Case study on adoption of new technology for innovation: Perspective of institutional and corporate entrepreneurship
Asia Pacific Journal of Innovation and Entrepreneurship
ISSN : 2398-7812
Article publication date: 7 August 2017
This paper aims at investigating the role of institutional entrepreneurship and corporate entrepreneurship to cope with firm’ impasses by adoption of the new technology ahead of other firms. Also, this paper elucidates the importance of own specific institutional and corporate entrepreneurship created from firm’s norm.
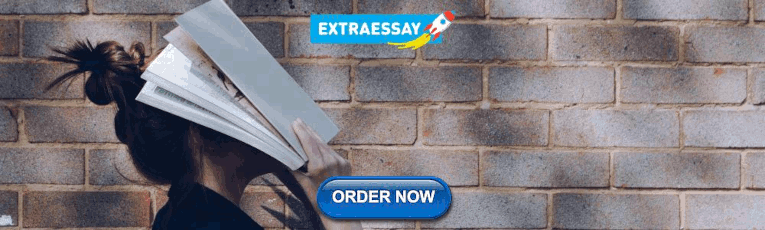
Design/methodology/approach
The utilized research frame is as follows: first, perspective of studies on institutional and corporate entrepreneurship are performed using prior literature and preliminary references; second, analytical research frame was proposed; finally, phase-based cases are conducted so as to identify research objective.
Kumho Tire was the first tire manufacturer in the world to exploit the utilization of radio-frequency identification for passenger carâ’s tire. Kumho Tire takes great satisfaction in lots of failures to develop the cutting edge technology using advanced information and communication technology cultivated by heterogeneous institution and corporate entrepreneurship.
Originality/value
The firm concentrated its resources into building the organization’s communication process and enhancing the quality of its human resources from the early stages of their birth so as to create distinguishable corporate entrepreneurship.
- Corporate entrepreneurship
- Institutional entrepreneurship
Han, J. and Park, C.-m. (2017), "Case study on adoption of new technology for innovation: Perspective of institutional and corporate entrepreneurship", Asia Pacific Journal of Innovation and Entrepreneurship , Vol. 11 No. 2, pp. 144-158. https://doi.org/10.1108/APJIE-08-2017-031
Emerald Publishing Limited
Copyright © 2017, Junghee Han and Chang-min Park.
Published in the Asia Pacific Journal of Innovation and Entrepreneurship . Published by Emerald Publishing Limited. This article is published under the Creative Commons Attribution (CC BY 4.0) licence. Anyone may reproduce, distribute, translate and create derivative works of this article (for both commercial & non-commercial purposes), subject to full attribution to the original publication and authors. The full terms of this licence may be seen at http://creativecommons.org/licenses/by/4.0/legalcode
1. Introduction
Without the entrepreneur, invention and new knowledge possibly have lain dormant in the memory of persons or in the pages of literature. There is a Korean saying, “Even if the beads are too much, they become treasure after sewn”. This implies importance of entrepreneurship. In general, innovativeness and risk-taking are associated with entrepreneurial activity and, more importantly, are considered to be important attributes that impact the implementation of new knowledge pursuing.
Implementation of cutting edge technology ahead of other firms is an important mechanism for firms to achieve competitive advantage ( Capon et al. , 1990 ; D’Aveni, 1994 ). Certainly, new product innovation continues to play a vital role in competitive business environment and is considered to be a key driver of firm performance, especially as a significant form of corporate entrepreneurship ( Srivastava and Lee, 2005 ). Corporate entrepreneurship is critical success factor for a firm’s survival, profitability and growth ( Phan et al. , 2009 ).
The first-mover has identified innovativeness and risk-taking as important attributes of first movers. Lumpkin and Dess (1996) argued that proactiveness is a key entrepreneurial characteristic related to new technology adoption and product. This study aims to investigate the importance of corporate and institutional entrepreneurship through analyzing the K Tire’s first adaptation of Radio-frequency identification (RFID) among the world tire manufactures. Also, this paper can contribute to start ups’ readiness for cultivating of corporate and institutional entrepreneurship from initial stage to grow and survive.
K Tire is the Korean company that, for the first time in the world, applied RFID to manufacturing passenger vehicle tires in 2013. Through such efforts, the company has built an innovation model that utilizes ICTs. The adoption of the technology distinguishes K Tire from other competitors, which usually rely on bar codes. None of the global tire manufacturers have applied the RFID technology to passenger vehicle tires. K Tire’s decision to apply RFID to passenger vehicle tires for the first time in the global tire industry, despite the uncertainties associated with the adoption of innovative technologies, is being lauded as a successful case of innovation. In the global tire market, K Tire belongs to the second tier, rather than the leader group consisting of manufacturers with large market shares. Then, what led K Tire to apply RFID technology to the innovation of its manufacturing process? A company that adopts innovative technologies ahead of others, even if the company is a latecomer, demonstrates its distinguishing characteristics in terms of innovation. As such, this study was motivated by the following questions. With regard to the factors that facilitate innovation, first, what kind of the corporate and institutional situations that make a company more pursue innovation? Second, what are the technological situations? Third, how do the environmental situations affect innovation? A case study offers the benefit of a closer insight into the entrepreneurship frame of a specific company. This study has its frame work rooted in corporate entrepreneurship ( Guth and Ginsberg, 1990 ; Shane and Venkataraman, 2000 ) and institutional entrepreneurship ( Battilana, 2006 ; Fligstein, 1997 ; Rojas, 2010 ). As mentioned, we utilized qualitative research method ( Yin, 2008 ). This paper is structured as follows. Section two presents the literature review, and section three present the methodology and a research case. Four and five presents discussion and conclusions and implications, respectively.
2. Theoretical review and analysis model
RFID technology is to be considered as not high technology; however, it is an entirely cutting edged skills when combined with automotive tire manufacturing. To examine why and how the firm behaves like the first movers, taking incomparable high risks to achieve aims unlike others, we review three kinds of prior literature. As firms move from stage to stage, they have to revamp innovative capabilities to survive and ceaseless stimulate growth.
2.1 Nature of corporate entrepreneurship
Before reviewing the corporate entrepreneurship, it is needed to understand what entrepreneurship is. To more understand the role that entrepreneurship plays in modern economy, one need refer to insights given by Schumpeter (1942) or Kirzner (1997) . Schumpeter suggests that entrepreneurship is an engine of economic growth by utilization of new technologies. He also insists potential for serving to discipline firms in their struggle to survive gale of creative destruction. While Schumper argued principle of entrepreneurship, Kirzner explains the importance of opportunities. The disruptions generated by creative destruction are exploited by individuals who are alert enough to exploit the opportunities that arise ( Kirzner, 1997 ; Shane and Venkataraman, 2000 ).
Commonly all these perspectives on entrepreneurship is an appreciation that the emergence of novelty is not an easy or predictable process. Based on literature review, we note that entrepreneurship is heterogeneous interests and seek “something new” associated with novel outcomes. Considering the literature review, we can observe that entrepreneurship is the belief in individual autonomy and discretion, and a mindset that locates agency in individuals for creating new activities ( Meyer et al. ,1994 ; Jepperson and Meyer, 2001 ).
the firm’s commitment to innovation (including creation and introduction of products, emphasis on R&D investments and commitment to patenting);
the firm’s venturing activities, such as entry into new business fields by sponsoring new ventures and creating new businesses; and
strategic renewal efforts aimed at revitalizing the firm’s ability to compete.
developing innovation an organizational tool;
allowing the employees to propose ideas; and
encouraging and nurturing the new knowledge ( Hisrich, 1986 ; Kuratko, 2007 ).
Consistent with the above stream of research, our paper focuses on a firm’s new adaptation of RFID as a significant form of corporate entrepreneurial activity. Thus, CE refers to the activities a firm undertakes to stimulate innovation and encourage calculated risk taking throughout its operations. Considering prior literature reviews, we propose that corporate entrepreneurship is the process by which individuals inside the organization pursuing opportunities without regards to the resources they control.
If a firm has corporate entrepreneurship, innovation (i.e. transformation of the existing firm, the birth of new business organization and innovation) happens. In sum, corporate entrepreneurship plays a role to pursue to be a first mover from a latecomer by encompassing the three phenomena.
2.2 Institution and institutional entrepreneurship
Most literature regarding entrepreneurship deals with the attribute of individual behavior. More recently, scholars have attended to the wider ecosystem that serves to reinforce risk-taking behavior. Institution and institutional entrepreneurship is one way to look at ecosystem that how individuals and groups attempt to try to become entrepreneurial activities and innovation.
Each organization has original norm and intangible rules. According to the suggestion by Scott (1995) , institutions constrain behavior as a result of processes associated with institutional pillars. The question how actors within the organizations become motivated and enabled to transform the taken-for-granted structures has attracted substantial attention for institutionalist. To understand why some firms are more likely to seek innovation activities despite numerous difficulties and obstacles, we should take look at the institutional entrepreneurship.
the regulative, which induces worker’s action through coercion and formal sanction;
the normative, which induces worker’s action through norms of acceptability and ethics; and
the cognitive, which induces worker’s action through categories and frames by which actors know and interpret their world.
North (1990) defines institutions as the humanly devised constraints that structure human action. Actors within some organization with sufficient resources have intend to look at them an opportunity to realize interests that they value highly ( DiMaggio, 1988 ).
It opened institutional arguments to ideas from the co-evolving entrepreneurship literature ( Aldrich and Fiol, 1994 ; Aldrich and Martinez, 2001 ). The core argument of the institutional entrepreneurship is mechanisms enabling force to motivate for actors to act difficult task based on norm, culture and shared value. The innovation, adopting RFID, a technology not verified in terms of its effectiveness for tires, can be influenced by the institution of the society.
A firm is the organizations. An organization is situated within an institution that has social and economic norms. Opportunity is important for entrepreneurship. The concept of institutional entrepreneurship refer to the activities of worker or actor who have new opportunity to realize interest that they values highly ( DiMaggio, 1988 ). DiMaggio (1988) argues that opportunity for institutional entrepreneurship will be “seen” and “exploited” by within workers and not others depending on their resources and interests respectively.
Despite that ambiguity for success was given, opportunity and motivation for entrepreneurs to act strategically, shape emerging institutional arrangements or standards to their interests ( Fligstein and Mara-Drita, 1996 ; Garud et al. , 2002 ; Hargadon and Douglas, 2001 ; Maguire et al. , 2004 ).
Resource related to opportunity within institutional entrepreneurship include formal or informal authority and power ( Battilana, 2006 ; Rojas, 2010 ). Maguire et al. (2004) suggest legitimacy as an important ingredient related to opportunity for institutional entrepreneurship. Some scholars suggest opportunity resources for institutional entrepreneurship as various aspects. For instance, Marquire and Hardy (2009) show that knowledge and expertise is more crucial resources. Social capital, including market leadership and social network, is importance resource related to opportunity ( Garud et al. , 2002 ; Lawrence et al. , 2005 ; Townley, 2002 ). From a sociological perspective, change associated with entrepreneurship implies deviations from some norm ( Garud and Karnøe, 2003 ).
Institutional entrepreneurship is therefore a concept that reintroduces agency, interests and power into institutional analyses of organizations. Based on the previous discussion, this study defines institution as three processes of network activity; coercion and formal sanction, normative and cognitive, to acquire the external knowledge from adopting common goals and rules inside an organization. It would be an interesting approach to look into a specific company to see whether it is proactive towards adopting ICTs (e.g. RFID) and innovation on the basis of such theoretical background.
2.3. Theoretical analysis frame
Companies innovate themselves in response to the challenges of the ever-changing markets and technologies, so as to ensure their survival and growth ( Tushman and Anderson, 1986 ; Tidd and Bessant, 2009 ; Teece, 2014 ). As illustrated above, to achieve the purpose of this study, the researcher provides the following frames of analyses based on the theoretical background discussed above ( Figure 1 ).
3. Case study
3.1 methodology.
It is a highly complicated and tough task to analyze the long process of innovation at a company. In this paper, we used analytical approach rather than the problem-oriented method because the case is examined to find and understand what has happened and why. It is not necessary to identify problems or suggest solutions. Namely, this paper analyzes that “why K Tire becomes a first mover from a late comer through first adoption of RFID technology for automotive tire manufacture with regards to process and production innovations”.
To study the organizational characteristics such as corporate entrepreneurship, institutional entrepreneurship, innovation process of companies, the qualitative case study is the suitable method. This is because a case study is a useful method when verifying or expanding well-known theories or challenging a specific theory ( Yin, 2008 ). This study seeks to state the frame of analysis established, based on previously established theories through a single case. K Tire was selected as the sample because it is the first global tire manufacturer, first mover to achieve innovation by developing and applying RFID.
The data for the case study were collected as follows. First, this study was conducted from April 2015 to the end of December 2015. Additional expanded data also were collected from September 12 to November 22, 2016, to pursue the goal of this paper. Coauthor worked for K Tire for more than 30 year, and currently serves as the CEO of an affiliate company. As such, we had the most hands-on knowledge and directed data in the process of adoption RFID. This makes this case study a form of participant observation ( Yin, 2008 ). To secure data on institutional entrepreneurship, in-depth interviews were conducted with the vice president of K Tire. The required data were secured using e-mail, and the researchers accepted the interviewees’ demand to keep certain sensitive matters confidential. The interviewees agreed to record the interview sessions. In this way, a 20-min interview data were secured for each interviewee. In addition, apart from the internal data of the subject company, other objective data were obtained by investigating various literatures published through the press.
3.2 Company overview
In September 1960, K Tire was established in South Korea as the name of Samyang Tire. In that time, the domestic automobile industry in Korea was at a primitive stage, as were auto motive parts industries like the tire industry. K Tire products 20 tires a day, depending on manual labor because of our backward technology and shortage of facilities.
The growth of K Tire was astonishment. Despite the 1974 oil shock and difficulties in procuring raw materials, K Tire managed to achieve remarkable growth. In 1976, K Tire became the leader in the tire sector and was listed on the Korea Stock Exchange. Songjung plant II was added in 1977. Receiving the grand prize of the Korea Quality Control Award in 1979, K Tire sharpened its corporate image with the public. The turmoil of political instability and feverish democratization in the 1980s worsened the business environment. K Tire also underwent labor-management struggles but succeeded in straightening out one issue after another. In the meantime, the company chalked up a total output of 50 million tires, broke ground for its Koksung plant and completed its proving ground in preparation for a new takeoff.
In the 1990s, K Tire expanded its research capability and founded technical research centers in the USA and the United Kingdom to establish a global R&D network. It also concentrated its capabilities in securing the foundation as a global brand, by building world-class R&D capabilities and production systems. Even in the 2000s, the company maintained its growth as a global company through continued R&D efforts by securing its production and quality capabilities, supplying tires for new models to Mercedes, Benz, Volkswagen and other global auto manufacturers.
3.3 Implementation of radio-frequency identification technology
RFID is radio-frequency identification technology to recognize stored information by using a magnetic carrier wave. RFID tags can be either passive, active or battery-assisted passive (BAP). An active tag has an on-board battery and periodically transmits its ID signal. A BAP has a small battery on board and is activated when in the presence of an RFID reader. A passive tag is cheaper and smaller because it has no battery; instead, the tag uses the radio energy transmitted by the reader. However, to operate a passive tag, it must be illuminated with a power level roughly a thousand times stronger than for signal transmission. That makes a difference in interference and in exposure to radiation.
an integrated circuit for storing and processing information, modulating and demodulating a radio frequency signal, collecting DC power from the incident reader signal, and other specialized functions; and
an antenna for receiving and transmitting the signal.
capable of recognizing information without contact;
capable of recognizing information regardless of the direction;
capable of reading and saving a large amount of data;
requires less time to recognize information;
can be designed or manufactured in accordance with the system or environmental requirements;
capable of recognizing data unaffected by contamination or the environment;
not easily damaged and cheaper to maintain, compared with the bar code system; and
tags are reusable.
3.3.1 Phase 1. Background of exploitation of radio-frequency identification (2005-2010).
Despite rapid growth of K Tire since 1960, K Tire ranked at the 13th place in the global market (around 2 per cent of the global market share) as of 2012. To enlarge global market share is desperate homework. K Tire was indispensable to develop the discriminated technologies. When bar code system commonly used by the competitors, and the industry leaders, K Tire had a decision for adoption of RFID technology instead of bar code system for tires as a first mover strategy instead of a late comer with regard to manufacture tires for personal vehicle. In fact, K Tire met two kinds of hardship. Among the top 20, the second-tier companies with market shares of 1-2 per cent are immersed in fiercer competitions to advance their ranks. The fierceness of the competition is reflected in the fact that of the companies ranked between the 11th and 20th place, only two maintained their rank from 2013.
With the demand for stricter product quality control and manufacture history tracking expanding among the auto manufacturers, tire manufacturers have come to face the need to change their way of production and logistics management. Furthermore, a tire manufacturer cannot survive if it does not properly respond to the ever stricter and exacting demand for safe passenger vehicle tires of higher quality from customers and auto manufacturers. As mentioned above, K Tire became one of the top 10 companies in the global markets, recording fast growth until the early 2000. During this period, K Tire drew the attention of the global markets with a series of new technologies and innovative technologies through active R&D efforts. Of those new products, innovative products – such as ultra-high-performance tires – led the global markets and spurred the company’s growth. However, into the 2010s, the propriety of the UHP tire technology was gradually lost, and the effect of the innovation grew weaker as the global leading companies stepped forward to take the reign in the markets. Subsequently, K Tire suffered from difficulties across its businesses, owing to the failure to develop follow-up innovative products or market-leading products, as well as the aggressive activities by the company’s hardline labor union. Such difficulties pushed K Tire down to the 13th position in 2014, which sparked the dire need to bring about innovative changes within the company.
3.3.2 Phase 2. Ceaseless endeavor and its failure (2011-2012).
It needs to be lightweight : An RFID tag attached inside a vehicle may adversely affect the weight balance of the tires. A heavier tag has greater adverse impact on the tire performance. Therefore, a tag needs to be as light as possible.
It needs to be durable : Passenger vehicle tires are exposed to extensive bending and stretching, as well as high levels of momentum, which may damage a tag, particularly causing damage to or even loss of the antenna section.
It needs to maintain adhesiveness : Tags are attached on the inner surface, which increase the possibility of the tags falling off from the surface while the vehicle is in motion.
It needs to be resistant to high temperature and high pressure : While going through the tire manufacture process, a tag is exposed to a high temperature of around 200°C and high pressure of around 30 bars. Therefore, a tag should maintain its physical integrity and function at such high pressure and temperature.
It needs to be less costly : A passenger vehicle tire is smaller, and therefore cheaper than truck/bus tires. As a result, an RFID tag places are greater burden on the production cost.
Uncountable tag prototypes, were applied to around 200 test tires in South Korea for actual driving tests. Around 150 prototypes were sent to extremely hot regions overseas for actual driving tests. However, the driving tests revealed damage to the antenna sections of the tags embedded in tires, as the tires reached the end of their wear life. Also, there was separation of the embedded tags from the rubber layers. This confirmed the risk of tire separation, resulting in the failure of the tag development attempt.
3.3.3 Phase 3. Success of adoption RFID (2013-2014).
Despite the numerous difficulties and failures in the course of development, the company ultimately emerged successful, owing to its institutional entrepreneurship and corporate entrepreneurship the government’s support. Owing to the government-led support project, K Tire resumed its RFID development efforts in 2011. This time, the company discarded the idea of the embedded-type tag, which was attempted during the first development. Instead, the company turned to attached-type tag. The initial stages were marked with numerous failures: the size of a tag was large at 20 × 70 mm, which had adverse impact on the rotation balance of the tires, and the attached area was too large, causing the attached sections to fall off as the tire stretched and bent. That was when all personnel from the technical, manufacturing, and logistics department participated in creating ideas to resolve the tag size and adhesiveness issues. Through cooperation across the different departments and repeated tests, K Tire successfully developed its RFID tag by coming up with new methods to minimize the tag size to its current size (9 × 45 mm), maintain adhesiveness and lower the tag price. Finally, K Tire was success the adoption RFID.
3.3.4 Phase 4. Establishment of the manufacture, logistics and marketing tracking system.
Whenever subtle and problematic innovation difficulties arise, every worker and board member moves forward through networking and knowledge sharing within intra and external.
While a bar code is only capable of storing the information on the nationality, manufacturer and category of a product, an RFID tag is capable of storing a far wider scope of information: nationality, manufacturer, category, manufacturing date, machines used, lot number, size, color, quantity, date and place of delivery and recipient. In addition, while the data stored in a bar code cannot be revised or expanded once the code is generated, an RFID tag allows for revisions, additions and removal of data. As for the recognition capability, a bar code recognizes 95per cent of the data at the maximum temperature of 70°C. An RFID tag, on the other hand, recognizes 99.9 per cent of the data at 120°C.
The manufacture and transportation information during the semi-finished product process before the shaping process is stored in the RFID tags, which is attached to the delivery equipment to be provided to the MLMTS;
Logistics Products released from the manufacture process are stored in the warehouses, to be released and transported again to logistics centers inside and outside of South Korea. The RFID tags record the warehousing information, as the products are stored into the warehouses, as well as the release information as the products are released. The information is instantly delivered to the MLMTS;
As a marketing, the RFID tags record the warehousing information of the products supplied and received by sales branches from the logistics centers, as well as the sales information of the products sold to consumers. The information is instantly delivered to the MLMTS; and
As a role of integrative Server, MLM Integrative Server manages the overall information transmitted from the infrastructures for each section (production information, inventory status and release information, product position and inventory information, consumer sales information, etc.).
The MLMTS provides the company with various systemic functions to integrate and manage such information: foolproof against manufacture process errors, manufacture history and quality tracking for each individual product, warehousing/releasing and inventory status control for each process, product position control between processes, real-time warehouse monitoring, release control and history information tracking across products of different sizes, as well as link/control of sales and customer information. To consumers, the system provides convenience services by providing production and quality information of the products, provision of the product history through full tracking in the case of a claim, as well as a tire pressure monitoring system:
“South korea’s K Tire Co. Inc. has begun applying radio-frequency identification (RFID) system tags on: half-finished” tire since June 16. We are now using an IoT based production and distribution integrated management system to apply RFID system on our “half-finished products” the tire maker said, claiming this is a world-first in the industry. The technology will enable K Tire to manage products more efficiently than its competitors, according to the company. RFID allows access to information about a product’s location, storage and release history, as well as its inventory management (London, 22, 2015 Tire Business).
4. Discussions
Originally, aims of RFID adoption for passenger car “half-finished product” is to chase the front runners, Hankook Tire in Korea including global leading companies like Bridgestone, Michaelin and Goodyear. In particular, Hankook Tire, established in 1941 has dominated domestic passenger tire market by using the first mover’s advantage. As a late comer, K Tire needs distinguishable innovation strategy which is RFID adoption for passenger car’s tire, “half-finished product” to overcome shortage of number of distribution channels. Adoption of RFID technology for passenger car’s tire has been known as infeasible methodologies according to explanation by Changmin Park, vice-CTO (chief technology officer) until K Tire’s success.
We lensed success factors as three perspectives; institutional entrepreneurship, corporate entrepreneurship and innovation. First, as a corporate entrepreneurship perspective, adopting innovative technologies having uncertainties accompanies by a certain risk of failure. Corporate entrepreneurship refers to firm’s effort that inculcate and promote innovation and risk taking throughout its operations ( Burgelman, 1983 ; Guth and Ginsberg, 1990 ). K Tire’s success was made possible by overcome the uncountable difficulties based on shared value and norms (e.g. Fligstein and Mara-Drita, 1996 ; Garud et al. , 2002 ; Hargadon and Douglas, 2001 ; Maguire et al. , 2004 ).
An unsuccessful attempt at developing innovative technologies causes direct loss, as well as loss of the opportunity costs. This is why many companies try to avoid risks by adopting or following the leading companies’ technologies or the dominant technologies. Stimulating corporate entrepreneurship requires firms to acquire and use new knowledge to exploit emerging opportunities. This knowledge could be obtained by joining alliances, selectively hiring key personnel, changing the composition or decision-making processes of a company’s board of directors or investing in R&D activities. When the firm uses multiple sources of knowledge ( Branzei and Vertinsky, 2006 ; Thornhill, 2006 ), some of these sources may complement one another, while others may substitute each other ( Zahra and George, 2002 ). Boards also provide managers with appropriate incentives that better align their interests with those of the firm. Given the findings, K Tire seeks new knowledge from external organizations through its discriminative corporate entrepreneurship.
When adopting the RFID system for its passenger vehicle tires, K Tire also had to develop new RFID tags suitable for the specific type of tire. The company’s capabilities were limited by the surrounding conditions, which prevented the application of existing tire RFID tag technologies, such as certain issues with the tire manufacturing process, the characteristic of its tires and the price of RFID tags per tire. Taking risks and confronting challenges are made from board member’s accountability. From the findings, we find that entrepreneurship leadership can be encouraged in case of within the accountability frame work.
Despite its status as a second-tier company, K Tire attempted to adopt the RFID system to its passenger vehicle tires, a feat not achieved even by the leading companies. Thus, the company ultimately built and settled the system through numerous trials and errors. Such success was made possible by the entrepreneurship of K Tire’s management, who took the risk of failure inherent in adopting innovative technologies and confronting challenges head on.
Second, institutional entrepreneurship not only involves the “capacity to imagine alternative possibilities”, it also requires the ability “to contextualize past habits and future projects within the contingencies of the moment” if existing institutions are to be transformed ( Emirbayer and Mische, 1998 ). New technologies, the technical infrastructure, network activities to acquire the new knowledge, learning capabilities, creating a new organization such as Pioneer Lab and new rules to create new technologies are the features. To qualify as institutional entrepreneurs, individuals must break with existing rules and practices associated with the dominant institutional logic(s) and institutionalize the alternative rules, practices or logics they are championing ( Garud and Karnøe, 2003 ; Battilana, 2006 ). K Tire established new organization, “Special lab” to obtain the know technology and information as CEO’s direct sub-committees. Institutional entrepreneurship arise when actors, through their filed position, recognize the opportunity circumstance so called “norms” ( Battilana et al. , 2009 ). To make up the deficit of technologies for RFID, knowledge stream among workers is more needed. Destruction of hierarch ranking system is proxy of the institutional entrepreneurship. Also, K Tire has peculiar norms. Namely, if one requires the further study such as degree course or non-degree course education services, grant systems operated via short screen process. Third, as innovation perspectives, before adopting the RFID system, the majority of K Tire’s researchers insisted that the company use the bar code technology, which had been widely used by the competitors. Such decision was predicated on the prediction that RFID technology would see wider use in the future, as well as the expected effect coming from taking the leading position, with regard to the technology.
Finally, K Tire’s adoption of the RFID technology cannot be understood without government support. The South Korean government has been implementing the “Verification and Dissemination Project for New u-IT Technologies” since 2008. Owing to policy support, K Tire can provide worker with educational service including oversea universities.
5. Conclusions and implications
To cope with various technological impasses, K Tire demonstrated the importance of institutional and corporate entrepreneurship. What a firm pursues more positive act for innovation is a research question.
Unlike firms, K Tire has strongly emphasized IT technology since establishment in 1960. To be promotion, every worker should get certification of IT sectors after recruiting. This has become the firm’s norm. This norm was spontaneously embedded for firm’s culture. K Tire has sought new ICT technology become a first mover. This norm can galvanize to take risk to catch up the first movers in view of institutional entrepreneurship.
That can be cultivated both by corporate entrepreneurship, referred to the activities a firm undertakes to stimulate innovation and encourage calculated risk taking throughout its operations within accountabilities and institutional entrepreneurship, referred to create its own peculiar norm. Contribution of our paper shows both importance of board members of directors in cultivating corporate entrepreneurship and importance of norm and rules in inducing institutional entrepreneurship.
In conclusion, many of them were skeptical about adopting RFID for its passenger vehicle tires at a time when even the global market and technology leaders were not risking such innovation, citing reasons such as risk of failure and development costs. However, enthusiasm and entrepreneurship across the organization towards technical innovation was achieved through the experience of developing leading technologies, as well as the resolve of the company’s management and its institutional entrepreneurship, which resulted in the company’s decision to adopt the RFID technology for small tires, a technology with unverified effects that had not been widely used in the markets. Introduction of new organization which “Special lab” is compelling example of institutional entrepreneurship. Also, to pursue RFID technology, board members unanimously agree to make new organization in the middle of failing and unpredictable success. This decision was possible since K Tire’s cultivated norm which was to boost ICT technologies. In addition, at that time, board of director’s behavior can be explained by corporate entrepreneurship.
From the findings, this paper also suggests importance of firms’ visions or culture from startup stage because they can become a peculiar norm and become firm’s institutional entrepreneurship. In much contemporary research, professionals and experts are identified as key institutional entrepreneurs, who rely on their legitimated claim to authoritative knowledge or particular issue domains. This case study shows that authoritative knowledge by using their peculiar norm, and culture as well as corporate entrepreneurship.
This paper has some limitations. Despite the fact that paper shows various fruitful findings, this study is not free from that our findings are limited to a single exploratory case study. Overcoming such limitation requires securing more samples, including the group of companies that attempt unprecedented innovations across various industries. In this paper, we can’t release all findings through in-depth interview and face-to-face meetings because of promise for preventing the secret tissues.
Nevertheless, the contribution of this study lies in that it shows the importance of corporate entrepreneurship and institutional entrepreneurship for firm’s innovative capabilities to grow ceaselessly.
Integrated frame of analysis
Aldrich , H.E. and Fiol , C.M. ( 1994 ), “ Fools rush in? The institutional context of industry creation ”, Academy of Management Review , Vol. 19 No. 4 , pp. 645 - 670 .
Aldrich , H.E. and Martinez , M.A. ( 2001 ), “ Many are called, but few are chosen: an evolutionary perspective for the study of entrepreneurship ”, Entrepreneurship Theory and Practice , Vol. 25 No. 4 , pp. 41 - 57 .
Battilana , J. ( 2006 ), “ Agency and institutions: the enabling role of individuals’ social position ”, Organization , Vol. 13 No. 5 , pp. 653 - 676 .
Battilana , J. , Leca , B. and Boxenbaum , E. ( 2009 ), “ How actors change institutions: towards a theory of institutional entrepreneurship ”, The Academy of Management Annals , Vol. 3 No. 1 , pp. 65 - 107 .
Branzei , O. and Vertinsky , I. ( 2006 ), “ Strategic pathways to product innovation capabilities in SMEs ”, Journal of Business Venturing , Vol. 21 No. 1 , pp. 75 - 105 .
Burgelman , R.A. ( 1983 ), “ A model of the interaction of strategic behavior, corporate context, and the context of strategy ”, Academy of Management Review , Vol. 8 No. 1 , pp. 61 - 70 .
Capon , N. , Farley , J.U. and Hoenig , S. ( 1990 ), “ Determinants of financial performance: a meta-analysis ”, Management Science , Vol. 36 , pp. 1143 - 1159 .
Covin , J. and Miles , M. ( 1999 ), “ Corporate entrepreneurship and the pursuit of competitive advantage ”, Entrepreneurship Theory and Practice , Vol. 23 , pp. 47 - 63 .
D’Aveni , R.A. ( 1994 ), Hyper-competition: Managing the Dynamics of Strategic Maneuvering . Free Press , New York, NY .
DiMaggio , P.J. ( 1988 ), “ Interest and agency in institutional theory ”, in Zucker , L.G. (Ed.), Institutional Patterns and Organizations , Ballinger , Cambridge, MA .
Emirbayer , M. and Mische , A. ( 1998 ), “ What is agency? ”, American Journal of Sociology , Vol. 103 , pp. 962 - 1023 .
Fligstein , N. ( 1997 ), “ Social skill and institutional theory ”, American Behavioral Scientist , Vol. 40 , pp. 397 - 405 .
Fligstein , N. and Mara-Drita , I. ( 1996 ), “ How to make a market: reflections on the attempt to create a single market in the European Union ”, American Journal of Sociology , Vol. 102 No. 1 , pp. 1 - 33 .
Garud , R. and Karnøe , P. ( 2003 ), “ Bricolage versusbreakthrough: distributed and embedded agency in technology entrepreneurship ”, Research Policy , Vol. 32 , pp. 277 - 300 .
Garud , R. , Jain , S. and Kumaraswamy , A. ( 2002 ), “ Institutional entrepreneurship in the sponsorship of common technological standards: the case of sun microsystems and Java ”, Academy of Management Journal , Vol. 45 No. 1 , pp. 196 - 214 .
Guth , W. and Ginsberg , A. ( 1990 ), “ Guest editors’ introduction: corporate entrepreneurship ”, Strategic Management Journal , Vol. 11 , pp. 5 - 15 .
Hargadon , A.B. and Douglas , Y. ( 2001 ), “ When innovations meet institutions: Edison and the design of the electric light ”, Administrative Science Quarterly , Vol. 46 No. 3 , pp. 476 - 502 .
Hisrich , R.D. ( 1986 ), Entrepreneurship, Intrapreneurship and Venture Capital , Lexington Books , MA .
Hoffman , A.J. ( 1999 ), “ Institutional evolution and change: environmentalism and the US chemical industry ”, Academy of Management Journal , Vol. 42 No. 4 , pp. 351 - 371 .
Jennings , D.F. and Lumpkin , J.R. ( 1989 ), “ Functioning modeling corporate entrepreneurship: an empirical integrative analysis ”, Journal of Management , Vol. 15 , pp. 485 - 502 .
Jepperson , R. and Meyer , J. ( 2001 ), “ The ‘actors’ of modern society: the cultural construction of social agency ”, Sociological Theory , Vol. 18 , pp. 100 - 120 .
Kirzner , I.M. ( 1997 ), “ Entrepreneurial discovery and the competitive market process: an Austrian approach ”, Journal of Economic Literature , Vol. 35 No. 1 , pp. 60 - 85 .
Kuratko , D. ( 2007 ), Corporate Entrepreneurship: Foundations and Trends in Entrepreneurship , Cambridge, MA .
Lawrence , T.B. , Hardy , C. and Phillips , N. ( 2002 ), “ Institutional effects of inter-organizational collaboration: the emergence of proto-institutions ”, Academy of Management Journal , Vol. 45 No. 1 , pp. 281 - 290 .
Lumpkin , G.T. and Dess , G.G. ( 1996 ), “ Clarifying the entrepreneurial orientation construct and linking it to performance ”, Academic. Management Review , Vol. 21 , pp. 135 - 172 .
Maguire , S. , Hardy , C. and Lawrence , T.B. ( 2004 ), “ Institutional entrepreneurship in emerging fields: HIV/AIDS treatment advocacy in Canada ”, The Academy of Management , Vol. 47 No. 5 , pp. 657 - 679 .
Meyer , J.W. , Boli , J. and Thomas , G.M. ( 1994 ), “ Ontology and rationalization in the western cultural account ”, in Richard Scott , W. and Meyer , J.W. (Eds), Institutional Environments and Organizations: Structural Complexity and Individualism , Sage Publications , Thousand Oaks, CA , pp. 9 - 27 .
North , D.C. ( 1990 ), Institutions, Institutional Change and Economic Performance , Cambridge University Press , Cambridge .
Phan , P.H. , Wright , M. , Ucbasaran , D. and Tan , W.L. ( 2009 ), “ Corporate entrepreneurship: current research and future directions ”, Journal of Business Venturing , Vol. 29 , pp. 197 - 205 .
Rojas , F. ( 2010 ), “ Power through institutional work: acquiring academic authority in the 1968 third world strike ”, Academy of Management Journal , Vol. 53 No. 6 , pp. 1263 - 1280 .
Srivastava , A. and Lee , H. ( 2005 ), “ Predicting order and timing of new product moves: the role of top management in corporate entrepreneurship ”, Journal of Business Venturing , Vol. 20 No. 1 , pp. 459 - 481 .
Schumpeter , J.A. ( 1942 ), Capitalism, Socialism, and Democracy , Harper and Brothers , New York, NY .
Scott , R. ( 1995 ), Institutions and Organizations , Sage , Thousand Oaks, CA .
Shane , S. and Venkataraman , S. ( 2000 ), “ The promise of entrepreneurship as a field of research ”, Academy of Management Review , Vol. 25 No. 1 , pp. 217 - 226 .
Teece , D.J. ( 2014 ), “ The foundations of enterprise performance: dynamic and ordinary capabilities in an (economic) theory of firms ”, Academy of Management Perspectives , Vol. 28 No. 4 , pp. 328 - 352 .
Thornhill , S. ( 2006 ), “ Knowledge, innovation and firm performance in high- and low-technology regimes ”, Journal of Business Venturing , Vol. 21 No. 5 , pp. 687 - 703 .
Tidd , J. and Bessant , J. ( 2009 ), Managing Innovation: Integrating Technological, Market and Organizational Change , 4th ed. , John Wiley & Sons , Sussex .
Tushman , M.L. and Anderson , P. ( 1986 ), “ Technological discontinuities and organizational environments ”, Administrative Science Quarterly , Vol. 31 No. 3 , pp. 439 - 465 .
Townley , B. ( 2002 ), “ The role of competing rationalities in institutional change ”, Academy of Management Journal , Vol. 45 No. 1 , pp. 163 - 179 .
Yin , R.K. ( 2008 ), Case Study Research: Design and Methods (Applied Social Research Methods) , 4th ed. , Sage, Thousand Oaks , CA .
Zahra , S.A. ( 1991 ), “ Predictors and financial outcomes of corporate entrepreneurship: an exploratory study ”, Journal of Business Venturing , Vol. 6 , pp. 259 - 285 .
Zahra , S.A. ( 1996 ), “ Governance, ownership, and corporate entrepreneurship: the moderating impact of industry technological opportunities ”, Academy of Management Journal , Vol. 39 No. 6 , pp. 1713 - 1735 .
Zahra , S.A. and George , G. ( 2002 ), “ Absorptive capacity: a review, reconceptualization, and extension ”, Academy of Management Review , Vol. 27 , pp. 185 - 203 .
Further reading
Bresnahan , T.F. , Brynjolfsson , E. and Hitt , L.M. ( 2002 ), “ Information technology, workplace organization, and the demand for skilled labor: firm-level evidence ”, The Quarterly Journal of Economics , Vol. 117 No. 1 , pp. 339 - 376 .
DiMaggio , P.J. ( 1984 ), “ Institutional isomorphism and structural conformity ”, paper presented at the 1984 American Sociological Association meetings, San Antonio, Texas .
Garud , R. ( 2008 ), “ Conferences as venues for the configuration of emerging organizational fields: the case of cochlear implants ”, Journal of Management Studies , Vol. 45 No. 6 , pp. 1061 - 1088 .
Maguire , S. and Hardy , C. ( 2009 ), “ Discourse and deinstitutionalization: the decline of DDT ”, Academy of Management Journal , Vol. 52 No. 1 , pp. 148 - 178 .
Acknowledgements
This work was supported by 2017 Hongik University Research Fund.
Corresponding author
Related articles, we’re listening — tell us what you think, something didn’t work….
Report bugs here
All feedback is valuable
Please share your general feedback
Join us on our journey
Platform update page.
Visit emeraldpublishing.com/platformupdate to discover the latest news and updates
Questions & More Information
Answers to the most commonly asked questions here
Machines of mind: The case for an AI-powered productivity boom
Subscribe to the economic studies bulletin, martin neil baily , martin neil baily senior fellow emeritus - economic studies , center on regulation and markets erik brynjolfsson , and erik brynjolfsson director - stanford digital economy lab, jerry yang and akiko yamazaki professor and senior fellow - stanford institute for human centered ai anton korinek anton korinek nonresident fellow - economic studies , center on regulation and markets @akorinek.
May 10, 2023
Large language models such as ChatGPT are emerging as powerful tools that not only make workers more productive but also increase the rate of innovation, laying the foundation for a significant acceleration in economic growth. As a general purpose technology, AI will impact a wide array of industries, prompting investments in new skills, transforming business processes, and altering the nature of work. However, official statistics will only partially capture the boost in productivity because the output of knowledge workers is difficult to measure. The rapid advances can have great benefits but may also lead to significant risks, so it is crucial to ensure that we steer progress in a direction that benefits all of society.
On a recent Friday morning, one of us sat down in his favorite coffee shop to work on a new research paper regarding how AI will affect the labor market. To begin, he pulled up ChatGPT , a generative AI tool. After entering a few plain-English prompts, the system was able to provide a suitable economic model, draft code to run the model, and produce potential titles for the work. By the end of the morning, he had achieved a week’s worth of progress on his research.
We expect millions of knowledge workers, ranging from doctors and lawyers to managers and salespeople to experience similar ground-breaking shifts in their productivity within a few years, if not sooner.
The potential of the most recent generation of AI systems is illustrated vividly by the viral uptake of ChatGPT, a large language model (LLM) that captured public attention by its ability to generate coherent and contextually appropriate text. This is not an innovation that is languishing in the basement. Its capabilities have already captivated hundreds of millions of users.
Other LLMs that were recently rolled out publicly include Google’s Bard and Anthropic’s Claude . But generative AI is not limited to text: in recent years, we have also seen generative AI systems that can create images, such as Midjourney , Stable Diffusion or DALL-E , and more recently multi-modal systems that combine text, images, video, audio and even robotic functions . These technologies are foundation models , which are vast systems based on deep neural networks that have been trained on massive amounts of data and can then be adapted to perform a wide range of different tasks. Because information and knowledge work dominates the US economy, these machines of the mind will dramatically boost overall productivity.
The power of productivity growth
The primary determinant of our long-term prosperity and welfare is the rate of productivity growth: the amount of output created per hour worked. This holds even though changes in productivity are not immediately felt by everyone and, in the short run, workers’ perceptions of the economy are dominated by the business cycle. From World War II until the early 1970s, labor productivity grew at over 3% a year, more than doubling over the period, ushering in an era of prosperity for most Americans. In the early 1970s productivity growth slowed dramatically, rebounding in the 1990s, only to slow again since the early 2000s.
Figure 1 illustrates the story. It decomposes the overall growth in labor productivity into two components: total factor productivity (which is a measure of the impact of technology) and the contribution of the labor composition and capital intensity. The figure illustrates that the key driver of changes in labor productivity is changes total factor productivity (TFP). There are many reasons for America’s recent economic struggles, but slow TFP growth is a key cause, slowly eating away at the country’s prosperity, making it harder to fight inflation, eroding workers’ wages and worsening budget deficits.
The generally slow pace of economic growth, together with the outsized profits of tech companies, has resulted in skepticism about the benefits of digital technologies for the broad economy. However, for about 10 years starting in the 1990s there was a surge in productivity growth, as shown in Figure 1, driven primarily by a huge wave of investment in computers and communications , which in turn drove business transformations. Even though there was a stock market bubble as well as significant reallocation of labor and resources, workers were generally better off. Furthermore, the federal budget was balanced from 1998 to 2001 —a double win. Digital technology can drive broad economic growth, and it happened less than thirty years ago.
Early estimates of AI’s productivity effects
The recent advances in generative AI have been driven by progress in software, hardware, data collection, and growing amounts of investment in cutting-edge models. Sevilla et al. (2022) observe that the amount of compute (computing power) used to train cutting-edge AI systems has been doubling every six months over the past decade. The capabilities of generative AI systems have grown in tandem, allowing them to perform many tasks that used to be reserved for cognitive workers, such as writing well-crafted sentences, creating computer code, summarizing articles, brainstorming ideas, organizing plans, translating other languages, writing complex emails, and much more.
Generative AI has broad applications that will impact a wide range of workers, occupations, and activities. Unlike most advances in automation in the past, it is a machine of the mind affecting cognitive work. As noted in a recent research paper (Eloundou et al., 2023) , LLMs could affect 80% of the US workforce in some form.
There is an emerging literature that estimates the productivity effects of AI on specific occupations or tasks. Kalliamvakou (2022) finds that software engineers can code up to twice as fast using a tool called Codex, based on the previous version of the large language model GPT-3. That’s a transformative effect. Noy and Zhang (2023) find that many writing tasks can also be completed twice as fast and Korinek (2023) estimates, based on 25 use cases for language models, that economists can be 10-20% more productive using large language models.
Related Content
David Kiron, Elizabeth J. Altman, Christoph Riedl
April 13, 2023
David Autor, Anna Salomons, Bryan Seegmiller
March 9, 2023
Sanjay Patnaik, James Kunhardt, Richard G. Frank
April 18, 2023
But can these gains in specific tasks translate into significant gains in a real-world setting? The answer appears to be yes. Brynjolfsson, Li, and Raymond (2023) show that call center operators became 14% more productive when they used the technology, with the gains of over 30% for the least experienced workers. What’s more, customer sentiment was higher when interacting with operators using generative AI as an aid, and perhaps as a result, employee attrition was lower. The system appears to create value by capturing and conveying some of the tacit organizational knowledge about how to solve problems and please customers that previously was learned only via on-the-job experience.
Criticism of large language models as merely “stochastic parrots” is misplaced. Most cognitive work involves drawing on past knowledge and experience and applying it to the problem at hand. It is true that generative AI programs are prone to certain types of mistakes, but the form of these mistakes is predictable. For example, language models tend to engage in “hallucinations,” i.e., to make up facts and references. As a result, they clearly require human oversight. However, their economic value depends not on whether they are flawless, but on whether they can be used productively. By that criterion, they are already poised to have a massive impact. Moreover, the accuracy of generative AI models continues to improve rapidly.
Quantifying the productivity effects
A recent report by Goldman Sachs suggests that generative AI could raise global GDP by 7%, a truly significant effect for any single technology. Based on our analysis of a variety of use cases and the share of the workforce doing mainly cognitive work, this estimate strikes us as being reasonable, though there remains great uncertainty about the ultimate productivity and growth effects of AI.
It is useful to rigorously break down the channels through which we expect generative AI to produce growth in productivity, output, and ultimately in social welfare in a model.
The first channel is the increased efficiency of output production. By making cognitive workers engaged in production more efficient, the level of output increases. Economic theory tells us that, in competitive markets, the effect of a productivity boost in a given sector on aggregate productivity and output is equal to the size of the productivity boost multiplied by the size of the sector ( Hulten’s theorem ). For instance, if generative AI makes cognitive workers on average 30% more productive over a decade or two and cognitive work makes up about 60% of all value added in the economy (as measured by the wage bill attributable to cognitive tasks), this amounts to a 18% increase in aggregate productivity and output, spread out over those years.
The second, and ultimately more important, channel is the acceleration of innovation and thus future productivity growth. Cognitive workers not only produce current output but also invent new things, engage in discoveries, and generate the technological progress that boosts future productivity. This includes R&D—what scientists do—and perhaps more importantly, the process of rolling out new innovations into production activities throughout the economy—what managers do. If cognitive workers are more efficient, they will accelerate technological progress and thereby boost the rate of productivity growth—in perpetuity. For example, if productivity growth was 2% and the cognitive labor that underpins productivity growth is 20% more productive, this would raise the growth rate of productivity by 20% to 2.4%. In a given year, such a change is barely noticeable and is usually swamped by cyclical fluctuations.
But productivity growth compounds. After a decade, the described tiny increase in productivity growth would leave the economy 5% larger, and the growth would compound further every year thereafter. What’s more, if the acceleration applied to the growth rate of the growth rate (for instance if one of the applications of AI was to improving AI itself ), then of course, growth would accelerate even more over time.
Figure 2 schematically illustrates the effects of the two channels of productivity growth over a twenty year horizon. The baseline follows the current projection of the Congressional Budget Office (CBO) of 1.5% productivity growth , giving rise to a total of 33% productivity growth over 20 years. The projection labeled “Level” assumes that generative AI raises the level of productivity and output by an additional 18% over ten years, as suggested by the illustrative numbers we discussed for the first channel. After ten years, growth reverts to the baseline rate. The third projection labeled “Level+Growth” additionally includes a one percentage point boost in the rate of growth over the baseline rate, resulting from the additional innovation triggered by generative AI. At first, the resulting growth trajectory is barely distinguishable from the “Level” projection, but through the power of compounding, the effects grow bigger over time, leading to a near doubling of output after 20 years, far greater than the baseline projection.
Barriers and drivers of adoption
For the productivity gains to materialize, advances in AI have to disseminate throughout the economy. Traditionally, this has always taken time, so we would not expect potential productivity gains to show up immediately. The advances need to be taken up and rolled out by businesses and organizations that employ cognitive labor throughout the economy, including small and medium-sized businesses, some of which may be slow to realize the potential of adapting advanced new technologies or may lack the required skills to use them well. For example, the Goldman report assumes it takes 10 years for the gains to fully materialize.
The “productivity J-curve” (Brynjolfsson et al., 2021) describes how new technologies, especially general purpose technologies, deliver productivity gains only after a period of investment in complementary intangible goods, such as business processes and new skills. In fact, this can temporarily even drag down measured productivity. As a result, earlier general purpose technologies like electricity and the first wave of computers took decades to have a significant effect on productivity. Additional barriers to adoption and rollout include concerns about job losses and institutional inertia and regulation, in areas from the medicine to finance and law.
However, in the case of generative AI there are also factors that can mitigate these barriers, or even accelerate adoption. First, in contrast to physical automation, one benefit of cognitive automation is that it can often be rolled out quickly via software. This is particularly true now that a ubiquitous digital infrastructure is available: the Internet. ChatGPT famously was the most rapid product launch in history—it gained 100 million users in just two months —because it was accessible to anyone with an internet connection and did not require any hardware investment on the users’ side.
Both Microsoft and Google are in the process of rolling out Generative AI tools as part of their search engines and office suites, offering access to generative AI to a large fraction of the cognitive workforce in advanced countries who regularly use these tools. Furthermore, application programming interfaces (APIs) are increasingly available to enable seamless modularization and connectivity between systems, and a marketplace for plug-ins and extensions is rapidly growing, making it much easier to add functionality. Finally, in contrast to other technologies, users of generative AI can interact with the technology in natural language rather than special codes or commands, making it easier to learn and adopt these tools.
These reasons for optimism suggest that the rollout of these new technologies may be faster than in the past. Still, the importance of training to make optimal use of these tools cannot be overstated.
Problems of measurement – silent productivity growth
The most common measure of productivity, non-farm business productivity, is quite adept at capturing increases in productivity in the industrial sector where inputs and outputs are tangible and easy to account for. However, productivity of cognitive labor is harder to measure. Statisticians who compile GDP and productivity statistics sometimes resort to valuing the output of cognitive activity simply by assuming it is proportional to the quantity of labor input being used to produce it, which of course eliminates any scope for productivity growth.
For example, generative AI enables economists to write more thought pieces and provide deeper analyses of the economy than before, yet this output would not directly show up in GDP statistics. Readers may feel that they have access to better and deeper economic analyses (contributing to channel 1 above). Moreover, the analyses may also play a part in enabling business leaders and policymakers to better harness the positive productivity effects of generative AI (contributing to channel 2 above). Neither of these positive productivity effects of such work would be directly captured in official GDP or productivity statistics, yet the benefits of economists’ productivity gains would still lead to greater social welfare.
The same holds true for many other cognitive workers throughout the economy. This may give rise to significant under-measurement or “silent productivity growth.”
Productivity growth, labor markets, and income distribution
A bigger pie does not automatically mean everyone benefits evenly, or at all. The productivity effects of generative AI are likely to go hand in hand with significant disruption in the job market as many workers may see downward wage pressures. For example, the Eloundou et al. paper cited earlier predicts that up to 49% of the workforce could eventually have half or more of their job tasks performed by AI. Will the demand for these tasks increase enough to compensate for such efficiency gains? Will the workers find other tasks to do? The answers are far from certain. In past technological transformations, workers who lost their jobs could transition to new jobs, and on average pay increased. However, given the scale of the impending disruption and the labor-saving nature of it, it remains to be seen whether this will be the case in the age of generative AI.
Moreover, the current wave of cognitive automation marks a change from most earlier waves of automation, which focused on physical jobs or routine cognitive tasks. Now, creative and unstructured cognitive jobs are also being impacted. Instead of the lowest paid workers bearing the brunt of the disruption, now many of the highest-paying occupations will be affected. These workers may find the disruption to be quite unexpected. If their skills are general, they may find it easier to adjust to displacement than blue-collar workers. However, if they have acquired a significant amount of human capital that becomes obsolete, they may experience much larger income losses than blue-collar workers who were displaced by previous rounds of automation.
The idea of jobs created versus jobs displaced is the most tangible manifestation of job market disruption for lay people. Job losses are indeed a significant social concern, and we need policies to facilitate adjustment. However, as economists, we note that the key factor in determining the influence of new technologies on the labor market is ultimately their effect on labor demand. Counting how many jobs are created versus how many are destroyed misses that employment is determined as the equilibrium of labor demand and labor supply. Labor supply is quite inelastic, reflecting that most working-age people want to or have to work independently of whether their incomes go up or down. Workers who lose their jobs as a result of changing technology will seek alternative employment. And, to the extent that changing technology raises productivity, this will increase national income and spur the demand for labor. Over the long run, the labor market can be expected to equilibrate, meaning that the supply of jobs, the demand for jobs and the level of wages will adjust to maintain full employment. This is evidenced by the fact that the unemployment rate in the United States has remained consistently low in the postwar period (with help from monetary and fiscal policy to recover from recessions). Job destruction has always been offset by job creation. Instead, the effects of automation and augmentation tend to be reflected in wages and income.
The effect of generative AI on labor demand depends on whether the systems complement or substitute for labor . Substitution occurs when AI models automate most or all tasks of certain jobs, while complementing occurs if they automate small parts of certain jobs, leaving humans indispensable. Additionally, AI systems can be complementary to human labor if they enable new tasks or increase quality.
As companies invest more in generative AI, they often have choices about whether to emphasize substitution or complementarity. For example, if call centers can use AI to complement human operators, or, as AI improves, they may restructure their processes to have the systems address more and more queries without human operators being involved. At the same time, higher productivity growth across the economy may make the overall effects more complementary by increasing overall labor demand and may mitigate the disruption.
In recent decades, there have been three main forces impacting income distribution. First, there has been an overall shift of income away from wages and towards corporate capital. Second, there has been an increase in the return to the skills that are valued by companies (reflected in part by higher returns to education). Third, there has been a shift caused by increased foreign competition .
It is hard to predict how generative AI will impact this mix. A positive interpretation is that workers who currently struggle with aspects of math and writing will become more productive with the help of these new tools and will be able to take better-paid jobs with the help of the new technology. A negative interpretation is that companies will use the technology to eliminate or de-skill more and more positions pushing a larger fraction of the workforce into unfulfilling jobs, raising the share of profits in income and, perhaps, increasing the demand for the most elite members of the workforce.
No doubt technological progress will not stop with the current wave of generative AI. Instead, we can expect even more dramatic advances in AI, bringing the technology closer to what is called artificial general intelligence (AGI). This will lead to even more radical transformations of life and work . The scarcity of human labor has been a double-edged sword throughout our history : on the one hand, it has held back economic growth because greater production would require more labor; on the other hand, it has been highly beneficial for income distribution since wages represent the market value of scarce labor. If labor can be replaced by machines across a wide range of tasks in the future, both points may no longer hold, and we may experience an AI-powered growth take-off at the same time as that the value of labor declines. This would present a significant challenge for our society . Moreover, AGI may also impose large risks on humanity if not aligned with human objectives .
Large language models and other forms of generative AI are still at an early stage, making it difficult to predict with great confidence the exact productivity effects they will have. Yet as we have argued, we expect that generative AI will have tremendous positive productivity effects, both by increasing the level of productivity and accelerating future productivity growth.
For policymakers, the goal should be to allow for the positive productivity gains while mitigating the risks and downsides of ever-more powerful AI. Faster productivity growth is an elixir that can solve or mitigate many of our society’s challenges, from raising living standards and addressing poverty to providing healthcare for all and strengthening our defenses. Indeed, it will be nearly impossible to fix some of our budgetary challenges, including the growing deficits, without sufficiently stronger growth.
AI-powered productivity growth will also create challenges. There may be a need for updating social programs and tax policy to soften the welfare costs of labor market disruptions and ensure that the benefits of AI give rise to shared prosperity rather than concentration of wealth. Other harms will also need to be addressed, including the amplification of misinformation and polarization, potentially destabilizing our democracy, and the creation of new biological and other weapons that could injure or kill untold numbers of people.
Therefore, we cannot let the capabilities of AI outstrip our understanding of their potential impacts. Economists and other social scientists will need to accelerate their work on AI’s impacts to keep up with our colleagues in AI research who are rapidly advancing the technologies. If we do that, we are optimistic our society can harness the productivity benefits and growth acceleration delivered by artificial intelligence to substantially advance human welfare in the coming years.
The authors used GPT4 for writing assistance in producing this text but assume full responsibility for its content and accuracy.
The Brookings Institution is financed through the support of a diverse array of foundations, corporations, governments, individuals, as well as an endowment. A list of donors can be found in our annual reports published online here . The findings, interpretations, and conclusions in this report are solely those of its author(s) and are not influenced by any donation.
Artificial Intelligence Technology Policy & Regulation
Regulatory Policy
Economic Studies
Center on Regulation and Markets
Ajay Agrawal, Joshua S. Gans, Avi Goldfarb
June 12, 2023
Sarah Kreps, Paul Timmers
December 20, 2022
Joshua Foust
October 20, 2022
30+ Digital Transformation Case Studies & Success Stories [2024]

