Lesson 6: The \(2^k\) Factorial Design
Introduction.
The \(2^k\) designs are a major set of building blocks for many experimental designs. These designs are usually referred to as screening designs. The \(2^k\) refers to designs with k factors where each factor has just two levels. These designs are created to explore a large number of factors, with each factor having the minimal number of levels, just two. By screening we are referring to the process of screening a large number of factors that might be important in your experiment, with the goal of selecting those important for the response that you're measuring. We will see that k can get quite large. So far we have been looking at experiments that have one, two or three factors, maybe a blocking factor and one or two treatment factors, but when using screening designs k can be as large as 8, 10 or 12. For those of you familiar with chemical or laboratory processes, it would not be hard to come up with a long list of factors that would affect your experiment. In this context we need to decide which factors are important.
In these designs we will refer to the levels as high and low, +1 and -1, to denote the high and the low level of each factor. In most cases the levels are quantitative, although they don't have to be. Sometimes they are qualitative, such as gender, or two types of variety, brand or process. In these cases the +1 and -1 are simply used as labels.
- The idea of 2-level Factorial Designs as one of the most important screening designs
- Defining a “contrast” which is an important concept and how to derive Effects and Sum of Squares using the Contrasts
- Process of analyzing Unreplicated or Single replicated factorial designs, and
- How to use Transformation as a tool in dealing with inadequacy of either variance homogeneity or normality of the data as major hypothetical assumptions.
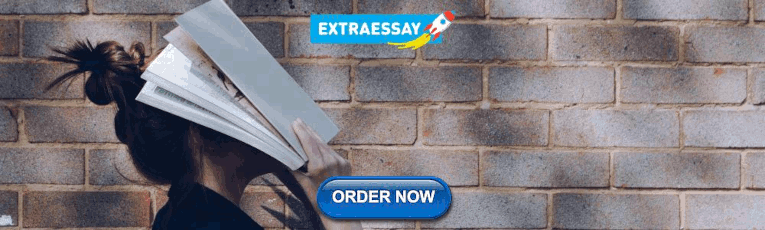
6.1 - The Simplest Case
The simplest case is \(2^k\) where \(k = 2\). We will define a new notation which is known as Yates notation. We will refer to our factors using the letters A, B, C, D, etc. as arbitrary labels of the factors. In the chemical process case, A is the concentration of the reactant and B is the amount of catalyst, both of which are quantitative. The yield of the process is our response variable.
Since there are two levels of each of two factors, \(2^k\) equals four. Therefore, there are four treatment combinations and the data are given below:
You can see that we have 3 observations at each of \(4 = 2^k\) combinations for \(k = 2\). So we have \(n = 3\) replicates.
The table above gives the data with the factors coded for each of the four combinations and below is a plot of the region of experimentation in two dimensions for this case.
The Yates notation used for denoting the factor combinations is as follows:
We use "(1)" to denote that both factors are at the low level, "a" for when A is at its high level and B is at its low level, "b" for when B is at its high level and A is at its low level, and "ab" when both A and B factors are at their high level.
The use of this Yates notation indicates the high level of any factor simply by using the small letter of that level factor. This notation actually is used for two purposes. One is to denote the total sum of the observations at that level. In the case below \(b = 60\) is the sum of the three observations at the level b .
This shortcut notation, using the small letters, shows which level for each of our k factors we are at just by its presence or absence.
We will also connect this to our previous notation for the two-factor treatment design:
\(Y_{ijk} = \mu + \alpha_{i} + \beta_{j} + (\beta \beta)_{ij} + e_{ijk}\)
What is the primary goal of these screening experiments?
The goal is to decide which of these factors is important. After determining which factors are important, then we will typically plan for a secondary experiment where the goal is to decide what level of the factors gives us the optimal response. Thus the screening \(2^k\) experiment is the first stage, generally, of an experimental sequence. In the second stage, one is looking for a response surface or an experiment to find the optimal level of the important factors.
Estimation of Factors Effects (in the Yates tradition)
The definition of an effect in the \(2^k\) context is the difference in the means between the high and the low level of a factor. From this notation, A is the difference between the averages of the observations at the high level of A minus the average of the observations at the low level of A.
Therefore, \(A=\bar{y}_{A^+}-\bar{y}_{A^-}\), in the example above:
\(A = 190/6 - 140/6 = 50/6 = 8.33\)
Similarly, \(B=\bar{y}_{B^+}-\bar{y}_{B^-}\), is similar only looking in the other direction. In our example:
\(B = 150/6 - 180/6 = 25 - 30 = -5\)
and finally, \(AB=\dfrac{ab+(1)}{2n}-\dfrac{a+b}{2n}\)
\(AB = [(90 + 80)/6 - (100 + 60)/6] = 10/6 = 1.67\)
Therefore in the Yates notation, we define an effect as the difference in the means between the high and the low levels of a factor whereas in previous models we defined an effect as the coefficients of the model, which are the differences between the marginal mean and the overall mean. To restate this, in terms of A, the A effect is the difference between the means at the high levels of A versus the low levels of A, whereas the coefficient, \(\alpha_i\), in the model is the difference between the marginal mean and the overall mean. So the Yates "effect" is twice the size of the estimated coefficient α i in the model, which is also usually called the effect of factor A.
The confusion is all in the notation used in the definition.
Let's look at another example in order to reinforce your understanding of the notation for these types of designs. Here is an example in three dimensions, with factors A, B and C. Below is a figure of the factors and levels as well as the table representing this experimental space.
In the table you can see the eight points coded by the factor levels +1 and -1. This example has two replicates so n = 2. Notice that the Yates notation is included as the total of the two replicates.
One nice feature of the Yates notation is that every column has an equal number of pluses and minuses so these columns are contrasts of the observations. For instance, take a look at the A column. This column has four pluses and four minuses, therefore, the A effect is a contrast.
This is the principle that gives us all sorts of useful characterizations in these \(2^k\) designs.
In the example above the A, B and C each are defined by a contrast of the data observation totals. Therefore you can define the contrast AB as the product of the A and B contrasts, the contrast AC by the product of the A and C contrasts, and so forth.
Therefore all the two-way and three-way interaction effects are defined by these contrasts. The product of any two gives you the other contrast in that matrix.
From these contrasts we can define the effect of A, B, and C, using these coefficients. The general form of an effect for k factors is:
\(\text{Effect} = (1/2^{(k-1)}n)\) [contrast of the totals]
The sum of the products of the contrast coefficients times the totals will give us an estimate of the effects.
We can also write the variance of the effect using the general form used previously. This would be:
\(\begin{eqnarray} Variance(Effect)&=&[1/(2^{(k-1)}n)^2] V(contrast),or \nonumber\\ &=&[1/(2^{(k-1)}n)^2] 2^k n \sigma^2 \nonumber\\ &=&\sigma^2 / 2^{(k-2)}n \nonumber \end{eqnarray}\)
Also, we can write the sum of squares for the effects which looks like:
\(SS(\text{effect}) = (\text{contrast})^2 / 2^{k}n\)
To summarize what we have learned in this lesson thus far, we can write a contrast of the totals which defines an effect, we can estimate the variance for this effect and we can write the sum of squares for an effect. We can do this very simply using Yates notation which historically has been the value of using this notation.
6.2 - Estimated Effects and the Sum of Squares from the Contrasts
How can we apply what we learned in the preceding section?
In general for \(2^k\) factorials the effect of each factor and interaction is:
We also defined the variance as follows:
\(\text{Variance(Effect)} = \sigma^2 / 2^{(k-2)}n\)
The true but unknown residual variance \(\sigma^2\), which is also called the within-cell variance, can be estimated by the MSE.
If we want to test an effect, for instance, say A = 0, then we can construct a t -test which is the effect over the square root of the estimated variance of the effect as follows:
\(t^{\ast}=\dfrac{Effect}{\sqrt{\frac{MSE}{n2^{k-2}}}} \sim t(2^k (n-1))\)
where ~ means that it has a t distribution with \(2^{k}(n-1)\) degrees of freedom.
