- Your Stories
Evidence Based Living
Bridging the gap between research and real life
- In The Media
- The Learning Center
- Cornell Cooperative Extension
- Youth Development
- Health and Wellness
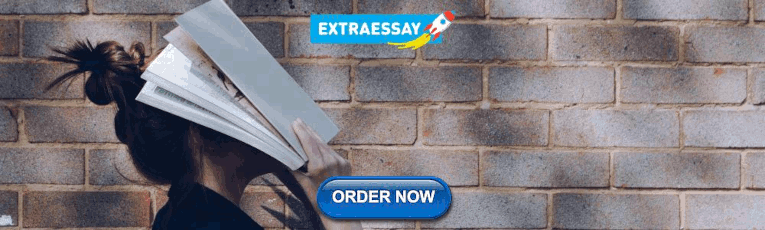
What is translational research?

Cornell’s College of Human Ecology is pursuing a translational research model to better link social and behavioral science research to extension and outreach, creating a more seamless link between science and service. But the question arises: What is “translational research?”
Evidence-Based Living sat down with Wethington to talk about the growing field of translational research.
To start off, what exactly is translational research?
Many definitions have been given for translational research, but the definition I like best is that it is a systematic effort to convert basic research knowledge into practical applications to enhance human health and well being.
Translational research was designed for the medical world. It emerged in response to concern over the long time lag between scientific discoveries and changes in treatments, practices, and health policies that incorporate the new discoveries.
What is applied research, and how does it differ?
Translational research is broader than the traditional term “applied research.” Applied research is any research that may possibly be useful for enhancing health or well-being. It does not necessarily have to have any effort connected with it to take the research to a practical level.
For example, an applied research study might analyze longitudinal data that tracks participants’ health and social relationships. The researchers would report their findings in an academic journal.
But in translational research, the same study would include some “action steps.” The researchers would partner with a community and ask for ideas about how their findings might apply there. Together, they would come up with an intervention plan that would also include scientific evaluation of its effectiveness.
Why are social science researchers slower to adopt these models compared to the medical community?
I think the answer to this question is that researchers have followed where the money has been allocated. The opportunities for social and behavioral scientists have not been established as rapidly.
More recently, three major government institutions have been funding projects that emphasize public health outreach using translational research – the Centers for Disease Control, the National Institutes of Health and the National Institute on Aging. All three have been establishing translational research centers across the country, primarily focused on underserved communities and health disparities.
Thus, social scientists are only now being encouraged to take part. More recently economic stimulus funds dispersed the National Institute of Health funded a number of translational research projects headed by social scientists, including three funded at Cornell. I predict that soon there will be social scientists engaged in translational research across the country, not just at funded centers.
What are the benefits of moving toward translational research?
For researchers, there is benefit to being affiliated with a center that provides seed funding for projects, methodological assistance, advice on developing proposals and experience in getting community input into research projects.
For universities, translational research centers provide a tactical advantage for attracting more funding. Translational research centers also provide a way for universities to meet public service goals in their strategic plans.
For communities, translational research provides opportunities to make a difference in their own communities. As part of one of the Cornell centers, we engaged public service agency directors in events where they could contribute to our research agenda. With a stake in the research, communities feel that they are making a valued and important contribution. We heard over and over from the community members that this was a real source of pride and accomplishment for them.
How can extension programs participate?
One way local extension programs can participate in translational research is to take part in community stakeholder groups that meet with researchers who are designing intervention and prevention research programs. Typically, a wide variety of stakeholders need to be engaged. County Cooperative Extension offices have many collaborative relationships in their counties and can work with researchers to make contacts.
Typically, local extension professionals do not have time to engage in research themselves. Yet they have valuable experience that can be shared. This makes Cooperative Extension an ideal contributor for implementing programs.
Nice reading! Thank you
A remarkable extraordinary read, ideally other huge schools can take an interest in something like this.
Quite the great read, hopefully other large schools are able to participate in something like this.
Speak Your Mind Cancel reply
A project at…, evidence-based blogs.
- Bandolier Evidence-Based Health Care
- British Psychological Society Research Digest
- Economics in Cooperative Extension
- EPB Exchange
- New Voices for Research
- Research Blogging
- Trust the Evidence
Subscribe by e-mail
Please, insert a valid email.
Thank you, your email will be added to the mailing list once you click on the link in the confirmation email.
Spam protection has stopped this request. Please contact site owner for help.
This form is protected by reCAPTCHA and the Google Privacy Policy and Terms of Service apply.
Return to top of page
Copyright © 2024 · Magazine Theme on Genesis Framework · WordPress · Log in
Introduction: What Is Translational Research
- First Online: 01 January 2014
Cite this chapter
- Dennis V. Cokkinos MD, FESC 2
1269 Accesses
1 Citations
There are many definitions of Research, Basic, Clinical and Translational Research. A very practical and short definition of Translational Research could be, the application of findings from Basic Research to patient, community and population care and to the advancement of the delivery of health services.
Usually three steps can be defined:
T1 (T for translation): is the transfer of new understanding of disease mechanisms gained in the laboratory into the development of new methods for diagnosis and therapy.
T2 is the translation of results from clinical studies into everyday clinical practice and health decision making.
T3 is the dissemination and implementation of research translation into practice/community/large populations.
Corresponding blocks or impediments are delineated to the successful employment of these steps. A newly introduced concept is the Valley of Death, separating research results from successful innovation-application.
To overcome these problems, the foundation and collaboration of centers able to conduct Translational Research, such as the National Institutes of Health and the National Clinical and Translational Science Award Consortium is important.
The teaching, training, and formation of translational researchers is difficult, varied and a matter of constant effort.
To overcome increasing costs the combination of “wet” –i.e. biological labs with “dry” or computational data is being increasingly employed.
This is a preview of subscription content, log in via an institution to check access.
Access this chapter
- Available as PDF
- Read on any device
- Instant download
- Own it forever
- Available as EPUB and PDF
- Compact, lightweight edition
- Dispatched in 3 to 5 business days
- Free shipping worldwide - see info
- Durable hardcover edition
Tax calculation will be finalised at checkout
Purchases are for personal use only
Institutional subscriptions
Abbreviations
Association for Clinical Research Training
Academic Health Centers
Biomedical Research Foundation Academy of Athens
Clinical and Translational Science Awards
General Clinical Research Centers
National Center for Advancing Translating Services
Translational Research Institute
National Institutes of Health
Translational Research
NSF. Definition of research. National Science Foundation 2007. http://www.Nsf.gov/statistics/randdef/business. cfm .
Oxford English Dictionary. www.oed.com .
Popper KR. Objective knowledge. 2nd ed. Oxford: Clarendon press; 1972. p. 191–205.
Google Scholar
Illing J. Thinking about research: frameworkers, ethics and scholarships. Edinburgh: Association for the Study of Medical Education; 2007. p. 2–37.
Bush V. Science: the endless frontier; a report to the President by Vannevar Bush, Director of the Office of Scientific research and Development. Washington, DC: United states Government Printing Office; 1945. Accessed 13 Nov 2009. Section 3 (the importance of basic research), chapter 3 (science and the public welfare) http://nsf.gov/about/history/nsf50/vbush1945_content.jsp .
Hecker L, Birla RV. Intangible factors leading to success in research: strategy, innovation and leadership. J Cardiovasc Transl Res. 2008;1:85–92.
Article PubMed Google Scholar
Glossary of themes for human subjects protection and inclusion issues based on the 1997 report of the NIH Director’s Panel on clinical research, entry: “clinical research”. http://grants.nih.gov/grants/peer/tree_glossary.pdf . Accessed 13 Nov 2009.
Schteingart DE. Introduction to principles of clinical and translational research. Athens: ESCI Core Course on Clinical Research; 2010.
Seely WE, Grinspoon S. Patient-oriented research: clinical pathophysiology and clinical therapeutics. In: Robertson D, Williams GH, editors. Clinical and translational science. 1st ed. London: Elsevier; 2009. p. 3–12.
Chapter Google Scholar
Califf RM, Zarin DA, Kramer JM, Sherman RE, Aberle LH, Tasneem A. Characteristics of clinical trials registered in ClinicalTrials.gov, 2007–2010. JAMA. 2012;307:1838–47.
Article CAS PubMed Google Scholar
Karp JE, McCaffrey RP. New avenues of translational research in leukemia and lymphoma: outgrowth of a Leukemia Society of America-National Cancer Institute workshop. J Natl Cancer Inst. 1994;86:1196–201.
Feldman AM, Koch WJ, Force TL. Developing strategies to link basic cardiovascular sciences with clinical drug development: another opportunity for translational sciences. Clin Pharmacol Ther. 2007;81:887–92.
Littman BH, Di Mario L, Plebani M, Marincola FM. What’s next in translational medicine? Clin Sci (Lond). 2007;112:217–27.
Article Google Scholar
Woolf SH. The meaning of translational research and why it matters. JAMA. 2008;290:211–3.
Dische S, Saunders M. Translational research–a new entity? Acta Oncol. 2001;40:995–9.
Coller BS. Translational research: forging a new cultural identity. Mt Sinai J Med. 2008;75:478–87.
Article PubMed Central PubMed Google Scholar
Coller BS. Translating from the rivers of Babylon to the coronary bloodstream. J Clin Invest. 2012;122:4293–9.
Article CAS PubMed Central PubMed Google Scholar
Williams DR. Introduction to clinical research. In: Robertson D, Williams GH, editors. Clinical and translational science. 1st ed. London: Elsevier; 2009. p. xvii–xx.
Williams GH, Robertson D. Clinical and translational science in infrastructure. In: Robertson D, Williams GH, editors. Clinical and translational science. 1st ed. London: Elsevier; 2009. p. 171–81.
Davis D, Evans M, Jadad A, Perrier L, Rath D, Ryan D, et al. The case for knowledge translation: shortening the journey from evidence to effect. BMJ. 2003;327(7405):33–5.
National Cancer Institute. Translational research working group definition of translational research. http://www.cancer.gov/trwg/TRWG-definition–ND-tr-continuum .
Sung NS, Crowley Jr WF, Genel M, Salber P, Sandy L, Sherwood LM, et al. Central challenges facing the national clinical research enterprise. JAMA. 2003;289:1278–87.
Lauer M, Scarlatos S. Translational research for cardiovascular diseases at the national heart lung and blood institute. Moving from bench to bedside and from bedside to community. Circulation. 2010;121:929–33.
Westfall JM, Mold J, Fagnan L. Practice-based research–“Blue Highways” on the NIH roadmap. JAMA. 2007;297:403–6.
Malliaras K, Kreke M, Marbán E. The stuttering progress of cell therapy for heart disease. Clin Pharmacol Ther. 2011;90:532–41.
Waldman SA, Terzic A. Clinical and translational science: from bench-bedside to global village. Clin Transl Sci. 2010;5:254–7.
Mc Garland Rubio D, Schoenbaum E, Lee S, Schteinggard DE, Marantz PR, Anderson KE, et al. Defining translational research: implications for training. Acad Med. 2010;85:470–5.
Koch WJ. Targeting the beta-adrenergic receptor kinase in heart failure. Lecture presented at the 1st international scientific conference on cardiovascular biotechnology: from cell to man. Biomedical Research Foundation of the Academy of Athens, 31 May 2013.
Kieburtz K, Olanow CW. Translational experimental therapeutics: the translation of laboratory-based discovery into disease-related therapy. Mt Sinai J Med. 2007;74:7–14.
Antoniades CH. Bridging the gap between basic science and clinical practice: the role of translational medicine. Lecture presented at the Biomedical Research Foundation Academy of Athens, 31 Oct 2012.
Murry CE, Jennings KA, Reimer KA. Preconditioning with ischemia: a delay of lethal cell injury in ischemic myocardium. Circulation. 1986;74:1124–36.
Chautard E, Thierry-Mieg N, Ricard-Blum S. Interaction networks: from protein functions to drug discovery. A review. Pathol Biol (Paris). 2009;57:324–33.
Article CAS Google Scholar
Kerner JF, Hall KL. Research dissemination and diffusion. Res Soc Work Practice 2009;19:519–30.
Zucker DR. What is needed to promote translational research and how do we get it? J Investig Med. 2009;57:468–70.
PubMed Google Scholar
Bernard GR. Preparedness of the CTSA’s structural and scientific assets to support the mission of the National Center for Advancing Translational Sciences (NCATS). Clin Transl Sci. 2012;5:121–9.
Hartmann KE, Heitman E, Brown NJ. Training basic, clinical and translational investigators. In: Robertson D, Williams GH, editors. Clinical and translational science. 1st ed. London: Elsevier; 2009. p. 191–9.
Williams GH, Robertson D. The future of clinical research. In: Robertson D, Williams GH, editors. Clinical and translational science. 1st ed. London: Elsevier; 2009. p. 565–70.
Nadler LM, Roberts WC. Lee Marshall Nadler, MD: a conversation with the editor. Proc Baylor Univ Med Cent. 2007;20:381–9.
Verkoeijen PPJL, Tabbers HK. Good research requires productive theories and guidelines. Med Educ. 2013;47:858–65.
Pang T, Terry RF, The PLoS Medicine Editors WHO/PLoS Collection. No health without research: a call for papers. PLoS Med. 2011;8:4 e1001008.
White KL, Williams TF, Greenberg BG. The ecology of medical care. N Engl J Med. 1961;265:885–92.
Green LA, Fryer Jr GE, Yawn BP, Lanier D, Dovey SM. The ecology of medical care revisited. N Engl J Med. 2001;344:2021–5.
Martin SS, Ou FS, Newby LK, Sutton V, Adams P, Felker GM, Wang TY. Patient- and trial-specific barriers to participation in cardiovascular randomized clinical trials. J Am Coll Cardiol. 2013;61:762–9.
Brutsaert DL. Heart failure: Quo Vadis. Lecture presented at cardiovascular biotechnology: from cell to man. Biomedical Research Foundation Academy of Athens, 31 May–1 June 2013.
Califf RM. Clinical trials. In: Robertson D, Williams GH, editors. Clinical and translational science. 1st ed. London: Elsevier; 2009. p. 13–37.
Welke KF, Ferguson Jr TB, Cooms LP, Dokholygan RS, Murray CJ, Sehrader MI, et al. Validity of the society of thoracic surgeons national adult cardiac surgery database. Ann Thorac Surg. 2004;77:1137–9.
Moss AJ, Francis CW, Rayan D. Collaborative clinical trials. N Engl J Med. 2011;364:789791.
Sipido KR, Casadei B, Holvoet P, Janssens S, Luttun A, Sampaolesi M. Bedside to bench: a look at experimental research with a clinical trial checklist. Cardiovasc Res. 2014;101:1–3.
Kaul S, Diamond GA. Trial and error. How to avoid commonly encountered limitations of published clinical trials. J Am Coll Cardiol. 2010;55:415–27.
Balas EA, Boren SA. In: Balas EA, editor. Yearbook of medical informatics: managing clinical knowledge for health care improvement. Stuttgart: Shattauer Verlagsgesellschaft mbH; 2000, p. 65–70.
Contopoulos-Ioannidis DG, Alexiou GA, Grouvias TC, Ioannidis JPA. Life circle of translational research for medical interventions. Science. 2008;321:1298–9.
Hudson J, Khazhragui HF. Into the valley of death; research to innovation. Drug Discov Today. 2012;18:610–3.
Butler D. Translational research; crossing the valley of death. Nature. 2008;453:840–2.
Roberts SF, Fischhoff MA, Sakowski SA, Feldman EL. Perspective: transforming science into medicine: how clinician-scientists can build bridges across research’s “valley of death”. Acad Med. 2012;87:266–70.
Coller BS, Califf RM. Traversing the valley of death: a guide to assessing prospects for translational success. Sci Transl Med. 2009;1:10cm9. doi: 10.1126/scitranslmed.3000265 .
Bodi V, Marrachelli VG, Husser O, Chorro FJ, Viña JR, Monleon D. Metabolomics in the diagnosis of acute myocardial ischemia. J Cardiovasc Trans Res. 2013;6:808–15.
Moses 3rd H, Dorsey ER, Matheson DH, Their SO. Financial anatomy of biomedical research. JAMA. 2005;294:1333–42.
Quinn T, Kohl P. Combining wet and dry research: experience with model development for cardiac mechano-electric structure-function studies. Cardiovasc Res. 2013;97:601–11.
The Royal Swedish Academy of Science. press release: pressmeddecande, 9 october 2013. Nobel prize in Chemistry. 2013.
Curry SH. Translational science: past, present, and future. Bio Tech. 2008;44:Pii–vii.
Braunwald E. Cardiovascular science: opportunities for translating research into improved care. J Clin Invest. 2013;123:6–10.
Stamler JS, Taber RL, Califf RM. Translation of academic discovery into societal benefit: proposal for a balanced approach–part 1. Am J Med. 2003;115:596–9.
Download references
Author information
Authors and affiliations.
Heart and Vessel Department, Biomedical Research Foundation, Academy of Athens, Athens, Greece
Dennis V. Cokkinos MD, FESC
You can also search for this author in PubMed Google Scholar
Corresponding author
Correspondence to Dennis V. Cokkinos MD, FESC .
Editor information
Editors and affiliations.
Academy of Athens, Biomedical Research Foundation, Athens, Greece
Dennis V. Cokkinos
Rights and permissions
Reprints and permissions
Copyright information
© 2015 Springer International Publishing Switzerland
About this chapter
Cokkinos, D.V. (2015). Introduction: What Is Translational Research. In: Cokkinos, D. (eds) Introduction to Translational Cardiovascular Research. Springer, Cham. https://doi.org/10.1007/978-3-319-08798-6_1
Download citation
DOI : https://doi.org/10.1007/978-3-319-08798-6_1
Published : 22 September 2014
Publisher Name : Springer, Cham
Print ISBN : 978-3-319-08797-9
Online ISBN : 978-3-319-08798-6
eBook Packages : Medicine Medicine (R0)
Share this chapter
Anyone you share the following link with will be able to read this content:
Sorry, a shareable link is not currently available for this article.
Provided by the Springer Nature SharedIt content-sharing initiative
- Publish with us
Policies and ethics
- Find a journal
- Track your research
- To save this word, you'll need to log in. Log In
translational research
Definition of translational research
called also translational medicine
Examples of translational research in a Sentence
These examples are programmatically compiled from various online sources to illustrate current usage of the word 'translational research.' Any opinions expressed in the examples do not represent those of Merriam-Webster or its editors. Send us feedback about these examples.
Word History
1986, in the meaning defined above
Dictionary Entries Near translational research
translation
translative
Cite this Entry
“Translational research.” Merriam-Webster.com Dictionary , Merriam-Webster, https://www.merriam-webster.com/dictionary/translational%20research. Accessed 12 May. 2024.
Medical Definition
Medical definition of translational research.
Subscribe to America's largest dictionary and get thousands more definitions and advanced search—ad free!
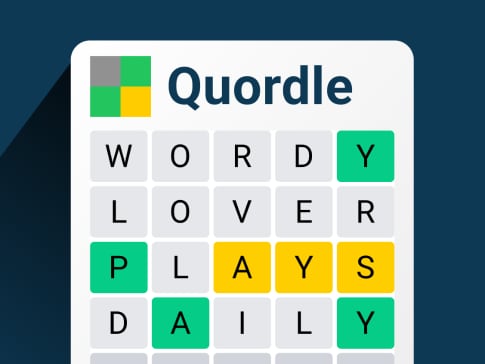
Can you solve 4 words at once?
Word of the day.
See Definitions and Examples »
Get Word of the Day daily email!
Popular in Grammar & Usage
More commonly misspelled words, your vs. you're: how to use them correctly, every letter is silent, sometimes: a-z list of examples, more commonly mispronounced words, how to use em dashes (—), en dashes (–) , and hyphens (-), popular in wordplay, the words of the week - may 10, a great big list of bread words, 10 scrabble words without any vowels, 8 uncommon words related to love, 9 superb owl words, games & quizzes.
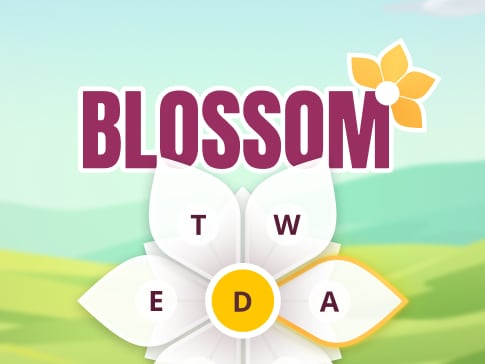