Digital transformation has been on the executive agenda for the past decade and ~ 90% of companies have already initiated their first digital strategy. However, given the increasing pace of technological innovation, there are numerous areas to focus on. A lack of focus leads to failed initiatives. Digital transformation leaders need to focus their efforts but they are not clear about in which areas to focus their digital transformation initiatives.
We see that digital transformation projects focusing on customer service and operations tend to be more heavily featured in case studies and we recommend enterprises to initially focus on digitally transforming these areas.
Research findings:
- Outsourcing is an important strategy for many companies’ digital transformation initiatives.
- Most successful digital transformation projects focus on customer service and operations
Michelin-EFFIFUEL
Michelin, a global tire manufacturer, launched its EFFIFUEL initiative in 2013 to reduce the fuel consumption of trucks. In this context, vehicles were equipped with telematics systems that collect and process data on the trucks, tires, drivers habits and fuel consumption conditions. By analyzing this data, fleet managers and executives at the trucking companies were able to make adjustments to reduce oil consumption.
- Business challenge : Inability to improve customer retention rates to target levels, due to trucks’ fuel consumption and CO2 emissions .
- Target customers : Fleet managers and operations managers at truck companies in Europe
- Line of business function : Customer success management and sales.
- Solution : By using smart devices, truck and tire performance degradation is detected and maintained from the start. The solution also nudges truck drivers into more cost and environmentally friendly driving.
- Business result : Enhanced customer retention and satisfaction. EFFIFUEL has brought fuel savings of 2.5 liters per 100 kilometers per truck. The company also reduced the environmental costs of transportation activities. According to Michellin, if all European trucking companies had been using the EFFIFUEL initiative, it would have caused a 9 tons of CO2 emission reduction.
Schneider Electric-Box
Schneider Electric is a global company with employees all over the world. Prior to the Box initiative , which is a cloud-based solution, business processes were relatively slow because it is difficult to process the same documents from different locations at the same time. Schneider Electric also needed a way to provide data management and security for its globally dispersed workforce. So Schneider Electric outsourced its own custom cloud environment that integrates with Microsoft Office applications to Box. The platform also ensures tight control of corporate data with granular permissions, content controls and the use of shared links. Thanks to this initiative, the company has moved from 80% of its content hosted on-premises to 90% in the cloud and has a more flexible workforce.
- Business challenge : Inability to increase operational efficiency of the global workforce without capitulating to data security.
- Solution : Outsourcing company’s cloud-based platform to Box, that ensures data security and integration with Microsoft Office programs to ensure ease of doing business.
- Business result : Schneider Electric connects its 142.000 workers within one platform which hosts 90% of its documents.
Thomas Pink-Fits.me
British shirt maker Thomas Pink, part of the Louis Vuitton Moet Hennessey group, has outsourced the development of its online sales platform to Fits.me Virtual Fitting Room . The aim of the initiative was to gain a competitive advantage over its competitors in e-commerce. Thanks to the online platform developed, customers can determine how well the shirt they are buying fits them by entering their body size.
The platform also helps Fits.me gain better customer insight as previously unknown customer data, including body measurements and fit preferences, becomes available. In this way, the platform can offer customers the clothes that fit them better.
- Business challenge : Lack of visibility into online sales and customers’ preferences.
- Target customers : Online buyers and users.
- Line of business function : Sales and customer success management.
- Solution : Outsourcing the development of the online platform to Fits.me Virtual Fitting Room .
- Business result : Improved customer satisfaction and engagement. Thomas Pink reports that customers who enter the virtual fitting room are more likely to purchase a product than those who do not. There are many successful digital transformation projects from different industries, but we won’t go into every case study. Therefore, we provide you with a sortable list of 31 successful case studies. We categorized them as:
- System Improvement : changing the way existing businesses work by introducing new technologies.
- Innovation : creating new business practices, based on the latest technology.
If you are ready to start you digital transformation journey, you can check our data-driven and comprehensive list of digital transformation consultant companies .
To find out more about digital transformation, you can also read our digital transformation best practices , digital transformation roadmap and digital transformation culture articles.
You can also check our sustainability case studies article which include ESG related success stories.
For any further assistance please contact us:
This article was drafted by former AIMultiple industry analyst Görkem Gençer.

