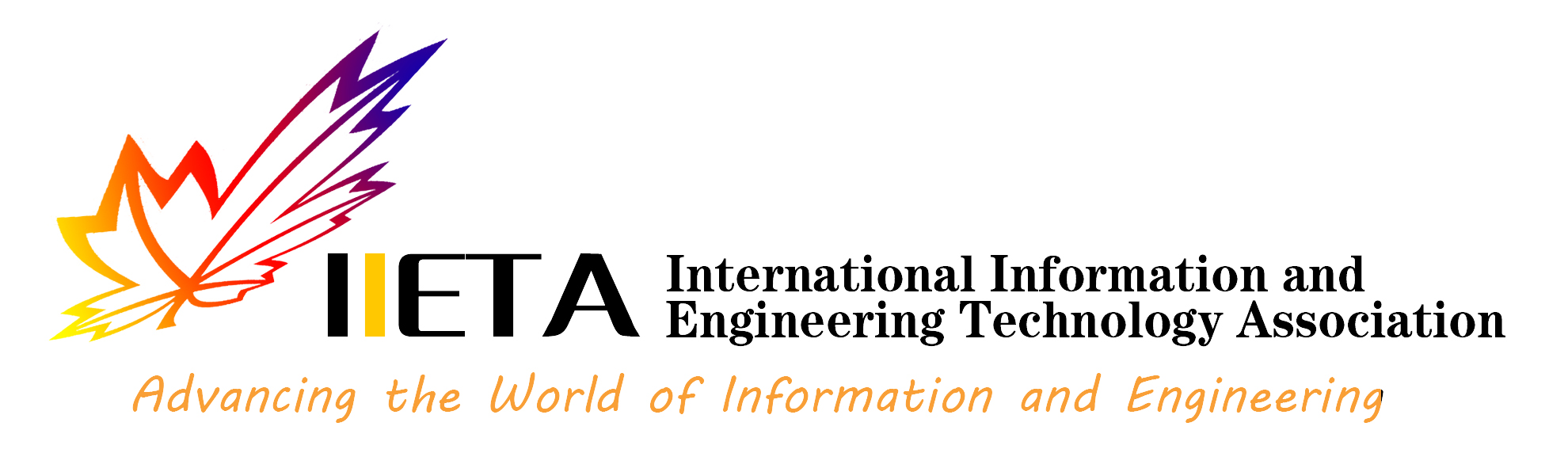
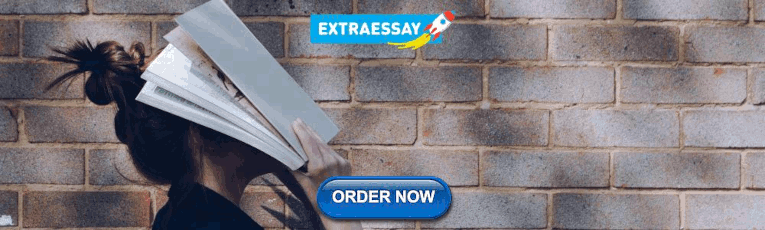
Search form
A real-time machine learning-based public transport bus-passenger information system.
Menzi Skhosana | Absalom E. Ezugwu *
© 2021 IIETA. This article is published by IIETA and is licensed under the CC BY 4.0 license ( http://creativecommons.org/licenses/by/4.0/ ).
OPEN ACCESS
The problems faced daily by the public transportation sector can be addressed or mitigated by collecting appropriate data and applying predictive analytics. This paper primarily focuses on problems affecting the public transport buses. These include the unavailability of real-time information to commuters about the current status of a given bus or travel route; and the inability of bus operators to efficiently assign available buses to routes for a given day based on expected demand for a particular route. A cloud-based system is developed to address the aforementioned issues. The proposed system comprises two subsystems, namely mobile and web applications interfaces. The mobile application interface provides commuters with the current location and availability of a given bus and other related information, and it is also used by drivers so that the bus can be tracked in real-time and collect ridership information throughout the day. Moreover, the web application serves as a dashboard for bus operators to gain insights from the collected ridership data. The new integrated system was developed using the Firebase Backend-as-a-Service (BaaS) platform and integrated with a machine learning model trained on collected ridership data to predict the daily ridership for a given route. The novel system provides a holistic solution to problems in the public transport sector. It is highly scalable, cost-efficient, and takes full advantage of the current technologies compared to other related application platforms.
bus location tracking, mobile tracking, short-term forecasting
Public transportation efficiency is essential for the economic growth and sustainability of urban areas. However, in developing countries, there are little or no advancements being made in this sector. The sizable capacity of public transport vehicles results in fewer trips and reduced fuel consumption.
According to Statistics South Africa, more than 76.7% of South African households rely on public transport for daily commutes [1]. This again highlights the importance of public transport and why problems in this sector need to be addressed. Stockin, in his article [2], outlines that the development of physical infrastructure, e.g. more highways or bigger roads, does not make traffic congestion better but may worsen it; this is a phenomenon known as the Induced Travel Demand (ITD). This leads to the point that the development of proper infostructure - which is defined as an electronic infrastructure that enables sharing knowledge and information among various societal actors [3] - is just as important. This has led to the recent trend and drive to modernize public transportation by integrating it with the latest technologies for more viable and eco-friendly environments. However, this trend has only been more prevalent in developed countries around the world and not so much in still developing countries like South Africa. This lag in developing countries is because many cities lack financial resources to implement these technologies [4]. Furthermore, it is noteworthy that approximately 55% of the world's population resides in urban areas, and this figure is expected to increase to 68% by the year 2050 [5].
Moreover, according to the Independent Communications Authority of South Africa (ICASA), smartphone usage has increased rapidly in the past five years. As of September 2019, 91.2% of the South African population owned a smartphone, which is nearly a 10% increase from the previous year. ICASA also reports on the state of the ICT sector in South Africa in terms of internet access coverage across the country, with the national population coverage for 3G mobile connection having increased from 99.5% in 2018 to 99.7% in 2019, and the coverage for 4G/LTE increased from 85.7% in 2018 to 92.8% in 2019 [6]. Given the widespread availability of smartphones and relatively good network coverage, smartphones as a platform for implementing a public transport management system (as part of the infostructure) will allow for easy access to transit information and lower implementation costs favouring developing countries.
Commuters - mostly in developing countries - are often left stranded at pickup spots and clueless about the availability and proximity of their mode of the public vehicle hence the bad stigma public transport has. This results from the unavailability of real-time information to commuters, poorly managed fleets, varying demands, traffic congestion and rigid schedules. Rapid population growth and urbanization lead to overwhelming travel demands [4, 7]. A better understanding of the commuters' behaviour helps transport operators estimate the demand and propose better services to improve the commuting experience [8]. However, in developing countries, the public sector's finances are generally so minimal that funding for transportation innovation is insufficient [9]. This calls for a cost-efficient and quickly implementable public transport solution to cater for these specific conditions. Previous work done in this sector [10-13] partially offers solutions to the challenges mentioned above but still lacks practicability. They require cumbersome hardware modules to be installed in buses, the use of outdated technologies with complicated implementation and high maintenance and lack of scalability.
This research demonstrates how a pragmatic intelligent public transport management system (Irenbus) can be developed and implemented, especially in South Africa and other developing countries. To achieve the aim set for the proposed study, this research has been divided into the following specific objectives:
- Design and develop an Android mobile application to inform commuters about the current status of a given bus through live location tracking.
- Develop an approach to collect daily ridership data using only a mobile application without external hardware modules.
- Develop an approach to incorporate a continuously trained machine learning model to forecast daily ridership per route.
- Design and develop a web-based application to monitor buses and drivers and present the machine learning model's predictions for a given route to bus operators.
- Evaluate the feasibility of the proposed system (Irenbus).
Furthermore, this research is focused on the design and implementation of a low-cost intelligent real-time public transport system that could be easily deployed in developing countries. However, for this research's purpose, the system's implementation and evaluation will mainly focus on buses, with three user roles - the bus user or commuter, the bus driver, and lastly, the bus manager or operator overseeing everything. The proposed Irenbus system could be considered as a holistic and intelligent real-time public transport system that keeps commuters informed about the current location and estimated arrival time of a given bus, while collecting daily ridership data that is used to provide predictive capabilities through the use of machine learning that renders valuable insights based on the ridership patterns to public transport authorities.
In summary, the technical contributions of this research are as follows: first, a system that provides a communication portal between bus users, drivers and operators and serves as a data management tool to store and organize collected daily ridership data intelligently. This system is accessible in a mobile application for bus users and drivers and a web application for bus operators [14]. The second is an approach to better incorporate machine learning models into the system to understand the collected data and foresight [15]. Third, an approach to efficiently collect daily ridership data, unlike some previous work in this topic [16], that uses only a mobile application without using cumbersome hardware modules.
The remaining part of this paper is structured as follows: Section 2 looks into related work in both published academic work and software products in the industry. Section 3 describes the overall structure of the proposed system - Irenbus. Section 4 presents the results of qualitative and quantitative evaluation of the new real-time public transport system. Section 5 provides a summary of the current work and describes the contribution of this research. We also explain the resulting Irenbus system prototype and how it relates to previous work and the objectives of this research, the limitations of the current system, and possible improvements in future work.
2.1 Bus tracking
Using GPS modules and embedded mini-computer systems, in [10], a bus monitoring system was developed that provided commuters with real-time information such as the estimated bus arrival time and the route name. This information was displayed on an LCD screen mounted at the bus stop. Although this system was stable and provided useful information to commuters, it had a few shortfalls, such as being fixed at one place, i.e. it could not provide information to commuters who were not at the bus stop. Furthermore, implementing this system would be costly since every bus stop had an LCD screen mounted.
A Web-based system was developed for tracking buses in transit using a GPS tracker installed on the bus. Users received real-time information straight to their mobile phones. Using Google Maps, users could see the bus on the map as it moved [13]. Their system ensured punctuality - which has been proven to be an essential factor in making buses more reliable [17]. Their system also seemed to be less expensive to implement than other previously done work but still lacked ease of use - as it only offered a web application with no native mobile application, which did not give insight to public transport authorities about future demands and ridership patterns.
2.2 Ridership forecasting
In Bacciu et al. [18], the authors discussed how a machine learning approach could be used to implement and assess predictive services for the users of a bike-sharing system. The models used in this study were trained on real-world historical usage data comprising more than 280 000 entries covering all hires in Pisa for two years. Seasonality manifests sharp changes in the usage patterns (e.g. bikes tend to be used more in spring than winter). The learning models captured these seasonal patterns by appropriately encoding the bike usage time, which explicitly modelled cyclic information such as weekdays and holidays.
In Hsu et al. [19], a system was proposed that used a camera mounted overhead to count passengers by combining a Convolutional Neural Network detection model and a spatiotemporal context model to address the counting problem in low resolution scenes and with a variation of illumination, pose and scale. Experimental results showed better performance than previously published results, and they planned to extend the current method with more in-depth learning algorithms. The only drawback to their system was that it might not work in a very dense and crowded scene, and in that case, they planned to explore crowd density map estimation for their future work.
In van Oort et al. [20], the authors explored using smart card data for performing simple analyses by using transport planning software. The data was converted to represent passengers per line and matrixes between stops. This matrix was then taken into the network to produce the measured passenger flows. Their method turned out to be valuable to operators to gain insights into small changes but could not give accurate insights into long-term changes.
2.3 Implemented real-world systems
Over the past few years, the City of Cape Town has put in efforts to improve its public transport by introducing the MyCiTi bus service. This service has a mobile application to accompany it that offers access to live updates to track the arrival of buses and allows users to conveniently save their favourite routes, stops and destinations for quick and easy access [21]. This led McCann et al. [22] to claim that Cape Town has the best public transport service in Africa. Nevertheless, it still follows a rigid schedule and does not collect ridership data from forecasting future ridership.
A software-as-a-service platform, Optibus, leverages artificial intelligence and historical data to predict and analyze on-time performance. It then automatically generates running times to help schedulers and operations executives create better schedules. Better on-time performance improves the reliability of the transit service - one of the main factors shown to increase ridership [23]. This software offers excellent insight and flexibility with schedules but is focused only on scheduling and ridership prediction and does not cater for commuters directly by providing relevant information and tracking.
To the best of the authors' knowledge, there has not been any published work that discusses how a holistic public transportation system can be designed and developed to relay useful transit information amongst commuters, drivers and bus operators while at the same time collecting and studying commuter boarding data to enable the prediction of future ridership patterns, and hence allowing for the development of better and more efficient bus schedules by integrating machine learning forecasting abilities.
3.1 System architecture
The proposed system is composed of two subsystems, viz. the mobile subsystem and the web subsystem. The mobile system is presented in an Android application platform and has two users, namely, commuters and bus drivers. Commuters use the application to get real-time information about the current status of buses in transit. Bus drivers use the application to capture daily ridership data; also, the live location of the device will be sent periodically in the background throughout the bus trip. The web subsystem is in the form of a web application. The bus operators use it to get a detailed view of all buses in transit, perform administrative tasks and view ridership forecasts for a given route.
The machine learning model is continuously trained with the daily ridership data collected by the mobile application, and the resulting model is used in the web application to forecast ridership, as shown in Figure 1.
The proposed system aimed to take full advantage of cloud-based services. Cloud computing allows for the delivery of different services through the Internet, including data storage, servers, databases and networking infrastructure [24]. A myriad of benefits come with cloud services, including cost savings, security, mobility, competitive edge, and sustainability. For the proposed system, we used Google's Firebase, which is a Backend-as-a-Service platform. Firebase was chosen over Amazon Web Services' AppSync and other similar platforms because it allows both mobile and web applications access to shared data and computing infrastructures at lower costs and requires minimal setup and maintenance. Firebase provides real-time syncing of data across all the devices - Android, iOS, and the web.
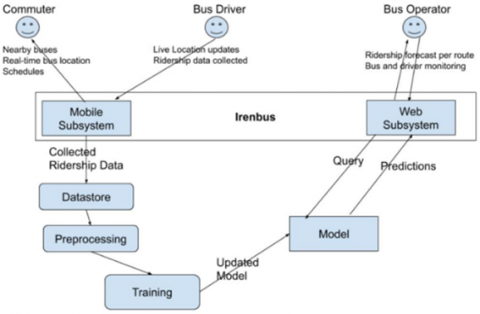
Figure 1. Irenbus system overview
3.2 Database design
A NoSQL database was used for the proposed system, which is non-tabular, and stores data differently compared to traditional relational tables. NoSQL databases come in various types, with the main types being document, key-value, wide-column, and graph-based databases. After assessing the data collected and stored by the proposed system, we decided to employ the key-value database. A key-value database is a simpler type of database where each item contains keys and values. A value can typically only be retrieved by referencing its key. The key-value database is great for use in cases where we need to store large amounts of data, but we do not need to perform complex queries to retrieve it [25]. This type of database is offered as the Realtime Database within the Firebase platform, mentioned in subsection 3.1. The real-time database additionally offers the following features [26, 27]:
- Optimization for offline use: The Realtime Database SDKs use local cache on the device to serve and store changes. When the device comes online, the local data is automatically synchronized.
- Collaboration across devices with ease: instead of typical HTTP requests, the Firebase Realtime Database uses data synchronization—every time data changes, any connected device receives that update within milliseconds.
- Accessible from Client Devices: This can be accessed directly from a mobile device or web browser; there is no need for an application server.
Based on the objectives we set out for the Irenbus system, the following nodes that resulted from the analysis carried out on the developed system are shown in Table 1.
The proposed Irenbus mobile application dependency graphs and data model representation are presented in Figures 5.1-5.15 under the appendix section.
