Starting Year: Feb. 2014
Articles Published: 404
Reviewed Articles: 27
Frequency: Quarterly
Language: Urdu
" Urdu Research Journal " is an open access refereed journal published quarterly. The Journal strives to publish work of high quality in research and literature works across the globe in Urdu language and literary theory. The aim of the journal is to provide high quality research material in Urdu for scholars and researchers.
EDITORIAL STAFF
Patron Prof. Ibne Kanwal
Chief Editor Dr. Uzair Israeel
Technical Assistant Nafees Ahmed
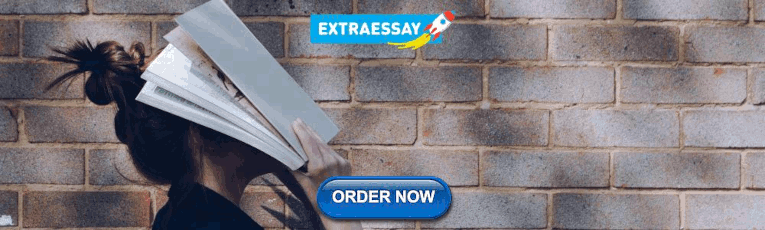
← ایسا کہا ں سے لاؤں کہ تجھ سا کہیں جسے
ڈاکٹر عزیر اسرائیل
Tags: اداریہ , پروفیسر ابن کنول
← قلمی چہرہ :پروفیسر ابن کنول
ڈاکٹر شفیع ایوب ، سی آئی ایل، جے این یو، نئی دہلی۔ 110067
Tags: پروفیسر ابن کنول
← منظوم خراج عقیدت
← کیوں؟ پروفیسر ابن کنول کے سانحہء ارتحال پر, ← ابنِ کنول صاحب (مثنوی کی ہئیت میں تعزیتی نظم).
ارشاد احمد ارشاد
← پہنچی وہیں پہ خاک جہاں کا خمیر تھا
متین امروہی
← رحلت ابن کنول (نظم بقید صنعت توشیح)
احمد امتیاز
← ابن کنول (خاص وضع قطع کا مخلص انسان)
صغیر افراہیم سابق صدر شعبہ اردو ، علی گڑھ مسلم یونی ورسٹی، علی گڑھ
← ہمدمِ دیرینہ – پروفیسر ابنِ کنول
ڈاکٹر صابر گودڑ
← منفی ماحول کا مثبت استعارہ: ابن کنول
پروفیسر محمد کاظم
← پیدا کہاں ہیں ایسے پراگندہ طبع لوگ
اکمل شاداب اسسٹنٹ پروفیسر شعبہ اردو، خواجہ معین الدین چشتی لسان یونی ورسٹی، کھنؤ
← آہ پروفیسر ابن کنول ۔۔۔۔۔دل کو کئی کہانیاں یاد سی آکے رہ گئیں
شبنم شمشاد اسسٹنٹ پروفیسر،شعبۂ اردو مانو،آرٹس اینڈ سائنس کالج فار وومن،سری نگر
← آتی رہے گی یاد ہمارے قابل ستائش استاد محترم ابن کنول
محمد جنید شکروی نائب پرنسپل آر بی جالان انٹر کالج دربھنگہ
← پروفیسرابن کنول:کچھ یادیں،کچھ باتیں
ڈاکٹرافضل مصباحی اسسٹنٹ پروفیسروسیکشن انچارج آف اردو ایم ایم وی، بنارس ہندویونیورسٹی، وارانسی، اترپردیش، بھارت
← زندہ رہتا ہے زمانے میں عمل اور کردار
ڈاکٹر ممتاز عالم رضوی مدیر اعلی روزنامہ قومی بھارت
← پروفیسر ابن کنول: ایک مشفق استاد کی باتیں اور یادیں
ڈاکٹر یامین انصاری ایڈیٹر، روزنامہ انقلاب، نوئیڈا، یوپی
← پروفیسر ابن کنول: ایک بے مثال استاد، لاثانی شخصیت
ڈاکٹر محمد شمس الدین اسسٹنٹ ڈائرکٹر، مولانا آزاد نیشنل اردو یونی ورسٹی اسٹدی سنٹر، بنارس، اترپردیش
← مخلص استاد پروفیسر ابن کنول
ڈاکٹر سدھارتھ سدیپ اسسٹنٹ پروفیسر شعبۂ اردو، خواجہ معین الدین چشتی لینگویج یونیورسٹی، لکھنؤ
← ابن کنول کی کہانیوں میں داستانوی اثرات
ڈاکٹر محمد ارشدندوی اسسٹنٹ پروفیسر(ایڈہاک )،شعبۂ اردو ، دیال سنگھ کا لج ،(دہلی یونیور سٹی ) لودھی روڈ،نئی دہلی ۳
← افسانہ’’ پہلا آدمی‘‘ ایک تجزیہ
عبید الرحمن نصیر ریسرچ اسکالر شعبہ اردو ،دہلی یونیورسٹی ،نئی دہلی(۱۱۰۰۰۷)
← ابن کنول کا افسانہ ’’بند راستے ‘‘کا تنقیدی مطالعہ
وجے کمار۔ریسرچ اسکالر شعبہ اردو جموں یونیورسٹی ،جموں و کشمیر
← بساط نشاط دل: ایک جائزہ
پروفیسر فاروق بخشی سابق صدر شعبہ اردو مولانا آزاد نیشنل اردو یونیورسٹی، حیدرآباد
← ابن کنول بحیثیت خاکہ نگار
شاہد اقبال ریسرچ اسکالر،دہلی یونیورسٹی،دہلی
← ’’کچھ شگفتگی کچھ سنجیدگی‘‘خاکوں کا گنجینۂ گوہر
ابراہیم افسر، میرٹھ، اترپردیش
← پروفیسرابن کنول کے سفر ناموں کا تجزیاتی مطالعہ
محمد یوسف ۔پی ۔ایچ ۔ڈی اسکالر بین الاقوامی اسلامی یونی ورسٹی اسلام آباد پروفیسرڈاکٹر کامران عباس کاظمی صدر شعبہ اردو و فارسی بین الاقوامی اسلامی یونی ورسٹی اسلام آباد
← ابن کنول کا سفر نامہ چار کھونٹ
آفاق حیدر گیسٹ لیکچرر سریندر ناتھ کالج فار ویمن کولکاتا
← پروفیسر ابن کنول کی سفرنامہ نگاری
ڈاکٹرمحمد عامر،002-نرمدا ہاسٹل، جے این یو، نئی دہلی
← ابن کنول کا ڈرامہ ’’خواب‘‘: ایک تنقیدی مطالعہ
ڈِمپلا دیوی ۔ ریسرچ اسکالر شعبہ اردو جموں یونیورسٹی
← داستانوی رنگ و آہنگ کا تخلیق کار:ابنِ کنول
پروفیسر آفتاب احمد آفاقی شعبۂ اردو ،بنارس ہندو یونیورسٹی، وارانسی
← پروفیسر ’’ابن کنول‘‘ اردو ادب کی روشنی میں
ڈاکٹر محمد طالب انصاری، ایسوسیٹ پروفیسر، کالج آف ایجوکیشن، مولانا آزاد نیشنل اردو یونی ورسٹی، حیدرآباد
← ابن کنول: ادبی خدمات
ڈاکٹر عبدالرّحمٰن، ریختہ فاؤنڈیشن، نوئیڈا، اترپردیش
← ابن کنول:اردو ادب کا ایک روشن باب
تنویر احمد، ریسرچ اسکالرشعبہ اردو دہلی یونیورسٹی،دہلی-۱۱۰۰۰۷
← پروفیسر ابن کنول : تعلیمی خیالات اور ادبی خدمات”
سونو رجک ریسرچ اسکالر مانو کالج آف ٹیچر ایجوکیشن دربھنگہ(بہار)
← ابن کنول کی شخصیت اور ادبی خدمات
ڈاکٹر محمد شاہد زیدی ، اسٹنٹ پروفیسر اردو گورنمنٹ پی ۔ جی۔ کالج سوائی مادھوپور (راجستھان )
Subscribe to our newsletter
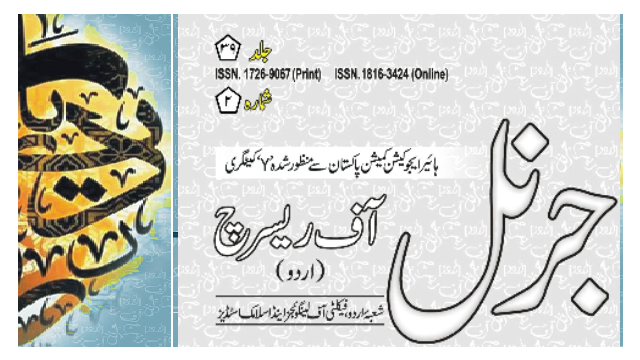
Vol.39,No.2(Dec 2023) has been Published
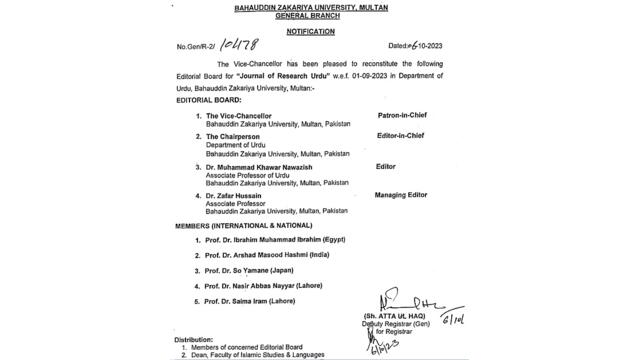
EDITORIAL BOARD HAS BEEN RECONSTITUTED
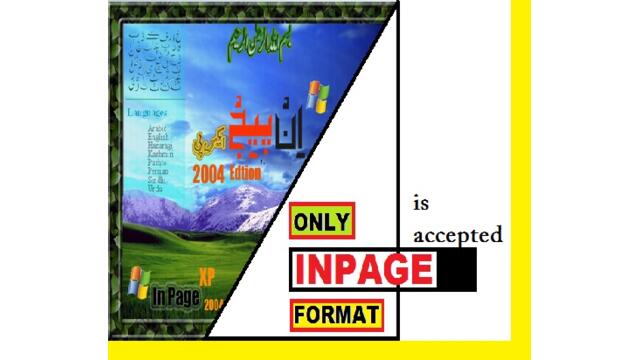
JOR (URDU) ACCEPTS ONLY INPAGE FORMAT
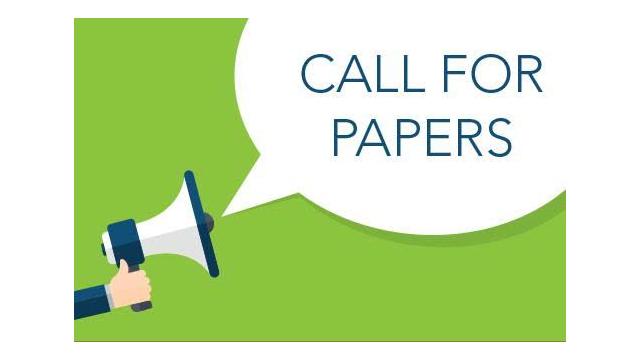
CALL FOR PAPER(S) IS OPEN
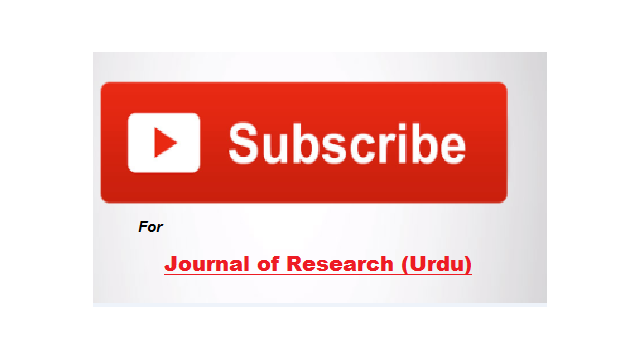
Subscribe JOR (Urdu) for your liberary
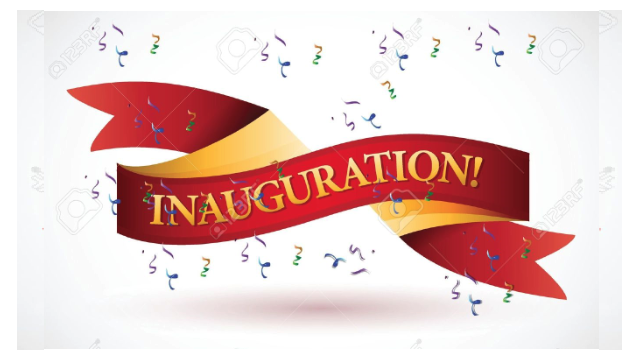
Inauguration of the website
Editor's choice.

اُردو کے تہذیبی معاشرے کا زوال (مہمان اداریہ)
- Dr. Athar Farouqui /
- December 31, 2023
اُردو غزلیات میں فارسی اَدبیات سے ماخوذ تلمیحات سے اِستفادے کا رُجحان :مختصر جائزہ
- Muhammad Mohsin Khalid /
Useful links
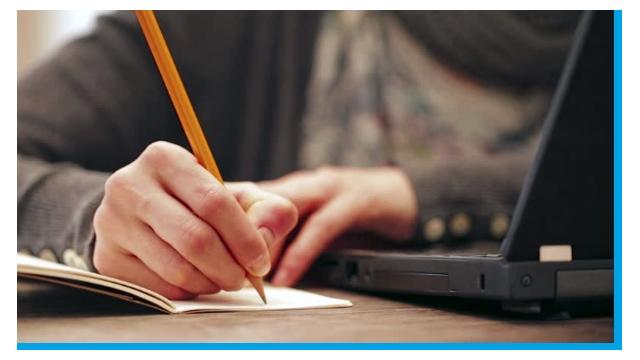
AUTHOR GUIDELINES
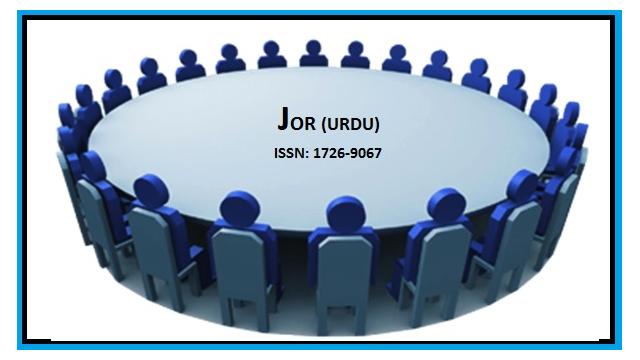
EDITORIAL BOARD
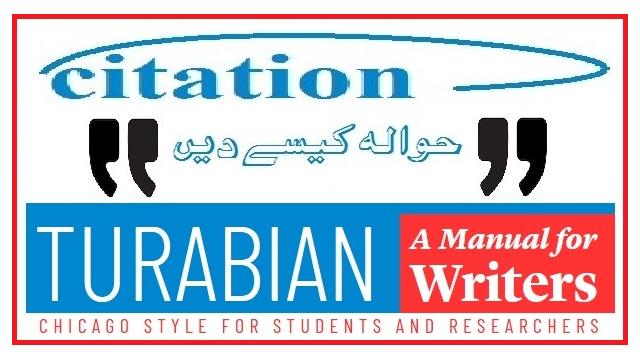
Citation Style
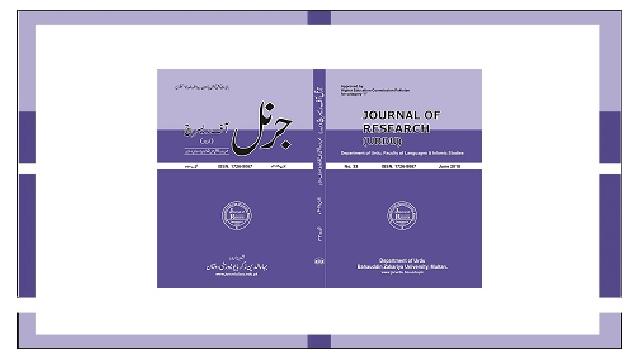
Current Issue
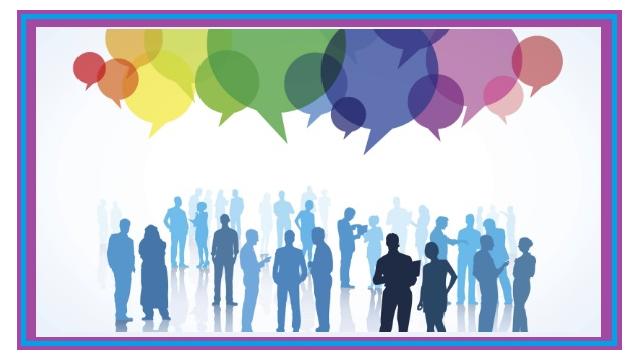
Advisory Board
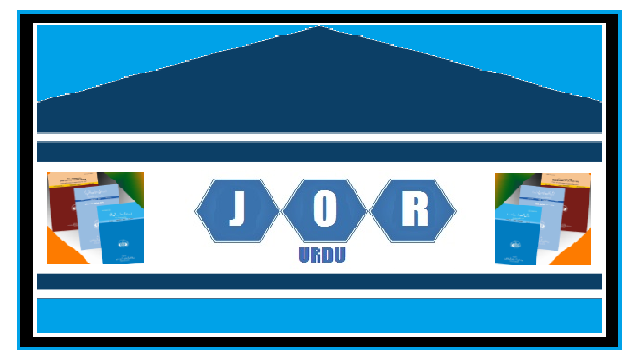
HEC Recognized Journals
Disclaimer .
- Journal of Research (Urdu)
Page activity
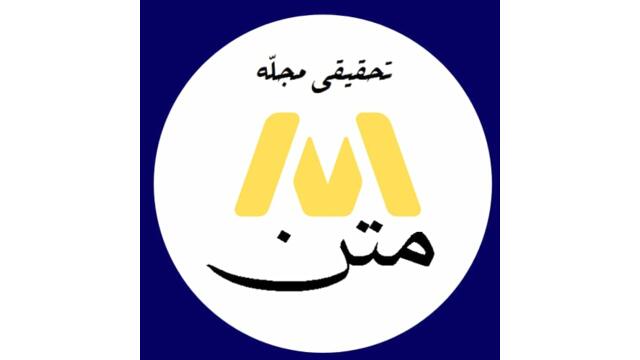
متْن (اردو ریسرچ جرنل)
شش ماہی تحقیقی مجلّہ.
Biannual Double Blind Peer Reviewed Urdu Research Journal of Urdu Department, The Islamia University of Bahawalpur.
MATAN (متْن), Department of Urdu, IUB.