Cem has been the principal analyst at AIMultiple since 2017. AIMultiple informs hundreds of thousands of businesses (as per similarWeb) including 60% of Fortune 500 every month. Cem's work has been cited by leading global publications including Business Insider , Forbes, Washington Post , global firms like Deloitte , HPE, NGOs like World Economic Forum and supranational organizations like European Commission . You can see more reputable companies and media that referenced AIMultiple. Throughout his career, Cem served as a tech consultant, tech buyer and tech entrepreneur. He advised businesses on their enterprise software, automation, cloud, AI / ML and other technology related decisions at McKinsey & Company and Altman Solon for more than a decade. He also published a McKinsey report on digitalization. He led technology strategy and procurement of a telco while reporting to the CEO. He has also led commercial growth of deep tech company Hypatos that reached a 7 digit annual recurring revenue and a 9 digit valuation from 0 within 2 years. Cem's work in Hypatos was covered by leading technology publications like TechCrunch and Business Insider . Cem regularly speaks at international technology conferences. He graduated from Bogazici University as a computer engineer and holds an MBA from Columbia Business School.
To stay up-to-date on B2B tech & accelerate your enterprise:
Next to Read
6 easy process mining digital transformation tips [2024], healthcare apis in 2024: top 7 use cases & case studies, digital transformation pharma trends & technologies in 2024.
Your email address will not be published. All fields are required.
Related research
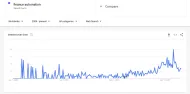
Top 10 Technologies Enabling Finance Digital Transformation in 2024
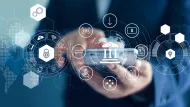
Digital Transformation in Banking: Top Trends & Best Practices
- Browse All Articles
- Newsletter Sign-Up
InformationTechnology →
No results found in working knowledge.
- Were any results found in one of the other content buckets on the left?
- Try removing some search filters.
- Use different search filters.

- SUGGESTED TOPICS
- The Magazine
- Newsletters
- Managing Yourself
- Managing Teams
- Work-life Balance
- The Big Idea
- Data & Visuals
- Reading Lists
- Case Selections
- HBR Learning
- Topic Feeds
- Account Settings
- Email Preferences
Case Study: How Aggressively Should a Bank Pursue AI?
- Thomas H. Davenport
- George Westerman

A Malaysia-based CEO weighs the risks and potential benefits of turning a traditional bank into an AI-first institution.
Siti Rahman, the CEO of Malaysia-based NVF Bank, faces a pivotal decision. Her head of AI innovation, a recent recruit from Google, has a bold plan. It requires a substantial investment but aims to transform the traditional bank into an AI-first institution, substantially reducing head count and the number of branches. The bank’s CFO worries they are chasing the next hype cycle and cautions against valuing efficiency above all else. Siti must weigh the bank’s mixed history with AI, the resistance to losing the human touch in banking services, and the risks of falling behind in technology against the need for a prudent, incremental approach to innovation.
Two experts offer advice: Noemie Ellezam-Danielo, the chief digital and AI strategy at Société Générale, and Sastry Durvasula, the chief information and client services officer at TIAA.
Siti Rahman, the CEO of Malaysia-headquartered NVF Bank, hurried through the corridors of the university’s computer engineering department. She had directed her driver to the wrong building—thinking of her usual talent-recruitment appearances in the finance department—and now she was running late. As she approached the room, she could hear her head of AI innovation, Michael Lim, who had joined NVF from Google 18 months earlier, breaking the ice with the students. “You know, NVF used to stand for Never Very Fast,” he said to a few giggles. “But the bank is crawling into the 21st century.”

- Thomas H. Davenport is the President’s Distinguished Professor of Information Technology and Management at Babson College, a visiting scholar at the MIT Initiative on the Digital Economy, and a senior adviser to Deloitte’s AI practice. He is a coauthor of All-in on AI: How Smart Companies Win Big with Artificial Intelligence (Harvard Business Review Press, 2023).
- George Westerman is a senior lecturer at MIT Sloan School of Management and a coauthor of Leading Digital (HBR Press, 2014).
Partner Center

McKinsey Technology Trends Outlook 2023
After a tumultuous 2022 for technology investment and talent, the first half of 2023 has seen a resurgence of enthusiasm about technology’s potential to catalyze progress in business and society. Generative AI deserves much of the credit for ushering in this revival, but it stands as just one of many advances on the horizon that could drive sustainable, inclusive growth and solve complex global challenges.
To help executives track the latest developments, the McKinsey Technology Council has once again identified and interpreted the most significant technology trends unfolding today. While many trends are in the early stages of adoption and scale, executives can use this research to plan ahead by developing an understanding of potential use cases and pinpointing the critical skills needed as they hire or upskill talent to bring these opportunities to fruition.
Our analysis examines quantitative measures of interest, innovation, and investment to gauge the momentum of each trend. Recognizing the long-term nature and interdependence of these trends, we also delve into underlying technologies, uncertainties, and questions surrounding each trend. This year, we added an important new dimension for analysis—talent. We provide data on talent supply-and-demand dynamics for the roles of most relevance to each trend. (For more, please see the sidebar, “Research methodology.”)
New and notable
All of last year’s 14 trends remain on our list, though some experienced accelerating momentum and investment, while others saw a downshift. One new trend, generative AI, made a loud entrance and has already shown potential for transformative business impact.
Research methodology
To assess the development of each technology trend, our team collected data on five tangible measures of activity: search engine queries, news publications, patents, research publications, and investment. For each measure, we used a defined set of data sources to find occurrences of keywords associated with each of the 15 trends, screened those occurrences for valid mentions of activity, and indexed the resulting numbers of mentions on a 0–1 scoring scale that is relative to the trends studied. The innovation score combines the patents and research scores; the interest score combines the news and search scores. (While we recognize that an interest score can be inflated by deliberate efforts to stimulate news and search activity, we believe that each score fairly reflects the extent of discussion and debate about a given trend.) Investment measures the flows of funding from the capital markets into companies linked with the trend. Data sources for the scores include the following:
- Patents. Data on patent filings are sourced from Google Patents.
- Research. Data on research publications are sourced from the Lens (www.lens.org).
- News. Data on news publications are sourced from Factiva.
- Searches. Data on search engine queries are sourced from Google Trends.
- Investment. Data on private-market and public-market capital raises are sourced from PitchBook.
- Talent demand. Number of job postings is sourced from McKinsey’s proprietary Organizational Data Platform, which stores licensed, de-identified data on professional profiles and job postings. Data is drawn primarily from English-speaking countries.
In addition, we updated the selection and definition of trends from last year’s study to reflect the evolution of technology trends:
- The generative-AI trend was added since last year’s study.
- We adjusted the definitions of electrification and renewables (previously called future of clean energy) and climate technologies beyond electrification and renewables (previously called future of sustainable consumption).
- Data sources were updated. This year, we included only closed deals in PitchBook data, which revised downward the investment numbers for 2018–22. For future of space technologies investments, we used research from McKinsey’s Aerospace & Defense Practice.
This new entrant represents the next frontier of AI. Building upon existing technologies such as applied AI and industrializing machine learning, generative AI has high potential and applicability across most industries. Interest in the topic (as gauged by news and internet searches) increased threefold from 2021 to 2022. As we recently wrote, generative AI and other foundational models change the AI game by taking assistive technology to a new level, reducing application development time, and bringing powerful capabilities to nontechnical users. Generative AI is poised to add as much as $4.4 trillion in economic value from a combination of specific use cases and more diffuse uses—such as assisting with email drafts—that increase productivity. Still, while generative AI can unlock significant value, firms should not underestimate the economic significance and the growth potential that underlying AI technologies and industrializing machine learning can bring to various industries.
Investment in most tech trends tightened year over year, but the potential for future growth remains high, as further indicated by the recent rebound in tech valuations. Indeed, absolute investments remained strong in 2022, at more than $1 trillion combined, indicating great faith in the value potential of these trends. Trust architectures and digital identity grew the most out of last year’s 14 trends, increasing by nearly 50 percent as security, privacy, and resilience become increasingly critical across industries. Investment in other trends—such as applied AI, advanced connectivity, and cloud and edge computing—declined, but that is likely due, at least in part, to their maturity. More mature technologies can be more sensitive to short-term budget dynamics than more nascent technologies with longer investment time horizons, such as climate and mobility technologies. Also, as some technologies become more profitable, they can often scale further with lower marginal investment. Given that these technologies have applications in most industries, we have little doubt that mainstream adoption will continue to grow.
Organizations shouldn’t focus too heavily on the trends that are garnering the most attention. By focusing on only the most hyped trends, they may miss out on the significant value potential of other technologies and hinder the chance for purposeful capability building. Instead, companies seeking longer-term growth should focus on a portfolio-oriented investment across the tech trends most important to their business. Technologies such as cloud and edge computing and the future of bioengineering have shown steady increases in innovation and continue to have expanded use cases across industries. In fact, more than 400 edge use cases across various industries have been identified, and edge computing is projected to win double-digit growth globally over the next five years. Additionally, nascent technologies, such as quantum, continue to evolve and show significant potential for value creation. Our updated analysis for 2023 shows that the four industries likely to see the earliest economic impact from quantum computing—automotive, chemicals, financial services, and life sciences—stand to potentially gain up to $1.3 trillion in value by 2035. By carefully assessing the evolving landscape and considering a balanced approach, businesses can capitalize on both established and emerging technologies to propel innovation and achieve sustainable growth.
Tech talent dynamics
We can’t overstate the importance of talent as a key source in developing a competitive edge. A lack of talent is a top issue constraining growth. There’s a wide gap between the demand for people with the skills needed to capture value from the tech trends and available talent: our survey of 3.5 million job postings in these tech trends found that many of the skills in greatest demand have less than half as many qualified practitioners per posting as the global average. Companies should be on top of the talent market, ready to respond to notable shifts and to deliver a strong value proposition to the technologists they hope to hire and retain. For instance, recent layoffs in the tech sector may present a silver lining for other industries that have struggled to win the attention of attractive candidates and retain senior tech talent. In addition, some of these technologies will accelerate the pace of workforce transformation. In the coming decade, 20 to 30 percent of the time that workers spend on the job could be transformed by automation technologies, leading to significant shifts in the skills required to be successful. And companies should continue to look at how they can adjust roles or upskill individuals to meet their tailored job requirements. Job postings in fields related to tech trends grew at a very healthy 15 percent between 2021 and 2022, even though global job postings overall decreased by 13 percent. Applied AI and next-generation software development together posted nearly one million jobs between 2018 and 2022. Next-generation software development saw the most significant growth in number of jobs (exhibit).