Finally, here is the equation for the sum of squares due to an effect to complete the story here:
\(\text{SS(Effect)} = \text{(contrast of totals)}^{2} / 2^{k}n\)
Where does all of this come from? Each effect in a \(2^k\) model has one degree of freedom. In the simplest case, we have two main effects and one interaction. They each have 1 degree of freedom. So the t statistic is the ratio of the effect over its estimated standard error (standard deviation of the effect). You will recall that if you have a t statistic with \(\nu\) degrees of freedom and square it, you get an F distribution with one and \(\nu\) degrees of freedom.
\(t^2(v)=F(1,v)\)
We can use this fact to confirm the formulas just developed. We see that the
\((t^{\ast}(\nu))^2=\dfrac{(Effect)^2}{MSE/n2^{k-2}}\)
and from the definition of an F-test, when the numerator has 1 degree of freedom:
\(F(1,\nu)=\dfrac{SS(Effect)/1}{MSE}=\dfrac{(contrast)^2}{2^kn(MSE)}\)
But from the definition of an Effect, we can write \(\text{(Effect)}^2 = \text{(contrast)}^2 / (n2^{k-1})^{2}\) and thus \(F(1, \nu) = (t*(\nu))^2\) which you can show by some algebra or by calculating an example.
Hint: Multiply \(F(1, \nu)\) by \((2^{(k-1)}n)^{2} / (2^{(k-1)}n)^{2}\) and simplify.
Once you have these contrasts, you can easily calculate the effect, you can calculate the estimated variance of the effect and the sum of squares due to the effect as well.
Creating a Factorial Design in Minitab
Let's use Minitab to help us create a factorial design and then add data so that we can analyze it. The data come from Figure 6.1.
In Minitab we use the software under Stat > Design of Experiments to create our full factorial design. We will come back to this command another time to look at fractional factorial and other types of factorial designs.
In the example that was shown above, we did not randomize the runs but kept them in standard order for the purpose of seeing more clearly the order of the runs. In practice, you would want to randomize the order of run when you are designing the experiment.
Once we have created a factorial design within the Minitab worksheet we then need to add the response data so that the design can be analyzed. These response data, Yield, are the individual observations, not the totals. So, we again go to the Stat > DOE > Factorial Menu where we will analyze the data set from the factorial design.
We began with the full model with all the terms included, both the main effects and all of the interactions. From here we were able to determine which effects were significant and should remain in the model and which effects were not significant and can be removed to form a simpler reduced model.
A Second Example - The Plasma Etch Experiment
Similar to the previous example, in this second industrial process example we have three factors, A equals Gap, B = Flow, C = Power and our response y = Etch Rate. ( The data are from Table 6-4 in the text.) Once again in Minitab we will create a similar layout for a full factorial design for three factors with two replicates which gives us 16 observations. Next, we add the response data, Etch Rate, to this worksheet and analyze this data set. These are the results we get:
Factorial Fit: EtchRate versus A, B, C
Estimated effects and coefficients for etchrate (coded units), analysis of variance for etchrate (coded units).
The analysis of variance shows the individual effects and the coefficients, (which are half of the effects), along with the corresponding t -tests. Now we can see from these results that the A effect and C effect are highly significant. The B effect is not significant. In looking at the interactions, AB , is not significant, BC is not significant, and the ABC are not significant. However the other interaction, AC is significant.
This is a nice example to illustrate the purpose of a screening design. You want to test a number of factors to see which ones are important. So what have we learned here? Two of these factors are clearly important, A and C . But B appears not to be important either as a main effect or within any interaction. It simply looks like random noise. B was the rate of gas flow across the edging process and it does not seem to be an important factor in this process, at least for the levels of the factor used in the experiment.
The analysis of variance summary table results show us that the main effects overall are significant. That is because two of them, A and C , are highly significant. The two-way interactions overall are significant. That is because one of them is significant. So, just looking at this summary information wouldn't tell us what to do except that we could drop the 3-way interaction.
Now we can go back to Minitab and use the Analyze command under Design of Experiments and we can remove all the effects that were seemingly not important such as any term having to do with B in the model. In running this new reduced model we get:
Factorial Fit: EtchRate versus A, C
For this model, all three terms are significant.
6.3 - Unreplicated \(2^k\) Factorial Designs
These are \(2^k\) factorial designs with one observation at each corner of the "cube". An unreplicated \(2^k\) factorial design is also sometimes called a "single replicate" of the \(2^k\) experiment.
You would find these types of designs used where k is very large or the process, for instance, is very expensive or takes a long time to run. In these cases, for the purpose of saving time or money, we want to run a screening experiment with as few observations as possible. When we introduced this topic we wouldn't have dreamed of running an experiment with only one observation. As a matter of fact, the general rule of thumb is that you would have at least two replicates. This would be a minimum in order to get an estimate of variation - but when we are in a tight situation, we might not be able to afford this due to time or expense. We will look at an example with one observation per cell, no replications, and what we can do in this case.
Where are we going with this? We have first discussed factorial designs with replications, then factorial designs with one replication, now factorial designs with one observation per cell and no replications, which will lead us eventually to fractional factorial designs. This is where we are headed, a steady progression to designs with more and more factors, but fewer observations and less direct replication.
Unreplicated \(2^k\) Factorial Designs
Let's look at the situation where we have one observation per cell. We need to think about where the variation occurs within this design. These designs are very widely used. However, there are risks…if there is only one observation at each corner, there is a high chance of an unusual response observation spoiling the results. What about an outlier? There would be no way to check if this was the case and thus it could distort the results fairly significantly. You have to remind yourself that these are not the definitive experiments but simply just screening experiments to determine which factors are important.
In these experiments one really cannot model the "noise" or variability very well. These experiments cannot really test whether or not the assumptions are being met - again this is another shortcoming, or the price of the efficiency of these experiment designs.
Spacing of Factor Levels in the Unreplicated \(2^k\) Factorial Designs
When choosing the levels of your factors, we only have two options - low and high. You can pick your two levels low and high close together or you can pick them far apart. As most of you know from regression the further apart your two points are the less variance there is in the estimate of the slope. The variance of the slope of a regression line is inversely related the distance between the extreme points. You can reduce this variance by choosing your high and low levels far apart.
However, consider the case where the true underlying relationship is curved, i.e., more like this:
... and you picked your low and high level as illustrated above, then you would have missed capturing the true relationship. Your conclusion would probably be that there is no effect of that factor. You need to have some understanding of what your factor is to make a good judgment about where the levels should be. In the end, you want to make sure that you choose levels in the region of that factor where you are actually interested and are somewhat aware of a functional relationship between the factor and the response. This is a matter of knowing something about the context for your experiment.
How do we analyze our experiment when we have this type of situation? We must realize that the lack of replication causes potential problems in statistical testing:
- Replication provides an estimate of "pure error" (a better phrase is an internal estimate of error), and
- With no replication, fitting the full model results in zero degrees of freedom for error.
Potential solutions to this problem might be:
- Pooling high-order interactions to estimate error, (something we have done already in randomized block design),
- Normal probability plotting of effects (Cuthbert and Daniels, 1959), and/or
- Dropping entire factors from the model and other methods.
Example of an Unreplicated \(2^k\) Design
The following \(2^4\) factorial (Example 6-2 in the text) was used to investigate the effects of four factors on the filtration rate of a resin for a chemical process plant. The factors are A = temperature, B = pressure, C = mole ratio (concentration of chemical formaldehyde), D = stirring rate. This experiment was performed in a pilot plant.
Here is the dataset for this Resin Plant experiment. You will notice that all of these factors are quantitative.
Notice also the use of the Yates notation here that labels the treatment combinations where the high level for each factor is involved. If only A is high then that combination is labeled with the small letter a . In total, there are 16 combinations represented.
Here is a visual representation of this - it would be impossible to show this in a 4-dimensional cube but here are two cubes which attempt to do the same thing.
Sequential Procedure for Strategically Finding a Model
Let's use the dataset ( Ex6-2.csv ) and work at finding a model for this data with Minitab...
Video Tutorial
Even with just one observation per cell, by carefully looking at the results we can come to some understanding as to which factors are important. We do have to take into account that these actual p -values are not something that you would consider very reliable because you are fitting this sequence of models, i.e., fishing for the best model. We have optimized with several decisions that invalidates the actual p -value of the true probability that this could have occurred by chance.
This is one approach to assume that some interactions are not important and use this to test lower order terms of the model and finally come up with a model that is more focused. Based on this for this example that we have just looked at, we can conclude that following factors are important, A , C , D , (of the main effects) and AC and AD of the two-way interactions.
Now I suggest you try this procedure and then go back and check to see what the final model looks like. Here is what we get when we drop factor B and all the interactions that we decided were not important:
Factorial Fit: Rate versus A, C, D
Estimated effects and coefficients for rate (coded units), analysis of variance for rate (coded units).
The important factors didn't change much here. However, we have slightly higher degrees of freedom for error. But now what the design looks like, by having dropped B totally, is that we now have a \(2^3\) design with 2 replicates per cell. We have moved from a four-factor with one observation per cell, to a three-factor with two observations per cell.
So, we have looked at two strategies here. The first is to take a higher order interaction out of the model and use them as the estimate of error. Next, what we did at the end of the process is drop that factor entirely. If a particular factor in the screening experiment turns out to be not important either as a main effect or as part of any interaction we can remove it. This is the second strategy, and for instance in this example we took out factor B completely from the analysis.
Graphical Approaches to Finding a Model
Let's look at some more procedures - this time graphical approaches for us to look at our data in order to find the best model. This technique is really cool. Get a cup of coffee and click:
Normal Probability Plot for the Effects
Having included all the terms back into a full model we have shown how to produce a normal plot. Remember that all of these effects are 1 degree of freedom contrasts of the original data, each one of these is a linear combination of the original observations, which are normally distributed with constant variance. Then these 15 linear combinations or contrasts are also normally distributed with some variance. If we assume that none of these effects are significant, the null hypothesis for all of the terms in the model, then we simply have 15 normal random variables, and we will do a normal random variable plot for these. That is what we will ask Minitab to plot for us. We get a normal probability plot, not of the residuals, not of the original observations but of the effects. We have plotted these effects against what we would expect if they were normally distributed.
In the middle - the points in black, they are pretty much in a straight line - they are following a normal distribution. In other words, their expectation or percentile is proportionate to the size of the effect. The ones in red are like outliers and stand away from the ones in the middle and indicate that they are not just random noise but there must be an actual affect. Without making any assumptions about any of these terms this plot is an overall test of the hypothesis based on simply assuming all of the effects are normal. This is a very helpful - a good quick and dirty first screen - or assessment of what is going on in the data, and this corresponds exactly with what we found in our earlier screening procedures.
The Pareto Plot
Let's look at another plot - the Pareto plot. This is simply a plot that can quickly show you what is important. It looks at the size of the effects and plots the effect size on a horizontal axis ranked from largest to smallest effect.
Having dropped some of the terms out of the model, for instance the three and four way interactions, Minitab plots the remaining effects, but now it is the standardized effect. Basically it is plotting the t -value, the effect over its standard deviation and then plotting it in ranked order. It also displays the t critical point as a red line at alpha = 0.05.
Effects and Interaction Plots
Another Minitab command that we can take a look at is the subcommand called Factorial Plots. Here we can create plots for main effects telling Minitab which factors you want to plot. As well you can plot two-way interactions. Here is a plot of the interactions (which are more interesting to interpret), for the example we've been looking at:
You can see that the C and D interaction plot the lines are almost parallel and therefore do not indicate interaction effects that are significant. However the other two combinations, A and C and A and D, indicate that significant interaction exists. If you just looked at the main effects plot you would likely miss the interactions that are obvious here.
Checking Residuals Using Minitab's Four in One Plot
We have reduced the model to include only those terms that we found were important. Now we want to check the residuals in order to make sure that our assumptions are not out of line with any conclusions that we are making. We can ask Minitab to produce a Four in One residuals plot which, for this example, looks like this:
In visually checking the residuals we can see that we have nothing to complain about. There does not seem to be any great deviation in the normal probability plot of the residuals. There's nothing here that is very alarming and it seems acceptable. In looking at the residuals versus the fitted values plot in the upper right of this four in one plot - except for the lower values on the left where there are smaller residuals and you might be somewhat concerned here, the rest do not set off any alarms - but we will come back to this later.
Contour and Surface Plots
We may also want contour plots of all pairs of our numeric factors. These can be very helpful to understand and present the relationship between several factors on the response. The contour plots below for our example show the color coded average response over the region of interest. The effect of these changes in colors is to show the twist in the plane.
In the D*C plot area you can see that there is no curvature in the colored areas, hence no evidence of interaction. However, if you look at C*A display you can see that if C is low you get a dramatic change. If C is high it makes very little difference. In other words, the response due to A depends on the level of C. This is what the interaction means and it shows up nicely in this contour plot.
Finally, we can also ask Minitab to give us a surface plot. We will set this up the same way in Minitab and this time Minitab will show the plot in three dimensions, two variables at a time.