Status: approved
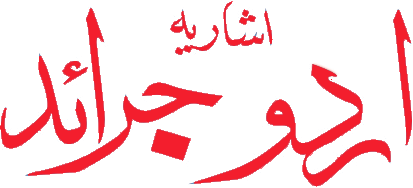
Status: applied
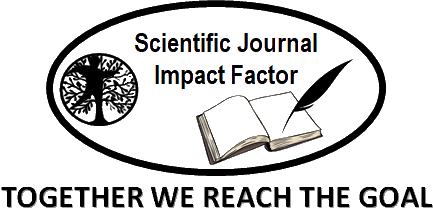

Brill | Nijhoff
Brill | Wageningen Academic
Brill Germany / Austria
Böhlau
Brill | Fink
Brill | mentis
Brill | Schöningh
Vandenhoeck & Ruprecht
V&R unipress
Open Access
Open Access for Authors
Open Access and Research Funding
Open Access for Librarians
Open Access for Academic Societies
Discover Brill’s Open Access Content
Organization
Stay updated
Corporate Social Responsiblity
Investor Relations
Policies, rights & permissions
Review a Brill Book
Author Portal
How to publish with Brill: Files & Guides
Fonts, Scripts and Unicode
Publication Ethics & COPE Compliance
Data Sharing Policy
Brill MyBook
Ordering from Brill
Author Newsletter
Piracy Reporting Form
Sales Managers and Sales Contacts
Ordering From Brill
Titles No Longer Published by Brill
Catalogs, Flyers and Price Lists
E-Book Collections Title Lists and MARC Records
How to Manage your Online Holdings
LibLynx Access Management
Discovery Services
KBART Files
MARC Records
Online User and Order Help
Rights and Permissions
Latest Key Figures
Latest Financial Press Releases and Reports
Annual General Meeting of Shareholders
Share Information
Specialty Products
Press and Reviews
Share link with colleague or librarian
Stay informed about this journal!
- Get New Issue Alerts
- Get Advance Article Alerts
Journal of Urdu Studies
- History & Culture
Institutional pricing (2024)
- Print Only €275.00 $318.00
- Print + Online €300.00 $349.00
- Online only €250.00 $290.00
- To place an order, please contact [email protected]
Individual pricing (2024)
- Online only €84.00 $97.00
- Print Only €84.00 $97.00
Call for Papers: Volume 2
Instructions for Authors
Journal menu
Submit article, editorial board, subject list.
- View PDF Flyer
Latest Articles
Front matter.
- Download PDF
Introduction: Seeing the World in Urdu
Debating caliphs and kings in the twentieth century: ʿabd ul-ḥalīm sharar’s essays on empire and governance, sayyid aḥmad dihlavī (1846–1918) on “the initial, intermediate, and final language of mankind” (1908), “can’t touch this”: early indian muslim responses to the saudi conquest of the hijaz, translating al-andalus: medieval muslim spain and urdu modernity, narrating economic history and the history of economic thought in urdu, historicizing the miraculous: muslim traditionalism and colonial modernity, tears of the begums: stories of survivors of the uprising of 1857 , written by niz̤āmī, ḳhvājah ḥasan, a most noble life: the biography of ashrafunnisa begum (1840–1903) , translated and edited by naim, c. m., bibi’s room: hyderabadi women and twentieth-century urdu prose , written by akhtar, nazia, back matter.
Reference Works
Primary source collections
COVID-19 Collection
How to publish with Brill
Open Access Content
Contact & Info
Sales contacts
Publishing contacts
Stay Updated
Newsletters
Social Media Overview
Terms and Conditions
Privacy Statement
Cookie Settings
Accessibility
Legal Notice
Terms and Conditions | Privacy Statement | Cookie Settings | Accessibility | Legal Notice | Copyright © 2016-2024
Copyright © 2016-2024
- [66.249.64.20|195.158.225.244]
- 195.158.225.244
Character limit 500 /500
Thank you for visiting nature.com. You are using a browser version with limited support for CSS. To obtain the best experience, we recommend you use a more up to date browser (or turn off compatibility mode in Internet Explorer). In the meantime, to ensure continued support, we are displaying the site without styles and JavaScript.
- View all journals
- My Account Login
- Explore content
- About the journal
- Publish with us
- Sign up for alerts
- Open access
- Published: 31 March 2022
Multi-class sentiment analysis of urdu text using multilingual BERT
- Lal Khan 1 ,
- Ammar Amjad 1 ,
- Noman Ashraf 2 &
- Hsien-Tsung Chang 1 , 3 , 4 , 5
Scientific Reports volume 12 , Article number: 5436 ( 2022 ) Cite this article
10k Accesses
30 Citations
1 Altmetric
Metrics details
- Computational science
- Computer science
- Information technology
- Machine learning
Sentiment analysis (SA) is an important task because of its vital role in analyzing people’s opinions. However, existing research is solely based on the English language with limited work on low-resource languages. This study introduced a new multi-class Urdu dataset based on user reviews for sentiment analysis. This dataset is gathered from various domains such as food and beverages, movies and plays, software and apps, politics, and sports. Our proposed dataset contains 9312 reviews manually annotated by human experts into three classes: positive, negative and neutral. The main goal of this research study is to create a manually annotated dataset for Urdu sentiment analysis and to set baseline results using rule-based, machine learning (SVM, NB, Adabbost, MLP, LR and RF) and deep learning (CNN-1D, LSTM, Bi-LSTM, GRU and Bi-GRU) techniques. Additionally, we fine-tuned Multilingual BERT(mBERT) for Urdu sentiment analysis. We used four text representations: word n -grams, char n -grams,pre-trained fastText and BERT word embeddings to train our classifiers. We trained these models on two different datasets for evaluation purposes. Finding shows that the proposed mBERT model with BERT pre-trained word embeddings outperformed deep learning, machine learning and rule-based classifiers and achieved an F1 score of 81.49%.
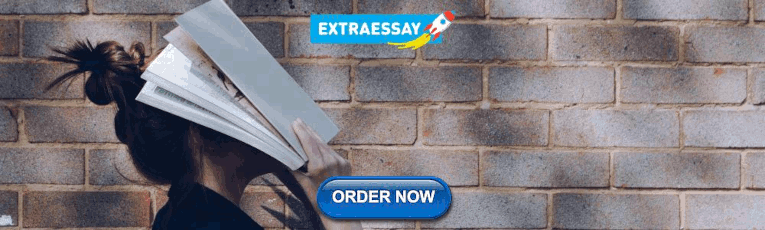
Similar content being viewed by others
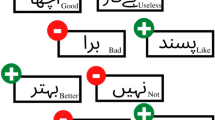
A hybrid dependency-based approach for Urdu sentiment analysis
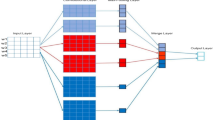
Amharic political sentiment analysis using deep learning approaches
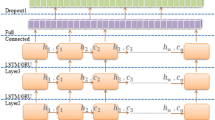
Character gated recurrent neural networks for Arabic sentiment analysis
Introduction.
Social networks (SNs) such as Blogs, Forums, Facebook, YouTube, Twitter, Instagram, and others have recently emerged as the most important platforms for social communication between diverse people 1 , 2 . As technology and awareness grow, more people are using the internet for global communication, online shopping, sharing their experiences and thoughts, remote education, and correspondence on numerous aspects of life 3 , 4 , 5 . Users are increasingly using SNs to communicate their views, opinions, and thoughts, as well as participate in discussion groups 6 . The inconspicuousness of the World Wide Web (WWW) has permitted single user to engage in aggressive SNs speech data that has made text conversation 7 , 8 or, more precisely, sentiment analysis (SA) is vital to understand the behaviors of people 9 , 10 , 11 , 12 , 13 , 14 , 15 .
The significance of sentiment analysis may be seen in our desire to know what they think and how others feel about the problem 16 . Firms and governments are looking for useful information in these user comments such as the feelings behind client comments 17 . SA refers to the application of machine and deep learning and computational linguistics to investigate the feelings or views expressed in user-written comments 18 , 19 . Because of increasing interest in SA, businesses are interested in driving campaigns, having more clients, overcoming their weaknesses, and winning marketing tactics. Business firms are interested to know the individual’s feedback and sentiments about their product and services 20 . Furthermore, politicians and their political parties are interested in learning about their public reputations. Due to the recent surge in SNs, sentiment analysis focus has shifted to social media data research. The importance of SA has increased in several fields, including movies, plays, sports, news chat shows, politics, harassment, services, and medical 21 . SA includes enhanced techniques for NLP, data mining for predictive studies, and topic modeling becomes an exciting domain of research 22 .
In terms of linguistics and technology, English and particular other European dialects are recognized as rich dialects. Yet, many other languages are classified as resource-deprived 23 , Urdu is one of them. The Urdu language requires a standard dataset, but unfortunately, scholars face a shortage of language resources. The Urdu language is Pakistan’s national and one of the official languages spoken in some state and union territories of India.
Sentiment analysis is as important for Urdu dialects as it is for any other dialect. Many obstacles make SA of the Urdu language difficult such as Urdu contains both formal and informal verb forms as well as masculine and feminine genders for each noun. Similarly, the Persian, Arabic, and Sanskrit languages have their terms in Urdu. Urdu is written from right to left, and the distinction between words is not always clear. The scarcity of acknowledged lexical resources 24 , 25 and the lack of Urdu text data due to morphological concerns. Rather than a conventional text encoding scheme, most Urdu websites are organized in an illustrated manner, which complicates the task of producing a state-of-the-art machine-readable corpus. The well-known sentiment lexicon database is an essential component for constructing sentiment analysis classification applications in any dialect. SentiWordNet is one of the several sentiment lexicons available in English. Urdu, on the other hand, is a resource-poor language with a severe lack of sentiment lexicon. Problems with Urdu word segmentation, morphological structure and vocabulary variances are among the main deterrents to developing a fully effective Urdu sentiment analysis model.
Research objective
This research aims to classify the semantic orientation of Urdu reviews. Our purposed model is inspired by 26 . In the cited paper, sentiment analysis of Arabic text was performed using pre-trained word embeddings. Recently, pre-trained algorithms have shown the state of the art results on NLP-related tasks 27 , 28 , 29 , 30 . These pre-trained models are trained on large corpus in order to capture long-term semantic dependencies.
The objective of this research study is to answer the following questions:
Is it possible to utilize a deep learning model in combination with a pre-trained word embedding strategy to identify the sentiment expressed by a social network user in Urdu?
Does the deep learning approach with fastText and BERT word embedding effective than the machine learning-based approaches and the rule-based approach to sentiment analysis for the Urdu language that have been studied so far?
To answer the first study question, the use of pre-trained word embeddings for sentiment analysis of Urdu language reviews is investigated. A deep learning model based on pre-trained word embedding captures long-term semantic relationships between words, unlike rule-based and machine learning-based approaches. To answer the second question, the deep learning models were compared to the machine learning-based methods and the rule-based method of Urdu sentiment analysis.
The main contribution of our research are as follows:
A new Multi-class sentiment analysis dataset for Urdu language based on user reviews. It is gathered from various domains such as food and beverages, movies and plays, software and apps, politics and sports. To the best of our knowledge, no such public Urdu corpus exists. The corpus will be made publicly available.
Fine-tuning a multilingual BERT model for Urdu sentiment classification, which has been trained on 104 languages, including Urdu, and is based on a BERT base with 12 layers, 768 hidden heads, and 110M parameters.
A set of baseline results of rule-based approach, machine-learning models (LR, MLP, Ada-Boost, RF, SVM) and deep learning models (1D-CNN, LSTM, Bi-LSTM, GRU and Bi-GRU) to create a benchmark for multi-class sentiment analysis using different text representations: fastText pre-trained word embeddings, char n -gram and word n -gram features.
The rest of the paper is organized as follows. Section “ Related work ” explains the related work for sentiment analysis. Section “ Corpus generation ” describes the creation of dataset and its statistics. Section “ Proposed methodology ” presents the proposed methodology. Section “ Results analysis ” analyze the experimental results and evaluation measures. Section “ Conclusion and implications ” concludes the paper.
Related work
In this section, we give a quick overview of existing datasets and popular techniques for sentiment analysis.
Sentiment analysis datasets
SemEval challenges are the most prominent efforts taken in the existing literature to create standard datasets for SA. In each competition, scholars accomplish different tasks to examine semantic analysis classifications using different corpora. The outcome of such competitions is a group of standard datasets and diverse approaches for SA. These benchmark corpora have been created in the English and Arabic languages 31 . Mainly, user tweets/reviews belong to various genres such as hotel, restaurants and laptops.
Every time, the SemEval contests series comes up with the various size of corpora. In the 2013 edition, the SemEval competition used SMS and Twitter corpora, and the Twitter corpus contains a total of 15,195 reviews, was split into training, development, and testing data are 9728, 1654, and 3813, respectively, while the SMS corpus consists of 2093 reviews was only used for testing purpose. The Twitter corpus comprises a total of 1853 reviews in the 2014 edition, including 86 sarcastic tweets for testing 32 . There were five separate subtasks in the 2016 and 2017 competition series. Each task’s corpus was divided into three sections: training, development, and testing. Subtask A, B, and D and subtask C and E sentences 30,632, 17,639, and 30,632 were used, respectively. There are 332 news articles in the Korean corpus for SA. Human experts manually annotated these news articles for sentiment analysis. The dataset contains 7713 subjectively annotated sentences and 17,615 opinionated expression tags utilizing the Korean Subjectivity Markup Language annotation method, reflecting the characteristics of Korean languages 33 .
Another corpus has been created in the Indonesian language. The Twitter streaming API was used to collect 3.5 million tweets 34 . A Roman Urdu corpus has been created, contains 10,021 user comments belonging to various domains such as politics, sports, food and recipes, software, and movies. All these sentences were manually annotated by three native speakers 35 .
Methods for sentiment analysis
Several methods have been proposed in the existing literature to solve SA tasks, such as supervised and unsupervised machine learning. In SemEval 2014 competition, both Support Vector Machine (SVM) and rule-based machine learning methods were applied. The lexicons were utilized to find the sentiment polarities of reviews using the rule-based technique. The overall polarity of the review was computed by summing the polarity scores of all words in the review and dividing by their distance from the aspect term. If a sentence’s polarity score is less than zero (0), it is classified as negative; if the score is equal to zero, it is defined as neutral; and if the score is equal to or more than one, it is defined as positive. These classified features and n -gram features have been used to train machine learning algorithms. In SemEval 2016 contest edition, many machine learning algorithms such as Linear Regression (LR), Random Forest (RF), and Gaussian Regression (GR) were used 31 . The word embeddings are enhanced Natural Language Processing (NLP) method representing words or phrases into numerical numbers names as vector. Machine learning algorithms such as SVM will determine a hyperplane that classifies tweets/reviews according to their sentiment. Similarly, RF generates various decision trees, and each tree is examined before a final choice is made. In the same way, Nave Bayes (NB) is a probabilistic machine learning method that is based on the Bayes theorem 36 .
Many research studies have been published to execute SA of various resource-deprived dialects like as Khmer, Thai, Roman Urdu, Arabic and Hindi. Based on the negation and discourse relationship, a study on Hindi dialect has been conducted for sentiment analysis. A corpus of human-annotated reviews in Hindi was created. An accuracy of 80.21% was achieved using a polarity-based method 37 . Similarly, few research studies have been conducted in the Thai dialect, also considered resource-deprived languages 38 . Another study was carried out to identify abusive words in the Thai dialect. Eighty-six percent of the f-measure was attained using the machine learning method. Similarly, a research study has been conducted in the Bengali dialect 39 . In this study, the SA of Bengali reviews is executed using the word2vec embedding model. Results reveal that their proposed algorithm achieved an accuracy of 75.5%.
Urdu datasets and machine learning techniques
The essential component of any sentiment analysis solution is a computer-readable benchmark corpus of consumer reviews. One of the most significant roadblocks for Urdu SA is a lack of resources, such as the lack of a gold-standard dataset of Urdu reviews. The truth is that most Urdu websites are designed in illustrative patterns rather than using standard Urdu encoding 40 . We recognized two methods for dataset creation from the existing literature, named as (1) automatic and (2) manual.
A research study focusing on Urdu sentiment analysis 41 created two datasets of user reviews to examine the efficiency of the proposed model. Only 650 movie reviews are included in the C1 dataset, with each review averaging 264 words in length. There are 322 positive and 328 negative reviews in corpus C1. The other dataset named C2, contains 700 reviews about refrigerators, air conditions, and televisions. The average length of words per review is 196 words.
Another study 42 used a corpus collected from the BBC Urdu news website to work on Urdu text classification. Two types of filters were successfully implemented to collect the required data. They concentrate on words like “Ghusa” (anger) and “Pyar” (love). A HTML parser is used to parse the obtained data, which yielded 500 news stories with 700 sentences containing the keywords mentioned above. These sentences were annotated for emotions. Nearly 6000 sentences not annotated with emotions were discarded from those 500 news articles.
Another study 43 on Urdu sentiment analysis subjectivity developed a corpus consisting of 6025 sentences from151 Urdu blogs from 14 various domains. Three human specialists manually classified these comments into three categories: neutral, negative, and positive. Additionally, they have implemented five supervised machine learning algorithms like SVM, Lib, NB (KNN, IBK), PART, and decision tree. Results reveal that KNN achieves the highest accuracy of 67.01% and performs better than other supervised machine learning algorithms. However, the performance of models can be enhanced by increasing the corpus size and using deep learning methods with pre-trained word embedding models.
Similarly, in work 44 , the comparison of NB versus SVM for the language preprocessing steps of Urdu documents reveals that SVM performs better than NB regarding accuracy. Additionally, normalized term frequency gives much improved results for feature selection. The major drawback of the proposed system is that the tokenization is done based on punctuation marks and white spaces. However, due to the grammatical structure of the Urdu language, the writer may put white space between a single word such as (Khoubsorat, beautiful), which will cause the tokenizer to tokenize the single word as two words (khoub) and (sorat), which is incorrect.
According to this study 45 , authors used three classic machine learning algorithms, such as NB, SVM, and Decision tree followed by a supervised machine learning approach to create Word Sense Disambiguation (WSD) in Urdu text. They test their theories using a corpus generated from Urdu news websites. They attain an f-measure of 0.71%. However, by implanting an adaptive mechanism, the system’s accuracy could be increased.
Urdu datasets and deep learning techniques
Deep learning approaches have recently been investigated for classification of Urdu text. In this study 46 , authors used deep learning methods to classify Urdu documents for product manufacturing. Stop words and infrequent words were deleted, which increased performance for medium and small datasets but decreased performance for large corpora. According to their findings, CNN with several filters (3,4,5) outperformed the competition, whereas BiLSTM outperformed CLSTM and LSTM. The authors of 47 used a single layer CNN with several filters to classify documents at the document level, and the results outperformed the baseline approaches. For document classification 48 , compared the performance of hybrid, machine learning, and deep learning models. According to their findings, the normalized difference measure-based feature selection strategy increases the accuracies of all models.
In this study 49 , authors recently suggested a model for Urdu SA by examining deep learning methods along with various word embeddings. For sentiment analysis, the effectiveness of deep learning algorithms such as LSTM, BiLSTM-ATT, CNN, and CNN-LSTM was evaluated.
The most significant work 50 has recently been performed on SA of Urdu text using various machine learning and deep learning techniques. Initially, Urdu user reviews of six various domains were collected from various social media platforms to build a state of art corpus. Later on, the whole Urdu corpus was manually annotated by human experts. Finally, a set of machine learning algorithms such as RF, NB, SVM, AdaBoost, MLP, LR, and deep learning algorithms such LSTM and CNN-1D were applied to validate the generated Urdu corpus. LR algorithms achieve the highest accuracy out of all others machine learning and deep learning algorithms.
A few research employing deep learning, semantic graphs and multimodal based system (MBS) have been undertaken on the areas of emotion classification 51 , concept extraction 52 , and user behavior analysis 53 . A unique CNN Text word2vec model was proposed in the research study 51 to analyze emotion in microblog texts. According to the testing results the suggested MBS 52 has a remarkable ability to learn the normal pattern of users’ everyday activities and detect anomalous behaviors.
There have been very few research studies on Urdu SA, and it is still in its early stages of maturation compared to other resource-rich languages like English. Because of the scarcity of linguistic resources, this can be discouraging for language engineering scholars. The majority of previous research papers 47 focused on various areas of language processing such as stemming, stop word recognition and removal, and Urdu word segmentation and normalization. The summery of the existing literature is presented in Table 1 .
