- Resources Home 🏠
- Try SciSpace Copilot
- Search research papers
- Add Copilot Extension
- Try AI Detector
- Try Paraphraser
- Try Citation Generator
- April Papers
- June Papers
- July Papers

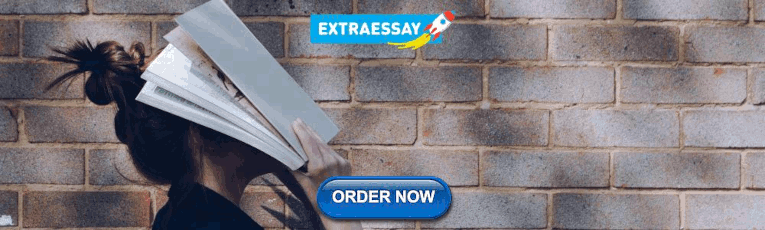
The Craft of Writing a Strong Hypothesis

Table of Contents
Writing a hypothesis is one of the essential elements of a scientific research paper. It needs to be to the point, clearly communicating what your research is trying to accomplish. A blurry, drawn-out, or complexly-structured hypothesis can confuse your readers. Or worse, the editor and peer reviewers.
A captivating hypothesis is not too intricate. This blog will take you through the process so that, by the end of it, you have a better idea of how to convey your research paper's intent in just one sentence.
What is a Hypothesis?
The first step in your scientific endeavor, a hypothesis, is a strong, concise statement that forms the basis of your research. It is not the same as a thesis statement , which is a brief summary of your research paper .
The sole purpose of a hypothesis is to predict your paper's findings, data, and conclusion. It comes from a place of curiosity and intuition . When you write a hypothesis, you're essentially making an educated guess based on scientific prejudices and evidence, which is further proven or disproven through the scientific method.
The reason for undertaking research is to observe a specific phenomenon. A hypothesis, therefore, lays out what the said phenomenon is. And it does so through two variables, an independent and dependent variable.
The independent variable is the cause behind the observation, while the dependent variable is the effect of the cause. A good example of this is “mixing red and blue forms purple.” In this hypothesis, mixing red and blue is the independent variable as you're combining the two colors at your own will. The formation of purple is the dependent variable as, in this case, it is conditional to the independent variable.
Different Types of Hypotheses

Types of hypotheses
Some would stand by the notion that there are only two types of hypotheses: a Null hypothesis and an Alternative hypothesis. While that may have some truth to it, it would be better to fully distinguish the most common forms as these terms come up so often, which might leave you out of context.
Apart from Null and Alternative, there are Complex, Simple, Directional, Non-Directional, Statistical, and Associative and casual hypotheses. They don't necessarily have to be exclusive, as one hypothesis can tick many boxes, but knowing the distinctions between them will make it easier for you to construct your own.
1. Null hypothesis
A null hypothesis proposes no relationship between two variables. Denoted by H 0 , it is a negative statement like “Attending physiotherapy sessions does not affect athletes' on-field performance.” Here, the author claims physiotherapy sessions have no effect on on-field performances. Even if there is, it's only a coincidence.
2. Alternative hypothesis
Considered to be the opposite of a null hypothesis, an alternative hypothesis is donated as H1 or Ha. It explicitly states that the dependent variable affects the independent variable. A good alternative hypothesis example is “Attending physiotherapy sessions improves athletes' on-field performance.” or “Water evaporates at 100 °C. ” The alternative hypothesis further branches into directional and non-directional.
- Directional hypothesis: A hypothesis that states the result would be either positive or negative is called directional hypothesis. It accompanies H1 with either the ‘<' or ‘>' sign.
- Non-directional hypothesis: A non-directional hypothesis only claims an effect on the dependent variable. It does not clarify whether the result would be positive or negative. The sign for a non-directional hypothesis is ‘≠.'
3. Simple hypothesis
A simple hypothesis is a statement made to reflect the relation between exactly two variables. One independent and one dependent. Consider the example, “Smoking is a prominent cause of lung cancer." The dependent variable, lung cancer, is dependent on the independent variable, smoking.
4. Complex hypothesis
In contrast to a simple hypothesis, a complex hypothesis implies the relationship between multiple independent and dependent variables. For instance, “Individuals who eat more fruits tend to have higher immunity, lesser cholesterol, and high metabolism.” The independent variable is eating more fruits, while the dependent variables are higher immunity, lesser cholesterol, and high metabolism.
5. Associative and casual hypothesis
Associative and casual hypotheses don't exhibit how many variables there will be. They define the relationship between the variables. In an associative hypothesis, changing any one variable, dependent or independent, affects others. In a casual hypothesis, the independent variable directly affects the dependent.
6. Empirical hypothesis
Also referred to as the working hypothesis, an empirical hypothesis claims a theory's validation via experiments and observation. This way, the statement appears justifiable and different from a wild guess.
Say, the hypothesis is “Women who take iron tablets face a lesser risk of anemia than those who take vitamin B12.” This is an example of an empirical hypothesis where the researcher the statement after assessing a group of women who take iron tablets and charting the findings.
7. Statistical hypothesis
The point of a statistical hypothesis is to test an already existing hypothesis by studying a population sample. Hypothesis like “44% of the Indian population belong in the age group of 22-27.” leverage evidence to prove or disprove a particular statement.
Characteristics of a Good Hypothesis
Writing a hypothesis is essential as it can make or break your research for you. That includes your chances of getting published in a journal. So when you're designing one, keep an eye out for these pointers:
- A research hypothesis has to be simple yet clear to look justifiable enough.
- It has to be testable — your research would be rendered pointless if too far-fetched into reality or limited by technology.
- It has to be precise about the results —what you are trying to do and achieve through it should come out in your hypothesis.
- A research hypothesis should be self-explanatory, leaving no doubt in the reader's mind.
- If you are developing a relational hypothesis, you need to include the variables and establish an appropriate relationship among them.
- A hypothesis must keep and reflect the scope for further investigations and experiments.
Separating a Hypothesis from a Prediction
Outside of academia, hypothesis and prediction are often used interchangeably. In research writing, this is not only confusing but also incorrect. And although a hypothesis and prediction are guesses at their core, there are many differences between them.
A hypothesis is an educated guess or even a testable prediction validated through research. It aims to analyze the gathered evidence and facts to define a relationship between variables and put forth a logical explanation behind the nature of events.
Predictions are assumptions or expected outcomes made without any backing evidence. They are more fictionally inclined regardless of where they originate from.
For this reason, a hypothesis holds much more weight than a prediction. It sticks to the scientific method rather than pure guesswork. "Planets revolve around the Sun." is an example of a hypothesis as it is previous knowledge and observed trends. Additionally, we can test it through the scientific method.
Whereas "COVID-19 will be eradicated by 2030." is a prediction. Even though it results from past trends, we can't prove or disprove it. So, the only way this gets validated is to wait and watch if COVID-19 cases end by 2030.
Finally, How to Write a Hypothesis
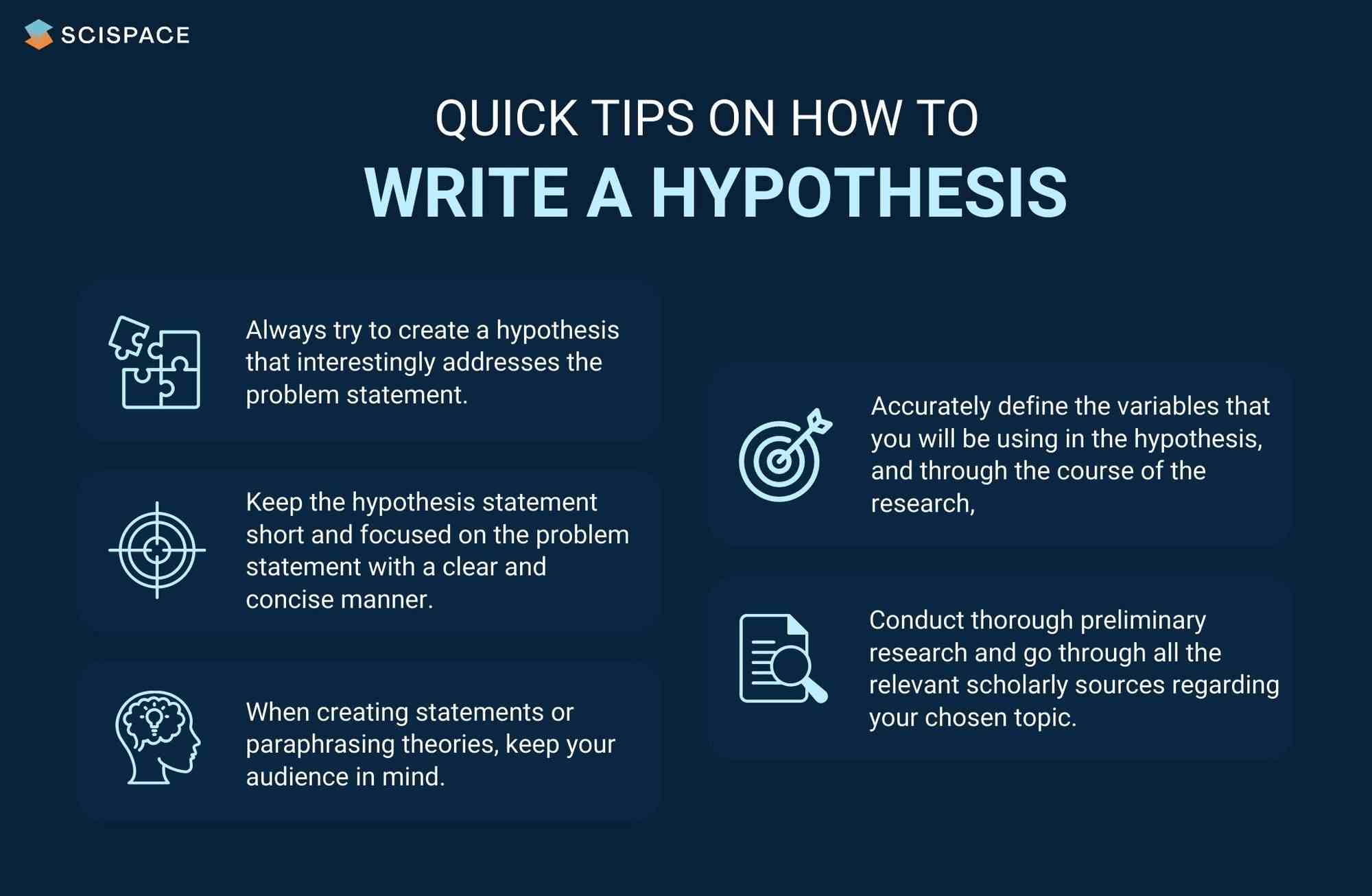
Quick tips on writing a hypothesis
1. Be clear about your research question
A hypothesis should instantly address the research question or the problem statement. To do so, you need to ask a question. Understand the constraints of your undertaken research topic and then formulate a simple and topic-centric problem. Only after that can you develop a hypothesis and further test for evidence.
2. Carry out a recce
Once you have your research's foundation laid out, it would be best to conduct preliminary research. Go through previous theories, academic papers, data, and experiments before you start curating your research hypothesis. It will give you an idea of your hypothesis's viability or originality.
Making use of references from relevant research papers helps draft a good research hypothesis. SciSpace Discover offers a repository of over 270 million research papers to browse through and gain a deeper understanding of related studies on a particular topic. Additionally, you can use SciSpace Copilot , your AI research assistant, for reading any lengthy research paper and getting a more summarized context of it. A hypothesis can be formed after evaluating many such summarized research papers. Copilot also offers explanations for theories and equations, explains paper in simplified version, allows you to highlight any text in the paper or clip math equations and tables and provides a deeper, clear understanding of what is being said. This can improve the hypothesis by helping you identify potential research gaps.
3. Create a 3-dimensional hypothesis
Variables are an essential part of any reasonable hypothesis. So, identify your independent and dependent variable(s) and form a correlation between them. The ideal way to do this is to write the hypothetical assumption in the ‘if-then' form. If you use this form, make sure that you state the predefined relationship between the variables.
In another way, you can choose to present your hypothesis as a comparison between two variables. Here, you must specify the difference you expect to observe in the results.
4. Write the first draft
Now that everything is in place, it's time to write your hypothesis. For starters, create the first draft. In this version, write what you expect to find from your research.
Clearly separate your independent and dependent variables and the link between them. Don't fixate on syntax at this stage. The goal is to ensure your hypothesis addresses the issue.
5. Proof your hypothesis
After preparing the first draft of your hypothesis, you need to inspect it thoroughly. It should tick all the boxes, like being concise, straightforward, relevant, and accurate. Your final hypothesis has to be well-structured as well.
Research projects are an exciting and crucial part of being a scholar. And once you have your research question, you need a great hypothesis to begin conducting research. Thus, knowing how to write a hypothesis is very important.
Now that you have a firmer grasp on what a good hypothesis constitutes, the different kinds there are, and what process to follow, you will find it much easier to write your hypothesis, which ultimately helps your research.
Now it's easier than ever to streamline your research workflow with SciSpace Discover . Its integrated, comprehensive end-to-end platform for research allows scholars to easily discover, write and publish their research and fosters collaboration.
It includes everything you need, including a repository of over 270 million research papers across disciplines, SEO-optimized summaries and public profiles to show your expertise and experience.
If you found these tips on writing a research hypothesis useful, head over to our blog on Statistical Hypothesis Testing to learn about the top researchers, papers, and institutions in this domain.
Frequently Asked Questions (FAQs)
1. what is the definition of hypothesis.
According to the Oxford dictionary, a hypothesis is defined as “An idea or explanation of something that is based on a few known facts, but that has not yet been proved to be true or correct”.
2. What is an example of hypothesis?
The hypothesis is a statement that proposes a relationship between two or more variables. An example: "If we increase the number of new users who join our platform by 25%, then we will see an increase in revenue."
3. What is an example of null hypothesis?
A null hypothesis is a statement that there is no relationship between two variables. The null hypothesis is written as H0. The null hypothesis states that there is no effect. For example, if you're studying whether or not a particular type of exercise increases strength, your null hypothesis will be "there is no difference in strength between people who exercise and people who don't."
4. What are the types of research?
• Fundamental research
• Applied research
• Qualitative research
• Quantitative research
• Mixed research
• Exploratory research
• Longitudinal research
• Cross-sectional research
• Field research
• Laboratory research
• Fixed research
• Flexible research
• Action research
• Policy research
• Classification research
• Comparative research
• Causal research
• Inductive research
• Deductive research
5. How to write a hypothesis?
• Your hypothesis should be able to predict the relationship and outcome.
• Avoid wordiness by keeping it simple and brief.
• Your hypothesis should contain observable and testable outcomes.
• Your hypothesis should be relevant to the research question.
6. What are the 2 types of hypothesis?
• Null hypotheses are used to test the claim that "there is no difference between two groups of data".
• Alternative hypotheses test the claim that "there is a difference between two data groups".
7. Difference between research question and research hypothesis?
A research question is a broad, open-ended question you will try to answer through your research. A hypothesis is a statement based on prior research or theory that you expect to be true due to your study. Example - Research question: What are the factors that influence the adoption of the new technology? Research hypothesis: There is a positive relationship between age, education and income level with the adoption of the new technology.
8. What is plural for hypothesis?
The plural of hypothesis is hypotheses. Here's an example of how it would be used in a statement, "Numerous well-considered hypotheses are presented in this part, and they are supported by tables and figures that are well-illustrated."
9. What is the red queen hypothesis?
The red queen hypothesis in evolutionary biology states that species must constantly evolve to avoid extinction because if they don't, they will be outcompeted by other species that are evolving. Leigh Van Valen first proposed it in 1973; since then, it has been tested and substantiated many times.
10. Who is known as the father of null hypothesis?
The father of the null hypothesis is Sir Ronald Fisher. He published a paper in 1925 that introduced the concept of null hypothesis testing, and he was also the first to use the term itself.
11. When to reject null hypothesis?
You need to find a significant difference between your two populations to reject the null hypothesis. You can determine that by running statistical tests such as an independent sample t-test or a dependent sample t-test. You should reject the null hypothesis if the p-value is less than 0.05.

You might also like

Consensus GPT vs. SciSpace GPT: Choose the Best GPT for Research
Literature Review and Theoretical Framework: Understanding the Differences