The surface plot shows us the same interaction effect in three dimensions in the twisted plane. This might be a bit easier to interpret. In addition you can ask Minitab to provide you with 3-D graphical tools that will allow you to grab these boxes and twist them around so that you can look at these boxes in space from different perspectives. Pretty cool! Give it a try. These procedures are all 'illustrated in the "Inspect" Flash movie at the beginning of this section.
Another Example - The Drilling Example 6.3
This is another fairly similar example to the one we just looked at. This drilling example (Example 6-3) is a \(2^4\) design - again, the same design that we looked at before. It is originally from C. Daniel, 1976. It has four factors, A = Drill load, B = Flow of a lubricant, C = Speed of drill, D = Type of mud, Y is the Response - the advance rate of the drill, (how fast can you drill an oil or gas well?).
We've used Minitab to create the factorial design and added the data from the experiment into the Minitab worksheet. First, we will produce a normal probability plot of the effects for this data with all terms included in a full model.
Here's what it looks like. It shows a strange pattern! No negative and all positive effects. All of the black dots are in fairly straight order except for perhaps the top two. If we look at these closer we can see that these are the BD and the BC terms, in addition to B, C, and D as our most important terms. Let's go back to Minitab and take out of our model the higher order interactions, (i.e. the 3-way and 4-way interactions), and produce this plot again (see below) just to see what we learn.
The normal probability plot of residuals looks okay. There is a gap in the histogram of other residuals but it doesn't seem to be a big problem.
When we look at the normal probability plot below, created after removing 3-way and 4-way interactions, we can see that now BD and BC are significant.
We can also see this in the statistical output of this model as shown below:
Factorial Fit: Rate versus A, B, C, D
Estimate effects and coefficients for rate (coded units).
The combined main effects are significant as seen in the combined summary table. And the individual terms, B, C, D, BC and BD, are all significant, just as shown on the normal probability plot above.
Now let's go one step farther and look at the completely reduced model. We'll go back into Minitab and get rid of everything except for the significant terms. Here is what you get:
What do you think?
Residuals versus the plot of the fitted values in the upper right-hand corner has now a very distinct pattern. It seems to be a classic as the response gets larger the residuals get more spread apart.
What does this suggest is needed? For those of you who have studied heteroscedastic variance patterns in regression models you should be thinking about possible transformations.
A transformation - the large values are more variable than smaller values. But why does this only show up now? Well, when we fit a full model it only has one observation per cell and there's no pure way to test for residuals. But when we fit a reduced model, now there is inherent replication and this pattern becomes apparent.
Take a look at the data set and you will find the square root and the log already added in order to analyze the same model using this transformed data. What do you find happens?
6.4 - Transformations
When you look at the graph of the residuals as shown below you can see that the variance is small at the low end and the variance is quite large on the right side producing a fanning effect. Consider the family of transformations that can be applied to the response \(y_{ij}\).
Transformations towards the bottom of the list are stronger in how they shrink large values more than they shrink small values that are represented on the plot. This pattern of the residuals is one clue to get you to be thinking about the type of transformations you would select.
The other consideration and thinking about transformations of the response \(y_{ij}\) is what it does to the relationship itself. Some of you will recall from other classes the Tukey one-degree-of-freedom test for interaction. This is a test for interaction where you have one observation per cell such as with a randomized complete block design. But with one observation per cell and two treatments our model would be :
\(Y_{ijk}= \mu+\alpha_{i}+\beta_{j}+(\alpha\beta)_{ij}+\epsilon_{ijk}\)
i = 1 ... a ,
j = 1 ... b , with
k = 1 ... 1, (only have one observation per cell)
There is no estimate of pure error so we cannot fit the old model. The model proposed by Tukey's has one new parameter (γ) gamma :
\(Y_{ij}= \mu+\alpha_{i}+\beta_{j}+\gamma\alpha_{i}\beta_{j}+\epsilon_{ij}\)
This single parameter, gamma, is the 1 degree of freedom term and so our error,\(\epsilon_{ij}\), has ( a -1)( b -1) -1 degrees of freedom. This model allows for just a single additional parameter which is based on a multiplicative effect on the two factors.
Now, when is this applicable?
Let's go back to the drill rate example ( Ex6-3.MTW | Ex6-3.csv ) where we saw the fanning effect in the plot of the residuals. In this example B, C and D were the three main effects and there were two interactions BD and BC. From Minitab we can reproduce the normal probability plot for the full model.
But let's first take a look at the residuals versus our main effects B, C and D.
All three of these residuals versus the main effects show same pattern, the large predicted values tend to have larger variation.
Next, what we really want to look at is the factorial plots for these three factors, B, C and D and the interactions among these, BD and BC.
What you see in the interaction plot above is a pattern that is non-parallel showing there is interaction present. But, from what you see in the residual graph what would you expect to see on this factor plot?
The tell-tale pattern that is useful here is an interaction that does not have crossing lines - a fanning effect - and it is exactly the same pattern that allows the Tukey model to fit. In both cases, it is a pattern of interaction that you can remove by transformation. If we select a transformation that will shrink the large values more than it does the small values and the overall result would be that we would see less of this fan effect in the residuals.
We can look at either the square root or log transformation. It turns out that the log transformation is the one that seems to fit the best. On a log scale it looks somewhat better - it might not be perfect but it is certainly better than what we had before.
Let's also look at the analysis of variance.
Factorial Fit: LOGT versus B, C, D
Estimated effects and coefficients for logt (coded units), analysis of variance for logt (coded units).
The overall main effects are still significant. But the two 2-way interactions effects combined are no longer significant, and individually, the interactions are not significant here either. So, the log transformation which improved the unequal variances pulled the higher responses down more than the lower values and therefore resulted in more of a parallel shape. What's good for variance is good for a simple model. Now we are in a position where we can drop the interactions and reduce this model to a main effects only model.
Now our residual plots are nearly homoscedastic for B, C and D. See below...
Serendipity - good things come in packages! When you pick the correct transformation, you sometimes achieve constant variance and a simpler model.
Many times you can find a transformation that will work for your data - giving you a simpler analysis but it doesn't always work.
Transformations are typically performed to:
- Stabilize variance - to achieve equal variance
- Improve normality - this is often violated because it is easy to have an outlier when variance is large which can be 'reined in' with a transformation
- Simplify the model
Sometimes transformations will solve a couple of these problems.
Is there always a transformation that can be applied to equalize variance? Not really ... there are two approaches to solving this question. First, we could use some non-parametric method. Although non-parametric methods have fewer assumptions about the distribution, you still have to worry about how you are measuring the center of the distribution. When you have a non-parametric situation you may have a different shaped distribution in different parts of the experiment. You have to be careful about using the mean in one case, and the media in another ... but that is one approach.
The other approach is a weighted analysis, where you weight the observations according to the inverse of their variance. There are situations where you have unequal variation for maybe a known reason or unknown reason, but if you have repeated observations and you can get weights, then you can do a weighted analysis.
