- Privacy Policy

Home » Research Design – Types, Methods and Examples
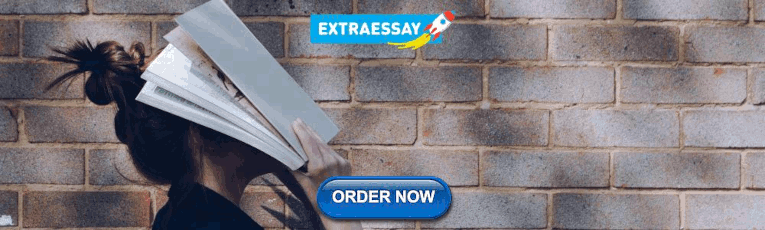
Research Design – Types, Methods and Examples
Table of Contents

Research Design
Definition:
Research design refers to the overall strategy or plan for conducting a research study. It outlines the methods and procedures that will be used to collect and analyze data, as well as the goals and objectives of the study. Research design is important because it guides the entire research process and ensures that the study is conducted in a systematic and rigorous manner.
Types of Research Design
Types of Research Design are as follows:
Descriptive Research Design
This type of research design is used to describe a phenomenon or situation. It involves collecting data through surveys, questionnaires, interviews, and observations. The aim of descriptive research is to provide an accurate and detailed portrayal of a particular group, event, or situation. It can be useful in identifying patterns, trends, and relationships in the data.
Correlational Research Design
Correlational research design is used to determine if there is a relationship between two or more variables. This type of research design involves collecting data from participants and analyzing the relationship between the variables using statistical methods. The aim of correlational research is to identify the strength and direction of the relationship between the variables.
Experimental Research Design
Experimental research design is used to investigate cause-and-effect relationships between variables. This type of research design involves manipulating one variable and measuring the effect on another variable. It usually involves randomly assigning participants to groups and manipulating an independent variable to determine its effect on a dependent variable. The aim of experimental research is to establish causality.
Quasi-experimental Research Design
Quasi-experimental research design is similar to experimental research design, but it lacks one or more of the features of a true experiment. For example, there may not be random assignment to groups or a control group. This type of research design is used when it is not feasible or ethical to conduct a true experiment.
Case Study Research Design
Case study research design is used to investigate a single case or a small number of cases in depth. It involves collecting data through various methods, such as interviews, observations, and document analysis. The aim of case study research is to provide an in-depth understanding of a particular case or situation.
Longitudinal Research Design
Longitudinal research design is used to study changes in a particular phenomenon over time. It involves collecting data at multiple time points and analyzing the changes that occur. The aim of longitudinal research is to provide insights into the development, growth, or decline of a particular phenomenon over time.
Structure of Research Design
The format of a research design typically includes the following sections:
- Introduction : This section provides an overview of the research problem, the research questions, and the importance of the study. It also includes a brief literature review that summarizes previous research on the topic and identifies gaps in the existing knowledge.
- Research Questions or Hypotheses: This section identifies the specific research questions or hypotheses that the study will address. These questions should be clear, specific, and testable.
- Research Methods : This section describes the methods that will be used to collect and analyze data. It includes details about the study design, the sampling strategy, the data collection instruments, and the data analysis techniques.
- Data Collection: This section describes how the data will be collected, including the sample size, data collection procedures, and any ethical considerations.
- Data Analysis: This section describes how the data will be analyzed, including the statistical techniques that will be used to test the research questions or hypotheses.
- Results : This section presents the findings of the study, including descriptive statistics and statistical tests.
- Discussion and Conclusion : This section summarizes the key findings of the study, interprets the results, and discusses the implications of the findings. It also includes recommendations for future research.
- References : This section lists the sources cited in the research design.
Example of Research Design
An Example of Research Design could be:
Research question: Does the use of social media affect the academic performance of high school students?
Research design:
- Research approach : The research approach will be quantitative as it involves collecting numerical data to test the hypothesis.
- Research design : The research design will be a quasi-experimental design, with a pretest-posttest control group design.
- Sample : The sample will be 200 high school students from two schools, with 100 students in the experimental group and 100 students in the control group.
- Data collection : The data will be collected through surveys administered to the students at the beginning and end of the academic year. The surveys will include questions about their social media usage and academic performance.
- Data analysis : The data collected will be analyzed using statistical software. The mean scores of the experimental and control groups will be compared to determine whether there is a significant difference in academic performance between the two groups.
- Limitations : The limitations of the study will be acknowledged, including the fact that social media usage can vary greatly among individuals, and the study only focuses on two schools, which may not be representative of the entire population.
- Ethical considerations: Ethical considerations will be taken into account, such as obtaining informed consent from the participants and ensuring their anonymity and confidentiality.
How to Write Research Design
Writing a research design involves planning and outlining the methodology and approach that will be used to answer a research question or hypothesis. Here are some steps to help you write a research design:
- Define the research question or hypothesis : Before beginning your research design, you should clearly define your research question or hypothesis. This will guide your research design and help you select appropriate methods.
- Select a research design: There are many different research designs to choose from, including experimental, survey, case study, and qualitative designs. Choose a design that best fits your research question and objectives.
- Develop a sampling plan : If your research involves collecting data from a sample, you will need to develop a sampling plan. This should outline how you will select participants and how many participants you will include.
- Define variables: Clearly define the variables you will be measuring or manipulating in your study. This will help ensure that your results are meaningful and relevant to your research question.
- Choose data collection methods : Decide on the data collection methods you will use to gather information. This may include surveys, interviews, observations, experiments, or secondary data sources.
- Create a data analysis plan: Develop a plan for analyzing your data, including the statistical or qualitative techniques you will use.
- Consider ethical concerns : Finally, be sure to consider any ethical concerns related to your research, such as participant confidentiality or potential harm.
When to Write Research Design
Research design should be written before conducting any research study. It is an important planning phase that outlines the research methodology, data collection methods, and data analysis techniques that will be used to investigate a research question or problem. The research design helps to ensure that the research is conducted in a systematic and logical manner, and that the data collected is relevant and reliable.
Ideally, the research design should be developed as early as possible in the research process, before any data is collected. This allows the researcher to carefully consider the research question, identify the most appropriate research methodology, and plan the data collection and analysis procedures in advance. By doing so, the research can be conducted in a more efficient and effective manner, and the results are more likely to be valid and reliable.
Purpose of Research Design
The purpose of research design is to plan and structure a research study in a way that enables the researcher to achieve the desired research goals with accuracy, validity, and reliability. Research design is the blueprint or the framework for conducting a study that outlines the methods, procedures, techniques, and tools for data collection and analysis.
Some of the key purposes of research design include:
- Providing a clear and concise plan of action for the research study.
- Ensuring that the research is conducted ethically and with rigor.
- Maximizing the accuracy and reliability of the research findings.
- Minimizing the possibility of errors, biases, or confounding variables.
- Ensuring that the research is feasible, practical, and cost-effective.
- Determining the appropriate research methodology to answer the research question(s).
- Identifying the sample size, sampling method, and data collection techniques.
- Determining the data analysis method and statistical tests to be used.
- Facilitating the replication of the study by other researchers.
- Enhancing the validity and generalizability of the research findings.
Applications of Research Design
There are numerous applications of research design in various fields, some of which are:
- Social sciences: In fields such as psychology, sociology, and anthropology, research design is used to investigate human behavior and social phenomena. Researchers use various research designs, such as experimental, quasi-experimental, and correlational designs, to study different aspects of social behavior.
- Education : Research design is essential in the field of education to investigate the effectiveness of different teaching methods and learning strategies. Researchers use various designs such as experimental, quasi-experimental, and case study designs to understand how students learn and how to improve teaching practices.
- Health sciences : In the health sciences, research design is used to investigate the causes, prevention, and treatment of diseases. Researchers use various designs, such as randomized controlled trials, cohort studies, and case-control studies, to study different aspects of health and healthcare.
- Business : Research design is used in the field of business to investigate consumer behavior, marketing strategies, and the impact of different business practices. Researchers use various designs, such as survey research, experimental research, and case studies, to study different aspects of the business world.
- Engineering : In the field of engineering, research design is used to investigate the development and implementation of new technologies. Researchers use various designs, such as experimental research and case studies, to study the effectiveness of new technologies and to identify areas for improvement.
Advantages of Research Design
Here are some advantages of research design:
- Systematic and organized approach : A well-designed research plan ensures that the research is conducted in a systematic and organized manner, which makes it easier to manage and analyze the data.
- Clear objectives: The research design helps to clarify the objectives of the study, which makes it easier to identify the variables that need to be measured, and the methods that need to be used to collect and analyze data.
- Minimizes bias: A well-designed research plan minimizes the chances of bias, by ensuring that the data is collected and analyzed objectively, and that the results are not influenced by the researcher’s personal biases or preferences.
- Efficient use of resources: A well-designed research plan helps to ensure that the resources (time, money, and personnel) are used efficiently and effectively, by focusing on the most important variables and methods.
- Replicability: A well-designed research plan makes it easier for other researchers to replicate the study, which enhances the credibility and reliability of the findings.
- Validity: A well-designed research plan helps to ensure that the findings are valid, by ensuring that the methods used to collect and analyze data are appropriate for the research question.
- Generalizability : A well-designed research plan helps to ensure that the findings can be generalized to other populations, settings, or situations, which increases the external validity of the study.
Research Design Vs Research Methodology
About the author.
Muhammad Hassan
Researcher, Academic Writer, Web developer
You may also like

Purpose of Research – Objectives and Applications

Data Interpretation – Process, Methods and...

Research Paper Outline – Types, Example, Template

Implications in Research – Types, Examples and...

Figures in Research Paper – Examples and Guide

Data Analysis – Process, Methods and Types
Leave a comment x.
Save my name, email, and website in this browser for the next time I comment.
Have a language expert improve your writing
Run a free plagiarism check in 10 minutes, automatically generate references for free.
- Knowledge Base
- Methodology
Research Design | Step-by-Step Guide with Examples
Published on 5 May 2022 by Shona McCombes . Revised on 20 March 2023.
A research design is a strategy for answering your research question using empirical data. Creating a research design means making decisions about:
- Your overall aims and approach
- The type of research design you’ll use
- Your sampling methods or criteria for selecting subjects
- Your data collection methods
- The procedures you’ll follow to collect data
- Your data analysis methods
A well-planned research design helps ensure that your methods match your research aims and that you use the right kind of analysis for your data.
Table of contents
Step 1: consider your aims and approach, step 2: choose a type of research design, step 3: identify your population and sampling method, step 4: choose your data collection methods, step 5: plan your data collection procedures, step 6: decide on your data analysis strategies, frequently asked questions.
- Introduction
Before you can start designing your research, you should already have a clear idea of the research question you want to investigate.
There are many different ways you could go about answering this question. Your research design choices should be driven by your aims and priorities – start by thinking carefully about what you want to achieve.
The first choice you need to make is whether you’ll take a qualitative or quantitative approach.
Qualitative research designs tend to be more flexible and inductive , allowing you to adjust your approach based on what you find throughout the research process.
Quantitative research designs tend to be more fixed and deductive , with variables and hypotheses clearly defined in advance of data collection.
It’s also possible to use a mixed methods design that integrates aspects of both approaches. By combining qualitative and quantitative insights, you can gain a more complete picture of the problem you’re studying and strengthen the credibility of your conclusions.
Practical and ethical considerations when designing research
As well as scientific considerations, you need to think practically when designing your research. If your research involves people or animals, you also need to consider research ethics .
- How much time do you have to collect data and write up the research?
- Will you be able to gain access to the data you need (e.g., by travelling to a specific location or contacting specific people)?
- Do you have the necessary research skills (e.g., statistical analysis or interview techniques)?
- Will you need ethical approval ?
At each stage of the research design process, make sure that your choices are practically feasible.
Prevent plagiarism, run a free check.
Within both qualitative and quantitative approaches, there are several types of research design to choose from. Each type provides a framework for the overall shape of your research.
Types of quantitative research designs
Quantitative designs can be split into four main types. Experimental and quasi-experimental designs allow you to test cause-and-effect relationships, while descriptive and correlational designs allow you to measure variables and describe relationships between them.
With descriptive and correlational designs, you can get a clear picture of characteristics, trends, and relationships as they exist in the real world. However, you can’t draw conclusions about cause and effect (because correlation doesn’t imply causation ).
Experiments are the strongest way to test cause-and-effect relationships without the risk of other variables influencing the results. However, their controlled conditions may not always reflect how things work in the real world. They’re often also more difficult and expensive to implement.
Types of qualitative research designs
Qualitative designs are less strictly defined. This approach is about gaining a rich, detailed understanding of a specific context or phenomenon, and you can often be more creative and flexible in designing your research.
The table below shows some common types of qualitative design. They often have similar approaches in terms of data collection, but focus on different aspects when analysing the data.
Your research design should clearly define who or what your research will focus on, and how you’ll go about choosing your participants or subjects.
In research, a population is the entire group that you want to draw conclusions about, while a sample is the smaller group of individuals you’ll actually collect data from.
Defining the population
A population can be made up of anything you want to study – plants, animals, organisations, texts, countries, etc. In the social sciences, it most often refers to a group of people.
For example, will you focus on people from a specific demographic, region, or background? Are you interested in people with a certain job or medical condition, or users of a particular product?
The more precisely you define your population, the easier it will be to gather a representative sample.
Sampling methods
Even with a narrowly defined population, it’s rarely possible to collect data from every individual. Instead, you’ll collect data from a sample.
To select a sample, there are two main approaches: probability sampling and non-probability sampling . The sampling method you use affects how confidently you can generalise your results to the population as a whole.
Probability sampling is the most statistically valid option, but it’s often difficult to achieve unless you’re dealing with a very small and accessible population.
For practical reasons, many studies use non-probability sampling, but it’s important to be aware of the limitations and carefully consider potential biases. You should always make an effort to gather a sample that’s as representative as possible of the population.
Case selection in qualitative research
In some types of qualitative designs, sampling may not be relevant.
For example, in an ethnography or a case study, your aim is to deeply understand a specific context, not to generalise to a population. Instead of sampling, you may simply aim to collect as much data as possible about the context you are studying.
In these types of design, you still have to carefully consider your choice of case or community. You should have a clear rationale for why this particular case is suitable for answering your research question.
For example, you might choose a case study that reveals an unusual or neglected aspect of your research problem, or you might choose several very similar or very different cases in order to compare them.
Data collection methods are ways of directly measuring variables and gathering information. They allow you to gain first-hand knowledge and original insights into your research problem.
You can choose just one data collection method, or use several methods in the same study.
Survey methods
Surveys allow you to collect data about opinions, behaviours, experiences, and characteristics by asking people directly. There are two main survey methods to choose from: questionnaires and interviews.
Observation methods
Observations allow you to collect data unobtrusively, observing characteristics, behaviours, or social interactions without relying on self-reporting.
Observations may be conducted in real time, taking notes as you observe, or you might make audiovisual recordings for later analysis. They can be qualitative or quantitative.
Other methods of data collection
There are many other ways you might collect data depending on your field and topic.
If you’re not sure which methods will work best for your research design, try reading some papers in your field to see what data collection methods they used.
Secondary data
If you don’t have the time or resources to collect data from the population you’re interested in, you can also choose to use secondary data that other researchers already collected – for example, datasets from government surveys or previous studies on your topic.
With this raw data, you can do your own analysis to answer new research questions that weren’t addressed by the original study.
Using secondary data can expand the scope of your research, as you may be able to access much larger and more varied samples than you could collect yourself.
However, it also means you don’t have any control over which variables to measure or how to measure them, so the conclusions you can draw may be limited.
As well as deciding on your methods, you need to plan exactly how you’ll use these methods to collect data that’s consistent, accurate, and unbiased.
Planning systematic procedures is especially important in quantitative research, where you need to precisely define your variables and ensure your measurements are reliable and valid.
Operationalisation
Some variables, like height or age, are easily measured. But often you’ll be dealing with more abstract concepts, like satisfaction, anxiety, or competence. Operationalisation means turning these fuzzy ideas into measurable indicators.
If you’re using observations , which events or actions will you count?
If you’re using surveys , which questions will you ask and what range of responses will be offered?
You may also choose to use or adapt existing materials designed to measure the concept you’re interested in – for example, questionnaires or inventories whose reliability and validity has already been established.
Reliability and validity
Reliability means your results can be consistently reproduced , while validity means that you’re actually measuring the concept you’re interested in.
For valid and reliable results, your measurement materials should be thoroughly researched and carefully designed. Plan your procedures to make sure you carry out the same steps in the same way for each participant.
If you’re developing a new questionnaire or other instrument to measure a specific concept, running a pilot study allows you to check its validity and reliability in advance.
Sampling procedures
As well as choosing an appropriate sampling method, you need a concrete plan for how you’ll actually contact and recruit your selected sample.
That means making decisions about things like:
- How many participants do you need for an adequate sample size?
- What inclusion and exclusion criteria will you use to identify eligible participants?
- How will you contact your sample – by mail, online, by phone, or in person?
If you’re using a probability sampling method, it’s important that everyone who is randomly selected actually participates in the study. How will you ensure a high response rate?
If you’re using a non-probability method, how will you avoid bias and ensure a representative sample?
Data management
It’s also important to create a data management plan for organising and storing your data.
Will you need to transcribe interviews or perform data entry for observations? You should anonymise and safeguard any sensitive data, and make sure it’s backed up regularly.
Keeping your data well organised will save time when it comes to analysing them. It can also help other researchers validate and add to your findings.
On their own, raw data can’t answer your research question. The last step of designing your research is planning how you’ll analyse the data.
Quantitative data analysis
In quantitative research, you’ll most likely use some form of statistical analysis . With statistics, you can summarise your sample data, make estimates, and test hypotheses.
Using descriptive statistics , you can summarise your sample data in terms of:
- The distribution of the data (e.g., the frequency of each score on a test)
- The central tendency of the data (e.g., the mean to describe the average score)
- The variability of the data (e.g., the standard deviation to describe how spread out the scores are)
The specific calculations you can do depend on the level of measurement of your variables.
Using inferential statistics , you can:
- Make estimates about the population based on your sample data.
- Test hypotheses about a relationship between variables.
Regression and correlation tests look for associations between two or more variables, while comparison tests (such as t tests and ANOVAs ) look for differences in the outcomes of different groups.
Your choice of statistical test depends on various aspects of your research design, including the types of variables you’re dealing with and the distribution of your data.
Qualitative data analysis
In qualitative research, your data will usually be very dense with information and ideas. Instead of summing it up in numbers, you’ll need to comb through the data in detail, interpret its meanings, identify patterns, and extract the parts that are most relevant to your research question.
Two of the most common approaches to doing this are thematic analysis and discourse analysis .
There are many other ways of analysing qualitative data depending on the aims of your research. To get a sense of potential approaches, try reading some qualitative research papers in your field.
A sample is a subset of individuals from a larger population. Sampling means selecting the group that you will actually collect data from in your research.
For example, if you are researching the opinions of students in your university, you could survey a sample of 100 students.
Statistical sampling allows you to test a hypothesis about the characteristics of a population. There are various sampling methods you can use to ensure that your sample is representative of the population as a whole.
Operationalisation means turning abstract conceptual ideas into measurable observations.
For example, the concept of social anxiety isn’t directly observable, but it can be operationally defined in terms of self-rating scores, behavioural avoidance of crowded places, or physical anxiety symptoms in social situations.
Before collecting data , it’s important to consider how you will operationalise the variables that you want to measure.
The research methods you use depend on the type of data you need to answer your research question .
- If you want to measure something or test a hypothesis , use quantitative methods . If you want to explore ideas, thoughts, and meanings, use qualitative methods .
- If you want to analyse a large amount of readily available data, use secondary data. If you want data specific to your purposes with control over how they are generated, collect primary data.
- If you want to establish cause-and-effect relationships between variables , use experimental methods. If you want to understand the characteristics of a research subject, use descriptive methods.
Cite this Scribbr article
If you want to cite this source, you can copy and paste the citation or click the ‘Cite this Scribbr article’ button to automatically add the citation to our free Reference Generator.
McCombes, S. (2023, March 20). Research Design | Step-by-Step Guide with Examples. Scribbr. Retrieved 28 October 2024, from https://www.scribbr.co.uk/research-methods/research-design/
Is this article helpful?
Shona McCombes
- USC Libraries
- Research Guides
Organizing Your Social Sciences Research Paper
- Types of Research Designs
- Purpose of Guide
- Design Flaws to Avoid
- Independent and Dependent Variables
- Glossary of Research Terms
- Reading Research Effectively
- Narrowing a Topic Idea
- Broadening a Topic Idea
- Extending the Timeliness of a Topic Idea
- Academic Writing Style
- Applying Critical Thinking
- Choosing a Title
- Making an Outline
- Paragraph Development
- Research Process Video Series
- Executive Summary
- The C.A.R.S. Model
- Background Information
- The Research Problem/Question
- Theoretical Framework
- Citation Tracking
- Content Alert Services
- Evaluating Sources
- Primary Sources
- Secondary Sources
- Tiertiary Sources
- Scholarly vs. Popular Publications
- Qualitative Methods
- Quantitative Methods
- Insiderness
- Using Non-Textual Elements
- Limitations of the Study
- Common Grammar Mistakes
- Writing Concisely
- Avoiding Plagiarism
- Footnotes or Endnotes?
- Further Readings
- Generative AI and Writing
- USC Libraries Tutorials and Other Guides
- Bibliography
Introduction
Before beginning your paper, you need to decide how you plan to design the study .
The research design refers to the overall strategy and analytical approach that you have chosen in order to integrate, in a coherent and logical way, the different components of the study, thus ensuring that the research problem will be thoroughly investigated. It constitutes the blueprint for the collection, measurement, and interpretation of information and data. Note that the research problem determines the type of design you choose, not the other way around!
De Vaus, D. A. Research Design in Social Research . London: SAGE, 2001; Trochim, William M.K. Research Methods Knowledge Base. 2006.
General Structure and Writing Style
The function of a research design is to ensure that the evidence obtained enables you to effectively address the research problem logically and as unambiguously as possible . In social sciences research, obtaining information relevant to the research problem generally entails specifying the type of evidence needed to test the underlying assumptions of a theory, to evaluate a program, or to accurately describe and assess meaning related to an observable phenomenon.
With this in mind, a common mistake made by researchers is that they begin their investigations before they have thought critically about what information is required to address the research problem. Without attending to these design issues beforehand, the overall research problem will not be adequately addressed and any conclusions drawn will run the risk of being weak and unconvincing. As a consequence, the overall validity of the study will be undermined.
The length and complexity of describing the research design in your paper can vary considerably, but any well-developed description will achieve the following :
- Identify the research problem clearly and justify its selection, particularly in relation to any valid alternative designs that could have been used,
- Review and synthesize previously published literature associated with the research problem,
- Clearly and explicitly specify hypotheses [i.e., research questions] central to the problem,
- Effectively describe the information and/or data which will be necessary for an adequate testing of the hypotheses and explain how such information and/or data will be obtained, and
- Describe the methods of analysis to be applied to the data in determining whether or not the hypotheses are true or false.
The research design is usually incorporated into the introduction of your paper . You can obtain an overall sense of what to do by reviewing studies that have utilized the same research design [e.g., using a case study approach]. This can help you develop an outline to follow for your own paper.
NOTE: Use the SAGE Research Methods Online and Cases and the SAGE Research Methods Videos databases to search for scholarly resources on how to apply specific research designs and methods . The Research Methods Online database contains links to more than 175,000 pages of SAGE publisher's book, journal, and reference content on quantitative, qualitative, and mixed research methodologies. Also included is a collection of case studies of social research projects that can be used to help you better understand abstract or complex methodological concepts. The Research Methods Videos database contains hours of tutorials, interviews, video case studies, and mini-documentaries covering the entire research process.
Creswell, John W. and J. David Creswell. Research Design: Qualitative, Quantitative, and Mixed Methods Approaches . 5th edition. Thousand Oaks, CA: Sage, 2018; De Vaus, D. A. Research Design in Social Research . London: SAGE, 2001; Gorard, Stephen. Research Design: Creating Robust Approaches for the Social Sciences . Thousand Oaks, CA: Sage, 2013; Leedy, Paul D. and Jeanne Ellis Ormrod. Practical Research: Planning and Design . Tenth edition. Boston, MA: Pearson, 2013; Vogt, W. Paul, Dianna C. Gardner, and Lynne M. Haeffele. When to Use What Research Design . New York: Guilford, 2012.
Action Research Design
Definition and Purpose
The essentials of action research design follow a characteristic cycle whereby initially an exploratory stance is adopted, where an understanding of a problem is developed and plans are made for some form of interventionary strategy. Then the intervention is carried out [the "action" in action research] during which time, pertinent observations are collected in various forms. The new interventional strategies are carried out, and this cyclic process repeats, continuing until a sufficient understanding of [or a valid implementation solution for] the problem is achieved. The protocol is iterative or cyclical in nature and is intended to foster deeper understanding of a given situation, starting with conceptualizing and particularizing the problem and moving through several interventions and evaluations.
What do these studies tell you ?
- This is a collaborative and adaptive research design that lends itself to use in work or community situations.
- Design focuses on pragmatic and solution-driven research outcomes rather than testing theories.
- When practitioners use action research, it has the potential to increase the amount they learn consciously from their experience; the action research cycle can be regarded as a learning cycle.
