9.1 Null and Alternative Hypotheses
The actual test begins by considering two hypotheses . They are called the null hypothesis and the alternative hypothesis . These hypotheses contain opposing viewpoints.
H 0 , the — null hypothesis: a statement of no difference between sample means or proportions or no difference between a sample mean or proportion and a population mean or proportion. In other words, the difference equals 0.
H a —, the alternative hypothesis: a claim about the population that is contradictory to H 0 and what we conclude when we reject H 0 .
Since the null and alternative hypotheses are contradictory, you must examine evidence to decide if you have enough evidence to reject the null hypothesis or not. The evidence is in the form of sample data.
After you have determined which hypothesis the sample supports, you make a decision. There are two options for a decision. They are reject H 0 if the sample information favors the alternative hypothesis or do not reject H 0 or decline to reject H 0 if the sample information is insufficient to reject the null hypothesis.
Mathematical Symbols Used in H 0 and H a :
H 0 always has a symbol with an equal in it. H a never has a symbol with an equal in it. The choice of symbol depends on the wording of the hypothesis test. However, be aware that many researchers use = in the null hypothesis, even with > or < as the symbol in the alternative hypothesis. This practice is acceptable because we only make the decision to reject or not reject the null hypothesis.
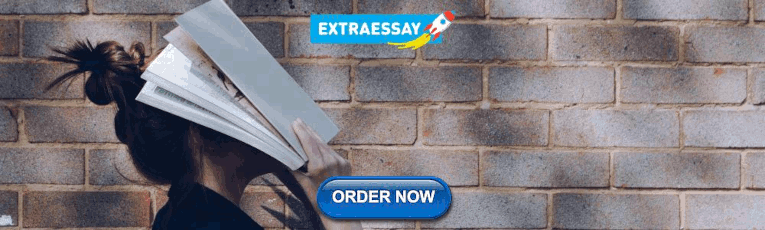
Example 9.1
H 0 : No more than 30 percent of the registered voters in Santa Clara County voted in the primary election. p ≤ 30 H a : More than 30 percent of the registered voters in Santa Clara County voted in the primary election. p > 30
A medical trial is conducted to test whether or not a new medicine reduces cholesterol by 25 percent. State the null and alternative hypotheses.
Example 9.2
We want to test whether the mean GPA of students in American colleges is different from 2.0 (out of 4.0). The null and alternative hypotheses are the following: H 0 : μ = 2.0 H a : μ ≠ 2.0
We want to test whether the mean height of eighth graders is 66 inches. State the null and alternative hypotheses. Fill in the correct symbol (=, ≠, ≥, <, ≤, >) for the null and alternative hypotheses.
- H 0 : μ __ 66
- H a : μ __ 66
Example 9.3
We want to test if college students take fewer than five years to graduate from college, on the average. The null and alternative hypotheses are the following: H 0 : μ ≥ 5 H a : μ < 5
We want to test if it takes fewer than 45 minutes to teach a lesson plan. State the null and alternative hypotheses. Fill in the correct symbol ( =, ≠, ≥, <, ≤, >) for the null and alternative hypotheses.
- H 0 : μ __ 45
- H a : μ __ 45
Example 9.4
An article on school standards stated that about half of all students in France, Germany, and Israel take advanced placement exams and a third of the students pass. The same article stated that 6.6 percent of U.S. students take advanced placement exams and 4.4 percent pass. Test if the percentage of U.S. students who take advanced placement exams is more than 6.6 percent. State the null and alternative hypotheses. H 0 : p ≤ 0.066 H a : p > 0.066
On a state driver’s test, about 40 percent pass the test on the first try. We want to test if more than 40 percent pass on the first try. Fill in the correct symbol (=, ≠, ≥, <, ≤, >) for the null and alternative hypotheses.
- H 0 : p __ 0.40
- H a : p __ 0.40
Collaborative Exercise
Bring to class a newspaper, some news magazines, and some internet articles. In groups, find articles from which your group can write null and alternative hypotheses. Discuss your hypotheses with the rest of the class.
As an Amazon Associate we earn from qualifying purchases.
This book may not be used in the training of large language models or otherwise be ingested into large language models or generative AI offerings without OpenStax's permission.
Want to cite, share, or modify this book? This book uses the Creative Commons Attribution License and you must attribute Texas Education Agency (TEA). The original material is available at: https://www.texasgateway.org/book/tea-statistics . Changes were made to the original material, including updates to art, structure, and other content updates.
Access for free at https://openstax.org/books/statistics/pages/1-introduction
- Authors: Barbara Illowsky, Susan Dean
- Publisher/website: OpenStax
- Book title: Statistics
- Publication date: Mar 27, 2020
- Location: Houston, Texas
- Book URL: https://openstax.org/books/statistics/pages/1-introduction
- Section URL: https://openstax.org/books/statistics/pages/9-1-null-and-alternative-hypotheses
© Jan 23, 2024 Texas Education Agency (TEA). The OpenStax name, OpenStax logo, OpenStax book covers, OpenStax CNX name, and OpenStax CNX logo are not subject to the Creative Commons license and may not be reproduced without the prior and express written consent of Rice University.
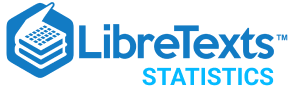
- school Campus Bookshelves
- menu_book Bookshelves
- perm_media Learning Objects
- login Login
- how_to_reg Request Instructor Account
- hub Instructor Commons
- Download Page (PDF)
- Download Full Book (PDF)
- Periodic Table
- Physics Constants
- Scientific Calculator
- Reference & Cite
- Tools expand_more
- Readability
selected template will load here
This action is not available.
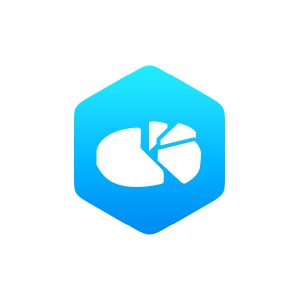
9.1: Null and Alternative Hypotheses
- Last updated
- Save as PDF
- Page ID 23459
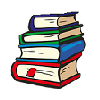
The actual test begins by considering two hypotheses . They are called the null hypothesis and the alternative hypothesis . These hypotheses contain opposing viewpoints.
\(H_0\): The null hypothesis: It is a statement of no difference between the variables—they are not related. This can often be considered the status quo and as a result if you cannot accept the null it requires some action.
\(H_a\): The alternative hypothesis: It is a claim about the population that is contradictory to \(H_0\) and what we conclude when we reject \(H_0\). This is usually what the researcher is trying to prove.
Since the null and alternative hypotheses are contradictory, you must examine evidence to decide if you have enough evidence to reject the null hypothesis or not. The evidence is in the form of sample data.
After you have determined which hypothesis the sample supports, you make a decision. There are two options for a decision. They are "reject \(H_0\)" if the sample information favors the alternative hypothesis or "do not reject \(H_0\)" or "decline to reject \(H_0\)" if the sample information is insufficient to reject the null hypothesis.
\(H_{0}\) always has a symbol with an equal in it. \(H_{a}\) never has a symbol with an equal in it. The choice of symbol depends on the wording of the hypothesis test. However, be aware that many researchers (including one of the co-authors in research work) use = in the null hypothesis, even with > or < as the symbol in the alternative hypothesis. This practice is acceptable because we only make the decision to reject or not reject the null hypothesis.
Example \(\PageIndex{1}\)
- \(H_{0}\): No more than 30% of the registered voters in Santa Clara County voted in the primary election. \(p \leq 30\)
- \(H_{a}\): More than 30% of the registered voters in Santa Clara County voted in the primary election. \(p > 30\)
Exercise \(\PageIndex{1}\)
A medical trial is conducted to test whether or not a new medicine reduces cholesterol by 25%. State the null and alternative hypotheses.
- \(H_{0}\): The drug reduces cholesterol by 25%. \(p = 0.25\)
- \(H_{a}\): The drug does not reduce cholesterol by 25%. \(p \neq 0.25\)
Example \(\PageIndex{2}\)
We want to test whether the mean GPA of students in American colleges is different from 2.0 (out of 4.0). The null and alternative hypotheses are:
- \(H_{0}: \mu = 2.0\)
- \(H_{a}: \mu \neq 2.0\)
Exercise \(\PageIndex{2}\)
We want to test whether the mean height of eighth graders is 66 inches. State the null and alternative hypotheses. Fill in the correct symbol \((=, \neq, \geq, <, \leq, >)\) for the null and alternative hypotheses.
- \(H_{0}: \mu \_ 66\)
- \(H_{a}: \mu \_ 66\)
- \(H_{0}: \mu = 66\)
- \(H_{a}: \mu \neq 66\)
Example \(\PageIndex{3}\)
We want to test if college students take less than five years to graduate from college, on the average. The null and alternative hypotheses are:
- \(H_{0}: \mu \geq 5\)
- \(H_{a}: \mu < 5\)
Exercise \(\PageIndex{3}\)
We want to test if it takes fewer than 45 minutes to teach a lesson plan. State the null and alternative hypotheses. Fill in the correct symbol ( =, ≠, ≥, <, ≤, >) for the null and alternative hypotheses.
- \(H_{0}: \mu \_ 45\)
- \(H_{a}: \mu \_ 45\)
- \(H_{0}: \mu \geq 45\)
- \(H_{a}: \mu < 45\)
Example \(\PageIndex{4}\)
In an issue of U. S. News and World Report , an article on school standards stated that about half of all students in France, Germany, and Israel take advanced placement exams and a third pass. The same article stated that 6.6% of U.S. students take advanced placement exams and 4.4% pass. Test if the percentage of U.S. students who take advanced placement exams is more than 6.6%. State the null and alternative hypotheses.
- \(H_{0}: p \leq 0.066\)
- \(H_{a}: p > 0.066\)
Exercise \(\PageIndex{4}\)
On a state driver’s test, about 40% pass the test on the first try. We want to test if more than 40% pass on the first try. Fill in the correct symbol (\(=, \neq, \geq, <, \leq, >\)) for the null and alternative hypotheses.
- \(H_{0}: p \_ 0.40\)
- \(H_{a}: p \_ 0.40\)
- \(H_{0}: p = 0.40\)
- \(H_{a}: p > 0.40\)
COLLABORATIVE EXERCISE
Bring to class a newspaper, some news magazines, and some Internet articles . In groups, find articles from which your group can write null and alternative hypotheses. Discuss your hypotheses with the rest of the class.
In a hypothesis test , sample data is evaluated in order to arrive at a decision about some type of claim. If certain conditions about the sample are satisfied, then the claim can be evaluated for a population. In a hypothesis test, we:
- Evaluate the null hypothesis , typically denoted with \(H_{0}\). The null is not rejected unless the hypothesis test shows otherwise. The null statement must always contain some form of equality \((=, \leq \text{or} \geq)\)
- Always write the alternative hypothesis , typically denoted with \(H_{a}\) or \(H_{1}\), using less than, greater than, or not equals symbols, i.e., \((\neq, >, \text{or} <)\).
- If we reject the null hypothesis, then we can assume there is enough evidence to support the alternative hypothesis.
- Never state that a claim is proven true or false. Keep in mind the underlying fact that hypothesis testing is based on probability laws; therefore, we can talk only in terms of non-absolute certainties.
Formula Review
\(H_{0}\) and \(H_{a}\) are contradictory.
- If \(\alpha \leq p\)-value, then do not reject \(H_{0}\).
- If\(\alpha > p\)-value, then reject \(H_{0}\).
\(\alpha\) is preconceived. Its value is set before the hypothesis test starts. The \(p\)-value is calculated from the data.References
Data from the National Institute of Mental Health. Available online at http://www.nimh.nih.gov/publicat/depression.cfm .
Null Hypothesis Definition and Examples
PM Images / Getty Images
- Chemical Laws
- Periodic Table
- Projects & Experiments
- Scientific Method
- Biochemistry
- Physical Chemistry
- Medical Chemistry
- Chemistry In Everyday Life
- Famous Chemists
- Activities for Kids
- Abbreviations & Acronyms
- Weather & Climate
- Ph.D., Biomedical Sciences, University of Tennessee at Knoxville
- B.A., Physics and Mathematics, Hastings College
In a scientific experiment, the null hypothesis is the proposition that there is no effect or no relationship between phenomena or populations. If the null hypothesis is true, any observed difference in phenomena or populations would be due to sampling error (random chance) or experimental error. The null hypothesis is useful because it can be tested and found to be false, which then implies that there is a relationship between the observed data. It may be easier to think of it as a nullifiable hypothesis or one that the researcher seeks to nullify. The null hypothesis is also known as the H 0, or no-difference hypothesis.
The alternate hypothesis, H A or H 1 , proposes that observations are influenced by a non-random factor. In an experiment, the alternate hypothesis suggests that the experimental or independent variable has an effect on the dependent variable .
How to State a Null Hypothesis
There are two ways to state a null hypothesis. One is to state it as a declarative sentence, and the other is to present it as a mathematical statement.
For example, say a researcher suspects that exercise is correlated to weight loss, assuming diet remains unchanged. The average length of time to achieve a certain amount of weight loss is six weeks when a person works out five times a week. The researcher wants to test whether weight loss takes longer to occur if the number of workouts is reduced to three times a week.
The first step to writing the null hypothesis is to find the (alternate) hypothesis. In a word problem like this, you're looking for what you expect to be the outcome of the experiment. In this case, the hypothesis is "I expect weight loss to take longer than six weeks."
This can be written mathematically as: H 1 : μ > 6
In this example, μ is the average.