Research Types Explained: Basic, Clinical, Translational
“Research” is a broad stroke of a word, the verbal equivalent of painting a wall instead of a masterpiece. There are important distinctions among the three principal types of medical research — basic, clinical and translational.
Whereas basic research is looking at questions related to how nature works, translational research aims to take what’s learned in basic research and apply that in the development of solutions to medical problems. Clinical research, then, takes those solutions and studies them in clinical trials. Together, they form a continuous research loop that transforms ideas into action in the form of new treatments and tests, and advances cutting-edge developments from the lab bench to the patient’s bedside and back again.
Basic Research
When it comes to science, the “basic” in basic research describes something that’s an essential starting point. “If you think of it in terms of construction, you can’t put up a beautiful, elegant house without first putting in a foundation,” says David Frank, MD , Associate Professor of Medicine, Medical Oncology, at Dana-Farber Cancer Institute. “In science, if you don’t first understand the basic research, then you can’t move on to advanced applications.”
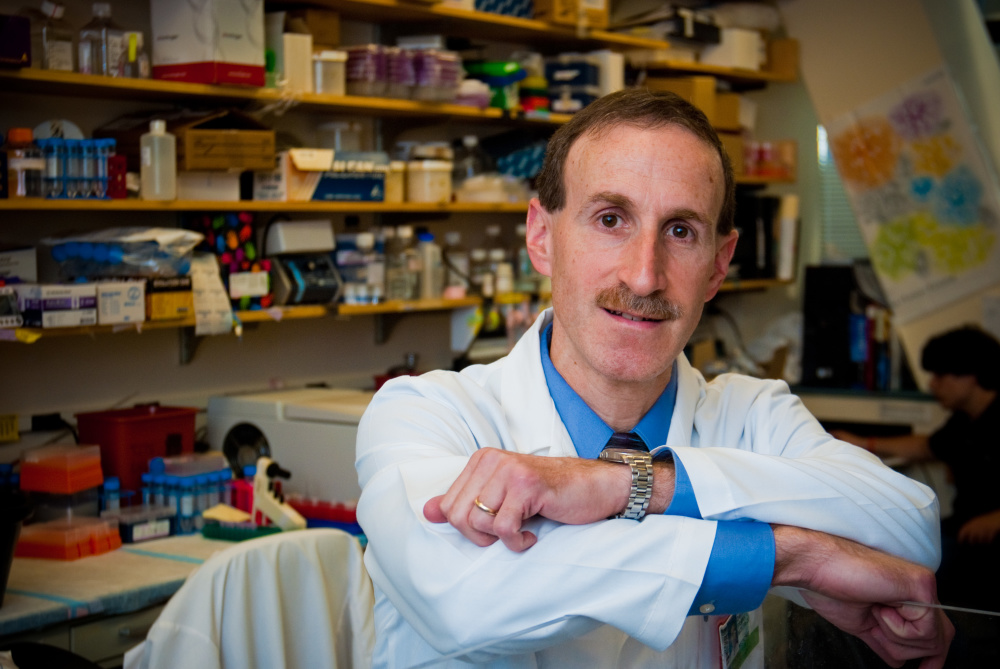
Basic medical research is usually conducted by scientists with a PhD in such fields as biology and chemistry, among many others. They study the core building blocks of life — DNA, cells, proteins, molecules, etc. — to answer fundamental questions about their structures and how they work.
For example, oncologists now know that mutations in DNA enable the unchecked growth of cells in cancer. A scientist conducting basic research might ask: How does DNA work in a healthy cell? How do mutations occur? Where along the DNA sequence do mutations happen? And why?
“Basic research is fundamentally curiosity-driven research,” says Milka Kostic, Program Director, Chemical Biology at Dana-Farber Cancer Institute. “Think of that moment when an apple fell on Isaac Newton’s head. He thought to himself, ‘Why did that happen?’ and then went on to try to find the answer. That’s basic research.”
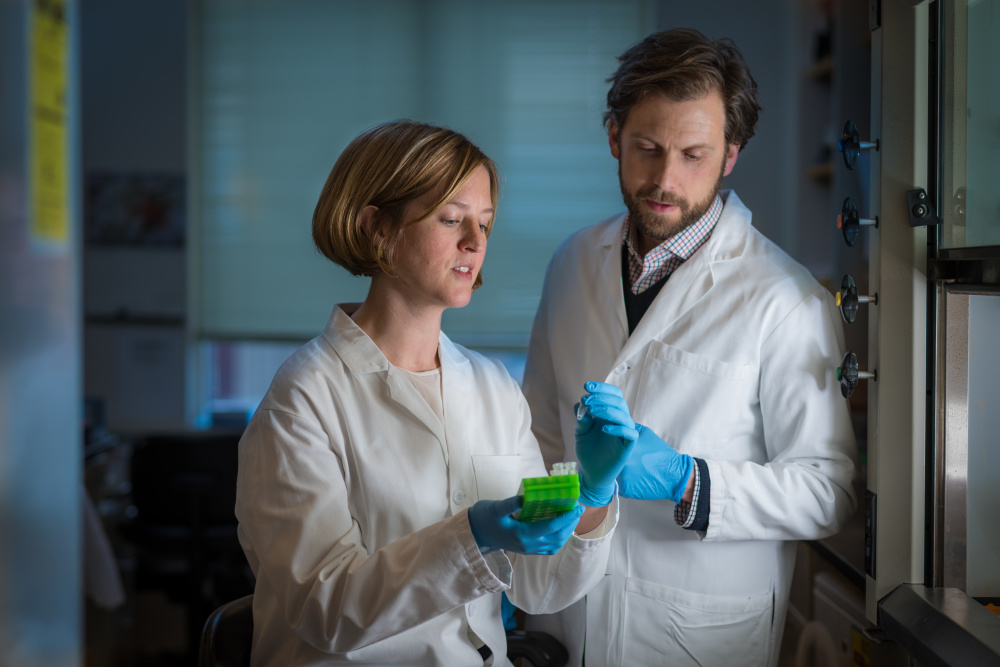
Clinical Research
Clinical research explores whether new treatments, medications and diagnostic techniques are safe and effective in patients. Physicians administer these to patients in rigorously controlled clinical trials, so that they can accurately and precisely monitor patients’ progress and evaluate the treatment’s efficacy, or measurable benefit.
“In clinical research, we’re trying to define the best treatment for a patient with a given condition,” Frank says. “We’re asking such questions as: Will this new treatment extend the life of a patient with a given type of cancer? Could this supportive medication diminish nausea, diarrhea or other side effects? Could this diagnostic test help physicians detect cancer earlier or distinguish between fast- and slow-growing cancers?”
Successful clinical researchers must draw on not only their medical training but also their knowledge of such areas as statistics, controls and regulatory compliance.
Translational Research
It’s neither practical nor safe to transition directly from studying individual cells to testing on patients. Translational research provides that crucial pivot point. It bridges the gap between basic and clinical research by bringing together a number of specialists to refine and advance the application of a discovery. “Biomedical science is so complex, and there’s so much knowledge available.” Frank says. “It’s through collaboration that advances are made.”
For example, let’s say a basic researcher has identified a gene that looks like a promising candidate for targeted therapy. Translational researchers would then evaluate thousands, if not millions, of potential compounds for the ideal combination that could be developed into a medicine to achieve the desired effect. They’d refine and test the compound on intermediate models, in laboratory and animal models. Then they would analyze those test results to determine proper dosage, side effects and other safety considerations before moving to first-in-human clinical trials. It’s the complex interplay of chemistry, biology, oncology, biostatistics, genomics, pharmacology and other specialties that makes such a translational study a success.
Collaboration and technology have been the twin drivers of recent quantum leaps in the quality and quantity of translational research. “Now, using modern molecular techniques,” Frank says, “we can learn so much from a tissue sample from a patient that we couldn’t before.”
Translational research provides a crucial pivot point after clinical trials as well. Investigators explore how the trial’s resulting treatment or guidelines can be implemented by physicians in their practice. And the clinical outcomes might also motivate basic researchers to reevaluate their original assumptions.
“Translational research is a two-way street,” Kostic says. “There is always conversation flowing in both directions. It’s a loop, a continuous cycle, with one research result inspiring another.”
Learn more about research at Dana-Farber .
Thank you for visiting nature.com. You are using a browser version with limited support for CSS. To obtain the best experience, we recommend you use a more up to date browser (or turn off compatibility mode in Internet Explorer). In the meantime, to ensure continued support, we are displaying the site without styles and JavaScript.
- View all journals
- My Account Login
- Explore content
- About the journal
- Publish with us
- Sign up for alerts
- Open access
- Published: 04 June 2020
Everyday characterizations of translational research: researchers’ own use of terminology and models in medical research and practice
- Dixi Louise Strand ORCID: orcid.org/0000-0003-0524-2466 1 , 2
Palgrave Communications volume 6 , Article number: 110 ( 2020 ) Cite this article
8271 Accesses
2 Citations
2 Altmetric
Metrics details
- Medical humanities
- Science, technology and society
Biomedical literature and policy are highly concerned with encouraging and improving the clinical application and clinical benefit of new scientific knowledge. Debates, theorizing, and policy initiatives aiming to close the “bench-to-bedside gap” have led to the development of “Translational Research” (TR), an emerging set of research-related discourses and practices within biomedicine. Studies in social science and the humanities have explored and challenged the assumptions underpinning specific TR models and policy initiatives, as well as the socio-material transformations involved. However, only few studies have explored TR as a productive ongoing process of meaning-making taking place as part of the everyday practices of the actual researchers located at the very nexus of science and clinic. This article therefore asks the question of how the discourse and promise of translation is embedded and performed within the practices and perspective of the specific actors involved. The findings are based on material from ethnographic fieldwork among translational researchers situated in a Danish hospital research setting. The analysis draws on the analytical notion of performativity in order to approach statements and models of TR in the light of their performative dimension. This analytical approach thus helps to highlight how the characterizations of TR also contain prescriptions for how the world must change for these characterizations to become true. The analysis provides insights into four different characterizations of TR and reflects on the associated practices where performative success is achieved in practice. With the presentation of these four characterizations, this paper illustrates different uses of the term TR among the actual actors engaged in research-clinic activities and contributes insight into the complex processes of conceptual and material reorganization that form part of the emergence of TR in biomedicine.
Similar content being viewed by others
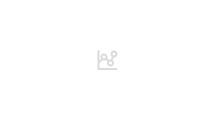
The translational lag narrative in policy discourse in the United States and the European Union: a comparative study
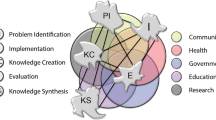
Wicked problems in a post-truth political economy: a dilemma for knowledge translation
The gains of reduction in translational processes: illness blogs and clinical-ethics cases, introduction.
Translational research has become subject to widespread debates in biomedical literature and politics, evoking high expectations, promises, and concerns. The term “translational research” was first used in a US national cancer program in the 90’s and has since appeared in research programs, research strategies, academic articles and journals, policy reports, and educational programs globally. The main interest underlying the concept in this normative policy oriented debate derives from a perceived series of gaps between life sciences, medical research, clinical practices, and effects in the form of, e.g., measurable health improvements. The rationale and promise of TR is to ensure and encourage that public investments in health science are turned into improved care practice and improved public health. The term TR is used interchangeably with other terms such as translational medicine, translational science, academic medicine, medical knowledge translation etc. TR is closely linked to research policy, funding incentives as well as organizational transformation in Europe, US, Australia, and more recently the Nordic countries, where Academic Health Science Centers (AHSCs) have been established in recent years to encourage translational interaction between research and clinic. As such, TR is important as a pervasive discourse in medical science and as a socio-economic reorganization of research practices. Existing studies have pointed to the multiple meanings of translational research in ongoing academic and policy debates—and to the way in which this concept is tied to a range of varying problems and possible solutions, not only in different medical fields but also in different national contexts (Crabu, 2018 ; Greenhalgh and Wieringa, 2011 ; Krüger et al., 2018 ; van der Laan and Boenink, 2015 ). Very few studies, however, focus empirically on how these expectations and characterizations of TR hold true in the context of actual researcher practices.
This article reports on an ethnographic case study of translational research networks in a Danish university hospital setting. The investigation focused empirically on the nature of these translational activities and on how translational research was “made to work” in a specific research-hospital setting. This article presents a particular sub-set of the data in order to explore how the translational researchers themselves understood and used the concept of translational research (TR). Focus is thus on how actors in a Danish research setting are entangled in wider discourses of TR and how they take part in performing TR discursively and materially. The analysis draws on key ideas from the work of Science and Technology Studies in order to understand TR as a set of performative statements and ideas that generate their own practices and thereby create the world they describe (Mackenzie et al., 2007 ; Mol, 2002 ). As such, the article contributes empirical insights into the researchers’ own descriptions and models regarding the concept, how the concept was performed in the setting studied, how researchers engaged with the concept, and what they made of it.
The article is structured as follows. First, I briefly present the literature on the concept of TR. The next section presents the methodological and theoretical backdrop for the research reported and the areas of investigation, translational research grounded in the fields of psychiatry and oncology. Hereafter, key statements and examples from the data are presented and analyzed. Lastly, the discussion reflects upon TR as a complex process of meaning production and material reorganization.
Translational research
In an important article from 2012, Van der Laan and Boenik “disentangle” the concept and rhetoric of TR and its different meanings, both historically and philosophically (van der Laan and Boenink, 2015 ). They focus on the extensive and exponential use of the concept in biomedical scientific literature during the years 1993–2010 and present different epistemic dimensions regarding the way the concept is interpreted and used. Krueger, Hendriks, and Gauch’s more recent literature survey of the term in biomedical and clinical research also finds a “kaleidoscope” of different dimensions, understandings, and applications related to the term (Krüger et al., 2018 ).
Despite the variances, one focal figure in this literature is the trope of “bench to bedside”. Here, TR is a science-clinic-public relationship conceptualized as a set of translational phases through which knowledge moves from basic biomedical research into diagnosis or treatment, subsequent development into evidence-based protocols, following deployment in clinical practice, and, ultimately, benefits for the individual and society through improvement of public health. The term implies a relocation and translation of knowledge across what are conceived of as somewhat separate domains. Yet, as noted in the existing literature studies, the way in which the specific gaps, models, and problematic barriers are constructed vary greatly. Likewise, the understanding of the very domains involved differs. Basic science, clinical research, clinical practice, the public, society, and politics are also defined and delineated in varying ways.
The social sciences and humanities have entered into and contributed to the biomedical debate on translational research, as recently reviewed by Crabu ( 2018 ). Work in these areas has challenged the transfer notion implicitly found in much of the literature on translational science—as well as the fundamental distinction between basic and applied science. Qualitative empirical studies also bring our attention to the very complex collaborations and recursive pathways of TR, where valuable breakthroughs in science and treatments can emerge by way of the clinical staff and their daily questions and puzzles, from patients or patient groups, commercial activity, or policy demands. The creation of new medical knowledge can thus have many “starting points” in addition to basic science, thus challenging the linearity implied in many discussions and policies on TR. In their study of laboratory and clinical practices related to Huntington Disease, Lewis, Hughes, and Atkinson, for example, point to TR as a complex of clusters and multiple processes of relocation and reconfiguration as objects, knowledge, practices, and resources are circulated between multiple sites (Lewis et al., 2014 ). Based on a study of health care innovation through extended translational networks in Canada, Lander and Atkinson-Grosjean ( 2011 ) likewise describe various hybrid domains of translational science that cut across presumed divides between basic science, clinic, as well as commercial and civic areas. Their study also illustrates how translational pathways flow through the interactions and relations among a complex collection of actors and organizations (Lander and Atkinson-Grosjean, 2011 ). This complexity is less visible in the normative depiction of TR as a unidimensional line from basic research to clinical practice and then to public health.
The study reported on in this paper converges with this line in the literature located in social science and the humanities, both openly exploring the complexities of TR and challenging the foundations and presuppositions of a normative TR agenda. The article focusses on the question of how actors involved in TR in a specific research-hospital setting engage with and use the concept. The exploration asks open questions as to which statements and models of TR circulate among these actors? What does TR mean in the context studied? How do these statements and models participate in shaping research and clinical practice? How does TR relate to other concepts and concerns? In exploring these questions, different understandings have emerged from the data, summarized here as four themes: TR as knowledge flow, TR as a political buzzword, TR as collaboration and exchange, and TR as competency and skills. Each of these understandings is depicted in turn in the analysis section. Based on this analysis, the paper contributes to existing social science and humanities explorations of TR and adds to existing work by illustrating the ways in which the concept of TR circulates in a particular setting and how the concept is adopted and used by actors in this setting as part of their everyday practice. The paper argues that these performative uses of the term are material and productive as they contribute to organizing work, as well as attaching value to specific kinds of work and specific skills.
The study reported here is based on ethnographic fieldwork in Danish hospitals carried out between January 2018 and March 2019. I conducted interviews and observations and collected a broad range of organizational and project documents. Observations included research team meetings, departmental meetings, public presentations of research, two academic conferences, patient testing and treatment, lab visits and informal conversations (~100 h). I took hand written notes during observation and subsequently wrote these out in text files—with concurrent memoing. Observations and informal conversations provided data on daily experiences and were linked to formal interviews that were conducted in parallel (n20). Interviews were conducted with various team members, primarily clinician-scientists (n11) but also research team members such as Ph.D. students (n4), biologists (n2), an engineer (n1), and lastly two department managers (n2). The interviews lasted 1–2 h, were recorded and transcribed with the respondents’ consent. All interviews were conducted at the hospitals and were semi-structured and included questions about the participants’ definitions, understandings, and models regarding the concept of TR. Data was stored, organized, and coded in the qualitative data analysis software NVivo using grounded theory and analytical tools from situational analysis (Clarke, 2005 ). The findings presented in this paper draw on a sub-set of the data regarding the way in which the translational researchers themselves understood and used the concept TR.
Ethical approval and consent were obtained in writing from the principle investigators of the research networks and from the informants. The project was also approved by the hospital management and reported to the regional ethics committee. Throughout the research project, I was simultaneously working as a consultant in a crosscutting research and innovation support unit at the hospitals. This involved weekly visits, meetings, workshops, and communication with staff and management at the hospital departments on issues related to research development and support in the region. This concurring consultancy work gave me a background understanding of the organization and the research infrastructure of the hospitals, but it is not included as a formalized part of the dataset due to research ethics of a dual role of employee and researcher.
Oncology and psychiatry
The setting for the research here is Region Zealand in Denmark and in particular two research networks based in the regional hospitals. These research networks connect different research projects or research protocols within a joint vision of changing and improving diagnosis and/or treatment within two very different medical areas, child and youth psychiatric diagnosis and cancer treatment. The two translational research networks were interdisciplinary, yet anchored in the two domains of oncology and psychiatry, referred to here as the electroporation and autism networks. The electroporation case was an international collaborative network working to develop and improve a new type of treatment for cancer, electroporation. This technique creates an electrostatic field in cancer cells in order to increase the permeability of the cell membrane, allowing chemicals, drugs or DNA to be introduced into the cell. When applied locally, this type of treatment has been found effective in killing the cancer cells of the tumor, and the treatment with this technique combined with administering calcium was found to release patterns into the immune system, possibly hindering a recurrence of the tumor and slowing further spreading of the cancer. A range of related projects sought to refine the technique in relation to specific cancer types and in relation to different types of chemicals, and to explore systemic immune responses of the treatment found clinically as an unexpected outcome of the treatment. This network played an important role in developing and implementing this particular type of cancer treatment internationally. The group was involved in developing European guidelines for clinical practices and in various political and practical implementation efforts to establish the treatment type as part of the standardized treatment program for specific cancer types in Denmark. The researchers were thus deeply engaged in research, but they were also focused on realizing its application in the clinic. Most of the researchers were also responsible for everyday clinical practices of examining patients and determining treatment strategies.
The second research network I studied conducted research on autism disorders in children and youth through a translational research design combining behavioral, psychological, and neurobiological approaches. The project was situated within a broader disciplinary debate regarding the Diagnostic and Statistical Manual (DSM) and concerning controversies as to the way in which to categorize symptoms of mental disorders. Autism in particular presents a contested diagnostic category, appearing clinically in a variety of forms and with varying professional understandings of its nature and appropriate treatments. The research network was concerned with this broader questioning of the very notion of autism as a singular disorder category and was critical of current diagnostic criteria and classification. Furthermore, the research network formed part of a shift in the clinic and the field more generally towards investigating mental disorders such as autism through laboratory practices and technologies like new IT-based cognitive function testing, electroencephalography (EEG), and magnetic resonance imaging (MRI). The researchers’ work was exploratory, seeking to find new ways of understanding autism as both symptoms and pathology, e.g., through a key psychiatric concept of cognitive flexibility that was investigated as part of the project.
Although several of the projects within both of these research networks included industrial partners, the lead researchers themselves stressed that their research was “investigator-initiated” and thus different in nature from clinical trials and medical research driven by industry. The lead researchers themselves framed their research, the research designs and approaches, as “translational”, e.g., in presentations and funding applications (“translational forskning” in Danish).
