprehension after performing the writing from sources task may only assess comprehension and/or learning (when the task involves retention of source information). However, since reading might lead to learning, as noted by Nelson and King ( 2022 ), in the following review we have considered that tasks placed after the reading-to-write activity that assess learning are also assessing the comprehension, in a general sense, of the source information.
Bearing the aforementioned precautions in mind, we will review studies that have compared reading-to-write from sources with just reading, and which have included comprehension assessments. Wiley and Voss ( 1999 ) found that asking undergraduate students to write an argumentative synthesis after reading multiple history sources about nineteenth-century Ireland improved their performance on inference comprehension and analogy tasks more than writing narratives, summaries or explanations. The authors attributed these results to the fact that the argument text written by the students contained more connected, integrated and transformed ideas than the other types of texts written under the other research conditions.
Following on from the study by Wiley and Voss, Le Bigot and Rouet ( 2007 ) asked 52 university students to write either a summary (presenting the main ideas) or an argument (expressing an opinion) on social influence after reading multisource hypertexts. After producing their text, they were asked to complete a comprehension questionnaire. The results from this study differ from those of Wiley and Voss ( 1999 ). Although argument texts were found to show more causal/consequence connectives and more transformed ideas than summary texts (which contained a greater use of temporal connectives and more borrowed ideas from the source texts), they did not improve comprehension, as measured by the identification of main ideas and detection of local details. Nevertheless, writing a summary enhanced both kinds of comprehension.
Gil et al. ( 2010 ) also compared writing a summary to writing an argument from several texts on climate change. Unlike Le Bigot and Rouet ( 2007 ), they found that students who wrote a summary text produced more transformations, covered all the information in the text materials and integrated the information to a greater extent than students asked to write in the argument text condition. Furthermore, students in the summary group obtained higher scores on the measures that were developed to assess either superficial or inferential comprehension. Notably, in these two latter studies, the summary task was closer to a synthesis task than the argumentative activity. For the summary task, Le Bigot and Rouet ( 2007 ) asked students to collect the main ideas from the texts, while Gil et al. ( 2010 ) asked for a report summarizing the causes of climate change presented in the texts. Therefore, students were able to integrate information from the texts without losing the intrinsic meaning of the information contained in them. In contrast, to write the argumentative proposal, in both studies students had to provide their own opinion, which requires them to adopt an external and critical stance and to distance themselves from the meaning of the texts. Cerdán and Vidal-Abarca ( 2008 ) decided to compare two clearly distinct tasks: writing an essay in order to answer an intertextual open question after reading three sources, and answering four intratextual open questions (the responses were placed in only one text). Students performed the reading and writing tasks using a special software program, Read&Answer , and were allowed to go back and forth from their products to the source texts and vice versa. A sample of the students performed the tasks while thinking aloud. After finishing the tasks, students completed a superficial comprehension task (sentence verification task), and a deep learning task (answering several open questions related to a problem situation). As predicted by the researchers, examination of the students’ processes revealed that those who wrote the essay integrated more relevant units of information than those who answered the intratextual questions, given that the latter showed more single-unit processing. In terms of comprehension, writing the essay produced better results in the deep learning task than answering intratextual questions; in contrast, there were no differences between the two tasks in the superficial understanding test. Cerdán and Vidal-Abarca ( 2008 ) concluded that writing the response to intertextual questions fosters deeper learning than intratextual ones, and that the two kinds of activities did not differ at the level of superficial comprehension.
In short, task instructions seem to be relevant to the way students understand the specificities of the writing task (Barzilai et al., 2018 ; Spivey, 1997 ). Asking students to pay attention to relevant information from the texts and to integrate it (i.e., by writing a synthesis) might help them to process information in greater depth, through building more integrations between the information contained in the texts, than asking them to give their opinion on a subject (which does not necessarily require them to select, organize and connect information). In this regard, Miras et al. ( 2013 ) found that higher education students who wrote better syntheses than their peers after reading three texts responded better to different kinds of questions that involved retrieving, interpreting and reflecting on information. Since the processes conducted by students when writing a synthesis allow them to connect more intra- and intertextual information, they perform better on deep comprehension measures. However, to the best of our knowledge there is no evidence of the different impact of only reading multiple documents versus reading and writing a synthesis on comprehension.
One final concern related to the previous studies would be that all of them ask some or all of their participants to read on a computer, in some cases using special software such as Read&Answer, in order to collect data from them all (Cerdán & Vidal-Abarca, 2008 ), or from a sample of their participants (Gil et al., 2010 ). For years, researchers have asked participants to read on screen or on paper without specifically addressing the possible influence of the media on the level of comprehension. However, some recent meta-analyses comparing students’ comprehension outcomes when reading expository texts on paper or on screen have concluded that solving the tasks on screen negatively influences the level of comprehension finally achieved (Clinton, 2019 ; Delgado et al., 2018 ). It is important to add that these results were obtained under time constraints, but were not evident when participants had all the time they wanted to solve the tasks. Additionally, Gil et al. ( 2010 ) and Vidal-Abarca et al. ( 2011 ) have pointed out that using Read&Answer software does not negatively influence students’ performance when compared with paper-and-pencil tasks.
In a different vein, with the exception of Cerdán and Vidal-Abarca ( 2008 ), the studies reviewed provide little information on the specific strategies involved in solving the tasks analyzed (e.g., rereading and note-taking). Is it the task in itself that leads to deep-level comprehension results? Or is it the specific strategies deployed by the students in order to solve the tasks that are responsible for the results?
Strategies to deal with multiple sources: rereading and note-taking
Just as the research suggests that essentially the same cognitive processes (generating ideas, questioning, hypothesizing, making inferences and supervising, etc.; see Tierney & Shanahan, 1996 ) come into play in both reading and writing, albeit with different weights, students may decide to use some or other of these processes or skills in a strategic way to solve the tasks they are assigned. According to Kirby ( 1988 ) and Dinsmore ( 2018 ), strategies are skills intentionally and consciously used to achieve an aim. Comprehension strategies refer to intentional attempts to control and modify meaning construction when reading a text (Afflerbach et al., 2008 ). Writing strategies involve the use of processes by the writer to improve the success of their writing (Baker & Boonkit, 2004 ). In this study, two strategies are prioritized: rereading and note-taking.
Rereading the text or parts of it is a strategy that has been highlighted as useful for achieving deep comprehension (Stine-Morrow et al., 2004 ). Goldman and Saul ( 1990 ), Hyöna et al. ( 2002 ) and Minguela et al. ( 2015 ) have described different overall reading processes that readers spontaneously engage in when approaching texts before performing a post-reading task, among which rereading seems to be key. In the Minguela et al. ( 2015 ) study, students who selectively reread some specific parts of the text obtained better results in a deep comprehension task containing questions that required integration of information across the text and/or reasoning beyond its literal content.
In the case of writing from various texts, researchers have also stressed the relevance of rereading to explain the differences in quality of the texts produced. Working from this perspective, McGinley ( 1992 ) was the first to mention the relevance of rereading (i.e., when students read either the source texts or their own products once again) for composing from sources. The importance of rereading documents in the synthesis task has been stressed by several research studies (see Lenski & Johns, 1997 ; Martínez et al., 2015 ; Solé et al., 2013 ; Vandermeulen et al., 2019 ). In addition to writing better products themselves, students who reread the texts (sometimes together with performing other activities, such as writing a draft or taking notes) showed either better learning of the contents of the texts (in the intervention study conducted by Martínez et al., 2015 ) or better comprehension (Solé et al., 2013 ).
Other studies in the area of writing a synthesis from sources have examined another strategy that students can performed spontaneously: note-taking (Hagen et al., 2014 ). However, the role of taking notes in writing a synthesis is less clear than the role of rereading (Bednall & Kehoe, 2011 ; Dovey, 2010 ). In single text reading, Gil et al. ( 2008 ) compared students who were required to take notes to those who did not take notes; they found that, firstly, taking notes reduced the time students devoted to processing the information in the source, and secondly, students who took notes identified fewer inferential sentences than students who did not. This finding was also replicated by some studies that required students to read from multiple sources while allowing them to take notes. These studies found that students who mostly copy from the texts tended to obtain poorer comprehension results (Hagen et al., 2014 ) and wrote weak synthesis essays (Luo & Kiewra, 2019 ). However, results for this question are not conclusive, because Kobayashi ( 2009 ) found that when students were given a clear purpose (i.e., finding relations between texts), those who used external strategy tools (e.g., taking notes—Kobayashi, 2009 ), outperformed students who did not use tools in a test with intertextual questions.
To summarize, these studies suggest the importance of strategies when students read texts or write from sources. Producing a synthesis from different texts requires them to read and reread them in order to identify the relevant information and to elaborate and integrate it (Nelson, 2008 ; Solé et al., 2013 ). In this demanding task, taking notes may be of considerable help. However, the strategies involved in reading, and in reading and writing from multiple sources, require fuller examination, as does the impact of these practices on comprehension.
This paper aims to explore this issue in depth. Specifically, it pursues two sets of aims: one for a general sample of students, and another one for a subsample of these students that performed the task on a computer and using the Read&Answer (R&A) software.