Now, the null hypothesis is what you expect if this hypothesis does not happen. In this case, if weight loss isn't achieved in greater than six weeks, then it must occur at a time equal to or less than six weeks. This can be written mathematically as:
H 0 : μ ≤ 6
The other way to state the null hypothesis is to make no assumption about the outcome of the experiment. In this case, the null hypothesis is simply that the treatment or change will have no effect on the outcome of the experiment. For this example, it would be that reducing the number of workouts would not affect the time needed to achieve weight loss:
H 0 : μ = 6
- Null Hypothesis Examples
"Hyperactivity is unrelated to eating sugar " is an example of a null hypothesis. If the hypothesis is tested and found to be false, using statistics, then a connection between hyperactivity and sugar ingestion may be indicated. A significance test is the most common statistical test used to establish confidence in a null hypothesis.
Another example of a null hypothesis is "Plant growth rate is unaffected by the presence of cadmium in the soil ." A researcher could test the hypothesis by measuring the growth rate of plants grown in a medium lacking cadmium, compared with the growth rate of plants grown in mediums containing different amounts of cadmium. Disproving the null hypothesis would set the groundwork for further research into the effects of different concentrations of the element in soil.
Why Test a Null Hypothesis?
You may be wondering why you would want to test a hypothesis just to find it false. Why not just test an alternate hypothesis and find it true? The short answer is that it is part of the scientific method. In science, propositions are not explicitly "proven." Rather, science uses math to determine the probability that a statement is true or false. It turns out it's much easier to disprove a hypothesis than to positively prove one. Also, while the null hypothesis may be simply stated, there's a good chance the alternate hypothesis is incorrect.
For example, if your null hypothesis is that plant growth is unaffected by duration of sunlight, you could state the alternate hypothesis in several different ways. Some of these statements might be incorrect. You could say plants are harmed by more than 12 hours of sunlight or that plants need at least three hours of sunlight, etc. There are clear exceptions to those alternate hypotheses, so if you test the wrong plants, you could reach the wrong conclusion. The null hypothesis is a general statement that can be used to develop an alternate hypothesis, which may or may not be correct.
- What Are Examples of a Hypothesis?
- What Is a Hypothesis? (Science)
- What 'Fail to Reject' Means in a Hypothesis Test
- What Are the Elements of a Good Hypothesis?
- Scientific Hypothesis Examples
- Null Hypothesis and Alternative Hypothesis
- What Is a Control Group?
- Understanding Simple vs Controlled Experiments
- Six Steps of the Scientific Method
- Scientific Method Vocabulary Terms
- Definition of a Hypothesis
- Understanding Experimental Groups
- Type I and Type II Errors in Statistics
- An Example of a Hypothesis Test
- How to Conduct a Hypothesis Test
If you're seeing this message, it means we're having trouble loading external resources on our website.
If you're behind a web filter, please make sure that the domains *.kastatic.org and *.kasandbox.org are unblocked.
To log in and use all the features of Khan Academy, please enable JavaScript in your browser.
AP®︎/College Statistics
Course: ap®︎/college statistics > unit 10.
- Idea behind hypothesis testing
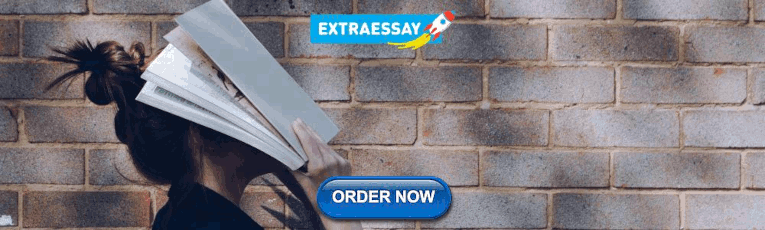
Examples of null and alternative hypotheses
- Writing null and alternative hypotheses
- P-values and significance tests
- Comparing P-values to different significance levels
- Estimating a P-value from a simulation
- Estimating P-values from simulations
- Using P-values to make conclusions
Want to join the conversation?
- Upvote Button navigates to signup page
- Downvote Button navigates to signup page
- Flag Button navigates to signup page

Video transcript
SYSTEMATIC REVIEW article
The flash effect—an evaluation of preclinical studies of ultra-high dose rate radiotherapy.
- 1 Department of Physics, Imperial College London, London, United Kingdom
- 2 Science and Technology Facilities Council (STFC), Rutherford Appleton Laboratory, Oxford, United Kingdom
- 3 Institut Curie, Universite Paris-Saclay, Centre national de la recherche scientifique (CNRS) UMR3347, Inserm U1021, Signalisation radiobiologie et cancer, Orsay, France
- 4 Universite Paris-Saclay, Centre national de la recherche scientifique (CNRS) UMR3347, Inserm U1021, Signalisation radiobiologie et cancer, Orsay, France
FLASH radiotherapy (FLASH-RT) is a novel radiotherapy approach based on the use of ultra-high dose radiation to treat malignant cells. Although tumours can be reduced or eradicated using radiotherapy, toxicities induced by radiation can compromise healthy tissues. The FLASH effect is the observation that treatment delivered at an ultra-high dose rate is able to reduce adverse toxicities present at conventional dose rates. While this novel technique may provide a turning point for clinical practice, the exact mechanisms underlying the causes or influences of the FLASH effect are not fully understood. The study presented here uses data collected from 41 experimental investigations (published before March 2024) of the FLASH effect. Searchable databases were constructed to contain the outcomes of the various experiments in addition to values of beam parameters that may have a bearing on the FLASH effect. An in-depth review of the impact of the key beam parameters on the results of the experiments was carried out. Correlations between parameter values and experimental outcomes were studied. Pulse Dose Rate had positive correlations with almost all end points, suggesting viability of FLASH-RT as a new modality of radiotherapy. The collective results of this systematic review study suggest that beam parameter qualities from both FLASH and conventional radiotherapy can be valuable for tissue sparing and effective tumour treatment.
1 Introduction
Cancer is an accumulation of abnormal, malignant cells which reproduce uncontrollably. In radiotherapy, a beam of ionising radiation is used to control or eliminate these malignant cells. The beam characteristics are optimised to maximise the impact on the cancer while minimising damage to healthy tissues. Radiotherapy is commonly delivered over several days in fractions of ∼ 2Gy, each fraction being delivered at a rate of < ∼ 10Gy/min. There is now a body of evidence that suggests that the delivery of the therapeutic dose at very high rates in FLASH Radiotherapy (FLASH-RT) yields a degree of tumour control equivalent to conventional radiotherapy while significantly reducing the adverse toxicities ( 1 ).
Studies of FLASH-RT are usually categorised using two parameters: tumour response and normal-tissue response. Previous studies have found that the normal-tissue response to FLASH-RT includes less clustered DNA damage sites, fewer dicentric chromosomes and a smaller fraction of G2 cells than are present in the response to conventional radiotherapy ( 2 ). While there are hypotheses on the processes that may give rise to the FLASH effect ( 3 ), its origin is unknown and the current understanding of the factors that influence the FLASH effect is limited.
In conjunction with dose rates, contradictory biological results from experiments performed with different beams resulted in the hypothesis that the beam parameters influence the appearance of the FLASH effect. This review explores the dependency of the FLASH effect on key irradiation parameters. The biological response induced by FLASH radiotherapy (FLASH-RT) is discussed, providing insights into its potential clinical applications.
2 Materials and methods
The primary focus of this study was the search for patterns in the data from experiments in which the FLASH effect had been investigated. Searchable databases were created in which the outcomes of FLASH radiotherapy experiments were stored alongside the parameters that determined the irradiation conditions. The database was then used to search for patterns in the results to determine which parameters most influence the normal-tissue and tumour responses to FLASH-RT.
A critical investigation of 41 experiments was performed to derive quantitative measures of the eradication of malignant cells and the creation of lasting side effects (e.g. skin damage and other toxicities). Examining each study in isolation allowed parameters to be identified that might be correlated to the measures of tumour control and normal-tissue sparing. By combining the data from all experiments, the analysis presented here seeks to overcome the statistical limitations of the individual studies, each of which is insufficient on its own to establish a clear connection between a particular parameter and the onset of the FLASH effect.
The crux of FLASH-RT is the increase of the therapeutic window through the delivery of the therapeutic dose at ultra-high dose rate. It has therefore been presupposed that the parameter that would be most directly correlated to the FLASH effect would be the dose rate. A number of preliminary studies such as the 2017 experiment conducted by Montay-Gruel et al. ( 4 ), which showed that higher dose rates were correlated with memory sparing, suggested this correlation. In order to elucidate the conditions in which a FLASH effect can be observed, it is imperative to expand these data sets and look for an overarching trend that is present in all, or many, studies.
2.1 Search criteria
The parameters identified as potentially correlated with the FLASH effect and considered in this study are:
● Mean Dose Rate (Gy/s)–the average dose rate across the duration of the irradiation;
● Pulse Dose Rate (Gy/s)–the dose rate delivered by each individual pulse, each pulse being composed of a number of bunches from the accelerator;
● Pulse Dose (Gy)–the dose in each pulse;
● Total Dose (Gy)–the total administered dose;
● Pulse Width ( µ s)–the temporal duration of each pulse;
● Total Duration (s)–the total time taken to administer the full dose;
● Repetition Frequency (Hz)–the frequency at which pulses are delivered; and
● Number of Pulses–the number of pulses delivered.
Data from papers published before March 2024 were considered for inclusion in the present study. The study evaluated each paper using the categories of: population, intervention, comparison, outcome (PICO) as a search strategy tool. The categories used to collect data for this systematic review study are listed in Table 1 .
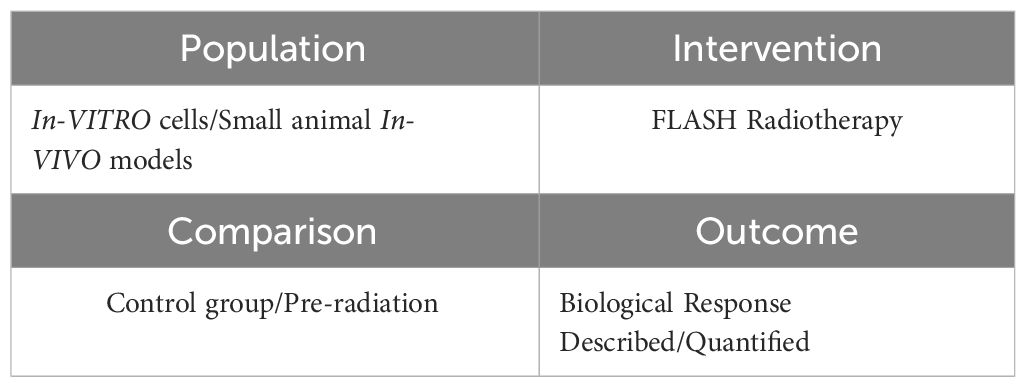
Table 1 Population, Intervention, Comparison, Outcome (PICO) search strategy used to select relevant experiments.
A more extensive description of each study and an explanation of the coherence to these qualities is listed in the Supplementary Materials . The specific tumour, tissue and cell types are listed in Tables 2 , 3 ; Table 2 displays the normal-tissue experiments and Table 3 displays the tumour experiments.
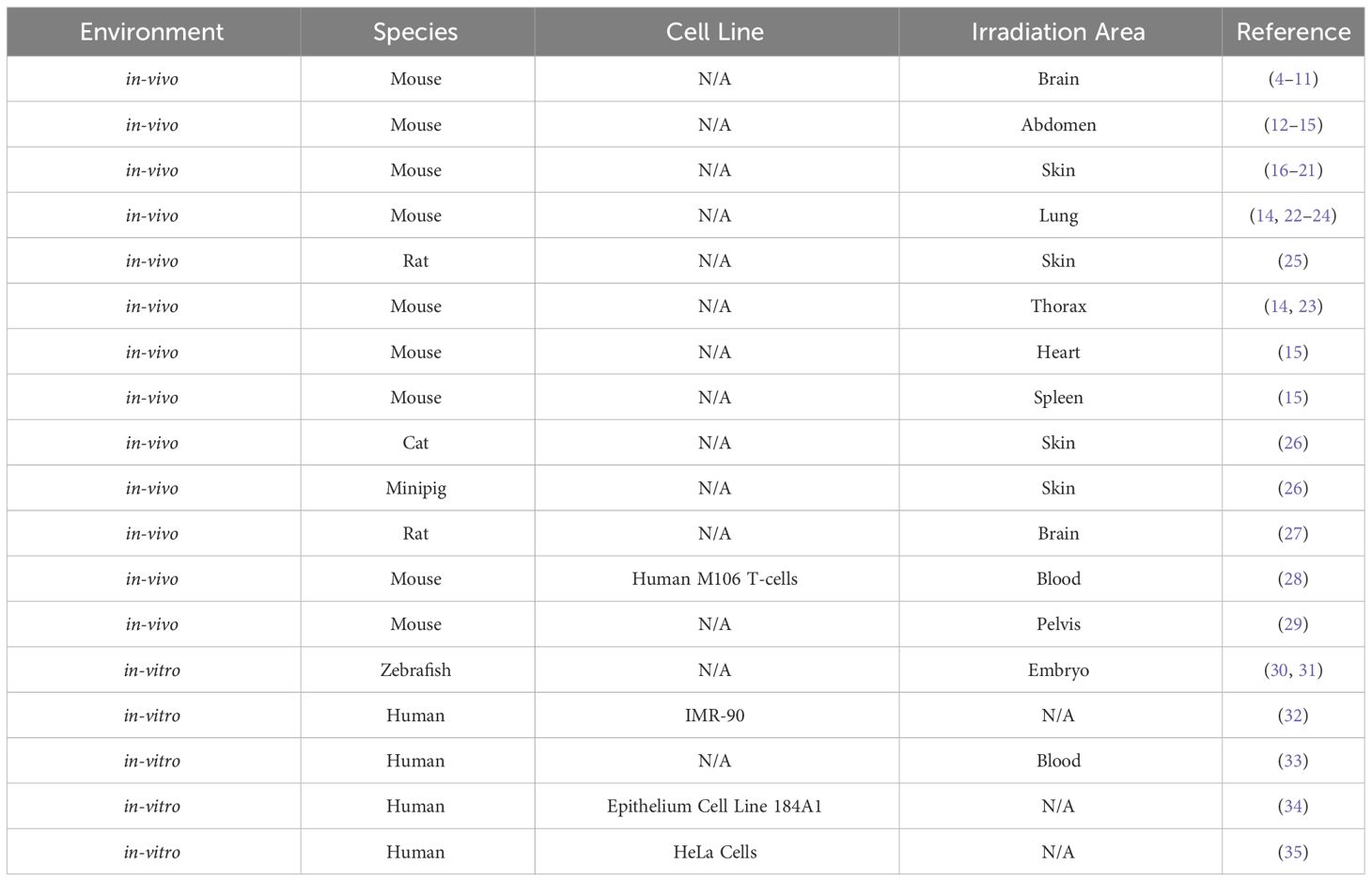
Table 2 A table of normal-tissue types in relevant experiments.