Analytical framework
Science Studies, Feminist Theory, and Cultural Studies have explored empirically and theoretically the way in which discourse, statements, and representations have productive consequences and effects upon reality (Foucault, 1990 ; Haraway, 1988 ; Latour, 1987 ; Mol, 2002 ). In their book on economics, Mackenzie et al. ( 2007 ) develop the term performativity to examine how economic theory takes part in shaping economic realities—how statements, models, concepts, and formulas over time shape the very worlds they describe. These authors illustrate how reality—socially and materially—over time becomes reshaped to fit with theoretical models and inherent presumptions. This analytical lens is highly relevant for understanding how the language of TR has been, and continually is, an agent for modifying the reality it describes. This analytical lens also leads us to study how actors involved in TR research take part in the actualization and “putting into motion” of TR through their use of TR concepts, models, and research designs. This is the focus in the following analysis where significant examples and excerpts related to the use of TR terminology are presented and discussed. According to Mol ( 2002 ), conversations and interviews are a way of listening to informants as if they were their own ethnographers, telling how their work of TR is understood and carried out. Interviews and conversations are thus analyzed as a way of learning about objects, events, and practices that are material and productive.
The analysis is organized according to four main understandings emerging from the data—TR as knowledge flow, TR as a political buzzword, TR as knowledge collaboration and exchange, and TR as competencies and skills. The quotes and excerpts are anonymized with regard to informant names and field of expertize and only attributed to the research network—named here as the autism and electroporation network, respectively. Throughout the analysis, the two networks also serve as analytical prisms for one another, juxtaposing their similarities and differences.
TR as knowledge flow from theory to practice
During the study, a dominant understanding of TR that appeared during observations, informal conversations, and interview questioning on the topic was TR as knowledge flow from theory to practice . This understanding aligns with the notion and modeling of TR that is pervasive in biomedical literature and the aforementioned bench to bedside trope. TR is conceived of as a set of transfers or flows of knowledge from basic biomedical research into clinical diagnosis and improved health for patients—and also concerns closing the gaps hindering this flow. This understanding was particularly prevalent in the electroporation network. A lead researcher involved in setting up laboratory studies, clinical trials, and implementation efforts in relation to the treatment technique electroporation explains her understanding of translational research to me in an interview:
It is about closing the gap between lab research and the patient’s everyday life. Moving knowledge from the laboratory over into clinical practice, as well as ensuring how we can take the biological tests that afterwards can go back to the researchers… moving from idea, to laboratory research, to clinical research and all the way into guidelines for new treatment. (Electroporation network)
This quote resonates with the dominant understanding of TR as knowledge that flows through a set of otherwise separate contexts and knowledge domains framed as laboratory, clinical practice (with associated guidelines), and the patient’s everyday life. She describes TR as the moving of laboratory research into clinical practice, and how TR also produces the biological data in the clinic to move back into the lab.
At a research event and presentation at the hospital, this researcher presents a timeline of her research on electroporation. She explains how she has been involved in the basic science in order to understand the cells and cell membranes and how they can be briefly destabilized by applying electrical pulses to the area. Based on these insights into cellular behavior, they developed new experimental electro-engineering tools for applying electricity directly to the skin and tumor and then for injecting drugs and chemicals into the area so these can enter into the tumor and cells creating a local and very effective new treatment form. These tools have subsequently been refined and developed and are part of standard treatment regimes for specific types of cancer, such as skin cancer. In addition, the technique and technology are being developed further for other types of cancers. For example, stomach and colon cancer, where the tumor is more difficult to access directly with electrodes. Here, the electrodes were undergoing further development in order for them to work with endoscopic devices.
In a timeline figure, a presentation slide sketches the steps of translation from basic science over into techniques tested on mice in order, for example, to refine how to administer the electricity and chemicals in an appropriate way and with the correct dosages. This led to further experimental treatments on patients, and later on, randomized trials with a larger number of patients proving both the safety and efficacy of this specific type of treatment. In 2013, the UK National Institute for Health and Care Excellence (NICE) guidelines state that sufficient evidence has been established for this particular treatment for cancer spreading in the skin. In conclusion, she notes that this process has been a relatively “rapid process of translation from the first discoveries to a treatment in widespread use.” She moves on to explain their more recent work on using calcium with this type of treatment to determine how this might have effects upon the immune system beyond the local effects of cancer cell death. The timeline and story of electroporation reiterates a temporal TR image of knowledge flow from theory to practice—and back again—over time.
When asked about definitions of the term translational, another researcher, part of the oncology research networks but involved in the study and treatment of other cancer types, similarly notes:
I think translational covers when we turn over basic science into practical science. I see it as research where we get out and onward from the lab. We have an aim that is out of the lab, and it is important for us to do something that potentially can get out and work in society. (Electroporation network)
This quote links up to an understanding of making science applicable and “something that can be used.” Other informants likewise recognized TR as the clinical application of theoretical or experimental knowledge—or “moving new knowledge closer to the clinic, so “it can be used and tested further.” One informant notes that TR in this way consists of a practical achievement that moves theory into clinical practice:
I define translational as the clinical application of theoretical or experimental knowledge. So translational science is that one translates, really a utilitarian term, that you translate something proven ex vivo or proven in a petri dish to something that has clinical consequences for patients.” (Electroporation network)
In the accounts recorded during the study, an understanding of TR as knowledge flow from theory to practice thus is in agreement with the dominant discourse in the literature and “promise of translation” envisioned in the future (Brown et al., 2000 ). The hope is that public investments in health science can and should be paid back in the form of improved care practice and improved public health. “Making a difference” was often mentioned as motivation for the career choice of working with translational hospital-based research, along with the value of participating in research that might be practically applicable to and an improvement of current clinical practice. This applicable-practice motivation was notable in both the electroporation and the autism network.
Following Mackenzie et al. ( 2007 ), the accounts and ideas also speak of actual practices where, in the electroporation network, knowledge artefacts, as well as biological material are relocated and exchanged. There are also accounts of how experimental research is connected to changing procedures for cancer treatment strategies and new standardized treatment programs.
TR as a political buzzword
Several of the informants in both research networks noted the buzzword character of the word, smiling or laughing at my question of their understanding in the interview thereby distancing themselves from the concept and instead locating the concept in a world of politics and funding bodies with interests different from their own. They reiterated statements resembling the objectives discussed above regarding knowledge flow and transfer, but then added that these ideas did not necessarily match the way TR projects actually played out according to their experience. A researcher from the autism network notes:
I know what it means, but that is not how I see it implemented. It is research that supposedly builds bridges between experimental research… over into clinical research, maybe back again. However, when I see translational programs implemented, it does not really get out to the patients, and it does not really get out into the clinic. (Autism network)
Several informants noted that the TR had a political buzzword quality and was about justifying funding for very specific types of research. Therefore, for some there was a discrepancy between the rhetoric of TR and the way it was realized in ongoing projects. Accounts such as the above imply a critical position—that the translational agenda is a way of living up to demands of funders or creating political enthusiasm for research—but that the actual move into clinic and patient benefits could be questioned.
One informant described TR as a specific kind of research funding to expand “the evidence base” rather than research enabling knowledge to flow between basic science and the clinic.
Really, a buzzword is about creating the funding to make the evidence. It is relatively inexpensive to fund the small, experimental studies but gets expensive when you want to test an intervention on a larger group. It is complicated to create the evidence to prove that this really should be part of the standard treatment plan. So it is a way of creating funding for this difficult phase, where promising research needs to create the evidence base for it to get used. (Electroporation network)
Here, TR is a way of creating political support for particular types of research funding—a way of filling an evidence gap that is necessary for something to be legitimate and justifiable as a diagnostic or treatment option in the clinic. Thus, in this understanding TR is understood as a rhetorical device serving particular interests and political agendas. This resonates with the political move to put significant resources into TR to increase the clinical relevance and application of research. Using research relies on the creation of very specific types of “robust” evidence such as randomized controlled trials—and these are a necessary intermediate stage for research findings to reach decision-makers and potentially have societal health impacts. So here, TR is about the political support for specific types of research, for creating particular research infrastructures that can enable credibility, validity, and paths of impacts for medical research.
Conversations and interviews where TR was referred to as a political buzzword can also be seen as part of the actors’ own reflections on a broader knowledge economy where colleagues, or they themselves, attempt to position themselves strategically in relation to political and funding agendas. They adhere to, draw on, but also smile at this—since what they see “play out” sometimes is a different scenario. The actors in both research networks involved were thus attentive to how one as a researcher strategically can link up to political agendas and adjust projects so they match the demands of policy and funding trends. Here, TR is pointed to as rhetorically powerful in justifying specific types of research resonating related analyses of how TR currently is mobilized in other national academic medical settings (Rushforth, 2016 ; Wilson-Kovacs and Hauskeller, 2012 ).
TR as interdisciplinary collaboration and exchange
A third understanding of TR that appeared in both networks was TR as a framing of interdisciplinary collaboration. Models and research designs of TR enabled and encouraged collaborations and exchanges across different medical specializations and across distinct departments and organizations. A researcher from the autism research network replies to the question of how she understands the term translational as follows:
I understand it broadly, that you try to connect knowledge and understanding from different levels—psychological, biological, social etc.—into a joint understanding of a phenomenon… The same phenomenon might really be the result of many different processes. (Autism network)
She moves on to explain how such processes can be captured at different levels and through different investigational methodologies deriving from different disciplines. Methods in her project included preclinical and clinical testing, neurobiological approaches, brain imaging, and at a later stage, if the funding becomes available, possibly also genetic testing. She explains that a translational approach is the next step in developing new knowledge on autism. Here, the promise of TR is to connect different knowledge forms to create radically new understandings.
The idea here is that the translational can dismantle diagnoses, as they are today. If we understand the translational levels, we will understand that the diagnostic system and the psychiatric system should be put together differently. (Autism network)
The research design of the autism project is therefore organized around a translational model that the lead researcher sketches for me on the whiteboard in her office, and later, after the interview, she sends me the model figure used in applications and when presenting the research (Fig. 1 ). Here, different translational levels are depicted along with the examinations, methods, and procedures applied to produce knowledge about these levels. The research project is designed around these translational levels through which the children included in the study move during a series of examinations marked in the figure as “assessments 1–5” under the column with procedures, ranging from standardized clinical screening tests and questionnaires, IT-based cognitive tests and paraclinical methods, including electroencephalography (EEG) and magnetic resonance imaging (MRI). These methods provide knowledge on different translational levels from the “psychosocial down to something increasingly biologically based”, she explains.
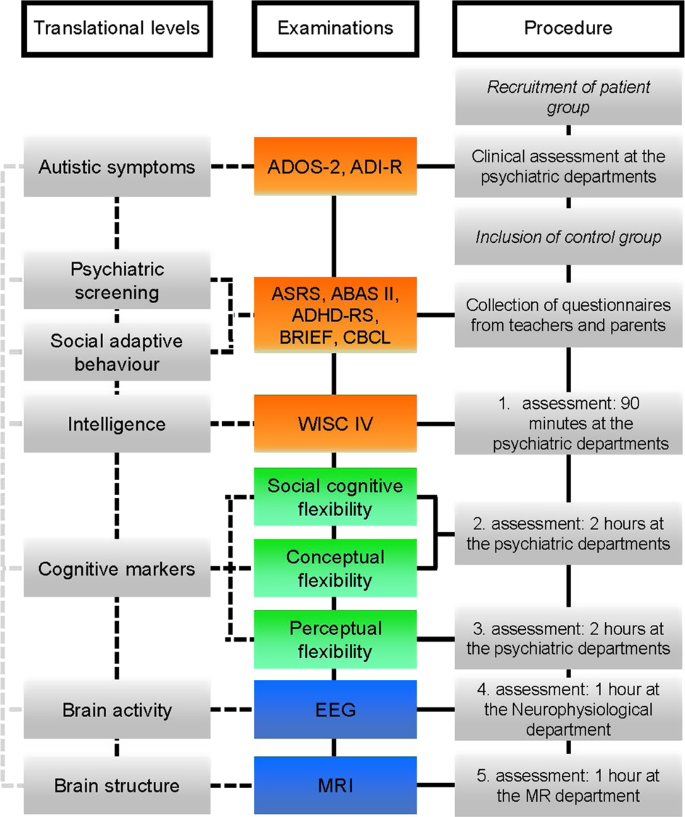
The translational model was shared by an informant in the autism research network and derives from their project description. The model depicts different translational levels along with the examinations, methods, and procedures applied to produce knowledge about these levels. The research project is designed around these translational levels and a series of examinations marked in the figure as “assessments 1–5” under the column with procedures, ranging from standardized clinical screening tests and questionnaires, IT-based cognitive testing, electroencephalography (EEG) to magnetic resonance imaging (MRI).
The model organizes the research project, the series of tests and examinations the patients and control subjects go through in which different kinds of technologies and expertize are involved. The tests take place in the childrens’ homes and in their school settings (using standardized questionnaires filled out by parents and teachers), in the psychiatric clinic (again using standardized screening and new cognitive tests developed as part of the project), in a neurobiology lab at the neurology department and at the department of medical imaging (where the MRI scanning technologies are located). The studies require personnel and expertize from these various disciplines in order to carry out the examinations and analyze the results. The autism research network thus spanned different departments and disciplinary specializations of child and youth psychiatry, psychology, psychophysiology, radiology, neurology, engineering, and screening software/IT development.
“It is like we take a cross-section and look at the same phenomenon at different levels”. (Autism network)
The translational research design brings together different methods and techniques for investigating many different parameters associated with autism. In this sense, the model of the translational research design can be seen as a workable boundary object (Star and Griesemer, 1989 ) enabling collaboration across a complex of scientific inquiry methods and knowledge forms into a joint workable research design. The model helps to make possible collaboration across specializations and departments and facilitates a joint study that combines approaches where autism is framed in different ways: as related to social, behavioral, and clinical symptoms, as a disease with a possible neurological basis, and as a disease with a possible brain structural basis. Through the translational research model, it becomes possible to relocate the phenomenon of autism into different disciplines and as such offers a tool towards collaboration. The language of TR in the autism network can thus be seen as a contact language enabling a joint interdisciplinary project to be planned and agreed upon (Galison, 1999 ).
In the same way, projects within the electroporation network were framed and depicted in translational models and research designs. This network also involved collaboration between a range of disciplines and areas of expertize, requiring collaboration between researchers based in oncology, surgery, dermatology, pathology, biochemistry, molecular biology, immunology, physics, engineering, IT, and palliation. A model (Fig. 2 ) was used in a research presentation to depict and present a subproject taking place within this translational network. The project was set up as a collaboration between the oncology and surgery department at the Danish hospital and included several other partners such as universities, industry, and e.g., a leading immunological research institute in France working to develop new methods to characterize the immune characteristics of cancer tumors, “the cellular landscape of the tumor”. Like the model from the autism network, it looks “across” and combines different types of examination and methodologies in a new way.
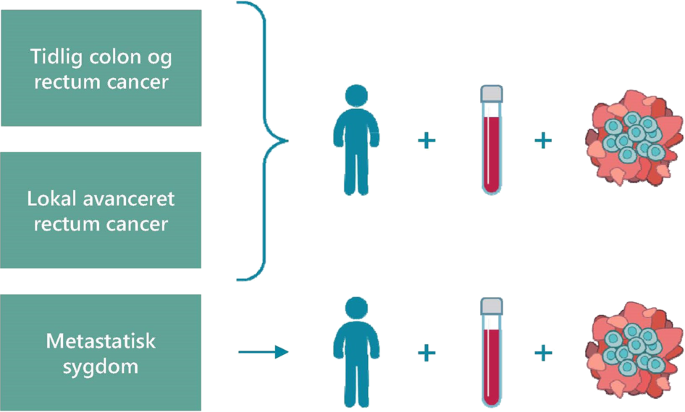
The translational model was shared by an informant in the electropration research network and derives from their project description. The model depicts the translational set-up for the study and different methods for examination that include and “sum up” patient outcomes, blood samples, and tumor biopsies across different stages of cancer—early cancer (Tidlig colon og rectum cancer), advanced cancer (Lokal avanceret rectum cancer), and cancer that has spread in the body (Metastatisk sygdom).
One of the lead researchers explains the translational set-up for the study in which the patient is treated with calcium electroporation before the planned surgical removal of a cancer tumor. The treatment prior to surgery aims to stop the tumor growth and hinder spreading of the cancer. Blood samples and tumor biopsies are taken, and biological data is recorded before and after the surgery to assess the effect of the intervention. In the boxes on the left, the figure presents the different stages of cancer development to be examined—early cancer, advanced cancer, and cancer that has spread in the body (metastasized). The investigations then focus on three ways of “reading” the intervention. Firstly, what happens to the patients? Is it safe? How is the treatment experienced by the patient, e.g., pain? Are the side effects short term and long term? Does the tumor grow or spread (as seen and measured through imaging technologies)? Secondly, what happens as the result of this treatment at the molecular level in the tumor and in the blood, examined through tumor biopsy investigations and blood profiling testing before and after the treatment intervention? These different “readings” of the interventions’ effects are summed up (+). The researcher explains that this can be a new way of producing knowledge about the variation they find clinically, for example of how the same intervention works differently in two patients, by looking at the cellular level and the biological markers to explain and understand the variation and the immunological changes involved. Translation is a way of making the effects of an intervention visible and documentable, as a way of proving a link between treatment and specific effects. As such “translational” is a set of methods and tools that can be used to compare and evaluate treatments with the potentiality of providing a new and different kind of knowledge of what works, and sometimes how it works.
In the electroporation network, several researchers noted that the research is fragmented and separate (e.g., in relation to the different stages of cancers or in relation to different treatments before or after surgery). A researcher explains that not a lot of research focuses on all three phases, but that looking at the immune system as a whole rather than the tumor as an isolated entity to be treated or removed is often neglected. Looking at the effects of the treatment overall requires what he notes as a “helicopter view”. Another researcher in the project similarly refers to this work as grasping the “bigger picture”.
It is about designing the study so you see the bigger picture and get a 360-degree view… If you want to make a difference and do research that moves the way we think, then you have to include all the parts and include the whole spectrum. (Electroporation network)
Ideally, for example, results from patient-reported outcomes, molecular biological examinations of blood samples and immunological investigations of tumor material are linked up in the research project—as are different stages of cancer and phases of cancer treatment like pre, during, and post operation, thereby encompassing the “whole spectrum” by working across disciplines and joining differing techniques and niches of research. For both networks, bringing together these different investigational techniques and methods was where the research had the break-through potential to be a “game changer”, as one researcher puts it—in the sense of a new way of thinking about cancer or an entirely new approach to psychiatric diagnosis. This is where there is a promise and potential to change the foundations for existing classifications, diagnostics, and treatment strategies of illnesses. In both networks, TR is a productive and adaptable way of framing interdisciplinary research and multifaceted research problems. TR holds a promise of not only creating usefulness of research, but also of changing fundamental paradigms of both clinical research and practice.