Table 1. NoSQL database nodes description
3.3 Mobile application
The mobile subsystem of Irenbus is in the form of an Android application. The most crucial component of an application in Android development is the Activity class. In contrast to most programming paradigms in which apps are launched with a main ( ) method, the Android system initiates code in an Activity instance by invoking specific callback methods that correspond to specific stages of its life cycle [28]. In Google LLC [29], the Activity life cycle is described in detail. The mobile application has four main activities:
- StartActivity: manages other activities and controls which activity is started based on the application's state when launched.
- LoginActivity: handles all the user authentication tasks of the application.
- CommuterMainActivity: handles all functionality that pertains to the commuter user.
- DriverMainActivity: handles all functionality that pertains to the bus driver user.
Authentication: The mobile application has two types of users: commuters and drivers. They each have different tasks they can carry out on the application, so we added an authentication process to give convenient access to different screens based on what type of user was currently logged in. Figure 2 illustrates some of the proposed system’s authentication processes.
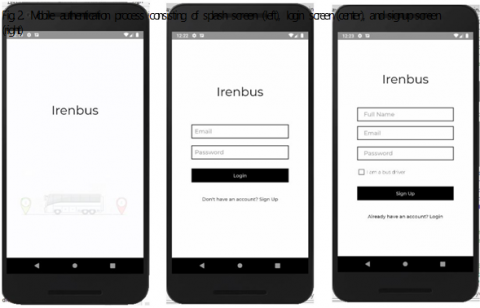
Figure 2. Mobile authentication process consisting of the splash screen (left), login screen (center), and signup screen (right)
The splash screen is shown when the application is launched, keeping the user company while running a background authentication process. In C¸ORAK [30], the authors claim the splash screen dramatically improves the user experience. The login screen allows the user to log in with their email and password. The signup screen enables the user to create a new user account. If the user on sign up checks the 'I am a bus driver' box, a 16 character alphanumeric code for the bus assigned to is required to prevent unauthorized access.
On launch, the mobile application requests the Firebase Authentication SDK to get the current user, as shown in the code snippet below. If the returned user object is null, the user has not logged in before the application. They are then presented with a login screen using an instance of the LoginActivity class. If the returned user object is not null, the user has logged in before. The application will then request the current user's userType value from the database with their userid, which is obtained using firebase user.getUid( ). After the current user's details have been read from the database, the application will access the appropriate screens based on their type.
Upon successful login, the authentication token is stored locally on the device so that the next time the user launches the application, they will not be required to enter in their password. Furthermore, this improves the user experience as the user can log in even without an internet connection.
Commuter: The primary purpose of the mobile application for the commuter is to keep them informed with real-time information about the current status of all buses currently in transit. There are no strict requirements to use the mobile application as a commuter; no personal information is required other than an email address and name, so anyone can sign up without worrying about their privacy. As a fair share of commuters is of age, the user interface is designed to be as minimal as possible and provide simple navigation, which will result in a better experience for the elderly. Additionally, the application is compatible with Google's TalkBack, an accessibility service that helps blind and visually impaired users interact with their devices. When the commuter is logged in, the bottom navigation offers three tabs which each show the fragments in Figure 3.
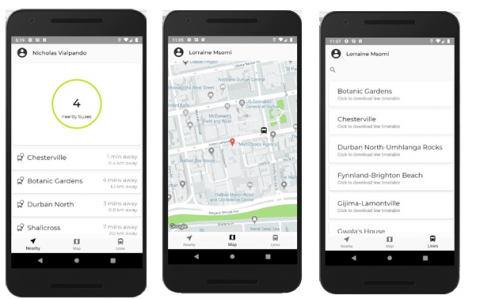
Figure 3. Computer view of the mobile application consisting of the Nearby Fragment (left), Map Fragment (center), and Lines Fragment (right)
Each fragment (Figure 3) in the application for the commuter provides the following functionality:
Nearby Fragment: shows all nearby buses, which are buses within a three kilometer radius from the commuter's current location. The distance between each online bus and the commuter is calculated using the Haversine geolocation equation.
$d=\left(\begin{array}{c}\sqrt{\sin ^{2}\left(\frac{\varphi_{2}-\varphi_{1}}{2}\right)+\cos \left(\varphi_{1}\right)} \cos \left(\varphi_{2}\right) \sin ^{2} \\ \left(\frac{\lambda_{2}-\lambda_{1}}{2}\right)\end{array}\right)$
Every three seconds, the bus location is updated on the database; this means that the distance is calculated each time the location changes. Additionally, through the use of the Google Maps' Directions API - a service that calculates directions between locations and it is accessed through an HTTP interface, with requests constructed as a URL string, and latitude/longitude coordinates to identify the locations [31] - the estimated time of arrival is obtained, which is updated based on real-time traffic conditions.
Map Fragment: graphically shows all the buses currently in transit as they move around, in real-time. The commuter's current location is indicated by a red pin icon and each bus with a black bus icon. When the bus icon on the map is clicked, it will show the bus' route. The user can use a combination of gestures to zoom in and out of the map to see a detailed view and a broader view. The map was created using the Maps SDK for Android API, which handles access to Google Maps servers, data downloading, map display, and response to map gestures.
Lines Fragment: shows all bus lines available for the commuter to browse. This is shown in the form of a list of bus schedules that can each be downloaded at any time. To make the navigation easier and improve user experience, a search functionality was added that can be used to filter from hundreds of bus lines to the specified bus lines in the search term. Figure 4 shows the bus lines tab in action.
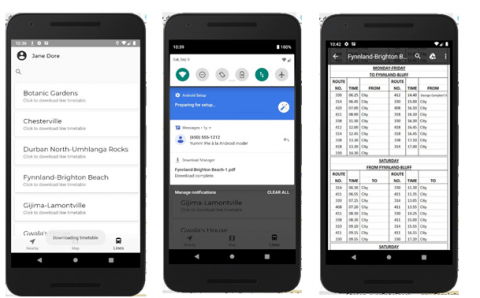
Figure 4. The application bus lines tab consisting of timetable download (left), download complete (center), and timetable viewer (right)
When the user has found the bus schedule/timetable they want, they can click on its name, and it will be downloaded in a PDF format, as shown in sequential order in Figure 4. As there are hundreds of bus schedules PDF files available, this requires a lot of storage space and could make the application bulky. In Wilson [32], the authors claim that users do not download applications because they do not have enough storage on their devices. If downloading an application means having to sacrifice precious photos or messages, the users are not likely to proceed with that download. So to keep the size of the developed application at a minimum, we stored those bus schedules on the Firebase Storage service. Each bus schedule will only be downloaded to the user's request and can be deleted at any time to free up space without deleting the whole application.
Driver : For the bus driver, the main purpose of the mobile application is to record daily ridership and allow the bus' location to be tracked in real-time. The application can be in one of two states that can be changed with the 'Go Online/Offline' button:
- Offline State: in this state, shown in Figure 5, the bus' location is not being tracked, which means it will not be visible to commuters. The bus driver should be in this state when they are not in service. This also means the bus driver will not be able to board commuters or record ridership, as shown in Figure 5.
- Online State: in this state, shown in Figure 5, the bus' location is being actively tracked, and the bus is visible to all nearby commuters. The bus driver will be able to record ridership in this state.
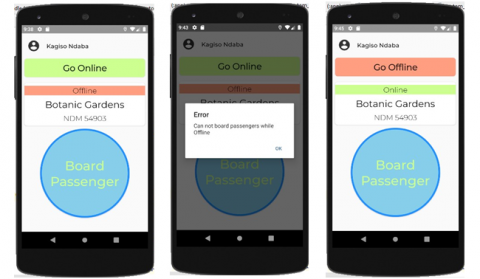
Figure 5. The three application service states: Offline State (left), Board Error (center), Online State (right)
The user interface for the bus driver is designed to be as simple as possible so as not to distract the driver. To record ridership, the bus driver taps on the 'Board Passenger' circle button the number of times that correspond to the number of passengers being boarded. For example, when the bus stops and three passengers board, the bus driver will tap on the button three times.
As mentioned previously, the bus driver is required to enter the bus code when signing up. The bus code is used to identify each bus uniquely and can only be obtained by the bus drivers from the bus operator or manager. The bus driver may be assigned to a different bus after they have signed up; in that instance, the 'Change Bus' option would be used to change to a different bus, given that the bus code entered is valid. This process is shown in order in Figure 6.
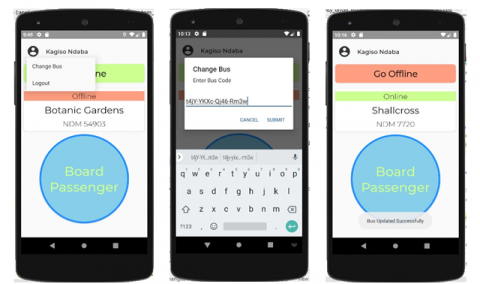
Figure 6. Board Passenger code validation: Bus Change Option (left), Bus Code Input (center), After Bus Change (right)
3.4 Web application
The second subsystem of Irenbus is a web application developed mainly with pure JavaScript and a few other technologies. It is hosted on the Firebase Hosting service, which provides production-grade web content hosting and automatically provisions and configures an SSL certificate. The primary purpose of this application is to provide bus managers and operators with the ability to monitor buses, drivers and perform administrative tasks.
Authentication: The web application only has one type of user - the bus operator/manager - with full administrative access. Hence proper functioning security features are mandatory for this application. The code snippet below shows how authentication state persistence is handled in the application using the Firebase JavaScript SDK.
Driver and Bus Management: The Map tab on the web application provides an overview of all online buses currently in transit. This is presented in a minimally styled map, developed using the Maps JavaScript API, as shown in Figure 7. The bus icons on the map are updated in real-time, and they show the bus as it moves. For a detailed view, the user can click on the bus icon to view where the bus is heading, the number plate and the current driver's full name. This map view will also come in handy when emergencies arise, as the bus' precise location can be tracked. The user can pan around, zoom in and out and set the map to a full-screen view if required.
Additionally, Google Street View is integrated into the map, enabling users to view and navigate through a 360-degree horizontal and a 290-degree vertical panoramic street-level imagery of cities.
The Drivers tab shows a searchable list of all registered drivers within the Irenbus system, including their picture, full name, and bus code of the bus they are assigned to.
Ridership Forecasting: The Ridership tab shows the predicted ridership figures for a given route. These predictions are made by a continuously trained Keras model, described in detail in subsection 3.5. These predictions help bus managers/operators dynamically assign drivers and buses to routes based on expected demand. Furthermore, this will potentially improve the availability and service of buses to commuters. An example of these predictions is shown in Figure 8.
The graph representation of the predictions was achieved through the use of chart.js, which is an open-source JavaScript library for data visualization. This library provides excellent rendering performance across all modern browsers and redraws charts on window re-size events for perfect scale granularity.
3.5 Machine learning pipeline
The machine learning pipeline for the Irenbus system attempts to provide Continuous Delivery for Machine Learning (CD4ML) which is a software engineering approach in which a cross-functional team produces machine learning applications based on code, data, and models in small and safe increments that can be reproduced and reliably released at any time, in short, adaptation cycles [33]. The study’s proposed approach is shown in Figure 9.
Data collection: this refers to the collection of daily ridership data carried out by bus drivers using the mobile application. This data is continuously saved on the Realtime Database.
Data Extraction and Analysis: the collected ridership data on the Realtime Database is periodically exported in a JSON format and then converted into CSV with tools such as https://konklone.io/json/. The CSV is then analyzed and checked for inconsistencies.
Model Training: This is carried out on a Python Notebook using the CSV file with ridership data from the previous step. The resulting Keras model is converted from HDF5 to JSON, as shown in code listing 1.
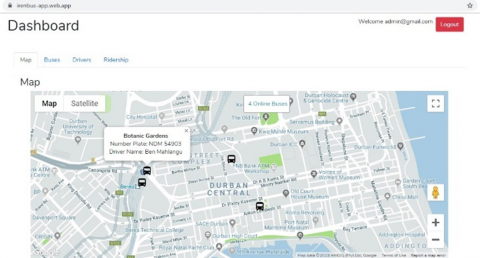
Figure 7. Online buses overview
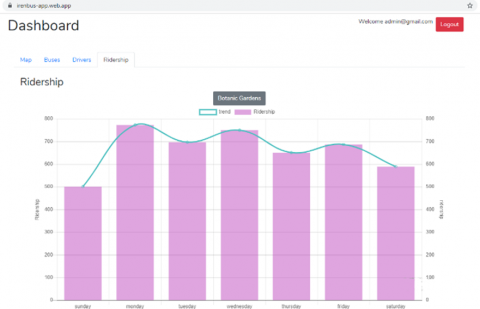
Figure 8. Ridership forecast for the Botanic Gardens route
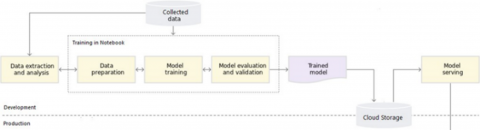
Figure 9. Irenbus machine learning pipeline
Code Listing 1: Training in Python
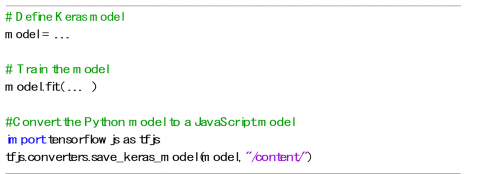
Trained model: the Keras model ( model.json ) is produced after training is uploaded to Firebase's Cloud Storage service. The sample dataset and detailed description, training and evaluation of the model used in the proposed system is described in subsection 4.3.
Prediction Service: this is carried out on the web application by a JavaScript function forecastRidership(...) , which takes values shown in code listing 2 and returns the predicted ridership value through the use of the Keras model saved on Cloud Storage.
Code Listing 2: Ridership forecast function in the Web Application
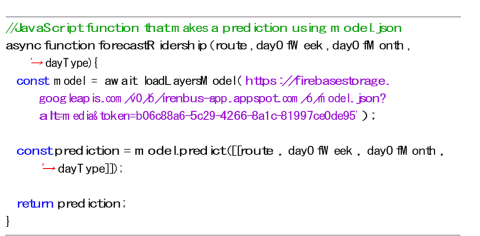
This section presents the qualitative and quantitative evaluations of the newly developed real-time public transport system.
4.1 Mobile application evaluation
We used the Android Studio Emulator and Firebase's Test Lab to test the Android application. The Android Emulator simulates Android devices on the computer, allowing applications on various simulated devices and Android API levels without needing to have each physical device. The Emulator provides the ability to simulate incoming phone calls and text messages, simulate different network speeds, specify the device's location, and simulate rotation and other hardware sensors [34]. Firebase Test Lab is a cloud-based app-testing infrastructure that uses real production devices running in a Google data center [35]. The Test Lab was used to supplement the Emulator with its test automation features and scalable testing.
Figure 10 shows the simulated route of the bus on an actual map. During the simulation, the bus location updates on the Realtime Database were monitored closely, and there were no abnormalities in the reported location points. The simulation was run for the second time, on which we now monitored the location updates on the web application dashboard map, and no errors on the bus' path were observed. So when the bus driver is online and the bus' location is being tracked, we expect no errors, as observed in the simulation.
Location Simulation: Since the mobile application depends heavily on location tracking, we needed to test if the application reported valid and accurate location points across all connected devices. We needed a cost-effective and reproducible approach to testing location tracking instead of physically driving around with the device, so we used the GPS Data Playback to simulate a bus moving around the city. The GPS Data Playback allows GPX files to be loaded and played back. A GPX (the GPS Exchange Format) is a lightweight XML data format for the interchange of GPS data (waypoints, routes, and tracks) between applications and Web services on the Internet. The bus' path was loaded into simulated_route.gpx, is as shown in code listing 3.
