Have a language expert improve your writing
Run a free plagiarism check in 10 minutes, generate accurate citations for free.
- Knowledge Base
Methodology
- Inductive vs. Deductive Research Approach | Steps & Examples
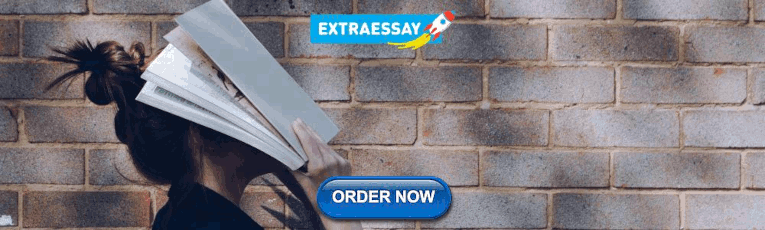
Inductive vs. Deductive Research Approach | Steps & Examples
Published on April 18, 2019 by Raimo Streefkerk . Revised on June 22, 2023.
The main difference between inductive and deductive reasoning is that inductive reasoning aims at developing a theory while deductive reasoning aims at testing an existing theory .
In other words, inductive reasoning moves from specific observations to broad generalizations . Deductive reasoning works the other way around.
Both approaches are used in various types of research , and it’s not uncommon to combine them in your work.
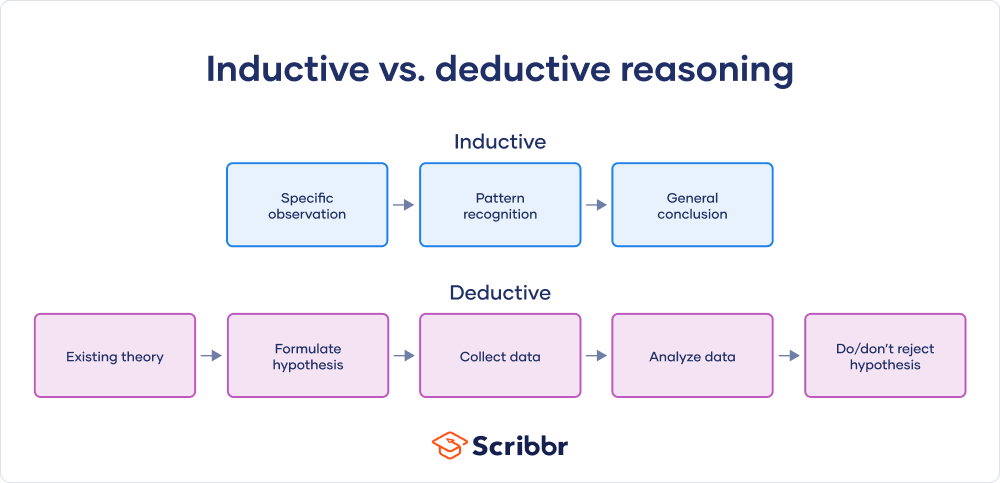
Table of contents
Inductive research approach, deductive research approach, combining inductive and deductive research, other interesting articles, frequently asked questions about inductive vs deductive reasoning.
When there is little to no existing literature on a topic, it is common to perform inductive research , because there is no theory to test. The inductive approach consists of three stages:
- A low-cost airline flight is delayed
- Dogs A and B have fleas
- Elephants depend on water to exist
- Another 20 flights from low-cost airlines are delayed
- All observed dogs have fleas
- All observed animals depend on water to exist
- Low cost airlines always have delays
- All dogs have fleas
- All biological life depends on water to exist
Limitations of an inductive approach
A conclusion drawn on the basis of an inductive method can never be fully proven. However, it can be invalidated.
Prevent plagiarism. Run a free check.
When conducting deductive research , you always start with a theory. This is usually the result of inductive research. Reasoning deductively means testing these theories. Remember that if there is no theory yet, you cannot conduct deductive research.
The deductive research approach consists of four stages:
- If passengers fly with a low cost airline, then they will always experience delays
- All pet dogs in my apartment building have fleas
- All land mammals depend on water to exist
- Collect flight data of low-cost airlines
- Test all dogs in the building for fleas
- Study all land mammal species to see if they depend on water
- 5 out of 100 flights of low-cost airlines are not delayed
- 10 out of 20 dogs didn’t have fleas
- All land mammal species depend on water
- 5 out of 100 flights of low-cost airlines are not delayed = reject hypothesis
- 10 out of 20 dogs didn’t have fleas = reject hypothesis
- All land mammal species depend on water = support hypothesis
Limitations of a deductive approach
The conclusions of deductive reasoning can only be true if all the premises set in the inductive study are true and the terms are clear.
- All dogs have fleas (premise)
- Benno is a dog (premise)
- Benno has fleas (conclusion)
Many scientists conducting a larger research project begin with an inductive study. This helps them develop a relevant research topic and construct a strong working theory. The inductive study is followed up with deductive research to confirm or invalidate the conclusion. This can help you formulate a more structured project, and better mitigate the risk of research bias creeping into your work.
Remember that both inductive and deductive approaches are at risk for research biases, particularly confirmation bias and cognitive bias , so it’s important to be aware while you conduct your research.
If you want to know more about statistics , methodology , or research bias , make sure to check out some of our other articles with explanations and examples.
- Chi square goodness of fit test
- Degrees of freedom
- Null hypothesis
- Discourse analysis
- Control groups
- Mixed methods research
- Non-probability sampling
- Quantitative research
- Inclusion and exclusion criteria
Research bias
- Rosenthal effect
- Implicit bias
- Cognitive bias
- Selection bias
- Negativity bias
- Status quo bias
Receive feedback on language, structure, and formatting
Professional editors proofread and edit your paper by focusing on:
- Academic style
- Vague sentences
- Style consistency
See an example
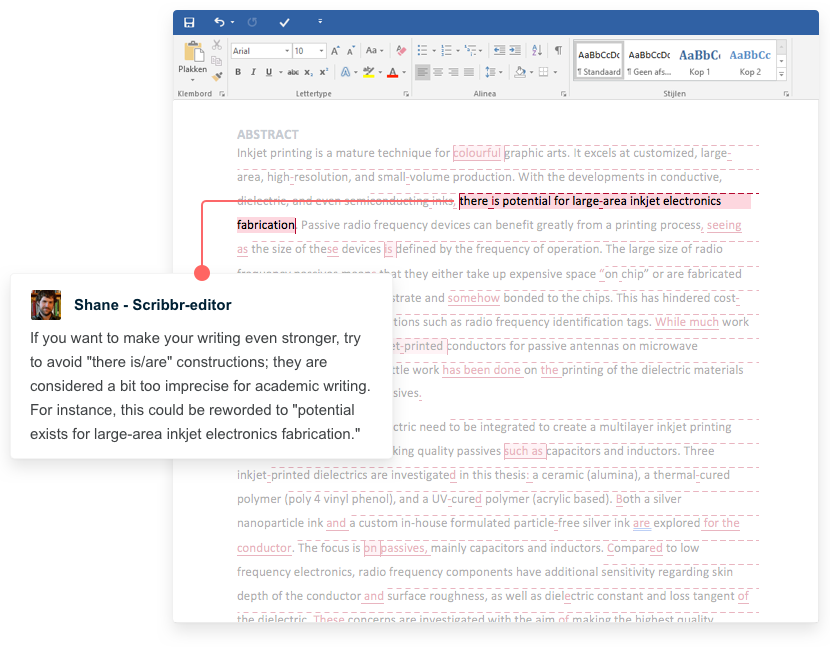
Inductive reasoning is a bottom-up approach, while deductive reasoning is top-down.
Inductive reasoning takes you from the specific to the general, while in deductive reasoning, you make inferences by going from general premises to specific conclusions.
Inductive reasoning is a method of drawing conclusions by going from the specific to the general. It’s usually contrasted with deductive reasoning, where you proceed from general information to specific conclusions.
Inductive reasoning is also called inductive logic or bottom-up reasoning.
Deductive reasoning is a logical approach where you progress from general ideas to specific conclusions. It’s often contrasted with inductive reasoning , where you start with specific observations and form general conclusions.
Deductive reasoning is also called deductive logic.
Exploratory research aims to explore the main aspects of an under-researched problem, while explanatory research aims to explain the causes and consequences of a well-defined problem.
Explanatory research is used to investigate how or why a phenomenon occurs. Therefore, this type of research is often one of the first stages in the research process , serving as a jumping-off point for future research.
Exploratory research is often used when the issue you’re studying is new or when the data collection process is challenging for some reason.
You can use exploratory research if you have a general idea or a specific question that you want to study but there is no preexisting knowledge or paradigm with which to study it.
A research project is an academic, scientific, or professional undertaking to answer a research question . Research projects can take many forms, such as qualitative or quantitative , descriptive , longitudinal , experimental , or correlational . What kind of research approach you choose will depend on your topic.
Cite this Scribbr article
If you want to cite this source, you can copy and paste the citation or click the “Cite this Scribbr article” button to automatically add the citation to our free Citation Generator.
Streefkerk, R. (2023, June 22). Inductive vs. Deductive Research Approach | Steps & Examples. Scribbr. Retrieved April 8, 2024, from https://www.scribbr.com/methodology/inductive-deductive-reasoning/
Is this article helpful?
Raimo Streefkerk
Other students also liked, qualitative vs. quantitative research | differences, examples & methods, explanatory research | definition, guide, & examples, exploratory research | definition, guide, & examples, unlimited academic ai-proofreading.
✔ Document error-free in 5minutes ✔ Unlimited document corrections ✔ Specialized in correcting academic texts
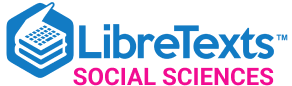
- school Campus Bookshelves
- menu_book Bookshelves
- perm_media Learning Objects
- login Login
- how_to_reg Request Instructor Account
- hub Instructor Commons
- Download Page (PDF)
- Download Full Book (PDF)
- Periodic Table
- Physics Constants
- Scientific Calculator
- Reference & Cite
- Tools expand_more
- Readability
selected template will load here
This action is not available.
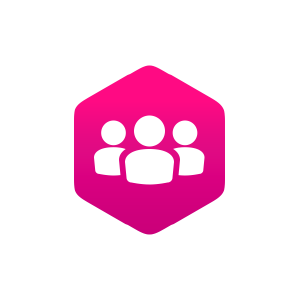
2.3: Inductive or Deductive? Two Different Approaches
- Last updated
- Save as PDF
- Page ID 12547

Learning Objectives
- Describe the inductive approach to research, and provide examples of inductive research.
- Describe the deductive approach to research, and provide examples of deductive research.
- Describe the ways that inductive and deductive approaches may be complementary.
Theories structure and inform sociological research. So, too, does research structure and inform theory. The reciprocal relationship between theory and research often becomes evident to students new to these topics when they consider the relationships between theory and research in inductive and deductive approaches to research. In both cases, theory is crucial. But the relationship between theory and research differs for each approach. Inductive and deductive approaches to research are quite different, but they can also be complementary. Let’s start by looking at each one and how they differ from one another. Then we’ll move on to thinking about how they complement one another.
Inductive Approaches and Some Examples
In an inductive approach to research, a researcher begins by collecting data that is relevant to his or her topic of interest. Once a substantial amount of data have been collected, the researcher will then take a breather from data collection, stepping back to get a bird’s eye view of her data. At this stage, the researcher looks for patterns in the data, working to develop a theory that could explain those patterns. Thus when researchers take an inductive approach, they start with a set of observations and then they move from those particular experiences to a more general set of propositions about those experiences. In other words, they move from data to theory, or from the specific to the general. Figure 2.5 outlines the steps involved with an inductive approach to research.
Figure 2.5 Inductive Research
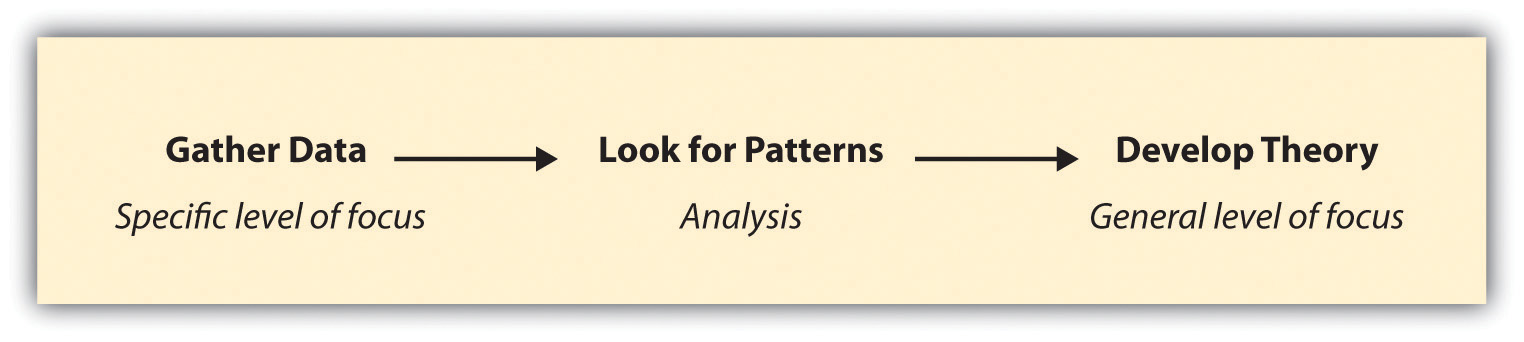
There are many good examples of inductive research, but we’ll look at just a few here. One fascinating recent study in which the researchers took an inductive approach was Katherine Allen, Christine Kaestle, and Abbie Goldberg’s study (2011)Allen, K. R., Kaestle, C. E., & Goldberg, A. E. (2011). More than just a punctuation mark: How boys and young men learn about menstruation. Journal of Family Issues, 32 , 129–156. of how boys and young men learn about menstruation. To understand this process, Allen and her colleagues analyzed the written narratives of 23 young men in which the men described how they learned about menstruation, what they thought of it when they first learned about it, and what they think of it now. By looking for patterns across all 23 men’s narratives, the researchers were able to develop a general theory of how boys and young men learn about this aspect of girls’ and women’s biology. They conclude that sisters play an important role in boys’ early understanding of menstruation, that menstruation makes boys feel somewhat separated from girls, and that as they enter young adulthood and form romantic relationships, young men develop more mature attitudes about menstruation.
In another inductive study, Kristin Ferguson and colleagues (Ferguson, Kim, & McCoy, 2011)Ferguson, K. M., Kim, M. A., & McCoy, S. (2011). Enhancing empowerment and leadership among homeless youth in agency and community settings: A grounded theory approach. Child and Adolescent Social Work Journal, 28 , 1–22. analyzed empirical data to better understand how best to meet the needs of young people who are homeless. The authors analyzed data from focus groups with 20 young people at a homeless shelter. From these data they developed a set of recommendations for those interested in applied interventions that serve homeless youth. The researchers also developed hypotheses for people who might wish to conduct further investigation of the topic. Though Ferguson and her colleagues did not test the hypotheses that they developed from their analysis, their study ends where most deductive investigations begin: with a set of testable hypotheses.
Deductive Approaches and Some Examples
Researchers taking a deductive approach take the steps described earlier for inductive research and reverse their order. They start with a social theory that they find compelling and then test its implications with data. That is, they move from a more general level to a more specific one. A deductive approach to research is the one that people typically associate with scientific investigation. The researcher studies what others have done, reads existing theories of whatever phenomenon he or she is studying, and then tests hypotheses that emerge from those theories. Figure 2.6 outlines the steps involved with a deductive approach to research.
Figure 2.6 Deductive Research
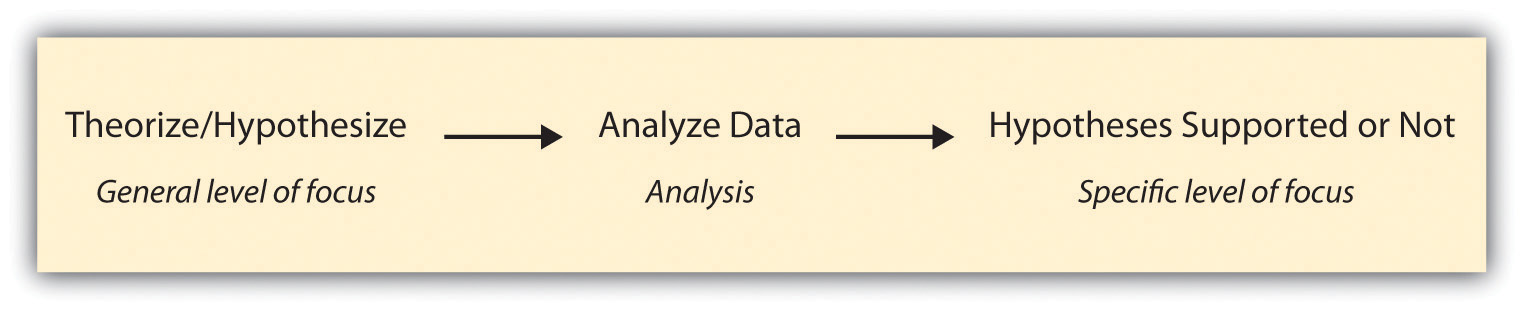
While not all researchers follow a deductive approach, as you have seen in the preceding discussion, many do, and there are a number of excellent recent examples of deductive research. We’ll take a look at a couple of those next.
In a study of US law enforcement responses to hate crimes, Ryan King and colleagues (King, Messner, & Baller, 2009)King, R. D., Messner, S. F., & Baller, R. D. (2009). Contemporary hate crimes, law enforcement, and the legacy of racial violence. American Sociological Review, 74 , 291–315.hypothesized that law enforcement’s response would be less vigorous in areas of the country that had a stronger history of racial violence. The authors developed their hypothesis from their reading of prior research and theories on the topic. Next, they tested the hypothesis by analyzing data on states’ lynching histories and hate crime responses. Overall, the authors found support for their hypothesis.
In another recent deductive study, Melissa Milkie and Catharine Warner (2011)Milkie, M. A., & Warner, C. H. (2011). Classroom learning environments and the mental health of first grade children. Journal of Health and Social Behavior, 52 , 4–22. studied the effects of different classroom environments on first graders’ mental health. Based on prior research and theory, Milkie and Warner hypothesized that negative classroom features, such as a lack of basic supplies and even heat, would be associated with emotional and behavioral problems in children. The researchers found support for their hypothesis, demonstrating that policymakers should probably be paying more attention to the mental health outcomes of children’s school experiences, just as they track academic outcomes (American Sociological Association, 2011).The American Sociological Association wrote a press release on Milkie and Warner’s findings: American Sociological Association. (2011). Study: Negative classroom environment adversely affects children’s mental health. Retrieved from asanet.org/press/Negative_Cla...tal_Health.cfm
Complementary Approaches?
While inductive and deductive approaches to research seem quite different, they can actually be rather complementary. In some cases, researchers will plan for their research to include multiple components, one inductive and the other deductive. In other cases, a researcher might begin a study with the plan to only conduct either inductive or deductive research, but then he or she discovers along the way that the other approach is needed to help illuminate findings. Here is an example of each such case.
In the case of my collaborative research on sexual harassment, we began the study knowing that we would like to take both a deductive and an inductive approach in our work. We therefore administered a quantitative survey, the responses to which we could analyze in order to test hypotheses, and also conducted qualitative interviews with a number of the survey participants. The survey data were well suited to a deductive approach; we could analyze those data to test hypotheses that were generated based on theories of harassment. The interview data were well suited to an inductive approach; we looked for patterns across the interviews and then tried to make sense of those patterns by theorizing about them.
For one paper (Uggen & Blackstone, 2004),Uggen, C., & Blackstone, A. (2004). Sexual harassment as a gendered expression of power. American Sociological Review, 69 , 64–92. we began with a prominent feminist theory of the sexual harassment of adult women and developed a set of hypotheses outlining how we expected the theory to apply in the case of younger women’s and men’s harassment experiences. We then tested our hypotheses by analyzing the survey data. In general, we found support for the theory that posited that the current gender system, in which heteronormative men wield the most power in the workplace, explained workplace sexual harassment—not just of adult women but of younger women and men as well. In a more recent paper (Blackstone, Houle, & Uggen, 2006),Blackstone, A., Houle, J., & Uggen, C. “At the time I thought it was great”: Age, experience, and workers’ perceptions of sexual harassment. Presented at the 2006 meetings of the American Sociological Association. Currently under review. we did not hypothesize about what we might find but instead inductively analyzed the interview data, looking for patterns that might tell us something about how or whether workers’ perceptions of harassment change as they age and gain workplace experience. From this analysis, we determined that workers’ perceptions of harassment did indeed shift as they gained experience and that their later definitions of harassment were more stringent than those they held during adolescence. Overall, our desire to understand young workers’ harassment experiences fully—in terms of their objective workplace experiences, their perceptions of those experiences, and their stories of their experiences—led us to adopt both deductive and inductive approaches in the work.
Researchers may not always set out to employ both approaches in their work but sometimes find that their use of one approach leads them to the other. One such example is described eloquently in Russell Schutt’s Investigating the Social World (2006).Schutt, R. K. (2006). Investigating the social world: The process and practice of research . Thousand Oaks, CA: Pine Forge Press. As Schutt describes, researchers Lawrence Sherman and Richard Berk (1984)Sherman, L. W., & Berk, R. A. (1984). The specific deterrent effects of arrest for domestic assault. American Sociological Review, 49 , 261–272. conducted an experiment to test two competing theories of the effects of punishment on deterring deviance (in this case, domestic violence). Specifically, Sherman and Berk hypothesized that deterrence theory would provide a better explanation of the effects of arresting accused batterers than labeling theory . Deterrence theory predicts that arresting an accused spouse batterer will reduce future incidents of violence. Conversely, labeling theory predicts that arresting accused spouse batterers will increase future incidents. Figure 2.7 summarizes the two competing theories and the predictions that Sherman and Berk set out to test.
Figure 2.7 Predicting the Effects of Arrest on Future Spouse Battery
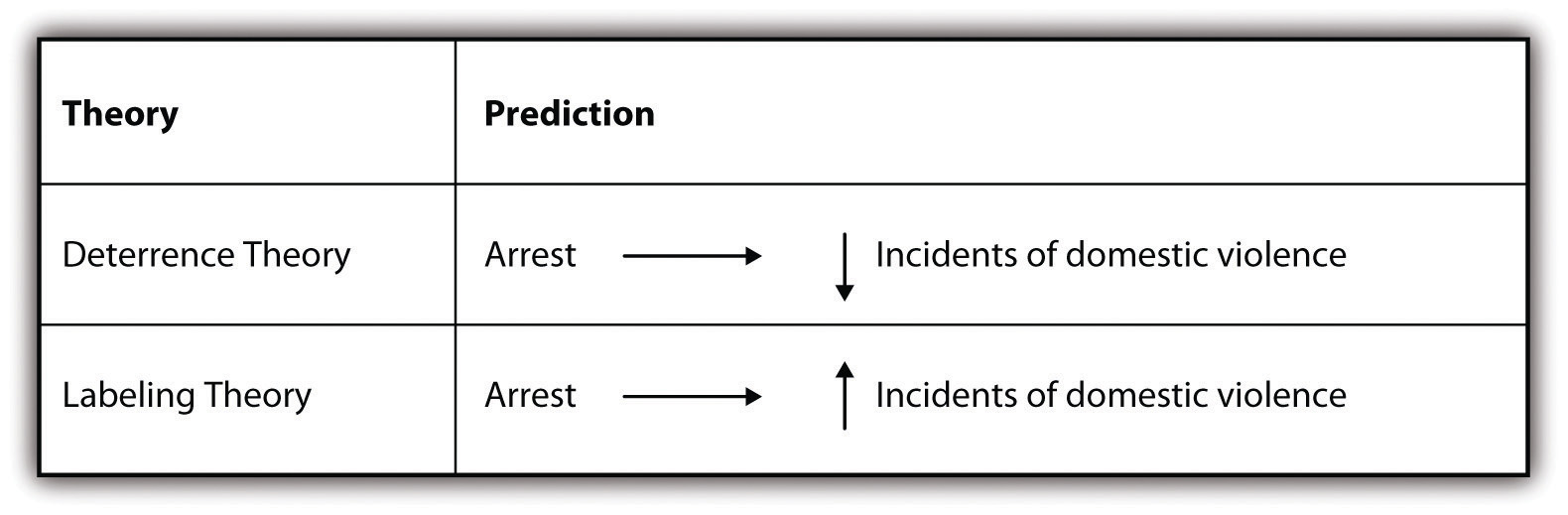
Sherman and Berk found, after conducting an experiment with the help of local police in one city, that arrest did in fact deter future incidents of violence, thus supporting their hypothesis that deterrence theory would better predict the effect of arrest. After conducting this research, they and other researchers went on to conduct similar experimentsThe researchers did what’s called replication. We’ll learn more about replication in Chapter 3. in six additional cities (Berk, Campbell, Klap, & Western, 1992; Pate & Hamilton, 1992; Sherman & Smith, 1992).Berk, R., Campbell, A., Klap, R., & Western, B. (1992). The deterrent effect of arrest in incidents of domestic violence: A Bayesian analysis of four field experiments. American Sociological Review, 57 , 698–708; Pate, A., & Hamilton, E. (1992). Formal and informal deterrents to domestic violence: The Dade county spouse assault experiment. American Sociological Review, 57 , 691–697; Sherman, L., & Smith, D. (1992). Crime, punishment, and stake in conformity: Legal and informal control of domestic violence. American Sociological Review, 57 , 680–690. Results from these follow-up studies were mixed. In some cases, arrest deterred future incidents of violence. In other cases, it did not. This left the researchers with new data that they needed to explain. The researchers therefore took an inductive approach in an effort to make sense of their latest empirical observations. The new studies revealed that arrest seemed to have a deterrent effect for those who were married and employed but that it led to increased offenses for those who were unmarried and unemployed. Researchers thus turned to control theory, which predicts that having some stake in conformity through the social ties provided by marriage and employment, as the better explanation.
Figure 2.8 Predicting the Effects of Arrest on Future Spouse Battery: A New Theory
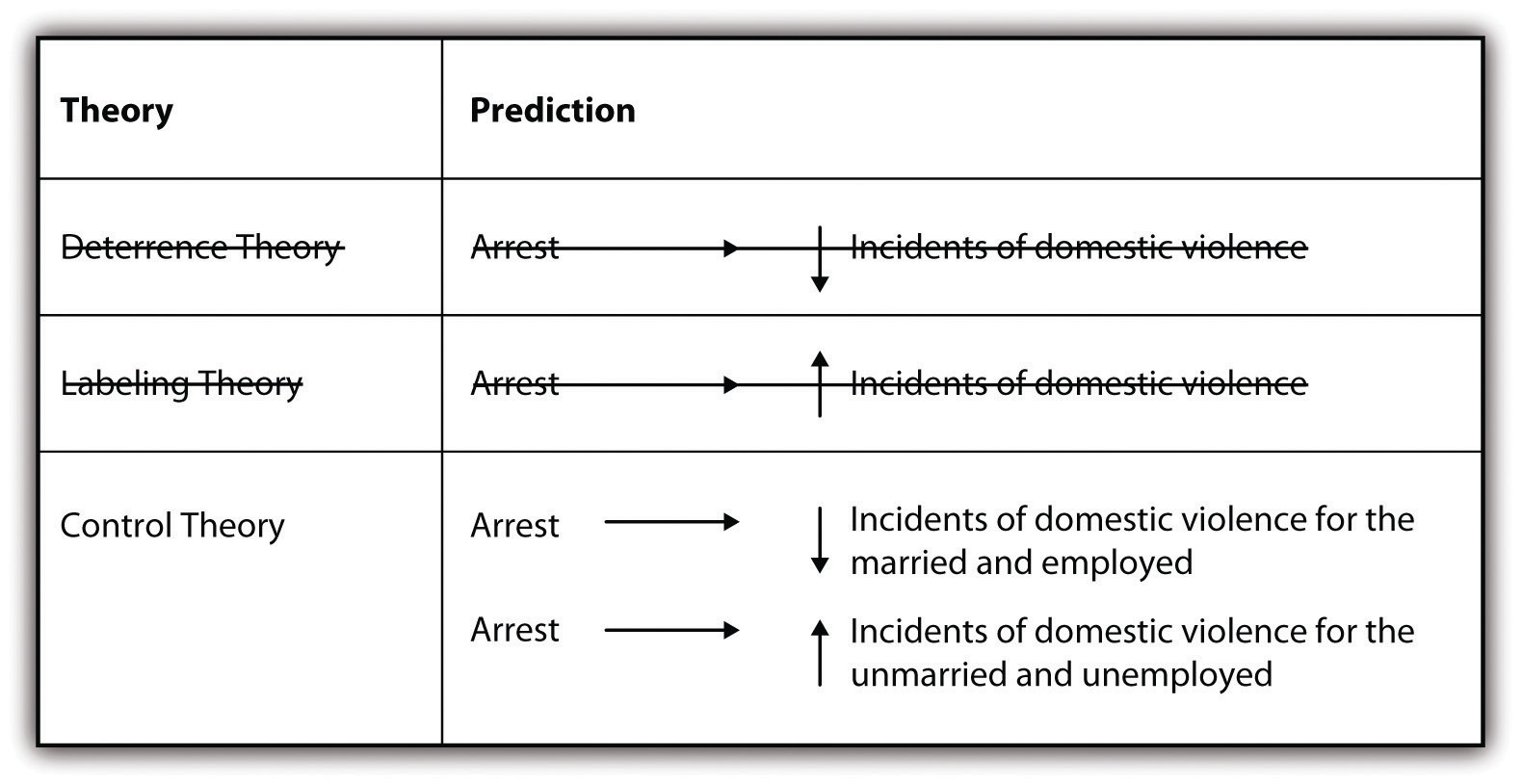
What the Sherman and Berk research, along with the follow-up studies, shows us is that we might start with a deductive approach to research, but then, if confronted by new data that we must make sense of, we may move to an inductive approach. Russell Schutt depicts this process quite nicely in his text, and I’ve adapted his depiction here, in Figure 2.9.
KEY TAKEAWAYS
- The inductive approach involves beginning with a set of empirical observations, seeking patterns in those observations, and then theorizing about those patterns.
- The deductive approach involves beginning with a theory, developing hypotheses from that theory, and then collecting and analyzing data to test those hypotheses.
- Inductive and deductive approaches to research can be employed together for a more complete understanding of the topic that a researcher is studying.
- Though researchers don’t always set out to use both inductive and deductive strategies in their work, they sometimes find that new questions arise in the course of an investigation that can best be answered by employing both approaches.
Monty Python and Holy Grail :
(click to see video)
Do the townspeople take an inductive or deductive approach to determine whether the woman in question is a witch? What are some of the different sources of knowledge (recall Chapter 1) they rely on?
- Think about how you could approach a study of the relationship between gender and driving over the speed limit. How could you learn about this relationship using an inductive approach? What would a study of the same relationship look like if examined using a deductive approach? Try the same thing with any topic of your choice. How might you study the topic inductively? Deductively?
Have a language expert improve your writing
Run a free plagiarism check in 10 minutes, automatically generate references for free.
- Knowledge Base
- Methodology
- Inductive vs Deductive Research Approach (with Examples)
Inductive vs Deductive Reasoning | Difference & Examples
Published on 4 May 2022 by Raimo Streefkerk . Revised on 10 October 2022.
The main difference between inductive and deductive reasoning is that inductive reasoning aims at developing a theory while deductive reasoning aims at testing an existing theory .
Inductive reasoning moves from specific observations to broad generalisations , and deductive reasoning the other way around.
Both approaches are used in various types of research , and it’s not uncommon to combine them in one large study.
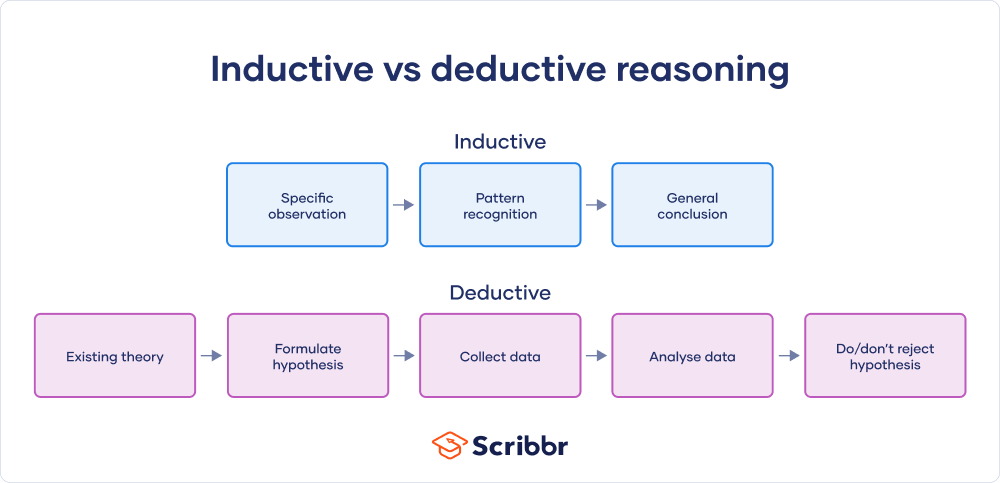
Table of contents
Inductive research approach, deductive research approach, combining inductive and deductive research, frequently asked questions about inductive vs deductive reasoning.
When there is little to no existing literature on a topic, it is common to perform inductive research because there is no theory to test. The inductive approach consists of three stages:
- A low-cost airline flight is delayed
- Dogs A and B have fleas
- Elephants depend on water to exist
- Another 20 flights from low-cost airlines are delayed
- All observed dogs have fleas
- All observed animals depend on water to exist
- Low-cost airlines always have delays
- All dogs have fleas
- All biological life depends on water to exist
Limitations of an inductive approach
A conclusion drawn on the basis of an inductive method can never be proven, but it can be invalidated.
Example You observe 1,000 flights from low-cost airlines. All of them experience a delay, which is in line with your theory. However, you can never prove that flight 1,001 will also be delayed. Still, the larger your dataset, the more reliable the conclusion.
Prevent plagiarism, run a free check.
When conducting deductive research , you always start with a theory (the result of inductive research). Reasoning deductively means testing these theories. If there is no theory yet, you cannot conduct deductive research.
The deductive research approach consists of four stages:
- If passengers fly with a low-cost airline, then they will always experience delays
- All pet dogs in my apartment building have fleas
- All land mammals depend on water to exist
- Collect flight data of low-cost airlines
- Test all dogs in the building for fleas
- Study all land mammal species to see if they depend on water
- 5 out of 100 flights of low-cost airlines are not delayed
- 10 out of 20 dogs didn’t have fleas
- All land mammal species depend on water
- 5 out of 100 flights of low-cost airlines are not delayed = reject hypothesis
- 10 out of 20 dogs didn’t have fleas = reject hypothesis
- All land mammal species depend on water = support hypothesis
Limitations of a deductive approach
The conclusions of deductive reasoning can only be true if all the premises set in the inductive study are true and the terms are clear.
- All dogs have fleas (premise)
- Benno is a dog (premise)
- Benno has fleas (conclusion)
Many scientists conducting a larger research project begin with an inductive study (developing a theory). The inductive study is followed up with deductive research to confirm or invalidate the conclusion.
In the examples above, the conclusion (theory) of the inductive study is also used as a starting point for the deductive study.
Inductive reasoning is a bottom-up approach, while deductive reasoning is top-down.
Inductive reasoning takes you from the specific to the general, while in deductive reasoning, you make inferences by going from general premises to specific conclusions.
Inductive reasoning is a method of drawing conclusions by going from the specific to the general. It’s usually contrasted with deductive reasoning, where you proceed from general information to specific conclusions.
Inductive reasoning is also called inductive logic or bottom-up reasoning.
Deductive reasoning is a logical approach where you progress from general ideas to specific conclusions. It’s often contrasted with inductive reasoning , where you start with specific observations and form general conclusions.
Deductive reasoning is also called deductive logic.
Cite this Scribbr article
If you want to cite this source, you can copy and paste the citation or click the ‘Cite this Scribbr article’ button to automatically add the citation to our free Reference Generator.
Streefkerk, R. (2022, October 10). Inductive vs Deductive Reasoning | Difference & Examples. Scribbr. Retrieved 8 April 2024, from https://www.scribbr.co.uk/research-methods/inductive-vs-deductive-reasoning/
Is this article helpful?
Raimo Streefkerk
Other students also liked, inductive reasoning | types, examples, explanation, what is deductive reasoning | explanation & examples, a quick guide to experimental design | 5 steps & examples.
- Skip to main content
- Skip to primary sidebar
- Skip to footer
- QuestionPro

