Hydrology Workbook
Exercise 4 - visualising groundwater, learning objectives.
In this exercise you will investigate what controls the direction and rate of groundwater flow, and investigate groundwater storage and flow within a layered groundwater system.
At the completion of this exercise students will be able to
calculate groundwater flow rates
describe the range of flow rates within natural groundwater systems and explain the key factors controlling these rates
identify patterns of groundwater flow and hydraulic head contours within layered groundwater systems
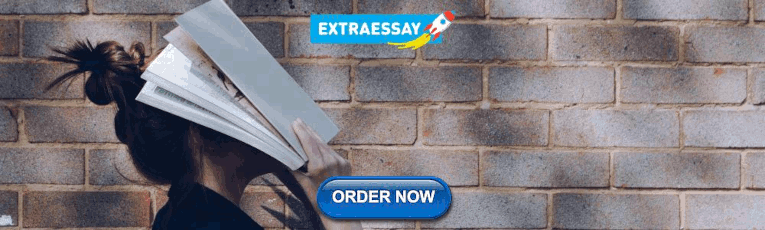
Groundwater flow rates
As a first step lets consider the flux law for groundwater flow. Groundwater flow is driven by the distribution of water pressure (hydraulic head, which has units of length) within the groundwater system. The flow of groundwater follows a flux law;
Where \(q\) is the specific discharge (or Darcy Flux) with units of L T -1 , \(Q\) is the volumetric flow rate (L 3 T -1 ), \(A\) is the cross-sectional area through which the water flow (L 2 ), and \(\frac{\Delta h}{ \Delta l}\) is the hydraulic gradient. This gradient is simply the change in water pressure ( \(h_1-h_2\) ) over a given distance (L/L, unitless). The key system property in this flux law is the hydraulic conductivity ( \(K\) ), which has units of L T -1 . In natural groundwater systems the value of hydraulic conductivity can vary over 12 orders of magnitude. Even though this water flux \(q\) has units of L T -1 , it’s not actually the velocity of the groundwater, because the water wasn’t flowing through the whole area, just the pore space. To correct for this and calculate the average velocity we need to use the area of the pore space ( \(An\) , where \(n\) is porosity), instead of the total area. This gives us;
Where \(\bar{v}\) is the average linear velocity of the water flowing through the porous media.
Now that we know these simple equations let’s look at some experimental data from simple “Darcy tubes” that allow us to measure the relationships between rates of groundwater flow, hydraulic gradients and the hydraulic conductivity of the porous media that it flows through. This is how the idea of a “hydraulic conductivity” was first defined, empirically, and it was only decades later that the physical basis for hydraulic conductivity and Darcy’s Law was fully elucidated from first principles (see Hubbert 1940 for some light bedtime reading).

Figure 5: a) Photo of simple Darcy Tube and b) diagram showing terms in Darcy’s Law Equation
Let’s have a go at using this experimental setup to estimate the hydraulic conductivity of different sedimentary materials. To do this all we need to do is measure the flow rate through the tube for our chosen porous media. For each porous material, the slope determines the hydraulic gradient and we just need to measure the volume that flows out of the tube over a set time interval.
During this experiment;
the diameter of the tubes was 40 mm
the inlet at the top of the tube was 25 cm above the outlet at the bottom of the tube, and the lateral distance between the inlet and the outlet was 0.5 m
Over a period 24 hours 168 mL was collected from the tube filled with sand, 3.6 mL was collected from the tube filled with silty sand, and 814 mL of water was collected from the tube filled with gravel.
- Based on this information you can rearrange Eq (10) to calculate the hydraulic conductivity of each porous media. Are you results consistent with the range of values for similar porous media in the literature? Could you measure the hydraulic conductivity of a clay sample using this experimental setup? What could you do to improve this experiment?
Using Darcy’s Law to calculate flow to a stream
Now we understand the concept of Darcy’s Law within a small-scale context, lets think about how we can apply it in the “real world”. The below diagram shows the flow in an aquifer towards a stream.

Figure 6: Water table and topography of a idealised hillslope
- Consider the digram above. If the slope of the water table hydraulic gradient perpendicular to the stream is on average approximately 1/1000 (m/m), what is the Darcy flux, q, of groundwater towards the river? Use a hydraulic conductivity, K, of 1 m /day.
Creating a flow net
We can trace the path of a “parcel” of water at any point in a groundwater system by creating a “flow net”. To do this we map the lines of “equipotential” (i.e., lines of constant head), like height contours on a topographic map, but instead of land height, its water height. Based on the gradient in water height (hydraulic gradient) we can work out the path that a parcel of wtare would take, as water will flow from high to low pressure.
Before we go further, lets check out this cool flow-net tool called “ TopoDrive ” . This is an online interactive tool that allows you to visulise groundwater flow nets!
.](https://aquaticecodynamics.github.io/images/exercise4/pictureY.png)
Figure 7: Creating a hillslope flow-net. For an overview, check out this youtube instruction video here .
- Create a hypothetical hillslope water table cross-section with the tool. Use the tool to show the water flow pathways. Make a note of your observations - is there anythign unexpected? Depending on your flow-net you may be surprised that water does not always flow in a straight line!
One thing to note with flow-lines (or sometimes we call them streamlines), water flow paths must cross lines of equipotetnial at right-angles (i.e. perpendicular).
- Now look at the below image - a plan view of the water table contours around Perth. Given the sort of hydraulic conductivities estimated above, what would the range of linear velocity we could expect in a groundwater system like the Gnangara Mound? Use the following watertable map (taken from Davidson and Yu 2008, HG20 ) to estimate a hydraulic conductivity, and then calculate linear velocity using Eq (11). You will need to assume a porosity - look these up, or just use 0.25 (which is a good indicative value for back-of-the-envelope calculations in aquifer materials - why not in clays?). Note that it’s probably ok to assume a porosity value because the range of porosity is within one order of magnitude, whereas hydraulic conductivity will vary by multiple orders of magnitude, even within the same type of material, so this will be the larger source of uncertainty in your calculation.

Figure 8: Section of a map of the watertable across Perth
Physical model of groundwater system
In this part of the exercise we will look at a model of a layered groundwater system. Each layer has a different hydraulic conductivity, \(K\) , forming two aquifers and an aquitard. Within each layer there will also be variation in hydraulic conductivity. This spatial variation is called “heterogeneity”, and it is an important feature of real groundwater systems.
Start by annotating the photo of this model (Figure 8 ) to show the following;
Aquifers and aquitards
Recharge and discharge zones
Water levels in each of the wells
The water table
Hydraulic head boundaries

Figure 9: Physical model of a layered groundwater system.
Now watch this video to see how water moves through the model when dye is injected to see the direction of groundwater flow. Does the flow direction vary spatially? Does the flow direction change over time?
Draw arrows on the following screen-shot to show the direction of groundwater flow within the model domain. We have learnt that the measure of potential for groundwater flow is “hydraulic head” and we also know that groundwater flows from high hydraulic head to low hydraulic head. In fact, groundwater flow will be perpendicular to the equipotentials (lines of equal hydraulic head). Based on your inferred flow paths, try now to add in the hydraulic head contours (or equipotentials).
It might help for you to think about topographic contours on a landscape map - in this case you have elevation contours, and if you placed a ball at the top of a hill it would roll (or flow) from the higher elevation to the lower elevation. As a general rule, we also know that flow through high conductivity layers will be primarily horizontal, and flow through low conductivity layers will be primarily vertical.

Figure 10: Physical model of a layered groundwater system.
What can you learn from this physical model about groundwater systems? By using the physical model we have been able to see directly in the subsurface, but in the real world, the only information we have would be the wells.
If you only had the hydraulic head measurements in the wells what would you know about the groundwater system?
What else would you need to know about this system to calculate groundwater flow velocities (and therefore contaminant transport)?
How important is it that we understand the geology as well as having measurements of hydraulic heads?
What else might you measure in a real groundwater system to get a fuller picture of what is happening?
Computer model of groundwater system
We can also run a “digital twin” of the physical model that we have built in the lab. For this activity we will now use the ParFlow Sandtank model. This is a computer model simulating groundwater flow, and allows users to change aquifer configurations and explore the essential features of groundwater and contaminant flow.
Once you have watched the introductory video lets take a look at running the model. Navigate to this model website . You can learn how to customise the sandtank and run a simulation here . Have a go at manipulating the soil conditions and the hydraulic head sliders, and run simulations pumping or injecting groundwater. Can you reproduce our lab-based physical model?
Example calculation of groundwater baseflow to a river
In this section is an example of how we can apply Darcy’s Law to work out how an aquifer is “working”, i.e., how much water it is conducting and entering the river as baseflow. Consider the following.
After a prolonged period without rain, the discharge of a river at a measuring station A is 10 m3/s and at a downstream station B it is 15 m3/s. The distance between A and B is 2 km.

Figure 11: Plan and cross sectional view of a river valley
Between the stations, the river receives water from both sides, from an unconfined aquifer, and the equipotentials are assumed to be parallel to the river.
The slope of the water table (hydraulic gradient) on average is approximately 1/1000 (m/m).
Based on this information, use Darcy’s Law to determine the transmissivity of the aquifer, in m2/s.
Note that, Transmissivity of an aquifer is the depth integrated hydraulic conductivity
Start by drawing a diagram and annotate with the information that you know.
You must log in to edit PetroWiki. Help with editing
Content of PetroWiki is intended for personal use only and to supplement, not replace, engineering judgment. SPE disclaims any and all liability for your use of such content. More information
Water influx models
Water influx models are mathematical models that simulate and predict aquifer performance. Most importantly, they predict the cumulative water influx history. When successfully integrated with a reservoir simulator , the net result is a model that effectively simulates performance of water drive reservoirs .
- 1 Aquifer models
- 2.1 Discretization
- 2.2 Cumulative water influx
- 2.3.1 Infinite radial aquifer
- 2.3.2 Finite radial aquifer
- 2.4 Linear aquifer
- 2.5 Calculation procedure
- 3.1 Direct measurement
- 3.2 History matching
- 4 Example: History matching water influx
- 5 Aquifer performance
- 6 Nomenclature
- 7 Subscripts
- 8 References
- 9 Noteworthy papers in OnePetro
- 10 External links
- 11 See also
Aquifer models
There are several popular aquifer models:
- van Everdingen-Hurst (VEH) model [1]
- Carter-Tracy model [2]
- Fetkovich model [3]
- Schilthuis model [4]
- Small- or pot-aquifer model [5]
The first three models are unsteady-state models and are the most realistic. They attempt to simulate the complex pressure changes that gradually occur within the aquifer and between the aquifer and reservoir. As pressure depletion proceeds, the pressure difference between the reservoir and aquifer grows rapidly and then abates as the aquifer and reservoir eventually equilibrate. This pressure interaction causes the water influx rate to start at zero, grow steadily, reach a maximum, and then dissipate. This particular water influx rate history behavior applies to initially saturated oil reservoirs; the behavior for initially undersaturated oil reservoirs is often slightly but distinctly different. The effects of undersaturation on the water-influx performance are discussed below. The unsteady-state models are far more successful at capturing the real dynamics than other models. In contrast, Schilthuis’ steady-state model assumes the aquifer pressure remains constant. The small-aquifer model, however, assumes the aquifer and reservoir pressures are equal.
The VEH model is the most sophisticated of all these models. Its main advantage is its realism. Originally, its main disadvantage was its cumbersome nature. Charts or tables had to be consulted repeatedly to execute a single calculation. To address this limitation, the Carter-Tracy and Fetkovich models were alternatives that were free of tables and charts. These models, however, were only approximations to and simplifications of the VEH model. Since the VEH charts and tables were digitized, [6] [7] [8] the need for alternatives has diminished.
Allard and Chen [9] proposed an aquifer model specifically for bottomwater drives. This model included 2D flow. In comparison, the VEH model considered only 1D flow. Simulation practitioners, however, have found that the VEH model is satisfactory in simulating bottomwater drives. [10]
van Everdingen-Hurst (VEH) model
van Everdingen and Hurst considered two geometries: radial- and linear-flow systems. The radial model assumes that the reservoir is a right cylinder and that the aquifer surrounds the reservoir. Fig. 1 illustrates the radial aquifer model, where:
- r o = reservoir radius
- r a = aquifer radius

Fig. 1 – Radial aquifer model.
Flow between the aquifer and reservoir is strictly radial. This model is especially effective in simulating peripheral and edgewater drives but also has been successful in simulating bottomwater drives. [9]
In contrast, the linear model assumes the reservoir and aquifer are juxtaposed rectangular parallelepipeds. Fig. 2 shows examples. Flow between the aquifer and reservoir is strictly linear. This model is intended to simulate edgewater and bottomwater drives. The model definition depends on the application. For edgewater drives, the thicknesses of the reservoir and aquifer are identical; the widths of the reservoir and aquifer are also the same, and the aquifer and reservoir lengths are L a and L r , respectively ( Fig. 2a ). For bottomwater drives, the width of the reservoir and aquifer are identical; the length ( L ) of the reservoir and aquifer are also the same; the aquifer depth is L a , and the reservoir thickness is h ( Fig. 2b ).