Types of Essays in Academic Writing - Quick Guide (2024)
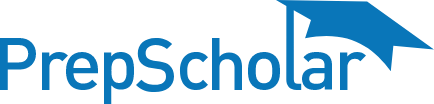
Choose Your Test
Sat / act prep online guides and tips, what is a hypothesis and how do i write one.
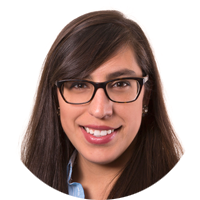
General Education
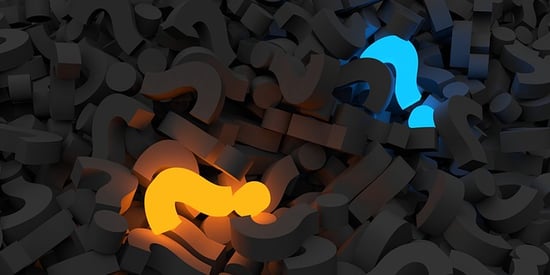
Think about something strange and unexplainable in your life. Maybe you get a headache right before it rains, or maybe you think your favorite sports team wins when you wear a certain color. If you wanted to see whether these are just coincidences or scientific fact, you would form a hypothesis, then create an experiment to see whether that hypothesis is true or not.
But what is a hypothesis, anyway? If you’re not sure about what a hypothesis is--or how to test for one!--you’re in the right place. This article will teach you everything you need to know about hypotheses, including:
- Defining the term “hypothesis”
- Providing hypothesis examples
- Giving you tips for how to write your own hypothesis
So let’s get started!
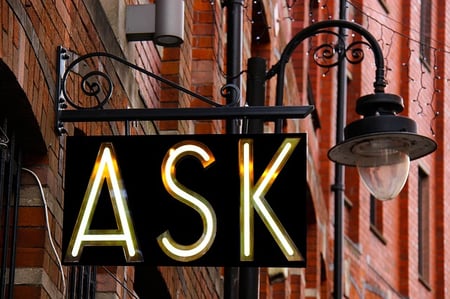
What Is a Hypothesis?
Merriam Webster defines a hypothesis as “an assumption or concession made for the sake of argument.” In other words, a hypothesis is an educated guess . Scientists make a reasonable assumption--or a hypothesis--then design an experiment to test whether it’s true or not. Keep in mind that in science, a hypothesis should be testable. You have to be able to design an experiment that tests your hypothesis in order for it to be valid.
As you could assume from that statement, it’s easy to make a bad hypothesis. But when you’re holding an experiment, it’s even more important that your guesses be good...after all, you’re spending time (and maybe money!) to figure out more about your observation. That’s why we refer to a hypothesis as an educated guess--good hypotheses are based on existing data and research to make them as sound as possible.
Hypotheses are one part of what’s called the scientific method . Every (good) experiment or study is based in the scientific method. The scientific method gives order and structure to experiments and ensures that interference from scientists or outside influences does not skew the results. It’s important that you understand the concepts of the scientific method before holding your own experiment. Though it may vary among scientists, the scientific method is generally made up of six steps (in order):
- Observation
- Asking questions
- Forming a hypothesis
- Analyze the data
- Communicate your results
You’ll notice that the hypothesis comes pretty early on when conducting an experiment. That’s because experiments work best when they’re trying to answer one specific question. And you can’t conduct an experiment until you know what you’re trying to prove!
Independent and Dependent Variables
After doing your research, you’re ready for another important step in forming your hypothesis: identifying variables. Variables are basically any factor that could influence the outcome of your experiment . Variables have to be measurable and related to the topic being studied.
There are two types of variables: independent variables and dependent variables. I ndependent variables remain constant . For example, age is an independent variable; it will stay the same, and researchers can look at different ages to see if it has an effect on the dependent variable.
Speaking of dependent variables... dependent variables are subject to the influence of the independent variable , meaning that they are not constant. Let’s say you want to test whether a person’s age affects how much sleep they need. In that case, the independent variable is age (like we mentioned above), and the dependent variable is how much sleep a person gets.
Variables will be crucial in writing your hypothesis. You need to be able to identify which variable is which, as both the independent and dependent variables will be written into your hypothesis. For instance, in a study about exercise, the independent variable might be the speed at which the respondents walk for thirty minutes, and the dependent variable would be their heart rate. In your study and in your hypothesis, you’re trying to understand the relationship between the two variables.
Elements of a Good Hypothesis
The best hypotheses start by asking the right questions . For instance, if you’ve observed that the grass is greener when it rains twice a week, you could ask what kind of grass it is, what elevation it’s at, and if the grass across the street responds to rain in the same way. Any of these questions could become the backbone of experiments to test why the grass gets greener when it rains fairly frequently.
As you’re asking more questions about your first observation, make sure you’re also making more observations . If it doesn’t rain for two weeks and the grass still looks green, that’s an important observation that could influence your hypothesis. You'll continue observing all throughout your experiment, but until the hypothesis is finalized, every observation should be noted.
Finally, you should consult secondary research before writing your hypothesis . Secondary research is comprised of results found and published by other people. You can usually find this information online or at your library. Additionally, m ake sure the research you find is credible and related to your topic. If you’re studying the correlation between rain and grass growth, it would help you to research rain patterns over the past twenty years for your county, published by a local agricultural association. You should also research the types of grass common in your area, the type of grass in your lawn, and whether anyone else has conducted experiments about your hypothesis. Also be sure you’re checking the quality of your research . Research done by a middle school student about what minerals can be found in rainwater would be less useful than an article published by a local university.
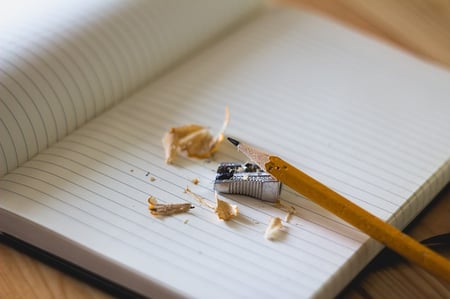
Writing Your Hypothesis
Once you’ve considered all of the factors above, you’re ready to start writing your hypothesis. Hypotheses usually take a certain form when they’re written out in a research report.
When you boil down your hypothesis statement, you are writing down your best guess and not the question at hand . This means that your statement should be written as if it is fact already, even though you are simply testing it.
The reason for this is that, after you have completed your study, you'll either accept or reject your if-then or your null hypothesis. All hypothesis testing examples should be measurable and able to be confirmed or denied. You cannot confirm a question, only a statement!
In fact, you come up with hypothesis examples all the time! For instance, when you guess on the outcome of a basketball game, you don’t say, “Will the Miami Heat beat the Boston Celtics?” but instead, “I think the Miami Heat will beat the Boston Celtics.” You state it as if it is already true, even if it turns out you’re wrong. You do the same thing when writing your hypothesis.
Additionally, keep in mind that hypotheses can range from very specific to very broad. These hypotheses can be specific, but if your hypothesis testing examples involve a broad range of causes and effects, your hypothesis can also be broad.
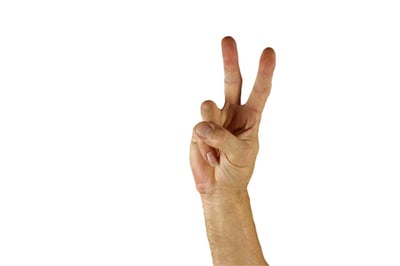
The Two Types of Hypotheses
Now that you understand what goes into a hypothesis, it’s time to look more closely at the two most common types of hypothesis: the if-then hypothesis and the null hypothesis.
#1: If-Then Hypotheses
First of all, if-then hypotheses typically follow this formula:
If ____ happens, then ____ will happen.
The goal of this type of hypothesis is to test the causal relationship between the independent and dependent variable. It’s fairly simple, and each hypothesis can vary in how detailed it can be. We create if-then hypotheses all the time with our daily predictions. Here are some examples of hypotheses that use an if-then structure from daily life:
- If I get enough sleep, I’ll be able to get more work done tomorrow.
- If the bus is on time, I can make it to my friend’s birthday party.
- If I study every night this week, I’ll get a better grade on my exam.
In each of these situations, you’re making a guess on how an independent variable (sleep, time, or studying) will affect a dependent variable (the amount of work you can do, making it to a party on time, or getting better grades).
You may still be asking, “What is an example of a hypothesis used in scientific research?” Take one of the hypothesis examples from a real-world study on whether using technology before bed affects children’s sleep patterns. The hypothesis read s:
“We hypothesized that increased hours of tablet- and phone-based screen time at bedtime would be inversely correlated with sleep quality and child attention.”
It might not look like it, but this is an if-then statement. The researchers basically said, “If children have more screen usage at bedtime, then their quality of sleep and attention will be worse.” The sleep quality and attention are the dependent variables and the screen usage is the independent variable. (Usually, the independent variable comes after the “if” and the dependent variable comes after the “then,” as it is the independent variable that affects the dependent variable.) This is an excellent example of how flexible hypothesis statements can be, as long as the general idea of “if-then” and the independent and dependent variables are present.
#2: Null Hypotheses
Your if-then hypothesis is not the only one needed to complete a successful experiment, however. You also need a null hypothesis to test it against. In its most basic form, the null hypothesis is the opposite of your if-then hypothesis . When you write your null hypothesis, you are writing a hypothesis that suggests that your guess is not true, and that the independent and dependent variables have no relationship .
One null hypothesis for the cell phone and sleep study from the last section might say:
“If children have more screen usage at bedtime, their quality of sleep and attention will not be worse.”
In this case, this is a null hypothesis because it’s asking the opposite of the original thesis!
Conversely, if your if-then hypothesis suggests that your two variables have no relationship, then your null hypothesis would suggest that there is one. So, pretend that there is a study that is asking the question, “Does the amount of followers on Instagram influence how long people spend on the app?” The independent variable is the amount of followers, and the dependent variable is the time spent. But if you, as the researcher, don’t think there is a relationship between the number of followers and time spent, you might write an if-then hypothesis that reads:
“If people have many followers on Instagram, they will not spend more time on the app than people who have less.”
In this case, the if-then suggests there isn’t a relationship between the variables. In that case, one of the null hypothesis examples might say:
“If people have many followers on Instagram, they will spend more time on the app than people who have less.”
You then test both the if-then and the null hypothesis to gauge if there is a relationship between the variables, and if so, how much of a relationship.
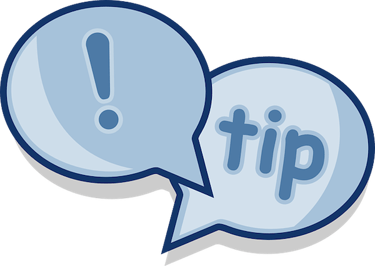
4 Tips to Write the Best Hypothesis
If you’re going to take the time to hold an experiment, whether in school or by yourself, you’re also going to want to take the time to make sure your hypothesis is a good one. The best hypotheses have four major elements in common: plausibility, defined concepts, observability, and general explanation.
#1: Plausibility
At first glance, this quality of a hypothesis might seem obvious. When your hypothesis is plausible, that means it’s possible given what we know about science and general common sense. However, improbable hypotheses are more common than you might think.
Imagine you’re studying weight gain and television watching habits. If you hypothesize that people who watch more than twenty hours of television a week will gain two hundred pounds or more over the course of a year, this might be improbable (though it’s potentially possible). Consequently, c ommon sense can tell us the results of the study before the study even begins.
Improbable hypotheses generally go against science, as well. Take this hypothesis example:
“If a person smokes one cigarette a day, then they will have lungs just as healthy as the average person’s.”
This hypothesis is obviously untrue, as studies have shown again and again that cigarettes negatively affect lung health. You must be careful that your hypotheses do not reflect your own personal opinion more than they do scientifically-supported findings. This plausibility points to the necessity of research before the hypothesis is written to make sure that your hypothesis has not already been disproven.
#2: Defined Concepts
The more advanced you are in your studies, the more likely that the terms you’re using in your hypothesis are specific to a limited set of knowledge. One of the hypothesis testing examples might include the readability of printed text in newspapers, where you might use words like “kerning” and “x-height.” Unless your readers have a background in graphic design, it’s likely that they won’t know what you mean by these terms. Thus, it’s important to either write what they mean in the hypothesis itself or in the report before the hypothesis.
Here’s what we mean. Which of the following sentences makes more sense to the common person?
If the kerning is greater than average, more words will be read per minute.
If the space between letters is greater than average, more words will be read per minute.
For people reading your report that are not experts in typography, simply adding a few more words will be helpful in clarifying exactly what the experiment is all about. It’s always a good idea to make your research and findings as accessible as possible.
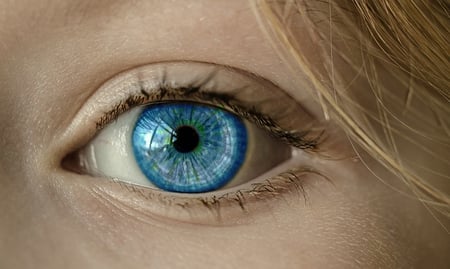
Good hypotheses ensure that you can observe the results.
#3: Observability
In order to measure the truth or falsity of your hypothesis, you must be able to see your variables and the way they interact. For instance, if your hypothesis is that the flight patterns of satellites affect the strength of certain television signals, yet you don’t have a telescope to view the satellites or a television to monitor the signal strength, you cannot properly observe your hypothesis and thus cannot continue your study.
Some variables may seem easy to observe, but if you do not have a system of measurement in place, you cannot observe your hypothesis properly. Here’s an example: if you’re experimenting on the effect of healthy food on overall happiness, but you don’t have a way to monitor and measure what “overall happiness” means, your results will not reflect the truth. Monitoring how often someone smiles for a whole day is not reasonably observable, but having the participants state how happy they feel on a scale of one to ten is more observable.
In writing your hypothesis, always keep in mind how you'll execute the experiment.
#4: Generalizability
Perhaps you’d like to study what color your best friend wears the most often by observing and documenting the colors she wears each day of the week. This might be fun information for her and you to know, but beyond you two, there aren’t many people who could benefit from this experiment. When you start an experiment, you should note how generalizable your findings may be if they are confirmed. Generalizability is basically how common a particular phenomenon is to other people’s everyday life.
Let’s say you’re asking a question about the health benefits of eating an apple for one day only, you need to realize that the experiment may be too specific to be helpful. It does not help to explain a phenomenon that many people experience. If you find yourself with too specific of a hypothesis, go back to asking the big question: what is it that you want to know, and what do you think will happen between your two variables?
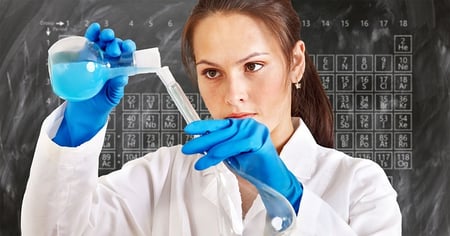
Hypothesis Testing Examples
We know it can be hard to write a good hypothesis unless you’ve seen some good hypothesis examples. We’ve included four hypothesis examples based on some made-up experiments. Use these as templates or launch pads for coming up with your own hypotheses.
Experiment #1: Students Studying Outside (Writing a Hypothesis)
You are a student at PrepScholar University. When you walk around campus, you notice that, when the temperature is above 60 degrees, more students study in the quad. You want to know when your fellow students are more likely to study outside. With this information, how do you make the best hypothesis possible?
You must remember to make additional observations and do secondary research before writing your hypothesis. In doing so, you notice that no one studies outside when it’s 75 degrees and raining, so this should be included in your experiment. Also, studies done on the topic beforehand suggested that students are more likely to study in temperatures less than 85 degrees. With this in mind, you feel confident that you can identify your variables and write your hypotheses:
If-then: “If the temperature in Fahrenheit is less than 60 degrees, significantly fewer students will study outside.”
Null: “If the temperature in Fahrenheit is less than 60 degrees, the same number of students will study outside as when it is more than 60 degrees.”
These hypotheses are plausible, as the temperatures are reasonably within the bounds of what is possible. The number of people in the quad is also easily observable. It is also not a phenomenon specific to only one person or at one time, but instead can explain a phenomenon for a broader group of people.
To complete this experiment, you pick the month of October to observe the quad. Every day (except on the days where it’s raining)from 3 to 4 PM, when most classes have released for the day, you observe how many people are on the quad. You measure how many people come and how many leave. You also write down the temperature on the hour.
After writing down all of your observations and putting them on a graph, you find that the most students study on the quad when it is 70 degrees outside, and that the number of students drops a lot once the temperature reaches 60 degrees or below. In this case, your research report would state that you accept or “failed to reject” your first hypothesis with your findings.
Experiment #2: The Cupcake Store (Forming a Simple Experiment)
Let’s say that you work at a bakery. You specialize in cupcakes, and you make only two colors of frosting: yellow and purple. You want to know what kind of customers are more likely to buy what kind of cupcake, so you set up an experiment. Your independent variable is the customer’s gender, and the dependent variable is the color of the frosting. What is an example of a hypothesis that might answer the question of this study?
Here’s what your hypotheses might look like:
If-then: “If customers’ gender is female, then they will buy more yellow cupcakes than purple cupcakes.”
Null: “If customers’ gender is female, then they will be just as likely to buy purple cupcakes as yellow cupcakes.”
This is a pretty simple experiment! It passes the test of plausibility (there could easily be a difference), defined concepts (there’s nothing complicated about cupcakes!), observability (both color and gender can be easily observed), and general explanation ( this would potentially help you make better business decisions ).
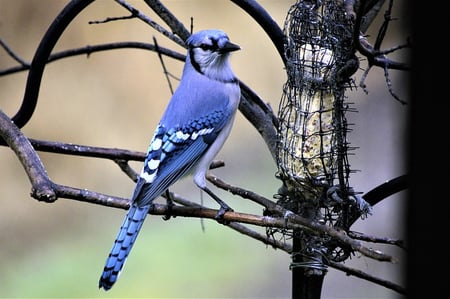
Experiment #3: Backyard Bird Feeders (Integrating Multiple Variables and Rejecting the If-Then Hypothesis)
While watching your backyard bird feeder, you realized that different birds come on the days when you change the types of seeds. You decide that you want to see more cardinals in your backyard, so you decide to see what type of food they like the best and set up an experiment.
However, one morning, you notice that, while some cardinals are present, blue jays are eating out of your backyard feeder filled with millet. You decide that, of all of the other birds, you would like to see the blue jays the least. This means you'll have more than one variable in your hypothesis. Your new hypotheses might look like this:
If-then: “If sunflower seeds are placed in the bird feeders, then more cardinals will come than blue jays. If millet is placed in the bird feeders, then more blue jays will come than cardinals.”
Null: “If either sunflower seeds or millet are placed in the bird, equal numbers of cardinals and blue jays will come.”
Through simple observation, you actually find that cardinals come as often as blue jays when sunflower seeds or millet is in the bird feeder. In this case, you would reject your “if-then” hypothesis and “fail to reject” your null hypothesis . You cannot accept your first hypothesis, because it’s clearly not true. Instead you found that there was actually no relation between your different variables. Consequently, you would need to run more experiments with different variables to see if the new variables impact the results.
Experiment #4: In-Class Survey (Including an Alternative Hypothesis)
You’re about to give a speech in one of your classes about the importance of paying attention. You want to take this opportunity to test a hypothesis you’ve had for a while:
If-then: If students sit in the first two rows of the classroom, then they will listen better than students who do not.
Null: If students sit in the first two rows of the classroom, then they will not listen better or worse than students who do not.
You give your speech and then ask your teacher if you can hand out a short survey to the class. On the survey, you’ve included questions about some of the topics you talked about. When you get back the results, you’re surprised to see that not only do the students in the first two rows not pay better attention, but they also scored worse than students in other parts of the classroom! Here, both your if-then and your null hypotheses are not representative of your findings. What do you do?
This is when you reject both your if-then and null hypotheses and instead create an alternative hypothesis . This type of hypothesis is used in the rare circumstance that neither of your hypotheses is able to capture your findings . Now you can use what you’ve learned to draft new hypotheses and test again!
Key Takeaways: Hypothesis Writing
The more comfortable you become with writing hypotheses, the better they will become. The structure of hypotheses is flexible and may need to be changed depending on what topic you are studying. The most important thing to remember is the purpose of your hypothesis and the difference between the if-then and the null . From there, in forming your hypothesis, you should constantly be asking questions, making observations, doing secondary research, and considering your variables. After you have written your hypothesis, be sure to edit it so that it is plausible, clearly defined, observable, and helpful in explaining a general phenomenon.
Writing a hypothesis is something that everyone, from elementary school children competing in a science fair to professional scientists in a lab, needs to know how to do. Hypotheses are vital in experiments and in properly executing the scientific method . When done correctly, hypotheses will set up your studies for success and help you to understand the world a little better, one experiment at a time.
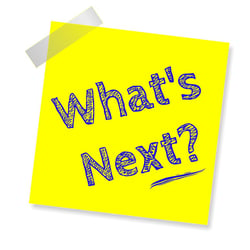
What’s Next?
If you’re studying for the science portion of the ACT, there’s definitely a lot you need to know. We’ve got the tools to help, though! Start by checking out our ultimate study guide for the ACT Science subject test. Once you read through that, be sure to download our recommended ACT Science practice tests , since they’re one of the most foolproof ways to improve your score. (And don’t forget to check out our expert guide book , too.)
If you love science and want to major in a scientific field, you should start preparing in high school . Here are the science classes you should take to set yourself up for success.
If you’re trying to think of science experiments you can do for class (or for a science fair!), here’s a list of 37 awesome science experiments you can do at home
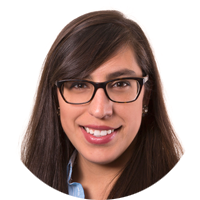
Ashley Sufflé Robinson has a Ph.D. in 19th Century English Literature. As a content writer for PrepScholar, Ashley is passionate about giving college-bound students the in-depth information they need to get into the school of their dreams.
Student and Parent Forum
Our new student and parent forum, at ExpertHub.PrepScholar.com , allow you to interact with your peers and the PrepScholar staff. See how other students and parents are navigating high school, college, and the college admissions process. Ask questions; get answers.

Ask a Question Below
Have any questions about this article or other topics? Ask below and we'll reply!
Improve With Our Famous Guides
- For All Students
The 5 Strategies You Must Be Using to Improve 160+ SAT Points
How to Get a Perfect 1600, by a Perfect Scorer
Series: How to Get 800 on Each SAT Section:
Score 800 on SAT Math
Score 800 on SAT Reading
Score 800 on SAT Writing
Series: How to Get to 600 on Each SAT Section:
Score 600 on SAT Math
Score 600 on SAT Reading
Score 600 on SAT Writing
Free Complete Official SAT Practice Tests
What SAT Target Score Should You Be Aiming For?
15 Strategies to Improve Your SAT Essay
The 5 Strategies You Must Be Using to Improve 4+ ACT Points
How to Get a Perfect 36 ACT, by a Perfect Scorer
Series: How to Get 36 on Each ACT Section:
36 on ACT English
36 on ACT Math
36 on ACT Reading
36 on ACT Science
Series: How to Get to 24 on Each ACT Section:
24 on ACT English
24 on ACT Math
24 on ACT Reading
24 on ACT Science
What ACT target score should you be aiming for?
ACT Vocabulary You Must Know
ACT Writing: 15 Tips to Raise Your Essay Score
How to Get Into Harvard and the Ivy League
How to Get a Perfect 4.0 GPA
How to Write an Amazing College Essay
What Exactly Are Colleges Looking For?
Is the ACT easier than the SAT? A Comprehensive Guide
Should you retake your SAT or ACT?
When should you take the SAT or ACT?
Stay Informed