- Solutions Industries Gaming Automotive Sports and events Education Government Travel & Hospitality Financial Services Healthcare Cannabis Technology Use Case NPS+ Communities Audience Contactless surveys Mobile LivePolls Member Experience GDPR Positive People Science 360 Feedback Surveys
- Resources Blog eBooks Survey Templates Case Studies Training Help center

Home Market Research
Inductive vs Deductive Research: Difference of Approaches
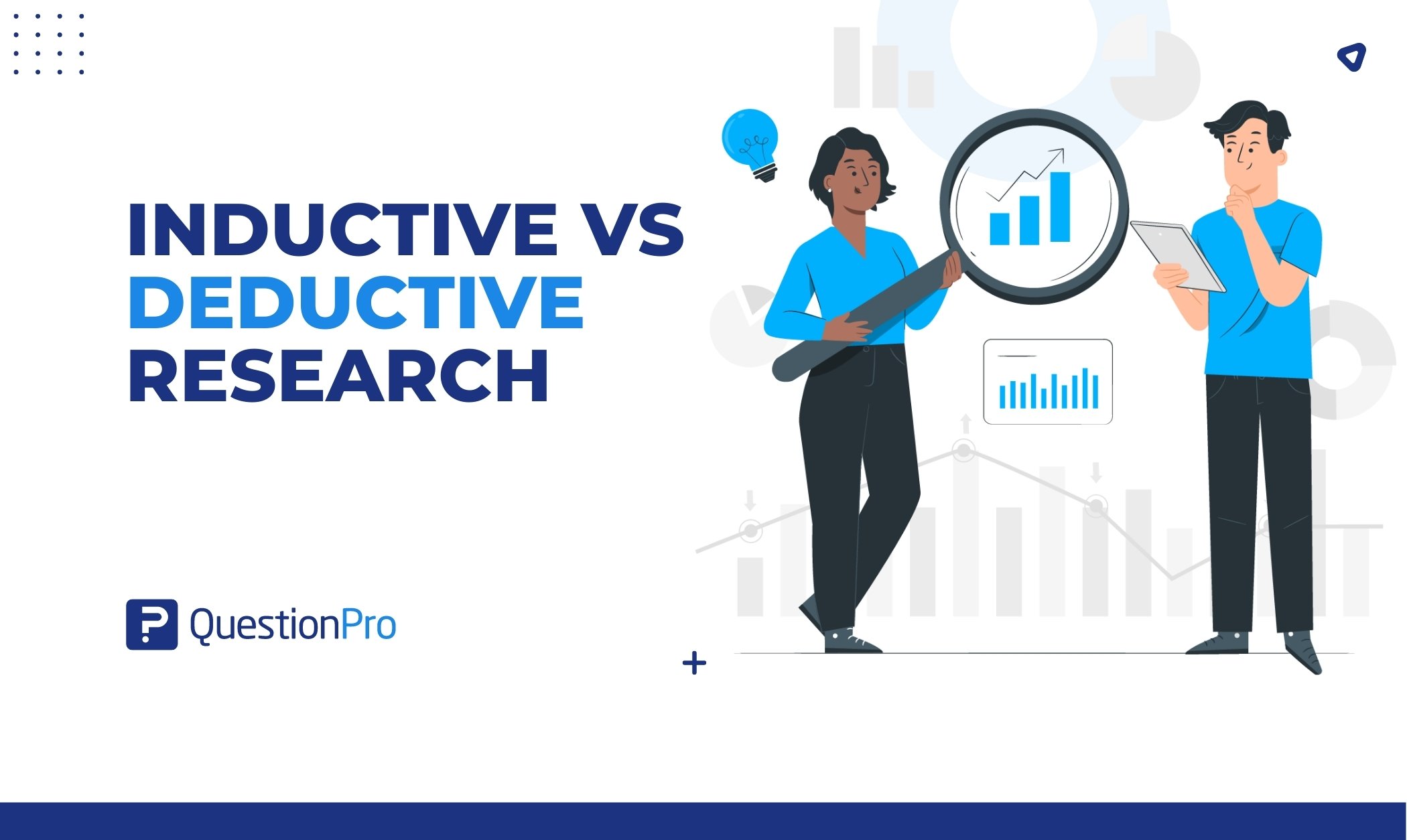
The terms “inductive” and “deductive” are often used in logic, reasoning, and science. Scientists use both inductive and deductive research methods as part of the scientific method.
Famous fictional detectives like Sherlock Holmes are often associated with deduction, even though that’s not always what Holmes does (more on that later). Some writing classes include both inductive and deductive essays.
But what’s the difference between inductive vs deductive research? The difference often lies in whether the argument proceeds from the general to the specific or the specific to the general.
Both methods are used in different types of research, and it’s not unusual to use both in one project. In this article, we’ll describe each in simple yet defined terms.
Content Index:
What is inductive research, stages of inductive research process, what is deductive research, stages of deductive research process, difference between inductive vs deductive research.
Inductive research is a method in which the researcher collects and analyzes data to develop theories, concepts, or hypotheses based on patterns and observations seen in the data.
It uses a “bottom-up” method in which the researcher starts with specific observations and then moves on to more general theories or ideas. Inductive research is often used in exploratory studies or when not much research has been done on a topic before.
LEARN ABOUT: Research Process Steps
The three steps of the inductive research process are:
- Observation:
The first step of inductive research is to make detailed observations of the studied phenomenon. This can be done in many ways, such as through surveys, interviews, or direct observation.
- Pattern Recognition:
The next step is to look at the data in detail once the data has been collected. This means looking at the data for patterns, themes, and relationships. The goal is to find insights and trends that can be used to make the first categories and ideas.
- Theory Development:
At this stage, the researcher will start to create initial categories or concepts based on the patterns and themes from the data analysis. This means putting the data into groups based on their similarities and differences to make a framework for understanding the thing being studied.
LEARN ABOUT: Data Management Framework
These three steps are often repeated in a cycle, so the researcher can improve their analysis and understand the phenomenon over time. Inductive research aims to develop new theories and ideas based on the data rather than testing existing theories, as in deductive research.
Deductive research is a type of research in which the researcher starts with a theory, hypothesis, or generalization and then tests it through observations and data collection.
It uses a top-down method in which the researcher starts with a general idea and then tests it through specific observations. Deductive research is often used to confirm a theory or test a well-known hypothesis.
The five steps in the process of deductive research are:
- Formulation of a hypothesis:
The first step in deductive research is to develop a hypothesis and guess how the variables are related. Most of the time, the hypothesis is built on theories or research that have already been done.
- Design of a research study:
The next step is designing a research study to test the hypothesis. This means choosing a research method, figuring out what needs to be measured, and figuring out how to collect and look at the data.
- Collecting data:
Once the research design is set, different methods, such as surveys, experiments, or observational studies, are used to gather data. Usually, a standard protocol is used to collect the data to ensure it is correct and consistent.
- Analysis of data:
In this step, the collected data are looked at to see if they support or disprove the hypothesis. The goal is to see if the data supports or refutes the hypothesis. You need to use statistical methods to find patterns and links between the variables to do this.
- Drawing conclusions:
The last step is drawing conclusions from the analysis of the data. If the hypothesis is supported, it can be used to make generalizations about the population being studied. If the hypothesis is wrong, the researcher may need to develop a new one and start the process again.
The five steps of deductive research are repeated, and researchers may need to return to earlier steps if they find new information or new ways of looking at things. In contrast to inductive research, deductive research aims to test theories or hypotheses that have already been made.
The main differences between inductive and deductive research are how the research is done, the goal, and how the data is analyzed. Inductive research is exploratory, flexible, and based on qualitative observation analysis. Deductive research, on the other hand, is about proving something and is structured and based on quantitative analysis .
Here are the main differences between inductive vs deductive research in more detail:
LEARN ABOUT: Theoretical Research
Inductive research and deductive research are two different types of research with different starting points, goals, methods, and ways of looking at the data.
Inductive research uses specific observations and patterns to come up with new theories. On the other hand, deductive research starts with a theory or hypothesis and tests it through observations.
Both approaches have advantages as well as disadvantages and can be used in different types of research depending on the question and goals.
QuestionPro is a responsive online platform for surveys and research that can be used for both inductive and deductive research. It has many tools and features to help you collect and analyze data, such as customizable survey templates, advanced survey logic, and real-time reporting.
With QuestionPro, researchers can do surveys, send them out, analyze the results, and draw conclusions that help them make decisions and learn more about their fields.
The platform has advanced data analysis and reporting tools that can be used with both qualitative and quantitative methods of data analysis.
Whether researchers do inductive or deductive research, QuestionPro can help them design, run, and analyze their projects completely and powerfully. So sign up now for a free trial!
LEARN MORE FREE TRIAL
MORE LIKE THIS
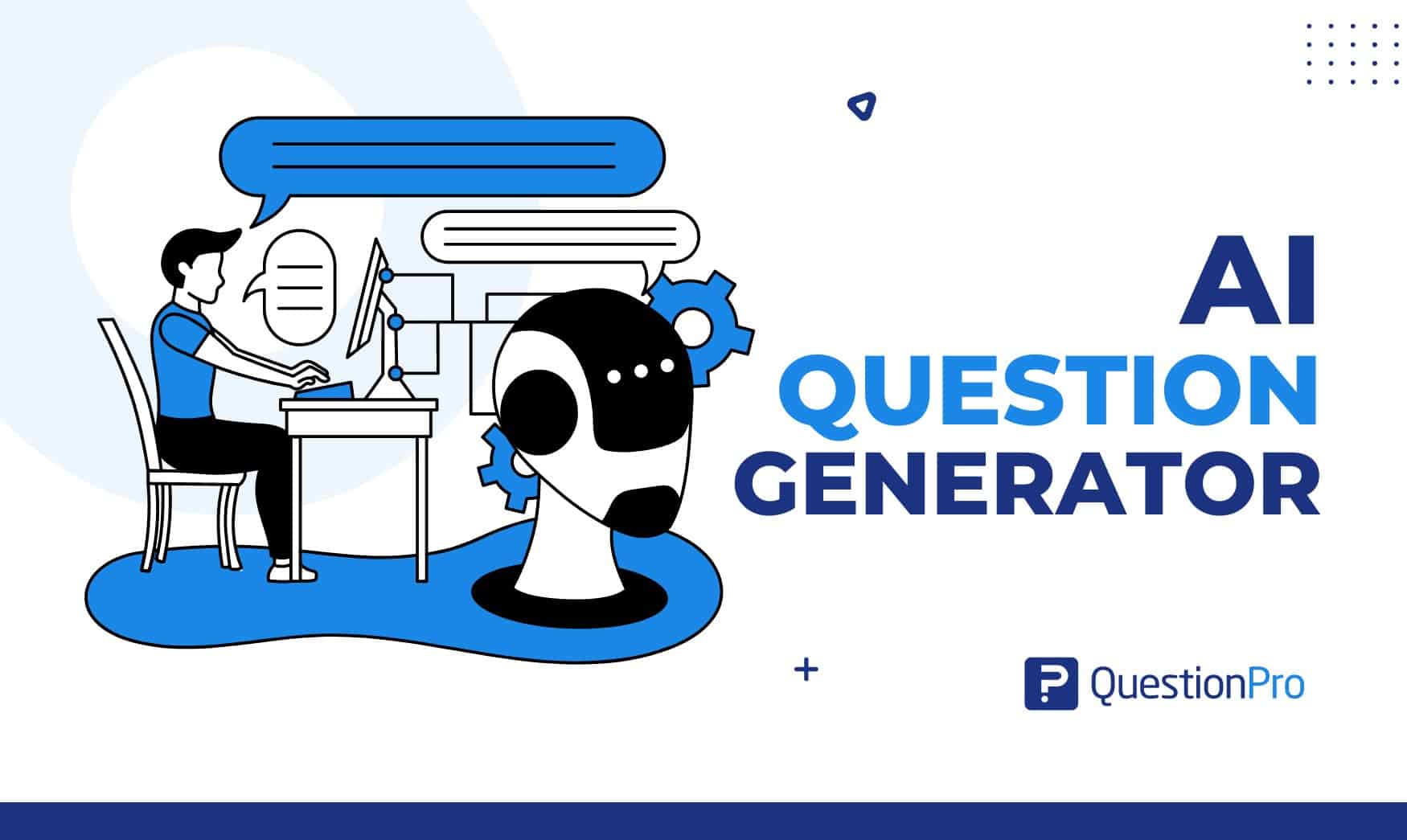
AI Question Generator: Create Easy + Accurate Tests and Surveys
Apr 6, 2024
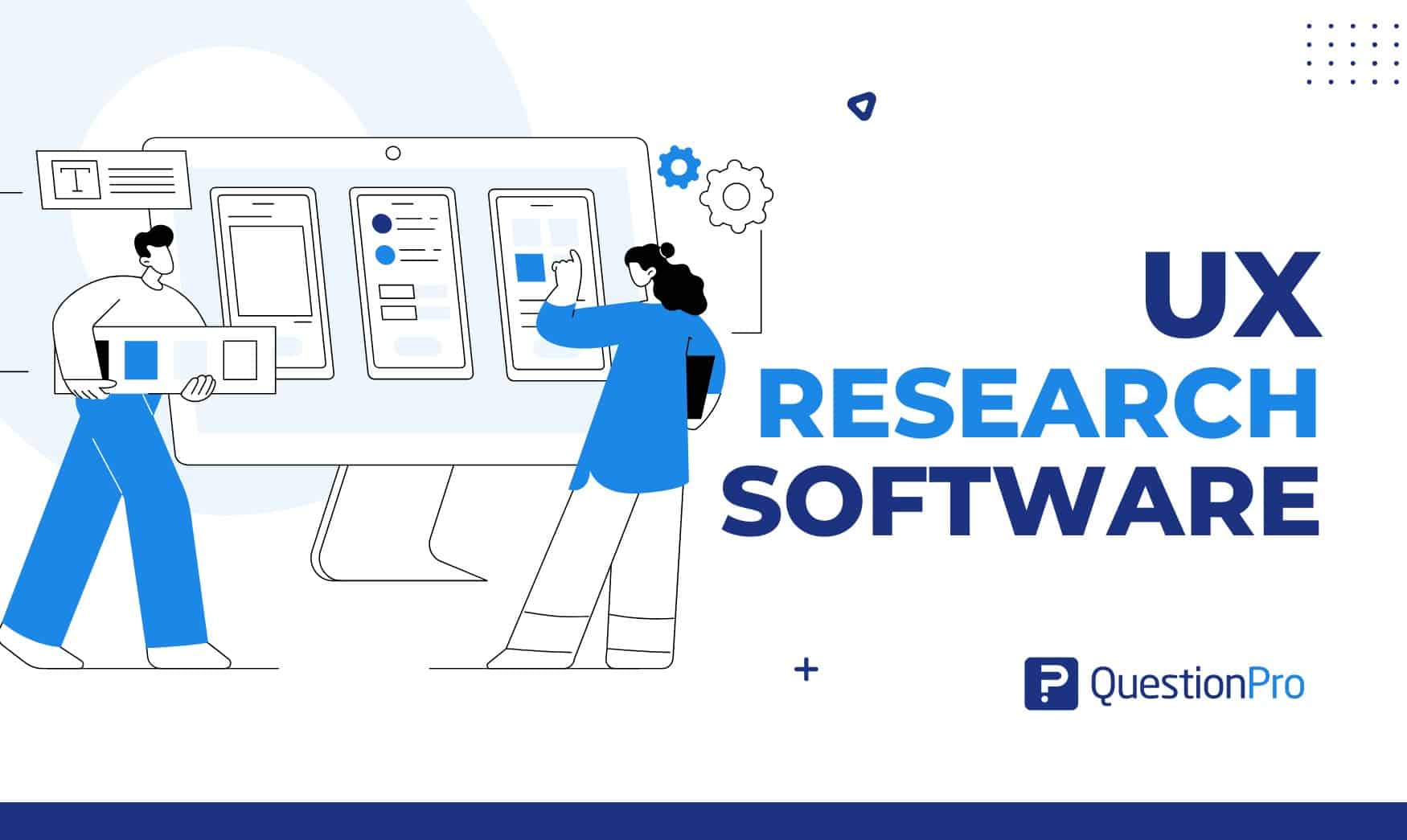
Top 17 UX Research Software for UX Design in 2024
Apr 5, 2024
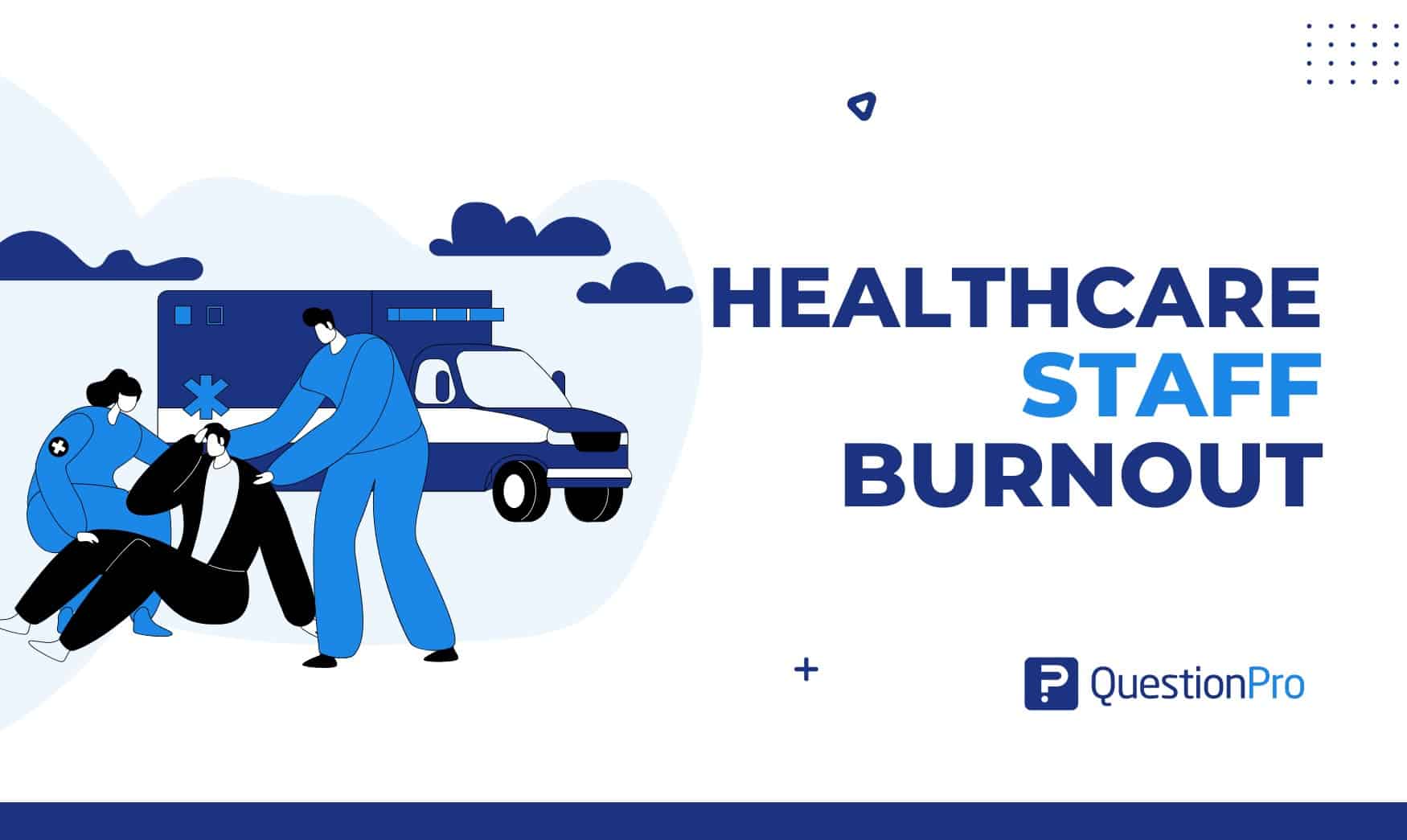
Healthcare Staff Burnout: What it Is + How To Manage It
Apr 4, 2024
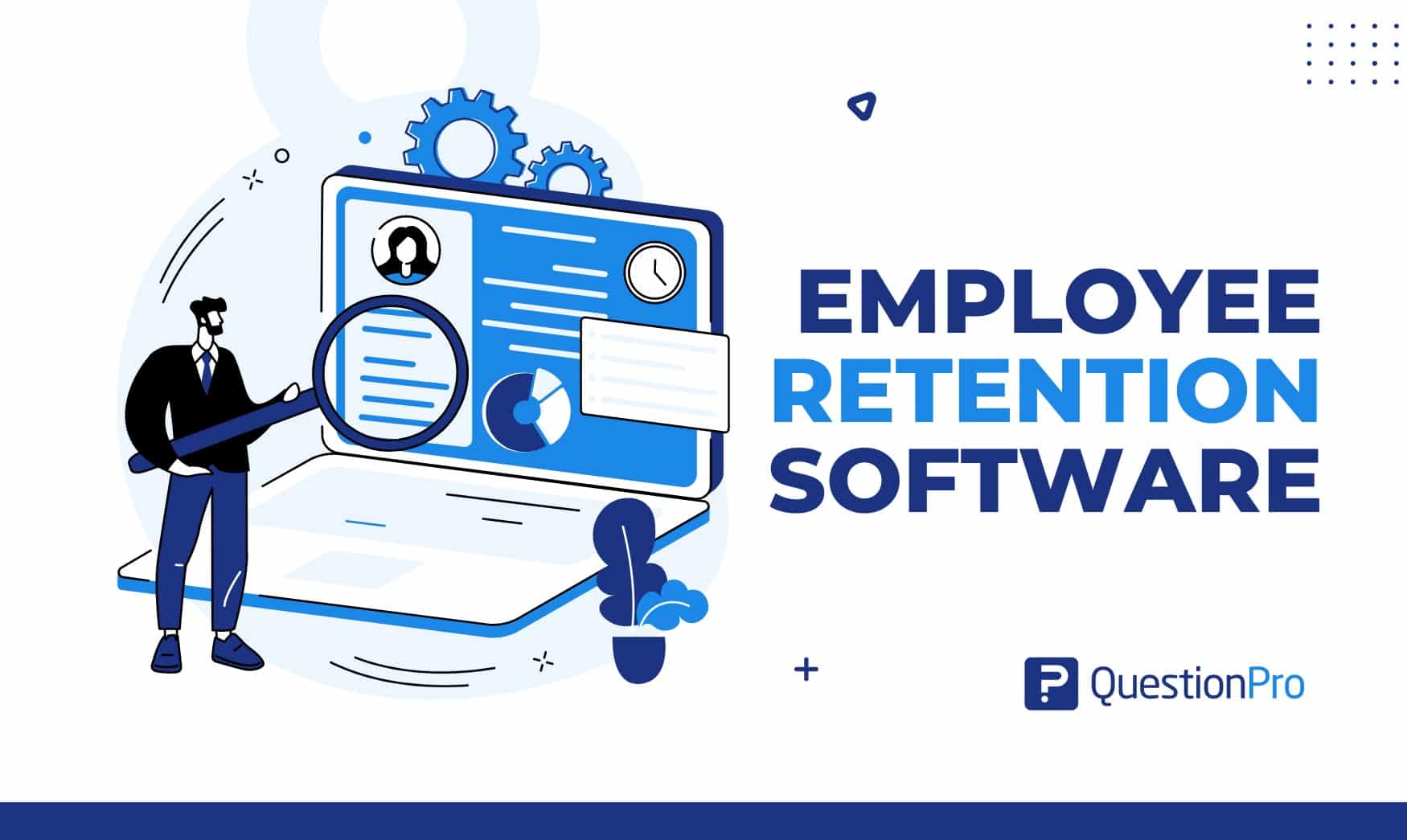
Top 15 Employee Retention Software in 2024
Other categories.
- Academic Research
- Artificial Intelligence
- Assessments
- Brand Awareness
- Case Studies
- Communities
- Consumer Insights
- Customer effort score
- Customer Engagement
- Customer Experience
- Customer Loyalty
- Customer Research
- Customer Satisfaction
- Employee Benefits
- Employee Engagement
- Employee Retention
- Friday Five
- General Data Protection Regulation
- Insights Hub
- Life@QuestionPro
- Market Research
- Mobile diaries
- Mobile Surveys
- New Features
- Online Communities
- Question Types
- Questionnaire
- QuestionPro Products
- Release Notes
- Research Tools and Apps
- Revenue at Risk
- Survey Templates
- Training Tips
- Uncategorized
- Video Learning Series
- What’s Coming Up
- Workforce Intelligence
- Privacy Policy
Buy Me a Coffee