Image description:
Small multiples of 15 slope charts show the number of job postings in different fields related to tech trends from 2021 to 2022. Overall growth of all fields combined was about 400,000 jobs, with applied AI having the most job postings in 2022 and experiencing a 6% increase from 2021. Next-generation software development had the second-highest number of job postings in 2022 and had 29% growth from 2021. Other categories shown, from most job postings to least in 2022, are as follows: cloud and edge computing, trust architecture and digital identity, future of mobility, electrification and renewables, climate tech beyond electrification and renewables, advanced connectivity, immersive-reality technologies, industrializing machine learning, Web3, future of bioengineering, future of space technologies, generative AI, and quantum technologies.
End of image description.
This bright outlook for practitioners in most fields highlights the challenge facing employers who are struggling to find enough talent to keep up with their demands. The shortage of qualified talent has been a persistent limiting factor in the growth of many high-tech fields, including AI, quantum technologies, space technologies, and electrification and renewables. The talent crunch is particularly pronounced for trends such as cloud computing and industrializing machine learning, which are required across most industries. It’s also a major challenge in areas that employ highly specialized professionals, such as the future of mobility and quantum computing (see interactive).
Michael Chui is a McKinsey Global Institute partner in McKinsey’s Bay Area office, where Mena Issler is an associate partner, Roger Roberts is a partner, and Lareina Yee is a senior partner.
The authors wish to thank the following McKinsey colleagues for their contributions to this research: Bharat Bahl, Soumya Banerjee, Arjita Bhan, Tanmay Bhatnagar, Jim Boehm, Andreas Breiter, Tom Brennan, Ryan Brukardt, Kevin Buehler, Zina Cole, Santiago Comella-Dorda, Brian Constantine, Daniela Cuneo, Wendy Cyffka, Chris Daehnick, Ian De Bode, Andrea Del Miglio, Jonathan DePrizio, Ivan Dyakonov, Torgyn Erland, Robin Giesbrecht, Carlo Giovine, Liz Grennan, Ferry Grijpink, Harsh Gupta, Martin Harrysson, David Harvey, Kersten Heineke, Matt Higginson, Alharith Hussin, Tore Johnston, Philipp Kampshoff, Hamza Khan, Nayur Khan, Naomi Kim, Jesse Klempner, Kelly Kochanski, Matej Macak, Stephanie Madner, Aishwarya Mohapatra, Timo Möller, Matt Mrozek, Evan Nazareth, Peter Noteboom, Anna Orthofer, Katherine Ottenbreit, Eric Parsonnet, Mark Patel, Bruce Philp, Fabian Queder, Robin Riedel, Tanya Rodchenko, Lucy Shenton, Henning Soller, Naveen Srikakulam, Shivam Srivastava, Bhargs Srivathsan, Erika Stanzl, Brooke Stokes, Malin Strandell-Jansson, Daniel Wallance, Allen Weinberg, Olivia White, Martin Wrulich, Perez Yeptho, Matija Zesko, Felix Ziegler, and Delphine Zurkiya.
They also wish to thank the external members of the McKinsey Technology Council.
This interactive was designed, developed, and edited by McKinsey Global Publishing’s Nayomi Chibana, Victor Cuevas, Richard Johnson, Stephanie Jones, Stephen Landau, LaShon Malone, Kanika Punwani, Katie Shearer, Rick Tetzeli, Sneha Vats, and Jessica Wang.
Explore a career with us
Related articles.
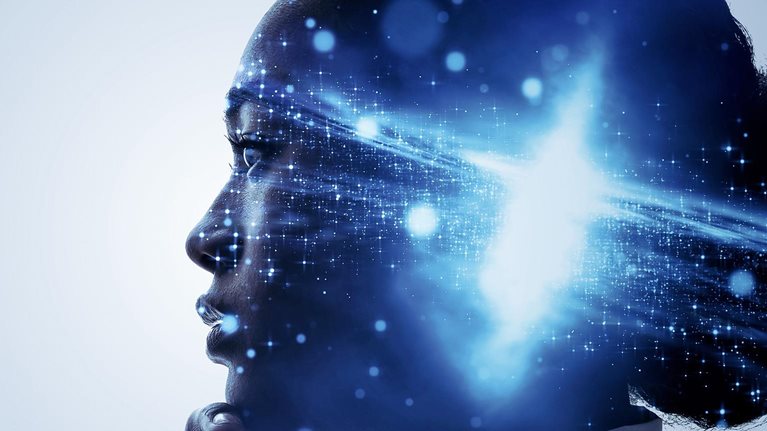
McKinsey Technology Trends Outlook 2022
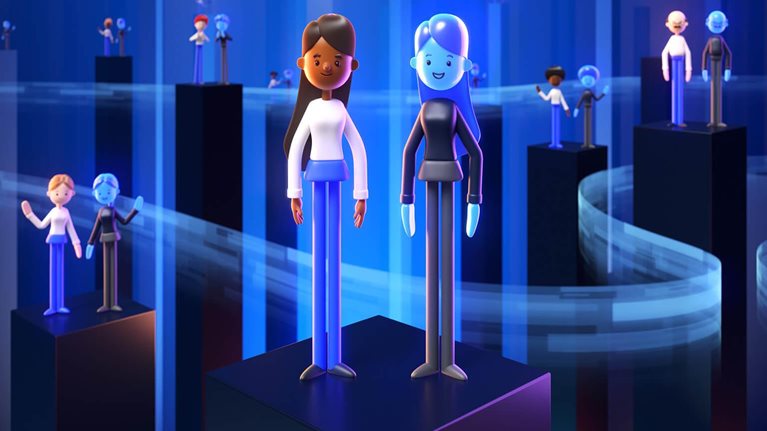
Value creation in the metaverse
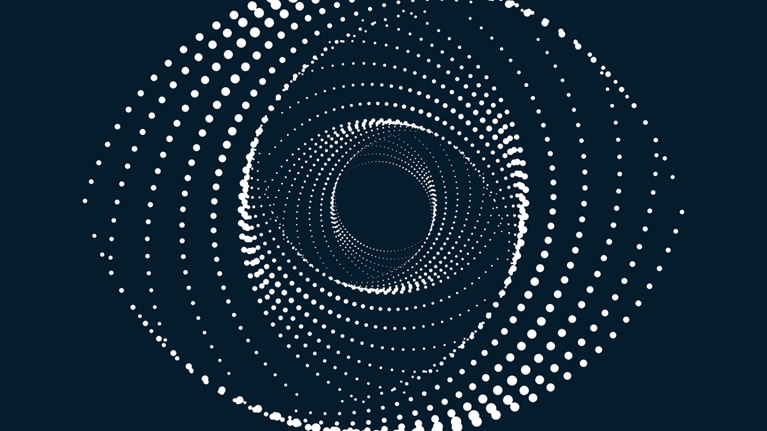
Quantum computing funding remains strong, but talent gap raises concern
3 lessons from IBM on designing responsible, ethical AI
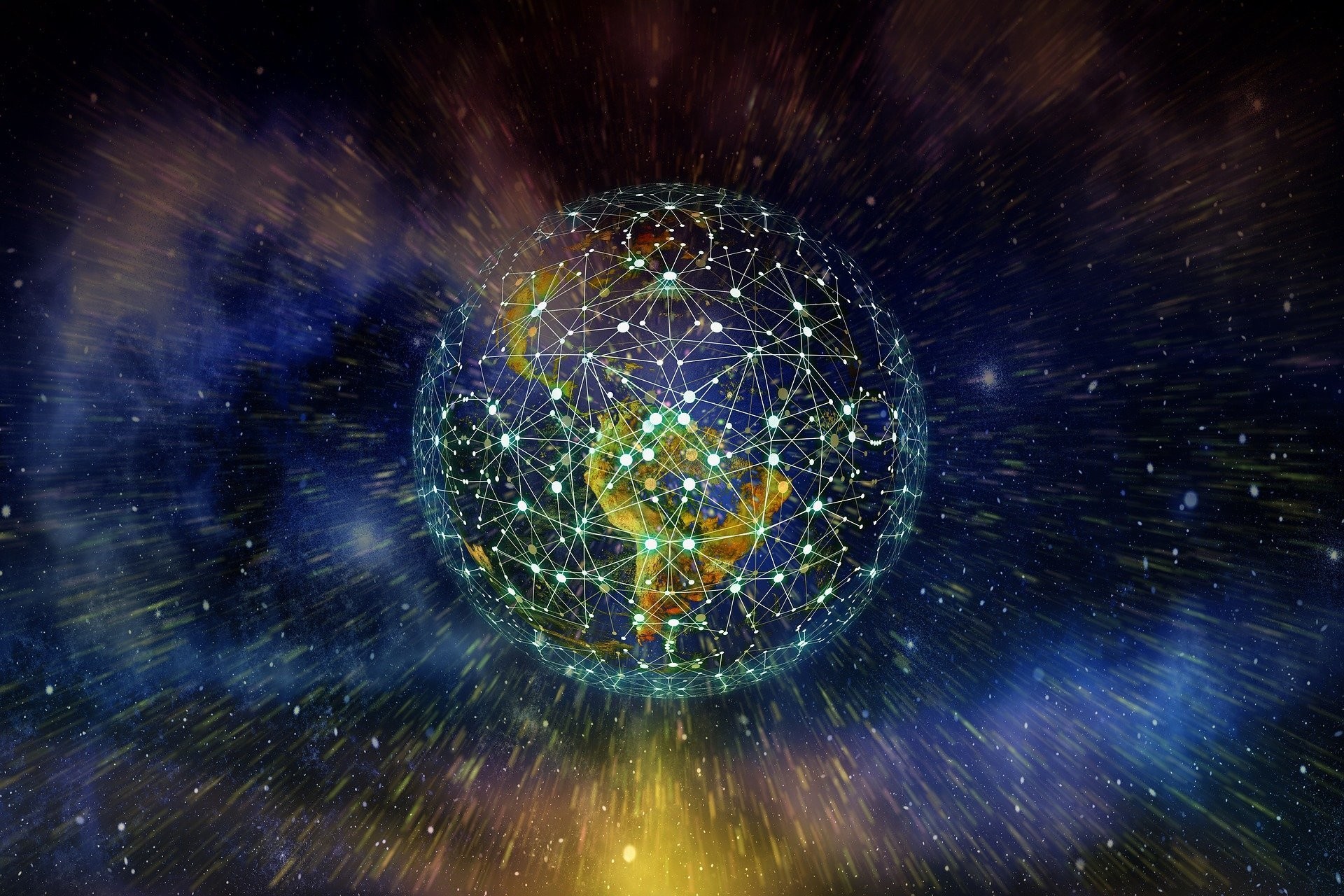
AI technology will affect all of human society - so it's crucial to put the work in to get it right. Image: Gerd Altmann / Pixabay
.chakra .wef-1c7l3mo{-webkit-transition:all 0.15s ease-out;transition:all 0.15s ease-out;cursor:pointer;-webkit-text-decoration:none;text-decoration:none;outline:none;color:inherit;}.chakra .wef-1c7l3mo:hover,.chakra .wef-1c7l3mo[data-hover]{-webkit-text-decoration:underline;text-decoration:underline;}.chakra .wef-1c7l3mo:focus,.chakra .wef-1c7l3mo[data-focus]{box-shadow:0 0 0 3px rgba(168,203,251,0.5);} Daniel Lim
Brian green.
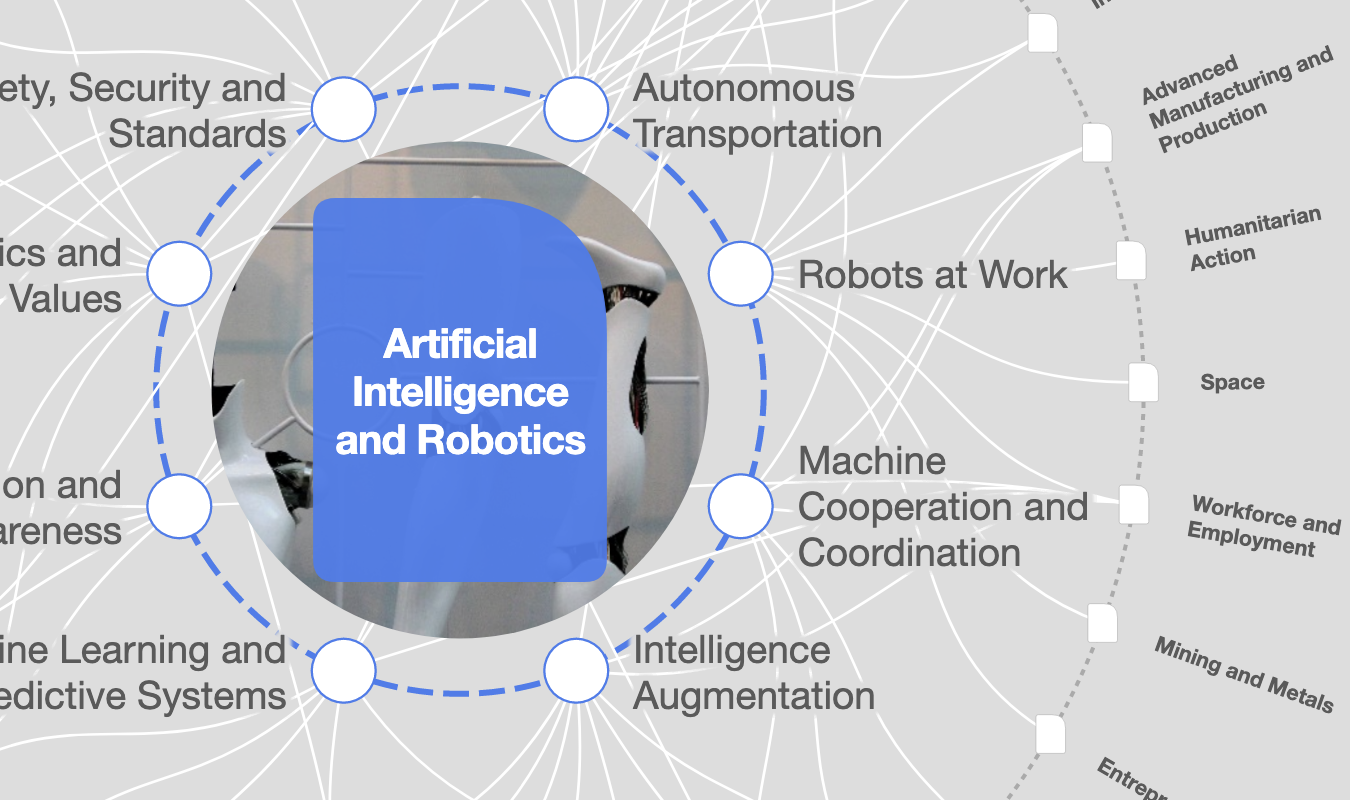
.chakra .wef-9dduvl{margin-top:16px;margin-bottom:16px;line-height:1.388;font-size:1.25rem;}@media screen and (min-width:56.5rem){.chakra .wef-9dduvl{font-size:1.125rem;}} Explore and monitor how .chakra .wef-15eoq1r{margin-top:16px;margin-bottom:16px;line-height:1.388;font-size:1.25rem;color:#F7DB5E;}@media screen and (min-width:56.5rem){.chakra .wef-15eoq1r{font-size:1.125rem;}} Artificial Intelligence is affecting economies, industries and global issues
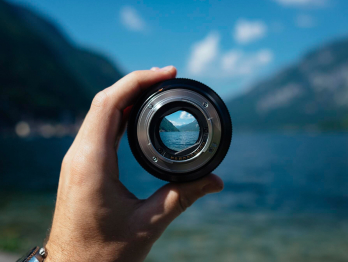
.chakra .wef-1nk5u5d{margin-top:16px;margin-bottom:16px;line-height:1.388;color:#2846F8;font-size:1.25rem;}@media screen and (min-width:56.5rem){.chakra .wef-1nk5u5d{font-size:1.125rem;}} Get involved with our crowdsourced digital platform to deliver impact at scale
Stay up to date:, artificial intelligence.
- IBM is well underway on its mission to develop and advance ethical AI technology.
- A new report , co-authored by the World Economic Forum and the Markkula Center for Applied Ethics at Santa Clara University, details these efforts.
- Here are its main lessons for other organizations in this space.
Over the past two years, the World Economic Forum has been working with a multi-stakeholder group to advance ethics in technology under a project titled Responsible Use of Technology . This group has identified a need to highlight and share best practices in the responsible design, development, deployment and use of technology. To this end, we have embarked on publishing a series of case studies that feature organizations that have made meaningful contributions and progress in technology ethics. Earlier this year, we began this series with a deep dive into Microsoft’s approach to responsible innovation .
In the second edition of this series, we focus on IBM’s journey towards ethical AI technology. The insights from this effort are detailed in a report titled Responsible Use of Technology: The IBM Case Study , which is jointly authored by the World Economic Forum and the Markkula Center for Applied Ethics at Santa Clara University. Below are the key lessons learned from our research, along with a brief overview of IBM's historical journey towards ethical technology.
1. Trusting your employees to think and act ethically
When Francesca Rossi joined IBM in 2015 with the mandate to work on AI ethics, she convened 40 colleagues to explore this topic. The work of this group of employees set in motion a critical chapter of IBM’s ethical AI technology journey.
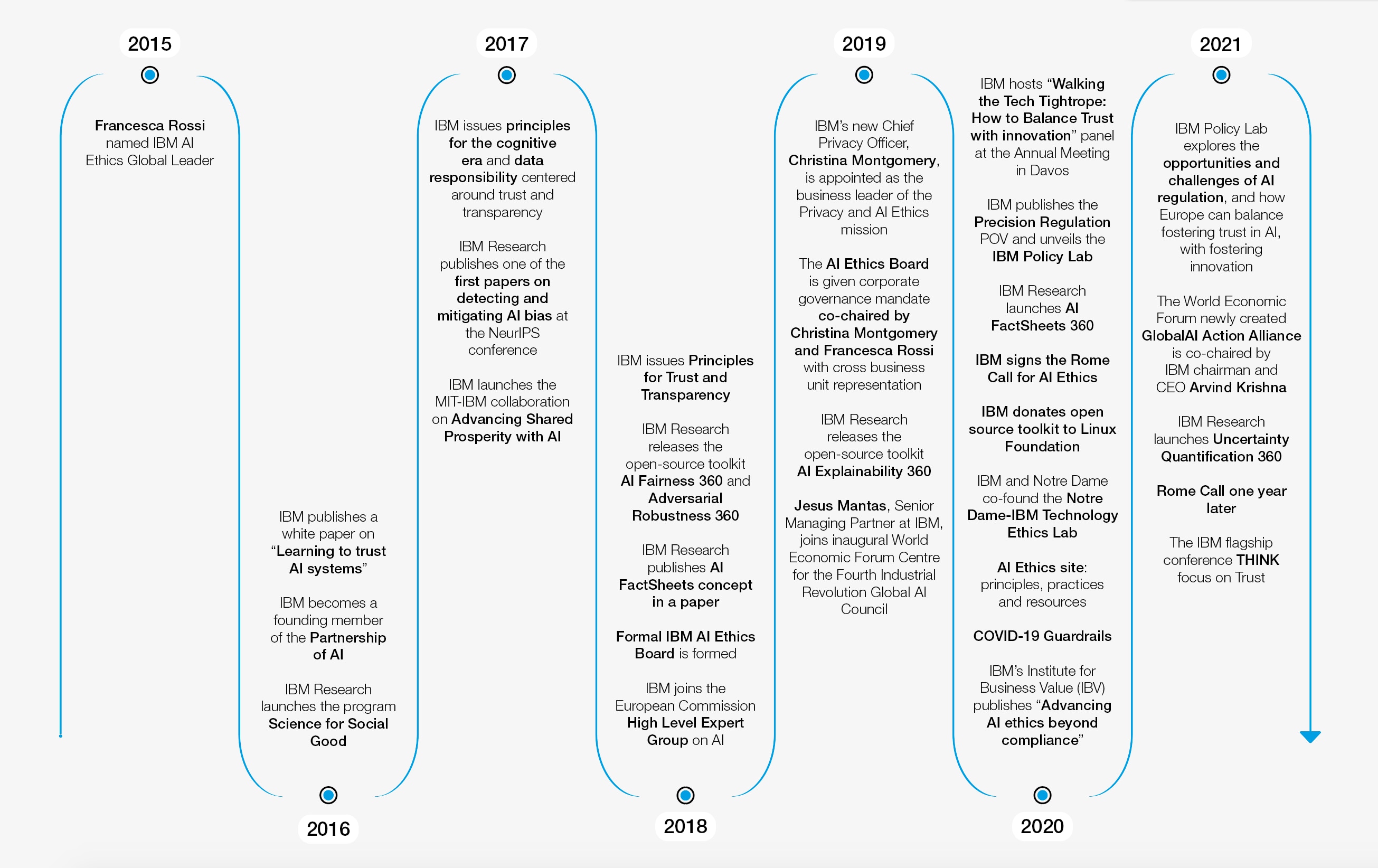
Initially, the group researched and published a paper titled Learning to trust AI systems , in which the company makes a set of commitments to advance its understanding and to put into effect the ethical development of AI. One of these commitments is to establish an internal 'AI ethics board', to discuss, advise and guide the ethical development and deployment of AI systems. With the leadership of Dr. Rossi and IBM’s chief privacy officer Christina Montgomery, this board is responsible for governing the company’s technology ethics efforts globally. However, a centralized ethics governance board is insufficient to oversee more than 345,000 employees working in over 175 countries. All employees are being trained in the 'ethics by design' methodology, and it is also a mandate for all of IBM's business units to adopt this methodology. The AI ethics board relies on a group of employees called 'focal points', who have official roles to support their business units on issues related to ethics.
There is also a network of employee volunteers called the 'advocacy network', which promote the culture of ethical, responsible, and trustworthy technology. Empowering employees is an important part of making technology ethics work at IBM.
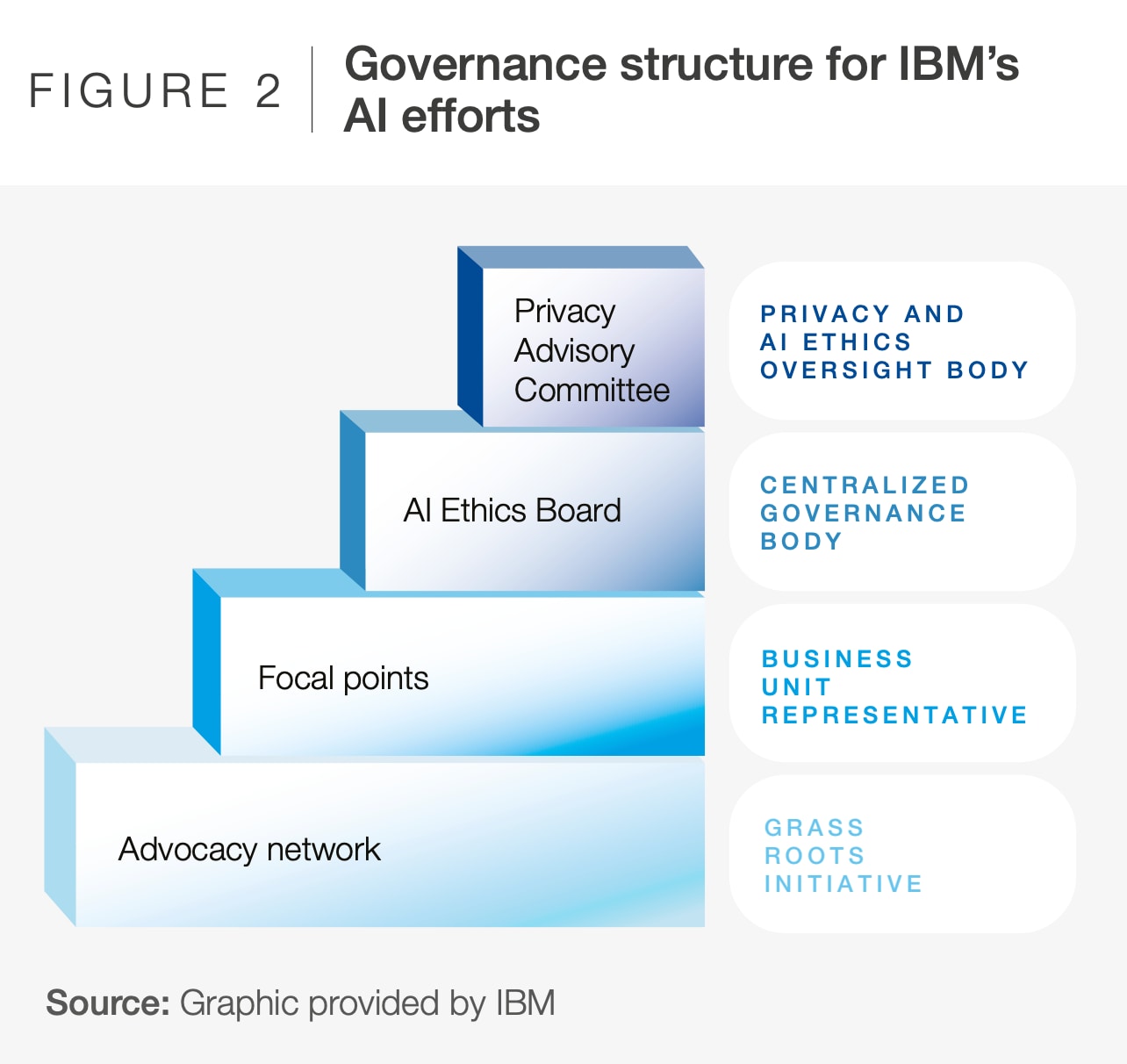
2. Operationalizing values and principles on AI ethics
To operationalize ethics in an organizational setting, there must first be commitments made to values. To this end, IBM developed a set of 'principles and pillars' to direct its research and business towards supporting key values in AI. Implementation of these principles and pillars led to the development of technical toolkits so that ethics could penetrate to the level of code; IBM Research created five open-source toolkits, freely available to anyone:
1. AI Explainability 360: eight algorithms for making machine learning models more explainable
2. AI Fairness 360: a set of 70 fairness metrics and 10 bias-mitigation algorithms
3. Adversarial Robustness Toolbox: a large set of tools for overcoming an array of adversarial attacks on machine learning models.
4. AI FactSheets 360: to make AI models transparent, factsheets collect data about the model in one place; this includes examples of FactSheets as well as a methodology for creating them.
5. Uncertainty Quantification 360: a set of tools to test how reliable AI predictions are, which helps to place boundaries on model confidence.
Any organization can make principles or other ethical commitments, but they have to be operationalized to become a reality. These toolkits help IBM fulfill its own ethical commitments, and by making them open-source IBM hopes to help other tech companies, and the machine learning community more broadly.
3. Aiming for broad impact on AI ethics
IBM recognizes that it is not separate from the tech industry or the world. Its actions will affect the reputation of the industry – and vice versa – and, ultimately, the entire tech industry together will affect human society. All this is to say that the stakes are high, so it is worth putting in the effort to get it right.
One of the ways that IBM has been attempting to promote the broad positive impact of technology has been through partnerships with educational and research organizations and multistakeholder organizations.
Within education, in 2011 IBM launched P-TECH, a programme that promotes career-applicable skill-building for high school students while working with an IBM mentor. Eventually, they can participate in paid summer internships at IBM and enroll in an associate’s degree programme at no cost.
At the university level, IBM has several partnerships with schools, such as the Notre Dame - IBM Tech Ethics Lab , to support underrepresented communities in STEM subjects and their teachers, and to improve relevant curricula. By teaming up with research organizations, IBM is helping to train the next generation of technology professionals and creates communications channels, between academia and industry, which include promoting the discussion of ethical concerns from both sides.
IBM is a founding member of several multistakeholders organizations, including the Partnership on AI to Benefit People and Society (2017) and the Vatican’s 'Rome Call for AI Ethics' (2020). IBM also works on many World Economic Forum initiatives related to AI ethics, including the Global Future Council on AI for Humanity, the Global AI Council, and the Global AI Action Alliance.
Besides these institutional partnerships, individual IBM employees are also involved in the IEEE’s Ethics in Action Initiative, the Future of Life Institute, the AAAI/ACM AI, Ethics, and Society conference, and the ITU AI for Good Global Summit.
Have you read?
How ethical, inclusive tech can help us create a better world, 9 ethical ai principles for organizations to follow, it’s time to change the debate around ai ethics. here's how.
IBM finds it worth its time and money to support these many initiatives for at least three reasons. First, IBM is committed to educating people and society about how technology and AI work. A better-educated society and especially better educated decision-makers are capable of making better decisions regarding the future role of AI in society.
Second, IBM wants to gather input from society about the ways AI is affecting the world. In this way, IBM can be more responsive towards the social impact of AI and act more quickly to prevent or react to harms. This helps to make sure that AI is truly delivering on its promise as a beneficial technology.
Third, IBM and its employees are committed to developing, deploying and using AI to benefit the world. By making sure that society is educated about AI and that tech companies know about the immediate impacts of AI on society, AI will be more likely to be directed towards good uses.
More and more people are taking a longer look at ethics and responsible innovation – and in many cases, they and their organizations are making these ideas an integral part of their corporate culture. All of these efforts contribute to making AI a better – and therefore more trustworthy – technology.
Looking ahead
Although IBM’s size and scale allow it to invest significant resources in these important areas, the lessons learned from IBM’s journey around the ethical use of AI technology are relevant to any organization. As companies evolve and grow, it is unfeasible for leaders to oversee every aspect of their business. Leaders must trust and empower their employees to make ethical decisions. Leaders need to provide the principles, guidelines, training, tools and support that will enable their employees to feel empowered and ready to handle ethical issues. Lastly, the overall purpose of responsible technology is to deliver positive impacts to society. The scale and scope of the impacts will vary by organization, but the goal towards improving the state of society should be consistent.
As more organizations share their experiences in adopting ethical technology practices, there is an opportunity to investigate the similarities and differences in their approaches. Over time, there might be ways to measure their successes and failures, while analyzing the paths that led them to such outcomes. This effort is in the hope that more organizations can learn from the experiences of others so that everyone is adopting methods and tools for the responsible use of technology.
Don't miss any update on this topic
Create a free account and access your personalized content collection with our latest publications and analyses.
License and Republishing
World Economic Forum articles may be republished in accordance with the Creative Commons Attribution-NonCommercial-NoDerivatives 4.0 International Public License, and in accordance with our Terms of Use.
The views expressed in this article are those of the author alone and not the World Economic Forum.
The Agenda .chakra .wef-n7bacu{margin-top:16px;margin-bottom:16px;line-height:1.388;font-weight:400;} Weekly
A weekly update of the most important issues driving the global agenda
.chakra .wef-1dtnjt5{display:-webkit-box;display:-webkit-flex;display:-ms-flexbox;display:flex;-webkit-align-items:center;-webkit-box-align:center;-ms-flex-align:center;align-items:center;-webkit-flex-wrap:wrap;-ms-flex-wrap:wrap;flex-wrap:wrap;} More on Artificial Intelligence .chakra .wef-17xejub{-webkit-flex:1;-ms-flex:1;flex:1;justify-self:stretch;-webkit-align-self:stretch;-ms-flex-item-align:stretch;align-self:stretch;} .chakra .wef-nr1rr4{display:-webkit-inline-box;display:-webkit-inline-flex;display:-ms-inline-flexbox;display:inline-flex;white-space:normal;vertical-align:middle;text-transform:uppercase;font-size:0.75rem;border-radius:0.25rem;font-weight:700;-webkit-align-items:center;-webkit-box-align:center;-ms-flex-align:center;align-items:center;line-height:1.2;-webkit-letter-spacing:1.25px;-moz-letter-spacing:1.25px;-ms-letter-spacing:1.25px;letter-spacing:1.25px;background:none;padding:0px;color:#B3B3B3;-webkit-box-decoration-break:clone;box-decoration-break:clone;-webkit-box-decoration-break:clone;}@media screen and (min-width:37.5rem){.chakra .wef-nr1rr4{font-size:0.875rem;}}@media screen and (min-width:56.5rem){.chakra .wef-nr1rr4{font-size:1rem;}} See all
UN and EU both agree new AI rules, and other digital technology stories you need to know
April 8, 2024
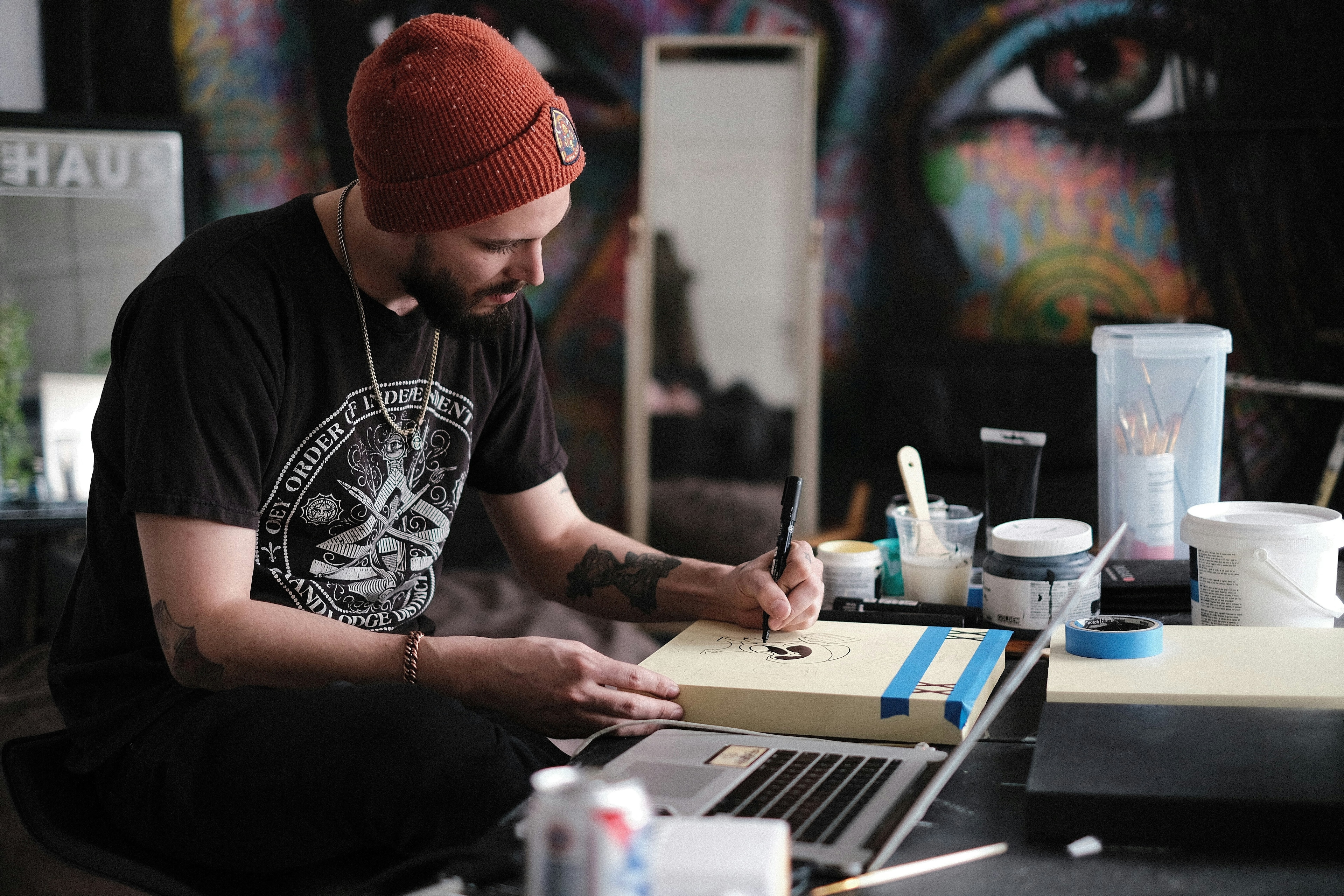
AI vs Art: Will AI help or hinder human expression?
Robin Pomeroy and Sophia Akram
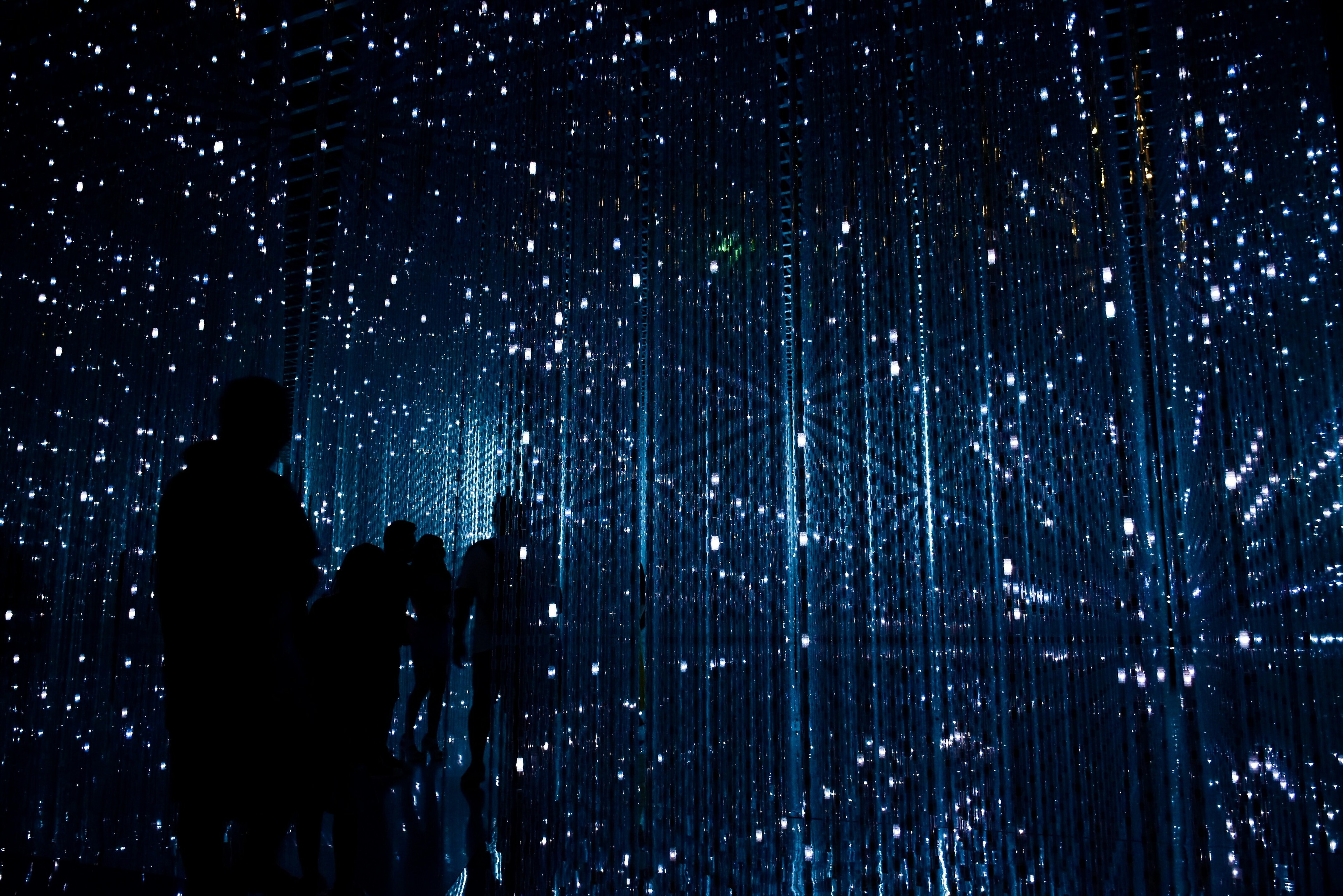
Weekend Reads: Funding AI’s future, imperfect environmentalists and Jane Goodall’s lessons on hope
Linda Lacina
April 5, 2024
To fully appreciate AI expectations, look to the trillions being invested
John Letzing
April 3, 2024
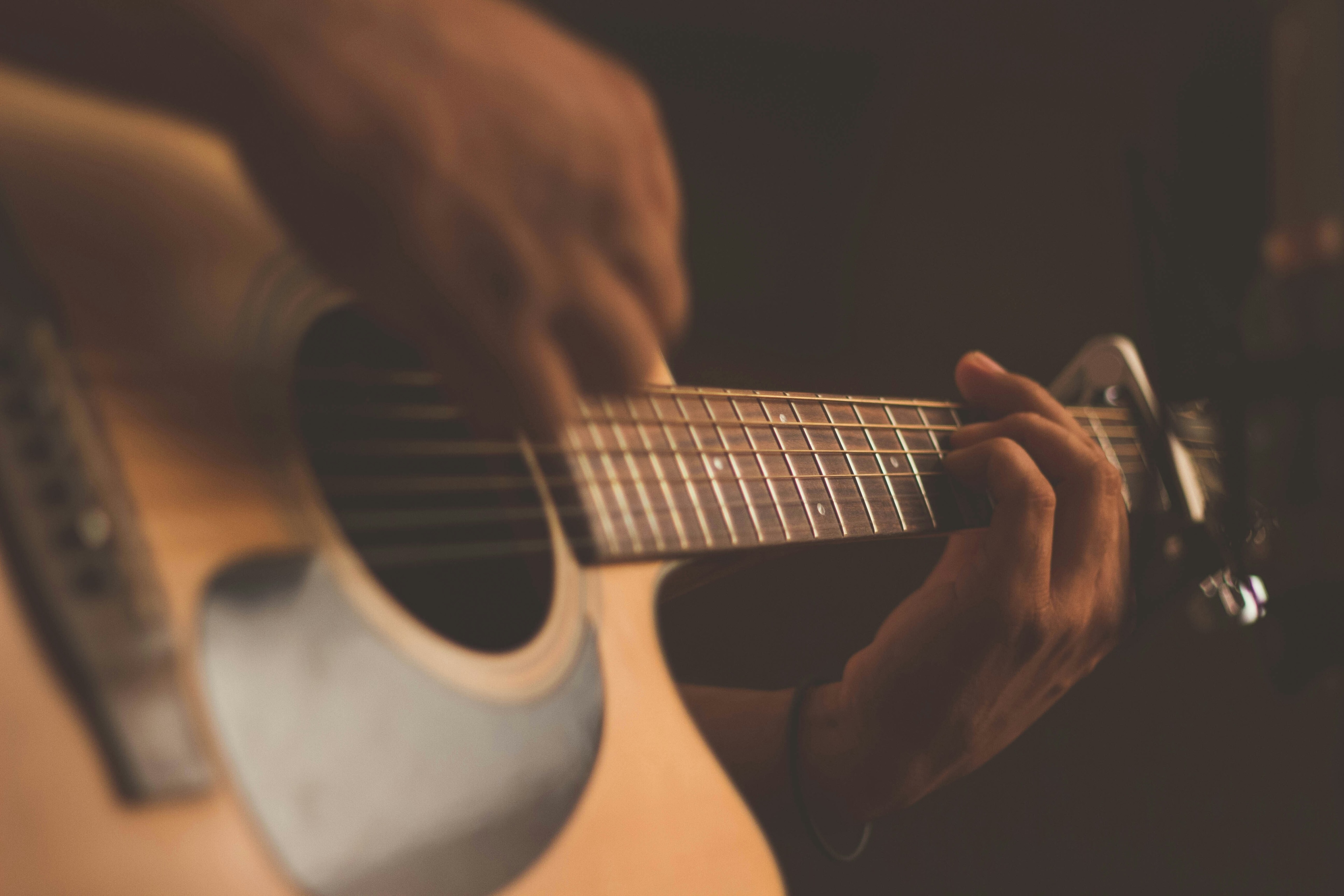
Can there be creative equity in the age of AI?
Faisal Kazim
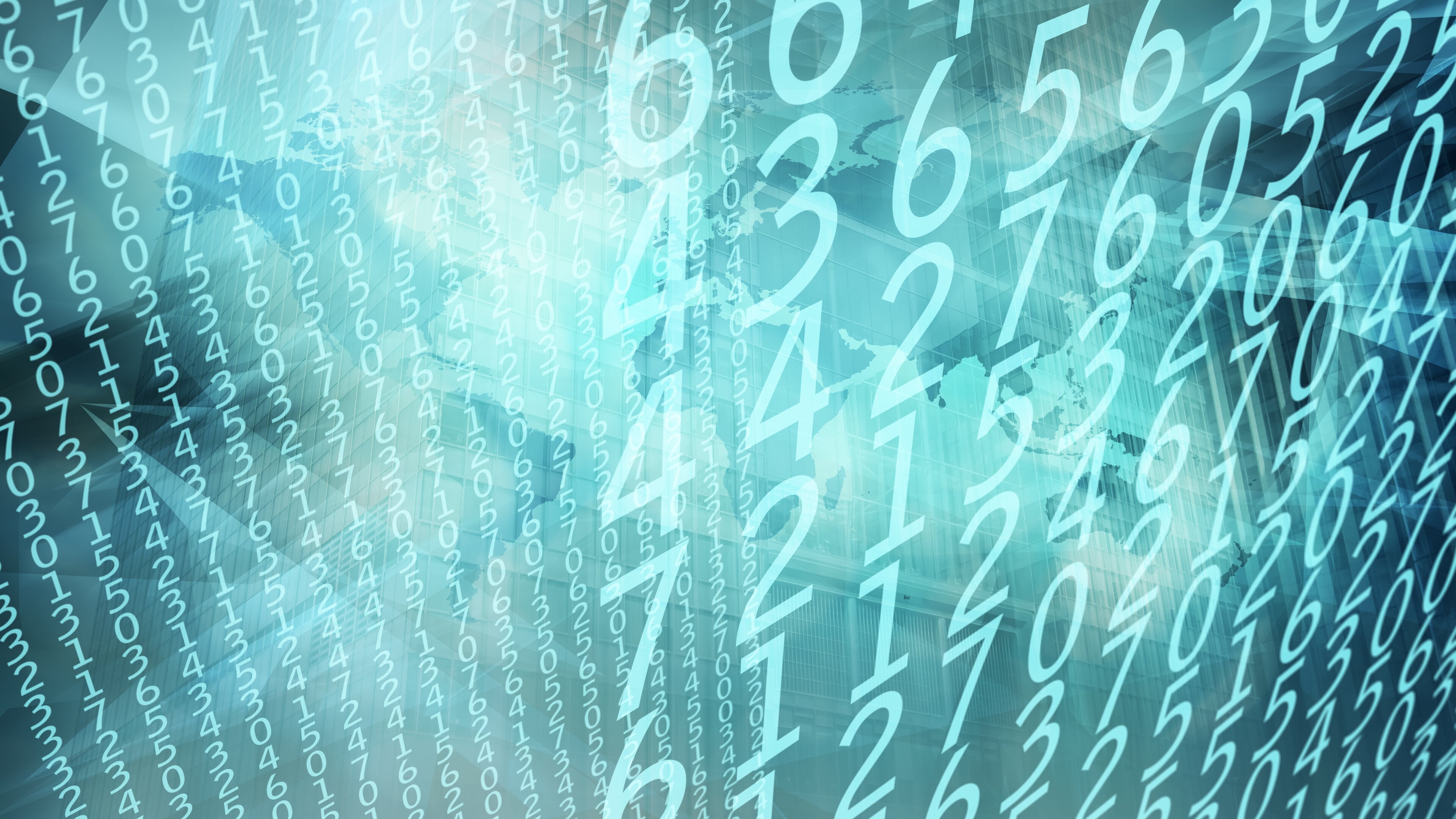
Building trust in AI means moving beyond black-box algorithms. Here's why
Eugenio Zuccarelli
April 2, 2024