Furthermore, the size of available annotated datasets is insufficient for successful sentiment analysis. However, the majority of the datasets and reviews from limited domains are only from negative and positive classes. To address this issue, this work focuses on the creation of an Urdu text corpus that includes sentences from several genres. To accomplish sentiment analysis task, we have applied various machine learning models with various features, deep learning models with combination of pre-trained word vectors and a rule-based algorithm on our created corpus UCSA-21 which has not yet investigated completely for the Urdu sentiment analysis text.
Corpus generation
This section explains how a manually annotated Urdu dataset was created to achieve Urdu SA. The collection of user comments and reviews from multiple websites, the compilation of human annotation rules, the execution of manual annotation, standardization, and finally, the description of the dataset’s features are all phases involved in creating the Urdu Corpus for Sentiment Analysis (UCSA-21).
We gathered data from websites that offered unfettered access and allowed users to remark in Urdu to create a benchmark dataset for assessing Urdu sentiment. Table 2 summarizes all of the websites that we visited to get user reviews. Movies, Pakistani and Indian drama, TV discussion shows, food and recipes, politicians and Pakistani political parties, sport, software, blogs and forums and gadgets were among the genres from which we gathered data. During a 5- to 6-month period, three people who were well-versed in the objective manually collected user comments. Initially, the data was gathered into an excel sheet along with the following details: (1) the review ID; (2) the review’s domain; and (3) the annotation label.
To implement Urdu SA, we need an annotated corpus containing user comments with their sentiments. Initially, annotations rules were defined then the corpus was annotated manually by three native speakers of the Urdu language keeping in mind those guidelines. All three native Urdu speakers were well aware of the purpose of annotation, annotated the complete dataset. Annotations guidelines were made for Urdu SA from existing literature. Figure 1 shows some samples of comments from the neutral, negative, and positive categories.
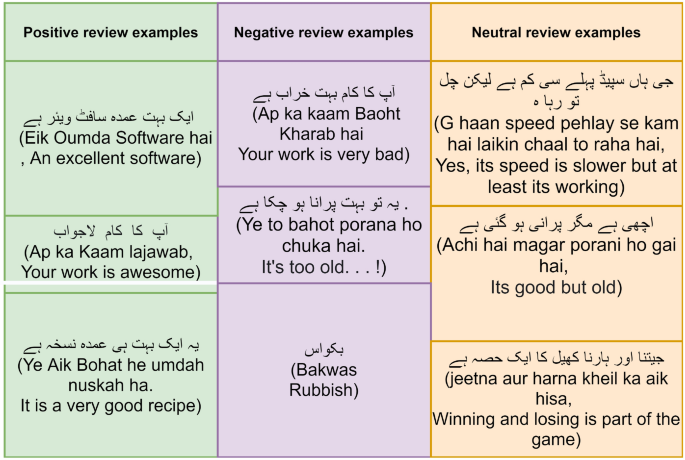
Examples of customer reviews label as neutral, positive and negative.
Annotation rules
A review is considered positive if the specified review expresses a positive meaning for all the characteristic terms. Suppose it contains words such as “acha” good, “Khoubsoorat” beautiful without containing negations like “Na” “Nahi” no as these words change the polarity 55 .
If any review expressing mutually neutral and positive classes, the review is marked as positive.
If any review expressing any agreement, then that review is classified as positive 56 .
If the user review expresses the negative sentiment in all aspects, then the review is marked as negative if it contains terms like “Bora” bad, “bukwas” rubbish, “zolum” cruelness, “ganda” dirty, without containing the negations as negations invert the polarity of the whole sentence 57 .
If a user comment comprises more negative words than any other class, it is classified as a negative review.
If a sentence contained straight unsoftened disagreements, then that sentence is classified as negative 56 .
If a review contained words such as banning, penalizing, assessing, and bidding, then that review is marked as a negative review 56 .
If a review comprises a denial, then that review is tagged as a negative review.
If a review contains a negative term with a positive adjective, then that sentence is marked as a negative review 58 .
Mockery: sentence such as “MashaAllah se koy to rank milli ha na hamari cricket team ko ...akhiri he sahi” (By the grace of God, our cricket team got at least some rank. may that be last) as classified as negative sentences 59 .
If a sentence contains a question such as “eis team ka kia banay ga” what will happen to this team? Showing frustrations is marked as a negative review 59 .
If a piece of factual information is presented in a sentence, then the sentence is marked as a neutral sentence?.
If assumptions, beliefs, or thoughts are shared in a review, then that review is identified as a neutral sentence 60 .
If words like maybe (Shaid) are present in a review, they are classified as neutral 56 .
A review containing both negative and positive opinions regarding the aspects is considered a neutral sentence 55 .
Corpus characteristics
To create the standard corpora, three human experts annotated the whole UCSA-21 dataset. Master graduates annotated each user review; they are native Urdu speakers and are well familiar with SA. To ensure that our annotation guidelines were proper, we gave a random sample of 100 reviews to two annotators (X and Y) and asked them to mark and mention which ones came under which conditions. Individualistically, both annotators classified these sentences into one of three categories: negative, neutral, and positive. The conflicting reviews among annotator x and annotator y were resolved by third annotator z keeping in mind the above-discussed annotations guidelines. For the entire dataset, we achieved an Inter-Annotator Agreement (IAA) of 71.45 percent using Cohens Kappa method. The findings of the IAA score and moderate scores show that the manual annotations rules were adequately drafted, well understood, and followed by annotation specialists during the annotation stage. After evaluating the data, it was shown that the majority of the disagreement occurred between the negative and neutral (11.60%) and positive and neutral (12.01%) classifications. Summary of the corpus presented in Table 3 and 4 , the UCSA-21 corpus comprises 9312 Urdu reviews, with 3,422 positive ratings, 2787 negative reviews, and 3103 neutral reviews. The statistics of corpus UCSA-21 show a class balance. Academics have worked hard to create datasets for sentiment analysis studies. Still, most of the available annotated datasets are too small and contain sentences from only a few domains, rather than multiple domains like UCSA-21. The other drawback of most of the existing corpora is they contain only two classes, negative and positive.
Proposed methodology
This section contains the experimental description of applied machine learning, rule-based, deep learning algorithms and our proposed two-layer stacked Bi-LSTM model. These algorithms have been trained and tested on our proposed UCSA-21 corpus and UCSA 50 datasets which are publically available.
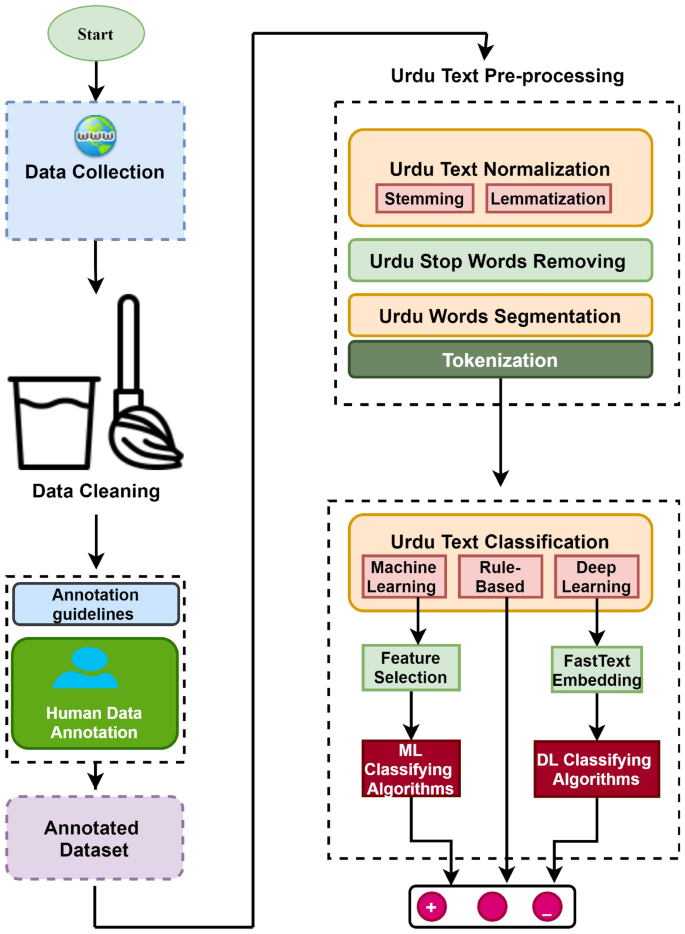
Proposed abstract level architecture for Urdu sentiment analysis.
Experimental datasets
In this research study, we used two urdu datasets UCSA-21(Our Proposed) and UCSA 50 to validate our proposed model. The proposed UCSA-21 dataset contains 9,312 Urdu reviews belonging to various genres such as food and recipes, movies, dramas, TV talk shows, politics, software and gadgets, and sports gathered from different social media websites. Each review in UCSA-21 belongs to one of three classes: neutral represented by 0, positive symbolized by 1, and negative reviews represented by 2. Tertiary classifications have experimented on the proposed corpus. The UCSA corpus compromises with total 9601 positive and negative user comments, contains 4843 positive and 4758 negative reviews. Tables 3 and 4 summarized the details of the used datasets in experiments.
Pre-processing
The primary goal of pre-processing is to prepare input text for subsequent tasks using various steps such as spelling correction, Urdu text cleaning, tokenization, Urdu word segmentation, normalization of Urdu text, and stop word removal. Tokenization is the process of separating each Uni-gram from sentences. The text is tokenized based on punctuation marks and white spaces. Stop words are vital words of any dialect and have no means in the context of sentiment classifications. They all are removed from the corpus to minimize corpus size. Segmentation is the method to find the boundaries among Urdu words. Due to the morphological structure of the Urdu language, the space between words does not specify a word boundary. Therefore, determining word boundaries in Urdu is essential 41 . Space-omission and Space-insertion are two main issues are linked with Urdu word segmentation. An example of a space omission among two words such as “Alamgeir”, universal and similarly space insertion in a single word such as “Khoub Sorat”, beautiful. In Urdu dialect, many words contain more than one string, such as “Khosh bash,” which means happiness is a Uni-gram with two strings. If during typing, that space between two strings is somehow omitted, then it will become “Khoshbash,” which is wrong syntactically and semantically either.The normalization part can be applied to fix the problem of correct encodings for the Arabic and Urdu characters with appropriate characters. Normalization brings each character in the designated uni-code array (0600-06FF) for the Urdu dialect.
Features extraction
Text is often indicated as a vector of weighted features in NLP tasks such as text classification. Different n -gram models are utilized in this study; these are models that assign probability to a series of words.A unigram is a model that has a series of one word, such as “Natural”; similarly, a bigram is a sequence of two words, such as “Natural Language,” and a trigram model is a sequence of three words, such as “Natural Language Processing.” On our dataset, we looked at n -gram features like unigram, bigram, trigram and variouse combination of these n -gram features. Additionally, we also investigate various character gram feattures to gain best results. Recently, pre-trained word embeddings approaches 61 have experimented with several NLP-related tasks, outperforming the existing systems. The main idea behind these word embedding models is to train them on large amounts of text data and fine-tune them for specific applications. The Wikipedia and Common Crawl (CC) data were used to train the fastText word embedding model. Wikipedia is the biggest free online data source, written in more than 200 dialects. After downloading and cleaning data, the model was trained. CC is a non-profit organization, which crawls web data and makes data freely available. fastText has been trained to understand more than 150 dialects, including Urdu. This is why we choose to use the fastText word vector model in our proposed research. fastText word to vector model was trained using Skipgram 61 and extension of Continuous Bag of Words (CBOW) methods 61 . In the Skipgram method, word representations are extended with character n-grams. A vector is associated with all n-gram characters, and vectors associated with words are obtained by adding the n-gram characters in the word. Similarly, the CBOW method denotes words as bags of character n-gram.
Classification techniques
This section explains the details of the proposed set of machine learning, rule-based, a set of deep learning algorithms and proposed mBERT model. The set of machine learning algorithms such as KNN, RF, NB, LR, MLP, SVM, and AdaBoost are used to classify Urdu reviews. Additionally, some deep learning algorithms such as CNN, LSTM, Bi-LSTM, GRU and Bi-GRU with fastText embeddings were also implemented. Figure 2 explains the abstract-level framework from data collection to classification.
The rule-based approach
Pure Urdu lexicon list containing 4728 negative and 2607 positive opinion words are publicly available. Figure 3 explains the algorithm of this approach in detail. Initially, each sentence is tokenized, and then each token is classified into one of three classes by comparing it to the available opinion words in the Urdu lexicon. The accessible Urdu lexicon and the words are used to determine the overall sentiment of the user review. If the text contains more positive tokens, the review is categorized as positive with a polarity score of 1. A review is characterized as negative with a polarity score of 2 if it contains more negative tokens (words) than positive tokens (words). Finally, a review is defined as neutral with a polarity score of 0 if it contains the same number of negative and positive words.
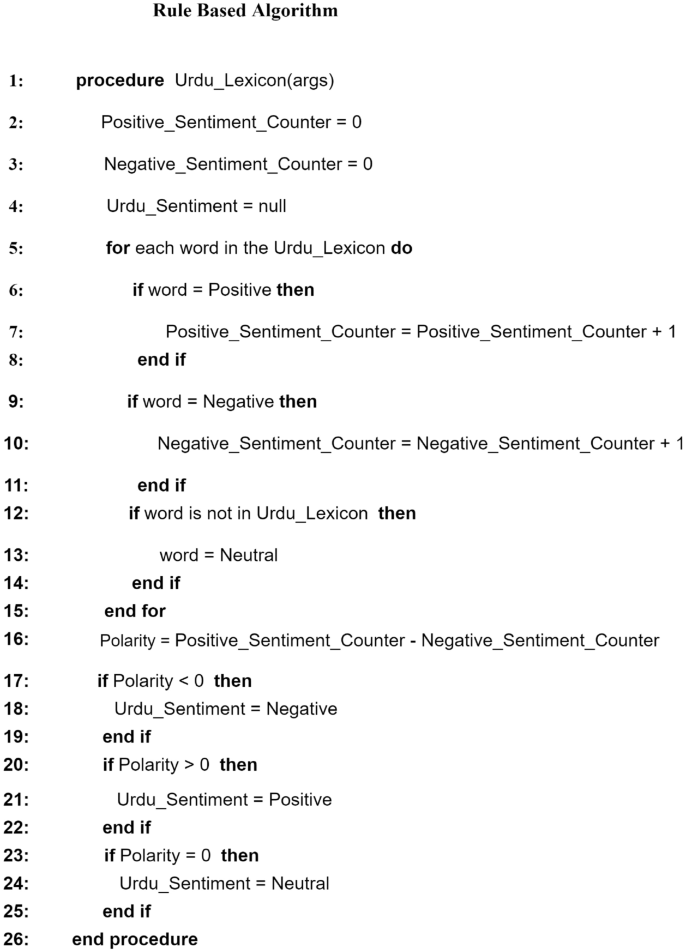
Rule-based Urdu sentiment analysis algorithm using Urdu Lexicon.
Deep learning models
The deep learning methods such CNN-1D, LSTM, GRU, BI-GRU, Bi-LSTM and mBERT model with word embedding model (fastText) were implemented using keras neural network library 4 for Urdu sentiment analysis to validate our proposed corpus. The technical and experimental information of deep learning algorithms are presented in this section. CNN-1D is mostly utilized in computer vision, but it also excels at classification problems in the natural language processing field. A CNN-1D is particularly capable If you intend to obtain new attributes from brief fixed-length chunks of the entire data set and the position of the feature is irrelevant 62 , 63 .
Study 64 introduced GRU to overcome the shortcomings of recurrent neural networks, such as resolving the vanishing gradient problem using update and reset gate mechanisms.Both update and reset gates are essentially vectors that govern what information should be transmitted to the output unit. The most exciting aspect of GRU is that it can be properly trained to keep information for an extended period of time without losing track of timestamps. A sequence processing model with two GRUs is known as Bi-GRU. One takes information in a forward direction, whereas the other takes it backwards. Only the input and forget gates are present in this bidirectional recurrent neural network.
LSTM 65 is a recurrent neural network design that displays state-of-the-art sequential data findings. LSTM is a technique for capturing long-term dependencies between text data. The LSTM model acquires the current word’s input for each time step, and the prior or last word’s output creates an output, which is utilized to feed to the next state. The prior state’s hidden layer (and, in some cases, all hidden layers) is then used for classification.We use Bi-LSTM model to classify each comment according to its class. Generally, Bi-LSTM used to capture more contextual information from both previous and future time sequences. In this study we used two-layer (Forward and Backward) Bi-LSTM, which obtain word embeddings from FastText.
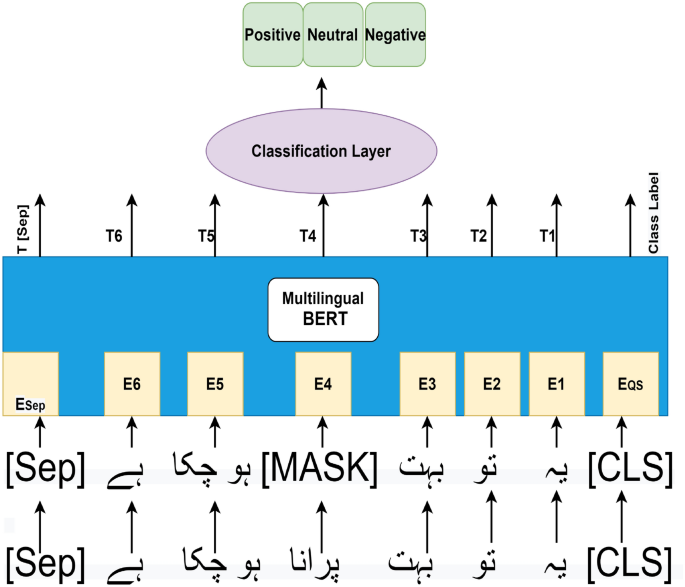
Multilingual BERT high level architecture for Urdu sentiment analysis.
mBERT: BERT 66 is one of the most widely used current language modeling architectures. Its generalization capabilities allows it to be modified to a variety of downstream tasks based on the demands of the user, whether it’s NER or relation extraction, question answering, or sentiment analysis. Figure 4 shows high level architecture of our Proposed model based on Multilingual BERT 67 . We fine-tune the latest multilingual (mBERT) model for Urdu sentiment recognition using supervised training data. The model mBERT developed based on single language base BERT 66 , which consists of 12 transformer layers and 768 hidden layers. The top 104 languages including Urdu with the largest Wikipedias were used to train the mBERT model. The training data for every dialect was gathered from a complete Wikipedia dump (except user and talk pages).
Transformers: The BERT small or base has 12 transformer layers, whereas the BERT large has 24 transformer layers. The Transformer is a natural language processing paradigm that aims to do sequence-to-sequence activities with long-range dependencies. The transformers made up with encoders and decoders. Furthermore, an encoder is made up of two pieces. Multi-Head Attention is the first part, while Feed Forward Neural Network is the second part. Masked Multi-Head Attention with Multi-Head Attention Feed Forward Neural Network is also included in Decoder. Encoders and decoders are implemented as stacked on top of each other.
Attention: The Transformer relies heavily on attention. Transformers’ self-attention obtains context comprehension of a word in the text based on neighboring words in the sentence. Attention uses Eq. ( 1 ) to determine the context of every word.
where Q, K, and V are abstract vectors that extract various components from an input word. The special classification token <CLS> in our proposed mBERT model captures the entire sentence, e.g., “Ye tou......” into a fixed-dimensional pooling representation and which produced an output vector with the equal size as the hidden size and the transformers’ output then fed into the fully-connected classification layer, which is the first token’s ultimate hidden state, whereas the special classification token <SEP> indicates the end of this particular sentence, as illustrated in Fig. 4 . The second stage is to replace 15% of tokens in each sentence with a [MASK] token (for example, the word ’Porana’ is substituted with a [MASK] token). The context of non-masked tokens is then used by the mBERT model to infer the original values of masked tokens. The encoders assign a unique representation to each token. For instance, the E1 is the fixed presenter of the sentence’s first word, “ye”. The model is made up of many levels, each of which performs multi-headed attention on the output of the preceding layer, for example, mBERT has 12 layers. T1 is the last representation of the first token or word of every sentence in Fig. 4 . The classification layer or softmax layer that has been added here. The classification layer has a dimension of K x H, where K is the number of classes (Positive, negative and neutral) and H is the size of the hidden state.
Model Training and Fine-Tuning: The entire sentiment classification mBERT model has been trained in two phases, with the first phase involving the pre-training of the mBERT language model and the second phase involving the fine-tuning of the outmost classification layer.The Urdu mBERT has been pre-trained on the Urdu Wikipedia. The mBERT model has been fine-tuned using the training set of the proposed and UCSA datasets, which are Comprised with labelled user reviews. Especially, the fully connected classification layer has been trained in this way. During training, categorical cross-entropy was utilized as the loss function. Table 5 presents lists the hyper-parameters adopted for this research.
Evaluation measures
In this study, Urdu sentiment analysis text classification experiments have been performed to evaluate our proposed dataset by using a set of machine learning, rule-based and deep learning algorithms. As a baseline algorithm for better assessment, we performed tertiary classifications experiment with 9312 reviews from our suggested UCSA-21 dataset. We depict four evaluation measures applied for evaluations of a bunch of machine learning, rule-based, and deep learning algorithms such as accuracy, precision, recall, and F1-measure.
where TN, TP, FN, and FP represent number of True Negative, True Positive, False Negative and False Positive respectively.
Results analysis
This section explains the results of various experiments that have been executed in this study, the usefulness of our proposed architecture for Urdu SA, and the discussion of revealed results. In the evaluation of various implemented machine learning, deep learning, and rule-based algorithms, it is observed that the mBERT algorithm perform better than all other models.
Tables 6 and 7 presents the obtained results using various machine learning techniques with different features on our proposed UCSA-21 corpus. The results reveal that SVM performance is slightly better on the UCSA-21 dataset than other machine learning algorithms, with an accuracy of 72.71% using combination (1-2) features. The gained results clearly show that all the machine learning classifiers perform better with word feature combination (1-2) and unigram. On the other hand, obtained results indicating that the set of machine learning algorithms performance is not satisfiable with trigram and bigram word feature. RF gain 55.00 % accuracy using trigram features had the lowest accuracy of all machine learning classifiers. When compared to bigram and trigram word features, all machine learning classifiers perform better using unigram word features which is consistent with 50 .The outcomes of several machine learning methods using character gram features are represented in Table 7 . Using the Char-3-gram feature, the findings demonstrated that NB and SVM outperformed all other machine learning classifiers with an accuracy of 68.29% and 67.50% respectively. on the other hand, LR had the poorest performance, with an accuracy of 58.40% when employing the char-5-gram feature.
Table 8 presents the baseline results achieved using a rule-based approach to validate our proposed UCSA-21 dataset. The rule-based approach achieved an accuracy (64.20%), precision (60.50%), recall (68.09%), and F1 score (64.07. It is observed that the rule-based technique didn’t achieve high scores in terms of accuracy as compared to machine learning and deep learning approaches. The lousy performance of the rule-based approach in this experiment is mere because of not considering the semantic information during the experiment; the experiment is only based on the terms in the lexicons database. One of the biggest flaws with rule-based algorithms is that it cannot distinguish humorous reviews with more positive words.The satirical reviews such as “MashaAllah se koy to rank milli ha na hamari cricket team ko. . . akhiri he sahi” translated as “By the grace of God, our cricket team got at least some rank. may that be last)” is a negative review which is wrongly classified as a positive review by rule-based approach.
Finally, this section contains the baseline results generated using many deep learning algorithms such as CNN-1D, LSTM,GRU, Bi-GRU, Bi-LSTM and our proposed model based on mBERT model. According to the results presented in Table 9 , deep learning models outperforms machine learning and rule-based approach. The obtained results reveal that our proposed model fine-tuned based on mBERT with SoftMax supersedes all other deep learning models with accuracy, precision, recall, and F1 score of 77.61%, 76.15%, 78.25%, and 77.18% respectively. It is Observed that Bi-LSTM and Bi-GRU can be effective for Urdu sentiment analysis compared to other traditional machine learning, rule-based, and deep learning algorithms merely because Bi-LSTM and Bi-GRU can capture information from backward and forward ways. Bi-LSTM produces slightly better results because it understands context better than LSTM and CNN-1D. It is also observed that LSTM and CNN-1D achieves slightly better results with Attention (ATT)layer as compared Max-polling (MP) layer.