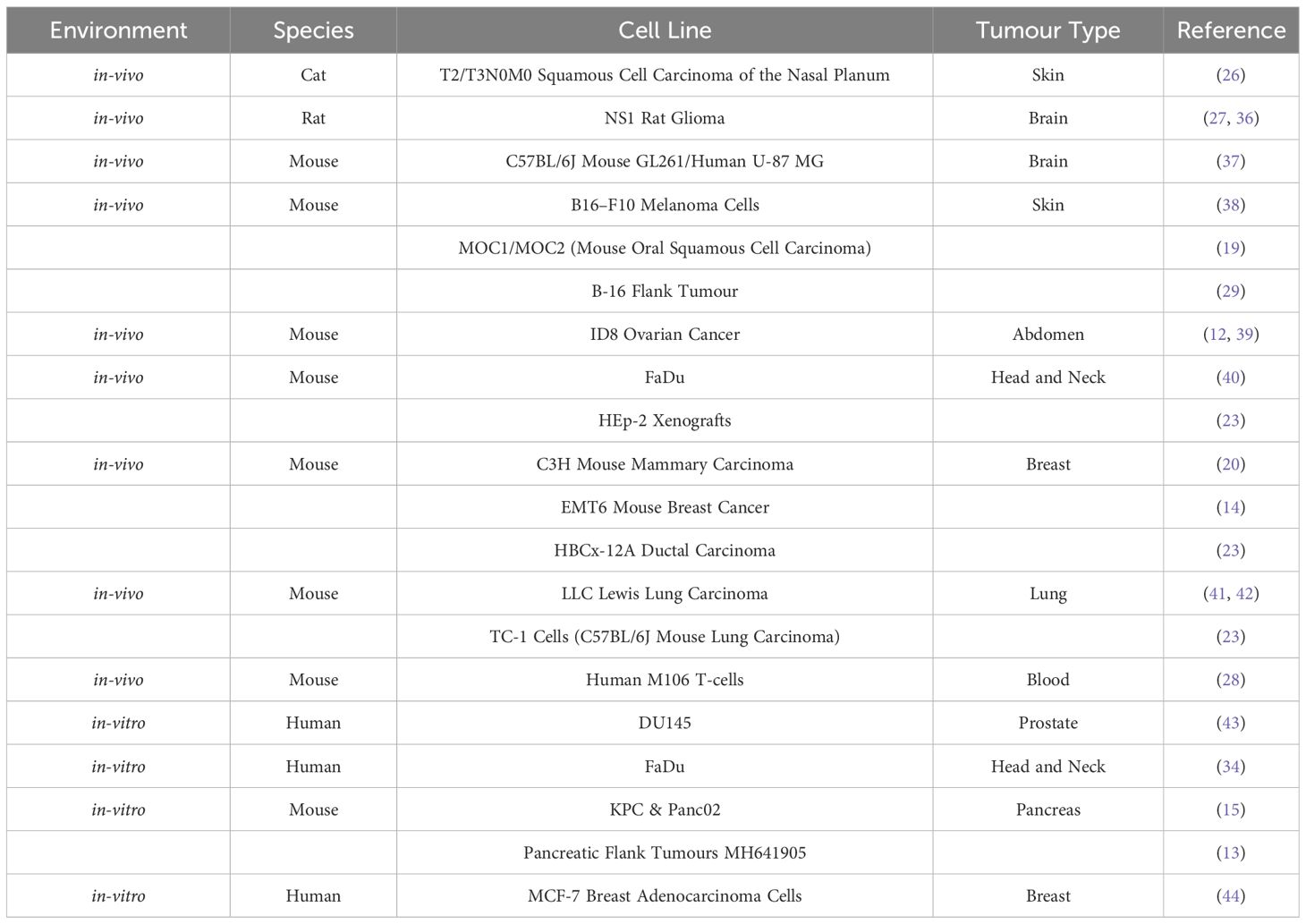
Table 3 A table of tumour types in relevant experiments.
2.2 Data analysis
A set of metrics was developed to allow a quantitative comparison of the varying endpoints that have been reported. Experiments which had determined the degree to which irradiation induced moist desquamation, impaired cognitive function, induced changes in stool level, tumour size, survival fractions, and/or caused fibrosis were included in this study. Unfortunately, each of these endpoints was examined in not more than one or two of the experiments. Scores were manually assigned to these endpoints to reflect the degree to which tumour control had been generated and normal-tissue sparing observed.
Some experiments did not record both tumour control and normal-tissue sparing, meaning that some scores had to be based on tumour control or normal-tissue sparing alone. Consequently, tumour response and normal-tissue sparing were individually scored. Scoring in this way would also account for the fact that larger doses and dose-delivery periods would typically result in more effective tumour control at the expense of increased normal-tissue toxicity. The criteria against which the scores were assigned in the two categories “Tumour Control” and “Normal-tissue Sparing” are defined in Table 4 . The end-point in each experiment was evaluated, converting the qualitative result into a number between 1 and 5 (fractional scores were awarded if the result fell between two categories). The overall score (to evaluate the degree to which FLASH-RT is effective as a radiotherapy treatment modality) was created by averaging the tumour-control and normal-tissue-sparing scores from experiments that evaluated both tumour control and normal-tissue sparing.
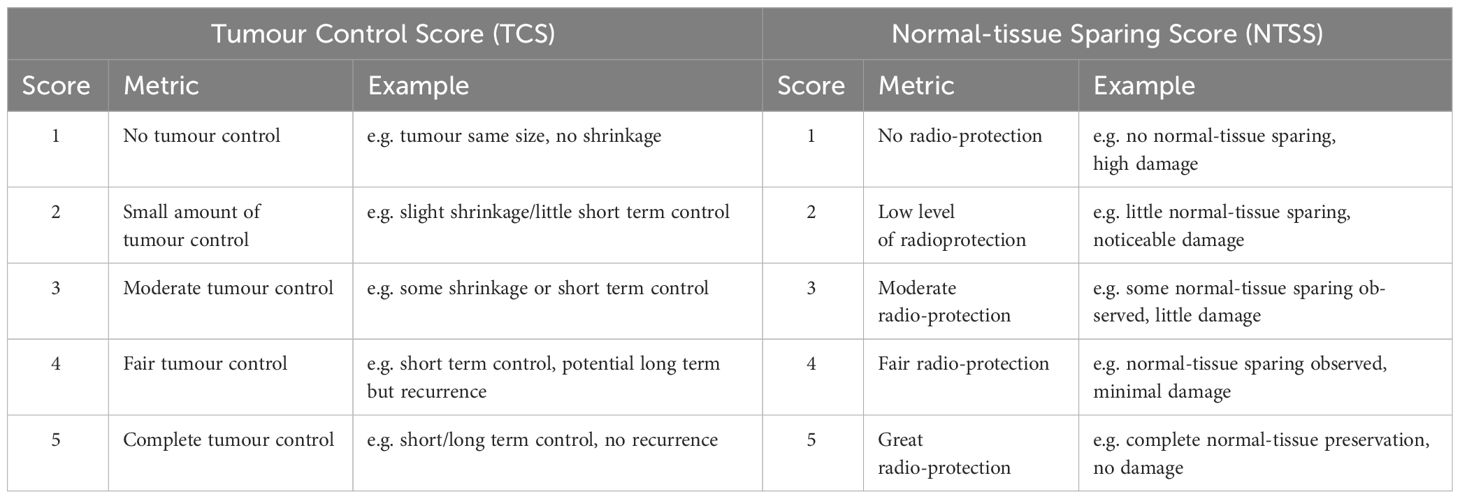
Table 4 Scoring system used to quantify the experimental biological responses.
In this paper, the quantitative tumour-control score is labelled “Tumour Control Score” or TCS, the normal-tissue-sparing score is labelled “Normal-tissue Sparing Score” or NTSS, and the overall score was labelled “Therapeutic Index Score” or TIS. The scores were used to evaluate the hypothesis that FLASH irradiation yields reduced normal-tissue sparing but maintains tumour control for each beam parameter identified in section 2.1. The database, including the calculated Therapeutic Index Score (with explanations of each score), is given in the Supplementary Materials .
The assessment of small animal survival in this review study allows for a comprehensive examination of the late-stage impacts of FLASH-RT, emphasising a focus beyond the commonly explored acute effects. This approach defines the two modes of survival statistics: “Survival Score” (S M ) and “Increased Lifespan” (ILS). S M refers to the percentage of survivors (S) still alive at different time points (M) after treatment and is defined by Equation 1 :
In this study, survivors were recorded at M = 1, 2 and 3 months for each experiment. ILS is defined in Equation 2 as the ratio of treated to untreated median survival time (MST):
where FLASH MST is the “median survival time”, the time at which the number of survivors drops below 50% post FLASH-RT and Control MST is the median survival time of the untreated group.
The bivariate Pearson’s correlation coefficient, r , was calculated to quantify the degree of any correlation between the chosen parameter and the FLASH response. Pearson’s correlation coefficient is given by Equation 3 :
where n is the number of measurements in the sample, the x i are the values of the beam parameters, the y i are the associated quantitative outcomes, and the mean of the x i and y i are x ¯ and y ¯ respectively. In the following, | r | > 0.5 is categorised as a “strong correlation”, r in the range 0.3<| r | ≤ 0.5 is categorised as a moderate correlation, and | r | ≤ 0.3 is categorised as a weak correlation.
A confidence-level analysis to establish the degree to which the null hypothesis, that the outcome is uncorrelated with the beam parameter, can be rejected was carried out by calculating the test statistic, t , given by Equation 4 :
where r is the Pearson correlation coefficient and n is the size of the sample.
For the null hypothesis, r = 0 and t follows the Student’s t distribution with n −2 degrees of freedom. The confidence level, or “ p -value”, was evaluated as the probability that a value with magnitude ≤ | t | would occur by chance. For this review study, statistical significance is characterised as a p-value of less than 0.05.
As a cross-check, the standard deviation of the data points from the value expected based on the null hypothesis ( σ null ), that the points and beam parameter are uncorrelated and the alternative hypothesis ( σ ), that the points are correlated with the beam parameter, were calculated. The standard deviations are defined by Equations 5 and 6 respectively:
where y est is the estimate of the end-point score obtained from the line of best fit, and n is the number of data points. If σ < σ null , it is more likely that the end point is correlated with the beam parameter, while, if σ null < σ , it is more likely that the data and beam parameter are uncorrelated.
To visually examine the trends observed in the data, each scored endpoint was evaluated and plotted as a function of the most significant beam parameter of the dataset. A straight line fit was performed on each graph to allow the correlation to be visualised. The straight-line fit was used to determine the 95% confidence interval CI and the 95% prediction interval PI calculated using Equations 7 and 8 respectively.:
where y ^ is the end point values, t crit is the statistic of interval confidence also known as the critical value of the t distribution [ ( 45 )], σ is the squared deviation of the end point values, x is an array of evenly spaced x values for the range of each beam parameter, x ¯ is the mean of the beam parameters. The expected distribution of the data is not known; therefore, this approach is primarily employed to investigate correlated trends and illustrate their spread, rather than presuming linearity or normal distribution of the data.
The experiments included in this study were carried out at 17 different beam lines and the data was collected using a wide variety of beam parameters. The value of some of the beam parameters varied significantly between experiments so the values of some of the beam parameters spanned a very wide range. It was therefore convenient to study the correlation of the various scored end-points for each beam parameter, x b , as well as for its logarithm, log 10 ( x b ), in order to compress the range of values and spread them out more evenly along the axis. The analysis of the data as a function of log 10 x b was more compelling for this reason (see Supplementary Materials ) and the analysis presented in this study will use log 10 x b .
3.1 Therapeutic Index Scores- TIS
The statistical analysis of the correlation of Therapeutic Index Scores (TIS) with the beam parameters is presented in Table 5 . TIS is most significantly correlated with Pulse Dose Rate ( r = 0.491). TIS is plotted as a function of Pulse Dose Rate in Figure 1 . The statistical significance for this correlation ( p = 0.038) combined with the value of σ being smaller than σ null validates the strength of the correlation, providing evidence of a potential FLASH effect. Pulse Dose is also statistically significant, with a p-value of p = 0.046 and moderate positive correlation of r = 0.476. σ is also much smaller than σ null for this correlation.