Get the latest articles and test prep tips!
Looking for Graduate School Test Prep?
Check out our top-rated graduate blogs here:
GRE Online Prep Blog
GMAT Online Prep Blog
TOEFL Online Prep Blog
Holly R. "I am absolutely overjoyed and cannot thank you enough for helping me!”
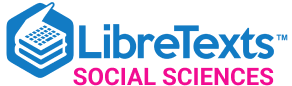
- school Campus Bookshelves
- menu_book Bookshelves
- perm_media Learning Objects
- login Login
- how_to_reg Request Instructor Account
- hub Instructor Commons
- Download Page (PDF)
- Download Full Book (PDF)
- Periodic Table
- Physics Constants
- Scientific Calculator
- Reference & Cite
- Tools expand_more
- Readability
selected template will load here
This action is not available.
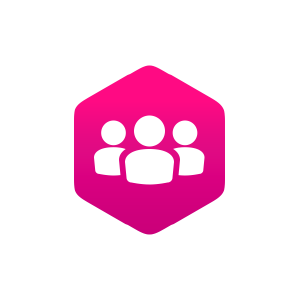
3.1.3: Developing Theories and Hypotheses
- Last updated
- Save as PDF
- Page ID 109843
2.5: Developing a Hypothesis
Learning objectives.
- Distinguish between a theory and a hypothesis.
- Discover how theories are used to generate hypotheses and how the results of studies can be used to further inform theories.
- Understand the characteristics of a good hypothesis.
Theories and Hypotheses
Before describing how to develop a hypothesis, it is important to distinguish between a theory and a hypothesis. A theory is a coherent explanation or interpretation of one or more phenomena. Although theories can take a variety of forms, one thing they have in common is that they go beyond the phenomena they explain by including variables, structures, processes, functions, or organizing principles that have not been observed directly. Consider, for example, Zajonc’s theory of social facilitation and social inhibition (1965) [1] . He proposed that being watched by others while performing a task creates a general state of physiological arousal, which increases the likelihood of the dominant (most likely) response. So for highly practiced tasks, being watched increases the tendency to make correct responses, but for relatively unpracticed tasks, being watched increases the tendency to make incorrect responses. Notice that this theory—which has come to be called drive theory—provides an explanation of both social facilitation and social inhibition that goes beyond the phenomena themselves by including concepts such as “arousal” and “dominant response,” along with processes such as the effect of arousal on the dominant response.
Outside of science, referring to an idea as a theory often implies that it is untested—perhaps no more than a wild guess. In science, however, the term theory has no such implication. A theory is simply an explanation or interpretation of a set of phenomena. It can be untested, but it can also be extensively tested, well supported, and accepted as an accurate description of the world by the scientific community. The theory of evolution by natural selection, for example, is a theory because it is an explanation of the diversity of life on earth—not because it is untested or unsupported by scientific research. On the contrary, the evidence for this theory is overwhelmingly positive and nearly all scientists accept its basic assumptions as accurate. Similarly, the “germ theory” of disease is a theory because it is an explanation of the origin of various diseases, not because there is any doubt that many diseases are caused by microorganisms that infect the body.
A hypothesis , on the other hand, is a specific prediction about a new phenomenon that should be observed if a particular theory is accurate. It is an explanation that relies on just a few key concepts. Hypotheses are often specific predictions about what will happen in a particular study. They are developed by considering existing evidence and using reasoning to infer what will happen in the specific context of interest. Hypotheses are often but not always derived from theories. So a hypothesis is often a prediction based on a theory but some hypotheses are a-theoretical and only after a set of observations have been made, is a theory developed. This is because theories are broad in nature and they explain larger bodies of data. So if our research question is really original then we may need to collect some data and make some observations before we can develop a broader theory.
Theories and hypotheses always have this if-then relationship. “ If drive theory is correct, then cockroaches should run through a straight runway faster, and a branching runway more slowly, when other cockroaches are present.” Although hypotheses are usually expressed as statements, they can always be rephrased as questions. “Do cockroaches run through a straight runway faster when other cockroaches are present?” Thus deriving hypotheses from theories is an excellent way of generating interesting research questions.
But how do researchers derive hypotheses from theories? One way is to generate a research question using the techniques discussed in this chapter and then ask whether any theory implies an answer to that question. For example, you might wonder whether expressive writing about positive experiences improves health as much as expressive writing about traumatic experiences. Although this question is an interesting one on its own, you might then ask whether the habituation theory—the idea that expressive writing causes people to habituate to negative thoughts and feelings—implies an answer. In this case, it seems clear that if the habituation theory is correct, then expressive writing about positive experiences should not be effective because it would not cause people to habituate to negative thoughts and feelings. A second way to derive hypotheses from theories is to focus on some component of the theory that has not yet been directly observed. For example, a researcher could focus on the process of habituation—perhaps hypothesizing that people should show fewer signs of emotional distress with each new writing session.
Among the very best hypotheses are those that distinguish between competing theories. For example, Norbert Schwarz and his colleagues considered two theories of how people make judgments about themselves, such as how assertive they are (Schwarz et al., 1991) [2] . Both theories held that such judgments are based on relevant examples that people bring to mind. However, one theory was that people base their judgments on the number of examples they bring to mind and the other was that people base their judgments on how easily they bring those examples to mind. To test these theories, the researchers asked people to recall either six times when they were assertive (which is easy for most people) or 12 times (which is difficult for most people). Then they asked them to judge their own assertiveness. Note that the number-of-examples theory implies that people who recalled 12 examples should judge themselves to be more assertive because they recalled more examples, but the ease-of-examples theory implies that participants who recalled six examples should judge themselves as more assertive because recalling the examples was easier. Thus the two theories made opposite predictions so that only one of the predictions could be confirmed. The surprising result was that participants who recalled fewer examples judged themselves to be more assertive—providing particularly convincing evidence in favor of the ease-of-retrieval theory over the number-of-examples theory.
Theory Testing
The primary way that scientific researchers use theories is sometimes called the hypothetico-deductive method (although this term is much more likely to be used by philosophers of science than by scientists themselves). Researchers begin with a set of phenomena and either construct a theory to explain or interpret them or choose an existing theory to work with. They then make a prediction about some new phenomenon that should be observed if the theory is correct. Again, this prediction is called a hypothesis. The researchers then conduct an empirical study to test the hypothesis. Finally, they reevaluate the theory in light of the new results and revise it if necessary. This process is usually conceptualized as a cycle because the researchers can then derive a new hypothesis from the revised theory, conduct a new empirical study to test the hypothesis, and so on. As Figure \(\PageIndex{1}\) shows, this approach meshes nicely with the model of scientific research in psychology presented earlier in the textbook—creating a more detailed model of “theoretically motivated” or “theory-driven” research.
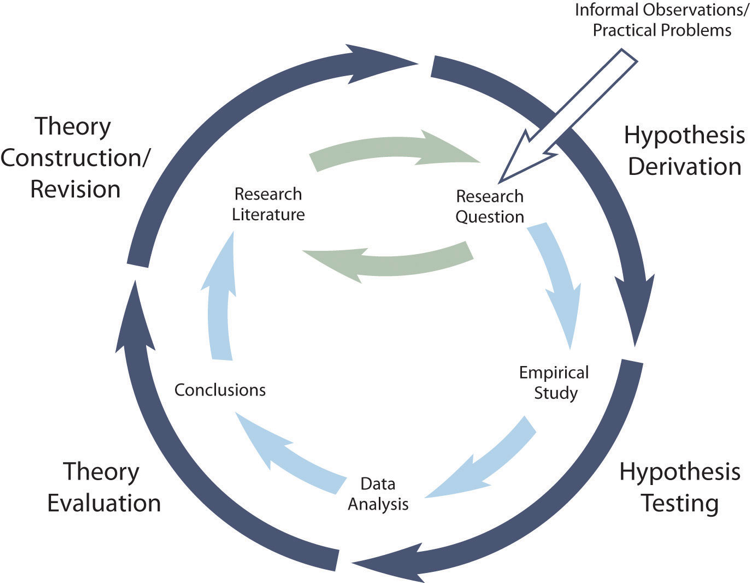
As an example, let us consider Zajonc’s research on social facilitation and inhibition. He started with a somewhat contradictory pattern of results from the research literature. He then constructed his drive theory, according to which being watched by others while performing a task causes physiological arousal, which increases an organism’s tendency to make the dominant response. This theory predicts social facilitation for well-learned tasks and social inhibition for poorly learned tasks. He now had a theory that organized previous results in a meaningful way—but he still needed to test it. He hypothesized that if his theory was correct, he should observe that the presence of others improves performance in a simple laboratory task but inhibits performance in a difficult version of the very same laboratory task. To test this hypothesis, one of the studies he conducted used cockroaches as subjects (Zajonc, Heingartner, & Herman, 1969) [3] . The cockroaches ran either down a straight runway (an easy task for a cockroach) or through a cross-shaped maze (a difficult task for a cockroach) to escape into a dark chamber when a light was shined on them. They did this either while alone or in the presence of other cockroaches in clear plastic “audience boxes.” Zajonc found that cockroaches in the straight runway reached their goal more quickly in the presence of other cockroaches, but cockroaches in the cross-shaped maze reached their goal more slowly when they were in the presence of other cockroaches. Thus he confirmed his hypothesis and provided support for his drive theory. (Zajonc also showed that drive theory existed in humans [Zajonc & Sales, 1966] [4] in many other studies afterward).
Incorporating Theory into Your Research
When you write your research report or plan your presentation, be aware that there are two basic ways that researchers usually include theory. The first is to raise a research question, answer that question by conducting a new study, and then offer one or more theories (usually more) to explain or interpret the results. This format works well for applied research questions and for research questions that existing theories do not address. The second way is to describe one or more existing theories, derive a hypothesis from one of those theories, test the hypothesis in a new study, and finally reevaluate the theory. This format works well when there is an existing theory that addresses the research question—especially if the resulting hypothesis is surprising or conflicts with a hypothesis derived from a different theory.
To use theories in your research will not only give you guidance in coming up with experiment ideas and possible projects, but it lends legitimacy to your work. Psychologists have been interested in a variety of human behaviors and have developed many theories along the way. Using established theories will help you break new ground as a researcher, not limit you from developing your own ideas.
There are three general characteristics of a good hypothesis. First, a good hypothesis must be testable and falsifiable . We must be able to test the hypothesis using the methods of science and if you’ll recall Popper’s falsifiability criterion, it must be possible to gather evidence that will disconfirm the hypothesis if it is indeed false. Second, a good hypothesis must be logical. As described above, hypotheses are more than just a random guess. Hypotheses should be informed by previous theories or observations and logical reasoning. Typically, we begin with a broad and general theory and use deductive reasoning to generate a more specific hypothesis to test based on that theory. Occasionally, however, when there is no theory to inform our hypothesis, we use inductive reasoning which involves using specific observations or research findings to form a more general hypothesis. Finally, the hypothesis should be positive. That is, the hypothesis should make a positive statement about the existence of a relationship or effect, rather than a statement that a relationship or effect does not exist. As scientists, we don’t set out to show that relationships do not exist or that effects do not occur so our hypotheses should not be worded in a way to suggest that an effect or relationship does not exist. The nature of science is to assume that something does not exist and then seek to find evidence to prove this wrong, to show that it really does exist. That may seem backward to you but that is the nature of the scientific method. The underlying reason for this is beyond the scope of this chapter but it has to do with statistical theory.
- Zajonc, R. B. (1965). Social facilitation. Science, 149 , 269–274 ↵
- Schwarz, N., Bless, H., Strack, F., Klumpp, G., Rittenauer-Schatka, H., & Simons, A. (1991). Ease of retrieval as information: Another look at the availability heuristic. Journal of Personality and Social Psychology, 61 , 195–202. ↵
- Zajonc, R. B., Heingartner, A., & Herman, E. M. (1969). Social enhancement and impairment of performance in the cockroach. Journal of Personality and Social Psychology, 13 , 83–92. ↵
- Zajonc, R.B. & Sales, S.M. (1966). Social facilitation of dominant and subordinate responses. Journal of Experimental Social Psychology, 2 , 160-168. ↵
2.4 Developing a Hypothesis
Learning objectives.
- Distinguish between a theory and a hypothesis.
- Discover how theories are used to generate hypotheses and how the results of studies can be used to further inform theories.
- Understand the characteristics of a good hypothesis.
Theories and Hypotheses
Before describing how to develop a hypothesis it is imporant to distinguish betwee a theory and a hypothesis. A theory is a coherent explanation or interpretation of one or more phenomena. Although theories can take a variety of forms, one thing they have in common is that they go beyond the phenomena they explain by including variables, structures, processes, functions, or organizing principles that have not been observed directly. Consider, for example, Zajonc’s theory of social facilitation and social inhibition. He proposed that being watched by others while performing a task creates a general state of physiological arousal, which increases the likelihood of the dominant (most likely) response. So for highly practiced tasks, being watched increases the tendency to make correct responses, but for relatively unpracticed tasks, being watched increases the tendency to make incorrect responses. Notice that this theory—which has come to be called drive theory—provides an explanation of both social facilitation and social inhibition that goes beyond the phenomena themselves by including concepts such as “arousal” and “dominant response,” along with processes such as the effect of arousal on the dominant response.
Outside of science, referring to an idea as a theory often implies that it is untested—perhaps no more than a wild guess. In science, however, the term theory has no such implication. A theory is simply an explanation or interpretation of a set of phenomena. It can be untested, but it can also be extensively tested, well supported, and accepted as an accurate description of the world by the scientific community. The theory of evolution by natural selection, for example, is a theory because it is an explanation of the diversity of life on earth—not because it is untested or unsupported by scientific research. On the contrary, the evidence for this theory is overwhelmingly positive and nearly all scientists accept its basic assumptions as accurate. Similarly, the “germ theory” of disease is a theory because it is an explanation of the origin of various diseases, not because there is any doubt that many diseases are caused by microorganisms that infect the body.
A hypothesis , on the other hand, is a specific prediction about a new phenomenon that should be observed if a particular theory is accurate. It is an explanation that relies on just a few key concepts. Hypotheses are often specific predictions about what will happen in a particular study. They are developed by considering existing evidence and using reasoning to infer what will happen in the specific context of interest. Hypotheses are often but not always derived from theories. So a hypothesis is often a prediction based on a theory but some hypotheses are a-theoretical and only after a set of observations have been made, is a theory developed. This is because theories are broad in nature and they explain larger bodies of data. So if our research question is really original then we may need to collect some data and make some observation before we can develop a broader theory.
Theories and hypotheses always have this if-then relationship. “ If drive theory is correct, then cockroaches should run through a straight runway faster, and a branching runway more slowly, when other cockroaches are present.” Although hypotheses are usually expressed as statements, they can always be rephrased as questions. “Do cockroaches run through a straight runway faster when other cockroaches are present?” Thus deriving hypotheses from theories is an excellent way of generating interesting research questions.
But how do researchers derive hypotheses from theories? One way is to generate a research question using the techniques discussed in this chapter and then ask whether any theory implies an answer to that question. For example, you might wonder whether expressive writing about positive experiences improves health as much as expressive writing about traumatic experiences. Although this question is an interesting one on its own, you might then ask whether the habituation theory—the idea that expressive writing causes people to habituate to negative thoughts and feelings—implies an answer. In this case, it seems clear that if the habituation theory is correct, then expressive writing about positive experiences should not be effective because it would not cause people to habituate to negative thoughts and feelings. A second way to derive hypotheses from theories is to focus on some component of the theory that has not yet been directly observed. For example, a researcher could focus on the process of habituation—perhaps hypothesizing that people should show fewer signs of emotional distress with each new writing session.
Among the very best hypotheses are those that distinguish between competing theories. For example, Norbert Schwarz and his colleagues considered two theories of how people make judgments about themselves, such as how assertive they are (Schwarz et al., 1991) [1] . Both theories held that such judgments are based on relevant examples that people bring to mind. However, one theory was that people base their judgments on the number of examples they bring to mind and the other was that people base their judgments on how easily they bring those examples to mind. To test these theories, the researchers asked people to recall either six times when they were assertive (which is easy for most people) or 12 times (which is difficult for most people). Then they asked them to judge their own assertiveness. Note that the number-of-examples theory implies that people who recalled 12 examples should judge themselves to be more assertive because they recalled more examples, but the ease-of-examples theory implies that participants who recalled six examples should judge themselves as more assertive because recalling the examples was easier. Thus the two theories made opposite predictions so that only one of the predictions could be confirmed. The surprising result was that participants who recalled fewer examples judged themselves to be more assertive—providing particularly convincing evidence in favor of the ease-of-retrieval theory over the number-of-examples theory.
Theory Testing
The primary way that scientific researchers use theories is sometimes called the hypothetico-deductive method (although this term is much more likely to be used by philosophers of science than by scientists themselves). A researcher begins with a set of phenomena and either constructs a theory to explain or interpret them or chooses an existing theory to work with. He or she then makes a prediction about some new phenomenon that should be observed if the theory is correct. Again, this prediction is called a hypothesis. The researcher then conducts an empirical study to test the hypothesis. Finally, he or she reevaluates the theory in light of the new results and revises it if necessary. This process is usually conceptualized as a cycle because the researcher can then derive a new hypothesis from the revised theory, conduct a new empirical study to test the hypothesis, and so on. As Figure 2.2 shows, this approach meshes nicely with the model of scientific research in psychology presented earlier in the textbook—creating a more detailed model of “theoretically motivated” or “theory-driven” research.

Figure 2.2 Hypothetico-Deductive Method Combined With the General Model of Scientific Research in Psychology Together they form a model of theoretically motivated research.
As an example, let us consider Zajonc’s research on social facilitation and inhibition. He started with a somewhat contradictory pattern of results from the research literature. He then constructed his drive theory, according to which being watched by others while performing a task causes physiological arousal, which increases an organism’s tendency to make the dominant response. This theory predicts social facilitation for well-learned tasks and social inhibition for poorly learned tasks. He now had a theory that organized previous results in a meaningful way—but he still needed to test it. He hypothesized that if his theory was correct, he should observe that the presence of others improves performance in a simple laboratory task but inhibits performance in a difficult version of the very same laboratory task. To test this hypothesis, one of the studies he conducted used cockroaches as subjects (Zajonc, Heingartner, & Herman, 1969) [2] . The cockroaches ran either down a straight runway (an easy task for a cockroach) or through a cross-shaped maze (a difficult task for a cockroach) to escape into a dark chamber when a light was shined on them. They did this either while alone or in the presence of other cockroaches in clear plastic “audience boxes.” Zajonc found that cockroaches in the straight runway reached their goal more quickly in the presence of other cockroaches, but cockroaches in the cross-shaped maze reached their goal more slowly when they were in the presence of other cockroaches. Thus he confirmed his hypothesis and provided support for his drive theory. (Zajonc also showed that drive theory existed in humans (Zajonc & Sales, 1966) [3] in many other studies afterward).
Incorporating Theory into Your Research
When you write your research report or plan your presentation, be aware that there are two basic ways that researchers usually include theory. The first is to raise a research question, answer that question by conducting a new study, and then offer one or more theories (usually more) to explain or interpret the results. This format works well for applied research questions and for research questions that existing theories do not address. The second way is to describe one or more existing theories, derive a hypothesis from one of those theories, test the hypothesis in a new study, and finally reevaluate the theory. This format works well when there is an existing theory that addresses the research question—especially if the resulting hypothesis is surprising or conflicts with a hypothesis derived from a different theory.
To use theories in your research will not only give you guidance in coming up with experiment ideas and possible projects, but it lends legitimacy to your work. Psychologists have been interested in a variety of human behaviors and have developed many theories along the way. Using established theories will help you break new ground as a researcher, not limit you from developing your own ideas.
Characteristics of a Good Hypothesis
There are three general characteristics of a good hypothesis. First, a good hypothesis must be testable and falsifiable . We must be able to test the hypothesis using the methods of science and if you’ll recall Popper’s falsifiability criterion, it must be possible to gather evidence that will disconfirm the hypothesis if it is indeed false. Second, a good hypothesis must be logical. As described above, hypotheses are more than just a random guess. Hypotheses should be informed by previous theories or observations and logical reasoning. Typically, we begin with a broad and general theory and use deductive reasoning to generate a more specific hypothesis to test based on that theory. Occasionally, however, when there is no theory to inform our hypothesis, we use inductive reasoning which involves using specific observations or research findings to form a more general hypothesis. Finally, the hypothesis should be positive. That is, the hypothesis should make a positive statement about the existence of a relationship or effect, rather than a statement that a relationship or effect does not exist. As scientists, we don’t set out to show that relationships do not exist or that effects do not occur so our hypotheses should not be worded in a way to suggest that an effect or relationship does not exist. The nature of science is to assume that something does not exist and then seek to find evidence to prove this wrong, to show that really it does exist. That may seem backward to you but that is the nature of the scientific method. The underlying reason for this is beyond the scope of this chapter but it has to do with statistical theory.
Key Takeaways
- A theory is broad in nature and explains larger bodies of data. A hypothesis is more specific and makes a prediction about the outcome of a particular study.
- Working with theories is not “icing on the cake.” It is a basic ingredient of psychological research.
- Like other scientists, psychologists use the hypothetico-deductive method. They construct theories to explain or interpret phenomena (or work with existing theories), derive hypotheses from their theories, test the hypotheses, and then reevaluate the theories in light of the new results.
- Practice: Find a recent empirical research report in a professional journal. Read the introduction and highlight in different colors descriptions of theories and hypotheses.
- Schwarz, N., Bless, H., Strack, F., Klumpp, G., Rittenauer-Schatka, H., & Simons, A. (1991). Ease of retrieval as information: Another look at the availability heuristic. Journal of Personality and Social Psychology, 61 , 195–202. ↵
- Zajonc, R. B., Heingartner, A., & Herman, E. M. (1969). Social enhancement and impairment of performance in the cockroach. Journal of Personality and Social Psychology, 13 , 83–92. ↵
- Zajonc, R.B. & Sales, S.M. (1966). Social facilitation of dominant and subordinate responses. Journal of Experimental Social Psychology, 2 , 160-168. ↵

Share This Book
- Increase Font Size
Crafting a hypothesis: understanding its types and how to write your own
Dec 15, 2022

A hypothesis serves as a statement that elucidates the predictions and reasoning of your research, akin to an 'educated guess' about the outcomes of your scientific experiments. Within the scientific method, a well-crafted hypothesis holds great significance, even though expressing even the simplest ones in words can be challenging.
Curious about how to construct a hypothesis for your academic paper? Below, we delve into the various types of hypotheses, the essential elements of a good hypothesis, step-by-step guidelines for creating your own, and numerous examples.
What is a Hypothesis?
As one of the ten essential words for university success, a hypothesis plays a foundational role in the scientific method. It essentially represents an educated guess based on observations, predicting the results of your experiment or research.
Examples of Hypotheses:
1. If I water plants daily, they will grow faster.
2. Adults can more accurately guess the temperature than children can.
3. Butterflies prefer white flowers to orange ones.
For instance, if you have noticed that daily watering promotes faster plant growth, your hypothesis might be: "Plants grow better with regular watering." Subsequently, you can perform experiments to test this hypothesis, such as comparing two plants - one watered regularly and the other not - and recording the results to observe differences.
Understanding Variables:
The language of hypotheses invariably revolves around variables, which are the elements being tested. Variables can encompass objects, events, concepts, or anything observable. There are two types of variables: independent and dependent. The independent variable is manipulated in the experiment, while the dependent variable is observed and measured for potential changes. In the earlier example, the frequency of watering represents the independent variable, while the plant's growth is the dependent variable.
The Role of Hypotheses in Research:
Hypotheses play a crucial role in determining the direction and organization of your subsequent research methods, making them integral to writing a research paper. Ultimately, readers are keen to know whether your hypothesis was validated or disproven, underscoring the importance of clearly articulating it in the introduction and/or abstract of your paper.
Seven Examples of Hypotheses:
Depending on the nature of your research and your expected findings, your hypothesis may fall into one or more of the following seven main categories. Keep in mind that a single hypothesis can qualify under several categories:
1. Simple Hypothesis:
This type suggests a relationship between two variables - one independent and one dependent.
- If you stay up late, then you feel tired the next day.
- Turning off your phone makes it charge faster.
2. Complex Hypothesis:
A complex hypothesis posits the relationship between more than two variables, such as two independent variables and one dependent variable, or vice versa.
- People who both eat a lot of fatty foods and have a family history of health problems are more likely to develop heart diseases.
- Older people who live in rural areas are happier than younger people who live in rural areas.
3. Null Hypothesis:
The null hypothesis (H0) suggests that no relationship exists between variables.
- There is no difference in plant growth when using either bottled water or tap water.
- Professional psychics do not win the lottery more than other people.
4. Alternative Hypothesis:
The alternative hypothesis (H1 or HA) is used alongside a null hypothesis and states the opposite, allowing only one of the two to be true.
- Plants grow better with bottled water than tap water.
- Professional psychics win the lottery more than other people.
5. Logical Hypothesis:
A logical hypothesis suggests a relationship between variables based on reasoning or deduction, without concrete evidence.
- An alien raised on Venus would have trouble breathing in Earth's atmosphere.
- Dinosaurs with sharp, pointed teeth were probably carnivores.
6. Empirical Hypothesis:
Also known as a 'working hypothesis,' an empirical hypothesis is currently being tested and relies on concrete data, unlike logical hypotheses.
- Customers at restaurants will tip the same even if the wait staff's base salary is raised.
- Washing your hands every hour can reduce the frequency of illness.
7. Statistical Hypothesis:
Involves testing only a sample of a population and applying statistical evidence to draw conclusions about the entire population.
- In humans, the birth-gender ratio of males to females is 1.05 to 1.00.
- Approximately 2% of the world population has natural red hair.
Characteristics of a Good Hypothesis:
Regardless of what you are testing, a good hypothesis adheres to the following five characteristics:
1. Cause and Effect:
Hypotheses always present a cause-and-effect relationship, where one variable influences another to change (or remain unchanged for null hypotheses). It can often be expressed as an if-then statement, such as: If one variable occurs, then another variable changes.
2. Testable Prediction:
Most hypotheses are designed to be tested (with the exception of logical hypotheses). Ensure that your hypothesis can be experimentally tested, and choose an independent variable that you can control completely.
3. Independent and Dependent Variables:
Define the variables in your hypothesis clearly to provide a comprehensive understanding to your readers. Though you need not explicitly state which ones are independent or dependent, it is essential to mention them all.
4. Candid Language:
Keep your hypothesis simple and clear, avoiding convoluted writing. The hypothesis serves as a contextual pillar, unifying your entire paper, so clarity is crucial. If unsure about your phrasing, try explaining your hypothesis to a friend to check for comprehension.
5. Adherence to Ethics:
Consider not only what can be tested but also what should be tested. Avoid hypotheses that require questionable or taboo experiments to maintain ethical standards and credibility.
Steps to Write a Hypothesis:
Follow these six steps to create a well-structured hypothesis:
1. Ask a Question:
Begin by asking questions about the world around you. Seek to understand why things are the way they are and what factors cause certain phenomena. Choose a research topic that genuinely interests you, as curiosity can naturally fuel your inquiry.
2. Conduct Preliminary Research:
Gather background information on your chosen topic. The extent of your preliminary research depends on the scope of your experiment. Collect only the information necessary to test or disprove your hypothesis.
3. Define Your Variables:
Once you have an idea for your hypothesis, identify the independent and dependent variables. Remember that independent variables must be controllable factors within your experiment.
4. Phrase it as an If-Then Statement:
Structure your hypothesis using an if-then format, clearly presenting the cause-and-effect relationship you are testing. For example: "If I water a plant every day, then it will grow better."
5. Collect Data to Support Your Hypothesis:
Conduct your experiments to gather data that either supports or refutes your hypothesis. Remember that a hypothesis serves as a means to an end, with the ultimate focus being on drawing conclusions from your research.
6. Write with Confidence:
Document your findings in a research paper for others to review. Effective writing is essential to presenting your hypothesis and research coherently.
In conclusion, a hypothesis is a fundamental aspect of the scientific method and should be written with precision and care. By following the outlined guidelines, you can construct a well-structured hypothesis that contributes to the success of your academic paper."
What Is a Hypothesis? (Science)
If...,Then...
Angela Lumsden/Getty Images
- Scientific Method
- Chemical Laws
- Periodic Table
- Projects & Experiments
- Biochemistry
- Physical Chemistry
- Medical Chemistry
- Chemistry In Everyday Life
- Famous Chemists
- Activities for Kids
- Abbreviations & Acronyms
- Weather & Climate
- Ph.D., Biomedical Sciences, University of Tennessee at Knoxville
- B.A., Physics and Mathematics, Hastings College
A hypothesis (plural hypotheses) is a proposed explanation for an observation. The definition depends on the subject.
In science, a hypothesis is part of the scientific method. It is a prediction or explanation that is tested by an experiment. Observations and experiments may disprove a scientific hypothesis, but can never entirely prove one.
In the study of logic, a hypothesis is an if-then proposition, typically written in the form, "If X , then Y ."
In common usage, a hypothesis is simply a proposed explanation or prediction, which may or may not be tested.
Writing a Hypothesis
Most scientific hypotheses are proposed in the if-then format because it's easy to design an experiment to see whether or not a cause and effect relationship exists between the independent variable and the dependent variable . The hypothesis is written as a prediction of the outcome of the experiment.
- Null Hypothesis and Alternative Hypothesis
Statistically, it's easier to show there is no relationship between two variables than to support their connection. So, scientists often propose the null hypothesis . The null hypothesis assumes changing the independent variable will have no effect on the dependent variable.
In contrast, the alternative hypothesis suggests changing the independent variable will have an effect on the dependent variable. Designing an experiment to test this hypothesis can be trickier because there are many ways to state an alternative hypothesis.
For example, consider a possible relationship between getting a good night's sleep and getting good grades. The null hypothesis might be stated: "The number of hours of sleep students get is unrelated to their grades" or "There is no correlation between hours of sleep and grades."
An experiment to test this hypothesis might involve collecting data, recording average hours of sleep for each student and grades. If a student who gets eight hours of sleep generally does better than students who get four hours of sleep or 10 hours of sleep, the hypothesis might be rejected.
But the alternative hypothesis is harder to propose and test. The most general statement would be: "The amount of sleep students get affects their grades." The hypothesis might also be stated as "If you get more sleep, your grades will improve" or "Students who get nine hours of sleep have better grades than those who get more or less sleep."
In an experiment, you can collect the same data, but the statistical analysis is less likely to give you a high confidence limit.
Usually, a scientist starts out with the null hypothesis. From there, it may be possible to propose and test an alternative hypothesis, to narrow down the relationship between the variables.
Example of a Hypothesis
Examples of a hypothesis include:
- If you drop a rock and a feather, (then) they will fall at the same rate.
- Plants need sunlight in order to live. (if sunlight, then life)
- Eating sugar gives you energy. (if sugar, then energy)
- White, Jay D. Research in Public Administration . Conn., 1998.
- Schick, Theodore, and Lewis Vaughn. How to Think about Weird Things: Critical Thinking for a New Age . McGraw-Hill Higher Education, 2002.
- Null Hypothesis Definition and Examples
- Definition of a Hypothesis
- What Are the Elements of a Good Hypothesis?
- Six Steps of the Scientific Method
- What Are Examples of a Hypothesis?
- Understanding Simple vs Controlled Experiments
- Scientific Method Flow Chart
- Scientific Method Vocabulary Terms
- What Is a Testable Hypothesis?
- Null Hypothesis Examples
- What 'Fail to Reject' Means in a Hypothesis Test
- How To Design a Science Fair Experiment
- What Is an Experiment? Definition and Design
- Hypothesis Test for the Difference of Two Population Proportions
- How to Conduct a Hypothesis Test
Research Hypothesis: Elements, Format, Types