Regarding the general sample, firstly, we aim to explore whether there were differences in the comprehension performance of participants depending on two variables (i.e., task—reading multiple texts and writing a synthesis vs. reading multiple texts only; and media—paper vs. screen with R&A software). Secondly, we aim to analyze whether there were differences in the quality of the synthesis written by participants in the reading/synthesis condition depending on the media used, and the relationship between the quality of their texts and the reading comprehension they achieved.
In line with previous research (Cerdán & Vidal-Abarca, 2008 ; Le Bigot & Rouet, 2007 ; Wiley & Voss, 1999 ), we expect students who write a synthesis to obtain better results in a deeper-level, inferential comprehension test of multiple sources than students who only read the texts. We also predict that the media used for performing the two tasks would not have any differential impact on students’ comprehension results (Gil et al., 2010 ; Vidal-Abarca et al., 2011 ).
In the subsample that used R&A software, we assess the strategies used by students to solve reading/no synthesis and reading/synthesis tasks. In particular, for this subsample of participants, we aim to: (1) explore whether their use of rereading and note-taking was associated with the task they had been assigned; and (2) explore the relationship between the use of these strategies (and the quality of the synthesis written by those who did so) and students’ comprehension performance.
Firstly, we expect that participants in the reading/synthesis condition would engage in more rereading and note-taking than those in the reading/no synthesis condition. Secondly, we expect that rereading and note-taking (provided that the notes are not mere copies of the source texts) would be associated with a higher level of inferential comprehension in each condition. For participants in the reading/synthesis condition, we hypothesize that the use of these strategies would be associated with the quality of the synthesis written by the students in terms of selection and integration of relevant and accurate information and organization, if the notes taken and the synthesis text produced are not copied. This text quality would be associated with the level of inferential comprehension finally achieved.
Participants
The sample was comprised of 155 first-year undergraduate psychology students at a state-run Spanish university who volunteered to take part in the study at the beginning of the academic year. The sample included 115 women and 40 men with an overall mean age of 19.64 years ( SD = 4.92). All participants received all the details about the aims and tasks of the project. After giving their informed consent, they were randomly assigned to one of the four conditions depending on the task they were assigned and on the media they used to perform it (see Table 1 ): (1) reading/no synthesis, paper and pencil; (2) reading/synthesis, paper and pencil; (3) reading/no synthesis, Read&Answer ; and (4) reading/synthesis, Read&Answer .
Thus, some participants (n = 79; age: M = 20.19; SD = 6.16) performed the assigned task (either reading/no synthesis or reading/synthesis) on a computer and using the Read&Answer (R&A) software. We used this software as a means of keeping track of the strategies they used to face the assigned task—specifically, in order to register their rereading without making them read aloud (reading/no synthesis condition: 62.5% women; reading/synthesis condition: 71.8% women).
Finally, participants in the four conditions did not differ in their prior knowledge regarding the content of the texts they were asked to read to perform the task they were assigned (see Table 2 ) according to an ANOVA performed with task and media as factors. No main effects of the two factors were found either (task: F (1,156) = 0.001, p = 0.990, partial η 2 < 0.001; media: F (1,156) = 0.003, p = 0.955, partial η 2 < 0.001).
Three complementary texts about the current conception of intelligence, taken from reliable sources used in psychology, were adapted for the study. The first was an argumentative piece that makes a case for theories upholding the diverse character of intelligence as against unitary theories (973 words). The second was an expository piece that presented Gardner’s theory of multiple intelligences (909 words). The third, which was also expository, presented Sternberg’s concept of successful intelligence (611 words). The level of difficulty of the texts was considered suitable for higher education students by a panel of seven experts and according to the Flesch-Szigriszt Index (INFLESZ; adaptation by Barrio-Cantalejo et al., 2008 ) of readability. According to Barrio-Cantalejo et al. ( 2008 ), an INFLESZ value between 40 and 55 is considered appropriate for this age group, and the values obtained ranged from 43 to 47.
Understanding these three texts involved comparing, contrasting and integrating information. Three intertextual ideas that appeared in the texts were required for the correct completion of the task: (1) the contrast between intelligence as a unitary quality measured by testing and intelligence as a multiple, diverse competence; (2) the contrast between intelligence as an inherited trait and intelligence as a factor that can be modified by the influence of the environment; (3) the importance of maintaining a balance between intelligences and knowing how to use them appropriately (see “Appendix A” with two excerpts of the texts).
Prior knowledge questionnaire
A prior knowledge questionnaire about “intelligence” was produced, consisting of 15 statements whose reliability was based on CFA and corrected for test length using the Spearman-Brown extension = 0.64, see “Appendix C”). The participants had to indicate whether the statements were true or false, and the total number of correct answers was recorded (see examples in “Appendix B”).
Inferential comprehension test
We applied a verification task that consisted of 25 items that required students to make inferences of diverse complexity (intratextual and intertextual) (reliability based on CFA and corrected for test length using the Spearman–Brown extension = 0.83, see “Appendix C”). The intratextual inferential questions required students to infer information that was not explicit but could be deduced and was consistent with the text content, and/or to integrate information spread throughout the text (Graesser, et al., 2010 ). The intertextual inferential questions required students to link information contained in different source texts and/or combining prior knowledge with the information provided by the text (Castells et al., 2021 ). We decided to create a single test with deep-level inferential questions because this is the kind of task in which previous research has found the most significant differences (Cerdán & Vidal-Abarca, 2008 ; Gil et al., 2010 ; Wiley & Voss, 1999 ). The appropriateness of the questions asked in the test was assessed by a panel of seven experts. A pilot study was carried out to obtain additional information on appropriateness. As in the prior knowledge questionnaire, participants had to indicate whether the statements were true or false, and the total number of correct answers was recorded (see “Appendix B”).
The reliability of the prior knowledge test measure was lower than desired. However, other research in this area has used similar reliability scores (see Gil et al., 2010 ; Bråten & Strømsø, 2009 ), because methodologists (Gronlund, 1985 ; Kerlinger & Lee, 2000 ) argue that a reliability estimate may or may not be acceptable depending on how the measure is used and what type of decision is based on the measurements.
All participants performed the tasks in collective sessions lasting approximately 90 min. We collected data in a total of four sessions, one per condition (paper and pencil reading/no synthesis; paper and pencil reading/synthesis; R&A reading/no synthesis; R&A reading/synthesis). Each subsample performed the task in a different room supervised by a researcher, and once the students ended the task, they left the room. For participants in the paper and pencil conditions, the prior knowledge questionnaire was administered first. Once the students had finished it, they were given the three texts presented in the same handout in a counterbalanced form. The instructions for the reading task were: Below you will find three texts with information on the concept of intelligence. Read them carefully, paying attention to the most important information they provide on the subject (intelligence) because afterwards you will have to answer questions related to the content of the texts. You may read them as many times as you wish, highlighting phrases, writing notes, etc ., but you must answer the questions without the texts or your notes in front of you.
The instructions for the synthesis task added the following requirement to the instructions above: Write a text including and, above all, integrating, what is most important in the texts you have read on the topic (intelligence).
Students were also informed that when answering the comprehension questions they would not be allowed access to any of the materials (e.g., the synthesis text or the notes, if they had taken any).
The participants in the Read&Answer (R&A) conditions (i.e., computer-based environment) performed the assigned task (reading/no synthesis or reading/synthesis) in front of a computer on separate R&A screens, following the same procedure. Footnote 1
The R&A software (Vidal-Abarca et al., 2011 ) allows the collection of online data by registering some indicators of the reading process, e.g., the sequence of actions that were followed (sequence of segments of the text or specific question that participants read), the number of actions performed and the fragment of the text that was unmasked. R&A presents the texts and the questions or task on different screens in masked form. To unmask readers have to click on each segment of the text or task; only one segment is visible at a time. The participants can go back and forth through the text in any order, either on the same screen or between screens in longer texts (or when there is more than one source-text, as in this study) by clicking on the arrow icons. Likewise, they can go back and forth from the texts to the task screen by clicking on the question mark icon (see Fig. 1 ). The kind of masking that is used enables participants to see the layout of the text (i.e., the text structure, the form of paragraphs, headings, subheadings, etc.) even though the text is masked (as shown in Fig. 1 ).
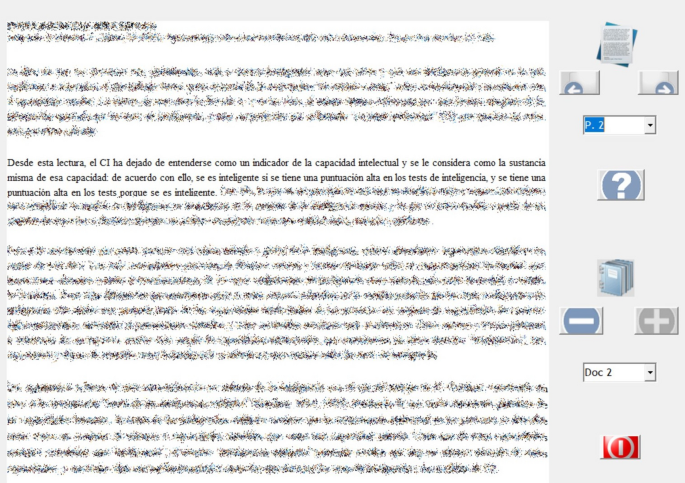
Read&Answer screen
The use of this software allows the collection of online data by recording all the participants’ actions. It provides some indicators of the reading process, such as the segments of the text or questions that were unmasked, the sequence in which they were accessed, and the time spent on each action.
