
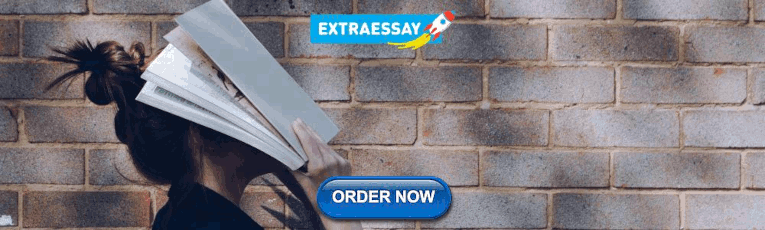
Research Topics & Ideas: Healthcare
100+ Healthcare Research Topic Ideas To Fast-Track Your Project
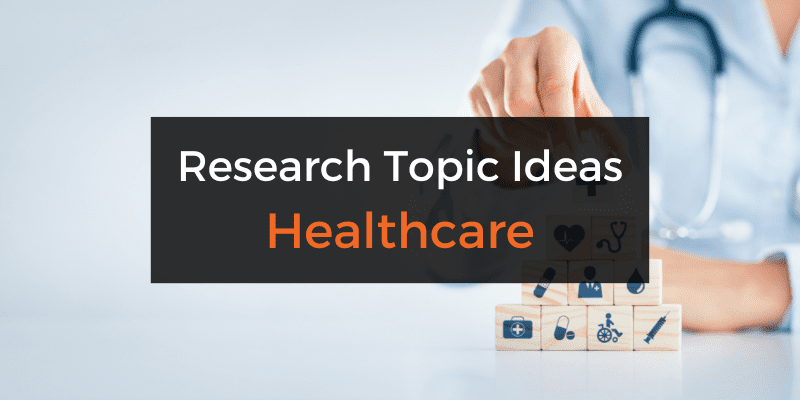
Finding and choosing a strong research topic is the critical first step when it comes to crafting a high-quality dissertation, thesis or research project. If you’ve landed on this post, chances are you’re looking for a healthcare-related research topic , but aren’t sure where to start. Here, we’ll explore a variety of healthcare-related research ideas and topic thought-starters across a range of healthcare fields, including allopathic and alternative medicine, dentistry, physical therapy, optometry, pharmacology and public health.
NB – This is just the start…
The topic ideation and evaluation process has multiple steps . In this post, we’ll kickstart the process by sharing some research topic ideas within the healthcare domain. This is the starting point, but to develop a well-defined research topic, you’ll need to identify a clear and convincing research gap , along with a well-justified plan of action to fill that gap.
If you’re new to the oftentimes perplexing world of research, or if this is your first time undertaking a formal academic research project, be sure to check out our free dissertation mini-course. In it, we cover the process of writing a dissertation or thesis from start to end. Be sure to also sign up for our free webinar that explores how to find a high-quality research topic.
Overview: Healthcare Research Topics
- Allopathic medicine
- Alternative /complementary medicine
- Veterinary medicine
- Physical therapy/ rehab
- Optometry and ophthalmology
- Pharmacy and pharmacology
- Public health
- Examples of healthcare-related dissertations
Allopathic (Conventional) Medicine
- The effectiveness of telemedicine in remote elderly patient care
- The impact of stress on the immune system of cancer patients
- The effects of a plant-based diet on chronic diseases such as diabetes
- The use of AI in early cancer diagnosis and treatment
- The role of the gut microbiome in mental health conditions such as depression and anxiety
- The efficacy of mindfulness meditation in reducing chronic pain: A systematic review
- The benefits and drawbacks of electronic health records in a developing country
- The effects of environmental pollution on breast milk quality
- The use of personalized medicine in treating genetic disorders
- The impact of social determinants of health on chronic diseases in Asia
- The role of high-intensity interval training in improving cardiovascular health
- The efficacy of using probiotics for gut health in pregnant women
- The impact of poor sleep on the treatment of chronic illnesses
- The role of inflammation in the development of chronic diseases such as lupus
- The effectiveness of physiotherapy in pain control post-surgery
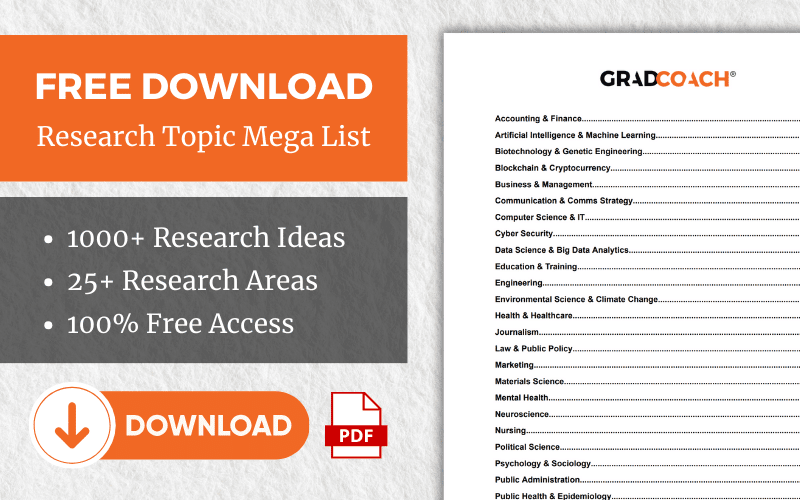
Topics & Ideas: Alternative Medicine
- The benefits of herbal medicine in treating young asthma patients
- The use of acupuncture in treating infertility in women over 40 years of age
- The effectiveness of homoeopathy in treating mental health disorders: A systematic review
- The role of aromatherapy in reducing stress and anxiety post-surgery
- The impact of mindfulness meditation on reducing high blood pressure
- The use of chiropractic therapy in treating back pain of pregnant women
- The efficacy of traditional Chinese medicine such as Shun-Qi-Tong-Xie (SQTX) in treating digestive disorders in China
- The impact of yoga on physical and mental health in adolescents
- The benefits of hydrotherapy in treating musculoskeletal disorders such as tendinitis
- The role of Reiki in promoting healing and relaxation post birth
- The effectiveness of naturopathy in treating skin conditions such as eczema
- The use of deep tissue massage therapy in reducing chronic pain in amputees
- The impact of tai chi on the treatment of anxiety and depression
- The benefits of reflexology in treating stress, anxiety and chronic fatigue
- The role of acupuncture in the prophylactic management of headaches and migraines
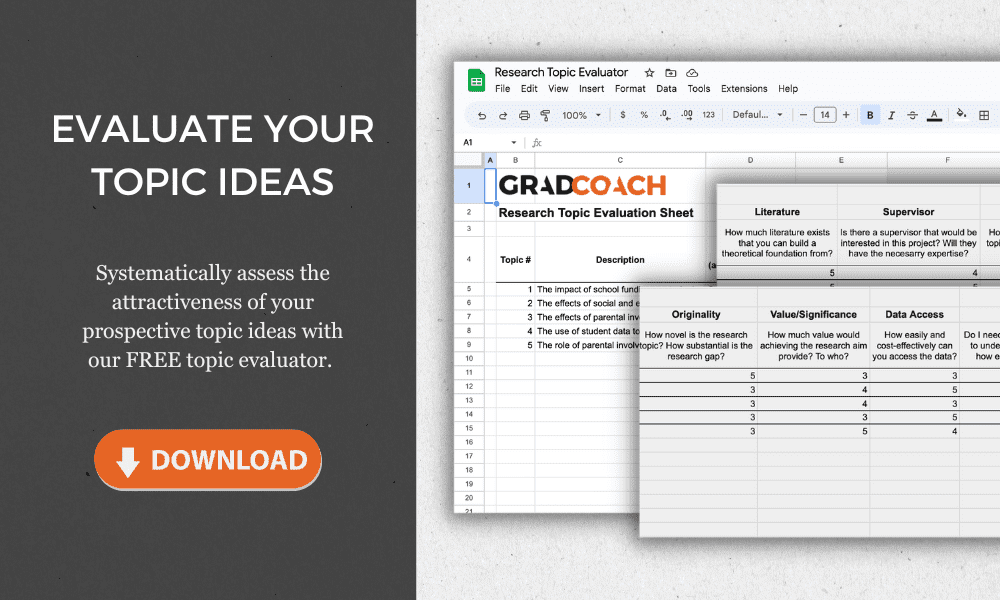
Topics & Ideas: Dentistry
- The impact of sugar consumption on the oral health of infants
- The use of digital dentistry in improving patient care: A systematic review
- The efficacy of orthodontic treatments in correcting bite problems in adults
- The role of dental hygiene in preventing gum disease in patients with dental bridges
- The impact of smoking on oral health and tobacco cessation support from UK dentists
- The benefits of dental implants in restoring missing teeth in adolescents
- The use of lasers in dental procedures such as root canals
- The efficacy of root canal treatment using high-frequency electric pulses in saving infected teeth
- The role of fluoride in promoting remineralization and slowing down demineralization
- The impact of stress-induced reflux on oral health
- The benefits of dental crowns in restoring damaged teeth in elderly patients
- The use of sedation dentistry in managing dental anxiety in children
- The efficacy of teeth whitening treatments in improving dental aesthetics in patients with braces
- The role of orthodontic appliances in improving well-being
- The impact of periodontal disease on overall health and chronic illnesses
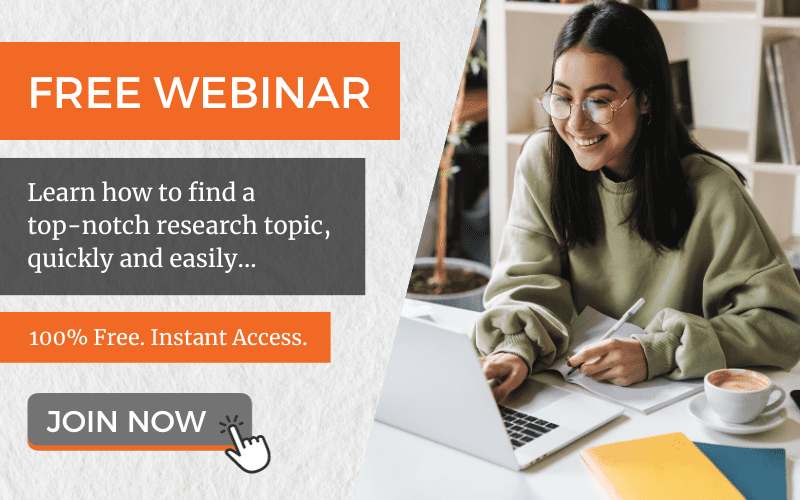
Tops & Ideas: Veterinary Medicine
- The impact of nutrition on broiler chicken production
- The role of vaccines in disease prevention in horses
- The importance of parasite control in animal health in piggeries
- The impact of animal behaviour on welfare in the dairy industry
- The effects of environmental pollution on the health of cattle
- The role of veterinary technology such as MRI in animal care
- The importance of pain management in post-surgery health outcomes
- The impact of genetics on animal health and disease in layer chickens
- The effectiveness of alternative therapies in veterinary medicine: A systematic review
- The role of veterinary medicine in public health: A case study of the COVID-19 pandemic
- The impact of climate change on animal health and infectious diseases in animals
- The importance of animal welfare in veterinary medicine and sustainable agriculture
- The effects of the human-animal bond on canine health
- The role of veterinary medicine in conservation efforts: A case study of Rhinoceros poaching in Africa
- The impact of veterinary research of new vaccines on animal health
Topics & Ideas: Physical Therapy/Rehab
- The efficacy of aquatic therapy in improving joint mobility and strength in polio patients
- The impact of telerehabilitation on patient outcomes in Germany
- The effect of kinesiotaping on reducing knee pain and improving function in individuals with chronic pain
- A comparison of manual therapy and yoga exercise therapy in the management of low back pain
- The use of wearable technology in physical rehabilitation and the impact on patient adherence to a rehabilitation plan
- The impact of mindfulness-based interventions in physical therapy in adolescents
- The effects of resistance training on individuals with Parkinson’s disease
- The role of hydrotherapy in the management of fibromyalgia