It is this course author's experience many times you can find a transformation when you have this kind of pattern. Also, sometimes when you have unequal variance you just have a couple of bad outliers, especially when you only have one or a few observations per cell. In this case it is difficult to the distinguish whether you have a couple of outliers or the data is heteroscedastic - it is not always clear.
Empirical Selection of Lambda
Prior (theoretical) knowledge or experience can often suggest the form of a transformation. However, another method for the analytical selection of lambda for the exponent used in the transformation is the Box-Cox (1964). This method simultaneously estimates the model parameters and the transformation parameter lambda.
Box-Cox method is implemented in some statistical software applications.
Example 6.4
This example is a four-factor design in a manufacturing situation where injection molding is the focus. Injection molding is a very common application in the industry; a \(2^k\) design where you have many factors influencing the quality which is measured by how many defects are created by the process. Almost anything that you can think of which has been made out of plastic was created through the injection molding process.
See the example in ( Ex6-4.mwx | Ex6-4.csv )
In this example we have four factors again: A = temperature of the material, B = clamp time for drying, C = resin flow, and D = closing time of the press. What we are measuring as the response is number of defects. This is recorded as an index of quality in terms of percent. As you look through the data in Figure 6.29 (7th edition) you can see percent of defects as high as 15.5% or as low as 0.5%. Let's analyze the full model in Minitab.
The normal probability plot of the effects shows us that two of the factors A and C are both significant and none of the two-way interactions are significant.
What we want to do next is look at the residuals vs. variables A, B, C, D in a reduced model with just the main effects as none of the interactions seemed important.
For each factor, you see that the residuals are more dispersed (higher variance) to the right than to the left. Overall, however, the residuals do not look too bad and the normal plot also does not look too bad. When we look at the p -values we find that A and C are significant but B and D are not.
But there is something else that can be learned here. The point of this example is that although the B factor is not significant as it relates to the response, percentage of product defects - however if you are looking for a recommended setting for B you should use the low level for B. A and C, are significant and will reduce the number of defects. However, by choosing B at the low level you will produce a more homogeneous product, products with less variability. What is important in product manufacturing is not only reducing the number of defects but also producing products that are uniform. This is a secondary consideration that should be taken into account after the primary considerations related to the percent of product defects.
Have a language expert improve your writing
Run a free plagiarism check in 10 minutes, generate accurate citations for free.
- Knowledge Base
Methodology
- How to Write a Strong Hypothesis | Steps & Examples
How to Write a Strong Hypothesis | Steps & Examples
Published on May 6, 2022 by Shona McCombes . Revised on November 20, 2023.
A hypothesis is a statement that can be tested by scientific research. If you want to test a relationship between two or more variables, you need to write hypotheses before you start your experiment or data collection .
Example: Hypothesis
Daily apple consumption leads to fewer doctor’s visits.
Table of contents
What is a hypothesis, developing a hypothesis (with example), hypothesis examples, other interesting articles, frequently asked questions about writing hypotheses.
A hypothesis states your predictions about what your research will find. It is a tentative answer to your research question that has not yet been tested. For some research projects, you might have to write several hypotheses that address different aspects of your research question.
A hypothesis is not just a guess – it should be based on existing theories and knowledge. It also has to be testable, which means you can support or refute it through scientific research methods (such as experiments, observations and statistical analysis of data).
Variables in hypotheses
Hypotheses propose a relationship between two or more types of variables .
- An independent variable is something the researcher changes or controls.
- A dependent variable is something the researcher observes and measures.
If there are any control variables , extraneous variables , or confounding variables , be sure to jot those down as you go to minimize the chances that research bias will affect your results.
In this example, the independent variable is exposure to the sun – the assumed cause . The dependent variable is the level of happiness – the assumed effect .
Prevent plagiarism. Run a free check.
Step 1. ask a question.
Writing a hypothesis begins with a research question that you want to answer. The question should be focused, specific, and researchable within the constraints of your project.
Step 2. Do some preliminary research
Your initial answer to the question should be based on what is already known about the topic. Look for theories and previous studies to help you form educated assumptions about what your research will find.
At this stage, you might construct a conceptual framework to ensure that you’re embarking on a relevant topic . This can also help you identify which variables you will study and what you think the relationships are between them. Sometimes, you’ll have to operationalize more complex constructs.
Step 3. Formulate your hypothesis
Now you should have some idea of what you expect to find. Write your initial answer to the question in a clear, concise sentence.
4. Refine your hypothesis
You need to make sure your hypothesis is specific and testable. There are various ways of phrasing a hypothesis, but all the terms you use should have clear definitions, and the hypothesis should contain:
- The relevant variables
- The specific group being studied
- The predicted outcome of the experiment or analysis
5. Phrase your hypothesis in three ways
To identify the variables, you can write a simple prediction in if…then form. The first part of the sentence states the independent variable and the second part states the dependent variable.
In academic research, hypotheses are more commonly phrased in terms of correlations or effects, where you directly state the predicted relationship between variables.
If you are comparing two groups, the hypothesis can state what difference you expect to find between them.
6. Write a null hypothesis
If your research involves statistical hypothesis testing , you will also have to write a null hypothesis . The null hypothesis is the default position that there is no association between the variables. The null hypothesis is written as H 0 , while the alternative hypothesis is H 1 or H a .
- H 0 : The number of lectures attended by first-year students has no effect on their final exam scores.
- H 1 : The number of lectures attended by first-year students has a positive effect on their final exam scores.
If you want to know more about the research process , methodology , research bias , or statistics , make sure to check out some of our other articles with explanations and examples.
- Sampling methods
- Simple random sampling
- Stratified sampling
- Cluster sampling
- Likert scales
- Reproducibility
Statistics
- Null hypothesis
- Statistical power
- Probability distribution
- Effect size
- Poisson distribution
Research bias
- Optimism bias
- Cognitive bias
- Implicit bias
- Hawthorne effect
- Anchoring bias
- Explicit bias
Here's why students love Scribbr's proofreading services
Discover proofreading & editing
A hypothesis is not just a guess — it should be based on existing theories and knowledge. It also has to be testable, which means you can support or refute it through scientific research methods (such as experiments, observations and statistical analysis of data).
Null and alternative hypotheses are used in statistical hypothesis testing . The null hypothesis of a test always predicts no effect or no relationship between variables, while the alternative hypothesis states your research prediction of an effect or relationship.
Hypothesis testing is a formal procedure for investigating our ideas about the world using statistics. It is used by scientists to test specific predictions, called hypotheses , by calculating how likely it is that a pattern or relationship between variables could have arisen by chance.