- Action research studies often have direct and obvious relevance to improving practice and advocating for change.
- There are no hidden controls or preemption of direction by the researcher.
What these studies don't tell you ?
- It is harder to do than conducting conventional research because the researcher takes on responsibilities of advocating for change as well as for researching the topic.
- Action research is much harder to write up because it is less likely that you can use a standard format to report your findings effectively [i.e., data is often in the form of stories or observation].
- Personal over-involvement of the researcher may bias research results.
- The cyclic nature of action research to achieve its twin outcomes of action [e.g. change] and research [e.g. understanding] is time-consuming and complex to conduct.
- Advocating for change usually requires buy-in from study participants.
Coghlan, David and Mary Brydon-Miller. The Sage Encyclopedia of Action Research . Thousand Oaks, CA: Sage, 2014; Efron, Sara Efrat and Ruth Ravid. Action Research in Education: A Practical Guide . New York: Guilford, 2013; Gall, Meredith. Educational Research: An Introduction . Chapter 18, Action Research. 8th ed. Boston, MA: Pearson/Allyn and Bacon, 2007; Gorard, Stephen. Research Design: Creating Robust Approaches for the Social Sciences . Thousand Oaks, CA: Sage, 2013; Kemmis, Stephen and Robin McTaggart. “Participatory Action Research.” In Handbook of Qualitative Research . Norman Denzin and Yvonna S. Lincoln, eds. 2nd ed. (Thousand Oaks, CA: SAGE, 2000), pp. 567-605; McNiff, Jean. Writing and Doing Action Research . London: Sage, 2014; Reason, Peter and Hilary Bradbury. Handbook of Action Research: Participative Inquiry and Practice . Thousand Oaks, CA: SAGE, 2001.
Case Study Design
A case study is an in-depth study of a particular research problem rather than a sweeping statistical survey or comprehensive comparative inquiry. It is often used to narrow down a very broad field of research into one or a few easily researchable examples. The case study research design is also useful for testing whether a specific theory and model actually applies to phenomena in the real world. It is a useful design when not much is known about an issue or phenomenon.
- Approach excels at bringing us to an understanding of a complex issue through detailed contextual analysis of a limited number of events or conditions and their relationships.
- A researcher using a case study design can apply a variety of methodologies and rely on a variety of sources to investigate a research problem.
- Design can extend experience or add strength to what is already known through previous research.
- Social scientists, in particular, make wide use of this research design to examine contemporary real-life situations and provide the basis for the application of concepts and theories and the extension of methodologies.
- The design can provide detailed descriptions of specific and rare cases.
- A single or small number of cases offers little basis for establishing reliability or to generalize the findings to a wider population of people, places, or things.
- Intense exposure to the study of a case may bias a researcher's interpretation of the findings.
- Design does not facilitate assessment of cause and effect relationships.
- Vital information may be missing, making the case hard to interpret.
- The case may not be representative or typical of the larger problem being investigated.
- If the criteria for selecting a case is because it represents a very unusual or unique phenomenon or problem for study, then your interpretation of the findings can only apply to that particular case.
Case Studies. Writing@CSU. Colorado State University; Anastas, Jeane W. Research Design for Social Work and the Human Services . Chapter 4, Flexible Methods: Case Study Design. 2nd ed. New York: Columbia University Press, 1999; Gerring, John. “What Is a Case Study and What Is It Good for?” American Political Science Review 98 (May 2004): 341-354; Greenhalgh, Trisha, editor. Case Study Evaluation: Past, Present and Future Challenges . Bingley, UK: Emerald Group Publishing, 2015; Mills, Albert J. , Gabrielle Durepos, and Eiden Wiebe, editors. Encyclopedia of Case Study Research . Thousand Oaks, CA: SAGE Publications, 2010; Stake, Robert E. The Art of Case Study Research . Thousand Oaks, CA: SAGE, 1995; Yin, Robert K. Case Study Research: Design and Theory . Applied Social Research Methods Series, no. 5. 3rd ed. Thousand Oaks, CA: SAGE, 2003.
Causal Design
Causality studies may be thought of as understanding a phenomenon in terms of conditional statements in the form, “If X, then Y.” This type of research is used to measure what impact a specific change will have on existing norms and assumptions. Most social scientists seek causal explanations that reflect tests of hypotheses. Causal effect (nomothetic perspective) occurs when variation in one phenomenon, an independent variable, leads to or results, on average, in variation in another phenomenon, the dependent variable.
Conditions necessary for determining causality:
- Empirical association -- a valid conclusion is based on finding an association between the independent variable and the dependent variable.
- Appropriate time order -- to conclude that causation was involved, one must see that cases were exposed to variation in the independent variable before variation in the dependent variable.
- Nonspuriousness -- a relationship between two variables that is not due to variation in a third variable.
- Causality research designs assist researchers in understanding why the world works the way it does through the process of proving a causal link between variables and by the process of eliminating other possibilities.
- Replication is possible.
- There is greater confidence the study has internal validity due to the systematic subject selection and equity of groups being compared.
- Not all relationships are causal! The possibility always exists that, by sheer coincidence, two unrelated events appear to be related [e.g., Punxatawney Phil could accurately predict the duration of Winter for five consecutive years but, the fact remains, he's just a big, furry rodent].
- Conclusions about causal relationships are difficult to determine due to a variety of extraneous and confounding variables that exist in a social environment. This means causality can only be inferred, never proven.
- If two variables are correlated, the cause must come before the effect. However, even though two variables might be causally related, it can sometimes be difficult to determine which variable comes first and, therefore, to establish which variable is the actual cause and which is the actual effect.
Beach, Derek and Rasmus Brun Pedersen. Causal Case Study Methods: Foundations and Guidelines for Comparing, Matching, and Tracing . Ann Arbor, MI: University of Michigan Press, 2016; Bachman, Ronet. The Practice of Research in Criminology and Criminal Justice . Chapter 5, Causation and Research Designs. 3rd ed. Thousand Oaks, CA: Pine Forge Press, 2007; Brewer, Ernest W. and Jennifer Kubn. “Causal-Comparative Design.” In Encyclopedia of Research Design . Neil J. Salkind, editor. (Thousand Oaks, CA: Sage, 2010), pp. 125-132; Causal Research Design: Experimentation. Anonymous SlideShare Presentation; Gall, Meredith. Educational Research: An Introduction . Chapter 11, Nonexperimental Research: Correlational Designs. 8th ed. Boston, MA: Pearson/Allyn and Bacon, 2007; Trochim, William M.K. Research Methods Knowledge Base. 2006.
Cohort Design
Often used in the medical sciences, but also found in the applied social sciences, a cohort study generally refers to a study conducted over a period of time involving members of a population which the subject or representative member comes from, and who are united by some commonality or similarity. Using a quantitative framework, a cohort study makes note of statistical occurrence within a specialized subgroup, united by same or similar characteristics that are relevant to the research problem being investigated, rather than studying statistical occurrence within the general population. Using a qualitative framework, cohort studies generally gather data using methods of observation. Cohorts can be either "open" or "closed."
- Open Cohort Studies [dynamic populations, such as the population of Los Angeles] involve a population that is defined just by the state of being a part of the study in question (and being monitored for the outcome). Date of entry and exit from the study is individually defined, therefore, the size of the study population is not constant. In open cohort studies, researchers can only calculate rate based data, such as, incidence rates and variants thereof.
- Closed Cohort Studies [static populations, such as patients entered into a clinical trial] involve participants who enter into the study at one defining point in time and where it is presumed that no new participants can enter the cohort. Given this, the number of study participants remains constant (or can only decrease).
- The use of cohorts is often mandatory because a randomized control study may be unethical. For example, you cannot deliberately expose people to asbestos, you can only study its effects on those who have already been exposed. Research that measures risk factors often relies upon cohort designs.
- Because cohort studies measure potential causes before the outcome has occurred, they can demonstrate that these “causes” preceded the outcome, thereby avoiding the debate as to which is the cause and which is the effect.
- Cohort analysis is highly flexible and can provide insight into effects over time and related to a variety of different types of changes [e.g., social, cultural, political, economic, etc.].
- Either original data or secondary data can be used in this design.
- In cases where a comparative analysis of two cohorts is made [e.g., studying the effects of one group exposed to asbestos and one that has not], a researcher cannot control for all other factors that might differ between the two groups. These factors are known as confounding variables.
- Cohort studies can end up taking a long time to complete if the researcher must wait for the conditions of interest to develop within the group. This also increases the chance that key variables change during the course of the study, potentially impacting the validity of the findings.
- Due to the lack of randominization in the cohort design, its external validity is lower than that of study designs where the researcher randomly assigns participants.
Healy P, Devane D. “Methodological Considerations in Cohort Study Designs.” Nurse Researcher 18 (2011): 32-36; Glenn, Norval D, editor. Cohort Analysis . 2nd edition. Thousand Oaks, CA: Sage, 2005; Levin, Kate Ann. Study Design IV: Cohort Studies. Evidence-Based Dentistry 7 (2003): 51–52; Payne, Geoff. “Cohort Study.” In The SAGE Dictionary of Social Research Methods . Victor Jupp, editor. (Thousand Oaks, CA: Sage, 2006), pp. 31-33; Study Design 101. Himmelfarb Health Sciences Library. George Washington University, November 2011; Cohort Study. Wikipedia.
Cross-Sectional Design
Cross-sectional research designs have three distinctive features: no time dimension; a reliance on existing differences rather than change following intervention; and, groups are selected based on existing differences rather than random allocation. The cross-sectional design can only measure differences between or from among a variety of people, subjects, or phenomena rather than a process of change. As such, researchers using this design can only employ a relatively passive approach to making causal inferences based on findings.
- Cross-sectional studies provide a clear 'snapshot' of the outcome and the characteristics associated with it, at a specific point in time.
- Unlike an experimental design, where there is an active intervention by the researcher to produce and measure change or to create differences, cross-sectional designs focus on studying and drawing inferences from existing differences between people, subjects, or phenomena.
- Entails collecting data at and concerning one point in time. While longitudinal studies involve taking multiple measures over an extended period of time, cross-sectional research is focused on finding relationships between variables at one moment in time.
- Groups identified for study are purposely selected based upon existing differences in the sample rather than seeking random sampling.
- Cross-section studies are capable of using data from a large number of subjects and, unlike observational studies, is not geographically bound.
- Can estimate prevalence of an outcome of interest because the sample is usually taken from the whole population.
- Because cross-sectional designs generally use survey techniques to gather data, they are relatively inexpensive and take up little time to conduct.
- Finding people, subjects, or phenomena to study that are very similar except in one specific variable can be difficult.
- Results are static and time bound and, therefore, give no indication of a sequence of events or reveal historical or temporal contexts.
- Studies cannot be utilized to establish cause and effect relationships.
- This design only provides a snapshot of analysis so there is always the possibility that a study could have differing results if another time-frame had been chosen.
- There is no follow up to the findings.
Bethlehem, Jelke. "7: Cross-sectional Research." In Research Methodology in the Social, Behavioural and Life Sciences . Herman J Adèr and Gideon J Mellenbergh, editors. (London, England: Sage, 1999), pp. 110-43; Bourque, Linda B. “Cross-Sectional Design.” In The SAGE Encyclopedia of Social Science Research Methods . Michael S. Lewis-Beck, Alan Bryman, and Tim Futing Liao. (Thousand Oaks, CA: 2004), pp. 230-231; Hall, John. “Cross-Sectional Survey Design.” In Encyclopedia of Survey Research Methods . Paul J. Lavrakas, ed. (Thousand Oaks, CA: Sage, 2008), pp. 173-174; Helen Barratt, Maria Kirwan. Cross-Sectional Studies: Design Application, Strengths and Weaknesses of Cross-Sectional Studies. Healthknowledge, 2009. Cross-Sectional Study. Wikipedia.
Descriptive Design
Descriptive research designs help provide answers to the questions of who, what, when, where, and how associated with a particular research problem; a descriptive study cannot conclusively ascertain answers to why. Descriptive research is used to obtain information concerning the current status of the phenomena and to describe "what exists" with respect to variables or conditions in a situation.
- The subject is being observed in a completely natural and unchanged natural environment. True experiments, whilst giving analyzable data, often adversely influence the normal behavior of the subject [a.k.a., the Heisenberg effect whereby measurements of certain systems cannot be made without affecting the systems].
- Descriptive research is often used as a pre-cursor to more quantitative research designs with the general overview giving some valuable pointers as to what variables are worth testing quantitatively.
- If the limitations are understood, they can be a useful tool in developing a more focused study.
- Descriptive studies can yield rich data that lead to important recommendations in practice.
- Appoach collects a large amount of data for detailed analysis.
- The results from a descriptive research cannot be used to discover a definitive answer or to disprove a hypothesis.
- Because descriptive designs often utilize observational methods [as opposed to quantitative methods], the results cannot be replicated.
- The descriptive function of research is heavily dependent on instrumentation for measurement and observation.
Anastas, Jeane W. Research Design for Social Work and the Human Services . Chapter 5, Flexible Methods: Descriptive Research. 2nd ed. New York: Columbia University Press, 1999; Given, Lisa M. "Descriptive Research." In Encyclopedia of Measurement and Statistics . Neil J. Salkind and Kristin Rasmussen, editors. (Thousand Oaks, CA: Sage, 2007), pp. 251-254; McNabb, Connie. Descriptive Research Methodologies. Powerpoint Presentation; Shuttleworth, Martyn. Descriptive Research Design, September 26, 2008; Erickson, G. Scott. "Descriptive Research Design." In New Methods of Market Research and Analysis . (Northampton, MA: Edward Elgar Publishing, 2017), pp. 51-77; Sahin, Sagufta, and Jayanta Mete. "A Brief Study on Descriptive Research: Its Nature and Application in Social Science." International Journal of Research and Analysis in Humanities 1 (2021): 11; K. Swatzell and P. Jennings. “Descriptive Research: The Nuts and Bolts.” Journal of the American Academy of Physician Assistants 20 (2007), pp. 55-56; Kane, E. Doing Your Own Research: Basic Descriptive Research in the Social Sciences and Humanities . London: Marion Boyars, 1985.
Experimental Design
A blueprint of the procedure that enables the researcher to maintain control over all factors that may affect the result of an experiment. In doing this, the researcher attempts to determine or predict what may occur. Experimental research is often used where there is time priority in a causal relationship (cause precedes effect), there is consistency in a causal relationship (a cause will always lead to the same effect), and the magnitude of the correlation is great. The classic experimental design specifies an experimental group and a control group. The independent variable is administered to the experimental group and not to the control group, and both groups are measured on the same dependent variable. Subsequent experimental designs have used more groups and more measurements over longer periods. True experiments must have control, randomization, and manipulation.
- Experimental research allows the researcher to control the situation. In so doing, it allows researchers to answer the question, “What causes something to occur?”
- Permits the researcher to identify cause and effect relationships between variables and to distinguish placebo effects from treatment effects.
- Experimental research designs support the ability to limit alternative explanations and to infer direct causal relationships in the study.
- Approach provides the highest level of evidence for single studies.
- The design is artificial, and results may not generalize well to the real world.
- The artificial settings of experiments may alter the behaviors or responses of participants.
- Experimental designs can be costly if special equipment or facilities are needed.
- Some research problems cannot be studied using an experiment because of ethical or technical reasons.
- Difficult to apply ethnographic and other qualitative methods to experimentally designed studies.
Anastas, Jeane W. Research Design for Social Work and the Human Services . Chapter 7, Flexible Methods: Experimental Research. 2nd ed. New York: Columbia University Press, 1999; Chapter 2: Research Design, Experimental Designs. School of Psychology, University of New England, 2000; Chow, Siu L. "Experimental Design." In Encyclopedia of Research Design . Neil J. Salkind, editor. (Thousand Oaks, CA: Sage, 2010), pp. 448-453; "Experimental Design." In Social Research Methods . Nicholas Walliman, editor. (London, England: Sage, 2006), pp, 101-110; Experimental Research. Research Methods by Dummies. Department of Psychology. California State University, Fresno, 2006; Kirk, Roger E. Experimental Design: Procedures for the Behavioral Sciences . 4th edition. Thousand Oaks, CA: Sage, 2013; Trochim, William M.K. Experimental Design. Research Methods Knowledge Base. 2006; Rasool, Shafqat. Experimental Research. Slideshare presentation.
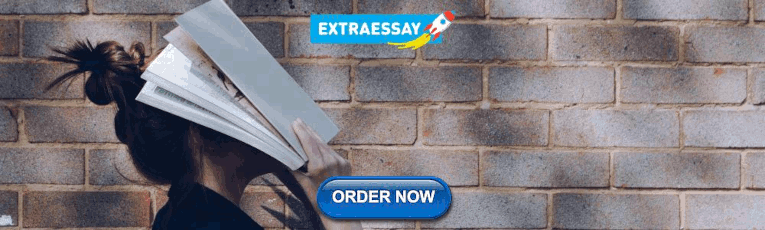
Exploratory Design
An exploratory design is conducted about a research problem when there are few or no earlier studies to refer to or rely upon to predict an outcome . The focus is on gaining insights and familiarity for later investigation or undertaken when research problems are in a preliminary stage of investigation. Exploratory designs are often used to establish an understanding of how best to proceed in studying an issue or what methodology would effectively apply to gathering information about the issue.
The goals of exploratory research are intended to produce the following possible insights:
- Familiarity with basic details, settings, and concerns.
- Well grounded picture of the situation being developed.
- Generation of new ideas and assumptions.
- Development of tentative theories or hypotheses.
- Determination about whether a study is feasible in the future.
- Issues get refined for more systematic investigation and formulation of new research questions.
- Direction for future research and techniques get developed.
- Design is a useful approach for gaining background information on a particular topic.
- Exploratory research is flexible and can address research questions of all types (what, why, how).
- Provides an opportunity to define new terms and clarify existing concepts.
- Exploratory research is often used to generate formal hypotheses and develop more precise research problems.
- In the policy arena or applied to practice, exploratory studies help establish research priorities and where resources should be allocated.
- Exploratory research generally utilizes small sample sizes and, thus, findings are typically not generalizable to the population at large.
- The exploratory nature of the research inhibits an ability to make definitive conclusions about the findings. They provide insight but not definitive conclusions.
- The research process underpinning exploratory studies is flexible but often unstructured, leading to only tentative results that have limited value to decision-makers.
- Design lacks rigorous standards applied to methods of data gathering and analysis because one of the areas for exploration could be to determine what method or methodologies could best fit the research problem.
Cuthill, Michael. “Exploratory Research: Citizen Participation, Local Government, and Sustainable Development in Australia.” Sustainable Development 10 (2002): 79-89; Streb, Christoph K. "Exploratory Case Study." In Encyclopedia of Case Study Research . Albert J. Mills, Gabrielle Durepos and Eiden Wiebe, editors. (Thousand Oaks, CA: Sage, 2010), pp. 372-374; Taylor, P. J., G. Catalano, and D.R.F. Walker. “Exploratory Analysis of the World City Network.” Urban Studies 39 (December 2002): 2377-2394; Exploratory Research. Wikipedia.
Field Research Design
Sometimes referred to as ethnography or participant observation, designs around field research encompass a variety of interpretative procedures [e.g., observation and interviews] rooted in qualitative approaches to studying people individually or in groups while inhabiting their natural environment as opposed to using survey instruments or other forms of impersonal methods of data gathering. Information acquired from observational research takes the form of “ field notes ” that involves documenting what the researcher actually sees and hears while in the field. Findings do not consist of conclusive statements derived from numbers and statistics because field research involves analysis of words and observations of behavior. Conclusions, therefore, are developed from an interpretation of findings that reveal overriding themes, concepts, and ideas. More information can be found HERE .
- Field research is often necessary to fill gaps in understanding the research problem applied to local conditions or to specific groups of people that cannot be ascertained from existing data.
- The research helps contextualize already known information about a research problem, thereby facilitating ways to assess the origins, scope, and scale of a problem and to gage the causes, consequences, and means to resolve an issue based on deliberate interaction with people in their natural inhabited spaces.
- Enables the researcher to corroborate or confirm data by gathering additional information that supports or refutes findings reported in prior studies of the topic.
- Because the researcher in embedded in the field, they are better able to make observations or ask questions that reflect the specific cultural context of the setting being investigated.
- Observing the local reality offers the opportunity to gain new perspectives or obtain unique data that challenges existing theoretical propositions or long-standing assumptions found in the literature.
What these studies don't tell you
- A field research study requires extensive time and resources to carry out the multiple steps involved with preparing for the gathering of information, including for example, examining background information about the study site, obtaining permission to access the study site, and building trust and rapport with subjects.
- Requires a commitment to staying engaged in the field to ensure that you can adequately document events and behaviors as they unfold.
- The unpredictable nature of fieldwork means that researchers can never fully control the process of data gathering. They must maintain a flexible approach to studying the setting because events and circumstances can change quickly or unexpectedly.
- Findings can be difficult to interpret and verify without access to documents and other source materials that help to enhance the credibility of information obtained from the field [i.e., the act of triangulating the data].
- Linking the research problem to the selection of study participants inhabiting their natural environment is critical. However, this specificity limits the ability to generalize findings to different situations or in other contexts or to infer courses of action applied to other settings or groups of people.
- The reporting of findings must take into account how the researcher themselves may have inadvertently affected respondents and their behaviors.
Historical Design
The purpose of a historical research design is to collect, verify, and synthesize evidence from the past to establish facts that defend or refute a hypothesis. It uses secondary sources and a variety of primary documentary evidence, such as, diaries, official records, reports, archives, and non-textual information [maps, pictures, audio and visual recordings]. The limitation is that the sources must be both authentic and valid.
- The historical research design is unobtrusive; the act of research does not affect the results of the study.
- The historical approach is well suited for trend analysis.
- Historical records can add important contextual background required to more fully understand and interpret a research problem.
- There is often no possibility of researcher-subject interaction that could affect the findings.
- Historical sources can be used over and over to study different research problems or to replicate a previous study.
- The ability to fulfill the aims of your research are directly related to the amount and quality of documentation available to understand the research problem.
- Since historical research relies on data from the past, there is no way to manipulate it to control for contemporary contexts.
- Interpreting historical sources can be very time consuming.
- The sources of historical materials must be archived consistently to ensure access. This may especially challenging for digital or online-only sources.
- Original authors bring their own perspectives and biases to the interpretation of past events and these biases are more difficult to ascertain in historical resources.
- Due to the lack of control over external variables, historical research is very weak with regard to the demands of internal validity.
- It is rare that the entirety of historical documentation needed to fully address a research problem is available for interpretation, therefore, gaps need to be acknowledged.
Howell, Martha C. and Walter Prevenier. From Reliable Sources: An Introduction to Historical Methods . Ithaca, NY: Cornell University Press, 2001; Lundy, Karen Saucier. "Historical Research." In The Sage Encyclopedia of Qualitative Research Methods . Lisa M. Given, editor. (Thousand Oaks, CA: Sage, 2008), pp. 396-400; Marius, Richard. and Melvin E. Page. A Short Guide to Writing about History . 9th edition. Boston, MA: Pearson, 2015; Savitt, Ronald. “Historical Research in Marketing.” Journal of Marketing 44 (Autumn, 1980): 52-58; Gall, Meredith. Educational Research: An Introduction . Chapter 16, Historical Research. 8th ed. Boston, MA: Pearson/Allyn and Bacon, 2007.
Longitudinal Design
A longitudinal study follows the same sample over time and makes repeated observations. For example, with longitudinal surveys, the same group of people is interviewed at regular intervals, enabling researchers to track changes over time and to relate them to variables that might explain why the changes occur. Longitudinal research designs describe patterns of change and help establish the direction and magnitude of causal relationships. Measurements are taken on each variable over two or more distinct time periods. This allows the researcher to measure change in variables over time. It is a type of observational study sometimes referred to as a panel study.
- Longitudinal data facilitate the analysis of the duration of a particular phenomenon.
- Enables survey researchers to get close to the kinds of causal explanations usually attainable only with experiments.
- The design permits the measurement of differences or change in a variable from one period to another [i.e., the description of patterns of change over time].
- Longitudinal studies facilitate the prediction of future outcomes based upon earlier factors.
- The data collection method may change over time.
- Maintaining the integrity of the original sample can be difficult over an extended period of time.
- It can be difficult to show more than one variable at a time.
- This design often needs qualitative research data to explain fluctuations in the results.
- A longitudinal research design assumes present trends will continue unchanged.
- It can take a long period of time to gather results.
- There is a need to have a large sample size and accurate sampling to reach representativness.
Anastas, Jeane W. Research Design for Social Work and the Human Services . Chapter 6, Flexible Methods: Relational and Longitudinal Research. 2nd ed. New York: Columbia University Press, 1999; Forgues, Bernard, and Isabelle Vandangeon-Derumez. "Longitudinal Analyses." In Doing Management Research . Raymond-Alain Thiétart and Samantha Wauchope, editors. (London, England: Sage, 2001), pp. 332-351; Kalaian, Sema A. and Rafa M. Kasim. "Longitudinal Studies." In Encyclopedia of Survey Research Methods . Paul J. Lavrakas, ed. (Thousand Oaks, CA: Sage, 2008), pp. 440-441; Menard, Scott, editor. Longitudinal Research . Thousand Oaks, CA: Sage, 2002; Ployhart, Robert E. and Robert J. Vandenberg. "Longitudinal Research: The Theory, Design, and Analysis of Change.” Journal of Management 36 (January 2010): 94-120; Longitudinal Study. Wikipedia.