Home » Inductive Vs Deductive Research
Inductive Vs Deductive Research
Table of Contents

Inductive and deductive research are two different approaches to conducting a research study. While they are different in their orientation and methods, they can both be used to generate new knowledge and advance scientific understanding.
Inductive Research
Inductive research is a bottom-up approach to research where a researcher starts with specific observations and data and then works to generate general principles or theories. This approach involves collecting data and analyzing it to identify patterns, themes, or categories. From these patterns, the researcher can develop theories or concepts that can explain the observations made in the data. Inductive research is often used in qualitative research, case studies, and grounded theory research.
Deductive Research
Deductive research is a top-down approach to research where a researcher starts with a theory or hypothesis and then tests it through data collection and analysis. This approach involves testing a specific hypothesis or theory and then drawing conclusions based on the results of the analysis. Deductive research is often used in quantitative research, experimental research, and survey research.
Difference between Inductive and Deductive Research
Here are some key differences between inductive and deductive research:
Both inductive and deductive research have their strengths and weaknesses, and the choice of which to use depends on the nature of the research question, the research objectives, and the available resources. Inductive research is useful when a researcher wants to explore a new area of inquiry or when there is limited theory or previous research available, while deductive research is useful when a researcher wants to test a specific theory or hypothesis.
Also see Research Methods
About the author
Muhammad Hassan
Researcher, Academic Writer, Web developer
You may also like

Exploratory Vs Explanatory Research

Basic Vs Applied Research

Generative Vs Evaluative Research

Reliability Vs Validity

Longitudinal Vs Cross-Sectional Research

Qualitative Vs Quantitative Research
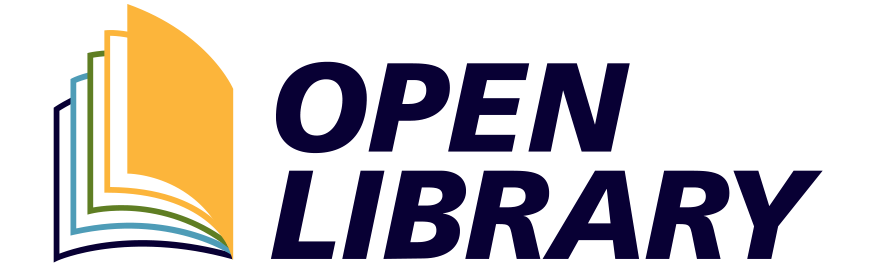
Want to create or adapt books like this? Learn more about how Pressbooks supports open publishing practices.
1-2 The Nature of Evidence: Inductive and Deductive Reasoning
Renate Kahlke; Jonathan Sherbino; and Sandra Monteiro
Different philosophies of science (Chapter 1-1) inform different approaches to research, characterized by different goals, assumptions, and types of data. In this chapter, we discuss how post-positivist, interpretivist, constructivist, and critical philosophies form a foundation for two broad approaches to generating knowledge: quantitative and qualitative research. There are several often-discussed distinctions between these two approaches, stemming from their philosophical roots, specifically, a commitment to objectivity versus subjective interpretation, numbers versus words, generalizability versus transferability, and deductive versus inductive reasoning. While these distinctions often distinguish qualitative and quantitative research, there are always exceptions. These exceptions demonstrate that quantitative and qualitative research approaches have more in common than what superficial descriptions imply.
Key Points of the Chapter
By the end of this chapter the learner should be able to:
- Describe quantitative research methodologies
- Describe qualitative research methodologies
- Compare and contrast these two approaches to interpreting data
Rayna (they/their) stared at the blinking cursor on her screen. They had been recently invited to revise and resubmit their first qualitative research manuscript. Most of the editor and reviewer comments had been relatively easy to handle, but when Rayna reached Reviewer 2’s comments, they were caught off guard. The reviewer acknowledged that they came from a quantitative background, but then went on to write: “I am worried about the generalizability of this study, with a sample of only 25 residents. Wouldn’t it be better to do a survey to get more perspectives?”
Rayna hadn’t seen any other qualitative studies talk about generalizability, so they weren’t entirely sure how to address this comment. Maybe the study won’t be valuable if the results aren’t generalizable! Reviewer 2 might be right that the sample size is too small! They immediately panic-email one of their co-authors:
Hi Rayna, We get these kinds of reviews all the time. Don’t worry, it’s not a flaw in your study. That said, I think we can build our field’s knowledge about qualitative research approaches by explaining some of these concepts. Start with the one by Monteiro et al. and then read the one by Wright and colleagues. These are both good primers and will give you some language to help respond to this reviewer.
With a sigh of relief, Rayna reads through the attached papers (1-8) and continues her mission to craft a response.
Deeper Dive on this Concept
Qualitative and quantitative research are often talked about as two different ways of thinking and generating knowledge. We contrast a reliance on words with a reliance on numbers, a focus on subjectivity with a focus on objectivity. And to some extent these approaches are different, in precisely the ways described. However, many experienced researchers on both sides of the line will tell you that these differences only go so far.
Drawing on Chapter 1-1, there are different philosophies of science that inform researchers and their research products. Generally, quantitative research is associated with post-positivism; researchers seek to be objective and reduce their bias in order to ensure that their results are as close as possible to the truth. Post-positivists, unlike positivists, acknowledge that a singular truth is impossible to define. However, truth can be defined within a spectrum of probability. Quantitative researchers generate and test hypotheses to make conclusions about a theory they have developed. They conduct experiments that generate numerical data that define the extent to which an observation is “true.” The strength of the numerical data suggests whether the observation can be generalized beyond the study population. This process is called deductive reasoning because it starts with a general theory that narrows to a hypothesis that is tested against observations that support (or refute) the theory.
Qualitative researchers, on the other hand, tend to be guided by interpretivism, constructivism, critical theory or other perspectives that value subjectivity. These analytic approaches do not address bias because bias assumes a misrepresentation of “truth” during collection or analysis of data. Subjectivity emphasizes the position and perspective assumed during analysis, articulating that there is no external objective truth, uninformed by context. ( See Chapter 1-1 .) Qualitative methods seek to deeply understand a phenomenon, using the rich meaning provided by words, symbols, traditions, structures, identity constructs and power dynamics (as opposed to simply numbers). Rather than testing a hypothesis, they generate knowledge by inductively generating new insights or theory (i.e. observations are collected and analyzed to build a theory). These insights are contextual, not universal. Qualitative researchers translate their results within a rich description of context so that readers can assess the similarities and differences between contexts and determine the extent to which the study results are transferable to their setting.
While these distinctions can be helpful in distinguishing quantitative and qualitative research broadly, they also create false divisions. The relationships between these two approaches are more complex, and nuances are important to bear in mind, even for novices, lest we exacerbate hierarchies and divisions between different types of knowledge and evidence.
As an example, the interpretation of quantitative results is not always clear and obvious – findings do not always support or refute a hypothesis. Thus, both qualitative and quantitative researchers need to be attentive to their data. While quantitative research is generally thought to be deductive, quantitative researchers often do a bit of inductive reasoning to find meaning in data that hold surprises. Conversely, qualitative data are stereotypically analyzed inductively, making meaning from the data rather than proving a hypothesis. However, many qualitative researchers apply existing theories or theoretical frameworks, testing the relevance of existing theory in a new context or seeking to explain their data using an existing framework. These approaches are often characterized as deductive qualitative work.
As another example, quantitative researchers use numbers, but these numbers aren’t always meaningful without words. In surveys, interpretation of numerical responses may not be possible without analyzing them alongside free-text responses. And while qualitative researchers rarely use numbers, they do need to think through the frequency with which certain themes appear in their dataset. An idea that only appears once in a qualitative study may have great value to the analysis, but it is also important that researchers acknowledge the views that are most prevalent in a given qualitative dataset.
Key Takeaways
- Quantitative research is generally associated with post-positivism. Since researchers seek to get as close to ‘the truth’, as they can, they value objectivity and seek generalizable results. They generate hypotheses and use deductive reasoning and numerical data numbers to prove or disprove their hypotheses. Replicating patterns of data to validate theories and interpretations is one way to evaluate ‘the truth’.
- Qualitative research is generally associated with worldviews that value subjectivity. Since qualitative researchers seek to understand the interaction between person, place, history, power, gender and other elements of context, they value subjectivity (e.g. interpretivism, constructivism, critical theory). Qualitative research does not seek generalizability of findings (i.e. a universal, decontextualized result), rather it produces results that are inextricably linked to the context of the data and analysis. Data tend to take the form of words, rather than numbers, and are analyzed inductively.
- Qualitative and quantitative research are not opposites. Qualitative and quantitative research are often marked by a set of apparently clear distinctions, but there are always nuances and exceptions. Thus, these approaches should be understood as complementary, rather than diametrically opposed.
Vignette Conclusion
Rayna smiled and read over their paper once more. They had included an explanation of qualitative research, and how it’s about depth of information and nuance of experience. The depth of data generated per participant is significant, therefore fewer people are typically recruited in qualitative studies. Rayna also articulated that while the interpretivist approach she used for analysis isn’t focused on generalizing the results beyond a specific context, they were able to make an argument for how the results can transfer to other, similar contexts. Cal provided a bunch of margin comments in her responses to the reviews, highlighting how savvy Rayna had been in addressing the concerns of Reviewer 2 without betraying their epistemic roots. The editor certainly was right – adding more justification and explanation had made the paper stronger, and would likely help others grow to better understand qualitative work. Now the only challenge left was figuring out how to upload the revised documents to the journal submission portal…
- Wright, S., O’Brien, B. C., Nimmon, L., Law, M., & Mylopoulos, M. (2016). Research Design Considerations. Journal of Graduate Medical Education, 8(1), 97–98. doi: 10.4300/JGME-D-15-00566.1
- Monteiro S, Sullivan GM, Chan TM. Generalizability theory made simple (r): an introductory primer to G-studies. Journal of graduate medical education. 2019 Aug;11(4):365-70. doi: 10.4300/JGME-D-19-00464.1
- Goldenberg MJ. On evidence and evidence-based medicine: lessons from the philosophy of science. Social science & medicine. 2006 Jun 1;62(11):2621-32. doi: 10.1016/j.socscimed.2005.11.031
- Varpio L, MacLeod A. Philosophy of science series: Harnessing the multidisciplinary edge effect by exploring paradigms, ontologies, epistemologies, axiologies, and methodologies. Academic Medicine. 2020 May 1;95(5):686-9. doi: 10.1097/ACM.0000000000003142
- Morse JM, Mitcham C. Exploring qualitatively-derived concepts: Inductive—deductive pitfalls. International journal of qualitative methods. 2002 Dec;1(4):28-35. https://doi.org/10.1177/160940690200100404
- Armat MR, Assarroudi A, Rad M, Sharifi H, Heydari A. Inductive and deductive: Ambiguous labels in qualitative content analysis. The Qualitative Report. 2018;23(1):219-21. https://www.proquest.com/docview/2122314268
- Tavakol M, Sandars J. Quantitative and qualitative methods in medical education research: AMEE Guide No 90: Part I. Medical Teacher. 2014 Sep 1;36(9):746-56. doi: 10.3109/0142159X.2014.915298
- Tavakol M, Sandars J. Quantitative and qualitative methods in medical education research: AMEE Guide No 90: Part II. Medical teacher. 2014 Oct 1;36(10):838-48. doi: 10.3109/0142159X.2014.915297
About the Authors
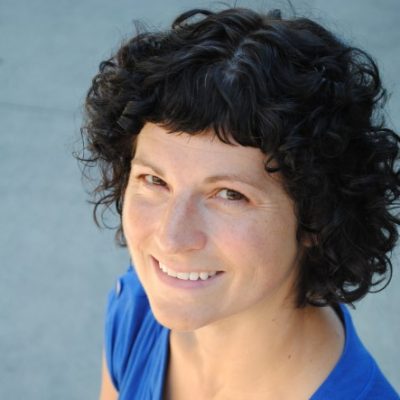
name: Renate Kahlke
institution: McMaster University
Renate Kahlke is an Assistant Professor within the Division of Education & Innovation, Department of Medicine. She is a scientist within the McMaster Education Research, Innovation and Theory (MERIT) Program.
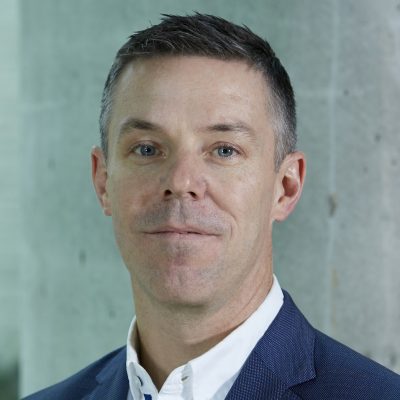
name: Jonathan Sherbino
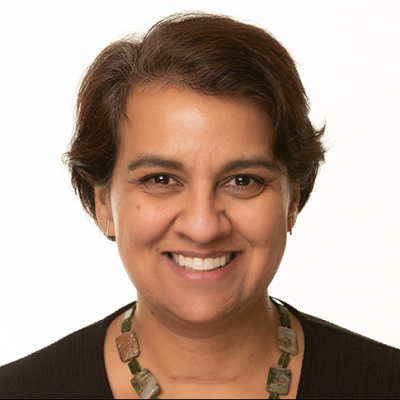
name: Sandra Monteiro
Sandra Monteiro is an Associate Professor within the Department of Medicine, Division of Education and Innovation, Faculty of Health Sciences, McMaster University. She holds a joint appointment within the Department of Health Research Methods, Evidence, and Impact , Faculty of Health Sciences, McMaster University.
1-2 The Nature of Evidence: Inductive and Deductive Reasoning Copyright © 2022 by Renate Kahlke; Jonathan Sherbino; and Sandra Monteiro is licensed under a Creative Commons Attribution-NonCommercial-ShareAlike 4.0 International License , except where otherwise noted.
Share This Book
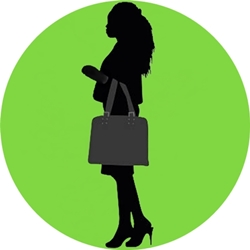
Dr Deborah Gabriel
Inductive and deductive approaches to research
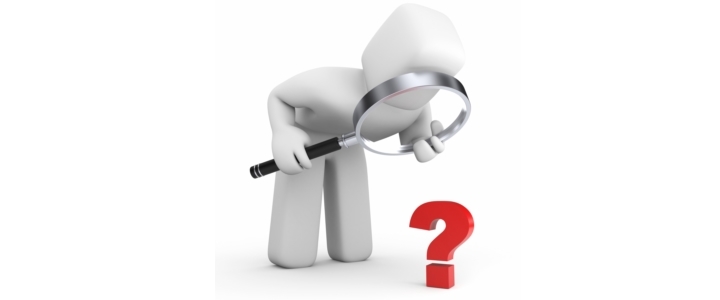
The main difference between inductive and deductive approaches to research is that whilst a deductive approach is aimed and testing theory, an inductive approach is concerned with the generation of new theory emerging from the data.
A deductive approach usually begins with a hypothesis, whilst an inductive approach will usually use research questions to narrow the scope of the study.
For deductive approaches the emphasis is generally on causality, whilst for inductive approaches the aim is usually focused on exploring new phenomena or looking at previously researched phenomena from a different perspective.
Inductive approaches are generally associated with qualitative research, whilst deductive approaches are more commonly associated with quantitative research. However, there are no set rules and some qualitative studies may have a deductive orientation.
One specific inductive approach that is frequently referred to in research literature is grounded theory, pioneered by Glaser and Strauss.
This approach necessitates the researcher beginning with a completely open mind without any preconceived ideas of what will be found. The aim is to generate a new theory based on the data.
Once the data analysis has been completed the researcher must examine existing theories in order to position their new theory within the discipline.
Grounded theory is not an approach to be used lightly. It requires extensive and repeated sifting through the data and analysing and re-analysing multiple times in order to identify new theory. It is an approach best suited to research projects where there the phenomena to be investigated has not been previously explored.
The most important point to bear in mind when considering whether to use an inductive or deductive approach is firstly the purpose of your research; and secondly the methods that are best suited to either test a hypothesis, explore a new or emerging area within the discipline, or to answer specific research questions.
Citing This Article
Gabriel, D. (2013). Inductive and deductive approaches to research. Accessed on ‘date’ from https://deborahgabriel.com/2013/03/17/inductive-and-deductive-approaches-to-research/
Gabriel, D., 2013. Inductive and deductive approaches to research. Accessed on ‘date’ from https://deborahgabriel.com/2013/03/17/inductive-and-deductive-approaches-to-research/
You May Also Like
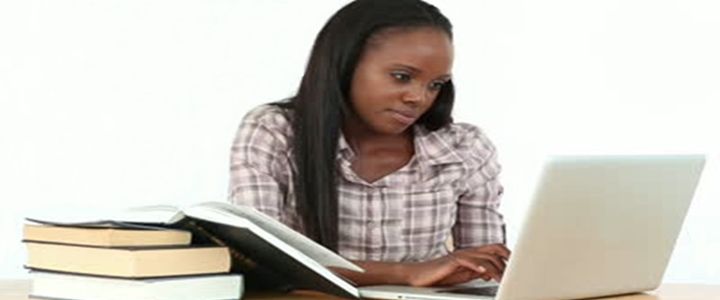
How to write a literature review
Whether you are writing a 2000-word literature review for an undergraduate dissertation or something closer to 10,000-words for a PhD thesis, the process is the same.

Using Conceptual Frameworks in Qualitative Research
I recently delivered this presentation to final year undergraduate students about to embark on their dissertation projects.