Cite this Scribbr article
If you want to cite this source, you can copy and paste the citation or click the “Cite this Scribbr article” button to automatically add the citation to our free Citation Generator.
McCombes, S. (2023, November 20). How to Write a Strong Hypothesis | Steps & Examples. Scribbr. Retrieved April 11, 2024, from https://www.scribbr.com/methodology/hypothesis/
Is this article helpful?
Shona McCombes
Other students also liked, construct validity | definition, types, & examples, what is a conceptual framework | tips & examples, operationalization | a guide with examples, pros & cons, unlimited academic ai-proofreading.
✔ Document error-free in 5minutes ✔ Unlimited document corrections ✔ Specialized in correcting academic texts
share this!
March 11, 2021
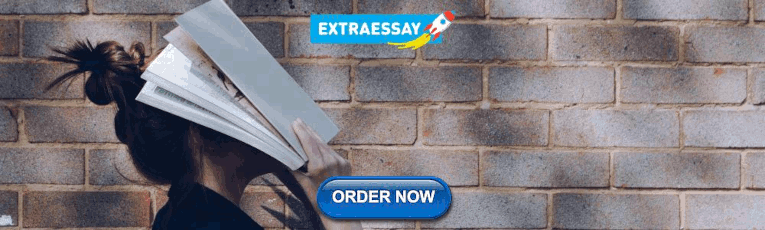
After cracking the 'sum of cubes' puzzle for 42, researchers discover a new solution for 3
by Jennifer Chu, Massachusetts Institute of Technology
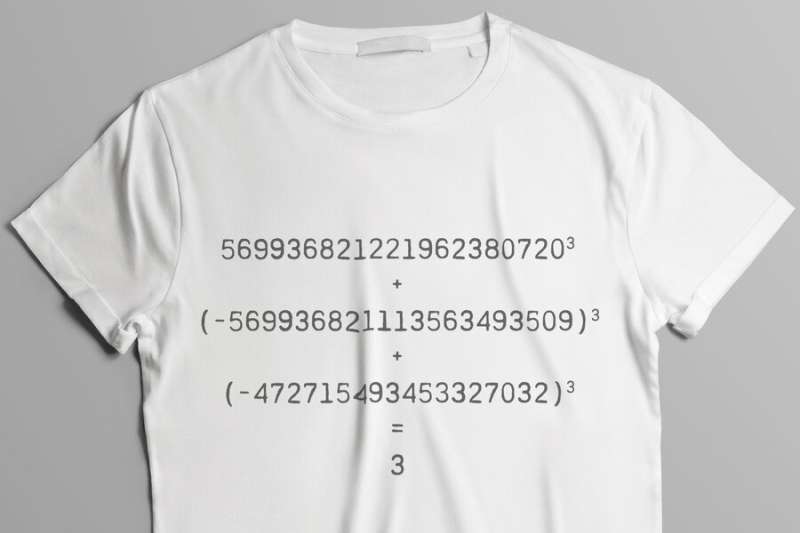
What do you do after solving the answer to life, the universe, and everything? If you're mathematicians Drew Sutherland and Andy Booker, you go for the harder problem.
In 2019, Booker, at the University of Bristol, and Sutherland, principal research scientist at MIT, were the first to find the answer to 42. The number has pop culture significance as the fictional answer to "the ultimate question of life, the universe, and everything," as Douglas Adams famously penned in his novel "The Hitchhiker's Guide to the Galaxy." The question that begets 42, at least in the novel, is frustratingly, hilariously unknown.
In mathematics, entirely by coincidence, there exists a polynomial equation for which the answer, 42, had similarly eluded mathematicians for decades. The equation x 3 +y 3 +z 3 =k is known as the sum of cubes problem. While seemingly straightforward, the equation becomes exponentially difficult to solve when framed as a "Diophantine equation"—a problem that stipulates that, for any value of k, the values for x, y, and z must each be whole numbers .
When the sum of cubes equation is framed in this way, for certain values of k, the integer solutions for x, y, and z can grow to enormous numbers. The number space that mathematicians must search across for these numbers is larger still, requiring intricate and massive computations.
Over the years, mathematicians had managed through various means to solve the equation, either finding a solution or determining that a solution must not exist, for every value of k between 1 and 100—except for 42.
In September 2019, Booker and Sutherland, harnessing the combined power of half a million home computers around the world, for the first time found a solution to 42. The widely reported breakthrough spurred the team to tackle an even harder, and in some ways more universal problem: finding the next solution for 3.
Booker and Sutherland have now published the solutions for 42 and 3, along with several other numbers greater than 100, this week in the Proceedings of the National Academy of Sciences .
Picking up the gauntlet
The first two solutions for the equation x 3 +y 3 +z 3 = 3 might be obvious to any high school algebra student, where x, y, and z can be either 1, 1, and 1, or 4, 4, and -5. Finding a third solution, however, has stumped expert number theorists for decades, and in 1953 the puzzle prompted pioneering mathematician Louis Mordell to ask the question: Is it even possible to know whether other solutions for 3 exist?
"This was sort of like Mordell throwing down the gauntlet," says Sutherland. "The interest in solving this question is not so much for the particular solution, but to better understand how hard these equations are to solve. It's a benchmark against which we can measure ourselves."
As decades went by with no new solutions for 3, many began to believe there were none to be found. But soon after finding the answer to 42, Booker and Sutherland's method, in a surprisingly short time, turned up the next solution for 3:
569936821221962380720 3 + (-569936821113563493509) 3 + (-472715493453327032) 3 = 3
The discovery was a direct answer to Mordell's question: Yes, it is possible to find the next solution to 3, and what's more, here is that solution. And perhaps more universally, the solution, involving gigantic, 21-digit numbers that were not possible to sift out until now, suggests that there are more solutions out there, for 3, and other values of k.
"There had been some serious doubt in the mathematical and computational communities, because [Mordell's question] is very hard to test," Sutherland says. "The numbers get so big so fast. You're never going to find more than the first few solutions. But what I can say is, having found this one solution, I'm convinced there are infinitely many more out there."
A solution's twist
To find the solutions for both 42 and 3, the team started with an existing algorithm, or a twisting of the sum of cubes equation into a form they believed would be more manageable to solve:
k - z 3 = x 3 + y 3 = (x + y)(x 2 - xy + y 2 )
This approach was first proposed by mathematician Roger Heath-Brown, who conjectured that there should be infinitely many solutions for every suitable k. The team further modified the algorithm by representing x+y as a single parameter, d. They then reduced the equation by dividing both sides by d and keeping only the remainder—an operation in mathematics termed "modulo d"—leaving a simplified representation of the problem.
"You can now think of k as a cube root of z, modulo d," Sutherland explains. "So imagine working in a system of arithmetic where you only care about the remainder modulo d, and we're trying to compute a cube root of k."
With this sleeker version of the equation, the researchers would only need to look for values of d and z that would guarantee finding the ultimate solutions to x, y, and z, for k=3. But still, the space of numbers that they would have to search through would be infinitely large.
So, the researchers optimized the algorithm by using mathematical "sieving" techniques to dramatically cut down the space of possible solutions for d.
"This involves some fairly advanced number theory, using the structure of what we know about number fields to avoid looking in places we don't need to look," Sutherland says.
A global task
The team also developed ways to efficiently split the algorithm's search into hundreds of thousands of parallel processing streams. If the algorithm were run on just one computer, it would have taken hundreds of years to find a solution to k=3. By dividing the job into millions of smaller tasks, each independently run on a separate computer, the team could further speed up their search.
In September 2019, the researchers put their plan in play through Charity Engine, a project that can be downloaded as a free app by any personal computer, and which is designed to harness any spare home computing power to collectively solve hard mathematical problems. At the time, Charity Engine's grid comprised over 400,000 computers around the world, and Booker and Sutherland were able to run their algorithm on the network as a test of Charity Engine's new software platform.
"For each computer in the network, they are told, 'your job is to look for d's whose prime factor falls within this range, subject to some other conditions,'" Sutherland says. "And we had to figure out how to divide the job up into roughly 4 million tasks that would each take about three hours for a computer to complete."
Very quickly, the global grid returned the very first solution to k=42, and just two weeks later, the researchers confirmed they had found the third solution for k=3—a milestone that they marked, in part, by printing the equation on t-shirts.
The fact that a third solution to k=3 exists suggests that Heath-Brown's original conjecture was right and that there are infinitely more solutions beyond this newest one. Heath-Brown also predicts the space between solutions will grow exponentially, along with their searches. For instance, rather than the third solution's 21-digit values, the fourth solution for x, y, and z will likely involve numbers with a mind-boggling 28 digits.
"The amount of work you have to do for each new solution grows by a factor of more than 10 million, so the next solution for 3 will need 10 million times 400,000 computers to find, and there's no guarantee that's even enough," Sutherland says. "I don't know if we'll ever know the fourth solution. But I do believe it's out there."
Journal information: Proceedings of the National Academy of Sciences
Provided by Massachusetts Institute of Technology
Explore further
Feedback to editors