Meta-Analysis Design
Meta-analysis is an analytical methodology designed to systematically evaluate and summarize the results from a number of individual studies, thereby, increasing the overall sample size and the ability of the researcher to study effects of interest. The purpose is to not simply summarize existing knowledge, but to develop a new understanding of a research problem using synoptic reasoning. The main objectives of meta-analysis include analyzing differences in the results among studies and increasing the precision by which effects are estimated. A well-designed meta-analysis depends upon strict adherence to the criteria used for selecting studies and the availability of information in each study to properly analyze their findings. Lack of information can severely limit the type of analyzes and conclusions that can be reached. In addition, the more dissimilarity there is in the results among individual studies [heterogeneity], the more difficult it is to justify interpretations that govern a valid synopsis of results. A meta-analysis needs to fulfill the following requirements to ensure the validity of your findings:
- Clearly defined description of objectives, including precise definitions of the variables and outcomes that are being evaluated;
- A well-reasoned and well-documented justification for identification and selection of the studies;
- Assessment and explicit acknowledgment of any researcher bias in the identification and selection of those studies;
- Description and evaluation of the degree of heterogeneity among the sample size of studies reviewed; and,
- Justification of the techniques used to evaluate the studies.
- Can be an effective strategy for determining gaps in the literature.
- Provides a means of reviewing research published about a particular topic over an extended period of time and from a variety of sources.
- Is useful in clarifying what policy or programmatic actions can be justified on the basis of analyzing research results from multiple studies.
- Provides a method for overcoming small sample sizes in individual studies that previously may have had little relationship to each other.
- Can be used to generate new hypotheses or highlight research problems for future studies.
- Small violations in defining the criteria used for content analysis can lead to difficult to interpret and/or meaningless findings.
- A large sample size can yield reliable, but not necessarily valid, results.
- A lack of uniformity regarding, for example, the type of literature reviewed, how methods are applied, and how findings are measured within the sample of studies you are analyzing, can make the process of synthesis difficult to perform.
- Depending on the sample size, the process of reviewing and synthesizing multiple studies can be very time consuming.
Beck, Lewis W. "The Synoptic Method." The Journal of Philosophy 36 (1939): 337-345; Cooper, Harris, Larry V. Hedges, and Jeffrey C. Valentine, eds. The Handbook of Research Synthesis and Meta-Analysis . 2nd edition. New York: Russell Sage Foundation, 2009; Guzzo, Richard A., Susan E. Jackson and Raymond A. Katzell. “Meta-Analysis Analysis.” In Research in Organizational Behavior , Volume 9. (Greenwich, CT: JAI Press, 1987), pp 407-442; Lipsey, Mark W. and David B. Wilson. Practical Meta-Analysis . Thousand Oaks, CA: Sage Publications, 2001; Study Design 101. Meta-Analysis. The Himmelfarb Health Sciences Library, George Washington University; Timulak, Ladislav. “Qualitative Meta-Analysis.” In The SAGE Handbook of Qualitative Data Analysis . Uwe Flick, editor. (Los Angeles, CA: Sage, 2013), pp. 481-495; Walker, Esteban, Adrian V. Hernandez, and Micheal W. Kattan. "Meta-Analysis: It's Strengths and Limitations." Cleveland Clinic Journal of Medicine 75 (June 2008): 431-439.
Mixed-Method Design
- Narrative and non-textual information can add meaning to numeric data, while numeric data can add precision to narrative and non-textual information.
- Can utilize existing data while at the same time generating and testing a grounded theory approach to describe and explain the phenomenon under study.
- A broader, more complex research problem can be investigated because the researcher is not constrained by using only one method.
- The strengths of one method can be used to overcome the inherent weaknesses of another method.
- Can provide stronger, more robust evidence to support a conclusion or set of recommendations.
- May generate new knowledge new insights or uncover hidden insights, patterns, or relationships that a single methodological approach might not reveal.
- Produces more complete knowledge and understanding of the research problem that can be used to increase the generalizability of findings applied to theory or practice.
- A researcher must be proficient in understanding how to apply multiple methods to investigating a research problem as well as be proficient in optimizing how to design a study that coherently melds them together.
- Can increase the likelihood of conflicting results or ambiguous findings that inhibit drawing a valid conclusion or setting forth a recommended course of action [e.g., sample interview responses do not support existing statistical data].
- Because the research design can be very complex, reporting the findings requires a well-organized narrative, clear writing style, and precise word choice.
- Design invites collaboration among experts. However, merging different investigative approaches and writing styles requires more attention to the overall research process than studies conducted using only one methodological paradigm.
- Concurrent merging of quantitative and qualitative research requires greater attention to having adequate sample sizes, using comparable samples, and applying a consistent unit of analysis. For sequential designs where one phase of qualitative research builds on the quantitative phase or vice versa, decisions about what results from the first phase to use in the next phase, the choice of samples and estimating reasonable sample sizes for both phases, and the interpretation of results from both phases can be difficult.
- Due to multiple forms of data being collected and analyzed, this design requires extensive time and resources to carry out the multiple steps involved in data gathering and interpretation.
Burch, Patricia and Carolyn J. Heinrich. Mixed Methods for Policy Research and Program Evaluation . Thousand Oaks, CA: Sage, 2016; Creswell, John w. et al. Best Practices for Mixed Methods Research in the Health Sciences . Bethesda, MD: Office of Behavioral and Social Sciences Research, National Institutes of Health, 2010Creswell, John W. Research Design: Qualitative, Quantitative, and Mixed Methods Approaches . 4th edition. Thousand Oaks, CA: Sage Publications, 2014; Domínguez, Silvia, editor. Mixed Methods Social Networks Research . Cambridge, UK: Cambridge University Press, 2014; Hesse-Biber, Sharlene Nagy. Mixed Methods Research: Merging Theory with Practice . New York: Guilford Press, 2010; Niglas, Katrin. “How the Novice Researcher Can Make Sense of Mixed Methods Designs.” International Journal of Multiple Research Approaches 3 (2009): 34-46; Onwuegbuzie, Anthony J. and Nancy L. Leech. “Linking Research Questions to Mixed Methods Data Analysis Procedures.” The Qualitative Report 11 (September 2006): 474-498; Tashakorri, Abbas and John W. Creswell. “The New Era of Mixed Methods.” Journal of Mixed Methods Research 1 (January 2007): 3-7; Zhanga, Wanqing. “Mixed Methods Application in Health Intervention Research: A Multiple Case Study.” International Journal of Multiple Research Approaches 8 (2014): 24-35 .
Observational Design
This type of research design draws a conclusion by comparing subjects against a control group, in cases where the researcher has no control over the experiment. There are two general types of observational designs. In direct observations, people know that you are watching them. Unobtrusive measures involve any method for studying behavior where individuals do not know they are being observed. An observational study allows a useful insight into a phenomenon and avoids the ethical and practical difficulties of setting up a large and cumbersome research project.
- Observational studies are usually flexible and do not necessarily need to be structured around a hypothesis about what you expect to observe [data is emergent rather than pre-existing].
- The researcher is able to collect in-depth information about a particular behavior.
- Can reveal interrelationships among multifaceted dimensions of group interactions.
- You can generalize your results to real life situations.
- Observational research is useful for discovering what variables may be important before applying other methods like experiments.
- Observation research designs account for the complexity of group behaviors.
- Reliability of data is low because seeing behaviors occur over and over again may be a time consuming task and are difficult to replicate.
- In observational research, findings may only reflect a unique sample population and, thus, cannot be generalized to other groups.
- There can be problems with bias as the researcher may only "see what they want to see."
- There is no possibility to determine "cause and effect" relationships since nothing is manipulated.
- Sources or subjects may not all be equally credible.
- Any group that is knowingly studied is altered to some degree by the presence of the researcher, therefore, potentially skewing any data collected.
Atkinson, Paul and Martyn Hammersley. “Ethnography and Participant Observation.” In Handbook of Qualitative Research . Norman K. Denzin and Yvonna S. Lincoln, eds. (Thousand Oaks, CA: Sage, 1994), pp. 248-261; Observational Research. Research Methods by Dummies. Department of Psychology. California State University, Fresno, 2006; Patton Michael Quinn. Qualitiative Research and Evaluation Methods . Chapter 6, Fieldwork Strategies and Observational Methods. 3rd ed. Thousand Oaks, CA: Sage, 2002; Payne, Geoff and Judy Payne. "Observation." In Key Concepts in Social Research . The SAGE Key Concepts series. (London, England: Sage, 2004), pp. 158-162; Rosenbaum, Paul R. Design of Observational Studies . New York: Springer, 2010;Williams, J. Patrick. "Nonparticipant Observation." In The Sage Encyclopedia of Qualitative Research Methods . Lisa M. Given, editor.(Thousand Oaks, CA: Sage, 2008), pp. 562-563.
Philosophical Design
Understood more as an broad approach to examining a research problem than a methodological design, philosophical analysis and argumentation is intended to challenge deeply embedded, often intractable, assumptions underpinning an area of study. This approach uses the tools of argumentation derived from philosophical traditions, concepts, models, and theories to critically explore and challenge, for example, the relevance of logic and evidence in academic debates, to analyze arguments about fundamental issues, or to discuss the root of existing discourse about a research problem. These overarching tools of analysis can be framed in three ways:
- Ontology -- the study that describes the nature of reality; for example, what is real and what is not, what is fundamental and what is derivative?
- Epistemology -- the study that explores the nature of knowledge; for example, by what means does knowledge and understanding depend upon and how can we be certain of what we know?
- Axiology -- the study of values; for example, what values does an individual or group hold and why? How are values related to interest, desire, will, experience, and means-to-end? And, what is the difference between a matter of fact and a matter of value?
- Can provide a basis for applying ethical decision-making to practice.
- Functions as a means of gaining greater self-understanding and self-knowledge about the purposes of research.
- Brings clarity to general guiding practices and principles of an individual or group.
- Philosophy informs methodology.
- Refine concepts and theories that are invoked in relatively unreflective modes of thought and discourse.
- Beyond methodology, philosophy also informs critical thinking about epistemology and the structure of reality (metaphysics).
- Offers clarity and definition to the practical and theoretical uses of terms, concepts, and ideas.
- Limited application to specific research problems [answering the "So What?" question in social science research].
- Analysis can be abstract, argumentative, and limited in its practical application to real-life issues.
- While a philosophical analysis may render problematic that which was once simple or taken-for-granted, the writing can be dense and subject to unnecessary jargon, overstatement, and/or excessive quotation and documentation.
- There are limitations in the use of metaphor as a vehicle of philosophical analysis.
- There can be analytical difficulties in moving from philosophy to advocacy and between abstract thought and application to the phenomenal world.
Burton, Dawn. "Part I, Philosophy of the Social Sciences." In Research Training for Social Scientists . (London, England: Sage, 2000), pp. 1-5; Chapter 4, Research Methodology and Design. Unisa Institutional Repository (UnisaIR), University of South Africa; Jarvie, Ian C., and Jesús Zamora-Bonilla, editors. The SAGE Handbook of the Philosophy of Social Sciences . London: Sage, 2011; Labaree, Robert V. and Ross Scimeca. “The Philosophical Problem of Truth in Librarianship.” The Library Quarterly 78 (January 2008): 43-70; Maykut, Pamela S. Beginning Qualitative Research: A Philosophic and Practical Guide . Washington, DC: Falmer Press, 1994; McLaughlin, Hugh. "The Philosophy of Social Research." In Understanding Social Work Research . 2nd edition. (London: SAGE Publications Ltd., 2012), pp. 24-47; Stanford Encyclopedia of Philosophy . Metaphysics Research Lab, CSLI, Stanford University, 2013.
Sequential Design
- The researcher has a limitless option when it comes to sample size and the sampling schedule.
- Due to the repetitive nature of this research design, minor changes and adjustments can be done during the initial parts of the study to correct and hone the research method.
- This is a useful design for exploratory studies.
- There is very little effort on the part of the researcher when performing this technique. It is generally not expensive, time consuming, or workforce intensive.
- Because the study is conducted serially, the results of one sample are known before the next sample is taken and analyzed. This provides opportunities for continuous improvement of sampling and methods of analysis.
- The sampling method is not representative of the entire population. The only possibility of approaching representativeness is when the researcher chooses to use a very large sample size significant enough to represent a significant portion of the entire population. In this case, moving on to study a second or more specific sample can be difficult.
- The design cannot be used to create conclusions and interpretations that pertain to an entire population because the sampling technique is not randomized. Generalizability from findings is, therefore, limited.
- Difficult to account for and interpret variation from one sample to another over time, particularly when using qualitative methods of data collection.
Betensky, Rebecca. Harvard University, Course Lecture Note slides; Bovaird, James A. and Kevin A. Kupzyk. "Sequential Design." In Encyclopedia of Research Design . Neil J. Salkind, editor. (Thousand Oaks, CA: Sage, 2010), pp. 1347-1352; Cresswell, John W. Et al. “Advanced Mixed-Methods Research Designs.” In Handbook of Mixed Methods in Social and Behavioral Research . Abbas Tashakkori and Charles Teddle, eds. (Thousand Oaks, CA: Sage, 2003), pp. 209-240; Henry, Gary T. "Sequential Sampling." In The SAGE Encyclopedia of Social Science Research Methods . Michael S. Lewis-Beck, Alan Bryman and Tim Futing Liao, editors. (Thousand Oaks, CA: Sage, 2004), pp. 1027-1028; Nataliya V. Ivankova. “Using Mixed-Methods Sequential Explanatory Design: From Theory to Practice.” Field Methods 18 (February 2006): 3-20; Bovaird, James A. and Kevin A. Kupzyk. “Sequential Design.” In Encyclopedia of Research Design . Neil J. Salkind, ed. Thousand Oaks, CA: Sage, 2010; Sequential Analysis. Wikipedia.
Systematic Review
- A systematic review synthesizes the findings of multiple studies related to each other by incorporating strategies of analysis and interpretation intended to reduce biases and random errors.
- The application of critical exploration, evaluation, and synthesis methods separates insignificant, unsound, or redundant research from the most salient and relevant studies worthy of reflection.
- They can be use to identify, justify, and refine hypotheses, recognize and avoid hidden problems in prior studies, and explain data inconsistencies and conflicts in data.
- Systematic reviews can be used to help policy makers formulate evidence-based guidelines and regulations.
- The use of strict, explicit, and pre-determined methods of synthesis, when applied appropriately, provide reliable estimates about the effects of interventions, evaluations, and effects related to the overarching research problem investigated by each study under review.
- Systematic reviews illuminate where knowledge or thorough understanding of a research problem is lacking and, therefore, can then be used to guide future research.
- The accepted inclusion of unpublished studies [i.e., grey literature] ensures the broadest possible way to analyze and interpret research on a topic.
- Results of the synthesis can be generalized and the findings extrapolated into the general population with more validity than most other types of studies .
- Systematic reviews do not create new knowledge per se; they are a method for synthesizing existing studies about a research problem in order to gain new insights and determine gaps in the literature.
- The way researchers have carried out their investigations [e.g., the period of time covered, number of participants, sources of data analyzed, etc.] can make it difficult to effectively synthesize studies.
- The inclusion of unpublished studies can introduce bias into the review because they may not have undergone a rigorous peer-review process prior to publication. Examples may include conference presentations or proceedings, publications from government agencies, white papers, working papers, and internal documents from organizations, and doctoral dissertations and Master's theses.
Denyer, David and David Tranfield. "Producing a Systematic Review." In The Sage Handbook of Organizational Research Methods . David A. Buchanan and Alan Bryman, editors. ( Thousand Oaks, CA: Sage Publications, 2009), pp. 671-689; Foster, Margaret J. and Sarah T. Jewell, editors. Assembling the Pieces of a Systematic Review: A Guide for Librarians . Lanham, MD: Rowman and Littlefield, 2017; Gough, David, Sandy Oliver, James Thomas, editors. Introduction to Systematic Reviews . 2nd edition. Los Angeles, CA: Sage Publications, 2017; Gopalakrishnan, S. and P. Ganeshkumar. “Systematic Reviews and Meta-analysis: Understanding the Best Evidence in Primary Healthcare.” Journal of Family Medicine and Primary Care 2 (2013): 9-14; Gough, David, James Thomas, and Sandy Oliver. "Clarifying Differences between Review Designs and Methods." Systematic Reviews 1 (2012): 1-9; Khan, Khalid S., Regina Kunz, Jos Kleijnen, and Gerd Antes. “Five Steps to Conducting a Systematic Review.” Journal of the Royal Society of Medicine 96 (2003): 118-121; Mulrow, C. D. “Systematic Reviews: Rationale for Systematic Reviews.” BMJ 309:597 (September 1994); O'Dwyer, Linda C., and Q. Eileen Wafford. "Addressing Challenges with Systematic Review Teams through Effective Communication: A Case Report." Journal of the Medical Library Association 109 (October 2021): 643-647; Okoli, Chitu, and Kira Schabram. "A Guide to Conducting a Systematic Literature Review of Information Systems Research." Sprouts: Working Papers on Information Systems 10 (2010); Siddaway, Andy P., Alex M. Wood, and Larry V. Hedges. "How to Do a Systematic Review: A Best Practice Guide for Conducting and Reporting Narrative Reviews, Meta-analyses, and Meta-syntheses." Annual Review of Psychology 70 (2019): 747-770; Torgerson, Carole J. “Publication Bias: The Achilles’ Heel of Systematic Reviews?” British Journal of Educational Studies 54 (March 2006): 89-102; Torgerson, Carole. Systematic Reviews . New York: Continuum, 2003.
- << Previous: Purpose of Guide
- Next: Design Flaws to Avoid >>
- Last Updated: Oct 24, 2024 10:02 AM
- URL: https://libguides.usc.edu/writingguide

What Is Research Design?
A Plain-Language Explainer (With Examples)
By: Derek Jansen (MBA) | Reviewers: Eunice Rautenbach (DTech) & Kerryn Warren (PhD) | April 2023
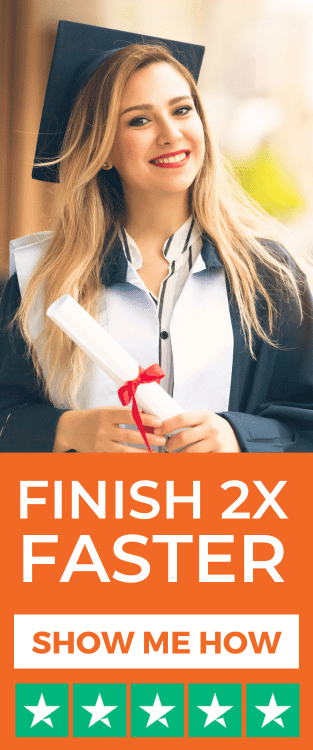
Overview: Research Design 101
What is research design.
- Research design types for quantitative studies
- Video explainer : quantitative research design
- Research design types for qualitative studies
- Video explainer : qualitative research design
- How to choose a research design
- Key takeaways
Research design refers to the overall plan, structure or strategy that guides a research project , from its conception to the final data analysis. A good research design serves as the blueprint for how you, as the researcher, will collect and analyse data while ensuring consistency, reliability and validity throughout your study.
Understanding different types of research designs is essential as helps ensure that your approach is suitable given your research aims, objectives and questions , as well as the resources you have available to you. Without a clear big-picture view of how you’ll design your research, you run the risk of potentially making misaligned choices in terms of your methodology – especially your sampling , data collection and data analysis decisions.
The problem with defining research design…
One of the reasons students struggle with a clear definition of research design is because the term is used very loosely across the internet, and even within academia.
Some sources claim that the three research design types are qualitative, quantitative and mixed methods , which isn’t quite accurate (these just refer to the type of data that you’ll collect and analyse). Other sources state that research design refers to the sum of all your design choices, suggesting it’s more like a research methodology . Others run off on other less common tangents. No wonder there’s confusion!
In this article, we’ll clear up the confusion. We’ll explain the most common research design types for both qualitative and quantitative research projects, whether that is for a full dissertation or thesis, or a smaller research paper or article.
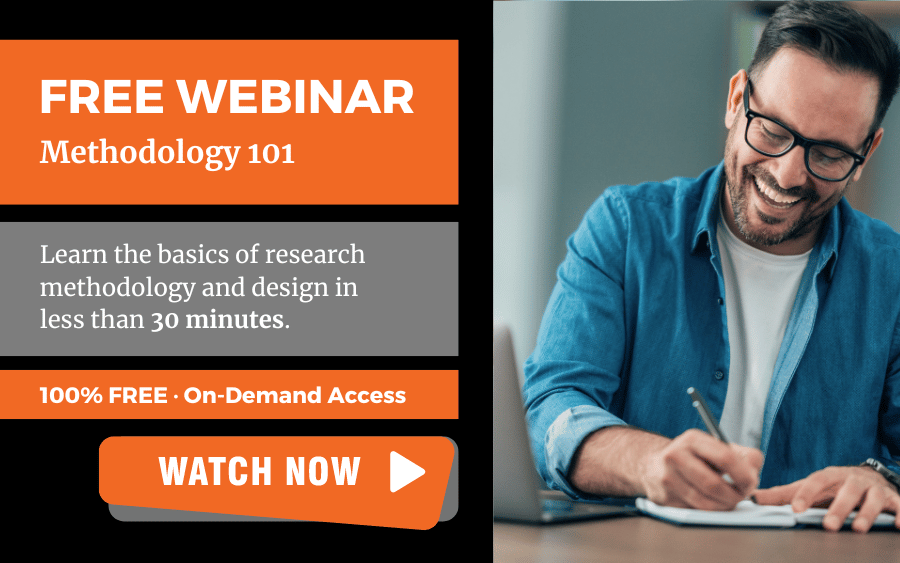
Research Design: Quantitative Studies
Quantitative research involves collecting and analysing data in a numerical form. Broadly speaking, there are four types of quantitative research designs: descriptive , correlational , experimental , and quasi-experimental .
As the name suggests, descriptive research design focuses on describing existing conditions, behaviours, or characteristics by systematically gathering information without manipulating any variables. In other words, there is no intervention on the researcher’s part – only data collection.
For example, if you’re studying smartphone addiction among adolescents in your community, you could deploy a survey to a sample of teens asking them to rate their agreement with certain statements that relate to smartphone addiction. The collected data would then provide insight regarding how widespread the issue may be – in other words, it would describe the situation.
The key defining attribute of this type of research design is that it purely describes the situation . In other words, descriptive research design does not explore potential relationships between different variables or the causes that may underlie those relationships. Therefore, descriptive research is useful for generating insight into a research problem by describing its characteristics . By doing so, it can provide valuable insights and is often used as a precursor to other research design types.
Correlational Research Design
Correlational design is a popular choice for researchers aiming to identify and measure the relationship between two or more variables without manipulating them . In other words, this type of research design is useful when you want to know whether a change in one thing tends to be accompanied by a change in another thing.
For example, if you wanted to explore the relationship between exercise frequency and overall health, you could use a correlational design to help you achieve this. In this case, you might gather data on participants’ exercise habits, as well as records of their health indicators like blood pressure, heart rate, or body mass index. Thereafter, you’d use a statistical test to assess whether there’s a relationship between the two variables (exercise frequency and health).
As you can see, correlational research design is useful when you want to explore potential relationships between variables that cannot be manipulated or controlled for ethical, practical, or logistical reasons. It is particularly helpful in terms of developing predictions , and given that it doesn’t involve the manipulation of variables, it can be implemented at a large scale more easily than experimental designs (which will look at next).
Need a helping hand?
Experimental research design is used to determine if there is a causal relationship between two or more variables . With this type of research design, you, as the researcher, manipulate one variable (the independent variable) while controlling others (dependent variables). Doing so allows you to observe the effect of the former on the latter and draw conclusions about potential causality.
For example, if you wanted to measure if/how different types of fertiliser affect plant growth, you could set up several groups of plants, with each group receiving a different type of fertiliser, as well as one with no fertiliser at all. You could then measure how much each plant group grew (on average) over time and compare the results from the different groups to see which fertiliser was most effective.
Overall, experimental research design provides researchers with a powerful way to identify and measure causal relationships (and the direction of causality) between variables. However, developing a rigorous experimental design can be challenging as it’s not always easy to control all the variables in a study. This often results in smaller sample sizes , which can reduce the statistical power and generalisability of the results.
Moreover, experimental research design requires random assignment . This means that the researcher needs to assign participants to different groups or conditions in a way that each participant has an equal chance of being assigned to any group (note that this is not the same as random sampling ). Doing so helps reduce the potential for bias and confounding variables . This need for random assignment can lead to ethics-related issues . For example, withholding a potentially beneficial medical treatment from a control group may be considered unethical in certain situations.
Quasi-Experimental Research Design
Quasi-experimental research design is used when the research aims involve identifying causal relations , but one cannot (or doesn’t want to) randomly assign participants to different groups (for practical or ethical reasons). Instead, with a quasi-experimental research design, the researcher relies on existing groups or pre-existing conditions to form groups for comparison.
For example, if you were studying the effects of a new teaching method on student achievement in a particular school district, you may be unable to randomly assign students to either group and instead have to choose classes or schools that already use different teaching methods. This way, you still achieve separate groups, without having to assign participants to specific groups yourself.