Top 40 Most Popular Case Studies of 2021
Two cases about Hertz claimed top spots in 2021's Top 40 Most Popular Case Studies
Two cases on the uses of debt and equity at Hertz claimed top spots in the CRDT’s (Case Research and Development Team) 2021 top 40 review of cases.
Hertz (A) took the top spot. The case details the financial structure of the rental car company through the end of 2019. Hertz (B), which ranked third in CRDT’s list, describes the company’s struggles during the early part of the COVID pandemic and its eventual need to enter Chapter 11 bankruptcy.
The success of the Hertz cases was unprecedented for the top 40 list. Usually, cases take a number of years to gain popularity, but the Hertz cases claimed top spots in their first year of release. Hertz (A) also became the first ‘cooked’ case to top the annual review, as all of the other winners had been web-based ‘raw’ cases.
Besides introducing students to the complicated financing required to maintain an enormous fleet of cars, the Hertz cases also expanded the diversity of case protagonists. Kathyrn Marinello was the CEO of Hertz during this period and the CFO, Jamere Jackson is black.
Sandwiched between the two Hertz cases, Coffee 2016, a perennial best seller, finished second. “Glory, Glory, Man United!” a case about an English football team’s IPO made a surprise move to number four. Cases on search fund boards, the future of malls, Norway’s Sovereign Wealth fund, Prodigy Finance, the Mayo Clinic, and Cadbury rounded out the top ten.
Other year-end data for 2021 showed:
- Online “raw” case usage remained steady as compared to 2020 with over 35K users from 170 countries and all 50 U.S. states interacting with 196 cases.
- Fifty four percent of raw case users came from outside the U.S..
- The Yale School of Management (SOM) case study directory pages received over 160K page views from 177 countries with approximately a third originating in India followed by the U.S. and the Philippines.
- Twenty-six of the cases in the list are raw cases.
- A third of the cases feature a woman protagonist.
- Orders for Yale SOM case studies increased by almost 50% compared to 2020.
- The top 40 cases were supervised by 19 different Yale SOM faculty members, several supervising multiple cases.
CRDT compiled the Top 40 list by combining data from its case store, Google Analytics, and other measures of interest and adoption.
All of this year’s Top 40 cases are available for purchase from the Yale Management Media store .
And the Top 40 cases studies of 2021 are:
1. Hertz Global Holdings (A): Uses of Debt and Equity
2. Coffee 2016
3. Hertz Global Holdings (B): Uses of Debt and Equity 2020
4. Glory, Glory Man United!
5. Search Fund Company Boards: How CEOs Can Build Boards to Help Them Thrive
6. The Future of Malls: Was Decline Inevitable?
7. Strategy for Norway's Pension Fund Global
8. Prodigy Finance
9. Design at Mayo
10. Cadbury
11. City Hospital Emergency Room
13. Volkswagen
14. Marina Bay Sands
15. Shake Shack IPO
16. Mastercard
17. Netflix
18. Ant Financial
19. AXA: Creating the New CR Metrics
20. IBM Corporate Service Corps
21. Business Leadership in South Africa's 1994 Reforms
22. Alternative Meat Industry
23. Children's Premier
24. Khalil Tawil and Umi (A)
25. Palm Oil 2016
26. Teach For All: Designing a Global Network
27. What's Next? Search Fund Entrepreneurs Reflect on Life After Exit
28. Searching for a Search Fund Structure: A Student Takes a Tour of Various Options
30. Project Sammaan
31. Commonfund ESG
32. Polaroid
33. Connecticut Green Bank 2018: After the Raid
34. FieldFresh Foods
35. The Alibaba Group
36. 360 State Street: Real Options
37. Herman Miller
38. AgBiome
39. Nathan Cummings Foundation
40. Toyota 2010
A Systems View Across Time and Space
- Open access
- Published: 17 July 2023
New product development process and case studies for deep-tech academic research to commercialization
- Pravee Kruachottikul 1 , 2 ,
- Poomsiri Dumrongvute ORCID: orcid.org/0009-0009-7461-5888 3 ,
- Pinnaree Tea-makorn 4 ,
- Santhaya Kittikowit 5 &
- Arisara Amrapala 6
Journal of Innovation and Entrepreneurship volume 12 , Article number: 48 ( 2023 ) Cite this article
8048 Accesses
1 Citations
1 Altmetric
Metrics details
This research proposes a new product development (NPD) framework for innovation-driven deep-tech research to commercialization and tested it with three case studies of different exploitation methods. The proposed framework, called Augmented Stage-Gate, integrates the next-generation Agile Stage-Gate development process with lean startup and design thinking approaches. The framework consists of six stages and five gates and focuses on critical thinking to help entrepreneurs avoid psychological traps and make the right decisions. Early activities focus on scouting for potential socioeconomically impactful deep-tech research, developing a business case, market analysis, and strategy for problem–solution fit, and then, moving to a build–measure–learn activity with a validated learning feedback loop. Next, suitable exploitation methods are decided using weight factor analysis, developing intellectual property (IP) strategy, completing the university technology transfer process, and participating in fundraising. To pass each gate, the committee board members, consisting of tech, business, IP and regulatory, and domain experts, will evaluate the passing criteria to decide Go/No-Go. Applying the framework to the case studies results in successful university research commercialization. The model, case study, and lessons learned in this paper can be useful for other deep-tech incubator programs to successfully launch deep-tech research for commercialization. The case studies’ positive outcomes validate the Augmented Stage-Gate framework, yet their success is not entirely guaranteed due to external factors like regulatory constraints, entrepreneur characteristics, timing, and the necessary ecosystem or infrastructure, particularly in emerging markets. These factors should be taken into account for future research purposes.
Introduction
Deep-tech innovation is a new wave of impactful innovation that drives the economy and society. Unlike digital innovations such as mobile apps and digital platforms that disrupted many old-fashioned businesses in past decades, deep-tech is unique, high-value, hard-to-reproduce technological or scientific advances that will improve the technological frontier or disrupt existing solutions and result in socio-economic impacts (De la Tour et al., 2017 ). Deep-tech innovation is usually led by megatrends and unmet needs (Linden & Fenn, 2003 ).
Thailand, a developing country, relies heavily on traditional businesses such as sales, marketing, and services. Thailand’s gross expenditure on R&D (GERD) is lower than that of other middle-to-high income countries. In 2018, Thailand spent 1.11% of gross domestic product (GDP) (182 billion baht) compared with an average of 1.41% for the upper-middle-income group and 2.43% for high income countries. GERD was expected to reach 2% of GPD in 2027 but this was revised to 1.46% due to the COVID-19 pandemic, assuming no new measures to boost R&D investment. Nevertheless, various government policies require stimulus to R&D spending, especially for SMEs and innovation-driven enterprises through the Thai Bay-Dole Act (Office of National Higher Education Science Research and Innovation Policy Council, 2021 ). Therefore, deep-tech innovation applied to Thai businesses could be a potent new driver for its economy. Since most deep-tech originates from academia, researchers, patents, or publications, it is unlikely to be successful and sustainable without real demand from users or direction from the business side. This is because traditional academia focuses heavily on research, publication, and prototype development (Fellnhofer, 2016 ), rather than building a product that is ready for commercial use (Hicks et al., 2009 ). Promoting entrepreneurship, which is a combination of art and process to pursue opportunities and turn into a business regardless of resources, among academia can be helpful to create environments that support innovation development (Barringer & Ireland, 2012 ).
Moreover, many deep-tech innovations require a large amount of funding at the initial stage to build a prototype, perform user validation, and develop a business strategy. Additionally, deep-tech innovation is new, and the industry may not be clear about market needs or potential buyers. Therefore, the technology acceptance model (TAM) is used to understand predictors of human behavior toward potential acceptance or rejection of the technology, particularly technologies related to information and communication technology (ICT) (Lee et al., 2003 ). It can also provide a useful tool to assess the success of new technology introductions and help understand the drivers of acceptance to proactively design interventions targeted at users that may be less inclined to adopt new systems (Venkatesh et al., 2003 ). After validating the market and technology, it is time to decide on commercialization options (Yaldiz & Bailey, 2019 ).
For deep-tech innovation to become successful exploitation from the research ideation stage until commercialization, it requires a product development model suitable for university research initiation and developing market environment. Meanwhile, many pieces of prior research on the NPD model and case studies were primarily conducted based on developed countries where the product development was done within the established company ecosystem (Cocchi et al., 2021 ; Cooper, 2016 ; Cooper & Sommer, 2016 , 2018 ; Salvato & Laplume, 2020 ; Walrave et al., 2022 ; Wuest et al., 2014 ). However, this study highlighted the importance of a specific NPD model in the academic initiative context with low resources and a lack of infrastructure setting, which generally happens within developing countries (Ravi & Janodia, 2022a ). This study is essential to promote deep-tech in Thailand and to help other developing countries that require a new growth potential to drive the economy. Consequently, to accelerate deep-tech innovation in Thailand, the Chulalongkorn University Technology Center (UTC) was established in 2019 as a platform to spring-board academic research to commercialization and facilitate among stakeholders within the ecosystem based on triple helix model, which promotes the way of working that the government, private sector, and academia must collaborate to form a solid, deep-tech innovation ecosystem (Leydesdorff & Etzkowitz, 1998 ) to support manpower, finance, know-how, production facilities, regulation, and sandbox testing in order to expedite the speed of innovation development.
This study uses qualitative research and observation based on the actual case studies of the UTC portfolio research teams. The goal is to understand the pain points, needs, obstacles, and processes required for the successful exploitation of their project and then extract the vital insightful factors for applying to the NPD model, which will be later discussed in the Methods section.
To develop the proposed NPD model, several related NPD studies have been reviewed. Then the next-generation stage-gate development system integrated with agile development, lean startup, and design thinking methods is selected and then applied together with the insights obtained from qualitative research as the NPD model to develop successful business-driven deep-tech innovation. The effectiveness of the model is later tested and confirmed using both experts and observation, which will be later described further in the Results section. This framework, which we call the Augmented Stage-Gate framework, is important for successful innovation and is based on critical thinking. Because human decisions are influenced by the subconscious, it is essential to make decisions based on the results of logical reasoning and avoid psychological traps (Linden & Fenn, 2003 ).
In addition, three case studies are explained and discussed. Applying the Augmented Stage-Gate framework results in successful commercialization process in all three cases where the teams transferred the technology via a spin-off startup with a patent, non-profit use with trade secret, and licensing. The benefits of this study can be used as a framework and case study for successful deep-tech innovation development and commercialization, especially in the context of developing markets and academic research initiation. Several options are proposed and discussed. Finally, the study makes several recommendations for future research, including its application to other vertical deep-tech innovation areas.
Literature review
In this section, the literature on the NPD model, TAM model, and product readiness assessment is discussed. Generally, the NPD model, is a nonlinear and iterative process based on a problem-solving approach that is used for the conception, development, and launch of new products or services. It can help management understand user insights, challenge assumptions, redefine problems, and create innovative solutions to prototype and test with target users to successfully launch in the market. In addition, the NPD process is based on critical thinking, which is the ability to look at events, conditions, or thoughts with a careful eye and make decisions about the reliability and validity of the knowledge according to standards of logic (Seferoglu & Akbiyik, 2006 ). It involves identifying and analyzing informational sources for credibility, indicating previous knowledge, making connections, and deducing conclusions (Thurman, 2009 ). Higher-order thinking ability provides the opportunity to analyze the existing knowledge or situation to correct mistakes and complete deficits to reach correct conclusions (Howard et al., 2015 ). In this study, the authors select Stage-Gate, which is a macro idea-to-launch product development planning process that involves the Go/No-Go decision-making (Cooper & Kleinschmidt, 2001 ), as the baseline NPD framework because the model is easy to understand among stakeholders in a simple linear system format that consists of detailed guidelines for every stage and explains the criteria for management to make a decision whether to allow the development to pass each gate. These unique characteristics of Stage-Gate model strongly fit within the context of our study. While its principles can be applied, the Stage-Gate model, including the number of stages, activities, and gate criteria, has to be adjusted according to our objectives using the insights obtained from this study.
After the core concept of Stage-Gate model was chosen, several modern State-Gate models were reviewed. The next-generation Stage-Gate process that comes with the Triple A system and spiral concept that promotes the development process to be adaptive, flexible, iterative, and accelerated using a feedback loop from user validation (Cooper, 2016 ) can be applied to the model. Furthermore, there was a study of applying Agile project management methods, which highlights a process that is a dynamic planning process that is adaptive and flexible to changes in product development, into a traditional Stage-Gate system, called Agile-Stage-Gate Hybrids. The results looked promising for faster product releases, quicker and better responses to changing customer requirements, and improved team communication and morale (Cooper, 2016 ). Moreover, case studies in manufacturers conducted by R. Cooper in 2018 also supported the earlier finding; yet it also added some challenges in terms of management buy-in, resources needed and allocation, and fluid product definitions and development plans (Cooper & Sommer, 2018 ). These insights are also similar to the study by Zasa et al. ( 2020 ) who highlighted that agile project management will increase interaction among project stakeholders and help break big tasks into small and achievable action items (called sprints ) within a short period of time. They also suggested that successful implementation required the integration between traditional project planning modes and the agile method, cultural change, and perceptions of all stakeholders in the organization (Zasa et al., 2020 ).
Therefore, by applying modern concepts of Stage-Gate like triple A system with spiral concept and agile development, the earlier Stage-Gate baseline model can be improved in many ways. That is, the model becomes more adaptive and flexible to changing customer requirements and situations, increasingly improved team communication and morale, and further highlights on an iterative process to promote interfacing between the development team and the target user. Moreover, the importance of interfacing with users iteratively for business assumption validation is also similar to the principle of lean startup and design thinking. The lean startup encourages startups to challenge business growth hypotheses and use them to build the minimal viable product (MVP), then test and validate with the real user to learn whether it is required to pivot or preserve. This can be repeated many times during the NPD process; an approach called build–measure–learn (Ries, 2011 ). On the other hand, design thinking uses a designer’s sensibility and methods to match people’s needs to what is technologically feasible and a viable business strategy that can be converted into customer value and market opportunity (Brown, 2008 ).
In addition, the TAM can be useful to consider during the NPD process, in particular with ICT-related technologies. It can provide information regarding the probability of success during the introduction of a new technology and the key drivers of user acceptance to enable proactively designed interventions and strategies targeted at populations of users who may not be inclined to adopt new systems (Venkatesh et al., 2003 ).
Lastly, the authors review the study of product readiness assessment. This is important for our context because there is a misalignment issue from different stakeholders when evaluating the readiness of the new product development. This is a typical problem found when the product is not ready for commercial. Yet the team has to communicate readiness level with stakeholders for different purposes such as fundraising, selling, field testing, etc. The first assessment is the technology readiness level (TRL) which was introduced by the National Aeronautics and Space Administration (NASA) in the 1970s. It is a well-recognized and useful tool to determine the maturity of new technologies. It is also a discipline-independent program that enables more effective assessment and communication. Its nine assessment levels are beneficial to determine the readiness of new technology and/or capability during the technology life cycle, which includes the completion of systems analysis and conceptual design studies, determination from several design options, and decision to start full-scale development (Mankins, 2009 ). Another assessment is the investment readiness level (IRL) proposed by Steve Blank in 2013, which is also divided into nine levels. IRL is used to evaluate how investment-ready a technology is by validating its business model to help investors assess the risk of investment (Blank, 2014 ). Investment readiness can be defined as a set of business development processes that increase business venture readiness as candidates for equity investors (Aernoudt et al., 2007 ). Alternatively, it is the capacity of the business venture to look for external funding, especially from an equity investor, to understand the specific needs required by an investor and be able to give an investor an attractive business proposal with high confidence (European Commission, 2006 ). Entrepreneurs need information and advice on the advantages of raising equity financing, what it means, and how to become investment-ready (Mason & Kwok, 2010 ). In addition, Australia National Investment Council. & Marsden Jacob Associates ( 1995 ) proposed that businesses that are not investment-ready are primarily the result of a lack of information. This means that they do not know about the role of equity finance and are unaware of what is involved in raising money, what is required to attract investors, and how to convincingly express their investment proposals (Australia National Investment Council. & Marsden Jacob Associates., 1995 ).
In this research, the authors use the next-generation stage-gate process as the baseline for the NPD process and then propose the modified NPD framework for new deep technologies that are more suitable for academic research initiation to commercialization in developing markets, called the Augmented Stage-Gate framework. The framework was designed using the insights obtained from in-depth interviews of 19 research teams who had been working on deep tech research and entered the three-month entrepreneurship development program in 2019. The interview was conducted at the end of the program and focused on understanding the pain points in the research-to-commercialization process in terms of entrepreneurship, business development, networking, financial, technology transfer process, progress assessment, and goal. After careful analysis, several recommendations were proposed and integrated into the Augmented Stage-Gate framework as shown in Table 1 .
The Augmented Stage-Gate framework highlights more on the Agile development process, flexible entrepreneurial development program, progress assessment using TRL and IRL, process management specialist to guide along the academic research to commercialization journey and bring in a network of business partners and legal experts to support. Its structure is divided into six stages (innovation ideation, build business case, development, test and validation, launch, and scale-up) with five gates (screening, go to development, go to test, go to commercial, and post-launch review). Here, stage means the process for work to be completed, and gate is for the Go or No-Go decision-making. TRL and IRL assessments, as shown in Table 2 , can be used to evaluate progress in terms of technology and business readiness at each stage.
The Augmented Stage-Gate framework applies the principle of the next-generation Stage-Gate’s triple A system and spiral development, which aims to overcome the typical challenges when handling undefined requirements during initial development, and Agile development, which aims to increase interaction among project stakeholders and help break big tasks into small and achievable action items (Sprints). This is because most customers are uncertain about their needs and so the product definition prior to development is unclear. The triple A model promotes each stage to be adaptive and flexible, agile, and accelerated while the spiral development concept promotes experimentation. This is also similar to what Isaacson ( 2011 ) described Steve Jobs’ philosophy during his development career at Apple that encouraged project teams to fail often, fail quickly, and fail cheaply. With the benefits obtained from the Augmented Stage-Gate core concept, the product design and definition can adapt to new information, customer feedback, and changing conditions along with multiple iterations of validation activities with users or customers throughout the NPD cycle. In addition, it is important to understand that the details of the process and its functions may differ from project to project, especially with deep tech, academic research initiative, and emerging market environment. Therefore, a flexible gating process must be leaner, faster, adaptive, and risk based. Experienced project teams, mentors, and stage-gate committees are also important to guide startup work throughout the NPD process. Additionally, even though the NPD model is represented in a simple linear format, in reality, it is common that each step can be repeated many times and also go back and forth between stages, depending on the readiness, criteria, and requirement to pass each stage.
Then the effectiveness of the Augmented Stage-Gate framework was tested with three cases, to be discussed in Sect. 4. The cases were research teams that joined UTC in 2019 after the new framework had been designed and completed the final stage of the framework by September 2022. The teams were willing to participate in the study. We gathered the information for the cases via observations and interviews.
The authors directly observed the teams as they moved through each stage of the framework. Tangible results such as actual sales, contract execution, regulatory approval, and certifications, were recorded. The authors also had access to relevant documents related to the development process since the teams were required to submit a progress checklist and presentation slides. Information reported (as appropriate to each stage) includes team, research and development progress, regulatory process, business plan, project planning and concept, product design, milestones, risk assessment, technology verification and validation (MVP), market validation, legal activities, IP status, implementation and operations, sales and marketing, and financial activities. These documents were collected and analyzed for the case studies.
In addition to observation, the authors interviewed the stage-gate committees and two or three people from each team (the principal investigator and 1–2 team members). The interviewees were asked to describe the team’s journey, how they applied the Augmented-Stage-Gate framework, and the results they achieved. The interviews also explored any significant challenges encountered during implementation, along with the solutions that the teams developed.
The interviews were recorded and transcribed, with the transcriptions used to create a final summary of the case. The summary was then reviewed and approved by the interviewees. In some cases, we went back to the interviewees multiple times to get additional information or to conduct follow-up interviews when the implementation and results had become clearer.
The Augmented Stage-Gate process of new product development
The proposed Augmented Stage-Gate process, as shown in Fig. 1 , is divided into six stages. In addition, the below detail explains the objective, activity, and criteria to pass the gate of each stage (as also summarized in Table 3 ).
Stage 0: innovation ideation stage. As a technology incubation office, one of the important roles at UTC is to search for impactful deep-tech research in focused areas that potentially impact our way of life and attitudes in all aspects. To achieve this, UTC has been working with various business partners and consultants to gain market insights while studying market research information for mega trends. Using this information, UTC scouts, classifies, and prioritizes potential research projects. After finding candidates, UTC works closely with them through various programs such as boot camp, workshop, and mentoring to develop the entrepreneurial knowledge and skill in order to help conduct an initial business feasibility study. Another advantage is to give entrepreneurs an understanding of the business journey, challenges, and exit plan so that they can prepare themselves with both skills and morale to be ready before launching. Moreover, the entrepreneurial development program is provided in a flexible format both online and offline to suit with the availability of researchers who might have other full-time jobs at the beginning. Usually, the business model canvas (Osterwalder et al., 2005 ), with its nine building blocks template, is used to communicate a firm’s or product’s value proposition, infrastructure, customers, and finances to stakeholders. After completion, the team is ready for the official screening, where the committee board consisting of business, technology, and legal experts will evaluate each research project.
The first step is to identify the target customer and study the user journey to understand the pain points and user insights. Additionally, lead users—advanced users who deal with an individual problem very intensively (von Hippel, 1986 )—are a subset of target users and can be helpful for the research team to test, validate, and gain valuable feedback on the early development product. Like design thinking, the concept starts with understanding the way customers do things and why, their physical and emotional needs, how they think about the world, and what is meaningful to them. This can be done by carefully observing, engaging, watching, and listening to the users and stakeholders, and then crafting a meaningful and actionable problem statement that focuses on the insights and needs (Brown, 2008 ).
The second step is to analyze internal and external market data. This process aims to understand the business environment and will allow us to better plan so that the threats and opportunities associated with the target area of the business are understood. An internal analysis examines factors within the research project and its co-founding team. The preferred analysis is a SWOT (Strength, Weakness, Opportunity, Threat). Meanwhile, an external analysis examines the wider business environment outside the research project. A popular tool for this is the PESTEL five-force analysis. The key to this process is to ensure that there is market demand to continue the tech-market fit development process.
The third step is to complete an initial financial management strategy, including profit and loss analysis, cash flow planning, and fundraising, that can help the entrepreneur understand the business from a financial perspective in different scenarios and help the business thrive. Because deep-tech product development usually requires a large amount of money and lengthy development time, careful planning in this step is much cheaper regarding business risk. It can avoid cash flow issues that may cause the company to go bankrupt or project delays. Moreover, financial planning can be used to estimate how much investment is needed in each venture development stage so that the entrepreneur can develop a successful fundraising strategy for investors or government grant agencies.
The next step is a preliminary study of the IP landscape. This gives the research project a high-level perspective on the constraints and opportunities regarding the potential exploitation and freedom to operate of IP rights. The researchers can conduct this by themselves or consult with the university IP office since normally the university provides IP support through its Technology Transfer Office (TTO) and IP Practicum Clinic, or by outsourcing services to specialized law firms.
After that, it is time for regulatory planning to help the research team understand and anticipate what regulations are required for each target market. For instance, Med Tech requires FDA (Food and Drug Administration) for commercialization, IRB (Institutional Review Board) for conducting a clinical trial in humans, and GMP (Good Manufacturing Practice) for manufacturing medical devices. Meanwhile, the PDPA (Personal Data Protection Act) is required to use personal data. Generally, the university technology office can be a helpful resource for regulatory advice.
Finally, since deep-tech initiates from academic research by nature, the original research team usually consists mainly of tech-savvy people. Therefore, to become a successful venture, it is crucial to find co-founders with business skills to join the team. Business case competitions or networking events within the university ecosystem can help form an organic partnership.
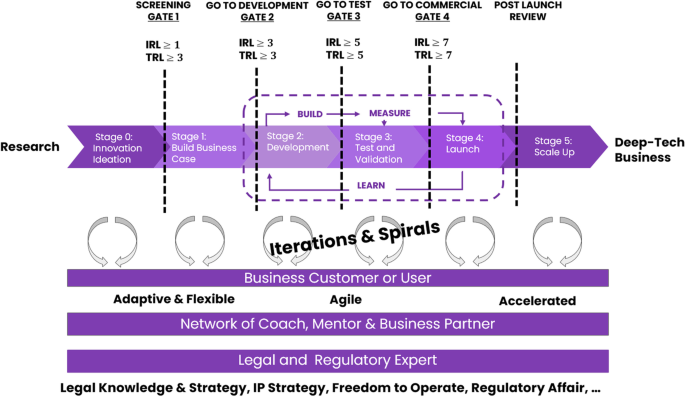
Augmented Stage-Gate framework
Stage 1: build business case stage. The main activity focuses on developing and validating the business model with target users by demonstrating the prototype and then measuring customer satisfaction, interest, or purchase intent. Usually, the prototype in this stage can be nonfunctional and developed based on the concepts of rapid, rough, and right. For example, AI and computer science technology can use UX/UI (user experience and user interface) and wireframe, which is a schematic or blueprint that is useful for thinking and communicating about the software structure among team members, as a prototype to validate the end-to-end solution idea with the user. Moreover, a network of mentors, domain experts, or key opinion leaders, which are mostly university alumni, can be useful resource because they are knowledgeable and experienced, in which they can give truthful advice and validate the solution idea. Another important thing is to interact with real users or customers as early as possible because today users’ roles have become more significant as a new source of innovation than in the past, when innovation was created solely from producers and supplied to consumers via goods and services, as described in Schumpeter’s theory of innovation in 1934 (Schumpeter, 1934 ). By working together, the research team can provide product knowledge, engineering, and manufacturing for innovative users to think and be creative (von Hippel, 1976 ), which means innovators receive an incentive to engage with users to develop innovative designs (Baldwin & von Hippel, 2011 ).