Code Listing 3: Simulated Route
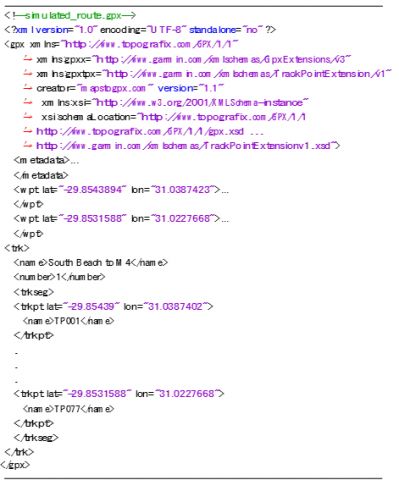
Figure 10. Played back bus route
Robo Test: We subjected it to several Robo tests to ensure that the mobile application users did not encounter unexpected results or had a poor experience when interacting with the newly developed application. These are automated tests that analyze the user interface structure and explore it methodically, simulating user activities. Robo tests are made possible by the Espresso and UI Automator 2.0 user experience testing frameworks.
Two tests were carried out separately as Robo tests on a Galaxy S7 Edge, Android API Level 23, to assess CPU usage, Memory consumption and Network usage in the two main activities of the Irenbus mobile application. These are the Commuter Activity and the Driver Activity, as shown in Figures 11 and 12.
Two tests were carried out separately as Robo tests on a Galaxy S7 Edge, Android API Level 23, to assess CPU usage, Memory consumption and Network usage in the two main activities of the Irenbus mobile application, which are the Commuter Activity and the Driver Activity.
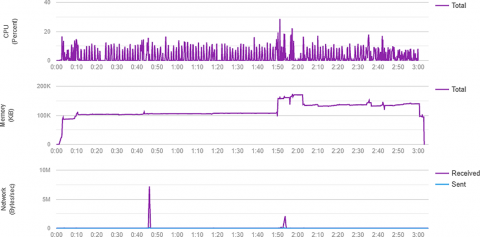
Figure 11. Commuter Activity CPU, Network and Memory statistics for 3 min 4 sec
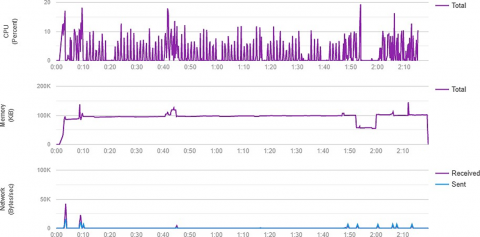
Figure 12. Driver Activity CPU, Network and Memory statistics for 2 min 19 sec
4.2 Web application evaluation
The web application performance was evaluated using Google's PageSpeed Insights tool, which reports a performance score of a web application on both mobile and desktop devices. This score is determined by running Lighthouse, which is an open-source, automated tool for improving the quality of web applications.
Six metrics were used to assess the performance of the application. First, Contentful Paint (FCP) marks the time at which the first text or image is painted. Time to Interactive is the amount of time it takes for the page to become fully interactive. Speed Index shows how quickly the contents of a page are visibly populated. Blocking Time is the sum of all periods between FCP and Time to Interactive when task length exceeded 50ms, expressed in milliseconds. Largest Contentful Paint marks the time at which the most extensive text or image is painted. Cumulative Layout Shift measures the movement of visible elements within the viewport. These metrics were selected because they are user-centric, as mentioned in Ref. [36].
The results of the performance assessment are shown in Table 2. The web application obtained a relatively better score on desktop devices than on mobile devices, which was expected as the web application was developed to be used on desktops by bus managers/operators. To improve the FCP, the amount of imagery and styling can be reduced, but all other metrics reported good results on the desktop.
Table 2. Page speed insights results
4.3 Ridership forecasting evaluation
This section describes and evaluates the machine learning model integrated into the newly implemented public transport system. Since the adopted machine learning model required data to be trained on, we utilized an already existing real-world public transport dataset instead of collecting the ridership data ourselves with the mobile application, which would have been very demanding and costly.
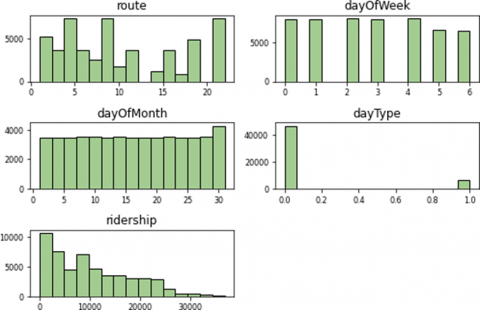
Figure 13. Dataset columns histograms
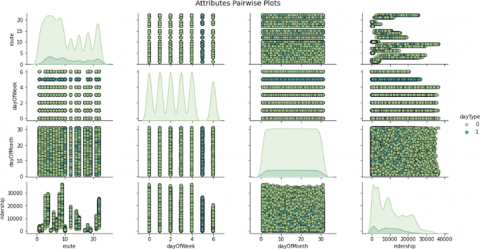
Figure 14. Pairwise correlogram for daytype
Dataset: The dataset used for this study was obtained from the Chicago Transit Authority's open data portal, which is an operator of mass transit in Chicago, Illinois and surrounding suburbs with a fleet of 1879 buses and a 242 million annual bus ridership [37, 38]. The obtained data shows the daily ridership total for each route from mid-2019 back to 2001. The dataset was filtered and only left relevant columns, namely ridership (daily), day type (working day or not), day of the month, day of the week and route (number). Figure 13 shows the value range and distribution for each attribute in the dataset. Specifically, Figures 14 and 15 visually describe the pairwise correlogram dataset for day Type and dataset correlation matrix.
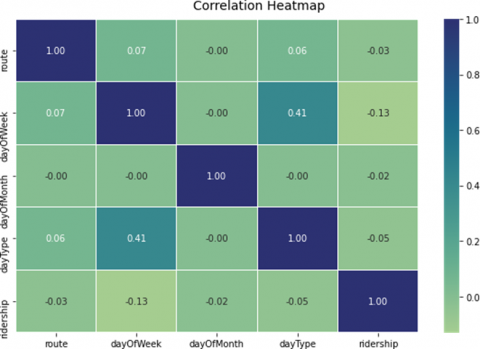
Figure 15. Dataset correlation matrix
Preprocessing : The varying scale of values in the dataset used led us to resort to preprocessing to resolve this. It is best practice to prepare the data before modelling it with a neural network model. The quality of data that a machine learning model is trained with greatly affects the resulting model. The following two well-known methods were employed to rescale the dataset's attributes:
- Normalization : In this case, the data is rescaled from the original range so that all attributes have values within the range of 0 and 1. A normalized value of an attribute is calculated as $Z=\frac{x-\min (x)}{\max (x)-\min (x)}\quad$.
- Standardization : This concerns rescaling the distribution of values so that the mean of observed values is 0 with a standard deviation of 1. A standardized value is obtained using $Z=\frac{x-\operatorname{mean}(x)}{\text { standarddeviation }(x)}\quad$.
Model Architecture: The adopted machine learning model is Keras' Sequential model with four densely connected hidden layers, with a single output that returns a continuous value. The input layer takes in four values for the developed system: route, day Type, day Of Week and day Of Month. The first hidden layer has four inputs and 50 output nodes. The second hidden layer has 50 inputs and 100 output nodes. The third hidden layer has 100 inputs and 50 output nodes. The output layer has 50 inputs and one output node - which is the ridership prediction - as shown in Figure 16.
The learning algorithm used to adjust weights in the network was the Adaptive moment estimation (Adam) optimization algorithm, chosen because of its computational efficiency, little memory requirements and straightforward implementation.
Training: The aforementioned two preprocessed (normalized and standardized) datasets and the unaltered dataset were used in the training process. The training was done three times with a different dataset to have three resulting models. This was done so we could observe the effect of preprocessing the dataset on the model's ability to learn, and we could pick the best model to be used in the new system. The models were trained on Google Colab - an online Python Notebook - with the system specification shown in Table 3.
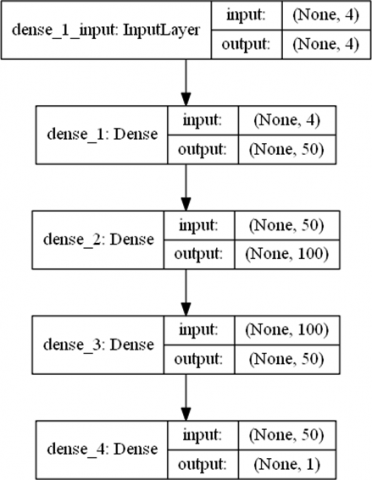
Figure 16. Model architecture
Table 3. Training system hardware specifications
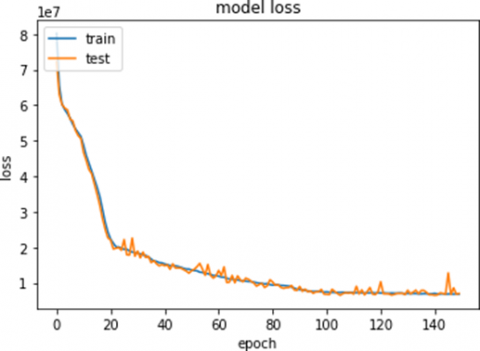
Figure 17. MSE for the model trained with an unscaled dataset
The model training was carried out for 150 epochs, which was found to be optimal. Each model used the Adam optimization algorithm, which automatically tunes itself to provide better results. The training used 80 per cent of the dataset, and testing used the remaining 20 per cent. For validation, we use 30 per cent of the 80 per cent for training. The batch size was set to 10 samples. To determine the optimal number of epochs to be used in the training process, for each model, we experimented with the number of epochs starting from 50 and increasing by 50 in each try while observing the amount of loss from each model, and when we reached 150, the models showed stability. The graphs in Figures 17, 18 and 19 show each model's Mean Square Error fluctuations for a duration of 150 epochs, which indicates how well the model was learning.
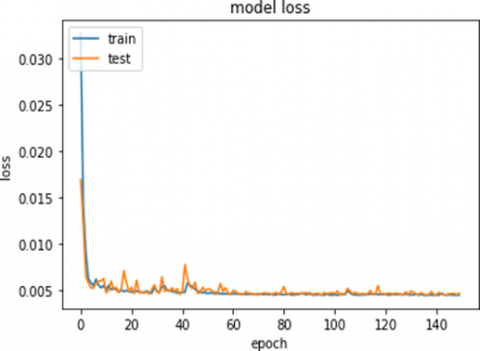
Figure 18. MSE for the model trained with a normalized dataset
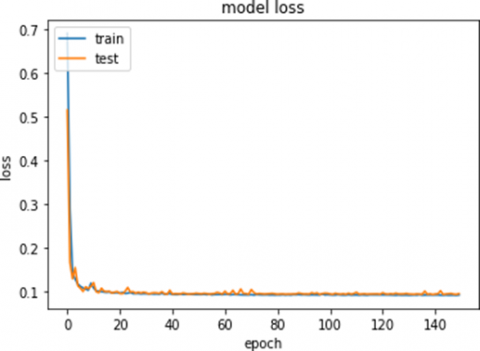
Figure 19. MSE for the model trained with a standardized dataset
The model's 150 epochs training for the Unscaled, Standardized and Normalized datasets took 14 mins 32 secs, 13 mins 49 secs and 13 mins and 20 secs, respectively. Generally, the lower the loss, the better the model is unless the model has overfitted the training data, which was not the case for current models since they were all tested with unseen data. The standardization of the dataset dramatically improved the model's learning ability compared to normalization. The non-preprocessed dataset visibly showed a more significant loss, hence more inferior learning for the model as expected in comparison to the preprocessed ones. The best model, which was trained from the standardized dataset, was used by the proposed web application to forecast future ridership.
4.4 Overall system evaluation
The quality of a system is the degree to which the system satisfies the stated and implied needs of its various stakeholders and thus provides value [39]. The most prominent models for assessing software quality include the Boehem, Mc-Call, FURPS, Dromey, ISO 9126 and the recent ISO 25010, which is defined as the cornerstone of a product quality evaluation [40-44]. The ISO 25010 is an international standard that defines software quality in two models: quality in use and product quality. The quality in use model is composed of five characteristics (further subdivided into sub-characteristics) that relate to the outcome of interaction when a product is used in a particular context. The product quality model comprises eight characteristics (further subdivided into sub-characteristics) that relate to static properties of software and dynamic properties of the computer system [45].
The Irenbus system was rated based on the ISO 2501O standards by five volunteers. For an accurate assessment, the selected volunteers were experienced in software design and development. Experienced volunteers were selected because most of the ISO 25010 standard metrics are technical. Due to the broadness of the Irenbus system - as it has three types of users, viz. the commuter, the bus driver and the bus manager - each volunteer was provided with authentication credentials for all three system users and asked to assess the system from the perspective of each of the users. So each of the volunteers completed three different assessments, which gave us fifteen assessments in total. The assessments contained sub-characteristics of the aforementioned ISO 25010 characteristics. The volunteers provided ratings using the Likert Scale (1-Not Satisfied, 2-Less Satisfied, 3-Neutral, 4-Satisfied, 5-Very Satisfied) on how much each sub- characteristic was satisfied by the Irenbus system. All assessments' results were compiled into Table 4, with µ being the mean and σ being the standard deviation of ratings for each sub-characteristic.
The volunteers evaluated the new system and scored the system on each characteristic's feasibility in the South African context. For the obtained results shown in Table 4, the ratings in all characteristics had a very low standard deviation. They were all less than one, which implies that the mean ratings resemble individual volunteer ratings closely. Based on the results, the new Irenbus is functionally suitable for the needs of commuters, bus drivers and bus operators. The performance efficiency of the system was rated high except for resource utilization, where volunteers reported heavy battery drainage on their mobile devices when logged in as bus drivers. This was due to background location tracking processes on which the GPS receiver, a small chip antenna in the mobile device, was always listening to the cell towers to decide where the device was located geographically at all times [46].
Furthermore, this is a prevalent issue in most location tracking mobile applications. For example, the popular cab-hailing application - Uber - explains in Ref. [47] how they are at- tempting to solve this problem. The Irenbus system achieved relatively good ratings on compatibility, reliability, security and the usability sub-characteristic - user interface aesthetics scoring the highest rating. The system's maintainability and portability characteristics achieved decent ratings; this implies that the new system can be deemed feasible and cost-efficient. Overall, the ratings show that the volunteers were fairly satisfied with the current system.
Table 4. Irenbus system evaluation
This paper aimed to demonstrate a practical and implementable intelligent public transport management system in the context of developing countries. Five objectives were listed as part of this research. The first involved designing and developing an Android application to inform commuters about the current status of a given bus through live location tracking. The second objective was to develop an approach to collect daily ridership by using only a mobile application and no external hardware modules. Third, developing an approach to incorporate continuously trained machine learning to forecast ridership per route. Fourth, designing and developing a web-based application to monitor buses, drivers and present the machine learning model's predictions to bus operators. The fifth objective was evaluating the feasibility of the newly developed system.
As this research aimed to develop the new system so that it could be easily implemented in South Africa and other developing countries, we needed to make sure that unnecessary implementation and maintainability costs were reduced as much as possible. This then led to the proposed system being purely cloud-based and run on just a mobile application and requiring no extra external hardware components. We chose smartphones as the platform to implement the new system because more than 91% of the South African population owned smartphones as of 2019, 3G coverage was almost 100%, and 4G/LTE coverage was nearly 93% in 2019 [6]. Furthermore, the cloud-based system means system updates and new features can be rolled out effortlessly without physically tinkering with the device. The system evaluation in subsection 4.4 showed that experienced software developers who volunteered to assess the Irenbus system deemed it feasible within the South African context.
The contribution of this research was to fill in the gap in previously published work with the development of a 360 degree and cohesive public transportation system that caters for the needs of commuters and bus operators; the exploration and development of a daily ridership collection approach; and the use of a machine learning model to predict future ridership based on the data collected by the system itself. The developed system took full advantage of cloud computing services while requiring only a smartphone to work, thereby eliminating the need and costs of installing and maintaining separate GPS trackers on the vehicles.