- The impact of cognitive-behavioural therapy in physical rehabilitation for individuals with chronic pain
- The use of virtual reality in physical rehabilitation of sports injuries
- The effects of electrical stimulation on muscle function and strength in athletes
- The role of physical therapy in the management of stroke recovery: A systematic review
- The impact of pilates on mental health in individuals with depression
- The use of thermal modalities in physical therapy and its effectiveness in reducing pain and inflammation
- The effect of strength training on balance and gait in elderly patients
Topics & Ideas: Optometry & Opthalmology
- The impact of screen time on the vision and ocular health of children under the age of 5
- The effects of blue light exposure from digital devices on ocular health
- The role of dietary interventions, such as the intake of whole grains, in the management of age-related macular degeneration
- The use of telemedicine in optometry and ophthalmology in the UK
- The impact of myopia control interventions on African American children’s vision
- The use of contact lenses in the management of dry eye syndrome: different treatment options
- The effects of visual rehabilitation in individuals with traumatic brain injury
- The role of low vision rehabilitation in individuals with age-related vision loss: challenges and solutions
- The impact of environmental air pollution on ocular health
- The effectiveness of orthokeratology in myopia control compared to contact lenses
- The role of dietary supplements, such as omega-3 fatty acids, in ocular health
- The effects of ultraviolet radiation exposure from tanning beds on ocular health
- The impact of computer vision syndrome on long-term visual function
- The use of novel diagnostic tools in optometry and ophthalmology in developing countries
- The effects of virtual reality on visual perception and ocular health: an examination of dry eye syndrome and neurologic symptoms
Topics & Ideas: Pharmacy & Pharmacology
- The impact of medication adherence on patient outcomes in cystic fibrosis
- The use of personalized medicine in the management of chronic diseases such as Alzheimer’s disease
- The effects of pharmacogenomics on drug response and toxicity in cancer patients
- The role of pharmacists in the management of chronic pain in primary care
- The impact of drug-drug interactions on patient mental health outcomes
- The use of telepharmacy in healthcare: Present status and future potential
- The effects of herbal and dietary supplements on drug efficacy and toxicity
- The role of pharmacists in the management of type 1 diabetes
- The impact of medication errors on patient outcomes and satisfaction
- The use of technology in medication management in the USA
- The effects of smoking on drug metabolism and pharmacokinetics: A case study of clozapine
- Leveraging the role of pharmacists in preventing and managing opioid use disorder
- The impact of the opioid epidemic on public health in a developing country
- The use of biosimilars in the management of the skin condition psoriasis
- The effects of the Affordable Care Act on medication utilization and patient outcomes in African Americans
Topics & Ideas: Public Health
- The impact of the built environment and urbanisation on physical activity and obesity
- The effects of food insecurity on health outcomes in Zimbabwe
- The role of community-based participatory research in addressing health disparities
- The impact of social determinants of health, such as racism, on population health
- The effects of heat waves on public health
- The role of telehealth in addressing healthcare access and equity in South America
- The impact of gun violence on public health in South Africa
- The effects of chlorofluorocarbons air pollution on respiratory health
- The role of public health interventions in reducing health disparities in the USA
- The impact of the United States Affordable Care Act on access to healthcare and health outcomes
- The effects of water insecurity on health outcomes in the Middle East
- The role of community health workers in addressing healthcare access and equity in low-income countries
- The impact of mass incarceration on public health and behavioural health of a community
- The effects of floods on public health and healthcare systems
- The role of social media in public health communication and behaviour change in adolescents
Examples: Healthcare Dissertation & Theses
While the ideas we’ve presented above are a decent starting point for finding a healthcare-related research topic, they are fairly generic and non-specific. So, it helps to look at actual dissertations and theses to see how this all comes together.
Below, we’ve included a selection of research projects from various healthcare-related degree programs to help refine your thinking. These are actual dissertations and theses, written as part of Master’s and PhD-level programs, so they can provide some useful insight as to what a research topic looks like in practice.
- Improving Follow-Up Care for Homeless Populations in North County San Diego (Sanchez, 2021)
- On the Incentives of Medicare’s Hospital Reimbursement and an Examination of Exchangeability (Elzinga, 2016)
- Managing the healthcare crisis: the career narratives of nurses (Krueger, 2021)
- Methods for preventing central line-associated bloodstream infection in pediatric haematology-oncology patients: A systematic literature review (Balkan, 2020)
- Farms in Healthcare: Enhancing Knowledge, Sharing, and Collaboration (Garramone, 2019)
- When machine learning meets healthcare: towards knowledge incorporation in multimodal healthcare analytics (Yuan, 2020)
- Integrated behavioural healthcare: The future of rural mental health (Fox, 2019)
- Healthcare service use patterns among autistic adults: A systematic review with narrative synthesis (Gilmore, 2021)
- Mindfulness-Based Interventions: Combatting Burnout and Compassionate Fatigue among Mental Health Caregivers (Lundquist, 2022)
- Transgender and gender-diverse people’s perceptions of gender-inclusive healthcare access and associated hope for the future (Wille, 2021)
- Efficient Neural Network Synthesis and Its Application in Smart Healthcare (Hassantabar, 2022)
- The Experience of Female Veterans and Health-Seeking Behaviors (Switzer, 2022)
- Machine learning applications towards risk prediction and cost forecasting in healthcare (Singh, 2022)
- Does Variation in the Nursing Home Inspection Process Explain Disparity in Regulatory Outcomes? (Fox, 2020)
Looking at these titles, you can probably pick up that the research topics here are quite specific and narrowly-focused , compared to the generic ones presented earlier. This is an important thing to keep in mind as you develop your own research topic. That is to say, to create a top-notch research topic, you must be precise and target a specific context with specific variables of interest . In other words, you need to identify a clear, well-justified research gap.
Need more help?
If you’re still feeling a bit unsure about how to find a research topic for your healthcare dissertation or thesis, check out Topic Kickstarter service below.