Stage 2: development stage. The main objective in this stage is to develop a workable and functional MVP, validate with the target user, and refine the business model. That is, it aims to improve technology progress and business strategy so that business risk can be reduced. However, it is noted that due to the Agile concept, the startup should target to break the development plan into small and achievable action items so that their hypothesis can be tested and learned often. In addition, validating the MVP in the closest real environment or sandbox, which refers to the environment that allows some players under specific conditions, to enter the market with fewer administrative constraints (e.g., licenses) or legislative requirements (Tsai et al., 2020 ), is recommended to move the MVP and business closer to the commercial version.
Stage 3: test and validation stage. The goal in this stage is to obtain a commercial version of the MVP and business model. To do that, the lean startup’s validated learning concept is applied to this stage because it can show whether the innovation development and business are moving in the correct direction according to the business model. If not, the innovation can be pivoted; a structural course correction to test a new fundamental hypothesis about the product, strategy, and engine of growth. To make the validated learning successful, cause-and-effect questions with actionable and quantitative metrics are essential. After the new features of the MVP are developed, it will be measured with the user to determine if it demonstrates business growth according to the underlying hypothesis, a process can be repeated many times. The benefit of embracing validated learning is to substantially shorten the developmental cycle.
Stage 4: launch stage. The main goal for this stage is to introduce the market of commercial products. The technology development team participates in a build–measure–learn activity to reach the closest version of a commercial product, while the business development team focuses on delivering a commercial final version of the business plan, sales and marketing strategy, IP strategy, regulatory planning, team formation and financial strategy to select the best commercial option with the highest probability of success and return on investment. In addition, if the university IP is used, the team must complete the technology transfer process. Moreover, according to the business model canvas template, this step must ensure that all nine blocks are validated with stakeholders in a way that leads to business growth and the commercial version of the MVP is refined accordingly. The next step is to finalize the IP submission and strategy, consisting of the final IP draft, valuation, and portfolio management, to obtain optimal legal protection and manage the IP effectively. IP valuation, calculated using either cost-based, income-based, or market-based methods, is useful for the entrepreneur to decide on a proper commercialization option and IP valuation for fundraising. Thus, it should be finalized before going to market. Even though IP services can be particularly expensive and time consuming for such early-stage endeavors, the benefit obtained from IP valuation and protection with a well-managed IP strategy generally increases company competitive advantages tremendously after successful exploitation.
The university technology transfer process is an intrinsic part of the technological innovation process. It is the process of conveying results stemming from scientific and technological research to the marketplace and to the wider society along with associated skills and procedures. To achieve a successful technological transfer, many factors must be considered. Souder et al. ( 1990 ) described seven best practices as analytical, facilities, pro-actions, people roles, conditions, technology quality, and organization. Meanwhile, Gorschek et al., ( 2006 ) recommended close cooperation and collaboration between researchers and practitioners. However, both entrepreneurs and tech transfer officers must discuss and plan each option carefully for the benefit of all stakeholders.
After completing the previous steps, it is time to decide on commercialization. Exploiting an innovation is not only about starting a new company, but there are also many other pathways to bring ideas to markets, such as licensing, joint ventures, and M&A (Schaufeld, 2015 ). Thus, to choose which option is suitable, the entrepreneur needs to consider factors such as market opportunity, IP protection, operation risk, time commitment, return on investment, and investment amount. A complete business plan should be developed and carefully verified, so that entrepreneurs can understand the business opportunities and risks in advance. Table 4 shows an example of an option comparison with a weight matrix between spin-offs and licenses. Briefly, the Option A spin-off scores higher than the Option B license, which means it is the more desirable commercial option to an entrepreneur.
Stage 5: scale-up. This activity focuses on collecting and analyzing the feedback obtained after launch, providing newer and better versions of commercial products or business plans using market feedback, and fully penetrating the target market. Several considerations can be analyzed. The first is to assess whether the product is performing according to pre-defined expectations in terms of technical and business aspects such as functionality, revenues, costs, profits, and so on. The second is to check customer satisfaction or anything that affects the company’s value chain, including purchasing raw material, selling the product, and delivering the goods to the customer. Finally, we examine the strengths and weaknesses of the entire NPD process to learn and improve.
Results and discussion
Case studies.
The case studies below highlight the importance of having an NPD framework that is adaptable to deep-tech within university research and emerging market contexts, yet extensive enough to cover all the essential components to transform deep-tech research into an innovation that has a high-fidelity MVP, an accomplished business and market strategy, a clear pathway towards implementation in the real world, and a complete IP strategy and technology transfer process from academia IP.
ReadMe is an artificial intelligence (AI) research project application that began in 2013 to perform Thai object character recognition (OCR) in any scene image, which often has high perspective and distortion error, uneven illumination, and different image resolutions. Additionally, the Thai character structure itself is very difficult to read automatically, particularly using software algorithms, because it consists of a syntactic structure of up to four layers and a strict relationship between words. The research team was conducting research and development internally and working with various industry partners. An e-commerce platform and a railway engineering company were contracted to help understand business demand as well as to improve and optimize the AI model for real-world applications. Nevertheless, after many years the technology remained a research project; early customers did not have purchase intent with a long-term commitment although the Thai OCR reading accuracy was high. Upon applying our Augmented Stage-Gate Framework to ReadMe in 2019, we successfully transformed the deep-tech research into a tech startup named Eikonnex AI ( https://www.eikonnex.ai/ ) that has now secured business deals for commercial use in private companies.
At the screening stage, the project’s potential for exploitation, validity, market feasibility, and technological feasibility was assessed and found to fulfill all the framework’s criteria. ReadMe, a national award-winning research project, was a deep-tech text reader that was in development for six years, had a research prototype proven well in the lab with a TRL of 4 and an IRL of 1, was the state-of-the-art Thai text reader that was more accurate than other better-known OCR technologies, and is a high-potential technology that could impact the business, medical, and transport industries.
Following their selection, the research team carried out innovation framework activities starting with continuous customer validation, that later helped them develop their market research and business plans. A large majority of their customers were banks, driven by the digital transformation trend and strong competition in the financial industry. One of the most challenging and high-volume applications is the personal loan approval credit scoring. Most were unable to automatically read Thai bank statements correctly due to statement template differences from different banks and Thai character challenges, increasing the time required for loan approval. The team saw this opportunity and pivoted their target customer and core technology to become an OCR with automatic template detection to read bank statements instead. After this decision, the team quickly redeveloped their MVP and carried out multiple user validations using the build–measure–learn process. In the meantime, the team worked closely with a network of mentors to adjust and validate the product idea and business plan.
After rigorously applying the framework’s validation activities, the technology underwent a complete transformation and reached commercial readiness. The technology now had a TRL of 7 and an IRL of 7, completed the IP strategy by obtaining a patent for their technique, concluded the technology transfer process, and set up a spin-off tech startup. Moreover, in early 2021 a few months after their establishment as a startup, the company received its first business deal from one of the biggest banks and completed the technology transfer process. Currently, the company is making its first sales by providing Thai document reader solution services either as an API or as a customized technology. They will continue to move towards digital transformation and expand into a coherent document digitization platform.
It is clear that with the support, guidance, and structure provided by the Augmented Stage-Gate Framework as explained in Table 5 , deep-tech research can be transformed into an innovative, high-impact, commercializable product and company in one to two years.
Chest X-ray AI reporter for COVID-19
Following the trend in the use of AI for healthcare, the chest X-ray reporter was an R&D project by physicians and computational researchers that aimed to create AI software that could classify and report abnormalities for physicians to consider as part of their diagnosis. Nonetheless, the technology remained a research project as it lacked a workforce to develop the complete application software and system integration and had no exit strategy.
With the application of our framework and the outbreak of the coronavirus (COVID-19) pandemic, the technology met the immediate needs of society by being able to detect COVID-19 and numerous other conditions from chest X-rays. As of the end of 2021, this innovation was used as a not-for-profit technology in the King Chulalongkorn Memorial Hospital, helping many patients in need.
The technology had a TRL of three and an IRL of one at the time of screening with an alpha version of the AI algorithm. As this project is led by physicians and computational researchers who are experts in the field, it is considered a deep technology with high potential for use in hospitals, especially rural government hospitals that sometimes lack healthcare personnel or technology to analyze chest X-rays efficiently. This innovation may also be adapted for use in other types of X-rays for other diseases and undoubtedly has large potential to improve the accuracy of medical diagnosis. Thus, this research is a good candidate for our Augmented Stage-Gate framework as explained in Table 6 .
Following the development and validation activities of our framework, the research team recruited more AI engineers to develop their algorithms and UX/UI to enable intuitive use of the technology. Here, the code and interface were continuously revised with frequent customer and domain expert validations to select the most relevant features and data for physicians. To protect intellectual property, the technique was kept a trade secret. After using the framework for only one year, the work reached a TRL level of 7 and an IRL level of 7 and gained acceptance for not-for-profit use in the hospital for preliminary screening of COVID-19 and other chest X-ray abnormalities. At present, the innovation is used at Chulalongkorn Hospital. We believe that, with its initial success, the technology can be implemented in other hospitals to help improve patients’ quality of life. The project team is now involved in the process of technology transfer and spin-off.
Progesterone test kit
The progesterone test kit for swine is a medical technology that began with a contracted research project between the Chulalongkorn University Faculty of Veterinary Medicine and a multinational science and technology company. The research team has in-depth knowledge and IP for developing a test kit that can easily test the progesterone level of animals from serum samples. In this research, the industry partner wanted to detect swine progesterone in the form of a strip test as it is a cheap and convenient method for mass adoption. The company promised to license the technology for sales and marketing purposes after the prototype showed promising results.
This research project has a potentially high impact on the local livestock industry. It is a new state-of-the-art technology and is an easy, effective, and low-cost solution that addresses many pain points faced by the swine farm industry. Moreover, we foresaw that the technology could be adapted to detect other hormones and health- or disease-related biomolecules in other livestock, increasing the market size and potential customers in the future. Finally, the initial readiness assessment revealed a TRL of 6 and an IRL of 1.
With our Augmented Stage-Gate framework, as explained in Table 7 , and business directions from the industry partner, the project established its market and business strategy and financial analysis. Moreover, the project team also brought in the qualified diagnostic development (QDD) center of Chulalongkorn University to support strip test design and small-scale manufacturing. Furthermore, with continuous iterations of customer validation, the researchers were able to fit the technology to the user’s needs and better understand the type of collaboration the industry was looking for. Thus, the team had business matching opportunities and discussed plausible deals with potential customers.
After more than 6 months of fine-tuning all aspects of the innovation, the project had a TRL of 7 and an IRL of 7 with a final prototype and licensed their technology to an international company that will use the kit for real-world applications. With the success of their first deal, the team has leverage to make future deals with other private companies.
The Augmented Stage-Gate Framework was used in these cases to validate the potential for exploitation, validity, market feasibility, and technological feasibility. All projects had low levels of investment readiness and different levels of technological readiness at the time of screening but were all considered deep technologies with high potential for use in their respective industries. The framework helped the teams carry out innovation framework activities, including continuous customer validation, market research, and business plans. All projects underwent a complete transformation after rigorously applying the framework’s validation activities, which included developing their MVP, carrying out multiple user validations, and adjusting their product idea and business plan with a network of mentors. In terms of commercial success, ReadMe successfully transformed into a tech startup named Eikonnex AI and secured business deals for commercial use in private companies. Chest X-ray AI Reporter for COVID-19 remained a not-for-profit technology used in King Chulalongkorn Memorial Hospital to detect COVID-19 and other chest X-ray abnormalities. Progesterone Test Kit licensed their technology to an international company. It is shown that the Augmented Stage-Gate Framework effectively transformed research projects into innovative, high-impact, commercialized products and companies.
Past literature has mentioned that traditional Stage-Gate models are not suitable for many of today’s businesses due to fast-changing user needs, uncertain market requirements (Cooper & Sommer, 2018 ), or industry complexity that requires highly iterative cycles and external collaboration (Sommer et al., 2015) and requires a more flexible and adaptive Stage-Gate model such as integrating agile process (Cocchi et al., 2021 ). Case studies leveraging these models were mostly conducted in corporates in developed economies. Directly adopting successful models from developed countries’ academic institutions require a well-established technology transfer office (Ravi & Janodia, 2022b ). Other studies that focus on the academic context in developing countries made suggestions in the policy level, recommending that the government encourage technology transfer by connecting industry and academia (Kirby & El Hadidi, 2019 ; Ravi & Janodia, 2022b ). None has given practical, step-by-step guideline model for technology initiated from academic institutions like ours.
Therefore, our work provides the first proved example of a new product development model that can be applied in similar contexts—commercializing university technology in an emerging economy. It solves the problems that persist in developing countries, Thailand especially, of lack of literature, lack of evaluation from key stakeholders, and a design-actuality gap (Abbasi et al., 2022 ; Heeks, 2002 ; Kalyanasundaram et al., 2021 ; Ravi & Janodia, 2022a ). However, we believe this model can also be applied to ecosystems with better infrastructure and maturity. Once research can be stably commercialized, building a strong infrastructure for technology transfer office like those in developed countries is a task recommended in the long run.
Lastly, even though the result from these case studies can confirm the validity of the proposed NPD model, it is not a hundred percent guarantee of successful exploitation. There might be other factors or circumstances that can affect the result such as market or technology that is highly regulated by local law, certain requirements of entrepreneur characteristics, appropriate timing for market or technology readiness, ecosystem or infrastructure that is required for research to commercial process, especially in emerging markets that might have no mature standard yet, etc. Those mentioned can be considered for future research.
Theoretical implications
This study develops a modified NPD framework that incorporates agile, lean startup, and design thinking to the Stage-Gate model for effective research to commercialization process generated from within the university in developing markets. Using the proposed Augmented Stage-Gate framework that has six stages (Innovation Ideation, Build Business Case, Development, Test and Validation, Launch, and Scale-up), we have presented three case studies from the Chulalongkorn University Technology Center. The approach is structural and based on critical thinking, which helps the technology incubator to accelerate the idea-to-launch process, decide the Go/No-Go of each innovation project stage to prioritize resource contribution, and reduce the risk of failure. Applying an open innovation concept can be beneficial during the NPD process of exchanging internal and external ideas. For example, introducing market demand to guide the direction of research, bringing in high-quality human resources from outside firms to accelerate the research and development, engaging users or customers to trial the product at an early stage, and co-creating the sandbox area to test and validate the innovation. Nevertheless, the project team must have an open mindset and absorptive capability to capture the value of this approach. In addition, university or business incubators should engage legal experts to supervise each activity to avoid conflicts of interest with external parties.
Managerial implications
The actual journey from idea to launch can be different from project to project. Engaging the Next-generation Stage-Gate’s Triple A System, (Adaptive, Agile and Accelerated) and Agile development to the NPD process is very important. Especially during early stages, each project team should focus on setting up a problem statement and then experimenting to learn and fail early, fast, and cheaply. Additionally, we summarized the key lessons learned during the first few batches of the UTC incubation program. First, the importance of the stage-gate committee role and organization as they are the gatekeepers in deciding the Go/No-Go of each project’s stage. The team needs to understand each project very well and be able to effectively track development progress and milestones. Project management software tools can be helpful in sharing ideas and tracking progress among teams, mentors, and committees whose roles must be considered carefully. Second, the incubator is usually responsible for providing NPD guidelines and mentoring for each stage; yet the incubator must also sometimes play a hands-on role solving issues by working closely with each team, especially for topics that they are unfamiliar with or that are at high risk such as regulatory and IP issues. Third, especially during the COVID-19 pandemic period, many activities were conducted online, such as business matching, mentoring, and customer meetings. Online activities lack many of the emotional and social aspects of work done in person. Therefore, the community manager had to work hard to build a supportive environment, maintain momentum and create positive team dynamics. Still, our experience suggests that it is possible to practice a hybrid onsite/online model while maintaining social distancing during the COVID-19 period. Fourth, legal considerations such as NDAs (Non-disclosure Agreements) and co-founder agreements should be considered as early as possible to avoid any conflicts that could cause project delay or failure. Finally, creating an environment where research, business partners, investors, and mentors can get to know each other is very important. These relationships can be developed informally and can lead to successful business deals. However, tech incubators should be able to identify, understand, and manage the expectations and relationships of each party before organizing networking events so that win–win situations can be realized.
Ideas for future research
Further research on the deep-tech NPD framework applied to specific technologies such as Med Tech that require extraordinary activities or have important limitations is needed. Case studies of successes and failures can be very useful. Challenges involving multiple stakeholders in different development journeys can lead to project failure due to miscommunication, lack of transparency, and a lack of legal knowledge. Thus, integrating legal perspectives and creating legal readiness levels in each NPD journey is essential. Finally, an analysis of co-founder characteristics, such as personality and working style, can suggest suitable ways of commercialization to maximize the probability of success.
Availability of data and materials
Not applicable.
Abbreviations
Artificial intelligence
Food and Drug Administration
Gross domestic product
Gross expenditure on R&D
Good manufacturing practice
- Intellectual property
Institutional review board
Investment readiness level
Minimal viable product
National Aeronautics and Space Administration
Non-disclosure agreement
- New product development
Object character recognition
Personal Data Protection Act
Politics, economics, social, technology, environment and legal
Qualified diagnostic development
Strength, weakness, opportunity, and threat
Technology acceptance model
Technology readiness level
Technology transfer office
User interface
Chulalongkorn University Technology Center
User experience
Abbasi, E., Amin, I., & Siddiqui, S. (2022). Towards developing innovation management framework (IMF) for ICT organizations at Pakistan. Journal of Innovation and Entrepreneurship . https://doi.org/10.1186/s13731-022-00231-6
Article Google Scholar
Aernoudt, R., San José, A., & Roure, J. (2007). Executive forum: Public support for the business angel market in Europe—a critical review. Venture Capital, 9 (1), 71–84. https://doi.org/10.1080/13691060600996723
Australia National Investment Council., & Marsden Jacob Associates. (1995). Financing growth : policy options to improve the flow of capital to Australia’s small and medium enterprises. 66. https://books.google.com/books/about/Financing_Growth.html?id=a_PkAAAACAAJ
Baldwin, C., & von Hippel, E. (2011). Modeling a Paradigm Shift: From producer innovation to user and open collaborative innovation. Organization Science, 22 (6), 1399–1417. https://doi.org/10.1287/ORSC.1100.0618
Barringer, B. R., & Ireland, R. D. (2012). Entrepreneurship: Successfully Launching New Ventures. In Zhurnal Eksperimental’noi i Teoreticheskoi Fiziki . Pearson Education.
Blank, S. (2014). How Investors Make Better Decisions: The Investment Readiness Level . https://steveblank.com/2014/07/01/how-investors-make-better-decisions-the-investment-readiness-level/
Brown, T. (2008). Design Thinking. Harvard Business Review , 84–92.
Cocchi, N., Dosi, C., & Vignoli, M. (2021). The Hybrid Model MatrixEnhancing Stage-Gate with Design Thinking, Lean Startup, and Agile. Research Technology Management . https://doi.org/10.1080/08956308.2021.1942645
Cooper, R. (2016). Agile-Stage-Gate Hybrids: The Next Stage for Product Development. Research-Technology Management .
Cooper, R. G., & Kleinschmidt, E. J. (2001). Stage-gate process for new product success. Innovation Management U , 3 .
Cooper, R. G., & Sommer, A. F. (2016). The Agile–Stage-Gate Hybrid Model: A promising new approach and a new research opportunity. Journal of Product Innovation Management . https://doi.org/10.1111/jpim.12314
Cooper, R. G., & Sommer, A. F. (2018). Agile–Stage-Gate for Manufacturers. Research-Technology Management, 61 (2), 17–26. https://doi.org/10.1080/08956308.2018.1421380
De la Tour, A., Soussan, P., Harlé, N., Chevalier, R., & Duportet, X. (2017). From tech to deep tech . Boston Consulting Group.
Google Scholar
European Commission. (2006). Investment readiness: Summary report of the workshop .
Fellnhofer, K. (2016). Literature review: Investment readiness level of small and medium sized companies. International Journal of Managerial and Financial Accounting, 7 (3–4), 268–284. https://doi.org/10.1504/IJMFA.2015.074904
Gorschek, T., Garre, P., Larsson, S., & Wohlin, C. (2006). A model for technology transfer in practice. IEEE Software, 23 (6), 88–95. https://doi.org/10.1109/MS.2006.147
Heeks, R. (2002). Information systems and developing countries: Failure, success, and local improvisations. Information Society . https://doi.org/10.1080/01972240290075039
Hicks, B., Larsson, A., Culley, S., & Larsson, T. (2009). A methodology for evaluating Technology Readiness during product development. DS 58-3: Proceedings of ICED 09, the 17th International Conference on Engineering Design , 3 .
Howard, L. W., Tang, T. L. P., & Jill Austin, M. (2015). Teaching critical thinking skills: Ability, motivation, intervention, and the Pygmalion effect. Journal of Business Ethics . https://doi.org/10.1007/s10551-014-2084-0
Isaacson, W. (2011). Steve Jobs . Simon & Schuster.
Kalyanasundaram, G., Ramachandrula, S., & Mungila Hillemane, B. S. (2021). The life expectancy of tech start-ups in India: What attributes impact tech start-ups’ failures? International Journal of Entrepreneurial Behaviour and Research . https://doi.org/10.1108/IJEBR-01-2021-0025
Kirby, D. A., & El Hadidi, H. H. (2019). University technology transfer efficiency in a factor driven economy: The need for a coherent policy in Egypt. Journal of Technology Transfer . https://doi.org/10.1007/s10961-019-09737-w
Lee, Y., Kozar, K. A., & Larsen, K. R. T. (2003). The technology acceptance model: Past, present, and future. Communications of the Association for Information Systems . https://doi.org/10.17705/1cais.01250
Leydesdorff, L., & Etzkowitz, H. (1998). The Triple Helix as a model for innovation studies. Science and Public Policy, 25 (3), 195–203. https://doi.org/10.1093/SPP/25.3.195
Linden, A., & Fenn, J. (2003). Understanding Gartner’s Hype Cycles (Strategic Analysis Report No. R-20-1971) .
Mankins, J. C. (2009). Technology readiness assessments: A retrospective. Acta Astronautica . https://doi.org/10.1016/j.actaastro.2009.03.058
Mason, C., & Kwok, J. (2010). Investment readiness programmes and access to finance: A critical review of design issues. Local Economy . https://doi.org/10.1080/02690942.2010.504570
Office of National Higher Education Science Research and Innovation Policy Council. (2021). Results of survey on R&D expenditure and manpower in 2019 announced . https://www.nxpo.or.th/th/en/7981/
Osterwalder, A., Pigneur, Y., & Tucci, C. L. (2005). Clarifying business models: Origins, present, and future of the concept. Communications of the Association for Information Systems . https://doi.org/10.17705/1cais.01601
Ravi, R., & Janodia, M. D. (2022a). Factors Affecting Technology Transfer and Commercialization of University Research in India: A Cross-sectional Study. Journal of the Knowledge Economy . https://doi.org/10.1007/s13132-021-00747-4
Ravi, R., & Janodia, M. D. (2022b). University-Industry Technology Transfer in India: A Plausible Model Based on Success Stories from the USA, Japan, and Israel. Journal of the Knowledge Economy . https://doi.org/10.1007/s13132-022-00908-z
Ries, E. (2011). The lean startup (1st ed.). Crown Business.
Salvato, J. J., & Laplume, A. O. (2020). Agile Stage-Gate Management (ASGM) for physical products. R and D Management . https://doi.org/10.1111/radm.12426
Schaufeld, J. (2015). Commercializing innovation: Turning technology breakthroughs into products. In Commercializing Innovation: Turning Technology Breakthroughs into Products . https://doi.org/10.1007/978-1-4302-6353-1
Schumpeter, J. A. (1934). The theory of economic development. Harvard economic studies, Harvard Economic Studies , 34 .
Seferoglu, S. S., & Akbiyik, C. (2006). Teaching critical thinking [in Turkish]. Hacettepe University Journal of Education , 30 , 193–200. https://www.researchgate.net/publication/257655812_Seferoglu_S_S_Akbiyik_C_2006_Teaching_critical_thinking_in_Turkish_Hacettepe_University_Journal_of_Education_30_193-200
Souder, W. E., Nashar, A. S., & Padmanabhan, V. (1990). A guide to the best technology-transfer practices. The Journal of Technology Transfer . https://doi.org/10.1007/BF02377652
Thurman, B. A. (2009). Teaching of critical thinking skills in the English content area in South Dakota public high schools and colleges [University of South Dakota]. https://www.proquest.com/openview/8f7c02105eefefde326ae67612838bf2/1?pq-origsite=gscholar&cbl=18750
Tsai, C. H., Lin, C. F., & Liu, H. W. (2020). The diffusion of the sandbox approach to disruptive innovation and its limitations. Cornell International Law Journal . https://doi.org/10.2139/ssrn.3487175
Venkatesh, V., Morris, M. G., Davis, G. B., & Davis, F. D. (2003). User acceptance of information technology: Toward a unified view. MIS Quarterly Management Information Systems . https://doi.org/10.2307/30036540
von Hippel, E. (1976). The dominant role of users in the scientific instrument innovation process. Research Policy . https://doi.org/10.1016/0048-7333(76)90028-7
von Hippel, E. (1986). Lead users: A source of novel product concepts. Management Science, 32 (7), 791–805. https://doi.org/10.1287/mnsc.32.7.791
Walrave, B., Dolmans, S., Van Oorschot, K. E., Nuijten, A. L. P., Keil, M., & Van Hellemond, S. (2022). Dysfunctional agile-stage-gate hybrid development: keeping up appearances. International Journal of Innovation and Technology Management . https://doi.org/10.1142/S0219877022400041
Wuest, T., Liu, A., Lu, S. C. Y., & Thoben, K. D. (2014). Application of the stage gate model in production supporting quality management. Procedia CIRP . https://doi.org/10.1016/j.procir.2014.01.071
Yaldiz, N., & Bailey, M. (2019). The effect of critical thinking on making the right decisions in the new venture process. Procedia Computer Science . https://doi.org/10.1016/j.procs.2019.09.053
Zasa, F. P., Patrucco, A., & Pellizzoni, E. (2020). Managing the hybrid organization: How can agile and traditional project management coexist? Research Technology Management . https://doi.org/10.1080/08956308.2021.1843331
Download references
Acknowledgements