This research was limited to demonstrating the current implemented system on only one mode of public transport - buses; this was done to simplify the scope of the system for now. However, it will be interesting to see how the new system can be implemented for other public transport modes and training and testing done on a large scale.
For future work, the functionality of the Irenbus can be improved by way of incorporating other modes of public transport; creating a communication channel between the bus managers and commuters in the form of a noticeboard for public announcements; by having a mobile application for not only Android but iOS devices too; and by also exploring other machine learning models to improve the ridership predictions.
This work is supported by the Huawei Technologies Africa (Pty) Ltd, Masters Bursary (Menzi Skhosana, student number: 216032734).
The source code, documentation, Python Notebook and training data for Irenbus is available in the following GitHub repository: https://github.com/m3n2ie/ Irenbus.
Irenbus Mobile Application Dependency Graphs
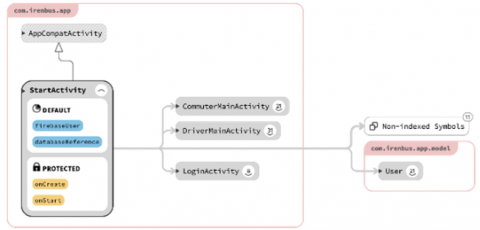
Figure 5.1. Start activity
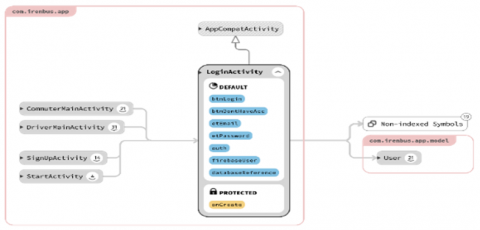
Figure 5.2. Login activity
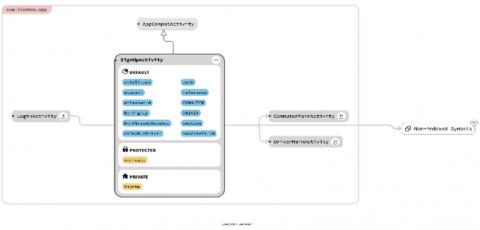
Figure 5.3. SignUp activity
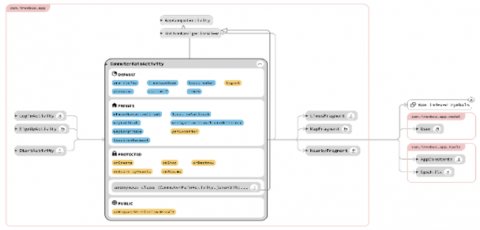
Figure 5.4. Commuter main activity
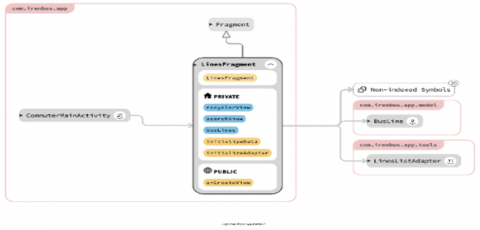
Figure 5.5. Lines fragment activity
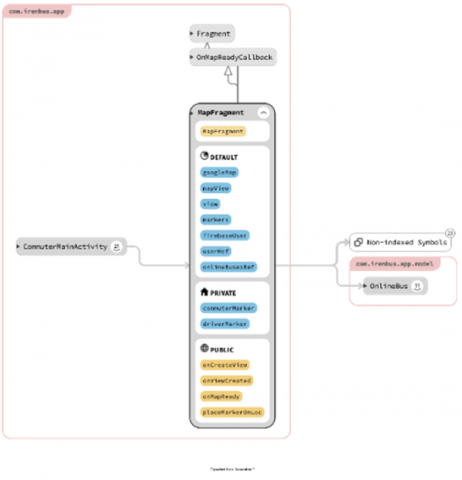
Figure 5.6. Map fragment activity
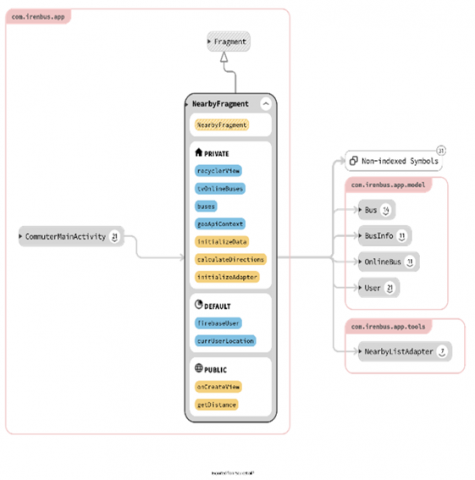
Figure 5.7. Nearby fragment activity
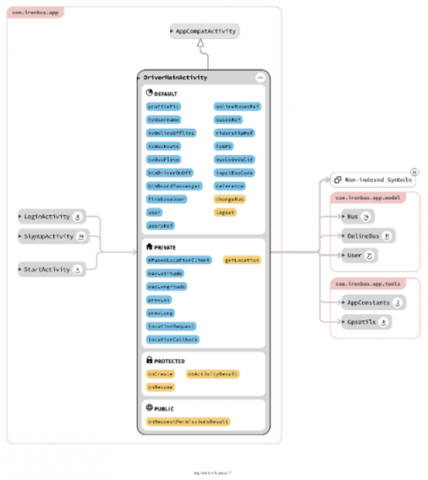
Figure 5.8. Driver main activity
IRENBUS DATA MODELS
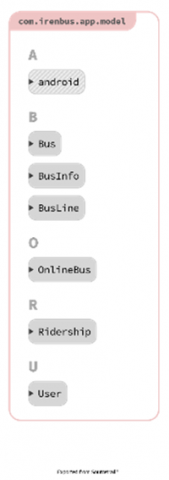
Figure 5.9. All data models
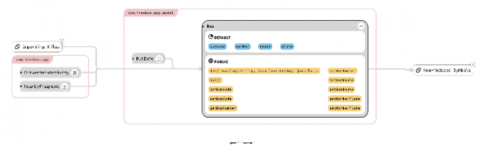
Figure 5.10. Bus data model
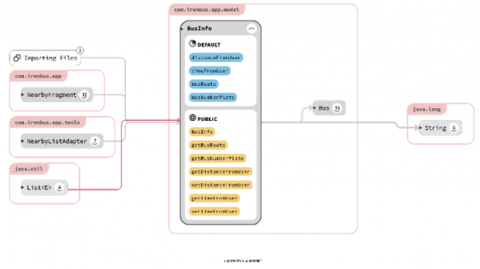
Figure 5.11. Bus info model
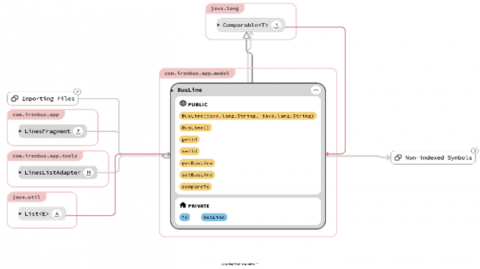
Figure 5.12. Bus line model
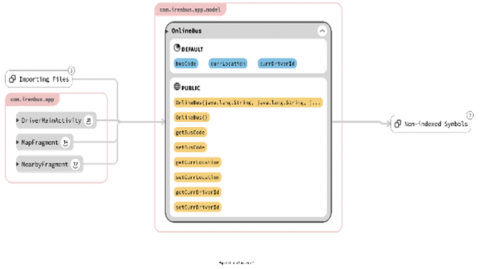
Figure 5.13. Online bus model
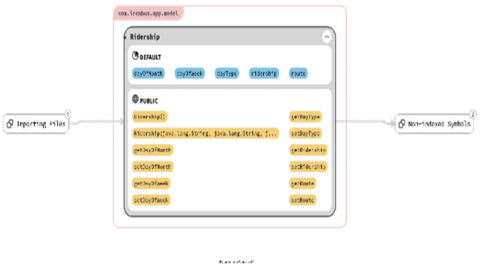
Figure 5.14. Ridership model
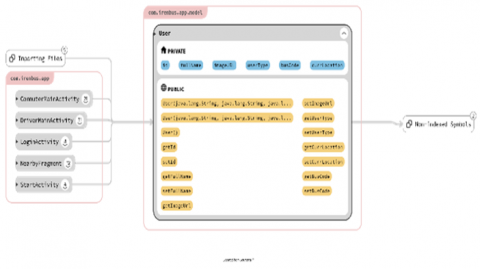
Figure 5.15. User model
[1] Stats, S.A. (2015). Measuring household expenditure on public transport. http://www.statssa.gov.za/?p=5943. [2] Stockin, D. (2020). Does adding an extra driving lane make traffic worse? https://drivetribe.com/p/does-adding-an-extra-driving-lane-E6FPiVJnQSCPun1-pS-Q-A?iid= LEiNsk8oQqOPNAOQ1{_}hVqg. [3] Hanna, N. (2010). e-Transformation: Enabling new Development Strategies, New York: Springer, pp. 281-309. ISBN: 9781441911858. [4] Skhosana, M., Ezugwu, A. (2021). A Real-Time Machine Learning Based Public Transport Bus-Passenger Information System. https://doi.org/10.31224/osf.io/2ubv9 [5] United Nations Department of Economic and Social Affairs. (2020). 68% of the World Population Projected To Live in Urban Areas By 2050. https://www.un.org/development/desa/en/news/population/2018-revision-of-world-urbanization-prospects.html. [6] ICASA. (2020). The state of the ICT sector in South Africa. Independent Communications Authority of South Africa, 83. https://www.icasa.org.za/uploads/files/State-of-ICT-Sector-Report-March-2018.pdf. [7] Motta, R.A., Da Silva, P.C.M., Santos, M.P.D.S. (2013). Crisis of public transport by bus in developing countries: a case study from Brazil. International Journal of Sustainable Development and Planning, 8(3): 348-361. https://doi.org/10.2495/SDP-V8-N3-348-361 [8] Ngoc, A.M., Hung, K.V., Tuan, V.A. (2017). Towards the development of quality standards for public transport service in developing countries: Analysis of public transport users’ behavior. Transportation Research Procedia, 25: 4560-4579. https://doi.org/10.1016/j.trpro.2017.05.354 [9] Pucher, J., Korattyswaropam, N., Mittal, N., Ittyerah, N. (2005). Urban transport crisis in India. Transport Policy, 12(3): 185-198. https://doi.org/10.1016/j.tranpol.2005.02.008 [10] Sungur, C., Babaoglu, I., Sungur, A. (2015). Smart bus station-passenger information system. In 2015 2nd International Conference on Information Science and Control Engineering, pp. 921-925. https://doi.org/10.1109/ICISCE.2015.209 [11] Manikandan, R., Niranjani, S. (2014). Implementation on real time public transportation information using GSM query response system. Contemporary Engineering Sciences, 5(7): 509-514. [12] Shirisha, K., Sivaprasad, T. (2016). Acquire bus information using GSM technology. International Journal of Advancements in Technology. [13] Kumbhar, M., Survase, M., Mastud, P., Salunke, A., Sirdeshpande, S. (2016). Real time web based bus tracking system. International Research Journal of Engineering and Technology, 3(2): 632-635. [14] Skhosana, M. (2020). Irenbus: A real-time public transport management system. In 2020 Conference on Information Communications Technology and Society (ICTAS), pp. 1-7. https://doi.org/10.1109/ICTAS47918.2020.234000 [15] Skhosana, M., Ezugwu, A.E., Rana, N., Shafi’i, M.A. (2020). An intelligent machine learning-based real-time public transport system. In International Conference on Computational Science and Its Applications, pp. 649-665. https://doi.org/10.1007/978-3-030-58817-5_47 [16] Ryu, S., Park, B.B., El-Tawab, S. (2020). WiFi sensing system for monitoring public transportation ridership: A case study. KSCE Journal of Civil Engineering, 24(10): 3092-3104. https://doi.org/10.1007/s12205-020-0316-7 [17] Monchambert, G., De Palma, A. (2014). Public transport reliability and commuter strategy. Journal of Urban Economics, 81: 14-29. https://doi.org/10.1016/j.jue.2014.02.001 [18] Bacciu, D., Carta, A., Gnesi, S., Semini, L. (2017). An experience in using machine learning for short-term predictions in smart transportation systems. Journal of Logical and Algebraic Methods in Programming, 87: 52-66. https://doi.org/10.1016/j.jlamp.2016.11.002 [19] Hsu, Y.W., Chen, Y.W., Perng, J.W. (2020). Estimation of the number of passengers in a bus using deep learning. Sensors, 20(8): 2178. https://doi.org/10.3390/s20082178 [20] van Oort, N., Brands, T., de Romph, E. (2015). Short-term prediction of ridership on public transport with smart card data. Transportation Research Record, 2535(1): 105-111. https://doi.org/10.3141/2535-12 [21] MyCiti. (2020). Using MyCiti on your phone. Available from: https://www.myciti.org.za/en/discover-myciti/using-myciti-on-your-phone/. [22] McCann A. (2020). Cities with the best & worst public transportation. https://wallethub.com/edu/cities-with-the-best-worst-public-transportation/65028/{#}main-findings. [23] Optibus. (2020). The optibus platform. https://www.optibus.com/product/platform/. [24] Frankenfield, J. (2020). What is cloud computing? - Types of Cloud Computing Services & More. https://www.trianz.com/insights/revolution-that-is-cloud-computing. [25] Mongo, D.B. (2020). NoSQL Explained. https://www.mongodb.com/nosql-explained. [26] Firebase. (2020). Store and sync data in real time. https://firebase.google.com/products/realtime-database. [27] Firebase. (2020). Firebase realtime database. https://firebase.google.com/docs/database. [28] Google, LLC. (2020). Introduction to Activities. https://developer.android.com/guide/components/activities/intro-activities.html. [29] Google, LLC. (2020). Developer guides — android developers. https://developer.android.com/reference/ android/app/Activity. [30] C¸ORAK, A. (2020). Splash screen is more important than you think how to use. https://uxplanet.org/splash-screen-is-more-important-than-you-think-855e78da3c2c. [31] Google, LLC. (2020). Get started - Directions API. https://developers.google.com/maps/documentation/directions/start. [32] Wilson, M. (2020). App download stats reveal reason behind low number of apps downloaded. https://www.zipwhip.com/blog/app-download-statistics-reveal-why-people-dont-download-apps/. [33] Sato, D., Wider, A., Windheuser, C. (2019). Continuous delivery for machine learning. https://martinfowler.com/articles/cd4ml.html. [34] Android Developers. (2020). Run apps on the android emulator. https://developer.android.com/studio/run/emulator. [35] Firebase. (2020). Firebase test. https://firebase.google.com/docs/test-lab. [36] Google Developers. (2020). About pagespeed insights. https://developers.google.com/speed/docs/insights/v5/about. [37] Chicago Transit Authority. (2020). CTA - Ridership - bus routes - daily totals by route about this dataset columns in this dataset. https://data.cityofchicago.org/Transportation/CTA-Ridership-Bus-Routes-Daily-Totals-by-Route/jyb9-n7fm. [38] Chicago Transit Authority. (2020). Annual Ridership Report Calendar Year 2015. https://www.transitchicago.com/assets/1/6/2018_Annual_Report_-_v3_04.03.2019.pdf. [39] SYSQA. (2020). Iso 25010: 2011. http://www.gripoprequirements.nl/downloads iso-25010-2011-een-introductie-v1{_}0.pdf. [40] Boehm, B.W., Brown, J.R., Lipow, M. (1976). Quantitative evaluation of software quality. In Proceedings of the 2nd International Conference on Software Engineering, pp. 592-605. [41] Walters, G.F., McCall, J.A. (1979). Software quality metrics for life-cycle cost-reduction. IEEE Transactions on Reliability, 28(3): 212-220. https://doi.org/10.1109/TR.1979.5220569 [42] Saini, R., Dubey, S.K., Rana, A. (2011). Analytical study of maintainability models for quality evaluation. Indian Journal of Computer Science and Engineering, 2(3): 449-454. [43] Dromey, R.G. (1995). A model for software product quality. IEEE Transactions on Software Engineering, 21(2): 146-162. https://doi.org/10.1109/32.345830 [44] Coallier, F. (2001). Software engineering–product quality–part 1: quality model. International Organization for Standardization: Geneva, Switzerland. 2(1): 1-25. [45] Messer-Misak, K., de Bruin, J.S., Hanke, S. (2020). A systematic approach to quality requirement management in medical software. In dHealth 2020–Biomedical Informatics for Health and Care (pp. 129-136). IOS Press. [46] Liao, S. (2018). Why GPS-dependent apps deplete your smartphone battery. https://www.theverge.com/2018/8/17/17630872/smartphone-battery-gps-location-services. [47] Hartanto, Y., Attwell, B. (2020). Activity / Service as a dependency: Re-thinking android architecture for the uber driver app. https://eng.uber.com/activity-service-dependency-android-app-architecture/.