Using the UCSA corpus, Table 10 compares the results of our proposed mBERT model with those of other commonly used deep learning algorithms. The obtained results shows that mBERT with SoftMax outperform all other deep learning algorithms with accuracy, precision, recall, and F1 score of 82.50%, 81.35%, 81.65%, and 81.49% respectively.We did not apply traditional machine learning algorithms to validate UCSA corpus because in study 50 authors already set baseline results. The findings shows that deep learning and our proposed model comparatively perform better by using UCSA corpus, due to less number of classification classes. As mentioned above the UCSA corpus compromises with only two classes: Positive and Negative on the other hand our proposed UCSA-21 corpus comprises with additional neutral class. After evaluating the data, achieving highest performance on both datasets shows the effectiveness of our proposed model for Urdu sentiment analysis (Fig. 5 ).
The confusion matrix is a measure for assessing the validity of a classification. Figure 6 present the confusion matrix of our proposed mBERT by using UCSA-21 Urdu corpus. In Fig. 6 , 78.10% of positive sentences are correctly classified as positive, while only 11.90% of positive reviews are incorrectly classified as negative, and 10.00% as neutral. Out of all reviews 78.40% of negative reviews are correctly identified as negative, while only 11.40% and 10.20% of negative reviews are incorrectly classified as neutral and positive respectively. Only 12.00% and 11.65% of neutral reviews are misclassified as negative and positive respectively, while 76.35 % of neutral reviews are accurately classified by our proposed model against UCSA-21 corpus. Similarly, Fig. 7 represents the confusion matrix of our proposed mBERT model using UCSA corpus which has only two classes: positive and Negative.
Machine learning models, on average, contain less trainable parameters than deep neural networks, which explains why they train so quickly. Instead than employing semantic information, these classifiers define class boundaries based on the discriminative power of words in relation to their classes. Furthermore, SVM performs pretty well among all adopted machine learning approaches because it not only handles outliers significantly better than other machine learning algorithms by deriving maximum margin hyperplanes, However, it also supports the kernel technique, which allows for effective tuning of a number of hyper-parameters to reach optimal performance. In addition, SVM employs Hinge loss, which outperforms LR’s log loss. Similarly, SVM’s capacity to capture feature interactions to some extent makes it superior to NB, which typically treats features independently.
On the other hand, deep learning algorithms, not only automate the feature engineering process, but they are also significantly more capable of extracting hidden patterns than machine learning classifiers. Due to a lack of training data, machine learning approaches are invariably less successful than deep learning algorithms. This is exactly the situation with the hand-on Urdu sentiment analysis assignment, where proposed and customized deep learning approaches significantly outperform machine learning methodologies. Bi-LSTM and Bi-Gru are the adaptable deep learning approach that can capture information in both backward and forward directions. The proposed mBERT used BERT word vector representation which is highly effectiv for NLP tasks. Eventually this approach which is based on transformers and encoder-decoder based technology beats other deep learning, machine learning and rule-based models. Figure 5 compare the overall accuracy of three various approaches and with proposed model used for Urdu sentiment analysis. The results reveals that the proposed mBERT model beats the deep learning, machine learning and rule-based algorithms.
As previously said, the Urdu language has a morphological structure that is highly unique, exceedingly rich, and complex when compared to other resource-rich languages. Urdu is a blend of several languages, including Hindi, Arabic, Turkish, Persian, and Sanskrit, and contains loan words from these languages. These are the most common causes of algorithm misclassifications. Other reasons for incorrect classifications include the fact that the normalization of Urdu text is not yet perfect. To tokenize Urdu text, spaces between words must be removed/inserted because the boundary between words is not visibly apparent. Similarly, in an Urdu sentence, the order of words can be changed but the sense/meaning stays the same, as in “Meeithay aam hain” and “Aam meeithay hain,” both of which have the same meaning “Mangos are sweet”. Manual annotation of user reviews also one of the reasons for miss classification.
The primary purpose for using a set of machine learning algorithms with word and character n-gram features to establish baseline results against our proposed Urdu corpus. Our proposed dataset comprises with short and long type of user reviews that’s why we used various deep learning algroithms such GRU and LSTM to investigate the performance of algroithms against Urdu text. GRU is typically used to categorize short sentences, whereas LSTM is thought to perform better versus long sentences because to its core structure. Similarly, BERT is currently one of the highest performing models for unsupervised pre-training. To address the Masked Language Modelling objective, this model is based on the Transformer architecture and trained on a huge amount of unlabeled texts from Wikipedia. It shows outstanding performance on a variety of NLP tasks. Motivation using mBERT is to investigate its performance against resource deprived languages such as Urdu.
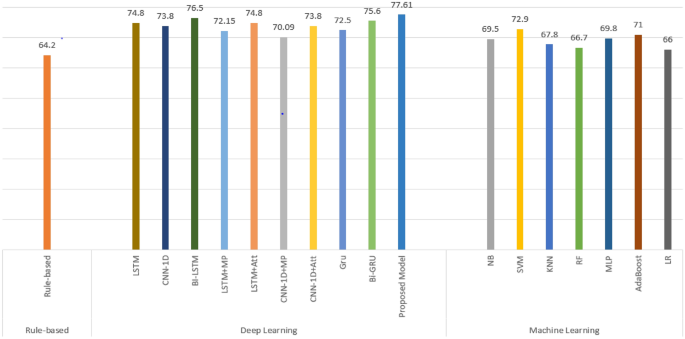
Accuracy Comparison of Machine, Deep Learning and Rule-Based Approaches with Proposed Model using UCSA-21 Corpus.
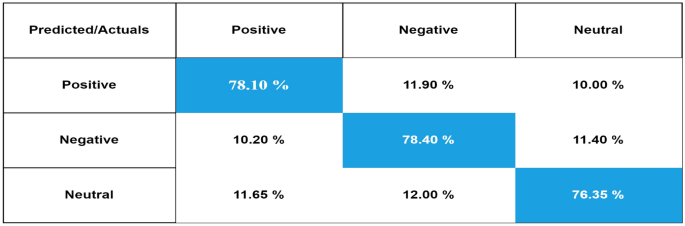
Confusion matrix of our proposed model using our proposed UCSA-21 corpus.
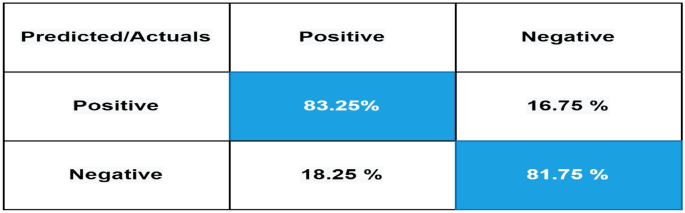
Confusion matrix of our proposed model using UCSA corpus.
As previously stated, there is a paucity of research on using deep learning approaches to analyze Urdu sentiment. Only a few studies have been published in this field, and they all used various machine learning classifiers on a small dataset with limited domains and have only positive and negative classes. On the other hand, our dataset, contains more user reviews than earlier studies, and it includes several genres with three classifications classes: positive, negative, and neutral. Table 1 shows a summery and comparison of our research with previous research.
Conclusion and implications
A huge amount of data has been generated on social media platforms, which contains crucial information for various applications. As a result, sentiment analysis is critical for analyzing public perceptions of any product or service. We observed that in the Urdu language, majority of studies focused on language processing tasks, with only a few experiments done in the domain of Urdu sentiment analysis utilizing several classical machine learning methodologies relatively with a small data corpus with only two data classes. In contrast, we proposed a multi-class Urdu sentiment analysis dataset and used various machine and deep learning algorithms to create baseline results. Additionally, our proposed mBERT classifier, achieves F1 score of 81.49% and 77.18% using UCSA and UCSA-21 datasets respectively.
This paper lays the path for more deep learning research into constructing language-independent models for languages with limited resources. Our findings reveal an essential insight: deep learning with pre-trained word embedding is a viable strategy for dealing with complicated and resource-poor languages like Urdu. In future, our plan is to use models such as GPT, GPT2 and GPT3 to improve the results. We believe that our publicly available dataset will serve as a baseline for sentiment analysis in Urdu.
Liu, Y. et al. Identifying social roles using heterogeneous features in online social networks. J. Assoc. Inf. Sci. Technol. 70 , 660–674 (2019).
Google Scholar
Lytos, A., Lagkas, T., Sarigiannidis, P. & Bontcheva, K. The evolution of argumentation mining: From models to social media and emerging tools. Inf. Process. Manage. 56 , 102055 (2019).
Vuong, T., Saastamoinen, M., Jacucci, G. & Ruotsalo, T. Understanding user behavior in naturalistic information search tasks. J. Assoc. Inf. Sci. Technol. 70 , 1248–1261 (2019).
CAS Google Scholar
Amjad, A., Khan, L. & Chang, H.-T. Effect on speech emotion classification of a feature selection approach using a convolutional neural network. PeerJ Comput. Sci. 7 , e766 (2021).
PubMed PubMed Central Google Scholar
Amjad, A., Khan, L. & Chang, H.-T. Semi-natural and spontaneous speech recognition using deep neural networks with hybrid features unification. Processes 9 , 2286 (2021).
Al-Smadi, M., Al-Ayyoub, M., Jararweh, Y. & Qawasmeh, O. Enhancing aspect-based sentiment analysis of Arabic hotels’ reviews using morphological, syntactic and semantic features. Inf. Process. Manage. 56 , 308–319 (2019).
Hassan, S.-U., Safder, I., Akram, A. & Kamiran, F. A novel machine-learning approach to measuring scientific knowledge flows using citation context analysis. Scientometrics 116 , 973–996 (2018).
Ashraf, M. et al. A study on usability awareness in local it industry. Int. J. Adv. Comput. Sci. Appl 9 , 427–432 (2018).
Shardlow, M. et al. Identification of research hypotheses and new knowledge from scientific literature. BMC Med. Inform. Decis. Mak. 18 , 1–13 (2018).
Thompson, P., Nawaz, R., McNaught, J. & Ananiadou, S. Enriching news events with meta-knowledge information. Lang. Resour. Eval. 51 , 409–438 (2017).
Mateen, A., Khalid, A., Khan, L., Majeed, S. & Akhtar, T. Vigorous algorithms to control urban vehicle traffic. In 2016 IEEE/ACIS 15th International Conference on Computer and Information Science (ICIS) , 1–5 (IEEE, 2016).
Bashir, F., Ashraf, N., Yaqoob, A., Rafiq, A. & Mustafa, R. U. Human aggressiveness and reactions towards uncertain decisions. Int. J. Adv. Appl. Sci. 6 , 112–116 (2019).
Mustafa, R. U. et al. A multiclass depression detection in social media based on sentiment analysis. In Latifi, S. (ed.) 17th International Conference on Information Technology–New Generations (ITNG 2020) , 659–662 (Springer International Publishing, Cham, 2020).
Ameer, I., Ashraf, N., Sidorov, G. & Gómez Adorno, H. Multi-label emotion classification using content-based features in Twitter. Comput. Sist. 24 , 25 (2020).
Ashraf, N. et al. Youtube based religious hate speech and extremism detection dataset with machine learning baselines. J. Intell. Fuzzy Syst. 20:1–9.
Sailunaz, K. & Alhajj, R. Emotion and sentiment analysis from twitter text. J. Comput. Sci. 36 , 101003 (2019).
Khan, Z., Iltaf, N., Afzal, H. & Abbas, H. Enriching non-negative matrix factorization with contextual embeddings for recommender systems. Neurocomputing 380 , 246–258 (2020).
Devi, B. & Pattabiraman, V. Soft cosine gradient and gaussian mixture joint probability recommender system for online social networks. Int. J. Intell. Eng. Syst. 13 , 301311 (2020).
Zhang, B. et al. Sentiment analysis through critic learning for optimizing convolutional neural networks with rules. Neurocomputing 356 , 21–30 (2019).
Luo, Z., Huang, S. & Zhu, K. Q. Knowledge empowered prominent aspect extraction from product reviews. Inf. Process. Manage. 56 , 408–423 (2019).
Araque, O., Zhu, G. & Iglesias, C. A. A semantic similarity-based perspective of affect lexicons for sentiment analysis. Knowl.-Based Syst. 165 , 346–359 (2019).
Safder, I. & Hassan, S.-U. Bibliometric-enhanced information retrieval: A novel deep feature engineering approach for algorithm searching from full-text publications. Scientometrics 119 , 257–277 (2019).
Al-Ayyoub, M., Khamaiseh, A. A., Jararweh, Y. & Al-Kabi, M. N. A comprehensive survey of Arabic sentiment analysis. Inf. Process. Manage. 56 , 320–342 (2019).
Asghar, M. Z. et al. Creating sentiment lexicon for sentiment analysis in Urdu: The case of a resource-poor language. Expert Syst. 36 , e12397 (2019).
Masroor, H., Saeed, M., Feroz, M., Ahsan, K. & Islam, K. Transtech: Development of a novel translator for roman Urdu to English. Heliyon 5 , e01780 (2019).
Ombabi, A. H., Ouarda, W. & Alimi, A. M. Deep learning CNN-LSTM framework for Arabic sentiment analysis using textual information shared in social networks. Soc. Netw. Anal. Min. 10 , 1–13 (2020).
Ashraf, N., Mustafa, R., Sidorov, G. & Gelbukh, A. Individual vs. group violent threats classification in online discussions. In Companion Proceedings of the Web Conference 2020 , WWW ’20, 629–633 (Association for Computing Machinery, New York, NY, USA, 2020).
Ashraf, N., Zubiaga, A. & Gelbukh, A. Abusive language detection in youtube comments leveraging replies as conversational context. PeerJ Comput. Sci. 7 , e742 (2021).
Amjad, M., Ashraf, N., Zhila, A., Sidorov, G, & Zubiaga, A. Threatening language detection and target identification in Urdu tweets. IEEE Access . https://doi.org/10.1109/ACCESS.2021.3112500 (2021).
Article Google Scholar
Ashraf, N., Butt, S., Sidorov, G. & Gelbukh, A. CIC at CheckThat! 2021: Fake news detection using machine learning and data augmentation. In CLEF 2021—Conference and Labs of the Evaluation Forum (Bucharest, Romania, 2021).
Kiritchenko, S., Mohammad, S. & Salameh, M. Semeval-2016 task 7: Determining sentiment intensity of English and Arabic phrases. In Proceedings of the 10th international workshop on semantic evaluation (SEMEVAL-2016) , 42–51 (2016).
Fernández, J., Gutiérrez, Y., Gómez, J. M. & Martinez-Barco, P. Gplsi: Supervised sentiment analysis in twitter using skipgrams. In Proceedings of the 8th International Workshop on Semantic Evaluation (SemEval 2014) , 294–299 (2014).
Jang, H., Kim, M. & Shin, H. Kosac: A full-fledged Korean sentiment analysis corpus. In Proceedings of the 27th Pacific Asia Conference on Language, Information, and Computation (PACLIC 27) , 366–373 (2013).
Wicaksono, A. F., Vania, C., Distiawan, B. & Adriani, M. Automatically building a corpus for sentiment analysis on Indonesian tweets. In Proceedings of the 28th Pacific Asia Conference on Language, Information and Computing , 185–194 (2014).
Mahmood, Z. et al. Deep sentiments in roman Urdu text using recurrent convolutional neural network model. Inf. Process. Manage. 57 , 102233 (2020).
Ayata, D., Saraclar, M. & Özgür, A. Busem at semeval-2017 task 4a sentiment analysis with word embedding and long short term memory rnn approaches. In Proceedings of the 11th International Workshop on Semantic Evaluation (SemEval-2017) , 777–783 (2017).
Mittal, N., Agarwal, B., Chouhan, G., Bania, N. & Pareek, P. Sentiment analysis of Hindi reviews based on negation and discourse relation. In Proceedings of the 11th Workshop on Asian Language Resources , 45–50 (2013).
Tuarob, S. & Mitrpanont, J. L. Automatic discovery of abusive Thai language usages in social networks. In International Conference on Asian Digital Libraries , 267–278 (Springer, 2017).
Al-Amin, M., Islam, M. S. & Uzzal, S. D. Sentiment analysis of Bengali comments with word2vec and sentiment information of words. In 2017 International Conference on Electrical, Computer and Communication Engineering (ECCE) , 186–190 (IEEE, 2017).
Ijaz, M. & Hussain, S. Corpus based Urdu lexicon development. In the Proceedings of Conference on Language Technology (CLT07), University of Peshawar, Pakistan , vol. 73 (2007).
Syed, A. Z., Aslam, M. & Martinez-Enriquez, A. M. Associating targets with sentiunits: A step forward in sentiment analysis of Urdu text. Artif. Intell. Rev. 41 , 535–561 (2014).
Mukund, S., Srihari, R. & Peterson, E. An information-extraction system for Urdu—a resource-poor language. ACM Trans. Asian Lang. Inf. Process. 9 , 1–43 (2010).
Mukhtar, N. & Khan, M. A. Urdu sentiment analysis using supervised machine learning approach. Int. J. Pattern Recognit. Artif. Intell. 32 , 1851001 (2018).
MathSciNet Google Scholar
Ali, A. R. & Ijaz, M. Urdu text classification. In Proceedings of the 7th International Conference on Frontiers of Information Technology , 1–7 (2009).
Abid, M., Habib, A., Ashraf, J. & Shahid, A. Urdu word sense disambiguation using machine learning approach. Cluster Comput. 21 , 515–522 (2018).
Akhter, M. P., Jiangbin, Z., Naqvi, I. R., Abdelmajeed, M. & Fayyaz, M. Exploring deep learning approaches for Urdu text classification in product manufacturing. Enterprise Inf. Syst. 20 , 1–26 (2020).
Nasim, Z. & Ghani, S. Sentiment analysis on Urdu tweets using Markov chains. SN Comput. Sci. 1 , 1–13 (2020).
Asim, M. N. et al. Benchmarking performance of machine and deep learning-based methodologies for Urdu text document classification. Neural Comput. Appl. 33 , 5437–5469 (2021).
Naqvi, U., Majid, A. & Abbas, S. A. Utsa: Urdu text sentiment analysis using deep learning methods. IEEE Access (2021).
Khan, L., Amjad, A., Ashraf, N., Chang, H.-T. & Gelbukh, A. Urdu sentiment analysis with deep learning methods. IEEE Access (2021).
Xu, D. et al. Deep learning based emotion analysis of microblog texts. Inf. Fusion 64 , 1–11 (2020).
Tian, Z. et al. User and entity behavior analysis under urban big data. ACM Trans. Data Sci. 1 , 1–19 (2020).
Qiu, J., Chai, Y., Tian, Z., Du, X. & Guizani, M. Automatic concept extraction based on semantic graphs from big data in smart city. IEEE Trans. Comput. Soc. Syst. 7 , 225–233 (2019).
Hashim, F. & Khan, M. Sentence Level Sentiment Analysis Using Urdu Nouns 101–108 (Department of Computer Science, University of Peshawar, 2016).
Do, H. H., Prasad, P., Maag, A. & Alsadoon, A. Deep learning for aspect-based sentiment analysis: A comparative review. Expert Syst. Appl. 118 , 272–299 (2019).
Abdul-Mageed, M. & Diab, M. T. Awatif: A multi-genre corpus for modern standard Arabic subjectivity and sentiment analysis. LREC 515 , 3907–3914 (2012).
Maynard, D. & Bontcheva, K. Challenges of evaluating sentiment analysis tools on social media. In Proceedings of the Tenth International Conference on Language Resources and Evaluation (LREC 2016) , 1142–1148 (LREC, 2016).
Ganapathibhotla, M. & Liu, B. Mining opinions in comparative sentences. In Proceedings of the 22nd International Conference on Computational Linguistics (Coling 2008) , 241–248 (2008).
Mehmood, K., Essam, D., Shafi, K. & Malik, M. K. Sentiment analysis for a resource poor language-roman Urdu. ACM Trans. Asian Low-Resour. Lang. Inf. Process. 19 , 1–15 (2019).
Sorgente, A., Vettigli, G. & Mele, F. An italian corpus for aspect based sentiment analysis of movie reviews, 349–353 (2014).
Bojanowski, P., Grave, E., Joulin, A. & Mikolov, T. Enriching word vectors with subword information. Trans. Assoc. Comput. Linguist. 5 , 135–146 (2017).
Kalchbrenner, N., Grefenstette, E. & Blunsom, P. A convolutional neural network for modelling sentences. arXiv:1404.2188 (arXiv preprint) (2014).
Rakhlin, A. Convolutional neural networks for sentence classification. GitHub (2016).
Cho, K. et al. Learning phrase representations using rnn encoder-decoder for statistical machine translation. arXiv:1406.1078 (arXiv preprint) (2014).
Hochreiter, S. & Schmidhuber, J. Long short-term memory. Neural Comput. 9 , 1735–1780 (1997).
CAS PubMed Google Scholar
Devlin, J., Chang, M.-W., Lee, K. & Toutanova, K. Bert: Pre-training of deep bidirectional transformers for language understanding. arXiv:1810.04805 (arXiv preprint) (2018).
Pires, T., Schlinger, E. & Garrette, D. How multilingual is multilingual bert? arXiv:1906.01502 (arXiv preprint) (2019).
Download references
Author information
Authors and affiliations.
Department of Computer Science and Information Engineering, Chang Gung University, Taoyuan, Taiwan
Lal Khan, Ammar Amjad & Hsien-Tsung Chang
CIC, Instituto Politécnico Nacional, Mexico City, Mexico
Noman Ashraf
Department of Physical Medicine and Rehabilitation, Chang Gung Memorial Hospital, Taoyuan, Taiwan
- Hsien-Tsung Chang
Artificial Intelligence Research Center, Chang Gung University, Taoyuan, Taiwan
Bachelor Program in Artificial Intelligence, Chang Gung University, Taoyuan, Taiwan
You can also search for this author in PubMed Google Scholar
Contributions
L.K. draft the main manuscript text. H.-T.C. set the experimental strategies. L.K., A.A., N.A. d****esigned and applied the experiments. All authors reviewed the manuscript. H.-T.C. handled the process and paper publication issues.
Corresponding author
Correspondence to Hsien-Tsung Chang .
Ethics declarations
Competing interests.
The authors declare no competing interests.
Additional information
Publisher's note.
Springer Nature remains neutral with regard to jurisdictional claims in published maps and institutional affiliations.
Rights and permissions
Open Access This article is licensed under a Creative Commons Attribution 4.0 International License, which permits use, sharing, adaptation, distribution and reproduction in any medium or format, as long as you give appropriate credit to the original author(s) and the source, provide a link to the Creative Commons licence, and indicate if changes were made. The images or other third party material in this article are included in the article's Creative Commons licence, unless indicated otherwise in a credit line to the material. If material is not included in the article's Creative Commons licence and your intended use is not permitted by statutory regulation or exceeds the permitted use, you will need to obtain permission directly from the copyright holder. To view a copy of this licence, visit http://creativecommons.org/licenses/by/4.0/ .
Reprints and permissions
About this article
Cite this article.
Khan, L., Amjad, A., Ashraf, N. et al. Multi-class sentiment analysis of urdu text using multilingual BERT. Sci Rep 12 , 5436 (2022). https://doi.org/10.1038/s41598-022-09381-9
Download citation
Received : 16 September 2021
Accepted : 22 March 2022
Published : 31 March 2022
DOI : https://doi.org/10.1038/s41598-022-09381-9
Share this article
Anyone you share the following link with will be able to read this content:
Sorry, a shareable link is not currently available for this article.
Provided by the Springer Nature SharedIt content-sharing initiative
This article is cited by
Migraine headache (mh) classification using machine learning methods with data augmentation.
- Moudasra Shahreen
Scientific Reports (2024)
Unlocking travel narratives: a fusion of stacking ensemble deep learning and neural topic modeling for enhanced tourism comment analysis
- Nassera Habbat
- Hicham Nouri
Social Network Analysis and Mining (2024)
Correcting spelling mistakes in Persian texts with rules and deep learning methods
- Sa. Kasmaiee
- Si. Kasmaiee
- M. Homayounpour
Scientific Reports (2023)
- Urooba Sehar
- Summrina Kanwal
- Osama A. Khashan
Computationally efficient recognition of unconstrained handwritten Urdu script using BERT with vision transformers
- Aejaz Farooq Ganai
- Farida Khursheed
Neural Computing and Applications (2023)
By submitting a comment you agree to abide by our Terms and Community Guidelines . If you find something abusive or that does not comply with our terms or guidelines please flag it as inappropriate.
Quick links
- Explore articles by subject
- Guide to authors
- Editorial policies
Sign up for the Nature Briefing: AI and Robotics newsletter — what matters in AI and robotics research, free to your inbox weekly.