The experimental demonstration of a verifiable blind quantum computing protocol

A machine learning-based approach to discover nanocomposite films for biodegradable plastic alternatives

Saturday Citations: Listening to bird dreams, securing qubits, imagining impossible billiards
2 hours ago

Physicists solve puzzle about ancient galaxy found by Webb telescope
3 hours ago

Researchers study effects of solvation and ion valency on metallopolymers

Chemists devise easier new method for making a common type of building block for drugs

Research team discovers more than 50 potentially new deep-sea species in one of the most unexplored areas of the planet
20 hours ago

New study details how starving cells hijack protein transport stations

New species of ant found pottering under the Pilbara named after Voldemort
21 hours ago

Searching for new asymmetry between matter and antimatter
Relevant physicsforums posts, part to whole comparison.
Apr 11, 2024
How to pick some numbers out of 13 integers, by a 4 digits code
Apr 10, 2024
Calculating the inverse of a function involving the error function
Apr 5, 2024
Reprise: Calculate the distance between two points without using a coordinate system
Apr 4, 2024
Non-sinusoidal waveform model
Apr 3, 2024
I've been trying to understand the proof for the binomial theorem
More from General Math
Related Stories

Bristol mathematician cracks Diophantine puzzle
Apr 2, 2019

Sum of three cubes for 42 finally solved—using real life planetary computer
Sep 6, 2019

Mathematicians proposed an express method for calculation of the propagation of light
Sep 13, 2019

Mathematician suggests a scheme for solving telegraph equations
Feb 11, 2021

Using computers to crack open centuries-old mathematical puzzles
Apr 3, 2019

Mathematicians prove a theorem that would help calculate the movement of water in porous rock
Oct 23, 2019
Recommended for you

A periodic table of primes: Research team claims that prime numbers can be predicted

'I had such fun!', says winner of top math prize
Mar 20, 2024

Ice-ray patterns: A rediscovery of past design for the future

Paper offers a mathematical approach to modeling a random walker moving across a random landscape
Mar 13, 2024

How do neural networks learn? A mathematical formula explains how they detect relevant patterns
Mar 12, 2024