The authors would like to thank Eikonnex AI Co., Ltd., Chulalongkorn University Center for Artificial Intelligence in Medicine (CU-AIM), Chulalongkorn University Center of Excellence in Swine Reproduction, and Qualified Diagnostic Development (QDD) Center of Chulalongkorn University for assisting the required information and being used in the selected case studies. We would like to express our gratitude to the Second Century Fund (C2F) of Chulalongkorn University and the Program Management Unit for National Competitiveness Enhancement (PMU-C) of The Office of National Higher Education Science Research and Innovation Policy Council (NXPO) to support this research project. Lastly, we would like to thank the staffs of UTC, which now forms a research group called Ignite Innovation Lab.
Second Century Fund (C2F) of Chulalongkorn University and the Program Management Unit for National Competitiveness Enhancement (PMU-C) of The Office of National Higher Education Science Research and Innovation Policy Council (NXPO) to support this research project.
Author information
Authors and affiliations.
Graduate Affairs, Faculty of Medicine, Chulalongkorn University, Bangkok, 10330, Thailand
Pravee Kruachottikul
University Technology Center (UTC), Chulalongkorn University, Bangkok, 10330, Thailand
Faculty of Law, Chulalongkorn University, Debdvaravati Building 254 Soi Chula 42, Phayathai Road, Wangmai, Pathumwan, Bangkok, 10330, Thailand
Poomsiri Dumrongvute
Sasin School of Management, Chulalongkorn University, Bangkok, 10330, Thailand
Pinnaree Tea-makorn
Faculty of Accounting and Commerce, Chulalongkorn University, Bangkok, 10330, Thailand
Santhaya Kittikowit
Department of Psychiatry, Faculty of Medicine, Chulalongkorn University, Bangkok, 10330, Thailand
Arisara Amrapala
You can also search for this author in PubMed Google Scholar
Contributions
PK, PD, and SK conceived the concept of new product development and entrepreneurship for academic research and technology transfer. PT wrote the manuscript. AA collected data from each research team and the publication templating.
Corresponding author
Correspondence to Poomsiri Dumrongvute .
Ethics declarations
Competing interests.
The authors declared no potential conflicts of interest with respect to the research, authorship, and/or publication of this article.
Additional information
Publisher's note.
Springer Nature remains neutral with regard to jurisdictional claims in published maps and institutional affiliations.
Rights and permissions
Open Access This article is licensed under a Creative Commons Attribution 4.0 International License, which permits use, sharing, adaptation, distribution and reproduction in any medium or format, as long as you give appropriate credit to the original author(s) and the source, provide a link to the Creative Commons licence, and indicate if changes were made. The images or other third party material in this article are included in the article's Creative Commons licence, unless indicated otherwise in a credit line to the material. If material is not included in the article's Creative Commons licence and your intended use is not permitted by statutory regulation or exceeds the permitted use, you will need to obtain permission directly from the copyright holder. To view a copy of this licence, visit http://creativecommons.org/licenses/by/4.0/ .
Reprints and permissions
About this article
Cite this article.
Kruachottikul, P., Dumrongvute, P., Tea-makorn, P. et al. New product development process and case studies for deep-tech academic research to commercialization. J Innov Entrep 12 , 48 (2023). https://doi.org/10.1186/s13731-023-00311-1
Download citation
Received : 08 November 2022
Accepted : 16 June 2023
Published : 17 July 2023
DOI : https://doi.org/10.1186/s13731-023-00311-1
Share this article
Anyone you share the following link with will be able to read this content:
Sorry, a shareable link is not currently available for this article.
Provided by the Springer Nature SharedIt content-sharing initiative
- Entrepreneurship
- Technopreneurship
- Research to commercialization
- Technology transfer
Case studies
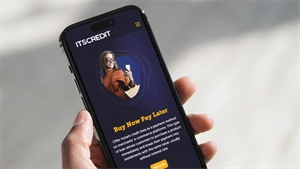
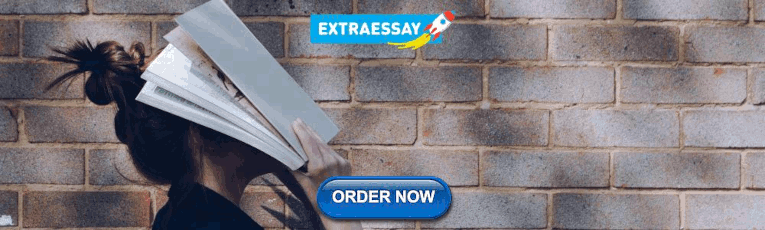
ITSCREDIT helps Polish payments company to manage credit risk
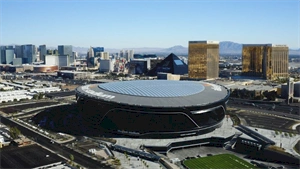
Allegiant Stadium uses Microsoft Power BI to control the indoor climate and monitor sustainability
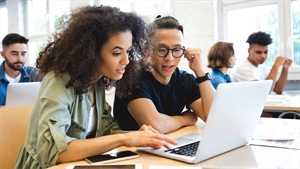
How Forta’s Terranova Security helped improve security for a Canadian university
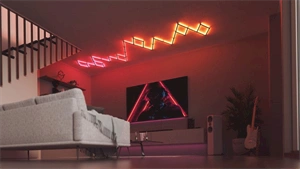
Nanoleaf improves productivity by 33 per cent by using Dynamics 365 Business Central
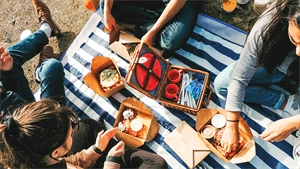
Ontinue helps AmerCareRoyal to protect merger and acquisition growth with MXDR
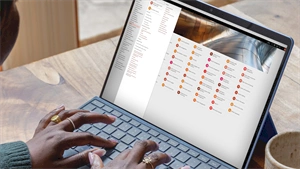
Velosio accommodates growth with accounts payable automation
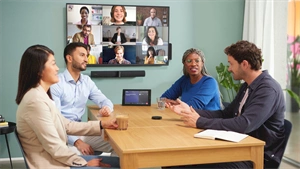
How Jabra helped Wunderman Thompson Studios Copenhagen enhance Teams collaboration
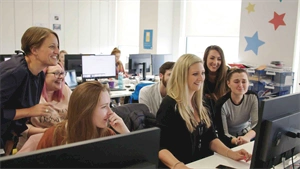
How Tollring helped Make-A-Wish UK to grant life-changing wishes
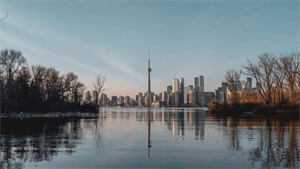
Ontario Government reduces its reliance on police with Microsoft Dynamics 365-based tool
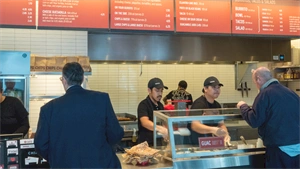
TTEC Digital helps Chipotle Mexican Grill to deliver high-quality customer service
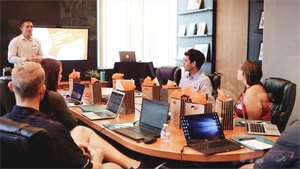
Fortra’s Terranova Security provides accessible cybersecurity training for global manufacturing firm
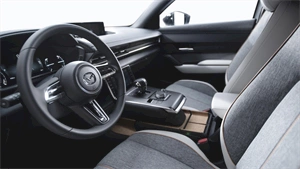
Mazda saves $1.8 million and enhances customer experiences with help from Resonate
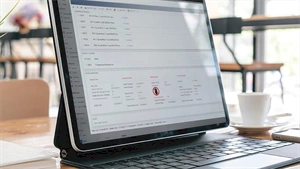
How Lexmark used Experlogix CPQ and Microsoft Dynamics 365 to streamline sales
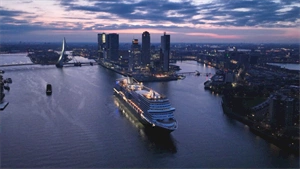
Cruise company Carnival Corporation deploys Microsoft Azure for efficient operations
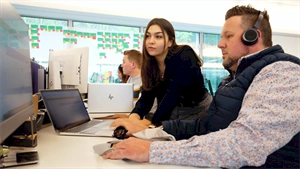
Foyer Global Health transforms services for multilingual customers with Anywhere365
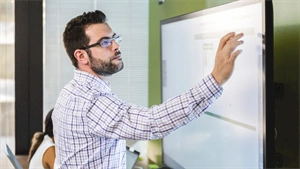
Siemens develops AI models faster with Microsoft Azure-based platform
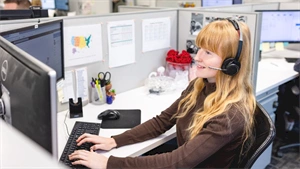
LightBulbs.com updates order management systems to automate 10 million transactions per year
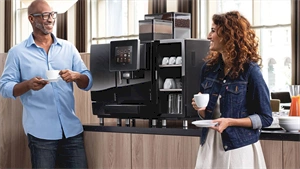
Franke is securing its connected services with Ontinue MXDR
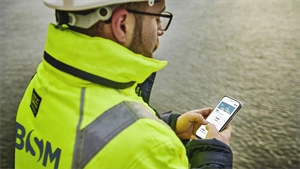
How Kroll helped BSM improve security with managed detection and response
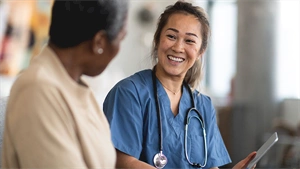
The PSA overhauls IT to ease hybrid working and reduce overheads
Recommended.
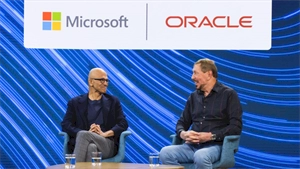
Microsoft and Oracle expand partnership to meet demand for Oracle Database@Azure
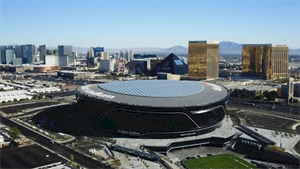
Microsoft invests $5.6 billion in AI and cloud infrastructure for Spain and Germany
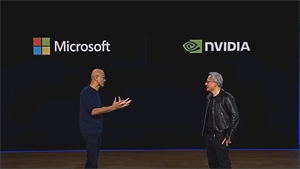
New Microsoft and Nvidia solutions to boost AI innovation
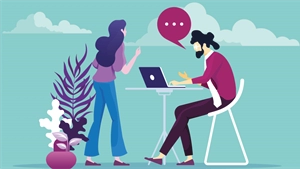
What three key AI trends does Microsoft expect to grow in 2024?
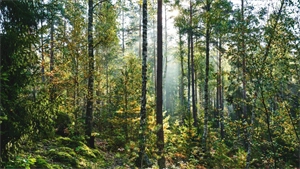
Microsoft reveals new sustainability solutions for ‘all analytics needs’
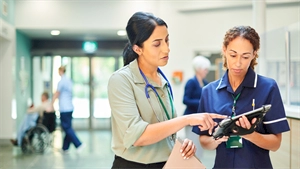
Streamlining healthcare and life sciences operations with the cloud
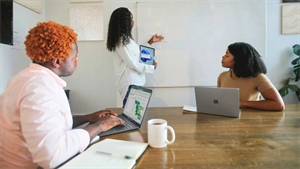
Adobe and Microsoft to bring generative AI capabilities to Microsoft 365
Subscribe to the technology record newsletter.
The most popular stories of the month delivered to your inbox
- About Technology Record
- Newsletters
- ©2024 Tudor Rose. All Rights Reserved. Technology Record is published by Tudor Rose with the support and guidance of Microsoft.
- About Tudor Rose
- Terms of Use
- Privacy policy
- Cookie policy
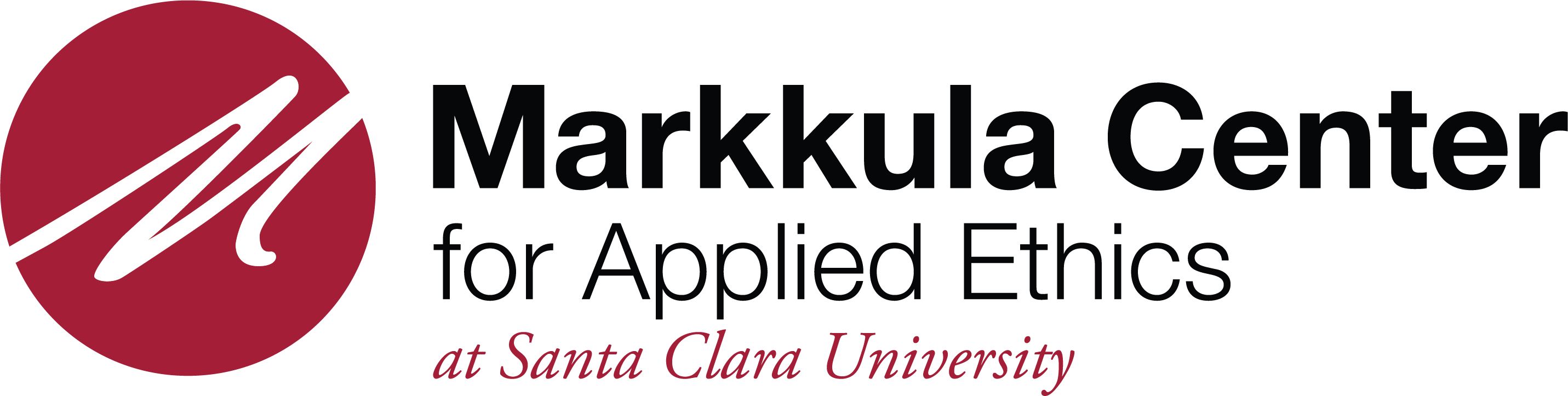
- Technology Ethics Cases
- Markkula Center for Applied Ethics
- Focus Areas
- Technology Ethics
- Technology Ethics Resources
Find case studies illustrating dilemmas in technology and ethics.
Case studies are also available on Internet Ethics .
For permission to reprint cases, submit requests to [email protected] .
Looking to draft your own case studies? This template provides the basics for writing ethics case studies in technology (though with some modification it could be used in other fields as well).
Ethical questions arise in interactions among students, instructors, administrators, and providers of AI tools.
Which stakeholders might benefit from a new age of VR “travel”? Which stakeholders might be harmed?
Case study on the history making GameStop short and stock price surge that occurred during January 2021.
As PunkSpider is pending re-release, ethical issues are considered about a tool that is able to spot and share vulnerabilities on the web, opening those results to the public.
With URVR recipients can capture and share 360 3D moments and live them out together.
VR rage rooms may provide therapeutic and inexpensive benefits while also raising ethical questions.
A VR dating app intended to help ease the stress and awkwardness of early dating in a safe and comfortable way.
Ethical questions pertaining to the use of proctoring software in online teaching.
Concern is high over possible misuses of new AI technology which generates text without human intervention.
As cities collect, analyze, and share more data, new ethical questions arise.
- More pages:
Suggestions or feedback?
MIT News | Massachusetts Institute of Technology
- Machine learning
- Social justice
- Black holes
- Classes and programs
Departments
- Aeronautics and Astronautics
- Brain and Cognitive Sciences
- Architecture
- Political Science
- Mechanical Engineering
Centers, Labs, & Programs
- Abdul Latif Jameel Poverty Action Lab (J-PAL)
- Picower Institute for Learning and Memory
- Lincoln Laboratory
- School of Architecture + Planning
- School of Engineering
- School of Humanities, Arts, and Social Sciences
- Sloan School of Management
- School of Science
- MIT Schwarzman College of Computing
Fostering ethical thinking in computing
Press contact :.
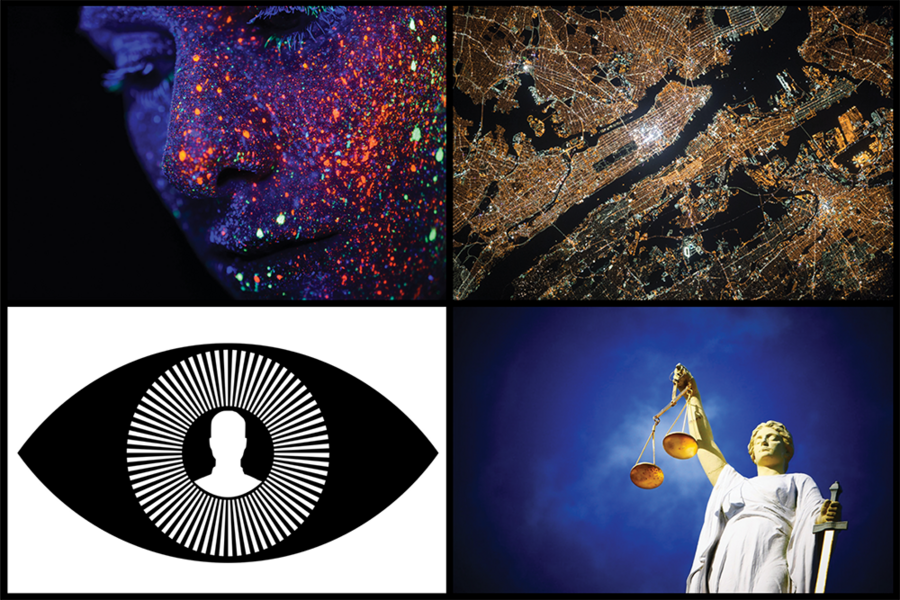
Previous image Next image
Traditional computer scientists and engineers are trained to develop solutions for specific needs, but aren’t always trained to consider their broader implications. Each new technology generation, and particularly the rise of artificial intelligence, leads to new kinds of systems, new ways of creating tools, and new forms of data, for which norms, rules, and laws frequently have yet to catch up. The kinds of impact that such innovations have in the world has often not been apparent until many years later.
As part of the efforts in Social and Ethical Responsibilities of Computing (SERC) within the MIT Stephen A. Schwarzman College of Computing, a new case studies series examines social, ethical, and policy challenges of present-day efforts in computing with the aim of facilitating the development of responsible “habits of mind and action” for those who create and deploy computing technologies.
“Advances in computing have undeniably changed much of how we live and work. Understanding and incorporating broader social context is becoming ever more critical,” says Daniel Huttenlocher, dean of the MIT Schwarzman College of Computing. “This case study series is designed to be a basis for discussions in the classroom and beyond, regarding social, ethical, economic, and other implications so that students and researchers can pursue the development of technology across domains in a holistic manner that addresses these important issues.”
A modular system
By design, the case studies are brief and modular to allow users to mix and match the content to fit a variety of pedagogical needs. Series editors David Kaiser and Julie Shah, who are the associate deans for SERC, structured the cases primarily to be appropriate for undergraduate instruction across a range of classes and fields of study.
“Our goal was to provide a seamless way for instructors to integrate cases into an existing course or cluster several cases together to support a broader module within a course. They might also use the cases as a starting point to design new courses that focus squarely on themes of social and ethical responsibilities of computing,” says Kaiser, the Germeshausen Professor of the History of Science and professor of physics.
Shah, an associate professor of aeronautics and astronautics and a roboticist who designs systems in which humans and machines operate side by side, expects that the cases will also be of interest to those outside of academia, including computing professionals, policy specialists, and general readers. In curating the series, Shah says that “we interpret ‘social and ethical responsibilities of computing’ broadly to focus on perspectives of people who are affected by various technologies, as well as focus on perspectives of designers and engineers.”
The cases are not limited to a particular format and can take shape in various forms — from a magazine-like feature article or Socratic dialogues to choose-your-own-adventure stories or role-playing games grounded in empirical research. Each case study is brief, but includes accompanying notes and references to facilitate more in-depth exploration of a given topic. Multimedia projects will also be considered. “The main goal is to present important material — based on original research — in engaging ways to broad audiences of non-specialists,” says Kaiser.
The SERC case studies are specially commissioned and written by scholars who conduct research centrally on the subject of the piece. Kaiser and Shah approached researchers from within MIT as well as from other academic institutions to bring in a mix of diverse voices on a spectrum of topics. Some cases focus on a particular technology or on trends across platforms, while others assess social, historical, philosophical, legal, and cultural facets that are relevant for thinking critically about current efforts in computing and data sciences.
The cases published in the inaugural issue place readers in various settings that challenge them to consider the social and ethical implications of computing technologies, such as how social media services and surveillance tools are built; the racial disparities that can arise from deploying facial recognition technology in unregulated, real-world settings; the biases of risk prediction algorithms in the criminal justice system; and the politicization of data collection.
"Most of us agree that we want computing to work for social good, but which good? Whose good? Whose needs and values and worldviews are prioritized and whose are overlooked?” says Catherine D’Ignazio, an assistant professor of urban science and planning and director of the Data + Feminism Lab at MIT.
D’Ignazio’s case for the series, co-authored with Lauren Klein, an associate professor in the English and Quantitative Theory and Methods departments at Emory University, introduces readers to the idea that while data are useful, they are not always neutral. “These case studies help us understand the unequal histories that shape our technological systems as well as study their disparate outcomes and effects. They are an exciting step towards holistic, sociotechnical thinking and making."
Rigorously reviewed
Kaiser and Shah formed an editorial board composed of 55 faculty members and senior researchers associated with 19 departments, labs, and centers at MIT, and instituted a rigorous peer-review policy model commonly adopted by specialized journals. Members of the editorial board will also help commission topics for new cases and help identify authors for a given topic.
For each submission, the series editors collect four to six peer reviews, with reviewers mostly drawn from the editorial board. For each case, half the reviewers come from fields in computing and data sciences and half from fields in the humanities, arts, and social sciences, to ensure balance of topics and presentation within a given case study and across the series.
“Over the past two decades I’ve become a bit jaded when it comes to the academic review process, and so I was particularly heartened to see such care and thought put into all of the reviews," says Hany Farid, a professor at the University of California at Berkeley with a joint appointment in the Department of Electrical Engineering and Computer Sciences and the School of Information. “The constructive review process made our case study significantly stronger.”
Farid’s case, “The Dangers of Risk Prediction in the Criminal Justice System,” which he penned with Julia Dressel, recently a student of computer science at Dartmouth College, is one of the four commissioned pieces featured in the inaugural issue.
Cases are additionally reviewed by undergraduate volunteers, who help the series editors gauge each submission for balance, accessibility for students in multiple fields of study, and possibilities for adoption in specific courses. The students also work with them to create original homework problems and active learning projects to accompany each case study, to further facilitate adoption of the original materials across a range of existing undergraduate subjects.
“I volunteered to work with this group because I believe that it's incredibly important for those working in computer science to include thinking about ethics not as an afterthought, but integrated into every step and decision that is made, says Annie Snyder, a mathematical economics sophomore and a member of the MIT Schwarzman College of Computing’s Undergraduate Advisory Group. “While this is a massive issue to take on, this project is an amazing opportunity to start building an ethical culture amongst the incredibly talented students at MIT who will hopefully carry it forward into their own projects and workplace.”
New sets of case studies, produced with support from the MIT Press’ Open Publishing Services program, will be published twice a year via the Knowledge Futures Group’s PubPub platform . The SERC case studies are made available for free on an open-access basis, under Creative Commons licensing terms. Authors retain copyright, enabling them to reuse and republish their work in more specialized scholarly publications.
“It was important to us to approach this project in an inclusive way and lower the barrier for people to be able to access this content. These are complex issues that we need to deal with, and we hope that by making the cases widely available, more people will engage in social and ethical considerations as they’re studying and developing computing technologies,” says Shah.
Share this news article on:
Related links.
- MIT Case Studies in Social and Ethical Responsibilities of Computing
- Program in Science, Technology, and Society
Related Topics
- Technology and society
- Education, teaching, academics
- Artificial intelligence
- Computer science and technology
- Diversity and inclusion
- Program in STS
- History of science
- Aeronautical and astronautical engineering
- Electrical Engineering & Computer Science (eecs)
- Urban studies and planning
- Human-computer interaction
- MIT Sloan School of Management
- School of Architecture and Planning
- School of Humanities Arts and Social Sciences
Related Articles
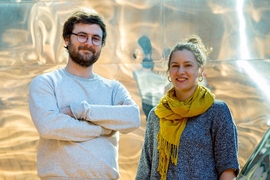
3 Questions: Marion Boulicault and Milo Phillips-Brown on ethics in a technical curriculum
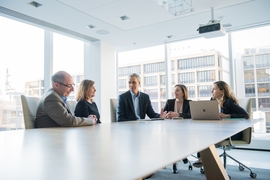
A college for the computing age
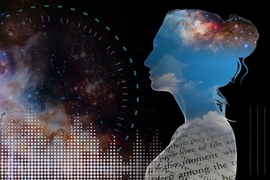
Computing and artificial intelligence: Humanistic perspectives from MIT
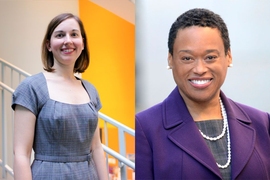
3 Questions: The social implications and responsibilities of computing
Previous item Next item
More MIT News
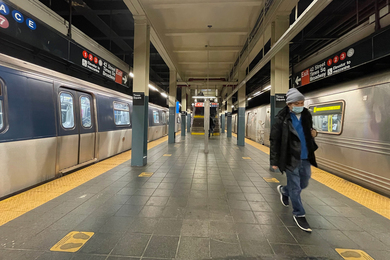
Has remote work changed how people travel in the U.S?
Read full story →
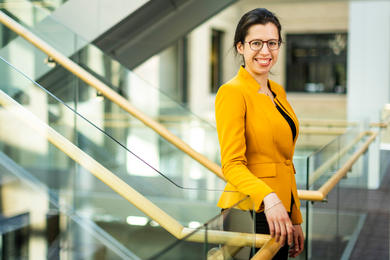
Physicist Netta Engelhardt is searching black holes for universal truths
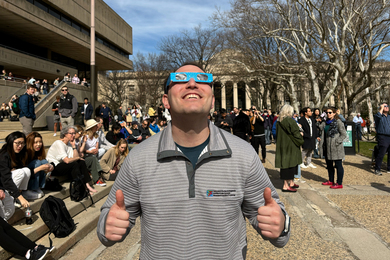
MIT community members gather on campus to witness 93 percent totality
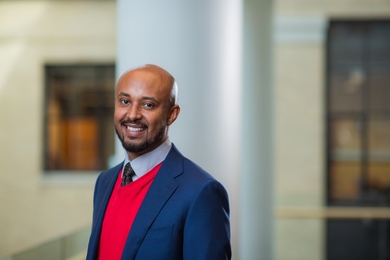
Extracting hydrogen from rocks
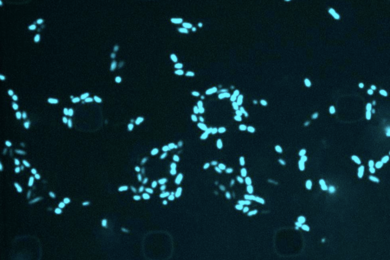
When an antibiotic fails: MIT scientists are using AI to target “sleeper” bacteria
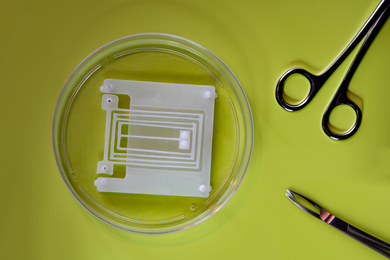
MIT engineers design flexible “skeletons” for soft, muscle-powered robots
- More news on MIT News homepage →
Massachusetts Institute of Technology 77 Massachusetts Avenue, Cambridge, MA, USA
- Map (opens in new window)
- Events (opens in new window)
- People (opens in new window)
- Careers (opens in new window)
- Accessibility
- Social Media Hub
- MIT on Facebook
- MIT on YouTube
- MIT on Instagram
Cookies on the NHS England website
We’ve put some small files called cookies on your device to make our site work.
We’d also like to use analytics cookies. These send information about how our site is used to a service called Google Analytics. We use this information to improve our site.
Let us know if this is OK. We’ll use a cookie to save your choice. You can read more about our cookies before you choose.
Change my preferences I'm OK with analytics cookies
Boosted GP practice access for patients thanks to new phone technology
- General practice
- GP online services
- Primary care
More patients will be able to get through to their GP surgery for an appointment, thanks to upgraded phone technology rolled out across the country.
Almost every GP practice in England has upgraded their phone systems – a key part of the GP access recovery plan.
More than 5,800 GP practices use a digital system for answering patients’ calls – enabling GP teams to manage multiple calls and helping to end the ‘8am rush’ for appointments.
During trials, this increased patients’ ability to get through to their practice by almost a third.
More than nine in ten ( 92 per cent) of GP practices in England now have cloud based systems – this means phone lines can be expanded and won’t ever be engaged. The remaining practices are agreeing dates within the next month for upgrades to happen with tech suppliers and are expected to happen from next month.
Extra training was also provided to staff answering calls at surgeries, so that people who need to see their family doctor are prioritised while those who would be better seen by other staff such as physiotherapists or mental health specialists can do so more quickly.
Abbey Medical Centre in Kenilworth in Warwickshire reduced the number of abandoned calls to their practice by 90% by using data from their upgraded telephone system to identify when lines were busiest and to ensure more reception staff were available at peak times.
The GP practice also focused on their most frequent callers – offering vulnerable patients more support from the same clinicians with their appointment usage reducing by three-quarters. The 25 patients who had the most appointments accounted for 8% of the total number of appointments available (50 out of 625 in a week)
Latest figures show that GPs delivered 364 million appointments in the last year – 57 million more annually than before the pandemic.
The primary care access recovery plan also set out plans for patients who need prescription medication to be able to get it directly from a pharmacy, without a GP appointment, for seven common conditions including earache, sore throat, or urinary tract infections.
The actions set out in the plan are expected to free up around 15 million GP appointments over the next two years for patients who need them most.
Dr Amanda Doyle, National Director of Primary Care and Community Services for NHS England, said:
“The NHS has delivered on its promise to upgrade GP telephone systems to make it easier for patients to contact their surgery. This is welcome news for patients and just one of a range of measures to make it quicker for people to get the help they need from their local GP team.
“Thanks to the hard work of staff more than 1.4 million appointments in general practice take place each working day which is a significant increase in the last four years and should really help patients trying to access their surgery.”
Health Minister Andrea Leadsom said:
“Backed by £240 million and the support of NHS England, nearly every general practice now has the ability to answer multiple calls efficiently with these upgrades.
“This will significantly reduce the 8am rush to book an appointment – with trials showing these measures could improve the number of people accessing GP services by almost a third.
“GPs carry out vital work to support communities and patients, and I’m pleased that there are now 800 more doctors working in general practice compared to last February. Last year, 50 million more GP appointments were delivered than five years ago, and the first ever Long Term Workforce Plan will increase GP training places by 50% to 6,000.”
Abbey Medical Centre has transformed the way patients access care following participation in the General Practice Improvement Programme.
The general practice in Kenilworth, Warwickshire, used data provided by their new digital telephony system to identify when telephone lines were busiest and to ensure more reception staff were available at peak times. Over 6 months, the number of abandoned calls fell from 247 to just 17 a day, a reduction of more than 90%.
They also promoted the use of forms on their website to request appointments and other kinds of care. The number of patients who used them grew from an average of 10 a day to 70, further reducing telephone demand and the “8am rush” for appointments – one of the key priorities of the Primary care recovery plan.
A key initiative to improve access for all patients focussed on the care they provided to 25 patients who had the most appointments. Their appointments at the practice accounted for 8% of the total number available (50 out of 625 in a week) to the practice’s 16,000 patients.
Over 6 months, the practice team steadily improved how they managed the care of these vulnerable patients, focussing on ensuring care continuity from the right clinical professional, usually a GP or nurse. Many of these patients needed wound dressing and care.
The practice developed a whole-team protocol and approach for managing wound care, including consistent steps for practice nurses, new communications methods to support team working and clear guidance on referral options so that, if required, patients could get timely access to specialist care. After 6 months, the number of appointments needed by these patients fell to 2% of all appointments (15/800), a reduction of 75%. As well as providing better, safer care for them, the new approach freed up 48 nurse and GP appointments (18 GP, 30 nurse) each week for other needy patients.
Ryan Smith, Non-Clinical Partner at the practice said: “The General Practice Improvement Programme has really helped us continue to improve our services so that more patients can get the care they need more easily. It’s particularly helped the patients who we previously saw most frequently, many as often as twice a week, by enabling us to focus on how we provide the best care for them, so they get the care they need with fewer appointments, which is better for them and means there are more appointments available for others who also need them.”
- Search entire site
- Search for a course
- Browse study areas
Analytics and Data Science
- Data Science and Innovation
- Postgraduate Research Courses
- Business Research Programs
- Undergraduate Business Programs
- Entrepreneurship
- MBA Programs
- Postgraduate Business Programs
Communication
- Animation Production
- Business Consulting and Technology Implementation
- Digital and Social Media
- Media Arts and Production
- Media Business
- Media Practice and Industry
- Music and Sound Design
- Social and Political Sciences
- Strategic Communication
- Writing and Publishing
- Postgraduate Communication Research Degrees
Design, Architecture and Building
- Architecture
- Built Environment
- DAB Research
- Public Policy and Governance
- Secondary Education
- Education (Learning and Leadership)
- Learning Design
- Postgraduate Education Research Degrees
- Primary Education
Engineering
- Civil and Environmental
- Computer Systems and Software
- Engineering Management
- Mechanical and Mechatronic
- Systems and Operations
- Telecommunications
- Postgraduate Engineering courses
- Undergraduate Engineering courses
- Sport and Exercise
- Palliative Care
- Public Health
- Nursing (Undergraduate)
- Nursing (Postgraduate)
- Health (Postgraduate)
- Research and Honours
- Health Services Management
- Child and Family Health
- Women's and Children's Health
Health (GEM)
- Coursework Degrees
- Clinical Psychology
- Genetic Counselling
- Good Manufacturing Practice
- Physiotherapy
- Speech Pathology
- Research Degrees
Information Technology
- Business Analysis and Information Systems
- Computer Science, Data Analytics/Mining
- Games, Graphics and Multimedia
- IT Management and Leadership
- Networking and Security
- Software Development and Programming
- Systems Design and Analysis
- Web and Cloud Computing
- Postgraduate IT courses
- Postgraduate IT online courses
- Undergraduate Information Technology courses
- International Studies
- Criminology
- International Relations
- Postgraduate International Studies Research Degrees
- Sustainability and Environment
- Practical Legal Training
- Commercial and Business Law
- Juris Doctor
- Legal Studies
- Master of Laws
- Intellectual Property
- Migration Law and Practice
- Overseas Qualified Lawyers
- Postgraduate Law Programs
- Postgraduate Law Research
- Undergraduate Law Programs
- Life Sciences
- Mathematical and Physical Sciences
- Postgraduate Science Programs
- Science Research Programs
- Undergraduate Science Programs
Transdisciplinary Innovation
- Creative Intelligence and Innovation
- Diploma in Innovation
- Transdisciplinary Learning
- Postgraduate Research Degree
HTI launches AI Governance Lighthouse Case Study Series
HTI’s Lighthouse Case Study Series shines a light on organisations that are actively exploring different approaches to human-centred AI governance. In the first case study, HTI presents insights from Telstra.
Telstra's approach to AI Governance
Since HTI’s AI Corporate Governance Program (AIGCP) began in September 2022, we have engaged with over 1000 organisations and individuals across Australia. A consistent request from business leaders has been to hear from their peers across diverse organisations who are in the process of tackling the challenge of AI governance.
In response, HTI is launching its Lighthouse Case Study Series, as part of the AICGP, to highlight the insights generated and challenges faced by organisations on the frontier of human-centred AI development and deployment.
“In a rapidly evolving technological and regulatory landscape around artificial intelligence, no single organisation has all the answers to making sure AI use is responsible and safe,” said HTI Co-Director and Industry Professor Nicholas Davis.
One of the fastest ways that HTI can support this is to facilitate peer-to-peer learning around what works when it comes to AI governance, sharing practical examples from organisations that are prioritising human-centred AI development and deployment. - Professor Nicholas Davis
HTI’s Lighthouse Case Study Series will initially feature three leading organisations – Telstra, KPMG Australia, and the University of Technology Sydney.
The first case study explores how Telstra is currently using AI, its approach to AI governance, the governance structures it has set up that support decision-making, and the complementary support provided to date.
Important insights include:
When assessing the strategic value of AI, organisations should consider the problem they are trying to solve and whether AI is the right tool to solve that problem.
Effective data governance is critical to AI governance.
Robust system architecture is essential to operationalise governance controls.
A rapidly evolving technology landscape requires continuous improvement.
Telstra has strategically invested in AI governance, particularly through its creation of internal governance structures that oversee all data and AI initiatives. These aim to ensure that Telstra’s commitment to Australia’s AI Ethics Principles and responsible AI are given practical effect.
Beyond the new governance structures created to formalise accountability for AI systems, such as the Risk Council for AI and Data, Telstra has aimed to foster a culture of safe and responsible AI and data use, strengthened by investment in employee training.
HTI’s work with leading Australian organisations demonstrates that there is not a ‘one size fits all approach’ to AI governance. However, for many businesses, the journey will start where Telstra’s did - not getting caught up in the hype, building a solid data and AI system inventory, and being strategic in how and where to deploy AI. - Professor Nicholas Davis
Over April 2024, HTI will publish further case studies featuring KPMG and UTS as part of HTI’s Lighthouse Case Study Series on AI Governance.
Read the AI Governance Lighthouse Case Study on Telstra
UTS acknowledges the Gadigal people of the Eora Nation, the Boorooberongal people of the Dharug Nation, the Bidiagal people and the Gamaygal people, upon whose ancestral lands our university stands. We would also like to pay respect to the Elders both past and present, acknowledging them as the traditional custodians of knowledge for these lands.