Phone: + 1 825 436 9306
Email: [email protected]
Subscription
Language support
Please sign up to receive notifications on new issues and newsletters from IIETA
Select Journal/Journals:
Copyright © 2024 IIETA. All Rights Reserved.
Thank you for visiting nature.com. You are using a browser version with limited support for CSS. To obtain the best experience, we recommend you use a more up to date browser (or turn off compatibility mode in Internet Explorer). In the meantime, to ensure continued support, we are displaying the site without styles and JavaScript.
- View all journals
- My Account Login
- Explore content
- About the journal
- Publish with us
- Sign up for alerts
- Data Descriptor
- Open access
- Published: 10 October 2023
ZTBus: A Large Dataset of Time-Resolved City Bus Driving Missions
- Fabio Widmer ORCID: orcid.org/0000-0003-0604-3856 1 na1 ,
- Andreas Ritter ORCID: orcid.org/0000-0002-7073-7118 1 na1 &
- Christopher H. Onder 1
Scientific Data volume 10 , Article number: 687 ( 2023 ) Cite this article
834 Accesses
Metrics details
- Electrical and electronic engineering
- Mechanical engineering
- Scientific data
This paper presents the Zurich Transit Bus (ZTBus) dataset, which consists of data recorded during driving missions of electric city buses in Zurich, Switzerland. The data was collected over several years on two trolley buses as part of multiple research projects. It involves more than a thousand missions across all seasons, each mission usually covering a full day of operation. The ZTBus dataset contains detailed information on the vehicle’s power demand, propulsion system, odometry, global position, ambient temperature, door openings, number of passengers, dispatch patterns within the public transportation network, etc. All signals are synchronized in time and include an absolute timestamp in tabular form. The dataset can be used as a foundation for a variety of studies and analyses. For example, the data can serve as a basis for simulations to estimate the performance of different public transit vehicle types, or to evaluate and optimize control strategies of hybrid electric vehicles. Furthermore, numerous influencing factors on vehicle operation, such as traffic, passenger volume, etc., can be analyzed in detail.
Similar content being viewed by others
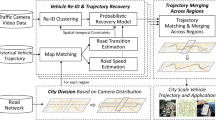
City-scale Vehicle Trajectory Data from Traffic Camera Videos
Fudan Yu, Huan Yan, … Yong Li
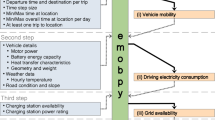
An open tool for creating battery-electric vehicle time series from empirical data, emobpy
Carlos Gaete-Morales, Hendrik Kramer, … Alexander Zerrahn
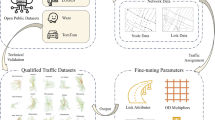
A unified dataset for the city-scale traffic assignment model in 20 U.S. cities
Xiaotong Xu, Zhenjie Zheng, … Wei Ma
Background & Summary
Public transportation is an effective solution for reducing traffic in growing cities. It significantly reduces the number of vehicles on the road, resulting in less congestion, shorter travel times, minimal ecological footprint, and reduced overall energy consumption. In the near future, the need for such efficient urban transportation systems is likely to increase, as an estimated two-thirds of the world’s population is expected to live in cities by 2050 1 .
In this context, detailed driving and operational data are of great value to assist cities and transportation operators in making informed decisions on the vehicles’ ideal propulsion technology and charging strategy for the respective public transportation network. Furthermore, for the development and tuning of intelligent vehicle state estimation algorithms or energy management strategies, time-resolved data of the traction system is necessary for both the vehicle manufacturers and the research community. While there are publicly available datasets describing urban traffic conditions and human mobility 2 , 3 , 4 , as well as time-series data of personal cars 5 , 6 , 7 or taxis 8 , publicly available time-series data of urban transit buses is lacking.
The goal of this publication is to fill this gap by presenting the ZTBus dataset 9 , which is composed of data recorded throughout the course of the projects «SwissTrolley plus» 10 and ISOTHERM 11 , both of which were collaborations between industry partners and public research institutions and were financially supported by the Swiss Federal Office of Energy (SFOE). The dataset covers more than a thousand driving missions of two trolley buses that were in operation between April 2019 and December 2022. It consists of detailed time series that represent the power demand, propulsion system, odometry, global position, ambient temperature, door openings, number of passengers, and the dispatch patterns within the public transportation network of the two vehicles. The time series are provided in a synchronized form and are sampled every second. Aggregated quantities for each of the missions are provided in a metadata table. A schematic overview of the data acquisition and curation procedure, which is explained in greater detail below, is shown in Fig. 1 . Figure 2 presents the full extent of the dataset.
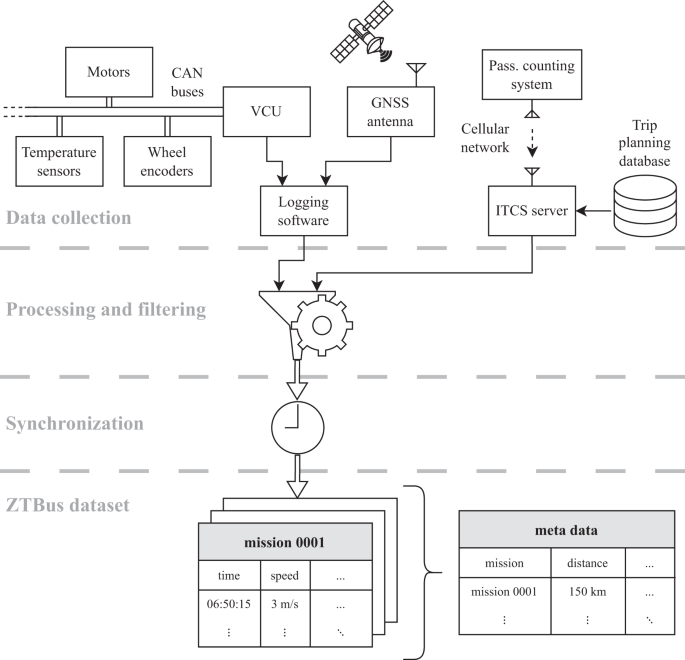
Data acquisition and curation. Signals from three different sources, i.e., the vehicle control unit (VCU), the global navigation satellite system (GNSS) antenna, and the passenger counting system, are used in the definition of the driving missions. Various filtering steps are added to reject erroneous and unrepresentative data. Finally, time synchronization and sampling are performed to present the data in a tabular format.
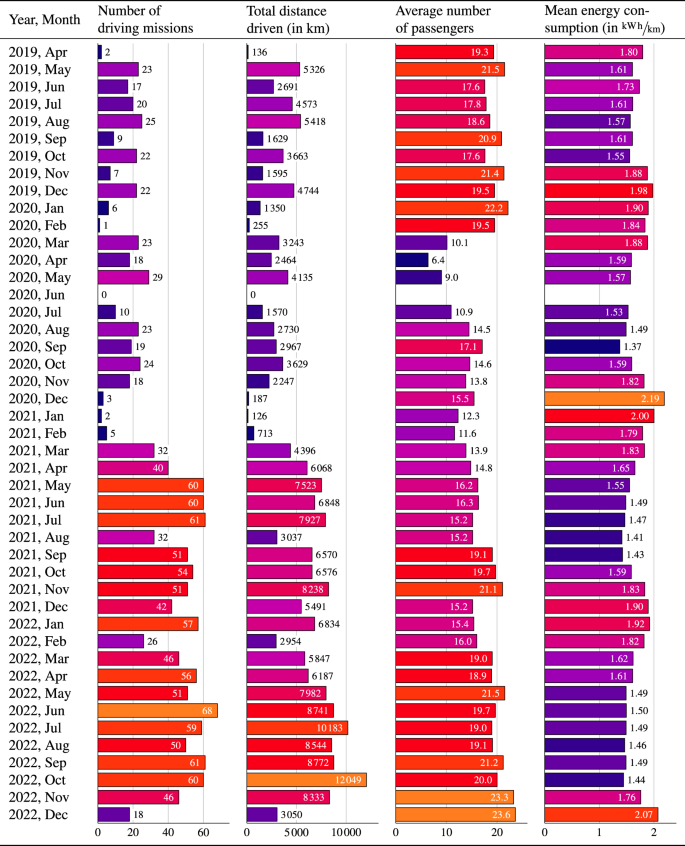
Visualization of the extent of the ZTBus dataset 9 , which includes a total of 1409 driving missions over the period of over 3.5 years between April 2019 and December 2022.
This data offers the potential to be used in a broad variety of fields. For example, the time-resolved global navigation satellite system (GNSS) data can be combined with odometry signals, such as the wheel speeds and the steering angle, and processed using sensor fusion approaches. Such algorithms can significantly improve the raw pose estimates provided by the GNSS sensor, and facilitate the use of dead reckoning approaches in case of momentary signal outage. Additionally, the large amount of data on a set of given routes is suitable for the examination of algorithms for trajectory filtering and map matching in machine learning contexts.
Machine learning may also be utilized to predict various influence factors in public transportation, such as the number of passengers that travel a certain distance at a given time, the traffic levels on specific roads and at specific times of the day, or the expected speed profiles of the vehicles in the near future or in general on certain road segments.
Finally, the aggregated data enables the examination of long-term correlations such as the impact of COVID-19 mitigation measures on passenger numbers, the effect of weather conditions on energy consumption, etc.
The dataset presented in this manuscript has been used in several of our own publications in the context of the research activities mentioned above: (1) The position, odometry, and velocity data served to develop and evaluate a real-time incremental graph construction algorithm 12 . (2) Time-resolved speed, torque, and braking pressure signals were used for the development of the model-based vehicle mass and road grade estimation method 13 . (3) The spatio-temporal nature of the power request signal was used to quantify the relation between grid and battery energy usage on certain road segments, which then served to derive a stochastic model predictive control approach 14 . (4) The optimal design and control of a thermal energy buffer in an electric city bus was studied based on the passenger loads, velocity and elevation profiles 15 . (5) A set of 16 representative all-day driving missions served to optimize the bus operation in terms of managing battery degradation throughout the vehicle lifetime 16 . (6) Hourly-averaged data was used to conduct a large-scale sensitivity analysis of the thermal comfort systems, allowing a comparison of various heating, ventilation and air conditioning (HVAC) systems 17 .
Data collection
The ZTBus dataset 9 was recorded on two trolley buses during regular operation by Verkehrsbetriebe Zurich (VBZ). Both are “HESS lighTram® 19 DC” buses, which are single-articulated, have an overall length of about 19 m, a curb weight of about 19 t, and a maximum passenger capacity of about 160. They are equipped with traction batteries, which allow them to run for a few kilometers without the overhead power grid. The dataset covers the operation of the buses on various bus routes in Zurich’s public transportation network, which are visualized in Fig. 3 .
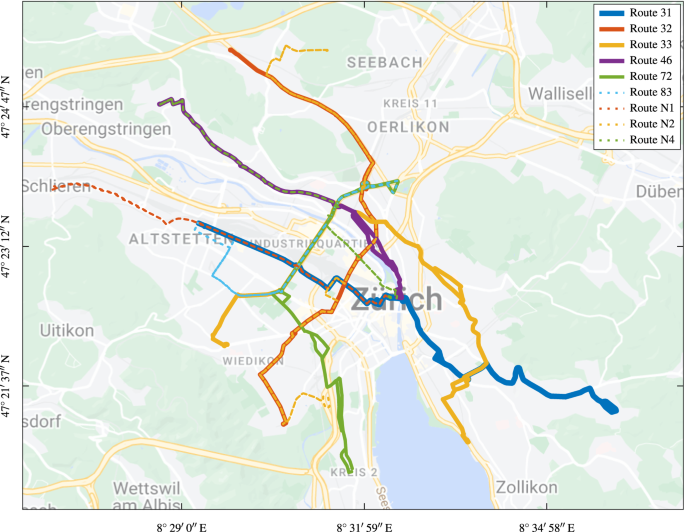
Path of the nine bus routes covered by the dataset. The route data is publicly available 18 . Map data ©2023 Google.
The data included in the ZTBus dataset 9 originates from the three systems described below and schematically shown in Fig. 1 . It is recorded via onboard logging systems specifically developed for that purpose.
The majority of the data is provided by the vehicle control unit (VCU) to which the raw measurement data is directly transmitted via multiple controller area network (CAN) buses from the various vehicle components. As this data is used during the normal operation of the bus, these signals are always available if the attached logging system works as intended.
The data related to the global positioning of the vehicles is provided by a GNSS antenna mounted on their roofs. The GNSS data may be temporarily unavailable if no reliable connection to the satellites can be established, which may be the case, e.g., during bad weather, between tall buildings, or in underpasses.
The passenger counts are estimated via onboard infrared-based passenger counting systems that transmit their estimates to the public transportation operator’s server computer via the local cellular network. This data is then automatically synchronized and augmented with the data from the intermodal transport control system (ITCS), i.e., the corresponding bus route number and stop names. We refer to this combined data as “ITCS data”.
The data is organized in “driving missions”, which we define as the entire period from the moment the bus is switched on until the moment it is switched off.
Selection of data records
To ensure that the dataset meets the requisite integrity and quality standards, we include only those records that represent complete driving missions during regular public transportation operation. For example, we reject test drives, short trips within depots, and missions that are completely missing any data from the three systems described above. The details of this selection step are described in the section on technical validation below.
We aim to reduce the processing of data to a minimum. In particular, instead of applying sophisticated filtering and smoothing techniques, we publish the raw measurement data received from the sensory devices, which allows its use for the development or tuning of such smoothing and filtering algorithms as well. The processing steps that were nevertheless considered necessary and were carried out are as follows:
On our vehicles, the most accurate indicator of the vehicle speed is provided by the rotational speed sensors mounted on the motor shafts. As we aim to present our data in a manner that is independent of the vehicle’s specific drivetrain, we use an estimate of the “compound” transmission ratio γ to convert the rotational speed measurements ω to the longitudinal vehicle speed v :
The compound transmission ratio thus combines the effects of the transmission, final drive, and wheel radius. For estimating γ , we analyze measurement data of perfectly straight driving sections, where the traveled distance according to filtered GNSS data is compared to the total angle covered by the electric machine. Note that we use the rotational speed measurements of the motor at the middle axle for the calculation of the travel speed in Eq. 1 . That way, errors induced due to offtracking during tight turns are minimized. The estimated transmission ratio is also used to calculate the traction force
where T trac represents the total torque provided by the electric machines. Since the vehicle acceleration in city buses is generally low so as to allow for a comfortable ride even for standing passengers, our experience shows that the error induced by tire slip is typically negligible.