Vol. 5 No. 2 (2021): Al-Aijaz Research Journal of Islamic Studies & Humanities (April to June 2021)
Urdu-1 the role of women in the formation and stability of a just social system: a research study, urdu-2 the impact of private schools on the islamic thoughts of student’s at district shaheed banzirabad, urdu-3 role of the shariah academy in family laws, urdu-4 unilateral promise & its application in islamic banking and finance, urdu-5 the development of spouse in the light of holy prophet muhammad (pbuh)’s relation with sayyedah aisha (r.a), urdu-6 an analytical study on the muslim youth in the poetry of allama muhammad iqbal in the light of quran and hadith, urdu-7 a critical study on the methodology of ibn abdulbar in his book al-istī’āb fī m’rifat al-asḥāb, urdu-8 prediction in the holy ghazwat of muhammadi arabi (s.a.w) and righteousness of his hadiths, urdu-9 comparative study of steps taken by muslims and western people about child rights in pakistan and on international level, urdu-10 music and its instruments an analytical study in the light of qura’nic verses, urdu-11 the main features of woman in hindustani society, urdu-12 persian elements in iftikhar arif’s religious poetry: an analysis, urdu-13 a critical analysis of the constitutions of pakistan's religious political parties, urdu-14 reasons for nonpayment of parental rights and their remedy research study in the light of quran and sunnah, urdu-15 the analytical study of the rights and duties of the widows of district charsadda in contemporary and islamic context, urdu-16 a scientific and research review of the different stages and procedures of jurisprudence in the history of complication and a scholarly research review of abdul rahman al-jazeera’s book al-fiqh ali al-madhahib al-arba’ah, urdu-17 the role of sufis of sahiwal in publishing islam and promoting urdu language and literature, urdu-18 moral teachings and values in divine and non-divine religions, urdu-19 inorate,literary style, manner and procedure of the commentary of "lame-ud-durari" (sharh-e-sahih bukhari), urdu-20 juma -tul- mubarak is an islamic festival, urdu-21 interrogative verses in the holy qur’an: an analytical study of style and purpose, urdu-22 problems and solutions in contemporary da'wah analytical review in the light of sirah-e-tayeba, urdu-23 critical perspective of meerza adeeb’s dramas on islamic historic personalities, urdu-24 an interpretation of the different stages and procedures of contracts in the era of ignorance and the duration of islam, urdu-25 a principles of amendment of the society in the teaching / light of the addresses of hazrat abu bakar siddique (r.a), urdu-26 a study of the book of mumtaz shireen, “manto: noori na nari” according islamic values, urdu-27 analytical study of arguments from quranic verses in ashiaat-ul-lamaat, urdu-28 an analysis of administrative matters of the state of madina in the light of the teachings of holy prophet (p.b.u.h), urdu-29 social and economical influences of tafaseer of sub-continent ulama, urdu-30 the method and style of tafsir ruhul bayan is permissible, arabic-1 the fatwa crisis at the present time, its causes and how to get out of it, an applied study, arabic-2 the rules of preference in context in tafseer moahib-ur-rehman of shiekh syed amir ali (elected models), arabic-3 the effect of the qur'anic context in resolving of conflict and contradiction between the qur'anic verses in the light of the rooh ul maani, arabic-4 authenticity of the exegeses of the holy quran by sunnah and its types, arabic-5 the impact of sectarian nervousness on the individual and society from the perspective of the holy quran, arabic-6 explaining the ascertainment of the philosophers and their words containing in the order of (al-tamyeez) by abdulaziz (from first to thirty), arabic-7 un-accepted chains of tafseer by hazrat abdullah bin abbas (r.a), arabic-8 metonymy examples in al-arbaeen al-navaviyah, arabic-9 the significans of semantics in the form of derived verbs in the holy quran, arabic-10 al-imam al-a'mash al-kufi-and some examples from his odd readings (qiraat shadhah) with its guidance from arabic language, arabic-11 breach of the knowledge through the distorition in the view of islamic shariah, arabic-12 methodology of abu zaid al-qarashi in “jamharahti ashaar il arab”, arabic-13 introductory review of books on synonyms to help in understanding the qur’ān تعارف الکتب حول المترادفات التي تساعد في فهم القرآن, english-1 the role of a teacher in the light of seerah is an essential element of personality building (the analytical study of the current scenario and the views of students of university of sufism and modern sciences, bhitshah, matiari), english-2 the socio-political & economic contribution of sufis in society; a case of district muzaffargarh, english-3 the portrayal of human rights in islam –multi-perspective disposition, english-4 learning religious education through practice: impact of co-curricular activities in teaching of religious studies at university level ., english-5 principles of electronic evidence in sharī‘ah and law-a comparative study, english-6 the concept of tortuous liability under islamic law: an inquiry into question of compensatory damages, english-7 the merger and acquisition financial performance analysis of conventional and islamic banks case of kasb and bank islami case of kasb and bank islami, english-8 religious minorities, their status in pakistan with reference to the teachings of islam and constitution of pakistan.