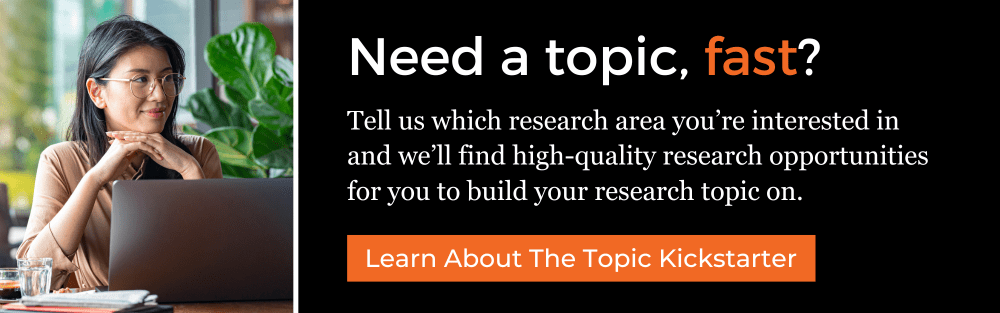
You Might Also Like:
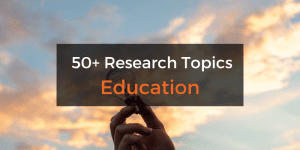
15 Comments
I need topics that will match the Msc program am running in healthcare research please
Hello Mabel,
I can help you with a good topic, kindly provide your email let’s have a good discussion on this.
Can you provide some research topics and ideas on Immunology?
Thank you to create new knowledge on research problem verse research topic
Help on problem statement on teen pregnancy
This post might be useful: https://gradcoach.com/research-problem-statement/
can you provide me with a research topic on healthcare related topics to a qqi level 5 student
Please can someone help me with research topics in public health ?
Hello I have requirement of Health related latest research issue/topics for my social media speeches. If possible pls share health issues , diagnosis, treatment.
I would like a topic thought around first-line support for Gender-Based Violence for survivors or one related to prevention of Gender-Based Violence
Please can I be helped with a master’s research topic in either chemical pathology or hematology or immunology? thanks
Can u please provide me with a research topic on occupational health and safety at the health sector
Good day kindly help provide me with Ph.D. Public health topics on Reproductive and Maternal Health, interventional studies on Health Education
may you assist me with a good easy healthcare administration study topic
May you assist me in finding a research topic on nutrition,physical activity and obesity. On the impact on children
Submit a Comment Cancel reply
Your email address will not be published. Required fields are marked *
Save my name, email, and website in this browser for the next time I comment.
- Print Friendly
77 interesting medical research topics for 2024
Last updated
25 November 2023
Reviewed by
Brittany Ferri, PhD, OTR/L
Medical research is the gateway to improved patient care and expanding our available treatment options. However, finding a relevant and compelling research topic can be challenging.
Use this article as a jumping-off point to select an interesting medical research topic for your next paper or clinical study.
- How to choose a medical research topic
When choosing a research topic , it’s essential to consider a couple of things. What topics interest you? What unanswered questions do you want to address?
During the decision-making and brainstorming process, here are a few helpful tips to help you pick the right medical research topic:
Focus on a particular field of study
The best medical research is specific to a particular area. Generalized studies are often too broad to produce meaningful results, so we advise picking a specific niche early in the process.
Maybe a certain topic interests you, or your industry knowledge reveals areas of need.
Look into commonly researched topics
Once you’ve chosen your research field, do some preliminary research. What have other academics done in their papers and projects?
From this list, you can focus on specific topics that interest you without accidentally creating a copycat project. This groundwork will also help you uncover any literature gaps—those may be beneficial areas for research.
Get curious and ask questions
Now you can get curious. Ask questions that start with why, how, or what. These questions are the starting point of your project design and will act as your guiding light throughout the process.
For example:
What impact does pollution have on children’s lung function in inner-city neighborhoods?
Why is pollution-based asthma on the rise?
How can we address pollution-induced asthma in young children?
- 77 medical research topics worth exploring in 2023
Need some research inspiration for your upcoming paper or clinical study? We’ve compiled a list of 77 topical and in-demand medical research ideas. Let’s take a look.
- Exciting new medical research topics
If you want to study cutting-edge topics, here are some exciting options:
COVID-19 and long COVID symptoms
Since 2020, COVID-19 has been a hot-button topic in medicine, along with the long-term symptoms in those with a history of COVID-19.
Examples of COVID-19-related research topics worth exploring include:
The long-term impact of COVID-19 on cardiac and respiratory health
COVID-19 vaccination rates
The evolution of COVID-19 symptoms over time
New variants and strains of the COVID-19 virus
Changes in social behavior and public health regulations amid COVID-19
Vaccinations
Finding ways to cure or reduce the disease burden of chronic infectious diseases is a crucial research area. Vaccination is a powerful option and a great topic to research.
Examples of vaccination-related research topics include:
mRNA vaccines for viral infections
Biomaterial vaccination capabilities
Vaccination rates based on location, ethnicity, or age
Public opinion about vaccination safety
Artificial tissues fabrication
With the need for donor organs increasing, finding ways to fabricate artificial bioactive tissues (and possibly organs) is a popular research area.
Examples of artificial tissue-related research topics you can study include:
The viability of artificially printed tissues
Tissue substrate and building block material studies
The ethics and efficacy of artificial tissue creation
- Medical research topics for medical students
For many medical students, research is a big driver for entering healthcare. If you’re a medical student looking for a research topic, here are some great ideas to work from:
Sleep disorders
Poor sleep quality is a growing problem, and it can significantly impact a person’s overall health.
Examples of sleep disorder-related research topics include:
How stress affects sleep quality
The prevalence and impact of insomnia on patients with mental health conditions
Possible triggers for sleep disorder development
The impact of poor sleep quality on psychological and physical health
How melatonin supplements impact sleep quality
Alzheimer’s and dementia
Cognitive conditions like dementia and Alzheimer’s disease are on the rise worldwide. They currently have no cure. As a result, research about these topics is in high demand.
Examples of dementia-related research topics you could explore include:
The prevalence of Alzheimer’s disease in a chosen population
Early onset symptoms of dementia
Possible triggers or causes of cognitive decline with age
Treatment options for dementia-like conditions
The mental and physical burden of caregiving for patients with dementia
- Lifestyle habits and public health
Modern lifestyles have profoundly impacted the average person’s daily habits, and plenty of interesting topics explore its effects.
Examples of lifestyle and public health-related research topics include:
The nutritional intake of college students
The impact of chronic work stress on overall health
The rise of upper back and neck pain from laptop use
Prevalence and cause of repetitive strain injuries (RSI)
- Controversial medical research paper topics
Medical research is a hotbed of controversial topics, content, and areas of study.
If you want to explore a more niche (and attention-grabbing) concept, here are some controversial medical research topics worth looking into:
The benefits and risks of medical cannabis
Depending on where you live, the legalization and use of cannabis for medical conditions is controversial for the general public and healthcare providers.
Examples of medical cannabis-related research topics that might grab your attention include:
The legalization process of medical cannabis
The impact of cannabis use on developmental milestones in youth users
Cannabis and mental health diagnoses
CBD’s impact on chronic pain
Prevalence of cannabis use in young people
The impact of maternal cannabis use on fetal development
Understanding how THC impacts cognitive function
Human genetics
The Human Genome Project identified, mapped, and sequenced all human DNA genes. Its completion in 2003 opened up a world of exciting and controversial studies in human genetics.
Examples of human genetics-related research topics worth delving into include:
Medical genetics and the incidence of genetic-based health disorders
Behavioral genetics differences between identical twins
Genetic risk factors for neurodegenerative disorders
Machine learning technologies for genetic research
Sexual health studies
Human sexuality and sexual health are important (yet often stigmatized) medical topics that need new research and analysis.
As a diverse field ranging from sexual orientation studies to sexual pathophysiology, examples of sexual health-related research topics include:
The incidence of sexually transmitted infections within a chosen population
Mental health conditions within the LGBTQIA+ community
The impact of untreated sexually transmitted infections
Access to safe sex resources (condoms, dental dams, etc.) in rural areas
- Health and wellness research topics
Human wellness and health are trendy topics in modern medicine as more people are interested in finding natural ways to live healthier lifestyles.
If this field of study interests you, here are some big topics in the wellness space:
Gluten sensitivity
Gluten allergies and intolerances have risen over the past few decades. If you’re interested in exploring this topic, your options range in severity from mild gastrointestinal symptoms to full-blown anaphylaxis.
Some examples of gluten sensitivity-related research topics include:
The pathophysiology and incidence of Celiac disease
Early onset symptoms of gluten intolerance
The prevalence of gluten allergies within a set population
Gluten allergies and the incidence of other gastrointestinal health conditions
Pollution and lung health
Living in large urban cities means regular exposure to high levels of pollutants.
As more people become interested in protecting their lung health, examples of impactful lung health and pollution-related research topics include:
The extent of pollution in densely packed urban areas
The prevalence of pollution-based asthma in a set population
Lung capacity and function in young people
The benefits and risks of steroid therapy for asthma
Pollution risks based on geographical location
Plant-based diets
Plant-based diets like vegan and paleo diets are emerging trends in healthcare due to their limited supporting research.
If you’re interested in learning more about the potential benefits or risks of holistic, diet-based medicine, examples of plant-based diet research topics to explore include:
Vegan and plant-based diets as part of disease management
Potential risks and benefits of specific plant-based diets
Plant-based diets and their impact on body mass index
The effect of diet and lifestyle on chronic disease management
Health supplements
Supplements are a multi-billion dollar industry. Many health-conscious people take supplements, including vitamins, minerals, herbal medicine, and more.
Examples of health supplement-related research topics worth investigating include:
Omega-3 fish oil safety and efficacy for cardiac patients
The benefits and risks of regular vitamin D supplementation
Health supplementation regulation and product quality
The impact of social influencer marketing on consumer supplement practices
Analyzing added ingredients in protein powders
- Healthcare research topics
Working within the healthcare industry means you have insider knowledge and opportunity. Maybe you’d like to research the overall system, administration, and inherent biases that disrupt access to quality care.