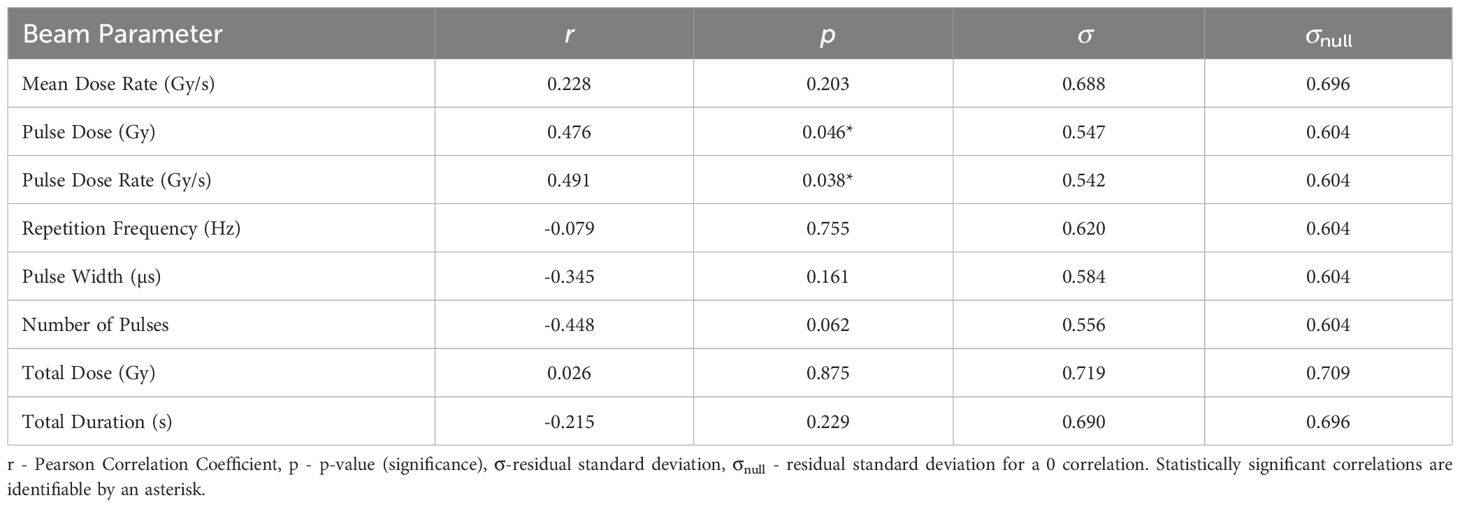
Table 5 Statistical analysis for TIS data.
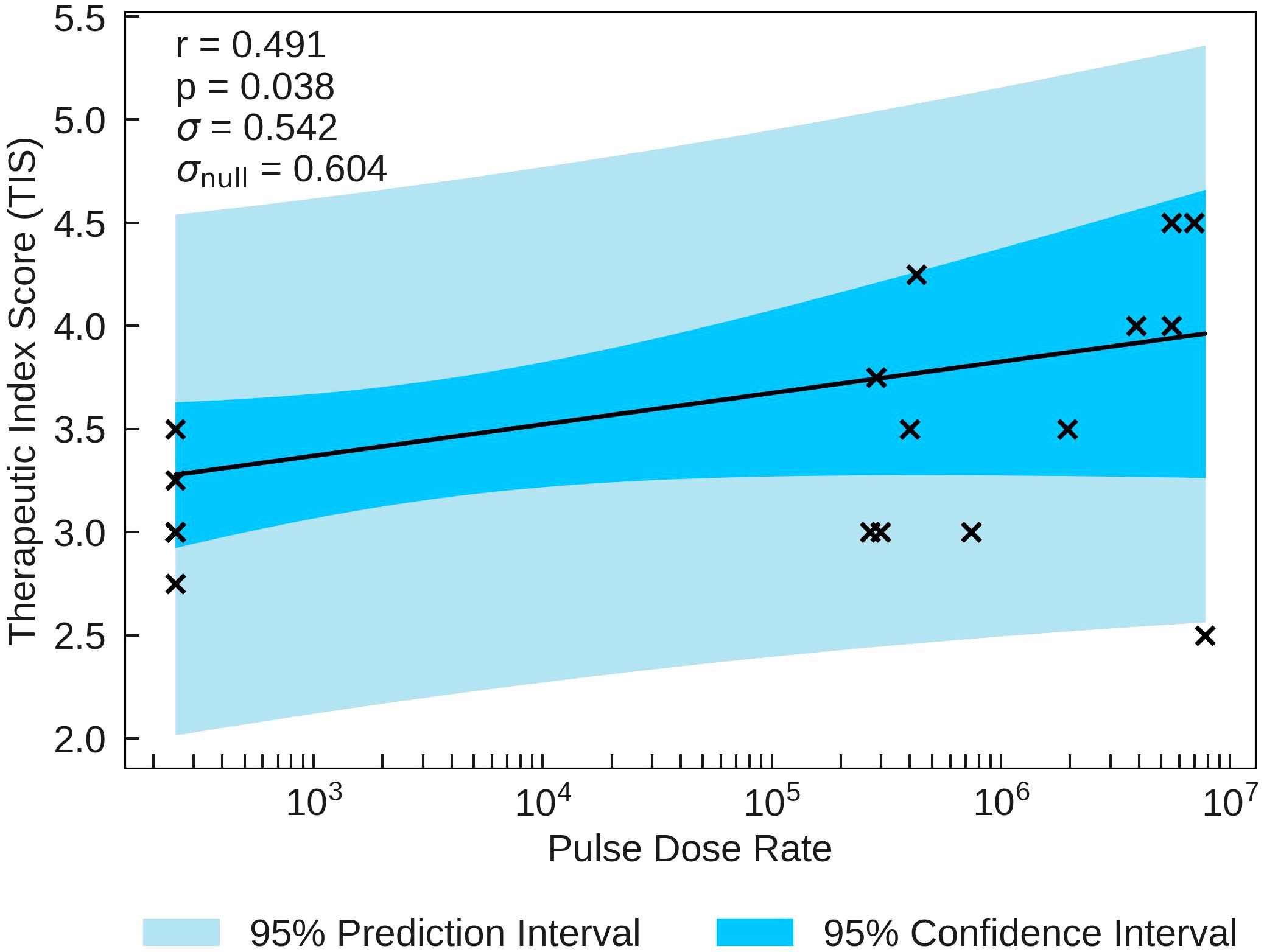
Figure 1 TIS plotted against the most significant and strongest beam parameter, Pulse Dose Rate. A positive correlation between the parameters suggests that an increase in dose rate will increase the chance of observing a higher therapeutic index, as predicted in section 2.
3.2 Tumour Control Scores- TCS
The correlations of TCS with the various beam parameters are illustrated in Table 6 . The most significant correlation
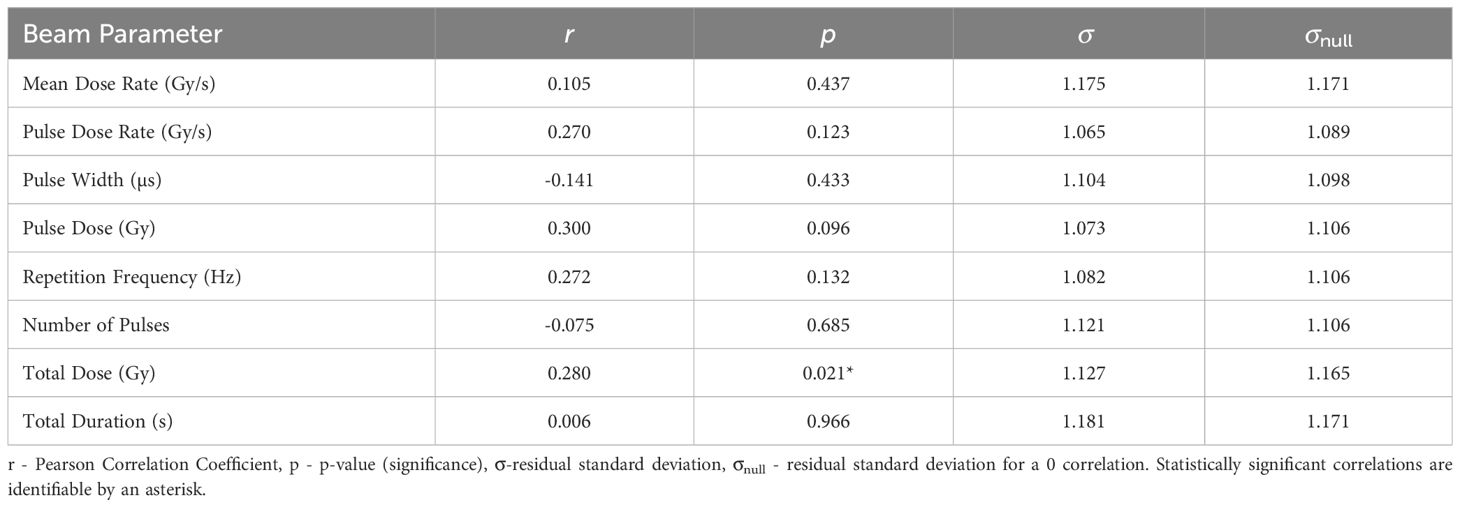
Table 6 Statistical analysis for TCS data.
between TCS and the beam parameters on a logarithmic scale is with Total Dose ( r = 0.280, p = 0.021). The σ value being smaller than σ null along with the statistical significance indicate that the correlation, while not strong, gives a reasonable description of the data. TCS is plotted as a function of Total Duration in Figure 2 . This correlation suggests that the tumour response is as expected in classical radiobiology, supported by the non-significant moderately positive correlation between Pulse Dose and TCS ( r = 0.300). Conversely, Pulse Dose Rate and TCS also held a non-significant weak positive correlation ( r = 0.270). With a stronger, statistically significant correlation, this would suggest that new radiotherapy models such as FLASH has the potential for effective tumour treatment.
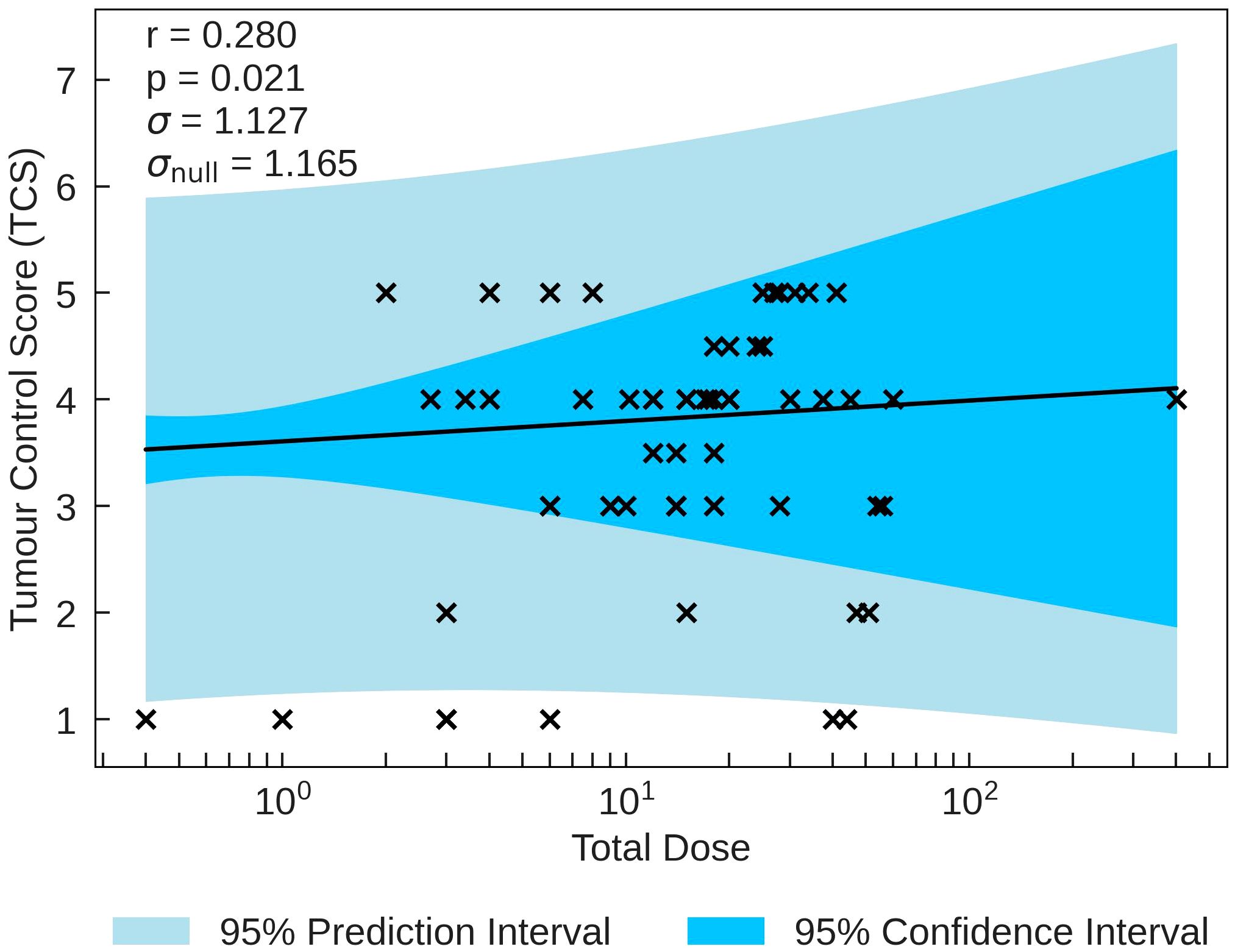
Figure 2 TCS plotted against the most significant and strongest beam parameter, Total Dose. A positive correlation between the parameters suggests that an increase in irradiation dose increases tumour control.
3.3 Normal-tissue Sparing Scores- NTSS
The statistics characterising the correlations of NTSS with the various parameters are summarised in Table 7 . NTSS has the most statistically significant correlation with Mean Dose Rate ( r = 0.286, p = 0.0001). This correlation, plotted in Figure 3 , suggests that an increase in the dose rate may increase the chance of observing sparing in normal-tissue, showing evidence of a FLASH effect, supported by NTSS statistically significant correlations with Pulse Dose Rate ( r = 0.226, p = 0.019) and Total Duration ( r = −0.222, p = 0.004). This is challenged by the two other statistically significant NTSS correlations; Number of Pulses ( r = −0.410, p = 0.001) and Pulse Dose ( r = 0.367, p = 0.001), suggesting attributes of conventional therapy are sufficient for tissue sparing.