HEC Approved by "Y" Category

About the Journal
- Al-Aijaz Research Journal of Islamic Studies and Humanities
- ISSN (Print) : 2707-1200
- ISSN (Electronic) : 2707-1219
- DOI: https://doi.org/10.53575
- Frequency : Quarterly (4 issues per year)
- Nature : Print and Online
- Submission Email : [email protected]
- Languages of Publication: Arabic, English, Urdu
Current Issue
Make a submission, information.
- For Readers
- For Authors
- For Librarians
Developed By
- An account of the believers: In the light of Surah al-Furqan 61
- Article 62, 63 of Constitution of Pakistan and Moral Standards for Leadership in an Islamic State: An Analytical Study 13
- Maulana Obaidullah Sindhi's Theory of Ethics and the Formation of Society (In Modern Context) 12
- URDU-30 The Method and Style of Tafsir Ruhul Bayan is Permissible 10
- The Economic Conditions of Arabs in pre-Islamic Era 10
urdu ocr Recently Published Documents
Total documents.
- Latest Documents
- Most Cited Documents
- Contributed Authors
- Related Sources
- Related Keywords
A novel normal to tangent line (NTL) algorithm for scale invariant feature extraction for Urdu OCR
A comparative analysis on nastaliq style urdu character recognition.
Optical Character Recognition (OCR) has emerged as an interesting research field. Lot of work has been declared in Urdu script based on various approaches and diverse methodologies have been put forward on Nastaliq font style to get the desired output. The paper presents a survey on different techniques of OCR and ends up with the comparative analysis of Urdu character recognition based on accuracy and other performance parameters. This exploration directly implies that the nonpresence of Urdu OCR has restricted the idea on advanced Urdu library and thus, drives a pathway for enormous research in this field.
Comparative Analysis of Raw Images and Meta Feature based Urdu OCR using CNN and LSTM
Ligature analysis-based urdu ocr framework, impact of ligature coverage on training practical urdu ocr systems, projection profile based ligature segmentation of nastaleeq urdu ocr, character segmentation for nastaleeq urdu ocr: a review, offline urdu ocr using ligature based segmentation for nastaliq script, nastalique segmentation-based approach for urdu ocr, ligature segmentation for urdu ocr, export citation format, share document.
Submissions
Submission preparation checklist.
- The submission has not been previously published, nor is it before another journal for consideration (or an explanation has been provided in Comments to the Editor).
- The submission file is in OpenOffice, Microsoft Word, or RTF document file format.
- Where available, URLs for the references have been provided.
- The text is single-spaced; uses a 12-point font; employs italics, rather than underlining (except with URL addresses); and all illustrations, figures, and tables are placed within the text at the appropriate points, rather than at the end.
- The text adheres to the stylistic and bibliographic requirements outlined in the Author Guidelines.
Author Guidelines
- Manuscripts should not exceed 20 pages and must conform to the style of the publication manual of the American psychological Association (APA 6 th edition)
- An Abstract of 200 to 250 words should be submitted with the manuscript
- The name (s), affiliation (s) and phone, fax email address, permanent and postal address and a brief biography of the author (s) should appear on cover page.
- The authors are requested to check the article thoroughly, its spellings, grammar and illustration etc.
- Incomplete article will not be entertained.
- The research papers are accepted for publications on the understanding that they have not been published earlier.
- Research paper should be an original piece, including methodology, contents, data analysis and interpretation.
- Authors should be clearly specified as main/corresponding author, co-author and supporting author.
- Manuscripts will be reviewed by at least three consulting editors.
Formatting:
1-Margins: one inch on all sides expects left side with 1.5 inch.
2-Front size and Types: 12-pt Time New Roman.
3-Line spacing: Double space throughout the paper, including the title page, abstract, body of the document, references, appendixes, footnotes and tables.
4-Spacing after punctuations: space once after commas, colon and semicolons within sentences. Insert two spaces after full stop.
5-Paragraph: indentation 5-7 spaces
6- Pagination: The page numbers appears one inch from the right edge of the paper on the first line of every page.
7-Alignment: Flush left
8- Running head: The running head is the short title that appears at the top of the page or published article.
9- Referencing: Sources cited appear in parentheses after each reference giving author’s name, year of publication, and page number in the case of direct quotes. List all sources alphabetically at the End of the manuscript under the heading references using APA style.
10- Footnotes are not allowed, and the use of endnotes is discouraged
11- Notes: Citation in notes follows the same format.
11- Graphics: Mathematical symbols should be clearly marked. Numbers the tables and figure with Arabic numerals, Prepare tables using tabs without vertical lines; provide figures, charts, and diagrams in camera-ready form.
The soft copy of the article complete in all respect and all correspondence regarding contribution to the journal and other information should be email:
Section of Research Article:
Research articles should present innovative research that clearly addresses selected hypothesis. A research article should be divided into the following sections;
- Introduction
- Literature review
- Methodology
- Results and discussion
- Conclusions
- Acknowledgement
The contributors are advised to follow the publication manual APA 6 th edition for referencing. The accuracy and completeness of all the references are the responsibility of the author. A reference list should contain only those references that are cited in the text.
Tables, Figures and illustrations
The purpose of tables and figures is to present data to the reader in a clear and unambiguous manner. The author should not describe the data in the text. Tables should each be typed on a separate sheet and attached at the end of the manuscript.
Originality of Manuscript
Manuscripts are accepted for consideration with the understanding that they are original material and are not under consideration for publication elsewhere.
Review process:
After a preliminary editorial review, article will be sent to references that have expertise in the subject of the article. Author will be informed about comments of the referees to revise the article accordingly if required.
Copyright Notice
Plagiarism policy:
As per HEC policy, plagiarism will not be accepted in any journal. With the submission author is requested to declare the originality of his/her work.
Conflict of interest
Authors’ reviewers or editorial board have to disclose every situation which could potentially affect the impartial review and publication procedure.
Open access policy
It is an unrestricted online model that allows the distribution of research papers through the internet to everyone in the global community with no price restrain.
Privacy Statement
The names and email addresses entered in this journal site will be used exclusively for the stated purposes of this journal and will not be made available for any other purpose or to any other party.
Make a Submission
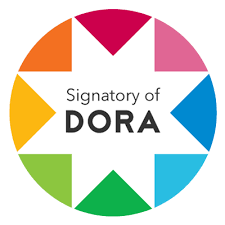
Our Journal ARMAGHAN is providing unrestricted access to knowledge and education for all and thereby follows OPEN ACCESS POLICY to showcase its content.
Article Publication Charges (APC):
The Women University Multan has approved the Article Publishing fee of Rs. 20000/- on the recommendation of F&PC and Syndicate. The valuable researchers are requested to submit their research papers online or via journal email. and submit publication fees after the expectance of the paper by depositing through a bank challan form or ATM.
The Researchers/ scholars/ authors may deposit the fee in the BANK OF PUNJAB (MDA Branch) MULTAN.
Account Title: THE WOMEN UNIVERSITY MULTAN ARMAGHAN
Account Number: 6580103434200208
Bank Name: Bank of Punjab, MDA Branch Multan.