To focus on the valuable information recorded between the initial departure and the final arrival of each driving mission, we discard any data recorded more than 1 min before and more than 1 min after the actual driving.
When combining numbers of passengers (estimated by the on-board infrared-based counting systems) with bus route and stop names (provided by the ITCS), some inconsistencies are filtered out by the public transportation operator. For example, trips are discarded if the total number of passengers boarding differs too much from the total number of passengers alighting throughout one trip, or if a recorded trip cannot be uniquely matched to a trip in the ITCS database. To exclude any remaining erroneous ITCS data, which may occur, for instance, when stops are skipped, we use the locations of the reported stops according to publicly available general transit feed specification (GTFS) data 18 and compare them to the location estimates provided by the GNSS sensors. If the locations are farther than 100 m apart for at least three stops in a row, the ITCS data associated with these stops is discarded.
Finally, the data from the three different sources introduced above, i.e., the VCU, GNSS, and the ITCS, is synchronized and resampled. For this purpose, we first generate a new date-time vector in coordinated universal time (UTC) with a uniform sampling period of 1 s covering the time window highlighted above. The signals are then mapped to this time vector as follows:
The ITCS data is only given at discrete time events approximately matching the moments the bus is leaving a stop. As the processing of the raw data is to be kept to a minimum, the ITCS data is not interpolated. Instead, the nearest sample times of the new date-time vector are identified and the discrete values are mapped accordingly.
All binary (status) signals are interpreted as piecewise constant signals and are thus resampled via previous neighbor interpolation.
All other signals are linearly interpolated.
Data Records
The ZTBus dataset is published on the repository for publications and research data of ETH Zurich 9 . It is organized in two different types of comma-separated values (CSV) text files, the first of which describes the 1409 individual driving missions, while the second contains metadata of all driving missions.
The names of the files that describe the individual driving missions are based on the vehicle identification number (either 183 or 208) and the time period in which the data was collected. For example, the data collected on the bus numbered 183 between 16 Oct 2019 02:52:43 and 16 Oct 2019 07:10:12, both given in UTC, is available in the following file:
B183_2019-10-16_02-52-43_2019-10-16_07-10-12.csv
The metadata describing all driving missions is provided as metaData.csv.
Detailed description of the time-resolved measurement data
The ZTBus dataset 9 consists of 1409 driving missions, each of which is described in a separate CSV file. All files have the same structure and format, where the first row contains the headers of the corresponding columns and the remaining rows describe the set of data samples recorded at a specific moment in time. This time index is represented in the first column as absolute UTC time, expressed according to ISO 8601.
The columns are described in Table 1 , where NaN represents unavailable data, unless specified otherwise.
Detailed description of the metadata
The metadata of the driving missions is tabulated as described in Table 2 . The first row contains the headers of the corresponding columns. The remaining rows contain metadata of the driving missions, indexed via the corresponding file name in the first column.
Technical Validation
In this section, we explain the various measures we have taken to ensure the requisite integrity and quality of the ZTBus dataset 9 . In particular, we have developed the following selection criteria.
We start by considering only records without any known issues in the logging toolchain, i.e., issues such as erroneous timestamps, or bugs in any of the involved software components. For this initial selection, we additionally reject records with corrupt file contents. Furthermore, we only consider records that span a time interval of at least 1 h each, as all shorter records do not represent any regular public transportation operation. This initial selection contains a total of 2046 missions.
We reject missions where either VCU, GNSS, or ITCS data is unavailable throughout the entire mission, which reduces the dataset by 189 missions.
If we detect a gap of at least 10 s in any of the VCU data, we reject the data of the entire mission, as this hints at a potential issue either in the logging toolchain or with the onboard clock. Such a gap is detected in 13 missions.
During normal operation, the bus is expected to be at a standstill for some time at both the beginning and the end of each record. If this is not the case, parts of the logging toolchain may have failed to start in time or might have terminated unexpectedly. Thus, we reject 152 missions, where the bus operation does not meet this standstill criterion.
In some of the records, the bus is found to be not driving at all. This might happen, for instance, if the bus is started during maintenance work. As such records do not represent a regular public transportation operation, they are rejected. This reduces the dataset by 42 missions.
To exclude any test drives and short missions to, from, or within a depot, we require each driving mission to last at least 3 h. This reduces the dataset by 211 missions.
During regular operation on the trolley bus routes covered in this dataset, no prolonged standstills are to be expected. Therefore, we filter all missions with any standstill time of more than 30 min. This reduces the dataset by 30 missions.
The selection criteria listed above were established iteratively over the years that we have been working on the data. We believe that these simple criteria are adequate to consistently remove all data records that are contaminated due to software malfunction or that are not representative of a regular public transportation operation, such as drives within a garage or to a workshop. In the following two subsections, we provide some visualizations of the data in the ZTBus dataset 9 . These visualizations offer valuable insights into the dataset’s contents and quality. Additionally, they help identify anomalies and outliers, thus guiding the determination of the data selection steps discussed above.
Time series inspection
Throughout the activities within our research projects 10 , 11 , we visually analyzed hundreds of time windows that are similar to the one shown in Fig. 4 . Such visualizations reveal, for example, the consistency of the wheel speed signals w.r.t. the steering and articulation angles. In particular, a left turn is evident at around second 40, with a negative articulation angle and a positive steering angle, according to the definitions given in Table 1 . Accordingly, as expected in a left turn, the right front wheel turns slightly faster than the left.
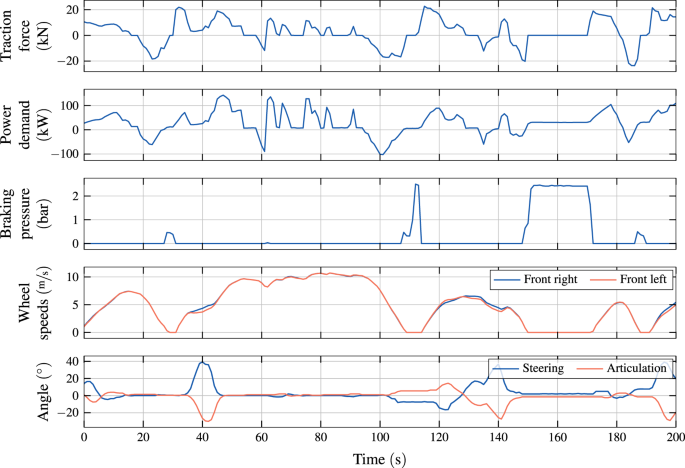
Detailed view on example time series recorded in the morning of 7 Mar 2021 on bus numbered 183 on route 72 of VBZ.
A slightly coarser time scale is used in Fig. 5 , which visualizes the proper synchronization of the VCU signals with the ITCS signals. This figure shows that the times at which the stop names and the passenger counts are reported correspond to the times at which the bus departs from the respective stops.
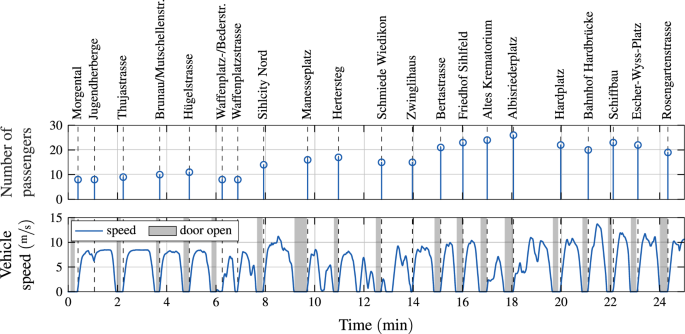
Exemplary ITCS data aligned with the speed profile recorded in the morning of 7 Mar 2021 on the bus numbered 183 on route 72 of VBZ. The names of the bus stops are shown above the graph. The areas shaded in gray indicate that at least one door is open.
Some signals are best visualized over the course of the daily operation of a bus, as exemplified in Fig. 6 . The repetitive nature of the driving profile is clearly observable in both the pronounced elevation profile of the depicted bus route 72 and the passenger volume. The measured ambient temperature indicates on the one hand that the bus started its mission directly from a depot whose temperature lies significantly above the outside temperature, and on the other hand that the thermal inertia experienced by the sensor is quite significant due to its placement behind bodywork. Due to the sensor resolution, the temperature is only available in increments of 1 K. However, as a result of the linear interpolation used during processing, the dataset may contain single temperature values between these increments.
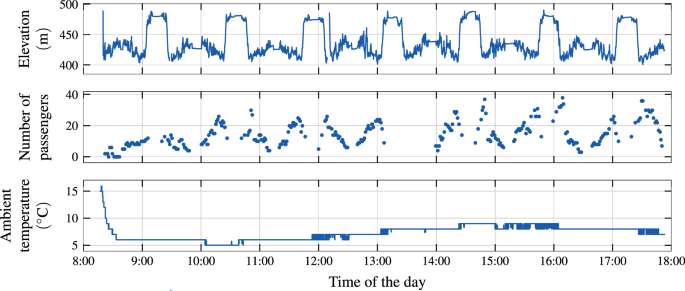
Example time series recorded throughout the day on 7 Mar 2021 on the bus numbered 183 on VBZ route 72.
The GNSS data deliberately was not modified and is provided as raw data, except for the linear interpolation necessary for time synchronization. Therefore, the data might be noisy or imprecise in some locations or may be missing completely during certain time windows. Hence, for certain types of applications, such data may have to be excluded or pre-processed by applying dedicated smoothing or filtering algorithms. Conversely, incomplete and imprecise data can be used as valuable training or validation data, e.g., for dead-reckoning and map-matching algorithms. Anyways, the GNSS data is mostly of good quality, as the exemplary visualization in Fig. 7 clearly shows. Despite the complicated installation of the overhead infrastructure and the footbridges around the crossing depicted, the quality of the raw measurements is by far sufficient to determine which roads were taken.
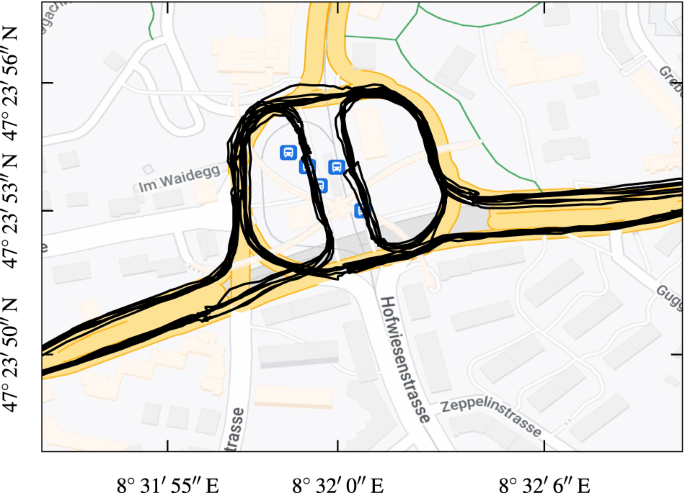
Example GNSS trajectories of 7 outbound and return trips each, recorded on 7 Mar 2021 around Bucheggplatz in Zurich, Switzerland. The square is an important transportation hub in the center of Zurich, connecting two tram routes, two bus routes, and three trolley bus routes. Map data ©2023 Google.
Statistical analysis
In order verify and validate the integrity of the individual signals, we perform a rudimentary statistical analysis on the large amount of collected data. In particular, we examine a multitude of histograms, three of which exemplified in Fig. 8 . Such an analysis of the respective minimum, mean, and maximum values of all driving missions lends itself to detect anomalies and outliers relatively quickly. These visualizations were a helpful tool in the development of the selection criteria mentioned above.
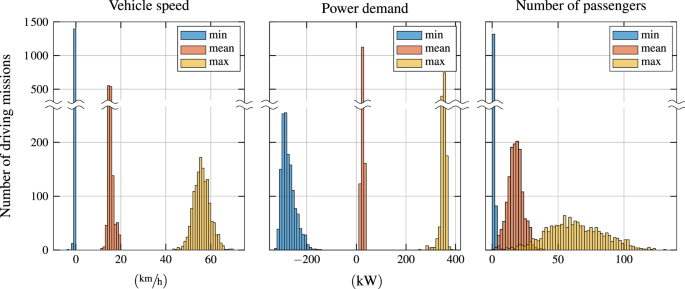
Example histograms granting a representative overview of all data values contained in the dataset. Each of the three categories “min”, “mean”, and “max” refers to the corresponding values of all driving missions, visualized as a histogram. For example, the data values shown for the minimum vehicle speed are the smallest values of odometry_vehicleSpeed of each driving mission. To increase the clarity of the graph, the scale of the y-axes changes above the indicated discontinuities.
An inspection of the three histograms shown in Fig. 8 reveals that the vehicle speed is slightly negative in some situations. This typically occurs when the vehicle is starting or stopping. From experience, we also know that the average speed of a transit bus in Zurich is around 15 km/h, while the maximum speed rarely exceeds 65 km/h. These facts are well represented and confirmed in the dataset. The distinct peaks shown in the maximum and minimum power demand levels are mainly due to the combined power capacity of the two electric motors of the buses, which is around 320 kW for both negative and positive values. Assuming some auxiliary power consumption in the range of 20 kW to 30 kW, these power limits perfectly explain the peaks observed at around −300 kW and 350 kW, respectively. The average power demand is in the range of 15 kW to 35 kW, which corresponds to the expected range of 1.5 kWh/km to 2.0 kWh/km for transit buses driving at the mean vehicle speed mentioned above. Finally, the distribution of the number of passenger shows that the average occupation ranges between 10 and 30 people. On most missions, the vehicle is both empty and about half full at least once.
Usage Notes
All files of the ZTBus dataset 9 described in this paper are provided in the CSV format using UTF-8 encoding. No special tools are necessary to load or interpret this data and most data processing tools can seamlessly work with data in this format. For convenience, sample Matlab code is provided along with the dataset to recreate the figures shown in this paper. This code can serve as a starting point for numerous new analyses.
Code availability
The code used to collect, store, filter, and synchronize the data is not published, as it can only be used with the raw data recorded on the specific prototype vehicles, which contains partly proprietary data. As large portions of the code deal with engineering challenges, such as translating data between formats used in different programming languages, ensuring compatibility between software versions, and performing operations in our custom log data base, we do not expect it to be interesting to the readers or useful for any other applications. Instead we directly explain the relevant processing and filtering steps in the respective sections above.
As mentioned above, no specific code is necessary to load or interpret the ZTBus dataset 9 . However, for convenience, the sample Matlab code provided allows to load some parts of the data and recreate most of the figures shown in this manuscript. The code has been developed with Matlab version 9.12 (R2022a) and does not require any specialized toolboxes. It is distributed under the GNU General Public License version 3 (GPLv3) alongside the ZTBus dataset 9 .
United Nations. World urbanization prospects (United Nations, 2014), https://doi.org/10.18356/527e5125-en .
Mon, E. E., Ochiai, H., Komolkiti, P. & Aswakul, C. Real-world sensor dataset for city inbound-outbound critical intersection analysis. Scientific Data 9 , https://doi.org/10.1038/s41597-022-01448-6 (2022).
Huang, Q. et al . The temporal geographically-explicit network of public transport in Changchun City, Northeast China. Scientific Data 6 , 190026, https://doi.org/10.1038/sdata.2019.26 (2019).
Article PubMed PubMed Central Google Scholar
Guo, F., Zhang, D., Dong, Y. & Guo, Z. Urban link travel speed dataset from a megacity road network. Scientific Data 6 , 61, https://doi.org/10.1038/s41597-019-0060-3 (2019).
Oh, G., Leblanc, D. J. & Peng, H. Vehicle energy dataset (VED), a large-scale dataset for vehicle energy consumption research. IEEE Transactions on Intelligent Transportation Systems 23 , 3302–3312, https://doi.org/10.1109/TITS.2020.3035596 (2022).