When a proposition is formulated for empirical testing, we call it a hypothesis. Almost all studies begin with one or more hypotheses.
Let’s Understand Research Hypothesis.
What is a hypothesis.
A hypothesis, specifically a research hypothesis, is formulated to predict an assumed relationship between two or more variables of interest.
If we reasonably guess that a relationship exists between the variables of interest, we first state it as a hypothesis and then test it in the field.
Hypotheses are stated in terms of the particular dependent and independent variables that are going to be used in the study.
Research Hypothesis Definition
A research hypothesis is a conjectural statement, a logical supposition, a reasonable guess, and an educated prediction about the nature of the relationship between two or more variables that we expect to happen in our study.
Unless you are creating an exploratory study, your hypothesis should always explain what you expect to happen during your experiment or research.
Remember, a hypothesis does not have to be correct. While the hypothesis predicts what the researchers expect to see, the research aims to determine whether this guess is right or wrong.
When experimenting, researchers might explore different factors to determine which ones might contribute to the outcome.
In many cases, researchers may find that the results of an experiment do not support the original hypothesis. When writing up these results, the researchers might suggest other options that should be explored in future studies.
Elements of a Good Hypothesis
Regardless of the type of hypothesis, the goal of a good hypothesis is to help explain the focus and direction of the experiment or research. As such, a good hypothesis will
- State the purpose of the research.
- Identify which variables are to be used.
A good hypothesis;
- Needs to be logical.
- Must be precise in language.
- It should be testable with research or experimentation.
A hypothesis is usually written in a form where it proposes that if something is done, then something will occur.
Finally, when you are trying to come up with a good hypothesis for your research or experiments, ask yourself the following questions:
- Is your hypothesis based on any previous research on a topic?
- Can your hypothesis be tested?
- Does your hypothesis include independent and dependent variables?
Before you come up with a specific hypothesis, spend some time doing background research on your topic.
Once you have completed a literature review, start thinking of potential questions you still have. Pay attention to the discussion section in the journal articles you read. Many authors will suggest questions that still need to be explored.
Basic Format of a Good Hypothesis
A hypothesis often follows a basic format of “If {this happens}, then {this will happen}.” One way to structure your hypothesis is to describe what will happen to the dependent variable if you change the independent variable.
The basic format might be:
“If {these changes are made to a certain independent variable}, then we will observe {a change in a specific dependent variable}.”
A few examples:
- Students who eat breakfast will perform better on a math test than students who do not eat breakfast.
- Students who experience test anxiety before an exam get higher scores than students who do not experience test anxiety.
- Drivers who talk on their mobile phones while driving will be more likely to make errors when driving than those who do not talk on the phone.
- People with high exposure to ultraviolet light will have a higher frequency of skin cancer than those who do not have such exposure.
Look at the last example.
Here is the independent variable (exposure to ultraviolet light)) is specified, and the dependent variable (skin cancer) is also specified.
Notice also that this research hypothesis specifies a direction in that it predicts that people exposed to ultraviolet light will have a higher risk of cancer.
This is not always the case. Research hypotheses can also specify a difference without saying which group will be better or higher than the other.
For example, one might formulate a hypothesis of the type: ‘Religion does not make any significant difference in the performance of cultural activities.’
In general, however, it is considered a better hypothesis if you can specify a direction.
Research hypotheses serve several important functions. The most important one is to direct and guide the research.
A few of the other functions of the research hypothesis are enumerated below:
- A research hypothesis indicates the major independent variables to be included in the study;
- A research hypothesis suggests the type of data that must be collected and the type of analysis that must be conducted to measure the relationship;
- A research hypothesis identifies facts that are relevant and that are not;
- A research hypothesis suggests the type of research design to be employed.
Types of Research Hypothesis
Two types of research hypotheses are;
- Descriptive hypothesis.
- Relational hypothesis.
Descriptive Hypotheses
Descriptive hypotheses are propositions that typically state some variables’ existence, size, form, or distribution.
These hypotheses are formulated in the form of statements in which we assign variables to cases.
For example,
- The prevalence of contraceptive use among currently married women in India exceeds 60%.
In this example, the case is ‘currently married women,’ and the variable is ‘prevalence of contraceptives.’ As a second example,
- The public universities are currently experiencing budget difficulties.
Here,’ public universities’ is the case, and ‘budget difficulties’ is the variable.
- The National Board of Revenue claims that over 15% of potential taxpayers falsify in their income tax returns.
- At most, 75% of the pre-school children in community A have a protein-deficient diet.
- The average sales in a superstore exceed taka 25 lac per month.
- Smoking increases the risk of lung cancer.
- The average longevity of women is higher among females than among males.
- Gainfully employed women tend to have lower than average fertility.
- Women with child loss experience will have higher fertility than those who do not have such experiences.
All examples of descriptive hypotheses.
It is important to note that the Descriptive hypothesis does not always have variables that can be designated as independent or dependent.
Relational Hypotheses
Relational hypotheses, on the other hand, are statements that describe the relationship between variables concerning some cases.
- Communities with many modern facilities will have a higher rate of contraception than communities with few modern facilities.
In this instance, the case is ‘communities,’ and the variables are ‘rate of contraception’ and ‘modern facilities.’
Similarly, “People who use chewing tobacco have a higher risk of oral carcinoma than people who have never used chewing tobacco” is a relational hypothesis.
A relational hypothesis is again of two types: correlational hypothesis and the causal hypothesis.
A correlational hypothesis states that variables occur in some predictable relationships without implying that one variable causes the other to change or take on different values.
Here is an example of a co-relational hypothesis:
- Males are more efficient than their female counterparts in typing.
In making such a statement, we do not claim that sex (male-female) as a variable influences the other variable,’ typing efficiency’ (less efficient-more efficient). Here is one more example of a correlational hypothesis:
- Saving habit is more pronounced among Christians than the people of other religions.
Once again, religion is not believed to be a factor in saving habits, although a positive relationship has been observed.
Look at the following example:
- The participation of women in household decision making increases with age, their level of education, and the number of surviving children.
Here too, women’s education, several surviving children, or education does not guarantee their decision-making autonomy.
With causal hypotheses (also called explanatory hypotheses), on the other hand, there is an implication that a change in one variable causes a change or leads to an effect on the other variable.
A causal variable is typically called an independent variable, and the other is the dependent variable. It is important to note that the term “cause” roughly means “help make happen.” So, the independent variable need not be the sole reason for the existence of or change in the dependent variable. Here are some examples of causal hypotheses:
- An increase in family income leads to an increase in the income saved.
- Exposure of mothers to mass media increases their knowledge of malnutrition among their children.
- An offer of a discount in a department store enhances the sales volume.
- Chewing tobacco increases the risk of oral carcinoma.
- Goat farming contributes to poverty alleviation of rural people.
- The utilization of child welfare clinics is the lowest in those clinics in which the clinic personnel are poorly motivated to provide preventive services.
- An increase in bank interest rate encourages the customers for increased savings.
In the above example, we have ample reasons to believe that one variable (family income and savings, misuse of credit, and farm size) has a bearing on the other variable.
We cite two more examples to illustrate the hypothesis, general objective, ultimate objective, and a few specific objectives.
General objective:
- To compare the complications of acceptors of laparoscopic sterilization and mini-laparotomy among American women.
Research hypothesis:
- The risk of complications is higher in the mini-laparotomy method of sterilization than in laparoscopic sterilization.
Specific objectives:
- To assess the complications of laparoscopic sterilization and mini-laparotomy.
- To assess service providers’ knowledge and perception regarding the complications, preferences, and convenience of the two methods.
Ultimate Objectives:
- To introduce and popularize the laparoscopic female sterilization method in the National Family Planning Program to reduce the rapid population growth rate.
In a study designed to examine the living and working conditions of the overseas migrant workers from India and the pattern of remittances from overseas migrant workers, the general objective, specific objectives, and the ultimate objective were formulated as follows:
- To examine the living and working conditions of the overseas migrant workers from India.”
- Characteristics of migrant workers by significant migration channels;
- Countries of destination;
- The occupational skill of the workers;
- Pattern and procedures of remittances;
- Impact of remittances on government revenue;
- Better utilization of remittances.
Ultimate objective:
- To suggest ways and means to minimize the differences in the policy adopted by the public and private sectors in their recruitment process in the interest of the workers;
- To ascertain the possible exploitation of the workers by the private agencies and suggest remedies for such exploitation.
- Private agencies, in most cases, exploit migrant workers.
What are the elements of a good hypothesis?
A good hypothesis should state the purpose of the research, identify which variables are to be used, be logical, precise in language, and be testable with research or experimentation.
How is a hypothesis typically structured?
A hypothesis often follows a basic format of “If {this happens}, then {this will happen}.” It proposes that if something is done, then a specific outcome will occur.
What is a Descriptive hypothesis?
Descriptive hypotheses are propositions that typically state some variables’ existence, size, form, or distribution. They are formulated in the form of statements in which variables are assigned to cases.
What distinguishes a Relational hypothesis?
Relational hypotheses describe the relationship between variables concerning some cases. They can be correlational, where variables occur in a predictable relationship without implying causation, or causal, where a change in one variable causes a change in another.
What is the difference between a correlational hypothesis and a causal hypothesis?
A correlational hypothesis states that variables occur in some predictable relationships without implying that one variable causes the other to change. A causal hypothesis, on the other hand, implies that a change in one variable causes a change or leads to an effect on the other variable.
What are the two main types of research hypotheses?
The two main types of research hypotheses are Descriptive hypothesis and Relational hypothesis
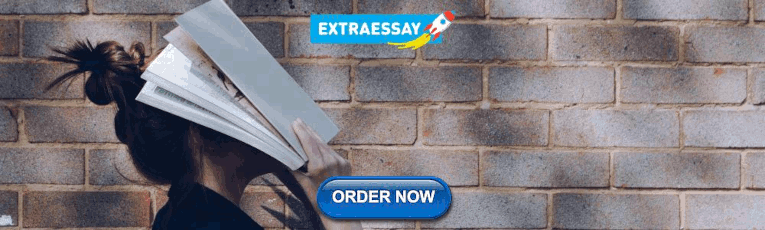
What is a hypothesis in the context of academic research?
A hypothesis is a statement about an expected relationship between variables or an explanation of an occurrence that is clear, specific, and testable.
How does a research hypothesis differ from a general hypothesis?
A research hypothesis is more specific and clear about what’s being assessed and the expected outcome. It must also be testable, meaning there should be a way to prove or disprove it.
What are the essential attributes of a good research hypothesis?
A good research hypothesis should have specificity, clarity, and testability.
Why is testability crucial for a research hypothesis?
Testability ensures that empirical research can prove or disproven the hypothesis. If a statement isn’t testable, it doesn’t qualify as a research hypothesis.
What is the null hypothesis?
The null hypothesis is the counter-proposal to the original hypothesis. It predicts that there is no relationship between the variables in question.
How can one ensure that a hypothesis is clear and specific?
A hypothesis should clearly identify the variables involved, the parties involved, and the expected relationship type, leaving no ambiguity about its intent or meaning.
Why is it essential to avoid value judgments in a research hypothesis?
Value judgments are subjective and not appropriate for a hypothesis. A research hypothesis should strive to be objective, avoiding personal opinions.
What is the basic definition of a hypothesis in research?
A research hypothesis is a statement about an expected relationship between variables, or an explanation of an occurrence, that is clear, specific, and testable.
While a general hypothesis is an idea or explanation based on known facts but not yet proven, a research hypothesis is a clear, specific, and testable statement about the expected outcome of a study.
What are the essential characteristics of a good research hypothesis?
A good research hypothesis should possess specificity, clarity, and testability. It should clearly define what’s being assessed and the expected outcome, and it must be possible to prove or disprove the statement through experimentation.
How can one ensure that a hypothesis is testable?
A hypothesis is testable if there’s a possibility to prove both its truth and falsity. The results of the hypothesis should be reproducible, and it should be specific enough to allow for clear testing procedures.
What is the difference between a null hypothesis and an alternative hypothesis?
The null hypothesis proposes that no statistical significance exists in a set of observations, suggesting any differences are due to chance alone. The alternative hypothesis, on the other hand, predicts a relationship between the variables of the study and states that the results are significant to the research topic.
How should one formulate an effective research hypothesis?
To formulate an effective research hypothesis, one should state the problem clearly, use an ‘if-then’ statement structure, define the variables as dependent or independent, and scrutinize the hypothesis to ensure it meets the criteria of specificity, clarity, and testability.
What are some types of hypotheses in research?
Types of hypotheses include simple, complex, directional, non-directional, associative and causal, empirical, and statistical hypotheses. Each type serves a specific purpose and is used based on the nature of the research question or problem.