Naturally, quasi-experimental research designs have limitations when compared to experimental designs. Given that participant assignment is not random, it’s more difficult to confidently establish causality between variables, and, as a researcher, you have less control over other variables that may impact findings.
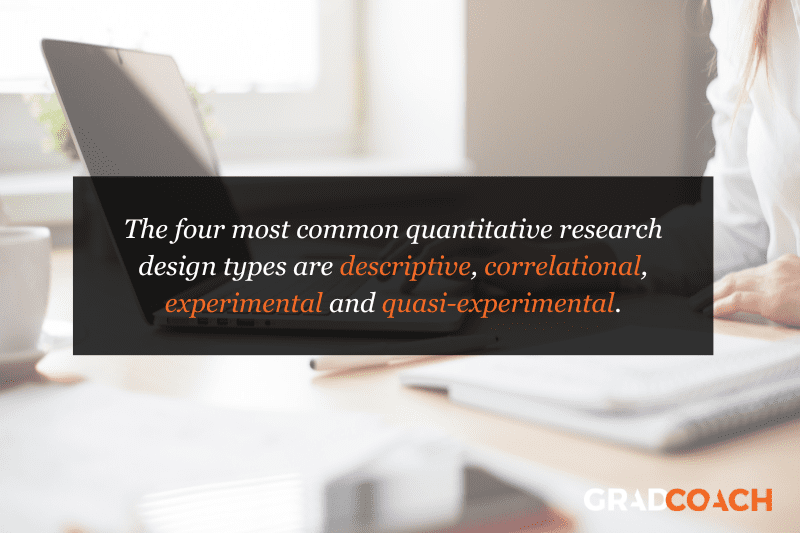
Research Design: Qualitative Studies
There are many different research design types when it comes to qualitative studies, but here we’ll narrow our focus to explore the “Big 4”. Specifically, we’ll look at phenomenological design, grounded theory design, ethnographic design, and case study design.
Phenomenological design involves exploring the meaning of lived experiences and how they are perceived by individuals. This type of research design seeks to understand people’s perspectives , emotions, and behaviours in specific situations. Here, the aim for researchers is to uncover the essence of human experience without making any assumptions or imposing preconceived ideas on their subjects.
For example, you could adopt a phenomenological design to study why cancer survivors have such varied perceptions of their lives after overcoming their disease. This could be achieved by interviewing survivors and then analysing the data using a qualitative analysis method such as thematic analysis to identify commonalities and differences.
Phenomenological research design typically involves in-depth interviews or open-ended questionnaires to collect rich, detailed data about participants’ subjective experiences. This richness is one of the key strengths of phenomenological research design but, naturally, it also has limitations. These include potential biases in data collection and interpretation and the lack of generalisability of findings to broader populations.
Grounded Theory Research Design
Grounded theory (also referred to as “GT”) aims to develop theories by continuously and iteratively analysing and comparing data collected from a relatively large number of participants in a study. It takes an inductive (bottom-up) approach, with a focus on letting the data “speak for itself”, without being influenced by preexisting theories or the researcher’s preconceptions.
As an example, let’s assume your research aims involved understanding how people cope with chronic pain from a specific medical condition, with a view to developing a theory around this. In this case, grounded theory design would allow you to explore this concept thoroughly without preconceptions about what coping mechanisms might exist. You may find that some patients prefer cognitive-behavioural therapy (CBT) while others prefer to rely on herbal remedies. Based on multiple, iterative rounds of analysis, you could then develop a theory in this regard, derived directly from the data (as opposed to other preexisting theories and models).
Grounded theory typically involves collecting data through interviews or observations and then analysing it to identify patterns and themes that emerge from the data. These emerging ideas are then validated by collecting more data until a saturation point is reached (i.e., no new information can be squeezed from the data). From that base, a theory can then be developed .
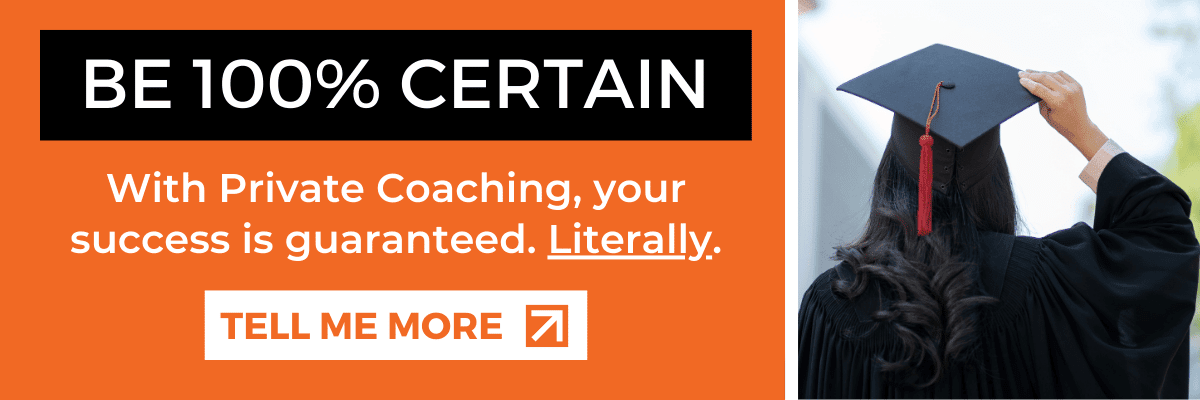
Ethnographic design involves observing and studying a culture-sharing group of people in their natural setting to gain insight into their behaviours, beliefs, and values. The focus here is on observing participants in their natural environment (as opposed to a controlled environment). This typically involves the researcher spending an extended period of time with the participants in their environment, carefully observing and taking field notes .
All of this is not to say that ethnographic research design relies purely on observation. On the contrary, this design typically also involves in-depth interviews to explore participants’ views, beliefs, etc. However, unobtrusive observation is a core component of the ethnographic approach.
As an example, an ethnographer may study how different communities celebrate traditional festivals or how individuals from different generations interact with technology differently. This may involve a lengthy period of observation, combined with in-depth interviews to further explore specific areas of interest that emerge as a result of the observations that the researcher has made.
As you can probably imagine, ethnographic research design has the ability to provide rich, contextually embedded insights into the socio-cultural dynamics of human behaviour within a natural, uncontrived setting. Naturally, however, it does come with its own set of challenges, including researcher bias (since the researcher can become quite immersed in the group), participant confidentiality and, predictably, ethical complexities . All of these need to be carefully managed if you choose to adopt this type of research design.
Case Study Design
With case study research design, you, as the researcher, investigate a single individual (or a single group of individuals) to gain an in-depth understanding of their experiences, behaviours or outcomes. Unlike other research designs that are aimed at larger sample sizes, case studies offer a deep dive into the specific circumstances surrounding a person, group of people, event or phenomenon, generally within a bounded setting or context .
As an example, a case study design could be used to explore the factors influencing the success of a specific small business. This would involve diving deeply into the organisation to explore and understand what makes it tick – from marketing to HR to finance. In terms of data collection, this could include interviews with staff and management, review of policy documents and financial statements, surveying customers, etc.
While the above example is focused squarely on one organisation, it’s worth noting that case study research designs can have different variation s, including single-case, multiple-case and longitudinal designs. As you can see in the example, a single-case design involves intensely examining a single entity to understand its unique characteristics and complexities. Conversely, in a multiple-case design , multiple cases are compared and contrasted to identify patterns and commonalities. Lastly, in a longitudinal case design , a single case or multiple cases are studied over an extended period of time to understand how factors develop over time.
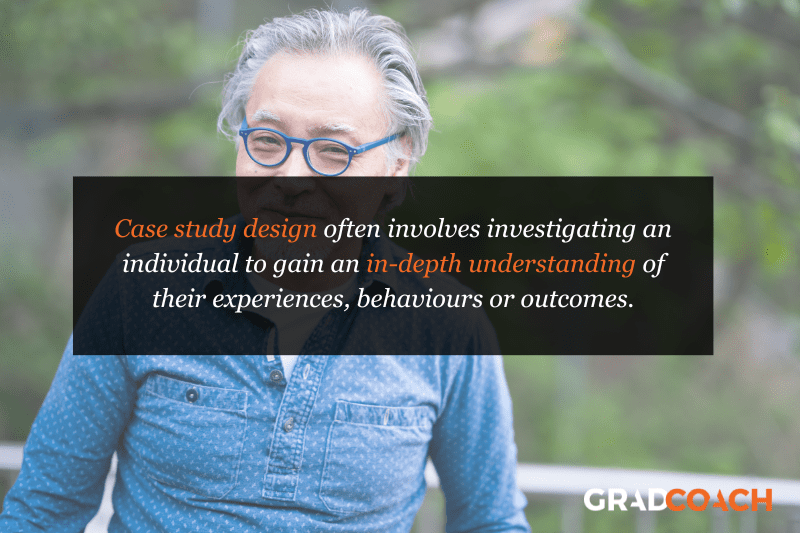
How To Choose A Research Design
Having worked through all of these potential research designs, you’d be forgiven for feeling a little overwhelmed and wondering, “ But how do I decide which research design to use? ”. While we could write an entire post covering that alone, here are a few factors to consider that will help you choose a suitable research design for your study.
Data type: The first determining factor is naturally the type of data you plan to be collecting – i.e., qualitative or quantitative. This may sound obvious, but we have to be clear about this – don’t try to use a quantitative research design on qualitative data (or vice versa)!
Research aim(s) and question(s): As with all methodological decisions, your research aim and research questions will heavily influence your research design. For example, if your research aims involve developing a theory from qualitative data, grounded theory would be a strong option. Similarly, if your research aims involve identifying and measuring relationships between variables, one of the experimental designs would likely be a better option.
Time: It’s essential that you consider any time constraints you have, as this will impact the type of research design you can choose. For example, if you’ve only got a month to complete your project, a lengthy design such as ethnography wouldn’t be a good fit.
Resources: Take into account the resources realistically available to you, as these need to factor into your research design choice. For example, if you require highly specialised lab equipment to execute an experimental design, you need to be sure that you’ll have access to that before you make a decision.
Keep in mind that when it comes to research, it’s important to manage your risks and play as conservatively as possible. If your entire project relies on you achieving a huge sample, having access to niche equipment or holding interviews with very difficult-to-reach participants, you’re creating risks that could kill your project. So, be sure to think through your choices carefully and make sure that you have backup plans for any existential risks. Remember that a relatively simple methodology executed well generally will typically earn better marks than a highly-complex methodology executed poorly.
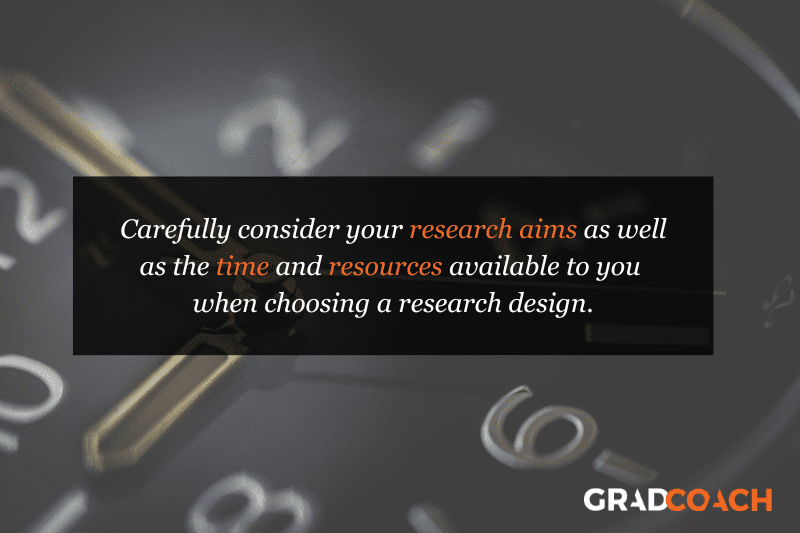
Recap: Key Takeaways
We’ve covered a lot of ground here. Let’s recap by looking at the key takeaways:
- Research design refers to the overall plan, structure or strategy that guides a research project, from its conception to the final analysis of data.
- Research designs for quantitative studies include descriptive , correlational , experimental and quasi-experimenta l designs.
- Research designs for qualitative studies include phenomenological , grounded theory , ethnographic and case study designs.
- When choosing a research design, you need to consider a variety of factors, including the type of data you’ll be working with, your research aims and questions, your time and the resources available to you.
If you need a helping hand with your research design (or any other aspect of your research), check out our private coaching services .
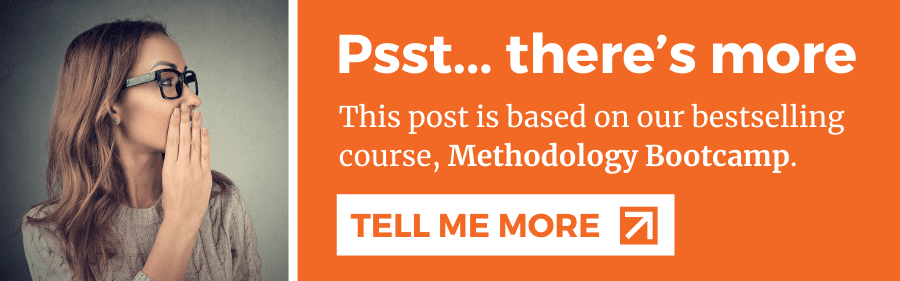
Learn More About Methodology
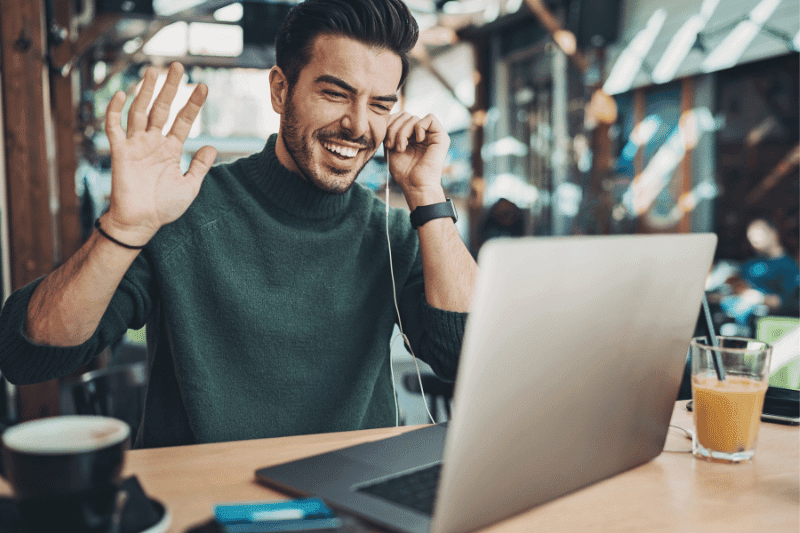
How To Choose A Tutor For Your Dissertation
Hiring the right tutor for your dissertation or thesis can make the difference between passing and failing. Here’s what you need to consider.
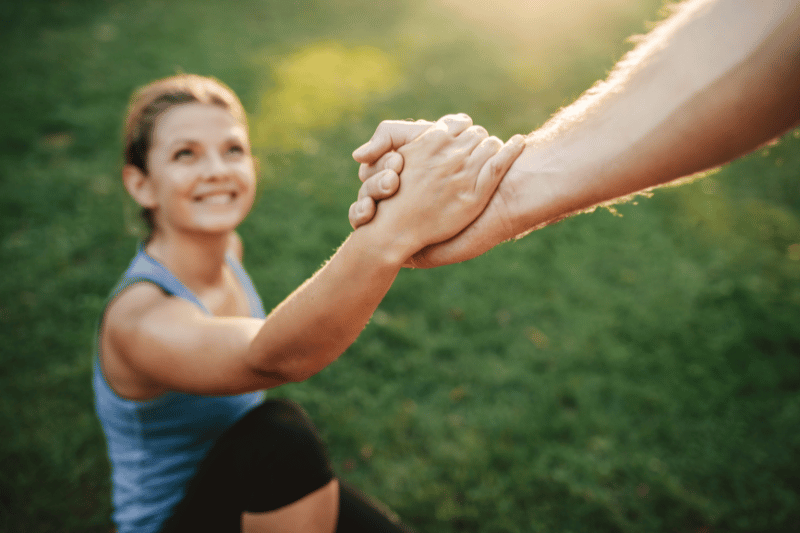
5 Signs You Need A Dissertation Helper
Discover the 5 signs that suggest you need a dissertation helper to get unstuck, finish your degree and get your life back.
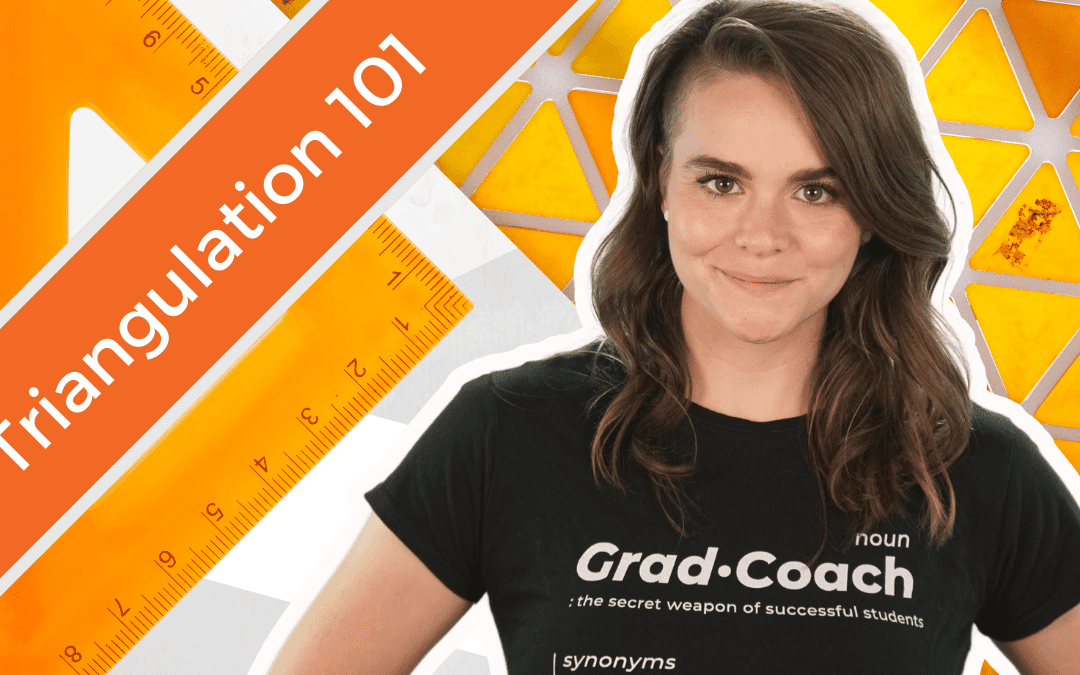
Triangulation: The Ultimate Credibility Enhancer
Triangulation is one of the best ways to enhance the credibility of your research. Learn about the different options here.
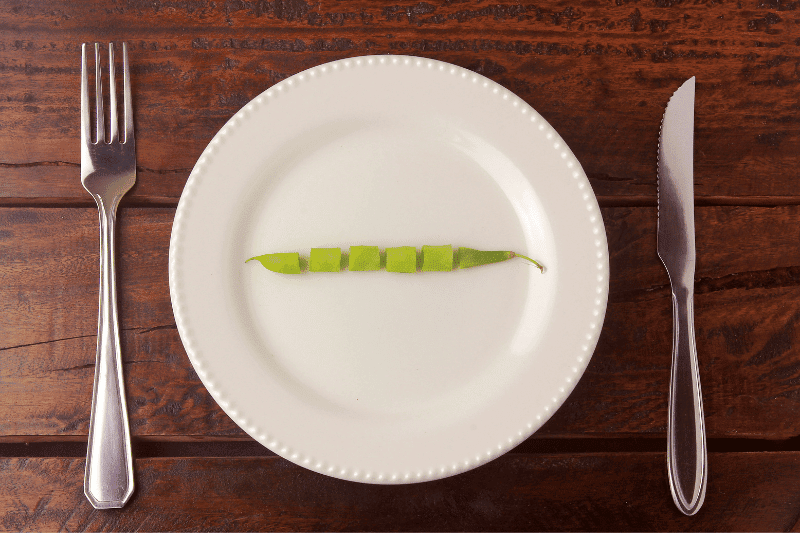
Research Limitations 101: What You Need To Know
Learn everything you need to know about research limitations (AKA limitations of the study). Includes practical examples from real studies.
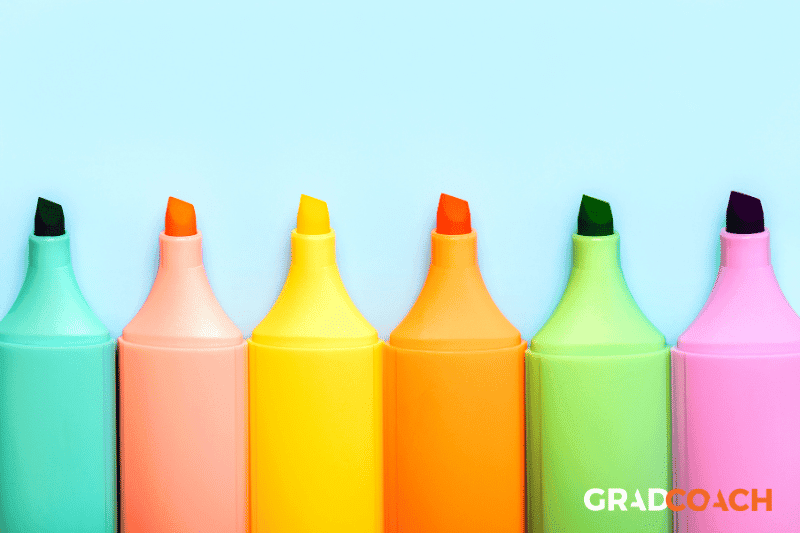
In Vivo Coding 101: Full Explainer With Examples
Learn about in vivo coding, a popular qualitative coding technique ideal for studies where the nuances of language are central to the aims.
📄 FREE TEMPLATES
Research Topic Ideation
Proposal Writing
Literature Review
Methodology & Analysis
Academic Writing
Referencing & Citing
Apps, Tools & Tricks
The Grad Coach Podcast
18 Comments
Is there any blog article explaining more on Case study research design? Is there a Case study write-up template? Thank you.
Thanks this was quite valuable to clarify such an important concept.
Thanks for this simplified explanations. it is quite very helpful.
This was really helpful. thanks
Thank you for your explanation. I think case study research design and the use of secondary data in researches needs to be talked about more in your videos and articles because there a lot of case studies research design tailored projects out there.
Please is there any template for a case study research design whose data type is a secondary data on your repository?
This post is very clear, comprehensive and has been very helpful to me. It has cleared the confusion I had in regard to research design and methodology.
This post is helpful, easy to understand, and deconstructs what a research design is. Thanks
This post is really helpful.
how to cite this page
Thank you very much for the post. It is wonderful and has cleared many worries in my mind regarding research designs. I really appreciate .
how can I put this blog as my reference(APA style) in bibliography part?
This post has been very useful to me. Confusing areas have been cleared
This is very helpful and very useful!
Wow! This post has an awful explanation. Appreciated.
Thanks This has been helpful
Micah on 29, September, 2024 this is really helpful
This article is on point. Very well articulated and simply to understand. thanks for pointing out the term has been used very loosely across the internet, and even within academia. This is why so many students find it difficult to explain their study design
Thank you for these useful materials on how to designs the research
Submit a Comment Cancel reply
Your email address will not be published. Required fields are marked *
Save my name, email, and website in this browser for the next time I comment.
Submit Comment
- Print Friendly
An official website of the United States government
Official websites use .gov A .gov website belongs to an official government organization in the United States.
Secure .gov websites use HTTPS A lock ( Lock Locked padlock icon ) or https:// means you've safely connected to the .gov website. Share sensitive information only on official, secure websites.
- Publications
- Account settings
- Advanced Search
- Journal List

Declaring and Diagnosing Research Designs
Graeme blair, jasper cooper, alexander coppock, macartan humphreys.
- Author information
- Article notes
- Copyright and License information
Jasper Cooper, Assistant Professor of Political Science, University of California, San Diego, http://jasper-cooper.com .
Alexander Coppock, Assistant Professor of Political Science, Yale University, https://alexandercoppock.com .
Macartan Humphreys, WZB Berlin, Professor of Political Science, Columbia University, http://www.macartan.nyc .
Graeme Blair, Assistant Professor of Political Science, University of California, Los Angeles, [email protected] , https://graemeblair.com.
Issue date 2019 Aug.
This is an Open Access article, distributed under the terms of the Creative Commons Attribution licence ( http://creativecommons.org/licenses/by/4.0/ ), which permits unrestricted re-use, distribution, and reproduction in any medium, provided the original work is properly cited.
Researchers need to select high-quality research designs and communicate those designs clearly to readers. Both tasks are difficult. We provide a framework for formally “declaring” the analytically relevant features of a research design in a demonstrably complete manner, with applications to qualitative, quantitative, and mixed methods research. The approach to design declaration we describe requires defining a model of the world ( M ), an inquiry ( I ), adatastrategy( D ), andananswerstrategy( A ). Declaration of these features in code provides sufficient information for researchers and readers to use Monte Carlo techniques to diagnose properties such as power, bias, accuracy of qualitative causal inferences, and other “diagnosands.” Ex ante declarations can be used to improve designs and facilitate preregistration, analysis, and reconciliation of intended and actual analyses. Ex post declarations are useful for describing, sharing, reanalyzing, and critiquing existing designs. We provide open-source software, DeclareDesign, to implement the proposed approach.