Article Google Scholar
Zhang, S., Fatih, D., Abdulqadir, F., Schwarz, T. & Ma, X. Extended vehicle energy dataset (eVED): An enhanced large-scale dataset for deep learning on vehicle trip energy consumption https://doi.org/10.48550/ARXIV.2203.08630 (2022).
Calearo, L., Marinelli, M. & Ziras, C. A review of data sources for electric vehicle integration studies. Renewable and Sustainable Energy Reviews 151 , 111518, https://doi.org/10.1016/j.rser.2021.111518 (2021).
Piorkowski, M., Sarafijanovic-Djukic, N. & Grossglauser, M. CRAWDAD dataset epfl/mobility (v. 2009-02-24). Downloaded from https://crawdad.org/epfl/mobility/20090224 , https://doi.org/10.15783/C7J010 (2009).
Widmer, F., Ritter, A. & Onder, CH. ZTBus: A Large Dataset of Time-Resolved City Bus Driving Missions, ETH Zurich , https://doi.org/10.3929/ethz-b-000626723 (2023).
Ritter, A. et al . SwissTrolley plus. Schlussbericht (F&E) SI/501321, Bundesamt für Energie BFE URL https://www.aramis.admin.ch/Grunddaten/?ProjectID=37064 (2019).
Widmer, F., Onder, C., Amacker, N. & Böhm, A. ISOTHERM. Schlussbericht (F&E) SI/501979, Bundesamt für Energie BFE URL https://www.aramis.admin.ch/Grunddaten/?ProjectID=44962 (2023).
Ritter, A., Widmer, F., Niam, J. W., Elbert, P. & Onder, C. Real-time graph construction algorithm for probabilistic predictions in vehicular applications. IEEE Transactions on Vehicular Technology 70 , 5483–5498, https://doi.org/10.1109/TVT.2021.3077063 (2021).
Ritter, A., Widmer, F., Vetterli, B. & Onder, C. H. Optimization-based online estimation of vehicle mass and road grade: Theoretical analysis and experimental validation. Mechatronics 80 , 102663, https://doi.org/10.1016/j.mechatronics.2021.102663 (2021).
Ritter, A., Widmer, F., Duhr, P. & Onder, C. H. Long-term stochastic model predictive control for the energy management of hybrid electric vehicles using Pontryagin’s minimum principle and scenario-based optimization. Applied Energy 322 , 119192, https://doi.org/10.1016/j.apenergy.2022.119192 (2022).
Widmer, F., Ritter, A., Duhr, P. & Onder, C. H. Battery lifetime extension through optimal design and control of traction and heating systems in hybrid drivetrains. eTransportation 14 , 100196, https://doi.org/10.1016/j.etran.2022.100196 (2022).
Widmer, F., Ritter, A., Ritzmann, J., Gerber, D. & Onder, C. H. Battery health target tracking for HEVs: Closed-loop control approach, simulation framework, and reference trajectory optimization. eTransportation 17 , 100244, https://doi.org/10.1016/j.etran.2023.100244 (2023).
Widmer, F. et al . Highly efficient year-round energy and comfort optimization of HVAC systems in electric city buses. https://doi.org/10.48550/arXiv.2303.00571. Preprint, presented at the 2023 IFAC World Congress (2023).
Zürcher Verkehrsverbund (ZVV). ZVV Fahrplan Tram und Bus (Static GTFS). Downloaded from https://opendata.swiss/perma/ec7bb57c-f0aa-4e8e-9266-f0b7112f6355@stadt-zurich (2023).
Download references
Acknowledgements
This work was supported by the SFOE (contract numbers SI/501321-01 and SI/501979-01) and the industrial partners Carrosserie HESS AG and VBZ.
Author information
These authors contributed equally: Fabio Widmer, Andreas Ritter.
Authors and Affiliations
ETH Zurich, Institute for Dynamic Systems and Control, Zurich, 8092, Switzerland
Fabio Widmer, Andreas Ritter & Christopher H. Onder
You can also search for this author in PubMed Google Scholar
Contributions
F.W. and A.R. developed the methodology and software, curated, analyzed, and visualized the data, and wrote the original draft. C.O. was responsible for funding acquisition and supervision. All authors reviewed the manuscript.
Corresponding author
Correspondence to Fabio Widmer .
Ethics declarations
Competing interests.
The authors declare no competing interests.
Additional information
Publisher’s note Springer Nature remains neutral with regard to jurisdictional claims in published maps and institutional affiliations.
Rights and permissions
Open Access This article is licensed under a Creative Commons Attribution 4.0 International License, which permits use, sharing, adaptation, distribution and reproduction in any medium or format, as long as you give appropriate credit to the original author(s) and the source, provide a link to the Creative Commons licence, and indicate if changes were made. The images or other third party material in this article are included in the article’s Creative Commons licence, unless indicated otherwise in a credit line to the material. If material is not included in the article’s Creative Commons licence and your intended use is not permitted by statutory regulation or exceeds the permitted use, you will need to obtain permission directly from the copyright holder. To view a copy of this licence, visit http://creativecommons.org/licenses/by/4.0/ .
Reprints and permissions
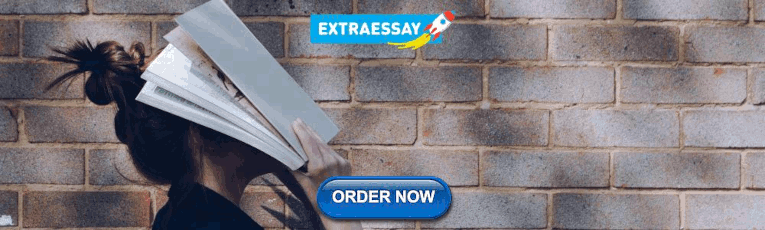
About this article
Cite this article.
Widmer, F., Ritter, A. & Onder, C.H. ZTBus: A Large Dataset of Time-Resolved City Bus Driving Missions. Sci Data 10 , 687 (2023). https://doi.org/10.1038/s41597-023-02600-6
Download citation
Received : 15 March 2023
Accepted : 26 September 2023
Published : 10 October 2023
DOI : https://doi.org/10.1038/s41597-023-02600-6
Share this article
Anyone you share the following link with will be able to read this content:
Sorry, a shareable link is not currently available for this article.
Provided by the Springer Nature SharedIt content-sharing initiative
Quick links
- Explore articles by subject
- Guide to authors
- Editorial policies
Sign up for the Nature Briefing newsletter — what matters in science, free to your inbox daily.

Machine Learning for Bus Travel Prediction
- Conference paper
- First Online: 15 June 2022
- Cite this conference paper
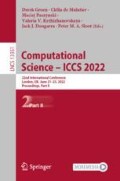
- Łukasz Pałys 13 ,
- Maria Ganzha ORCID: orcid.org/0000-0001-7714-4844 13 &
- Marcin Paprzycki ORCID: orcid.org/0000-0002-8069-2152 14
Part of the book series: Lecture Notes in Computer Science ((LNCS,volume 13351))
Included in the following conference series:
- International Conference on Computational Science
996 Accesses
3 Citations
Nowadays, precise data of movements of public transport can be collected. Specifically, for each bus, geoposition can be regularly obtained and stored. In this context, an attempt to build a model to represent behavior of busses, and predict their delays, is discussed.
This is a preview of subscription content, log in via an institution to check access.
Access this chapter
- Available as PDF
- Read on any device
- Instant download
- Own it forever
- Available as EPUB and PDF
- Compact, lightweight edition
- Dispatched in 3 to 5 business days
- Free shipping worldwide - see info
Tax calculation will be finalised at checkout
Purchases are for personal use only
Institutional subscriptions
https://api.um.warszawa.pl/ .
https://www.wtp.waw.pl .
All reported models have been implemented in Python, using Keras library.
Fan, W., Gurmu, Z.: Dynamic travel time prediction models for buses using only GPS data. Int. J. Transp. Sci. Technol. 4 (4), 353–366 (2015). https://www.sciencedirect.com/science/article/pii/S204604301630168X
Dahl, E., Sjåfjell, A., Skogen, S.: On implementations of bus travel time prediction utilizing methods in artificial intelligence, NUST (2014)
Google Scholar
Sjåfjell, A., Dahl, E., Skogen, S.: Intelligent transportation systems and artificial intelligence - a state of the art review, NUST (2013)
Lin, Y., Yang, X., Zou, N., Jia, L.: Real-time bus arrival time prediction: case study for Jinan, China. J. Transp. Eng. 139 (11), 1133–1140 (2013)
Zychowski, A., Junosza-Szaniawski, K., Kosicki, A.: Travel time prediction for trams in Warsaw. In: Kurzynski, M., Wozniak, M., Burduk, R. (eds.) CORES 2017. AISC, vol. 578, pp. 53–62. Springer, Cham (2018). https://doi.org/10.1007/978-3-319-59162-9_6
Chapter Google Scholar
Yang, J.S.: Travel time prediction using the GPS test vehicle and Kalman filtering techniques. In: Proceedings of the 2005, American Control Conference, 2005, pp. 2128–2133. IEEE (2005)
Jiwon, M., Kim, D., Kho, S., Park, C.: Travel time prediction using k nearest neighbor method with combined data from vehicle detector system and automatic toll collection system. Transp. Res. Rec. J. Transp. Res. Board 2256 , 51–59 (2012)
Haykin, S.: Neural Networks: A Comprehensive Foundation. Prentice Hall PTR, Upper Saddle River, NJ, USA (1998)
MATH Google Scholar
Shaji, H.E., Tangirala, A.K., Vanajakshi, L.: Prediction of trends in bus travel time using spatial patterns. Transp. Res. Procedia 48 , 998–1007 (2020)
Article Google Scholar
Comi, A., Zhuk, M., Kovalyshyn, V., Hilevych, V.: Investigating bus travel time and predictive models: a time series-based approach. Transp. Res. Procedia 45 , 692–699 (2020)
Fei, J., Lu, Y., Guo, Y., Zhang, H.: Predicting bus arrival time using BP neural network and dynamic transfer. Procedia Comput. Sci. 174 , 95–100 (2019)
Wang, L., Zuo, Z., Fu, J.: Bus arrival time prediction using RBF neural networks adjusted by online data. Procedia. Soc. Behav. Sci. 138 , 67–75 (2014)
Wang, L., Zuo, Z., Fu, J.: Bus dynamic travel time prediction: using a deep feature extraction framework based on RNN and DNN. Electronics 9 (11), 1876 (2020). https://doi.org/10.3390/electronics9111876 . https://www.mdpi.com/2079-9292/9/11/1876
Vidnerová, P.: RBF-Keras: an RBF Layer for Keras Library (2019). https://github.com/PetraVidnerova/rbf_keras
Pałys, Ł., Ganzha, M., Paprzycki, M.: Applying machine learning to predict behavior of bus transport in Warsaw, Poland. https://arxiv.org/submit/4254888
Download references
Author information
Authors and affiliations.
Warsaw University of Technology, Warsaw, Poland
Łukasz Pałys & Maria Ganzha
Systems Research Institute Polish Academy of Sciences, Warsaw, Poland
Marcin Paprzycki
You can also search for this author in PubMed Google Scholar
Corresponding author
Correspondence to Maria Ganzha .
Editor information
Editors and affiliations.
Brunel University London, London, UK
Derek Groen
University of Amsterdam, Amsterdam, The Netherlands
Clélia de Mulatier
AGH University of Science and Technology, Krakow, Poland
Maciej Paszynski
Valeria V. Krzhizhanovskaya
University of Tennessee at Knoxville, Knoxville, TN, USA
Jack J. Dongarra
Peter M. A. Sloot
Rights and permissions
Reprints and permissions
Copyright information
© 2022 The Author(s), under exclusive license to Springer Nature Switzerland AG
About this paper
Cite this paper.
Pałys, Ł., Ganzha, M., Paprzycki, M. (2022). Machine Learning for Bus Travel Prediction. In: Groen, D., de Mulatier, C., Paszynski, M., Krzhizhanovskaya, V.V., Dongarra, J.J., Sloot, P.M.A. (eds) Computational Science – ICCS 2022. ICCS 2022. Lecture Notes in Computer Science, vol 13351. Springer, Cham. https://doi.org/10.1007/978-3-031-08754-7_72
Download citation
DOI : https://doi.org/10.1007/978-3-031-08754-7_72
Published : 15 June 2022
Publisher Name : Springer, Cham
Print ISBN : 978-3-031-08753-0
Online ISBN : 978-3-031-08754-7
eBook Packages : Computer Science Computer Science (R0)
Share this paper
Anyone you share the following link with will be able to read this content:
Sorry, a shareable link is not currently available for this article.
Provided by the Springer Nature SharedIt content-sharing initiative
- Publish with us
Policies and ethics
- Find a journal
- Track your research
Help | Advanced Search
Computer Science > Computation and Language
Title: uni-smart: universal science multimodal analysis and research transformer.
Abstract: In scientific research and its application, scientific literature analysis is crucial as it allows researchers to build on the work of others. However, the fast growth of scientific knowledge has led to a massive increase in scholarly articles, making in-depth literature analysis increasingly challenging and time-consuming. The emergence of Large Language Models (LLMs) has offered a new way to address this challenge. Known for their strong abilities in summarizing texts, LLMs are seen as a potential tool to improve the analysis of scientific literature. However, existing LLMs have their own limits. Scientific literature often includes a wide range of multimodal elements, such as molecular structure, tables, and charts, which are hard for text-focused LLMs to understand and analyze. This issue points to the urgent need for new solutions that can fully understand and analyze multimodal content in scientific literature. To answer this demand, we present Uni-SMART (Universal Science Multimodal Analysis and Research Transformer), an innovative model designed for in-depth understanding of multimodal scientific literature. Through rigorous quantitative evaluation across several domains, Uni-SMART demonstrates superior performance over leading text-focused LLMs. Furthermore, our exploration extends to practical applications, including patent infringement detection and nuanced analysis of charts. These applications not only highlight Uni-SMART's adaptability but also its potential to revolutionize how we interact with scientific literature.
Submission history
Access paper:.
- HTML (experimental)
- Other Formats

References & Citations
- Google Scholar
- Semantic Scholar
BibTeX formatted citation

Bibliographic and Citation Tools
Code, data and media associated with this article, recommenders and search tools.
- Institution
arXivLabs: experimental projects with community collaborators
arXivLabs is a framework that allows collaborators to develop and share new arXiv features directly on our website.
Both individuals and organizations that work with arXivLabs have embraced and accepted our values of openness, community, excellence, and user data privacy. arXiv is committed to these values and only works with partners that adhere to them.
Have an idea for a project that will add value for arXiv's community? Learn more about arXivLabs .
- Share full article
Advertisement
Supported by
What the Data Says About Pandemic School Closures, Four Years Later
The more time students spent in remote instruction, the further they fell behind. And, experts say, extended closures did little to stop the spread of Covid.

By Sarah Mervosh , Claire Cain Miller and Francesca Paris
Four years ago this month, schools nationwide began to shut down, igniting one of the most polarizing and partisan debates of the pandemic.
Some schools, often in Republican-led states and rural areas, reopened by fall 2020. Others, typically in large cities and states led by Democrats, would not fully reopen for another year.
A variety of data — about children’s academic outcomes and about the spread of Covid-19 — has accumulated in the time since. Today, there is broad acknowledgment among many public health and education experts that extended school closures did not significantly stop the spread of Covid, while the academic harms for children have been large and long-lasting.
While poverty and other factors also played a role, remote learning was a key driver of academic declines during the pandemic, research shows — a finding that held true across income levels.