Fig. 2 – Linear aquifer model for (a) an edgewater drive and (b) a bottomwater drive.
van Everdingen and Hurst solved the applicable differential equations analytically to determine the water influx history for the case of a constant pressure differential at the aquifer/reservoir boundary. This case assumes the reservoir pressure is constant. They called this case the "constant terminal pressure" and reported their results in terms of tables and charts. This solution is not immediately applicable to actual reservoirs because it does not consider a declining reservoir pressure. To address this limitation, van Everdingen and Hurst applied the superposition theorem to a specific reservoir pressure history. This adaptation usually requires that the reservoir’s pressure history be known. The first step in applying their model is to discretize the time and pressure domains.
Discretization

Table 1- Time and pressure domain discretization for van Everdingen-Hurst model.
Cumulative water influx
The cumulative water influx at k th level is

where U is the aquifer constant and W D is the dimensionless cumulative water influx. This equation is based on the superposition theorem. The term W D ( t Dk – t Dj ) is not a product but refers to the evaluation of W D at a dimensionless time difference of ( t Dk – t Dj ). If we apply Eq. 3 for k = 1, 2, and 3, we obtain

The length of the equation grows with the time. The aquifer constant, U , and the dimensionless cumulative water influx, W D ( t D ), depend on whether the radial or linear model is applied.
Radial model
The radial model is based on the following equations. The effective reservoir radius is a function of the reservoir PV and is

- r o is expressed in ft
- V pr is the reservoir PV expressed in RB
- ϕ r is the reservoir porosity (fraction)
- h is the pay thickness in ft
The constant f is θ /360, where θ is the angle that defines the portion of the right cylinder. Fig. 3 illustrates the definition of θ for a radial aquifer model. The dimensionless time is

- k a = aquifer permeability (md)
- μ w = water viscosity (cp)
- c t = total aquifer compressibility (psi–1)
- ϕ a = aquifer porosity (fraction)
- t is expressed in years

where U is in units of RB/psi if h is in ft, r o is in ft, and c t is in psi –1 .

Fig. 3 – Definition of angle, θ , for radial aquifer model.
The dimensionless aquifer radius is

The dimensionless water influx, W D , is a function of t D and r eD and depends on whether the aquifer is infinite acting or finite.
Infinite radial aquifer
The aquifer is infinite acting if r e approaches infinity or if the pressure disturbance within the aquifer never reaches the aquifer’s external boundary. If either of these conditions is met, then W D is

where a 7 = 4.8534 × 10 –12 , a 6 = –1.8436 × 10 –9 , a 5 = 2.8354 × 10 –7 , a 4 = –2.2740 × 10 –5 , a 3 = 1.0284 × 10 –3 , a 2 = –2.7455 × 10 –2 , a 1 = 8.5373 × 10 –1 , a 0 = 8.1638 × 10 –1 , or

Marsal [7] presented Eqs. 8 and 10 . Walsh [11] [8] presented Eq. 9 .
Finite radial aquifer
For finite aquifers, Eqs. 8 through 10 apply if t D < t D *, where

If t D > t D *, then

Marsal [7] gave Eqs. 11 through 13 . These equations are effective in approximating the charts and tables by van Everdingen and Hurst. Minor discontinuities exist at some of the equation boundaries. A slightly more accurate but much more lengthy set of equations has been offered by Klins et al. [6] Fig. 4 shows W D as a function of t D for r eD = 5, 7.5, 10, 20, and ∞. These equations simplify the application of the VEH model enormously.

Fig. 4 – W D vs. t D for a radial aquifer model.
A finite aquifer can be treated effectively as an infinite aquifer if

where t D max is the maximum value of t D . These equations follow from Eq. 11 . For example, if t D max is 540 and corresponds to a time of 8 years, then Eq. 15 yields ≥ r eD = 38. Therefore, if the aquifer has a dimensionless radius greater than 38, then the aquifer acts indistinguishably from and equivalent to an infinite aquifer at all times less than 8 years.
Linear aquifer
The aquifer size in the linear model is given in terms of the aquifer/reservoir pore-volume ratio, V pa / V pr .

The aquifer constant is

For edgewater drives, the aquifer length is

where L a and L r are defined in Fig. 2a . For bottomwater drives, the aquifer depth is

where L a is defined in Fig. 2b . The dimensionless time is

Eqs. 20 and 5 use the same units except L a is given in ft. One difference between the linear and radial models is that t D is a function of the aquifer size for the linear model, whereas t D is independent of the aquifer size for the radial model. This difference forces a recalculation of t D in the linear model if the aquifer size is changed. The dimensionless cumulative water influx is

Eq. 21 is by Marsal, [7] and Eq. 22 is by Walsh. [11] [8] Fig. 5 shows W D as a function of t D . The aquifer can be treated as infinite if the aquifer length is greater than the critical length.

where t max is the maximum time expressed in years and L ac is in units of ft. Eqs. 20 and 23 use the same units. Alternatively, the aquifer is infinite-acting if t D ≤ 0.50. If infinite-acting and an edgewater drive, W e can be evaluated directly without computing W D and is

where the units in Eq. 5 apply, and W e is in units of RB and h and w are in units of ft.

Fig. 5 – W D vs. t D for linear aquifer model.
Calculation procedure

- Compute the time-averaged incremental pressure differential Δ p j for ( j = 1, 2, ...., n ) with Eq. 2 .
- Compute t Dj for ( j = 0, 1, ..., n ) with Eq. 5 for radial aquifers or with Eq. 20 for linear aquifers.
- Steps 5 through 9 create a computational loop that is repeated n times. The loop index is k , where k = 1, ..., n . For the k th time level, compute ( t Dk – t Dj ) for ( j = 0, ..., k – 1).
- For the k th time level, compute W D ( t Dk – t Dj ) for ( j = 0, ..., k – 1).
- For the k th time level, compute Δ p j + 1 W D ( t Dk – t Dj ) for ( j = 0, ..., k – 1).
- For the k th time level, compute W ek with Eq. 3 .
- Increment the time from level k to k + 1, and return to Step 5 until k > n .
This procedure is highly repetitive and well suited for spreadsheet calculation. The example below illustrates the procedure.
Determining water-influx model parameters
The minimum parameters that need to be specified in the radial model are the aquifer constant, U , the time constant, k t , and the dimensionless aquifer radius, r eD . The time constant combines a number of constants, is the proportionality constant between the dimensionless and real time, and is defined by

Physically, the time constant represents the aquifer conductivity. In summary, U and k t are defined as

Eq. 26 assumes the same units as Eq. 6 , and V pr is given in res bbl. Eq. 27 assumes the same units as Eq. 5 .
The minimum parameters that need to be specified in the linear model are the aquifer constant, time constant, and aquifer/reservoir PV ratio ( V pa / V pr ). The aquifer constant and time constant are

Eq. 29 assumes the same units as Eq. 5 .
There are three common methods to estimate model parameters:
Direct measurement
History matching.
- Material balance
The first two methods are described in the following sections. The material-balance method through the McEwen method, [12] is described on the material balance in water drive reservoirs page.
This method estimates model parameters from direct measurement of the independent constants. Though ideally preferred, this method is rarely possible because of the uncertainty of some of the constants.
For the radial model, the model parameters ( U , k t , and r eD ) are a function of the following constants:
These constants follow from inspection of Eqs. 6 , 7 , and 27 . The uncertainty among these constants varies. Of these constants, r e , r o , and k a are perhaps the most uncertain. Qualitatively, these constants are related to the aquifer size, reservoir size, and aquifer conductivity (i.e., V pa , V pr , and k t ).
For the linear model, the model parameters ( U , k t , and V pa / V pr ) are a function of the following constants:
This list follows from inspection of Eqs. 17 and 29 . Of these constants, V pa , V pr , L a , and k a are the most uncertain. If h is approximately known, then V pa and L a are not independent but related through Eqs. 18 or 19 . Thus, V pa , V pr , and k a are the most uncertain independent constants. Qualitatively, these constants are related to the aquifer size, reservoir size, and aquifer conductivity (i.e., V pa , V pr , and k t , respectively). Note the similarity between the radial and linear models.
In summary, because of the uncertainty of the aquifer size and conductivity and reservoir size, it is difficult to estimate reliably the water-influx model parameters. Nevertheless, every attempt should be made to estimate the median, variance, and range of each constant and the model parameters. This information is helpful in the history-matching method.
If the water influx history can be estimated, then model parameters can be estimated from history matching. When history matching is used, only the most uncertain constants should be treated as adjustable parameters: preferably only:
- r e , r o , and k a for the radial model
- V pa , V pr , and k a for the linear model
Unless only one adjustable parameter exists, history matching is usually complicated by nonuniqueness. [13] [12] [14] [15] [16] Nonuniqueness, however, can be minimized by limiting the range of parameter adjustment to realistic ranges. The example below illustrates the history-matching procedure.
Example: History matching water influx
Table 2 summarizes the cumulative water influx and average reservoir pressure as a function of time for an initially saturated, black oil reservoir. Areally, the reservoir is approximately semicircular, bounded on one side by a sealing fault and the other side by an aquifer. Fig. 6a shows a schematic representation of the reservoir. Assume the reservoir and aquifer properties in Table 3 apply.

Table 2 - Reservoir performance data for example.

Table 3 - Reservoir and fluid properties for example.

Fig. 6 – Areal schematic of a reservoir partially surrounded by a radial aquifer; (b) interpretation of reservoir geometry when linear aquifer model is applied.
Assume a radial-flow aquifer with f = 0.50. Find the optimal aquifer size ( V pa and r eD ) that best simulates the water-influx performance. Plot and compare the actual and predicted water-influx histories.
Find the optimal aquifer size ( V pa / V pr and L a ) that best matches the water-influx performance assuming a linear-flow aquifer. Assume the reservoir width is w = 2 r o and length is L r = πr o /4, where r o is given by Eq. 4 . Fig. 6b schematically shows the areal interpretation. Plot and compare the actual and predicted water-influx histories. Which model (linear or radial) best matches the data?
Solution . Compute the effective reservoir radius from

Table 4 - Predicted water influx history for radial aquifer if r eD =5.0.
The solution procedure varies r eD until the best match is obtained. Tables 5 through 7 give the details of the calculation for r eD = 5.0. Table 5 tabulates ( t Dk – t Dj ) for k and j = 0, 1, ..., 7. Table 6 tabulates W D ( t Dk – t Dj ) for k and j = 0, 1, ..., 7. Table 7 tabulates Δ p j + 1 W D ( t Dk – t Dj ) for k and j = 0, 1, ..., 7. Table 4 tabulates ΣΔ p j + 1 W D ( t Dk – t Dj ) for k = 1, 2, ..., 7 for r eD = 5 and W ej for j = 0, 1, ..., 7 for r eD = 5. Fig. 7 plots W e vs. t and compares model and actual results; excellent agreement is noted. These calculations were repeated for other values of r eD but r eD = 5.0 was found to give the best fit of the actual and model water-influx histories. Notice how well the radial model matches the history. A dimensionless aquifer radius of r eD = 5.0 corresponds to V pa / V pr = 20.8. This means that the aquifer is 20.8 times larger than the reservoir.