The segmentation of the text that the participant can make visible by clicking on it is decided by the researcher during the planning stage of the experiment. For instance, we chose to segment the texts as follows: Text 1 was divided into 16 fragments, of which seven dealt with the intertextual ideas; Text 2 was divided into 12 fragments, of which four dealt with the intertextual ideas, and Text 3 was divided into nine fragments, of which three dealt with the intertextual ideas.
For both tasks, and as in the paper conditions, the participants were allowed to spend as long as they wanted on reading and on producing their written texts. Only when they decided to answer the reading comprehension test were the texts, notes and the synthesis text (for students in the reading/synthesis conditions) made inaccessible.
Analysis of text quality
Following on from previous studies (Boscolo et al., 2007 ; Nadal et al., 2021 ), we analyzed the quality of the texts, considering the dimensions presented in Table 3 . First, following on from Magliano et al. ( 1999 ), we segmented each synthesis into idea units. An idea unit contained a main verb. If an utterance had two verbs and one agent, it was treated as having two separate idea units (Gil et al., 2010 ). Then, following on from Luo and Kiewra ( 2019 ), the ideas were related to the source text they came from so as to obtain a picture of how students had organized and connected them in their own text. In this way, we were able to identify the number of main ideas that required intertextual connections (in a similar vein as Luo & Kiewra, 2019 ), which could be up to three. It also allowed us to identify the diverse types of organization (following on from Martínez et al., 2015 ; Nadal et al., 2021 ). Like Reynolds and Perin ( 2009 ) and Zhang ( 2013 ), we found mistakes or incomplete and incomprehensible ideas. In addition, we analyzed the degree of copying from the source texts, since this has been shown to be a relevant factor for explaining comprehension results (Luo & Kiewra, 2019 ).
Two independent raters, researchers who were not otherwise involved in the study, coded the first 20 texts. Two of the authors trained them in two sessions, providing them with the categories and the specific criteria, and using three written syntheses from the study that were not included in the random sampling. After that, the raters coded the first 20 texts (25% of all the texts, written either on paper or on screen). Interrater reliability was adequate for most dimensions (Relevant ideas, K = 0.67; Accuracy of content, K = 0.81; Textual organization, K = 0.84). Disagreements were resolved through discussion and then the rest of the syntheses were distributed between the two raters for evaluation.
Additionally, the percentage of verbatim copying from the three source texts in the synthesis text was calculated using the WCopyfind tool and by applying the criterion that seven words copied literally (subject [article + noun], verb, predicate [article/preposition + noun + complement]) represented one instance of literal copying (following on Nadal et al., 2021 ).
Finally, the percentage of copying from the notes in the synthesis was calculated using the same tool and applying a criterion of four words (subject [noun], verb, predicate [noun + complement]). In this case, we used this criterion because the notes were shorter than the three source texts (following on Van Weijen et al., 2018 ).
Analysis of the strategies in the R&A subsample
We analyzed the participants’ use of rereading and note-taking. In particular:
Rereading strategy
Students were allowed to go back and forth in the texts as they wanted (during reading and/or, for those in the reading/synthesis condition, while they wrote their synthesis as well). Looking at the R&A output, all unmasking actions of less than 1 s that were performed repeatedly and consecutively on the same segment were deleted, as they reflected accidental double-clicks on a segment when participants intended to unmask it. After doing so, all the unmasking actions registered by the software that constituted a revisit of a segment that had already been previously unmasked were considered as “rereading”. Regarding this strategy, we analyzed the number of rereadings , i.e., the number of times in which participants revisited some segment of the texts.
This variable was considered at two points of the process:
Number of rereadings during the initial reading of the text Rereadings that occurred before facing the assigned task (either taking the comprehension test or writing the synthesis and then taking the reading test, depending on the condition). We focused on this initial rereading because it allowed us to compare rereading between participants in the two conditions (reading/no synthesis vs. reading/synthesis).
Number of rereadings during writing of the synthesis text Additionally, for the students in the reading/synthesis condition, this variable was also considered by focusing on participants’ rereading while they composed their text, which gave us a measure of how hybrid their synthesis writing was.
Note-taking strategy
Students were allowed to take notes during the reading/no synthesis and the reading/synthesis task. Regarding this strategy, we analyzed the following variables:
Note-taking The presence or absence of note-taking during the execution of the task was coded dichotomously.
Percentage of copy from the source texts contained in the notes We used the WCopyfind tool, applying the criterion that four words copied literally (subject [noun], verb, predicate [noun + complement]) represented one instance of literal copying (following on Van Weijen et al., 2018 ).
Statistical analysis
After the analyses of the reading strategies and the written products, we performed different statistical analyses to meet our research aims.
Comprehension results for participants in the different conditions
Regarding the general sample (n = 155), and to explore whether there were differences in the comprehension performance of participants depending on the task and the media, we performed an ANCOVA analysis with inferential reading comprehension as the dependent variable (DV), tasks (reading/no synthesis vs. reading/synthesis) and media (paper and pencil vs. R&A) as factors, and prior knowledge as covariate.
Text quality in the different conditions and its relation to comprehension
In all participants assigned to the reading/synthesis task (n = 77) we tested whether there were differences in the textual organization, the accuracy of content, and the relevance of the ideas included in the synthesis they produced depending on the media used. To do so, we performed a Mann Whitney U test comparing the results obtained by participants using the R&A and those in the paper and pencil condition for each of the dimensions used to assess text quality (i.e., relevance of ideas, accuracy of content, and textual organization). Furthermore, and to test the relation between the different dimensions of text quality (textual organization, accuracy of content, and relevance of ideas) and inferential comprehension, we performed Spearman’s correlation analysis. Non-parametric analyses were chosen for these data because the variables related to text quality are ordinal.
Strategy used to address different tasks in the R&A participants
We explored whether participants in the R&A subsample used rereading and note-taking differently depending on the task they had been assigned by means of chi-square analysis and mean comparison analyses (either Student’s t test or Mann–Whitney U test, depending on the compliance of assumptions). Specifically, on the one hand, a chi-square analysis was performed to determine whether there was any association between the task (reading/no synthesis vs. reading/synthesis) and the presence or absence of note-taking. On the other hand, we compared participants in the reading/no synthesis task condition and participants in the reading/synthesis conditions on the remaining variables concerning the use of strategies (i.e., a Mann–Whitney U test was performed to compare the number of rereadings in the two groups of participants, due to non-normality of the DV; and a Student’s t test was done to compare the percentage of copying from the source texts contained in the notes).
Relationship between the use of strategies, the written products and students’ inferential comprehension performance in the R&A participants
Finally, we performed bi-serial correlational analyses to explore the associations between the participants’ use of strategies (and the quality of the synthesis written by those who did so) and their inferential comprehension test score. Based on the results of these correlations, we conducted regression and mediation analyses to identify possible impacts of independent variables on inferential comprehension.
Descriptive statistics for the inferential comprehension test score obtained by participants depending on the tasks they had been assigned to, and the media with which they performed the task are shown in Table 4 . We conducted analyses of covariance (ANCOVA), with the task (reading/synthesis vs. reading/no synthesis) and the media (paper and pencil/R&A) as the factors, prior knowledge as a covariate, and the inferential comprehension test score as the dependent variable.
The covariate, prior knowledge, was not significantly related to the comprehension test score, F (1, 149) = 2.195, p = 0.141, partial η 2 = 0.015. No statistically significant main effects were found either for the task, F (1, 149) = 1.183, p = 0.279, partial η 2 = 0.008, or for the media, F (1, 149) = 0.452, p = 0.502, partial η 2 = 0.003. Finally, no interaction was found between task and the media for the comprehension test score, F (1, 149) = 0.470, p = 0.494, partial η 2 = 0.003.
This result suggests that the media in which participants performed the task did not affect the comprehension results they obtained.
As explained in the Method section, participants in the reading/synthesis conditions wrote a synthesis after reading the source texts, and completed the inferential comprehension test once they had finished this text. Figure 2 presents the results of the analysis of the quality of their synthesis, according to the different categories established for doing so. Students tended to write short texts in terms of the number of words ( M = 285.28; SD = 106.81), without mistakes or incomprehensible ideas in most cases (59.5%), but few included only relevant ideas (8.1%); forty per cent produced juxtaposed summaries, while only 23% wrote a text with a clear structural axis).
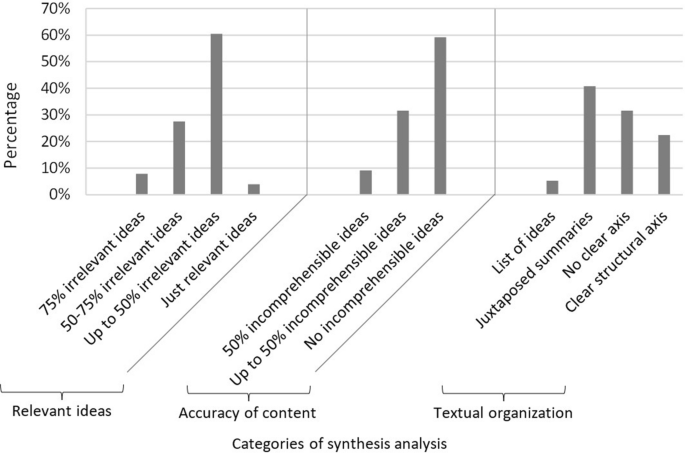
Percentage of participants in each of the categories for the synthesis analysis
The three dimensions considered for analyzing text quality significantly correlated with inferential comprehension (relevance of ideas: rho = 0.227, p = 0.049; accuracy of content: rho = 0.257, p = 0.025; textual organization: rho = 0.285, p = 0.013).