TR as competencies and skills
A final and fourth understanding reappearing in the data material is TR as competencies and skills. When explaining their TR understandings and research activities it was often noted by the informants that such work required a set of specific competencies and skills. This concerned the ability to develop and use translational tools and methodologies in the research project and at the hospital. In the excerpt below, a lead researcher stresses the aspect of “ability to use”:
“To me, translation is the ability to have a tool to translate an effect of something, a clinical intervention, a clinical problem—to be able to translate that into an effect on the genetic, cellular, or molecular level. So translation to me is using that methodology, that method, to find an effect of something that happens clinically.” (Electroporation network)
As part of the electroporation study, Ph.D. students and young researchers went abroad and participated in research courses, seminars and visits—one in an electro-engineering institute in Slovenia to learn the electrophysics behind the technique, the other in an immunology laboratory in France to learn how to measure immune cells in the tumor with a novel prognostic technique. These kinds of exchanges were vital to developing the skills necessary to realize the TR objectives of the project. One of the Ph.D. students explains this as somewhat different from other kinds of clinical research at the department.
When you are a researcher at a hospital, you stand there with your patient, and then you send off your tests, I have heard jokes about this in the lab, you send off your tests into a black box, and we get a bunch of numbers back. I would like to go into that black box and see what happens, to understand how the tests are practically handled in the lab, because I think that the very numbers I get out of that black box, well I will to a greater degree understand them, if I have been there in the lab, where I see it and have it in my hands. (Electroporation network)
In this quote, the physician in research training points to her own movement between research located in a clinic and in a laboratory. Part of her research plan is to move into the laboratory and acquire the skills to understand laboratory-based analysis, results and possibilities. In order to gain these skills and competencies, and apply them to the clinical focus of her research, she notes how it is necessary to physically “move into the black box” and “have it in my hands”. As such, her training involves a shift from more “traditional” medical research into a new kind of translational research.
Another team member in the project has a background in human biology. She has been hired to support the translational research at the department and highlights that it is the exchanges between the different professional groups in the project that are so necessary and where we “really make the most of the knowledge we have”. She explains her mediating work and role and how her educational background in human biology has equipped her to be a clinical research biologist.
It is really about understanding a little bit of everybody else’s areas or field, so you can be precisely that link between chemists, physicists, and doctors. (Electroporation network)
In the electroporation network, a TR research agenda was thus closely linked with recruiting or educating the “TR agents” that could facilitate research activities and exchanges across disciplinary boundaries. A crucial supporting aim of the TR networks was thus to develop these skills in house—at the department and at the hospital, rendering the opportunities in the technologies and the lab more accessible.
Likewise, Ph.D. students in the autism network were trained thoroughly in the working of the neuro-lab by experts at the hospital and from abroad, setting up and using the physical equipment for carrying out EEG examinations, as well as the technologies and software involved in data analysis of the EEG results. As noted by one of the Ph.D. students, this was really a very different set of “much more technical skills” than those he was trained in as medical doctor in child and youth psychiatry. A key participant in the autism network was for example a trained psychologist and had experience from a previous job with MRI brain scanning techniques. His expertize in both child and youth psychiatry and imaging technologies made it possible to connect the psychiatric research interests with possibilities in the MRI devices for testing and analysis. He could speak the necessary highly specialized language related to diagnostic imaging, such as multi-slicing, pulse-sequences, and fiber tracking etc. He enabled the interactions and exchanges among the psychiatrists and the MRI engineer and imaging professor. Such ability to apply new methodologies across disciplines and to converse and move expertly across more than one discipline is thus a fourth way in which the discourse and promise of translation was embedded within the practices of both research networks.
This article has presented and explored different uses of the TR terminology in two specific settings of biomedical clinic-academic work practices. This type of inquiry is underexplored in the literature, and this empirical contribution thus adds to related efforts into studying how the actors in the field respond to political agendas of TR and changes in the biomedical research-clinic landscapes (Rushforth, 2016 ; Vignola-Gagne, 2014 ; Wilson-Kovacs and Hauskeller, 2012 ). The analysis has put forth a set of performative statements on TR along with the models of translation presented by these actors. Here we see how TR is embedded in the practices and perspectives of a set of particular actors involved in TR.
The first characterization of TR as knowledge flow can be viewed as an adaptation of the normative language of TR debates and policies. The goal and value of bench to bedside work enters into the actors’ own characterizations and meaning-making of their everyday work—as it also productively shapes and organizes these practices. Researchers involved in TR take on and seek to fulfill expectations and visions of the TR discourse and assumptions that circulate among funders, evaluators, management, and in health care prioritization and politics. TR also becomes part of the actors’ own sense-making and vision of how value can be created through their work for patients and for society. This brings our attention to how actors involved in carrying out TR take part in actualizing and putting into motion theories, models, and the very propositions of TR. This characterization places positive value upon specific kinds of work in the hospitals and among the hospital-based researchers. Translational research work that can ensure that research results are used, integrated into practice, and can produce benefits for patients and society is thus also characterized and performed as desirable and good.
The second characterization points to the critical position of TR as associated with a political agenda and something that can be used strategically to secure funding. TR offers an opportunity for researchers to position themselves advantageously in relation to TR policy and funding—thus potentially gaining a privileged professional status as key leaders of change, as noted in related studies (Vignola-Gagne, 2014 ; Wainwright and Williams, 2009 ; Wilson-Kovacs and Hauskeller, 2012 ). The researchers themselves take part in critically reflecting upon political agendas, funding flows and the consequences for their work, their career, and their field of expertize. They thus participate in questioning the very promises and hopes of the TR dominant discourse and the transformations they experience.
In the third characterization presented here, TR as collaboration and exchange, TR becomes a way of seeing and analyzing the subject matter across disciplinary divides. As such, the translational research design combines several different investigational methodologies from different and otherwise somewhat distinct disciplines. In the terms of Latour, these techniques mediate the object studied in different ways, rendering it visible for science in particular ways (Latour, 1987 ), and these different mediations are brought together in the promise of “seeing the bigger picture”—and “changing the game” in radical ways. TR discourse and promises become an organizing factor for research and research activities in the hospital studied. TR serves to facilitate epistemological boundary spanning (Evans and Scarbrough, 2014 ) and is a productive way of framing interdisciplinary research and multifaceted research problems that cannot be solved with traditional research frameworks. In this understanding TR perhaps opens for alternative ways of creating medical knowledge and evidence other than—or in combination with—the gold standard of randomized controlled trials (Timmermans and Berg, 2003 ; Wieringa et al., 2017 ).
Lastly, TR is closely linked to the building and expanding of interdisciplinary and transactional skills in the hospital setting studied. This is discussed under the fourth characterization, TR as competencies and skills. This theme highlights the integration of new knowledge forms into the hospital research setting, as well as the very practical, material, and embodied abilities of TR such as handling the equipment, delivering the electric pulses to the tumor areas as in electroporation, and learning to administer the details of the EEG equipment and devices. New skills must be learned and entered into clinical practice and experimentation. Likewise, medically trained employees move into the lab and learn to work with the biopsies, blood samples, and cells “in their hands”. Scientific investigation of cancers and autism is shifted out of the clinic into the laboratories of for example neurobiology and brain imaging—and the techniques and skills from these disciplines are relocated into clinical and medical practice and achieve new value here.
With the presentation of these four understandings, this article illustrates different uses of the term TR among actors engaged in research-clinic activities in two settings, that of clinical oncology and that of clinical psychiatry. The analysis presented in this article does, of course, not cover all the ways in which actors involved in TR use the term. Rather it illustrates some specific, situated uses—uses that reappeared and were focal in the data material produced in this study. The analysis provides new insights into TR as a”force of example” (Flyvbjerg, 2006 ). Rather than providing a total overview or mapping generalized patterns, the analysis explores specific context-dependent appearances of TR. The study brings out differences and connections for further juxtaposition to other studies in different specializations in different national contexts. It is important to note that the characterizations were not mutually exclusive, but overlapping and entangled in the setting studied. The understandings were mobilized, in turn, to bring out different aspects and values of the hospital-based research work in specific situations. All characterizations circulated in both networks—thus seemingly co-existing within these networks, as well as sometimes in the course of a single interview.
Notable are however also some of the differences in the two research networks. The networks were not analyzed as comparative cases (several cases of the same), but selected due to differences and analyzed in juxtaposition to bring out differences. In the electroporation network, the dominant understanding of TR as knowledge flow was more prevalent than in the autism network. In the electroporation network TR as knowlegde flow appeared as the primary understanding of the term and also as an important guiding rationale of conducting hospital-based translational research. This might be linked to the ways in which the field of cancer research historically has been tied to the political and funding agenda of TR. Historical studies have analyzed how the rise of translational research and a translational agenda, particularly in the US, is closely linked to cancer research and to the promise of cancer cures based on research into new drugs and treatment therapies (Fujimura, 1996 ; Keating and Cambrosio, 2012 ; Löwy, 1996 ). All early publications 1992–1997 using the term are also related to cancer research, in particular research on biomarkers in relation to cancer prevention and the establishment of tissue banks and cancer research centers in this period (van der Laan and Boenink, 2015 ). Historically, TR in this version seems more closely linked to the electroporation network than to that of psychiatry—where the understanding of TR as collaboration and exchange was foregrounded more often. These differences bring our attention to the ways in which TR is situated differently in different disciplines and underscores the contextual nature of the concept.
This article has presented selected findings from an ethnographic study of the everyday practices of TR in a specific setting. The discourse of TR, including the policy initiatives and organizational transformations linked to the TR discourse, can be seen as a paradigmatic shift in medical science. Yet little is known about how such a paradigm shift plays out in concrete settings, what it means to the actors involved, how it changes what constitutes meaningful and valuable research—as well as meaningful and valuable everyday work practices. This article proposes that actors take part in performing the emergence of TR and possible paradigm shifts by foregrounding and valuing specific versions of TR along with specific practices, specific skills. This entails that other practices and skills perhaps are backgrounded and become less visible and less valued. That which the less visible and less-valued practices are composed of (e.g., perspectives of patients or other working groups at the hospitals) constitutes a pressing question for further work beyond the scope of this paper. One of the important points highlighted here is the different ways in which TR discourses, promises, and expectations form an active part of the researchers’ sense-making and practices. The analysis presented in this article also allows us to reflect on how these actors take part in fulfilling a societal obligation, encouraging and improving the clinical application and clinical benefit of new scientific knowledge. They share the concerns found in the TR debates and policy regarding the closing of a “bench-to-bedside gap”, but also rework these orientations in relation to their specific projects and practices. Applying the notion of performativity, statements and models of TR have been approached in the light of their agency and their performative dimension. This analytical approach thus helps to understand how TR characterizations—and statements—also contain a prescription for how the world must change for them to become true. Mackenzie et.al. suggest that performative success is when there is created both a new language and theory, as well as new reality ( 2007 ). This article provides insights into four co-existing characterizations where such performative success was achieved in the setting studied. The analysis also points to a disciplinary difference through the juxtaposition of two different research networks. New languages, theories and realities were successfully in the making in these networks along with changing implications for the way in which research knowledge is produced and applied, as well as cultural shifts in what constitutes good and valuable research. In conclusion, this performative lens is proposed as a potential step forward toward developing a social science and humanities understanding of TR, how usefulness of research is characterized and realized through practice while keeping in sight the complexity and materiality of such processes.
Data availability
The dataset generated and analyzed during the current study is not publicly available due to the sensitive nature of the content and the use/consent agreed with informants. Selected anonymized extracts and summaries are available from the corresponding author on reasonable request and signing of a MOU to ensure the ethical use of data.
Brown N, Rappert B, Webster A (2000) Contested futures: a sociology of prospective techno-science. Ashgate, Farnham
Google Scholar
Clarke AE (2005) Situational analysis: grounded theory after the postmodern turn. Sage Publications, California
Book Google Scholar
Crabu S (2018) Rethinking biomedicine in the age of translational research: organisational, professional, and epistemic encounters. Sociology Compass. https://doi.org/10.1111/soc4.12623
Article Google Scholar
Evans S, Scarbrough, H (2014) Supporting knowledge translation through collaborative translational research initiatives: “bridging” versus “blurring” boundary-spanning approaches in the UK CLAHRC initiative. Soc Sci Med. https://doi.org/10.1016/j.socscimed.2014.01.025
Flyvbjerg B (2006) Five misunderstandings about case-study research. Qual Inq. https://doi.org/10.1177/1077800405284363
Foucault M (1990) The history of sexuality. Volume 1, An introduction. Vintage, New York
Fujimura JH (1996) Crafting science. a sociohistory of the quest for the genetics of cancer. Harvard University Press, Cambridge
Galison P (1999) Trading Zone-coordinating action and belief. In: Biagolo M (ed) The Science Studies Reader. Abingdon-on-Thames, Routledge, pp. 137–160
Greenhalgh T, Wieringa S (2011) Is it time to drop the ‘knowledge translation’ metaphor? A critical literature review. J R Soc Med. https://doi.org/10.1258/jrsm.2011.110285
Haraway D (1988) Situated knowledges: the science question in feminism and the privilege of partial perspective. Fem Stud. https://doi.org/10.2307/3178066
Keating P, Cambrosio A (2012) Cancer on trial: oncology as a new style of practice. University of Chicago Press, Chicago
Krüger AK, Hendriks B, Gauch S (2018) The multiple meanings of translational research. negotiating medical science. https://doi.org/10.31235/OSF.IO/W6XJN
Lander B, Atkinson-Grosjean, J (2011) Translational science and the hidden research system in universities and academic hospitals: a case study. Soc Sci Med. https://doi.org/10.1016/j.socscimed.2010.11.019
Latour B (1987) Science in action: how to follow scientists and engineers through society. Harvard University Press, Cambridge
Lewis J, Hughes J, Atkinson P (2014) Relocation, realignment and standardisation: circuits of translation in Huntington’s disease. Soc Theor Health. https://doi.org/10.1057/sth.2014.13
Löwy I (1996) Between bench and bedside: science, healing, and interleukin-2 in a cancer ward. Harvard University Press, Cambridge
Mackenzie D, Muniesa F, Siu L (2007) Do economists make markets. Do economists make markets? On the Performativity of Economics. Princeton University Press, New Jersey. https://doi.org/10.1086/597458
Mol A (2002) The body multiple: ontology in medical practice. Duke University Press, Durham
Rushforth A (2016) What’s in a slogan? Translational science and the rhetorical work of cancer researchers in a UK university. Nord J Sci Technol Stud. https://doi.org/10.5324/njsts.v4i1.2170
Star SL, Griesemer JR (1989) Institutional ecology, ‘translations’ and boundary objects: amateurs and professionals in Berkeley’s Museum of Vertebrate Zoology, 1907–39. Soc Stud Sci. https://doi.org/10.1177/030631289019003001
Timmermans S, Berg M (2003) The gold standard: the challenge of evidence-based medicine and standardization in health care. Temple University Press, Philadephia
van der Laan AL, Boenink M (2015). Beyond bench and bedside: disentangling the concept of translational research. Health Care Analysis: HCA: J Health Philos Policy. https://doi.org/10.1007/s10728-012-0236-x
Vignola-Gagne E (2014) Argumentative practices in science, technology and innovation policy: the case of clinician-scientists and translational research. Sci Pub Policy. https://doi.org/10.1093/scipol/sct039
Wainwright S, Williams C (2009) Stem cells, translational research and the sociology of science. In: Atkinson P, Glasner P, Lock M. Handbook of genetics and society: mapping the new genomic era. Routledge, Abingdon-on-Thames, pp. 41–58
Wieringa S, Engebretsen E, Heggen K, Greenhalgh T (2017) Has evidence-based medicine ever been modern? A Latour-inspired understanding of a changing EBM. J Eval Clin Practice. https://doi.org/10.1111/jep.12752
Wilson-Kovacs DM, Hauskeller C (2012) The clinician-scientist: professional dynamics in clinical stem cell research. Sociol Health Illn. https://doi.org/10.1111/j.1467-9566.2011.01389.x
Download references
Acknowledgements
This study was funded by Data and Development Support, Region Zealand, and hosted by Department of People and Technology, Roskilde University. The study was carried out with support and supervision from Jesper Grarup, Peter Kjær, and the two research groups Health Promotion Research and Dialogical Communication at Roskilde University. Special thanks are also extended to the participants in the study.
Author information
Authors and affiliations.
Data and Development Support, Region Zealand, Soroe, Denmark
Dixi Louise Strand
Department of People and Technology, Roskilde University, Roskilde, Denmark
You can also search for this author in PubMed Google Scholar
Corresponding author
Correspondence to Dixi Louise Strand .
Ethics declarations
Competing interests.
The author declares no competing interests.
Additional information
Publisher’s note Springer Nature remains neutral with regard to jurisdictional claims in published maps and institutional affiliations.
Rights and permissions
Open Access This article is licensed under a Creative Commons Attribution 4.0 International License, which permits use, sharing, adaptation, distribution and reproduction in any medium or format, as long as you give appropriate credit to the original author(s) and the source, provide a link to the Creative Commons license, and indicate if changes were made. The images or other third party material in this article are included in the article’s Creative Commons license, unless indicated otherwise in a credit line to the material. If material is not included in the article’s Creative Commons license and your intended use is not permitted by statutory regulation or exceeds the permitted use, you will need to obtain permission directly from the copyright holder. To view a copy of this license, visit http://creativecommons.org/licenses/by/4.0/ .
Reprints and permissions
About this article
Cite this article.
Strand, D.L. Everyday characterizations of translational research: researchers’ own use of terminology and models in medical research and practice. Palgrave Commun 6 , 110 (2020). https://doi.org/10.1057/s41599-020-0489-1
Download citation
Received : 04 December 2019
Accepted : 22 April 2020
Published : 04 June 2020
DOI : https://doi.org/10.1057/s41599-020-0489-1
Share this article
Anyone you share the following link with will be able to read this content:
Sorry, a shareable link is not currently available for this article.
Provided by the Springer Nature SharedIt content-sharing initiative
Quick links
- Explore articles by subject
- Guide to authors
- Editorial policies