Table 5 - Summary of ( t Dk - t Dj ) calculations for radial aquifer.

Table 6 - Summary of W D ( t Dk - t Dj )calculations for radial aquifer.

Table 7 - Summary of Δ p j +1 W D ( t Dk - t Dj )calculations for radial aquifer.

Fig. 7 – Comparison of actual and predicted water-influx histories for Example 1.
To determine whether this aquifer can be treated as infinite acting, we evaluate Eq. 15 with t D max = 26.08. This calculation yields r eD = 8.1. Because this value of r eD is greater than the history-matched value of r eD = 5.0, this aquifer cannot be treated as infinite.
For the linear aquifer model, the geometry dictates that L r = 11,696 ft if L r = πr o /4 = π (14,892)/4.With the same trial-and-error procedure as used for the radial aquifer, the linear aquifer yields V pa / V pr = 12 for the best match between the actual and predicted water-influx data. This value of V pa / V pr yields L a = 161,145 ft, U = 27,271 RB/psi, and k t = 0.0074 years –1 . Fig. 7 compares the predicted and actual data and shows that the match is poor. This comparison reveals that the linear model is not preferable to simulate water influx for this reservoir.
Aquifer performance
The aquifer performance is described in terms of the:
- Delivery rate
- Average aquifer pressure
- Cumulative water-influx volume as a function of time
The aquifer pressure characteristically lags behind the reservoir pressure and is estimated by

The aquifer delivery rate is q w = ∂W e ( t )/ ∂t , which is determined from the slope of the W e vs. t curve.
Fig. 8 shows the aquifer pressure and delivery rate history for the data in the example above. This figure includes the reservoir pressure history for comparison. The qualitative results in Fig. 8 are representative of many aquifers. The water-delivery rate is initially zero and increases rapidly. It peaks after approximately 12 to 14 years and then slowly decreases. The aquifer and reservoir pressures start at equivalent values. The reservoir pressure declines more quickly than the aquifer pressure. The pressure differential between the aquifer and reservoir grows and is approximately 250, 350, and 500 psia, respectively, after 2, 5, and 10 years. The pressure differential peaks after 12 to 14 years and then begins to dissipate. The pressure differential and delivery rate decline together.