Mann–Whitney U tests to compare participants performing this task on different media showed no significant differences between them in the different dimensions considered for analyzing text quality (Relevant ideas: U = 595.5, p = 0.278; Accuracy of content: U = 504.5; p = 0.07; Textual organization: U = 581.5; p = 0.247). Thus, considering both this result and the ones presented in the previous section, in the following sections we will focus on the subsample of participants who performed the task with the R&A software.
Strategies used to address different tasks in the R&A participants
Note-taking was used by 40.51% of participants in the R&A subsample. The notes tended to be schematic, with a mean length of 210.38 words for those who did the reading/synthesis task ( SD = 134.47), and of 107 words for those who were assigned the reading/no synthesis task ( SD = 59.16).
A chi-square analysis was performed to determine whether there was any association between the task and the presence or absence of note-taking. The analysis revealed an association between these two variables [ χ 2 (1) = 5.688, p = 0.017, Cramer’s V = 0.268]: as shown in Table 5 , 65.6% of participants who decided to take notes had been told they had to write a synthesis later; conversely, 61.7% of the participants who did not take notes were in the reading/no synthesis condition. No expected values were below 5.
Student’s t test to compare participants performing the different tasks found no significant differences between them, either in the percentage of copying of source texts information in the notes, t (30) = 0.458, p = 0.650 (reading/no synthesis: M = 23.09 , SD = 13.51; reading/synthesis: M = 25.81, SD = 17.03) or in the number of rereadings during the initial reading of the text, U = 592.5, p = 0.830 (reading/no synthesis: M = 10.65, SD = 14.13; reading/synthesis: M = 10.12, SD = 14.06).
Relationship between the use of strategies, the written products and students’ inferential comprehension performance among R&A participants
Reading/no synthesis condition.
Table 6 displays the results of bi-serial correlations between the strategies carried out by participants (number of rereadings during the initial reading of the text, and presence/absence of note-taking), the percentage of copy in the notes that participants took, and the results obtained on different tests (prior knowledge and inferential comprehension).
Table 6 shows that none of the considered variables correlated with the inferential comprehension test scores obtained by students in the reading/no synthesis condition.
Reading/synthesis condition
For participants in the reading/synthesis condition, results for the different categories used to analyze the quality of the synthesis can be observed in Fig. 3 . Students tended to write short texts in terms of the number of words ( M = 285.28; SD = 106.81), without mistakes or incomprehensible ideas in most cases (71.8%), but few included only relevant ideas (5.1%) and a clear structural axis (28.2%).
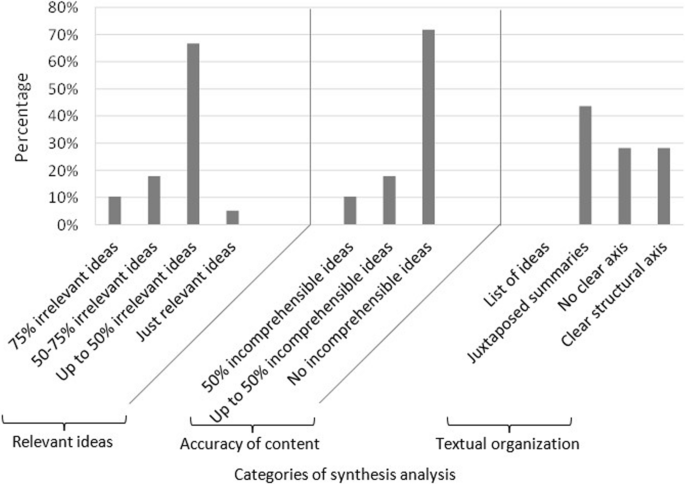
For this subsample of participants, bi-serial correlations were calculated between the variables referring to the strategies used by participants (i.e., number of rereadings during the initial reading, number of rereadings during writing of the synthesis, presence/absence of note-taking, percentage of copy from the source texts contained in the notes); the variables related to the written products [the three categories of synthesis analysis—relevant ideas, accuracy of content, and textual organization—the percentage of copying from the source texts ( M = 4.13, SD = 7.2), and the percentage of copying from the notes ( M = 24, SD = 25.71)]; and the prior knowledge and the inferential comprehension test scores (see Table 7 ).
Looking at the strategies used by the reading/synthesis condition participants, we observed that none of them correlates with inferential comprehension.
The only variables that correlate with the inferential comprehension scores are the categories of quality of the synthesis. To identify whether the three categories for analyzing the quality of the text (textual organization, accuracy of content and relevance of ideas) had a similar impact on inferential comprehension, we performed a stepwise regression analysis. The analysis of standard residuals showed that the data contained no outliers (Std. Residual Min = − 2.22, Std. Residual Max = 1.37). Tests to see whether the data met the assumption of collinearity indicated that multicollinearity was not a concern (Tolerance = 0.996, VIF = 1.004). The data met the assumption of independent errors (Durbin-Watson value = 1.927). The first model of the stepwise regression included the accuracy of content, and explained 9% of the variance ( R 2 = 0.097, F (1, 37) = 5.081, p = 0.03), while the second model included text structure, excluded relevant ideas, and explained 20% of the variance in inferential comprehension: R 2 = 0.203, F (1, 36) = 5.924, p = 0.02 (accuracy of content: β = 0.369, t (38) = 2.542, p = 0.015; textual organization: β = 0.353, t (38) = 2.434, p = 0.02).
Several categories of the quality of the synthesis correlated with the strategies assessed. Thus, we decided to conduct additional analyses in order to identify other potential relations. As shown in Table 7 , textual organization negatively correlated with note-taking ( p = 0.002, meaning that note-takers wrote syntheses with a worse textual organization) and with the percentage of copy from the source text in the synthesis ( p < 0.001, indicating that those who copied the source text most wrote syntheses with a worse textual organization). In order to look more closely at these results for textual organization, we performed a linear regression with textual organization as the dependent variable, and two independent variables: percentage of copy from the source texts in the synthesis and note-taking (a categorical variable: not taking notes was considered the reference group). We did not include other variables that correlated with the percentage of copy from the source text in the synthesis (specifically, the percentage of copy from the source texts in the notes, and the percentage of copy from the notes in the synthesis text), because the information for these two variables is only accessible for those who took notes and the variable note-taking becomes constant. An analysis of standard residuals showed that the data contained no outliers (Std. Residual Min = − 1.91, Std. Residual Max = 1.71). Tests to see whether the data met the assumption of collinearity indicated that multicollinearity was not a concern (Tolerance = 0.809, VIF = 1.236). The data met the assumption of independent errors (Durbin–Watson value = 2.145). The regression showed that these variables explained a significant proportion of variance in textual organization, R 2 = 0.371, F (2, 36) = 10.60, p < 0.001 (percentage of copy from source texts: β = − 0.298, t (36) = − 2.836, p = 0.007; note-taking: β = − 0.417, t (36) = − 2.025, p = 0.049).
Another result worth noting was that rereading during the initial reading correlated with the percentage of copy from the source text. To test whether this last variable could be mediating the effect of rereading during initial reading on textual organization, we conducted a mediation analysis (Hayes, 2013 ). The number of rereadings during the initial reading was the independent variable, the percentage of copy from the source texts was the mediator, and textual organization was the dependent variable. No significant indirect effect of the number of rereadings during the initial reading was found on textual organization through the percentage of copy from the source texts, ab = − 0.0153, BCa CI [− 0.04, 0.0022].
Finally, in view of the results shown in Table 7 , we performed a parallel multiple mediation analysis, to test whether the categories for text quality textual organization and accuracy of content could be mediating the effect of prior knowledge on inferential comprehension. The indirect effect of prior knowledge through textual organization is estimated as ab = 0.176, BCa CI [0.036, 0.37]. A second indirect effect of prior knowledge on inferential comprehension is modeled through accuracy of content, estimated as ab = 0.192, BCa CI [0.054, 0.36]. As a whole, 28.7% of the variance in inferential comprehension is explained by both proposed mediators and prior knowledge, p = 0.0017.
In this study, we aimed to establish whether asking students to write about the information available in three sources influenced their level of inferential reading comprehension compared to students who only read the three documents. We also assessed the potential impact of different media to solve the tasks, and the relationship between the categories used to analyze the quality of the synthesis (i.e., relevance of ideas; accuracy of content; and textual organization) and inferential reading comprehension. Additionally, in a subsample using R&A software, we sought to identify which of the strategies (i.e., rereading and note-taking) the students used to complete each task were associated with their inferential reading comprehension results.