Qualitative data analysis
The most important task that you will undertake in your PhD research project is the analysis of data. The integrity and rigour of your research is utterly dependent on getting the analysis right and it will be intensely scrutinised by the external examiners.
200 thoughts on “ Inductive and deductive approaches to research ”
- Pingback: Methods and methodology | Deborah Gabriel
- Pingback: PhD research: qualitative data analysis | Deborah Gabriel
Hi, yes the explanation was helpful because it was simple to read and pretty much, straight to the point. It has given me a brief understanding for what I needs. Thanks — Chantal
It was very supportive for me!! thank you!!
thank you so much for the information. it was simple to read and also brief concise and straight to the point. thank you.
Deborah, thanks for this elaboration. but I am asking is it possible to conduct a deductive inclined research and also generate a theory, or add to the theory. I have been asked by my supervisor whether I am just testing hypothesi or my aim is to contribute/generate a theory. my studies is more of a quantitative nature. Thanks
Deductive research is more aimed towards testing a hypothesis and therefore is an approach more suited to working with quantitative data. The process normally involves reproducing a previous study and seeing if the same results are produced. This does not lend itself to generating new theories since that is not the object of the research. Between inductive and deductive approaches there is also a third approach which I will write a post on shortly – abdductive.
Dear Deborah, it has been very long time since you posted this article. However, I can testify that it is very helpful for a novis reseracher like me.
Ms. Deborah, thank you for given info, but i was in confusion reg, differences between deductive, inductive, abductive and (new one) Hypothetico-deductive approaches. Can it be possible to email the differences, its applications, tools used and scientific nature, to build a theory using quantitative survey method. My email Id is: [email protected] Iam writing my PhD thesis based on this . it is part of my 3 chapter. Thanking you awaiting for your mail soon.
Hi Madhu. As a PhD student you need to take the time to read the appropriate literature on research approaches and attend workshops/conferences to better understand methodology. You cannot take short cuts by by asking someone (me) to simply provide you with ready answers to your queries – especially when I do not have the time to do so!
Great saying…every student at every level, should be critical analysts and critical thinkers.
Thank u.. but there is a little mistake which is deductive approach deals with qualitative and inductive appoech deals with quantitative
No – you have it the wrong way round – I suggest you read the article again and also engage in wider reading on research methods to gain a deeper understanding.
A clear cut concept with example.
Deborah I appreciate so much in this article…I got what I am looking for… thanks for your contribution.
Thanks so much
It is simple, easy to differentiate and understandable.
Thank u for the information, it really helps me.
Exactly, your work is simple and clear, that there are two research approaches, Inductive and deductive.Qualitative and Quantative approaches You gave clear differences in a balance, simple to understand, I suppose you are a teacher by profession. This is how we share knowledge,and you become more knowledgable
Thank you Lambawi, I am glad that these posts are proving useful. I will endeavour to add some more in the coming weeks!
This has been helpful. thanks for posting
Thank you very much for sharing knowledge with me. It really much helpful while preparing my college exam. Thank –:)
Thank you ever so much for making it simple and easily understandable. Would love to see more posts.
Best wishes
The explanation is simple and easy to understand it has helped to a lot thank you
very helpful and explained simply. thanks
Explanation is simple…. it was a great help for my exam preparation.
Excellent presentation please!
Very helpful information and a clear, simple explanation. Thank you
Thanks; this has been helpful in preparation for my forthcoming exams
This is fantastic, I have greatly beneffitted from this straight forward illustration
Thanks…i will benifited to read this
Thanks for your help. Keep it like that so that will be our guide towards our destinations.
Thank-you for your academic insights.
Thank you for your clarification. Well understood.
Hi, I had a question would you call process tracing technique an inductive or deductive approach? or maybe both? Hope you can help me with the same.
Hi Achin. Process tracing is a qualitative analytical tool and therefore inductive rather than deductive, since its purpose is to identify new phenomena. You might find this journal article useful:
http://www.ukcds.org.uk/sites/default/files/uploads/Understanding-Process-Tracing.pdf
Preparing for my Research Methods exams and I'm grateful for your explanations. This is a full lecture made simple. Thank you very much.
I am very thankful for this information, madam you are just good. If you are believer, allow me to say, May God bless you with more knowledge and good health.
Hi Rasol, glad that the post was useful and – yes – I am a believer so thank you for the blessing!
Dear Deborah
I am currently doing Btech in forensic with Unisa would you be so kind and help with this question below and may I use your services while Iam doing this degree Please
Hi I have question that goes like these "If the reseacher wanted to conduct reseach in a specific context to see whether it supports an establisblished theory" the reseacher would be conducting
1. case study
2. deductive research
3. exploratory resarch
4. inductive methods
please help me to choose the right one
Yours Faithfully
Baba Temba
Deductive, which is not exploratory but designed to test a hypothesis. So this is unlike to be case study research but a quantitative study.
Hi Deborah, I have been struggling with my research methods proposal, in finding the right methodology for my study. This is the only explanation out of all the books that I have read which really enables me to truly understand the meaning of Grounded Theory for which you describe as an inductive. I just would like to say thank you for your explanation as this has helped me in a way, which I thought I would never get. Thank you Destini
You are most welcome!
Very useful piece of information. Thank you!
Very impressing work, may god bless you with more mighty knowledge.
In fact this has been very usefull information for me in my research,. It's very clear and easy to understand looking at the choice of language ,etc God bless you!!
Hi Ms Deborah Gabriel, I am from the backward area of Pakistan, which is known as “North Waziristan”. Unfortunately famous for terrorism, as from that background, you can understand the weakness of my educational background. I am struggling for MBA degree, and I was searching for Deductive and Inductive approaches, and then I found your best explained article here, already praised by many people. I will just add this “Thank you, May GOD Bless You” I will be highly honoured if you would like to contact me on my email. My email is [email protected] Thanking you for your time and efforts.
Is it possible to use deductive approach in research concerning what has happened in an industry?
If you are seeking to test a hypothesis then yes.
Thank you very much this information has been extremely helpful. I can now progress with my dissertation.
Thanks for that good work Deborah. It has taken me quite a short time to read and understand. Kindly please help me understand what am required to write in this case where my teacher gave me this question: "Explain the process of deduction and induction research approaches".
Please refer to the recommended reading: https://deborahgabriel.com/recommended-reading/
Many thanks to you, I really appreciate u on ur information provided basically on theories and approaches to understanding research.
Thank you very much.
Good work Deborah.
Thank you so much!! The distinction between the two approaches is clear and concise. Most other websites tend to go into long discussions without really getting to the point. This was very helpful.
Thank you , useful explanation
It is a very fruitful post. I would like to ask if the objective of my research is to develop an extended process from the existing processes. And I am going to use qualitative and quantitative research methods, because my research phenomenon requires to study the individual meanings and perceptions and then uses the findings from the qualitative study and also the theoretical study as inputs for the quantitative study. Finally, I will use the findings of the theoretical study and the quantitative study in developing the extended process. So, which approach to follow in this case?
Dear Tamer, Your question is too hypothetical for me to offer a response. But in any event, you are the only one who can decide whether an inductive or deductive approach is appropriate for your research project. This is where methodology comes in – which is about determining what research methods will be most effective in answering your research questions and which are in sync with your approach (e.g. critical, feminist etc).
my dissertation is in the same situation. and I also feel struggle to choose my research approach. I guess its a combination of inductive and deductive. using the deductive approach to test what was found in the literature, and use an inductive approach to examine the themes that emerged from qualitative data.
Thanks much!
What do you think about the approach with quantitative analyses that start with data to generate theories? Typically data mining techniques fit into my example.
This is a question of methodology – research methods must be selected based on the discipline, research questions and approach to the study. For example, If you are seeking to ascertain how many people read the news on their smartphones then a quantitiative method is most appropriate. On the other hand. if you are seeking to delve into why some people read the news on their smartphones, then clearly a qualitative method is required.
Awesome response, I was looking at the same thing in my postgraduate class.
What if I’m using secondary sources? Which would be more appropriate qualitative or quantitative?
The question of inductive or deductive approaches arise only in relation to ‘primary’ research – that is when you are undertaking your own study. In your own study, secondary sources would appear under a Literature Review. However, if you are doing a dissertation, say for an undergraduate degree where you are not undertaking primary research then inductive or deductive approaches are not applicable. I hope this clarifies.
Your comments are really good and easy to understand. Hope to contact you for my project. keep up the good work. thank you
The last paragraph stated ‘The most important point to bear in mind when considering whether to use an inductive or deductive approach is firstly the purpose of your research; and secondly the methods that are best suited to either test a hypothesis, explore a new or emerging area within the discipline, or to answer specific research questions. However my question is if my research is about answering specific research questions in a qualitative research. Am I to use the inductive or the deductive or the mixture of the two?
Hi Ola, if your research questions are qualitatively focused – that is seeking to find out the whys and the hows as opposed to ‘what’ and ‘how many’ then certainly an inductive approach is most appropriate. This is because inductive aims to find new theories emerging from the data whereas deductive is centred on testing a hypothesis rather than exploring research questions.
Thank u so much.it was difficult for me to understand but with ur help the job is complete
Points of distinction top notch. Absolutely fantastic. Straight to the point. Was really helpful. Keep up the good work.
Thanks for the inforation Deborah. It was useful
Thank you so much, this was something I was never able to grasp so well! I found this site while searching the difference between the two on Google. I am a PHD Scholar, now it seems I will be visiting this site frequently and seeking your help 🙂
Hi Deborah. Thank you for the input.It clearly exemplifies the difference. In your response to one of the questions, you have highlighted a lot of 'what' will qualify the research as quantitative. I have developed 4 research questions, 3 are on 'what's and 1 'why'. The what is because my sample of analysis is multimodal text. Will my study still fall under qualitative? Thank you in advance, Deborah. I appreciate it very much.
Hi Zilla, It is hard to provide a definitive answer without knowing what your research questions are (although time does not permit me to provide individual responses). So I will reiterate that the question of whether to adopt an inductive or deductive approach to a research project is relevant for ‘primary’ research – that is, research that you undertake yourself. Factors that influence your decision should rest on whether you are seeking to explore the ‘whys’ and ‘hows’ of human experience, generating new levels or understanding or simply wish to test a hypothesis or use a large sample in order to generalise results to the wider population. You say that your sample is multimodal text – that is simply text plus media such as videos, pictures etc. My question to you is whether this multimodal text has been generated from primary research – i.e interviews you conducted, photos you took and/or videos that you filmed of research subjects? If that is the case then I would presume that this would be a qualitative research project that would lend itself to an inductive approach,since I cannot imagine that you would be able to work with a very large sample of multimodal text. If the multimodal text is not generated from your own primary resesarch then this is secondary research that might be included in your literature review but would fall outside the scope of your analysis.
Dear Deborah I just want to ask you to help me with generation of theory. Steps that need to be followed
Mongwai Michael
Thanks a lot for showing me the best way to understand the basic difference between two approaches of research.
Dear Aliyu, time does not permit me to provide responses on your individual projects. Therefore, my aim is to equip you with the understanding of different approaches so that you have both the confidence and competence to make appropriate decisions on the most suitable methodological approaches to your research.
Beautiful stuff you are giving us Deborah.
Deborah, thank you so much for your explanation, I'm clear now.
I am gathering quantitative data to develop a model to represent the behavior of a material using an existing model. I subsequently used this model to simulate the material behavior with a computer program. this is a reversal approach to previour reaeasch in these area. usually the computer simulation is used to obtain quantitative data without experiment. Could you please kindly let me know what is my reasearch method Thanks
Please see my response to Aliyu on 8 November.
Dr, your explanation about inductive research and deductive, is meaningful to postgraduate students. What is your suggestion on my research topic: use of handset by primary six pupils for games, rather for home works and readings, what is the research approach that will be suitable?
Very brief and well explained. Thank you Deborah.
Thank you Dr. Gabriel, good informationl; will come back.
It has actually helped – a similar question was asked last year in my schools,that prompted me to search for it while preparing for my exam. Today the same question appeared and I used your explanation as my response to the question. Thanks.
Hi Deborah, your explanations are comprehensible. I understand this topic thanks to you. May I ask you question? What are the similarities between inductive and deductive reasoning?
That’s like asking what the similarities are between quantitative and qualitative research! Focus on what your research objectives are and then choose the approach that will be most efefctive in meeting these objectives.
Thanks so much Have got what I really want here
Enlightening facts. Thank you.
Thanks Deborah for the explanation but, i want to ask if descriptive is inductive or deductive approach? God bless you
it is really good explanation
Can I ask one question? I am going to research how technology is changing the hotel industry particularly at the hotel front desk so is that inductive or deductive approach? I believe deductive approach because the aim of my research is to investigate current used technology at hotel front desk. So what do you think please let me know Thank you very much indeed.
Please refer to my post on conceptual frameworks to take you through the key steps in developing a research project – you will find your answer there: https://deborahgabriel.com/2015/02/14/using-conceptual-frameworks-in-qualitative-research/
The information provide is quite helpful, thanks after all….
I was confused about these approaches but your information has helped me a lot – reasonable and authentic.
I've got the answers,thx.
It is very clear and concise.
Thank you, it was right on point.
Thank you, I used this solution for my assignment.
Thank you so much Deborah. I am currently doing my dissertation and most of my lecturers have recommeded us to use Research Methods for Business Students by Mark Saunders, Philip Lewis and Adrian Thornhill. I have found the book very hard to understand especially when I'm wrtiting up the methodology section as I have to talk about deductive and inductive approaches. You have simplified it and explained it well. Also you have made it so so easy to understand. Everyone should be reading this. Thank you so so, so much.
You’re very welcome. Good luck with your dissertation!
Thank you D now I am aware of these two!
I found it so easy to understand the difference between deductive theory and inductive- it's so helpful. Many Thanks.
Deborah, your work is precise,well organized and relevant.Thank you very much.
Thanks for the explanation, it has cleared my doubts.
Thanks Dr. Deb, I am satisfied – it was really useful.
Hi Doc, thank you for making things simpler for me. I will always be incontact with your website. Stay forever blessed.
Thank you for the information. It really helps me.
Hi Deborah, i just went through the abductive approach which is combination of inductive and deductive Approach. I found it a little confusing when I tried to know by my own from e sources. But after going through the conversation in this page helped me a lot. Thank u very much. If u can share your email I can share my report made for my pre PhD comprehensive viva. My profession is teaching and my area of research is International HRM. Title is Knowledge and Learning Model among effective repatriation . If anybody is doing reserach in the same area, plse feel free to reach me at [email protected]. Thank u all again
Thx for the information.
Hi Deborah Thank you very much for the article. it is informative. My question is what approach am i supposed to take if i am doing a research that is both qualitative and quantitative. I am doing research on the feasibility of establishing renewable energy systems in a developing country. I am using a simulation software to generate a model to analyse the technical and economic data (Quantitative) but i have to use interviews to capture social and polical views from industry experts (Qualitative). So which approach is best in such a scenario? Thank you
In a mixed methods study, the quanitiative dimension of the study usually functions to capture preliminary data, with the qualitative dimension being the primary method that answers the research questions. In any case, in a mixed methods study you must peform both quantitative and qualitative data analysis – separately. In reference to your specifc study you need to refer back to your reearch questions and the aims and objectives of your study. Is your primary objective to develop a model for a renewable energy system or is it to determine whether industry experts see the viability of the model? If it is the latter then the approach should be inductive. I would advise you to consult your supervisor or someone in your discipline, as I am not an engineer.
Very informative.
Your explaination of inductive & deductive approaches to research is clear to understand.
Your explanation of concepts is succint and easily conceivable. Helpful.
Thank you so much Deborah. I am currently writing my research on resource curse theory and I will like to have your private mail for private discussions. Thank you
No problem – you can use the contact form and your message will go directly to my email address.
Thanks for differentiating the two in easy and pragmetic manner.
Thank you Deborah, that was a simple, clear explanation helpful for sure.
I like the way you simplified everything,was really helpful for my assignment. Please how do I reference this work? Thank you
Reference it as an online source:
Gabriel,D.(2013). Inductive and deductive approaches to research. Avaolable from: https://deborahgabriel.com/2013/03/17/inductive-and-deductive-approaches-to-research/
Thank you Deborah, this is very helpful to me and others.
Great insight, simple and clear; I now get the difference thanks for sharing.
Thanks for the very good explanation and comparison.
A very simple and straightforward guidance to students.
Hi Debrah , It is really interesting to get valuable points from your statments about deductive reasoning. However it seems short . It will be helpful for us if you write more. Thanks
Dear Almaz, thank you for your feedback. The post was only intended to provide a brief overview of the subject – to better understand inductive and deductive approaches to research I strongly recommend immersing yourself in the available literature.
Perhaps, you can suggest 1 or 2 widely cited scholars (to read) that argue that deductive approaches can also be used in a qualitative methodology which is interpretive/subjective in nature.
Thank you once again!
You are definitely on point.
Thank you so much.
Thank you so much Deborah.
Such inspiring work.
Your literature is helping us a lot here at the Ivory Tower Makerere University Kampala Uganda.
I am glad to hear that. What are you studying?
Hi Deborah. I am Iftikhar from United Arab Emirates (UAE). I am conducting a research on learning preferences of Generation Z youth, and one of my research questions is "What are the learning preferences of UAE Gen Z youth and how matching of L&D program delivery with these learning preferences affect Gen Z interest in organizational L&D programs?" Now as for existing literature, a lot has already been written on this but in the West; there is practically no formal research literature available on this topic in UAE. Therefore, I am taking the Western literature outcomes and applying these in UAE context to see the results. My questions are: a. Will this research be treated as "Deductive' or "Inductive"? b. Should I choose 'Quantitative" or "Qualitative' approach? Wishing you all the best.
Thanks Deborah. but I'm confused. You said deductive approach is used in quantitative research and it test a theory and inductive approach is used in qualitative research to generate a theory. So what is grounded theory?
Thanks a lot for such a good explanation, Deborah!
Thank you very much. It was simple to understand.
Lovely….this was very helpful…simple and straightforward.
Thanks. It is so useful. Best Regards.
This has been troubling me for a while. It is often said that the interpretive paradigm typically goes with inductive approaches and methods involving observation, interviews and research into archives. But then if concepts are to emerge from the data without theoretical preconceptions, how come it is often said that the research design, choice of case studies, and initial coding in thematic analysis can be theory driven? Wouldn’t that make the approach deductive (i.e. about testing theory). Or, how does theory coming before the research design fit with an inductive approach? In my experience so far authors seem to evade this point.
HALLO D! I REALLY LIKE YOUR WORK IT REALLY HELPED ME IN MY RESEARCH.
Thank You so very much Deborah. I really got to uncover what puzzled me on deductive versus inductive approaches.
Thanks Dr.Gabriel. It was very simple and useful. Now I understood the differecne b/w deductive and inductive method.
Thanx for sharing with us. It is very useful for my dissertation. Your topic clarifies the difference between inductive and deductive research.
Hi Dr.Gabriel, I am doing a research to apply a theory into service industry which is more commonly practiced in manufacturing industry ( known as Lean approach), my aim is to apply this approach into banking operation, the objective is to find the elements/processes in the bank operation that actually increase the cost or decrease the service quality. If I want to conduct a research to find those elements in a bank operation. should I use Inductive approach? what is your advise? Thanks
Hi Deborah, Thank you for a great article! It made it very clear the differenece between deductive and inductive. I'd like to ask you the following: – Is possible to have inductive study with hypotheses and use semi-structured interviews to answer these hypotheses and research questions? thank you very much for your reply! Alina
Hi Alina, I’m glad my post has been useful for you. In answer to your question, I think maybe you are confusing research questions with hypotheses. Research questions guide the overall study and ensure that when designing interview questions – they are structured in the most effective way to elicit responses that address the research questions. Hypotheses are linked to deductive studies where researchers aim to test presumptions/predictions about phenomenon – this is not the same as research questions.
Hi Deborah Thanks for an intersting piece of work presented. Am kindly inquiring how i can get along with literature review and conceptual framemework on the topic 'IDPs and Solid Waste Management' and objectives; exploring everyday practice around solid waste management; finding out how social networks move and merge into new spaces for waste management and establish connections between waste management and social lfestyle. Thanks Hakimu
Hi Deborah thank you for a great article . I have a question for you I am doing my research work and I have some issue about theory construction. Basically I am a beginner in social research – I have no idea about constructing new theory. Please let me know about theory construction and what is a procedure – how can I construct theory and also about steps of this method? I hope you will understand my words. Thank you.
Dear Amna, Welcome to the world of research – we all have to start somewhere! If you're new to social research I would recommend you join the Social Research Association (SRA) who provide training and a wealth of resources for researchers. With regards to theory – unless you are researching new phenomena that has never been researched before or are developing a completely new approach (unlikely) you will not be creating 'new' theory with your research project. You will be using existing theory in your approach and embed theoretical perspectives into your methodology. You will also likely use relevant theories when analysing your data. However, before you think about theory you need to develop your methodology – see my other post: methods and methodology .
Thanks so much. This post was very helpful and easy to understand!
Hi Deborah, Thank you for the precise and helpful information .. I need your help as I feel a little bit confused. i am doing a case study of airline corporate image. it is the newest crisis scenario in my country related to our regional carrier. I think, i among the pioneers doing the case study research for this airline company. I used the conceptual framework from other previous conducted study. It was conducted in quantitative manner. If i used the conceptual framework as my guidance for my literature review and interview question construction, is that okay if i do not use inductive for the case study because i do not build a new theory. If i just compare and argue with the previous finding and the model used, is it consider as deductive approach in case study? Based on my reading, i found some researchers used deductive approach in their case study. they tested the hypotheses..but i just compare my finding with the model used from the previous research. For your information, i did documentation, direct observation and interview (trigulation) with ex-passengers and aviation expert. What do you think? .Please help me..i am stuck. Thank you
Thank you Doctor, it’s straightforward valuable piece of knowledge. It may require a little bit of referencing. Furthermore, adding citation line below will be useful for academic use.
Thank you very much!
Thank you for a crisp and nice post. It helped me.
I am very beginner in research, and its really very helpful.
Thanks writer,
Thank you very much…
wow wow wow great work Deborah. I now have a clear insight of the differences,,,, kudos!
Thank you …it’s helpful for me.
Good job.It helped me to find the question’s answer in my mid-term. Thenk you.
My study is ethnographic research specifically it studies about culture, tradition and lifestyle of an ethnic groups. I think my research is inductive, is it right?
Thank you, I feel same as most the above commentators. Very well written – written in a way that I (who for the first time heard of these two types of researcher methods,) felt like I got a gist of what they are and how they are different.
Thanks Deborah G! Your articles have helped me a lot.
your article is simple to understand and please keep it up thanks.
Hi, It is really helpful me to get sorted these concepts in research field in simple manner.Thanks for that and really appreciate it.
Very clear explanation about inductive and deductive appraoches in research. I like it.
I appreciate your clear and precise explanations, thank you.
Thanks for this, it’s clear, concise and easy to understand – very helpful.
That was just perfect. I have been reading about these methods from my course book offered by university for 3 days but I couldn’t understand their differences. Now it is completely clear. Thanks a lot.
Is that possible to have both in our research? I mean, what if we choose an inductive approach and then when we go forward make some assumptions to answer research questions?
If you are undertaking inductive research then you don’t make any assumptions as you are looking for micro theories to emerge from your analysis of the data. You cannot start with inductive and then switch to deductive – it must be one or the other. You don’t make assumptions to answer research questions – you analyse the findings to do that.
It is precise and clear. Thanks
Great work and explanation and also the researcher herself is very energetic and motivated to help others… world is because of people like you. thanks
Dear madam, I’m a undergraduate studying engineering and I want to know famous researches done solely based on inductive and deductive separately….if you can give me some examples it will be very helpful to me….
You can look this up yourself, through your library and learning resources at your institution…
Dear Deborah, Thank you for the precise explanation of inductive and deductive approaches.
When analysing data in a qualitative study, could you use both inductive and deductive methods as a triangulation technique?
Hi Irene, a mixed methods study might involve both a quantitative method – e.g. survey and qualitative – e.g. interviews. But the overall approach would still be inductive as the quantitative element normally shapes the qualitative and the overall aim would still be to gain in-depth understandings rather than generalise findings. Mixed methods does as you say, create academic rigour through triangulation.
Dear Deborah Thank you in advance for using your precious time to reply to my question. God bless you. l am doing my master degree dissertation on Green Supply Chain Management practices in the United Kingdom automotive industry. The research philosophy that I adopt is this: interpretivist epistemological and constructivism ontological. The methodology is as follows: Interpretivism – inductive – mono-method qualitative -survey. My question is this: Can online survey questionnaire be used with the inductive approach? Most books that l am reading are linking online survey with quantitative data.
Quite educative. Thank you Dr Deborah, it’s so useful to my study.
Thank you Dr Gabriel. It was really useful abstract. Kindly help me to enlighten with more details on grounded theory, dependent and independent variables.
Thank you Dr Gabriel for sharing your knowledge with all of us. Highly appreciated. I have just started my Ph.D. program, and I’m still struggled to deciding which paradigm I have to use! I have one question: My research idea talks about the readiness of an organization toward IoT (factors that affect the organization readiness towards IoT). note*: The IoT technology is still not implemented in the organization context that I want to study that is why I’m going to study the readiness. so what is your suggestion for me regarding which paradigm I have to use?
Hi Mutasem, Congratulations on embarking on your PhD program! Your research paradigm should reflect your positionality, your values and in essence, how you view the world. You need to think critically and reflectively about this. For example, you say you plan to study the readiness of an organization to implement IT. One approach to this study could be examining what factors might shape that readiness – i.e power structures that confer equality/inequality, and there is also the question of how the adoption of IT could help to create more inclusivity and diversity (which contribute to greater productivity & profitability). This of course is a different proposition from merely focusing on technical issues as opposed to social/political ones that also shape technology use.
THANK YOU SO MUCH FOR YOUR KIND RESPONSE
Dear Dr Gabriel
I am currently busy with my Masters in Interior design. My research aim is to determine (possibly explore as Its not currently making sense) the discrepancy that exists between the designer and a specific user group of a Healthcare environment. I have used provisional coding as a first cycle method (which identified a set of themes by which to analyze a Healthcare environment). These themes (conceptual framework) informed my interviews etc. From there the findings were analysed to see to what extent the designer aims correspond with the way in which the user group experiences the space (through the various themes). I initially thought that it was a deductive study but as it is a qualitative study I was abit worried. From what I understood from previous comments, the inductive/deductive is only applicable in the primary research, would that then mean that my study takes the form of a inductive approach? (although my questions are ‘what’ and ‘how’).
Kind regards Anienke
Hi Deborah, your posts are quite simple and useful. Great! Thanks for posts!
Thanks for posting this, I would say that this article is one of the most useful explanation I have found so far. But I also have a question, hopefully you will be able to help me out. If my research question is about understanding “How important are loyalty programmes for customers in welness field. I use quantitative method (Online survey) to collect data from respondents, what is my approach for research inductive and deductive?
Dr Deborah Gabriel, Honestly, I have gone through your explanation on inductive and deductive approaches to research work and I’m very pleased with the write up. I want to sincerely thank you for your contribution to the existing body of knowledge. Regards, Clemduze.
Thank you Dr Gabriel for explaining the differences between inductive and deductive approaches in research. Your explanation helped me understand these two concepts as I am working on the early portions of my dissertation in General Psychology.
Thank you so much. This has been very useful. I now know I can use both Inductive and deductive if i am carrying out a mixed method research. Both can be used depending on my research questions.
It was helpful.
How can I be at this time replying to this worthy and simple explanation? It’s well placed and and explained. Thank you D.
Thanks for your article. How do I reference you in my work?
APA Gabriel, D. (2013). Inductive and deductive approaches to research. Accessed on ‘date’ from https://deborahgabriel.com/2013/03/17/inductive-and-deductive-approaches-to-research/
Harvard Gabriel, D., 2013. Inductive and deductive approaches to research. Accessed on ‘date’ from https://deborahgabriel.com/2013/03/17/inductive-and-deductive-approaches-to-research/
Can social research be purely deductive?
‘Social’ research – i.e research within the social sciences, can be qualitative or quantitative, and therefore can be inductive or deductive. It depends on what the research objectives are as to which approach is taken – and as my article states, these questions are explored through research methodology.
Very important points are discussed in your article in simplified terms.
Thanks very much!
Appreciated the discussion – it is well simplified and easy to understand.
Comments are closed.
To read this content please select one of the options below:
Please note you do not have access to teaching notes, qualitative research: deductive and inductive approaches to data analysis.
Qualitative Research Journal
ISSN : 1443-9883
Article publication date: 31 October 2018
Issue publication date: 15 November 2018
The purpose of this paper is to explain the rationale for choosing the qualitative approach to research human resources practices, namely, recruitment and selection, training and development, performance management, rewards management, employee communication and participation, diversity management and work and life balance using deductive and inductive approaches to analyse data. The paper adopts an emic perspective that favours the study of transfer of human resource management practices from the point of view of employees and host country managers in subsidiaries of western multinational enterprises in Ghana.
Design/methodology/approach
Despite the numerous examples of qualitative methods of data generation, little is known particularly to the novice researcher about how to analyse qualitative data. This paper develops a model to explain in a systematic manner how to methodically analyse qualitative data using both deductive and inductive approaches.
The deductive and inductive approaches provide a comprehensive approach in analysing qualitative data. The process involves immersing oneself in the data reading and digesting in order to make sense of the whole set of data and to understand what is going on.
Originality/value
This paper fills a serious gap in qualitative data analysis which is deemed complex and challenging with limited attention in the methodological literature particularly in a developing country context, Ghana.
- Qualitative
- Emic interviews documents
Azungah, T. (2018), "Qualitative research: deductive and inductive approaches to data analysis", Qualitative Research Journal , Vol. 18 No. 4, pp. 383-400. https://doi.org/10.1108/QRJ-D-18-00035
Emerald Publishing Limited
Copyright © 2018, Emerald Publishing Limited
Related articles
We’re listening — tell us what you think, something didn’t work….
Report bugs here
All feedback is valuable
Please share your general feedback
Join us on our journey
Platform update page.
Visit emeraldpublishing.com/platformupdate to discover the latest news and updates
Questions & More Information
Answers to the most commonly asked questions here
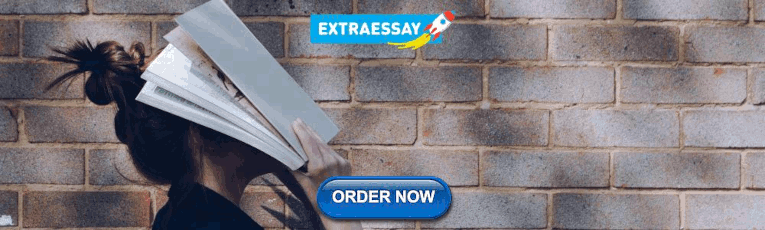
Inductive and/or Deductive Research Designs
- First Online: 27 October 2022
Cite this chapter
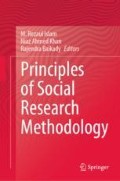
- Md. Shahidul Haque 4
2546 Accesses
1 Citations
This chapter aims to introduce the readers, especially the Bangladeshi undergraduate and postgraduate students to some fundamental considerations of inductive and deductive research designs. The deductive approach refers to testing a theory, where the researcher builds up a theory or hypotheses and plans a research stratagem to examine the formulated theory. On the contrary, the inductive approach intends to construct a theory, where the researcher begins by gathering data to establish a theory. In the beginning, a researcher must clarify which approach he/she will follow in his/her research work. The chapter discusses basic concepts, characteristics, steps and examples of inductive and deductive research designs. Here, also a comparison between inductive and deductive research designs is shown. It concludes with a look at how both inductive and deductive designs are used comprehensively to constitute a clearer image of research work.
- Deductive research design
- Inductive research design
- Research design
This is a preview of subscription content, log in via an institution to check access.
Access this chapter
- Available as PDF
- Read on any device
- Instant download
- Own it forever
- Available as EPUB and PDF
- Compact, lightweight edition
- Dispatched in 3 to 5 business days
- Free shipping worldwide - see info
- Durable hardcover edition
Tax calculation will be finalised at checkout
Purchases are for personal use only
Institutional subscriptions
Beiske, B. (2007). Research methods: Uses and limitations of questionnaires, interviews and case studies . GRIN Verlag.
Google Scholar
Bhattacherjee, A. (2012). Social science research: Principles, methods, and practices (2nd ed.). Global Text Project.
Brewer, J., & Hunter, A. (1989). Multi method research: A synthesis of styles . Sage Publications Ltd.
Burns, N., & Grove, S. K. (2003). Understanding nursing research (3rd ed.). Saunders.
Cambridge Dictionary. (2016a). Hypothesis. In Dictionary.cambridge.org . Retrieved October, 15, 2016, from http://dictionary.cambridge.org/dictionary/english/hypothesis .
Corbin, J. M., & Strauss, A. (1990). Grounded theory research: Procedures, canons, and evaluative criteria. Qualitative Sociology, 13 , 3–21. https://doi.org/10.1007/BF00988593
Article Google Scholar
Creswell, J. W., & Plano Clark, V. L. (2017). Designing and conducting mixed methods research (3rd ed.). Sage Publications Inc.
Crowther, D., & Lancaster, G. (2009). Research methods: A concise introduction to research in management and business . Butterworth-Heinemann.
Easterby-Smith, M., Thorpe, R., & Lowe, A. (2002). Management research: An introduction . Sage Publications Ltd.
Engel, R. J., & Schutt, R. K. (2005). The practice of research in social work . Sage Publications Inc.
Gill, J., & Johnson, P. (2010). Research Methods for Managers (4th ed.). Sage Publications Ltd.
Goddard, W., & Melville, S. (2004). Research methodology: An introduction (2nd ed.). Blackwell Publishing.
Godfrey, J., Hodgson, A., Tarca, A., Hamilton, J., & Holmes, S. (2010). Accounting theory (7th ed). Wiley. ISBN: 978-0-470-81815-2.
Gray, D. E. (2004). Doing research in the real world . Sage Publications Ltd.
Hackley, C. (2003). Doing research projects in marketing, management and consumer research . Routledge.
Book Google Scholar
Lodico, M. G., Spaulding, D. T., & Voegtle, K. H. (2006). Methods in educational research: From theory to practice . John Wiley & Sons.
Merriam-Webster. (2016a). Inductive. In Merriam-Webster.com dictionary . Retrieved October 12, 2016a, from http://www.merriam-webster.com/dictionary/inductive .
Merriam-Webster. (2016b). Deductive. In Merriam-Webster.com dictionary . Retrieved October 12, 2016b, from http://www.merriam-webster.com/dictionary/deductive .
Morgan, D. L. (2014). Integrating Qualitative and Quantitative Methods: A Pragmatic Approach. SAGE Publications, Inc. https://dx.doi.org/10.4135/9781544304533
Neuman, W. L. (2003). Social research methods: Qualitative and quantitative approaches . Allyn and Bacon.
Oxford Dictionary. (2016a). Inductive. In Oxford online dictionary . Retrieved October 15, 2016a, from https://en.oxforddictionaries.com/definition/inductive .
Oxford Dictionary. (2016b). Deductive. In Oxford online dictionary . Retrieved October 15, 2016b, from https://en.oxforddictionaries.com/definition/deductive .
Oxford Dictionary. (2016c). Theory. In Oxford online dictionary . Retrieved October 15, 2016c, from https://en.oxforddictionaries.com/definition/theory .
Saunders, M., Lewis, P., & Thornhill, A. (2007). Research methods for business students (5th ed.). Prentice Hall.
Sherman, L. W., & Berk, R. A. (1984). The specific deterrent effects of arrest for domestic assault. American Sociological Review, 49 (2), 261–272.
Singh, K. (2006). Fundamental of research methodology and statistics. New Age International (P) Limited.
Snieder, R., & Larner, K. (2009). The art of being a scientist: A guide for graduate students and their mentors . Cambridge University Press.
Strauss, A., & Corbin, J. (1998). Basics of qualitative research (2nd ed.). Sage Publications Ltd.
Thomas, D. R. (2006). A general inductive approach for analyzing qualitative evaluation data. American Journal of Evaluation, 27 (2), 237–246. https://doi.org/10.1177/1098214005283748
Trochim, W. M. K. (2006). Research methods knowledge base. Retrieved on October 12, 2016, from http://www.socialresearchmethods.net .
Wilson, J. (2010). Essentials of business research: A guide to doing your research project . Sage Publishers Ltd.
Download references
Author information
Authors and affiliations.
Department of Social Work, Jagannath University, Dhaka, 1100, Bangladesh
Md. Shahidul Haque
You can also search for this author in PubMed Google Scholar
Corresponding author
Correspondence to Md. Shahidul Haque .
Editor information
Editors and affiliations.
Centre for Family and Child Studies, Research Institute of Humanities and Social Sciences, University of Sharjah, Sharjah, United Arab Emirates
M. Rezaul Islam
Department of Development Studies, University of Dhaka, Dhaka, Bangladesh
Niaz Ahmed Khan
Department of Social Work, School of Humanities, University of Johannesburg, Johannesburg, South Africa
Rajendra Baikady
Rights and permissions
Reprints and permissions
Copyright information
© 2022 The Author(s), under exclusive license to Springer Nature Singapore Pte Ltd.
About this chapter
Haque, M.S. (2022). Inductive and/or Deductive Research Designs. In: Islam, M.R., Khan, N.A., Baikady, R. (eds) Principles of Social Research Methodology. Springer, Singapore. https://doi.org/10.1007/978-981-19-5441-2_5
Download citation
DOI : https://doi.org/10.1007/978-981-19-5441-2_5
Published : 27 October 2022
Publisher Name : Springer, Singapore
Print ISBN : 978-981-19-5219-7
Online ISBN : 978-981-19-5441-2
eBook Packages : Social Sciences
Share this chapter
Anyone you share the following link with will be able to read this content:
Sorry, a shareable link is not currently available for this article.
Provided by the Springer Nature SharedIt content-sharing initiative
- Publish with us
Policies and ethics
- Find a journal
- Track your research

An official website of the United States government
The .gov means it’s official. Federal government websites often end in .gov or .mil. Before sharing sensitive information, make sure you’re on a federal government site.
The site is secure. The https:// ensures that you are connecting to the official website and that any information you provide is encrypted and transmitted securely.
- Publications
- Account settings
Preview improvements coming to the PMC website in October 2024. Learn More or Try it out now .
- Advanced Search
- Journal List
- Front Psychol
Quantitative and Qualitative Approaches to Generalization and Replication–A Representationalist View
In this paper, we provide a re-interpretation of qualitative and quantitative modeling from a representationalist perspective. In this view, both approaches attempt to construct abstract representations of empirical relational structures. Whereas quantitative research uses variable-based models that abstract from individual cases, qualitative research favors case-based models that abstract from individual characteristics. Variable-based models are usually stated in the form of quantified sentences (scientific laws). This syntactic structure implies that sentences about individual cases are derived using deductive reasoning. In contrast, case-based models are usually stated using context-dependent existential sentences (qualitative statements). This syntactic structure implies that sentences about other cases are justifiable by inductive reasoning. We apply this representationalist perspective to the problems of generalization and replication. Using the analytical framework of modal logic, we argue that the modes of reasoning are often not only applied to the context that has been studied empirically, but also on a between-contexts level. Consequently, quantitative researchers mostly adhere to a top-down strategy of generalization, whereas qualitative researchers usually follow a bottom-up strategy of generalization. Depending on which strategy is employed, the role of replication attempts is very different. In deductive reasoning, replication attempts serve as empirical tests of the underlying theory. Therefore, failed replications imply a faulty theory. From an inductive perspective, however, replication attempts serve to explore the scope of the theory. Consequently, failed replications do not question the theory per se , but help to shape its boundary conditions. We conclude that quantitative research may benefit from a bottom-up generalization strategy as it is employed in most qualitative research programs. Inductive reasoning forces us to think about the boundary conditions of our theories and provides a framework for generalization beyond statistical testing. In this perspective, failed replications are just as informative as successful replications, because they help to explore the scope of our theories.
Introduction
Qualitative and quantitative research strategies have long been treated as opposing paradigms. In recent years, there have been attempts to integrate both strategies. These “mixed methods” approaches treat qualitative and quantitative methodologies as complementary, rather than opposing, strategies (Creswell, 2015 ). However, whilst acknowledging that both strategies have their benefits, this “integration” remains purely pragmatic. Hence, mixed methods methodology does not provide a conceptual unification of the two approaches.
Lacking a common methodological background, qualitative and quantitative research methodologies have developed rather distinct standards with regard to the aims and scope of empirical science (Freeman et al., 2007 ). These different standards affect the way researchers handle contradictory empirical findings. For example, many empirical findings in psychology have failed to replicate in recent years (Klein et al., 2014 ; Open Science, Collaboration, 2015 ). This “replication crisis” has been discussed on statistical, theoretical and social grounds and continues to have a wide impact on quantitative research practices like, for example, open science initiatives, pre-registered studies and a re-evaluation of statistical significance testing (Everett and Earp, 2015 ; Maxwell et al., 2015 ; Shrout and Rodgers, 2018 ; Trafimow, 2018 ; Wiggins and Chrisopherson, 2019 ).
However, qualitative research seems to be hardly affected by this discussion. In this paper, we argue that the latter is a direct consequence of how the concept of generalizability is conceived in the two approaches. Whereas most of quantitative psychology is committed to a top-down strategy of generalization based on the idea of random sampling from an abstract population, qualitative studies usually rely on a bottom-up strategy of generalization that is grounded in the successive exploration of the field by means of theoretically sampled cases.
Here, we show that a common methodological framework for qualitative and quantitative research methodologies is possible. We accomplish this by introducing a formal description of quantitative and qualitative models from a representationalist perspective: both approaches can be reconstructed as special kinds of representations for empirical relational structures. We then use this framework to analyze the generalization strategies used in the two approaches. These turn out to be logically independent of the type of model. This has wide implications for psychological research. First, a top-down generalization strategy is compatible with a qualitative modeling approach. This implies that mainstream psychology may benefit from qualitative methods when a numerical representation turns out to be difficult or impossible, without the need to commit to a “qualitative” philosophy of science. Second, quantitative research may exploit the bottom-up generalization strategy that is inherent to many qualitative approaches. This offers a new perspective on unsuccessful replications by treating them not as scientific failures, but as a valuable source of information about the scope of a theory.
The Quantitative Strategy–Numbers and Functions
Quantitative science is about finding valid mathematical representations for empirical phenomena. In most cases, these mathematical representations have the form of functional relations between a set of variables. One major challenge of quantitative modeling consists in constructing valid measures for these variables. Formally, to measure a variable means to construct a numerical representation of the underlying empirical relational structure (Krantz et al., 1971 ). For example, take the behaviors of a group of students in a classroom: “to listen,” “to take notes,” and “to ask critical questions.” One may now ask whether is possible to assign numbers to the students, such that the relations between the assigned numbers are of the same kind as the relations between the values of an underlying variable, like e.g., “engagement.” The observed behaviors in the classroom constitute an empirical relational structure, in the sense that for every student-behavior tuple, one can observe whether it is true or not. These observations can be represented in a person × behavior matrix 1 (compare Figure 1 ). Given this relational structure satisfies certain conditions (i.e., the axioms of a measurement model), one can assign numbers to the students and the behaviors, such that the relations between the numbers resemble the corresponding numerical relations. For example, if there is a unique ordering in the empirical observations with regard to which person shows which behavior, the assigned numbers have to constitute a corresponding unique ordering, as well. Such an ordering coincides with the person × behavior matrix forming a triangle shaped relation and is formally represented by a Guttman scale (Guttman, 1944 ). There are various measurement models available for different empirical structures (Suppes et al., 1971 ). In the case of probabilistic relations, Item-Response models may be considered as a special kind of measurement model (Borsboom, 2005 ).