What Is Translational Research?
- National Institutes of Health (NIH)
- Translational Research
C all it what you will – a “golden spike,” a “missing link,” a “crossroads” – translational research is a critically important trend at NIH.
Translational research activities are intended to bring knowledge from the lab into practice, and ideally, allow practice to influence what occurs in the laboratory. It is defined by NIH as “the process of applying ideas, insights, and discoveries generated through basic scientific inquiry to the treatment or prevention of human disease.” Several NIH institutes are stimulating new connections between basic and clinical behavioral research through such mechanisms as: Requests for Application, providing greater access to clinical populations and collaborators, workshops connecting basic researchers with public health and clinical investigators, and new peer review procedures that draw on experts from both clinical and basic perspectives.
These were the kind of results Congress was looking for when it chose to double the NIH budget: the fruits of research being used to treat patients with complex disorders in an effective and efficient manner. APS has paid close attention to this trend, not only by citing it in previous Observer articles, but through other means as well. APS co-hosted a Capitol Hill briefing dedicated to the subject ( Observer , November 2002), and was also very supportive of translational research in testimony before Congress last spring. In the Senate report that accompanied FY 03 appropriations for NIH, seven NIH institutes were commended for their focus on the importance of translating basic research into application and treatment.
Several NIH institutes are now working on translational research projects of their own, and also are collaborating with each other as well. This collaboration is indicative of the nature of this research, which must cross disciplinary boundaries if it is to be successful.
APS regularly opens certain online articles for discussion on our website. Effective February 2021, you must be a logged-in APS member to post comments. By posting a comment, you agree to our Community Guidelines and the display of your profile information, including your name and affiliation. Any opinions, findings, conclusions, or recommendations present in article comments are those of the writers and do not necessarily reflect the views of APS or the article’s author. For more information, please see our Community Guidelines .
Please login with your APS account to comment.