Source: Fahle, Kane, Patterson, Reardon, Staiger and Stuart, “ School District and Community Factors Associated With Learning Loss During the COVID-19 Pandemic .” Score changes are measured from 2019 to 2022. In-person means a district offered traditional in-person learning, even if not all students were in-person.
“There’s fairly good consensus that, in general, as a society, we probably kept kids out of school longer than we should have,” said Dr. Sean O’Leary, a pediatric infectious disease specialist who helped write guidance for the American Academy of Pediatrics, which recommended in June 2020 that schools reopen with safety measures in place.
There were no easy decisions at the time. Officials had to weigh the risks of an emerging virus against the academic and mental health consequences of closing schools. And even schools that reopened quickly, by the fall of 2020, have seen lasting effects.
But as experts plan for the next public health emergency, whatever it may be, a growing body of research shows that pandemic school closures came at a steep cost to students.
The longer schools were closed, the more students fell behind.
At the state level, more time spent in remote or hybrid instruction in the 2020-21 school year was associated with larger drops in test scores, according to a New York Times analysis of school closure data and results from the National Assessment of Educational Progress , an authoritative exam administered to a national sample of fourth- and eighth-grade students.
At the school district level, that finding also holds, according to an analysis of test scores from third through eighth grade in thousands of U.S. districts, led by researchers at Stanford and Harvard. In districts where students spent most of the 2020-21 school year learning remotely, they fell more than half a grade behind in math on average, while in districts that spent most of the year in person they lost just over a third of a grade.
( A separate study of nearly 10,000 schools found similar results.)
Such losses can be hard to overcome, without significant interventions. The most recent test scores, from spring 2023, show that students, overall, are not caught up from their pandemic losses , with larger gaps remaining among students that lost the most ground to begin with. Students in districts that were remote or hybrid the longest — at least 90 percent of the 2020-21 school year — still had almost double the ground to make up compared with students in districts that allowed students back for most of the year.
Some time in person was better than no time.
As districts shifted toward in-person learning as the year went on, students that were offered a hybrid schedule (a few hours or days a week in person, with the rest online) did better, on average, than those in places where school was fully remote, but worse than those in places that had school fully in person.
Students in hybrid or remote learning, 2020-21
80% of students
Some schools return online, as Covid-19 cases surge. Vaccinations start for high-priority groups.
Teachers are eligible for the Covid vaccine in more than half of states.
Most districts end the year in-person or hybrid.
Source: Burbio audit of more than 1,200 school districts representing 47 percent of U.S. K-12 enrollment. Note: Learning mode was defined based on the most in-person option available to students.
Income and family background also made a big difference.
A second factor associated with academic declines during the pandemic was a community’s poverty level. Comparing districts with similar remote learning policies, poorer districts had steeper losses.
But in-person learning still mattered: Looking at districts with similar poverty levels, remote learning was associated with greater declines.
A community’s poverty rate and the length of school closures had a “roughly equal” effect on student outcomes, said Sean F. Reardon, a professor of poverty and inequality in education at Stanford, who led a district-level analysis with Thomas J. Kane, an economist at Harvard.
Score changes are measured from 2019 to 2022. Poorest and richest are the top and bottom 20% of districts by percent of students on free/reduced lunch. Mostly in-person and mostly remote are districts that offered traditional in-person learning for more than 90 percent or less than 10 percent of the 2020-21 year.
But the combination — poverty and remote learning — was particularly harmful. For each week spent remote, students in poor districts experienced steeper losses in math than peers in richer districts.
That is notable, because poor districts were also more likely to stay remote for longer .
Some of the country’s largest poor districts are in Democratic-leaning cities that took a more cautious approach to the virus. Poor areas, and Black and Hispanic communities , also suffered higher Covid death rates, making many families and teachers in those districts hesitant to return.
“We wanted to survive,” said Sarah Carpenter, the executive director of Memphis Lift, a parent advocacy group in Memphis, where schools were closed until spring 2021 .
“But I also think, man, looking back, I wish our kids could have gone back to school much quicker,” she added, citing the academic effects.
Other things were also associated with worse student outcomes, including increased anxiety and depression among adults in children’s lives, and the overall restriction of social activity in a community, according to the Stanford and Harvard research .
Even short closures had long-term consequences for children.
While being in school was on average better for academic outcomes, it wasn’t a guarantee. Some districts that opened early, like those in Cherokee County, Ga., a suburb of Atlanta, and Hanover County, Va., lost significant learning and remain behind.
At the same time, many schools are seeing more anxiety and behavioral outbursts among students. And chronic absenteeism from school has surged across demographic groups .
These are signs, experts say, that even short-term closures, and the pandemic more broadly, had lasting effects on the culture of education.
“There was almost, in the Covid era, a sense of, ‘We give up, we’re just trying to keep body and soul together,’ and I think that was corrosive to the higher expectations of schools,” said Margaret Spellings, an education secretary under President George W. Bush who is now chief executive of the Bipartisan Policy Center.
Closing schools did not appear to significantly slow Covid’s spread.
Perhaps the biggest question that hung over school reopenings: Was it safe?
That was largely unknown in the spring of 2020, when schools first shut down. But several experts said that had changed by the fall of 2020, when there were initial signs that children were less likely to become seriously ill, and growing evidence from Europe and parts of the United States that opening schools, with safety measures, did not lead to significantly more transmission.
“Infectious disease leaders have generally agreed that school closures were not an important strategy in stemming the spread of Covid,” said Dr. Jeanne Noble, who directed the Covid response at the U.C.S.F. Parnassus emergency department.
Politically, though, there remains some disagreement about when, exactly, it was safe to reopen school.
Republican governors who pushed to open schools sooner have claimed credit for their approach, while Democrats and teachers’ unions have emphasized their commitment to safety and their investment in helping students recover.
“I do believe it was the right decision,” said Jerry T. Jordan, president of the Philadelphia Federation of Teachers, which resisted returning to school in person over concerns about the availability of vaccines and poor ventilation in school buildings. Philadelphia schools waited to partially reopen until the spring of 2021 , a decision Mr. Jordan believes saved lives.
“It doesn’t matter what is going on in the building and how much people are learning if people are getting the virus and running the potential of dying,” he said.
Pandemic school closures offer lessons for the future.
Though the next health crisis may have different particulars, with different risk calculations, the consequences of closing schools are now well established, experts say.
In the future, infectious disease experts said, they hoped decisions would be guided more by epidemiological data as it emerged, taking into account the trade-offs.
“Could we have used data to better guide our decision making? Yes,” said Dr. Uzma N. Hasan, division chief of pediatric infectious diseases at RWJBarnabas Health in Livingston, N.J. “Fear should not guide our decision making.”
Source: Fahle, Kane, Patterson, Reardon, Staiger and Stuart, “ School District and Community Factors Associated With Learning Loss During the Covid-19 Pandemic. ”
The study used estimates of learning loss from the Stanford Education Data Archive . For closure lengths, the study averaged district-level estimates of time spent in remote and hybrid learning compiled by the Covid-19 School Data Hub (C.S.D.H.) and American Enterprise Institute (A.E.I.) . The A.E.I. data defines remote status by whether there was an in-person or hybrid option, even if some students chose to remain virtual. In the C.S.D.H. data set, districts are defined as remote if “all or most” students were virtual.
An earlier version of this article misstated a job description of Dr. Jeanne Noble. She directed the Covid response at the U.C.S.F. Parnassus emergency department. She did not direct the Covid response for the University of California, San Francisco health system.
How we handle corrections
Sarah Mervosh covers education for The Times, focusing on K-12 schools. More about Sarah Mervosh
Claire Cain Miller writes about gender, families and the future of work for The Upshot. She joined The Times in 2008 and was part of a team that won a Pulitzer Prize in 2018 for public service for reporting on workplace sexual harassment issues. More about Claire Cain Miller
Francesca Paris is a Times reporter working with data and graphics for The Upshot. More about Francesca Paris
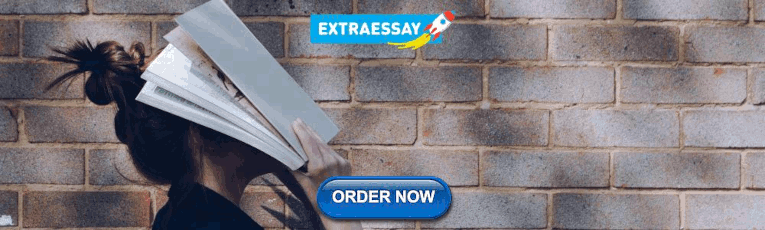
IMAGES
VIDEO
COMMENTS
This paper presents a survey of ML-based solutions for public bus transportation and details the modeling of these solutions (e.g., data types, ML algorithms). In addition, the problems tackled in the literature are categorized into four themes, and the solutions proposed to deal with them are schematized, highlighting problems that are little ...
This paper proposes two new encoders for data bus inversion (DBI), which conventionally uses a majority voter to pick a data representation that minimizes switching activities and thus reduces the corresponding energy consumption. The new encoders employ simpler approximate voters comprising only two gate levels, which improve latency more than twice while still achieving switching activity ...
Source Normalized Impact per Paper ... this refers to the collection of daily ridership data carried out by bus drivers using the mobile application. This data is continuously saved on the Realtime Database. ... Brands, T., de Romph, E. (2015). Short-term prediction of ridership on public transport with smart card data. Transportation Research ...
With the maturity of big data technology, analyzing residents' travel habits and tracks has become an important research direction in the field of intelligent transportation study. In this paper, based on the subway and bus ride data, a subway-bus double-layer network model was established using complex network theory, taking the optimal traffic efficiency as the goal, the structure of ...
This paper delves its roots in a vast research endeavor aimed at granting cyber resilience of current and future assets. Within such a broad and challenging domain, the work has a very precise scope: to address the vulnerabilities of a specific avionic platform protocol, namely the MIL-STD-1553 standard. ... Indeed, the 1553 data bus protocol ...
This paper aims at underscoring the momentum gained by Big Data for the transportation and mobility industry by surveying and analysing the latest research efforts related to this noted synergy. ... proposes a methodology based on four processes that collect and merge data from different sources into predefined data classes. Research efforts ...
Regarding the above reviewed papers, two main research gaps are identified here. First, sample segmentation is not implemented in some of them, which might lead to unstable prediction results caused by mixed distributions. ... The data set was collected by bus card readers and cameras from 159 bus lines in Shenzhen, a metropolis in China ...
Data cleaning is the foundation for downstream applications and is one of the most important stages of the data lifecycle. According to research, data scientists and analysts spend more than 80% of the time cost on data cleaning in their data analysis projects [].With the development of big data technology and industrial digitalization, the topics of DQ have attracted more and more attention.
This paper aims to review research on applications of AI that can be used to improve PT, i.e., to make PT better in some way. This ... de Almeida MC (2020) Data-driven solution for planning bus routes of the public transport in UNICAMP. In: Proceedings of the 33rd international conference on efficiency, cost, optimization, simulation and ...
This paper formulates the car-specific metro train crowding prediction problem based on real-time load data and evaluates the performance of several data-driven prediction methods (lasso, stepwise ...
The analysis of extensive vehicle location data in an urban bus system requires an efficient data-driven method so that its output can be used to improve both the information and operational reliabilities of the bus transit services. This research aims to design and implement an integrated model for forecasting bus arrival times using artificial intelligence techniques. The novelty of the ...
Research using big data and other disruptive technologies are flourishing in several fields, like healthcare (Abdel-Basset et al., 2021) and tourism (Liu et al., 2018). This paper is part of that trend, using big data and artificial intelligence to support transport planners.
The experimental results show that the MALNSN algorithm proposed in this paper can not only ensure the stability of the algorithm, but also formulate a reasonable route optimization strategy in a shorter time, effectively reducing the consumption of transport capacity resources, improving the operation efficiency of public transport and increasing the accessibility ofpublic transport. With the ...
Abstract. This paper presents the Zurich Transit Bus (ZTBus) dataset, which consists of data recorded during driving missions of electric city buses in Zurich, Switzerland. The data was collected ...
As a part of the project Open data in Warsaw Footnote 1, exact location of public buses, reported in real time, is available.From there, 30 days of data reporting bus movements was harvested (total of about 10 GB of data). Data was filtered, retaining: (1) line number, (2) departure time from the last stop, (3) current percentage of distance traveled between adjacent stops, (4) time of the ...
This paper, therefore, addresses the high packet loss experienced in bus topology by investigating the performance of bus topology in four practical scenarios consisting of 10, 20, 30, and 40 ...
Forecasting passenger flow is a time-series research field because bus data points (including smartcard and meteorology data) are indexed in time order and are therefore time-series data. A time series is a sequence of discrete time data, and the use of a time series model can help organizations understand the underlying causes of trends and ...
A large body of research in bus arrival/travel time prediction can be categorized as: ... The data used in this paper was the bus transit data collected from No. 261 bus route in Guangzhou, a cross-city route serving the eastern suburbs and the central business district (CBD) area, and the FCD of corresponding areas during the period from 1st ...
The data preparation involved in the paper contains bus smart card transaction data, bus location data, travel chain data and bus station data from May 1, 2020 to June 30, 2020, which was provided by Beijing Municipal Transportation Commission. In order to match the temporal and spatial relations, the data was pre-processed.
MAvdhutSalunk" have impl emented "Real Time Web Based. Bus Tracki ng System" The proposed syst em reduces the. waiting time of remote users for bus. A system is used to track. the bus at any ...
This research paper delves into the transformative potential of Vehicle-to-Grid (V2G) technology in the context of school bus transportation, focusing on enhancing energy efficiency and ...
To map eGrid electricity regions to the transit bus data for each agency, ... While we examined conventional buses in this paper, research has also shown that shared automated vehicles could improve equity in transit systems with reduced costs by acting as a complement to existing transit service to expand mobility and access. Continued ...
"Real-Time Bus Tracking System Based on IoT and GPS" by R. Velu and S. Venkatesan (2019): This research paper proposes a real-time bus tracking system using IoT and GPS technologies. The study focuses on the integration of GPS modules in buses to track their locations and transmit the data to a central server. The system also includes a web-based
In this work, we discuss building performant Multimodal Large Language Models (MLLMs). In particular, we study the importance of various architecture components and data choices. Through careful and comprehensive ablations of the image encoder, the vision language connector, and various pre-training data choices, we identified several crucial design lessons. For example, we demonstrate that ...
We present Stable Video 3D (SV3D) -- a latent video diffusion model for high-resolution, image-to-multi-view generation of orbital videos around a 3D object. Recent work on 3D generation propose techniques to adapt 2D generative models for novel view synthesis (NVS) and 3D optimization. However, these methods have several disadvantages due to either limited views or inconsistent NVS, thereby ...
Attack Mitigation. For a modern vehicle, if the sensor in a vehicle anti-lock braking system (ABS) or controller area network (CAN) bus is attacked during a brake process, the vehicle will lose driving direction control and the driver's life will be highly threatened. However, current methods for detecting attacks are not sufficiently ...
The interview data collection was anonymous. Reporting and quoting in the paper are also anonymous. The paper is part of the research project "App Cities". Ethics approval was received by the Norwegian Center for Research Data (NSD), prior to the beginning of this research, with reference number 869419.
In scientific research and its application, scientific literature analysis is crucial as it allows researchers to build on the work of others. However, the fast growth of scientific knowledge has led to a massive increase in scholarly articles, making in-depth literature analysis increasingly challenging and time-consuming. The emergence of Large Language Models (LLMs) has offered a new way to ...
Battery states are very important for the safe and reliable use of new energy vehicles. The estimation of power battery states has become a research hotspot in the development of electric buses and transportation safety management. This paper summarizes the basic workflow of battery states estimation tasks, compares, and analyzes the advantages and disadvantages of three types of data sources ...
The A.E.I. data defines remote status by whether there was an in-person or hybrid option, even if some students chose to remain virtual. In the C.S.D.H. data set, districts are defined as remote ...