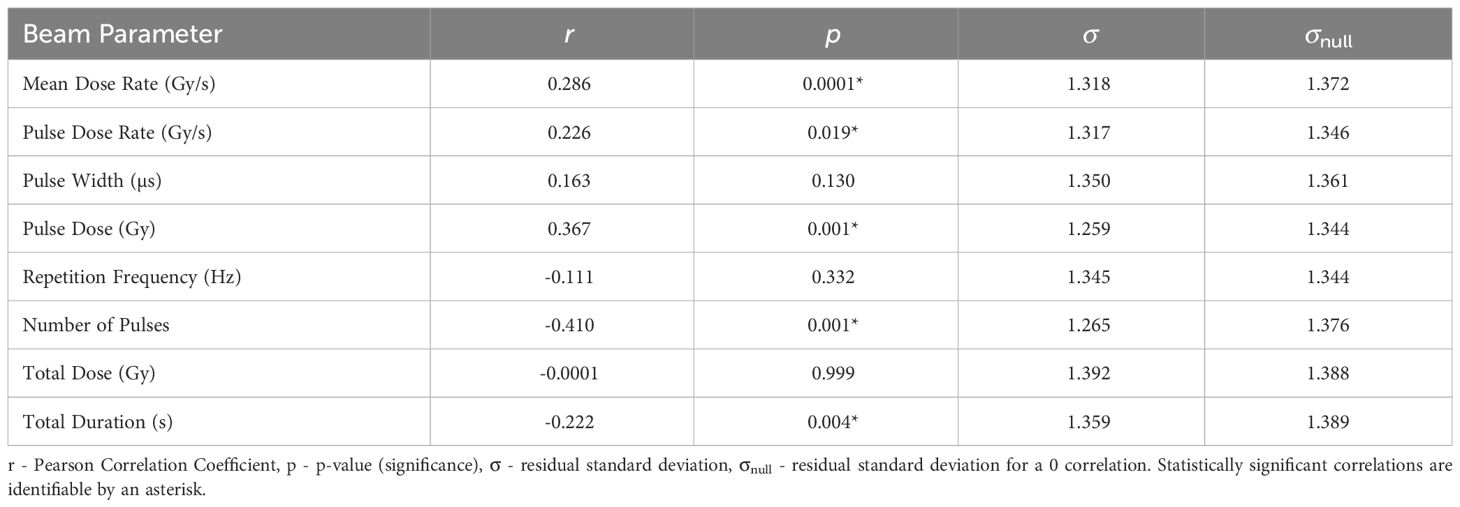
Table 7 Statistical analysis for Normal-tissue Sparing scored data.
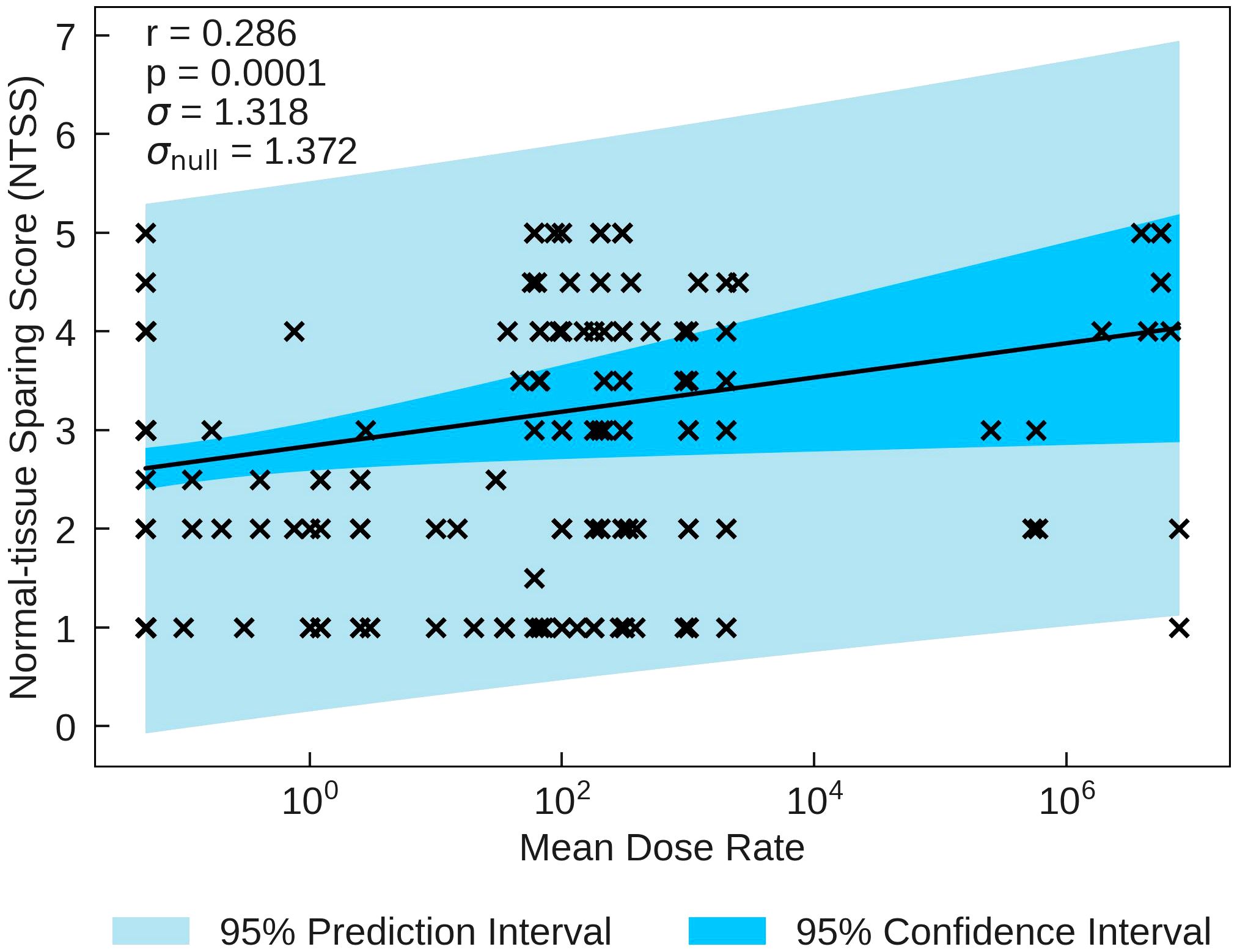
Figure 3 NTSS plotted against the most statistically significant beam parameter, Mean Dose Rate. A positive correlation between the parameters suggests that a decrease in pulse rate may increase the chance of observing a sparing effect in normal tissue.
3.4 Increased Lifespan Score- ILS
The statistics characterising the ILS correlation with the various parameters are summarised in Table 8 . The most statistically significant ILS correlation is with Total Dose ( r = 0.539, p = 0.017), closely followed by Total Duration ( r = 0.536, p = 0.022. These correlations are plotted in Figure 4 . The σ values for these correlations are less than σ null . In conjunction with other correlations presented in Table 8 , the results suggest that higher doses and dose times yield greater lifespan increases. This also suggests that ILS is dominated by tumour control.
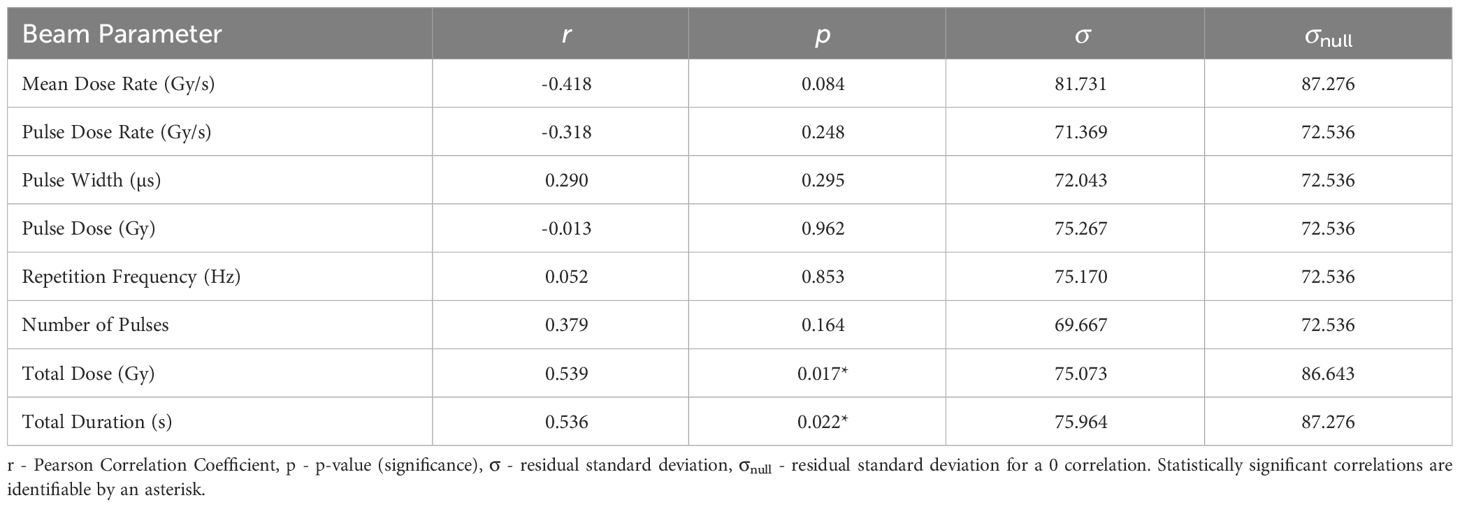
Table 8 Statistical analysis for Increased Lifespan.
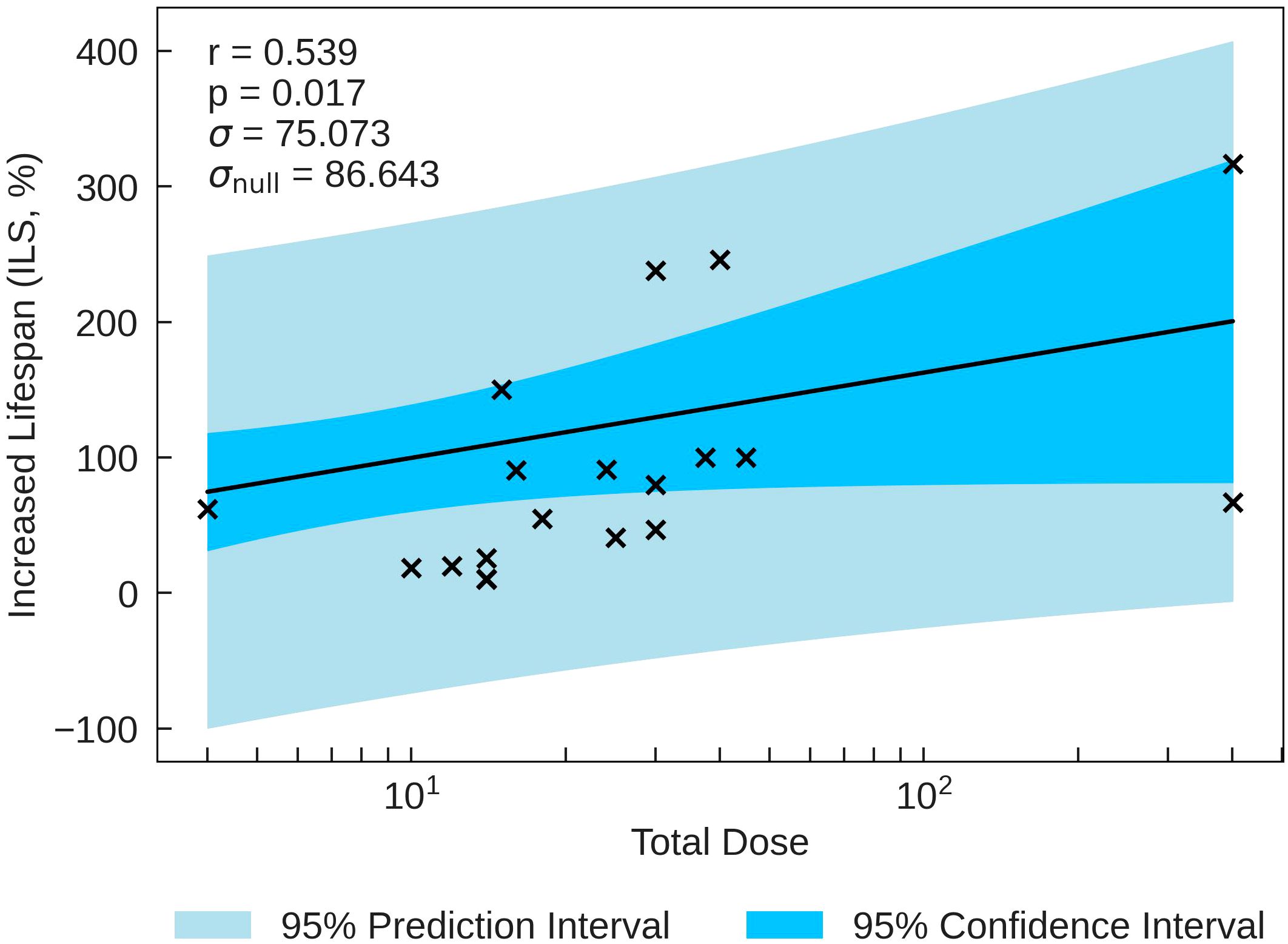
Figure 4 ILS plotted against the most significant and strongest beam parameter, Total Dose. A strong positive correlation between the parameters implies that an increase in dose can increase the lifespan of small animals.