Constructing a numerical representation from an empirical relational structure; Due to the unique ordering of persons with regard to behaviors (indicated by the triangular shape of the relation), it is possible to construct a Guttman scale by assigning a number to each of the individuals, representing the number of relevant behaviors shown by the individual. The resulting variable (“engagement”) can then be described by means of statistical analyses, like, e.g., plotting the frequency distribution.
Although essential, measurement is only the first step of quantitative modeling. Consider a slightly richer empirical structure, where we observe three additional behaviors: “to doodle,” “to chat,” and “to play.” Like above, one may ask, whether there is a unique ordering of the students with regard to these behaviors that can be represented by an underlying variable (i.e., whether the matrix forms a Guttman scale). If this is the case, we may assign corresponding numbers to the students and call this variable “distraction.” In our example, such a representation is possible. We can thus assign two numbers to each student, one representing his or her “engagement” and one representing his or her “distraction” (compare Figure 2 ). These measurements can now be used to construct a quantitative model by relating the two variables by a mathematical function. In the simplest case, this may be a linear function. This functional relation constitutes a quantitative model of the empirical relational structure under study (like, e.g., linear regression). Given the model equation and the rules for assigning the numbers (i.e., the instrumentations of the two variables), the set of admissible empirical structures is limited from all possible structures to a rather small subset. This constitutes the empirical content of the model 2 (Popper, 1935 ).

Constructing a numerical model from an empirical relational structure; Since there are two distinct classes of behaviors that each form a Guttman scale, it is possible to assign two numbers to each individual, correspondingly. The resulting variables (“engagement” and “distraction”) can then be related by a mathematical function, which is indicated by the scatterplot and red line on the right hand side.
The Qualitative Strategy–Categories and Typologies
The predominant type of analysis in qualitative research consists in category formation. By constructing descriptive systems for empirical phenomena, it is possible to analyze the underlying empirical structure at a higher level of abstraction. The resulting categories (or types) constitute a conceptual frame for the interpretation of the observations. Qualitative researchers differ considerably in the way they collect and analyze data (Miles et al., 2014 ). However, despite the diverse research strategies followed by different qualitative methodologies, from a formal perspective, most approaches build on some kind of categorization of cases that share some common features. The process of category formation is essential in many qualitative methodologies, like, for example, qualitative content analysis, thematic analysis, grounded theory (see Flick, 2014 for an overview). Sometimes these features are directly observable (like in our classroom example), sometimes they are themselves the result of an interpretative process (e.g., Scheunpflug et al., 2016 ).
In contrast to quantitative methodologies, there have been little attempts to formalize qualitative research strategies (compare, however, Rihoux and Ragin, 2009 ). However, there are several statistical approaches to non-numerical data that deal with constructing abstract categories and establishing relations between these categories (Agresti, 2013 ). Some of these methods are very similar to qualitative category formation on a conceptual level. For example, cluster analysis groups cases into homogenous categories (clusters) based on their similarity on a distance metric.
Although category formation can be formalized in a mathematically rigorous way (Ganter and Wille, 1999 ), qualitative research hardly acknowledges these approaches. 3 However, in order to find a common ground with quantitative science, it is certainly helpful to provide a formal interpretation of category systems.
Let us reconsider the above example of students in a classroom. The quantitative strategy was to assign numbers to the students with regard to variables and to relate these variables via a mathematical function. We can analyze the same empirical structure by grouping the behaviors to form abstract categories. If the aim is to construct an empirically valid category system, this grouping is subject to constraints, analogous to those used to specify a measurement model. The first and most important constraint is that the behaviors must form equivalence classes, i.e., within categories, behaviors need to be equivalent, and across categories, they need to be distinct (formally, the relational structure must obey the axioms of an equivalence relation). When objects are grouped into equivalence classes, it is essential to specify the criterion for empirical equivalence. In qualitative methodology, this is sometimes referred to as the tertium comparationis (Flick, 2014 ). One possible criterion is to group behaviors such that they constitute a set of specific common attributes of a group of people. In our example, we might group the behaviors “to listen,” “to take notes,” and “to doodle,” because these behaviors are common to the cases B, C, and D, and they are also specific for these cases, because no other person shows this particular combination of behaviors. The set of common behaviors then forms an abstract concept (e.g., “moderate distraction”), while the set of persons that show this configuration form a type (e.g., “the silent dreamer”). Formally, this means to identify the maximal rectangles in the underlying empirical relational structure (see Figure 3 ). This procedure is very similar to the way we constructed a Guttman scale, the only difference being that we now use different aspects of the empirical relational structure. 4 In fact, the set of maximal rectangles can be determined by an automated algorithm (Ganter, 2010 ), just like the dimensionality of an empirical structure can be explored by psychometric scaling methods. Consequently, we can identify the empirical content of a category system or a typology as the set of empirical structures that conforms to it. 5 Whereas the quantitative strategy was to search for scalable sub-matrices and then relate the constructed variables by a mathematical function, the qualitative strategy is to construct an empirical typology by grouping cases based on their specific similarities. These types can then be related to one another by a conceptual model that describes their semantic and empirical overlap (see Figure 3 , right hand side).