Fig. 8 – Predicted aquifer pressure and water-influx rate histories for example.
The aquifer performance noted in Fig. 8 is not without exception. The qualitative performance in Fig. 8 is characteristic of an initially saturated reservoir. Aquifers feeding initially undersaturated reservoirs may behave quite differently. The difference stems from the difference in the reservoir pressure histories. The reservoir pressure in initially undersaturated oil reservoirs initially declines much more quickly than in initially saturated reservoirs. Consequently, initially undersaturated reservoirs create a substantial pressure differential between the reservoir and aquifer much sooner than initially saturated reservoirs. Of course, this distinction depends on the degree of undersaturation. If the reservoir is significantly undersaturated, a large pressure differential between the reservoir and aquifer is quickly established. This large pressure differential, in turn, promotes water influx; consequently, the water-influx rate increases more rapidly in initially undersaturated reservoirs than initially saturated reservoirs. Once the bubblepoint is reached, the pressure differential between the aquifer and reservoir may decline temporarily. Later, the pressure differential may increase, reminiscent of an initially saturated reservoir, as in Fig. 8 . The net effect is that water recharge rate may oscillate in an initially undersaturated oil reservoir.
Nomenclature
- ↑ van Everdingen, A.F. and Hurst, W. 1949. The Application of the Laplace Transformation to Flow Problems in Reservoirs. Trans., AIME 186, 305.
- ↑ Carter, R.D. and Tracy, G.W. 1960. An Improved Method for Calculating Water Influx. Trans., AIME 219: 415.
- ↑ Fetkovich, M.J. 1971. A Simplified Approach to Water Influx Calculations—Finite Aquifer Systems. J Pet Technol 23 (7): 814–28. SPE-2603-PA. http://dx.doi.org/10.2118/2603-PA
- ↑ Schilthuis, R.J. 1936. Active Oil and Reservoir Energy. Trans., AIME 118: 33.
- ↑ Coats, K.H. 1970. Mathematical Methods for Reservoir Simulation. Presented by the College of Engineering, University of Texas at Austin, 8–12 June 1970.
- ↑ 6.0 6.1 Klins, M.A., Bouchard, A.J., and Cable, C.L. 1988. A Polynomial Approach to the van Everdingen-Hurst Dimensionless Variables for Water Encroachment. SPE Res Eng 3 (1): 320-326. SPE-15433-PA. http://dx.doi.org/10.2118/15433-PA
- ↑ 7.0 7.1 7.2 7.3 Marsal, D. 1982. Topics of Reservoir Engineering. Course Notes, Delft U. of Technology.
- ↑ 8.0 8.1 8.2 Walsh, M.P. 1996. A Generalized Approach to Petroleum Reservoir Engineering. Austin, Texas: Petroleum Recovery Research Inst.
- ↑ 9.0 9.1 Allard, D.R. and Chen, S.M. 1988. Calculation of Water Influx for Bottomwater Drive Reservoirs. SPE Res Eng 3 (2): 369-379. SPE-13170-PA. http://dx.doi.org/10.2118/13170-PA
- ↑ Field, M.B., Givens, J.W., and Paxman, D.S. 1970. Kaybob South - Reservoir Simulation of a Gas Cycling Project with Bottom Water Drive. J Pet Technol 22 (4): 481-492. SPE-2640-PA. http://dx.doi.org/10.2118/2640-PA
- ↑ 11.0 11.1 Walsh, M.P. and Lake, L.W. 2003. A Generalized Approach to Primary Hydrocarbon Recovery. Amsterdam: Elsevier.
- ↑ 12.0 12.1 McEwen, C.R. 1962. Material Balance Calculations With Water Influx in the Presence of Uncertainty in Pressures. SPE J. 2 (2): 120–128. SPE-225-PA. http://dx.doi.org/10.2118/225-PA
- ↑ Tehrani, D.H. 1985. An Analysis of a Volumetric Balance Equation for Calculation of Oil in Place and Water Influx. J Pet Technol 37 (9): 1664-1670. SPE-12894-PA. http://dx.doi.org/10.2118/12894-PA
- ↑ Woods, R.W. and Muskat, M.M. 1945. An Analysis of Material-Balance Calculations. Trans., AIME 160: 124.
- ↑ Chierici, G.L. and Ciucci, G.M. 1967. Water Drive Gas Reservoirs: Uncertainty in Reserves Evaluation From Past History. J Pet Technol 19 (2): 237-244. http://dx.doi.org/10.2118/1480-PA
- ↑ Sills, S.R. 1996. Improved Material-Balance Regression Analysis for Waterdrive Oil and Gas Reservoirs. SPE Res Eval & Eng 11 (2): 127–134. SPE-28630-PA. http://dx.doi.org/10.2118/28630-PA
Noteworthy papers in OnePetro
Use this section to list papers in OnePetro that a reader who wants to learn more should definitely read
External links
Use this section to provide links to relevant material on websites other than PetroWiki and OnePetro
Water drive reservoirs
Material balance in water drive reservoirs
Oil fluid characteristics
Oil fluid properties
PEH:Oil_Reservoir_Primary_Drive_Mechanisms
- 5.5 Reservoir simulation
Navigation menu
The Models:
1979 - Texas Water Development Board Finite-Difference Model (with 1992 refinements)
1983 - Discrete-State Compartment Model
1988 - United States Geological Survey Finite-Difference Model
1992 - Lumped Parameter Model
1993 - A & M University Economic / Hydrologic Model
1994 - United States Geological Survey Finite-Element Model
1995 - Edwards Underground Water District Edwards Aquifer Simulation
1999 - Bureau of Economic Geology 3D Virtual Reality Model
2004 - 3D Model of the Edwards in Northern Bexar County
2005 - HSPF Recharge Model for the San Antonio Segment of the Edwards
2015 - USGS Updated Numerical Model with Uncertainty Assessment of 1950-56 Drought Conditions on Brackish-Water Movement with the Edwards Aquifer
In the mid-1970's, researchers at the Texas Water Development Board evaluated and synthesized previously compiled geologic and hydrologic data, collected additional data, and initiated synthesis and analysis studies using digital computer modeling techniques. These pioneers were William B. Klemt, Tommy R. Knowles, Glenward R. Elder, and Thomas W. Sieh. Their objectives were to investigate the influence of projected water demands on Aquifer storage and spring flow, to determine the effectiveness of artificial recharge, and to discover whether ground-water management could protect spring flow at Comal and San Marcos Springs. Their model simulates water levels and spring flows based on the physical constraints of the system and on the recharge and pumpage rates for the Aquifer.
Finite Difference Grid for TWDB model
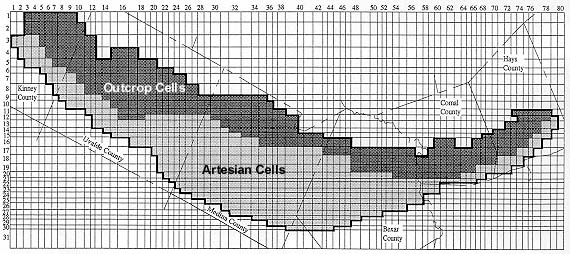
There are three types of cells in the model: outcrop cells, artesian cells, and boundary cells. In the graphic below, the boundary cells are the ones with at least one black, bold border; the outcrop cells are in dark grey; and the artesian cells are in light gray. Most of the grid cells lie outside the boundary, so only 856 of the cells in the grid were considered as part of the Edwards system and included in the simulation.
Critical steps in model building are the assignment of recharge and pumpage values to each of the cells. Recharge was divided into two components: water that recharges by going down through sinkholes and faults in creek beds, and water that becomes recharge by falling directly onto the outcrop. In the assignment of recharge, the amount of water known to have been lost from a stream to recharge was assigned evenly to the cells that contained the stream reach, and the amount recharged by direct infiltration was evenly divided among the remaining cells for that subbasin. Assignment of pumpage is also critical - it must be assigned so it approximates the distribution of pumpage that actually occurred. In this model, the pumpage assigned to each cell is the total pumpage of all wells located within the cell.
Model Calibration
After a model is initially built, there comes a calibration phase that involves comparing observed and simulated water levels. The idea is to find out how well the model can predict what actually happened in the past. For this model, the calibration period used data from 1947 to 1971. This period was chosen because the data was readily available and these years included a great variation in water levels. There was an extreme drought that lasted from 1947 until 1956, and 1957 and 1958 were years of unusually high recharge. It was felt that if the model could simulate water levels for this period, it would be well calibrated.
The calibration results are shown in the graphic below, and it is clear the measured flow and the simulated flow are very close. The difference amounts to only 4.3 percent of the total flow. Based on this comparison, it was decided the digital model was calibrated to a degree of accuracy sufficient to reproduce past events and that the model could therefore be used to predict future Aquifer conditions as a tool in evaluating and developing management plans.
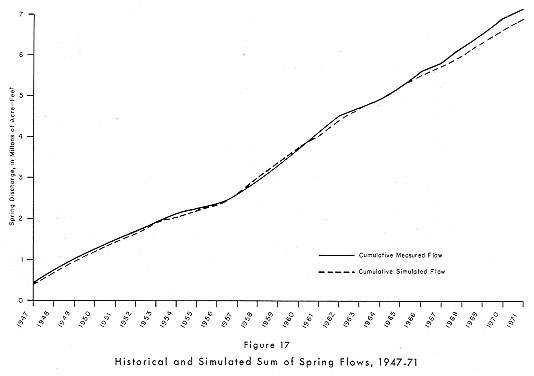
Future Simulation Phase
After calibration, a Future Simulation Phase involved using the model to predict what would happen in the Aquifer under a variety of different conditions. There were a total of 20 model runs that looked at the response of the Aquifer under a variety of pumpage and recharge conditions, various drought and flood conditions, several Aquifer management plans, and under augmentation pumping. Below are some selected results from Simulation Run 1. By the year 2000, total Aquifer pumpage was projected to be around 650,000 acre-feet. Comal Springs was projected to go dry in 1995, and San Marcos Springs would be dry by 2010. Pumpage has not really panned out as was projected back in the 70's when this model was built. Currently there is a cap on pumpage at 450,000 acre feet, and the Springs are still flowing.
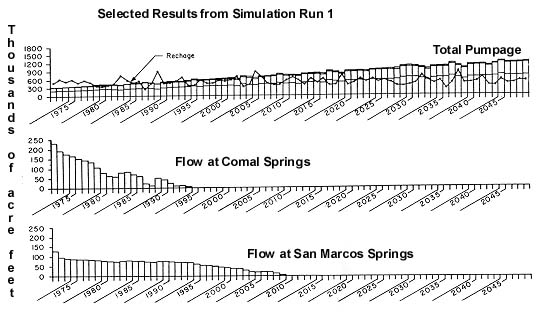
Model Conclusions
The 20 model runs suggested the Aquifer could indeed meet the demand projections, but the natural flow of Comal and San Marcos Springs would cease by 2020 if projected pumpage occurred as predicted. It also concluded the addition of artificial recharge that was being proposed at the time did not result in an appreciable increase in the Aquifer's available water. Further, it suggested the drought-flood sequence of the 1950's produced only minor effects compared to the long-term influence of pumpage by humans. The model also concluded that spring flows at Comal and San Marcos Aprings could be maintained through groundwater management plans. Finally, it suggested there is sufficient storage in the Aquifer to allow spring flow of Comal and San Marcos Springs to be replaced by augmentation pumpage.
1992 Model Refinement
In 1992, David Thorkildsen and Paul D. McElhaney revisited the 1970's model, refined it, and applied it under new conditions of reduced regional pumpage and under new Aquifer management plans. During the years between 1979 and 1992, the United States Geological Survey continued to collect new and better data on things like Aquifer transmissivity, storage coefficients, and anistropy, so these two geologists at the TWDB were able to incorporate the new data into their model to better represent the flow system and improve the model simulation.
For the new version of the model, the time period used for calibration was 1947 to 1959 instead of 1947 to 1971 as before. It was thought that since this period was one of extreme stress that included a major drought and a recovery period, it would be a good test for the parameters in the model. The time step for output results became monthly instead of annually.
After calibration, the model was used to simulate Aquifer response to several constant pumpage scenarios under recharge conditions of the drought and recovery sequence (1947-1959). The results indicated that large pumpage reductions would be necessary in order to ensure minimum springflows in the 40 to 50 cfs range at both San Marcos and Comal Springs. For a flow of 50 cfs or greater to be maintained at Comal Springs, the model indicated total pumpage would have to be in the 225,000 to 250,000 acre-feet per year range for the entire San Antonio region. This is much less than current pumpage. To maintain 100 cfs or greater, the model indicated maximum total pumpage would be about 165,000 acre feet per year.
The model also looked at the effect of reduced pumpage in the western, agricultural part of the Edwards region. Interestingly, the model indicated this would result in an increase in springflow at Comal Springs, but the increase was not equal to the amount of pumpage reduced. If the reduction in pumpage was placed in Uvalde county, only 34% of the reduction shows up as springflow at Comal Springs after nine years. If the reduction was placed in Medina county, 67% of the reduction was seen as increased springflow at Comal Springs after nine years. In both cases the remainder of the reduced pumpage either became increased Aquifer storage, interformational flow, or increased outflow. When the reductions were placed in Uvalde county, Leona springs began to flow again; but not when the reductions were placed in Medina county.
One of the ideas being floated in the early 90's by the Texas Water Commission was a mandatory water curtailment plan called the "dry-year option", under which pumpage for municipal, industrial, and aquaculture was adjusted (decreased or increased) on a monthly basis based on the simulated water level in the model cell representing the J17 monitoring well . Irrigation pumpage was to be adjusted on an annual basis, also using the simulated water level at J17. Under this plan, the model indicated that San Marcos Springs would always flow, but Comal Springs would cease to flow in an extreme drought.
UTSA's Decision Support System
In the mid-90's the University of Texas at San Antonio built something called the Decision Support System for the Edwards. It was actually the TWDB model converted to run on a personal computer, with minor embellishments and additions. This was the first model that had an interface sufficiently developed that a water manager could use it behind a desk. It has not been widely used outside academic circles.
United States Geological Survey Finite Difference Model