While these topics are essential to explore, it is important to note that these studies usually require approval and oversight from an Institutional Review Board (IRB). This ensures the study is ethical and does not harm any subjects.
For this reason, the IRB sets protocols that require additional planning, so consider this when mapping out your study’s timeline.
Here are some examples of trending healthcare research areas worth pursuing:
The pros and cons of electronic health records
The rise of electronic healthcare charting and records has forever changed how medical professionals and patients interact with their health data.
Examples of electronic health record-related research topics include:
The number of medication errors reported during a software switch
Nurse sentiment analysis of electronic charting practices
Ethical and legal studies into encrypting and storing personal health data
Inequities within healthcare access
Many barriers inhibit people from accessing the quality medical care they need. These issues result in health disparities and injustices.
Examples of research topics about health inequities include:
The impact of social determinants of health in a set population
Early and late-stage cancer stage diagnosis in urban vs. rural populations
Affordability of life-saving medications
Health insurance limitations and their impact on overall health
Diagnostic and treatment rates across ethnicities
People who belong to an ethnic minority are more likely to experience barriers and restrictions when trying to receive quality medical care. This is due to systemic healthcare racism and bias.
As a result, diagnostic and treatment rates in minority populations are a hot-button field of research. Examples of ethnicity-based research topics include:
Cancer biopsy rates in BIPOC women
The prevalence of diabetes in Indigenous communities
Access inequalities in women’s health preventative screenings
The prevalence of undiagnosed hypertension in Black populations
- Pharmaceutical research topics
Large pharmaceutical companies are incredibly interested in investing in research to learn more about potential cures and treatments for diseases.
If you’re interested in building a career in pharmaceutical research, here are a few examples of in-demand research topics:
Cancer treatment options
Clinical research is in high demand as pharmaceutical companies explore novel cancer treatment options outside of chemotherapy and radiation.
Examples of cancer treatment-related research topics include:
Stem cell therapy for cancer
Oncogenic gene dysregulation and its impact on disease
Cancer-causing viral agents and their risks
Treatment efficacy based on early vs. late-stage cancer diagnosis
Cancer vaccines and targeted therapies
Immunotherapy for cancer
Pain medication alternatives
Historically, opioid medications were the primary treatment for short- and long-term pain. But, with the opioid epidemic getting worse, the need for alternative pain medications has never been more urgent.
Examples of pain medication-related research topics include:
Opioid withdrawal symptoms and risks
Early signs of pain medication misuse
Anti-inflammatory medications for pain control
- Identify trends in your medical research with Dovetail
Are you interested in contributing life-changing research? Today’s medical research is part of the future of clinical patient care.
As your go-to resource for speedy and accurate data analysis , we are proud to partner with healthcare researchers to innovate and improve the future of healthcare.
Get started today
Go from raw data to valuable insights with a flexible research platform
Editor’s picks
Last updated: 21 December 2023
Last updated: 16 December 2023
Last updated: 6 October 2023
Last updated: 5 March 2024
Last updated: 25 November 2023
Last updated: 15 February 2024
Last updated: 11 March 2024
Last updated: 12 December 2023
Last updated: 6 March 2024
Last updated: 10 April 2023
Last updated: 20 December 2023
Latest articles
Related topics, log in or sign up.
Get started for free
Healthcare Research Paper Topics
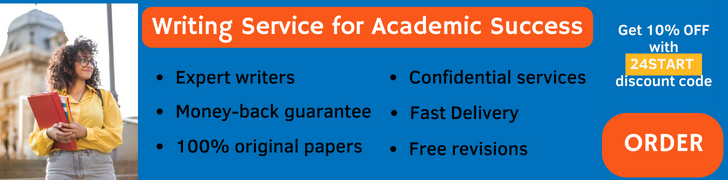
In this page, we provide a comprehensive list of healthcare research paper topics , expert advice on selecting compelling topics, guidance on writing an impactful research paper, and information about iResearchNet’s writing services. By exploring these resources, students in the health sciences field can choose relevant and significant healthcare research paper topics, develop their papers effectively, and access professional writing assistance to excel in their academic endeavors.
100 Healthcare Research Paper Topics
The field of healthcare research encompasses a vast array of topics that are crucial for understanding, improving, and transforming healthcare practices. As students in the health sciences, you have the opportunity to explore these diverse areas and contribute to the knowledge base of healthcare research. This comprehensive list aims to inspire and guide you in selecting healthcare research paper topics that align with your interests and academic goals. The topics are divided into ten distinct categories, each containing ten thought-provoking and relevant research ideas. Let this list serve as a springboard for your exploration and a catalyst for impactful research in the dynamic field of healthcare.
Academic Writing, Editing, Proofreading, And Problem Solving Services
Get 10% off with 24start discount code.
1. Healthcare Policy and Management
- The Impact of Health Policies on Access to Care
- Assessing the Effectiveness of Health Insurance Programs
- Analyzing the Role of Healthcare Leadership in Quality Improvement
- Exploring Strategies for Healthcare Cost Containment
- Investigating the Relationship Between Healthcare Regulations and Patient Outcomes
- Evaluating the Impact of Electronic Health Records on Healthcare Delivery
- Examining the Role of Public-Private Partnerships in Healthcare
- Analyzing the Influence of Political Factors on Healthcare Decision-Making
- Assessing the Ethical Implications of Resource Allocation in Healthcare
- Investigating the Effectiveness of Health Promotion Programs in Primary Care Settings
2. Healthcare Ethics and Legal Issues
- Analyzing the Ethical Challenges of Healthcare Research Involving Human Subjects
- Exploring the Impact of Cultural and Religious Beliefs on Healthcare Decision-Making
- Examining Legal Issues in End-of-Life Care and Advance Directives
- Investigating the Ethical Implications of Genetic Testing and Personalized Medicine
- Assessing the Ethical Dilemmas in Access to Experimental Treatments
- Exploring the Role of Ethics Committees in Healthcare Organizations
- Analyzing the Intersection of Healthcare Ethics and Artificial Intelligence
- Evaluating the Legal and Ethical Implications of Telemedicine
- Investigating the Ethics of Healthcare Resource Allocation during Public Health Emergencies
- Examining the Legal and Ethical Issues of Patient Privacy in the Digital Age
3. Healthcare Technology and Innovation
- Assessing the Impact of Artificial Intelligence in Healthcare Diagnostics
- Exploring the Potential of Wearable Devices for Remote Patient Monitoring
- Investigating the Role of Big Data Analytics in Healthcare Decision-Making
- Analyzing the Use of Robotics in Surgery and Patient Care
- Examining the Impact of Telehealth on Healthcare Access and Delivery
- Evaluating the Benefits and Challenges of Electronic Health Records Implementation
- Exploring the Applications of Virtual Reality in Healthcare Education and Training
- Investigating the Role of Mobile Health Applications in Health Behavior Change
- Assessing the Potential of Blockchain Technology in Healthcare Data Security
- Analyzing the Ethical and Social Implications of Genetic Engineering in Healthcare
4. Healthcare Quality and Patient Safety
- Evaluating the Impact of Patient-Centered Care on Health Outcomes
- Analyzing the Role of Quality Improvement Initiatives in Reducing Medical Errors
- Assessing the Effectiveness of Medication Safety Practices in Healthcare Settings
- Exploring Strategies to Improve Healthcare Communication and Interprofessional Collaboration
- Investigating the Relationship Between Nursing Workforce and Patient Safety
- Examining the Impact of Clinical Practice Guidelines on Healthcare Quality
- Analyzing the Role of Patient Engagement in Enhancing Healthcare Quality
- Evaluating the Effectiveness of Lean Six Sigma in Healthcare Process Improvement
- Exploring the Role of Health Information Technology in Enhancing Patient Safety
- Investigating the Influence of Organizational Culture on Healthcare Quality and Safety
5. Mental Health and Psychological Well-being
- Analyzing the Impact of Stigma on Mental Health Help-Seeking Behavior
- Exploring the Effectiveness of Psychotherapy Approaches in Treating Mental Health Disorders
- Assessing the Role of Early Intervention in Preventing Mental Health Disorders
- Investigating the Relationship Between Adverse Childhood Experiences and Mental Health Outcomes
- Examining the Intersection of Mental Health and Substance Abuse Disorders
- Evaluating the Impact of Mindfulness-Based Interventions on Psychological Well-being
- Exploring the Role of Social Support in Mental Health Recovery
- Analyzing the Effectiveness of Mental Health Awareness Campaigns
- Investigating the Influence of Cultural Factors on Mental Health Help-Seeking Behavior
- Examining the Mental Health Needs and Challenges among Specific Populations (e.g., LGBTQ+, Veterans, Refugees)
6. Chronic Diseases and their Management
- Assessing the Impact of Lifestyle Factors on Chronic Disease Prevention and Management
- Exploring the Role of Community-Based Interventions in Chronic Disease Control
- Investigating the Relationship Between Social Determinants of Health and Chronic Disease Burden
- Analyzing the Use of Digital Health Technologies in Chronic Disease Management
- Examining the Impact of Health Literacy on Chronic Disease Outcomes
- Evaluating the Effectiveness of Self-Management Programs for Chronic Conditions
- Exploring the Role of Healthcare Providers in Chronic Disease Prevention and Management
- Analyzing the Impact of Health Policies on Chronic Disease Prevention Efforts
- Investigating the Relationship Between Mental Health and Chronic Disease Management
- Examining the Disparities in Access to Chronic Disease Care and Treatment
7. Healthcare Disparities and Access to Care
- Analyzing Racial and Ethnic Disparities in Healthcare Access and Quality
- Exploring the Role of Socioeconomic Factors in Healthcare Disparities