Constructing a conceptual model from an empirical relational structure; Individual behaviors are grouped to form abstract types based on them being shared among a specific subset of the cases. Each type constitutes a set of specific commonalities of a class of individuals (this is indicated by the rectangles on the left hand side). The resulting types (“active learner,” “silent dreamer,” “distracted listener,” and “troublemaker”) can then be related to one another to explicate their semantic and empirical overlap, as indicated by the Venn-diagram on the right hand side.
Variable-Based Models and Case-Based Models
In the previous section, we have argued that qualitative category formation and quantitative measurement can both be characterized as methods to construct abstract representations of empirical relational structures. Instead of focusing on different philosophical approaches to empirical science, we tried to stress the formal similarities between both approaches. However, it is worth also exploring the dissimilarities from a formal perspective.
Following the above analysis, the quantitative approach can be characterized by the use of variable-based models, whereas the qualitative approach is characterized by case-based models (Ragin, 1987 ). Formally, we can identify the rows of an empirical person × behavior matrix with a person-space, and the columns with a corresponding behavior-space. A variable-based model abstracts from the single individuals in a person-space to describe the structure of behaviors on a population level. A case-based model, on the contrary, abstracts from the single behaviors in a behavior-space to describe individual case configurations on the level of abstract categories (see Table 1 ).
Variable-based models and case-based models.
From a representational perspective, there is no a priori reason to favor one type of model over the other. Both approaches provide different analytical tools to construct an abstract representation of an empirical relational structure. However, since the two modeling approaches make use of different information (person-space vs. behavior-space), this comes with some important implications for the researcher employing one of the two strategies. These are concerned with the role of deductive and inductive reasoning.
In variable-based models, empirical structures are represented by functional relations between variables. These are usually stated as scientific laws (Carnap, 1928 ). Formally, these laws correspond to logical expressions of the form
In plain text, this means that y is a function of x for all objects i in the relational structure under consideration. For example, in the above example, one may formulate the following law: for all students in the classroom it holds that “distraction” is a monotone decreasing function of “engagement.” Such a law can be used to derive predictions for single individuals by means of logical deduction: if the above law applies to all students in the classroom, it is possible to calculate the expected distraction from a student's engagement. An empirical observation can now be evaluated against this prediction. If the prediction turns out to be false, the law can be refuted based on the principle of falsification (Popper, 1935 ). If a scientific law repeatedly withstands such empirical tests, it may be considered to be valid with regard to the relational structure under consideration.
In case-based models, there are no laws about a population, because the model does not abstract from the cases but from the observed behaviors. A case-based model describes the underlying structure in terms of existential sentences. Formally, this corresponds to a logical expression of the form
In plain text, this means that there is at least one case i for which the condition XYZ holds. For example, the above category system implies that there is at least one active learner. This is a statement about a singular observation. It is impossible to deduce a statement about another person from an existential sentence like this. Therefore, the strategy of falsification cannot be applied to test the model's validity in a specific context. If one wishes to generalize to other cases, this is accomplished by inductive reasoning, instead. If we observed one person that fulfills the criteria of calling him or her an active learner, we can hypothesize that there may be other persons that are identical to the observed case in this respect. However, we do not arrive at this conclusion by logical deduction, but by induction.
Despite this important distinction, it would be wrong to conclude that variable-based models are intrinsically deductive and case-based models are intrinsically inductive. 6 Both types of reasoning apply to both types of models, but on different levels. Based on a person-space, in a variable-based model one can use deduction to derive statements about individual persons from abstract population laws. There is an analogous way of reasoning for case-based models: because they are based on a behavior space, it is possible to deduce statements about singular behaviors. For example, if we know that Peter is an active learner, we can deduce that he takes notes in the classroom. This kind of deductive reasoning can also be applied on a higher level of abstraction to deduce thematic categories from theoretical assumptions (Braun and Clarke, 2006 ). Similarly, there is an analog for inductive generalization from the perspective of variable-based modeling: since the laws are only quantified over the person-space, generalizations to other behaviors rely on inductive reasoning. For example, it is plausible to assume that highly engaged students tend to do their homework properly–however, in our example this behavior has never been observed. Hence, in variable-based models we usually generalize to other behaviors by means of induction. This kind of inductive reasoning is very common when empirical results are generalized from the laboratory to other behavioral domains.
Although inductive and deductive reasoning are used in qualitative and quantitative research, it is important to stress the different roles of induction and deduction when models are applied to cases. A variable-based approach implies to draw conclusions about cases by means of logical deduction; a case-based approach implies to draw conclusions about cases by means of inductive reasoning. In the following, we build on this distinction to differentiate between qualitative (bottom-up) and quantitative (top-down) strategies of generalization.
Generalization and the Problem of Replication
We will now extend the formal analysis of quantitative and qualitative approaches to the question of generalization and replicability of empirical findings. For this sake, we have to introduce some concepts of formal logic. Formal logic is concerned with the validity of arguments. It provides conditions to evaluate whether certain sentences (conclusions) can be derived from other sentences (premises). In this context, a theory is nothing but a set of sentences (also called axioms). Formal logic provides tools to derive new sentences that must be true, given the axioms are true (Smith, 2020 ). These derived sentences are called theorems or, in the context of empirical science, predictions or hypotheses . On the syntactic level, the rules of logic only state how to evaluate the truth of a sentence relative to its premises. Whether or not sentences are actually true, is formally specified by logical semantics.
On the semantic level, formal logic is intrinsically linked to set-theory. For example, a logical statement like “all dogs are mammals,” is true if and only if the set of dogs is a subset of the set of mammals. Similarly, the sentence “all chatting students doodle” is true if and only if the set of chatting students is a subset of the set of doodling students (compare Figure 3 ). Whereas, the first sentence is analytically true due to the way we define the words “dog” and “mammal,” the latter can be either true or false, depending on the relational structure we actually observe. We can thus interpret an empirical relational structure as the truth criterion of a scientific theory. From a logical point of view, this corresponds to the semantics of a theory. As shown above, variable-based and case-based models both give a formal representation of the same kinds of empirical structures. Accordingly, both types of models can be stated as formal theories. In the variable-based approach, this corresponds to a set of scientific laws that are quantified over the members of an abstract population (these are the axioms of the theory). In the case-based approach, this corresponds to a set of abstract existential statements about a specific class of individuals.
In contrast to mathematical axiom systems, empirical theories are usually not considered to be necessarily true. This means that even if we find no evidence against a theory, it is still possible that it is actually wrong. We may know that a theory is valid in some contexts, yet it may fail when applied to a new set of behaviors (e.g., if we use a different instrumentation to measure a variable) or a new population (e.g., if we draw a new sample).
From a logical perspective, the possibility that a theory may turn out to be false stems from the problem of contingency . A statement is contingent, if it is both, possibly true and possibly false. Formally, we introduce two modal operators: □ to designate logical necessity, and ◇ to designate logical possibility. Semantically, these operators are very similar to the existential quantifier, ∃, and the universal quantifier, ∀. Whereas ∃ and ∀ refer to the individual objects within one relational structure, the modal operators □ and ◇ range over so-called possible worlds : a statement is possibly true, if and only if it is true in at least one accessible possible world, and a statement is necessarily true if and only if it is true in every accessible possible world (Hughes and Cresswell, 1996 ). Logically, possible worlds are mathematical abstractions, each consisting of a relational structure. Taken together, the relational structures of all accessible possible worlds constitute the formal semantics of necessity, possibility and contingency. 7
In the context of an empirical theory, each possible world may be identified with an empirical relational structure like the above classroom example. Given the set of intended applications of a theory (the scope of the theory, one may say), we can now construct possible world semantics for an empirical theory: each intended application of the theory corresponds to a possible world. For example, a quantified sentence like “all chatting students doodle” may be true in one classroom and false in another one. In terms of possible worlds, this would correspond to a statement of contingency: “it is possible that all chatting students doodle in one classroom, and it is possible that they don't in another classroom.” Note that in the above expression, “all students” refers to the students in only one possible world, whereas “it is possible” refers to the fact that there is at least one possible world for each of the specified cases.
To apply these possible world semantics to quantitative research, let us reconsider how generalization to other cases works in variable-based models. Due to the syntactic structure of quantitative laws, we can deduce predictions for singular observations from an expression of the form ∀ i : y i = f ( x i ). Formally, the logical quantifier ∀ ranges only over the objects of the corresponding empirical relational structure (in our example this would refer to the students in the observed classroom). But what if we want to generalize beyond the empirical structure we actually observed? The standard procedure is to assume an infinitely large, abstract population from which a random sample is drawn. Given the truth of the theory, we can deduce predictions about what we may observe in the sample. Since usually we deal with probabilistic models, we can evaluate our theory by means of the conditional probability of the observations, given the theory holds. This concept of conditional probability is the foundation of statistical significance tests (Hogg et al., 2013 ), as well as Bayesian estimation (Watanabe, 2018 ). In terms of possible world semantics, the random sampling model implies that all possible worlds (i.e., all intended applications) can be conceived as empirical sub-structures from a greater population structure. For example, the empirical relational structure constituted by the observed behaviors in a classroom would be conceived as a sub-matrix of the population person × behavior matrix. It follows that, if a scientific law is true in the population, it will be true in all possible worlds, i.e., it will be necessarily true. Formally, this corresponds to an expression of the form
The statistical generalization model thus constitutes a top-down strategy for dealing with individual contexts that is analogous to the way variable-based models are applied to individual cases (compare Table 1 ). Consequently, if we apply a variable-based model to a new context and find out that it does not fit the data (i.e., there is a statistically significant deviation from the model predictions), we have reason to doubt the validity of the theory. This is what makes the problem of low replicability so important: we observe that the predictions are wrong in a new study; and because we apply a top-down strategy of generalization to contexts beyond the ones we observed, we see our whole theory at stake.
Qualitative research, on the contrary, follows a different strategy of generalization. Since case-based models are formulated by a set of context-specific existential sentences, there is no need for universal truth or necessity. In contrast to statistical generalization to other cases by means of random sampling from an abstract population, the usual strategy in case-based modeling is to employ a bottom-up strategy of generalization that is analogous to the way case-based models are applied to individual cases. Formally, this may be expressed by stating that the observed qualia exist in at least one possible world, i.e., the theory is possibly true:
This statement is analogous to the way we apply case-based models to individual cases (compare Table 1 ). Consequently, the set of intended applications of the theory does not follow from a sampling model, but from theoretical assumptions about which cases may be similar to the observed cases with respect to certain relevant characteristics. For example, if we observe that certain behaviors occur together in one classroom, following a bottom-up strategy of generalization, we will hypothesize why this might be the case. If we do not replicate this finding in another context, this does not question the model itself, since it was a context-specific theory all along. Instead, we will revise our hypothetical assumptions about why the new context is apparently less similar to the first one than we originally thought. Therefore, if an empirical finding does not replicate, we are more concerned about our understanding of the cases than about the validity of our theory.
Whereas statistical generalization provides us with a formal (and thus somehow more objective) apparatus to evaluate the universal validity of our theories, the bottom-up strategy forces us to think about the class of intended applications on theoretical grounds. This means that we have to ask: what are the boundary conditions of our theory? In the above classroom example, following a bottom-up strategy, we would build on our preliminary understanding of the cases in one context (e.g., a public school) to search for similar and contrasting cases in other contexts (e.g., a private school). We would then re-evaluate our theoretical description of the data and explore what makes cases similar or dissimilar with regard to our theory. This enables us to expand the class of intended applications alongside with the theory.
Of course, none of these strategies is superior per se . Nevertheless, they rely on different assumptions and may thus be more or less adequate in different contexts. The statistical strategy relies on the assumption of a universal population and invariant measurements. This means, we assume that (a) all samples are drawn from the same population and (b) all variables refer to the same behavioral classes. If these assumptions are true, statistical generalization is valid and therefore provides a valuable tool for the testing of empirical theories. The bottom-up strategy of generalization relies on the idea that contexts may be classified as being more or less similar based on characteristics that are not part of the model being evaluated. If such a similarity relation across contexts is feasible, the bottom-up strategy is valid, as well. Depending on the strategy of generalization, replication of empirical research serves two very different purposes. Following the (top-down) principle of generalization by deduction from scientific laws, replications are empirical tests of the theory itself, and failed replications question the theory on a fundamental level. Following the (bottom-up) principle of generalization by induction to similar contexts, replications are a means to explore the boundary conditions of a theory. Consequently, failed replications question the scope of the theory and help to shape the set of intended applications.
We have argued that quantitative and qualitative research are best understood by means of the structure of the employed models. Quantitative science mainly relies on variable-based models and usually employs a top-down strategy of generalization from an abstract population to individual cases. Qualitative science prefers case-based models and usually employs a bottom-up strategy of generalization. We further showed that failed replications have very different implications depending on the underlying strategy of generalization. Whereas in the top-down strategy, replications are used to test the universal validity of a model, in the bottom-up strategy, replications are used to explore the scope of a model. We will now address the implications of this analysis for psychological research with regard to the problem of replicability.
Modern day psychology almost exclusively follows a top-down strategy of generalization. Given the quantitative background of most psychological theories, this is hardly surprising. Following the general structure of variable-based models, the individual case is not the focus of the analysis. Instead, scientific laws are stated on the level of an abstract population. Therefore, when applying the theory to a new context, a statistical sampling model seems to be the natural consequence. However, this is not the only possible strategy. From a logical point of view, there is no reason to assume that a quantitative law like ∀ i : y i = f ( x i ) implies that the law is necessarily true, i.e.,: □(∀ i : y i = f ( x i )). Instead, one might just as well define the scope of the theory following an inductive strategy. 8 Formally, this would correspond to the assumption that the observed law is possibly true, i.e.,: ◇(∀ i : y i = f ( x i )). For example, we may discover a functional relation between “engagement” and “distraction” without referring to an abstract universal population of students. Instead, we may hypothesize under which conditions this functional relation may be valid and use these assumptions to inductively generalize to other cases.
If we take this seriously, this would require us to specify the intended applications of the theory: in which contexts do we expect the theory to hold? Or, equivalently, what are the boundary conditions of the theory? These boundary conditions may be specified either intensionally, i.e., by giving external criteria for contexts being similar enough to the ones already studied to expect a successful application of the theory. Or they may be specified extensionally, by enumerating the contexts where the theory has already been shown to be valid. These boundary conditions need not be restricted to the population we refer to, but include all kinds of contextual factors. Therefore, adopting a bottom-up strategy, we are forced to think about these factors and make them an integral part of our theories.
In fact, there is good reason to believe that bottom-up generalization may be more adequate in many psychological studies. Apart from the pitfalls associated with statistical generalization that have been extensively discussed in recent years (e.g., p-hacking, underpowered studies, publication bias), it is worth reflecting on whether the underlying assumptions are met in a particular context. For example, many samples used in experimental psychology are not randomly drawn from a large population, but are convenience samples. If we use statistical models with non-random samples, we have to assume that the observations vary as if drawn from a random sample. This may indeed be the case for randomized experiments, because all variation between the experimental conditions apart from the independent variable will be random due to the randomization procedure. In this case, a classical significance test may be regarded as an approximation to a randomization test (Edgington and Onghena, 2007 ). However, if we interpret a significance test as an approximate randomization test, we test not for generalization but for internal validity. Hence, even if we use statistical significance tests when assumptions about random sampling are violated, we still have to use a different strategy of generalization. This issue has been discussed in the context of small-N studies, where variable-based models are applied to very small samples, sometimes consisting of only one individual (Dugard et al., 2012 ). The bottom-up strategy of generalization that is employed by qualitative researchers, provides such an alternative.
Another important issue in this context is the question of measurement invariance. If we construct a variable-based model in one context, the variables refer to those behaviors that constitute the underlying empirical relational structure. For example, we may construct an abstract measure of “distraction” using the observed behaviors in a certain context. We will then use the term “distraction” as a theoretical term referring to the variable we have just constructed to represent the underlying empirical relational structure. Let us now imagine we apply this theory to a new context. Even if the individuals in our new context are part of the same population, we may still get into trouble if the observed behaviors differ from those used in the original study. How do we know whether these behaviors constitute the same variable? We have to ensure that in any new context, our measures are valid for the variables in our theory. Without a proper measurement model, this will be hard to achieve (Buntins et al., 2017 ). Again, we are faced with the necessity to think of the boundary conditions of our theories. In which contexts (i.e., for which sets of individuals and behaviors) do we expect our theory to work?
If we follow the rationale of inductive generalization, we can explore the boundary conditions of a theory with every new empirical study. We thus widen the scope of our theory by comparing successful applications in different contexts and unsuccessful applications in similar contexts. This may ultimately lead to a more general theory, maybe even one of universal scope. However, unless we have such a general theory, we might be better off, if we treat unsuccessful replications not as a sign of failure, but as a chance to learn.
Author Contributions
MB conceived the original idea and wrote the first draft of the paper. MS helped to further elaborate and scrutinize the arguments. All authors contributed to the final version of the manuscript.
Conflict of Interest
The authors declare that the research was conducted in the absence of any commercial or financial relationships that could be construed as a potential conflict of interest.
Acknowledgments
We would like to thank Annette Scheunpflug for helpful comments on an earlier version of the manuscript.
1 A person × behavior matrix constitutes a very simple relational structure that is common in psychological research. This is why it is chosen here as a minimal example. However, more complex structures are possible, e.g., by relating individuals to behaviors over time, with individuals nested within groups etc. For a systematic overview, compare Coombs ( 1964 ).
2 This notion of empirical content applies only to deterministic models. The empirical content of a probabilistic model consists in the probability distribution over all possible empirical structures.
3 For example, neither the SAGE Handbook of qualitative data analysis edited by Flick ( 2014 ) nor the Oxford Handbook of Qualitative Research edited by Leavy ( 2014 ) mention formal approaches to category formation.
4 Note also that the described structure is empirically richer than a nominal scale. Therefore, a reduction of qualitative category formation to be a special (and somehow trivial) kind of measurement is not adequate.
5 It is possible to extend this notion of empirical content to the probabilistic case (this would correspond to applying a latent class analysis). But, since qualitative research usually does not rely on formal algorithms (neither deterministic nor probabilistic), there is currently little practical use of such a concept.
6 We do not elaborate on abductive reasoning here, since, given an empirical relational structure, the concept can be applied to both types of models in the same way (Schurz, 2008 ). One could argue that the underlying relational structure is not given a priori but has to be constructed by the researcher and will itself be influenced by theoretical expectations. Therefore, abductive reasoning may be necessary to establish an empirical relational structure in the first place.
7 We shall not elaborate on the metaphysical meaning of possible worlds here, since we are only concerned with empirical theories [but see Tooley ( 1999 ), for an overview].
8 Of course, this also means that it would be equally reasonable to employ a top-down strategy of generalization using a case-based model by postulating that □(∃ i : XYZ i ). The implications for case-based models are certainly worth exploring, but lie beyond the scope of this article.
- Agresti A. (2013). Categorical Data Analysis, 3rd Edn. Wiley Series In Probability And Statistics . Hoboken, NJ: Wiley. [ Google Scholar ]
- Borsboom D. (2005). Measuring the Mind: Conceptual Issues in Contemporary Psychometrics . Cambridge: Cambridge University Press; 10.1017/CBO9780511490026 [ CrossRef ] [ Google Scholar ]
- Braun V., Clarke V. (2006). Using thematic analysis in psychology . Qual. Res. Psychol . 3 , 77–101. 10.1191/1478088706qp063oa [ CrossRef ] [ Google Scholar ]
- Buntins M., Buntins K., Eggert F. (2017). Clarifying the concept of validity: from measurement to everyday language . Theory Psychol. 27 , 703–710. 10.1177/0959354317702256 [ CrossRef ] [ Google Scholar ]
- Carnap R. (1928). The Logical Structure of the World . Berkeley, CA: University of California Press. [ Google Scholar ]
- Coombs C. H. (1964). A Theory of Data . New York, NY: Wiley. [ Google Scholar ]
- Creswell J. W. (2015). A Concise Introduction to Mixed Methods Research . Los Angeles, CA: Sage. [ Google Scholar ]
- Dugard P., File P., Todman J. B. (2012). Single-Case and Small-N Experimental Designs: A Practical Guide to Randomization Tests 2nd Edn . New York, NY: Routledge; 10.4324/9780203180938 [ CrossRef ] [ Google Scholar ]
- Edgington E., Onghena P. (2007). Randomization Tests, 4th Edn. Statistics. Hoboken, NJ: CRC Press; 10.1201/9781420011814 [ CrossRef ] [ Google Scholar ]
- Everett J. A. C., Earp B. D. (2015). A tragedy of the (academic) commons: interpreting the replication crisis in psychology as a social dilemma for early-career researchers . Front. Psychol . 6 :1152. 10.3389/fpsyg.2015.01152 [ PMC free article ] [ PubMed ] [ CrossRef ] [ Google Scholar ]
- Flick U. (Ed.). (2014). The Sage Handbook of Qualitative Data Analysis . London: Sage; 10.4135/9781446282243 [ CrossRef ] [ Google Scholar ]
- Freeman M., Demarrais K., Preissle J., Roulston K., St. Pierre E. A. (2007). Standards of evidence in qualitative research: an incitement to discourse . Educ. Res. 36 , 25–32. 10.3102/0013189X06298009 [ CrossRef ] [ Google Scholar ]
- Ganter B. (2010). Two basic algorithms in concept analysis , in Lecture Notes In Computer Science. Formal Concept Analysis, Vol. 5986 , eds Hutchison D., Kanade T., Kittler J., Kleinberg J. M., Mattern F., Mitchell J. C., et al. (Berlin, Heidelberg: Springer Berlin Heidelberg; ), 312–340. 10.1007/978-3-642-11928-6_22 [ CrossRef ] [ Google Scholar ]
- Ganter B., Wille R. (1999). Formal Concept Analysis . Berlin, Heidelberg: Springer Berlin Heidelberg; 10.1007/978-3-642-59830-2 [ CrossRef ] [ Google Scholar ]
- Guttman L. (1944). A basis for scaling qualitative data . Am. Sociol. Rev . 9 :139 10.2307/2086306 [ CrossRef ] [ Google Scholar ]
- Hogg R. V., Mckean J. W., Craig A. T. (2013). Introduction to Mathematical Statistics, 7th Edn . Boston, MA: Pearson. [ Google Scholar ]
- Hughes G. E., Cresswell M. J. (1996). A New Introduction To Modal Logic . London; New York, NY: Routledge; 10.4324/9780203290644 [ CrossRef ] [ Google Scholar ]
- Klein R. A., Ratliff K. A., Vianello M., Adams R. B., Bahník Š., Bernstein M. J., et al. (2014). Investigating variation in replicability . Soc. Psychol. 45 , 142–152. 10.1027/1864-9335/a000178 [ CrossRef ] [ Google Scholar ]
- Krantz D. H., Luce D., Suppes P., Tversky A. (1971). Foundations of Measurement Volume I: Additive And Polynomial Representations . New York, NY; London: Academic Press; 10.1016/B978-0-12-425401-5.50011-8 [ CrossRef ] [ Google Scholar ]
- Leavy P. (2014). The Oxford Handbook of Qualitative Research . New York, NY: Oxford University Press; 10.1093/oxfordhb/9780199811755.001.0001 [ CrossRef ] [ Google Scholar ]
- Maxwell S. E., Lau M. Y., Howard G. S. (2015). Is psychology suffering from a replication crisis? what does “failure to replicate” really mean? Am. Psychol. 70 , 487–498. 10.1037/a0039400 [ PubMed ] [ CrossRef ] [ Google Scholar ]
- Miles M. B., Huberman A. M., Saldaña J. (2014). Qualitative Data Analysis: A Methods Sourcebook, 3rd Edn . Los Angeles, CA; London; New Delhi; Singapore; Washington, DC: Sage. [ Google Scholar ]
- Open Science, Collaboration (2015). Estimating the reproducibility of psychological science . Science 349 :Aac4716. 10.1126/science.aac4716 [ PubMed ] [ CrossRef ] [ Google Scholar ]
- Popper K. (1935). Logik Der Forschung . Wien: Springer; 10.1007/978-3-7091-4177-9 [ CrossRef ] [ Google Scholar ]
- Ragin C. (1987). The Comparative Method : Moving Beyond Qualitative and Quantitative Strategies . Berkeley, CA: University Of California Press. [ Google Scholar ]
- Rihoux B., Ragin C. (2009). Configurational Comparative Methods: Qualitative Comparative Analysis (Qca) And Related Techniques . Thousand Oaks, CA: Sage Publications, Inc; 10.4135/9781452226569 [ CrossRef ] [ Google Scholar ]
- Scheunpflug A., Krogull S., Franz J. (2016). Understanding learning in world society: qualitative reconstructive research in global learning and learning for sustainability . Int. Journal Dev. Educ. Glob. Learn. 7 , 6–23. 10.18546/IJDEGL.07.3.02 [ CrossRef ] [ Google Scholar ]
- Schurz G. (2008). Patterns of abduction . Synthese 164 , 201–234. 10.1007/s11229-007-9223-4 [ CrossRef ] [ Google Scholar ]
- Shrout P. E., Rodgers J. L. (2018). Psychology, science, and knowledge construction: broadening perspectives from the replication crisis . Annu. Rev. Psychol . 69 , 487–510. 10.1146/annurev-psych-122216-011845 [ PubMed ] [ CrossRef ] [ Google Scholar ]
- Smith P. (2020). An Introduction To Formal Logic . Cambridge: Cambridge University Press. 10.1017/9781108328999 [ CrossRef ] [ Google Scholar ]
- Suppes P., Krantz D. H., Luce D., Tversky A. (1971). Foundations of Measurement Volume II: Geometrical, Threshold, and Probabilistic Representations . New York, NY; London: Academic Press. [ Google Scholar ]
- Tooley M. (Ed.). (1999). Necessity and Possibility. The Metaphysics of Modality . New York, NY; London: Garland Publishing. [ Google Scholar ]
- Trafimow D. (2018). An a priori solution to the replication crisis . Philos. Psychol . 31 , 1188–1214. 10.1080/09515089.2018.1490707 [ CrossRef ] [ Google Scholar ]
- Watanabe S. (2018). Mathematical Foundations of Bayesian Statistics. CRC Monographs On Statistics And Applied Probability . Boca Raton, FL: Chapman And Hall. [ Google Scholar ]
- Wiggins B. J., Chrisopherson C. D. (2019). The replication crisis in psychology: an overview for theoretical and philosophical psychology . J. Theor. Philos. Psychol. 39 , 202–217. 10.1037/teo0000137 [ CrossRef ] [ Google Scholar ]
What is inductive coding in qualitative research?
Last updated
9 March 2023
Reviewed by
Jean Kaluza
Inductive coding is a data analysis process that involves reading and interpreting raw contextual data to develop themes, concepts, or a process model via interpretations based on data.
Inductive coding can also be defined as a bottom-up approach where you start with nothing, developing the code as you analyze the dataset.
Streamline data coding
Use global data tagging systems in Dovetail so everyone analyzing research is speaking the same language
- How is inductive coding different from deductive coding?
The main difference between inductive and deductive coding is that inductive reasoning aims to develop a theory, whereas deductive reasoning aims to test an existing theory.
- Is qualitative coding inductive or deductive?
Qualitative coding can involve inductive coding, deductive coding, or a combination of both.
- Decide on an approach before coding
Before coding, it is important to decide if you want to develop the codes as you analyze the data (inductive), start with a set of codes and stick with them (deductive), or use a combination approach.
What is inductive coding?
Inductive coding is a ground-up approach where a researcher derives codes from the data. Researchers typically don't start with preconceived notions of what the codes ought to be, allowing the theory or narrative to emerge from the raw data.
This is ideal for exploratory research or times when researchers want to develop new theories, concepts, or ideas.
What is deductive coding?
This is a top-down approach where a researcher starts by developing a codebook with an initial set of codes. The set of codes could be based on the individual's research questions or an existing research framework/theory.
The researcher then reads through the data and assigns excerpts to codes. After analysis, the codes should still closely resemble the codebook they started with. This approach is ideal when a researcher has a predetermined structure for how they need their final findings to be.
- Steps in inductive coding
The inductive approach typically consists of three stages:
Observation: for example, a user can’t find the purchase button.
Seeking patterns: for example, four-fifths of users tested couldn’t find the purchase button.
Developing a theory or general (preliminary) conclusion: for example, the purchase button needs to be redesigned or repositioned to be found more easily.
Let’s look at the steps in inductive coding (how inductive coding works):
Start by breaking down the qualitative data set into smaller samples.
Read a sample of the data.
Develop codes to cover the sample.
Reread the samples, then apply the codes.
Read a new data sample and simultaneously apply the codes you developed for the first sample.
Take note of where codes don't match or if you need extra codes.
Develop new codes based on the second sample.
Recode all responses again.
If you add a new code, split an existing code into two, or change a code's description, review how this change will affect the coding of all responses. Similar responses at different points in the survey could result in different codes.
Inductive coding is an iterative process. It takes longer and is more thorough than deductive coding. Regardless, inductive coding provides a more complete, unbiased view of the themes throughout the data.
- Combining inductive and deductive coding approaches
Research studies typically combine deductive and inductive approaches to coding. You can deductively start with a set of codes, then inductively develop new ones and iterate on the existing codes as you sift through the data.
Researchers working on large projects typically start with an inductive approach to develop a relevant research topic and develop a strong working theory. Deductive research then follows to invalidate or confirm the conclusion. This can formulate a more structured project. It can also help to mitigate the risk of research bias creeping into the work.
Like any other qualitative research method , both deductive and inductive approaches are at risk of research bias, especially cognitive bias, and confirmation bias.
Inductive coding is an important approach in qualitative research. It allows the analysis of data when knowledge is limited.
Get started today
Go from raw data to valuable insights with a flexible research platform
Editor’s picks
Last updated: 21 December 2023
Last updated: 16 December 2023
Last updated: 6 October 2023
Last updated: 5 March 2024
Last updated: 25 November 2023
Last updated: 15 February 2024
Last updated: 11 March 2024
Last updated: 12 December 2023
Last updated: 6 March 2024
Last updated: 10 April 2023
Last updated: 20 December 2023
Latest articles
Related topics, log in or sign up.
Get started for free
Mastering Deductive Reasoning in Qualitative Research
Introduction.
Deductive reasoning is a fundamental aspect of qualitative research that serves as a guiding light in the quest to understand complex phenomena. It’s a structured approach that involves starting with a theory or hypothesis and then systematically collecting and analyzing data to test or confirm that theory. In the realm of qualitative research, this method enables researchers to explore existing knowledge and apply it to specific cases, helping to derive valuable insights. To truly master the art of deductive reasoning in qualitative research, one must understand its principles, techniques, and applications. In this article, we’ll delve into the world of deductive reasoning and explore its significance in qualitative research.
The Basics of Deductive Reasoning
Deductive reasoning operates on the premise that if a theory is true and relevant to a specific context, then certain observations or data points should support it. In qualitative research, this begins with the formulation of a clear and testable hypothesis. Researchers then gather data that is relevant to the hypothesis and analyze it systematically to draw conclusions. The process generally follows these steps:
- Develop a Clear Hypothesis: Deductive reasoning starts with a well-defined hypothesis or theory. This hypothesis serves as the foundation for the research and sets the direction for data collection and analysis.
- Collect Relevant Data : Researchers collect data through various methods, such as interviews, surveys, observations, or document analysis. The data collected should directly relate to the hypothesis or theory under investigation.
- Analyze the Data: The collected data is analyzed using appropriate qualitative research methods, such as thematic analysis, content analysis, or grounded theory. Researchers look for patterns, themes, or relationships that either support or refute the hypothesis.
- Draw Conclusions: Based on the analysis, researchers draw conclusions about the hypothesis. If the data supports the hypothesis, it is considered confirmed. If not, researchers may need to modify the hypothesis or explore alternative explanations.
- Refine and Iterate: Deductive reasoning often involves an iterative process. Researchers refine their hypothesis and repeat the data collection and analysis until they achieve a satisfactory level of confirmation or understanding.
Applications in Qualitative Research
Deductive reasoning finds numerous applications in qualitative research across various disciplines, including sociology, psychology, anthropology, and education. Here are some key applications:
- Theory Testing: Qualitative researchers often use deductive reasoning to test existing theories or concepts in real-world contexts. By applying established theories to specific cases, researchers can assess their validity and relevance.
- Hypothesis Confirmation: Deductive reasoning allows researchers to confirm or reject hypotheses based on empirical evidence. This helps in advancing knowledge and theory development.
- Comparative Analysis: Researchers can use deductive reasoning to compare and contrast findings across different cases or contexts. This approach enables a deeper understanding of the factors influencing a phenomenon.
- Policy Evaluation: In fields like public policy and social sciences, deductive reasoning can be applied to evaluate the effectiveness of policies or interventions by comparing them to established theories or expectations.
- Conceptual Frameworks: Deductive reasoning aids in the development of conceptual frameworks that guide qualitative research projects. These frameworks help structure the research process and ensure that data collection and analysis align with the research goals.
Resources for Further Learning
To truly master deductive reasoning in qualitative research, it’s essential to delve deeper into the methodology and practice. If you’re looking for comprehensive guidance and practical tips, consider visiting the dedicated resource on deductive reasoning in qualitative research at https://atlasti.com/guides/qualitative-research-guide-part-2/deductive-reasoning .
Deductive reasoning is a powerful tool in the qualitative researcher’s arsenal. It allows researchers to apply existing theories and concepts to real-world situations, facilitating a deeper understanding of complex phenomena. By following a structured process of hypothesis formulation, data collection, analysis, and conclusion drawing, qualitative researchers can harness the full potential of deductive reasoning. Whether you are a seasoned researcher or just starting your journey in qualitative research, mastering deductive reasoning will undoubtedly enhance the depth and quality of your research endeavors.
- Open access
- Published: 02 April 2022
A qualitative study of rural healthcare providers’ views of social, cultural, and programmatic barriers to healthcare access
- Nicholas C. Coombs 1 ,
- Duncan G. Campbell 2 &
- James Caringi 1
BMC Health Services Research volume 22 , Article number: 438 ( 2022 ) Cite this article
22k Accesses
19 Citations
10 Altmetric
Metrics details
Ensuring access to healthcare is a complex, multi-dimensional health challenge. Since the inception of the coronavirus pandemic, this challenge is more pressing. Some dimensions of access are difficult to quantify, namely characteristics that influence healthcare services to be both acceptable and appropriate. These link to a patient’s acceptance of services that they are to receive and ensuring appropriate fit between services and a patient’s specific healthcare needs. These dimensions of access are particularly evident in rural health systems where additional structural barriers make accessing healthcare more difficult. Thus, it is important to examine healthcare access barriers in rural-specific areas to understand their origin and implications for resolution.
We used qualitative methods and a convenience sample of healthcare providers who currently practice in the rural US state of Montana. Our sample included 12 healthcare providers from diverse training backgrounds and specialties. All were decision-makers in the development or revision of patients’ treatment plans. Semi-structured interviews and content analysis were used to explore barriers–appropriateness and acceptability–to healthcare access in their patient populations. Our analysis was both deductive and inductive and focused on three analytic domains: cultural considerations, patient-provider communication, and provider-provider communication. Member checks ensured credibility and trustworthiness of our findings.
Five key themes emerged from analysis: 1) a friction exists between aspects of patients’ rural identities and healthcare systems; 2) facilitating access to healthcare requires application of and respect for cultural differences; 3) communication between healthcare providers is systematically fragmented; 4) time and resource constraints disproportionately harm rural health systems; and 5) profits are prioritized over addressing barriers to healthcare access in the US.
Conclusions
Inadequate access to healthcare is an issue in the US, particularly in rural areas. Rural healthcare consumers compose a hard-to-reach patient population. Too few providers exist to meet population health needs, and fragmented communication impairs rural health systems’ ability to function. These issues exacerbate the difficulty of ensuring acceptable and appropriate delivery of healthcare services, which compound all other barriers to healthcare access for rural residents. Each dimension of access must be monitored to improve patient experiences and outcomes for rural Americans.
Peer Review reports
Unequal access to healthcare services is an important element of health disparities in the United States [ 1 ], and there remains much about access that is not fully understood. The lack of understanding is attributable, in part, to the lack of uniformity in how access is defined and evaluated, and the extent to which access is often oversimplified in research [ 2 ]. Subsequently, attempts to address population-level barriers to healthcare access are insufficient, and access remains an unresolved, complex health challenge [ 3 , 4 , 5 ]. This paper presents a study that aims to explore some of the less well studied barriers to healthcare access, particularly those that influence healthcare acceptability and appropriateness.