In 1988, Robert.W. Maclay and L.F. Land, working for the United States Geological Survey, developed an Edwards model that focused on concepts such as the effects of barrier faults and storage within the aquifer. Like the TWDB model, it was a finite-difference model, but it was modified to provide the capability of representing barrier faults. Rather than predicting Aquifer levels under different conditions as in the TWDB model, the main objective was to learn more about storage of water in different parts of the Aquifer and identification of flowpaths within the aquifer.
Finite Difference Grid for USGS model
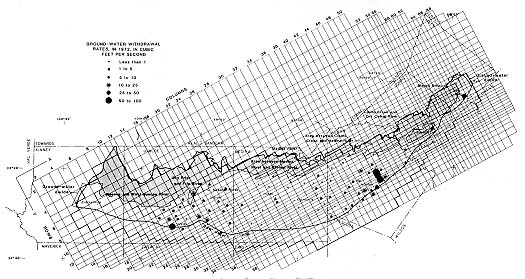
The important thing to notice about this grid is the orientation is about 65 degrees east of north. This was done to achieve the best alignment with the faults west of Cibolo Creek. The math model assumes that one of the x,y Cartesian coordinate axes is aligned along the principal component of the transmissivity.
More than 300 simulations were performed, and the modeling effort resulted in several major interpretations. One significant interpretation was that two essentially independent areas of regional flow exist in the west and central portions of the Aquifer, and flows from the two areas converge at Comal springs. Barrier faults deflect patterns of groundwater movement, and the most noticeable deflection is the convergence of flow through a geologic structural opening, the Knippa Gap, in eastern Uvalde county. A second significant interpretation is that groundwater flow in northeastern Bexar, Comal, and Hays counties is diverted by barrier faults toward San Marcos Springs. The simulations showed that several barrier faults in the northwestern part of the San Antonio area had a significant effect on storage, water levels, and springflow within the Edwards Aquifer.
Lumped Parameter Model
A lumped parameter model for the Edwards was developed in 1992 by Nisai Wanakule and Roberto Anaya with funding from various agencies. Their objective was to develop and test a simple and easy to use model to accurately simulate monthly water levels and springflows of the Edwards Aquifer. The model was formulated using a discrete, nonlinear, non-stationary system based on control theory. The main advantage of this simulation model was that it could utilize limited data to provide accurate, short-term estimates of Aquifer levels and springflows. Previous models required massive amounts of inputs and generated only annual estimates. Basically, the Edwards Aquifer was represented as a series of connected rock-filled tanks that represent eight major drainage basins of the Aquifer and springflows at Comal and San Marcos springs. Individual drainage basins included the Nueces River, the Frio River, the Sabinal River, Upper and Lower Seco and Hondo Creek (these represent flows on both sides of the Knippa Gap), the Medina River, Helotes and Salado Creek, Cibolo Creek, and the Blanco River. Data from the Guadalupe River basin were not available and thus were not included. Inputs to the model included real time measurements of recharge from river gauging stations. Outputs of the model included forecasts of levels in seven key observation wells and springflows. The model estimates monthly recharge, water levels, and pumping in each basin. It also allows for the interaction of ground and surface water between sub-basins. Monthly stream gauge and Aquifer level data from 1975 to 1990 were used to calibrate the model, while data from 1962 to 1974 were analyzed to verify its performance.
The simulation exercises showed the model is accurate and very easy to use because much less data is needed. When a Macintosh computer with a 68040 microprocessor was used, the lumped parameter model efficiently simulated 189 monthly iterations of water levels for nine drainage basins in less than four minutes. Recharge functions in the model provided good estimates of monthly recharge. Recharge estimates were very similar to results from the USGS model in most cases, although peak years were under-represented.
For a long time this model was available to actually be run on the web by any interested person.
A & M University Economic / Hydrologic Model
In 1993, Bruce McCarl and Lonnie Jones of the Texas A & M University Agricultural Economics Department worked with graduate students R. Lynn Williams and Carl Dillon to investigate the implications of management plans that were being proposed for the Aquifer at the time. At that time, hardly any research had been done that looked at the effect of different management plans on the regional economy.
In 1992, two management plans were drafted by the Texas Water Commission. The "Sector Plan" proposed specific cutbacks for agricultural, municipal, and industrial users when Aquifer levels at the J17 index well dropped to key levels, and the "Total Limit Plan" called for a total reduction in water use, but didn't specify where the cutbacks had to occur. Neither plan was adopted, but the Texas Legislature began considering plans similar to the TWC plans. A study was undertaken to evaluate the hydrologic and economic implications of the TWC plans. The A & M researchers analyzed four variants of the plans using an annual economic/hydrologic simulation model. The model simulated water use by the agricultural, industrial and municipal sectors while simultaneously forecasting annual spring flow and year-end water elevations. The model also accounted for uncertainty in recharge amounts and in the incidence of elevation-triggered pumping limits. A key feature of the model was that it optimized optimize water allocations among the various sectors of users based on maximizing economic welfare. In other words, water was allocated to the activities that generated the greatest economic value.
The model suggested that the simultaneous imposition of pumpage limits, water rights and water markets would be necessary to maintain economic efficiency. To allocate water toward the highest value use, the model made the assumption that low valued users would allow high value user to use their water instead. In reality, this would be highly unlikely without compensation for the low valued users. So, a rights structure was also examined where the irrigated agriculture sector (generally a low valued user) was guaranteed water usage at their 1988 level. The model showed that under these circumstances, there are gains in agricultural welfare, but municipal, industrial and total welfare is reduced by more than the agricultural gain. And without an agricultural guarantee for an allocation at their 1988 level, the municipal and industrial gains are achieved at agriculture's expense. Either way, the model suggested everyone loses out economically when pumping is no longer unrestricted and water is allocated. It indicated the management plans were likely to result in annual losses for current water users ranging from $0.73 million to $1.5 million (based on 1988 demands), rising to $2.3 to $6.7 million in the year 2000. When the value of water is optimized in an economic sense, the model predicted an annual loss in regional economic activity of between $6.26 and $19.58 per acre foot that pumpage is reduced. By the year 2000, agricultural use value was equal to about $19 per acre foot while non-agricultural values were about $109 per acre foot. This suggested that paying farmers not to use water would make economic sense.
The benefits of the management plans that were being proposed were increased flows at Comal and San Marcos Springs and higher year-end Aquifer levels. This model was the first attempt to quantify the economic cost of keeping springs flowing. It suggested costs would be very high, and there would still be no guarantee that Comal Springs would keep flowing in a severe drought.
United States Geological Survey Finite-Element Model
In 1994, Eve Kuniansky and Kelly Holligan, working for the United States Geological Survey, published a model that sought to understand and describe the regional flow system and examine how the Edwards Aquifer is connected to the Trinity Aquifer and other hydraulically connected units. The researchers wanted to gain a better understanding of how much water enters the Edwards from other Aquifers and how anistropy affects regional flows of groundwater. Anistropy occurs when water can move more easily through the rock matrix in one direction than another. In most Aquifers made up of flat-lying sedimentary rocks, water can move more easily horizontally than vertically. Because of this property, and because the Aquifer is many times wider than it is deep, groundwater flow through the Aquifer can be approximated with two dimensions even though the Aquifer actually exists in three dimensions. Unlike the previous models that used a finite-difference approach, this model used a finite-element method because it is one of the few numerical methods that can approximate anistropy.
In this model, the major departure from the earlier simulations was this one allowed a hydraulic connection from the Trinity Aquifer. The previous models assumed a no-flow boundary between the Edwards and the Trinity. Two simulations were performed. One was for the winter of 1974-1975 because there was adequate water-level data for model calibration for that period; and the other simulation was for predevelopment conditions. The simulations indicated that a significant amount of water enters the Balcones Fault Zone from other aquifers; about 97,500 acre-ft per from the Trinity and an additional 292,500 acre-ft per year from the Edwards-Trinity Plateau aquifer west of the Haby Crossing fault. Also, large irrigation withdrawals from the Edwards-Trinity Aquifer and the Cenozoic Pecos alluvial Aquifer in the Trans Pecos region have resulted in the drying up of several springs including Comanche and Leon Springs. Further, the results also suggested that anistropy is an important factor in groundwater movement in the Balcones Fault Zone.
Edwards Aquifer Simulation
In 1995 the Edwards Aquifer Simulation (EAS) was developed under the guidance of the Edwards Underground Water District. It was designed as an interactive tool to explore both the natural processes that influence and control the operation of the Aquifer and the human exploitation and management of the aquifer. It used a hands-on, role-playing environment that allowed users to model Aquifer response and springflows under many different scenarios such as varying rainfall patterns, institution of pumping restrictions, allocation of supply, and establishment of conservation and reuse programs. Unfortunately, this simulation was built for the Macintosh platform, so there were not many that could run it.
These are the two main display screens for the Edwards Aquifer Simulator. The Regional Map screen, at left, displays status indicators for springflow, municipal and agricultural pumpage, and downstream flow. The display would update annually during a model run. On the right, the Monitor Board screen displays graphical and numerical indicators of water level.
This model will on many platforms, including Linux, and it is a very cool tool for visualizing the structure of the Edwards in Bexar county. It is available from the USGS website at: http://pubs.usgs.gov/sir/2004/5226/
In cooperation with the San Antonio Water System, the United States Geological Survey developed a new model with a focus on simulating the interaction between freshwater and saline water where they mix in the area called the brackish-water transition zone.
The model results indicate that effects on fresh water during a severe drought would be minor.
USGS scientist Linzy Brakefield said "While the model shows little potential for movement of brackish water into freshwater, the research suggests there is a need for an improved understanding of some parts of the Edwards Aquifer flow system. Better knowledge of how the Aquifer is recharged and the relationship between recharge, pumping, and springflow is needed. With the new developments from this study there is potential to develop more accurate models in the future."
Materials used to prepare this section:
Edwards Underground Water District, (1995). Edwards Aquifer Simulator, Version 2 for Macintosh, User Manual. New Braunfels: TBEE Educational Consultants.
Klemt, William B., Knowles, Tommy R., Elder, Glenward R., and Sieh, Thomas W. (1979). Ground-Water Resources and Model Applications for the Edwards (Balcones Fault Zone) Aquifer in the San Antonio Region, Texas . Austin: Texas Department of Water Resources, Report 239.
Kuniansky, Eve L., and Holligan, Kelly Q. (1994). Simulations of Flow in the Edwards-Trinity Aquifer System and Contiguous Hydraulically Connected Units, West-Central Texas . Austin: US Geological Survey, Water-Resources Investigations Report 93-4039.
LBJ-Guyton Associates, (2005). HSPF Recharge Models for the San Antonio Segment of the Balcones Fault Zone Edwards Aquifer. EAA Contract 02-87-AS
McCarl, Bruce A., Jordan, Wayne R., Williams, R. Lynne, Jones, Lonnie L., and Dillon, Carl R. (1993). Economic and Hydrologic Implications of Proposed Edwards Aquifer Management Plans. College Station: Texas Water Resources Institute, Technical Report No. 158.
Maclay, R. W., and Land. L. F. (1988). Simulation of Flow in the Edwards Aquifer, San Antonio Region, Texas, and Refinement of Storage and Flow Concepts . Washington: US Government Printing Office, US Geological Survey Water-Supply Paper 2336-A.
Pantea, Michael P. and Cole, James C. (2004). Three-Dimensional Geologic Framework Modeling of Faulted Hydrostratigraphic Units within the Edwards Aquifer, Northern Bexar County, Texas. USGS Scientific Investigations Report 2004�5226.
Thorkildsen, David and McElhaney, Paul D. (1992). Model Refinement and Applications for the Edwards (Balcones Fault Zone) Aquifer in the San Antonio Region . Austin: Texas Water Development Board, Report 340.
Wanakule, Nisai, and Anaya, Roberto (1993). A Lumped Parameter Model for the Edwards Aquifer . College Station: Texas Water Resources Institute, Technical Report No. 163.