3.5 Survival Score- SS
The statistics characterising the correlation of the Survival Score (SS) at 3 months post FLASH-RT with the various parameters are summarised in Table 9 . SS is most significantly correlated with Number of Pulses ( r = 0.709, p = 0.0002), plotted in Figure 5 . The correlations with Pulse Dose and Mean Dose Rate are also statistically significant ( r = −0.600, p =0.003 and r =−0.442, p =0.035 respectively), suggesting that there is a negative relation between the survival of small animals and dose rates at 3 months.
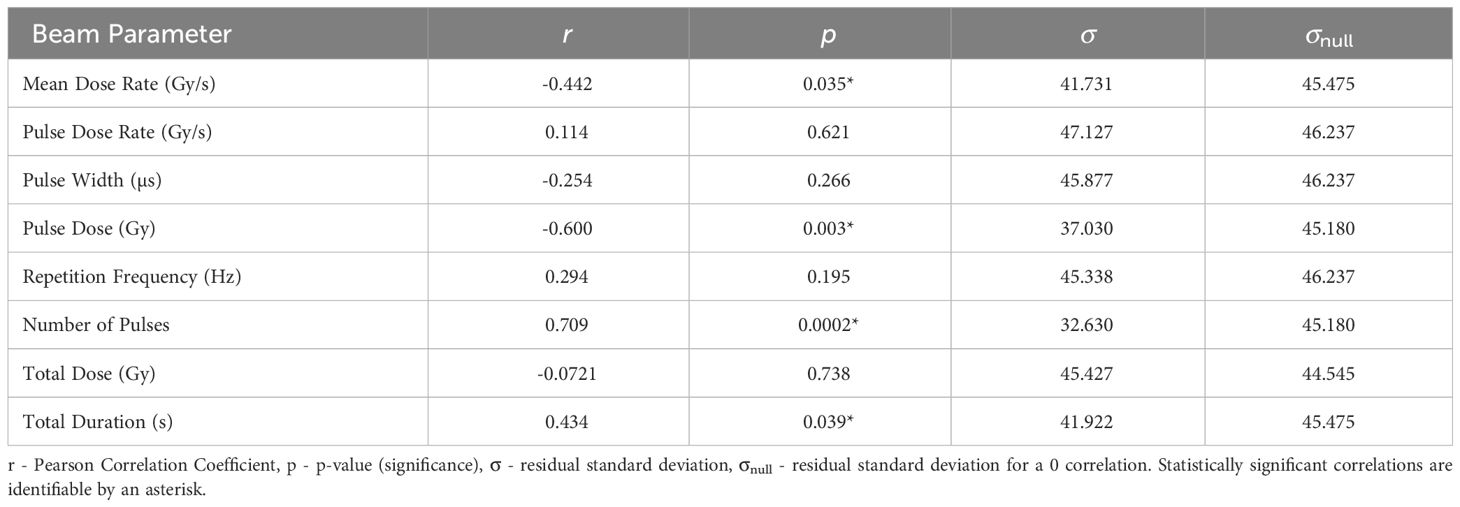
Table 9 Statistical analysis for survivors at 3 months.
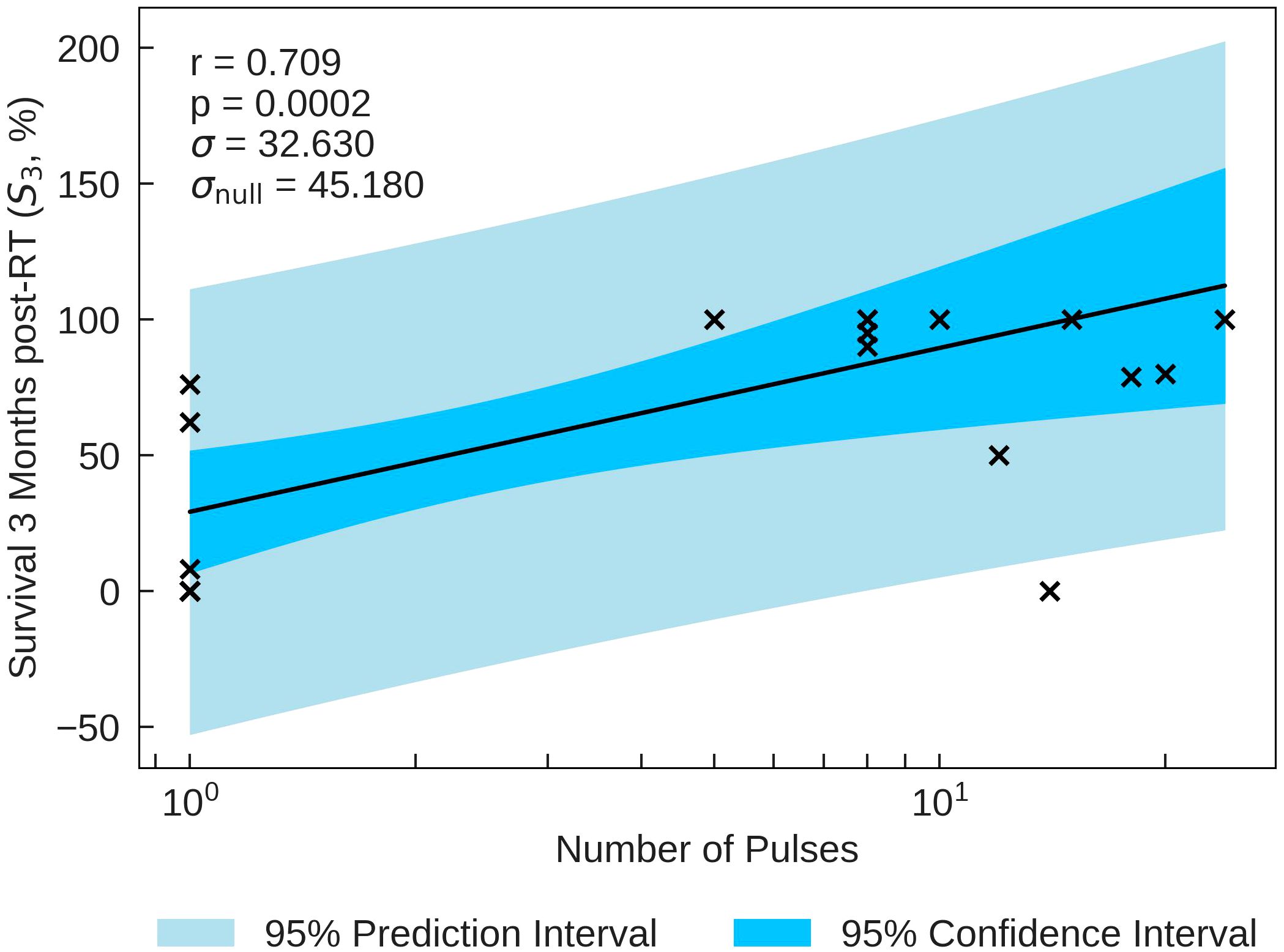
Figure 5 S 3 percentage plotted against the most significant and strongest beam parameter, Number of Pulses. A strong positive correlation between the parameters implies that an increase in the Number of Pulses can increase the survival time of small animals.
3.6 Discussion
The FLASH effect is an intriguing radiobiological effect, holding promise to revolutionise radiotherapy. However, the exact conditions to activate the FLASH effect are still unclear. For that purpose, a retrospective evaluation has been carried out to study the FLASH dependence on beam parameters.
This review study highlighted that TIS, TCS, and NTSS showed low correlations with most of the beam parameters. A possible reason for the observed low correlation was explored. This was the presence of a dose-rate threshold for the onset of the FLASH effect. For example, Boreham et al. ( 46 ) first explored the dose rate thresholds in early 2000. Their study demonstrated that decreasing the dose rate from 702mGy/min to 2.9 mGy/min had a negligible impact on the induction of lymphocyte apoptosis. However, once the dose rate surpassed 1.5 mGy/min, the rate of lymphocyte apoptosis was significantly reduced. This effect was thought to be related to the presence of slowly repaired lesions. The authors concluded that the linear relationship between the dose rate is only present above a minimum dose rate.
In the most recent studies, the threshold for the onset of the FLASH effect is estimated to be 30Gy/s ( 47 ), 35Gy/s or 40Gy/s ( 48 ). For the data used in the present study, around 20% of the data points of all the FLASH studies use Mean Dose Rates below these thresholds. Therefore, it was considered that the dose rate may not be high enough for some data points for the FLASH effect to be observed. The combination of the data taken at dose rates above and below the FLASH threshold may dilute any observed linear correlation. It is therefore possible that some of the data included in the study lies below the FLASH threshold and that as a result no FLASH effect can be observed. Moreover, it is important to determine whether the FLASH threshold depends on other beam parameters such as particle type, total dose etc. To investigate the possible impact of a threshold, subsets of the data obtained at dose rates above 30Gy/s and above 40Gy/s were analysed separately (see Supplementary Materials ). The trends observed were consistent with each other and with the trends observed in the full sample. This was equally apparent when examining Figures 1 , 3 . There is no therefore evidence for a threshold effect in the data included in the present review study and all data have been retained in analyses presented above.
In order to demonstrate a full picture of all data, trends or thresholds for isolated parameters were not investigated in this review study. With no knowledge of a distribution, the linear regression analysis, confidence/prediction intervals and correlation coefficients are presented to look for an overarching trend rather than a study based on specific distributions. All data for trend testing, searching for potential thresholds (e.g. Figure 1 ) or removing statistical outliers for certain distributions that could drive correlations (e.g. Figure 3 ) can be found in the Supplementary Materials .
An overview of the data is presented in Figure 6 , where the Pearson correlation coefficients characterising the relationship between the logarithmic scored endpoints and the beam parameters are presented.
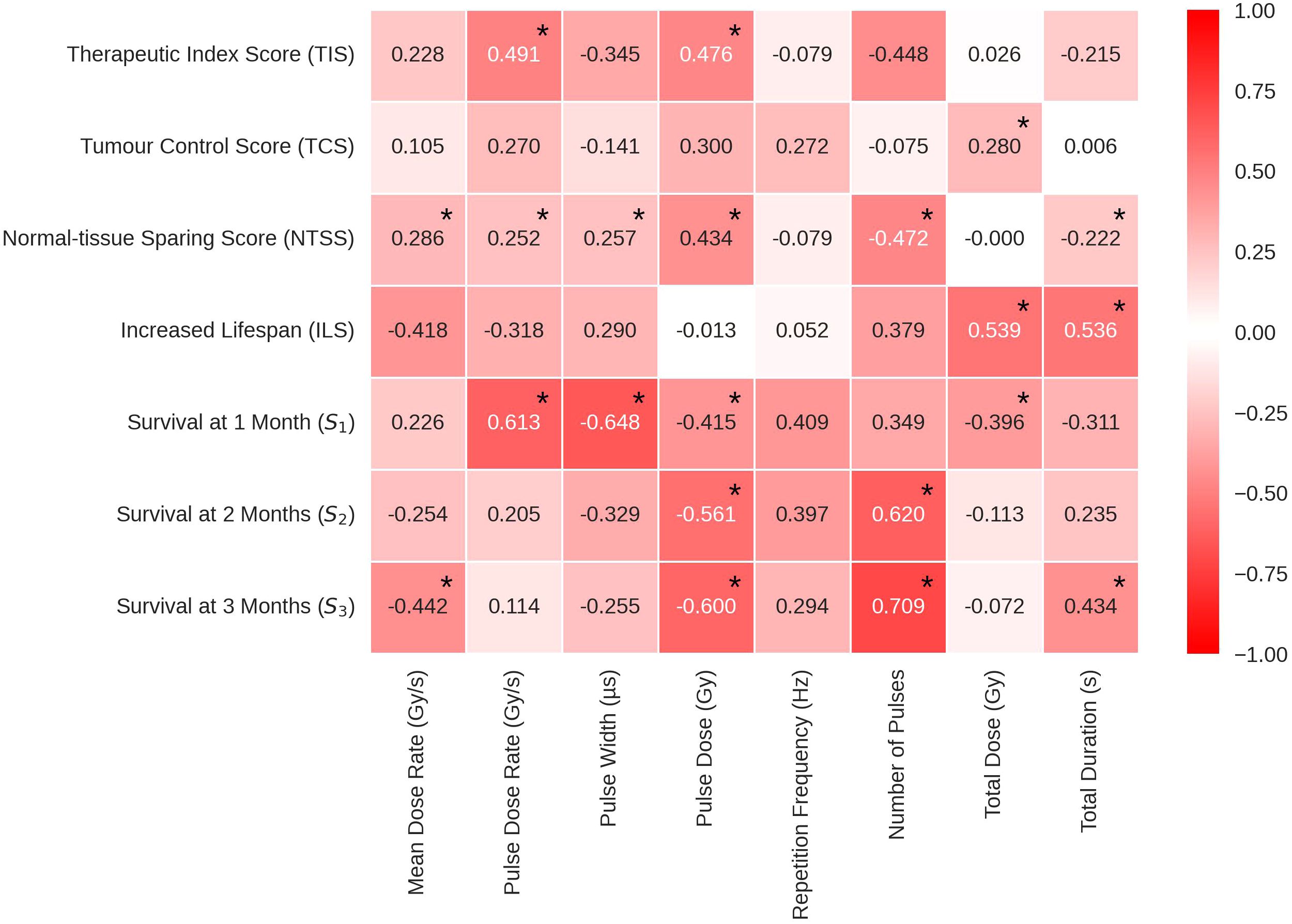
Figure 6 Pearson’s correlation coefficients in heat map form to show the correlations between the log of each beam parameter and the corresponding endpoint. The values range between -1 and 1, where the extremities (closest to -1 and 1) have the deepest colour and the weakest correlations (closer to 0) have a weak colour. Statistically significant correlations are identifiable by an asterisk at the top right of the corresponding correlation coefficient. TIS, Therapeutic Index Score; TCS, Tumour Control Score; NTSS, Normal-tissue Sparing Score; ILS, Increased Lifespan; S 1 , Survival % at 1 month; S 2 , Survival % at 2 month; S 3 , Survival % at 3 month.