In truth, healthcare access entails a complicated calculus that combines characteristics of individuals, their households, and their social and physical environments with characteristics of healthcare delivery systems, organizations, and healthcare providers. For one to fully ‘access’ healthcare, they must have the means to identify their healthcare needs and have available to them care providers and the facilities where they work. Further, patients must then reach, obtain, and use the healthcare services in order to have their healthcare needs fulfilled. Levesque and colleagues critically examined access conceptualizations in 2013 and synthesized all ways in which access to healthcare was previously characterized; Levesque et al. proposed five dimensions of access: approachability, acceptability, availability, affordability and appropriateness [ 2 ]. These refer to the ability to perceive, seek, reach, pay for, and engage in services, respectively.
According to Levesque et al.’s framework, the five dimensions combine to facilitate access to care or serve as barriers. Approachability indicates that people facing health needs understand that healthcare services exist and might be helpful. Acceptability represents whether patients see healthcare services as consistent or inconsistent with their own social and cultural values and worldviews. Availability indicates that healthcare services are reached both physically and in a timely manner. Affordability simplifies one’s capacity to pay for healthcare services without compromising basic necessities, and finally, appropriateness represents the fit between healthcare services and a patient’s specific healthcare needs [ 2 ]. This study focused on the acceptability and appropriateness dimensions of access.
Before the novel coronavirus (SARS-CoV-2; COVID-19) pandemic, approximately 13.3% of adults in the US did not have a usual source of healthcare [ 6 ]. Millions more did not utilize services regularly, and close to two-thirds reported that they would be debilitated by an unexpected medical bill [ 7 , 8 , 9 ]. Findings like these emphasized a fragility in the financial security of the American population [ 10 ]. These concerns were exacerbated by the pandemic when a sudden surge in unemployment increased un- and under-insurance rates [ 11 ]. Indeed, employer-sponsored insurance covers close to half of Americans’ total cost of illness [ 12 ]. Unemployment linked to COVID-19 cut off the lone outlet to healthcare access for many. Health-related financial concerns expanded beyond individuals, as healthcare organizations were unequipped to manage a simultaneous increase in demand for specialized healthcare services and a steep drop off for routine revenue-generating healthcare services [ 13 ]. These consequences of the COVID-19 pandemic all put additional, unexpected pressure on an already fragmented US healthcare system.
Other structural barriers to healthcare access exist in relation to the rural–urban divide. Less than 10% of US healthcare resources are located in rural areas where approximately 20% of the American population resides [ 14 ]. In a country with substantially fewer providers per capita compared to many other developed countries, persons in rural areas experience uniquely pressing healthcare provider shortages [ 15 , 16 ]. Rural inhabitants also tend to have lower household income, higher rates of un- or under-insurance, and more difficulty with travel to healthcare clinics than urban dwellers [ 17 ]. Subsequently, persons in rural communities use healthcare services at lower rates, and potentially preventable hospitalizations are more prevalent [ 18 ]. This disparity often leads rural residents to use services primarily for more urgent needs and less so for routine care [ 19 , 20 , 21 ].
The differences in how rural and urban healthcare systems function warranted a federal initiative to focus exclusively on rural health priorities and serve as counterpart to Healthy People objectives [ 22 ]. The rural determinants of health, a more specific expression of general social determinants, add issues of geography and topography to the well-documented social, economic and political factors that influence all Americans’ access to healthcare [ 23 ]. As a result, access is consistently regarded as a top priority in rural areas, and many research efforts have explored the intersection between access and rurality, namely within its less understood dimensions (acceptability and appropriateness) [ 22 ].
Acceptability-related barriers to care
Acceptability represents the dimension of healthcare access that affects a patient’s ability to seek healthcare, particularly linked to one’s professional values, norms and culture [ 2 ]. Access to health information is an influential factor for acceptable healthcare and is essential to promote and maintain a healthy population [ 24 ]. According to the Centers for Disease Control and Prevention, health literacy or a high ‘health IQ’ is the degree to which individuals have the ability to find, understand, and use information and services to inform health-related decisions and actions for themselves and others, which impacts healthcare use and system navigation [ 25 ]. The literature indicates that lower levels of health literacy contribute to health disparities among rural populations [ 26 , 27 , 28 ]. Evidence points to a need for effective health communication between healthcare organizations and patients to improve health literacy [ 24 ]. However, little research has been done in this area, particularly as it relates to technologically-based interventions to disseminate health information [ 29 ].
Stigma, an undesirable position of perceived diminished status in an individual’s social position, is another challenge that influences healthcare acceptability [ 30 ]. Those who may experience stigma fear negative social consequences in relation to care seeking. They are more likely to delay seeking care, especially among ethnic minority populations [ 31 , 32 ]. Social media presents opportunities for the dissemination of misleading medical information; this runs further risk for stigma [ 33 ]. Stigma is difficult to undo, but research has shown that developing a positive relationship with a healthcare provider or organization can work to reduce stigma among patients, thus promoting healthcare acceptability [ 34 ].
A provider’s attempts to engage patients and empower them to be active decision-makers regarding their treatment has also been shown to improve healthcare acceptability. One study found that patients with heart disease who completed a daily diary of weight and self-assessment of symptoms, per correspondence with their provider, had better care outcomes than those who did not [ 35 ]. Engaging with household family members and involved community healers also mitigates barriers to care, emphasizing the importance of a team-based approach that extends beyond those who typically provide healthcare services [ 36 , 37 ]. One study, for instance, explored how individuals closest to a pregnant woman affect the woman’s decision to seek maternity care; partners, female relatives, and community health-workers were among the most influential in promoting negative views, all of which reduced a woman’s likelihood to access care [ 38 ].
Appropriateness-related barriers to care
Appropriateness marks the dimension of healthcare access that affects a patient’s ability to engage, and according to Levesque et al., is of relevance once all other dimensions (the ability to perceive, seek, reach and pay for) are achieved [ 2 ]. The ability to engage in healthcare is influenced by a patient’s level of empowerment, adherence to information, and support received by their healthcare provider. Thus, barriers to healthcare access that relate to appropriateness are often those that indicate a breakdown in communication between a patient with their healthcare provider. Such breakdown can involve a patient experiencing miscommunication, confrontation, and/or a discrepancy between their provider’s goals and their own goals for healthcare. Appropriateness represents a dimension of healthcare access that is widely acknowledged as an area in need of improvement, which indicates a need to rethink how healthcare providers and organizations can adapt to serve the healthcare needs of their communities [ 39 ]. This is especially true for rural, ethnic minority populations, which disproportionately experience an abundance of other barriers to healthcare access. Culturally appropriate care is especially important for members of minority populations [ 40 , 41 , 42 ]. Ultimately, patients value a patient-provider relationship characterized by a welcoming, non-judgmental atmosphere [ 43 , 44 ]. In rural settings especially, level of trust and familiarity are common factors that affect service utilization [ 45 ]. Evidence suggests that kind treatment by a healthcare provider who promotes patient-centered care can have a greater overall effect on a patient’s experience than a provider’s degree of medical knowledge or use of modern equipment [ 46 ]. Of course, investing the time needed to nurture close and caring interpersonal connections is particularly difficult in under-resourced, time-pressured rural health systems [ 47 , 48 ].
The most effective way to evaluate access to healthcare largely depends on which dimensions are explored. For instance, a population-based survey can be used to measure the barrier of healthcare affordability. Survey questions can inquire directly about health insurance coverage, care-related financial burden, concern about healthcare costs, and the feared financial impacts of illness and/or disability. Many national organizations have employed such surveys to measure affordability-related barriers to healthcare. For example, a question may ask explicitly about financial concerns: ‘If you get sick or have an accident, how worried are you that you will not be able to pay your medical bills?’ [ 49 ]. Approachability and availability dimensions of access are also studied using quantitative analysis of survey questions, such as ‘Is there a place that you usually go to when you are sick or need advice about your health?’ or ‘Have you ever delayed getting medical care because you couldn’t get through on the telephone?’ In contrast, the remaining two dimensions–acceptability and appropriateness–require a qualitative approach, as the social and cultural factors that determine a patient’s likelihood of accepting aspects of the services that are to be received (acceptability) and the fit between those services and the patient’s specific healthcare needs (appropriateness) can be more abstract [ 50 , 51 ]. In social science, qualitative methods are appropriate to generate knowledge of what social events mean to individuals and how those individuals interact within them; these methods allow for an exploration of depth rather than breadth [ 52 , 53 ]. Qualitative methods, therefore, are appropriate tools for understanding the depth of healthcare providers’ experiences in the inherently social context of seeking and engaging in healthcare.
In sum, acceptability- and appropriateness-related barriers to healthcare access are multi-layered, complex and abundant. Ensuring access becomes even more challenging if structural barriers to access are factored in. In this study, we aimed to explore barriers to healthcare access among persons in Montana, a historically underserved, under-resourced, rural region of the US. Montana is the fourth largest and third least densely populated state in the country; more than 80% of Montana counties are classified as non-core (the lowest level of urban/rural classification), and over 90% are designated as health professional shortage areas [ 54 , 55 ]. Qualitative methods supported our inquiry to explore barriers to healthcare access related to acceptability and appropriateness.
Participants
Qualitative methods were utilized for this interpretive, exploratory study because knowledge regarding barriers to healthcare access within Montana’s rural health systems is limited. We chose Montana healthcare providers, rather than patients, as the population of interest so we may explore barriers to healthcare access from the perspective of those who serve many persons in rural settings. Inclusion criteria required study participants to provide direct healthcare to patients at least one-half of their time. We defined ‘provider’ as a healthcare organization employee with clinical decision-making power and the qualifications to develop or revise patients’ treatment plans. In an attempt to capture a group of providers with diverse experience, we included providers across several types and specialties. These included advanced practice registered nurses (APRNs), physicians (MDs and DOs), and physician assistants (PAs) who worked in critical care medicine, emergency medicine, family medicine, hospital medicine, internal medicine, pain medicine, palliative medicine, pediatrics, psychiatry, and urgent care medicine. We also included licensed clinical social workers (LCSWs) and clinical psychologists who specialize in behavioral healthcare provision.
Recruitment and Data Collection
We recruited participants via email using a snowball sampling approach [ 56 ]. We opted for this approach because of its effectiveness in time-pressured contexts, such as the COVID-19 pandemic, which has made healthcare provider populations hard to reach [ 57 ]. Considering additional constraints with the pandemic and the rural nature of Montana, interviews were administered virtually via Zoom video or telephone conferencing with Zoom’s audio recording function enabled. All interviews were conducted by the first author between January and September 2021. The average length of interviews was 50 min, ranging from 35 to 70 min. There were occasional challenges experienced during interviews (poor cell phone reception from participants, dropped calls), in which case the interviewer remained on the line until adequate communication was resumed. All interviews were included for analysis and transcribed verbatim into NVivo Version 12 software. All qualitative data were saved and stored on a password-protected University of Montana server. Hard-copy field notes were securely stored in a locked office on the university’s main campus.
Data analysis included a deductive followed by an inductive approach. This dual analysis adheres to Levesque’s framework for qualitative methods, which is discussed in the Definition of Analytic Domains sub-section below. Original synthesis of the literature informed the development of our initial deductive codebook. The deductive approach was derived from a theory-driven hypothesis, which consisted of synthesizing previous research findings regarding acceptability- and appropriateness-related barriers to care. Although the locations, patient populations and specific type of healthcare services varied by study in the existing literature, several recurring barriers to healthcare access were identified. We then operationalized three analytic domains based on these findings: cultural considerations, patient-provider communication, and provider-provider communication. These domains were chosen for two reasons: 1) the terms ‘culture’ and ‘communication’ were the most frequently documented characteristics across the studies examined, and 2) they each align closely with the acceptability and appropriateness dimensions of access to healthcare, respectively. In addition, ‘culture’ is included in the definition of acceptability and ‘communication’ is a quintessential aspect of appropriateness. These domains guided the deductive portion of our analysis, which facilitated the development of an interview guide used for this study.
Interviews were semi-structured to allow broad interpretations from participants and expand the open-ended characterization of study findings. Data were analyzed through a flexible coding approach proposed by Deterding and Waters [ 58 ]. Qualitative content analysis was used, a method particularly beneficial for analyzing large amounts of qualitative data collected through interviews that offers possibility of quantifying categories to identify emerging themes [ 52 , 59 ]. After fifty percent of data were analyzed, we used an inductive approach as a formative check and repeated until data saturation, or the point at which no new information was gathered in interviews [ 60 ]. At each point of inductive analysis, interview questions were added, removed, or revised in consideration of findings gathered [ 61 ]. The Standards for Reporting Qualitative Research (SRQR) was used for reporting all qualitative data for this study [ 62 ]. The first and third authors served as primary and secondary analysts of the qualitative data and collaborated to triangulate these findings. An audit approach was employed, which consisted of coding completed by the first author and then reviewed by the third author. After analyses were complete, member checks ensured credibility and trustworthiness of findings [ 63 ]. Member checks consisted of contacting each study participant to explain the study’s findings; one-third of participants responded and confirmed all findings. All study procedures were reviewed and approved by the Human Subjects Committee of the authors’ institution’s Institutional Review Board.
Definitions of Analytic Domains
Cultural considerations.
Western health systems often fail to consider aspects of patients’ cultural perspectives and histories. This can manifest in the form of a providers’ lack of cultural humility. Cultural humility is a process of preventing imposition of one’s worldview and cultural beliefs on others and recognizing that everyone’s conception of the world is valid. Humility cultivates sensitive approaches in treating patients [ 64 ]. A lack of cultural humility impedes the delivery of acceptable and appropriate healthcare [ 65 ], which can involve low empathy or respect for patients, or dismissal of culture and traditions as superstitions that interfere with standard treatments [ 66 , 67 ]. Ensuring cultural humility among all healthcare employees is a step toward optimal healthcare delivery. Cultural humility is often accomplished through training that can be tailored to particular cultural- or gender-specific populations [ 68 , 69 ]. Since cultural identities and humility have been marked as factors that can heavily influence patients’ access to care, cultural considerations composed our first analytic domain. To assess this domain, we asked participants how they address the unique needs of their patients, how they react when they observe a cultural behavior or attitude from a patient that may not directly align with their treatment plan, and if they have received any multicultural training or training on cultural considerations in their current role.
Patient-provider communication
Other barriers to healthcare access can be linked to ineffective patient-provider communication. Patients who do not feel involved in healthcare decisions are less likely to adhere to treatment recommendations [ 70 ]. Patients who experience communication difficulties with providers may feel coerced, which generates disempowerment and leads patients to employ more covert ways of engagement [ 71 , 72 ]. Language barriers can further compromise communication and hinder outcomes or patient progress [ 73 , 74 ]. Any miscommunication between a patient and provider can affect one’s access to healthcare, namely affecting appropriateness-related barriers. For these reasons, patient-provider communication composed our second analytic domain. We asked participants to highlight the challenges they experience when communicating with their patients, how those complications are addressed, and how communication strategies inform confidentiality in their practice. Confidentiality is a core ethical principle in healthcare, especially in rural areas that have smaller, interconnected patient populations [ 75 ].
Provider-Provider Communication
A patient’s journey through the healthcare system necessitates sufficient correspondence between patients, primary, and secondary providers after discharge and care encounters [ 76 ]. Inter-provider and patient-provider communication are areas of healthcare that are acknowledged to have some gaps. Inconsistent mechanisms for follow up communication with patients in primary care have been documented and emphasized as a concern among those with chronic illness who require close monitoring [ 68 , 77 ]. Similar inconsistencies exist between providers, which can lead to unclear care goals, extended hospital stays, and increased medical costs [ 78 ]. For these reasons, provider-provider communication composed our third analytic domain. We asked participants to describe the approaches they take to streamline communication after a patient’s hospital visit, the methods they use to ensure collaborative communication between primary or secondary providers, and where communication challenges exist.
Healthcare provider characteristics
Our sample included 12 providers: four in family medicine (1 MD, 1 DO, 1 PA & 1 APRN), three in pediatrics (2 MD with specialty in hospital medicine & 1 DO), three in palliative medicine (2 MDs & 1 APRN with specialty in wound care), one in critical care medicine (DO with specialty in pediatric pulmonology) and one in behavioral health (1 LCSW with specialty in trauma). Our participants averaged 9 years (range 2–15) as a healthcare provider; most reported more than 5 years in their current professional role. The diversity of participants extended to their patient populations as well, with each participant reporting a unique distribution of age, race and level of medical complexity among their patients. Most participants reported that a portion of their patients travel up to five hours, sometimes across county- or state-lines, to receive care.
Theme 1: A friction exists between aspects of patients’ rural identities and healthcare systems
Our participants comprised a collection of medical professions and reported variability among health-related reasons their patients seek care. However, most participants acknowledged similar characteristics that influence their patients’ challenges to healthcare access. These identified factors formed categories from which the first theme emerged. There exists a great deal of ‘rugged individualism’ among Montanans, which reflects a self-sufficient and self-reliant way of life. Stoicism marked a primary factor to characterize this quality. One participant explained:
True Montanans are difficult to treat medically because they tend to be a tough group. They don’t see doctors. They don’t want to go, and they don’t want to be sick. That’s an aspect of Montana that makes health culture a little bit difficult.
Another participant echoed this finding by stating:
The backwoods Montana range guy who has an identity of being strong and independent probably doesn’t seek out a lot of medical care or take a lot of medications. Their sense of vitality, independence and identity really come from being able to take care and rely on themselves. When that is threatened, that’s going to create a unique experience of illness.
Similar responses were shared by all twelve participants; stoicism seemed to be heavily embedded in many patient populations in Montana and serves as a key determinant of healthcare acceptability. There are additional factors, however, that may interact with stoicism but are multiply determined. Stigma is an example of this, presented in this context as one’s concern about judgement by the healthcare system. Respondents were openly critical of this perception of the healthcare system as it was widely discussed in interviews. One participant stated:
There is a real perception of a punitive nature in the medical community, particularly if I observe a health issue other than the primary reason for one’s hospital visit, whether that may be predicated on medical neglect, delay of care, or something that may warrant a report to social services. For many of the patients and families I see, it’s not a positive experience and one that is sometimes an uphill barrier that I work hard to circumnavigate.
Analysis of these factors suggest that low use of healthcare services may link to several characteristics, including access problems. Separately, a patient’s perceived stigma from healthcare providers may also impact a patient’s willingness to receive services. One participant put it best by stating
Sometimes, families assume that I didn’t want to see them because they will come in for follow up to meet with me but end up meeting with another provider, which is frustrating because I want to maintain patients on my panel but available time and resource occasionally limits me from doing so. It could be really hard adapting to those needs on the fly, but it’s an honest miss.
When a patient arrives for a healthcare visit and experiences this frustration, it may elicit a patient’s perceptions of neglect or disorganization. This ‘honest miss’ may, in turn, exacerbate other acceptable-related barriers to care.
Theme 2: Facilitating access to healthcare requires application of and respect for cultural differences
The biomedical model is the standard of care utilized in Western medicine [ 79 , 80 ]. However, the US comprises people with diverse social and cultural identities that may not directly align with Western conceptions of health and wellness. Approximately 11.5% of the Montana population falls within an ethnic minority group. 6.4% are of American Indian or Alaska Native origin, 0.5% are of Black or African American origin, 0.8% are of Asian origin and 3.8% are of multiple or other origins. [ 81 ]. Cultural insensitivity is acknowledged in health services research as an active deterrent for appropriate healthcare delivery [ 65 ]. Participants for this study were asked how they react when a patient brings up a cultural attitude or behavior that may impact the proposed treatment plan. Eight participants noted a necessity for humility when this occurs. One participant conceptualized this by stating:
When this happens, I learn about individuals and a way of life that is different to the way I grew up. There is a lot of beauty and health in a non-patriarchal, non-dominating, non-sexist framework, and when we can engage in such, it is really expansive for my own learning process.
The participants who expressed humility emphasized that it is best to work in tandem with their patient, congruently. Especially for those with contrasting worldviews, a provider and a patient working as a team poses an opportunity to develop trust. Without it, a patient can easily fall out of the system, further hindering their ability to access healthcare services in the future. One participant stated:
The approach that ends up being successful for a lot of patients is when we understand their modalities, and they have a sense we understand those things. We have to show understanding and they have to trust. From there, we can make recommendations to help get them there, not decisions for them to obey, rather views based on our experiences and understanding of medicine.
Curiosity was another reaction noted by a handful of participants. One participant said:
I believe patients and their caregivers can be engaged and loving in different ways that don’t always follow the prescribed approach in the ways I’ve been trained, but that doesn’t necessarily mean that they are detrimental. I love what I do, and I love learning new things or new approaches, but I also love being surprised. My style of medicine is not to predict peoples’ lives, rather to empower and support what makes life meaningful for them.
Participants mentioned several other characteristics that they use in practice to prevent cultural insensitivity and support a collaborative approach to healthcare. Table 1 lists these facilitating characteristics and quotes to explain the substance of their benefit.
Consensus among participants indicated that the use of these protective factors to promote cultural sensitivity and apply them in practice is not standardized. When asked, all but two participants said they had not received any culturally-based training since beginning their practice. Instead, they referred to developing skills through “on the job training” or “off the cuff learning.” The general way of medicine, one participant remarked, was to “throw you to the fire.” This suggested that use of standardized cultural humility training modules for healthcare providers was not common practice. Many attributed this to time constraints.
Individual efforts to gain culturally appropriate skills or enhance cultural humility were mentioned, however. For example, three participants reported that they attended medical conferences to discuss cultural challenges within medicine, one participant sought out cultural education within their organization, and another was invited by Native American community members to engage in traditional peace ceremonies. Participants described these additional efforts as uncommon and outside the parameters of a provider’s job responsibilities, as they require time commitments without compensation.
Additionally, eight participants said they share their personal contact information with patients so they may call them directly for medical needs. The conditions and frequency with which this is done was variable and more common among providers in specialized areas of medicine or those who described having a manageable patient panel. All who reported that they shared their personal contact information described it as an aspect of rural health service delivery that is atypical in other, non-rural healthcare systems.
Theme 3: Communication between healthcare providers is systematically fragmented
Healthcare is complex and multi-disciplinary, and patients’ treatment is rarely overseen by a single provider [ 82 ]. The array of provider types and specialties is vast, as is the range of responsibilities ascribed to providers. Thus, open communication among providers both within and between healthcare systems is vital for the success of collaborative healthcare [ 83 ]. Without effective communication achieved between healthcare providers, the appropriate delivery of healthcare services may be become compromised. Our participants noted that they face multiple challenges that complicate communication with other providers. Miscommunication between departments, often implicating the Emergency Department (ED), was a recurring point noted among participants. One participant who is a primary care physician said:
If one of my patients goes to the ER, I don’t always get the notes. They’re supposed to send them to the patient’s primary care doc. The same thing happens with general admissions, but again, I often find out from somebody else that my patient was admitted to the hospital.
This failure to communicate can negatively impact the patient, particularly if time sensitivity or medical complexity is essential to treatment. A patient’s primary care physician is the most accurate source of their medical history; without an effective way to obtain and synthesize a patient’s health information, there may be increased risk of medical error. One participant in a specialty field stated:
One of the biggest barriers I see is obtaining a concise description of a patient’s history and needs. You can imagine if you’re a mom and you’ve got a complicated kid. You head to the ER. The ER doc looks at you with really wide eyes, not knowing how to get information about your child that’s really important.
This concern was highlighted with a specific example from a different participant:
I have been unable to troubleshoot instances when I send people to the ER with a pretty clear indication for admission, and then they’re sent home. For instance, I had an older fellow with pretty severe chronic kidney disease. He presented to another practitioner in my office with shortness of breath and swelling and appeared to have newly onset decompensated heart failure. When I figured this out, I sent him to the ER, called and gave my report. The patient later came back for follow up to find out not only that they had not been admitted but they lost no weight with outpatient dialysis . I feel like a real opportunity was missed to try to optimize the care of the patient simply because there was poor communication between myself and the ER. This poor guy… He ended up going to the ER four times before he got admitted for COVID-19.
In some cases, communication breakdown was reported as the sole cause of a poor outcome. When communication is effective, each essential member of the healthcare team is engaged and collaborating with the same information. Some participants called this process ‘rounds’ when a regularly scheduled meeting is staged between a group of providers to ensure access to accurate patient information. Accurate communication may also help build trust and improve a patient’s experience. In contrast, ineffective communication can result in poor clarity regarding providers’ responsibilities or lost information. Appropriate delivery of healthcare considers the fit between providers and a patient’s specific healthcare needs; the factors noted here suggest that provider-provider miscommunication can adversely affect this dimension of healthcare access.
Another important mechanism of communication is the sharing of electronic medical records (EMRs), a process that continues to shift with technological advances. Innovation is still recent enough, however, for several of our study participants to be able to recall a time when paper charts were standard. Widespread adoption and embrace of the improvements inherent in electronic medical records expanded in the late 2000’s [ 84 ]. EMRs vastly improved the ability to retain, organize, safeguard, and transfer health information. Every participant highlighted EMRs at one point or another and often did so with an underlying sense of anger or frustration. Systematic issues and problems with EMRs were discussed. One participant provided historical context to such records:
Years back, the government aimed to buy an electronic medical record system, whichever was the best, and a number of companies created their own. Each were a reasonable system, so they all got their checks and now we have four completely separate operating systems that do not talk to each other. The idea was to make a router or some type of relay that can share information back and forth. There was no money in that though, so of course, no one did anything about it. Depending on what hospital, clinic or agency you work for, you will most likely work within one of these systems. It was a great idea; it just didn’t get finished.
Seven participants confirmed these points and their impacts on making coordination more difficult, relying on outdated communication strategies more often than not. Many noted this even occurs between facilities within the same city and in separate small metropolitan areas across the state. One participant said:
If my hospital decides to contract with one EMR and the hospital across town contracts with another, correspondence between these hospitals goes back to traditional faxing. As a provider, you’re just taking a ‘fingered crossed’ approach hoping that the fax worked, is picked up, was put in the appropriate inbox and was actually looked at. Information acquisition and making sure it’s timely are unforeseen between EMRs.
Participants reported an “astronomic” number of daily faxes and telephone calls to complete the communication EMRs were initially designed to handle. These challenges are even more burdensome if a patient moves from out of town or out of state; obtaining their medical records was repeatedly referred to as a “chore” so onerous that it often remains undone. Another recurring concern brought up by participants regarded accuracy within EMRs to lend a false sense of security. They are not frequently updated, not designed to be family-centered and not set up to do anything automatically. One participant highlighted these limitations by stating:
I was very proud of a change I made in our EMR system [EPIC], even though it was one I never should have had to make. I was getting very upset because I would find out from my nursing assistant who read the obituary that one of my patients had died. There was a real problem with the way the EMR was notifying PCP’s, so I got an EPIC-level automated notification built into our EMR so that any time a patient died, their status would be changed to deceased and a notification would be sent to their PCP. It’s just really awful to find out a week later that your patient died, especially when you know these people and their families really well. It’s not good care to have blind follow up.
Whether it be a physical or electronic miscommunication between healthcare providers, the appropriate delivery of healthcare can be called to question
Theme 4: Time and resource constraints disproportionately harm rural health systems
Several measures of system capacity suggest the healthcare system in the US is under-resourced. There are fewer physicians and hospital beds per capita compared to most comparable countries, and the growth of healthcare provider populations has stagnated over time [ 15 ]. Rural areas, in particular, are subject to resource limitations [ 16 ]. All participants discussed provider shortages in detail. They described how shortages impact time allocation in their day-to-day operations. Tasks like patient intakes, critical assessments, and recovering information from EMRs take time, of which most participants claimed to not have enough of. There was also a consensus in having inadequate time to spend on medically complex cases. Time pressures were reported to subsequently influence quality of care. One participant stated:
With the constant pace of medicine, time is not on your side. A provider cannot always participate in an enriching dialogue with their patients, so rather than listen and learn, we are often coerced into the mindset of ‘getting through’ this patient so we can move on. This echoes for patient education during discharge, making the whole process more arduous than it otherwise could be if time and resources were not as sparse.
Depending on provider type, specialty, and the size of patient panels, four participants said they have the luxury of extending patient visits to 40 + minutes. Any flexibility with patient visits was regarded as just that: a luxury. Very few providers described the ability to coordinate their schedules as such. This led some study participants to limit the number of patients they serve. One participant said:
We simply don’t have enough clinicians, which is a shame because these people are really skilled, exceptional, brilliant providers but are performing way below their capacity. Because of this, I have a smaller case load so I can engage in a level of care that I feel is in the best interest of my patients. Everything is a tradeoff. Time has to be sacrificed at one point or another. This compromise sets our system up to do ‘ok’ work, not great work.
Of course, managing an overly large number of patients with high complexity is challenging. Especially while enduring the burden of a persisting global pandemic, participants reflected that the general outlook of administering healthcare in the US is to “do more with less.” This often forces providers to delegate responsibilities, which participants noted has potential downsides. One participant described how delegating patient care can cause problems.
Very often will a patient schedule a follow up that needs to happen within a certain time frame, but I am unable to see them myself. So, they are then placed with one of my mid-level providers. However, if additional health issues are introduced, which often happens, there is a high-risk of bounce-back or need to return once again to the hospital. It’s an inefficient vetting process that falls to people who may not have specific training in the labs and imaging that are often included in follow up visits. Unfortunately, it’s a forlorn hope to have a primary care physician be able to attend all levels of a patient’s care.
Several participants described how time constraints stretch all healthcare staff thin and complicate patient care. This was particularly important among participants who reported having a patient panel exceeding 1000. There were some participants, however, who praised the relationships they have with their nurse practitioners and physician’s assistants and mark transparency as the most effective way to coordinate care. Collectively, these clinical relationships were built over long standing periods of time, a disadvantage to providers at the start of their medical career. All but one participant with over a decade of clinical experience mentioned the usefulness of these relationships. The factors discussed in Theme 4 are directly linked to the Availability dimension of access to healthcare. A patient’s ability to reach care is subject to the capacity of their healthcare provider(s). Additionally, further analysis suggests these factors also link to the Appropriateness dimension because the quality of patient-provider relationships may be negatively impacted if a provider’s time is compromised.
Theme 5: Profits are prioritized over addressing barriers to healthcare access in the US.
The US healthcare system functions partially for-profit in the public and private sectors. The federal government provides funding for national programs such as Medicare, but a majority of Americans access healthcare through private employer plans [ 85 ]. As a result, uninsurance rates influence healthcare access. Though the rate of the uninsured has dropped over the last decade through expansion of the Affordable Care Act, it remains above 8 percent [ 86 ]. Historically, there has been ethical criticism in the literature of a for-profit system as it is said to exacerbate healthcare disparities and constitute unfair competition against nonprofit institutions. Specifically, the US healthcare system treats healthcare as a commodity instead of a right, enables organizational controls that adversely affect patient-provider relationships, undermines medical education, and constitutes a medical-industrial complex that threatens influence on healthcare-related public policy [ 87 ]. Though unprompted by the interviewer, participants raised many of these concerns. One participant shared their views on how priorities stand in their practice:
A lot of the higher-ups in the healthcare system where I work see each patient visit as a number. It’s not that they don’t have the capacity to think beyond that, but that’s what their role is, making sure we’re profitable. That’s part of why our healthcare system in the US is as broken as it is. It’s accentuated focus on financially and capitalistically driven factors versus understanding all these other barriers to care.
Eight participants echoed a similar concept, that addressing barriers to healthcare access in their organizations is largely complicated because so much attention is directed on matters that have nothing to do with patients. A few other participants supported this by alluding to a “cherry-picking” process by which those at the top of the hierarchy devote their attention to the easiest tasks. One participant shared an experience where contrasting work demands between administrators and front-line clinical providers produces adverse effects:
We had a new administrator in our hospital. I had been really frustrated with the lack of cultural awareness and curiosity from our other leaders in the past, so I offered to meet and take them on a tour of the reservation. This was meant to introduce them to kids, families and Tribal leaders who live in the area and their interface with healthcare. They declined, which I thought was disappointing and eye-opening.
Analysis of these factors suggest that those who work directly with patients understand patient needs better than those who serve in management roles. This same participant went on to suggest an ulterior motive for a push towards telemedicine, as administrators primarily highlight the benefit of billing for virtual visits instead of the nature of the visits themselves.
This study explored barriers and facilitators to healthcare access from the perspective of rural healthcare providers in Montana. Our qualitative analysis uncovered five key themes: 1) a friction exists between aspects of patients’ rural identities and healthcare systems; 2) facilitating access to healthcare requires application of and respect for cultural differences; 3) communication between healthcare providers is systematically fragmented; 4) time and resource constraints disproportionately harm rural health systems; and 5) profits are prioritized over addressing barriers to healthcare access in the US. Themes 2 and 3 were directly supported by earlier qualitative studies that applied Levesque’s framework, specifically regarding healthcare providers’ poor interpersonal quality and lack of collaboration with other providers that are suspected to result from a lack of provider training [ 67 , 70 ]. This ties back to the importance of cultural humility, which many previous culture-based trainings have referred to as cultural competence. Cultural competence is achieved through a plethora of trainings designed to expose providers to different cultures’ beliefs and values but induces risk of stereotyping and stigmatizing a patient’s views. Therefore, cultural humility is the preferred idea, by which providers reflect and gain open-ended appreciation for a patient’s culture [ 88 ].