New to InTeGrate?
- Module 6: Groundwater Hydrology
In this two-part module, we focus on the occurrence and movement of groundwater. Key topics will include an overview of aquifer types and nomenclature, and the physical properties and processes that govern the storage, transport, rate of flow, and budgets of water in these systems.
Expand for more detail and links to related resources
Activity Classification and Connections to Related Resources Collapse
Grade level.
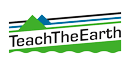
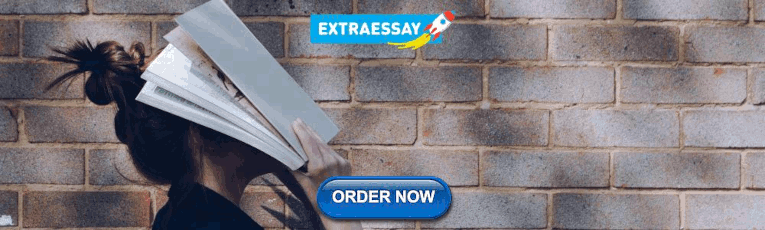
Learning Goals
- identify the properties of artesian wells and describe the conditions under which they form;
- explain the difference between porosity and permeability;
- list and describe the properties of aquifers that control the movement and storage of groundwater;
- explain the role of fractures in determining the transmission properties of aquifers;
- use Darcy's Law to explain the roles of aquifer properties and driving forces in governing the rate of groundwater flow;
- apply the concept of hydraulic head to draw flowlines on maps and cross sections;
- interpret the current and historical balance between groundwater recharge and water extraction from well hydrographs;
- propose a course of action to address overdraft in an aquifer.
Context for Use
Description and teaching materials.
In this module, students will:
- interact with online teaching materials pertaining to groundwater hydrology;
- interpret a cross-sectional aquifer diagram;
- complete a table associating given rock properties with hydraulic conductivity;
- interpret a diagram relating fracturing to well productivity;
- use a Darcy Tube to measure flow rate as a function of aquifer characteristics, or determine the same relationships using the original data set collected by Darcy;
- interpret hydraulic gradient from a potentiometric surface map.
All materials for students are available online using the Student Materials link below. These can be implemented entirely in the context of distance learning, with students completing any discussion questions in the form of a blog or discussion group. In a traditional or blended classroom setting, students can complete the online unit as homework, using class time for discussion and the Summative Assessment.
- Student Materials - Module 6: Groundwater Hydrology
Teaching Notes and Tips
What works best for the module? Module 6 provides a primer on basic aspects of groundwater flow, including an overview of aquifers and aquitards, types of aquifers, the aquifer physical properties that govern storage and transmission of water, the gradients in gravitational and pressure energy that control the direction and rate of water percolation, and the concepts of recharge and overdraft. The module material is basic, but is somewhat abstract, and a large number of individual terms, topics, and concepts are covered.
We have structured the module in two parts. Part One focuses on introductory material, various types of aquifers, and aquifer rock properties including porosity and permeability. Part Two introduces the dynamics of groundwater flow—notably the concepts of hydraulic head, recharge, and water budgets. Throughout the module, we rely on abundant schematics to illustrate aspects of subsurface geologic and hydrogeologic systems that are generally difficult to visualize, and we draw upon examples wherever possible to relate to students' common experiences. As one example, the discussion of well hydrographs incorporates data that link to recent/ongoing drought conditions in California, and compare a well response over multiple years in this area with one in an area in the eastern United States that has been unaffected by drought. Instructors might consider exploring the USGS well database in real time in class. The website is easy to navigate and interpret.
The formative assessments are designed to ensure that students have digested each individual concept (e.g., porosity, permeability, well hydrographs). The two summative assessments include: (1) a Darcy tube flow experiment (see additional instructions for construction of a simple Darcy tube apparatus for the lab activity—also available to download in the student version of the assessment ); and (2) a worksheet and discussion of hydraulic head (also available in the student version of the assessment ). In general, students found the first part of the module to be straightforward, but struggled with the second part—specifically the concepts of hydraulic head and its relationship to flow direction, the generation of cross-sectional diagrams illustrating the water table or potentiometric surface, and the role of pressure vs. gravitational energy in driving flow. The Darcy experiment is critical as a building block toward understanding—and developing intuition about—the physics of groundwater percolation, in particular the influence of driving gradient (controlled by the slope of the experimental tube) and aquifer conductivity (material in the tubes). The exercise works best as an interactive lab in which students are asked to make predictions and take turns running the experiment. We have also found it effective to prompt the students to take the lead by deciding what they need to measure, how to make the measurements, and where uncertainties may originate. As noted in the overall course notes, the lab itself requires at least 75 minutes to ensure ample time for setup, multiple experimental trials, and some discussion. The assigned summative assessment requires the students to synthesize concepts, and to plot their data with original data from Darcy's 1856 experiments and compare the two. The assigned exercise provides important context while also continuing to give students practice in plotting and interpreting graphical data.
Most students were able to complete the formative assessments in Part 1 correctly, though some had difficulty with Formative Assessment 3, which required them to piece together factors that control hydraulic conductivity, including grain size and sorting, fluid viscosity, and porosity. Most students were able to generate plots for the Darcy experiment and interpret them correctly in the context of hydraulic conductivity, and the formulation of Darcy's Law, by which flux is proportional to the hydraulic gradient. Some students struggled with the mechanics of generating plots, and others with the calculation of slope or the relationship between the slope of the plot and equation for a line.
The formative assessments in Part 2 posed a greater challenge to most students, and in particular assessments #2 and #3 related to well hydrographs and cones of depression. Many students did not understand the concept that groundwater would flow down gradient (perpendicular to contours of equal head); others did not understand the concept of a cross-sectional diagram or the idea that the potentiometric surface map is analogous to a topographic contour map. Confusion about the concept of hydraulic head was also apparent from some answers to formative assessment questions about confined vs. unconfined aquifers and artesian conditions. We found that by the time of the Part 2 Summative Assessment on hydraulic head, most students were able to complete the assignment and—with guidance and some individual explanation or discussion—developed a clear understanding of hydraulic head and the potentiometric surface.
Reflections In general, we found that the volume of material in this module, combined with the incorporation of several abstract/mathematical concepts including hydraulic head and Darcy's Law, necessitated in-class discussion to review and discuss the module content and Formative Assessments.
While Module 6, Part 1 is generally straightforward, students would probably benefit from additional discussion of aquifer properties. These might include: a review of key diagrams to explain porosity, grain size and sorting, and tortuosity; and some examples of rock specimens that serve as aquifers or aquitards—for example, hand specimens of sandstones, shales, and other rocks that the students can inspect with hand lenses or pour water onto. By the same token, devoting additional class time to the Darcy lab, either via a longer class period or a second class meeting devoted to follow-up discussion, would allow students time to generate plots and interpret them with their peers and with guidance from instructors. This would also avoid overlap of assigned "homework" from the Module 6 Summative Assessment with the start of readings and formative assessments in the next module.
For Module 6, Part 2, additional time devoted to interpretation of potentiometric surface maps, further developing the analogy to topographic maps (as covered in the Module 3 Summative Assessment ), tracing flowlines, and the effects of pumping wells would help to solidify key concepts. Drawing upon current events for discussion of the link between well hydrographs and climatic conditions would also be valuable in both illustrating the connection between surface processes (e.g., Module 2 ) and groundwater resources, and in making the relevance of the material clearer. If instructors have access to physical groundwater simulators (so called "ant farms"), these can also be a useful tool for in-class activity or demonstrations to help students visualize groundwater flow and the concept of hydraulic head. These can be constructed with simple materials as a groundwater model described by the NGWA ; or are available through teaching supply companies like Ward's.
Module 6.1 Formative Assessments
- Formative Assessment 1: Aquifers
- Formative Assessment 2: Permeability and Porosity
- Formative Assessment 3: Hydraulic Conductivity
Module 6.1 Summative Assessment
- The Summative assessment for Module 6.1 is Flow in Aquifers .
Module 6.2 Formative Assessments
- Formative Assessment 1: Hydraulic Gradient
- Formative Assessment 2: Well Hydrograph Records
- Formative Assessment 3: Cone of Depression
- Formative Assessment 4: High Plains Aquifer
Module 6.2 Summative Assessment
- The Summative assessment for Module 6.2 is Hydraulic Head and Aquifers .
References and Resources
- Readings from Student Materials — Module 6: Groundwater Hydrology
« Previous Page Next Page »
- Module 3: Rivers and Watersheds
- Module 4: Flood and Drought
- Module 5: Dam It All
- Student Materials
Teaching Themes
Already used some of these materials in a course? Let us know and join the discussion »
Considering using these materials with your students? Get advice for using GETSI modules in your courses » Get pointers and learn about how it's working for your peers in their classrooms »