Matching Psychology Training to Job Market Realities
APS President Wendy Wood discusses how graduate programs can change the habit of focusing on academic-career preparation.

Science in Service: Shaping Federal Support of Scientific Research
Social psychologist Elizabeth Necka shares her experiences as a program officer at the National Institute on Aging.

Finding Opportunities in Research Administration
In a conversation with APS President Wendy Wood, clinical scientist Christine Hunter shares how she’s applied skills learned in graduate school to her role as a government research director.
Privacy Overview

- Research Overview
- Research Activities
- Research Resources
- Training & Education
- Collaborate With NCATS
- Funding Overview
- Open Funding Opportunities
- Small Business Programs
- Funding Policy & Operating Guidelines
- Grant Information
- Challenges and Prize Competitions
- Scientific Review
- Conference Grant Information
- Resources for You
- Resources for Researchers
- Resources for Trainees
- Resources for Rare Disease Patients and Advocates
- News & Events Overview
- Newsletters
- Scientific Publications
- NCATS Overview
About Translational Science
- Advisory Committees
- Careers at NCATS
- Director's Corner
- Divisions & Offices
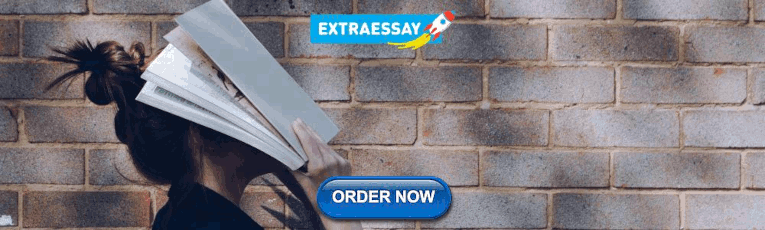
Translational Science Spectrum
The spectrum represents the stages of research involved in bringing more treatments to all people more quickly.
About the Translational Science Spectrum
The translational science spectrum shows the stages of translational research. The stages do not happen in a straight line or in one direction. Each stage builds upon and informs the others. At all stages, our staff and grantees come up with new approaches, prove their usefulness and share the findings. Patient involvement is a key feature of all stages in translation.

Basic Research
Basic research involves scientific exploration that can reveal fundamental mechanisms of biology, disease or behavior. Every stage of the translational research spectrum builds upon and informs basic research. NCATS scientists typically do not conduct basic research; however, insights gained from the center’s studies along the translational spectrum can inform basic research.

Preclinical Research
Preclinical research connects the basic science of disease with human medicine. During this stage, scientists develop model interventions to further understand the basis of a disease or disorder and find ways to treat it. Testing is carried out using cell or animal models of disease; samples of human or animal tissues; or computer-assisted simulations of drug, device or diagnostic interactions within living systems.
Related Research
- Assay Development
- Data Science and Informatics
- Platform Technologies
- Therapeutic Discovery and Development

Clinical Research
Clinical research includes studies to better understand a disease in humans and relate this knowledge to findings in cell or animal models; testing and refinement of new technologies in people; testing of interventions for safety and effectiveness in those with or without disease; behavioral and observational studies; and outcomes and health services research. The goal of many clinical trials is to obtain data to support regulatory approval for an intervention.
- Clinical Research Network
- Patient and Community Engagement
- Rare Diseases

Clinical Implementation
Clinical implementation involves the adoption of interventions that have been demonstrated to be useful in a research environment into routine clinical care for the general population. This stage also includes implementation research to evaluate the results of clinical trials and to identify new clinical questions and gaps in care.

Public Health
Public health includes studying health outcomes at the population level to determine the effects of diseases and efforts to prevent, diagnose and treat them. Findings help guide scientists working to assess the effects of current interventions and to develop new ones.
- Emergent Diseases

Translational Science Education Resources
We offer a collection of helpful educational resources and virtual classes to advance your knowledge of translational science.
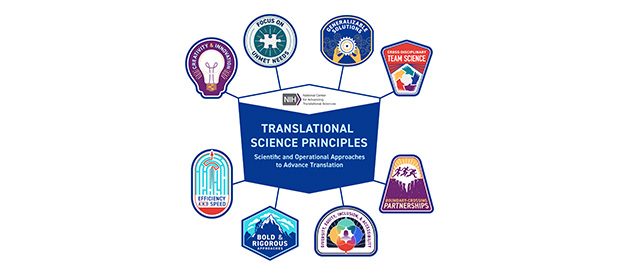
Translational Science Principles
Our [eight] translational science principals characterize effective translational science approaches.
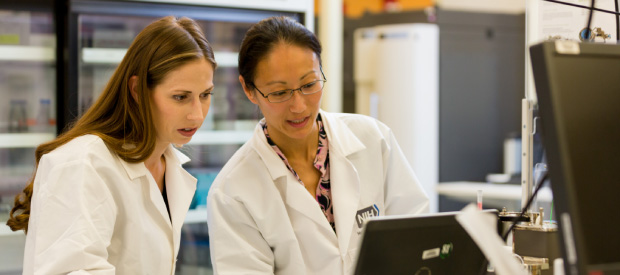
Translational science focuses on understanding the scientific principles underlying each step of the translational process.
Last updated on April 22, 2024
Featured Clinical Reviews
- Screening for Atrial Fibrillation: US Preventive Services Task Force Recommendation Statement JAMA Recommendation Statement January 25, 2022
- Evaluating the Patient With a Pulmonary Nodule: A Review JAMA Review January 18, 2022
Select Your Interests
Customize your JAMA Network experience by selecting one or more topics from the list below.
- Academic Medicine
- Acid Base, Electrolytes, Fluids
- Allergy and Clinical Immunology
- American Indian or Alaska Natives
- Anesthesiology
- Anticoagulation
- Art and Images in Psychiatry
- Artificial Intelligence
- Assisted Reproduction
- Bleeding and Transfusion
- Caring for the Critically Ill Patient
- Challenges in Clinical Electrocardiography
- Climate and Health
- Climate Change
- Clinical Challenge
- Clinical Decision Support
- Clinical Implications of Basic Neuroscience
- Clinical Pharmacy and Pharmacology
- Complementary and Alternative Medicine
- Consensus Statements
- Coronavirus (COVID-19)
- Critical Care Medicine
- Cultural Competency
- Dental Medicine
- Dermatology
- Diabetes and Endocrinology
- Diagnostic Test Interpretation
- Drug Development
- Electronic Health Records
- Emergency Medicine
- End of Life, Hospice, Palliative Care
- Environmental Health
- Equity, Diversity, and Inclusion
- Facial Plastic Surgery
- Gastroenterology and Hepatology
- Genetics and Genomics
- Genomics and Precision Health
- Global Health
- Guide to Statistics and Methods
- Hair Disorders
- Health Care Delivery Models
- Health Care Economics, Insurance, Payment
- Health Care Quality
- Health Care Reform
- Health Care Safety
- Health Care Workforce
- Health Disparities
- Health Inequities
- Health Policy
- Health Systems Science
- History of Medicine
- Hypertension
- Images in Neurology
- Implementation Science
- Infectious Diseases
- Innovations in Health Care Delivery
- JAMA Infographic
- Law and Medicine
- Leading Change
- Less is More
- LGBTQIA Medicine
- Lifestyle Behaviors
- Medical Coding
- Medical Devices and Equipment
- Medical Education
- Medical Education and Training
- Medical Journals and Publishing
- Mobile Health and Telemedicine
- Narrative Medicine
- Neuroscience and Psychiatry
- Notable Notes
- Nutrition, Obesity, Exercise
- Obstetrics and Gynecology
- Occupational Health
- Ophthalmology
- Orthopedics
- Otolaryngology
- Pain Medicine
- Palliative Care
- Pathology and Laboratory Medicine
- Patient Care
- Patient Information
- Performance Improvement
- Performance Measures
- Perioperative Care and Consultation
- Pharmacoeconomics
- Pharmacoepidemiology
- Pharmacogenetics
- Pharmacy and Clinical Pharmacology
- Physical Medicine and Rehabilitation
- Physical Therapy
- Physician Leadership
- Population Health
- Primary Care
- Professional Well-being
- Professionalism
- Psychiatry and Behavioral Health
- Public Health
- Pulmonary Medicine
- Regulatory Agencies
- Reproductive Health
- Research, Methods, Statistics
- Resuscitation
- Rheumatology
- Risk Management
- Scientific Discovery and the Future of Medicine
- Shared Decision Making and Communication
- Sleep Medicine
- Sports Medicine
- Stem Cell Transplantation
- Substance Use and Addiction Medicine
- Surgical Innovation
- Surgical Pearls
- Teachable Moment
- Technology and Finance
- The Art of JAMA
- The Arts and Medicine
- The Rational Clinical Examination
- Tobacco and e-Cigarettes
- Translational Medicine
- Trauma and Injury
- Treatment Adherence
- Ultrasonography
- Users' Guide to the Medical Literature
- Vaccination
- Venous Thromboembolism
- Veterans Health
- Women's Health
- Workflow and Process
- Wound Care, Infection, Healing
- Download PDF
- Share X Facebook Email LinkedIn
- Permissions
What Does the Proposed Causal Inference Framework for Observational Studies Mean for JAMA and the JAMA Network Journals?
- 1 Executive Managing Editor, JAMA and JAMA Network
- 2 Department of Emergency Medicine, Harbor-UCLA Medical Center, Torrance, California
- 3 Statistical Editor, JAMA
- 4 Deputy Editor, JAMA
- 5 Executive Editor, JAMA and JAMA Network
- Special Communication Causal Inference and Effects of Interventions From Observational Studies in Medical Journals Issa J. Dahabreh, MD, ScD; Kirsten Bibbins-Domingo, PhD, MD, MAS JAMA
The Special Communication “Causal Inferences About the Effects of Interventions From Observational Studies in Medical Journals,” published in this issue of JAMA , 1 provides a rationale and framework for considering causal inference from observational studies published by medical journals. Our intent is to invite discussion of this framework, explore its application in the context of specific study designs, and actively examine how this framework could be implemented and used by authors, peer reviewers, and editors of medical journals, including JAMA and the journals of the JAMA Network. Our overarching goal is to ensure that findings from observational designs may be appropriately interpreted in thoughtful and circumspect manners and applied by readers, other researchers, and clinicians, with the ultimate goal of improving patient care and public and global health.
Two points are worth underscoring in describing our intention with this publication. First, the proposal for causal interpretation of some observational studies should not be interpreted as diminished enthusiasm at JAMA for well-conducted randomized clinical trials that remain the foundation of evidence-based medicine. More than half of the Original Investigations published in JAMA last year were randomized clinical trials, and our examination of the reporting of observational studies does not signal an intent to depart from this practice. 2 JAMA and all of the journals of the JAMA Network also publish observational studies, many intending to provide evidence that addresses important causal clinical or public health questions, and some using designs and analytic approaches that produce results that may have a causal interpretation when key assumptions are plausible. Our responsibility to readers is to report scientific findings with precision and clarity. Part of this responsibility is to keep pace with methodological advances and to provide guidance and flexibility to authors to enable this precision and clarity in communicating the intent of the research and the carefully structured interpretation of the findings.
Second, while this framework could be applied to all observational studies published in JAMA and the JAMA Network, we anticipate that causal interpretations will be possible only in a select subset of these studies. Many observational studies do not address causal questions, and for some with this intent, causal inference may not be relevant, sufficiently well supported, or even possible. As acknowledged in the Special Communication: “For some observational studies that start with causal goals, causal inference may prove impossible; in these cases, estimates may be given associational interpretations. In addition, many important descriptive and predictive research questions can be answered by observational studies that do not require causal notions.” 1
So with excitement and trepidation, we will now consider how best to balance methodologic advances and semantic and interpretive flexibility in the reporting of research with the principles in our long-standing and often discussed reporting policy that generally limits use of causal language to well-done randomized clinical trials. 3 We anticipate that this will be a multistep process of considering potential changes, including how and when we can apply the proposed causal inference framework to select observational studies. Next steps will include reviewing when and how specific observational study designs and analyses can support causal inferences. To support this part of our process, JAMA will publish a new set of articles in the Guide to Statistics and Methods series that address how the proposed causal inference framework may be applied to specific study designs and methods. These guides will build on previous guidance 4 - 10 and include practical tips and concrete examples of specific study designs and analyses, such as target trial emulation, instrumental variable analysis, regression discontinuity, interrupted time series, difference-in-difference, and mediation analysis. Reports of these studies as well as nonrandomized controlled studies (or other “quasi-experimental” studies) will require specific conditions be met to support casual inferences, including clearly discussing the necessary assumptions in view of background knowledge; incorporating design elements to improve plausibility of assumptions; implementing statistical methods to address bias due to confounding, selection, missing data, and measurement error; and properly quantifying uncertainty.
In parallel, there will be continued discussion among our journal editors and statistical editors, engagement with researchers and authors, and plans to retain and identify additional peer reviewers with understanding of causal inference methods as well as knowledge to adequately judge the appropriateness of these methods as explicated in reports of studies.
We look forward to readers’ and other stakeholders’ comments about the proposed framework as we continue exploring how best to apply casual inference concepts and provide recommendations for authors, reviewers, editors, and readers.
Published Online: May 9, 2024. doi:10.1001/jama.2024.8107
Corresponding Author: Annette Flanagin, RN, MA ( [email protected] ).
Conflict of Interest Disclosures: Dr Lewis reported serving as senior medical scientist at Berry Consultants LLC, a statistical consulting firm focusing on the design, implementation, and analysis of adaptive and platform clinical trials. No other disclosures were reported.
See More About
Flanagin A , Lewis RJ , Muth CC , Curfman G. What Does the Proposed Causal Inference Framework for Observational Studies Mean for JAMA and the JAMA Network Journals? JAMA. Published online May 09, 2024. doi:10.1001/jama.2024.8107
Manage citations:
© 2024
Artificial Intelligence Resource Center
Cardiology in JAMA : Read the Latest
Browse and subscribe to JAMA Network podcasts!
Others Also Liked
- Register for email alerts with links to free full-text articles
- Access PDFs of free articles
- Manage your interests
- Save searches and receive search alerts
- UB Directory
- Office of the Provost >
- Academic & Administrative Units >
Daly and Jacobs receive IPRO Quality Award
By Samantha Nebelecky
Published May 9, 2024

L-R: Christopher Daly, PharmD/MBA, and David Jacobs, PharmD, PhD
Christopher Daly, PharmD/MBA, and David Jacobs, PharmD, PhD, along with their team, have received a 2024 IPRO Quality Award for their social determinants of health (SDoH) pharmacy program within New York Community Pharmacy Enhanced Services Network (CPESN) member pharmacies.
Related Links
- Pharmacies are ideal location for community social needs screening
- CTSI Translational Pilot Studies Program awards grants to SPPS faculty
IPRO is a non-profit healthcare quality evaluation organization that works with government agencies, providers and patients to implement innovative programs that bring policy ideas to life.
IPRO’s Quality Awards recognize outstanding performance by health care providers and stakeholders in New York State. These awards acknowledge exemplary healthcare quality improvement projects completed by healthcare organizations or providers in partnership with the IPRO Quality Innovation Network – Quality Improvement Organization (QIN-QIO), a collaboration of three organizations with decades of experience serving as Medicare QIOs.
Daly, clinical associate professor, and Jacobs, assistant professor, both of the UB School of Pharmacy and Pharmaceutical Sciences (SPPS) Department of Pharmacy Practice, along with their team members Durdana Iqbal, PharmD, SPPS Pharmaceutical Health Services and Outcomes Fellow, Walter Gibson, SPPS data analyst, John Croce, RPh, President, CPESN NY and Managing Network Facilitator, Jessica Anderson, PharmD, Network Facilitator, Upstate NY, CPESN NY, and Alec Gillies, BS ’94, Network Lead Luminary, WNY Network, CPESN NY, were recognized for their innovative work from their collaboration with NY CPESN member pharmacies, specifically the implementation of a SDoH pharmacy program.
The overarching project titled, “Effectiveness of a Social Determinants of Health Pharmacy Program Implemented in Community Pharmacies,” looked to explore how community pharmacy practice continues to expand its role in public health. Through a mixed method approach, findings will allow for a better understanding of the training, workflow integration, and overall program implementation, which is necessary as new social needs services continue to grow within accessible pharmacy settings.
SPPS researchers, working alongside collaborators, developed and evaluated the program focused on optimizing implementation strategies that led to greater screening acceptance and referral uptake. The preliminary results from January through December 2023 show more than 1,000 screenings conducted, with 64% of them carried out in rural areas across the state. The program demonstrates a high efficiency rate along with a 26% resolution referral rate. Common social needs identified included food insecurity, housing instability, and healthcare access. Further analysis evaluating health care utilization and economic measures are underway and will be completed by summer 2024.
Daly, Jacobs, and their team will be recognized at our SPPS Spring Celebration: Graduation and Awards Luncheon on May 17. They will also be honored at IPRO’s Annual Meeting and Luncheon on June 11 where they will be presented with their award.
For over 135 years, the University at Buffalo School of Pharmacy and Pharmaceutical Sciences has continually been a leader in the education of pharmacists and pharmaceutical scientists, renowned for innovation in clinical practice and research. The school is accredited by the American Council of Pharmaceutical Education and is the No. 1 ranked school of pharmacy in New York State and No. 19 in the United States by U.S. News & World Report.
Featured Centers and Institutes