The cells in Figure 6 are colour coded to indicate the strength of the correlation. The most significant TIS correlations were with Pulse Dose Rate and Pulse Dose, both positive, suggesting that a high pulse dose and dose rate increases therapeutic index. Pulse Dose Rate demonstrated positive correlations with nearly all endpoints, excluding ILS. This pattern suggests the potential viability of FLASH-RT as an effective and less toxic modality for radiotherapy. TCS and NTSS results suggest that beam parameter qualities from both FLASH and conventional radiotherapy can be useful to spare tissue and treat the tumour effectively.
While TCS correlations remained weak to moderate for all beam parameters, the correlation with Total Dose remains statistically significant. The correlation indicates that, in the data considered here, a larger dose administered to the tumour results in a higher level of tumour control and following a more classical model. Weak to moderate correlations are also observed for NTSS, having 5 statistically significant correlations. Thereby the FLASH effect showed statistically significant (although weak to moderate) correlations with Number of Pulses, Mean Dose Rate, Pulse Dose Rate, Pulse Dose and Total Duration. The NTSS correlations suggest that normal-tissue sparing in FLASH therapy can be favoured by both the use of short pulses with high dose rates and high doses with less pulses. These results suggest FLASH-RT could preserve tissue using a low amount of short, high dose pulses.
Negative correlations of ILS with Mean Dose Rate and Pulse Dose Rate are observed. Multivariate analyses carried out to investigate the correlation between the beam parameters displayed a strong positive relationships between these two parameters (see Supplementary Materials ). This is expected, assuming most accelerators do not have extensive lapses between pulses. In addition, the ILS logged data shows statistically significant, strong positive correlations with Total Dose and Total Duration, suggesting that the parameters of tumour control weighted more in this case.
The percentage of survivals at short term (S 1 - 1 month post-RT) correlates positively with Pulse Dose Rate (the higher the pulse dose rate, the higher the percentage of survivals) and negatively with Pulse Width suggesting that these parameters are possibly related to the acute toxicity. This was not reproduced in the long term. For correlations with percentage of survivals at 3 months post-RT (S 3 ), both Mean Dose Rate and Pulse Dose Rate held statistically significant negative correlations.
In addition, Pulse Dose Rate had a statistically significant negative correlation while Total Duration and Number of Pulses had a statistically significant positive correlation. In conjunction with the TCS scores, it appears that the survival difference long term (3 months post-RT) could be due to continuous beams having a more efficient tumour control. Observing the differences at 1 and 3 months post-RT, it appears that the variation in the time at which the endpoints are observed may be an additional source of inconsistency in the homogeneity of the manually scored data.
A recent study of the impact of FLASH-RT on glioblastoma investigated survival trends at 3 months ( 49 ). In order to compare the results of the present study with that presented in ( 49 ), glioblastoma studies were extracted from the S M database and survival was examined at 3 months. Survival at three months (S 3 ) was averaged separately for ultrahigh dose rate irradiations (dose rate greater than 30Gy/s) and compared to the average S 3 for conventional irradiations (CONV) of the same dose. It was found that the average survival at 3 months was very similar for FLASH and CONV, resulting in 54.8 ± 7.7% and 54.3 ± 7.6% respectively (see Supplementary Materials ). This suggests that the survival response for this type of tumour is similar for both radiation modalities. Despite these results not being significant, the indication that FLASH may have a slightly higher response is similar to the conclusion drawn in the recently published study by Böhlen et al. ( 49 ).
4 Conclusion
The FLASH effect is thought to reduce the adverse toxicities during the radiation process. This review study presents an extensive analysis of experiments including investigations of FLASH-RT and its potential influences. A semi-quantitative approach was developed to assess each study, wherein the normal-tissue preservation and tumour-control capabilities were evaluated considering the outcomes of each experiment. The study establishes a correlation between ultra-high dose rates and the observed FLASH effect. This is evident through the significant associations found between Normal-tissue Sparing Score and Therapeutic Index Score with Pulse Dose Rate.
Additional data extrapolation was carried out to enable survival to be studied in order to evaluate the papers with a set endpoint. The Survival Score correlations are indicative of a short term sparing effect and a long term tumour control efficiency. This is seen in the data at 1-month, where both Mean Dose Rate and Pulse Dose Rate exhibit positive correlations and then observing how the relationship reverses for 2 and 3 months post FLASH-RT. This phenomenon suggests a delicate balance between normal-tissue sparing and effective tumour control. In addition, the Increased Lifespan data provides further support, indicating a significant positive correlation with both Total Dose and Total Duration. These findings underscore the need for higher doses and extended radiation times for comprehensive tumour treatment.
Data availability statement
The original contributions presented in the study are included in the article/ Supplementary Material . Further inquiries can be directed to the corresponding authors.
Author contributions
JM: Writing – original draft, Writing – review & editing, Data curation, Formal analysis, Methodology. KL: Writing – original draft, Writing – review & editing, Supervision, Validation. YP: Writing – original draft, Writing – review & editing, Supervision.
The author(s) declare financial support was received for the research, authorship, and/or publication of this article. The work described here was carried out within the joint National de la Recherche Scientifique (CNRS)-Imperial College London (ICL) International Research Centre. The research was made possible by grants from CNRS, ICL and the UKRI Science and Technology Facilities Council and received funding from the European Research Council (ERC) under the European Union’s Horizon 2020 research and innovation program (Grant Agreement No 817908).
Acknowledgments
We gratefully acknowledge all sources of support. Special thanks are extended to Anthea MacIntosh-LaRocque for her invaluable contribution in initiating the development of the searchable database during her undergraduate study at Imperial College London and to the ITRF/LhARA collaborators for their insight and support.
Conflict of interest
The authors declare that the research was conducted in the absence of any commercial or financial relationships that could be construed as a potential conflict of interest.
Publisher’s note
All claims expressed in this article are solely those of the authors and do not necessarily represent those of their affiliated organizations, or those of the publisher, the editors and the reviewers. Any product that may be evaluated in this article, or claim that may be made by its manufacturer, is not guaranteed or endorsed by the publisher.
Supplementary material
The Supplementary Material for this article can be found online at: https://www.frontiersin.org/articles/10.3389/fonc.2024.1340190/full#supplementary-material
1. Hughes J, Parsons J. FLASH Radiotherapy: Current Knowledge and Future Insights Using Proton-Beam255 Therapy. Int J Molecular Sciences . (2020) 6492. doi: 10.3390/ijms21186492
CrossRef Full Text | Google Scholar
2. Lin B, Gao F, Yang Y, Wu D, Zhang Y, Feng G, et al. FLASH Radiotherapy: History andFuture. Front Oncol . (2021) 11. doi: 10.3389/fonc.2021.644400
3. Vozenin M-C, Hendry JH, Limoli CL. Biological benefits of ultra-high dose rate FLASH radiotherapy: Sleeping beauty awoken. Clin Oncol (R Coll Radiol.) . (2019) 31:407–15. doi: 10.1016/j.clon.2019.04.001
PubMed Abstract | CrossRef Full Text | Google Scholar
4. Montay-Gruel P, Petersson K, Jaccard M, Boivin G, Germond J-F, Petit B, et al. Irradiation in a flash: Unique sparing of memory in mice after whole brain irradiation with dose rates above 100 Gy/s. Radiother Oncol . (2017) 124:365–9. doi: 10.1016/j.radonc.2017.05.003
5. Montay-Gruel P, Acharya MM, Petersson K, Alikhani L, Yakkala C, Allen BD, et al. Long-term neurocognitive benefits of FLASH radiotherapy driven by reduced reactive oxygen species. Proc Natl Acad Sci U. S. A . (2019) 116:10943–51. doi: 10.1073/pnas.1901777116
6. Simmons DA, Lartey FM, Schüler E, Rafat M, King G, Kim A, et al. Reduced cognitive deficits after FLASH irradiation of whole mouse brain are associated with less hippocampal dendritic spine loss and neuroinflammation. Radiother. Oncol . (2019) 139:4–10. doi: 10.1016/j.radonc.2019.06.006
7. Montay-Gruel P, Bouchet A, Jaccard M, Patin D, Serduc R, Aim W, et al. X-rays can trigger the FLASH effect: Ultra-high dose-rate synchrotron light source prevents normal brain injury after whole brain irradiation in mice. Radiother Oncol . (2018) 129:582–8. doi: 10.1016/j.radonc.2018.08.016
8. Montay-Gruel P, Acharya MM, Gonçalves Jorge P, Petit B, Petridis IG, Fuchs P, et al. Hypofractionated FLASH-RT as an effective treatment against glioblastoma that reduces neurocognitive side effects in mice. Clin Cancer Res . (2021) 27:775–84. doi: 10.1158/1078-0432.CCR-20-0894
9. Alaghband Y, Cheeks SN, Allen BD, Montay-Gruel P, Doan N-L, Petit B, et al. Neuroprotection of radiosensitive juvenile mice by ultra-high dose rate FLASH irradiation. Cancers (Basel) . (2020) 12:1671. doi: 10.3390/cancers12061671
10. Montay-Gruel P, Markarian M, Allen BD, Baddour JD, Giedzinski E, Jorge PG, et al. Ultra-high-dose-rate FLASH irradiation limits reactive gliosis in the brain. Radiat Res . (2020) 194:636–45. doi: 10.1667/RADE-20-00067.1
11. Limoli CL, Kramár EA, Almeida A, Petit B, Grilj V, Baulch JE, et al. The sparing effect of FLASH-RT on synaptic plasticity is maintained in mice with standard fractionation. Radiother Oncol . (2023) 186:109767. doi: 10.1016/j.radonc.2023.109767
12. Levy K, Natarajan S, Wang J, Chow S, Eggold JT, Loo P, et al. FLASH irradiation enhances the therapeutic index of abdominal radiotherapy for the treatment of ovarian cancer. bioRxiv (2019). doi: 10.1101/2019.12.12.873414
13. Diffenderfer ES, Verginadis II, Kim MM, Shoniyozov K, Velalopoulou A, Goia D, et al. Design, implementation, and in vivo validation of a novel proton FLASH radiation therapy system. Int J Radiat Oncol Biol Phys . (2020) 106:440–8. doi: 10.1016/j.ijrobp.2019.10.049
14. Gao F, Yang Y, Zhu H, Wang J, Xiao D, Zhou Z, et al. First demonstration of the FLASH effect with ultrahigh dose rate high-energy X-rays. Radiother Oncol . (2022) 166:44–50. doi: 10.1016/j.radonc.2021.11.004
15. Venkatesulu BP, Sharma A, Pollard-Larkin JM, Sadagopan R, Symons J, Neri S, et al. Ultra high dose rate (35 Gy/sec) radiation does not spare the normal tissue in cardiac and splenic models of lymphopenia and gastrointestinal syndrome. Sci Rep . (2019) 9:17180. doi: 10.1038/s41598-019-53562-y
16. Hendry J, Moore J, Hodgson B, Keene J. The Constant Low Oxygen Concentration in All the Target Cells305 for Mouse Tail Radionecrosis. Radiat Res (1982) 92:172–181. doi: 10.2307/3575852
17. Soto LA, Casey KM, Wang J, Blaney A, Manjappa R, Breitkreutz D, et al. FLASH irradiation results in reduced severe skin toxicity compared to conventional-dose-rate irradiation. Radiat Res . (2020) 194:618–24. doi: 10.1667/RADE-20-00090
18. Inada T, Nishio H, Amino S, Abe K, Saito K. High dose-rate dependence of early skin reaction in mouse. Int J Radiat Biol Relat Stud Phys Chem Med . (1980) 38:139–45. doi: 10.1080/09553008014551031
19. Cunningham S, McCauley S, Vairamani K, Speth J, Girdhani S, Abel E, et al. FLASH proton pencil beam scanning irradiation minimizes radiation-induced leg contracture and skin toxicity in mice. Cancers (Basel) . (2021) 13:5. doi: 10.3390/cancers13051012
20. Sørensen BS, Sitarz MK, Ankjærgaard C, Johansen JG, Andersen CE, Kanouta E, et al. Pencil beam scanning proton FLASH maintains tumor control while normal tissue damage is reduced in a mouse model. Radiother Oncol . (2022) 175:178–84. doi: 10.1016/j.radonc.2022.05.014
21. Rudigkeit S, Schmid TE, Dombrowsky AC, Stolz J, Bartzsch S, Chen C-B, et al. Proton-FLASH: effects of ultra-high dose rate irradiation on an in-vivo mouse ear model. Sci Rep . (2024) 14:1418. doi: 10.1038/s41598-024-51951-6
22. Fouillade C, Curras-Alonso S, Giuranno L, Quelennec E, Heinrich S, Bonnet-Boissinot S, et al. FLASH irradiation spares lung progenitor cells and limits the incidence of radio-induced senescence. Clin Cancer Res . (2020) 26:1497–506. doi: 10.1158/1078-0432.CCR-19-1440
23. Favaudon V, Caplier L, Monceau V, Pouzoulet F, Sayarath M, Fouillade C, et al. Ultrahigh dose-rate FLASH irradiation increases the differential response between normal and tumor tissue in mice. Sci Transl Med . (2014) 6:245ra93. doi: 10.1126/scitranslmed.3008973