We couldn’t find any results matching your search.
Please try using other words for your search or explore other sections of the website for relevant information.
We’re sorry, we are currently experiencing some issues, please try again later.
Our team is working diligently to resolve the issue. Thank you for your patience and understanding.
News & Insights

How to Invest in OpenAI's ChatGPT (Updated 2024)

April 08, 2024 — 04:30 pm EDT
Written by Melissa Pistilli for Investing News Network ->
OpenAI’s ChatGPT is one of the latest technological breakthroughs in the artificial intelligence (AI) space. But is there a good investment case for a technology that has become so controversial?
This emerging technology is representative of a niche subsector of the AI industry known as generative AI — systems that can generate text, images or sounds in response to prompts given by users.
Precedence Research expects the global AI market to grow at a compound annual growth rate (CAGR) of 19 percent to reach US$2.57 trillion by 2032. Just how much of an impact OpenAI’s ChatGPT will have on this space is hard to predict, but S&P Global suggested in December 2023 that the total market revenue of generative AI as a whole will see a CAGR of 57.9 percent through 2028, increasing from US$3.7 billion last year to US$36.36 billion in 2028.
“The key trend last year was the rise of generative AI, and 2023 will go down as one of the most exciting years for AI yet! With the launch of ChatGPT late in 2022, the true scale of its disruptive potential was more realized across the world in 2023,” said Naseem Husain , senior vice president and exchange-traded fund (ETF) strategist at Horizons ETFs. “Its success has sparked a wave of generative and chat AI models, from Midjourney to Grok.”
Of course, OpenAi has also generated a lot of controversy, such as fears over job destruction and targeted disinformation campaigns . And let’s not forget the odd and abrupt, however brief, ousting of OpenAI CEO Sam Altman .
Many lawsuits have emerged as well. Multiple news outlets , including the the New York Times , have launched copyright lawsuits against OpenAI, and some of the plaintiffs are also seeking damages from the private tech firm’s very public partner Microsoft (NASDAQ: MSFT ). Additionally, the Authors Guild, which represents a group of prominent authors , launched a class-action lawsuit against OpenAI that is calling for a licensing system that would allow authors to opt out of having their books used to train AI, and would require AI companies to pay for the material they do use.
With all of that said, there's still a lot of excitement surrounding generative AI technology. Many investors are wondering if it's possible to invest in OpenAI's ChatGPT, and if there are other ways to invest in generative AI. Here the Investing News Network (INN) answers those questions and more, shedding light on this new landscape.
What is OpenAI's ChatGPT?
Created by San Francisco-based tech lab OpenAI, ChatGPT is a generative AI software application that uses a machine learning technique called reinforcement learning from human feedback (RLHF) to emulate human-written conversations based on a large range of user prompts. This kind of software is better known as an AI chatbot.
ChatGPT learns language by training on texts gleaned from across the internet, including online encyclopedias, books, academic journals, news sites and blogs. Based on this training, the AI chatbot generates text by making predictions about which words (or tokens) can be strung together to produce the most suitable response.
More than a million people engaged with ChatGPT within the first week of its launch for free public testing on November 30, 2022. Many were in awe of the chatbot’s seemingly natural language capabilities, not only in terms of understanding questions, but also because of its human-like responses. Users felt as if they were having a conversation with a human.
Besides being an excellent conversation partner, ChatGPT can write engaging short stories , develop catchy marketing materials, solve complicated math problems and even create code in various programming languages.
Based on this success, OpenAI has created a more powerful version of the ChatGPT system called GPT-4 , which was released in March 2023. It is currently only available to paid ChatGPT subscribers and Microsoft Copilot users .
This iteration of ChatGPT can accept visual inputs , is much more precise and can display a higher level of expertise in various subjects. Because of this, GPT-4 can describe images in vivid detail and ace standardized tests.
Unlike its predecessor, GPT-4 doesn't have any time limits on what information it can access; however, AI researcher and professor Dr. Oren Etzioni has said that the chatbot is still terrible at discussing the future and generating new ideas. It also hasn't lost its tendency to deliver incorrect information with too high a degree of confidence.
What is Elon Musk's relationship to OpenAI?
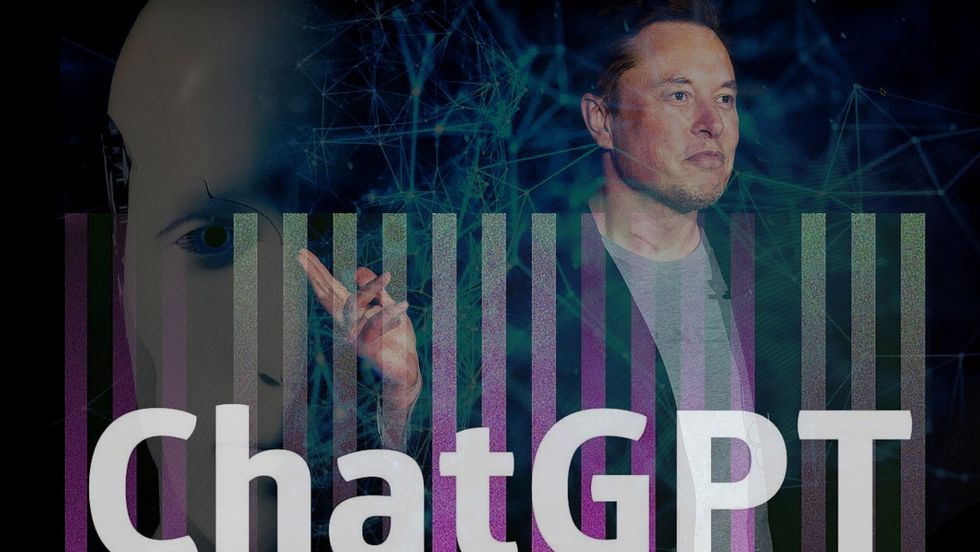
DIA TV / Shutterstock
OpenAI was founded in 2015 by Altman, its current CEO, as well as Tesla's (NASDAQ: TSLA ) Elon Musk and other big-name investors, such as venture capitalist Peter Thiel and LinkedIn co-founder Reid Hoffman. Musk left his position on OpenAI's board of directors in 2018 to focus on Tesla and its pursuit of autonomous vehicle technology.
A few days after ChatGPT became available for public testing, Musk took to X , formerly known as Twitter, to say, “ChatGPT is scary good. We are not far from dangerously strong AI.” That same day, he announced that X had shut the door on OpenAI’s access to its database so it could no longer use it for RLHF training.
His reason: “OpenAI was started as open-source & non-profit. Neither are still true.”
Furthering his feud with OpenAI, Musk filed a lawsuit against the company in March 2024 for an alleged breach of contract. The crux of his complaint was that OpenAI has broken the "founding agreement" made between the founders (Altman, Greg Brockman and himself) that the company would remain a non-profit. Altman and OpenAI have denied there was such an agreement and that Musk was keen on an eventual for-profit structure.
Is ChatGPT revolutionary or hype?
Is ChatGPT a revolutionary technology or just another hyped-up tech fad that will flop, much in the way of Google Glass or the Segway ? It may be too early to tell, but as with any new technology, there are plenty of wrinkles to iron out.
One of the most challenging bugs to fix before ChatGPT can be deployed more widely is the chatbot’s propensity to respond with “plausible-sounding but incorrect or nonsensical answers," admits OpenAI .
Remember, its selection of which words to string together are actually predictions — not as fallible as mere guesses, but still fallible. Even the latest 4.0 version is “ still is not fully reliable (it “hallucinates” facts and makes reasoning errors),” says the company, which emphasizes that users should exercise caution when employing the technology.
Indeed, ChatGPT's failings can have dangerous real-life consequences. Among other negative applications, the tech can be used to spread misinformation, carry out phishing email scams or write malicious code. What’s more, the AI-based technology is prone to racial and gender-based biases. Not only has this language learning model contributed to the human-like quality of its responses, but it has also picked up on some of humanity’s shortcomings.
“ChatGPT was trained on the collective writing of humans across the world, past and present. This means that the same biases that exist in the data, can also appear in the model,” explains Garling Wu , staff writer for online technology publication MUO, in a September 2023 article. “In fact, users have shown how ChatGPT can give produce some terrible answers, some, for example, that discriminate against women. But that's just the tip of the iceberg; it can produce answers that are extremely harmful to a range of minority groups.”
On the flip side, an August 2023 study by the University of East Anglia identified a left-wing bias in ChatGPT. Researchers at the school said their work shows that ChatGPT "favors Democrats in the U.S., the Labour Party in the U.K., and president Lula da Silva of the Workers’ Party in Brazil," according to Forbes.
There’s also the fear among teachers that the technology is leading to an unwelcome rise in academic dishonesty, with students using ChatGPT to write essays or complete their science homework.
“Teachers and school administrators have been scrambling to catch students using the tool to cheat, and they are fretting about the havoc ChatGPT could wreak on their lesson plans,” writes New York Times tech columnist Kevin Roose .
Despite these concerns, we’re likely to see new iterations of ChatGPT — hopefully without the aforementioned bugs — as OpenAI has the backing of tech giant Microsoft.
Why is Microsoft investing in OpenAI?
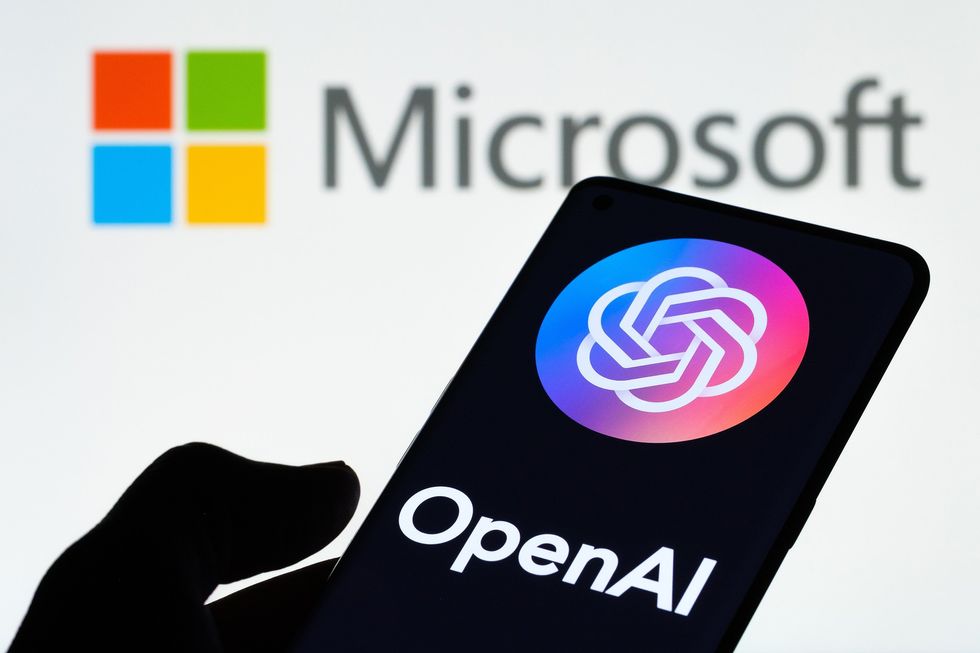
Ascannio / Shutterstock
Since 2019, Microsoft has invested at least US$3 billion in OpenAI to help the small tech firm create its ultra-powerful AI chatbot, as reported by New York Times technology correspondents Cade Metz and Karen Weise.
Microsoft announced in mid-January 2023 that as part of the third phase of its partnership with OpenAI, it will make "a multiyear, multibillion dollar investment." Although the company hasn't disclosed the total amount of its latest spend, reports at the time indicated that US$10 billion is on the table . According to a February article from Reuters , OpenAI was recently valued at US$80 billion, meaning Microsoft's US$10 billion move would be huge. However, as of late 2023 there were rumors that OpenAI has only received a fraction of that purported investment.
How could Microsoft benefit from its investment? It seems the tech giant is hopeful advancements in generative AI may increase revenues for its Azure cloud computing business, as OpenAI officially licensed its technologies to Microsoft in 2020. Indeed, Pitchbook has described the deal as an “ unprecedented milestone ” for generative AI technology.
The strength of Microsoft’s confidence in OpenAI’s Altman was definitely on display in late November, when it quickly moved him to the payroll of its advanced AI research team after he was fired from OpenAI . Barely a week passed before Altman was back at the helm of OpenAI with major board changes, including the addition of Dee Templeton , Microsoft's vice president of technology and research partnerships and operations, as a non-voting observer.
What's the future of OpenAI and ChatGPT?
The ChatGPT 3.5 platform is free to use, and can be accessed via the web. Those with an iPhone or iPad can also use ChatGPT through an app , and an Android version launched in July 2023 . OpenAI also launched a paid subscription, ChatGPT Plus for business use , in August 2023. ChatGPT Plus gives users access to the newest iteration, GPT-4.
In addition to Microsoft's use of the ChatGPT technology as part of Copilot, other companies are working with OpenAI to incorporate the technology into their platforms, including Canva, Duolingo (NASDAQ: DUOL ), Intercom, Salesforce (NYSE: CRM ), Scale, Stripe, and Upwork (NASDAQ: UPWK ).
As uptake increases, generative AI technology is replacing humans in the workplace, and will likely continue doing so in a number of fields, from content creation and customer service to transcription and translation services, and even in graphic design and paralegal fields. However, humans are hitting back, as evidenced by recent lawsuits launched against OpenAI and Microsoft. As mentioned, a growing group of prominent authors is suing the creator of ChatGPT and its financial backer for infringing on their copyright by using their books without permission to train the language models behind ChatGPT and other AI-based software.
The New York Times has also taken a stand by taking OpenAI and Microsoft to Manhattan Federal Court.
"Defendants seek to free-ride on the Times's massive investment in its journalism by using it to build substitutive products without permission or payment," states the complaint . "There is nothing 'transformative' about using the Times's content without payment to create products that substitute for the Times and steal audiences away from it."
What about the long-term goals for OpenAI and ChatGPT? Metz of the New York Times believes the end game is “artificial general intelligence, or AGI — a machine that can do anything the human brain can do.”
In keeping with this end goal, OpenAI made a major move by acquiring an AI creative firm with a deep talent bench, Global Illumination , in mid-August 2023. "Global Illumination is a company that has been leveraging AI to build creative tools, infrastructure, and digital experiences," states OpenAI on its website .
"The team previously designed and built products early on at Instagram and Facebook and have also made significant contributions at YouTube, Google, Pixar, Riot Games, and other notable companies."
In November 2023, OpenAI decided to give customers without coding skills the ability to create customized versions of its chatbot and access to large data sets for training. “OpenAI wants people to start innovating using the chatbots and creating special chatbots,” Hod Lipson, an engineering and data science professor at Columbia University, told CNBC .
Chatbot creators will eventually have the ability to share their custom chatbots through OpenAI’s GPT Store. “They’re really trying to create a marketplace, which will allow companies and people to innovate and play around with this incredible form of AI that they’ve just unleashed,” Lipson added.
What is Google's Bard AI?
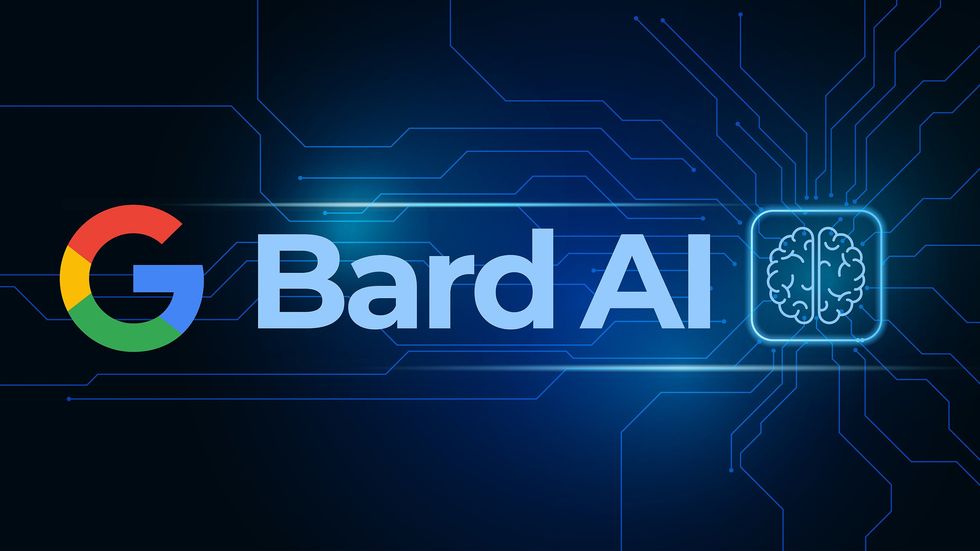
Carl DMaster / Shutterstock
While ChatGPT has been generating major buzz, it's definitely not the only chatbot out there.
Notably, Alphabet (NASDAQ: GOOGL ) subsidiary Google launched its answer to ChatGPT in March 2023. Known as Bard AI , the chatbot is built on Google’s Language Model for Dialogue Applications (or LaMDA). Google CEO Sundar Pichai has described Bard as an “experimental conversational AI service … (that) seeks to combine the breadth of the world’s knowledge with the power, intelligence and creativity of our large language models.”
As with ChatGPT, users can key in a query, request or prompt and Bard will provide a human-like response. One way in which Bard may have had a leg up on the original ChatGPT is that the latter could only use data up to 2021, while the former can access up-to-date information online; this is less relevant now that GPT-4 no longer has this limitation.
However, Bard's ability to access current data hasn’t spared it from ChatGPT’s biggest folly: confidently stating misinformation as fact. The Verge reported that when asked about new discoveries from the James Webb Space Telescope, Google’s Bard “made a factual error in its very first demo.”
In early in 2024, Google launched the latest iteration of its Bard Advanced AI chatbot with a new name, Gemini AI. The new version is powered by Google's Gemini Ultra large language model.
Which stocks will benefit the most from AI chatbot technology?
Other than companies directly tied to generative AI technology, which stocks are likely to get a boost from advances?
There are several verticals in the tech industry with indirect exposure to AI chatbot technology, such as semiconductors, network equipment providers, cloud providers, central processing unit manufacturers and internet of things.
Some of the publicly traded companies in these verticals include:
- Graphics processing unit leader Nvidia (NASDAQ: NVDA )
- The world's largest semiconductor chip manufacturer by revenue, Taiwan Semiconductor Manufacturing Company (NYSE: TSM )
- Computer memory and data storage producer Micron Technology (NASDAQ: MU )
- Digital communications firm Cisco Systems (NASDAQ: CSCO )
- Networking products provider Juniper Networks (NYSE: JNPR )
- Semiconductor producer Marvell Technology Group (NASDAQ: MRVL )
- Cloud-computing Amazon Web Services' parent company Amazon (NASDAQ: AMZN )
- Bluechip multinational technology company IBM (NYSE: IBM )
- Major semiconducter chip manufacturer Intel (NASDAQ: INTC )
While most companies specializing in generative AI remain in the venture capital stage, there are plenty of AI stocks for those interested in the space. INN's article 5 Canadian Artificial Intelligence Stocks , ASX AI Stocks: 5 Biggest Companies , and 12 Generative AI Stocks to Watch as ChatGPT Soars includes some examples.
Investors who don’t like to put all their eggs in one basket can check out these 5 Artificial Intelligence ETFs . And if you’re looking for a more general overview of the market, INN has you covered with How to Invest in Artificial Intelligence . You can also take a look back at the market in 2023 with our AI Market 2023 Year-End Review , or read projections for AI this year in our AI Market Forecast: 3 Top Trends that will Affect AI in 2024 .
FAQs for investing in OpenAI and ChatGPT
When will openai go public.
So, can you invest in OpenAI itself? The company is not currently a publicly traded stock; however, if Microsoft does take a large position in the company, investors will be able to gain indirect exposure to OpenAI by purchasing Microsoft shares.
For those seeking direct exposure, be on the lookout for news of an initial public offering (IPO). As of late-March 2024, there are no plans for an OpenAI IPO on the horizon.
How is OpenAI funded?
OpenAI raised US$11.3 billion over six funding rounds from 2016 to January 2024.
The three top investors are technology investment firm Thrive Capital, venture capital firm Andreessen Horowitz and revolutionary technology investment firm Founders Fund.
What is the market value of ChatGPT/OpenAI?
OpenAI has a market valuation of US$80 billion as of February 2024. The company’s 2023 revenue had reached US$2 billion mark in December 2023 to join the ranks of Google and Meta (NASDAQ: META ).
Does ChatGPT use Nvidia chips?
ChatGPT’s distributed computing infrastructure depends upon powerful servers with multiple graphics processing units (GPUs). High-performance Nvidia GPU chips are preferred for this application as they also provide excellent Compute Unified Device Architecture support.
Will ChatGPT cause another GPU shortage?
Most likely not. The type of GPUs used for machine learning models like ChatGPT are different from other types of GPUs, including those used to power gaming systems or crypto mining.
Can ChatGPT make stock predictions?
A University of Florida study recently highlighted the potential for advanced language models such as ChatGPT to accurately predict movements in the stock market using sentiment analysis. During the course of the study, ChatGPT outperformed traditional sentiment analysis methods, and the finance professors conducting the research concluded that “incorporating advanced language models into the investment decision-making process can yield more accurate predictions and enhance the performance of quantitative trading strategies.”
When to expect ChatGPT 5?
OpenAI filed a trademark application for ChatGPT-5 in mid-July 2023, which hinted that the next iteration of the generative AI technology is currently under development. There were rumours the company planned to complete training for ChatGPT-5 by the end of 2023, which did not materialize. Its anyone's guess when we may see its launch, but most likely not before Q3 2024 .
While PC Guide notes that OpenAI did release GPT-4V and GPT-4 Turbo in Q4 2023, there is little sign that ChatGPT-5 is close to market. However, the publication did share that, "In a March 2024 interview on the Lex Fridman podcast, Sam Altman teased an “ amazing new model this year ” but wouldn’t commit to it being called GPT 5 (or anything else)".
This is an updated version of an article first published by the Investing News Network in 2023.
Don't forget to follow us @INN_Technology for real-time news updates!
Securities Disclosure: I, Melissa Pistilli, hold no direct investment interest in any company mentioned in this article.
Editorial Disclosure: The Investing News Network does not guarantee the accuracy or thoroughness of the information reported in the interviews it conducts. The opinions expressed in these interviews do not reflect the opinions of the Investing News Network and do not constitute investment advice. All readers are encouraged to perform their own due diligence.
The views and opinions expressed herein are the views and opinions of the author and do not necessarily reflect those of Nasdaq, Inc.

More Related Articles
This data feed is not available at this time.
Sign up for the TradeTalks newsletter to receive your weekly dose of trading news, trends and education. Delivered Wednesdays.
To add symbols:
- Type a symbol or company name. When the symbol you want to add appears, add it to My Quotes by selecting it and pressing Enter/Return.
- Copy and paste multiple symbols separated by spaces.
These symbols will be available throughout the site during your session.
Your symbols have been updated
Edit watchlist.
- Type a symbol or company name. When the symbol you want to add appears, add it to Watchlist by selecting it and pressing Enter/Return.
Opt in to Smart Portfolio
Smart Portfolio is supported by our partner TipRanks. By connecting my portfolio to TipRanks Smart Portfolio I agree to their Terms of Use .
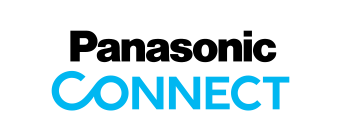
- Products/Solutions
- Projector Country&Region
- Projector Global
- Country & Region

Product Quick Search
- For Corporate
- For Education
- New Product / Event Information
- Optional Lens Guide
- Option Finder

Large-Venue

Installation

Short Throw

Ultra Short Throw

Wireless Presentation System

360 degree camera speakerphone

Sustainability

- Procurement
- Distribution, Sales, and Installation
- Lifetime Usage
- Disposal & Recycling
- April 3, 2024 Case Studies New case study (Ancient Shu Immersive Exhibition) added.
- April 2, 2024 Download Throw Distance Calculator updated.
- January 31, 2024 Product Preliminary Product Information for 2024 models added.
- January 31, 2024 Product PT-MZ882 Series webpage added.
- January 31, 2024 Press Release PT-MZ882 Series announced.
- January 31, 2024 Product PT-RQ7 Series webpage added.
- January 31, 2024 Press Release PT-RQ7 Series announced.
- January 31, 2024 Product ET-FMP50 Series webpage added.
- January 31, 2024 Press Release ET-FMP50 Series announced.
- December 6, 2023 Solution New Products, Case studies, Blogs, and Videos added to Corporate AV Solutions webpage.
- November 29, 2023 Solution Sustainability mini-site for our global projector business launched.
- October 27, 2023 Download Throw Distance Calculator updated.
- October 27, 2023 Case Studies New case study (Ukiyoe Motion Exhibition) added.
- October 27, 2023 Case Studies New case study (GOLFLAND CORPORATION) added.
- September 22, 2023 Download Throw Distance Calculator updated.
- September 22, 2023 Download Fisheye Lens Simulator updated.
- September 14, 2023 Product PT-RZ14K webpage updated.
- September 4, 2023 Download Throw Distance Calculator updated.
- July 28, 2023 Support List of tested SFP modules compatible with TY-SB01FB 12G-SDI Optical Function Board updated.
- July 28, 2023 Solution Webpage of Intel ® SDM Slots updated.
- July 27, 2023 Download Throw Distance Calculator updated.
- July 19, 2023 Support PT-FRQ60 Series added to Projector FAQ page.
- July 19, 2023 Product PT-REZ12 Series webpage updated.
- July 5, 2023 Case Studies New case study (Es Con Field Hokkaido) added.
- June 9, 2023 Product Preliminary Product Information for PT-RZ14K added.
- June 9, 2023 Press Release PT-RZ14K product announced.
- June 9, 2023 Product Preliminary Product Information for PT-REQ15/REZ15 added.
- June 9, 2023 Press Release PT-REQ15/REZ15 products announced.
- May 19, 2023 Product PT-MZ20K Series interview video added.
- May 8, 2023 Case Studies New case study (Subaru Kohsan EBiS303 Event Hall) added.
- April 26, 2023 Download Throw Distance Calculator updated.
- April 20, 2023 Product PT-REQ12 Series webpage updated.
- April 17, 2023 Product PT-CMZ50 webpage updated.
- April 12, 2023 Product PT-MZ20K Series webpage updated.
- March 27, 2023 Case Studies New case study (Chinggis Khaan National Museum) added.
- February 22, 2023 Download Throw Distance Calculator updated.
- February 17, 2023 Product PT-MZ20K Series product information updated (product information on optional lenses added).
- February 15, 2023 Case Studies New case study (Pavilion Bukit Jalil “Dome of Wonders”) added.
- February 10, 2023 Case Studies New case study (Grande Centre Point Space Pattaya) added.
- January 31, 2023 Product Preliminary Product Information for 2023 models added.
- January 31, 2023 Product PT-REQ12 Series webpage added.
- January 31, 2023 Product PT-REZ12 Series webpage added.
- January 12, 2023 Product PT-CMZ50 webpage added.
- January 6, 2023 Case Studies New case studies added.
- December 23, 2022 Download Throw Distance Calculator updated.
- December 22, 2022 Case Studies New case studies added.
- December 20, 2022 Product PT-MZ20K Series webpage updated.
- December 14, 2022 Solution New Useful Blogs, Case studies and Videos added to Corporate AV Solutions webpage.
- October 19, 2022 Product PT-RQ25K Series webpage updated.
- October 19, 2022 Download Throw Distance Calculator updated.
- October 19, 2022 Download Fisheye Lens Simulator updated.
- October 14, 2022 Product PT-MZ20K Series preliminary webpage updated.
- October 4, 2022 News Special Site for Olympic Games and Paralympic Games added.
- October 4, 2022 News Beijing 2022 Special Website launched.
- September 15, 2022 Download Throw Distance Calculator updated.
- September 15, 2022 Product PT-LMZ460 Series webpage added.
- September 15, 2022 Product PT-TMZ400 Series webpage added.
- September 12, 2022 Case Studies New case study (Australia Pavilion, Expo 2020 Dubai) added.
- September 12, 2022 Case Studies Seven case studies added.
- September 1, 2022 Support PT-MZ880 Series added to Projector FAQ page.
- July 26, 2022 Case Studies New case study (Portugal Pavilion, Expo 2020 Dubai) added.
- July 20, 2022 Product Option Finder added.
- July 19, 2022 Support Projector FAQ page renewed.
- June 30, 2022 Download Projector Network Setup Software is now available.
- June 29, 2022 Support Optional Lens Guide webpage added.
- June 10, 2022 Case Studies New case study (Thailand Pavilion, Expo 2020 Dubai) added.
- June 8, 2022 Case Studies New case study (Hungary Pavilion, Expo 2020 Dubai) added.
- June 7, 2022 Solution New blogs added to Panasonic Corporate AV Solutions webpage.
- June 7, 2022 Download Multi Monitoring and Control Software updated.
- June 6, 2022 Case Studies New case study (France Pavilion, Expo 2020 Dubai) added.
- May 23, 2022 Case Studies New case study (Japan Pavilion, Expo 2020 Dubai) added.
- May 17, 2022 Solution Education webpage renewed , blog links to Panasonic EU and NA added .
- May 10, 2022 Product Preliminary Product Information for 2022 models added.
- May 10, 2022 Download Throw Distance Calculator updated.
- April 20, 2022 Product PT-FRQ60 Series webpage updated.
- April 20, 2022 Download Throw Distance Calculator updated.
- March 25, 2022 Case Studies New case study (Juntendo University, Sakura Campus) added.
- March 16, 2022 Solution Sound devices added to the Office Environment with Visual Systems on Corporate AV Solutions webpage.
- March 10, 2022 Case Studies Barrier-Free VR Spectating: "Reserve Seat for the Future Stars" Case Study added.
- March 9, 2022 Solution Useful Blog page added to Corporate AV Solutions webpage.
- March 1, 2022 Product PT-RQ25K Series webpage added.
- March 1, 2022 Product PT-MZ20KL webpage added.
- February 18, 2022 Product PT-VMZ71 Series webpage updated.
- February 18, 2022 Download Throw Distance Calculator updated.
- February 18, 2022 Download Fisheye Lens Simulator updated.
- February 8, 2022 Case Studies New case studies added.
- January 27, 2022 Product PT-FRQ60 Series webpage added.
- January 24, 2022 Product PT-VMZ71 Series webpage added.
- January 19, 2022 Case Studies New case study (Fantasia City of Lights Huis Ten Bosch) added.
- January 12, 2022 Solution The Museum Experience on Panasonic Museum AV Solutions updated.
Case Studies
- See More Case Studies

Museum / Exhibition
Ancient Shu Immersive Exhibition (China)

Ukiyoe Motion Exhibition (Japan)

Corporate / Business
GOLFLAND CORPORATION (Japan)

Subaru Kohsan EBiS303 Event Hall (Japan)

Daiichi Sankyo Co., Ltd. “NEXUS HAYAMA” (Japan)
Contact Information

- North America
- Latin America
- Europe / CIS
- Middle East / Africa
Related Links

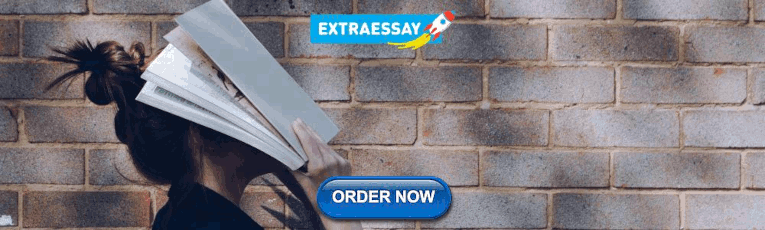
IMAGES
VIDEO
COMMENTS
Here are the 8 inspiring digital transformation case studies to consider when undertaking transformation projects in 2024: 1. Amazon extended the B2C model to embrace B2B transactions with a vision to improve the customer experience. Overview of the digital transformation initiative. Amazon Business is an example of how a consumer giant ...
To study the organizational characteristics such as corporate entrepreneurship, institutional entrepreneurship, innovation process of companies, the qualitative case study is the suitable method. This is because a case study is a useful method when verifying or expanding well-known theories or challenging a specific theory ( Yin, 2008 ).
The modern CIO creates new operating models and helps the organization become data-driven. 7 The CIO takes the organization forward "… in ways that extract the maximum value from the information on hand…to make better decisions, faster" 8 as articulated in the new data strategy. This case study articulates all the listed requirements of ...
Case Study: Will a Bank's New Technology Help or Hurt Morale? by. Leonard A. Schlesinger. From the Magazine (July-August 2021) Anuj Shrestha. Share. Save. Beth Daniels, the CEO of Michigan's ...
From World War II until the early 1970s, labor productivity grew at over 3% a year, more than doubling over the period, ushering in an era of prosperity for most Americans. In the early 1970s ...
New research on technology adoption from Harvard Business School faculty on issues including the use of educational technology, effects of the increasing ubiquity of smartphones, and methods for increasing technology adoption. ... A case study by Andy Wu and David Yoffie lays out the key challenges immersive 3D technology must overcome to be ...
Data is providing the fuel to power better and faster decisions. High-performing organizations are three times more likely than others to say their data and analytics initiatives have contributed at least 20 percent to EBIT (from 2016-19). Customer followers. Being "customer centric" is well established.
Tech Trends 2020 technology case studies | Deloitte Insights. Article 1 minute read 15 January 2020. Case studies and insights. 15 January 2020. Read insights from thought leaders and success stories from leading organizations. Get inspired by new ideas on ways to apply emerging technologies to reach business ambitions.
New research on technological innovation from Harvard Business School faculty on issues including using data mining to improve productivity, why business IT innovation is so difficult, and the business implications of the technology revolution. ... A case study by Andy Wu and David Yoffie lays out the key challenges immersive 3D technology must ...
In a recent McKinsey study, some 50 percent of surveyed companies reported moderate to significant impact on realizing new revenue streams, almost 70 percent reported impact on increasing existing revenue streams, and 76 percent reported impact on reducing costs. 1. Tech transformations, nonetheless, remain notoriously difficult and complex.
Enterprises use AIMultiple to identify new software and services, their use cases, benefits, best practices and case studies. AIMultiple shares data-driven insights on how solutions in AI / generative AI / machine learning / data science, cloud / cloud GPUs, cybersecurity / application security / network security / microsegmentation, data collection / web data / survey software, IoT, process ...
As a technology and innovation scholar, the author has studied how innovators commercialize new technologies and found that Tesla's strategy offers enduring lessons for any innovator, especially ...
New research on information technology from Harvard Business School faculty on issues including the HealthCare.gov fiasco, online privacy concerns, and the civic benefits of technologies that utilize citizen-created data. Page 1 of 59 Results →. 12 Mar 2024. HBS Case.
For many executives, this push to embrace innovation is perplexing due to the constant deluge of exciting new technologies. The U.S. economy grew 2.3% last year, but technology is expensive, and ...
Case Study Classroom Notes. A 2023 report by McKinsey found that increased productivity from AI could equal an additional $200 billion to ... Michael broke the silence. "This technology isn't ...
Technology Consulting Case Study #1: IBM. Technology consulting can help companies better use the data they already have. Woodside, a major oil and gas company in Australia, needed to retain senior engineers' institutional knowledge. Its goal was to collect decades of unique expertise and historical context that was previously stored in ...
Interest in the topic (as gauged by news and internet searches) increased threefold from 2021 to 2022. As we recently wrote, generative AI and other foundational models change the AI game by taking assistive technology to a new level, reducing application development time, and bringing powerful capabilities to nontechnical users. Generative AI ...
In the second edition of this series, we focus on IBM's journey towards ethical AI technology. The insights from this effort are detailed in a report titled Responsible Use of Technology: The IBM Case Study, which is jointly authored by the World Economic Forum and the Markkula Center for Applied Ethics at Santa Clara University.Below are the key lessons learned from our research, along with ...
Two cases about Hertz claimed top spots in 2021's Top 40 Most Popular Case Studies. ... Master's in Technology Management One-year business & leadership program designed exclusively for Yale College ... Yale School of Management Edward P. Evans Hall 165 Whitney Avenue New Haven, CT 06511-3729 Apply Now Get Yale SOM News. Footer navigation.
technology innovation outcomes might look like. Yet the need to define how technology products can be ethically made remains.3 This paper is the first in a series of case studies that investigate how companies have begun to incorporate ethical thinking into the development of technology. It focuses on Microsoft Corporation and
This research proposes a new product development (NPD) framework for innovation-driven deep-tech research to commercialization and tested it with three case studies of different exploitation methods. The proposed framework, called Augmented Stage-Gate, integrates the next-generation Agile Stage-Gate development process with lean startup and design thinking approaches.
TTEC Digital helps Chipotle Mexican Grill to deliver high-quality customer service. Case study | Manufacturing.
An AI Ethics Case Study. Concern is high over possible misuses of new AI technology which generates text without human intervention. Case studies illustrating ethical dilemmas in IT, biotechnology, and the Internet.
A case studies series from the Social and Ethical Responsibilities of Computing program at MIT delves into a range of topics, from social and ethical implications of computing technologies and the racial disparities that can arise from deploying facial recognition technology in unregulated, real-world settings to the biases of risk prediction algorithms in the criminal justice system and the ...
More patients will be able to get through to their GP surgery for an appointment, thanks to upgraded phone technology rolled out across the country. Almost every GP practice in England has upgraded their phone systems - a key part of the GP access recovery plan. More than 5,800 GP practices use a digital system for answering patients' calls ...
HTI's Lighthouse Case Study Series will initially feature three leading organisations - Telstra, KPMG Australia, and the University of Technology Sydney. The first case study explores how Telstra is currently using AI, its approach to AI governance, the governance structures it has set up that support decision-making, and the complementary ...
Just how much of an impact OpenAI's ChatGPT will have on this space is hard to predict, but S&P Global suggested in December 2023 that the total market revenue of generative AI as a whole will ...
Copilot for Security customers can use the incredible depth and breadth of Microsoft threat intelligence in MDTI with Generative AI to quickly understand the full scope of attacks, anticipate the next steps of an ongoing campaign, and drive an optimal security plan for their organizations. They can immediately begin using MDTI in the Copilot ...
The newest variant, the E-2D Advanced Hawkeye, brings the latest technology into the platform that can confront threats anywhere in the world, Janice Zilch, Northrop Grumman's vice-president for ...
January 31, 2024 Press Release ET-FMP50 Series announced. December 6, 2023 Solution New Products, Case studies, Blogs, and Videos added to Corporate AV Solutions webpage. November 29, 2023 Solution Sustainability mini-site for our global projector business launched. October 27, 2023 Download Throw Distance Calculator updated.