Focusing on the general sample, since some of our students performed the tasks using a special software program ( Read&Answer ), we compared the results obtained using this program with those obtained performing the task on paper. In line with other studies (Cerdán et al., 2009 ; Gil et al., 2010 ), and also with our expectations, we did not find significant differences between students who completed the task in the two different media. In addition, although several meta-analyses (Clinton, 2019 ; Delgado et al., 2018 ) have shown that reading on screen can decrease the level of comprehension, this effect was not visible in our study, possibly because we did not set time restrictions to accomplish the tasks. Focusing on the task variable, the students who had the opportunity to produce a written product of an integrative type did not obtain better comprehension results than those whose task consisted exclusively of reading. This unexpected result is at odds with the findings of other studies, such as Graham and Hebert ( 2011 ), and Hebert et al. ( 2013 ). However, it is important to note that those two meta-analyses compared writing interventions and their impact on comprehension, while in our study we did not instruct our students how to write the synthesis. As Graham and Hebert ( 2011 ) mentioned, it is possible that the impact of writing on comprehension is less visible among higher education students carrying out more complex tasks, such as synthesizing from multiple documents.
A possible explanation for this result, which contradicts our initial hypothesis, may be found not so much in the type of task the students were assigned, but in the strategies they chose in order to carry out the tasks, and the quality of the written product in the reading/synthesis condition. Starting with the last variable, the categories considered to assess the quality of the written product significantly correlated with the level of inferential reading comprehension, meaning that students who wrote a better synthesis, in terms of textual organization, accuracy of content and relevance of the ideas included, also obtained better results in the comprehension test whether they performed the task on paper or with R&A, a result that has also been observed in prior research (Martínez et al., 2015 ). In fact, the accuracy of content and the textual organization explained 20% of the variance of inferential comprehension for the students who wrote the synthesis. This result points to the importance of correctly selecting and organizing the information as a means to deepen and understand the sources, in line with other research findings (Solé et al., 2013 ).
Regarding the strategies deployed by participants in the reading/no synthesis versus the reading/synthesis condition, the results show that in both situations, independently of the task assigned and contrary to our expectations, students mostly reread the text several times. Nevertheless, and in line with our expectations, the students in the reading/synthesis condition tended to take notes more than their peers in the reading/no synthesis condition. Since these students were required to write a text that involved integrating information from several sources, it is logical that they used note-taking to a higher extent, as we expected.
Although neither of these strategies (rereading and note-taking) was related to inferential comprehension for the students in both conditions, for the reading/synthesis condition, note-taking and the percentage of copy from the source texts in the synthesis explained 37% of the variance of the textual organization, meaning that those students who took notes mostly copied from the source texts and achieved low textual organization scores. Conversely, students who did not take notes and did not copy from the source texts were able to provide a better structure to their synthesis. In agreement with prior research (Gil et al., 2008 ; Hagen et al., 2014 ), students’ notes in this condition were mostly copied fragments from the source texts, and note-takers apparently trusted these notes to write their synthesis instead of going back to the source texts, which hypothetically may have led them to write lower-quality syntheses and to obtain worse inferential comprehension results than students who did not take notes. Following on from Kobayashi ( 2009 ) and Luo and Kiewra ( 2019 ), it may be the case that, when students have not been taught how to use this tool strategically, they simply use it routinely, focusing mainly on superficial information from the texts and copying information instead of elaborating upon it. In contrast to other studies of text comprehension (Gil et al., 2010 ; Kintsch, 1998 ; Le Bigot & Rouet, 2007 ), we did not find a relationship between prior knowledge and the inferential comprehension results in the correlation analyses. Although it might be thought that the questionnaire lacked the sensitivity required to assess prior knowledge, one of the results seems to point to a more complex explanation. In fact, for the students in the reading/synthesis condition, prior knowledge correlated with two of the categories of the quality of the synthesis (text structure and accuracy of the content), meaning that the students who had a higher degree of prior knowledge were also able to better organize and include accurate information in their syntheses. For this reason, we decided to perform mediation analyses of prior knowledge on inferential comprehension scores, through the two previous synthesis categories. The results showed significant indirect effects of prior knowledge on inferential comprehension through text organization and accuracy of content. In sum, although background knowledge did not play a direct role in comprehending the texts in depth, it was relevant to helping students to produce better syntheses.
A final comment should be made regarding the category of the synthesis quality “relevant ideas”. Although this category correlated significantly with the inferential comprehension, it did not correlate with prior knowledge and did not show a clear impact on inferential comprehension when introduced in the regression analysis. This result may be related to the fact that identifying the three main ideas shared by the texts did not prevent students from just copying them in their text without expanding their understanding of the sources’ content.
Several limitations of the present study must be pointed out. The first is to do with the inferential comprehension test. Although it might be argued that using other comprehension scales (e.g., literal comprehension), might have produced additional results, higher education students are expected to achieve deep inferential comprehension when studying from several texts. Therefore, it seems important to focus on this level of comprehension, in which most studies on understanding multiple sources have found significant differences.
A second possible limitation is the “artificial” nature of the software program ( Read&Answer ). Although we did not find differences between the paper condition and the R&A condition in the inferential comprehension results, we cannot guarantee that the use of this software did not have an impact on the strategies students performed when solving the tasks. Although studies that have used this tool suggest that it does not have a relevant impact on participants’ cognitive processes (see Cerdán et al., 2009 ; Gil et al., 2010 ), and it has been validated against eye-tracking and paper and pencil testing (Vidal-Abarca et al., 2011 ), its effect may depend on the complexity of the task. A third important limitation is the possibility that the reading/synthesis condition may have appeared artificial to the students. It is conceivable that at least some of the students would not have spontaneously written a synthesis as a way of improving their comprehension of the texts. As this was an assigned task, neither decided by them, nor assessed by their professors, nor linked to a subject, its potential may be diminished because it was produced routinely and not conducted strategically, as shown by the students’ reliance on the notes which were mostly copied from the texts.
Despite its limitations, however, our study contributes to the understanding of the relationship of synthesis writing (and the strategies undertaken to perform it) on inferential comprehension. Our results suggest the importance not only of using specific strategies to carry out reading and/or writing tasks (e.g., rereading during writing, or note-taking) but of using them strategically to fulfil the aims and to adapt to the demands of the task. Thus, for example, students tended to take notes to a higher extent when they were required to write a synthesis, a practice that seems eminently suitable in view of the complexity of the task; however, as we argued above, the key issue is how these notes are used. On the other hand, the quality of the written products seems to be crucial to improving reading comprehension. Thus, it is not the task itself, but the way students meet its requirements, that determines the quality of the result. Our results for synthesis quality confirm that students’ ability to integrate information from different sources cannot be taken for granted, and emphasize once again the need for specific writing instruction in higher education.
Data availability
Availability of data and material under request.
Participants in the paper and pencil conditions received all instructions and source texts on paper, performed the assigned task on paper, and were allowed to take notes on a separate sheet. Participants in the R&A conditions received all instructions and source-text on screen, performed the assigned task on screen, and were allowed to take notes on paper if they wished. We chose not to include an additional screen on R&A to take notes so as to avoid complicating the procedure with this software for participants and causing confusion between the notes screen and the synthesis screen, or to avoid the risk that some students might regard this as a requirement rather than as a strategy that they could decide to use or not. The average time taken to complete the tasks by the students in the R&A condition was 60 min for the reading/synthesis and 40 min for the reading/no synthesis condition.
Afflerbach, P., Pearson, P. D., & Paris, S. G. (2008). Clarifying differences between reading skills and reading strategies. The Reading Teacher, 61 (5), 364–373. https://doi.org/10.1598/RT.61.5.1
Article Google Scholar
Applebee, A. N. (1984). Writing and reasoning. Review of Educational Research, 54 (4), 577–596. https://doi.org/10.3102/00346543054004577
Baker, W., & Boonkit, K. (2004). Learning strategies in reading and writing: EAP contexts. RELC Journal, 35 (3), 299–328. https://doi.org/10.1177/0033688205052143
Bangert-Drowns, R. L., Hurley, M. M., & Wilkinson, B. (2004). The effects of school-based writing-to-learn interventions on academic achievement: A meta-analysis. Review of Educational Research, 74 (1), 29–58. https://doi.org/10.3102/00346543074001029
Barrio-Cantalejo, I. M., Simón-Lorda, P., Melguizo, M., Escalona, I., Mirajúan, M. I., & Hernando, P. (2008). Validation of the INFLESZ scale to evaluate readability of texts aimed at the patient. Anales Del Sistema Sanitario De Navarra, 31 (2), 135–152. https://doi.org/10.4321/s1137-66272008000300004
Barzilai, S., Zohar, A. R., & Mor-Hagani, S. (2018). Promoting integration of multiple texts: A review of instructional approaches and practices. Educational Psychology Review, 30 (3), 1–27. https://doi.org/10.1007/s10648-018-9436-8
Basaraba, D., Yovanoff, P., Alonzo, J., & Tindal, G. (2013). Examining the structure of reading comprehension: Do literal, inferential, and evaluative comprehension truly exist? Reading and Writing, 26 , 349–379. https://doi.org/10.1007/s11145-012-9372-9
Bednall, T. C., & Kehoe, E. J. (2011). Effects of self-regulatory instructional aids on self-directed study. Instructional Science, 39 (2), 205–226. https://doi.org/10.1007/s11251-009-9125-6
Boscolo, P., Arfé, B., & Quarisa, M. (2007). Improving the quality of students’ academic writing: An intervention study. Studies in Higher Education, 32 (4), 419–438. https://doi.org/10.1080/03075070701476092
Bråten, I., & Strømsø, H. I. (2009). Effects of task instruction and personal epistemology on the understanding of multiple texts about climate change. Discourse Processes, 47 (1), 1–31. https://doi.org/10.1080/01638530902959646
Britt, M. A., Perfetti, C. A., Sandak, R., & Rouet, J. F. (1999). Content integration and source separation in learning from multiple texts. In S. R. Goldman, A. C. Graesser, & P. van den Broek (Eds.), Narrative comprehension, causality, and coherence: Essays in honor of Tom Trabasso (pp. 209–233). Erlbaum.