Your email address will not be published. Required fields are marked *

13 Different Types of Hypothesis
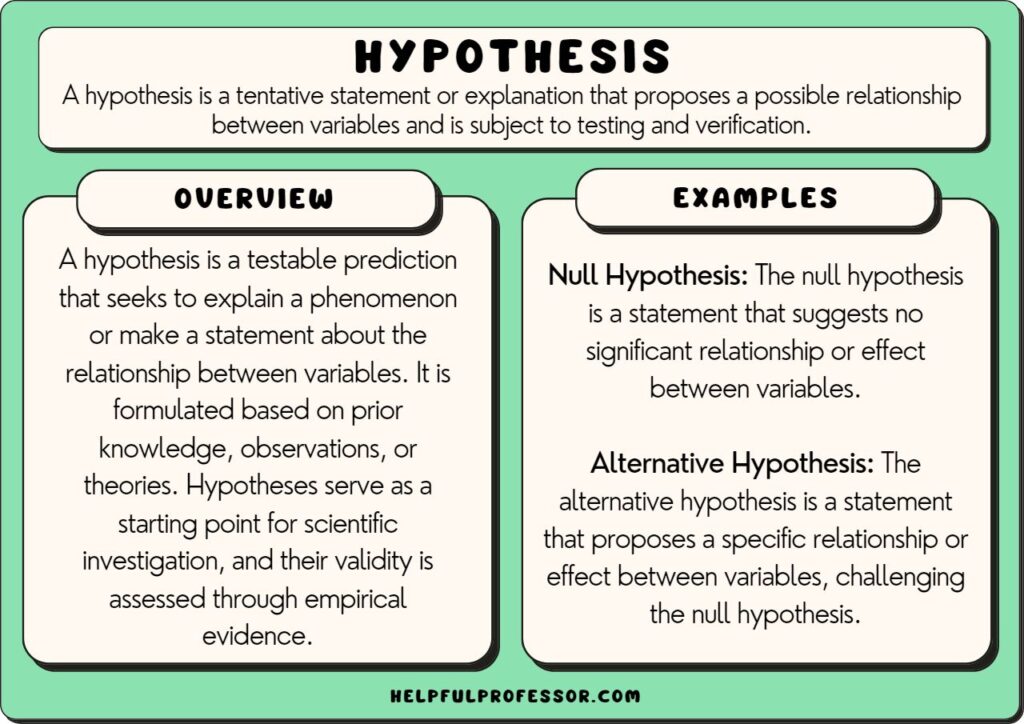
There are 13 different types of hypothesis. These include simple, complex, null, alternative, composite, directional, non-directional, logical, empirical, statistical, associative, exact, and inexact.
A hypothesis can be categorized into one or more of these types. However, some are mutually exclusive and opposites. Simple and complex hypotheses are mutually exclusive, as are direction and non-direction, and null and alternative hypotheses.
Below I explain each hypothesis in simple terms for absolute beginners. These definitions may be too simple for some, but they’re designed to be clear introductions to the terms to help people wrap their heads around the concepts early on in their education about research methods .
Types of Hypothesis
Before you Proceed: Dependent vs Independent Variables
A research study and its hypotheses generally examine the relationships between independent and dependent variables – so you need to know these two concepts:
- The independent variable is the variable that is causing a change.
- The dependent variable is the variable the is affected by the change. This is the variable being tested.
Read my full article on dependent vs independent variables for more examples.
Example: Eating carrots (independent variable) improves eyesight (dependent variable).
1. Simple Hypothesis
A simple hypothesis is a hypothesis that predicts a correlation between two test variables: an independent and a dependent variable.
This is the easiest and most straightforward type of hypothesis. You simply need to state an expected correlation between the dependant variable and the independent variable.
You do not need to predict causation (see: directional hypothesis). All you would need to do is prove that the two variables are linked.
Simple Hypothesis Examples
2. complex hypothesis.
A complex hypothesis is a hypothesis that contains multiple variables, making the hypothesis more specific but also harder to prove.
You can have multiple independent and dependant variables in this hypothesis.
Complex Hypothesis Example
In the above example, we have multiple independent and dependent variables:
- Independent variables: Age and weight.
- Dependent variables: diabetes and heart disease.
Because there are multiple variables, this study is a lot more complex than a simple hypothesis. It quickly gets much more difficult to prove these hypotheses. This is why undergraduate and first-time researchers are usually encouraged to use simple hypotheses.
3. Null Hypothesis
A null hypothesis will predict that there will be no significant relationship between the two test variables.
For example, you can say that “The study will show that there is no correlation between marriage and happiness.”
A good way to think about a null hypothesis is to think of it in the same way as “innocent until proven guilty”[1]. Unless you can come up with evidence otherwise, your null hypothesis will stand.
A null hypothesis may also highlight that a correlation will be inconclusive . This means that you can predict that the study will not be able to confirm your results one way or the other. For example, you can say “It is predicted that the study will be unable to confirm a correlation between the two variables due to foreseeable interference by a third variable .”
Beware that an inconclusive null hypothesis may be questioned by your teacher. Why would you conduct a test that you predict will not provide a clear result? Perhaps you should take a closer look at your methodology and re-examine it. Nevertheless, inconclusive null hypotheses can sometimes have merit.
Null Hypothesis Examples
4. alternative hypothesis.
An alternative hypothesis is a hypothesis that is anything other than the null hypothesis. It will disprove the null hypothesis.
We use the symbol H A or H 1 to denote an alternative hypothesis.
The null and alternative hypotheses are usually used together. We will say the null hypothesis is the case where a relationship between two variables is non-existent. The alternative hypothesis is the case where there is a relationship between those two variables.
The following statement is always true: H 0 ≠ H A .
Let’s take the example of the hypothesis: “Does eating oatmeal before an exam impact test scores?”
We can have two hypotheses here:
- Null hypothesis (H 0 ): “Eating oatmeal before an exam does not impact test scores.”
- Alternative hypothesis (H A ): “Eating oatmeal before an exam does impact test scores.”
For the alternative hypothesis to be true, all we have to do is disprove the null hypothesis for the alternative hypothesis to be true. We do not need an exact prediction of how much oatmeal will impact the test scores or even if the impact is positive or negative. So long as the null hypothesis is proven to be false, then the alternative hypothesis is proven to be true.
5. Composite Hypothesis
A composite hypothesis is a hypothesis that does not predict the exact parameters, distribution, or range of the dependent variable.
Often, we would predict an exact outcome. For example: “23 year old men are on average 189cm tall.” Here, we are giving an exact parameter. So, the hypothesis is not composite.
But, often, we cannot exactly hypothesize something. We assume that something will happen, but we’re not exactly sure what. In these cases, we might say: “23 year old men are not on average 189cm tall.”
We haven’t set a distribution range or exact parameters of the average height of 23 year old men. So, we’ve introduced a composite hypothesis as opposed to an exact hypothesis.
Generally, an alternative hypothesis (discussed above) is composite because it is defined as anything except the null hypothesis. This ‘anything except’ does not define parameters or distribution, and therefore it’s an example of a composite hypothesis.
6. Directional Hypothesis
A directional hypothesis makes a prediction about the positivity or negativity of the effect of an intervention prior to the test being conducted.
Instead of being agnostic about whether the effect will be positive or negative, it nominates the effect’s directionality.
We often call this a one-tailed hypothesis (in contrast to a two-tailed or non-directional hypothesis) because, looking at a distribution graph, we’re hypothesizing that the results will lean toward one particular tail on the graph – either the positive or negative.
Directional Hypothesis Examples
7. non-directional hypothesis.
A non-directional hypothesis does not specify the predicted direction (e.g. positivity or negativity) of the effect of the independent variable on the dependent variable.
These hypotheses predict an effect, but stop short of saying what that effect will be.
A non-directional hypothesis is similar to composite and alternative hypotheses. All three types of hypothesis tend to make predictions without defining a direction. In a composite hypothesis, a specific prediction is not made (although a general direction may be indicated, so the overlap is not complete). For an alternative hypothesis, you often predict that the even will be anything but the null hypothesis, which means it could be more or less than H 0 (or in other words, non-directional).
Let’s turn the above directional hypotheses into non-directional hypotheses.
Non-Directional Hypothesis Examples
8. logical hypothesis.
A logical hypothesis is a hypothesis that cannot be tested, but has some logical basis underpinning our assumptions.
These are most commonly used in philosophy because philosophical questions are often untestable and therefore we must rely on our logic to formulate logical theories.
Usually, we would want to turn a logical hypothesis into an empirical one through testing if we got the chance. Unfortunately, we don’t always have this opportunity because the test is too complex, expensive, or simply unrealistic.
Here are some examples:
- Before the 1980s, it was hypothesized that the Titanic came to its resting place at 41° N and 49° W, based on the time the ship sank and the ship’s presumed path across the Atlantic Ocean. However, due to the depth of the ocean, it was impossible to test. Thus, the hypothesis was simply a logical hypothesis.
- Dinosaurs closely related to Aligators probably had green scales because Aligators have green scales. However, as they are all extinct, we can only rely on logic and not empirical data.
9. Empirical Hypothesis
An empirical hypothesis is the opposite of a logical hypothesis. It is a hypothesis that is currently being tested using scientific analysis. We can also call this a ‘working hypothesis’.
We can to separate research into two types: theoretical and empirical. Theoretical research relies on logic and thought experiments. Empirical research relies on tests that can be verified by observation and measurement.
So, an empirical hypothesis is a hypothesis that can and will be tested.
- Raising the wage of restaurant servers increases staff retention.
- Adding 1 lb of corn per day to cows’ diets decreases their lifespan.
- Mushrooms grow faster at 22 degrees Celsius than 27 degrees Celsius.
Each of the above hypotheses can be tested, making them empirical rather than just logical (aka theoretical).
10. Statistical Hypothesis
A statistical hypothesis utilizes representative statistical models to draw conclusions about broader populations.
It requires the use of datasets or carefully selected representative samples so that statistical inference can be drawn across a larger dataset.
This type of research is necessary when it is impossible to assess every single possible case. Imagine, for example, if you wanted to determine if men are taller than women. You would be unable to measure the height of every man and woman on the planet. But, by conducting sufficient random samples, you would be able to predict with high probability that the results of your study would remain stable across the whole population.
You would be right in guessing that almost all quantitative research studies conducted in academic settings today involve statistical hypotheses.
Statistical Hypothesis Examples
- Human Sex Ratio. The most famous statistical hypothesis example is that of John Arbuthnot’s sex at birth case study in 1710. Arbuthnot used birth data to determine with high statistical probability that there are more male births than female births. He called this divine providence, and to this day, his findings remain true: more men are born than women.
- Lady Testing Tea. A 1935 study by Ronald Fisher involved testing a woman who believed she could tell whether milk was added before or after water to a cup of tea. Fisher gave her 4 cups in which one randomly had milk placed before the tea. He repeated the test 8 times. The lady was correct each time. Fisher found that she had a 1 in 70 chance of getting all 8 test correct, which is a statistically significant result.
11. Associative Hypothesis
An associative hypothesis predicts that two variables are linked but does not explore whether one variable directly impacts upon the other variable.
We commonly refer to this as “ correlation does not mean causation ”. Just because there are a lot of sick people in a hospital, it doesn’t mean that the hospital made the people sick. There is something going on there that’s causing the issue (sick people are flocking to the hospital).
So, in an associative hypothesis, you note correlation between an independent and dependent variable but do not make a prediction about how the two interact. You stop short of saying one thing causes another thing.
Associative Hypothesis Examples
- Sick people in hospital. You could conduct a study hypothesizing that hospitals have more sick people in them than other institutions in society. However, you don’t hypothesize that the hospitals caused the sickness.
- Lice make you healthy. In the Middle Ages, it was observed that sick people didn’t tend to have lice in their hair. The inaccurate conclusion was that lice was not only a sign of health, but that they made people healthy. In reality, there was an association here, but not causation. The fact was that lice were sensitive to body temperature and fled bodies that had fevers.
12. Causal Hypothesis
A causal hypothesis predicts that two variables are not only associated, but that changes in one variable will cause changes in another.
A causal hypothesis is harder to prove than an associative hypothesis because the cause needs to be definitively proven. This will often require repeating tests in controlled environments with the researchers making manipulations to the independent variable, or the use of control groups and placebo effects .
If we were to take the above example of lice in the hair of sick people, researchers would have to put lice in sick people’s hair and see if it made those people healthier. Researchers would likely observe that the lice would flee the hair, but the sickness would remain, leading to a finding of association but not causation.
Causal Hypothesis Examples
13. exact vs. inexact hypothesis.
For brevity’s sake, I have paired these two hypotheses into the one point. The reality is that we’ve already seen both of these types of hypotheses at play already.
An exact hypothesis (also known as a point hypothesis) specifies a specific prediction whereas an inexact hypothesis assumes a range of possible values without giving an exact outcome. As Helwig [2] argues:
“An “exact” hypothesis specifies the exact value(s) of the parameter(s) of interest, whereas an “inexact” hypothesis specifies a range of possible values for the parameter(s) of interest.”
Generally, a null hypothesis is an exact hypothesis whereas alternative, composite, directional, and non-directional hypotheses are all inexact.
See Next: 15 Hypothesis Examples
This is introductory information that is basic and indeed quite simplified for absolute beginners. It’s worth doing further independent research to get deeper knowledge of research methods and how to conduct an effective research study. And if you’re in education studies, don’t miss out on my list of the best education studies dissertation ideas .
[1] https://jnnp.bmj.com/content/91/6/571.abstract
[2] http://users.stat.umn.edu/~helwig/notes/SignificanceTesting.pdf
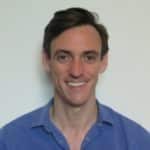
Chris Drew (PhD)
Dr. Chris Drew is the founder of the Helpful Professor. He holds a PhD in education and has published over 20 articles in scholarly journals. He is the former editor of the Journal of Learning Development in Higher Education. [Image Descriptor: Photo of Chris]
- Chris Drew (PhD) https://helpfulprofessor.com/author/chris-drew-phd/ 5 Top Tips for Succeeding at University
- Chris Drew (PhD) https://helpfulprofessor.com/author/chris-drew-phd/ 50 Durable Goods Examples
- Chris Drew (PhD) https://helpfulprofessor.com/author/chris-drew-phd/ 100 Consumer Goods Examples
- Chris Drew (PhD) https://helpfulprofessor.com/author/chris-drew-phd/ 30 Globalization Pros and Cons
2 thoughts on “13 Different Types of Hypothesis”
Wow! This introductionary materials are very helpful. I teach the begginers in research for the first time in my career. The given tips and materials are very helpful. Chris, thank you so much! Excellent materials!
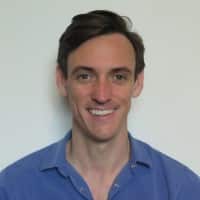
You’re more than welcome! If you want a pdf version of this article to provide for your students to use as a weekly reading on in-class discussion prompt for seminars, just drop me an email in the Contact form and I’ll get one sent out to you.
When I’ve taught this seminar, I’ve put my students into groups, cut these definitions into strips, and handed them out to the groups. Then I get them to try to come up with hypotheses that fit into each ‘type’. You can either just rotate hypothesis types so they get a chance at creating a hypothesis of each type, or get them to “teach” their hypothesis type and examples to the class at the end of the seminar.
Cheers, Chris
Leave a Comment Cancel Reply
Your email address will not be published. Required fields are marked *
Science and Hypothesis
- First Online: 13 June 2021
Cite this chapter
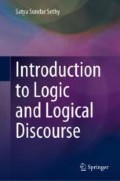
- Satya Sundar Sethy 2
322 Accesses
In this chapter, we will discuss the significance of a ‘hypothesis’ in a logical inquiry, a scientific investigation, and research work. We will enumerate some of the definitions of ‘hypothesis’. We will elaborate on the nature and scope of the ‘hypothesis’ and the sources to obtain a hypothesis. Further, we will explain the kinds of hypothesis with suitable examples. In the end, we will illustrate methods to verify a hypothesis in a logical inquiry and a scientific investigation.
This is a preview of subscription content, log in via an institution to check access.
Access this chapter
- Available as PDF
- Read on any device
- Instant download
- Own it forever
- Available as EPUB and PDF
- Compact, lightweight edition
- Dispatched in 3 to 5 business days
- Free shipping worldwide - see info
- Durable hardcover edition
Tax calculation will be finalised at checkout
Purchases are for personal use only
Institutional subscriptions
Werkmeister, W.H. (1948). The basis and structure of knowledge . New York: Haper and Bros Publication.
Lundberg, G.A. (1968). Social research: A study in methods of gathering data . New York: Greenwood Press.
Black, J. A., and Champion, D.J. (1976). Method and issues in social research . New York: John Wiley & Sons.
Goode, W.J., and Hatt, P.K. (1971). Methods in social research . New York: McGraw-Hill Publication.
https://www.merriam-webster.com/dictionary/hypothesis .
Sarantakos, S. (2005) (3rd Edition). Social research . New York: Palgrave Macmillan.
Author information
Authors and affiliations.
Department of Humanities and Social Sciences, Indian Institute of Technology Madras, Chennai, Tamil Nadu, India
Satya Sundar Sethy
You can also search for this author in PubMed Google Scholar
Rights and permissions
Reprints and permissions
Copyright information
© 2021 Springer Nature Singapore Pte Ltd.
About this chapter
Sethy, S.S. (2021). Science and Hypothesis. In: Introduction to Logic and Logical Discourse. Springer, Singapore. https://doi.org/10.1007/978-981-16-2689-0_17
Download citation
DOI : https://doi.org/10.1007/978-981-16-2689-0_17
Published : 13 June 2021
Publisher Name : Springer, Singapore
Print ISBN : 978-981-16-2688-3
Online ISBN : 978-981-16-2689-0
eBook Packages : Religion and Philosophy Philosophy and Religion (R0)
Share this chapter
Anyone you share the following link with will be able to read this content:
Sorry, a shareable link is not currently available for this article.
Provided by the Springer Nature SharedIt content-sharing initiative
- Publish with us
Policies and ethics
- Find a journal
- Track your research
- Maths Notes Class 12
- NCERT Solutions Class 12
- RD Sharma Solutions Class 12
- Maths Formulas Class 12
- Maths Previous Year Paper Class 12
- Class 12 Syllabus
- Class 12 Revision Notes
- Physics Notes Class 12
- Chemistry Notes Class 12
- Biology Notes Class 12
- Domain and Range of Trigonometric Functions
- Exponential Graph
- Line Integral
- Determinant of 2x2 Matrix
- Integral of Cos x
- Algebra of Matrices
- Random Sampling
- Derivative of Sin 2x
- Integration
- Derivative of Sec Square x
- Derivative Rules
- Derivative of Sec x
- Systematic Random Sampling
- Derivative of Tan Inverse x
- Derivative of Arctan
- Zero Vector
- Triple Integrals
- Local Maxima and Minima in Calculus
Hypothesis is a testable statement that explains what is happening or observed. It proposes the relation between the various participating variables. Hypothesis is also called Theory, Thesis, Guess, Assumption, or Suggestion. Hypothesis creates a structure that guides the search for knowledge.
In this article, we will learn what is hypothesis, its characteristics, types, and examples. We will also learn how hypothesis helps in scientific research.
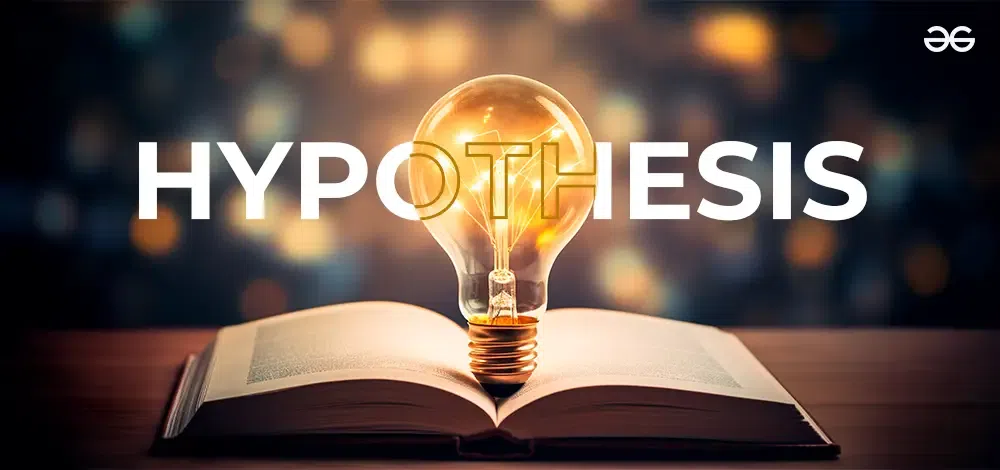
What is Hypothesis?
A hypothesis is a suggested idea or plan that has little proof, meant to lead to more study. It’s mainly a smart guess or suggested answer to a problem that can be checked through study and trial. In science work, we make guesses called hypotheses to try and figure out what will happen in tests or watching. These are not sure things but rather ideas that can be proved or disproved based on real-life proofs. A good theory is clear and can be tested and found wrong if the proof doesn’t support it.
Hypothesis Meaning
A hypothesis is a proposed statement that is testable and is given for something that happens or observed.
- It is made using what we already know and have seen, and it’s the basis for scientific research.
- A clear guess tells us what we think will happen in an experiment or study.
- It’s a testable clue that can be proven true or wrong with real-life facts and checking it out carefully.
- It usually looks like a “if-then” rule, showing the expected cause and effect relationship between what’s being studied.
Characteristics of Hypothesis
Here are some key characteristics of a hypothesis:
- Testable: An idea (hypothesis) should be made so it can be tested and proven true through doing experiments or watching. It should show a clear connection between things.
- Specific: It needs to be easy and on target, talking about a certain part or connection between things in a study.
- Falsifiable: A good guess should be able to show it’s wrong. This means there must be a chance for proof or seeing something that goes against the guess.
- Logical and Rational: It should be based on things we know now or have seen, giving a reasonable reason that fits with what we already know.
- Predictive: A guess often tells what to expect from an experiment or observation. It gives a guide for what someone might see if the guess is right.
- Concise: It should be short and clear, showing the suggested link or explanation simply without extra confusion.
- Grounded in Research: A guess is usually made from before studies, ideas or watching things. It comes from a deep understanding of what is already known in that area.
- Flexible: A guess helps in the research but it needs to change or fix when new information comes up.
- Relevant: It should be related to the question or problem being studied, helping to direct what the research is about.
- Empirical: Hypotheses come from observations and can be tested using methods based on real-world experiences.
Sources of Hypothesis
Hypotheses can come from different places based on what you’re studying and the kind of research. Here are some common sources from which hypotheses may originate:
- Existing Theories: Often, guesses come from well-known science ideas. These ideas may show connections between things or occurrences that scientists can look into more.
- Observation and Experience: Watching something happen or having personal experiences can lead to guesses. We notice odd things or repeat events in everyday life and experiments. This can make us think of guesses called hypotheses.
- Previous Research: Using old studies or discoveries can help come up with new ideas. Scientists might try to expand or question current findings, making guesses that further study old results.
- Literature Review: Looking at books and research in a subject can help make guesses. Noticing missing parts or mismatches in previous studies might make researchers think up guesses to deal with these spots.
- Problem Statement or Research Question: Often, ideas come from questions or problems in the study. Making clear what needs to be looked into can help create ideas that tackle certain parts of the issue.
- Analogies or Comparisons: Making comparisons between similar things or finding connections from related areas can lead to theories. Understanding from other fields could create new guesses in a different situation.
- Hunches and Speculation: Sometimes, scientists might get a gut feeling or make guesses that help create ideas to test. Though these may not have proof at first, they can be a beginning for looking deeper.
- Technology and Innovations: New technology or tools might make guesses by letting us look at things that were hard to study before.
- Personal Interest and Curiosity: People’s curiosity and personal interests in a topic can help create guesses. Scientists could make guesses based on their own likes or love for a subject.
Types of Hypothesis
Here are some common types of hypotheses:
Simple Hypothesis
Complex hypothesis, directional hypothesis.
- Non-directional Hypothesis
Null Hypothesis (H0)
Alternative hypothesis (h1 or ha), statistical hypothesis, research hypothesis, associative hypothesis, causal hypothesis.
Simple Hypothesis guesses a connection between two things. It says that there is a connection or difference between variables, but it doesn’t tell us which way the relationship goes.
Complex Hypothesis tells us what will happen when more than two things are connected. It looks at how different things interact and may be linked together.
Directional Hypothesis says how one thing is related to another. For example, it guesses that one thing will help or hurt another thing.
Non-Directional Hypothesis
Non-Directional Hypothesis are the one that don’t say how the relationship between things will be. They just say that there is a connection, without telling which way it goes.
Null hypothesis is a statement that says there’s no connection or difference between different things. It implies that any seen impacts are because of luck or random changes in the information.
Alternative Hypothesis is different from the null hypothesis and shows that there’s a big connection or gap between variables. Scientists want to say no to the null hypothesis and choose the alternative one.
Statistical Hypotheis are used in math testing and include making ideas about what groups or bits of them look like. You aim to get information or test certain things using these top-level, common words only.
Research Hypothesis comes from the research question and tells what link is expected between things or factors. It leads the study and chooses where to look more closely.
Associative Hypotheis guesses that there is a link or connection between things without really saying it caused them. It means that when one thing changes, it is connected to another thing changing.
Causal Hypothesis are different from other ideas because they say that one thing causes another. This means there’s a cause and effect relationship between variables involved in the situation. They say that when one thing changes, it directly makes another thing change.
Hypothesis Examples
Following are the examples of hypotheses based on their types:
Simple Hypothesis Example
- Studying more can help you do better on tests.
- Getting more sun makes people have higher amounts of vitamin D.
Complex Hypothesis Example
- How rich you are, how easy it is to get education and healthcare greatly affects the number of years people live.
- A new medicine’s success relies on the amount used, how old a person is who takes it and their genes.
Directional Hypothesis Example
- Drinking more sweet drinks is linked to a higher body weight score.
- Too much stress makes people less productive at work.
Non-directional Hypothesis Example
- Drinking caffeine can affect how well you sleep.
- People often like different kinds of music based on their gender.
- The average test scores of Group A and Group B are not much different.
- There is no connection between using a certain fertilizer and how much it helps crops grow.
Alternative Hypothesis (Ha)
- Patients on Diet A have much different cholesterol levels than those following Diet B.
- Exposure to a certain type of light can change how plants grow compared to normal sunlight.
- The average smarts score of kids in a certain school area is 100.
- The usual time it takes to finish a job using Method A is the same as with Method B.
- Having more kids go to early learning classes helps them do better in school when they get older.
- Using specific ways of talking affects how much customers get involved in marketing activities.
- Regular exercise helps to lower the chances of heart disease.
- Going to school more can help people make more money.
- Playing violent video games makes teens more likely to act aggressively.
- Less clean air directly impacts breathing health in city populations.
Functions of Hypothesis
Hypotheses have many important jobs in the process of scientific research. Here are the key functions of hypotheses:
- Guiding Research: Hypotheses give a clear and exact way for research. They act like guides, showing the predicted connections or results that scientists want to study.
- Formulating Research Questions: Research questions often create guesses. They assist in changing big questions into particular, checkable things. They guide what the study should be focused on.
- Setting Clear Objectives: Hypotheses set the goals of a study by saying what connections between variables should be found. They set the targets that scientists try to reach with their studies.
- Testing Predictions: Theories guess what will happen in experiments or observations. By doing tests in a planned way, scientists can check if what they see matches the guesses made by their ideas.
- Providing Structure: Theories give structure to the study process by arranging thoughts and ideas. They aid scientists in thinking about connections between things and plan experiments to match.
- Focusing Investigations: Hypotheses help scientists focus on certain parts of their study question by clearly saying what they expect links or results to be. This focus makes the study work better.
- Facilitating Communication: Theories help scientists talk to each other effectively. Clearly made guesses help scientists to tell others what they plan, how they will do it and the results expected. This explains things well with colleagues in a wide range of audiences.
- Generating Testable Statements: A good guess can be checked, which means it can be looked at carefully or tested by doing experiments. This feature makes sure that guesses add to the real information used in science knowledge.
- Promoting Objectivity: Guesses give a clear reason for study that helps guide the process while reducing personal bias. They motivate scientists to use facts and data as proofs or disprovals for their proposed answers.
- Driving Scientific Progress: Making, trying out and adjusting ideas is a cycle. Even if a guess is proven right or wrong, the information learned helps to grow knowledge in one specific area.
How Hypothesis help in Scientific Research?
Researchers use hypotheses to put down their thoughts directing how the experiment would take place. Following are the steps that are involved in the scientific method:
- Initiating Investigations: Hypotheses are the beginning of science research. They come from watching, knowing what’s already known or asking questions. This makes scientists make certain explanations that need to be checked with tests.
- Formulating Research Questions: Ideas usually come from bigger questions in study. They help scientists make these questions more exact and testable, guiding the study’s main point.
- Setting Clear Objectives: Hypotheses set the goals of a study by stating what we think will happen between different things. They set the goals that scientists want to reach by doing their studies.
- Designing Experiments and Studies: Assumptions help plan experiments and watchful studies. They assist scientists in knowing what factors to measure, the techniques they will use and gather data for a proposed reason.
- Testing Predictions: Ideas guess what will happen in experiments or observations. By checking these guesses carefully, scientists can see if the seen results match up with what was predicted in each hypothesis.
- Analysis and Interpretation of Data: Hypotheses give us a way to study and make sense of information. Researchers look at what they found and see if it matches the guesses made in their theories. They decide if the proof backs up or disagrees with these suggested reasons why things are happening as expected.
- Encouraging Objectivity: Hypotheses help make things fair by making sure scientists use facts and information to either agree or disagree with their suggested reasons. They lessen personal preferences by needing proof from experience.
- Iterative Process: People either agree or disagree with guesses, but they still help the ongoing process of science. Findings from testing ideas make us ask new questions, improve those ideas and do more tests. It keeps going on in the work of science to keep learning things.
People Also View:
Mathematics Maths Formulas Branches of Mathematics
Summary – Hypothesis
A hypothesis is a testable statement serving as an initial explanation for phenomena, based on observations, theories, or existing knowledge. It acts as a guiding light for scientific research, proposing potential relationships between variables that can be empirically tested through experiments and observations. The hypothesis must be specific, testable, falsifiable, and grounded in prior research or observation, laying out a predictive, if-then scenario that details a cause-and-effect relationship. It originates from various sources including existing theories, observations, previous research, and even personal curiosity, leading to different types, such as simple, complex, directional, non-directional, null, and alternative hypotheses, each serving distinct roles in research methodology. The hypothesis not only guides the research process by shaping objectives and designing experiments but also facilitates objective analysis and interpretation of data, ultimately driving scientific progress through a cycle of testing, validation, and refinement.
FAQs on Hypothesis
What is a hypothesis.
A guess is a possible explanation or forecast that can be checked by doing research and experiments.
What are Components of a Hypothesis?
The components of a Hypothesis are Independent Variable, Dependent Variable, Relationship between Variables, Directionality etc.
What makes a Good Hypothesis?
Testability, Falsifiability, Clarity and Precision, Relevance are some parameters that makes a Good Hypothesis
Can a Hypothesis be Proven True?
You cannot prove conclusively that most hypotheses are true because it’s generally impossible to examine all possible cases for exceptions that would disprove them.
How are Hypotheses Tested?
Hypothesis testing is used to assess the plausibility of a hypothesis by using sample data
Can Hypotheses change during Research?
Yes, you can change or improve your ideas based on new information discovered during the research process.
What is the Role of a Hypothesis in Scientific Research?
Hypotheses are used to support scientific research and bring about advancements in knowledge.
Please Login to comment...
Similar reads.
- Geeks Premier League 2023
- Maths-Class-12
- Geeks Premier League
- Mathematics
- School Learning
- What are Tiktok AI Avatars?
- Poe Introduces A Price-per-message Revenue Model For AI Bot Creators
- Truecaller For Web Now Available For Android Users In India
- Google Introduces New AI-powered Vids App
- 30 OOPs Interview Questions and Answers (2024)
Improve your Coding Skills with Practice
What kind of Experience do you want to share?
Data Analysis for Leadership & Public Affairs:
Chapter 8 the logic of hypothesis testing.
All of us hypothesize all the time. We look at the world around us, something that happened yesterday or might happen tomorrow, and try to concoct a story in our head about the chance of yesterday’s/tomorrow’s event happening. We may even weave together a silent, internalized narrative about how the event came about. These stories in our head are conjectures, our best guesses, maybe even biased thoughts, but they have not been tested with data. For example, you may be a city employee in the Streets and Sanitation department who feels the city has gotten dirtier over the past five years. You may be an admissions counselor who thinks the academic preparation of the pool of high school graduates applying to your university has gotten better over the years. Maybe you work as a program evaluation specialist in the Ohio Department of Education and need to find out if a dropout recovery program has made any difference in reducing high-school dropout rates. These suspicions, the motivating questions, are hypotheses that have not yet been tested with data. If you were keen to test your hypothesis, you would have to follow an established protocol that starts with a clear articulation of the hypotheses to be tested. Formally, we define hypothesis testing as an inferential procedure that uses sample data to evaluate the credibility of a hypothesis about a population parameter . The process involves (i) stating a hypothesis, (ii) drawing a sample to test the hypothesis, and (iii) carrying out the test to see if the hypothesis should be rejected (or not). Before we proceed, however, memorize the following statement: A hypothesis is an assumption that can neither be proven nor disproven without a reasonable shadow of doubt, a point that will become all too clear by the time we end this chapter. 10
8.1 Articulating the Hypotheses to be tested
Hypotheses are denoted by \(H\) , for example,
- \(H\) : Not more than 5% of GM trucks breakdown in under 10,000 miles
- \(H\) : Heights of North American adult males is distributed with \(\mu = 72\) inches
- \(H\) : Mean year-round temperature in Athens (OH) is \(> 62\)
- \(H\) : 10% of Ohio teachers have the highest rating (Accomplished)
- \(H\) : Mean county unemployment rate in an Appalachian county is 12.1%
- \(H\) : The Dropout Recovery program has reduced average dropout rates to be no higher than 30%
Hypotheses come in pairs, one the null hypothesis and the other the alternative hypothesis . The null hypothesis, often denoted as \(H_{0}\) , is the assumption believed to be true while the alternative hypothesis, often denoted as \(H_{a}\) or \(H_{1}\) is the statement believed to be true if \(H_{0}\) is rejected by the statistical test. Some expressions follow, all in the context of average heights of adult males in North America
- \(H_{0}\) : \(\mu > 72\) inches; \(H_{1}\) : \(\mu \leq 72\)
- \(H_{0}\) : \(\mu < 72\) inches; \(H_{1}\) : \(\mu \geq 72\)
- \(H_{0}\) : \(\mu \leq 72\) inches; \(H_{1}\) : \(\mu > 72\)
- \(H_{0}\) : \(\mu \geq 72\) inches; \(H_{1}\) : \(\mu < 72\)
- \(H_{0}\) : \(\mu = 72\) inches; \(H_{1}\) : \(\mu \neq 72\)
\(H_{0}\) and \(H_{1}\) are mutually exclusive and exhaustive , mutually exclusive in the sense that either \(H_{0}\) or \(H_{1}\) can be true at a given point in time (both cannot be true at the same point in time), and exhaustive in that \(H_{0} + H_{1}\) exhaust the sample space (there is no third or fourth possibility unknown to us).
When we setup our null and alternative hypotheses we must pay careful attention to how we word each hypothesis. In particular, we usually want the alternative hypothesis to be the one we hope is supported by the data and the statistical test while the null hypothesis is the one to be rejected. Why? Because rejecting the null implies the alternative must be true. As a rule, then, always setup the alternative hypothesis first and then setup the null hypothesis. For example, say I suspect that dropout rates have dropped below 30% and I hope to find this suspicion supported by the test. I would then setup the hypotheses as follows:
\[\begin{array}{l} H_0: \text{ mean dropout rate } \geq 30\% \\ H_1: \text{ mean dropout rate } < 30\% \end{array}\]
If I don’t know whether dropout rates have decreased or increased, I just suspect they are not what they were last year (which may have been, say, 50%), then I would set these up as follows:
\[\begin{array}{l} H_0: \text{ mean dropout rate } = 50\% \\ H_1: \text{ mean dropout rate } \neq 50\% \end{array}\]
Notice the difference between the two pairs of statements. On the first pass I had a specific suspicion, that rates had fallen below 30% and so I setup the hypotheses with this specificity in mind . On the second go around I had less information to work with, just a feeling that they weren’t 50% and hence I setup less specific hypotheses . This leads us to a very important point you should always bear in mind: Hypotheses testing is only as good as the underlying substantive knowledge used to develop the hypotheses. If you know nothing about the subject you are researching, your hypothesis development will be blind to reality and the resulting \(H_0\) and \(H_1\) almost guaranteed to be incorrectly setup. There is thus no substitute to reading as widely as possible before you embark on sampling and hypothesis testing.
8.2 Type I and Type II Errors
Hypothesis testing is fraught with the danger of drawing the wrong conclusion, and this is true in all research settings. This means that whenever you reject a null hypothesis, there is always some non-zero probability, however small it may be, that in fact you should not have rejected the null hypothesis. Similarly, every time you fail to reject the null hypothesis there is always some non-zero probability that in fact you should have rejected the null hypothesis. Why does this happen? It happens because we do not see the population and are forced to work with the sample we drew, and samples can mislead us by sheer chance. Let us formalize this tricky situation.
Assume there are two states of the world, one where the null hypothesis is true (but we don’t know this) and the other where the null hypothesis is false (but we don’t know this).
The correct decisions are shown in the green cells while the incorrect decisions are shown in the red cells. The two errors that could happen are labeled Type I and Type II errors, respectively. A Type I error is a false positive and occurs if you reject the null hypothesis when you shouldn’t have, and a Type II error is a false negative and occurs if you fail to reject the null hypothesis when you should have. When we carry out a hypothesis test we usually default to minimizing the probability of a Type I error but in some cases the probability of a Type II error may be the one we wish to minimize. Both cannot be minimized at the same time. Why these errors are omnipresent in every hypothesis test and why both cannot be minimized at the same time will become clear once we do a few hypothesis tests.
The probability of a Type I error given that the null hypothesis is true is labeled \(\alpha\) and the probability of a Type II error given that the null hypothesis is false is labeled \(\beta\) . The probability of correctly rejecting a false null hypothesis equals \(1- \beta\) and is called the power of a test . Estimating the power of a test is a fairly complicated task and taken up in some detail in a later Chapter.
We also refer to \(\alpha\) as the level of significance of a test, and conventionally two values are used for \(\alpha\) , \(\alpha = 0.05\) and \(\alpha = 0.01\) . With \(\alpha = 0.05\) we are saying we are comfortable with a false positive, with rejecting the null hypothesis when we shouldn’t have, in at most five out of every 100 tests of the same hypotheses with similarly sized random samples. With \(\alpha = 0.01\) we are saying we are comfortable with a false positive, with rejecting the null hypothesis when we shouldn’t have, in at most one out of every 100 tests of the same hypotheses with similarly sized random samples. If you want a lower false positive rate then go with \(\alpha = 0.01\) . There is a trade-off, however: If you choose a lower false positive rate \((\alpha = 0.01)\) then it will generally be harder to reject the null hypothesis than it would have been with a higher false positive rate \((\alpha = 0.05)\) .
8.3 The Process of Hypothesis Testing: An Example
Assume we want to know whether the roundabout on SR682 in Athens, Ohio, has had an impact on traffic accidents in Athens. We have historical data on the number of accidents in years past. Say the average per day used to be 6 (i.e., \(\mu = 6\) ). To see if the roundabout has had an impact, we could gather accident data for a random sample of 100 days \((n=100)\) from the period after the roundabout was built. Before we do that though, we will need to specify our hypotheses. What do we think might be the impact? Let us say the City Engineer argues that the roundabout should have decreased accidents. If he is correct then the sample mean ( \(\bar{x}\) ) should be less than the population mean ( \(\mu\) ) i.e., \(\bar{x} < \mu\) . If he is wrong then the sample mean ( \(\bar{x}\) ) should be at least as much as the population mean ( \(\mu\) ) i.e., \(\bar{x} \geq \mu\) .
We know from the theory of sampling distributions that the distribution of sample means, for all samples of size \(n\) , will be normally distributed. Most sample means would be in the middle of the distribution but, of course, we know that by sheer chance we could end up with a sample mean from the tails (the upper and lower extremes of the distribution). This will happen with a very small probability but it could happen, so bear this possibility in mind. The sampling distribution is shown below:

FIGURE 8.1: Sampling Distribution of Mean Traffic Accidents
If we believe the City Engineer, we would setup the hypotheses as \(H_0\) : The roundabout does not reduce accidents, i.e., \(\mu \geq \mu_0\) , and \(H_1\) : The roundabout does reduce accidents, i.e., \(\mu < \mu_0\) .
We then calculate the sample mean \((\bar{x})\) and the sample standard deviation \((s)\) , then the standard error of the sample mean: \(s_{\bar{x}} = \dfrac{s}{\sqrt{n}}\) , and finally the \(t_{calculated} = \dfrac{\bar{x} - \mu}{s_{\bar{x}}}\) . The next step would involve using \(df=n-1\) and finding the area to the left of \(t_{calculated})\) . If this area were very small then we could conclude that the roundabout must have worked to reduce accidents. How should we define ``very small’’? By setting \(\alpha\) either to 0.05 or to 0.01. Once we set \(\alpha\) we would Reject \(H_0\) if \(P(t_{calculated}) \leq \alpha\) because this would imply that the probability of getting a \(t\) value such as the one we calculated so far from the middle of the distribution, by sheer chance, is very small. Thus our findings must be reliable, the data must be providing sufficient evidence to conclude that the roundabout has reduced accidents. If, however, \(P(t_{calculated}) > \alpha\) then we would Fail to reject \(H_0\) ; the data would be providing us with insufficient evidence to conclude that the roundabout has reduced accidents.

FIGURE 8.2: Rejecting the Null

FIGURE 8.3: Failing to Reject the Null
In the plots above, the green region in the figure shows where we need our calculated \(t\) to fall before we can reject the null hypothesis, and the grey region shows the area where we need our calculated \(t\) to fall if we are not rejecting the null hypothesis. The green region is often referred to as the critical region or the rejection region .
What if the City Engineer had no clue whether accidents might have decreased or increased? Well, in that case we would we would setup the hypotheses as \(H_0\) : The roundabout has no impact on accidents, i.e., \(\mu = \mu_0\) , and \(H_1\) : The roundabout has an impact on accidents, i.e., \(\mu \neq \mu_0\) . Next steps would be to calculate the sample mean \((\bar{x})\) and the sample standard deviation \((s)\) , followed by the standard error of the sample mean: \(s_{\bar{x}} = \dfrac{s}{\sqrt{n}}\) , then \(t_{calculated} = \dfrac{\bar{x} - \mu}{s_{\bar{x}}}\) . Using \(df=n-1\) , we would then find the area to the left/right of \(\pm t_{calculated})\) . If this area were very small we would conclude that the roundabout must have worked to reduce accidents. Once again, how should we define “very small?” By setting \(\alpha\) either to 0.05 or to 0.01. We could then Reject \(H_0\) if \(P(\pm t_{calculated}) \leq \alpha\) ; the data would be providing us with sufficient evidence to conclude that the roundabout had an impact on accidents. If \(P(\pm t_{calculated}) > \alpha\) then we would Fail to reject \(H_0\) ; the data would be providing us with insufficient evidence to conclude that the roundabout had an impact on accidents. Watch the plots below to see the critical region in this particular scenario.

FIGURE 8.4: Rejecting the Null

FIGURE 8.5: Failing to Reject the Null
Note the difference between the first time we tested the City Engineer’s suspicions and the second time we did so.
- In the first instance he/she had a very specific hypothesis, that accidents would have decreased. This led us to specify \(H_1: \mu < \mu_{0}\) . Given the inequality \(<\) we focused only on the left side of the distribution to decide whether to reject the null hypothesis or not.
- In the second instance he/she had a vague hypothesis, that accidents will occur at a different rate post-construction of the roundabout. This led us to specify \(H_1: \mu \neq \mu_{0}\) . Given the possibility that accidents could have increased/decreased, we now focused on both sides of the distribution (left and right) to decide whether to reject the null hypothesis or not.
Hypothesis tests of the first kind are what we call one-tailed tests and those of the second kind are what we call two-tailed tests . Once we do a few hypothesis tests I will explain how two-tailed tests require more evidence from your data in order to reject the null hypothesis; one-tailed tests make it relatively easier to reject the null hypothesis. For now, here is recipe for specifying hypotheses …
- If we want to test whether something has “changed” then \(H_0\) must specify that nothing has changed \(\ldots H_0:\mu = \mu_{0}; H_1: \mu \neq \mu_{0} \ldots\) two-tailed
- If we want to test whether something is “different” then \(H_0\) must specify that nothing is different \(\ldots H_0:\mu = \mu_{0}; H_1: \mu \neq \mu_{0}\ldots\) two-tailed
- If we want to test whether something had an “impact” then \(H_0\) must specify that it had no impact \(\ldots H_0:\mu = \mu_{0}; H_1: \mu \neq \mu_{0}\ldots\) {two-tailed}
- If we want to test whether something has “increased” then \(H_0\) must specify that it has not increased \(\ldots H_0:\mu \leq \mu_{0}; H_1: \mu > \mu_{0}\ldots\) {one-tailed}
- If we want to test whether something has “decreased” then \(H_0\) must specify that it has not decreased \(\ldots H_0:\mu \geq \mu_{0}; H_1: \mu < \mu_{0}\ldots\) {one-tailed}
- Collect the sample and set \(\alpha=0.05\) or \(\alpha=0.01\)
- Calculate \(s_{\bar{x}} = \dfrac{s}{\sqrt{n}}\) , \(\bar{x}\) , \(df=n-1\)
- Calculate the \(t\)
- Reject \(H_0\) if calculated \(t\) falls in the critical region ; do not reject \(H_0\) otherwise
8.3.1 Example 1
Last year, Normal (IL) the city’s motor pool maintained the city’s fleet of vehicles at an average cost of \(\$346\) per car. This year Jack’s Crash Shop is doing the maintenance. The city engineer notices that in a random sample of 36 cars repaired by Jack, the mean repair cost is \(\$330\) with a standard deviation of \(\$120\) . Is going with Jack saving the city money?
Here are the hypotheses: \(H_0: \mu \geq 346\) and \(H_1: \mu < 346\)
Let us choose \(\alpha = 0.05\) and calculate the degrees of freedom: \(df=n-1=36-1=35\) . We need the standard error so let us calculate that: \(s_{\bar{x}} = \dfrac{s}{\sqrt{n}} = \dfrac{120}{\sqrt{36}} = 20\) . The calculated \(t\) will be \(t = \dfrac{\bar{x} - \mu_{0}}{s_{\bar{x}}} = \dfrac{330-346}{20} = \dfrac{-16}{20} = -0.80\)

FIGURE 8.6: Example 1
The green area shows the critical region, while the gray area shows the rest of the distribution. The red dot shows where our calculated \(t = -0.80\) falls. Since it does not fall in the critical region we fail to reject \(H_0\) . The data provide insufficient evidence to conclude that going with Jack’s shop is saving the city money.
How did we find the critical region? We found it by looking up what we call the critical t value for given degrees of freedom. Here we have \(df = 35\) . If I use an online table for finding \(t\) values and areas under the distribution, like the one here , I see that the critical region starts at \(t=-1.69\) and extends left to the end of the distribution’s left-tail. This is the green area in the plot.
So another way of deciding whether to reject the null or not is to compare the calculated \(t\) to the critical \(t\) using the following guidelines:
- \(H_1: \mu < \mu_{0}\) , reject \(H_0\) if \(-t_{calculated} \leq -t_{critical}\)
- \(H_1: \mu > \mu_{0}\) , reject \(H_0\) if \(t_{calculated} \geq t_{critical}\)
- \(H_1: \mu \neq \mu_{0}\) , reject \(H_0\) if \(\lvert{t_{calculated}}\rvert \geq \lvert{t_{critical}}\rvert\) Using this rule, my calculated \(t = -0.80\) is not less than or equal to the critical \(t = -1.69\) and so I cannot reject the null hypothesis.
I could have also just looked up my calculated \(t\) and then found \(P(t \leq -0.80)\) . If this area were smaller than \(\alpha\) , I could reject the null hypothesis. If this area were greater than \(\alpha\) I would fail to reject the null hypothesis. Using the online table if I enter values for the calculated \(t\) and the degrees of freedom I see the area to be \(0.2146\) . This is quite a bit larger than \(\alpha = 0.05\) so I fail to reject the null hypothesis. This is the more common way of making decisions about hypothesis tests so let us memorize this rule:
- \(P(t \leq t_{calculated}) \leq \alpha\) : Reject the null hypothesis
- \(P(t \leq t_{calculated}) > \alpha\) : Do not reject the null hypothesis
8.3.2 Example 2
Kramer’s (TX) Police Chief learns that her staff clear 46.2% of all burglaries in the city. She wants to benchmark their performance and to do this she samples 10 other demographically similar cities in Texas. She finds their clearance rates to be as follows:
Is Kramer’s clearance rate significantly different from those of other similar Texas cities?
\(H_0: \mu = 46.2\) and \(H_1: \mu \neq 46.2\) .
Set \(\alpha = 0.05\) and note \(df=n-1=10-1=9\) , \(\bar{x}=40.34\) , and \(s_{\bar{x}} = 2.4279\)
\(t = \dfrac{ \bar{x} - \mu_{0} }{ s_{\bar{x}} } = \dfrac{40.34-46.2}{2.4279} = -2.414\)
Critical \(t = \lvert{2.262}\rvert\) . Our calculated \(t = -2.414\) clearly surpasses critical \(t\) and so we can reject the null hypothesis; Kramer’s clearance rate seems to be different from that of demographically similar cities.
If I looked up \(P(t \leq t_{calculated})\) I would find this to be \(0.039\) . Since this is less than \(\alpha = 0.05\) I can easily reject the null hypothesis. The graphical depiction of the decision is shown below:

FIGURE 8.7: Example 2
8.4 Confidence Intervals for Hypothesis Tests
Confidence intervals can and should be used for hypothesis tests as well because they are more informative than simply providing the sample mean and our decision about rejecting/not rejecting the null hypothesis. They are used as follows:
- Calculate the appropriate confidence interval for the \(\alpha\) used for the hypothesis test. This interval will be calculated for the sample mean (or proportion).
- See if the confidence interval traps the null value used in the hypotheses. If it does, then the null should not be rejected. If it does not, then the null should be rejected.
Let us take the Kramer (TX) example for demonstration purposes. Our sample mean was 40.34 and the population mean was 46.2, the standard error was 2.4279, the critical \(t\) was 2.262, and \(\alpha\) was 0.05. Consequently, the, 95% interval would be \(40.34 \pm 2.262(2.4279) = 40.34 \pm 5.49191 = 34.84809 \text{ and } 45.83191\) . Note that the value of 46.2 does not fall in this interval and hence we can reject the null hypothesis.
If you calculate the appropriate interval for Jack’s Crash Shop in Example 1 you will see the two-tail critical value being \(\pm 2.03224451\) and the resulting interval calculated as 289.3551 and 370.6449. In Example 1 we failed to reject the null hypothesis. Since our confidence interval includes the population value of 346 we fail to reject the null here as well.
As a rule, then, if you are able to reject the null hypothesis your confidence interval will not subsume the population value used in the null hypothesis. Likewise, if you are unable to reject the null hypothesis your confidence interval will not subsume the population value used in the null hypothesis.
8.5 The One-Sample t-test
The preceding hypothesis tests are what we call a one-sample t-test where we have a population mean, a single sample to work with, and are looking to test whether the sample mean differs from the population mean. These tests are built upon some assumptions and if these assumptions are violated the test-based conclusions become wholly unreliable.
8.5.1 Assumptions
The assumptions that apply to the one-sample t-test are:
- The variable being tested is an interval-level or ratio-level measure
- The data represent a random sample
- There are no significant outliers
- The variable(s) being tested are approximately normally distributed
The random sample assumption we generally hold to be true because if we knew we had messed up and ended up with a non-random sample, I hope we would be smart enough to avoid doing any kind of a statistical test altogether. Similarly, one would (hopefully) know that our measures were neither interval nor ratio-level and hence we should not do a t-test. The assumptions that need to be tested are thus those of normality and significant outliers . We will do these shortly.
8.6 The Two-group (or Two-sample) t-test
The more interesting tests involve two groups such as, for example, comparing reading proficiency levels across male and female students, studying the wage gap between men and women, seeing if the outcome differs between those given some medicine versus those given a placebo, and so on. The logic underlying the hypothesis test is simple: If both groups come from a common population, their means should be identical Of course, the means could differ a little by chance but a large difference in the means would happen with a small probability. If this probability is less than or equal to the test \(\alpha\) , we reject the null hypothesis.If this probability is greater than the test \(\alpha\) we do not reject the null hypothesis. Let us see this logic by way of a visual representation.
In the plot below you see the bell-shaped distribution that characterizes the population and two dots, the red marking the mean reading score for female students and the blue marking the mean reading score for male students. Both are identical; \(\mu_{female} = \mu_{male}\) , and this would be true if there was no difference between the two groups.
FIGURE 8.8: A Normal Distribution
You could, of course, end up seeing the means some distance apart purely by chance even though in the population there is no difference between the groups. This is shown for four stylized situations although the possibilities of how far apart the means might be are countless.
FIGURE 8.9: Common Parent Population
Since chance can yield the two means some distance apart, the hypothesis test is designed to help us determine whether chance is driving the drift we see or is it really the case that the groups come from separate populations. If they do come from different populations, we would expect to see the following:
FIGURE 8.10: Separate Parent Populations
Note that here the sample means are in the middle of their respective distributions, exactly what you would expect to see in most of your sample. The question thus becomes whether any differences in sample means that we see should be attributed to chance or to the rejection of the belief that both groups share a common parent population. The hypotheses are setup as shown below:
- Two-Tailed Hypotheses: \(H_0\) : \(\mu_{1} = \mu_{2}\) ; \(H_A\) : \(\mu_{1} \neq \mu_{2}\) and these can be rewritten as \(H_0\) : \(\mu_{1} - \mu_{2} = 0\) ; \(H_A\) : \(\mu_{1} - \mu_{2} \neq 0\)
- One-Tailed Hypotheses: \(H_0\) : \(\mu_{1} \leq \mu_{2}\) ; \(H_A\) : \(\mu_{1} > \mu_{2}\) , rewritten as \(H_0\) : \(\mu_{1} - \mu_{2} \leq 0\) ; \(H_A\) : \(\mu_{1} - \mu_{2} > 0\)
- One-Tailed Hypotheses: \(H_0\) : \(\mu_{1} \geq \mu_{2}\) ; \(H_A\) : \(\mu_{1} < \mu_{2}\) , rewritten as \(H_0\) : \(\mu_{1} - \mu_{2} \geq 0\) ; \(H_A\) : \(\mu_{1} - \mu_{2} < 0\)
The test static is \(t = \dfrac{\left(\bar{x}_{1} - \bar{x}_{2} \right) - \left(\mu_{1} - \mu_{2} \right)}{SE_{\bar{x}_{1} - \bar{x}_{2}}}\) , with \(x_1\) and \(x_2\) representing the sample means of group 1 and group 2, respectively.
Given that we have two groups we calculate the standard errors and the degrees of freedom in a more nuanced manner. In particular, we have to decide whether, assuming the null hypothesis to be true (i.e., the groups do indeed come from two different parent populations), these populations have (i) equal variance , or (ii) unequal variances .
Assuming Equal Variances
With equal population variances, we calculate the standard error as:
\[s_{\bar{x}_1 - \bar{x}_2} = \sqrt{\dfrac{n_1 + n_2}{n_1n_2}}\sqrt{\dfrac{(n_1 -1)s^{2}_{x_{1}} + (n_2 -1)s^{2}_{x_2}}{\left(n_1 + n_2\right) -2}}\]
and the degrees of freedom are \(df = n_1 + n_2 - 2\) . Note that \(s^{2}_{x_1}\) and \(s^{2}_{x_2}\) are the variances of group 1 and group 2, respectively, while \(n-1\) and \(n_2\) are the sample sizes of groups 1 and 2, respectively.
Assuming Unequal Variances
With unequal populations variances things get somewhat tricky because now we calculate the standard error as
\[s_{\bar{x}_1 - \bar{x}_2} = \sqrt{\dfrac{s^{2}_{x_1}}{n_1} + \dfrac{s^{2}_{x_2}}{n_2}}\]
and the approximate degrees of freedom as
\[\dfrac{\left(\dfrac{s^{2}_{x_1}}{n_1} + \dfrac{s^{2}_{x_2}}{n_2} \right)^2}{\dfrac{1}{(n_1 -1)}\left(\dfrac{s^{2}_{x_1}}{n_1}\right)^2 + \dfrac{1}{(n_2 -1)}\left(\dfrac{s^{2}_{x_2}}{n_2}\right)^2}\]
These degrees of freedom are derived from the Welch-Satterthwaite equation , named for its authors, and hence the two-group t-test with unequal variances assumed is also called Welch's t-test .
Once again, regardless of equal or unequal variances assumed, our decision criteria do not change: Reject the null hypothesis if the p-value of the calculated \(t\) is \(\leq \alpha\) ; do not reject otherwise. Let us see a few examples, although I will not manually calculate the standard errors or degrees of freedom since these days statistical software would be used de jure .
Assumptions
The two-group t-test is driven by some assumptions.
- Random samples … usually assumed to be true otherwise there would be no point to doing any statistical test
- Variables are drawn from normally distributed Populations … this can be formally tested and we will see how to carry out these tests shortly. However, I wouldn’t worry too much if normality is violated. Bear this in mind.
- Variables have equal variances in the Population … this can be formally tested and we will see how to carry out these tests shortly
Although we will be testing two of the assumptions, you may want to bear the following rules of thumb in mind since testing assumptions is not always a cut-and-dried affair.
- Draw larger samples if you suspect the Population(s) may be skewed
- Assumption theoretically justified, standard deviations fairly close
- \(n_1 \geq 30\) and \(n_2 \geq 30\)
- One standard deviation is at least twice the other standard deviation
- \(n_1 < 30\) or \(n_2 < 30\)
Note also that the t-test is robust in large samples and survives some degree of skewness so long as the skew is of similar degree and direction in each group. How large is “large?” Excellent question but one without a formulaic answer since disciplines define large differently and the sample sample size that someone might see as “small” would appear “large” to others.
8.6.1 Example 1
The Athens County Public Library is trying to keep its bookmobile alive since it reaches readers who otherwise may not use the library. One of the library employees decides to conduct an experiment, running advertisements in 50 areas served by the bookmobile and not running advertisements in 50 other areas also served by the bookmobile. After one month, circulation counts of books are calculated and mean circulation counts are found to be 526 books for the advertisement group with a standard deviation of 125 books and 475 books for the non-advertisement group with a standard deviation of 115 books. Is there a statistically significant difference in mean book circulation between the two groups?
Since we are being asked to test for a “difference” it is a two-tailed test, with hypotheses given by:
\[\begin{array}{l} H_0: \text{ There is no difference in average circulation counts } (\mu_1 = \mu_2) \\ H_1: \text{ There is a difference in average circulation counts } (\mu_1 \neq \mu_2) \end{array}\]
Since both groups have sample sizes that exceed 30 we can proceed with the assumption of equal variances and calculate the standard error and the degrees of freedom. The degrees of freedom as easy: \(df = n_1 + n_2 - 2 = 50 + 50 - 2 = 98\) . The standard error is \(s_{\bar{x}_1 - \bar{x}_2} = \sqrt{\dfrac{n_1 + n_2}{n_1n_2}}\sqrt{\dfrac{(n_1 -1)s^{2}_{x_{1}} + (n_2 -1)s^{2}_{x_2}}{\left(n_1 + n_2\right) -2}}\) and plugging in the values we have
\[s_{\bar{x}_1 - \bar{x}_2} = \sqrt{\dfrac{50 + 50}{2500}} \sqrt{\dfrac{(50 -1)(125^2) + (50 -1)(115^2)}{\left(50 + 50\right) -2}} = (0.2)(120.1041) = 24.02082\]
The test statistic is
\[t = \dfrac{\left( \bar{x}_1 - \bar{x}_2 \right) - \left( \mu_1 - \mu_2 \right) }{s_{\bar{x}_1 - \bar{x}_2}} = \dfrac{(526 - 475) - 0}{24.02082} = \dfrac{51}{24.02082} = 2.123158\]
Since no \(\alpha\) is given let us use the conventional starting point of \(\alpha = 0.05\) . With \(df=98\) and \(\alpha = 0.05\) , two-tailed, the critical \(t\) value would be \(\pm 1.98446745\) . Since our calculated \(t = 2.1231\) exceeds the critical \(t = 1.9844\) , we can easily reject the null hypothesis of no difference. These data suggest there is a difference in average circulation counts between the advertisement and no advertisement groups.
We could have also used the the p-value approach, rejecting the null hypothesis of no difference if the p-value was \(\leq \alpha\) . The p-value of our calculated \(t\) turns out to be 0.0363 and so we can reject the null hypothesis. Note, in passing, that had we used \(\alpha = 0.01\) we would have been unable to reject the null hypothesis because \(0.0363\) is \(> 0.01\) .
The 95% confidence interval is given by \((\bar{x}_1 - \bar{x}_2) \pm t_{\alpha/2}(s_{\bar{x}_1 - \bar{x}_2}) = 51 \pm 1.9844(24.02082) = 51 \pm 47.66692 = 3.33308 \text{ and } 98.66692\) . We can be about 95% confident that the true difference between the groups lies in this interval. Had we used the 99% interval for a test with \(\alpha = 0.01\) the interval would be \(51 \pm 2.627(24.02082) = -12.10269 \text{ and } 114.1027\) , subsuming the null hypothesis difference of \(0\) and leaving us unable to reject the null hypothesis.
8.6.2 Example 2
Say we have a large data-set with a variety of information about several cars, gathered in 1974. One of the questions we have been tasked with testing is whether the miles per gallon yield of manual transmission cars in 1974 was greater than that of automatic transmission cars. Assume they want us to use \(\alpha = 0.05\) .
We have thirteen manual transmission cars and 19 automatic transmission cars, and the means and standard deviations are 24.3923 and 6.1665 for manual, and 17.1473 and 3.8339 for automatic cars, respectively. The hypotheses are:
\[\begin{array}{l} H_0: \text{Mean mpg of manual cars is } \leq \text{ the mean mpg of automatic cars} (\bar{x}_{man} \leq \bar{x}_{auto}) \\ H_1: \text{Mean mpg of manual cars is } > \text{ the mean mpg of automatic cars} (\bar{x}_{man} > \bar{x}_{auto}) \end{array}\]
The calculated \(t\) is 4.1061 and the p-value is 0.0001425, allowing us to reject the null hypothesis. The data suggest that average mpg of manual cars is not not \(\leq\) that of automatic cars.
Note a couple of things here: (i) We have a one-tailed hypothesis test, and (ii) we are assuming equal variances since both conditions are not met for assuming unequal variances. In addition, note that the confidence interval is found to be \((3.6415, 10.8483)\) , indicating that we can be 95% confident that the average manual mpg is higher than average automatic mpg by anywhere between 3.64 mpg and 10.84 mpg.
8.6.3 Caution
If, for two groups, the confidence intervals around each group mean do not overlap with that of the other group, we can safely infer the two groups are statistically significantly different. Be careful, however, to not automatically infer no statistically significant differences just because the confidence intervals of two groups overlap. Why, you ask? See here and here . This is a common mistake a lot of us slip into.
8.7 Paired t-tests
Assume, for example, that we have measured some outcome for the same set of individuals at two points in time. This situation could be akin to gathering a random sample of \(6^{th}\) grade students, recording their mathematics scores on the statewide assessment, and then some years later recording their \(8^{th}\) grade mathematics scores on the statewide assessment. We might be curious to know if on average, students’ mathematics performance has improved over time, either organically or because of some special mathematics tutoring program that implemented in the school. Or we may have a situation where the question is whether hospitalization tends to depress patients and so we measure their mental health before hospitalization and then again after hospitalization.
We need not have the same individuals measured twice; paired t-tests also work with two groups with different individuals in each group so long as the groups are similar . What does that mean? Well, think of it as follows. Say I am interested in the hospitalization question. I know that if I administer some mental health assessment (most likely with a battery of survey-type questions) prior to hospitalization, and then I go back with the same battery of questions post-hospitalization, the patients might catch on to the purpose of my study and tailor their responses accordingly. If they don’t respond honestly to my questions, the resulting data will be contaminated. How can I get around this hurdle? A common technique used in situations such as these, where you cannot go back to the same set of individuals, involves finding individuals who are similar to the first group such that both groups have the same average age of the patients, the same proportion of males versus females, similar distributions of income, pre-existing medical conditions, and so on. If we employ such an approach then we have what we call a matched pairs design .
The following assumptions apply to paired t-tests:
- Variables are drawn from normally distributed Populations … this can be formally tested and we will see how to carry out these tests shortly
The Testing Protocol
Let us see how the test is carried out with reference to a small data-set wherein we have six pre-school children’s scores on a vocabulary test before a reading program is introduced into the pre-school \((x_1)\) and then again after the reading program has been in place for a few months \((x_2)\) .
Note the column \(d_i\) has the difference of the scores such that for Child 1, \(6.0 - 5.4 = 0.6\) , for Child 2, \(5.0 - 5.2 = -0.2\) , and so on. The mean, variance and standard deviation of \(d\) are calculated as follows:
\[\begin{eqnarray*} d_{i} &=& x_{1} - x_{2} \\ \bar{d} &=& \dfrac{\sum{d_i}}{n} \\ s^{2}_{d} &=& \dfrac{\sum(d_i - \bar{d})^2}{n-1} \\ s_d &=& \sqrt{\dfrac{\sum(d_i - \bar{d})^2}{n-1}} \end{eqnarray*}\]
Note also that if the reading program is very effective, then we should see average scores being higher post-intervention, even if some students don’t necessarily improve their individual scores.
Say we have no idea what to expect from the program. In that case, our hypotheses would be:
\[\begin{array}{l} H_0: \mu_d = 0 \\ H_1: \mu_d \neq 0 \end{array}\]
The test statistic is given by \(t = \dfrac{\bar{d} - \mu_d}{s_d/\sqrt{n}}; df=n-1\) and the interval estimate calculated as \(\bar{d} \pm t_{\alpha/2}\left(\dfrac{s_d}{\sqrt{n}}\right)\) .
Once we have specified our hypotheses, selected \(\alpha\) , and calculated the test statistic, the usual decision rules apply: Reject the null hypothesis if the calculated \(p-value \leq \alpha\) ; do not reject the null hypothesis if the calculated \(p-value > \alpha\) .
In this particular example, it turns out that \(\bar{d}=0.30\) ; \(s_d=0.335\) ; \(t = 2.1958\) , \({\circled{\color{blue}{df = 5}}}\) , \(p-value = 0.07952\) and 95% CI: \(0.3 \pm 0.35 = (-0.0512, 0.6512)\) .
Given the large \(p-value\) we fail to reject \(H_0\) and conclude that these data do not suggest a statistically significant impact of the reading program.
8.7.1 Example 1
Over the last decade, has poverty worsened in Ohio’s public school districts? One way to test worsening poverty would be to compare the percent of children living below the poverty line in each school district across two time points. For the sake of convenience I will use two American Community Survey (ACS) data sets that measure Children Characteristics (Table S0901) , one the 2011-2015 ACS and the other the 2006-2010 ACS. While a small snippet of the data are shown below for the 35 school districts with data for both years, you can download the full dataset from here .
\[\begin{array}{l} H_0: \text{ Poverty has not worsened } (d \leq 0) \\ H_1: \text{ Poverty has worsened } (d > 0) \end{array}\]
Subtracting the 2006-2010 poverty rate from the 2011-2015 poverty rate for each district and then calculating the average difference \((d)\) yields \(\bar{d} = 4.328571\) and \(s_{d} = 3.876746\) . With \(n=35\) we have a standard error \(s_{\bar{d}} = \dfrac{s_d}{\sqrt{n}} = \dfrac{3.876746}{\sqrt{35}} = 0.6552897\) . The test statistic is \(t = \dfrac{\bar{d}}{s_{\bar{d}}} = \dfrac{4.328571}{0.6552897} = 6.605584\) and has a \(p-value = 0.0000001424\) , allowing us to easily reject the null hypothesis. These data suggest that school district poverty has indeed worsened over the intervening time period. The 95% confidence interval is \((2.9968 \text{ and } 5.6602)\) .
8.7.2 Example 2
A large urban school district in a Midwestern state implemented a reading intervention to boost the district’s scores on the state’s English Language Arts test. The intervention was motivated by poor performance of the district’s \(4^{th}\) grade cohort. Three years had passed before that cohort was tested in the \(8^{th}\) grade. Did the intervention boost ELA scores, on average?
\[\begin{array}{l} H_0: \text{ Intervention did not boost ELA scores } (d \leq 0) \\ H_1: \text{ Intervention did boost ELA scores } (d > 0) \end{array}\]
We have \(\bar{d} = 14.62594\) , \(s_d = 66.27296\) , \(df = 12955\) and the standard error is \(0.5822609\) . The test statistic then is \(t = \dfrac{\bar{d}}{s_{\bar{d}}} = \dfrac{14.62594}{0.5822609} = 25.11922\) and has a \(p-value\) that is very close to \(0\) . Hence we can reject the null hypothesis; these data suggest that the reading intervention did indeed boost English Language Arts scores on average.
8.8 Chapter 8 Practice Problems
Problem 1 In the last few years Ohio, like many other states, established a framework for rating teachers and principals/assistant principals. Prior to this policy shift, the existing approach to rating educators yielded very few ineffective teachers, a problem well documented by The Widget Effect . Under the new rating system, the expectation is that the percentage of ineffective teachers will be greater than in the past. Assume that the old system yielded an average of 0.5% of ineffective educators in any school district., (i.e., \(\mu = 0.5\) ). Given this reality, establish the appropriate null and alternative hypotheses.
Assume that your team of program evaluators carries out a statistical test and rejects the null hypothesis. Which error – Type I or Type II – should you be more concerned about?
- Explain, in the context of the problem, what committing a Type I error would mean.
- Explain, in the context of the problem, what committing a Type II error would mean.
Write the missing hypothesis for each of the hypotheses listed below, both in words and with appropriate mathematical notation:
- \(H_0\) : Average amount of rainfall per year is the same today as what it was in 1977
- \(H_1\) : Adult males’ average heights have increased in recent years
- \(H_0\) : College dropout rates of first generation students are either the same as or lower than those of their non-first generation peers
- \(H_1\) : A human can swim faster in syrup than in water
- \(H_1\) : Average waiting time (in minutes) between eruptions of The Old Faithful geyser exceed 20 minutes
- \(H_0\) : Average number of words typed per minute is not affected by typing tutorials
- \(H_0\) : The number of migrant deaths in Mediterranean waters have not changed since last year
What factor can a research control to reduce the risk of committing a Type I error? What factor can a researcher control to reduce the standard error?
A researcher carried out a one-tailed hypothesis test using \(\alpha = 0.01\) and rejected \(H_0\) . Another researcher repeated the hypothesis test but with \(\alpha = 0.05\) and as a two-tailed test, and failed to reject \(H_0\) . Can both analyses be correct? Explain your answer.
Is crime in America rising or falling? The answer is not as simple as politicians make it out to be because of how the FBI collects crime data from the country’s more than 18,000 police agencies. National estimates can be inconsistent and out of date, as the FBI takes months or years to piece together reports from those agencies that choose to participate in the voluntary program. To try to fill this gap, The Marshall Project collected and analyzed more than 40 years of data on the four major crimes the FBI classifies as violent — homicide, rape, robbery and assault — in 68 police jurisdictions with populations of 250,000 or greater. The research team calculated the rate of crime in each category and for all violent crime, per 100,000 residents in the jurisdiction.
- Setup the appropriate hypotheses to test the suggestion that violent crimes per 100,000 (violent_per_100k) exceeded 800 in 2015.
- Using \(\alpha = 0.05\) , carry out the hypothesis test and state your conclusion in words.
- Report and interpret the corresponding confidence interval for your estimate of average violent crimes per 100,000 in 2015.
With The Marshall Project data-set, answer the questions that follow .
- Setup the hypotheses to test the belief that in 2015, larger cities (defined as cities with population sizes \(\geq 500,000\) ) have more violent crimes per 100,000 than smaller cities (defined as cities with population sizes \(< 500,000\) ). The variable is called total_pop in the data-set.
- Carry out the appropriate test and state your conclusion in words. Use \(\alpha = 0.01\) .
- Report and interpret the corresponding confidence interval for your estimated difference in average violent crimes per 100,000 in small versus large cities.
Continuing on with the Marshall Project data-set,
- Setup the hypotheses to test the belief that cities’ homicides per 100,000 declined between 1975 and 2015.
- Carry out the appropriate test and state your conclusion in words. Use \(\alpha = 0.05\) .
- Report and interpret the corresponding confidence interval for your estimated difference.
The Evidence and Data for Gender Equality (EDGE) project is a joint initiative of the United Nations Statistics Division and UN Women that seeks to improve the integration of gender issues into the regular production of official statistics for better, evidence-based policies. EDGE aims to accelerate existing efforts to generate internationally comparable gender indicators on health, education, employment, entrepreneurship and asset ownership in three many ways, one of which is to develop an online interactive platform to disseminate gender-relevant data on education, employment, and health.
We will focus on the maternal mortality ratio (modeled estimate, per 100,000 live births), available for the 1990 - 2015 period by country and region. You can access this indicator from this file .
Two questions are of interest here:
- a belief that in 2015 the average maternal mortality ratio was \(< 160\) , and
- an interest in seeing if on average maternal mortality ratios declined between 1990 and 2015.
Setup the appropriate hypothesis for questions (1) and (2), carry out the test (choose an appropriate value for \(\alpha\) ), state your conclusion in words, and both report and interpret the corresponding confidence interval estimates.
On September \(25^{th}\) 2015, countries adopted a set of goals to end poverty, protect the planet, and ensure prosperity for all as part of a new sustainable development agenda. Each goal has specific targets to be achieved over the next 15 years. The World Bank has a wonderful database that provides country-level information on a variety of indicators that feature in The Atlas of Sustainable Development Goals .
Focus on a specific indicator – Access to a mobile phone or internet at home” for males and females aged 15 years old or older, respectively, in 2014. A casual observer suspects that gender discrimination leads to males having differential access a mobile phone or internet at home. Test this suspicion with the data-set available here . State your conclusions, including the interpretation of the confidence intervals for your estimated difference.
The Ohio Department of Education gathers and releases a wealth of data on public schools and community schools; see, for instance, here and here . A snippet of these data are provided for you in this file . Note that you have data for community schools and public school districts; private and parochial schools are excluded.
Thirty-two of our 88 counties are Appalachian, as flagged by the variable Appalachian in the data-set. Focusing on third-grade reading proficiency rates (X3rd.Grade.Reading) , test whether the proportion of third-grade students rated proficient or better in reading differs between Appalachian and non-Appalachian districts. Report and interpret your conclusion and the confidence interval for the estimated difference.
Problem 11 The Lalonde data-set with 722 observations on the following 12 variables
- age = age in years.
- educ = years of schooling.
- black = indicator variable for blacks (1 = Black).
- hisp = indicator variable for Hispanics (1 = Hispanic).
- married = indicator variable for martial status (1 = Married).
- nodegr = indicator variable for high school diploma (1 = no high school graduate or equivalent).
- re75 = real earnings in 1975.
- re78 = real earnings in 1978.
- treat = an indicator variable for treatment status (1 = treatment group).
“Key to this data-set is the treatment, which refers to the National Supported Work Demonstration (NSW), a temporary employment program designed to help disadvantaged workers lacking basic job skills move into the labor market by giving them work experience and counseling in a sheltered environment. Unlike other federally sponsored employment and training programs, the NSW program assigned qualified applicants to training positions randomly. Those assigned to the treatment group received all the benefits of the NSW program, while those assigned to the control group were left to fend for themselves.” Lalonde (1986) . Note that real earnings in 1975 are before the NSW program began and real earnings in 1978 are the outcome of interest because they were recorded after the NSW treatment had occurred. Using these data answer the following questions:
- Did the NSW program have an impact on the real earnings of participants?
- Did the NSW program increase the real earnings of participants?
Focusing only on participants in the NSW program,
- Did the real earnings in 1978 differ by marital status?
- What about between participants with/without a high school degree?
“The Student/Teacher Achievement Ratio (STAR) was a four-year longitudinal class-size study funded by the Tennessee General Assembly and conducted by the State Department of Education. Over 7,000 students in 79 schools were randomly assigned into one of three interventions: small class (13 to 17 students per teacher), regular class (22 to 25 students per teacher), and regular-with-aide class (22 to 25 students with a full-time teacher’s aide). Classroom teachers were also randomly assigned to the classes they would teach. The interventions were initiated as the students entered school in kindergarten and continued through third grade. The Project STAR public access data set contains data on test scores, treatment groups, and student and teacher characteristics for the four years of the experiment, from academic year 1985–1986 to academic year 1988–1989. The test score data analyzed in this chapter are the sum of the scores on the math and reading portion of the Stanford Achievement Test.” Source These data can be downloaded from here . Note the key variables as defined below:
- gender = student’s gender 1/male, 2/female
- ethnicity = student’s ethnicity 1/caucasian, 2/african-american, 3/asian, 4/hispanic, 5/american-indian, 6/other
- stark, star1, star2, star3 = whether the student was in a 2/small classroom, 1/a regular classroom, or 3/a regular classroom with a teaching aide
- readk, read1, read2, read3 = reading test scores in kindergarten/grade 1/grade 2/grade 3
- mathk, math1, math2, math3 = mathematics test scores in kindergarten/grade 1/grade 2/grade 3
- lunchk, lunch1, lunch2, lunch3 = student eligible for free/reduced lunch in kindergarten/grade 1/grade 2/grade 3 with 1/non-free, 2/free
- schoolk, school1, school2, school3 = school type student attended in kindergarten/grade 1/grade 2/grade 3 with 1/inner-city, 2/suburban, 3/rural, 4/urban
- degreek, degree1, degree2, degree3 = teacher’s highest degree earned in kindergarten/grade 1/grade 2/grade 3 with 1/bachelor, 2/master, 3/specialist, 4/phd
- ladderk, ladder1, ladder2, ladder3 = teacher’s career ladder status in kindergarten/grade 1/grade 2/grade 3 with 1/level 1, 2/level 2, 3/level 3, 4/apprentice, 5/probation, 6/pending
- experiencek, experience1, experience2, experience3 = teacher’s years of teaching experience in kindergarten/grade 1/grade 2/grade 3
- tethnicityk, tethnicity1, tethnicity2, tethnicity3 = teacher’s ethnicity in kindergarten/grade 1/grade 2/grade 3 with 1/caucasian, 2/african-american
Comparing students who were in a small classroom in all four grades to students in a regular classroom in all four grades,
- Did reading scores improve between grades k and 3?
- What about mathematics scores?
Comparing students who were in a small classroom in all four grades to students in a regular classroom (with our without an aide) in all four grades,
- Was there a difference in reading scores in the fourth grade?
The data for this analysis come from the University of Michigan Panel Study of Income Dynamics (hereafter PSID) for the year 1975 (interview year 1976). Although this year was atypical of most of the 1970’s, only in 1976 did PSID directly interview the wives in the households. During all other years the head of the household’s interview supplied information about the wife’s labor market experiences during the previous year. One suspects that the own reporting is more accurate, and it is for this reason that many recent studies of married women’s labor supply have used these data.
This sample consists of 753 married white women between the ages of 30 and 60 in 1975, with 428 working at some time during the year. This sample size is smaller than most used in the studies reported in Table I. The dependent variable is hours = the wife’s annual hours of work in 1975, and measured as the product of the number of weeks the wife worked for money in 1975 and the average number of hours of work per week during the weeks she worked. The measure of the wage rate is the average hourly earnings, defined by dividing the total labor income of the wife in 1975 by the above measure of her hours of work.
- inlf = labor force participation in 1975 = 1
- hours = wife’s hours of work in 1975
- kidslt6 = number of children less than 6 years old in household
- kidsge6 = number of children between ages 6 and 18 in household
- age = wife’s age
- educ = wife’s educational attainment, in years
- wage = wife’s average hourly earnings, in 1975 dollars
- repwage = wife’s wage reported at the time of the 1976 interview (not= 1975 estimated wage)
- hushrs = husband’s hours worked in 1975
- husage = husband’s age
- huseduc = husband’s educational attainment, in years
- huswage = husband’s wage, in 1975 dollars
- faminc = family income, in 1975 dollars
- mtr = Federal marginal tax rate facing women
- mothereduc = wife’s mother’s educational attainment, in years
- fathereduc = wife’s father’s educational attainment, in years
- unemprate = unemployment rate in county of residence, in percentage points
- city = lives in large city (SMSA) = 1
- exper = actual years of wife’s previous labor market experience
- nwifeinc = \(faminc - (wage \times hours)\) in thousand dollars
- lwage = log(wage)
- expersq = \(exper^2\)
- Did the wife’s annual hours of work differ according to whether the family lived in a city or not?
- Create a new variable \(= 1\) if the family had any children under 6 years of age and \(= 0\) otherwise. Then test whether the wife’s annual hours of work were greater for families with no children in this age bracket versus at least one child in this age bracket.
The following data-set contains information on 2.725 individuals’ criminal arrest histories. The variables include
- narr86 = number of times arrested, 1986
- nfarr86 = number of felony arrests, 1986
- nparr86 = number property crime arrests, 1986
- pcnv = proportion of prior convictions
- avgsen = average sentence length, months
- tottime = time in prison since 18 (months)
- ptime86 = months in prison during 1986
- qemp86 = number of quarters employed, 1986
- inc86 = legal income, 1986, in hundreds of dollars
- durat = recent unemployment duration
- black = 1 if Black
- hispan = 1 if Hispanic
- pcnvsq = pcnv \(^2\)
- pt86sq = ptime86 \(^2\)
- inc86sq = inc86 \(^2\)
- Was the average sentence length greater for Black versus non-Black individuals?
- What about for Hispanic versus non-Hispanic individuals?
It would be remiss of us not to recognize the existence of a very passionate and vigorous Null Hypothesis Significance Testing (NHST) controversy. While the details of the controversy are too advanced for our present purposes, here are some articles you should read: The Truth Wears Off: Is there something wrong with the scientific method? and Do We Really Need the S-word? ↩︎
Have a language expert improve your writing
Run a free plagiarism check in 10 minutes, generate accurate citations for free.
- Knowledge Base
Hypothesis Testing | A Step-by-Step Guide with Easy Examples
Published on November 8, 2019 by Rebecca Bevans . Revised on June 22, 2023.
Hypothesis testing is a formal procedure for investigating our ideas about the world using statistics . It is most often used by scientists to test specific predictions, called hypotheses, that arise from theories.
There are 5 main steps in hypothesis testing:
- State your research hypothesis as a null hypothesis and alternate hypothesis (H o ) and (H a or H 1 ).
- Collect data in a way designed to test the hypothesis.
- Perform an appropriate statistical test .
- Decide whether to reject or fail to reject your null hypothesis.
- Present the findings in your results and discussion section.
Though the specific details might vary, the procedure you will use when testing a hypothesis will always follow some version of these steps.
Table of contents
Step 1: state your null and alternate hypothesis, step 2: collect data, step 3: perform a statistical test, step 4: decide whether to reject or fail to reject your null hypothesis, step 5: present your findings, other interesting articles, frequently asked questions about hypothesis testing.
After developing your initial research hypothesis (the prediction that you want to investigate), it is important to restate it as a null (H o ) and alternate (H a ) hypothesis so that you can test it mathematically.
The alternate hypothesis is usually your initial hypothesis that predicts a relationship between variables. The null hypothesis is a prediction of no relationship between the variables you are interested in.
- H 0 : Men are, on average, not taller than women. H a : Men are, on average, taller than women.
Prevent plagiarism. Run a free check.
For a statistical test to be valid , it is important to perform sampling and collect data in a way that is designed to test your hypothesis. If your data are not representative, then you cannot make statistical inferences about the population you are interested in.
There are a variety of statistical tests available, but they are all based on the comparison of within-group variance (how spread out the data is within a category) versus between-group variance (how different the categories are from one another).
If the between-group variance is large enough that there is little or no overlap between groups, then your statistical test will reflect that by showing a low p -value . This means it is unlikely that the differences between these groups came about by chance.
Alternatively, if there is high within-group variance and low between-group variance, then your statistical test will reflect that with a high p -value. This means it is likely that any difference you measure between groups is due to chance.
Your choice of statistical test will be based on the type of variables and the level of measurement of your collected data .
- an estimate of the difference in average height between the two groups.
- a p -value showing how likely you are to see this difference if the null hypothesis of no difference is true.
Based on the outcome of your statistical test, you will have to decide whether to reject or fail to reject your null hypothesis.
In most cases you will use the p -value generated by your statistical test to guide your decision. And in most cases, your predetermined level of significance for rejecting the null hypothesis will be 0.05 – that is, when there is a less than 5% chance that you would see these results if the null hypothesis were true.
In some cases, researchers choose a more conservative level of significance, such as 0.01 (1%). This minimizes the risk of incorrectly rejecting the null hypothesis ( Type I error ).
The results of hypothesis testing will be presented in the results and discussion sections of your research paper , dissertation or thesis .
In the results section you should give a brief summary of the data and a summary of the results of your statistical test (for example, the estimated difference between group means and associated p -value). In the discussion , you can discuss whether your initial hypothesis was supported by your results or not.
In the formal language of hypothesis testing, we talk about rejecting or failing to reject the null hypothesis. You will probably be asked to do this in your statistics assignments.
However, when presenting research results in academic papers we rarely talk this way. Instead, we go back to our alternate hypothesis (in this case, the hypothesis that men are on average taller than women) and state whether the result of our test did or did not support the alternate hypothesis.
If your null hypothesis was rejected, this result is interpreted as “supported the alternate hypothesis.”
These are superficial differences; you can see that they mean the same thing.
You might notice that we don’t say that we reject or fail to reject the alternate hypothesis . This is because hypothesis testing is not designed to prove or disprove anything. It is only designed to test whether a pattern we measure could have arisen spuriously, or by chance.
If we reject the null hypothesis based on our research (i.e., we find that it is unlikely that the pattern arose by chance), then we can say our test lends support to our hypothesis . But if the pattern does not pass our decision rule, meaning that it could have arisen by chance, then we say the test is inconsistent with our hypothesis .
If you want to know more about statistics , methodology , or research bias , make sure to check out some of our other articles with explanations and examples.
- Normal distribution
- Descriptive statistics
- Measures of central tendency
- Correlation coefficient
Methodology
- Cluster sampling
- Stratified sampling
- Types of interviews
- Cohort study
- Thematic analysis
Research bias
- Implicit bias
- Cognitive bias
- Survivorship bias
- Availability heuristic
- Nonresponse bias
- Regression to the mean
Hypothesis testing is a formal procedure for investigating our ideas about the world using statistics. It is used by scientists to test specific predictions, called hypotheses , by calculating how likely it is that a pattern or relationship between variables could have arisen by chance.
A hypothesis states your predictions about what your research will find. It is a tentative answer to your research question that has not yet been tested. For some research projects, you might have to write several hypotheses that address different aspects of your research question.
A hypothesis is not just a guess — it should be based on existing theories and knowledge. It also has to be testable, which means you can support or refute it through scientific research methods (such as experiments, observations and statistical analysis of data).
Null and alternative hypotheses are used in statistical hypothesis testing . The null hypothesis of a test always predicts no effect or no relationship between variables, while the alternative hypothesis states your research prediction of an effect or relationship.
Cite this Scribbr article
If you want to cite this source, you can copy and paste the citation or click the “Cite this Scribbr article” button to automatically add the citation to our free Citation Generator.
Bevans, R. (2023, June 22). Hypothesis Testing | A Step-by-Step Guide with Easy Examples. Scribbr. Retrieved April 15, 2024, from https://www.scribbr.com/statistics/hypothesis-testing/
Is this article helpful?
Rebecca Bevans
Other students also liked, choosing the right statistical test | types & examples, understanding p values | definition and examples, what is your plagiarism score.