- Assessing the Impact of Geographic Location on Healthcare Access and Health Outcomes
- Investigating Gender Disparities in Healthcare Utilization and Treatment
- Examining the Influence of Health Insurance Status on Healthcare Disparities
- Evaluating the Effectiveness of Culturally Competent Care in Reducing Disparities
- Exploring the Relationship Between Language Barriers and Healthcare Access
- Analyzing the Impact of Implicit Bias on Healthcare Disparities
- Investigating the Role of Health Literacy in Healthcare Disparities
- Examining the Disparities in Mental Health Services and Access to Mental Healthcare
8. Healthcare Education and Training
- Assessing the Effectiveness of Simulation-Based Training in Healthcare Education
- Exploring the Role of Interprofessional Education in Improving Collaborative Practice
- Investigating the Impact of Technology-Enhanced Learning in Healthcare Education
- Analyzing the Use of Gamification in Healthcare Training and Skill Development
- Examining the Role of Continuing Education in Enhancing Healthcare Providers’ Competence
- Evaluating the Effectiveness of Mentorship Programs in Healthcare Education
- Exploring Strategies to Address Cultural Competence in Healthcare Education
- Analyzing the Role of Reflective Practice in Healthcare Professional Development
- Investigating the Use of Team-Based Learning in Healthcare Education
- Examining the Impact of Experiential Learning in Healthcare Training Programs
9. Public Health and Preventive Medicine
- Assessing the Impact of Vaccination Programs on Public Health Outcomes
- Exploring the Role of Health Promotion Campaigns in Preventing Non-communicable Diseases
- Investigating the Effectiveness of Community-Based Interventions in Disease Prevention
- Analyzing the Impact of Environmental Factors on Public Health
- Examining the Role of Social Determinants of Health in Health Disparities
- Evaluating the Effectiveness of Public Health Policies in Tobacco Control
- Exploring Strategies for Preventing and Managing Infectious Diseases
- Analyzing the Role of Health Education in Promoting Healthy Lifestyles
- Investigating the Influence of Media on Public Health Perceptions and Behaviors
- Examining the Challenges and Opportunities in Global Health Initiatives
10. Emerging Topics in Healthcare Research
- Assessing the Implications of Artificial Intelligence in Healthcare
- Exploring the Role of Precision Medicine in Personalized Healthcare
- Investigating the Impact of Genomic Research on Healthcare Delivery
- Analyzing the Use of Telemedicine in Rural and Underserved Areas
- Examining the Integration of Traditional and Complementary Medicine in Healthcare
- Evaluating the Potential of Digital Therapeutics in Disease Management
- Exploring the Ethical Considerations of Gene Editing Technologies in Healthcare
- Analyzing the Influence of Social Media on Healthcare Decision-Making
- Investigating the Role of Health Information Exchange in Coordinated Care
- Examining the Implications of Health Equity in Healthcare Research and Practice
This comprehensive list of healthcare research paper topics encompasses a wide range of areas within the healthcare field. Each category offers diverse research ideas that can inspire students in the health sciences to explore pressing issues, propose innovative solutions, and contribute to the advancement of healthcare knowledge. Whether you are interested in healthcare policy, ethics, technology, mental health, chronic diseases, healthcare disparities, education, public health, or emerging healthcare research paper topics, this list serves as a valuable resource to kickstart your research journey. Choose a topic that resonates with you, aligns with your academic goals, and enables you to make a meaningful impact in the field of healthcare research. Remember, the pursuit of knowledge and the drive to improve healthcare practices are at the heart of your journey as a student in the health sciences.
Choosing Healthcare Research Paper Topics
Choosing the right healthcare research paper topic is a crucial step in conducting a successful and impactful study. With the vast array of healthcare issues and areas to explore, it can be challenging to narrow down your focus. To help you navigate this process effectively, we have compiled expert advice and ten essential tips for selecting compelling healthcare research paper topics. Consider these insights as you embark on your research journey in the dynamic field of healthcare:
- Follow Your Passion : Choose a topic that genuinely interests you. Passion and enthusiasm will drive your motivation, ensuring that you remain engaged throughout the research process.
- Stay Informed : Keep up with the latest healthcare trends, emerging issues, and ongoing debates. Stay informed through reputable sources, academic journals, conferences, and professional networks to identify current and relevant research gaps.
- Identify a Research Gap : Conduct a thorough literature review to identify areas where there is a need for further research. Look for unanswered questions, controversies, or gaps in knowledge that you can address in your study.
- Consider Relevance and Significance : Choose a topic that is relevant to current healthcare challenges or contributes to improving healthcare practices, policies, or patient outcomes. Aim for a topic that has real-world implications and societal impact.
- Delve into Specific Areas : Narrow down your focus by selecting a specific aspect or subtopic within the broad field of healthcare. This allows for a more focused and in-depth analysis of the chosen area.
- Consult with Your Advisor or Faculty : Seek guidance from your research advisor or faculty members who specialize in healthcare research. They can provide valuable insights, help you refine your topic, and direct you to relevant literature and resources.
- Brainstorm with Peers : Engage in discussions with your peers and classmates to explore different perspectives and gain inspiration. Collaborative brainstorming sessions can generate new ideas and offer fresh insights.
- Consider Ethical Considerations : Take ethical considerations into account when selecting a healthcare research topic. Ensure that your research adheres to ethical guidelines and respects the rights and privacy of participants, especially in studies involving human subjects.
- Think Interdisciplinary : Consider interdisciplinary approaches to healthcare research. Explore how other disciplines, such as sociology, psychology, economics, or technology, intersect with healthcare, providing a broader perspective and enhancing the depth of your research.
- Feasibility and Available Resources : Assess the feasibility of your chosen topic, considering the resources, time, and data availability required for your research. Ensure that you have access to relevant data sources, research tools, and necessary support to carry out your study effectively.
By following these expert tips, you will be equipped to choose a healthcare research paper topic that aligns with your interests, is relevant to current healthcare challenges, and has the potential to make a meaningful impact in the field. Remember, selecting the right topic sets the foundation for a successful research endeavor, allowing you to contribute to the advancement of healthcare knowledge and practices.
How to Write a Healthcare Research Paper
Writing a healthcare research paper requires careful planning, organization, and attention to detail. To help you navigate the intricacies of the writing process, we have compiled ten essential tips to guide you towards crafting a well-written and impactful healthcare research paper. Follow these expert recommendations to enhance the quality and effectiveness of your research paper:
- Develop a Clear Research Question : Start by formulating a clear and concise research question that will serve as the central focus of your paper. Ensure that your question is specific, measurable, achievable, relevant, and time-bound (SMART).
- Conduct a Thorough Literature Review : Before diving into your research, conduct a comprehensive literature review to familiarize yourself with existing knowledge on the topic. Identify key theories, concepts, methodologies, and gaps in the literature that your research aims to address.
- Create a Solid Research Design : Design a robust research plan that aligns with your research question. Define your study population, sampling strategy, data collection methods, and statistical analyses. A well-designed research plan enhances the validity and reliability of your findings.
- Collect and Analyze Data : Implement your data collection methods, ensuring ethical considerations and adherence to research protocols. Once collected, analyze the data using appropriate statistical techniques and tools. Provide a clear description of your analytical methods.
- Structure your Paper Effectively : Organize your research paper into logical sections, including an introduction, literature review, methodology, results, discussion, and conclusion. Use headings and subheadings to enhance readability and guide the reader through your paper.
- Write a Compelling Introduction : Start your paper with a strong introduction that captures the reader’s attention and provides a concise overview of the research topic, objectives, and significance. Clearly state your research question and the rationale for your study.
- Present Clear and Concise Results : Present your research findings in a clear and concise manner. Use tables, graphs, and figures where appropriate to enhance the readability of your results. Provide a comprehensive interpretation of the results, highlighting key findings and their implications.
- Engage in Critical Analysis and Discussion : Analyze and interpret your findings in the context of existing literature. Discuss the strengths and limitations of your study, addressing potential biases or confounders. Consider alternative explanations and provide a thoughtful discussion of the implications of your findings.
- Follow Proper Citation and Referencing Guidelines : Adhere to the appropriate citation style (such as APA, MLA, or Chicago) consistently throughout your paper. Cite all sources accurately and include a comprehensive list of references at the end of your paper.
- Revise and Edit : Before finalizing your research paper, revise and edit it thoroughly. Pay attention to clarity, coherence, grammar, spelling, and punctuation. Ensure that your arguments flow logically and that your paper is well-structured and cohesive.