PhD Excellence Initiative
A campus-wide, student-centric effort to ensure that UB’s PhD programs remain among the strongest in the world.
Recent University News
- 5/10/24 Study: For treating retinopathy of prematurity, UB-led research proves that ranibizumab is safe
- 5/10/24 Neurology Educator Honored With AAN Innovation Award
- 5/10/24 Gresham lecturer shares personal definition of compassion
- 5/10/24 UB’s new mass spectrometer can accelerate discoveries
- 5/10/24 UB English professor reworks a classic literary anthology
- Open access
- Published: 08 May 2024
Deep learning imaging phenotype can classify metabolic syndrome and is predictive of cardiometabolic disorders
- Jacob S. Leiby 1 ,
- Matthew E. Lee 1 ,
- Manu Shivakumar 1 ,
- Eun Kyung Choe 2 &
- Dokyoon Kim ORCID: orcid.org/0000-0002-4592-9564 1 , 3
Journal of Translational Medicine volume 22 , Article number: 434 ( 2024 ) Cite this article
119 Accesses
Metrics details
Cardiometabolic disorders pose significant health risks globally. Metabolic syndrome, characterized by a cluster of potentially reversible metabolic abnormalities, is a known risk factor for these disorders. Early detection and intervention for individuals with metabolic abnormalities can help mitigate the risk of developing more serious cardiometabolic conditions. This study aimed to develop an image-derived phenotype (IDP) for metabolic abnormality from unenhanced abdominal computed tomography (CT) scans using deep learning. We used this IDP to classify individuals with metabolic syndrome and predict future occurrence of cardiometabolic disorders.
A multi-stage deep learning approach was used to extract the IDP from the liver region of unenhanced abdominal CT scans. In a cohort of over 2,000 individuals the IDP was used to classify individuals with metabolic syndrome. In a subset of over 1,300 individuals, the IDP was used to predict future occurrence of hypertension, type II diabetes, and fatty liver disease.
For metabolic syndrome (MetS) classification, we compared the performance of the proposed IDP to liver attenuation and visceral adipose tissue area (VAT). The proposed IDP showed the strongest performance (AUC 0.82) compared to attenuation (AUC 0.70) and VAT (AUC 0.80). For disease prediction, we compared the performance of the IDP to baseline MetS diagnosis. The models including the IDP outperformed MetS for type II diabetes (AUCs 0.91 and 0.90) and fatty liver disease (AUCs 0.67 and 0.62) prediction and performed comparably for hypertension prediction (AUCs of 0.77).
Conclusions
This study demonstrated the superior performance of a deep learning IDP compared to traditional radiomic features to classify individuals with metabolic syndrome. Additionally, the IDP outperformed the clinical definition of metabolic syndrome in predicting future morbidities. Our findings underscore the utility of data-driven imaging phenotypes as valuable tools in the assessment and management of metabolic syndrome and cardiometabolic disorders.
Cardiometabolic disorders, such as type II diabetes and cardiovascular disease, have become a global health concern with significant morbidity and mortality rates [ 1 , 2 , 3 , 4 ]. Metabolic syndrome, a condition characterized by a clustering of metabolic abnormalities, is a known risk factor for these diseases [ 5 , 6 , 7 , 8 ]. The overall prevalence of metabolic syndrome in the United States has moderately increased among all adults in the last decade, but has increased significantly among specific groups, including women, young adults, and Asian and Hispanic adults [ 9 ]. Clinical definitions of metabolic syndrome vary among organizations, but typically consist of a combination of high blood pressure, dyslipidemia, central obesity, and glucose intolerance [ 10 ]. Lifestyle modifications and medications have been shown to effectively mitigate these conditions, reducing the incidence of metabolic syndrome [ 11 , 12 , 13 ]. Therefore, early detection and intervention for individuals with metabolic abnormalities may help reduce the risk of developing more serious metabolic-related diseases.
Image-derived phenotypes (IDPs) are quantitative measurements extracted from medical imaging that are used as potential biomarkers for disease. Traditionally, hand-crafted features based on prior expert knowledge, such as volume, activation, or attenuation of regions of interest are extracted to overcome the high dimensionality of imaging data. IDPs from brain imaging modalities have shown strong associations with morbidity and genetic risk for Alzheimer’s disease [ 14 , 15 , 16 ]. Studies have also explored IDPs from computed tomography (CT) scans and magnetic resonance imaging and their associations with metabolic-related diseases [ 17 , 18 , 19 ]. In particular, hand-crafted features including abdominal fat and muscular measurements, aortic calcium quantification, and volumetric liver attenuation acquired from abdominal CT scans were found to be strongly associated with metabolic syndrome and subsequent cardiovascular events [ 18 , 20 ]. Unenhanced abdominal CT scans are a particularly favorable modality for extracting IDPs as they are performed for many indications and allow clinicians to extract additional biologically relevant information in a non-invasive manner.
Hand-crafted radiomic features, such a volumetric measurements, are efficient at converting high-dimensional data into simple representations that can be used for disease association or classification. However, imaging data consists of rich information, and these hand-crafted features rely on previous knowledge and may miss biologically relevant signal. Recent advances in machine learning have enabled data-driven approaches to derive IDPs using deep learning-based models to that are able to condense high-dimensional data into compact representations of unobservable information. Data-driven IDPs have been proposed in a variety of settings for both population-based and disease-specific phenotypes [ 21 , 22 , 23 , 24 , 25 ].
In this study, we aimed to develop a deep learning-derived IDP of metabolic abnormality and use it to predict future occurrence of cardiometabolic disorders. To do this, we treated the clinical definition of metabolic syndrome as a proxy of metabolic abnormality and trained a deep learning model to classify it from unenhanced abdominal CT scans. We focused on three-dimensional (3D) segmented liver regions to directly compare our results to the performance of liver attenuation (expressed as Hounsfield units), a hand-crafted feature that is linearly correlated with hepatic fat and is highly associated with metabolic syndrome [ 18 , 26 , 27 , 28 ]. Additionally, we included comparisons to visceral adipose tissue area (VAT), another traditional IDP strongly associated with metabolic syndrome [ 18 ]. Our IDP demonstrated significant superiority over attenuation and VAT in classifying metabolic syndrome. Furthermore, we performed an association analysis of the IDP with 115 clinical phenotypes and observed significant associations with anthropometric measurements and endocrine, metabolism, and digestive phenotypes. Lastly, we used the IDP to predict the future occurrence of metabolic-related morbidities. Our findings revealed that the IDP significantly enhanced the prediction of fatty liver disease and type II diabetes compared to relevant baseline covariates and the clinical definition of metabolic syndrome and performed comparably for prediction of hypertension.
Data and clinical definitions
Study population.
For the model development, we used data from a comprehensive health check-up cohort of a Korean population consisting of 2,268 individuals (Table 1 ). The details of the dataset are described elsewhere [ 29 ]. Briefly, at the Seoul National University Hospital Gangnam Center, individuals participate in comprehensive health screening for anthropometric, cardiovascular, digestive, endocrinological, metabolic, hematologic, lung, and renal conditions with various clinical tests including abdominal computed tomography (CT) scans. For 1,397 individuals, we collected the 5-year follow up data (Supplementary Table 1). For external replication of the developed model, we used data and CT scans of preoperative evaluation tests from stage I colorectal cancer patients, who underwent curative colectomy in Seoul National University hospital [ 30 ]. We used the non-contrast (unenhanced) abdominal CT scan stored in Digital Imaging and Communications in Medicine (DICOM) file format. Abdominal CT scans were performed using a 64-slice multi-detector CT scanner (Brilliance 64 scanners; Philips Healthcare, Amsterdam, Netherlands) with 3 mm thickness and increment.
Clinical assessment and definitions
All participants entered the test after at least ten hours of fasting. For the definition of metabolic syndrome, we used the criteria suggested by the American Heart Association [ 31 ]. In the population for model development, metabolic syndrome was defined when at least three of the following metabolic risk factors were positive: increased waist circumference (male > 102 cm, female > 88 cm); triglycerides > = 150 mg/dL; decreased high-density lipoprotein (HDL) cholesterol (male < 40 mg/dL, female < 50 mg/dL); fasting glucose > = 100 mg/dL or on medication for hyperglycemia; and blood pressure > = 130/85 mmHg or on medication for hypertension, resulting in sixteen possible combinations. Among individuals with metabolic syndrome, the most common contributing factors were increased blood glucose, blood pressure, and waist circumference; elevated blood glucose was the most common component overall (Supplementary Fig. 1 ). In the validation population from colorectal cancer patients, since the waist circumference, HDL cholesterol, triglyceride data were not available, we used a modified criteria for metabolic syndrome, indirectly; at least three of the following metabolic risk factors were positive: increased body mass index > = 25 kg/m2; on medication of dyslipidemia; fasting glucose > = 100 mg/dL or on medication for hyperglycemia; and blood pressure > = 130/85 mmHg or on medication for hypertension. The visceral adipose tissue area was measured at the level of the umbilicus of the abdominal CT scan, as previously described [ 32 ].
For all follow up analyses, we defined hypertension and type II diabetes as “on medication for the disease”, and abdominal ultrasound was used to diagnose fatty liver. The abdominal ultrasound procedure was performed by an experienced radiologist. The definition of fatty liver was based on the vascular blurring, attenuation, hepatorenal echo contrast and liver brightness in ultrasonography image [ 33 ]. We classified as “normal liver” versus “at least mild degree of fatty liver”, annotated by radiologists.
Liver segmentation in abdominal CT scans
Abdominal ct preprocessing.
All DICOM series were converted to 3D Neuroimaging Informatics Technology Initiative (NIfTI) file format using the dicom2nifti python package. 3D volumes were preprocessed using the nnU-Net software [ 34 ]. Briefly, the intensity values are clipped at the 0.5 and 99.5 percentiles, and each volume is resampled to the median values of the training data spacing in each axis.
Segmentation model architecture and optimization
The segmentation model followed the U-Net architecture (Supplementary Fig. 2 a) [ 34 ]. This model, popular in medical imaging segmentation, adopts an encoder-decoder structure aiming to learn a dense embedding of the input data (encoder) and localize the relevant information back into the input dimensions through an expanding path (decoder). We again used the nnU-Net software to optimize the network topology and the input patch size [ 34 ]. The final patch size was set to 28 × 256 × 256.
Training and validation
The segmentation model was trained and validated on 358 abdominal CT scans with the liver regions annotated. The annotations were generated by a technician using a commercially available segmentation software program (AVIEW Modeler, version 1.1.42, Corelinesoft Co. Ltd., Seoul, South Korea). We performed 5-fold cross validation and used the Dice coefficient as the performance metric.
The top-performing segmentation model was used to create liver segmentation masks for all remaining samples.
Image-derived phenotypes
Liver attenuation.
Liver attenuation values were extracted by applying the mask to the original CT volume and calculating the median attenuation of the voxels predicted as liver.
Visceral adipose tissue area
Visceral adipose tissue area was measured at the level of the umbilicus of the abdominal CT scan, as previously described [ 32 ]. We defined the visceral adipose tissue are as the adipose tissue area, in parameter cm2, located intra-abdominally, confined by the parietal peritoneum [ 35 ].
Deep learning image-derived phenotype
To derive the imaging phenotype, we initially masked the original volumes, selecting only the predicted liver region. Next, we employed a multi-stage deep learning pipeline [ 36 ]. First, we used the pre-trained encoder from the segmentation model to extract a latent representation of the data. Specifically, we performed global average pooling over each encoder block output to aggregate the spatial information and concatenated the pooled embeddings (Supplementary Fig. 2 b). Second, we input these embeddings into a neural network classifier with a single node output to classify metabolic syndrome using the binary cross-entropy loss function. (Supplementary Fig. 2 c). More details on the model architecture are described in Supplementary Fig. 2 . As the segmentation model was optimized for patch-based input, each input volume was divided into \( X\) three-dimensional patches, and a latent representation was created for each patch. The classifier incorporated a learned gated attention-pooling function to aggregate the patch information into a volume-level embedding [ 37 ]. The classifier was trained using the AdamW optimizer in PyTorch version 1.12.1 with default parameters. To validate the deep learning IDP, we used stratified five-fold cross validation repeated ten times for a total of fifty models. For each split we reserved 20% of the total dataset for testing and evaluation and with the remaining data, trained on 80% and used 20% as a validation set to tune the hyperparameters.
For the metabolic syndrome classification, the final hidden layer of the classifier, containing 256 nodes, was extracted as the IDP. For the phenotypic association and follow-up analyses, the output of the classifier was used as a condensed version of the IDP.
Baseline metabolic syndrome classification
We employed elastic-net logistic regression to incorporate the IDP with clinical covariates to predict metabolic syndrome using the R package glmnet version 4.1-1. Specifically, we used the default parameters of the cv.glmnet function to perform ten-fold cross-validation in training datasets only and used the optimal hyper parameters to evaluate the performance in the hold-out testing datasets (the validation sets used in hyperparameter tuning for IDP development are not used in these analyses). All numerical features were centered and scaled based on the training dataset means and standard deviations.
Phenome-wide association analysis
We conducted linear regression for each phenotype of interest, correcting for age and sex in R version 4.1.0. All reported p-values were corrected for multiple-testing using the Bonferroni correction.
Follow-up disease analyses
We conducted predictive analyses for three metabolic-related morbidities—hypertension, fatty liver disease, and type II diabetes. For each disease, we removed all individuals at baseline who already had the respective condition, extracted baseline condensed IDPs for the remaining individuals, and collected baseline metabolic syndrome status and covariate measurements. We compared models that included relevant baseline covariates, clinically defined metabolic syndrome, and the IDP.
We used the same training splits as in the previous analyses to fit a logistic regression, and then used that model to predict disease status in the hold-out datasets (the validation sets used in hyperparameter tuning for IDP development are not used in these analyses). All numerical features were centered and scaled based on the training dataset means and standard deviations.
To classify individuals as metabolically abnormal at baseline, the optimal cutoff value of the IDP was determined as the threshold value that maximized the sensitivity and specificity [ 38 ].
Performance evaluation and statistical tests
We employed the area under the receiver operating characteristic curve (AUC) and area under the precision-recall curve (AURPC) as measures of model performance. A paired t -test was used to compare the AUCs between different models.
For statistical tests comparing demographic and clinical information between metabolic syndrome positive and negative cases, we used the t -test for continuous variables and the Fisher exact test for categorical variables in R version 4.1.0.
IDP accurately classifies baseline metabolic syndrome
To develop the image-derived phenotype (IDP) of metabolic abnormality, we constructed a multi-stage deep learning pipeline that involved segmentation of the liver region from 3D unenhanced CT scans followed by training a metabolic syndrome classifier on the segmentation model embeddings (Fig. 1 a, Methods). The liver segmentation model was trained on 358 manually annotated CT scans and achieved a Dice score of 0.98 through five-fold cross-validation. The final hidden layer of the trained metabolic syndrome classifier is extracted as the IDP. (The classification network achieved a mean AUC of 0.81 (0.02) and AUPRC of 0.52 (0.05)). Using regularized logistic regression to classify metabolic syndrome, the IDP achieved an AUC of 0.82 through ten times repeated five-fold cross-validation compared to an AUC of 0.69 using extracted volumetric liver attenuation, an AUC of 0.80 using visceral adipose tissue area (VAT), and an AUC of 0.59 using age and sex (Fig. 1 b, top, Table 2 , and Methods). Additionally, we assessed the utility of our model in combination with VAT. When including VAT as an additional covariate the model achieved an AUC of 0.84, suggesting complementary information between the two IDPs (Fig. 1 b, bottom, and Table 2 ).
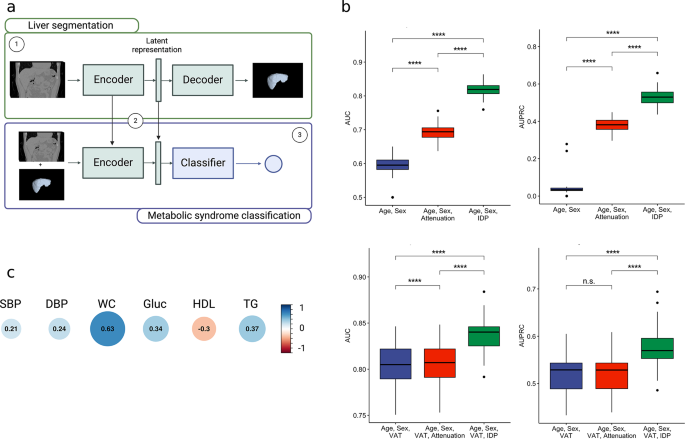
a , Overview of three-stage IDP development model: Stage 1 - Training the 3D liver segmentation model; Stage 2 - Using the pretrained encoder to extract latent representations from input CT scans; Stage 3 - Training the metabolic system classifier. b , Predictive performance comparing covariates and attenuation to the IDP. c , Correlation between the IDP and metabolic syndrome risk factors. IDP, image-derived phenotype; AUC, area under receiver-operating curve; Attenuation, median volumetric liver attenuation (expressed as Hounsfield units); VAT, visceral adipose tissue; SBP, systolic blood pressure; DBP, diastolic blood pressure; WC, waist circumference; Gluc, glucose; HDL, high-density lipoprotein; TG, triglycerides; **** P < 0.0001, n.s. not significant, paired t-test
The output of the deep learning model exhibited significant correlations with each of the individual metabolic syndrome components (Fig. 1 c). The direction of these correlations aligns with the definition of metabolic syndrome, with the strongest correlations observed for waist circumference and lipid levels. Many studies have previously shown the associations between waist circumference and lipid levels with liver diseases [ 39 , 40 , 41 ].
To validate the IDP, we predicted metabolic syndrome in 521 individuals from an external cohort (Supplementary Table 3). For this analysis, we used a modified definition of metabolic syndrome due to data availability (Methods). The IDP significantly outperformed covariate models, achieving an AUC of 0.66 compared to AUC of 0.62 and 0.54 for liver attenuation and age and sex, respectively (Supplementary Fig. 3 and Table 2 ). This decrease in performance compared to the development dataset may be due to the underlying disease profiles and imaging characteristics of the external dataset.
These results suggest that our framework learns a strong IDP for metabolic abnormality. Through cross-validation, it can better classify metabolic syndrome versus existing biomarkers and covariates and this trend holds when applied to an external validation cohort.
IDP shows sex-specific differences
To investigate potential variations in the IDP between sex, we performed stratified analyses. Males have a metabolic syndrome prevalence rate of 26% (410/1558) and females have a prevalence of 10% (74/710). We found that the IDP significantly improves prediction of metabolic syndrome in both males and females (Fig. 2 ; Table 3 ). Additionally, incorporating VAT with our IDP yielded improved performance in both sexes compared to a model utilizing VAT alone. Interestingly, for males the IDP alone achieved a higher AUC than VAT and for females VAT outperformed the IDP (Table 3 ).
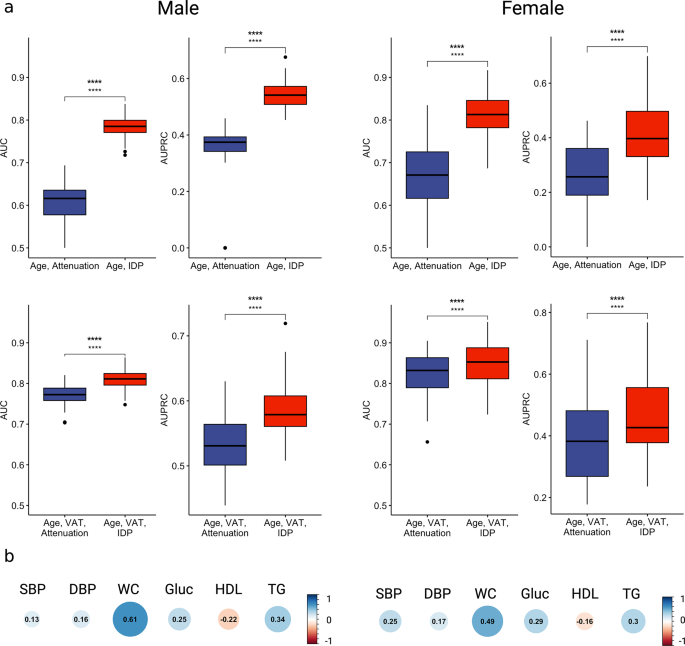
a , Predictive performance comparing covariate models to the IDP, stratified by sex. (Male, left column. Female, right column). b , Correlation between the IDP and metabolic syndrome risk factors. IDP, image-derived phenotype; AUC, area under receiver-operating curve; Attenuation, median volumetric liver attenuation (expressed as Hounsfield units); VAT, visceral adipose tissue; SBP, systolic blood pressure; DBP, diastolic blood pressure; WC, waist circumference; HDL, high-density lipoprotein; TG, triglycerides; **** P < 0.0001, paired t-test
To investigate the biological signal captured by the IDP, we analyzed the underlying differences in etiology between sex. Among males, the most prevalent components for diagnosing metabolic syndrome were glucose levels, blood pressure, and waist circumference (Supplementary Fig. 1 ). In females, the most prevalent components were glucose levels, HDL levels, and waist circumference (Supplementary Fig. 1 ). Consistently, the IDP demonstrated the strongest correlations with waist circumference, glucose, and triglycerides for both males and females (Fig. 2 b).
IDP is associated with metabolic-related phenotypes
To explore the biological relevance of the IDP, we performed a phenome-wide association analysis (PheWAS) between 115 phenotypes corroborated by comprehensive health check-ups and the output of the IDP classifier (Supplementary Table 4). We found that the IDP is significantly associated with 32 phenotypes after Bonferroni correction (Fig. 3 and Supplementary Table 4 ). Notably, the most significant associations were observed with anthropometric measurements, including fat and muscle measurements, which are highly correlated with waist circumference, a component of metabolic syndrome. Other metabolic syndrome related phenotypes, including hypertension, hemoglobin A1c, type II diabetes diagnosis, and dyslipidemia, are also strongly associated. Hematologic features, such as white blood cell counts, as well as the endocrine phenotype uric acid are also significant. Additionally, digestive features related to the liver, such as alanine transaminase (ALT), aspartate transaminase (AST), and gamma-glutamyl transferase (GGT), demonstrated notable associations with the IDP. Of particular interest, the IDP exhibited a strong association with fatty liver disease compared to its association with liver attenuation (Supplementary Table 4). These findings suggest that the IDP, developed to classify metabolic syndrome, may also possess predictive capabilities for other metabolic-related conditions.
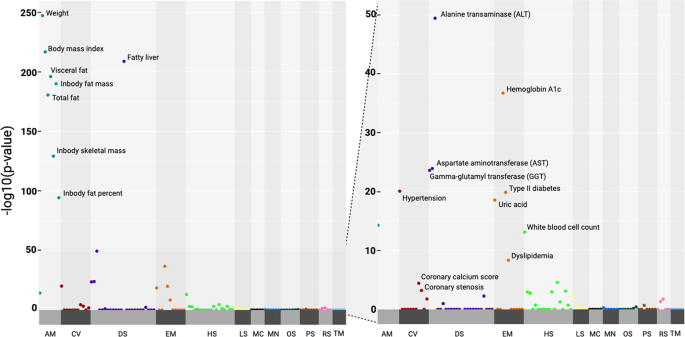
Phenotypic association analysis, corrected for age and sex. All P values are Bonferroni corrected. AM, anthropomorphic measurements; CV, cerebro-cardiovascular; DS, digestive system; EM, endocrine and metabolism; HS, hematologic system; LS, lifestyle; MC, musculoskeletal; OS, ophthalmic system; PS, pulmonary system; RS, renal system; TM, tumor markers
IDP predicts future occurrence of metabolic-related morbidities
We conducted predictive analyses for three morbidities—hypertension, fatty liver disease, and type II diabetes. Briefly, for each disease, we removed all individuals at baseline diagnosed with the respective condition, collected baseline IDP, covariates, and metabolic syndrome status from the remaining individuals, and predicted future disease (Fig. 4 a). For these analyses, we consider the output of the classifier as a condensed representation of the IDP.
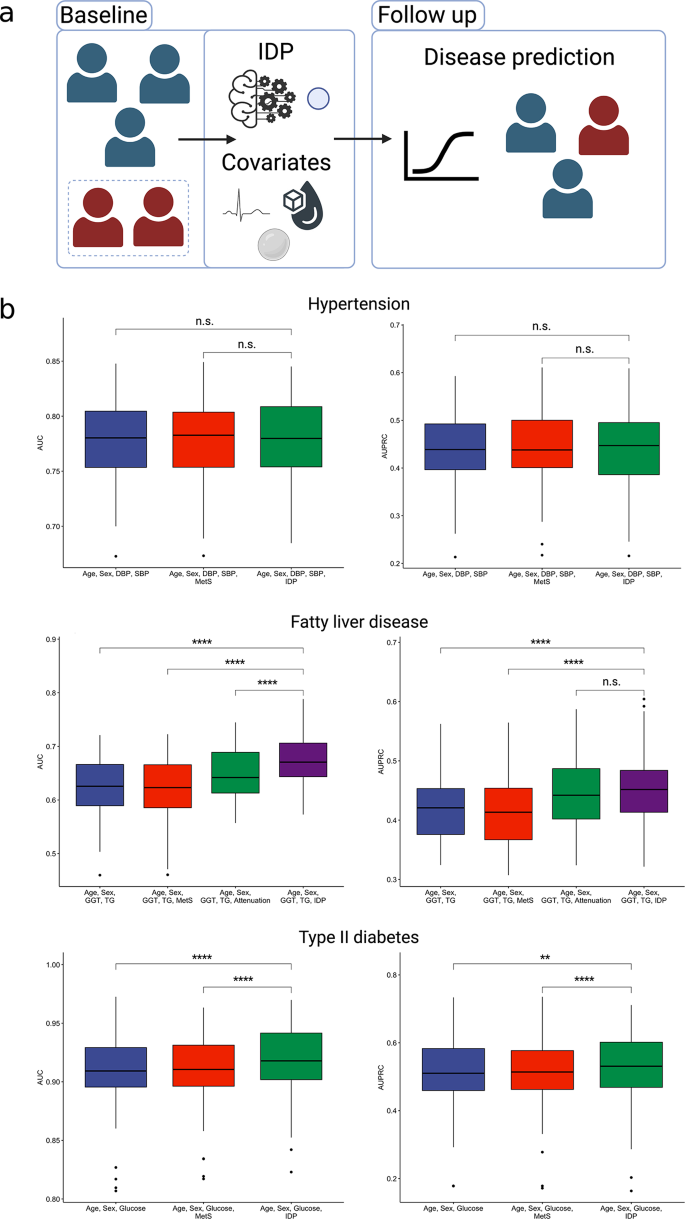
Overview of the follow-up disease prediction framework. Individuals diagnosed with the disease of interest as baseline are removed, and the baseline IDP and covariates are extracted from the remaining individuals. These are used as features to predict disease in the follow-up period. b , Predictive performance comparing relevant covariates and baseline metabolic syndrome diagnosis to the IDP. First row, hypertension; second row, type II diabetes; third row, fatty liver disease. SBP, systolic blood pressure; DBP, diastolic blood pressure; MetS, baseline metabolic syndrome; GGT, gamma-glutamyl transferase; TG, triglycerides; IDP, image-derived phenotype; AUC, area under receiver-operating curve.**** P < 0.0001, ** P < 0.001, n.s. not significant, paired t-test
For hypertension prediction, we incorporated baseline blood pressure measurements as additional covariates. We found that the deep learning IDP did not improve the performance of predicting hypertension (Fig. 4 b; Table 4 ). We included baseline GGT levels, TG levels, and liver attenuation as additional covariates for fatty liver disease prediction. Our analysis demonstrated that the IDP model significantly outperformed the model with covariates alone and the model including baseline clinical metabolic syndrome (Fig. 4 b; Table 4 ). For type II diabetes prediction, baseline glucose levels were included. Once again, we observed that the IDP outperformed the two baseline models (Fig. 4 b; Table 4 ). We see the same trends for disease prediction in models with only age and sex as covariates (Supplementary Table 5).
We performed additional analyses in which individuals who were not diagnosed with metabolic syndrome at baseline were removed. We used logistic regression to compare the performance of models containing baseline covariates alone and models including the IDP. In all cases except for hypertension, the models including the IDP demonstrated superior performance compared to the covariate models (Supplementary Table 6).
To illustrate the robustness of the deep learning IDP, we identified cases where individuals appeared clinically healthy at baseline but subsequently developed metabolic-related morbidities in the follow-up period. Specifically, we selected individuals who had an IDP value that classified them as metabolically abnormal (Methods), however no diagnosis of metabolic syndrome or disease at baseline, a total of 705. We identified 34% (50/149) of the individuals who developed hypertension, 66% (126/190) of the individuals who developed fatty liver disease, and 78% (31/40) of the individuals who developed type II diabetes. Many of these individuals had two metabolic risk factors at baseline, high glucose levels being the most prevalent (Supplementary Fig. 4 ).
Metabolic syndrome represents a significant risk factor for the development of severe cardiometabolic diseases. It is characterized by a set of potentially reversible metabolic abnormalities, emphasizing the importance of early and accurate detection for clinical management. In this study, we successfully developed a deep learning-based image-derived phenotype of metabolic abnormality that can classify metabolic syndrome directly from unenhanced abdominal CT scans in a large cohort of over 2,000 individuals. Our approach involved training a 3D segmentation model to localize the liver and utilizing this region to predict metabolic syndrome through a combination of a pretrained segmentation encoder and a deep learning classifier. In comparison to traditional hand-crafted radiomic features that are known to be significantly associated with metabolic syndrome, volumetric liver attenuation and visceral adipose tissue, our analysis demonstrated that the deep learning IDP was able to more accurately classify metabolic syndrome than the radiomic features and clinical covariates alone.