- About this Site
- Accessibility
Reuse of InTeGrate Materials
We encourage the reuse and dissemination of the material on this site for noncommercial purposes as long as attribution to the original material on the InTeGrate site is retained.
Material on this page is offered under a Creative Commons license unless otherwise noted below.
Show terms of use for text on this page »
Show terms of use for media on this page »
- None found in this page
Learn more about Citing, Reusing and Adapting InTeGrate materials for your classroom
- Last Modified: April 14, 2024
- Short URL: https://serc.carleton.edu/175919 What's This?
Disclaimer: Any opinions, findings, conclusions or recommendations expressed in this website are those of the author(s) and do not necessarily reflect the views of the National Science Foundation.
Estimation of Unconfined Aquifer Transmissivity Using a Comparative Study of Machine Learning Models
- Published: 24 August 2023
- Volume 37 , pages 4909–4931, ( 2023 )
Cite this article
- Zahra Dashti 1 ,
- Mohammad Nakhaei 1 , 2 ,
- Meysam Vadiati ORCID: orcid.org/0000-0003-0841-6919 3 ,
- Gholam Hossein Karami 1 &
- Ozgur Kisi 4 , 5
257 Accesses
3 Citations
Explore all metrics
Groundwater management is key to attaining sustainable development goals, especially in arid and semi-arid countries. Hence, a precise estimate of the aquifer hydrodynamic parameters (hydraulic conductivity, transmissivity, specific yield, and storage coefficient) is required for proper groundwater resource management. The central goal of this research is to utilize machine learning models to estimate transmissivity by pumping test data in unconfined alluvial aquifers. Artificial neural network (ANN), gene expression programming (GEP), adaptive neuro-fuzzy inference system (ANFIS), fuzzy logic (FL), least square support vector machine (LSSVM), and group method of data handling (GMDH) methods were utilized to estimate transmissivity. To achieve this goal, pumping tests and hydrogeological data from 96 pumping wells located in the central plateau of Iran were collected and normalized. Using the existing normalized data, several combinations were utilized as inputs to the models, and then randomly, 70% of the data were used for the training step and 30% for the testing step. Finally, ten combinations that provided a better answer were selected from whole combinations. The mean absolute error, root means square error and correlation coefficient were used to assess the models' precision. The comparison criteria revealed that while all developed methods could provide desirable transmissivity estimations, the GMDH model was the most accurate and precise. The result of the models suggested that the best combination in transmissivity estimating was combination 3 (well discharge, thickness, depth of pumping well, and minimum and maximum time-drawdown data). Therefore, using this method and without graphic methods, it is feasible to estimate the transmissivity with acceptable accuracy in unconfined aquifers with similar hydrogeological and geological characteristics.
This is a preview of subscription content, log in via an institution to check access.
Access this article
Price includes VAT (Russian Federation)
Instant access to the full article PDF.
Rent this article via DeepDyve
Institutional subscriptions
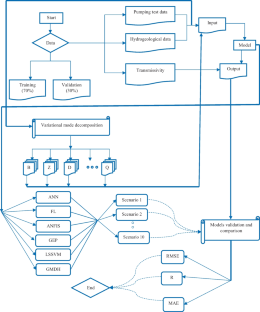
Similar content being viewed by others
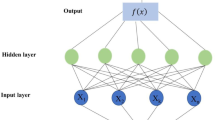
Application of artificial intelligence models for prediction of groundwater level fluctuations: case study (Tehran-Karaj alluvial aquifer)
Meysam Vadiati, Zahra Rajabi Yami, … Ozgur Kisi
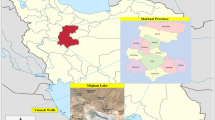
A Comparative Study of Artificial Intelligence Models and A Statistical Method for Groundwater Level Prediction
Mojtaba Poursaeid, Amir Houssain Poursaeid & Saeid Shabanlou
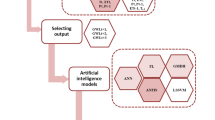
Groundwater Level Simulation Using Soft Computing Methods with Emphasis on Major Meteorological Components
Saeideh Samani, Meysam Vadiati, … Ozgur Kisi
Availability of Data and Materials
The data, models, and codes generated or used during the study are available from the corresponding author by request.
Abbreviations
Ant Colony Optimization
Artificial Intelligence
Adaptive Neuro-Fuzzy Inference System
Artificial Neural Network
Crow Search Algorithm
Differential Evolution
Fuzzy Logic
Genetic Algorithm
Gene Expression Programming
Group Method of Data Handling
Hydrodynamic Parameter
Levenberg–Marquardt
Least Square Support Vector Machine
Mean Absolute Error
Membership Functions
Machine Learning
Means Squared Error
Particle Swarm Optimization
Correlation Coefficient
Root Means Squared Error
Transmissivity
Arabameri A, Arora A, Pal SC, Mitra S, Saha A, Nalivan OA, Somayeh P, Moayedi H (2021) K-fold and state-of-the-art metaheuristic machine learning approaches for groundwater potential modelling. Water Resour Manag 35:1837–1869. https://doi.org/10.1007/s11269-021-02815-5
Article Google Scholar
Ayvaz MT (2007) Simultaneous determination of aquifer parameters and zone structures with fuzzy c-means clustering and meta-heuristic harmony search algorithm. Adv Water Resour 30(11):2326–2338. https://doi.org/10.1016/j.advwatres.2007.05.009
Azari T, Samani N (2018) Modeling the Neuman’s well function by an artificial neural network for the determination of unconfined aquifer parameters. Comput Geosci 22(4):1135–1148. https://doi.org/10.1007/s10596-018-9742-8
Azari T, Samani N, Mansoori E (2015) An artificial neural network model for the determination of leaky confined aquifer parameters: an accurate alternative to type curve matching methods. Iran J Sci Technol (Sci) 39(4):463–472. https://doi.org/10.22099/IJSTS.2015.3389
Bahmani R, Ouarda TB (2021) Groundwater level modeling with hybrid artificial intelligence techniques. J Hydrol 595:125659. https://doi.org/10.1016/j.jhydrol.2020.125659
Balkhair KS (2002) Aquifer parameters determination for large diameter wells using neural network approach. J Hydrol 265(1–4):118–128. https://doi.org/10.1016/S0022-1694(02)00103-8
Bateni SM, Mortazavi-Naeini M, Ataie-Ashtiani B, Jeng DS, Khanbilvardi R (2015) Evaluation of methods for estimating aquifer hydraulic parameters. Appl Soft Comput 28:541–549. https://doi.org/10.1016/j.asoc.2014.12.022
Bear J (1979) Hydraulics of Groundwater. McGraw Hill Inc, New York, NY, pp 432
Ch S, Mathur S (2012) Particle swarm optimization trained neural network for aquifer parameter estimation. KSCE J Civ Eng 16(3):298–307. https://doi.org/10.1007/s12205-012-1452-5
Chang CC, Lin CJ (2011) LIBSVM: a library for support vector machines. ACM Trans Intell Syst Technol (TIST) 2(3):1–27. https://doi.org/10.1145/1961189.1961199
Chiu SL (1994) Fuzzy model identification based on cluster estimation. J Intell Fuzzy Syst 2(3):267–278. https://doi.org/10.3233/IFS-1994-2306
Cui F, Al-Sudani ZA, Hassan GS, Afan HA, Ahammed SJ, Yaseen ZM (2022) Boosted artificial intelligence model using improved alpha-guided grey wolf optimizer for groundwater level prediction: Comparative study and insight for federated learning technology. J Hydrol 606:127384. https://doi.org/10.1016/j.jhydrol.2021.127384
Das M, Bhattacharjya RK, Kartha SA (2023) ANN-SFLA based parameter estimation method for an unsaturated–saturated simulation model. Model Earth Syst Environ 1–15. https://doi.org/10.1007/s40808-023-01797-0
Dashti Z, Nakhaei M, Vadiati M, Karami GH, Kisi O (2023) A literature review on pumping test analysis (2000–2022). Environ Sci Pollut Res 30(4):9184–9206. https://doi.org/10.1007/s11356-022-24440-4
Delnaz A, Rakhshandehroo G, Nikoo MR (2019) Optimal estimation of unconfined aquifer parameters in uncertain environment based on fuzzy transformation method. Water Supply 19(2):444–450. https://doi.org/10.2166/ws.2018.089
Delnaz A, Rakhshandehroo G, Nikoo MR (2020) Confined aquifer’s hydraulic parameters estimation by a generalized regression neural network. Iran J Sci Technol Trans Civil Eng 44(1):259–269. https://doi.org/10.1007/s40996-019-00238-2
Ferreira C (2001) Gene expression programming: a new adaptive algorithm for solving problems. arXiv preprint cs/0102027. https://doi.org/10.48550/arXiv.cs/0102027
Ghobadi A, Cheraghi M, Sobhanardakani S, Lorestani B, Merrikhpour H (2022) Groundwater quality modeling using a novel hybrid data-intelligence model based on gray wolf optimization algorithm and multilayer perceptron artificial neural network: a case study in Asadabad Plain, Hamedan, Iran. Environ Sci Pollut Res 29(6):8716–8730. https://doi.org/10.1007/s11356-021-16300-4
Gholami V, Khaleghi MR, Pirasteh S, Booij MJ (2022) Comparison of self-organizing map, artificial neural network, and co-active neuro-fuzzy inference system methods in simulating groundwater quality: Geospatial artificial intelligence. Water Resour Manag 1–19. https://doi.org/10.1007/s11269-021-02969-2
Gupta PK, Maiti S (2023) Enhancing the prediction of hydraulic parameters using machine learning, integrating multiple attributes of GIS and geophysics. Hydrogeol J 31(2):501–520. https://doi.org/10.1007/s10040-022-02567-5
Ha D, Zheng G, Zhou H, Zeng C, Zhang H (2020) Estimation of hydraulic parameters from pumping tests in a multiaquifer system. Underground Space 5(3):210–222. https://doi.org/10.1016/j.undsp.2019.03.006
Heddam S, Ptak M, Sojka M, Kim S, Malik A, Kisi O, Zounemat-Kermani M (2022) Least square support vector machine-based variational mode decomposition: a new hybrid model for daily river water temperature modeling. Environ Sci Pollut Res 1–28. https://doi.org/10.1007/s11356-022-20953-0
Ivakhnenko AG (1971) Polynomial theory of complex systems. IEEE Trans Syst Man Cybern 4:364–378. https://doi.org/10.1109/TSMC.1971.4308320
Jang JS (1993) ANFIS: adaptive-network-based fuzzy inference system. IEEE Trans Syst Man Cybern 23(3):665–685. https://doi.org/10.1109/21.256541
Karahan H, Ayvaz MT (2008) Simultaneous parameter identification of a heterogeneous aquifer system using artificial neural networks. Hydrogeol J 16(5):817–827. https://doi.org/10.1007/s10040-008-0279-0
Khalaf S, Ahmed AO, Abdalla MG, El Masry AA (2017) Identification of hydraulic parameters of wadi el natrun pliocene aquifer using artificial neural network. Hydrol Current Res 8(267):2. https://doi.org/10.4172/2157-7587.1000267
Khedri A, Kalantari N, Vadiati M (2020) Comparison study of artificial intelligence method for short term groundwater level prediction in the northeast Gachsaran unconfined aquifer. Water Supply 20(3):909–921. https://doi.org/10.2166/ws.2020.015
Khorrami B, Ali S, Gündüz O (2023) Investigating the local-scale fluctuations of groundwater storage by using downscaled GRACE/GRACE-FO JPL mascon product Based on Machine Learning (ML) Algorithm. Water Resour Manag 1–18. https://doi.org/10.1007/s11269-023-03509-w
Kisi O (2015) Pan evaporation modeling using least square support vector machine, multivariate adaptive regression splines and M5 model tree. J Hydrol 528:312–320. https://doi.org/10.1016/j.jhydrol.2015.06.052
Lin GF, Chen GR (2006) An improved neural network approach to the determination of aquifer parameters. J Hydrol 316(1–4):281–289. https://doi.org/10.1016/j.jhydrol.2005.04.023
Lin HT, Ke KY, Chen CH, Wu SC, Tan YC (2010) Estimating anisotropic aquifer parameters by artificial neural networks. Hydrol Process 24(22):3237–3250. https://doi.org/10.1002/hyp.7750
Mantoglou A (2003) Estimation of heterogeneous aquifer parameters from piezometric data using ridge functions and neural networks. Stoch Env Res Risk Assess 17(5):339–352. https://doi.org/10.1007/s00477-003-0155-3
Mathon BR, Ozbek MM, Pinder GF (2008) Transmissivity and storage coefficient estimation by coupling the Cooper-Jacob method and modified fuzzy least-squares regression. J Hydrol 353(3–4):267–274. https://doi.org/10.1016/j.jhydrol.2008.02.004
Mehdizadeh S, Behmanesh J, Khalili K (2017) Application of gene expression programming to predict daily dew point temperature. Appl Therm Eng 112:1097–1107. https://doi.org/10.1016/j.applthermaleng.2016.10.181
Minasny B, McBratney AB (2002) The neuro-m method for fitting neural network parametric pedotransfer functions. Soil Sci Soc Am J 66(2):352–361. https://doi.org/10.2136/sssaj2002.3520
Mohammed M, Sharafati A, Al-Ansari N, Yaseen ZM (2020) Shallow foundation settlement quantification: application of hybridized adaptive neuro-fuzzy inference system model. Adv Civil Eng. https://doi.org/10.1155/2020/7381617
Mozaffari S, Javadi S, Moghaddam HK, Randhir TO (2022) Forecasting groundwater levels using a hybrid of support vector regression and particle swarm optimization. Water Resour Manag 36(6):1955–1972. https://doi.org/10.1007/s11269-022-03118-z
Najafzadeh M, Homaei F, Mohamadi S (2022) Reliability evaluation of groundwater quality index using data-driven models. Environ Sci Pollut Res 29(6):8174–8190. https://doi.org/10.1007/s11356-021-16158-6
Nariman-Zadeh N, Darvizeh A, Darvizeh M, Gharababaei H (2002) Modelling of explosive cutting process of plates using GMDH-type neural network and singular value decomposition. J Mater Process Technol 128(1–3):80–87. https://doi.org/10.1016/S0924-0136(02)00264-9
Neuman SP (1975) Analysis of pumping test data from anisotropic unconfined aquifers considering delayed gravity response. Water Resour Res 11(2):329–342. https://doi.org/10.1029/WR011i002p00329
Nourani V, Mousavi S (2016) Spatiotemporal groundwater level modeling using hybrid artificial intelligence-meshless method. J Hydrol 536:10–25. https://doi.org/10.1016/j.jhydrol.2016.02.030
Ozturk M, Salman O, Koc M (2011) Artificial neural network model for estimating the soil temperature. Can J Soil Sci 91(4):551–562. https://doi.org/10.4141/cjss10073
Pan Z, Lu W, Wang H, Bai Y (2022) Fast inverse estimation of hydraulic conductivity field based on a deep convolutional-cycle generative adversarial neural network. J Hydrol 613:128420. https://doi.org/10.1016/j.jhydrol.2022.128420
Patel S, Eldho TI, Rastogi AK (2020) Hybrid-metaheuristics based inverse groundwater modelling to estimate hydraulic conductivity in a nonlinear real-field large aquifer system. Water Resour Manage 34:2011–2028. https://doi.org/10.1007/s11269-020-02540-5
Pham QB, Kumar M, Di Nunno F, Elbeltagi A, Granata F, Islam ARM, Talukdar S, Nguyen XC, Ahmed AN, Anh DT (2022) Groundwater level prediction using machine learning algorithms in a drought-prone area. Neural Comput Appl 1–23. https://doi.org/10.1007/s00521-022-07009-7
Prasad KL, Rastogi AK (2001) Estimating net aquifer recharge and zonal hydraulic conductivity values for Mahi Right Bank Canal project area, India by genetic algorithm. J Hydrol 243(3–4):149–161. https://doi.org/10.1016/S0022-1694(00)00364-4
Rahbar A, Mirarabi A, Nakhaei M, Talkhabi M, Jamali M (2022) A comparative analysis of data-driven models (SVR, ANFIS, and ANNs) for daily karst spring discharge prediction. Water Resour Manag 36(2):589–609. https://doi.org/10.1007/s11269-021-03041-9
Raheja H, Goel A, Pal M (2022) Prediction of groundwater quality indices using machine learning algorithms. Water Pract Technol 17(1):336–351. https://doi.org/10.2166/wpt.2021.120
Rajesh M, Kashyap D, Hari Prasad KS (2010) Estimation of unconfined aquifer parameters by genetic algorithms. Hydrol Sci J 55(3):403–413. https://doi.org/10.1080/02626661003738167
Razzagh S, Sadeghfam S, Nadiri AA, Busico G, Ntona MM, Kazakis N (2022) Formulation of Shannon entropy model averaging for groundwater level prediction using artificial intelligence models. Int J Environ Sci Technol 19(7):6203–6220. https://doi.org/10.1007/s13762-021-03793-2
Roshni T, Jha MK, Deo RC, Vandana A (2019) Development and evaluation of hybrid artificial neural network architectures for modeling spatio-temporal groundwater fluctuations in a complex aquifer system. Water Resour Manag 33:2381–2397. https://doi.org/10.1007/s11269-019-02253-4