Google Scholar
Britt, M. A., Rouet, J. F., & Durik, A. M. (2017). Literacy beyond text comprehension: A theory of purposeful reading . Taylor & Francis.
Book Google Scholar
Castells, N., Minguela, M., Solé, M., Miras, M., Nadal, E., & Rijlaarsdam, G. (2021). Improving questioning-answering strategies in learning from multiple complementary texts: An intervention study. Reading Research Quartery, 57 (3), 879–912. https://doi.org/10.1002/rrq.451
Cerdán, R., & Vidal-Abarca, E. (2008). The effects of tasks on integrating information from multiple documents. Journal of Educational Psychology, 100 (1), 209–222. https://doi.org/10.1037/0022-0663.100.1.209
Cerdán, R., Vidal-Abarca, E., Salmerón, L., Martínez, T., & Gilabert, R. (2009). Read&Answer: A tool to capture on-line processing of electronic texts. The Ergonomics Open Journal, 2 , 133–140.
Clinton, V. (2019). Reading from paper compared to screens: A systematic review and meta-analysis. Journal of Research in Reading, 42 (2), 288–325. https://doi.org/10.1111/1467-9817.12269
Delgado, P., Vargas, C., Ackerman, R., & Salmerón, L. (2018). Don’t throw away your printed books: A meta-analysis on the effects of reading media on reading comprehension. Educational Research Review, 25 , 23–38. https://doi.org/10.1016/j.edurev.2018.09.003
Dinsmore, D. L. (2018). Strategic processing in education . Routledge.
Dovey, T. (2010). Facilitating writing from sources: A focus on both process and product. Journal of English for Academic Purposes, 9 (1), 45–60. https://doi.org/10.1016/j.jeap.2009.11.005
Fitzgerald, J., & Shanahan, T. (2020). Reading and writing relationships and their development. Educational Psychologist, 35 (1), 39–50. https://doi.org/10.1207/S15326985EP3501_5
Galbraith, D., & Baaijen, V. M. (2018). The work of writing: Raiding the inarticulate. Educational Psychologist, 53 (4), 238–257. https://doi.org/10.1080/00461520.2018.1505515
Gil, L., Bråten, I., Vidal-Abarca, E., & Strømsø, H. I. (2010). Summary versus argument tasks when working with multiple documents: Which is better for whom? Contemporary Educational Psychology, 35 (3), 157–173. https://doi.org/10.1016/j.cedpsych.2009.11.002
Gil, L., Vidal-Abarca, E., & Martínez, T. (2008). Efficacy of note-taking to integrate information from multiple documents. Infancia y Aprendizaje, 31 (2), 259–272. https://doi.org/10.1174/021037008784132905
Goldman, S. R., & Saul, E. U. (1990). Flexibility in text processing: A strategy competition model. Learning and Individual Differences, 2 (2), 181–219. https://doi.org/10.1016/1041-6080(90)90022-9
Graham, S., & Hebert, M. (2011). Writing to read: A meta-analysis of the impact of writing and writing instruction on reading. Harvard Educational Review, 81 (4), 710–744. https://doi.org/10.17763/haer.81.4.t2k0m13756113566
Graesser, A., Ozuru, Y., & Sullins, J. (2010). What is a good question? In M. G. McKeown & L. Kucan (Eds.), Bringing research to life (pp. 112–141). Guilford Press.
Gronlund, N. E. (1985). Measurement and evaluation in teaching . MacMillan.
Hagen, Å. M., Braasch, J. L., & Bråten, I. (2014). Relationships between spontaneous note-taking, self-reported strategies and comprehension when reading multiple texts in different task conditions. Journal of Research in Reading, 37 (1), 141–157. https://doi.org/10.1111/j.1467-9817.2012.01536.x
Hayes, A. F. (2013). Introduction to mediation, moderation, and conditional process analysis: A regression based approach . Guilford Publications.
Hebert, M., Simpson, A., & Graham, S. (2013). Comparing effects of different writing activities on reading comprehension: A meta-analysis. Reading and Writing, 26 (1), 111–138. https://doi.org/10.1007/s11145-012-9386-3
Hyöna, J., Lorch, R. F., & Kaakinen, J. (2002). Individual differences in reading to summarize expository texts: Evidence from eye fixation patterns. Journal of Educational Psychology, 94 (1), 44–55. https://doi.org/10.1037/0022-0663.94.1.44
Kerlinger, F. N., & Lee, H. B. (2000). Foundations of behavioral research . Harcourt College Publishers.
Kintsch, W. (1998). Comprehension: A paradigm for cognition . Cambridge University Press.
Kirby, J. R. (1988). Style, strategy, and skill in reading. In R. R. Schmeck (Ed.), Learning strategies and learning styles (pp. 229–274). Springer.
Chapter Google Scholar
Kobayashi, K. (2009). Comprehension of relations among controversial texts: Effects of external strategy use. Instructional Science, 37 (4), 311–324. https://doi.org/10.1007/s11251-007-9041-6
Le Bigot, L., & Rouet, J. F. (2007). The impact of presentation format, task assignment, and prior knowledge on students’ comprehension of multiple online documents. Journal of Literacy Research, 39 (4), 445–470. https://doi.org/10.1080/10862960701675317
Lenski, S. D., & Johns, J. L. (1997). Patterns of reading-to-write. Reading Research and Instruction, 37 (1), 15–38. https://doi.org/10.1080/19388079709558252
List, A., & Alexander, P. A. (2019). Toward an integrated framework of multiple text use. Educational Psychologist, 54 , 20–39. https://doi.org/10.1080/00461520.2018.1505514
Lord, F. M., & Novick, M. R. (2008). Statistical theories of mental test scores . IAP.
Luo, L., & Kiewra, K. A. (2019). Soaring to successful synthesis writing: An investigation of SOAR strategies for college students writing from multiple sources. Journal of Writing Research, 11 (1), 163–209. https://doi.org/10.17239/jowr-2019.11.01.06
Magliano, J. P., Trabasso, T., & Graesser, A. C. (1999). Strategic processing during comprehension. Journal of Educational Psychology, 91 (4), 615–629. https://doi.org/10.1037/0022-0663.91.4.615
Martínez, I., Mateos, M., Martín, E., & Rijlaarsdam, G. (2015). Learning history by composing synthesis texts: Effects on an instructional programme on learning, reading and writing processes, and text quality. Journal of Writing Research, 7 , 275–302. https://doi.org/10.17239/jowr-2015.07.02.03
Mateos, M., & Solé, I. (2009). Synthesising information from various texts: A study of procedures and products at different educational levels. European Journal of Psychology of Education, 24 (4), 435–451. https://doi.org/10.1007/BF03178760
McGinley, W. (1992). The role of reading and writing while composing from multiple sources. Reading Research Quarterly, 27 (3), 227–248. https://doi.org/10.2307/747793
Minguela, M., Solé, I., & Pieschl, S. (2015). Flexible self-regulated reading as a cue for deep comprehension: Evidence from online and offline measures. Reading & Writing, 28 (5), 721–744. https://doi.org/10.1007/s11145-015-9547-2
Miras, M., Solé, I., & Castells, N. (2013). Creencias sobre lectura y escritura, producción de síntesis escritas y resultados de aprendizaje [Reading and writing beliefs, written synthesis production and learning results]. Revista Mexicana De Investigación Educativa, 18 (57), 437–459.
Moran, R., & Billen, M. (2014). The reading and writing connection: Merging two reciprocal content areas. Georgia Educational Researcher . https://doi.org/10.20429/ger.2014.110108
Nadal, E., Miras, M., Castells, N., & de la Paz, S. (2021). Intervención en escritura de síntesis a partir de fuentes: Impacto de la comprensión [Intervention in writing a synthesis based on sources: Impact of comprehension]. Revista Mexicana De Investigación Educativa, 26 (88), 95–122.
Nelson, N. (2008). The reading-writing nexus in discourse research. In C. Bazerman (Ed.), Handbook of research on writing: History, society, school, individual, text (pp. 435–450). Erlbaum.
Nelson, N., & King, J. R. (2022). Discourse synthesis: Textual transformations in writing from sources. Reading and Writing . https://doi.org/10.1007/s11145-021-10243-5
Reynolds, G., & Perin, D. (2009). A comparison of text structure and self-regulated writing strategies for composing from sources by middle school students. Reading Psychology, 30 (3), 265–300. https://doi.org/10.1080/02702710802411547
Scardamalia, M., & Bereiter, C. (1987). Knowledge telling and knowledge transforming in written composition. In S. Rosenberg (Ed.), Cambridge monographs and texts in applied psycholinguistics. Advances in applied psycholinguistics: Reading, writing and language learning (pp. 142–175). Cambridge University Press.
Schumacher, G. M., & Nash, J. G. (1991). Conceptualizing and measuring knowledge change due to writing. Research in the Teaching of English, 25 (1), 67–96.
Solé, I., Miras, M., Castells, N., Espino, S., & Minguela, M. (2013). Integrating information: An analysis of the processes involved and the products generated in a written synthesis task. Written Communication, 30 (1), 63–90. https://doi.org/10.1177/0741088312466532
Spivey, N. (1997). The constructivist metaphor: Reading, writing and the making of meaning . Academic Press.