An official website of the United States government
The .gov means it’s official. Federal government websites often end in .gov or .mil. Before sharing sensitive information, make sure you’re on a federal government site.
The site is secure. The https:// ensures that you are connecting to the official website and that any information you provide is encrypted and transmitted securely.
- Publications
- Account settings
Preview improvements coming to the PMC website in October 2024. Learn More or Try it out now .
- Advanced Search
- Journal List
- PeerJ Comput Sci

Recognition of Urdu sign language: a systematic review of the machine learning classification
1 Faculty of Engineering Science Technology and Management, Department of Biomedical Engineering and Department of Electrical Engineering, Ziauddin University, Karachi, Pakistan
Munaf Rashid
2 Faculty of Engineering Science Technology and Management, Department of Electrical Engineering and Department of Software Engineering, Ziauddin University, Karachi, Pakistan
Samreen Hussain
3 HESSA Project, US AID Program, Karachi, Pakistan
4 Faculty of Engineering Science Technology and Management, Electrical Engineering Department, Ziauddin University, Karachi, Pakistan
Sidra Abid Syed
5 Faculty of Engineering Science Technology and Management, Department of Biomedical Engineering, Ziauddin University, Karachi, Pakistan
Afshan Saad
6 Computer Science Department, Muhammad Ali Jinnah University, Karachi, Pakistan
Associated Data
The following information was supplied regarding data availability:
This is a literature review.
Background and Objective
Humans communicate with one another using language systems such as written words or body language (movements), hand motions, head gestures, facial expressions, lip motion, and many more. Comprehending sign language is just as crucial as learning a natural language. Sign language is the primary mode of communication for those who have a deaf or mute impairment or are disabled. Without a translator, people with auditory difficulties have difficulty speaking with other individuals. Studies in automatic recognition of sign language identification utilizing machine learning techniques have recently shown exceptional success and made significant progress. The primary objective of this research is to conduct a literature review on all the work completed on the recognition of Urdu Sign Language through machine learning classifiers to date.
Materials and methods
All the studies have been extracted from databases, i.e., PubMed, IEEE, Science Direct, and Google Scholar, using a structured set of keywords. Each study has gone through proper screening criteria, i.e. , exclusion and inclusion criteria. PRISMA guidelines have been followed and implemented adequately throughout this literature review.
This literature review comprised 20 research articles that fulfilled the eligibility requirements. Only those articles were chosen for additional full-text screening that follows eligibility requirements for peer-reviewed and research articles and studies issued in credible journals and conference proceedings until July 2021. After other screenings, only studies based on Urdu Sign language were included. The results of this screening are divided into two parts; (1) a summary of all the datasets available on Urdu Sign Language. (2) a summary of all the machine learning techniques for recognizing Urdu Sign Language.
Our research found that there is only one publicly-available USL sign-based dataset with pictures versus many character-, number-, or sentence-based publicly available datasets. It was also concluded that besides SVM and Neural Network, no unique classifier is used more than once. Additionally, no researcher opted for an unsupervised machine learning classifier for detection. To the best of our knowledge, this is the first literature review conducted on machine learning approaches applied to Urdu sign language.
Introduction
Everything in our world is imperfect, and there is no place for idealism, and many scientific data and figures demonstrate this. In the same way, humans are neither flawless nor ideal. Some people are born differently than others. We describe them as impaired since they are distinct, but in truth, they are unique and have particular requirements. It is estimated that over 72 million people worldwide ( World Federation of the Deaf, 2022 ) have hearing impairment difficulties, with approximately 10 million people in Pakistan ( Pakistan Association of the Deaf, 2022b ) being deaf, as per the International Federation of the Deaf. There is no all-encompassing international system that provides a comprehensive manner for deaf people to talk with one another worldwide. Since the beginning of time, visual communication has conveyed information. Generally, various new types of sign languages are being used worldwide. A sign language is a way of communication that, instead of using sonically transmissible sound patterns, uses visually transmis ( Disabled World, 2017 ) smoothly. To communicate effectively between the deaf community and the general public without paper and pencil, there are a variety of sign languages available in different countries ( U. S. Department of Justice, 2020 ), including American Sign Language, British Sign Language, Spanish Sign Language, and probably sign languages throughout every country. Even if you are not fluent in sign language, you have almost certainly come into contact with it, either through witnessing it in action or through using a translator at a seminar or a performance. There is still more sign language than strikes the eye, and several dialects other than American Sign Language (ASL) are used for sign language communication. It is estimated that around 60 sign languages are recognized and utilized worldwide ( Elakkiya, 2020 ). According to the National Institute on Deafness and Other Communication Disorders (NIDCD), ASL is “a complete and complex language that includes signals generated by moving the hands in conjunction with facial expressions and body postures”. It is more than just a translation of English into hand gestures; it has grammar and pronunciation norms and can handle varied ethnicities and accents ( American Sign Language, 2021 ).
Furthermore, there is a lot of reported in different languages like Chinese ( Jiang et al., 2020 ), American ( Zafrulla et al., 2011 ), or Indian ( Gupta & Kumar, 2021 ) that demonstrate that there is much work has been done on sign language recognition systems globally. The diversity of sign languages seen throughout the world indicates that local and regional language and culture are significant elements in the evolution of sign language, as is true of the development of any spoken language, regardless of its origin. However, many people have wondered why there isn’t a universal sign language for those who sign. This may be analogous to asking why there isn’t a universally accepted spoken language spoken all over the globe ( Elakkiya, 2020 ).
Individuals who are deaf in Pakistan communicate with one another through the Pakistani Sign Language (PSL). It is subject to the rules of linguistics, just like all other sign languages, and, like the spoken Urdu language, it has its grammar, letters and words, and gestures and complex sentences. It also has a distinct vocabulary of signs and a constantly evolving syntax, just like any other sign language system worldwide. PSL has matured into a full-fledged language due to its evolution over time. Many people speak Urdu in South Asia, and it is the official language of Pakistan. Nastaleeq and Naskh are the most popular Urdu writing systems. It is extensively used in old Urdu literature and newspapers to write in the Nastaleeq way. Many other ethnic languages, including Persian, Pashto, Punjabi, Baluchi, and Saraiki, also use the Nastaleeq writing style to write their texts too. Indo-European language Urdu has its roots in India. It is one of the most widely spoken languages on the Indian subcontinent. Urdu is one of India’s 23 official languages and one of Pakistan’s two. Also, Dubai has a large population of people who speak this language. A majority of the world’s population speaks it. This is a written form of Urdu derived from the Persian script, which is evolved from the Arabic script. Urdu is also written from right to left, like Arabic. As a practical medium of interaction for deaf people everywhere, sign languages have emerged as the backbone of individual Deaf cultures. Hearing people who cannot communicate verbally due to a disability or disorder like augmentative and alternative communication or have deaf family members, such as children of deaf adults, utilize signs in addition to those who are deaf or have hearing loss. Furthermore, a blind person can also be benefitted from this work through a text-image to speech technology. If a character in an image is automatically detected through a machine, then converting it in the sound can be life-support to blind people.
In contrast to Arabic and Persian, Urdu has more independent letters. Urdu has a more complex script than Arabic or Persian ( Hussain, Ali & Akram, 2015 ; Anwar, Wang & Wang, 2006 ). In Figs. 1A and and1B, 1B , Urdu sign language is represented and labeled with words and numbers.

Gesture recognition has found a significant usage in this field, allowing deaf and mute patients to interact with us more efficiently and effectively. A considerable time and effort have been made in sign recognition worldwide. However, in the case of Urdu Sign Language, no such work could be found. Nearly 0.2 million deaf and mute Pakistani citizens do not have access to assistive and rehabilitative technology. There have been two types of gestures: static gestures are those gestures that incorporate dynamic hand, body, and face motions. Static gestures are those that do not change. During static gestures, the noticeable gesture occurs within a specific period that the performer physically orchestrates. A succession of finger and hand stances are identified and analyzed ( Imtiaz et al., 2015 ; Subban & Mishra, 2013 ). In different parts of the world, other sign languages are used, including British Sign Language (BSL), American Sign Language (ASL), Arabic Sign Language (ArSL), and Spanish Sign Language ( Carol & Humphries, 1988 ). Each of these sign languages has developed independently of the others. Typically, gestures in sign languages are generated either by signs that are ideographic notional hand movements, such as the thumbs-up, which is frequently used for the word “ok”, or by spelling words letter by letter following specific sign language norms ( Li, Yang & Peng, 2009 ). Two key technologies are being deployed for hand posture or gesture recognition. There are two approaches: one is based on computer vision, which takes photographs of the signer and converts them into text using image analysis algorithms, and the other is based on machine learning. The third option is the use of a sensor-equipped glove ( Oudah, Al-Naji & Chahl, 2020 ).
Due to various factors, the current state of Sign Language Recognition (SLR) is around 30 years behind voice recognition systems. One of the critical reasons for this is that receiving and detecting two-dimensional video data is far more complex than analyzing linear audio signals. Furthermore, verbal communication lexical and grammatical objects have yet to be fully discovered, and no conventional vocabularies are available. Aside from this, there are no traditional definitions for such a considerable number of signs. Sign language classification and recognition reached a high point in terms of research papers in the early 1990s ( Elakkiya, 2020 ). The data collecting techniques are critical in categorizing the essential characteristics of various research on SLR. Due to the extreme dependability of sensor-based SLR systems, many studies have investigated data gloves or cyber gloves to extract the properties of the mechanical and non-mechanical components of the signs. Unfortunately, the usage of such sensors is unpleasant and restricting for the signer.
Furthermore, due to the high cost of sensors, real deployments of sensor-based SLR devices are impractical. On the other side, vision-based SLR systems have profoundly affected researchers due to their weight and capacity to handle crowded, dynamic heterogeneous situations and fluctuations under varied illuminations and occlusions in the feature extraction stage ( Elakkiya & Selvamani, 2015 ; Elakkiya & Selvamani, 2018 ). The population sampling methods are critical in categorizing various SLR works’ essential aspects. Due to the extreme dependability of sensor-based SLR systems, many researchers have employed electronic gloves or cyber gloves to extract data of the mechanical and non-mechanical components of the signs.
Nonetheless, for the signer, the usage of these sensors is somewhat uncomfortable and extremely limiting ( Elakkiya et al., 2012 ). In addition, due to the high cost of sensors, practical applications of sensor-based SLR systems are impractical. On the other hand, vision-based SLR systems have profoundly affected researchers due to their heaviness and capacity to manage crowded, dynamic heterogeneous surroundings and fluctuations in the segmentation stage under varying illuminations and occlusions ( Elakkiya, Kannan & Selvamani, 2013 ). The SLR solutions’ standard element automatically allows signer-dependent actions, i.e., all signers are trained before involving the patient. Signer independence or cross-validation among signers, on the other hand, entails the normalization of features to eliminate signer interactions. The range between some signers and the camera and the signer’s posture and magnification is rarely disclosed. SLR’s early phases were comparable to speech recognition in that they focused on individual signs.
Even though various SLR methods for identifying continuous phrases have been created, the detection accuracy has only achieved up to 90% for short dictionaries. The epenthesis motion occurs among adjacent signs in endless sign sentences. Previous studies have not specified if these are directly modeled, indirectly constructed, or just ignored. The action base will be expanded if transition movements are additionally simulated with the signs. Transition motions may be misclassified as signs unless they are modeled or ignored. The current recognition system recognizes a vast vocabulary simply utilizing sensor-based equipment, depending on the state-of-the-art sign language recognition. The classification performance is valid for the confined test situation, and many systems are signer-dependent. There isn’t much information about heftiness in real-time applications of SLR systems.
Furthermore, the specific vocabulary collections are unknown, and no common language for such speeches exists. In conclusion, none of the existing recognition systems meet the stringent real-world application requirements ( Elakkiya & Selvamani, 2017 ). Keeping in mind the following implications of sensor-based SLR systems, the machine learning-based recognition seems too helpful and most effective and accurate.
On the other hand, computer vision-based methods use bare hands without colored gloves or sensors. Compared to sensor-based methods, vision-based solutions offer more mobility and normalcy for signers and be more cost-efficient due to a single camera. The classification methods may be divided into two types based on machine learning techniques: supervised learning and unsupervised learning. The SLR system can detect static and dynamic gestures of signs using these methods. For SLR, there are many categorization methods available. Neural networks (NNs), Hidden Markov models, support vector machine (SVM), KNN, K-means clustering, self-organizing maps (SOM), dynamic time warping, finite state machines, Kalman filtering, particle filtering, the condensation algorithm, and Bayesian classifier are some special classification techniques ( Elakkiya, 2020 ; Gomes et al., 2016 ).
This literature review is needed to discover which classifiers have been utilized with what claimed accuracies and which sections of Urdu language have not been examined to locate data sources that other researchers have been using. To get insight into how others have defined and measured essential concepts. ‘Contribute to the advancement of knowledge in the area. It is a good idea to go over the literature to see what has been done previously and what worked and didn’t. Because of this, you may uncover gaps in the literature, which you can then seek to fix or address with your study by analyzing previous studies. To help future researchers in Urdu sign language, we have examined the merits and drawbacks of the previous research. This research study provides a literature review of all the previously published research studies in both journals ( n = 10) and conference proceedings ( n = 10), as shown in Tables 1 and and2, 2 , based on machine learning approaches for recognizing Urdu Sign language. This literature review will look at several published studies regarding their details, findings, and validity. We will explore them, summarize them, analyze them, and discuss them. Until July 2021, we will continue to conduct research based on research publications from databases such as PubMed, IEEE Xplore, ScienceDirect, and Google Scholar. The primary goal of this work is to evaluate the current efficacy of various machine learning approaches used to diagnose voice disorders and examine the development, weaknesses, and difficulties that have been identified and future research requirements. The following are the main contributions of this paper: (1) to review the classifiers, feature extracted, and accuracies of included articles. (2) To review the datasets and their types, no. of images, and accuracies of included articles. (3) Identify the gap.
The following is a breakdown of the structure of this paper: “Introduction” includes a brief overview of sign language and discusses Urdu Sign Language in detail. The technique used to perform this literature review is described in “Materials and Methods”. The findings of this systematic examination are discussed in greater detail in “Results” of this document. “Discussion” discusses the primary research questions we are pursuing. “Conclusion” contains the conclusion of this entire work, including limitations, research gaps, and suggestions for further exploration.
Materials and Methods
Search methodology.
For this literature review, the population (P), intervention (I), comparison (C), and outcome (O) base PICO method was taken into consideration which was previously used by Syed, Rashid & Hussain (2020) and clearly defined the goals and intervention of this literature review and for what population it is intended for. PICO was used to develop the search strategy, which was as follows: Population = deaf people in Pakistan, Intervention = recognition of Urdu Sign Language, Comparison = all the datasets developed for Urdu Sign Language and all the machine learning classifiers implemented on Urdu Sign Language, and Outcome = accuracies reported in the selected study. To construct a set of search strings, the Boolean operator combined relevant analogs and alternative words: AND focuses and limits the search, while OR widens and increases the investigation ( Syed, Rashid & Hussain, 2020 ). The following search term was created with the assistance of these Boolean operators:
- • (Pakistani sign language) OR (Urdu sign language) AND (“computer vision” OR “neural network” OR “artificial intelligence” OR “pattern recognition” OR “machine learning”)
- • (Pakistani sign language) AND (“computer vision” OR “neural network” OR “artificial intelligence” OR “pattern recognition” OR “machine learning”)
- • (Urdu sign language) AND (“computer vision” OR “neural network” OR “artificial intelligence” OR “pattern recognition” OR “machine learning”)
- • (Urdu sign language/Pakistani sign language) AND (computer vision)
- • (Urdu sign language/Pakistani sign language) AND (neural network)
- • (Urdu sign language/Pakistani sign language) AND (artificial intelligence)
- • (Urdu sign language/Pakistani sign language) AND (pattern recognition),
- • (Urdu sign language/Pakistani sign language) AND (machine learning)
Searches for peer-reviewed papers were conducted in four large databases: PubMed, IEEE Xplore, Google Scholar, and ScienceDirect (all of which are free to use). Review papers, research articles, conference abstracts, correspondences, data articles, debates, and case reports were the only types of articles that could be found in ScienceDirect. All three databases will be checked until July 2021. These databases have been searched using a set of keywords that have been shown above and utilized to do searches. The search results were found in PubMed ( n = 3), IEEE Xplore ( n = 9), Google Scholar ( n = 11), and ScienceDirect ( n = 49), with a total of 72 results when the initial search was performed, as shown in Fig. 2 . In Fig. 3 , it can be seen that most research work for the application of machine learning techniques for Urdu Sign Language Recognition has been conducted and published in the last fifteen years, from 2007 to 2021, which itself exhibits the significance of this investigation.

Survey methodology
To declutter the research studies extracted through the PICO search method defined in Syed, Rashid & Hussain (2020) , PRISMA ( Liberati et al., 2009 ) protocols were followed. Search results were collected and arranged using the online endnote system, as depicted in Fig. 4 . The endnote web system constructed a data table taken from each selected document. Full texts of articles that were deemed possibly appropriate were uploaded to the Endnote website for viewing (by Clarivate Analytics). In the first attempt, it was necessary to apply the search criteria ( Table 1 ) to each specified database to include the whole document in journals and conferences. There have been thousands of useless results from this approach, and as a result, a decision is taken to limit further the search to only the title and type of material contained within the page.