Saha S, Mallik S, Mishra U (2022) Groundwater depth forecasting using machine learning and artificial intelligence techniques: A survey of the literature. recent developments in sustainable infrastructure (ICRDSI-2020)—GEO-TRA-ENV-WRM 153–167. https://doi.org/10.1007/978-981-16-7509-6_13
Sahour S, Khanbeyki M, Gholami V, Sahour H, Kahvazade I, Karimi H (2023) Evaluation of machine learning algorithms for groundwater quality modeling. Environ Sci Pollut Res 30(16):46004–46021. https://doi.org/10.1007/s11356-023-25596-3
Samani N, Gohari-Moghadam M, Safavi AA (2007) A simple neural network model for the determination of aquifer parameters. J Hydrol 340(1–2):1–11. https://doi.org/10.1016/j.jhydrol.2007.03.017
Samani S, Vadiati M, Azizi F, Zamani E, Kisi O (2022) Groundwater level simulation using soft computing methods with emphasis on major meteorological components. Water Resour Manag 36(10):3627–3647. https://doi.org/10.1007/s11269-022-03217-x
Samuel MP, Jha MK (2003) Estimation of aquifer parameters from pumping test data by genetic algorithm optimization technique. J Irrig Drain Eng 129(5):348–359. https://doi.org/10.1061/(ASCE)0733-9437(2003)129:5(348)
Sanikhani H, Kisi O (2012) River flow estimation and forecasting by using two different adaptive neuro-fuzzy approaches. Water Resour Manag 26(6):1715–1729. https://doi.org/10.1007/s11269-012-9982-7
Shigidi A, Garcia LA (2003) Parameter estimation in groundwater hydrology using artificial neural networks. J Comput Civ Eng 17(4):281–289. https://doi.org/10.1061/(ASCE)0887-3801(2003)17:4(281)
Sobhani J, Najimi M, Pourkhorshidi AR, Parhizkar T (2010) Prediction of the compressive strength of no-slump concrete: A comparative study of regression, neural network and ANFIS models. Constr Build Mater 24(5):709–718. https://doi.org/10.1016/j.conbuildmat.2009.10.037
Suryanarayana C, Sudheer C, Mahammood V, Panigrahi BK (2014) An integrated wavelet-support vector machine for groundwater level prediction in Visakhapatnam, India. Neurocomputing 145:324–335. https://doi.org/10.1016/j.neucom.2014.05.026
Suykens JAK, Vanderwalle J (1999) Least square support vector machine classifier. Neural Process Lett 9:293–300. https://doi.org/10.1023/A:1018628609742
Tabari MR, M., Azari, T., & Dehghan, V. (2021) A supervised committee neural network for the determination of aquifer parameters: a case study of Katasbes aquifer in Shiraz plain. Iran Soft Computing 25(6):4785–4798. https://doi.org/10.1007/s00500-020-05487-2
Tadj W, Chettih M, Mouattah K (2021) A new hybrid algorithm for estimating confined and leaky aquifers parameters from transient time-drawdown data. Soft Comput 25(24):15463–15476. https://doi.org/10.1007/s00500-021-06224-z
Tao H, Hameed MM, Marhoon HA, Zounemat-Kermani M, Heddam S, Kim S, Sulaiman SO, Tan ML, Sa’adi, Z., Danandeh Mehr, A., Allawi, M. F., Abba, S.I., Zain, J. M., Falah, M. W., Jamei, M., Dhanraj Bokde, M., Bayatvarkeshi, M., Al-Mukhtar, M., Bhagat, S. K., Tiyasha, T., Khedher, K. M., Al-Ansari, N., Shahid, S., Yaseen, Z. M. (2022) Groundwater level prediction using machine learning models: a comprehensive review. Neurocomputing. https://doi.org/10.1016/j.neucom.2022.03.014
Vakhshouri B, Nejadi S (2018) Prediction of compressive strength of self-compacting concrete by ANFIS models. Neurocomputing 280:13–22. https://doi.org/10.1016/j.neucom.2017.09.099
Vu MT, Jardani A (2022) Multi-task neural network in hydrological tomography to map the transmissivity and storativity simultaneously: HT-XNET. J Hydrol 612:128167. https://doi.org/10.1016/j.jhydrol.2022.128167
Vu MT, Jardani A (2023) Multitasking neural network to jointly map discrete fracture structures and matrix transmissivity by inverting hydraulic data acquired in 2D fractured aquifers. XNET-fracture. Adv Water Resour 177:104463. https://doi.org/10.1016/j.advwatres.2023.104463
Wang WY, Kang JT, Li K, Fan YH, Lin P (2022) A Novel intelligent inversion method of hydrogeological parameters based on the disturbance-inspired equilibrium optimizer. Sustainability 14(6):3267. https://doi.org/10.3390/su14063267
Xue P, Wen Z, Zhao D, Jakada H, Liang X (2021) Determination of hydraulic conductivity and its spatial variability in the Jianghan Plain using a multi-format, multi-method approach. J Hydrol 594:125917. https://doi.org/10.1016/j.jhydrol.2020.125917
Yeh HD, Lin YC, Huang YC (2007) Parameter identification for leaky aquifers using global optimization methods. Hydrol Process Int J 21(7):862–872. https://doi.org/10.1002/hyp.6274
Yuan Z, Wang LN, Ji X (2014) Prediction of concrete compressive strength: Research on hybrid models genetic based algorithms and ANFIS. Adv Eng Softw 67:156–163. https://doi.org/10.1016/j.advengsoft.2013.09.004
Zheng G, Ha D, Loaiciga H, Zhou H, Zeng C, Zhang H (2019) Estimation of the hydraulic parameters of leaky aquifers based on pumping tests and coupled simulation/optimization: Verification using a layered aquifer in Tianjin, China. Hydrogeol J 27(8):3081–3095. https://doi.org/10.1007/s10040-019-02021-z
Download references
Acknowledgements
Iran Water Resources Management Company issued the data used in this research. The authors thank Iran Water Resources Management Company for providing the pumping test data.
Author information
Authors and affiliations.
Department of Applied Geology, Faculty of Earth Sciences, Kharazmi University, Tehran, Iran
Zahra Dashti, Mohammad Nakhaei & Gholam Hossein Karami
Head of Water Research Center, Kharazmi University, Tehran, Iran
Mohammad Nakhaei
Global Affairs, Hubert H. Humphrey Fellowship Program, University of California, 10 College Park, Davis, CA, 95616, USA
Meysam Vadiati
Department of Civil Engineering, Lübeck University of Applied Sciences, 23562, Lübeck, Germany
Department of Civil Engineering, Ilia State University, 0162, Tbilisi, Georgia
You can also search for this author in PubMed Google Scholar
Contributions
Z. Dashti and M. Vadiati analyzed and interpreted data and contributed to writing the manuscript. M. Nakhaei and G.H. Karami contributed to the interpretation and manuscript revision. O. Kisi was involved in revising the manuscript critically for important intellectual content. All authors read and approved the final manuscript.
Corresponding author
Correspondence to Meysam Vadiati .
Ethics declarations
Ethical approval.
Not applicable because this article does not contain any studies with human or animal subjects.
Consent to Participate
Not applicable.
Consent to Publish
We, the undersigned, give us consent to publish identifiable details, including text, figures, and tables, in the journal.
Conflict of Interest
The authors declare no conflict of interest.
Additional information
Publisher's note.
Springer Nature remains neutral with regard to jurisdictional claims in published maps and institutional affiliations.
Rights and permissions
Springer Nature or its licensor (e.g. a society or other partner) holds exclusive rights to this article under a publishing agreement with the author(s) or other rightsholder(s); author self-archiving of the accepted manuscript version of this article is solely governed by the terms of such publishing agreement and applicable law.
Reprints and permissions
About this article
Dashti, Z., Nakhaei, M., Vadiati, M. et al. Estimation of Unconfined Aquifer Transmissivity Using a Comparative Study of Machine Learning Models. Water Resour Manage 37 , 4909–4931 (2023). https://doi.org/10.1007/s11269-023-03588-9
Download citation
Received : 04 March 2023
Accepted : 12 August 2023
Published : 24 August 2023
Issue Date : September 2023
DOI : https://doi.org/10.1007/s11269-023-03588-9
Share this article
Anyone you share the following link with will be able to read this content:
Sorry, a shareable link is not currently available for this article.
Provided by the Springer Nature SharedIt content-sharing initiative
- Gene expression programming; Pumping test
- Least-square support vector machine
- Hydrodynamic characteristics
- Find a journal
- Publish with us
- Track your research
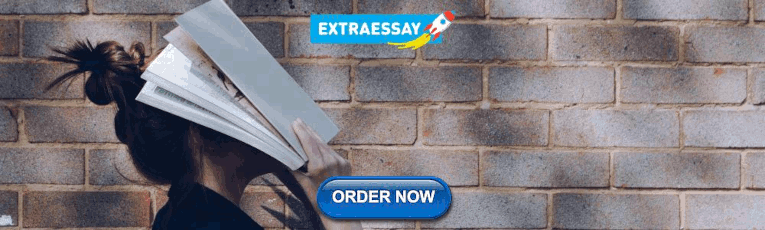
IMAGES
VIDEO
COMMENTS
Assignment 4: Exploring Flow with Aquifer Models Part I: Tabletop Aquifer Model (10 points) The first part of this assignment will use a table-top aquifer model to help you better understand hydraulic head and groundwater flow directions in unconfined and confined aquifers. This table-top model consists of: • A heterogeneous unconfined aquifer, which is mostly fine sand with some medium and ...
Assignment 4 Exploring Flow with Aquifer Models .pdf. Assignment 4: Exploring Flow with Aquifer Models Part I: Tabletop Aquifer Model (10 points) The first part of this assignment will use a table-top aquifer model to help you better understand hydraulic head and groundwater flow directions in unconfined and. Solutions available.
As a first step lets consider the flux law for groundwater flow. Groundwater flow is driven by the distribution of water pressure (hydraulic head, which has units of length) within the groundwater system. The flow of groundwater follows a flux law; q = Q A = −K Δh Δl (3) (3) q = Q A = − K Δ h Δ l. Where q q is the specific discharge (or ...
Description and Teaching Materials. Students use a virtual version of an analog model to explore the behavior of groundwater, using a virtual dye tracer. They explore the effects of pumping wells and observe the behavior of an artesian aquifer. Student handout for Online Groundwater Lab (Microsoft Word 2007 (.docx) 4.1MB Mar30 20)
Summative Assessment: Flow in Aquifers (In-Person Lab for Blended Class) Throughout Module 6.1, we have focused on the physical properties of aquifers that control the storage and movement of groundwater. Today's "laboratory" activity is designed to give you a first-hand look at how hydrogeologists actually quantify the ability of aquifers to ...
There are several popular aquifer models: van Everdingen-Hurst (VEH) model [1] Carter-Tracy model [2] Fetkovich model [3] Schilthuis model [4] Small- or pot-aquifer model [5] The first three models are unsteady-state models and are the most realistic. They attempt to simulate the complex pressure changes that gradually occur within the aquifer ...
We present a model of groundwater dynamics under stationary flow and, governed by Darcy's law of water motion through porous media, we apply it to study a 2D aquifer with water table of constant slope comprised of a homogeneous and isotropic media; the more realistic case of an homogeneous anisotropic soil is also considered. Taking into account some geophysical parameters we develop a ...
Assignment 4: Wells and Mapping Groundwater Flow Part I: An aquifer model The first part of this assignment will use a table-top aquifer model to help you better understand hydraulic head and groundwater flow directions in unconfined and confined aquifers. The table-top model consists of: a heterogeneous unconfined aquifer, which is mostly fine sand with some medium and coarse sand lenses; a ...
For further exploring the physical insight of τ, two new analytical models are developed to simulate confined flow for pumping in a stream-aquifer system. One model considers a flow equation for a single-zone aquifer and regards the streambed as the lagging Robin BC. On the other hand, the other considers a two-zone aquifer consisting of ...
Scoring and Rubric. Each answer will earn a maximum of 25 points, as described in the rubric below. Rubric. Work Shown. Possible Points. Provides a well-reasoned response to the question posed. 10. Uses correct grammar, spelling, and sentence structure. 5.
In this study, a sand-tank model as a physical analog of a real-world aquifer is presented for groundwater instruction. The sand tank is used for introducing flow nets and quantifying groundwater flow, for groundwater modeling and calibration via a spreadsheet, and for dye-transport modeling. Students learn from the sand tank through interaction and visualization, and by collecting and ...
Many small-scale ground water models are too small to incorporate distant aquifer boundaries. If a larger-scale model exists for the area of interest, flow and head values can be specified for boundaries in the smaller-scale model using values from the larger-scale model.
ALL GROUND-WATER HYDROLOGY WORK IS MODELING. A Model is a representation of a system. Modeling begins when one formulates a concept of a hydrologic system, continues with application of, for example, Darcy's Law or the Theis equation to the problem, and may culminate in a complex numerical simulation. All models are wrong, but some are useful.
As indicated in the flowchart in Fig. 4, we perform a qualitative assessment of the numerical model simulation from four distinct perspectives: the spatial distribution of groundwater recharge (Section 4.4.1), the regional groundwater flow direction (Section 4.4.2), groundwater age estimates (Section 4.4.3), and the identification of aquifer ...
Numerical Modeling Assignment 2 The goal of this homework is to understand the vertical flow and transport within the Bangladeshi aquifer by constructing a two-dimensional model in FEFLOW. The figure below illustrates the 2-D section that we are interested in modeling: 400 m 154 m 47m 73 m 98 m 200 m
Lumped Parameter Model. A lumped parameter model for the Edwards was developed in 1992 by Nisai Wanakule and Roberto Anaya with funding from various agencies. Their objective was to develop and test a simple and easy to use model to accurately simulate monthly water levels and springflows of the Edwards Aquifer.
The overall goal of Module 6, Groundwater Hydrology, is to develop a basic understanding of physical processes and properties that control the occurrence and movement of groundwater in the subsurface.The module has two parts and is designed to span a two-week session. The first part (Module 6.1) focuses on aquifers and their properties, with a series of case studies illustrating examples of ...
Name:_____ EVS-120 Exploring Aquifers through a Groundwater Model. Draw a diagram of the groundwater model and make labels for the following: ( points) A. Confined aquifer B. Unconfined aquifer C. Artesian aquifer D. Artesian wells E. Pollution sources F. Discharge area G. Recharge area H. Surface Waters. Questions:
the exception of the Van Everdingen & Hurst, whose model does not demand much computer power. 4.2.1 Pot Aquifer Model This method is one the simplest model for estimating the amount of water encroaching into hydrocarbon reservoirs. Mathematically, it is given as: W e¼ c wþc f fW iðÞP i 2P Where W i ¼ πðÞr a 2 2r e hθ 5:615,f ¼ θ 360 ...
0.001 ±7.03 m 3 s-1 for the Zwarte Beek, 0.06 ±20.4 m 3 s-1 for the Herk and Mombeek, and 1.23 ±29.1 m 3 s-1 for the Dijle (Fig. 105 3a) . The flow duration curve (FDC), which plots the cumulative frequency of the stream flow, represents the variability and
This model included a low-dip reservoir with a horizontal GWC surface—which accurately repli-cated actual edge-water drive reservoirs (Fig. 2). As seen in Figure 2, the reservoir radius varied in a range of 4-19 km. In the model, the aquifer radius and thickness were variable in the aquifer-reservoir interface region.
A fundamental goal of hydrogeophysics is to quantify the in-situ hydraulic conductivity of aquifers from remotely sensed geophysical data. There are a variety of established methods for quantifying hydraulic conductivity (Butler 2005), but most are point-scale methods that provide low horizontal resolution and are generally impractical for parameterizing large-scale groundwater-flow models ...
Groundwater management is key to attaining sustainable development goals, especially in arid and semi-arid countries. Hence, a precise estimate of the aquifer hydrodynamic parameters (hydraulic conductivity, transmissivity, specific yield, and storage coefficient) is required for proper groundwater resource management. The central goal of this research is to utilize machine learning models to ...