As empirical social scientists, we routinely face two research design problems. First, we need to select high-quality designs, given resource constraints. Second, we need to communicate those designs to readers and reviewers.
To select strong designs, we often rely on rules of thumb, simple power calculators, or principles from the methodological literature that typically address one component of a design while assuming optimal conditions for others. These relatively informal practices can result in the selection of suboptimal designs, or worse, designs that are simply too weak to deliver useful answers.
To convince others of the quality of our designs, we often defend them with references to previous studies that used similar approaches, with power analyses that may rely on assumptions unknown even to ourselves, or with ad hoc simulation code. In cases of dispute over the merits of different approaches, disagreements sometimes fall back on first principles or epistemological debates rather than on demonstrations of the conditions under which one approach does better than another.
In this paper we describe an approach to address these problems. We introduce a framework—MIDA—that asks researchers to specify information about their background model ( M ), their inquiry ( I ), their data strategy ( D ), and their answer strategy ( A ). We then introduce the notion of “diagnosands,” or quantitative summaries of design properties. Familiar diagnosands include statistical power, the bias of an estimator with respect to an estimand, or the coverage probability of a procedure for generating confidence intervals. We say a design declaration is “diagnosand-complete” when a diagnosand can be estimated from the declaration. We do not have a general notion of a complete design, but rather adopt an approach in which the purposes of the design determine which diagnosands are valuable and in turn what features must be declared. In practice, domain-specific standards might be agreed upon among members of particular research communities. For instance, researchers concerned about the policy impact of a given treatment might require a design that is diagnosand-complete for an out-of-sample diag- nosand, such as bias relative to the population average treatment effect. They may also consider a diagnosand directly related to policy choices, such as the probability of making the right policy decision after research is conducted.
We acknowledge that although many aspects of design quality can be assessed through design diagnosis, many cannot. For instance the contribution to an academic literature, relevance to a policy decision, and impact on public debate are unlikely to be quantifiable ex ante.
Using this framework, researchers can declare a research design as a computer code object and then diagnose its statistical properties on the basis of this declaration. We emphasize that the term “declare” does not imply a public declaration or even necessarily a declaration before research takes place. A researcher may declare the features of designs in our framework for their own understanding and declaring designs may be useful before or after the research is implemented. Researchers can declare and diagnose their designs with the companion software for this paper, DeclareDesign, but the principles of design declaration and diagnosis do not depend on any particular software implementation.
The formal characterization and diagnosis of designs before implementation can serve many purposes. First, researchers can learn about and improve their inferential strategies. Done at this stage, diagnosis of a design and alternatives can help a researcher select from a range of designs, conditional upon beliefs about the world. Later, a researcher may include design declaration and diagnosis as part of a preanalysis plan or in a funding request. At this stage, the full specification of a design serves a communication function and enables third parties to understand a design and an author’s intentions. Even if declared ex-post, formal declaration still has benefits. The complete characterization can help readers understand the properties of a research project, facilitate transparent replication, and can help guide future (re-)analysis of the study data.
The approach we describe is clearly more easily applied to some types of research than others. In prospective confirmatory work, for example, researchers may have access to all design-relevant information prior to launching their study. For more inductive research, by contrast, researchers may simply not have enough information about possible quantities of interest to declare a design in advance. Although in some cases the design may still be usefully declared ex post, in others it may not be possible to fully reconstruct the inferential procedure after the fact. For instance, although researchers might be able to provide compelling grounds for their inferences, they may not be able to describe what inferences they would have drawn had different data been realized. This may be particularly true of interpretivist approaches and approaches to process tracing that work backwards from outcomes to a set of possible causes that cannot be prespecified. We acknowledge from the outset that variation in research strategy limits the utility of our procedure for different types of research. Even still, we show that our framework can accommodate discovery, qualitative inference, and different approaches to mixed methods research, as well as designs that focus on “effects-of-causes” questions, often associated with quantitative approaches, and “causes-of-effects” questions, often associated with qualitative approaches.
Formally declaring research designs as objects in the manner we describe here brings, we hope, four benefits. It can facilitate the diagnosis of designs in terms of their ability to answer the questions we want answered under specified conditions; it can assist in the improvement of research designs through comparison with alternatives; it can enhance research transparency by making design choices explicit; and it can provide strategies to assist principled replication and reanalysis of published research.
RESEARCH DESIGNS AND DIAGNOSANDS
We present a general description of a research design as the specification of a problem and a strategy to answer it. We build on two influential research design frameworks. King, Keohane, and Verba (1994 , 13) enumerate four components of a research design: a theory, a research question, data, and an approach to using the data. Geddes (2003) articulates the links between theory formation, research question formulation, case selection and coding strategies, and strategies for case comparison and inference. In both cases, the set of components are closely aligned to those in the framework we propose. In our exposition, we also employ elements from Pearl’s (2009) approach to structural modeling, which provides a syntax for mapping design inputs to design outputs as well as the potential outcomes framework as presented, for example, in Imbens and Rubin (2015) , which many social scientists use to clarify their inferential targets. We characterize the design problem at a high level of generality with the central focus being on the relationship between questions and answer strategies. We further situate the framework within existing literature below.
Elements of a Research Design
The specification of a problem requires a description of the world and the question to be asked about the world as described. Providing an answer requires a description of what information is used and how conclusions are reached given this information.
At its most basic we think of a research design as including four elements 〈 M, I, D, A 〉:
A causal model , M, of how the world works. 1 In general, following Pearl’s definition of a probabilistic causal model ( Pearl 2009 ) we assume that a model contains three core elements. First, a specification of the variables X about which research is being conducted. This includes endogenous and exogenous variables ( V and U respectively) and the ranges of these variables. In the formal literature this is sometimes called the signature of a model (e.g., Halpern 2000 ). Second, a specification of how each endogenous variable depends on other variables (the “functional relations” or, as in Imbens and Rubin (2015) , “potential outcomes”), F. Third, a probability distribution over exogenous variables, P(U).
An inquiry , I , about the distribution of variables, X , perhaps given interventions on some variables. Using Pearl’s notation we can distinguish between questions that ask about the conditional values of variables, such as Pr( X 1 | X 2 = 1) and questions that ask about values that would arise under interventions: Pr( X 1 | do ( X 2 = 1)) 2 . We let a M denote the answer to I under the model. Conditional on the model, a M is the value of the estimand, the quantity that the researcher wants to learn about.
A data strategy, D , generates data d on X under model M with probability P M ( d | D ). The data strategy includes sampling strategies and assignment strategies, which we denote with P S and P Z respectively. Measurement techniques are also a part of data strategies and can be thought of as procedures by which unobserved latent variables are mapped (possibly with error) into observed variables.
An answer strategy, A , that generates answer a A using data d.
A key feature of this bare specification is that if M, D, and A are sufficiently well described, the answer to question I has a distribution P M (a A |D). Moreover, one can construct a distribution of comparisons of this answer to the correct answer, under M , for example by assessing P M ( a A − a M |D ). One can also compare this to results under different data or analysis strategies, P M ( a A − a M | D ′ ) and P M ( a A ′ − a M | D ) , and to answers generated under alternative models, P M ( a A − a M ′ | D ) , as long as these possess signatures that are consistent with inquiries and answer strategies.
MIDA captures the analysis-relevant features of a design, but it does not describe substantive elements, such as how theories are derived, how interventions are implemented, or even, qualitatively, how outcomes are measured. Yet many other aspects of a design that are not explicitly labeled in these features enter into this framework if they are analytically relevant. For example, if treatment effects decay, logistical details of data collection (such as the duration of time between a treatment being administered and endline data collection) may enter into the model. Similarly, if a researcher anticipates noncompliance, substantive knowledge of how treatments are taken up can be included in many parts of the design.
Diagnosands
The ability to calculate distributions of answers, given a model, opens multiple avenues for assessment and critique. How good is the answer you expect to get from a given strategy? Would you do better, given some desideratum, with a different data strategy? With a different analysis strategy? How good is the strategy if the model is wrong in one way or another?
To allow for this kind of diagnosis of a design, we introduce two further concepts, both functions of research designs. These are quantities that a researcher or a third party could calculate with respect to a design.
A diagnostic statistic is a summary statistic generated from a “run” of a design—that is, the results given a possible realization of variables, given the model and data strategy. For example the statistic: e = “difference between the estimated and the actual average treatment effect” is a diagnostic statistic that requires specifying an estimand. The statistic s = 1 ( p ≤ 0.05 ) , interpreted as “the result is considered statistically significant at the 5% level,” is a diagnostic statistic that does not require specifying an estimand, but it does presuppose an answer strategy that reports a p -value.
Diagnostic statistics are governed by probability distributions that arise because both the model and the data generation, given the model, may be stochastic.
A diagnosand is a summary of the distribution of a diagnostic statistic. For example, (expected) bias in the estimated treatment effect is E ( e ) and statistical power is E ( s ) .
To illustrate, consider the following design. A model M specifies three variables X , Y , and Z defined on the real number line that form the signature. In additional we assume functional relationships between them that allow for the possibility of confounding (for example, Y = bX + Z + ε Y ; X = Z + ε X , with Z, ε X , ε Z distributed standard normal). The inquiry I is “what would be the average effect of a unit increase in X on Y in the population?” The specification of this question depends on the signature of the model, but not the functional relations of the model. The answer provided by the model does of course depend on the functional relations. Consider now a data strategy, D , in which data are gathered on X and Y for n randomly selected units. An answer a A , is then generated using ordinary least squares as the answer strategy, A .
We have specified all the components of MIDA. We now ask: How strong is this research design? One way to answer this question is with respect to the diagnosand “bias.” Here the model provides an answer, a M , to the inquiry, so the distribution of bias given the model, a A – a M , can be calculated.
In this example, the expected performance of the design may be poor, as measured by the bias diag- nosand, because the data and analysis strategy do not handle the confounding described by the model (see Supplementary Materials Section 1 for a formal declaration and diagnosis of this design). In comparison, better performance may be achieved through an alternative data strategy (e.g., where D′ randomly assigned X before recording X and Y ) or an alternative analysis strategy (e.g., A′ conditions on Z). These design evaluations depend on the model, and so one might reasonably ask how performance would look were the model different (for example, if the underlying process involved nonlinearities).
In all cases, the evaluation of a design depends on the assessment of a diagnosand, and comparing the diagnoses to what could be achieved under alternative designs.
Choice of Diagnosands
What diagnosands should researchers choose? Although researchers commonly focus on statistical power, a larger range of diagnosands can be examined and may provide more informative diagnoses of design quality. We list and describe some of these in Table 1 , indicating for each the design information that is required in order to calculate them.
Examples of Diagnosands and the Elements of the Model (M), Inquiry (I), Data Strategy (D), and Answer Strategy (A) Required in Order for a Design to be Diagnosand-Complete for Each Diagnosand
The set listed here includes many canonical diagnosands used in classical quantitative analyses. Diagnosands can also be defined for design properties that are often discussed informally but rarely subjected to formal investigation. For example one might define an inference as “robust” if the same inference is made under different analysis strategies. One might conclude that an intervention gives “value for money” if estimates are of a certain size and be interested in the probability that a researcher in correct in concluding that an intervention provides value for money.
We believe there is not yet a consensus around diagnosands for qualitative designs. However, in certain treatments clear analogues of diagnosands exist, such as sampling bias or estimation bias (e.g., Herron and Quinn 2016 ). There are indeed notions of power, coverage, and consistency for QCA researchers (e.g., Baumgartner and Thiem 2017 ; Rohlfing 2018 ) and concerns around correct identification of causes of effects, or of causal pathways, for scholars using process-tracing (e.g., Bennett 2015 ; Fairfield and Charman 2017 ; Humphreys and Jacobs 2015 ; Mahoney 2012 ).
Though many of these diagnosands are familiar to scholars using frequentist approaches, analogous diagnosands can be used to assess Bayesian estimation strategies (see Rubin 1984 ), and as we illustrate below, some diagnosands are unique to Bayesian answer strategies.
Given that there are many possible diagnosands, the overall evaluation of a design is both multi-dimensional and qualitative. For some diagnosands, quality thresholds have been established through common practice, such as the standard power target of 0.80. Some researchers are unsatisfied unless the “bias” diagnosand is exactly zero. Yet for most diagnosands, we only have a sense of better and worse, and improving one can mean hurting another, as in the classic bias-variance tradeoff. Our goal is not to dichotomize designs into high and low quality, but instead to facilitate the assessment of design quality on dimensions important to researchers.
What is a Complete Research Design Declaration?
A declaration of a research design that is in some sense complete is required in order to implement it, communicate its essential features, and to assess its properties. Yet existing definitions make clear that there is no single conception of a complete research design: at the time of writing, the Consolidated Standards of Reporting Trials (CONSORT) Statement widely used in medicine includes 22 features, while other proposals range from nine to 60 components 3 .
We propose a conditional conception of completeness: we say a design is “diagnosand-complete” for a given diagnosand if that diagnosand can be calculated from the declared design. Thus a design that is diagnosand-complete for one diagnosand may not be for another. Consider, for example, the diagnosand statistical power. Power is the probability of obtaining a statistically significant result. Equivalently, it is the probability that the p -value is lower than a critical value (e.g., 0.005). Thus, power-completeness requires that the answer strategy return a p -value and a significance threshold be specified. It does not, however, require a well-defined estimand, such as a true effect size (see Table 1 where, for a power diagnosand, there is no check under I). In contrast, bias- or RMSE-completeness does not require a hypothesis test, but does require the specification of an estimand.
Diagnosand-completeness is a desirable property to the extent that it means a diagnosand can be calculated. How useful diagnosand-completeness is depends on whether the diagnosand is worth knowing. Thus, evaluating completeness should focus first on whether diagnosands for which completeness holds are indeed useful ones.
The utility of a diagnosis depends in part on whether the information underlying declaration is believable. For instance, a design may be bias-complete, but only under the assumptions of a given spillover structure. Readers might disagree with these assumptions. Even in this case, however, an advantage of declaration is a clarification of the conditions for completeness.
EXISTING APPROACHES TO LEARNING ABOUT RESEARCH DESIGNS
Much quantitative research design advice focuses on one aspect of design at a time, rather than on the ways in which multiple components of a research design relate to each other. Statistics articles and textbooks tend to focus on a specific class of estimators ( Angrist and Pischke 2008 ; Imbens and Rubin 2015 ; Rosenbaum 2002 ), set of estimands ( Heckman, Urzua, and Vytlacil 2006 ; Imai, King, and Stuart 2008 ; Deaton 2010 ; Imbens 2010 ), data collection strategies ( Lohr 2010 ), or ways of thinking about data-generation models ( Gelman and Hill 2006 ; Pearl 2009 ). In Shadish, Cook, and Campbell (2002 , 156), for example, the “elements of a design” consist of “assignment, measurement, comparison groups and treatments,” a definition that does not include questions of interest or estimation strategies. In some instances, quantitative researchers do present multiple elements of research design. Gerber and Green (2012) , for example, examine data-generating models, estimands, assignment and sampling strategies, and estimators for use in experimental causal inference; and Shadish, Cook, and Campbell (2002) and Dunning (2012) similarly describe the various aspects of designing quasi-experimental research and exploiting natural experiments.
In contrast, a number of qualitative treatments focus on integrating the many stages of a research design, from theory generation, to case selection, measurement, and inference. In an influential book on mixed method research design for comparative politics, for example, Geddes (2003) articulates the links between theory formation (M), research question formulation (I), case selection and coding strategies (D), and strategies for case comparison and inference (A). King, Keohane, and Verba (1994) and the ensuing discussion in Brady and Collier (2010) highlight how alternative qualitative strategies present tradeoffs in terms of diagnosands such as bias and generalizability. However, few of these texts investigate those diagnosands formally in order to measure the size of the tradeoffs between alternative qualitative strategies 4 . Qualitative approaches, including process tracing and qualitative comparative analysis, sometimes appear almost hermetic, complete with specific epistemologies, types of research questions, modes of data gathering, and analysis. Though integrated, these strategies are often not formalized. And if they are, it is seldom in a way that enables comparison with other approaches or quantification of design tradeoffs.
MIDA represents an attempt to thread the needle between these two traditions. Quantifying the strength of designs necessitates a language for formally describing the essential features of a design. The relatively fragmented manner in which the quantitative design is thought of in existing work may produce real research risks for individual research projects. In contrast, the more holistic approaches of some qualitative traditions offer many benefits, but formal design diagnosis can be difficult. Our hope is that MIDA provides a framework for doing both at once.
A useful way to illustrate the fragmented nature of thinking on research design among quantitative scholars is to examine the tools that are actually used to do research design. Perhaps the most prominent of these are “power calculators.” These have an all-design flavor in the sense that they ask whether, given an answer strategy, a data collection strategy is likely to return a statistically significant result. Power calculations like these are done using formulae (e.g., Cohen 1977 ; Haseman 1978 ; Lenth 2001 ; Muller et al. 1992 ; Muller and Peterson 1984 ); software tools such as Web applications and general statistical software (e.g., easy power for R and Power and Sample Size for Stata) as well as standalone tools (e.g., Optimal Design, G*Power, nQuery, SPSS Sample Power); and sometimes Monte Carlo simulations.
In most cases these tools, though touching on multiple parts of a design, in fact leave almost no scope to describe what the data generating processes can be, what the questions of interest are, and what types of analyses will be undertaken. We conducted a census of currently available diagnostic tools (mainly power calculators) and assessed their ability to correctly diagnose three variants of a common experimental design, in which assignment probabilities are heterogeneous by block. 5 The first variant simply uses a difference-in-means estimator (DIM), the second conditions on block fixed effects (BFE), and the third includes inverse- probability weighting to account for the heterogeneous assignment probabilities (BFE-IPW).
We found that the vast majority of tools used are unable to correctly characterize the tradeoffs these three variants present. As shown in Table 2 , none of the tools was able to diagnose the design while taking account of important features that bias unweighted estimators. 6 In our simulations the result is an overstatement of the power of the difference-in-means.
Existing Tools Cannot Declare Many Core Elements of Designs and, as a Result, Can Only Calculate Some Diagnosands
Note: Panel (a) indicates the number of tools that allow declaration of a particular feature of the design as part of the diagnosis. In the first row, for example, 0/30indicates that no tool allows researchers to declare correlated effect and block sizes. Panel (b) indicates the number of tools that can perform a particular diagnosis. Results correspond to design tool census concluded in July 2017 and do not include tools published since then.
Because no tool was able to account for weighting in the estimator, none was able to calculate the power for the IPW-BFE answer strategy. Moreover, no tool sought to calculate the design’s bias, root mean- squared-error, or coverage (which require information on I ). The companion software to this article, which was designed based on MIDA, illustrates that power is a misleading indicator of quality in this context. While the IPW-BFE estimator is better powered and less biased than the BFE estimator, its purported efficiency is misleading. IPW-BFE is better powered than DIM and BFE because it produces biased variance estimates that lead to a coverage probability that is too low. In terms of RMSE and the standard deviation of estimates, the IPW-BFE strategy does not outperform the BFE estimator. This exercise should not be taken as proof of the superiority of one strategy over another in general; instead we learn about their relative performance for particular diagnosands for the specific design declared.
We draw a number of conclusions from this review of tools.
First, researchers are generally not designing studies using the actual strategies that they will use to conduct analysis. From the perspective of the overall designs, the power calculations are providing the wrong answer.
Second, the tools can drive scholars toward relatively narrow design choices. The inputs to most power calculators are data strategy elements like the number of units or clusters. Power calculators do not generally focus on broader aspects of a design, like alternative assignment procedures or the choice of estimator. While researchers may have an awareness that such tradeoffs exist, quantifying the extent of the tradeoff is by no means obvious until the model, inquiry, data strategy, and answer strategy is declared in code.
Third, the tools focus attention on a relatively narrow set of questions for evaluating a design. While understanding power is important for some designs, the range of possible diagnosands of interest is much broader. Quantitative researchers tend to focus on power, when other diagnosands such as bias, coverage, or RMSE may also be important. MIDA makes clear, however, that these features of a design are often linked in ways that current practice obscures.
A second illustration of risks arising from a fragmented conceptualization of research design comes from debates over the disproportionate focus on estimators to the detriment of careful consideration of estimands. Huber (2013) , for example, worries that the focus on identification leads researchers away from asking compelling questions. In the extreme, the estimators themselves (and not the researchers) appear to select the estimand of interest. Thus, Deaton (2010) highlights how instrumental variables approaches identify effects for a subpopulation of compliers. Who the compliers are is jointly determined by the characteristics of the subjects and also by the data strategy. The implied estimand (the Local Average Treatment Effect, sometimes called the Complier Average Causal Effect) may or may not be of theoretical interest. Indeed, as researchers swap one instrument for another, the implied estimand changes. Deaton’s worry is that researchers are getting an answer, but they do not know what the question is. 7 Were the question posed as the average effect of a treatment, then the performance of the instrument would depend on how well the instrumental variables regression estimates that quantity, and not how well they answer the question for a different subpopulation. This is not done in usual practice, however, as estimands are often not included as part of a research design.
To illustrate risks arising from the combination of a fractured approach to design in the formal quantitative literature, and the holistic but often less formal approaches in the qualitative literature, we point to difficulties these approaches have in learning from each other.
Goertz and Mahoney (2012) tell a tale of two cultures in which qualitative and quantitative researchers differ not just in the analytic tools they use, but in very many ways, including, fundamentally, in their conceptualizations of causation and the kinds of questions they ask. The authors claim (though not all would agree) that qualitative researchers think of causation in terms of necessary and/or sufficient causes, whereas many quantitative researchers focus on potential outcomes, average effects, and structural equations. One might worry that such differences would preclude design declaration within a common framework, but they need not, at least for qualitative scholars that consider causes in counterfactual terms. 8
For example, a representation of a causal process in terms of causal configurations might take the form: Y = AB + C, meaning that the presence of A and B or the presence of C is sufficient to produce Y . This configuration statement maps directly into a potential outcomes function (or structural equation) of the form Y ( A, B, C ) = max( AB, C ). Given this, the marginal effect of one variable, conditional on others, can be translated to the conditions in which the variable is difference-making in the sense of altering relevant INUS 9 conditions: E(Y(A = 1| B , C) − Y(A = 0| B , C )) = E(B = 1, C = 0). 10 Describing these differences in notation as differences in notions of causality suggests that there is limited scope for considering designs that mix approaches, and that there is little that practitioners of one approach can say to practitioners of another approach. In contrast, clarification that the difference is one regarding the inquiry—i.e., which combinations of variables guarantee a given outcome and not the average marginal effect of a variable across conditions—opens up the possibility to assess how quantitative estimation strategies fare when applied to estimating this estimand.
A second point of difference is nicely summarized by Goertz and Mahoney (2012 , 230): “qualitative analysts adopt a ‘causes-of-effects’ approach to explanation [... whereas] statistical researchers follow the ‘effects-of- causes’ approach employed in experimental research.” We agree with this association, though from a MIDA perspective we see such distinctions as differences in estimands and not as differences in ontology. Conditioning on a given X and Y the effects-of-cause question is E(Yi(Xi = 1) − Y i ( X i = 0)). By contrast, the cause-of- effects question can be written Pr( Y i (0) = 0| X i = 1, Y i (1) = 1). This expression asks what are the chances that Y would have been 0 if X were 0for aunit i for which X was 1 and Y i (1) was 1. The two questions are of a similar form though the cause-of-effects question is harder to answer ( Dawid 2000 ). Once thought of as questions about what the estimand is, one can assess directly when one or another estimation strategy is more or less effective at facilitating inference about the estimand of interest. In fact, experiments are in general not able to solve the identification problem for cause-of-effects questions ( Dawid 2000 ) and this may be one reason for why these questions are often ignored by quantitative researchers. Exceptions include Yamamoto (2012) and Balke and Pearl (1994) .
Below, we demonstrate gains from declaration of designs in a common framework by providing examples of design declaration for crisp-set qualitative comparative analysis ( Ragin 1987 ), nested case analysis ( Lieberman 2005 ), and CPO (causal process observation) process-tracing ( Collier 2011 ; Fairfield 2013 ), alongside experimental and quasi-experimental designs.
Overall, this discussion suggests that the common ways in which designs are conceptualized produce three distinct problems. First, the different components of a design may not be chosen to work optimally together. Second, consideration is unevenly distributed across components of a design. Third, the absence of a common framework across research traditions obscures where the points of overlap and difference lie and may limit both critical assessment of approaches and cross-fertilization. We hope that the MIDA framework and tools can help address these challenges.
DECLARING AND DIAGNOSING RESEARCH DESIGNS IN PRACTICE
A design that can be declared in computer code can then be simulated in order to diagnose its properties. The approach to declaration that we advocate is one that conceives of a design as a concatenation of steps. To illustrate, the top panel of Table 3 shows how to declare a design in code using the companion software to this paper, DeclareDesign ( Blair etal. 2018 ). The resulting set of objects (p _U, f_Y, I, p _S, p _Z, R, and A ) are all steps. Formally, each of these steps is a function. The design is the concatenation of these, which we represent using the “ + ” operator: design <– p _U + f _Y + I + p _S + p _Z + R + A . A single simulation runs through these steps, calling each of the functions successively. A design diagnosis conducts m simulations, then summarizes the resulting distribution of diagnostic statistics in order to estimate the diagnosand.