Mathematicians prove Pólya's conjecture for the eigenvalues of a disk, a 70-year-old math problem
Mar 1, 2024
Let us know if there is a problem with our content
Use this form if you have come across a typo, inaccuracy or would like to send an edit request for the content on this page. For general inquiries, please use our contact form . For general feedback, use the public comments section below (please adhere to guidelines ).
Please select the most appropriate category to facilitate processing of your request
Thank you for taking time to provide your feedback to the editors.
Your feedback is important to us. However, we do not guarantee individual replies due to the high volume of messages.
E-mail the story
Your email address is used only to let the recipient know who sent the email. Neither your address nor the recipient's address will be used for any other purpose. The information you enter will appear in your e-mail message and is not retained by Phys.org in any form.
Newsletter sign up
Get weekly and/or daily updates delivered to your inbox. You can unsubscribe at any time and we'll never share your details to third parties.
More information Privacy policy
Donate and enjoy an ad-free experience
We keep our content available to everyone. Consider supporting Science X's mission by getting a premium account.
E-mail newsletter
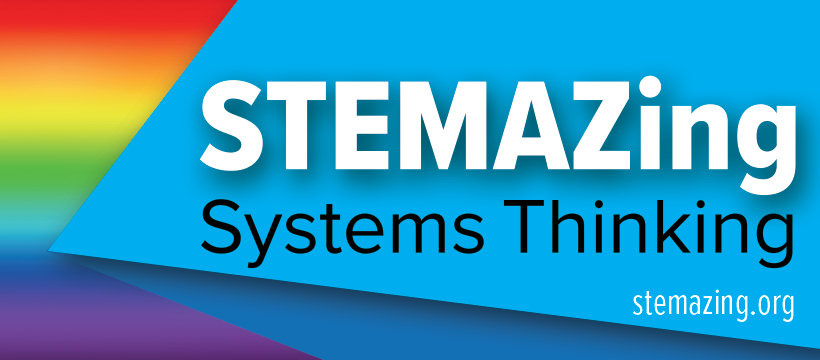
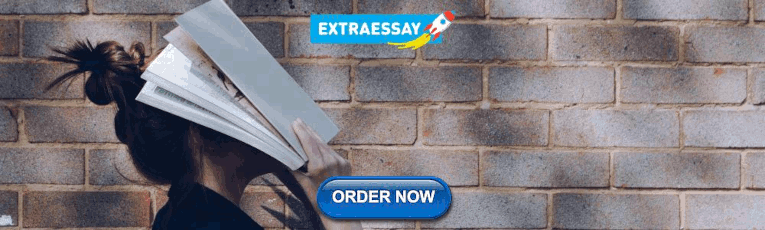
IMAGES
VIDEO
COMMENTS
A 2 on the bottom means it should be shaded grey as well. Cube 2 Hypotheses and Patterns Claim 1: It is red, has the numbers 4 on the bottom and 8 on the top and the name is. FRANCINE. Claim 2: It is red, has the numbers 4 on the bottom and 5 on the top and the name is. FRANN or FRANI or FRANY.
Mini Inquiry Cube Materials. The materials are relatively inexpensive and can be easily found on Amazon to make 50 cubes for under $20. These wooden cubes are nice because they come pre-cut to the size you need. These ones come in packs of 120 but you can find others in different quantities pretty easily as well.
The \ (2^k\) designs are a major set of building blocks for many experimental designs. These designs are usually referred to as screening designs. The \ (2^k\) refers to designs with k factors where each factor has just two levels. These designs are created to explore a large number of factors, with each factor having the minimal number of ...
Cube #2 FRANCENE ALMA FRANK ROBERTA ROB 3 3 5 4 ALFRED 6 2 7 3 4 8 2 4 Bottom. Created Date: 19980407131243Z ...
Honors Physics Lesson 1A.0 - Hypothesis Cube Claim-Evidence-Reasoning Student Name: Directions: Observing the five visible sides, determine what is on the bottom of the cube. Find as many patterns (evidence) ... Please refer to the attachment to answer this question. This question was created from Each person will be partnered with one other ...
1. Find the mass, volume, & density of each cube. 2. Use the table to hypothesize what material each cube is made of. 3. Find your teacher to check your answers & record the actual material of each cube. Cube # Mass (g) Volume (cm3) L x W x H Density = m/v g/cm3 Hypothesis: Cube Material Actual: Cube Material 1 2 3 4
0 2 . 2 The loss in mass shown in FIGURE 3 is due to osmosis. The rate of osmosis between 0 and 40 minutes is faster in B (the eight small cubes) than in A (single large cube). Is the rate of osmosis per mm2 per minute different between A and B during this time? Use appropriate calculations to support your answer. [3 marks]
5. Phrase your hypothesis in three ways. To identify the variables, you can write a simple prediction in if…then form. The first part of the sentence states the independent variable and the second part states the dependent variable. If a first-year student starts attending more lectures, then their exam scores will improve.
Study with Quizlet and memorize flashcards containing terms like The Scientific Method Question options: A) is based on continued observation and experiment. B) is a strict set of rules and procedures that lead to inarguable fact. C) is just a theory. D) isn't used much in modern chemistry. E) is a framework for proving an argument you know to be true., How many significant figures are in the ...
To find the solutions for both 42 and 3, the team started with an existing algorithm, or a twisting of the sum of cubes equation into a form they believed would be more manageable to solve: k - z ...
Check the temperature of the buckets before you begin the experiment. Use ice cubes from a refrigerator icemaker if possible because they are very uniform. If you make them in ice cube trays, each cube should be only about 1⁄2 to 3⁄4 of the compartment. Use a syringe to fill them if necessary to make them uniform.
Study with Quizlet and memorize flashcards containing terms like An empirical science is one that is based on a. hypothesis b. calculus c. computer models d. observed data, When Earth catches up to a slower-moving outer planet and passes it in its orbit, the planet a. exhibits retrograde motion b. slows down because it feels Earth's gravitational pull c. decreases in brightness as it passes ...
2-tailed test crit under Ho Allow use Of t method AWFW 0.99 to 1.00 (allow 1) Or z = 1.96 On their z and critical value Or t Accept Ho at 5% level Of significance. Sufficient evidence at the 5% level Of significance to support the manufacturer's belief. Total 6(a) 471 = 94.2 - 6.058 4 1 -tailed test --2.132 Ho Hi : p < 100 94.2-100 --2.14 6.058
Hypothesis: If the mass and volume of a cube is found, then the density of the cube will determine the material of the cube. Procedures: 1. Find the mass of each cube using a balance and record results in the data table. 2. Find the volume of each cube (Length x Width x Height) and record results in the data table. 3.
Expert-verified. Answer- Here the body is completely subm …. Stating a Hypothesis 2 1. Based on an understanding of geometry and Archimedes' Principle, which of the following is a reasonable hypothesis? If a solid is a cube whose side measures 1 cm and whose mass is 10.0g, then it will displace 10cm of water when submerged If a solid is a ...
Biology questions and answers. B. Osmosis: Determining the Tonicity of Unknown SolutionsPREDICTYou will be placing cubes of potato into beakers of unknown tonicity, and measuring the change in weight of the potato cubes after a period of time. Answer questions on the data sheet.PROTOCOLTonicity refers to the concentration of solutes in a solution.
View Hypothesis WS 2 answers from MATH 302 at High School Summer Program. Hypothesis Worksheet #2 Answers 1) fail to reject Ho 2) reject Ho 3) fail to reject Ho 4) reject Ho 5) p-value = .0202 6)
Hypothesis Cube 1. Click, hold, and move around the grey cube that says TOP and FRONT, or use your fingers on a cell phone or tablet touch screen, to rotate the cube around to explore the five faces of the cube you are given. Make a claim about what you think is on the missing face (which number and what color is the face). Support your claim ...