By following these tips, you will be well-equipped to write a high-quality healthcare research paper that effectively communicates your findings, contributes to the existing knowledge in the field, and engages readers with your insights and conclusions. Remember to seek feedback from your peers, professors, or research advisors to further refine your paper and ensure its overall excellence.
iResearchNet’s Custom Writing Services
At iResearchNet, we understand the challenges students face when it comes to writing healthcare research papers. To support you in your academic journey and ensure the highest quality of your work, we offer a comprehensive range of writing services. With a team of expert degree-holding writers and a commitment to excellence, we are dedicated to providing customized solutions tailored to your specific needs. Here are the features that set our writing services apart:
- Expert Degree-Holding Writers : Our team consists of highly qualified writers with advanced degrees in healthcare and related fields. They possess in-depth knowledge and expertise in various areas of healthcare, ensuring that your research paper is handled by professionals with subject matter expertise.
- Custom Written Works : We understand the importance of originality and uniqueness in academic writing. Our writers craft each research paper from scratch, tailoring it to your specific requirements and ensuring that it is entirely original and plagiarism-free.
- In-Depth Research : Our writers are skilled in conducting extensive research using reputable sources. They delve deep into the literature to gather the most relevant and up-to-date information, providing a solid foundation for your research paper.
- Custom Formatting : We offer custom formatting options to meet the specific guidelines of your institution and chosen citation style. Whether it’s APA, MLA, Chicago/Turabian, Harvard, or any other formatting style, our writers are well-versed in the intricacies of each.
- Top Quality : We are committed to delivering research papers of the highest quality. Our writers follow strict quality control measures to ensure that your paper meets the academic standards, including proper structure, clarity of writing, and logical flow of ideas.
- Customized Solutions : We recognize that every research paper is unique. Our writers work closely with you to understand your research objectives, guidelines, and preferences. They tailor their approach to ensure that your research paper reflects your vision and academic goals.
- Flexible Pricing : We offer flexible pricing options to accommodate students’ budgets. We understand the financial constraints students often face, and we strive to provide competitive and affordable pricing for our writing services.
- Short Deadlines : We understand that time is often a critical factor. We offer short turnaround times, allowing you to meet tight deadlines without compromising the quality of your research paper. With our dedicated team, we can handle urgent requests efficiently.
- Timely Delivery : We prioritize timely delivery to ensure that you receive your research paper well before your deadline. We understand the importance of submitting your work on time and offer our commitment to punctuality.
- 24/7 Support : Our customer support team is available 24/7 to assist you with any inquiries or concerns you may have. We are here to provide prompt and helpful assistance at any stage of the writing process.
- Absolute Privacy : We value your privacy and confidentiality. We have strict measures in place to protect your personal information and ensure that your identity remains anonymous throughout the process.
- Easy Order Tracking : We provide a user-friendly platform that allows you to track the progress of your order. You can stay updated on the status of your research paper and communicate directly with your assigned writer.
- Money-Back Guarantee : We are confident in the quality of our writing services. In the rare event that you are not satisfied with the final product, we offer a money-back guarantee, ensuring your peace of mind and commitment to your satisfaction.
At iResearchNet, we are dedicated to your success. We strive to exceed your expectations and provide you with a seamless and exceptional experience. Trust us with your healthcare research paper and let our expert writers bring your ideas to life with professionalism, accuracy, and academic excellence.
Customized Solutions for Your Research Needs
Are you a health sciences student in search of professional assistance for your healthcare research paper? Look no further than iResearchNet. We are here to empower your academic journey and help you excel in your research endeavors. With our comprehensive writing services and commitment to excellence, we provide the necessary tools and expert guidance to ensure your success.
At iResearchNet, we understand the unique challenges that come with writing healthcare research papers. Our team of expert degree-holding writers specializes in the health sciences field, allowing us to deliver customized solutions tailored to your specific research needs. Whether you need assistance in selecting a research topic, conducting in-depth literature reviews, analyzing data, or crafting a well-structured paper, we have the expertise to guide you every step of the way.
Take the next step in your healthcare research journey and unlock your academic potential with iResearchNet. Order your custom healthcare research paper today and let our expert writers bring your ideas to life with professionalism, accuracy, and academic excellence. Trust us to provide you with the guidance and support you need to achieve your research goals and make a meaningful impact in the field of healthcare.
ORDER HIGH QUALITY CUSTOM PAPER

- Research article
- Open access
- Published: 10 April 2021
The role of artificial intelligence in healthcare: a structured literature review
- Silvana Secinaro 1 ,
- Davide Calandra 1 ,
- Aurelio Secinaro 2 ,
- Vivek Muthurangu 3 &
- Paolo Biancone 1
BMC Medical Informatics and Decision Making volume 21 , Article number: 125 ( 2021 ) Cite this article
139k Accesses
236 Citations
24 Altmetric
Metrics details
Background/Introduction
Artificial intelligence (AI) in the healthcare sector is receiving attention from researchers and health professionals. Few previous studies have investigated this topic from a multi-disciplinary perspective, including accounting, business and management, decision sciences and health professions.
The structured literature review with its reliable and replicable research protocol allowed the researchers to extract 288 peer-reviewed papers from Scopus. The authors used qualitative and quantitative variables to analyse authors, journals, keywords, and collaboration networks among researchers. Additionally, the paper benefited from the Bibliometrix R software package.
The investigation showed that the literature in this field is emerging. It focuses on health services management, predictive medicine, patient data and diagnostics, and clinical decision-making. The United States, China, and the United Kingdom contributed the highest number of studies. Keyword analysis revealed that AI can support physicians in making a diagnosis, predicting the spread of diseases and customising treatment paths.
Conclusions
The literature reveals several AI applications for health services and a stream of research that has not fully been covered. For instance, AI projects require skills and data quality awareness for data-intensive analysis and knowledge-based management. Insights can help researchers and health professionals understand and address future research on AI in the healthcare field.
Peer Review reports
Artificial intelligence (AI) generally applies to computational technologies that emulate mechanisms assisted by human intelligence, such as thought, deep learning, adaptation, engagement, and sensory understanding [ 1 , 2 ]. Some devices can execute a role that typically involves human interpretation and decision-making [ 3 , 4 ]. These techniques have an interdisciplinary approach and can be applied to different fields, such as medicine and health. AI has been involved in medicine since as early as the 1950s, when physicians made the first attempts to improve their diagnoses using computer-aided programs [ 5 , 6 ]. Interest and advances in medical AI applications have surged in recent years due to the substantially enhanced computing power of modern computers and the vast amount of digital data available for collection and utilisation [ 7 ]. AI is gradually changing medical practice. There are several AI applications in medicine that can be used in a variety of medical fields, such as clinical, diagnostic, rehabilitative, surgical, and predictive practices. Another critical area of medicine where AI is making an impact is clinical decision-making and disease diagnosis. AI technologies can ingest, analyse, and report large volumes of data across different modalities to detect disease and guide clinical decisions [ 3 , 8 ]. AI applications can deal with the vast amount of data produced in medicine and find new information that would otherwise remain hidden in the mass of medical big data [ 9 , 10 , 11 ]. These technologies can also identify new drugs for health services management and patient care treatments [ 5 , 6 ].
Courage in the application of AI is visible through a search in the primary research databases. However, as Meskò et al. [ 7 ] find, the technology will potentially reduce care costs and repetitive operations by focusing the medical profession on critical thinking and clinical creativity. As Cho et al. and Doyle et al. [ 8 , 9 ] add, the AI perspective is exciting; however, new studies will be needed to establish the efficacy and applications of AI in the medical field [ 10 ].
Our paper will also concentrate on AI strategies for healthcare from the accounting, business, and management perspectives. The authors used the structured literature review (SLR) method for its reliable and replicable research protocol [ 11 ] and selected bibliometric variables as sources of investigation. Bibliometric usage enables the recognition of the main quantitative variables of the study stream [ 12 ]. This method facilitates the detection of the required details of a particular research subject, including field authors, number of publications, keywords for interaction between variables (policies, properties and governance) and country data [ 13 ]. It also allows the application of the science mapping technique [ 14 ]. Our paper adopted the Bibliometrix R package and the biblioshiny web interface as tools of analysis [ 14 ].
The investigation offers the following insights for future researchers and practitioners:
bibliometric information on 288 peer-reviewed English papers from the Scopus collection.
Identification of leading journals in this field, such as Journal of Medical Systems, Studies in Health Technology and Informatics, IEEE Journal of Biomedical and Health Informatics, and Decision Support Systems.
Qualitative and quantitative information on authors’ Lotka’s law, h-index, g-index, m-index, keyword, and citation data.
Research on specific countries to assess AI in the delivery and effectiveness of healthcare, quotes, and networks within each region.
A topic dendrogram study that identifies five research clusters: health services management, predictive medicine, patient data, diagnostics, and finally, clinical decision-making.
An in-depth discussion that develops theoretical and practical implications for future studies.
The paper is organised as follows. Section 2 lists the main bibliometric articles in this field. Section 3 elaborates on the methodology. Section 4 presents the findings of the bibliometric analysis. Section 5 discusses the main elements of AI in healthcare based on the study results. Section 6 concludes the article with future implications for research.
Related works and originality
As suggested by Zupic and Čater [ 15 ], a research stream can be evaluated with bibliometric methods that can introduce objectivity and mitigate researcher bias. For this reason, bibliometric methods are attracting increasing interest among researchers as a reliable and impersonal research analytical approach [ 16 , 17 ]. Recently, bibliometrics has been an essential method for analysing and predicting research trends [ 18 ]. Table 1 lists other research that has used a similar approach in the research stream investigated.