A Procedure for Declaring and Diagnosing Research Designs Using the Companion Software DeclareDesign ( Blair et al. 2018 )
Note: The top panel includes each element of a design that can be declared along with code used to declare them. The middle panel describes steps to simulate that design. The bottom panel includes the procedure to diagnose the design.
Diagnosands can be estimated with higher levels of precision by increasing m . However, simulations are often computationally expensive. In order to assess whether researchers have conducted enough simulations to be confident in their diagnosand estimates, we recommend estimating the sampling distributions of the diagnosands via the nonparametric bootstrap. 11 With the estimated diagnosand and its standard error, we can characterize our uncertainty about whether the range of likely values of the diagnosand compare favorably to reference values such as statistical power of 0.8. 12
Design diagnosis places a burden on researchers to come up with a causal model, M. Since researchers presumably want to learn about the model, declaring it in advance may seem to beg the question. Yet declaring a model is often unavoidable when diagnosing designs. In practice, doing so is already familiar to any researcher who has calculated the power of a design, which requires the specification of effect sizes. The seeming arbitrariness of the declared model can be mitigated by assessing the sensitivity of diagnosis to alternative models and strategies, which is relatively straightforward given a diagnosand-complete design declaration. Further, researchers can inform their substantive models with existing data, such as baseline surveys. Just as power calculators focus attention on minimum detectable effects, design declaration offers a tool to demonstrate design properties and how they change depending on researcher assumptions.
In the next sections, we illustrate how research designs that aim to answer descriptive, causal, and exploratory research questions can be declared and diagnosed in practice. We then describe how the estimand-focused approach we propose works with designs that focus less on estimand estimation and more on modeling data generating processes. In all cases, we highlight potential gains from declaring designs using the MIDA framework.
Descriptive Inference
Descriptive research questions often center on measuring a parameter in a sample or in the population, such as the proportion of voters in the United States who support the Democratic candidate for president. Although seemingly very different from designs that focus on causal inference, because of the lack of explanatory variables, the formal differences are not great.
Survey Designs
We examine an estimator of candidate support that conditions on being a “likely voter.” For this problem, the data that help researchers predict who will vote are of critical importance. In the Supplementary Materials Section 3.1 , we declare a model in which latent voters are likely to vote for a candidate, but overstate their true propensity to vote. The inquiry is the true underlying support for the candidate among those who will vote, while the data strategy involves taking a random sample from the national adult population and asking survey questions that measure vote intention and likelihood of voting. As an answer strategy, we estimate support for the candidate among likely voters. The diagnosis shows that when people misreport whether they vote, estimates of candidate support may be biased, a commonplace observation about the weaknesses of survey measures. The utility of design declaration here is that we can calibrate how far off our estimates will be under reasonable models of misreporting.
Bayesian Descriptive Inference
Although our simulation approach has a frequentist flavor, the MIDA framework itself can also be applied to Bayesian strategies. In Supplementary Materials Section 3.2 , we declare a Bayesian descriptive inference design. The model stipulates a latent probability of success for each unit, and makes one binomial draw for each according to this probability. The inquiry is the true latent probability, and the data strategy involves a random sample of relatively few units. We consider two answer strategies: first, we stipulate uniform priors, with a mean of 0.50 and a standard deviation of 0.29; in the second, we place more prior probability mass at0.50, with a standard deviation of 0.11.
Once declared, the design can be diagnosed not only in terms of its bias, but also as a function of quantities specific to Bayesian estimation approaches, such as the expected shift in the location and scale of the posterior distribution relative to the prior distribution. The diagnosis shows that the informative prior approach yields more certain and more biased inferences than the uniform prior approach. In terms of the bias-variance tradeoff, the informative priors decrease the posterior standard deviation by 40% relative to the uniform priors, but increase the bias by 33%.
Causal Inference
The approach to design diagnosis we propose can be used to declare and diagnose a range of research designs typically employed to answer causal questions in the social sciences.
Process Tracing
Although not all approaches to process tracing are readily amenable to design declaration (e.g., theory-building process tracing, see Beach and Pedersen 2013 , 16), some are. We focus here on Bayesian frameworks that have been used to describe process tracing logics (e.g., Bennett 2015 ; Humphreys and Jacobs 2015 ; Fairfield and Charman 2017 ). In these approaches, “causal process observations” (CPOs) are believed to be observed with different probabilities depending on the causal process that has played out in a case. Ideal-type CPOs as described by Van Evera (1997) are “hoop tests” (CPOs that are nearly certain to be seen if the hypothesis is true, but likely either way), “smoking-gun tests” (CPOs that are unlikely to be seen in general but are extremely unlikely if a hypothesis is false), and “doubly- decisive tests” (CPOs that are likely tobe seen if and only if a hypothesis is true) 13 . Unlike much quantitative inference, such studies often pose “causes-of-effects” inquiries (did the presence of a strong middle class cause a revolution?), and not “effects-of-causes” questions (what is the average effect of a strong middle class on the probability of a revolution happening?) ( Goertz and Mahoney 2012 ). Such inquiries often imply a hypothesis— “the strong middle class caused the revolution,” say—that can be investigated using Bayes’ rule.
Formalizing this kind of process-tracing exercise leads to non-obvious insights about the tradeoffs involved in committing to one or another CPO strategy ex ante. We declare a design based on a model of the world in which both the driver, X , and the outcome, Y , might be present in a given case either because X caused Y or because Y would have been present regardless of X (or perhaps, an alternative cause was responsible for Y). See Supplementary Materials Section 3.3 . The inquiry is whether X in fact caused Y in the specific case under analysis (i.e., would Y have been different if X were different?). The data strategy consists of selecting one case from a population of cases, based on the fact that both X and Y are present, and then collecting two causal process observations. Even before diagnosis, the declaration of the design illustrates an important point: the case selection strategy informs the answer strategy by enabling the researcher to narrow down the number of causal processes that might be at play. This greatly simplifies the application of Bayes’ rule to the case in question.
Importantly, the researcher attaches two different ex ante probabilities to the observation of confirmatory evidence in each CPO, depending on whether X did or did not cause Y . Specifically, the first CPO contains evidence that is more likely to be seen when the hypothesis is true, Pr(E 1 |H) = 0.75, but even when H is false and Y happened irrespective of X , there is some probability of observing the first piece of evidence: Pr(E 1 |¬H) = 0.25. The first CpO thus constitutes a “straw-in-the-wind” test (albeit a reasonably strong one). By contrast, the probability of observing the evidence in the second CPO when the hypothesis that X caused Y is true, Pr(E 2 |H) is 0.30, whereas the probability of observing the evidence when the hypothesis is false, Pr(E 2 |¬H) is only 0.05. The second CPO thus constitutes a “smoking gun” test of H . Observing the second piece of evidence is more informative than observing the first, because it is so unlikely to observe a smoking gun when the hypothesis is false.
Diagnosis reveals that a researcher who relied solely on the weaker “straw-in-the-wind” test would make better inferences on average than one who relied solely on the “smoking gun” test. One does better relying on the straw because, even if it is less informative when observed, it is much more commonly observed than the smoking gun, which is an informative, but rare, clue. The Collier (2011 , 826) assertion that, of the four tests, straws-in-the-wind are “the weakest and place the least demand on the researcher’s knowledge and assumptions” might thus be seen as an advantage rather than a disadvantage. In practice, of course, scholars often seek multiple CPOs, possibly of different strength (see, for example, Fairfield 2013 ). In such cases, the diagnosis suggests the learning depends on the ways in which these CPOs are correlated. There are large gains from seeking two CPOs when they are negatively correlated— for example, if they arise from alternative causal processes. But there are weak gains when CPOs arise from the same process. Presentations of process tracing rarely describe correlations between CPO probabilities yet the need to specify these (and the gain from doing so) presents itself immediately when a process tracing design is declared.
Qualitative Comparative Analysis (QCA)
One approach to mixed methods research focuses on identifying ways that causes combine to produce outcomes. What, for instance, are the combinations of demography, natural resource abundance, and institutional development that give rise to civil wars? An answer might be of the form: conflicts arise when there is natural resource abundance and weak institutional structure or when there are deep ethnic divisions. The key idea is that different configurations of conditions can lead to the same outcome (equifinality) and the interest is in assessing which combinations of conditions matter.
Many applications of qualitative comparative analysis use Boolean minimization algorithms to assess which configurations of factors are associated with different outcomes. Critics have highlighted that these algorithms are sensitive to measurement error ( Hug 2013 ). Pointing to such sensitivity, some even go as far as to call for the rejection of QCA as a framework for inquiry ( Lucas and Szatrowski 2014 ; for a nuanced response, see Baumgartner and Thiem 2017 ).
However, a formal declaration of a QCA design makes clear that these criticisms unnecessarily conflate QCA answer strategies with their inquiries (for a similar argument, see Collier 2014 ). Contrary to claims that regression analysis and QCA stem from fundamentally different ontologies ( Thiem, Baumgartner, and Bol 2016 ), we show that saturated regression analysis may mitigate measurement error concerns in QCA. This simple proof of concept joins efforts toward unifying QCA with aspects of mainstream statistics ( Braumoeller 2003 ; Rohlfing 2018 ) and other qualitative approaches ( Rohlfing and Schneider 2018 ).
In Supplementary Materials Section 3.4 we declare a QCA design, focusing on the canonical case of binary variables (“crisp-set QCA”). The model features an outcome Y that arises in a case if and only if cause A is absent and cause B is present (Y = a * B). The approach extends readily to cases with many causes in complex configurations. For our inquiry, we wish to know the true minimal set of configurations of conditions that are sufficient to cause Y . The data strategy involves measuring and encoding knowledge about Y in a truth table. We allow for some error in this process. As in Rohlfing (2018) , we are agnostic as to how this error arises: it may be that scholarly debate generates epistemic uncertainty about whether Y is truly present or absent in a given case, or that there is measurement error due to sampling variability.
For answer strategies, we compare two QCA minimization approaches. The first employs the classical Quine-McCluskey (QMC) minimization algorithm (see Duşa and Thiem 2015 , for a definition) and the second the “Consistency Cubes” (CCubes) algorithm ( Duşa 2018 ) to solve for the set of causal conditions that produces Y . This comparison demonstrates the utility of declaration and diagnosis for researchers using QCA algorithms, who might worry about whether their choice of algorithm will alter their inferences. 14 We show that, at least in simple cases such as this, such concerns are minimal.
We also consider how ordinary least squares minimization performs when targeting a QCA estimand. The right hand side of the regression includes indicators for membership in all feasible configurations of A and B. Configurations that predict the presence of Y with probability greater than 0.5 are then included in the set of sufficient conditions.
The diagnosis of this design shows that QCA algorithms can be successful at pinpointing exactly the combination of conditions that give rise to outcomes. When there is no error and the sample is large enough to ensure sufficient variation in the data, QMC and CCubes successfully recover the correct configuration 100% of the time. The diagnosis also confirms that QCA via saturated regression can recover the data generating process correctly and the configuration of causes esti- mand can then be computed, correctly, from estimated marginal effects.
This last point is important for thinking through the gains from employing the MIDA framework. The declaration clarifies that QCA is not equivalent to saturated regression: without substantial transformation, regression does not target the QCA estimands ( Thiem, Baumgartner, and Bol 2016 ). However, it also clarifies that regression models can be integrated into classical QCA inquiries, and do very well. Using regression to perform QCA is equivalent to QMC and CCubes when there is no error, and even slightly outperforms these algorithms (on the diagnosands we consider) in the presence of measurement error. More work is required to understand the conditions under which the approaches perform differently.
However, the declaration and diagnosis illustrate that there need not be a tension between regression as an estimation procedure and causal configurations as an estimand. Rather than seeing them as rival research paradigms, scholars interested in QCA estimands can combine the machinery developed in the QCA literature to characterize configurations of conditions with the machinery developed in the broader statistical literature to uncover data generating processes. Thus, for instance, in answer to critiques that the method does not have a strategy for causal identification ( Tanner 2014 ), one could in principle try to declare designs in which instrumental variables strategies, say, are used in combination with QCA estimands.
Nested Mixed Methods
A second approach to mixed methods research nests qualitative small N analysis within a strategy that involves movement back and forwards between large N theory testing and small N theory validation and theory generation. Lieberman (2005) describes a strategy of nested analysis of this form. In Supplementary Materials Section 3.5 , we specify the estimands and analysis strategies implied by the procedure proposed in Lieberman (2005) . In our declaration, we assume a model with binary variables and an inquiry focused on the relationship between X and Y (both causes-of-effects and effects-of-causes are studied). The model allows for the possibility that there are variables that are not known to the researcher when conducting large N analysis, but might modify or confound the relationship between X and Y . The data strategy and answer strategies are quite complex and integrated with each other. The researcher begins by analyzing a data set involving X and Y . If the quantitative analysis is “successful” (defined in terms of sufficient residual variance explained), the researcher engages in within- case “on the regression line” analysis. Using within-case data, the researcher assesses the extent to which X plausibly caused Y (or not X caused not Y ) in these cases. If the qualitative or quantitative analyses reject the model, then a new qualitative analysis is undertaken to better understand the relationship between X and Y . In the design, this qualitative exploration is treated as the possibility of discovering the importance of a third variable that may moderate the effect of X on Y . If an alternative model is successfully developed, it is then tested on the same large N data.
Diagnosis of this design illustrates some of its advantages. In particular, in some settings the within- case analysis can guide researchers to models that better capture data generating processes and improve identification. The declaration also highlights the design features that are left to researchers. How many cases should be gathered and how should they be selected? What thresholds should be used to decide whether a theory is successful or not? The design diagnosis suggests interesting interactions between these design elements. For instance, if the bar for success in the theory testing stage is low in terms of the minimum share of cases explained that are considered adequate, then the researcher might be better off sampling fewer qualitative cases in the testing stage and more in the development stage. More variability in the first stage makes it more likely that one would reject a theory, which might in turn lead to the discovery of a better theory.
Observational Regression-Based Strategies
Many observational studies seek to make causal claims, but do not explicitly employ the potential outcomes framework, instead describing inquiries in terms of model parameters. Sometimes studies describe their goal as the estimation of a parameter b from a model of the form y i = α + βx i + ε i . What is the estimand here? If we believe that this model describes the true data generating process, then β is an estimand: it is the true (constant) marginal effect of x on y . But what if we are wrong about the model? We run into a problem if we want to assess the properties of strategies under different assumptions about data generation when the inquiry itself depends on the data generating model.
To address this problem, we can declare an inquiry as a summary of differences in potential outcomes across conditions, β . Such a summary might derive from a simple comparison of potential outcomes—for example τ ≡ E x E i ( Y i ( x ) − Y i ( x − 1 ) ) captures the difference in outcomes between having income x and having a dollar less, x − 1, for different possible income levels. Or it could be a parameter from a model applied to the potential outcomes. For example we might define α and β as the solutions to:
Here Y i ( x ) is the (unknown) potential outcome for unit i in condition x . Estimand β can be thought of as the coefficient one would get on x if one were to able to regress all possible potential outcomes on all possible conditions for all units (given density of interest f ( x )). 15 Our data strategy will simply consist of the passive observation of units in the population, and we assess the performance of an answer strategy employing an OLS model to estimate β under different conditions.
To illustrate, we declare a design that lets us quickly assess the properties of a regression estimate under the assumption that in the true data-generating process y is in fact a nonlinear function of x ( Supplementary Materials Section 3.6 ). Diagnosis of the design shows that under uniform random assignment of x , the linear regression returns an unbiased estimate of a (linear) estimand, even though the true data generating process is nonlinear. Interestingly, with the design in hand, it is easy to see that unbiasedness is lost in a design in which different values of x i are assigned with differing probabilities. The benefit of declaration here is that, without defining I , it is hard to see the conditions under which A is biased or unbiased. Declaration and diagnosis clarify that, even though the answer strategy “assumes” a nonlinear relationship in M that does not hold, under certain conditions OLS is still able to estimate a linear summary of that relationship.
Matching on Observables
In many observational research designs, the processes by which units are assigned to treatment are not known with certainty. In matching designs, the effects of unknown assignment procedure may, for example, be assessed by matching units on their observable traits under an assumption of as-if random assignment between matched pairs. Diagnosis in such instances can shed light on risks when such assumptions are not justified. In Supplementary Materials Section 3.7 , we declare a design with a model in which three observable random variables are combined in a probit process that assigns the treatment variable, Z . The inquiry pertains to the average treatment effect of Z on the outcome Y among those actually assigned to treatment, which we estimate using an answer strategy that tries to reconstruct the assignment process to calculate a A . Our diagnosis shows that matching improves mean-squared- error ( E [( a A − a M ) 2 ]) relative to a naive difference-in-means estimator of the treatment effect on the treated (ATT), but can nevertheless remain biased (E[ a A − a M ] ≠ 0) if the matching algorithm does not successfully pair units with equal probabilities of assignment, i.e., if matching has not eliminated all sources of confounding. The chief benefit of the MIDA declaration here is to separate out beliefs about the data generating process ( M ) from the details of the answer strategy ( A ), whose robustness to alternative data generating processes can then be assessed.
Regression Discontinuity
While in many observational settings researchers do not know the assignment process, in others, researchers may know how assignment works without necessarily controlling it. In regression discontinuity designs, causal identification is often premised on the claim that potential outcomes are continuous at a critical threshold (see De la Cuesta and Imai 2016 ; Sekhon and Titiunik 2016 ). The declaration of such designs involves a model that defines the unknown potential outcomes functions mapping average outcomes to the running and treatment variables. Our inquiry is the difference in the conditional expectations of the two potential outcomes functions at the discontinuity. The data strategy involves passive observation and collection of the data. The answer strategy is a polynomial regression in which the assignment variable is linearly interacted with a fourth order polynomial transformation of the running variable. In Supplementary Materials Section 3.8 , we declare and diagnose such a design.
The declaration highlights a difference between this design and many others: the estimand here is not an average of potential outcomes of a set of sample units, but rather an unobservable quantity defined at the limit of the discontinuity. This feature makes the definition of diagnosands such as bias or external validity conceptually difficult. If researchers postulate unobservable counter-factuals, such as the “treated” outcome for a unit located below the treatment threshold, then the usefulness of the regression discontinuity estimate of the average treatment effect for a specific set of units can be assessed.
Experimental Design
In experimental research, researchers are in control of sample construction and assignment of treatments, which makes declaring these parts of the design straightforward. A common choice faced in experimental research is between employing a 2-by-2 factorial design or a three-arm trial where the “both” condition is excluded. Suppose we are interested in the effect of each of two treatments when the other condition is set to control. Should we choose a factorial design or a three- arm design? Focusing for simplicity on the effect of a single treatment, we declare two designs under a range of alternative models to help assess the tradeoffs. For both designs, we consider models M 1 ,..., M K , where we let the interaction between treatments vary over the range −0.2 to +0.2. Our inquiry is always the average treatment effect of treatment 1 given all units are in the control condition for treatment 2. We consider two alternative data strategies: an assignment strategy in which subjects are assigned to a control condition, treatment 1, or treatment 2, each with probability 1/3; and an alternative strategy in which we assign subjects to each of four possible combinations of factors with probability 1/4. The answer strategy in both cases involves a regression of the outcome on both treatment indicators with no interaction term included.
We declare and diagnose this design and confirm that neither design exhibits bias when the true interaction term is equal to zero ( Figure 1 left panel). The details of the declaration can be found in Supplementary Materials Section 3.9 . However, when the interaction between the two treatments is stronger, the factorial design renders estimates of the effect of treatment 1 that are more and more biased relative to the “pure” main effect estimand. Moreover, there is a bias-variance tradeoff in choosing between the two designs when the interaction is weak ( Figure 1 right panel). When the interaction term is close to zero, the factorial design is preferred, because it is more powerful: it compares one half of the subject pool to the other half, whereas the three-arm design only compares a third to a third. However, as the magnitude of the interaction term increases, the precision gains are offset by the increase in bias documented in the left-panel. When the true interaction between treatments is large, the three-arm design is then preferred. This exercise highlights key points of design guidance. Researchers often select factorial designs because they expect interaction effects, and indeed factorial designs are required to assess these. However if the scientific question of interest is the pure effect of each treatment, researchers should (perhaps counterintuitively) use a factorial design if they expect weak interaction effects. An integrated approach to design declaration here illustrates non-trivial interactions between the d ata strategy, on the one hand, and the ability of answers (a A ) to approximate the estimand ( a M ), on the other.
FIGURE 1. Diagnoses of Designs With Factorial or Three-Arm Assignment Strategies Illustrate a Bias-Variance Tradeoff.
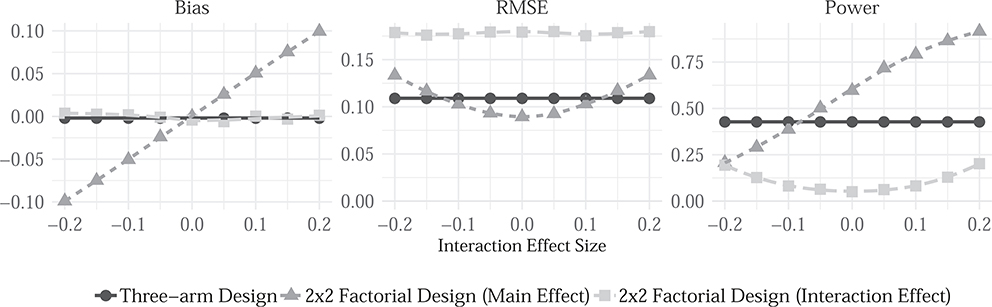
Bias (left), root mean-squared-error (center), and power (right) are displayed for two assignment strategies, a 2 × 2 treatment arm factorial design (black solid lines; circles) and a three-arm design (gray dashed lines; triangles) according to varying interaction effect sizes specified in the potential outcomes function ( x axis). The third panel also shows power for the interaction effect (squares) from the factorial design.
Designs for Discovery-Oriented Research
In some research projects, the ultimate hypotheses that are assessed are not known at the design stage. Some inductive designs are entirely unstructured and explore a variety of data sources with a variety of methods within a general domain of interest until a new insight is uncovered. Yet many can be described in a more structured way.
In studying textual data, for example, a researcher may have a procedure for discovering the “topics” that are discussed in a corpus of documents. Before beginning the research, the set of topics and even the number of topics is unknown. Instead, the researcher selects a model for estimating the content of a fixed number of topics (e.g., Blei, Ng, and Jordan 2003 ) and a procedure for evaluating the model fit used to select which number of topics fits the data best. Such a design is inductive, yet the analytical discovery process can be described and evaluated.
We examine a data analysis procedure in which the researcher assesses possible analysis strategies in a first stage on half of the data and in the second stage applies her preferred procedure to the second half of the data. Split-sample procedures such as this enable researchers to learn about the data inductively while protecting against Type I errors (for an early discussion of the design, see Cox 1975 ). In Supplementary Materials Section 3.10 , we declare a design in which the model stipulates a treatment of interest, but also specifies groups for which there might be heterogeneous treatment effects. The main inquiry pertains to the treatment effect, but the researchers anticipate that they may be interested in testing for heterogeneous treatment effects if they observe prima facie evidence for it. The data strategy involves random assignment. The answer strategy involves examination of main effects, but in addition the researchers examine heterogeneous treatment effects inside a random subgroup of the data. If they find evidence of differential effects they specify a new inquiry which is assessed on the remaining data. The results on heterogeneous effects are compared against a strategy that simply reports discoveries found using complete data, rather than on split data (we call this the “unprincipled” approach).
We see lower bias from principled discovery than from unprincipled discovery as one might expect. The declaration and diagnosis also highlight tradeoffs in terms of mean squared error. Mean squared error is not necessarily lower for the principled approach since fewer data are used in the final test. Moreover, the principled strategy is somewhat less likely to produce a result at all since it is less likely that a result would be discovered in a subset of the data than in the entire data set. With this design declared, one can assess what an optimal division of units into training and testing data might be given different hypothesized effect sizes.
Designs for Modeling Data Generation Processes
For most designs we have described, the estimand of interest is a number: an average level, a causal effect, or a summary of causal effects. Yet in some situations, researchers seek not to estimate a particular number, but rather to model a data generating process. For work of this kind, the data generating process is the estimand, rather than any particular comparison of potential outcomes. This was the case for the qualitative QCA design we looked at, in which the combination of conditions that produce an outcome was the estimand. This model- focused orientation is also common for quantitative researchers. In the example from Observational Regression-Based Strategies, we noted that a researcher might be interested not in the average effect resulting from a change in X over some range, but in estimating a function f Y * ( X ) (which itself might be used to learn about different quantities of interest). This kind of approach can be handled within the MIDA framework in two ways. One asks the researcher to identify the ultimate quantities of interest ex ante and to treat these as the estimands. In this case, the model generated to make inferences about quantities of interest is thought of as part of the answer strategy, a, and not part of i . A second approach posits a true underlying DGP as part of m , f Y * * . The estimand is then also a function, f Y * , which could be f Y * * itself or an approximation. 16 An estimate is a function f Y that aims to approximate f Y * . In this case, it is difficult to think of diagnosands like bias or coverage when comparing f Y * to f Y , but diagnosands can still be constructed that measure the success of the modeling. For instance, for a range of values of X we could compare values of f Y ( X ) to f Y * ( X ) , or employ familiar statistics of goodness of fit, such as the R 2 . The MIDA framework forces clarity regarding which of these approaches a design is using, and as a consequence, what kinds of criticisms of a design are on target. For instance, returning to the regression strategies example: if a linear model is used to estimate a linear estimand, it may behave well for that purpose even when the underlying process is very nonlinear. If, however, the goal is to estimate the shape of the data generating process, the linear estimator will surely fare poorly.