Spivey, N. N., & King, J. R. (1989). Readers as writers composing from sources. Reading Research Quarterly, 24 (1), 7–26.
Sternberg, R. J. (1997). Successful intelligence: How practical and creative intelligence determine success in life . Plume.
Stine-Morrow, E. A. L., Gagne, D. D., Morrow, D. G., & DeWall, B. H. (2004). Age differences inrereading. Memory and Cognition, 32 (5), 696–710. https://doi.org/10.3758/BF03195860
Tierney, R. J., & Shanahan, T. (1996). Research on the reading-writing relationship: Interactions, transactions, and outcomes. In R. Barr, M. L. Kamil, P. B. Mosenthal, & P. D. Pearson (Eds.), Handbook of Reading Research (pp. 246–280). Erlbaum.
Vandermeulen, N., Van den Broek, B., Van Steendam, E., & Rijlaarsdam, G. (2019). In search of an effective source use pattern for writing argumentative and informative synthesis texts. Reading and Writing, 33 (2), 239–266. https://doi.org/10.1007/s11145-019-09958-3
Van Weijen, D., Rijlaarsdam, G., & Van Den Bergh, H. (2018). Source use and argumentation behavior in L1 and L2 writing: A within-writer comparison. Reading and Writing, 32 (6), 1635–1655. https://doi.org/10.1007/s11145-018-9842-9
Vidal-Abarca, E., Martinez, T., Salmerón, L., Cerdán, R., Gilabert, R., Gil, L., Mañá, A., Llorens, A. C., & Ferris, R. (2011). Recording online processes in task-oriented reading with Read&Answer. Behavior Research Methods, 43 (1), 179–192. https://doi.org/10.3758/s13428-010-0032-1
Wiley, J., & Voss, J. F. (1999). Constructing arguments from multiple sources: Tasks that promote understanding and not just memory for text. Journal of Educational Psychology, 91 (2), 301–311. https://doi.org/10.1037//0022-0663.91.2.301
Zhang, C. (2013). Effect of instruction on ESL students’ synthesis writing. Journal of Second Language Writing, 22 (1), 51–67. https://doi.org/10.1016/j.jslw.2012.12.001
Download references
Open Access funding provided thanks to the CRUE-CSIC agreement with Springer Nature. This research was funded by the Spanish Ministery of Science, Innovation and Universities (Ref: RTI2018-097289-B-I00).
Author information
Authors and affiliations.
Department of Cognition, Developmental and Educational Psychology, Faculty of Psychology, Universitat de Barcelona, Pg. de la Vall d’Hebrón, 171, 08035, Barcelona, Spain
Núria Castells, Marta Minguela & Esther Nadal
You can also search for this author in PubMed Google Scholar
Corresponding author
Correspondence to Núria Castells .
Ethics declarations
Conflict of interest.
The authors declare that they have no conflict of interest.
Ethical approval
This manuscript has not been published elsewhere and is not under consideration by another journal.
Additional information
Publisher's note.
Springer Nature remains neutral with regard to jurisdictional claims in published maps and institutional affiliations.
Segments of the source texts in which the intertextual idea of the “contrast between intelligence as a unitary quality measured by testing, and intelligence as a multiple, diverse competence” can be found
Text 1: multiple intelligences.
“The idea that people are, globally, more or less intelligent than others, and that this general intelligence is what conditions or determines learning, is an essential part of the "common sense" conception of intelligence and its relationship with school learning. The strength of this idea has led to—and has been, at the same time, reinforced by—a simplistic interpretation of the information provided by intelligence tests, and in particular by the so-called "Intelligence Quotient" (IQ) that many of these tests make it possible to calculate.
From this interpretation, IQ has ceased to be understood as an indicator of intellectual capacity and is considered as the very substance of that ability: according to this, you are intelligent if you have a high score on intelligence tests, and you have a high score on tests because you are intelligent. With this, what at first was nothing but a more or less convenient artifact to simplify the measure of intelligence becomes the essence of intelligence itself, and what had been measured from a set of different aspects or factors ends up being conceived as a single, unitary and uniform entity.
As compelling as this unitary and global view of intelligence may seem, there are abundant scientific arguments against this idea. The most forceful come from various authors and recent theories on intellectual behavior, which have as a common element the questioning of traditional intelligence tests as an appropriate method of measuring and studying it. Despite the differences among them, these authors agree that traditional intelligence tests only measure a small part of people's intellectual abilities: a set of logical-mathematical capacities, associated with scientific thinking and the more academic school content. These authors and theories propose the existence of a much broader spectrum of intellectual capacities, which would constitute, in their own right, "intelligences", as important and worthy of attention as the academic intelligence to which all intelligence tests refer."
Text 2: two essential claims about intelligence
“The theory of multiple intelligences makes two complementary claims. First, the theory is a complete explanation of human cognition: it presents intelligences as a new definition of the nature of human beings from a cognitive point of view. Whereas Socrates saw man as a rational animal and Freud emphasised the irrationality of the human being, I have (with due caution) described the human being as an organism possessing a basic set of seven, eight or a dozen intelligences.
(…) The second assertion—that we all have a unique combination of intelligences—leads to the most important consequence of this theory for the next millennium. We can choose to ignore this uniqueness, we can choose to minimise it, or we can choose to enjoy it. I believe that the great challenge of deploying human resources is to find the best way to take advantage of the uniqueness we have been given as a species: that of having multiple intelligences.”
Text 3: successful intelligence
"Successful intelligence is, according to Sternberg ( 1997 ), that which is really important in life, that which is used to achieve important goals and that shown by those who have succeeded in life, either according to their personal patterns, or according to those of others. This intelligence has little to do with the intelligence measured by traditional tests and IQ scores. According to Sternberg, these tests refer only to a small and not very important (though academically overrated) part of a much broader and more complex intellectual spectrum, and essentially measure "inert intelligence", that is, potentialities that do not necessarily lead to a movement or a directed action, which one does not have to know how to use in order to produce real changes in life, for oneself or for others. According to Sternberg, the notion that there is a general intelligence factor that can be measured with IQ is false, and is based on the fact that all traditional intelligence tests measure essentially the same narrow range of skills.
For Sternberg, successful intelligence involves three aspects: an analytical aspect, a creative aspect and a practical aspect. The first is used to solve problems; the second, to decide which problems to solve; and the third, to put the solutions into practice. Conventional intelligence tests measure only the analytical aspect of intelligence, and not even completely. These three aspects are considered relatively independent of each other, and in fact each of them is conceptualized as a specific intelligence. In doing so, Sternberg points to the multiple, non-unitary character of intelligence."
Sample of prior knowledge test questions (true/false)
Intelligence is a general ability that each person has to a certain degree. T/F
What a person learns modifies their intelligence. T/F
Success in life is a more reliable indicator of a person’s intelligence than his/her own IQ. T/F
Sample of inferential reading comprehension questions (true/false)
From the pluralist theories of intelligence, the balance and relationship between the different types of intelligence of a person is more important than the fact that he/she may be more or less gifted in any one of them. T/F
Despite defending the plural nature of people's intellectual abilities, the theories of Gardner and Sternberg accept that IQ reflects the overall degree of these abilities. T/F
Reliability calculation process
According to Lord and Novick ( 2008 ), if the true-score variance of the items vary, Cronbach’s alpha is an underestimate of the true reliability to and unknown height. Therefore it is preferable to use and estimate of the reliability that is not affected by differences in true-score variance between items (H. Van den Bergh, personal communication, May 19, 2022). For this reason, one-factor CFA models were conducted with the Prior knowledge questionnaire (see Fig. 4 ), and with the Inferential comprehension test (see Fig. 5 ), and, since the models fitted the data (Prior knowledge questionnaire: RMSEA (95% CI) = 0.063 (0.044; 0.082); X 2 = 145.385; df = 90; p < 0.001; x 2 /df = 1.615; Inferential comprehension test: RMSEA (95% CI) = 0.04471 (0.03041; 0.05715); X 2 = 358.539; df = 275; p < 0.001; x 2 /df = 1.304), the regression of the items on the factor was interpreted as the proportion of true-score variance. Taking the average proportion of true-score variance, the Spearman–Brown extension for lengthening a test was calculated.