The scientific articles reported show substantial differences in keywords and research topics that have been previously studied. The bibliometric analysis of Huang et al. [ 19 ] describes rehabilitative medicine using virtual reality technology. According to the authors, the primary goal of rehabilitation is to enhance and restore functional ability and quality of life for patients with physical impairments or disabilities. In recent years, many healthcare disciplines have been privileged to access various technologies that provide tools for both research and clinical intervention.
Hao et al. [ 20 ] focus on text mining in medical research. As reported, text mining reveals new, previously unknown information by using a computer to automatically extract information from different text resources. Text mining methods can be regarded as an extension of data mining to text data. Text mining is playing an increasingly significant role in processing medical information. Similarly, the studies by dos Santos et al. [ 21 ] focus on applying data mining and machine learning (ML) techniques to public health problems. As stated in this research, public health may be defined as the art and science of preventing diseases, promoting health, and prolonging life. Using data mining and ML techniques, it is possible to discover new information that otherwise would be hidden. These two studies are related to another topic: medical big data. According to Liao et al. [ 22 ], big data is a typical “buzzword” in the business and research community, referring to a great mass of digital data collected from various sources. In the medical field, we can obtain a vast amount of data (i.e., medical big data). Data mining and ML techniques can help deal with this information and provide helpful insights for physicians and patients. More recently, Choudhury et al. [ 23 ] provide a systematic review on the use of ML to improve the care of elderly patients, demonstrating eligible studies primarily in psychological disorders and eye diseases.
Tran et al. [ 2 ] focus on the global evolution of AI research in medicine. Their bibliometric analysis highlights trends and topics related to AI applications and techniques. As stated in Connelly et al.’s [ 24 ] study, robot-assisted surgeries have rapidly increased in recent years. Their bibliometric analysis demonstrates how robotic-assisted surgery has gained acceptance in different medical fields, such as urological, colorectal, cardiothoracic, orthopaedic, maxillofacial and neurosurgery applications. Additionally, the bibliometric analysis of Guo et al. [ 25 ] provides an in-depth study of AI publications through December 2019. The paper focuses on tangible AI health applications, giving researchers an idea of how algorithms can help doctors and nurses. A new stream of research related to AI is also emerging. In this sense, Choudhury and Asan’s [ 26 ] scientific contribution provides a systematic review of the AI literature to identify health risks for patients. They report on 53 studies involving technology for clinical alerts, clinical reports, and drug safety. Considering the considerable interest within this research stream, this analysis differs from the current literature for several reasons. It aims to provide in-depth discussion, considering mainly the business, management, and accounting fields and not dealing only with medical and health profession publications.
Additionally, our analysis aims to provide a bibliometric analysis of variables such as authors, countries, citations and keywords to guide future research perspectives for researchers and practitioners, as similar analyses have done for several publications in other research streams [ 15 , 16 , 27 ]. In doing so, we use a different database, Scopus, that is typically adopted in social sciences fields. Finally, our analysis will propose and discuss a dominant framework of variables in this field, and our analysis will not be limited to AI application descriptions.
Methodology
This paper evaluated AI in healthcare research streams using the SLR method [ 11 ]. As suggested by Massaro et al. [ 11 ], an SLR enables the study of the scientific corpus of a research field, including the scientific rigour, reliability and replicability of operations carried out by researchers. As suggested by many scholars, the methodology allows qualitative and quantitative variables to highlight the best authors, journals and keywords and combine a systematic literature review and bibliometric analysis [ 27 , 28 , 29 , 30 ]. Despite its widespread use in business and management [ 16 , 31 ], the SLR is also used in the health sector based on the same philosophy through which it was originally conceived [ 32 , 33 ]. A methodological analysis of previously published articles reveals that the most frequently used steps are as follows [ 28 , 31 , 34 ]:
defining research questions;
writing the research protocol;
defining the research sample to be analysed;
developing codes for analysis; and
critically analysing, discussing, and identifying a future research agenda.
Considering the above premises, the authors believe that an SLR is the best method because it combines scientific validity, replicability of the research protocol and connection between multiple inputs.
As stated by the methodological paper, the first step is research question identification. For this purpose, we benefit from the analysis of Zupic and Čater [ 15 ], who provide several research questions for future researchers to link the study of authors, journals, keywords and citations. Therefore, RQ1 is “What are the most prominent authors, journal keywords and citations in the field of the research study?” Additionally, as suggested by Haleem et al. [ 35 ], new technologies, including AI, are changing the medical field in unexpected timeframes, requiring studies in multiple areas. Therefore, RQ2 is “How does artificial intelligence relate to healthcare, and what is the focus of the literature?” Then, as discussed by Massaro et al. [ 36 ], RQ3 is “What are the research applications of artificial intelligence for healthcare?”.
The first research question aims to define the qualitative and quantitative variables of the knowledge flow under investigation. The second research question seeks to determine the state of the art and applications of AI in healthcare. Finally, the third research question aims to help researchers identify practical and theoretical implications and future research ideas in this field.
The second fundamental step of the SLR is writing the research protocol [ 11 ]. Table 2 indicates the currently known literature elements, uniquely identifying the research focus, motivations and research strategy adopted and the results providing a link with the following points. Additionally, to strengthen the analysis, our investigation benefits from the PRISMA statement methodological article [ 37 ]. Although the SLR is a validated method for systematic reviews and meta-analyses, we believe that the workflow provided may benefit the replicability of the results [ 37 , 38 , 39 , 40 ]. Figure 1 summarises the researchers’ research steps, indicating that there are no results that can be referred to as a meta-analysis.
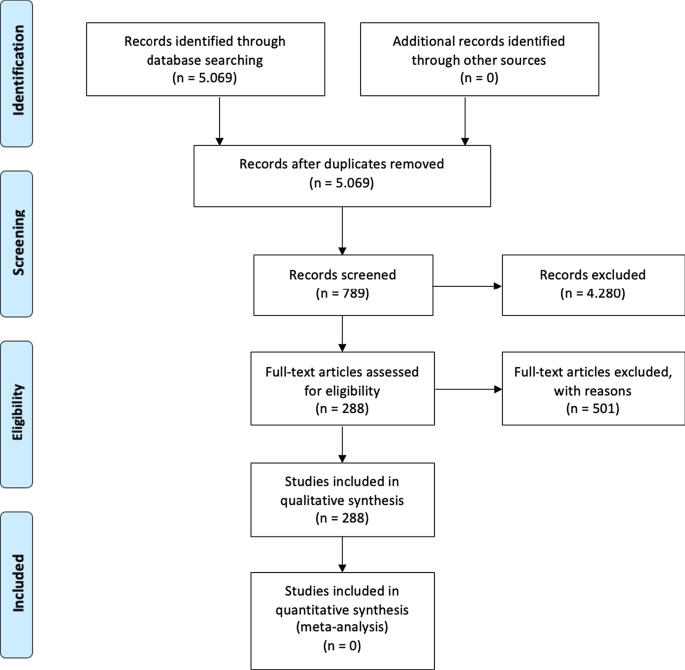
Source : Authors’ elaboration on Liberati et al. [ 37 ]
PRISMA workflow.
The third step is to specify the search strategy and search database. Our analysis is based on the search string “Artificial Intelligence” OR “AI” AND “Healthcare” with a focus on “Business, Management, and Accounting”, “Decision Sciences”, and “Health professions”. As suggested by [ 11 , 41 ] and motivated by [ 42 ], keywords can be selected through a top-down approach by identifying a large search field and then focusing on particular sub-topics. The paper uses data retrieved from the Scopus database, a multi-disciplinary database, which allowed the researchers to identify critical articles for scientific analysis [ 43 ]. Additionally, Scopus was selected based on Guo et al.’s [ 25 ] limitations, which suggest that “future studies will apply other databases, such as Scopus, to explore more potential papers” . The research focuses on articles and reviews published in peer-reviewed journals for their scientific relevance [ 11 , 16 , 17 , 29 ] and does not include the grey literature, conference proceedings or books/book chapters. Articles written in any language other than English were excluded [ 2 ]. For transparency and replicability, the analysis was conducted on 11 January 2021. Using this research strategy, the authors retrieved 288 articles. To strengthen the study's reliability, we publicly provide the full bibliometric extract on the Zenodo repository [ 44 , 45 ].
The fourth research phase is defining the code framework that initiates the analysis of the variables. The study will identify the following:
descriptive information of the research area;
source analysis [ 16 ];
author and citation analysis [ 28 ];
keywords and network analysis [ 14 ]; and
geographic distribution of the papers [ 14 ].
The final research phase is the article’s discussion and conclusion, where implications and future research trends will be identified.
At the research team level, the information is analysed with the statistical software R-Studio and the Bibliometrix package [ 15 ], which allows scientific analysis of the results obtained through the multi-disciplinary database.
The analysis of bibliometric results starts with a description of the main bibliometric statistics with the aim of answering RQ1, What are the most prominent authors, journal keywords and citations in the field of the research study?, and RQ2, How does artificial intelligence relate to healthcare, and what is the focus of the literature? Therefore, the following elements were thoroughly analysed: (1) type of document; (2) annual scientific production; (3) scientific sources; (4) source growth; (5) number of articles per author; (6) author’s dominance ranking; (7) author’s h-index, g-index, and m-index; (8) author’s productivity; (9) author’s keywords; (10) topic dendrogram; (11) a factorial map of the document with the highest contributions; (12) article citations; (13) country production; (14) country citations; (15) country collaboration map; and (16) country collaboration network.