We performed sex stratified analysis and found that for males, the IDP showed a stronger classification performance than visceral adipose tissue, while in females the opposite was shown, and visceral adipose tissue showed stronger performance. Studies suggest that visceral fat influences metabolic syndrome risk differently between sex, posing a greater risk for females. This risk is linked more to fat composition and distribution rather than total fat quantity [ 42 ].. Males tend to accumulate fat viscerally, predominantly in the abdomen, while females predominantly store it subcutaneously [ 43 , 44 ]. Thus, the risk of metabolic syndrome is often assessed based on sex-specific visceral fat thresholds rather than absolute amounts [ 45 ]. This implies that females may be more metabolically sensitive to visceral fat accumulation.
The IDP was strongly correlated with each of the metabolic risk components and showed significant associations with 32 clinical phenotypes, many of which are directly related to cardiometabolic abnormalities. Given that the IDP is derived from imaging focused on the liver, liver-specific features including alanine transaminase (ALT), aspartate transaminase (AST), and gamma-glutamyl transferase (GGT), and fatty liver disease were some of the most strongly associated phenotypes.
To explore the clinical utility of the IDP, we further investigated its predictive capabilities for future occurrences of cardiometabolic diseases, including hypertension, fatty liver disease, and type II diabetes in a subset of over 1,300 individuals who had follow-up data. Inclusion of the IDP significantly improved predictive performance for each of the diseases when compared to baseline covariate models for fatty liver disease and type II diabetes. Our analyses clearly demonstrated that the IDP outperformed the clinical definition of metabolic syndrome in terms of predictive accuracy. We hypothesize that this may be due to the nature of the clinical metabolic syndrome definition. There are sixteen possible combinations of five risk factors for diagnosis, but an individual can have up to two risk factors and not be diagnosed. Due to the heterogeneity of the diagnosis, the proposed IDP may be learning information at a more granular scale. Additionally, the IDP successfully identified a subset of individuals who appeared clinically healthy at baseline but later developed a cardiometabolic disease during the follow-up period. Specifically, the IDP identified 34%, 66%, and 78% of the individuals who developed hypertension, fatty liver disease, and type II diabetes, respectively, despite these individuals not meeting the criteria for a metabolic syndrome diagnosis at baseline.
We validated the IDP in an external validation dataset, which was collected for a different purpose (colorectal cancer patients) compared to the comprehensive health check-up cohort. This difference in data collection may have contributed to the reduced performance observed in the external validation, as the underlying characteristics and disease profiles of the cohorts varied. Although the performance in classifying metabolic syndrome decreased, it remained significantly higher than the hand-crafted feature and covariate models. The reduced performance could also be attributed to inherent differences in data resulting from variations in devices and acquisition protocols used for obtaining the abdominal CT scans. Domain shift, which refers to a change in the underlying data distribution, is a well-known phenomenon in machine learning research that can lead to challenges in model translation from the training data to external datasets. This issue is particularly prevalent especially in medical imaging research, where datasets are collected within specific scopes that encompass factors such as time-frame, demography, clinical settings, and acquisition devices [ 46 ]. It is important to note that while the external validation cohort differed in its intended use, it still provided valuable insights into the generalizability and robustness of the IDP model. The variations observed between the cohorts emphasize the need for further validation in diverse populations and contexts to establish the broader applicability of the IDP.
This study has some limitations that need to be addressed in future research, including the characteristics of the selected cohort and the interpretability of the model. The comprehensive health check-up population consisted mainly of individuals who were generally healthy, which limited our ability to study a broader range of cardiometabolic diseases. We chose hypertension, fatty liver disease, and type II diabetes due to their moderate representation in the dataset. While we were able to validate the IDP for metabolic syndrome prediction using an external cohort, we were unable to externally validate the prediction of subsequent diseases in the follow-up analyses. Additionally, all individuals in this study belonged to a Korean population, necessitating replication in more diverse populations. Further, due to lack of comprehensive annotated data, the proposed IDP pipeline focused only on the liver. We would expect additional information would be found from other regions of the scan, as suggested by previous work [ 18 ]. Finally, while the deep learning IDP is a stronger representation of metabolic abnormality, it lacks biological interpretability. In future work, additional analyses need to be performed in order to better understand the underlying biological processes driving the performance of the proposed IDP and validating its connections with individual metabolic risk factors. Additionally, it is necessary to conduct prospective studies in diverse clinical settings to further validate our method and explore the utility of the IDP guiding personalized treatment strategies.
This work establishes a robust framework for data-driven IDP discovery in the context of metabolic abnormality. It emphasizes the efficient approach of using non-specific CT scans for IDP extraction and underscores the utility of deep learning-driven imaging phenotypes as valuable tools in the assessment and management of metabolic syndrome and cardiometabolic disorders. Abdominal CT scans, performed for various reasons, currently provide insights restricted to the radiologist’s analysis, primarily concentrating on specific organ irregularities. However, providing the possibility of additional imaging phenotypes in patients undergoing abdominal CT for pathological reasons or routine check-up could provide additional valuable medical information for intervening before other complications arise, thus promoting overall health. While this work focuses on the liver region of unenhanced abdominal CT scans and cardiometabolic disorders, the general framework can be readily adapted for other diseases and regions of interest segmented from various imaging modalities.
Data availability
Code to reproduce the models described in this paper can be found at www.github.com/leibyj/MetabolicDisorders-IDP . The datasets analyzed during the current study are available from the corresponding author upon reasonable request.
Abbreviations
Three-dimensional
Alanine transaminase
Aspartate transaminase
Area under the receiver operating curve
Area under the precision-recall curve
- Computed tomography
Digital Imaging and Communications in Medicine
Gamma-glutamyl transferase
High-density lipoprotein
Image-derived phenotype
- Metabolic syndrome
Neuroimaging Informatics Technology Initiative
triglycerides
Visceral adipose tissue
Ozougwu O. The pathogenesis and pathophysiology of type 1 and type 2 diabetes mellitus. J Physiol Pathophysiology. 9 2013;4:46–57.
Guariguata L, Whiting DR, Hambleton I, Beagley J, Linnenkamp U, Shaw JE. Global estimates of diabetes prevalence for 2013 and projections for 2035. Diabetes Res Clin Pract. 2 2014;103:137–49.
Levenson JW, Skerrett PJ, Gaziano JM. Reducing the Global Burden of Cardiovascular Disease: the role of risk factors. Prev Cardiol. 10 2002;5:188–99.
Mensah GA, Roth GA, Fuster V. The Global Burden of Cardiovascular diseases and Risk factors. J Am Coll Cardiol. 11 2019;74:2529–32.
Wilson PWF, D’Agostino RB, Parise H, Sullivan L, Meigs JB. Metabolic Syndrome as a Precursor of Cardiovascular Disease and Type 2 Diabetes Mellitus, Circulation, vol. 112, pp. 3066–3072, 11 2005.
Ford ES, Li C, Sattar N. Metabolic syndrome and Incident Diabetes. Diabetes Care. 9 2008;31:1898–904.
Shin J-A, Lee J-H, Lim S-Y, Ha H-S, Kwon H-S, Park Y-M, Lee W-C, Kang M-I, Yim H-W, Yoon K-H, Son H-Y. Metabolic syndrome as a predictor of type 2 diabetes, and its clinical interpretations and usefulness. J Diabetes Invest. 5 2013;4:334–43.
Isomaa B, Almgren P, Tuomi T, Forse{\‘}n B, Lahti K, Nisse{\‘}n M, Taskinen M-R, Groop L. Cardiovascular Morbidity and Mortality Associated with the metabolic syndrome. Diabetes Care. 4 2001;24:683–9.
Hirode G, Wong RJ. Trends in the Prevalence of Metabolic Syndrome in the United States, 2011–2016, JAMA, vol. 323, p. 2526, 6 2020.
Kassi E, Pervanidou P, Kaltsas G, Chrousos G. Metabolic syndrome: definitions and controversies. BMC Med. 2011;9:5.
Article Google Scholar
Yamaoka K, Tango T. Effects of lifestyle modification on metabolic syndrome: a systematic review and meta-analysis. BMC Med. 2012;10:11.
Wijesuriya M, Fountoulakis N, Guess N, Banneheka S, Vasantharajah L, Gulliford M, Viberti G, Gnudi L, Karalliedde J. A pragmatic lifestyle modification programme reduces the incidence of predictors of cardio-metabolic disease and dysglycaemia in a young healthy urban south Asian population: a randomised controlled trial. BMC Med. 2017;15:8.
Han TS, Lean MEJ. A clinical perspective of obesity, metabolic syndrome and cardiovascular disease. JRSM Cardiovasc Disease. 2016;5:1.
Sintini I, Graff-Radford J, Senjem ML, Schwarz CG, Machulda MM, Martin PR, Jones DT, Boeve BF, Knopman DS, Kantarci K, Petersen RC, Jack CR, Lowe VJ, Josephs KA, Whitwell JL. Longitudinal neuroimaging biomarkers differ across Alzheimer’s disease phenotypes, Brain, vol. 143, pp. 2281–2294, 6 2020.
Zhou H, He L, Zhang Y, Shen L, Chen B. Interpretable Graph Convolutional Network Of Multi-Modality Brain Imaging For Alzheimer’s Disease Diagnosis, in 2022 IEEE 19th International Symposium on Biomedical Imaging (ISBI), 2022.
Kim M, Kim J, Qu J, Huang H, Long Q, Sohn K-A, Kim D, Shen L. Interpretable temporal graph neural network for prognostic prediction of Alzheimer’s disease using longitudinal neuroimaging data, in 2021 IEEE International Conference on Bioinformatics and Biomedicine (BIBM), 2021.
Basty N, Sorokin EP, Thanaj M, Whitcher B, Liu Y, Bell JD, Thomas EL, Cule M. Cardiovascular measures from abdominal MRI provide insights into abdominal vessel genetic architecture, medRxiv, 8 2022.
Pickhardt PJ, Graffy PM, Zea R, Lee SJ, Liu J, Sandfort V, Summers RM. Utilizing fully automated abdominal CT–Based biomarkers for opportunistic screening for metabolic syndrome in adults without symptoms. Am J Roentgenol. 1 2021;216:85–92.
Yao MS, Chae A, MacLean MT, Verma A, Duda J, Gee J, Torigian DA, Rader D, Kahn C, Witschey WR, Sagreiya H. SynthA1c: Towards Clinically Interpretable Patient Representations for Diabetes Risk Stratification, Predictive Intelligence in Medicine. PRIME 2023 Lecture Notes in Computer Science, vol 14277, 2022.
Pickhardt PJ, Graffy PM, Zea R, Lee SJ, Liu J, Sandfort V, Summers RM. Automated CT biomarkers for opportunistic prediction of future cardiovascular events and mortality in an asymptomatic screening population: a retrospective cohort study. Lancet Digit Health. 2020;2:e192–e.
Article PubMed PubMed Central Google Scholar
Gong W, Beckmann CF, Smith SM. Phenotype discovery from population brain imaging. Med Image Anal. 2021;71:7.
Cha KH, Hadjiiski L, Chan H-P, Weizer AZ, Alva A, Cohan RH, Caoili EM, Paramagul C, Samala RK. Bladder Cancer Treatment Response Assessment in CT using Radiomics with Deep-Learning. Sci Rep. 2017;7:8.
Lao J, Chen Y, Li Z-C, Li Q, Zhang J, Liu J, Zhai G. A deep learning-based Radiomics Model for Prediction of Survival in Glioblastoma Multiforme. Sci Rep. 2017;7:9.
Liang J, Zhang W, Yang J, Wu M, Dai Q, Yin H, Xiao Y, Kong L. Deep learning supported discovery of biomarkers for clinical prognosis of liver cancer. Nat Mach Intell. 4 2023;5:408–20.
Afshar P, Mohammadi A, Plataniotis KN, Oikonomou A, Benali H. From handcrafted to Deep-Learning-Based Cancer Radiomics: challenges and opportunities. IEEE Signal Process Mag. 7 2019;36:132–60.
Pickhardt PJ, Graffy PM, Reeder SB, Hernando D, Li K. Quantification of Liver Fat Content with Unenhanced MDCT: Phantom and clinical correlation with MRI Proton Density Fat Fraction. Am J Roentgenol. 2018;211:9.
Pickhardt PJ, Jee Y, O’Connor SD, del Rio AM. Visceral adiposity and Hepatic Steatosis at Abdominal CT: Association with the metabolic syndrome. Am J Roentgenol. 5 2012;198:1100–7.
Guo Z, Blake GM, Li K, Liang W, Zhang W, Zhang Y, Xu L, Wang L, Brown JK, Cheng X, Pickhardt PJ. Liver Fat Content Measurement with Quantitative CT Validated against MRI Proton Density Fat Fraction: A Prospective Study of 400 Healthy Volunteers, Radiology, vol. 294, pp. 89–97, 1 2020.
Lee C, Choe EK, Choi JM, Hwang Y, Lee Y, Park B, Chung SJ, Kwak M-S, Lee J-E, Kim JS, Park SK, Cho S-H. Health and Prevention Enhancement (H-PEACE): a retrospective, population-based cohort study conducted at the Seoul National University Hospital Gangnam Center, Korea, BMJ Open, vol. 8, p. e019327, 4 2018.
Choe EK, Park KJ, Chung SJ, Moon SH, Ryoo S-B, Oh H-K. Colonoscopic Surveillance after Colorectal Cancer Resection: who needs more intensive Follow-Up? Digestion. 2015;91:142–9.
Article PubMed Google Scholar
Grundy SM, Brewer HB, Cleeman JI, Smith SC, Lenfant C. Definition Metabolic Syndrome Circulation. 1 2004;109:433–8.
Chung SJ, Kim D, Park MJ, Kim YS, Kim JS, Jung HC, Song IS. Metabolic syndrome and visceral obesity as risk factors for reflux oesophagitis: a cross-sectional case-control study of 7078 Koreans undergoing health check-ups, Gut, vol. 57, pp. 1360–1365, 4 2008.
Hamaguchi M, Kojima T, Itoh Y, Harano Y, Fujii K, Nakajima T, Kato T, Takeda N, Okuda J, Ida K, Kawahito Y, Yoshikawa T, Okanoue T. The Severity of Ultrasonographic Findings in Nonalcoholic Fatty Liver Disease Reflects the Metabolic Syndrome and Visceral Fat Accumulation, The American Journal of Gastroenterology, vol. 102, pp. 2708–2715, 12 2007.
Isensee F, Jaeger PF, Kohl SAA, Petersen J, Maier-Hein KH. nnU-Net: a self-configuring method for deep learning-based biomedical image segmentation. Nat Methods. 12 2020;18:203–11.
Choe EK. Association of visceral obesity and early colorectal neoplasia. World J Gastroenterol. 2013;19:8349.
Article CAS PubMed PubMed Central Google Scholar
Leiby JS, Lee ME, Choe EK, Kim D. Data-Driven classification of fatty liver from 3D unenhanced abdominal CT scans. Mach Learn Med Imaging Lecture Notes Comput Sci, 2023, p. 264–72.
Ilse M, Tomczak JM, Welling M. Attention-based Deep Multiple Instance Learning, Proceedings of the 35th International Conference on Machine Learning, in Proceedings of Machine Learning Research, 80:2127–2136, 13 2 2018.
Liu C, Berry PM, Dawson TP, Pearson RG. Selecting thresholds of occurrence in the prediction of species distributions, Ecography, vol. 28, pp. 385–393, 6 2005.
Sheng G, Xie Q, Wang R, Hu C, Zhong M, Zou Y. Waist-to-height ratio and non-alcoholic fatty liver disease in adults. BMC Gastroenterol. 2021;21:5.
Milić S, Lulić D, Štimac D. Non-alcoholic fatty liver disease and obesity: biochemical, metabolic and clinical presentations. World J Gastroenterol. 7 2014;20(28):9330–7.
Zhu W, Shi P, Fu J, Liang A, Zheng T, Wu X, Yuan S. Development and application of a novel model to predict the risk of non-alcoholic fatty liver disease among lean pre-diabetics with normal blood lipid levels. Lipids Health Dis. 2022;21:12.
Oh YH, Choi S, Lee G, Son JS, Kim KH, Park SM. Changes in body composition are Associated with metabolic changes and the risk of metabolic syndrome. J Clin Med MDPI AG. 2021;10:745.
Article CAS Google Scholar
Nielson S, Guo Z, Johnson CM, Hensrud DD, Jensen MD. Splanchnic lipolysis in human obesity. J Clin Invest Am Soc Clin Invest. 2004;113:1582–8.
Lemieux S, Prud’homme D, Bouchard C, Tremblay A, Després J. Sex differences in the relation of visceral adipose tissue accumulation to total body fatness. Am J Clin Nutr Elsevier BV. 1993;58:463–7.
Kim HI, Kim JT, Yu HS, Kwak SH, Jang HC, Park KS, Kim SY, Lee HK, Cho YM. Gender Differences in Diagnostic Values of Visceral Fat Area and Waist Circumference for Predicting Metabolic Syndrome in koreans. J Korean Medicial Sci Korean Acad Med Sci. 2011;26:906.
Sahiner B, Chen W, Samala RK, Petrick N. Data drift in medical machine learning: implications and potential remedies. Br J Radiol, 3 2023.
Download references
The authors have no funding to disclose. The author(s) declare financial support was received for the research, authorship, and/or publication of this article. This work was supported by the National Institutes of Health (U01 AG068057).
Author information
Authors and affiliations.
Department of Biostatistics, Epidemiology, and Informatics, Perelman School of Medicine, University of Pennsylvania, 19104, Philadelphia, PA, USA
Jacob S. Leiby, Matthew E. Lee, Manu Shivakumar & Dokyoon Kim
Department of Surgery, Seoul National University Hospital Healthcare System Gangnam Center, 06236, Seoul, South Korea
Eun Kyung Choe
Institute for Biomedical Informatics, Perelman School of Medicine, University of Pennsylvania, 19104, Philadelphia, PA, USA
Dokyoon Kim
You can also search for this author in PubMed Google Scholar
Contributions
JSL: conceptualization, methodology, manuscript preparation and review; MEL: methodology, manuscript review; MS: methodology, manuscript review; EKC: conceptualization, methodology, supervision, data curation, manuscript preparation and review; DK: conceptualization, methodology, supervision, manuscript review.
Corresponding authors
Correspondence to Eun Kyung Choe or Dokyoon Kim .
Ethics declarations
The Institutional Review Board of Seoul National University Hospital approved the study protocol (H-1902-017-1008), and the study was conducted in accord with the Helsinki Declaration. Informed consent was waived by the board.
Competing interests
The authors declare that they have no competing interests.
Additional information
Publisher’s note.
Springer Nature remains neutral with regard to jurisdictional claims in published maps and institutional affiliations.
Electronic supplementary material
Below is the link to the electronic supplementary material.
Supplementary Material 1
Rights and permissions.
Open Access This article is licensed under a Creative Commons Attribution 4.0 International License, which permits use, sharing, adaptation, distribution and reproduction in any medium or format, as long as you give appropriate credit to the original author(s) and the source, provide a link to the Creative Commons licence, and indicate if changes were made. The images or other third party material in this article are included in the article’s Creative Commons licence, unless indicated otherwise in a credit line to the material. If material is not included in the article’s Creative Commons licence and your intended use is not permitted by statutory regulation or exceeds the permitted use, you will need to obtain permission directly from the copyright holder. To view a copy of this licence, visit http://creativecommons.org/licenses/by/4.0/ . The Creative Commons Public Domain Dedication waiver ( http://creativecommons.org/publicdomain/zero/1.0/ ) applies to the data made available in this article, unless otherwise stated in a credit line to the data.
Reprints and permissions
About this article
Cite this article.
Leiby, J.S., Lee, M.E., Shivakumar, M. et al. Deep learning imaging phenotype can classify metabolic syndrome and is predictive of cardiometabolic disorders. J Transl Med 22 , 434 (2024). https://doi.org/10.1186/s12967-024-05163-1
Download citation
Received : 08 January 2024
Accepted : 04 April 2024
Published : 08 May 2024
DOI : https://doi.org/10.1186/s12967-024-05163-1
Share this article
Anyone you share the following link with will be able to read this content:
Sorry, a shareable link is not currently available for this article.
Provided by the Springer Nature SharedIt content-sharing initiative
- Deep learning
- Machine learning
Journal of Translational Medicine
ISSN: 1479-5876
- Submission enquiries: Access here and click Contact Us
- General enquiries: [email protected]
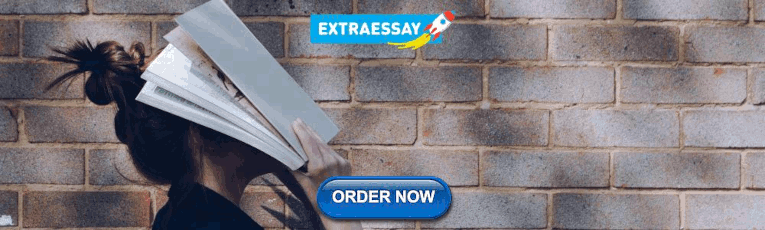
IMAGES
VIDEO
COMMENTS
Translational research. Translational research (also called translation research, translational science, or, when the context is clear, simply translation) [1] [2] is research aimed at translating (converting) results in basic research into results that directly benefit humans. The term is used in science and technology, especially in biology ...
Translational research is a systematic effort to convert basic research knowledge into practical applications to enhance human health and well being. Learn how Cornell Cooperative Extension engages in translational research and how extension programs can participate in it.
Defining Translational Research. TVST was established to provide a venue for multidisciplinary research that bridges the gap between basic research and clinical care. Although the journal's focus may have been unique in the vision science community at the time of its inception, the concept of translational research was not.
Translational research is the process of turning basic science discoveries into health interventions that improve human health. Learn about the different stages of translational research, the role of multidisciplinary collaboration and community engagement, and the resources provided by UAMS Translational Research Institute.
According to this definition, translational research is part of a unidirectional continuum in which research findings are moved from the researcher's bench to the patient's bedside and community. In the continuum, the first stage of translational research (T1) transfers knowledge from basic research to clinical research, while the second ...
Translational research is a rapidly evolving area of biomedical research that aims to facilitate and speed up the transfer of scientific discoveries into clinical practice. It has emerged as a scientific discipline rather recently, in order to fill the gap between clinical and basic research area.
Instead, all articles published in Pediatric Research represent translational research and categorized based on methodology: basic translational research, clinical translational research, or ...
Dische and Saunders [ 15] mention the definition given by Julie Denekamp: Translational Research is the application of scientific method to address a health need. Barry S. Coller has very simply defined [ 16] it as: Successful implementation of a laboratory concept into a clinical protocol.
Translational science is the field that generates innovations that overcome longstanding challenges along the translational research pipeline. These include scientific, operational, financial and administrative innovations that transform the way that research is done, making it faster, more efficient, and more impactful.
Translational science is the field that generates scientific and operational innovations that overcome longstanding challenges along the translational research pipeline. These include scientific, operational, financial and administrative innovations that transform the way that research is done, making it faster, more efficient, and more impactful.
Much relies on the definition of what is a translational researcher. Lee Nadler is senior vice president for Experimental Medicine at the Dana Farber Cancer Institute in Boston, and is charged ...
Translational research is medical research that applies scientific discoveries to prevent, diagnose, and treat disease. Learn the word history, usage, and synonyms of translational research from Merriam-Webster dictionary.
Translational Research. It's neither practical nor safe to transition directly from studying individual cells to testing on patients. Translational research provides that crucial pivot point. It bridges the gap between basic and clinical research by bringing together a number of specialists to refine and advance the application of a discovery ...
Translational research focuses more on the achievement of improved health outcomes of a particular scientific discovery. Translational research bridges the gap between basic research and clinical research. Clinical research, therefore, is a critical step in the translational process, as this stage determines if new medical solutions are both ...
Translational research is a medium wherein nuances of basic research can evolve to address gaps of knowledge in the clinical setting. There is minimal evidence in surgical literature to suggest a rubric within which TR can be conducted, which is the primary goal of this body of work.
Translational research definitions courtesy of ... The definition includes pharmacologic, non-pharmacologic, and behavioral interventions given for disease prevention, prophylaxis, diagnosis, or therapy. Community trials and other population-based intervention trials also are included.
Discussion. Although there is little agreement between definitions, we were able to identify an emerging consensus 5-phase (T0-T4) definition for translational research. T1 involves processes that bring ideas from basic research through early testing in humans. T2 involves the establishment of effectiveness in humans and clinical guidelines.
Translational research means different things to different people, but it seems important to almost everyone. The National Institutes of Health (NIH) has made translational research a priority, forming centers of translational research at its institutes and launching the Clinical and Translational Science Award (CTSA) program in 2006.
Translational research work that can ensure that research results are used, integrated into practice, and can produce benefits for patients and society is thus also characterized and performed as ...
Translational research activities are intended to bring knowledge from the lab into practice, and ideally, allow practice to influence what occurs in the laboratory. It is defined by NIH as "the process of applying ideas, insights, and discoveries generated through basic scientific inquiry to the treatment or prevention of human disease.".
The translational science spectrum shows the stages of translational research. The stages do not happen in a straight line or in one direction. Each stage builds upon and informs the others. At all stages, our staff and grantees come up with new approaches, prove their usefulness and share the findings. Patient involvement is a key feature of ...
Translational Research delivers original investigations in the broad fields of laboratory, clinical, and public health research. Published monthly since 1915, it keeps readers up-to-date on significant biomedical research from all subspecialties of medicine. Interdisciplinary and cross-disciplinary in scope, it aims to expedite the translation ...
Gene therapy aims to modify or manipulate gene expression or to alter the biological properties of living cells for therapeutic use, an innovative medical approach that currently sits at an inflection point (1, 2).After the first gene therapies developed in the 1990s, research and development (R&D) remained stagnant until the mid-2010s when the convergence of next-generation technologies ...
Clinical and translational research (CTR) contains four phases (T1-T4), involving the translation of basic research to humans, then to clinical settings, practice, and the population, with the ultimate goal of improving public health. ... One definition of CTR is moving research from bench to bedside to communities and back again. This ...
So with excitement and trepidation, we will now consider how best to balance methodologic advances and semantic and interpretive flexibility in the reporting of research with the principles in our long-standing and often discussed reporting policy that generally limits use of causal language to well-done randomized clinical trials. 3 We ...
Headquartered on the growing Buffalo Niagara Medical Campus and one of only a handful of such buildings in the nation, the state-of-the-art Clinical and Translational Research Center provides a centralized facility for the Clinical and Translational Science Institute and places clinicians adjacent to clinical and translational researchers.
Clinical assessment and definitions. All participants entered the test after at least ten hours of fasting. For the definition of metabolic syndrome, we used the criteria suggested by the American Heart Association [].In the population for model development, metabolic syndrome was defined when at least three of the following metabolic risk factors were positive: increased waist circumference ...