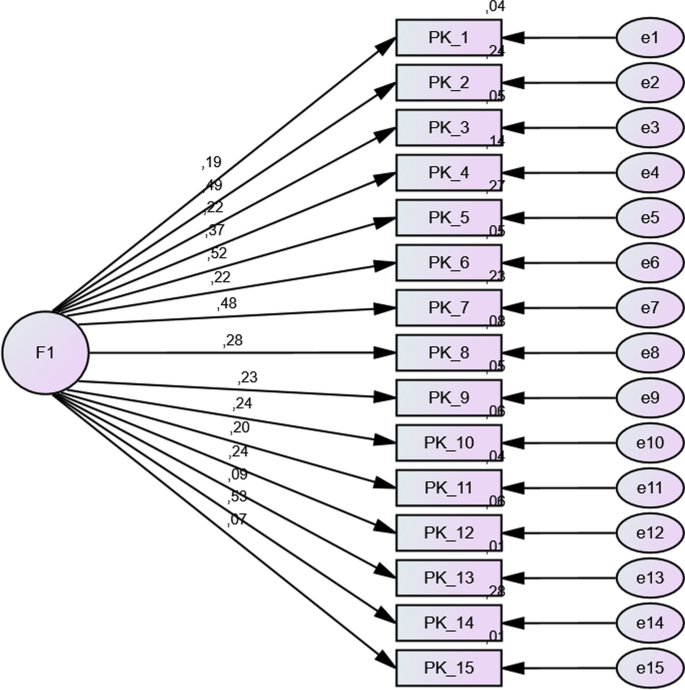
Inferential question items and their loadings (regression values of the items on the factor are presented in the middle of the arrows)
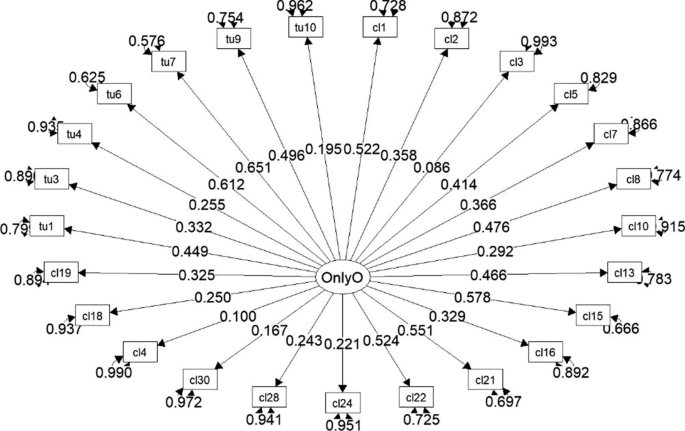
Rights and permissions
Open Access This article is licensed under a Creative Commons Attribution 4.0 International License, which permits use, sharing, adaptation, distribution and reproduction in any medium or format, as long as you give appropriate credit to the original author(s) and the source, provide a link to the Creative Commons licence, and indicate if changes were made. The images or other third party material in this article are included in the article's Creative Commons licence, unless indicated otherwise in a credit line to the material. If material is not included in the article's Creative Commons licence and your intended use is not permitted by statutory regulation or exceeds the permitted use, you will need to obtain permission directly from the copyright holder. To view a copy of this licence, visit http://creativecommons.org/licenses/by/4.0/ .
Reprints and permissions
About this article
Castells, N., Minguela, M. & Nadal, E. Writing a synthesis versus reading: strategies involved and impact on comprehension. Read Writ 36 , 849–880 (2023). https://doi.org/10.1007/s11145-022-10341-y
Download citation
Accepted : 31 July 2022
Published : 20 August 2022
Issue Date : April 2023
DOI : https://doi.org/10.1007/s11145-022-10341-y
Share this article
Anyone you share the following link with will be able to read this content:
Sorry, a shareable link is not currently available for this article.
Provided by the Springer Nature SharedIt content-sharing initiative
- Multiple source comprehension
- Synthesis text
- Note-taking
- Inferential comprehension
Advertisement
- Find a journal
- Publish with us
- Track your research
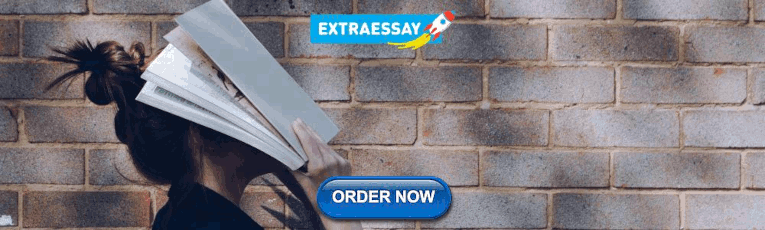
IMAGES
VIDEO
COMMENTS
Follow rules for precis writing. Stick to the word limit provided. Steps. Step 1: Read the given passage, highlight and underline the important points and note down the keywords in the same order as in the original passage. Step 2: Note down the central theme/gist of the passage and tone of the author.
After exploring the differences between composition and comprehension, it is evident that both are essential components of effective communication. While composition refers to the technical aspects of writing, such as grammar, syntax, and punctuation, comprehension involves understanding the meaning and context of a message.
Comprehension refers to an individual's ability to understand a text whereas composition is the creation of something like a written work. Thus, composition and comprehension are intrinsically linked together. The main difference between composition and comprehension is that composition involves creating something whereas comprehension ...
For many years, reading instruction was based on a concept of reading as the application of a set of isolated skills such as identifying words, finding main ideas, identifying cause and effect relationships, comparing and contrasting and sequencing. Comprehension was viewed as the mastery of these skills. One important classroom study conducted ...
An essay is a focused piece of writing designed to inform or persuade. There are many different types of essay, but they are often defined in four categories: argumentative, expository, narrative, and descriptive essays. Argumentative and expository essays are focused on conveying information and making clear points, while narrative and ...
Choosing between an essay and a composition can depend on the context in which they are used. Both terms are often used interchangeably, but the context can make a difference in the choice. Examples Of Different Contexts. Here are some examples of different contexts and how the choice between essay and composition might change:
Silva (1993), in a review of differences between writing in a first and second language, found that writing in a second ... (English grade, reading and listening comprehension, self-concept) are related to the length of their essays (word count). ... All students answered two independent and two integrated essay writing prompts of the internet ...
Comparison Table. The essay's main purpose is to cause the reader to reflect on a particular topic declaring the author's opinion. The composition's main purpose is to describe the topic and express the author's feelings. An author's position and thoughts on the current topic must be clearly understood from the essay.
Expert answer. There is a very strong relationship between reading and writing. First, reading and writing are both functional activities that can be used to accomplish a task. Second, reading and writing draw upon the same skills, knowledge, and processes in terms of being able to read and comprehend text and in terms of being able to write text.
Student with good background knowledge can open up a comprehension paper on any range of topics and quickly understand what the writer is talking about. In their composition paper, they can easily pull out interesting and well-developed ideas for any question they are asked. Unfortunately, Pokémon Go is unlikely to be the sole topic of exams.
Let us first look at the meaning of composition. A composition can refer to any creative work, be it a short story, poem, essay, research paper or a piece of music. Therefore, the main difference between essay and composition is that essay is a type of composition whereas composition refers to any creative work.
Based on these differences between stories and essays, researchers have long theorized that narratives might have an advantage over expository texts when it comes to memory and comprehension. ... results remained the same with the difference between memory and comprehension failing to attain statistical significance (p = .31). Publication bias ...
Source-based essays are evaluated both on the quality of the writing and the content appropriate interpretation and use of source material. Hence, composing a high-quality source-based essay (an essay written based on source material) relies on skills related to both reading (the sources) and writing (the essay) skills. As such, source-based writing must involve language comprehension and ...
Based on these differences between stories and essays, researchers have long theorized that narratives might have an advantage over expository texts when it comes to memory and comprehension. ... However, results remained the same with the difference between memory and comprehension failing to attain statistical significance (p = .31 ...
Inferential comprehension is necessary to connect ideas in a text together in a meaningful manner. There have been multiple studies on inferential comprehension involving texts of different genres (narrative and expository), but not a coherent overview of the findings of inferential comprehension by genre. The purpose of this study is to provide a coherent overview by conducting a meta ...
Abstract. We investigated developmental trajectories of and the relation between reading and writing (word reading, reading comprehension, spelling, and written composition), using longitudinal data from students in Grades 3 to 6 in the US. Results revealed that word reading and spelling were best described as having linear growth trajectories ...
Personal recount essay. Descriptive essay. Reflective essay. Discursive essay. Argumentative essay. 1. Narrative Essay. As the name suggests, the goal of the narrative essay is to narrate a fictional story. However, that doesn't mean you can't sprinkle in some personal experiences to spice up your writing.
To support students in this style of writing, typically, teachers introduce them to various strategies that target the writing portion of the standardized tests. These strategies and approaches often address the overall structure of the essay and provide a general idea of how to respond to the prompt.
related to both reading (the sources) and writing (the essay) skills. As such, source-based writing must involve language comprehension and production processes. The purpose of the current study is to examine the impact of reading, writing, and blended (i.e., reading and writing) strategy training on students' performance on a content-
The purposes of this review were to determine: (1) if different writing activities were more effective than others in improving students' reading comprehension, and (2) if obtained differences among writing activities was related to how reading comprehension was measured? Meta-analysis was used to examine these questions across studies involving students in grades 1-12. Nineteen studies ...
The corpus features essays for 90 topics from Essay Forum 42, an active community for providing writing feedback on different kinds of text and is frequented by high-school students to get ...
Writing an integrated essay based on multiple-documents requires students to both comprehend the documents and integrate the documents into a coherent essay. In the current study, we examined the effects of summarization as a potential reading strategy to enhance participants' multiple-document comprehension and integrated essay writing.
It is well established that many children and adolescents have inadequate reading comprehension or writing skills (NAEP, 2003; OECD, 2017).Several studies have attempted to explain the differences between individuals with proficient or poor performance in reading and writing, and the variables that influence these performances (Beauvais et al., 2011; Cain et al., 2004; Ferrari et al., 1998 ...
In terms of comprehension, writing the essay produced better results in the deep learning task than answering intratextual questions; in contrast, there were no differences between the two tasks in the superficial understanding test. ... Although we did not find differences between the paper condition and the R&A condition in the inferential ...