Further research is determined by referring to the sources of the linked studies discovered. Following the collection of leading search research, we evaluated the titles and abstracts of the studies to identify relevant ones. The current investigation results, including a comprehensive text, are being used to assess the relevant studies.
Table 2 depicts the details of all the datasets used in the selected studies, including a character-based, EMG signals-based, and sign images-based dataset. Figure 5 representing that which dataset is the most used and which is the least used and as per the resulting signal based EMG data has only used twice in Khan et al. (2020b) and Khan et al. (2020a) whereas dataset which contains the images of sign used four times in Halim & Abbas (2014) , Kanwal et al. (2014) , Nasir et al. (2014) and Imran et al. (2021a) . The most used type of dataset are the character-based datasets in Chandio et al. (2020) , Naseem et al. (2019) , Sagheer et al. (2010) , Ahmad et al. (2017) , Sami, (2014) , Husnain et al. (2019) , Gul et al. (2020) Arafat & Iqbal (2020) and Ahmed et al. (2017) . Also, in Fig. 6 , we can observe that only five datasets are publically available out of four datasets ( Chandio et al., 2020 ; Sagheer et al., 2010 ; Arafat & Iqbal, 2020 ; Ahmed et al., 2017 ) contain either character, numeral, or sentence-based images. Only one dataset ( Liberati et al., 2009 ) is publically available, which is based on images of the visually impaired individual making signs of Urdu Language and the highest accuracy reported in publically available datasets is 97% by Chandio et al. (2020) , which is reported on the text-based dataset and not on sign based dataset which is the actual lackness in this area.

Whereas in Fig. 7 , all the not publically available datasets, the highest reported accuracy 98%, again reported on the text-based dataset and not on the sign-based dataset. In Table 2 , Halim & Abbas (2014) , Kanwal et al. (2014) , Nasir et al. (2014) and Imran et al. (2021a) are the only authors who have used sign based language dataset. Generally, there are two types in recognizing the Urdu language, i.e., sign-based images and text-based images. Sign language recognition models employ two kinds of input data to extract the essential characteristics: static and dynamic. Several deep-based models using still or sequential inputs have been presented in recent years. While active inputs provide sequential information that might help increase the sign language recognition rate, there are still certain obstacles to overcome, such as the computational cost of input sequences. Dynamic inputs may also be divided into separate dynamical inputs and continuous inputs. Discrete active inputs are utilized at the word level, whereas continual inputs are used at the sentence level. Tokenization of sentences into individual words, identifying the start and conclusion of a phrase, and handling abbreviations and repetitions in the sentence are all issues with continuous dynamic inputs. We’ll go through the sign language recognition algorithms that have utilized these inputs in the following sections.

Table 3 summarizes all 20 studies that are included in this literature review. This table covers all the important details, i.e., classifier name, which feature is extracted, reported accuracy, reported sensitivity and reported specificity. The study is either published in a journal or conference. From Table 3 , we can analyzed that the SVM ( Chandio et al., 2020 ; Imran et al., 2021a ; Sagheer et al., 2010 ; Ahmad et al., 2017 ; Khan et al., 2020b ; Ahmed et al., 2017 ; Imran et al., 2021b ) and Neural Network ( Chandio et al., 2020 ; Naseem et al., 2019 ; Ahmad et al., 2007 ; Arafat & Iqbal, 2020 ; Sagheer et al., 2009 ; Naz et al., 2015 ; Ul-Hasan et al., 2013 ) is the commonly used classifier by researchers for the detection of Urdu Sign Language other than these both rest of the classifiers used only once i.e., DTW ( Halim & Abbas, 2014 ), HMM ( Gul et al., 2020 ).
Figure 8 represents the reported accuracies that have been generated after using SVM as a classifier, and it has been noted that the highest accuracy in SVM is written by Sagheer et al. in ( Ahmed et al., 2017 ) on a character-based dataset which is publically available by the name of UNHD (Urdu-Nasta’liq Handwritten Dataset). The Support Vector Machine (SVM) is an old classification technique that has piqued the research community’s attention, particularly in machine classification, regression, and learning, among other areas. SVM with the accompanying classes that are well-known. This is described as the process of filtering or extracting characteristics. Even if no prediction of unknown samples is required, feature evaluation and SVM classification have been utilized in conjunction with one another. In class differentiation, they can designate the main sets involved in the process. The SVM depicts the entrance space as a vast area with several doors. By generating an optimal hyperplane separation, the SVM determined the boundary between regions belonging to both classes of sites. The hyperplane is selected to optimize the separation between the closest samples of exercises. Initially, SVM models were developed to sort linear categories into subcategories. Because of the massive characteristics, it is impossible to use the function attributes to identify the separation hyperplane in their pure form.

The specific part is used to calculate non-linear mapping utilizing unique non-linear variables called the kernel, derived from the characteristic function. It has the advantage of operating in the input area where the weighted sum of the kernel function assessed by support vectors can be utilized to solve the classification problem. In contrast, the support vectors have the disadvantage of only functioning in the output area. The SVM algorithm can create a variety of learning machines by utilizing a variety of kernel functions. Compared to artificial neural networks, SVM tends to be significantly more accurate and produce more promising outcomes ( Uma Rani & Holi, 2014 ). Support vector machines (SVMs) have emerged as a popular machine learning technique for classification, regression, and novelty detection tasks. They exhibit outstanding performance and effectiveness on a wide range of real-world questions, and the approach is conceptually motivated by logic. It is not necessary to seek out the architecture of the learner machine through experimentation ( Huang et al., 2018 ) to achieve success. There are only a few free parameters available. Even though SVMs are incredibly effective classifiers that use non-linear kernels, they have drawbacks: (1). It is necessary to test alternative kernel configurations and model parameters to obtain the optimal model; (2).
In some cases, training might take a lengthy time, especially if there are many characteristics or examples in the data set; (3). Their inner workings are difficult to comprehend since the fundamental models are built on sophisticated mathematical frameworks, and their conclusions are tough to interpret. The selection of features using all available data, followed by the testing of classifier training, for example, results in an optimistic error estimate ( Yue, Li & Hao, 2003 ).
Figure 9 represents the reported accuracies that have been generated after using neural networks, which include RNN, CNN, DLN, MD-RNN, and BLSTM-RNN. In the CNN, numerous hierarchy levels are formed of routing groups and grouping layers, and each of these levels is defined by a different type of chart. A convolutional layer, which receives data at the input level, is the starting point for most CNNs. The convolution layer is responsible for convolutionary processes involving a small number of filtering maps of the same dimension. In addition, the result from this layer is passed to the sample layer, which reduces the scale of the subsequent layers in the sequence. CNN is closely associated with a wide range of deep neural networks locally ( Jan et al., 0000 ). These systems are then deployed on several hundred cores of GPU architecture based on the GPU architecture. Following the previous layer information blocks ( O’Shea & Nash, 2015 ), the appropriate people will assign the role maps. It is dependent on the size of the maps.

On the other hand, each thread is tied to a single neuron utilizing a suitable block that contains several lines. Similarly, neuron convolution, induction, and summation are performed on the input neurons throughout the procedure. Finally, the techniques described above are stored in global memory. A reverse and propagation model is used to handle results as efficiently as possible. On the other hand, pulling or moving activities lead to parallel spread because a single distribution would not result in a beneficial consequence. As mentioned previously, the neurons of a single layer communicate with a different number of neurons, which impacts the border effect ( Yang & Horie, 2015 ).
Figure 10 represents the reported accuracies using the UPTI (Urdu Printed Text Images) dataset ( Ahmad et al., 2017 ; Sabbour & Shafait, 2013 ; Naz et al., 2015 ; Ul-Hasan et al., 2013 ), as it can be observed in Table 2 that UPTI is the most used dataset and the highest reported accuracy is 96%. This dataset contains a total of 10,063 images of different sentences of the Urdu language, and this is also a publically available dataset. But the problem lies here that this is not the sign language dataset, which is the authors’ primary concern.

The author’s first concern is the lack of publicly available datasets containing images of individuals making the signs. Only one such dataset is available in Mendeley by Imran et al. in Sami (2014) in 2021, which is very recent. There are so many things that can be pointed out as a flaw. The number of participants used for this dataset is 40. Each individual contributes 37 pictures (one picture each of 37 Urdu characters), making only 1480 images in total considered a minimal dataset. Furthermore, only SVM as a classifier to validate this dataset.
Another lack of concern that the author has is the lackness of machine learning outcomes, i.e., specificity and sensitivity. Only one study, i.e., Khan et al. (2020a) , reports all three products: accuracy, specificity, and sensitivity. Naseem et al. (2019) didn’t write the accuracy, the primary machine learning outcome. The last noticeable thing is that not even a single author has used unsupervised techniques as a classifier in the screened studies, which means that a lot of work needs to be done in this area.
When it comes to the limitations of this literature review, we can’t ignore the fact that the number of papers included was far lower than expected. As a second point, only studies published in English were considered for inclusion, limiting the representation of work from non-English countries that speak and the generalizability of the findings. Third, there is a strong likelihood that the search technique used for this review overlooked some significant articles, given that papers published in conference proceedings were primarily disregarded.
When a person cannot hear, they are deaf, making communicating with others extremely difficult. More than 5% of the global population, including adults and children, is deaf, and around 10 million Pakistanis are deaf. Another impairment is muteness, which occurs when an individual cannot talk or communicate correctly. There are many other types of disabilities. People like this have a very distinct manner of connecting with the rest of the world. Through “Sign Language”, they communicate their feelings and thoughts to the rest of the world. Sign language is very distinct and not comprehended by others; many institutions and organizations worldwide teach people with disabilities and their families sign dialects to make one’s lives more accessible; however, learning sign language is not easy. Not everybody is familiar with it. This literature review summarized 20 screened studies included after the detailed screening. It is also concluded that SVM and Neural Network are the most common classifiers. The first identified gap is the lack of publically available datasets and, most specifically, datasets with images of signs of Urdu characters and not the actual characters. The second identified gap is that the authors can use unsupervised machine learning classifiers because this is an untouched territory, and a tremendous amount of work can be done here.
Funding Statement
The authors received no funding for this work.
Additional Information and Declarations
The authors declare there are no competing interests.
Hira Zahid conceived and designed the experiments, performed the experiments, analyzed the data, performed the computation work, prepared figures and/or tables, authored or reviewed drafts of the paper, and approved the final draft.
Munaf Rashid conceived and designed the experiments, performed the experiments, analyzed the data, performed the computation work, authored or reviewed drafts of the paper, and approved the final draft.
Samreen Hussain analyzed the data, prepared figures and/or tables, and approved the final draft.
Fahad Azim and Afshan Saad analyzed the data, authored or reviewed drafts of the paper, and approved the final draft.
Sidra Abid Syed analyzed the data, performed the computation work, authored or reviewed drafts of the paper, and approved the final draft.
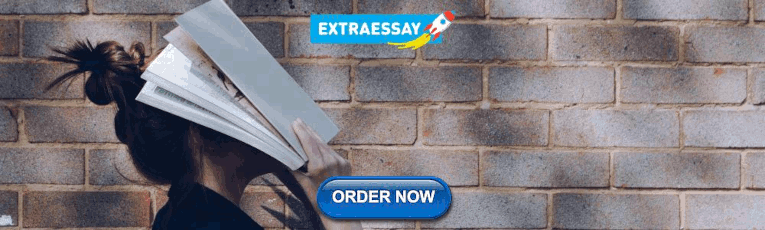
IMAGES
VIDEO
COMMENTS
"Urdu Research Journal" is an open access refereed journal published quarterly. The Journal strives to publish work of high quality in research and literature works across the globe in Urdu language and literary theory. The aim of the journal is to provide high quality research material in Urdu for scholars and researchers.
In the meeting of the editorial board of Journal of Research (Urdu) held on June 30, 2022, while approving the publishing of current issue of June 2022, i.e Vol. 38, No. 1, it has been decided that articles only composed in Inpage format will be accepted for processing of publication in JO...
Explore the latest full-text research PDFs, articles, conference papers, preprints and more on URDU. Find methods information, sources, references or conduct a literature review on URDU
شش ماہی تحقیقی مجلّہ. Name: MATAN (Urdu Research Journal) ISSN (print): 2708-5724. ISSN (online): 2708-5732. Publishing year: 2020. Online publishing year: 2020. Institute: Faculty of Arts & Language. Publisher: Department of Urdu, The Islamia University of Bahawalpur. Biannual Double Blind Peer Reviewed Urdu Research Journal ...
The Journal of Urdu Studies is a peer-reviewed, academic journal dedicated to the study of Urdu across a range of disciplines in the humanities and social sciences. The objective of the journal is to advance the field of Urdu Studies by publishing superior scholarship, setting and maintaining the highest standards in Urdu-English translation, developing new methods in Urdu research, and ...
A research Journal is a systematic record of scholarly works of researchers. It is an academic publication of peer reviewed articles in a given field, which presents research as a straight forward and clear process. Universities are research based institution and research journals of universities publish the research articles and encourage the ...
Explore the latest full-text research PDFs, articles, conference papers, preprints and more on URDU. Find methods information, sources, references or conduct a literature review on URDU
Explore the latest full-text research PDFs, articles, conference papers, preprints and more on URDU. Find methods information, sources, references or conduct a literature review on URDU
Khan et al. [43] conducted a survey on Urdu sentiment analysis by reviewing more than 14 articles published in sentiment analysis of Urdu language. The techniques required for Urdu SA were classified on the basis of machine learning, lexicon-based and hybrid approaches. However, still, there is a need to conduct a comprehensive survey, which can cover all aspects Urdu SA with respect to posed ...
This paper contains a comprehensive study of research conducted on Roman Urdu and Urdu text for a product review. This study is divided into categories, such as collection of relevant corpora, data preprocessing, feature extraction, classification platforms and approaches, limitations, and future work.
A research study focusing on Urdu sentiment analysis 41 created two datasets of user reviews to examine the efficiency of the proposed model. Only 650 movie reviews are included in the C1 dataset ...
URDU-16 A Scientific and Research Review of the Different Stages and Procedures of Jurisprudence in the History of Complication and a scholarly research review of Abdul Rahman Al-Jazeera's book Al-Fiqh Ali Al-Madhahib Al-Arba'ah ... Al-Aijaz Research Journal of Islamic Studies and Humanities; ISSN (Print) : 2707-1200; ISSN (Electronic ...
Indian Institute of Technology Delhi. [email protected]. Abstract. In this paper, we propose a novel approach to address the challenges of printed Urdu text recognition using high-resolution, multi-scale semantic feature extraction. Our proposed UTRNet architecture, a hybrid CNN-RNN model, demonstrates state-of-the-art performance on ...
Abstract. It has been published for the Department of Urdu, Jai Prakash University, Chapra (India), and it contains research papers written by Prof. Shahnaz Nabi, Zehra Mehdi, Dr. Najeeba Arif, Dr ...
languages, Urdu has also changed with the passage of time. This research paper aims to find out the dimension of semantic change in Urdu, discussing how the linguistic expressions change their meanings over time. The researchers observed that Urdu speakers are using lexis in different senses from the meanings given in dictionaries.
Urdu Ocr. Optical Character Recognition (OCR) has emerged as an interesting research field. Lot of work has been declared in Urdu script based on various approaches and diverse methodologies have been put forward on Nastaliq font style to get the desired output. The paper presents a survey on different techniques of OCR and ends up with the ...
URDU RESEARCH : ارمغان ISSN (Print): 2707-6288 ISSN (Online): 2788-8355 Skip to main content ... The research papers are accepted for publications on the understanding that they have not been published earlier. Research paper should be an original piece, including methodology, contents, data analysis and interpretation. ...
distinct interactions. Thus, this research paper analyses natural conversation on various levels of code-mixing and code-switching of Urdu-Punjabi among children's speech bearing the age of 2 to 5 in their daily life in Sahiwal city. Though "the language of global communication is English" (Aziz et al., 2021, p.884) but the
Hi, I am back with the latest video. In this video you will learn, how to write research paper. Numbers of persons are worried about the steps to write resea...
Research articles that use Urdu sign language as a language for detection: ... Review papers, research articles, conference abstracts, correspondences, data articles, debates, and case reports were the only types of articles that could be found in ScienceDirect. All three databases will be checked until July 2021. These databases have been ...
Very limited research work has been done in Urdu or Roman Urdu languages. Whereas, Hindi/Urdu is the third largest language in the world. In this paper, we focus on the sentiment analysis of ...
IIUI mission is to transform the society by promoting education, training, research, technology, and collaboration for reconstruction of human thought in all its forms on the foundations of Islam. IIUI vision to be an excellent University in diversity, knowledge, research, and innovation for the benefits of society and the Muslim Ummah.