The research designs we have described in this section are varied in the intellectual traditions as well as inferential goals they represent. Yet commonalities emerge, which enabled us to declare each design in terms of MIDA. Exploring this broad set of research practices through MIDA clarified non-obvious aspects of the designs, such as the target of inference (Inquiry) in QCA designs or regression discontinuity designs with finite units, as well as the subtle implications of beliefs about heterogeneity in treatment effects (Model) for selecting between three-arm and 2 × 2 factorial designs.
PUTTING DECLARATIONS AND DESIGN DIAGNOSIS TO USE
We have described and illustrated a strategy for declaring research designs for which “diagnosands” can be estimated given conjectures about the world. How might declaring and diagnosing research designs in this way affect the practices of authors, readers, and replication authors? We describe implications for how designs are chosen, communicated, and challenged.
Making Design Choices
The move toward increasing credibility of research in the social sciences places a premium on considering alternative data strategies and analysis strategies at early stages of research projects, not only because it reduces researcher discretion after observing outcomes, but more importantly because it can improve the quality of the final research design. While there is nothing new about the idea of determining features such as sampling and estimation strategies ex ante, in practice many designs are finalized late in the research process, after data are collected. Frontloading design decisions is difficult not only because existing tools are rudimentary and often misleading, but because it is not clear in current practice what features of a design must be considered ex ante.
We provide a framework for identifying which features affect the assessment of a design’s properties, declaring designs and diagnosing their inferential quality, and frontloading design decisions. Declaring the design’s features in code enables direct exploration of alternative data and analysis strategies using simulated data; evaluating alternative strategies through diagnosis; and exploring the robustness of a chosen strategy to alternative models. Researchers can undertake each step before study implementation or data collection.
Communicating Design Choices
Bias in published results can arise for many reasons. For example, researchers may deliberately or in advertently select analysis strategies because they produce statistically significant results. Proposed solutions to reduce this kind of bias focus on various types of preregistration of analysis strategies by researchers ( Casey, Glennerster, and Miguel 2012 ; Green and Lin 2016 ; Nosek et al. 2015 ; Rennie 2004 ; Zarin and Tse 2008 ). Study registries are now operating in numerous areas of social science, including those hosted by the American Economic Association, Evidence in Governance and Politics, and the Center for Open Science. Bias may also arise from reviewers basing publication recommendations on statistical significance. Results- blind review processes are being introduced in some journals to address this form of bias (e.g., Findley et al. 2016 ).
However, the effectiveness of design registries and results-blind review in reducing the scope for either form of publication bias depends on clarity over which elements must be included to describe the design. In practice, some registries rely on checklists and preanalysis plans exhibit great variation, ranging from lists of written hypotheses to all-but-results journal articles. In our view, the solution to this problem does not lie in ever-more-specific questionnaires, but rather in a new way of characterizing designs whose analytic features can be diagnosed through simulation.
The actions to be taken by researchers are described by the data strategy and the answer strategy; these two features of a design are clearly relevant elements of a preregistration document. In order to know which design choices were made ex ante and which were arrived at ex post, researchers need to communicate their data and answer strategies unambiguously. However, assessing whether the data and answer strategies are any good usually requires specifying a model and an inquiry. Design declaration can clarify for researchers and third parties what aspects of a study need to be specified in order to meet standards for effective preregistration. Rather than asking: “are the boxes checked?” the question becomes: “can it be diagnosed?” The relevant diagnosands will likely depend on the type of research design. However, if an experimental design is, for example, “bias complete,” then we know that sufficient information has been given to define the question, data, and answer strategy unambiguously.
Declaration of a design in code also enables a final and infrequently practiced step of the registration process, in which the researcher “reports and reconciles” the final with the planned analysis. Identifying how and whether the features of a design diverge between ex ante and ex post declarations highlights deviations from the preanalysis plan. The magnitude of such deviations determines whether results should be considered exploratory or confirmatory. At present, this exercise requires a review of dozens of pages of text, such that differences (or similarities) are not immediately clear even to close readers. Reconciliation of designs declared in code can be conducted automatically, by comparing changes to the code itself (e.g., a move from the use of a stratified sampling function to simple random sampling) and by comparing key variables in the design such as sample sizes.
Challenging Design Choices
The independent replication of the results of studies after their publication is an essential component of the shift toward more credible science. Replication — whether verification, reanalysis of the original data, or reproduction using fresh studies — provides incentives for researchers to be clear and transparent in their analysis strategies, and can build confidence in findings. 17
In addition to rendering the design more transparent, diagnosand-complete declaration can allow for a different approach to the re-analysis and critique of published research. A standard practice for replicators engaging in reanalysis is to propose a range of alternative strategies and assess the robustness of the data- dependent estimates to different analyses. The problem with this approach is that, when divergent results are found, third parties do not have clear grounds to decide which results to believe. This issue is compounded by the fact that, in changing the analysis strategy, replicators risk departing from the estimand of the original study, possibly providing different answers to different questions. In the worst case scenario, it can be difficult to determine what is learned both from the original study and from the replication.
A more coherent strategy facilitated by design simulations would be to use a diagnosand-complete declaration to conduct “design replication.” In a design replication, a scholar restates the essential design characteristics to learn about what the study could have revealed, not just what the original author reports was revealed. This helps to answer the question: under what conditions are the results of a study to be believed? By emphasizing abstract properties of the design, design replication provides grounds to support alternative analyses on the basis of the original authors’ intentions and not on the basis of the degree of divergence of results. Conversely, it provides authors with grounds to question claims made by their critics.
Table 4 illustrates situations that may arise. In a declared design an author might specify situation 1: a set of claims on the structure of the variables and their potential outcomes (the model) and an estimator (the answer strategy). A critic might then question the claims on potential outcomes (for example, questioning a nospillovers assumption) or question estimation strategies (for example, arguing for inclusion or exclusion of a control variable from an analysis), or both.
Diagnosis Results Given Alternative Assumptions About the Model and Alternative Answer Strategies
Note : Four scenarios encountered by researchers and reviewers of a study are considered depending on whetherthe model orthe answer strategy differ from the author’s original strategy and model.
In this context, there are several possible criteria for admitting alternative answer strategies:
Home Ground Dominance. If ex ante the diagnostics for situation 3 are better than for 1 then this gives grounds to switch to 3. That is, if a critic can demonstrate that an alternative estimation strategy outperforms an original estimation strategy even under the data generating process assumed by an original researcher, then they have strong grounds to propose a change in strategies. Conversely, if an alternative estimation strategy produces different results, conditional on the data, but does not outperform the original strategy given the original assumptions, this gives grounds to question the reanalysis.
Robustness to Alternative Models. If the diagnostics in situation 3 are as good as in 1 but are better in situation 4 than in situation 2 this provides a robustness argument for altering estimation strategies. For example, in a design with heterogeneous probabilities by block, an inverse propensity-weighted estimator will do about as well as a fixed effects estimator in terms of bias when treatment effects are constant, but will perform better on this dimension when effects are heterogeneous.
Model Plausibility. If the diagnostics in situation 1 are better than in situation 3, but the diagnostics in situation 4 are better than in situation 2, then things are less clear and the justification of a change in estimators depends on the plausibility of the different assumptions about potential outcomes.
The normative value or relative ranking of these criteria should be left to individual research communities. Without a declared design, in particular the model and inquiry, none of these criteria can be evaluated, complicating the defense of claims for both the critic and the original author.
APPLICATION: DESIGN REPLICATION OF Björkman and Svensson (2009)
We illustrate the insights that a formalized approach to design declaration can reveal through an application to the design of Björkman and Svensson (2009) , which investigated whether community-based monitoring can improve health outcomes in rural Uganda.
We conduct a “design replication:” using available information, we posit a Model, Inquiry, Data, and Answer strategy to assess properties of Björkman and Svensson (2009) . This design replication can be contrasted with the kind of reanalysis of the study’s data that has been conducted by Donato and Garcia Mosqueira (2016) or the reproduction by Raffler, Posner, and Parkerson (2019) in which the experiment was conducted again.
The exercise serves three purposes: first, it sheds light on the sorts of insights the design can produce without using the original study’s data or code; second, it highlights how difficulties can arise from designs in which the inquiry is not well-defined; third, we can assess the properties of replication strategies, notably those pursued by Donato and Garcia Mosqueira (2016) and Raffler, Posner, and Parkerson (2019) , in order to make clearer the contributions of such efforts.
In the original study, Björkman and Svensson (2009) estimate the effects of treatment on two important indicators: child mortality, defined as the number of deaths per 1,000 live births among under-5 year-olds (taken at the catchment-area-level) and weight-for-age z -scores, which are calculated by subtracting from an infant’s weight the median for their age from a reference population, and dividing by the standard deviation of that population. In the original design, the authors estimate a positive effect of the intervention on weight among surviving infants. They also find that the treatment greatly decreases child mortality.
We briefly outline the steps of our design replication here, and present more detail in Supplementary Materials Section 4 .
We began by positing a model of the world in which unobserved variables, “family health” and “community health,” determine both whether infants survive early childhood and whether they are malnourished.
Our attempt to define the study’s inquiry met with a difficulty: the weight of infants in control areas whose lives would have been saved if they had been in the treatment is undefined (for a discussion of the general problem known as “truncation-by-death,” see Zhang and Rubin 2003 ). Unless we are willing to make conjectures about undefined states of the world (such as the control weight of a child who would not have survived if assigned to the control), we can only define the average difference in individuals’ potential outcomes for those children whose survival is unaffected by the treatment: E [Weight( Z = 1) − Weight( Z = 0)|Alive( Z = 0) = Alive( Z = 1) = 1]. 18
As in the original article we stratify sampling on catchment area and cluster-assign households in 25 of the 50 catchment areas to the intervention.
We estimate mortality at the cluster level and weight- for-age among living children at the household level, as in Björkman and Svensson (2009) .
Figure 2 illustrates how the existence of an effect on mortality can pose problems for the unbiased estimation of an effect on weight-for-age. The histograms represent the sampling distributions of the average effect estimates of community monitoring on infant mortality and weight-for-age. The dotted vertical line represents the true average effect ( a M ). The mortality estimand is defined at the cluster level and the weight- for-age estimand is defined for infants who would survive regardless of treatment status. The dashed line represents the average answer, i.e., the answer we expect the design to provide ( E [ a A ]). The weight-for-age answer strategy simply compares the weights of surviving infants across treatment and control. Under our postulated model of the world, the estimates of the effect on weight-for-age are biased downwards because it is precisely those infants with low health outcomes whose lives are saved by the treatment.
FIGURE 2. Data-independent Replication of Estimates in Björkman and Svensson (2009) .
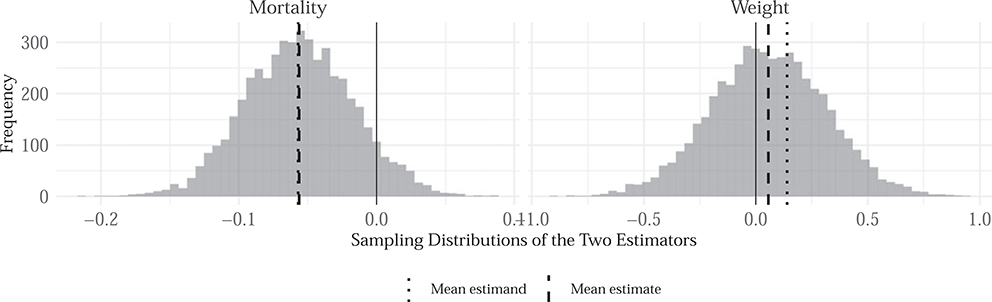
Histograms display the frequency of simulated estimates of the effect of community monitoring on infant mortality (left) and on weight-for-age (right). The dashed vertical line shows the average estimate, the dotted vertical line shows the average estimand.
We draw upon the “robustness to alternative models” criterion (described in the previous section) to argue for an alternative answer strategy that exhibits less bias under plausible conjectures about the world.
An alternative answer strategy is to attempt to subset the analysis of the weight effects to a group of infants whose survival does not depend on the treatment. This approach is equivalent to the “find always-responders” strategy for avoiding post-treatment bias in audit studies ( Coppock 2019 ). In the original study, for example, the effects on survival are much larger among infants younger than two years old. If indeed the survival of infants above this age threshold is unaffected by the treatment, then it is possible to provide unbiased estimates of the weight-for age effect, if only among this group. In terms of bias, such an approach does at least as well if we assume that there is no correlation between weight and mortality, and better if such a correlation does exist. It thus satisfies the “robustness to alternative models” criterion.
A reasonable counter to this replication effort might be to say that the alternative answer strategy does not meet the criterion of “home ground dominance” with respect to RMSE. The increase in variance from subsetting to a smaller group may outweigh the bias reduction that it entails. In both cases, transparent arguments can be made by formally declaring and comparing the original and modified designs.
The design replication also highlights the relatively low power of the weight-for-age estimator. As Gelman and Carlin (2014) have shown, conditioning on statistical significance in such contexts can pose risks of exaggerating the true underlying effect size. Based on our assumptions, what can we say here, specifically, about the risk of exaggeration? How effectively does a design such as that used in the replication by Raffler, Posner, and Parkerson (2019) mitigate this risk? To answer this question, we modify the sampling strategy of our simulation of the original study to include 187 clusters instead of 50. 19 We then define the diagnosand of interest as the “exaggeration ratio” ( Gelman and Carlin 2014 ): the ratio of the absolute value of the estimate to the absolute value of the estimand, given that the estimated effect is significant at the α = 0.05 level. This diagnosand thus provides a measure of how much the design exaggerates effect sizes conditional on statistical significance.
The original design exhibits a high exaggeration ratio, according to the assumptions employed in the simulations: on average, statistically significant estimates tend to exaggerate the true effect of the intervention on mortality by a factor of two and on weight-for-age by a factor of four. In other words, even though the study estimates effects on mortality in an unbiased manner, limiting attention to statistically significant effects provides estimates that are twice as large in absolute value as the true effect size on average. By contrast, using the same sample size as that employed in Raffler, Posner, and Parkerson (2019) reduces the exaggeration ratio on the mortality estimand to where it should be, around one.
Finally, we can also address the analytic replication by Donato and Garcia Mosqueira (2016) . The replicators (D&M) noted that the eighteen community-based organizations who carried out the original “power to the people” (P2P) intervention were active in 64% of the treatment communities and 48% of the control communities. Donato and Garcia Mosqueira (2016) posit that prior presence of these organizations may be correlated with health outcomes, and therefore include in their analytic replication of the mortality and weight- for-age regressions both an indicator for CBO presence and the interaction of the intervention with CBO presence. The inclusion of these terms into the regression reduces the magnitude of the coefficients on the intervention indicator and thereby increases the p -values above the α = 0.1 threshold in some cases. The original authors (B&S) criticized the replicators’ decision to include CBO presence as a regressor, on the grounds that in any such study it is possible to find some unrelated variable whose inclusion will increase standard error of the treatment effect estimate.
In short, the original replicators make a set of contrasting claims about the true model of the world: B&S claim that CBO presence is unrelated to the outcome of interest ( Björkman Nyqvist and Svensson 2016 ), whereas D&M claim that CBO presence might indeed affect (or be otherwise correlated with) health outcomes. As we argued in the previous section, diagnosis of the properties of the answer strategy under these competing claims should determine which answer strategy is best justified.
Since we do not know whether the replicators would have conditioned on CBO presence and its interaction with the intervention if it had not been imbalanced, we modify the original design to include four different replicator strategies: the first ignores CBO presence as in the original study; the second includes CBO presence irrespective of imbalance; the third includes an indicator for CBO presence only if the CBO presence is significantly imbalanced among the 50 treatment and control clusters at the α = 0.05 level; and the last strategy includes terms for both CBO presence and an interaction of CBO presence with the treatment irrespective of imbalance. We consider how these strategies perform under a model in which CBO presence is unrelated to health outcomes, and another in which, as claimed by the replicators, CBO presence is highly correlated with health outcomes.
Including the interaction term is a strictly dominated strategy from the standpoint of reducing mean squared error: irrespective of whether CBO presence is correlated with health outcomes or imbalanced, the RMSE expected under this strategy is higher than under any other strategy. Thus, based on a criterion of “Home Ground Dominance” in favor of B&S, one would be justified in discounting the importance of the replicators’ observation that “including the interaction term leads to a further reduction in magnitude and significance” of the estimated treatment effect ( Donato and Garcia Mosqueira 2016 ,19).
Supposing now that there is no correlation between CBO presence and health outcomes, inclusion of the CBO indicator does increase RMSE ever so slightly in those instances where there is imbalance, and the standard errors are ever so slightly larger. On average, however, the strategies of conditioning on CBO presence regardless of balance and conditioning on CBO presence only if imbalanced perform about as well as a strategy of ignoring CBO presence when there is no underlying correlation. However, when there is a correlation between health outcomes and CBO presence, strategies that include CBO presence improve RMSE considerably, especially when there is imbalance. Thus, D&M could make a “Robustness to Alternative Models” claim in defense of their inclusion of the CBO dummy: including CBO presence does not greatly diminish inferential quality on average, even if there is no correlation in CBO presence and outcomes; and if there is such a correlation, including CBO presence in the regression specification strictly improves inferences. In sum, a diagnostic approach to replication clarifies that one should resist updating beliefs about the study based on the use of interaction terms, but that the inclusion of the CBO indicator only harms inferences in a very small subset of cases. In general, including it does not worsen inferences and in many cases can improve them. This approach helps to clarify which points of disagreement are most critical for how the scientific community should interpret and learn from replication efforts.
We began with two problems faced by empirical social science researchers: selecting high quality designs and communicating them to others. The preceding sections have demonstrated how the MIDA framework can address both challenges. Once designs are declared in MIDA terms, diagnosing their properties and improving them becomes straightforward. Because MIDA describes a grammar of research designs that applies across a very broad range of empirical research traditions, it enables efficient sharing of designs with others.
Designing high quality research is difficult and comes with many pitfalls, only a subset of which are ameliorated by the MIDA framework. Others we fail to address entirely and in some cases, we may even exacerbate them. We outline four concerns.
The first is the worry that evaluative weight could get placed on essentially meaningless diagnoses. Given that design declaration includes declarations of conjectures about the world it is possible to choose inputs so that a design passes any diagnostic test set for it. For instance, a simulation-based claim to unbiasedness that incorporates all features of a design is still only good with respect to the precise conditions of the simulation (in contrast, analytic results, when available, may extend over general classes of designs). Still worse, simulation parameters might be selected because of their properties. A power analysis, for instance, may be useless if implausible parameters are chosen to raise power artificially. While MIDA may encourage more honest declarations, there is nothing in the framework that enforces them. As ever, garbage-in, garbage-out.
Second, we see a risk that research may get evaluated on the basis of a narrow, but perhaps inappropriate set of diagnosands. Statistical power is often invoked as a key design feature —but there may be little value in knowing the power of a study that is biased away from its target of inference. The appropriateness of the diagnosand depends on the purposes of the study. As MIDA is silent on the question of a study’s purpose, it cannot guide researchers or critics to the appropriate set of diagnosands by which to evaluate a design. An advantage of the approach is that the choice of diagnosands gets highlighted and new diagnosands can be generated in response to substantive concerns.
Third, emphasis on the statistical properties of a design can obscure the substantive importance of a question being answered or other qualitative features of a design. A similar concern has been raised regarding the “identification revolution” where a focus on identification risks crowding out attention to the importance of questions being addressed ( Huber 2013 ). Our framework can help researchers determine whether a particular design answers a question well (or at all), and it also nudges them to make sure that their questions are defined clearly and independently of their answer strategies . It cannot, however, help researchers choose good questions.
Finally, we see a risk that the variation in the suitability of design declaration to different research strategies may be taken as evidence of the relative superiority of different types of research strategies. While we believe that the range of strategies that can be declared and diagnosed is wider than what one might at first think possible, there is no reason to believe that all strong designs can be declared either ex ante or ex post. An advantage of our framework, we hope, is that it can help clarify when a strategy can or cannot be completely declared. When a design cannot be declared, non-declarability is all the framework provides, and in such cases we urge caution in drawing conclusions about design quality.
We conclude on a practical note. In the end, we are asking that scholars add a step to their workflow. We want scholars to formally declare and diagnose their research designs both in order to learn about them and to improve them. Much of the work of declaring and diagnosing designs is already part of how social scientists conduct research: grant proposals, IRB protocols, preanalysis plans, and dissertation prospectuses contain design information and justifications for why the design is appropriate for the question. The lack of a common language to describe designs and their properties, however, seriously hampers the utility of these practices for assessing and improving design quality. We hope that the inclusion of a declaration and diagnosis step to the research process can help address this basic difficulty.
Supplementary Material
Acknowledgments.
Authors are listed in alphabetical order. This work was supported in part by a grant from the Laura and John Arnold Foundation and seed funding from EGAP—Evidence in Governance and Politics. Errors remain the responsibility of the authors. We thank the Associate Editor and three anonymous reviewers for generous feedback. In addition, we thank Peter Aronow, Julian Brückner, Adrian Duşa Adam Glynn, Donald Green, Justin Grimmer, Kolby Hansen, Erin Hartman, Alan Jacobs, Tom Leavitt, Winston Lin, Matto Mildenberger, Matthias Orlowski, Molly Roberts, Tara Slough, Gosha Syunyaev, Anna Wilke, Teppei Yamamoto, Erin York, Lauren Young, and Yang-Yang Zhou; seminar audiences at Columbia, Yale, MIT, WZB, NYU, Mannheim, Oslo, Princeton, Southern California Methods Workshop, and the European Field Experiments Summer School; as well as participants at the EPSA2016,APSA2016,EGAP18,BITSS2017,andSPSP2018meetings for helpful comments. We thank Clara Bicalho, Neal Fultz, Sisi Huang, Markus Konrad, Lily Medina, Pete Mohanty, Aaron Rudkin, Shikhar Singh, Luke Sonnet, and John Ternovski for theirmany contributions to thebroaderproject.Themethodsproposedin thispaperareimplemented inanaccompanyingopen-sourcesoftwarepackage,DeclareDesign(Blair et al. 2018). Replication files are available at the American Political Science Review Dataverse: https://doi.org/10.7910/DVN/XYT1VB .
In this appendix, we demonstrate how each diagnosand-relevant feature of a simple design can be defined in code, with an application in which the assignment procedure is known, as in an experimental or quasi-experimental design.
M (1) The population . Defines the population variables, including both observed and unobserved X . In the example below we define a function that returns a normally distributed variable of a given size. Critically, the declaration is not a declaration of a particular realization of data but of a data generating process. Researchers will typically have a sense of the distribution of covariates from previous work, and may even have an existing data set of the units that will be in the study with background characteristics. Researchers should assess the sensitivity of their diagnosands to different assumptions about P(U).
M (2) The structural equations, or potential outcomes function . The potential outcomes function defines conjectured potential outcomes given interventions Z and parents. In the example below the potential outcomes function maps from a treatment condition vector ( Z ) and background data u , generated by P(U) to a vector of outcomes. In this example the potential outcomes function satisfies a SUTVA condition-each unit’s outcome depends on its own condition only, though in general since Z is a vector, it need not.
In many cases, the potential outcomes function (or its features) is the very thing that the study sets out to learn, so it can seem odd to assume features of it. We suggest two approaches to developing potential outcomes functions that will yield useful information about the quality of designs. First, consider a null potential outcomes function in which the variables of interest are set to have no effect on the outcome whatsoever. Diagnosands such as bias can then be assessed relative to a true estimand of zero. This approach will not work for diagnosands like power or the Type-S rate. Second, set a series of potential outcomes functions that correspond to competing theories. This approach enables the researcher to judge whether the design yields answers that help adjudicate between the theories.
I Estimands . The estimand function creates a summary of potential outcomes. In principle, the estimand function can also take realizations of assignments as arguments, in order to calculate post-treatment estimands. Below, the estimand is the Average Treatment Effect, or the average difference between treated and untreated potential outcomes.
D (1) The sampling strategy . Defines the distribution over possible samples for which outcomes are measured, p S .
In the example below each unit generated by P(U) is sampled with 10% probability. Again sampling describes a sampling strategy and not an actual sample.
D (2) The treatment assignment strategy . Defines the strategy for assigning variables under the notional control of researchers. In this example each sampled unit is assigned to treatment independently with probability 0.5. In designs in which the sampling process or the assignment process are in the control of researchers, p z is known. In observational designs, researchers either know or assume p z based on substantive knowledge. We make explicit here an additional step in which the outcome for Y is revealed after Z is determined.
A The answer strategies are functions that use information from realized data and the design, but do not have access to the full schedule of potential outcomes. In the declaration we associate estimators with estimands and we record a set of summary statistics that are required to compute diagnostic statistics. In the example below, an estimator function takes data and returns an estimate of a treatment effect using the difference-in-means estimator, as well as a set of associated statistics, including the standard error, p-value, and the confidence interval.