24. Kim Y-E, Gwak S-H, Hong B-J, Oh J-M, Choi H-S, Kim MS, et al. Effects of ultra-high doserate FLASH irradiation on the tumor microenvironment in Lewis lung carcinoma: Role of myosin light chain. Int J Radiat Oncol Biol Phys . (2021) 109:1440–53. doi: 10.1016/j.ijrobp.2020.11.012
25. Field S, Bewley D. Effects of dose-rate on the radiation response of rat skin. Int J Radiat Biol Related Stud Physics Chem Med . (1974) 26:259–67. doi: 10.1080/09553007414551221
26. Vozenin M, De Fornel P, Petersson K, Favaudon V, Jaccard M, Germond J, et al. The advantage of FLASH radiotherapy confirmed in mini-pig and cat-cancer patients. Clin Cancer Res. (2019) 25:35–42. doi: 10.1158/1078-0432.CCR-17-3375
27. Konradsson E, Liljedahl E, Gustafsson E, Adrian G, Beyer S, Ilaahi SE, et al. Comparable long-term tumor control for hypofractionated FLASH versus conventional radiation therapy in an immunocompetent rat glioma model. Adv Radiat Oncol . (2022) 7:101011. doi: 10.1016/j.adro.2022.101011
28. Chabi S, Van To TH, Leavitt R, Poglio S, Jorge PG, Jaccard M, et al. Ultra-high-dose-rate FLASH and conventional-dose-rate irradiation differentially affect human acute lymphoblastic leukemia and normal hematopoiesis. Int J Radiat Oncol Biol Phys . (2021) 109:819–29. doi: 10.1016/j.ijrobp.2020.10.012
29. Cao N, Erickson DPJ, Ford EC, Emery RC, Kranz M, Goff P, et al. Preclinical ultra-high dose rate (FLASH) proton radiotherapy system for small animal studies. Adv Radiat Oncol . (2023) 9:101425. doi: 10.1016/j.adro.2023.101425
30. Beyreuther E, Brand M, Hans S, Hideghéty K, Karsch L, Leßmann E, et al. Feasibility of proton FLASH effect tested by zebrafish embryo irradiation. Radiother. Oncol . (2019) 139:46–50. doi: 10.1016/j.radonc.2019.06.024
31. Karsch L, Pawelke J, Brand M, Hans S, Hideghéty K, Jansen J, et al. Beam pulse structure and dose rate as determinants for the flash effect observed in zebrafish embryo. Radiother Oncol . (2022) 173:49–54. doi: 10.1016/j.radonc.2022.05.025
32. Buonanno M, Grilj V, Brenner DJ. Biological effects in normal cells exposed to FLASH dose rate protons. Radiother Oncol . (2019) 139:51–5. doi: 10.1016/j.radonc.2019.02.009
33. Cooper CR, Jones D, Jones GD, Petersson K. FLASH irradiation induces lower levels of DNA damage ex vivo, an effect modulated by oxygen tension, dose, and dose rate. Br J Radiol . (2022) 95:20211150. doi: 10.1259/bjr.20211150
34. Laschinsky L, Baumann M, Beyreuther E, Enghardt W, Kaluza M, Karsch L, et al. Radiobiological effectiveness of laser accelerated electrons in comparison to electron beams from a conventional linear accelerator. J Radiat Res . (2012) 53:395–403. doi: 10.1269/jrr.11080
35. Auer S, Hable V, Greubel C, Drexler GA, Schmid TE, Belka C, et al. Survival of tumor cells after proton irradiation with ultra-high dose rates. Radiat Oncol . (2011) 6:139. doi: 10.1186/1748-717X-6-139
36. Liljedahl E, Konradsson E, Gustafsson E, Jonsson KF, Olofsson JK, Ceberg C, et al. Long-term anti-tumor effects following both conventional radiotherapy and FLASH in fully immunocompetent animals with glioblastoma. Sci Rep . (2022) 12. doi: 10.1038/s41598-022-16612-6
37. Almeida A, Godfroid C, Leavitt RJ, Montay-Gruel P, Petit B, Romero J, et al. Anti-tumor effect by either FLASH or conventional dose rate irradiation involves equivalent immune responses. Int J Radiat Oncol Biol Phys . (2023). doi: 10.1016/j.ijrobp.2023.10.031
38. Fernandez-Palomo C, Trappetti V, Potez M, Pellicioli P, Krisch M, Laissue J, et al. Complete remission of mouse melanoma after temporally fractionated Microbeam Radiotherapy. Cancers (Basel) . (2020) 12:2656. doi: 10.3390/cancers12092656
39. Levy K, Natarajan S, Wang J, Chow S, Eggold JT, Loo PE, et al. Abdominal FLASH irradiation reduces radiation-induced gastrointestinal toxicity for the treatment of ovarian cancer in mice. Sci Rep . (2020) 10:21600. doi: 10.1038/s41598-020-78017-7
40. Zlobinskaya O, Siebenwirth C, Greubel C, Hable V, Hertenberger R, Humble N, et al. The effects of ultra-high dose rate proton irradiation on growth delay in the treatment of human tumor xenografts in nude mice. Radiat Res . (2014) 181:177–83. doi: 10.1667/RR13464.1
41. Rama N, Saha T, Shukla S, Goda C, Milewski D, Mascia A, et al. Improved tumor control through T-cell infiltration modulated by ultra-high dose rate proton FLASH using a clinical pencil beam scanning proton system. Int J Radiat Oncol Biol Physics . (2019) 105:S164–5. doi: 10.1016/j.ijrobp.2019.06.187
42. Shukla S, Saha T, Rama N, Acharya A, Le T, Bian F, et al. Ultra-high dose-rate proton FLASH improves tumor control. Radiother Oncol . (2023) 186:109741. doi: 10.1016/j.radonc.2023.109741
43. Adrian G, Konradsson E, Lempart M, Bäck S, Ceberg C, Petersson K. The FLASH effect depends on oxygen concentration. Br J Radiol . (2020) 93:20190702. doi: 10.1259/bjr.20190702
44. Sumini M, Previti A, Galassi D, Ceccolini E, Rocchi F, Mostacci D, et al. Analysis and characterization of the X-ray beam produced by a PF device for radiotherapy applications. Xray Spectrom . (2015) 44:289–95. doi: 10.1002/xrs.2621
45. Confidence/prediction intervals— Real Statistics Using Excel. Available at: real-statistics.com. (Accessed 09-Jun-2023).
Google Scholar
46. Boreham DR, Dolling J-A, Maves SR, Siwarungsun N, Mitchel REJ. Dose-rate effects for apoptosis and micronucleus formation in gamma-irradiated human lymphocytes. Radiat Res . (2000) 153:579–86. doi: 10.1667/0033-7587(2000)153[0579:DREFAA]2.0.CO;2
47. Garty G, Obaid R, Deoli N, Royba E, Tan Y, Harken AD, et al. Ultra-high dose rate FLASH irradiator at the radiological research accelerator facility. Sci Rep . (2022) 12:1. doi: 10.1038/s41598-022-19211-7
48. Durante M, Brauer-Krisch E, Hill M. Faster and safer? FLASH ultra-high dose rate in radiotherapy. Br J Radiol . (2017) 91:20170628. doi: 10.1259/bjr.20170628
49. Böhlen TT, Germond J-F, Petersson K, Ozsahin EM, Herrera FG, Bailat C, et al. Effect of conventional and ultra-high dose rate “FLASH” irradiations on preclinical tumour models: A systematic analysis: Tumour response to CONV and UHDR irradiation. Int J Radiat Oncol Biol Phys . (2023) 116:1007–101. doi: 10.1016/j.ijrobp.2023.05.045
Keywords: ultra-high dose rate irradiation, ultra-high dose rate, FLASH-RT, normal-tissue sparing, FLASH, radiotherapy, therapeutic index, radiation - adverse effects
Citation: McGarrigle JM, Long KR and Prezado Y (2024) The FLASH effect—an evaluation of preclinical studies of ultra-high dose rate radiotherapy. Front. Oncol. 14:1340190. doi: 10.3389/fonc.2024.1340190
Received: 17 November 2023; Accepted: 20 March 2024; Published: 22 April 2024.
Reviewed by:
Copyright © 2024 McGarrigle, Long and Prezado. This is an open-access article distributed under the terms of the Creative Commons Attribution License (CC BY) . The use, distribution or reproduction in other forums is permitted, provided the original author(s) and the copyright owner(s) are credited and that the original publication in this journal is cited, in accordance with accepted academic practice. No use, distribution or reproduction is permitted which does not comply with these terms.
*Correspondence: Josie May McGarrigle, [email protected] ; Kenneth Richard Long, [email protected] ; Yolanda Prezado, [email protected]
Disclaimer: All claims expressed in this article are solely those of the authors and do not necessarily represent those of their affiliated organizations, or those of the publisher, the editors and the reviewers. Any product that may be evaluated in this article or claim that may be made by its manufacturer is not guaranteed or endorsed by the publisher.
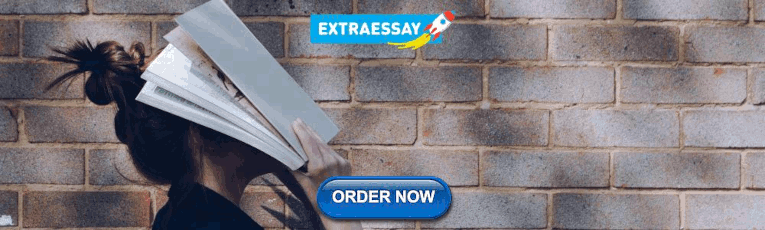
IMAGES
VIDEO
COMMENTS
Study with Quizlet and memorize flashcards containing terms like 1. The null hypothesis represents which of the following statements? a. No relationship between the variables under study b. A positive relationship between the independent and dependent variables c. A negative relationship between the independent and dependent variables d. A difference between the variables under study, 2 ...
The null hypothesis represents which of the following statements? A. No relationship between the variables under study. The significance level reported in a research study can be explained by? C. Risk associated with not being 100% confident the difference is due to the treatment. About us. About Quizlet; How Quizlet works;
Basic definitions. The null hypothesis and the alternative hypothesis are types of conjectures used in statistical tests to make statistical inferences, which are formal methods of reaching conclusions and separating scientific claims from statistical noise.. The statement being tested in a test of statistical significance is called the null hypothesis. . The test of significance is designed ...
The actual test begins by considering two hypotheses.They are called the null hypothesis and the alternative hypothesis.These hypotheses contain opposing viewpoints. H 0, the —null hypothesis: a statement of no difference between sample means or proportions or no difference between a sample mean or proportion and a population mean or proportion. In other words, the difference equals 0.
The actual test begins by considering two hypotheses.They are called the null hypothesis and the alternative hypothesis.These hypotheses contain opposing viewpoints. \(H_0\): The null hypothesis: It is a statement of no difference between the variables—they are not related. This can often be considered the status quo and as a result if you cannot accept the null it requires some action.
The null and alternative hypotheses offer competing answers to your research question. When the research question asks "Does the independent variable affect the dependent variable?": The null hypothesis ( H0) answers "No, there's no effect in the population.". The alternative hypothesis ( Ha) answers "Yes, there is an effect in the ...
Typically, the null hypothesis includes an equal sign. The null hypothesis states that the population parameter equals a particular value. That value is usually one that represents no effect. In the case of a one-sided hypothesis test, the null still contains an equal sign but it's "greater than or equal to" or "less than or equal to."
Null Hypothesis Examples. "Hyperactivity is unrelated to eating sugar " is an example of a null hypothesis. If the hypothesis is tested and found to be false, using statistics, then a connection between hyperactivity and sugar ingestion may be indicated. A significance test is the most common statistical test used to establish confidence in a ...
It is the opposite of your research hypothesis. The alternative hypothesis--that is, the research hypothesis--is the idea, phenomenon, observation that you want to prove. If you suspect that girls take longer to get ready for school than boys, then: Alternative: girls time > boys time. Null: girls time <= boys time.
The null hypothesis represents which of the following statements? Select one: a. a negative relationship between the independent and dependent variables b. no relationship between the variables under study c. a positive relationship between the independent and dependent variables d. a difference between the variables under study.
B. If the P-value is less than 0.05, the decision is to reject the null hypothesis. Otherwise, we fail to reject the null hypothesis. C. If the test statistic falls within the critical region, the decision is to reject the null hypothesis. D. If P-valueless than or equals≤alphaα , the decision is to reject the null hypothesis.
Which of the following is most likely to represent a statement of the null hypothesis? А. Но: н > 0 Ho: M < 0 Ho: M# 0 Ho: M = 0; Like. 0. All replies. Answer. 1 day ago. The null hypothesis is a general statement or default position that there is no relationship between two measured phenomena, or no association ...
See Answer. Question: Which of the following statements about the null hypothesis is false? a. It is the first of two opposing hypotheses in any hypothesis test. b. It is a vehicle for making startling new claims that contradict the conventional wisdom. c. It is a description of the status quo. d. It is the first of two opposing hypotheses in ...
The null hypothesis represents which of the following statements? a) no relationship between the variables under study b) a positive relationship between the independent and dependent variables c) a negative relationship between the independent and dependent variables d) a difference between the variables under study
where r is the Pearson correlation coefficient and n is the size of the sample.. For the null hypothesis, r = 0 and t follows the Student's t distribution with n−2 degrees of freedom.The confidence level, or "p-value", was evaluated as the probability that a value with magnitude ≤ |t| would occur by chance.For this review study, statistical significance is characterised as a p-value ...
Which of the following statements is FALSE regarding the "null hypothesis". a) It ideally includes a specific, quantitative statement that is falsifiable. b) Failing to reject it necessarily means that it is true. c) It is typically the statement that would be interesting to reject. d) It typically represents the status quo.
See Answer. Question: Which of the following statements is false? A. When the alternative hypothesis is two-sided, the null hypothesis is rejected for values of the test statistic located in either tail of that statistic's sampling distribution B. When the alternative hypothesis is two-sided, any hypothesis test is said to be a two-tailed test C.