Hypotheses Testing
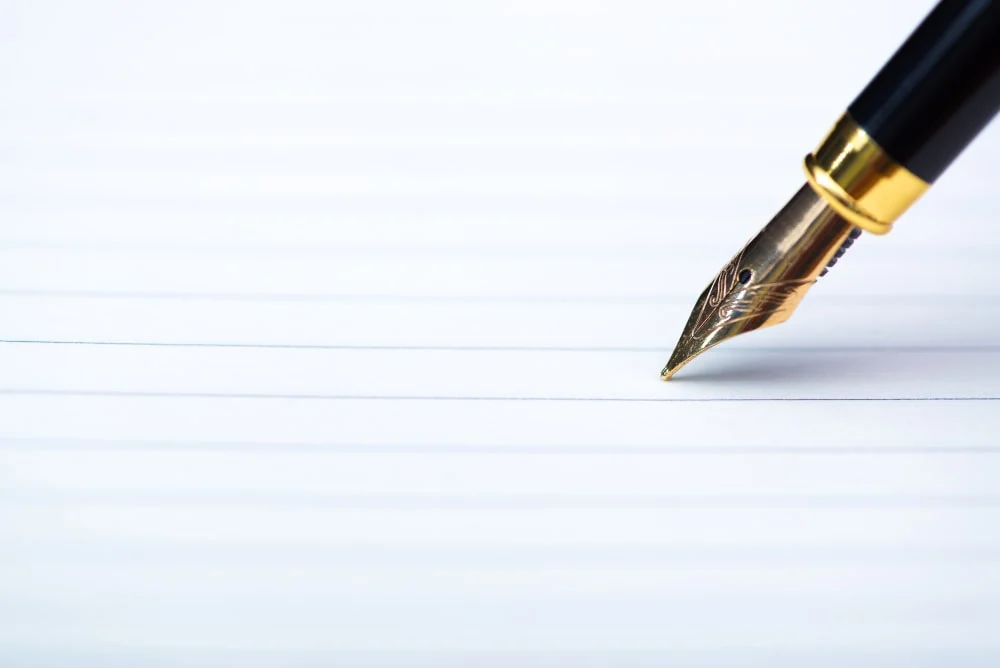
A Hypothesis A hypothesis is a tentative explanation that accounts for a set of facts and can be tested by further investigation. It may be defined as follows:
- A hypothesis may be precisely defined as a tentative proposition suggested as a solution to a problem or as an explanation of some phenomenon (Ary, Jacobs, and Razavieh, 1984).
- A hypothesis is a conjectural statement of the relation between two or more variables (Kerlinger, 1956). A hypothesis is a formal statement that presents the expected relationship between an independent and dependent variable. (Creswell, 1994).
- Hypothesis relates theory to observation and observation to theory. (Ary, Jacobs, and Razavieh, 1984).
- Hypotheses are relational propositions. (Kerlinger, 1956).
- A hypothesis is a tentative explanation that accounts for a set of facts and can be tested by further investigation.
- A hypothesis is a logical supposition, a reasonable guess, and an educated conjecture. It provides a tentative explanation for a phenomenon under investigation (Leedy and Ormrod, 2001).
Importance of hypothesis A hypothesis is important because it guides the research. An investigator may refer to the hypothesis to direct his or her thought process toward the solution of the research problem or sub-problems. The hypothesis helps an investigator to collect the right kinds of data needed for the investigation. Hypotheses are also important because they help an investigator locate information needed to resolve the research problem or sub-problems (Leedy and Ormrod, 2001)
Types of hypothesis A hypothesis (plural hypotheses) is a precise, testable statement of what the researchers predict will be the outcome of the study. This usually involves proposing a possible relationship between two variables: the independent variable (what the researcher changes) and the dependent variable (what the research measures). In research, there is a convention that the hypothesis is written in two forms, the null hypothesis, and the alternative hypothesis (called the experimental hypothesis when the method of investigation is an experiment). The hypotheses can be expressed in the following ways:
Null Hypothesis The null hypothesis states that there is no relationship between the two variables being studied (one variable does not affect the other). It states results are due to chance and are not significant in terms of supporting the idea being investigated. A null hypothesis also bears the features:
- Ho states the opposite of what the experimenter would expect or predict.
- The final conclusion of the investigator will either retain a null hypothesis or reject a null hypothesis in favor of an alternative hypothesis.
- Not rejecting Ho does not really mean that Ho is true. There might not be enough evidence against Ho.
- States the assumption (numerical) to be tested
- Begin with the assumption that the null hypothesis is TRUE
- Always contains the ‘=’ sign
Alternative Hypothesis The alternative hypothesis states that there is a relationship between the two variables being studied (one variable has an effect on the other). It states that the results are not due to chance and that they are significant in terms of supporting the theory being investigated. An alternative hypothesis is a statement that suggests a potential outcome that the researcher may expect (H1 or HA). An alternative hypothesis encompasses the followings:
- Is it the opposite of the null hypothesis?
- Challenges to the status quo
- Never contains just the ‘=’ sign
- Is generally the hypothesis that is believed to be true by the researcher?
- Comes from prior literature or studies.
- It is established only when a null hypothesis is rejected.
- Often an alternative Hypothesis is the desired conclusion of the investigator.
The two types of alternative hypotheses are directional hypotheses and non-directional Hypotheses. A non-directional alternative hypothesis states that the null hypothesis is wrong. A non-directional alternative hypothesis does not predict whether the parameter of interest is larger or smaller than the reference value specified in the null hypothesis. Whereas a directional alternative hypothesis states that the null hypothesis is wrong, and also specifies whether the true value of the parameter is greater than or less than the reference value specified in the null hypothesis. The advantage of using a directional hypothesis is increased power to detect the specific effect you are interested in. The disadvantage is that there is no power to detect an effect in the opposite direction.
Derivation of Hypothesis Inductive: The researcher notes the observations of behavior, thinks about the problem, turns to literature for clues, makes additional observations, derives probable relationships, and hypothesizes an explanation. A hypothesis is then tested. It may be limited in scope and can lead to unconnected findings, which could explain little about the research.
Deductive: The researcher begins by selecting a theory and derives a hypothesis leading to deductions derived through symbolic logic or mathematics. These deductions are then presented in the form of statements accompanied by an argument or a rationale for the particular proposition.
Hypothesis testing The following 5 steps are followed when testing hypotheses.
1. Specify H0 and HA – the null and alternative hypotheses (a) H0: E(X) = 10 (b) H0: E(X) = 10 (c) H0: E(X) = 10 HA: E(X) <> 10 HA: E(X) < 10 HA: E(X) > 10
Note that, in example (a), the alternative values for E(X) can be either above or below the value specified in H0. Hence, a two-tailed test is called for – that is, values for HA lie in both the upper and lower halves of the normal distribution. In example (b), the alternative values are below those specified in H0, while in example (c) the alternative values are above those specified in H0. Hence, for (b) and (c), a one-tailed test is called for.
2. Determine the appropriate test statistic A statistic to test the hypothesis. A ‘test statistic’ should follow a probability distribution (Z, t, χ2 or F) or there should be a threshold value based on which H0 is ejected or accepted.
3. Determine the critical region The region in which if the ‘test statistic’ falls, H0 is rejected. The critical region should be in the tail area. P-value- the tail probability; usually, the probability that the ‘test statistic’ is greater (less) than its calculated value. If the p-value is less than a given ‘level of significance’ (say 0.05), H0 is rejected.
- When p value > .10 → the observed difference is “not significant”
- When p value ≤ .10 → the observed difference is “marginally significant”
- When p value ≤ .05 → the observed difference is “significant”
- When p value ≤ .01 → the observed difference is “highly significant”

4. Compute the value of the test statistic Say, a value of z calculated on the basis of a sample result is called a computed z-value, and is denoted by the symbols zc or simply z.
5. Make a decision If the calculated value of the test statistic falls in the critical region, then H0is rejected. When the calculated value lies in the acceptance region, H0is not rejected.
Decision problem How do we choose between H0and HA? The standard procedure is to assume H0is true – just as we presume innocent until proven guilty. Using probability theory, we try to determine whether there is sufficient evidence to declare H0false. We reject only when the chance is small that H0is true. Since our decisions are based on probability rather than certainty, we can make errors.
Type I Error: A type I error occurs when the null hypothesis (H0) is wrongly rejected. For example, A type I error would occur if we concluded that the two drugs produced different effects when in fact there was no difference between them.
Type II Error: A type II error occurs when the null hypothesis H0, is not rejected when it is in fact false. For example, A type II error would occur if it were concluded that the two drugs produced the same effect, that is, there is no difference between the two drugs on average, when in fact they produced different ones.
In conclusion, we need the null hypothesis to determine if there is a difference between the groups being tested or not. Without it, we would be swamped with possibilities making it almost impossible to test.
| Journals | Instructions | Submission |
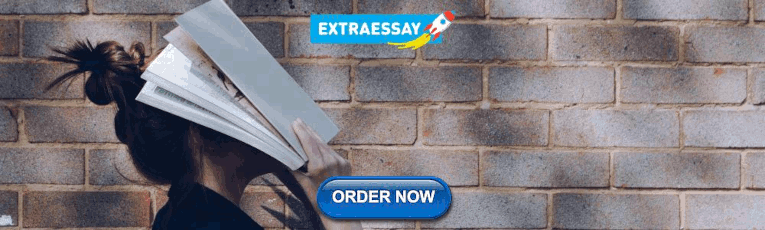
IMAGES
VIDEO
COMMENTS
A hypothesis is an educated guess or even a testable prediction validated through research. It aims to analyze the gathered evidence and facts to define a relationship between variables and put forth a logical explanation behind the nature of events.
So in hypothetical reasoning what we're doing is making a leap from the evidence we have available to the rule or principle or theory which explains that evidence. The hypothesis is the link between the two. We have some finite evidence available to us, and we hypothesize an explanation.
A hypothesis is a guess about what's going to happen. In research, the hypothesis is what you the researcher expects the outcome of an experiment, a study, a test, or a program to be. ... It can also be like an explanation for a phenomenon, or a logical prediction of a possible causal correlation among multiple factors. In science—or ...
Second, a good hypothesis must be logical. As described above, hypotheses are more than just a random guess. Hypotheses should be informed by previous theories or observations and logical reasoning. Typically, we begin with a broad and general theory and use deductive reasoning to generate a more specific hypothesis to test based on that theory.
Merriam Webster defines a hypothesis as "an assumption or concession made for the sake of argument.". In other words, a hypothesis is an educated guess. Scientists make a reasonable assumption--or a hypothesis--then design an experiment to test whether it's true or not.
Second, a good hypothesis must be logical. As described above, hypotheses are more than just a random guess. Hypotheses should be informed by previous theories or observations and logical reasoning. Typically, we begin with a broad and general theory and use deductive reasoning to generate a more specific hypothesis to test based on that theory.
Second, a good hypothesis must be logical. As described above, hypotheses are more than just a random guess. Hypotheses should be informed by previous theories or observations and logical reasoning. Typically, we begin with a broad and general theory and use deductive reasoning to
It essentially represents an educated guess based on observations, predicting the results of your experiment or research. Examples of Hypotheses: 1. If I water plants daily, they will grow faster. ... Logical Hypothesis: A logical hypothesis suggests a relationship between variables based on reasoning or deduction, without concrete evidence. ...
In science, a hypothesis is part of the scientific method. It is a prediction or explanation that is tested by an experiment. Observations and experiments may disprove a scientific hypothesis, but can never entirely prove one. In the study of logic, a hypothesis is an if-then proposition, typically written in the form, "If X, then Y ."
What is a hypothesis? A hypothesis is an educated guess or conclusion based on something you have witnessed. A complete hypothesis considers multiple aspects of observation and calls for facts, direct testing and evidence to either prove or disprove your conclusion. ... Logical hypothesis A logical hypothesis states a relationship between ...
Types of hypotheses include simple, complex, directional, non-directional, associative and causal, empirical, and statistical hypotheses. Each type serves a specific purpose and is used based on the nature of the research question or problem. The research hypothesis is a logical supposition and an educated prediction of the assumed relationship ...
There are 13 different types of hypothesis. These include simple, complex, null, alternative, composite, directional, non-directional, logical, empirical, statistical, associative, exact, and inexact. A hypothesis can be categorized into one or more of these types. However, some are mutually exclusive and opposites.
A hypothesis is regarded as a provisional supposition. It means a hypothesis is a suggestion or a possible explanation of a logical inquiry. It is a tentative solution and not a real solution to a logical inquiry until tested, verified and proved to be true. Thus, a hypothesis is subject to revision and rejection.
Testable: An idea (hypothesis) should be made so it can be tested and proven true through doing experiments or watching. It should show a clear connection between things. Specific: It needs to be easy and on target, talking about a certain part or connection between things in a study. Falsifiable: A good guess should be able to show it's wrong. This means there must be a chance for proof or ...
scientific hypothesis, an idea that proposes a tentative explanation about a phenomenon or a narrow set of phenomena observed in the natural world.The two primary features of a scientific hypothesis are falsifiability and testability, which are reflected in an "If…then" statement summarizing the idea and in the ability to be supported or refuted through observation and experimentation.
The hypothesis: An educated guess. Susan B Shipley, RN The hypothesis: An educated guess The purpose of research or scientific in- quiry is to determine how variables are related. We do not test variables; we test the relationship between them. A re- searcher’s prediction of how the vari- ables relate to each other is called the hypothesis.
Since hypothesis is an intelligent guess or a tentative solution hence the formulation of hypothesis becomes imperative as soon as a research question is formulated.iii Hypothesis is merely a tentative assumption made in order to draw and test its logical or empirical consequences. It is a tentative, testable statement.
Hypotheses come in pairs, one the null hypothesis and the other the alternative hypothesis. The null hypothesis, often denoted as \(H_{0}\), is the assumption believed to be true while the alternative hypothesis, often denoted as \(H_{a}\) or \(H_{1}\) is the statement believed to be true if \(H_{0}\) is rejected by the statistical test. Some ...
Present the findings in your results and discussion section. Though the specific details might vary, the procedure you will use when testing a hypothesis will always follow some version of these steps. Table of contents. Step 1: State your null and alternate hypothesis. Step 2: Collect data. Step 3: Perform a statistical test.
A hypothesis is a tentative explanation that accounts for a set of facts and can be tested by further investigation. A hypothesis is a logical supposition, a reasonable guess, and an educated conjecture. It provides a tentative explanation for a phenomenon under investigation (Leedy and Ormrod, 2001). Importance of hypothesis
It is a hypothesis that is based on incomplete or uncertain information. Conjecture is often used in situations where there is no concrete evidence to support a claim or theory. It is a form of educated guesswork that is based on logical reasoning and intuition. Define Guess. A guess is an estimate or prediction made without any supporting ...
The difference between hypothesis and prediction is explained through explanations & examples. Use our simple table for hypothesis vs prediction reference. Dictionary ... You could call a hypothesis a testable guess. Hypothesis Examples. Understanding a hypothesis can be hard.
A logical supposition, a reasonable guess, an educated conjecture. Hypothesis. Provides a tentative explanation for a phenomenon under investigation. ... Depends entirely on the researcher's hypothesis, assumptions, and logical reasoning processes. Data Interpretation. Essential to the research process.