We then declare the design by adding together the elements. Order matters. Since we have defined the estimand before the sampling step, our estimand is the Population Average Treatment Effect, not the Sample Average Treatment Effect. We have also included a declare_reveal() step between the assignment and estimation steps that reveals the outcome Y on the basis of the potential outcomes and a realized random assignment.
These six features represent the study. In order to assess the completeness of a declaration and to learn about the properties of the study, we also define functions for the diagnostic statistics, t(D, Y,f), anddiagnosands, θ ( D, Y , f, g). For simplicity, the two can be coded as a single function. For example, to calculate the bias of the design as a diagnosand is:
Diagnosing the design involves simulating the design many times, then calculating the value of the diagnosand from the resulting simulations.
The diagnosis returns an estimate of the diagnosands, along with other metadata associated with the simulations.
SUPPLEMENTARY MATERIAL
To view supplementary material for this article, please visit https://doi.org/10.1017/S0003055419000194 .
Replication materials can be found on Dataverse at: https://doi.org/10.7910/DVN/XYT1VB .
Though M is a causal model of the world, such a model can be used for both causal and non-causal questions of interest.
The distinction lies in whether the conditional probability is recorded through passive observation or active intervention to manipulate the probabilities of the conditioning distribution. For example, Pr( X 1 | X 2 = 1) might indicate the conditional probability that it is raining, given that Jack has his umbrella, whereas Pr( X 1 | do ( X 2 = 1))would indicate the probability of rain, given Jack is made to carry an umbrella.
See “Pre Analysis Plan Template” (60 features); World Bank Development Impact Blog (nine features).
Some exceptions are provided on page 4. Herron and Quinn (2016) , for example, conduct a formal investigation of the RMSE and bias exhibited by the alternative case selection strategies proposed in an influential piece by Seawright and Gerring (2008) .
We assessed tools listed in four reviews of the literature ( Greenand MacLeod 2016 ; Groemping 2016 ; Guo et al. 2013 ; Kreidler et al., 2013 ),in addition to the first thirty results from Google searches of the terms “statistical bias calculator,” “statistical power calculator,” and “sample size calculator.” We found no admissible tools using the term “statistical bias calculator.” Thirty of the 143 tools we identified were able to diagnose inferential properties of designs, such as their power. See Supplementary Materials Section 2 for further details on the tool survey.
For example, no design could account for: the posited correlation between block size and potential outcomes; the sampling strategy; the exact randomization procedure; the formal definition of the estimand as the population average treatment effect; or the use of inverse- probability weighting. The one tool (GLIMMPSE) that was able to account for the blocking strategy encountered an error and was unable to produce diagnostic statistics.
Aronow and Samii (2016) express a similar concern for models using regression with controls.
Schneider and Wagemann (2012 ,320–1) also note that there are not grounds to assume incommensurability, noting that “if set-theoretic, method-specific concepts.... can be translated into the potential outcomes framework, the communication between scholars from different research traditions will be facilitated.” See also Mahoney (2008) on the consistency of these conceptualizations.
An INUS condition is “an insufficient but non-redundant part of an unnecessary but sufficient condition” ( Mackie 1974 ).
Goertz and Mahoney (2012 , 59) also make the point that the difference is in practice, and is not fundamental: “Within quantitative research, it does not seem useful to group cases according to common causal configurations on the independent variables. Although one could do this, it is not a practice within the tradition.” (Emphasis added.)
In their paper on simulating clinical trials through Monte Carlo, Morris, White, and Crowther (2019) provide helpful analytic formula for deriving Monte Carlo standard errors for several diagnosands (“performance measures”). In the companion software, we adopt a non-parametric bootstrap approach that is able to calculate standard errors for any user-provided diagnosand.
This procedure depends on the researcher choosing a “good” diagnosand estimator. In nearly all cases, diagnosands will be features of the distribution of adiagnostic statistic that, given i.i.d. sampling, can be consistently estimated via plug-in estimation (for example taking sample means). Our simulation procedure, by construction, yields i.i.d. draws of the diagnostic statistic.
See also Collier, Brady, and Seawright (2004) , Mahoney (2012) , Bennett and Checkel (2014) , Fairfield (2013) .
For both methods, we use the “parsimonious” solution and not the “conservative” or“ intermediate” solutions that have been criticized in Baumgartner and Thiem (2017) , though our declaration could easily be modified to check the performance of these alternative solutions.
Analternative might be to imagine a marginal effect conditional on actual assignment: if x i is the observed treatment received by unit i , define, for small δ , τ ≡ E [ Y i ( x i ) − Y i ( x i − δ ) ] / δ .
For instance researchers might be interested in a “conditional expectation function,” or in locating a parameter vector that can render a model as good as possible—such as minimizing the Kullback-Leibler information criterion ( White 1982 ).
For a discussion of the distinctions between these different modes of replication, see Clemens (2017) .
Of course, we could define our estimand as the difference in average weights for any surviving children in either state of the world: E [Weight( Z = 1)|Alive( Z = 1) = 1] − E [Weight( Z = 0)|Alive( Z = 0) = 1].This estimand would lead to very aberrant conclusions. Suppose, for example, that only one child with a very healthy weight survived in the control and all children, with weights ranging from healthy to very unhealthy, survived in the treatment. Despite all those lives saved, this estimand would suggest that the treatment has a large negative impact on health.
Raffler, Posner, and Parkerson (2019) employ a factorial design which breaks down the original intervention into two subcomponents: interface meetings between the community and village health teams, on the one hand, and integration of report cards into the action plans of health centers, on the other. We augment the sample size here only by the number of clusters corresponding to the pure control and both-arm conditions, as the other conditions of the factorial were not included in the original design. Including those other 189 clusters would only strengthen the conclusions drawn.
Contributor Information
GRAEME BLAIR, University of California, Los Angeles.
JASPER COOPER, University of California, San Diego.
ALEXANDER COPPOCK, Yale University.
MACARTAN HUMPHREYS, WZB Berlin and Columbia University.
- Angrist Joshua D., and Pischke Jörn-Steffen. 2008. Mostly Harmless Econometrics: An Empiricist’s Companion. Princeton, NJ: Princeton University Press. [ Google Scholar ]
- Aronow Peter M., and Samii Cyrus. 2016. “Does Regression Produce Representative Estimates of Causal Effects?” American Journal of Political Science 60 (1): 250–67. [ Google Scholar ]
- Balke Alexander, and Pearl Judea. 1994. Counterfactual Probabilities: Computational Methods, Bounds and Applications. In Proceedings of the Tenth International Conference on Uncertainty in Artificial Intelligence Burlington, MA: Morgan Kaufmann Publishers, 46–54. [ Google Scholar ]
- Baumgartner Michael, and Thiem Alrik. 2017. “Often Trusted but Never (Properly) Tested: Evaluating Qualitative Comparative Analysis.” Sociological Methods & Research: 1–33. Published first online 3 May 2017. [ Google Scholar ]
- Beach Derek, and Brun Pedersen Rasmus. 2013. Process-Tracing Methods: Foundations and Guidelines. Ann Arbor, MI: University of Michigan Press. [ Google Scholar ]
- Bennett Andrew. 2015. Disciplining Our Conjectures: Systematizing Process Tracing with Bayesian Analysis In Process Tracing, eds. Bennett Andrew and Checkel Jeffrey T. Cambridge: Cambridge University Press, 276–98. [ Google Scholar ]
- Bennett Andrew, and Checkel Jeffrey T., eds. 2014. Process Tracing. Cambridge: Cambridge University Press. [ Google Scholar ]
- Björkman Martina, and Svensson Jakob. 2009. “Power to the People: Evidence from a Randomized Field Experiment of a Community- Based Monitoring Project in Uganda.” Quarterly Journal of Economics 124 (2): 735–69. [ Google Scholar ]
- Björkman Nyqvist Martina, and Svensson Jakob. 2016. “Comments on Donato and Mosqueira’s (2016) ‘additional Analyses’ of Björkman and Svensson (2009).” Unpublished research note.
- Blair Graeme, Cooper Jasper, Coppock Alexander, Humphreys Macartan, and Fultz Neal. 2018. “DeclareDesign.” Software package for R, available at http://declaredesign.org . [ Google Scholar ]
- Blei David M., Ng Andrew Y., and Jordan Michael I. 2003. “Latent Dirichlet Allocation.” Journal of Machine Learning Research 3: 993–1022. [ Google Scholar ]
- Brady Henry E., and Collier David. 2010. Rethinking Social Inquiry: Diverse Tools, Shared Standards. Lanham, MD: Rowman & Littlefield Publishers. [ Google Scholar ]
- Braumoeller Bear F. 2003. “Causal Complexity and the Study of Politics.” Political Analysis 11 (3): 209–33. [ Google Scholar ]
- Casey Katherine, Glennerster Rachel, and Miguel Edward. 2012. “Reshaping Institutions: Evidence on Aid Impacts Using a Pre-Analysis Plan.” Quarterly Journal of Economics 127 (4): 1755–812. [ Google Scholar ]
- Clemens Michael A. 2017. “The Meaning of Failed Replications: A Review and Proposal.” Journal of Economic Surveys 31 (1): 326–42. [ Google Scholar ]
- Cohen Jacob. 1977. Statistical Power Analysis for the Behavioral Sciences. New York, NY: Academic Press. [ Google Scholar ]
- Collier David. 2011. “Understanding Process Tracing.” PS: Political Science & Politics 44 (4): 823–30. [ Google Scholar ]
- Collier David. 2014. “Comment: QCA Should Set Aside the Algorithms.” Sociological Methodology 44 (1): 122–6. [ Google Scholar ]
- Collier David, Brady Henry E., and Seawright Jason. 2004. Sources of Leverage in Causal Inference: Toward an Alternative View of Methodology In Rethinking Social Inquiry: Diverse Tools, Shared Standards, eds. Collier David and Brady Henry E. Lanham, MD: Rowman and Littlefield, 229–66. [ Google Scholar ]
- Coppock Alexander. 2019. “Avoiding Post-Treatment Bias in Audit Experiments.” Journal of Experimental Political Science 6(1): 1–4. [ Google Scholar ]
- Cox David R. 1975. “A Note on Data-Splitting for the Evaluation of Significance Levels.” Biometrika 62 (2): 441–4. [ Google Scholar ]
- Dawid A.Philip. 2000. “Causal Inference without Counterfactuals.” Journal of the American Statistical Association 95 (450): 407–24. [ Google Scholar ]
- De la Cuesta Brandon, and Imai Kosuke. 2016. “Misunderstandings about the Regression Discontinuity Design in the Study of Close Elections.” Annual Review of Political Science 19: 375–96. [ Google Scholar ]
- Deaton Angus S. 2010. “Instruments, Randomization, and Learning about Development.” Journal of Economic Literature 48 (2): 424–55. [ Google Scholar ]
- Donato Katherine, and Mosqueira Adrian Garcia. 2016. “Power to the People? A Replication Study of a Community-Based Monitoring Programme in Uganda.” 3ie Replication Papers 11. [ Google Scholar ]
- Dunning Thad. 2012. Natural Experiments in the Social Sciences: A Design-Based Approach. Cambridge: Cambridge University Press. [ Google Scholar ]
- Duşa Adrian. 2018. QCA with R. A Comprehensive Resource. New York, NY: Springer. [ Google Scholar ]
- Duşa Adrian, and Thiem Alrik. 2015. “Enhancing the Minimization of Boolean and Multivalue Output Functions with e QMC.” Journal of Mathematical Sociology 39 (2): 92–108. [ Google Scholar ]
- Fairfield Tasha. 2013. “Going where the Money Is: Strategies for Taxing Economic Elites in Unequal Democracies.” World Development 47: 42–57. [ Google Scholar ]
- Fairfield Tasha, and Charman Andrew E. 2017. “Explicit Bayesian Analysis for Process Tracing: Guidelines, Opportunities, and Caveats.” Political Analysis 25 (3): 363–80. [ Google Scholar ]
- Findley Michael G., Jensen Nathan M., Malesky Edmund J., and Pepinsky Thomas B. 2016. “Can Results-Free Review Reduce Publication Bias? The Results and Implications of a Pilot Study.” Comparative Political Studies 49 (13): 1667–703. [ Google Scholar ]
- Geddes Barbara. 2003. Paradigms and Sand Castles: Theory Building and Research Design in Comparative Politics. Ann Arbor, MI: University of Michigan Press. [ Google Scholar ]
- Gelman Andrew, and Hill Jennifer. 2006. Data Analysis Using Regression and Multilevel/Hierarchical Models. Cambridge: Cambridge University Press. [ Google Scholar ]
- Gelman Andrew, and Carlin John. 2014. “Beyond Power Calculations Assessing Type S (Sign) and Type M (Magnitude) Errors.” Perspectives on Psychological Science 9 (6): 641–51. [ DOI ] [ PubMed ] [ Google Scholar ]
- Gerber Alan S., and Green Donald P. 2012. Field Experiments: Design, Analysis, and Interpretation. New York, NY: W.W. Norton. [ Google Scholar ]
- Goertz Gary, and Mahoney James. 2012. A Tale of Two Cultures: Qualitative and Quantitative Research in the Social Sciences. Princeton, NJ: Princeton University Press. [ Google Scholar ]
- Green Donald P., and Lin Winston. 2016. “Standard Operating Procedures: A Safety Net for Pre-analysis Plans.” PS: Political Science and Politics 49 (3): 495–9. [ Google Scholar ]
- Green Peter, and MacLeod Catriona J. 2016. “SIMR: An R Package for Power Analysis of Generalized Linear Mixed Models by Simulation.” Methods in Ecology and Evolution 7 (4): 493–8. [ Google Scholar ]
- Groemping Ulrike. 2016. “Design of Experiments (DoE) & Analysis of Experimental Data.” Last accessed May 11, 2017 https://cran.r-project.org/web/views/ExperimentalDesign.html .
- Guo Yi, Logan Henrietta L., Glueck Deborah H., and Muller Keith E. 2013. “Selecting a Sample Size for Studies with Repeated Measures.” BMC Medical Research Methodology 13 (1): 100. [ DOI ] [ PMC free article ] [ PubMed ] [ Google Scholar ]
- Halpern Joseph Y. 2000. “Axiomatizing Causal Reasoning.” Journal of Artificial Intelligence Research 12: 317–37. [ Google Scholar ]
- Haseman Joseph K. 1978. “Exact Sample Sizes for Use with the Fisher-Irwin Test for 2 × 2 Tables.” Biometrics 34 (1): 106–9. [ Google Scholar ]
- Heckman James J., Urzua Sergio, and Vytlacil Edward. 2006. “Understanding Instrumental Variables in Models with Essential Heterogeneity.” The Review of Economics and Statistics 88 (3): 389–432. [ Google Scholar ]
- Herron Michael C., and Quinn Kevin M. 2016. “A Careful Look at Modern Case Selection Methods.” Sociological Methods & Research 45 (3): 458–92. [ Google Scholar ]
- Huber John. 2013. “Is Theory Getting Lost in the ‘Identification Revolution’?” The Monkey Cage blog post. [ Google Scholar ]
- Hug Simon. 2013. “Qualitative Comparative Analysis: How Inductive Use and Measurement Error lead to Problematic Inference.” Political Analysis 21 (2): 252–65. [ Google Scholar ]
- Humphreys Macartan, and Jacobs Alan M. 2015. “Mixing Methods: A Bayesian Approach.” American Political Science Review 109 (4): 653–73. [ Google Scholar ]
- Imai Kosuke, King Gary, and Stuart Elizabeth A. 2008. “Misunderstandings between Experimentalists and Observationalists about Causal Inference.” Journal of the Royal Statistical Society: Series A 171 (2): 481–502. [ Google Scholar ]
- Imbens Guido W. 2010. “Better LATE Than Nothing: Some Comments on Deaton (2009) and Heckman and Urzua (2009).” Journal of Economic Literature 48 (2): 399–423. [ DOI ] [ PMC free article ] [ PubMed ] [ Google Scholar ]
- Imbens Guido W., and Rubin Donald B. 2015. Causal Inference in Statistics, Social, and Biomedical Sciences. Cambridge: Cambridge University Press. [ Google Scholar ]
- King Gary, Keohane Robert O., and Verba Sidney. 1994. Designing Social Inquiry: Scientific Inference in Qualitative Research. Princeton, NJ: Princeton University Press. [ Google Scholar ]
- Kreidler Sarah M., Muller Keith E., Grunwald Gary K., Ringham Brandy M., Coker-Dukowitz Zacchary T., Sakhadeo Uttara R., Barcón Anna E., and Glueck Deborah H. 2013. “GLIMMPSE: Online Power Computation for Linear Models with and without a Baseline Covariate.” Journal of Statistical Software 54 (10) 1–26. [ DOI ] [ PMC free article ] [ PubMed ] [ Google Scholar ]
- Lenth Russell V. 2001. “Some Practical Guidelines for Effective Sample Size Determination.” The American Statistician 55 (3): 187–93. [ Google Scholar ]
- Lieberman Evan S. 2005. “Nested Analysis as a Mixed-Method Strategy for Comparative Research.” American Political Science Review 99 (3): 435–52. [ Google Scholar ]
- Lohr Sharon. 2010. Sampling: Design and Analysis. Boston: Brooks Cole. [ Google Scholar ]
- Lucas Samuel R., and Szatrowski Alisa. 2014. “Qualitative Comparative Analysis in Critical Perspective.” Sociological Methodology 44 (1): 1–79. [ Google Scholar ]
- Mackie John Leslie. 1974. The Cement of the Universe: A Study of Causation. Oxford: Oxford University Press. [ Google Scholar ]
- Mahoney James. 2008. “Toward a Unified Theory of Causality.” Comparative Political Studies 41 (4–5): 412–36. [ Google Scholar ]
- Mahoney James. 2012. “The Logic of Process Tracing Tests in the Social Sciences.” Sociological Methods & Research 41 (4): 570–97. [ Google Scholar ]
- Morris Tim P., White Ian R., and Crowther Michael J. 2019. “Using Simulation Studies to Evaluate Statistical Methods.” Statistics in Medicine. [ DOI ] [ PMC free article ] [ PubMed ] [ Google Scholar ]
- Muller Keith E., and Peterson Bercedis L. 1984. “Practical Methods for Computing Power in Testing the Multivariate General Linear Hypothesis.” Computational Statistics & Data Analysis 2(2): 143–58. [ Google Scholar ]
- Muller Keith E., Lavange Lisa M., Ramey Sharon Landesman, and Ramey Craig T. 1992. “Power Calculations for General Linear Multivariate Models Including Repeated Measures Applications.” Journal of the American Statistical Association 87 (420): 1209–26. [ DOI ] [ PMC free article ] [ PubMed ] [ Google Scholar ]
- Nosek Brian A., Alter George, Banks George C., Borsboom Denny, Bowman Sara D., Breckler Steven J., Buck Stuart, et al. 2015. “Promoting an Open Research Culture: Author Guidelines for Journals Could Help to Promote Transparency, Openness, and Reproducibility.” Science 348 (6242): 1422. [ DOI ] [ PMC free article ] [ PubMed ] [ Google Scholar ]
- Pearl Judea. 2009. Causality. Cambridge: Cambridge University Press. [ Google Scholar ]
- Raffler Pia, Posner Daniel N., and Parkerson Doug. 2019. “The Weakness of Bottom-Up Accountability: Experimental Evidence from the Ugandan Health Sector.” Working Paper. [ Google Scholar ]
- Ragin Charles. 1987. The Comparative Method. Moving beyond Qualitative and Quantitative Strategies. Berkeley, CA: University of California Press. [ Google Scholar ]
- Rennie Drummond. 2004. “Trial Registration.” Journal of the American Medical Association: The Journal of the American Medical Association 292 (11): 1359–62.15355937 [ Google Scholar ]
- Rohlfing Ingo. 2018. “Power and False Negatives in Qualitative Comparative Analysis: Foundations, Simulation and Estimation for Empirical Studies.” Political Analysis 26 (1): 72–89. [ Google Scholar ]
- Rohlfing Ingo, and Schneider Carsten Q. 2018. “A Unifying Framework for Causal Analysis in Set-Theoretic Multimethod Research.” Sociological Methods & Research 47 (1): 37–63. [ Google Scholar ]
- Rosenbaum Paul R. 2002. Observational Studies. New York, NY: Springer. [ Google Scholar ]
- Rubin Donald B. 1984. “Bayesianly Justifiable and Relevant Frequency Calculations for the Applied Statistician.” Annals of Statistics 12 (4): 1151–72. [ Google Scholar ]
- Schneider Carsten Q., and Wagemann Claudius. 2012. Set-theoretic Methods for the Social Sciences: A Guide to Qualitative Comparative Analysis. Cambridge: Cambridge University Press. [ Google Scholar ]
- Seawright Jason, and Gerring John. 2008. “Case Selection Techniques in Case Study Research: A Menu of Qualitative and Quantitative Options.” Political Research Quarterly 61 (2): 294–308. [ Google Scholar ]
- Sekhon Jasjeet S., and Titiunik Rocio. 2016. “Understanding Regression Discontinuity Designs as Observational Studies.” Observational Studies 2: 173–81. [ Google Scholar ]
- Shadish William, Cook Thomas D., and Campbell Donald Thomas. 2002. Experimental and Quasi-Experimental Designs for Generalized Causal Inference. Boston, MA: Houghton Mifflin. [ Google Scholar ]
- Tanner Sean. 2014. “QCA Is of Questionable Value for Policy Research.” Policy and Society 33 (3): 287–98. [ Google Scholar ]
- Thiem Alrik, Baumgartner Michael, and Bol Damien. 2016. “Still Lost in Translation! A Correction of Three Misunderstandings between Configurational Comparativists and Regressional Analysts.” Comparative Political Studies 49 (6): 742–74. [ Google Scholar ]
- Van Evera Stephen. 1997. Guide to Methods for Students of Political Science. Ithaca, NY: Cornell University Press. [ Google Scholar ]
- White Halbert. 1982. “Maximum Likelihood Estimation of Mis- specified Models.” Econometrica: Journal of the Econometric Society 50 (1): 1–25. [ Google Scholar ]
- Yamamoto Teppei. 2012. “Understanding the Past: Statistical Analysis of Causal Attribution.” American Journal of Political Science 56 (1): 237–56. [ Google Scholar ]
- Zarin Deborah A., and Tse Tony. 2008. “Moving towards Transparency of Clinical Trials.” Science 319 (5868): 1340–2. [ DOI ] [ PMC free article ] [ PubMed ] [ Google Scholar ]
- Zhang Junni L., and Rubin Donald B. 2003. “Estimation of Causal Effects via Principal Stratification When Some Outcomes Are Truncated by ‘Death’.” Journal of Educational and Behavioral Statistics 28 (4): 353–68. [ Google Scholar ]
Associated Data
This section collects any data citations, data availability statements, or supplementary materials included in this article.
Supplementary Materials
- View on publisher site
- PDF (632.4 KB)
- Collections
Similar articles
Cited by other articles, links to ncbi databases.
- Download .nbib .nbib
- Format: AMA APA MLA NLM
Add to Collections
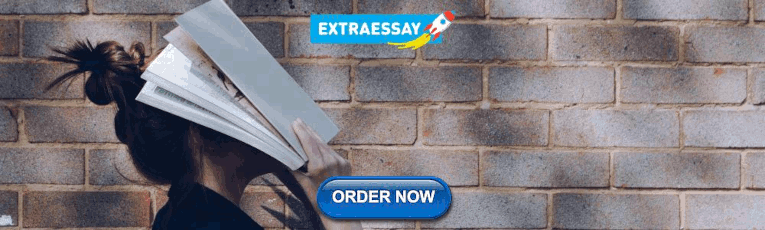
IMAGES
VIDEO
COMMENTS
A well-planned research design helps ensure that your methods match your research aims, that you collect high-quality data, and that you use the right kind of analysis to answer your questions, utilizing credible sources.
In no particular order, here are some common problems to avoid when designing a research study. Some are general issues you should think about as your organize your thoughts [e.g., developing a relevant problem to research] while other issues must be explicitly addressed in your paper [e.g., describing the limitations of your study].
Definition: Research design refers to the overall strategy or plan for conducting a research study. It outlines the methods and procedures that will be used to collect and analyze data, as well as the goals and objectives of the study.
A research design is a strategy for answering your research question using empirical data. Creating a research design means making decisions about: A well-planned research design helps ensure that your methods match your research aims and that you use the right kind of analysis for your data.
We first introduce a summary and key qualities of each approach. Then, using two common research contexts, we apply each approach to design a study, enabling comparisons among approaches and demonstrating the internal consistency within each approach.
Understood more as an broad approach to examining a research problem than a methodological design, philosophical analysis and argumentation is intended to challenge deeply embedded, often intractable, assumptions underpinning an area of study.
The essence of research design is to translate a research problem into data for analysis so as to provide relevant answers to research questions at a minimum cost.
We’ll explain the most common research design types for both qualitative and quantitative research projects, whether that is for a full dissertation or thesis, or a smaller research paper or article.
As empirical social scientists, we routinely face two research design problems. First, we need to select high-quality designs, given resource constraints. Second, we need to communicate those designs to readers and reviewers.
Sometimes the most basic fundamentals of research are the hardest to comprehend. Finding guidance on the initial steps of the research design process, namely identifying the basis of the study, is maddeningly difficult to find.