Implications for Practice
Perhaps the most substantial takeaway is how embedded rugged individualism is within rural patient populations and how difficult that makes the delivery of care in rural health systems. We heard from participants that stoicism and perceptions of stigma within the system contribute to this, but other resulting factors may be influential at the provider- and organizational-levels. Stoicism and perceived stigma both appear to arise, in part, from an understandable knowledge gap regarding the care system. For instance, healthcare providers understand the relations between primary and secondary care, but many patients may perceive both concepts as elements of a single healthcare system [ 89 ]. Any issue experienced by a patient when tasked to see both a primary and secondary provider may result in a patient becoming confused [ 90 ]. This may also overlap with our third theme, as a disjointed means of communication between healthcare providers can exacerbate patients’ negative experiences. One consideration to improve this is to incorporate telehealth programs into an existing referral framework to reduce unnecessary interfacility transfers; telehealth programs have proven effective in rural and remote settings [ 91 ].
In fact, telehealth has been rolled out in a variety of virtual platforms throughout its evolution, its innovation matched with continued technological advancement. Simply put, telehealth allows health service delivery from a distance; it allows knowledge and practice of clinical care to be in a different space than a patient. Because of this, a primary benefit of telehealth is its impact on improving patient-centered outcomes among those living in rural areas. For instance, text messaging technology improves early infant diagnosis, adherence to recommended diagnostic testing, and participant engagement in lifestyle change interventions [ 92 , 93 , 94 ]. More sophisticated interventions have found their way into smartphone-based technology, some of which are accessible even without an internet connection [ 95 , 96 ]. Internet accessibility is important because a number of study participants noted internet connectivity as a barrier for patients who live in low resource communities. Videoconferencing is another function of telehealth that has delivered a variety of health services, including those which are mental health-specific [ 97 ], and mobile health clinics have been used in rural, hard-to-reach settings to show the delivery of quality healthcare is both feasible and acceptable [ 98 , 99 , 100 ]. While telehealth has potential to reduce a number of healthcare access barriers, it may not always address the most pressing healthcare needs [ 101 ]. However, telehealth does serve as a viable, cost-effective alternative for rural populations with limited physical access to specialized services [ 102 ]. With time and resource limitations acknowledged as a key theme in our study, an emphasis on expanding telehealth services is encouraged as it will likely have significant involvement on advancing healthcare in the future, especially as the COVID-19 pandemic persists [ 103 ].
Implications for Policy
One could argue that most of the areas of fragmentation in the US healthcare system can be linked to the very philosophy on which it is based: an emphasis on profits as highest priority. Americans are, therefore, forced to navigate a health service system that does not work solely in their best interests. It is not surprising to observe lower rates of healthcare usage in rural areas, which may be a result from rural persons’ negative views of the US healthcare system or a perception that the system does not exist to support wellness. These perceptions may interact with ‘rugged individualism’ to squelch rural residents’ engagement in healthcare. Many of the providers we interviewed for this study appeared to understand this and strived to improve their patients’ experiences and outcomes. Though these efforts are admirable, they may not characterize all providers who serve in rural areas of the US. From a policy standpoint, it is important to recognize these expansive efforts from providers. If incentives were offered to encourage maximum efforts be made, it may lessen burden due to physician burnout and fatigue. Of course, there is no easy fix to the persisting limit of time and resources for providers, problems that require workforce expansion. Ultimately, though, the current structure of the US healthcare system is failing rural America and doing little to help the practice of rural healthcare providers.
Implications for Future Research
It is important for future health systems research efforts to consider issues that arise from both individual- and system-level access barriers and where the two intersect. Oftentimes, challenges that appear linked to a patient or provider may actually stem from an overarching system failure. If failures are critically and properly addressed, we may refine our understanding of what we can do in our professional spaces to improve care as practitioners, workforce developers, researchers and advocates. This qualitative study was exploratory in nature. It represents a step forward in knowledge generation regarding challenges in access to healthcare for rural Americans. Although mental health did not come up by design in this study, future efforts exploring barriers to healthcare access in rural systems should focus on access to mental healthcare. In many rural areas, Montana included, rates of suicide, substance use and other mental health disorders are highly prevalent. These characteristics should be part of the overall discussion of access to healthcare in rural areas. Optimally, barriers to healthcare access should continue to be explored through qualitative and mixed study designs to honor its multi-dimensional stature.
Strengths and Limitations
It is important to note first that this study interviewed healthcare providers instead of patients, which served as both a strength and limitation. Healthcare providers were able to draw on numerous patient-provider experiences, enabling an account of the aggregate which would have been impossible for a patient population. However, accounts of healthcare providers’ perceptions of barriers to healthcare access for their patients may differ from patients’ specific views. Future research should examine acceptability- and appropriateness-related barriers to healthcare access in patient populations. Second, study participants were recruited through convenience sampling methods, so results may be biased towards healthcare providers who are more invested in addressing barriers to healthcare access. Particularly, the providers interviewed for this study represented a subset who go beyond expectations of their job descriptions by engaging with their communities and spending additional uncompensated time with their patients. It is likely that a provider who exhibits these behavioral traits is more likely to participate in research aimed at addressing barriers to healthcare access. Third, the inability to conduct face-to-face interviews for our qualitative study may have posed an additional limitation. It is possible, for example, that in-person interviews might have resulted in increased rapport with study participants. Notwithstanding this possibility, the remote interview format was necessary to accommodate health risks to the ongoing COVID-19 pandemic. Ultimately, given our qualitative approach, results from our study cannot be generalizable to all rural providers’ views or other rural health systems. In addition, no causality can be inferred regarding the influence of aspects of rurality on access. The purpose of this exploratory qualitative study was to probe research questions for future efforts. We also acknowledge the authors’ roles in the research, also known as reflexivity. The first author was the only author who administered interviews and had no prior relationships with all but one study participant. Assumptions and pre-dispositions to interview content by the first author were regularly addressed throughout data analysis to maintain study integrity. This was achieved by conducting analysis by unique interview question, rather than by unique participant, and recoding the numerical order of participants for each question. Our commitment to rigorous qualitative methods was a strength for the study for multiple reasons. Conducting member checks with participants ensured trustworthiness of findings. Continuing data collection to data saturation ensured dependability of findings, which was achieved after 10 interviews and confirmed after 2 additional interviews. We further recognize the heterogeneity in our sample of participants, which helped generate variability in responses. To remain consistent with appropriate means of presenting results in qualitative research however, we shared minimal demographic information about our study participants to ensure confidentiality.
The divide between urban and rural health stretches beyond a disproportionate allocation of resources. Rural health systems serve a more complicated and hard-to-reach patient population. They lack sufficient numbers of providers to meet population health needs. These disparities impact collaboration between patients and providers as well as the delivery of acceptable and appropriate healthcare. The marker of rurality complicates the already cumbersome challenge of administering acceptable and appropriate healthcare and impediments stemming from rurality require continued monitoring to improve patient experiences and outcomes. Our qualitative study explored rural healthcare providers’ views on some of the social, cultural, and programmatic factors that influence access to healthcare among their patient populations. We identified five key themes: 1) a friction exists between aspects of patients’ rural identities and healthcare systems; 2) facilitating access to healthcare requires application of and respect for cultural differences; 3) communication between healthcare providers is systematically fragmented; 4) time and resource constraints disproportionately harm rural health systems; and 5) profits are prioritized over addressing barriers to healthcare access in the US. This study provides implications that may shift the landscape of a healthcare provider’s approach to delivering healthcare. Further exploration is required to understand the effects these characteristics have on measurable patient-centered outcomes in rural areas.
Availability of data and materials
The datasets generated and/or analyzed during the current study are not publicly available due to individual privacy could be compromised but are available from the corresponding author on reasonable request.
Ethics approval and consent to participate.
All study procedures and methods were carried out in accordance with relevant guidelines and regulations from the World Medical Association Declaration of Helsinki. Ethics approval was given by exempt review from the Institutional Review Board (IRB) at the University of Montana (IRB Protocol No.: 186–20). Participants received oral and written information about the study prior to interview, which allowed them to provide informed consent for the interviews to be recorded and used for qualitative research purposes. No ethical concerns were experienced in this study pertaining to human subjects.
Consent for publication.
The participants consented to the publication of de-identified material from the interviews.
Riley WJ. Health Disparities: Gaps in Access, Quality and Affordability of Medical Care. Trans Am Clin Climatol Assoc. 2012;123:167–74.
PubMed PubMed Central Google Scholar
Levesque J, Harris MF, Russell G. Patient-centred access to health care: conceptualising access at the interface of health systems and populations. Int J Equity Health. 2013;12:18. https://doi.org/10.1186/1475-9276-12-18 .
Article PubMed PubMed Central Google Scholar
Serban N. A Multidimensional Framework for Measuring Access. In: Serban N. Healthcare System Access: Measurement, Inference, and Intervention. New Jersey: John Wiley & Sons; 2019. p. 13–59.
Google Scholar
Roncarolo F, Boivin A, Denis JL, et al. What do we know about the needs and challenges of health systems? A scoping review of the international literature. BMC Health Serv Res. 2017;17:636. https://doi.org/10.1186/s12913-017-2585-5 .
Cabrera-Barona P, Blaschke T. Kienberger S. Explaining Accessibility and Satisfaction Related to Healthcare: A Mixed-Methods Approach. Soc Indic Res. 2017;133,719–739. https://doi.org/10.1007/s11205-016-1371-9 .
Coombs NC, Meriwether WE, Caringi J, Newcomer SR. Barriers to healthcare access among U.S. adults with mental health challenges: A population-based study. SSM Popul Health. 2021;15:100847. https://doi.org/10.1016/j.ssmph.2021.100847 .
Farietta TP, Lu B, Tumin R. Ohio’s Medicaid Expansion and Unmet Health Needs Among Low-Income Women of Reproductive Age. Matern Child Health. 2018;22(12):1771–9. https://doi.org/10.1007/s10995-018-2575-1 .
Article Google Scholar
Sommers BD, Blendon RJ, Orav EJ, Epstein AM. Changes in Utilization and Health Among Low-Income Adults After Medicaid Expansion or Expanded Private Insurance. JAMA Intern Med. 2016;1;176(10):1501–1509. https://doi.org/10.1001/jamainternmed.2016.4419 .
National Alliance on Mental Illness: The Doctor is Out. Continuing Disparities in Access to Mental and Physical Health Care. 2017. https://www.nami.org/Support-Education/Publications-Reports/Public-Policy-Reports/The-Doctor-is-Out . Accessed 29 Aug 2021.
Pollitz K, Lopes L, Kearney A, Rae M, Cox C, Fehr F, Rousseau D, Kaiser Family Foundation. US Statistics on Surprise Medical Billing. JAMA. 2020;323(6):498. https://doi.org/10.1001/jama.2020.0065 .
Kahraman C, Orobello C, Cirella GT. hanging Dynamics with COVID-19: Future Outlook. In: XX Cirella GT, editor. Human Settlements: Urbanization, Smart Sector Development, and Future Outlook. Singapore: Springer; 2022. p. 235–52.
Book Google Scholar
Blumenthal D, Fowler EJ, Abrams M, Collins SR. Covid-19 — Implications for the Health Care System. N Engl J Med. 2020;383(15):1483–8. https://doi.org/10.1056/NEJMsb2021088 .
Article CAS PubMed Google Scholar
Defêche J, Azarzar S, Mesdagh A, Dellot P, Tytgat A, Bureau F, Gillet L, Belhadj Y, Bontems S, Hayette M-P, Schils R, Rahmouni S, Ernst M, Moutschen M, Darcis G. In-Depth Longitudinal Comparison of Clinical Specimens to Detect SARS-CoV-2. Pathogens. 2021;10(11):1362. https://doi.org/10.3390/pathogens10111362 .
Article CAS PubMed PubMed Central Google Scholar
Rosenblatt RA, Hart LG. Physicians and rural America. West J Med. 2000;173(5):348–51. https://doi.org/10.1136/ewjm.173.5.348 .
Kaiser Family Foundation Analysis of OECD Data. 2020. https://www.healthsystemtracker.org/chart-collection/u-s-health-care-resources-compare-countries/#item-physicians-density-per-1000-population-2000-2018 . Accessed 31 Oct 2021.
Government Accounting Office. Physician Workforce: Locations and Types of Graduate Training Were Largely Unchanged, and Federal Efforts May Not Be Sufficient to Meet Needs. 2017. https://www.gao.gov/assets/gao-17-411.pdf . Accessed 31 Oct 2021.
The Kaiser Family Foundation: The Uninsured in Rural America. 2003. https://www.kff.org/wp-content/uploads/2013/01/the-uninsured-in-rural-america-update-pdf.pdf . Accessed 15 Sept 2021.
Wright B, Potter AJ, Trivedi AN, Mueller KJ. The Relationship Between Rural Health Clinic Use and Potentially Preventable Hospitalizations and Emergency Department Visits Among Medicare Beneficiaries. J Rural Health. 2018;34(4):423–30. https://doi.org/10.1111/jrh.12253 .
Article PubMed Google Scholar
Weichelt B, Bendixsen C, Patrick T. A Model for Assessing Necessary Conditions for Rural Health Care’s Mobile Health Readiness: Qualitative Assessment of Clinician-Perceived Barriers. JMIR Mhealth Uhealth. 2019;7(11): e11915. https://doi.org/10.2196/11915 .
Mangundu M, Roets L, Janse van Rensberg E. Accessibility of healthcare in rural Zimbabwe: The perspective of nurses and healthcare users. Afr J Prim Health Care Fam Med. 2020;12(1):e1-e7. https://doi.org/10.4102/phcfm.v12i1.2245 .
Rahayu YYS, Araki T, Rosleine D. Factors affecting the use of herbal medicines in the universal health coverage system in Indonesia. J Ethnopharmacol. 2020;260: 112974. https://doi.org/10.1016/j.jep.2020.112974 .
Bolin JN, Bellamy GR, Ferdinand AO, et al. Rural Healthy People 2020: New Decade, Same Challenges. Journal of Rural Health. 2015;31(3):326–33.
Reid S. The rural determinants of health: using critical realism as a theoretical framework. Rural Remote Health. 2019;19(3):5184. https://doi.org/10.22605/RRH5184 .
Wynia MK, Osborn CY. Health literacy and communication quality in health care organizations. J Health Commun. 2010;15 Suppl 2(Suppl 2):102–115. https://doi.org/10.1080/10810730.2010.499981 .
Centers for Disease Control and Prevention. What is Health Literacy? 2022. https://www.cdc.gov/healthliteracy/learn/index.html . Accessed 25 Feb 2022.
Davis TC, Arnold CL, Rademaker A, et al. Differences in barriers to mammography between rural and urban women. J Womens Health (Larchmt). 2012;21(7):748–55. https://doi.org/10.1089/jwh.2011.3397 .
Halverson J, Martinez-Donate A, Trentham-Dietz A, et al. Health literacy and urbanicity among cancer patients. J Rural Health. 2013;29(4):392–402. https://doi.org/10.1111/jrh.12018 .
Zahnd WE, Scaife SL, Francis ML. Health literacy skills in rural and urban populations. Am J Health Behav. 2009;33(5):550–7. https://doi.org/10.5993/ajhb.33.5.8 .
Dogba MJ, Dossa AR, Breton E, Gandonou-Migan R. Using information and communication technologies to involve patients and the public in health education in rural and remote areas: a scoping review. BMC Health Serv Res. 2019;19(1):128. https://doi.org/10.1186/s12913-019-3906-7 .
Committee on the Science of Changing Behavioral Health Social Norms, Board on Behavioral, Cognitive, and Sensory Sciences, Division of Behavioral and Social Sciences and Education; National Academies of Sciences, Engineering, and Medicine. In: Ending Discrimination Against People with Mental and Substance Use Disorders: The Evidence for Stigma Change. Washington: National Academics Press; 2016. p. 33–52.
Wu Y, Zhou H, Wang Q, Cao M, Medina A, Rozelle S. Use of maternal health services among women in the ethnic rural areas of western China. BMC Health Serv Res. 2019;19(1):179. https://doi.org/10.1186/s12913-019-3996-2 .
Meyer E, Hennink M, Rochat R, et al. Working Towards Safe Motherhood: Delays and Barriers to Prenatal Care for Women in Rural and Peri-Urban Areas of Georgia. Matern Child Health J. 2016;20(7):1358–65. https://doi.org/10.1007/s10995-016-1997-x .
Heinrich S. Medical science faces the post-truth era: a plea for the grassroot values of science. Curr Opin Anaesthesiol. 2020;33(2):198–202. https://doi.org/10.1097/ACO.0000000000000833 .
Esquivel MM, Chen JC, Woo RK, et al. Why do patients receive care from a short-term medical mission? Survey study from rural Guatemala. J Surg Res. 2017;215:160–6. https://doi.org/10.1016/j.jss.2017.03.056 .
Park LG, Dracup K, Whooley MA, et al. Symptom Diary Use and Improved Survival for Patients With Heart Failure [published correction appears in Circ Heart Fail. 2017 Dec;10(12):]. Circ Heart Fail. 2017;10(11):e003874. https://doi.org/10.1161/CIRCHEARTFAILURE.117.003874 .
Taleb F, Perkins J, Ali NA, et al. Transforming maternal and newborn health social norms and practices to increase utilization of health services in rural Bangladesh: a qualitative review. BMC Pregnancy Childbirth. 2015;15:75. https://doi.org/10.1186/s12884-015-0501-8 .
Billah SM, Hoque DE, Rahman M, et al. Feasibility of engaging “Village Doctors” in the Community-based Integrated Management of Childhood Illness (C-IMCI): experience from rural Bangladesh. J Glob Health. 2018;8(2): 020413. https://doi.org/10.7189/jogh.08.020413 .
Kaiser JL, Fong RM, Hamer DH, et al. How a woman’s interpersonal relationships can delay care-seeking and access during the maternity period in rural Zambia: An intersection of the Social Ecological Model with the Three Delays Framework. Soc Sci Med. 2019;220:312–21. https://doi.org/10.1016/j.socscimed.2018.11.011 .
Rao KD, Sheffel A. Quality of clinical care and bypassing of primary health centers in India. Soc Sci Med. 2018;207:80–8. https://doi.org/10.1016/j.socscimed.2018.04.040 .
Lee YT, Lee YH, Kaplan WA. Is Taiwan’s National Health Insurance a perfect system? Problems related to health care utilization of the aboriginal population in rural townships. Int J Health Plann Manage. 2019;34(1):e6–10. https://doi.org/10.1002/hpm.2653 .
Lyford M, Haigh MM, Baxi S, Cheetham S, Shahid S, Thompson SC. An Exploration of Underrepresentation of Aboriginal Cancer Patients Attending a Regional Radiotherapy Service in Western Australia. Int J Environ Res Public Health. 2018;15(2):337. https://doi.org/10.3390/ijerph15020337 .
Article PubMed Central Google Scholar
Rohr JM, Spears KL, Geske J, Khandalavala B, Lacey MJ. Utilization of Health Care Resources by the Amish of a Rural County in Nebraska. J Community Health. 2019;44(6):1090–7. https://doi.org/10.1007/s10900-019-00696-9 .
Johnston K, Harvey C, Matich P, et al. Increasing access to sexual health care for rural and regional young people: Similarities and differences in the views of young people and service providers. Aust J Rural Health. 2015;23(5):257–64. https://doi.org/10.1111/ajr.12186 .
Legido-Quigley H, Naheed A, de Silva HA, et al. Patients’ experiences on accessing health care services for management of hypertension in rural Bangladesh, Pakistan and Sri Lanka: A qualitative study. PLoS ONE. 2019;14(1): e0211100. https://doi.org/10.1371/journal.pone.0211100 .
Shaw B, Amouzou A, Miller NP, Bryce J, Surkan PJ. A qualitative exploration of care-seeking pathways for sick children in the rural Oromia region of Ethiopia. BMC Health Serv Res. 2017;17(1):184. https://doi.org/10.1186/s12913-017-2123-5 .
Larson E, Vail D, Mbaruku GM, Kimweri A, Freedman LP, Kruk ME. Moving Toward Patient-Centered Care in Africa: A Discrete Choice Experiment of Preferences for Delivery Care among 3,003 Tanzanian Women. PLoS ONE. 2015;10(8): e0135621. https://doi.org/10.1371/journal.pone.0135621 .
Spleen AM, Lengerich EJ, Camacho FT, Vanderpool RC. Health care avoidance among rural populations: results from a nationally representative survey. J Rural Health. 2014;30(1):79–88. https://doi.org/10.1111/jrh.12032 .
Weisgrau S. Issues in rural health: access, hospitals, and reform. Health Care Financ Rev. 1995;17(1):1–14.
CAS PubMed PubMed Central Google Scholar
National Center for Health Statistics. 2018. https://www.cdc.gov/nchs/surveys.htm . Accessed 29 Aug 2021.
Sekhon M, Cartwright M, Francis JJ. Acceptability of healthcare interventions: an overview of reviews and development of a theoretical framework. BMC Health Serv Res. 2017;17(1):88. https://doi.org/10.1186/s12913-017-2031-8 .
Dyer TA, Owens J, Robinson PG. The acceptability of healthcare: from satisfaction to trust. Community Dent Health. 2016;33(4):242–51. https://doi.org/10.1922/CDH_3902Dyer10 .
Padgett DK. Qualitative and Mixed Methods in Public Health. Thousand Oaks, California: SAGE Publications Inc.; 2012.
Tolley EE, Ulin PR, Mack N, Robinson ET, Succop SM. Qualitative Methods in Public Health: A Field Guide for Applied Research. 2nd ed. San Francisco, California: John Wiley & Sons, Inc.; 2016.
Ingram DD, Franco SJ. 2013 NCHS urban-rural classification scheme for counties. In: National Center for Health Statistics: Vital Health Statistics. 2014. https://www.cdc.gov/nchs/data/series/sr_02/sr02_166.pdf . Accessed 31 Oct 2021.
Health Resources & Services Administration. Health Professional Shortage Area Find. 2021. https://data.hrsa.gov/tools/shortage-area/hpsa-find . Accessed 31 Oct 2021.
Shaghaghi A, Bhopal RS, Sheikh A. Approaches to Recruiting “Hard-To-Reach” Populations into Re-search: A Review of the Literature. Health Promot Perspect. 2011;1(2):86–94. https://doi.org/10.5681/hpp.2011.009 .
Sadler GR, Lee HC, Lim RS, Fullerton J. Recruitment of hard-to-reach population subgroups via adaptations of the snowball sampling strategy. Nurs Health Sci. 2010;12(3):369–74. https://doi.org/10.1111/j.1442-2018.2010.00541.x .
Deterding NM, Waters MC. Flexible Coding of In-depth Interviews: A Twenty-first-century Approach. Sociological Methods & Research. 2021;50(2):708–39. https://doi.org/10.1177/0049124118799377 .
Schreier M, Stamann C, Janssen M, Dahl T, Whittal A. Qualitative Content Analysis: Conceptualizations and Challenges in Research Practice-Introduction to the FQS Special Issue" Qualitative Content Analysis I". InForum Qualitative Sozialforschung/Forum: Qualitative Social Research. 2019;20:26 DEU.
Guest G, Namey E, Chen M. A simple method to assess and report thematic saturation in qualitative research. PLoS ONE. 2020;15(5): e0232076. https://doi.org/10.1371/journal.pone.0232076 .
Mayring P. Qualitative Content Analysis. Forum Qualitative Sozialforschung/Forum: Qualitative Social Research. 2000;1(2). https://doi.org/10.17169/fqs-1.2.1089 .
O'Brien BC, Harris IB, Beckman TJ, Reed DA, Cook DA. Standards for reporting qualitative research: a synthesis of recommendations. Academic Medicine, Vol. 89, No. 9 / Sept 2014. https://doi.org/10.1097/ACM.0000000000000388 .
Carter N, Bryant-Lukosius D, DiCenso A, Blythe J, Neville AJ. The use of triangulation in qualitative research. Oncol Nurs Forum. 2014;41(5):545–7. https://doi.org/10.1188/14.ONF.545-547 .
Miller S. Cultural humility is the first step to becoming global care providers. J Obstet Gynecol Neonatal Nurs. 2009;38(1):92–3. https://doi.org/10.1111/j.1552-6909.2008.00311.x .
Prasad SJ, Nair P, Gadhvi K, Barai I, Danish HS, Philip AB. Cultural humility: treating the patient, not the illness. Med Educ Online. 2016;21:30908. https://doi.org/10.3402/meo.v21.30908 .
George MS, Davey R, Mohanty I, Upton P. “Everything is provided free, but they are still hesitant to access healthcare services”: why does the indigenous community in Attapadi, Kerala continue to experience poor access to healthcare? Int J Equity Health. 2020;19(1):105. https://doi.org/10.1186/s12939-020-01216-1 .
Romanelli M, Hudson KD. Individual and systemic barriers to health care: Perspectives of lesbian, gay, bisexual, and transgender adults. Am J Orthopsychiatry. 2017;87(6):714–28. https://doi.org/10.1037/ort0000306 .
Bailie J, Schierhout G, Laycock A, et al. Determinants of access to chronic illness care: a mixed-methods evaluation of a national multifaceted chronic disease package for Indigenous Australians. BMJ Open. 2015;5(11): e008103. https://doi.org/10.1136/bmjopen-2015-008103 .
Reeve C, Humphreys J, Wakerman J, Carter M, Carroll V, Reeve D. Strengthening primary health care: achieving health gains in a remote region of Australia. Med J Aust. 2015;202(9):483–7. https://doi.org/10.5694/mja14.00894 .
Swan LET, Auerbach SL, Ely GE, Agbemenu K, Mencia J, Araf NR. Family Planning Practices in Appalachia: Focus Group Perspectives on Service Needs in the Context of Regional Substance Abuse. Int J Environ Res Public Health. 2020;17(4):1198. https://doi.org/10.3390/ijerph17041198 .
Kabia E, Mbau R, Oyando R, et al. “We are called the et cetera”: experiences of the poor with health financing reforms that target them in Kenya. Int J Equity Health. 2019;18(1):98. https://doi.org/10.1186/s12939-019-1006-2 .
Ho JW, Kuluski K, Im J. “It’s a fight to get anything you need” - Accessing care in the community from the perspectives of people with multimorbidity. Health Expect. 2017;20(6):1311–9. https://doi.org/10.1111/hex.12571 .
Latif A, Mandane B, Ali A, Ghumra S, Gulzar N. A Qualitative Exploration to Understand Access to Pharmacy Medication Reviews: Views from Marginalized Patient Groups. Pharmacy (Basel). 2020;8(2):73. https://doi.org/10.3390/pharmacy8020073 .
Tschirhart N, Diaz E, Ottersen T. Accessing public healthcare in Oslo, Norway: the experiences of Thai immigrant masseuses. BMC Health Serv Res. 2019;19(1):722. https://doi.org/10.1186/s12913-019-4560-9 .
Ireland S, Belton S, Doran F. “I didn’t feel judged”: exploring women’s access to telemedicine abortion in rural Australia. J Prim Health Care. 2020;12(1):49–56. https://doi.org/10.1071/HC19050 .
Stokes T, Tumilty E, Latu ATF, et al. Improving access to health care for people with severe chronic obstructive pulmonary disease (COPD) in Southern New Zealand: qualitative study of the views of health professional stakeholders and patients. BMJ Open. 2019;9(11): e033524. https://doi.org/10.1136/bmjopen-2019-033524 .
Jafar TH, Ramakrishnan C, John O, et al. Access to CKD Care in Rural Communities of India: a qualitative study exploring the barriers and potential facilitators. BMC Nephrol. 2020;21(1):26. https://doi.org/10.1186/s12882-020-1702-6 .
Doetsch J, Pilot E, Santana P, Krafft T. Potential barriers in healthcare access of the elderly population influenced by the economic crisis and the troika agreement: a qualitative case study in Lisbon, Portugal. Int J Equity Health. 2017;16(1):184. https://doi.org/10.1186/s12939-017-0679-7 .
Engel GL. The need for a new medical model: a challenge for biomedicine. Science. 1977;196(4286):129–36. https://doi.org/10.1126/science.847460 .
Risberg G, Hamberg K, Johansson E.E. Gender perspective in medicine: a vital part of medical scientific rationality. A useful model for comprehending structures and hierarchies within medical science. BMC Med. 2006;4(1):1.
Montana Census & Economic Information Center. 2021. https://ceic.mt.gov/ . Accessed 18 Sept 2021.
Smith M, Saunders R, Stuckhardt L, McGinnis JM, Committee on the Learning Health Care System in America, Institute of Medicine. Best Care at Lower Cost: The Path to Continuously Learning Health Care in America. Washington: National Academies Press; 2013.
O’Daniel M, Rosenstein AH, Professional Communication and Team Collaboration. In: Hughes RG, editor. Chapter 33: Patient Safety and Quality: An Evidence-Based Handbook for Nurses. Agency for Healthcare Research and Quality: Rockville; 2008.
Evans RS. Electronic Health Records: Then, Now, and in the Future. Yearb Med Inform. 2016;Suppl 1(Suppl 1):S48-S61. https://doi.org/10.15265/IYS-2016-s006 .
Tikkanen R, Osborn R, Mossialos E, Djordjevic A, Wharton GA. International Health Care System Profiles: United States. The Commonwealth Fund. 2020. https://www.commonwealthfund.org/international-health-policy-center/countries/united-states . Accessed 19 Sept 2021.
Berchick ER, Barnett JC, Upton RD. Health Insurance Coverage in the United States. Washington: United States Census Bureau; 2018.
Brock DW, Buchanan A. Ethical Issues in For-Profit Health Care. In: Gray BH, editor. For-Profit Enterprise in Health Care. Washington: National Academics Press; 1986.
Lekas HM, Pahl K, Fuller Lewis C. Rethinking Cultural Competence: Shifting to Cultural Humility. Health Serv Insights. 2020;13:1178632920970580. https://doi.org/10.1177/1178632920970580 .
Beaulieu MD. Primary and secondary care: Breaking down barriers for our patients with chronic diseases. Can Fam Physician. 2013;59(2):221.
Crafford L, Jenkins LS. Why seek a second consultation at an emergency centre? A qualitative study. Afr J Prim Health Care Fam Med. 2017;9(1):e1–8. https://doi.org/10.4102/phcfm.v9i1.1397 .
Sorensen MJ, von Recklinghausen FM, Fulton G, et al. Secondary overtriage: The burden of unnecessary interfacility transfers in a rural trauma system. JAMA Surg. 2013;148:763–8.
Sutcliffe CG, Thuma PE, van Dijk JH, et al. Use of mobile phones and text messaging to decrease the turnaround time for early infant HIV diagnosis and notification in rural Zambia: an observational study. BMC Pediatr. 2017;17(1):66. https://doi.org/10.1186/s12887-017-0822-z .
Baldwin LM, Morrison C, Griffin J, et al. Bidirectional Text Messaging to Improve Adherence to Recommended Lipid Testing. J Am Board Fam Med. 2017;30(5):608–14. https://doi.org/10.3122/jabfm.2017.05.170088 .
Albright K, Krantz MJ, Backlund Jarquín P, DeAlleaume L, Coronel-Mockler S, Estacio RO. Health Promotion Text Messaging Preferences and Acceptability Among the Medically Underserved. Health Promot Pract. 2015;16(4):523–32. https://doi.org/10.1177/1524839914566850 .
Cramer ME, Mollard EK, Ford AL, Kupzyk KA, Wilson FA. The feasibility and promise of mobile technology with community health worker reinforcement to reduce rural preterm birth. Public Health Nurs. 2018;35(6):508–16. https://doi.org/10.1111/phn.12543 .
Gbadamosi SO, Eze C, Olawepo JO, et al. A Patient-Held Smartcard With a Unique Identifier and an mHealth Platform to Improve the Availability of Prenatal Test Results in Rural Nigeria: Demonstration Study. J Med Internet Res. 2018;20(1): e18. https://doi.org/10.2196/jmir.8716 .
Trondsen MV, Tjora A, Broom A, Scambler G. The symbolic affordances of a video-mediated gaze in emergency psychiatry. Soc Sci Med. 2018;197:87–94. https://doi.org/10.1016/j.socscimed.2017.11.056 .
Schwitters A, Lederer P, Zilversmit L, et al. Barriers to health care in rural Mozambique: a rapid ethnographic assessment of planned mobile health clinics for ART. Glob Health Sci Pract. 2015;3(1):109–16. https://doi.org/10.9745/GHSP-D-14-00145 .
Kojima N, Krupp K, Ravi K, et al. Implementing and sustaining a mobile medical clinic for prenatal care and sexually transmitted infection prevention in rural Mysore, India. BMC Infect Dis. 2017;17(1):189. https://doi.org/10.1186/s12879-017-2282-3 .
Gorman SE, Martinez JM, Olson J. An assessment of HIV treatment outcomes among utilizers of semi-mobile clinics in rural Kenya. AIDS Care. 2015;27(5):665–8. https://doi.org/10.1080/09540121.2014.986053 .
Lee S, Black D, Held ML. Factors Associated with Telehealth Service Utilization among Rural Populations. J Health Care Poor Underserved. 2019;30(4):1259–72. https://doi.org/10.1353/hpu.2019.0104 .
Agha Z, Schapira RM, Maker AH. Cost effectiveness of telemedicine for the delivery of outpatient pulmonary care to a rural population. Telemed J E Health. 2002;8(3):281–91. https://doi.org/10.1089/15305620260353171 .
Doraiswamy S, Abraham A, Mamtani R, Cheema S. Use of Telehealth During the COVID-19 Pandemic: Scoping Review. J Med Internet Res. 2020;22(12): e24087. https://doi.org/10.2196/24087 .
Download references
Acknowledgements
This research was supported by the Center for Biomedical Research Excellence award (P20GM130418) from the National Institute of General Medical Sciences of the National Institute of Health. The first author was also supported by the University of Montana Burnham Population Health Fellowship. We would like to thank Dr. Christopher Dietrich, Dr. Jennifer Robohm and Dr. Eric Arzubi for their contributions on determining inclusion criteria for the healthcare provider population used for this study.
This research did not receive any specific grant from funding agencies in the public, commercial, and not-for-profit sectors.
Author information
Authors and affiliations.
School of Public & Community Health Sciences, University of Montana, 32 Campus Dr, Missoula, MT, 59812, USA
Nicholas C. Coombs & James Caringi
Department of Psychology, University of Montana, 32 Campus Dr, Missoula, MT, 59812, USA
Duncan G. Campbell
You can also search for this author in PubMed Google Scholar
Contributions
The authors confirm contribution to the paper as follows: study conception and design: NC and JC; data collection: NC; analysis and interpretation of results: NC and JC; draft manuscript preparation: NC, DC and JC; and manuscript editing: NC, DC and JC. All authors reviewed the results and approved the final version of the manuscript.
Corresponding author
Correspondence to Nicholas C. Coombs .
Ethics declarations
Competing interests.
The authors declare that they have no competing interests.
Additional information
Publisher's note.
Springer Nature remains neutral with regard to jurisdictional claims in published maps and institutional affiliations.
Rights and permissions
Open Access This article is licensed under a Creative Commons Attribution 4.0 International License, which permits use, sharing, adaptation, distribution and reproduction in any medium or format, as long as you give appropriate credit to the original author(s) and the source, provide a link to the Creative Commons licence, and indicate if changes were made. The images or other third party material in this article are included in the article's Creative Commons licence, unless indicated otherwise in a credit line to the material. If material is not included in the article's Creative Commons licence and your intended use is not permitted by statutory regulation or exceeds the permitted use, you will need to obtain permission directly from the copyright holder. To view a copy of this licence, visit http://creativecommons.org/licenses/by/4.0/ . The Creative Commons Public Domain Dedication waiver ( http://creativecommons.org/publicdomain/zero/1.0/ ) applies to the data made available in this article, unless otherwise stated in a credit line to the data.
Reprints and permissions
About this article
Cite this article.
Coombs, N.C., Campbell, D.G. & Caringi, J. A qualitative study of rural healthcare providers’ views of social, cultural, and programmatic barriers to healthcare access. BMC Health Serv Res 22 , 438 (2022). https://doi.org/10.1186/s12913-022-07829-2
Download citation
Received : 11 January 2022
Accepted : 21 March 2022
Published : 02 April 2022
DOI : https://doi.org/10.1186/s12913-022-07829-2
Share this article
Anyone you share the following link with will be able to read this content:
Sorry, a shareable link is not currently available for this article.
Provided by the Springer Nature SharedIt content-sharing initiative
- Access to healthcare
- Rural health
- Qualitative methods
BMC Health Services Research
ISSN: 1472-6963
- General enquiries: [email protected]
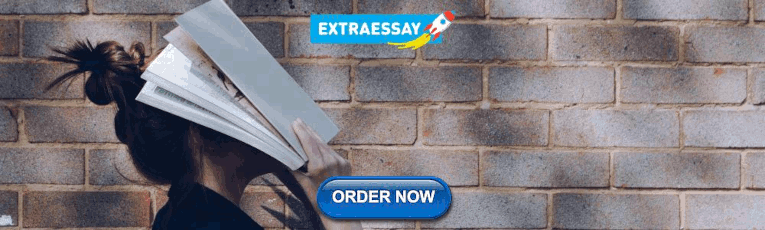
IMAGES
VIDEO
COMMENTS
In short, a data analysis process that draws on both deductive and inductive analysis supports a more organized, rigorous, and analytically sound qualitative study. See below for an example of how I organize deductive and inductive analytic practices into cycles. This figure, adapted from Bingham & Witkowsky (2022) and Bingham (2023), gives an ...
In short, a data analysis process that draws on both deductive and inductive analysis supports a more organized, rigorous, and analytically sound qualitative study. The figure below demonstrates how I organize deductive and inductive analytic practices into cycles. This figure gives an overview of my analysis process.
Revised on June 22, 2023. The main difference between inductive and deductive reasoning is that inductive reasoning aims at developing a theory while deductive reasoning aims at testing an existing theory. In other words, inductive reasoning moves from specific observations to broad generalizations. Deductive reasoning works the other way around.
The deductive approach involves beginning with a theory, developing hypotheses from that theory, and then collecting and analyzing data to test those hypotheses. Inductive and deductive approaches to research can be employed together for a more complete understanding of the topic that a researcher is studying.
Deductive qualitative analysis (DQA; Gilgun, 2005) is a specific approach to deductive qualitative research intended to systematically test, refine, or refute theory by integrating deductive and inductive strands of inquiry.The purpose of the present paper is to provide a primer on the basic principles and practices of DQA and to exemplify the methodology using two studies that were conducted ...
This article expands on ideas presented by Bingham and Witkowsky (2022) to explicate a process of qualitative analysis, rooted in both deductive and inductive strategies. Specifically, in this article, I outline a five-phase process of qualitative analysis that draws on deductive (codes developed a priori) and inductive (codes developed in the course of the analysis) coding strategies, as well ...
The main difference between inductive and deductive reasoning is that inductive reasoning aims at developing a theory while deductive reasoning aims at testing an existing theory. Inductive reasoning moves from specific observations to broad generalisations, and deductive reasoning the other way around. Both approaches are used in various types ...
Inductive and Deductive Research Approaches 7 Conclusions change and evolve continuously as more data is collected. Qualitative research is often said to employ inductive thinking or induction reasoning since it moves from specific observations about individual occurrences to broader generalizations and theories. In making
The Spectrum of Inductive and Deductive Research Approaches Using Quantitative and Qualitative Data. Young, Meredith PhD; Varpio, Lara PhD; Uijtdehaage, Sebastian PhD; Paradis, Elise PhD. Author Information. associate professor, Institute of Health Sciences Education and Department of Medicine, McGill University.
Difficulties stem from the nature of induction itself - Is analytic induction an impossible operation in qualitative research, as Popper (1963/65) suggests? In this section, we first discuss Popper's concern, followed by a discussion of two major threats that may prevent an inductive approach in qualitative research. 2 The first threat is the "pink elephant paradox;? the second is the ...
The main differences between inductive and deductive research are how the research is done, the goal, and how the data is analyzed. Inductive research is exploratory, flexible, and based on qualitative observation analysis. Deductive research, on the other hand, is about proving something and is structured and based on quantitative analysis.
Inductive research is often used in qualitative research, case studies, and grounded theory research. Deductive Research. Deductive research is a top-down approach to research where a researcher starts with a theory or hypothesis and then tests it through data collection and analysis.
While quantitative research is generally thought to be deductive, quantitative researchers often do a bit of inductive reasoning to find meaning in data that hold surprises. Conversely, qualitative data are stereotypically analyzed inductively, making meaning from the data rather than proving a hypothesis.
The main difference between inductive and deductive approaches to research is that whilst a deductive approach is aimed and testing theory, an inductive approach is concerned with the generation of new theory emerging from the data. A deductive approach usually begins with a hypothesis, whilst an inductive approach will usually use research ...
Exploratory research is generally considered to be inductive and qualitative (Stebbins 2001).Exploratory qualitative studies adopting an inductive approach do not lend themselves to a priori theorizing and building upon prior bodies of knowledge (Reiter 2013; Bryman 2004 as cited in Pearse 2019).Juxtaposed against quantitative studies that employ deductive confirmatory approaches, exploratory ...
Purpose. The purpose of this paper is to explain the rationale for choosing the qualitative approach to research human resources practices, namely, recruitment and selection, training and development, performance management, rewards management, employee communication and participation, diversity management and work and life balance using deductive and inductive approaches to analyse data.
In social research, two research designs may be followed; one is inductive, and another is deductive. Strauss and Corbin described the inductive analysis as, "the researcher begins with an area of study and allows the theory to emerge from the data" (p. 12).Deductive design is a form of data analysis that aims to see if the findings are consistent with the investigator's previous ...
Most often, qualitative research follows an inductive process. In most instances, however, theory developed from qualitative investigation is untested theory. Both quantitative and qualitative ...
Although inductive and deductive reasoning are used in qualitative and quantitative research, it is important to stress the different roles of induction and deduction when models are applied to cases. A variable-based approach implies to draw conclusions about cases by means of logical deduction; a case-based approach implies to draw ...
Furthermore, Miles and Huberman (1994) stated that its flexibility allows for both inductive and deductive approaches, the latter of which resonates with the structured and theory-testing nature of post-positivist inquiry, thereby solidifying thematic analysis as a reliable, rigorous, and adaptable method in deductive research.
Jean Kaluza. Inductive coding is a data analysis process that involves reading and interpreting raw contextual data to develop themes, concepts, or a process model via interpretations based on data. Inductive coding can also be defined as a bottom-up approach where you start with nothing, developing the code as you analyze the dataset.
Deductive reasoning operates on the premise that if a theory is true and relevant to a specific context, then certain observations or data points should support it. In qualitative research, this begins with the formulation of a clear and testable hypothesis. Researchers then gather data that is relevant to the hypothesis and analyze it systematically to draw conclusions.
Purpose The purpose of this paper is to address the imbalance between inductive and deductive research in management and organizational studies and to suggest changes in the journal review and ...
All qualitative data were saved and stored on a password-protected University of Montana server. Hard-copy field notes were securely stored in a locked office on the university's main campus. Procedure. Data analysis included a deductive followed by an inductive approach.
Deductive reasoning starts with a general theory and moves towards a specific conclusion, whereas inductive reasoning begins with specific observations and builds up to a general theory. Your ...