Main information
Table 3 shows the information on 288 peer-reviewed articles published between 1992 and January 2021 extracted from the Scopus database. The number of keywords is 946 from 136 sources, and the number of keywords plus, referring to the number of keywords that frequently appear in an article’s title, was 2329. The analysis period covered 28 years and 1 month of scientific production and included an annual growth rate of 5.12%. However, the most significant increase in published articles occurred in the past three years (please see Fig. 2 ). On average, each article was written by three authors (3.56). Finally, the collaboration index (CI), which was calculated as the total number of authors of multi-authored articles/total number of multi-authored articles, was 3.97 [ 46 ].
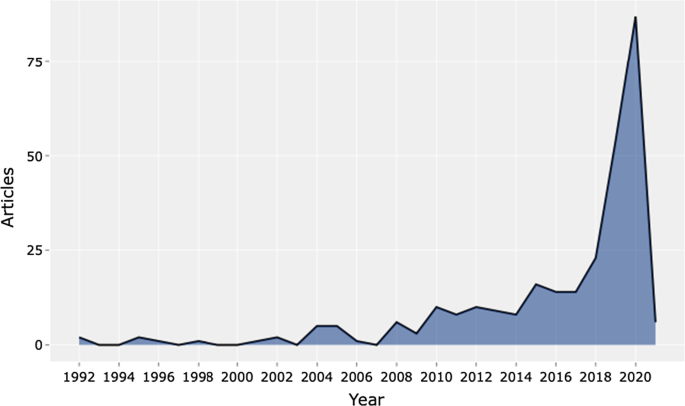
Source : Authors’ elaboration
Annual scientific production.
Table 4 shows the top 20 sources related to the topic. The Journal of Medical Systems is the most relevant source, with twenty-one of the published articles. This journal's main issues are the foundations, functionality, interfaces, implementation, impacts, and evaluation of medical technologies. Another relevant source is Studies in Health Technology and Informatics, with eleven articles. This journal aims to extend scientific knowledge related to biomedical technologies and medical informatics research. Both journals deal with cloud computing, machine learning, and AI as a disruptive healthcare paradigm based on recent publications. The IEEE Journal of Biomedical and Health Informatics investigates technologies in health care, life sciences, and biomedicine applications from a broad perspective. The next journal, Decision Support Systems, aims to analyse how these technologies support decision-making from a multi-disciplinary view, considering business and management. Therefore, the analysis of the journals revealed that we are dealing with an interdisciplinary research field. This conclusion is confirmed, for example, by the presence of purely medical journals, journals dedicated to the technological growth of healthcare, and journals with a long-term perspective such as futures.
The distribution frequency of the articles (Fig. 3 ) indicates the journals dealing with the topic and related issues. Between 2008 and 2012, a significant growth in the number of publications on the subject is noticeable. However, the graph shows the results of the Loess regression, which includes the quantity and publication time of the journal under analysis as variables. This method allows the function to assume an unlimited distribution; that is, feature can consider values below zero if the data are close to zero. It contributes to a better visual result and highlights the discontinuity in the publication periods [ 47 ].
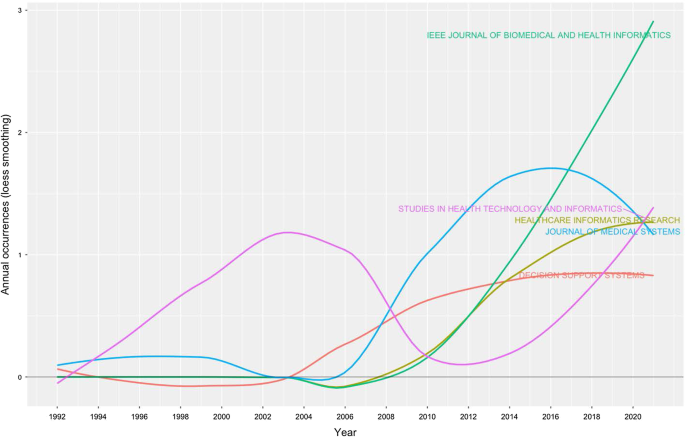
Source growth. Source : Authors’ elaboration
Finally, Fig. 4 provides an analytical perspective on factor analysis for the most cited papers. As indicated in the literature [ 48 , 49 ], using factor analysis to discover the most cited papers allows for a better understanding of the scientific world’s intellectual structure. For example, our research makes it possible to consider certain publications that effectively analyse subject specialisation. For instance, Santosh’s [ 50 ] article addresses the new paradigm of AI with ML algorithms for data analysis and decision support in the COVID-19 period, setting a benchmark in terms of citations by researchers. Moving on to the application, an article by Shickel et al. [ 51 ] begins with the belief that the healthcare world currently has much health and administrative data. In this context, AI and deep learning will support medical and administrative staff in extracting data, predicting outcomes, and learning medical representations. Finally, in the same line of research, Baig et al. [ 52 ], with a focus on wearable patient monitoring systems (WPMs), conclude that AI and deep learning may be landmarks for continuous patient monitoring and support for healthcare delivery.
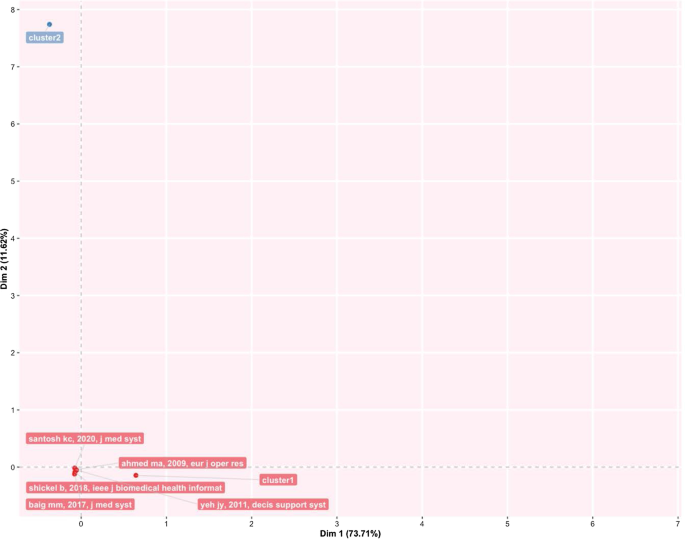
Factorial map of the most cited documents.
This section identifies the most cited authors of articles on AI in healthcare. It also identifies the authors’ keywords, dominance factor (DF) ranking, h-index, productivity, and total number of citations. Table 5 identifies the authors and their publications in the top 20 rankings. As the table shows, Bushko R.G. has the highest number of publications: four papers. He is the editor-in-chief of Future of Health Technology, a scientific journal that aims to develop a clear vision of the future of health technology. Then, several authors each wrote three papers. For instance, Liu C. is a researcher active in the topic of ML and computer vision, and Sharma A. from Emory University Atlanta in the USA is a researcher with a clear focus on imaging and translational informatics. Some other authors have two publications each. While some authors have published as primary authors, most have published as co-authors. Hence, in the next section, we measure the contributory power of each author by investigating the DF ranking through the number of elements.
Authors’ dominance ranking
The dominance factor (DF) is a ratio measuring the fraction of multi-authored articles in which an author acts as the first author [ 53 ]. Several bibliometric studies use the DF in their analyses [ 46 , 54 ]. The DF ranking calculates an author’s dominance in producing articles. The DF is calculated by dividing the number of an author’s multi-authored papers as the first author (Nmf) by the author's total number of multi-authored papers (Nmt). This is omitted in the single-author case due to the constant value of 1 for single-authored articles. This formulation could lead to some distortions in the results, especially in fields where the first author is entered by surname alphabetical order [ 55 ].
The mathematical equation for the DF is shown as:
Table 6 lists the top 20 DF rankings. The data in the table show a low level of articles per author, either for first-authored or multi-authored articles. The results demonstrate that we are dealing with an emerging topic in the literature. Additionally, as shown in the table, Fox J. and Longoni C. are the most dominant authors in the field.
Authors’ impact
Table 7 shows the impact of authors in terms of the h-index [ 56 ] (i.e., the productivity and impact of citations of a researcher), g-index [ 57 ] (i.e., the distribution of citations received by a researcher's publications), m-index [ 58 ] (i.e., the h-index value per year), total citations, total paper and years of scientific publication. The H-index was introduced in the literature as a metric for the objective comparison of scientific results and depended on the number of publications and their impact [ 59 ]. The results show that the 20 most relevant authors have an h-index between 2 and 1. For the practical interpretation of the data, the authors considered data published by the London School of Economics [ 60 ]. In the social sciences, the analysis shows values of 7.6 for economic publications by professors and researchers who had been active for several years. Therefore, the youthfulness of the research area has attracted young researchers and professors. At the same time, new indicators have emerged over the years to diversify the logic of the h-index. For example, the g-index indicates an author's impact on citations, considering that a single article can generate these. The m-index, on the other hand, shows the cumulative value over the years.
The analysis, also considering the total number of citations, the number of papers published and the year of starting to publish, thus confirms that we are facing an expanding research flow.
Authors’ productivity
Figure 5 shows Lotka’s law. This mathematical formulation originated in 1926 to describe the publication frequency by authors in a specific research field [ 61 ]. In practice, the law states that the number of authors contributing to research in a given period is a fraction of the number who make up a single contribution [ 14 , 61 ].
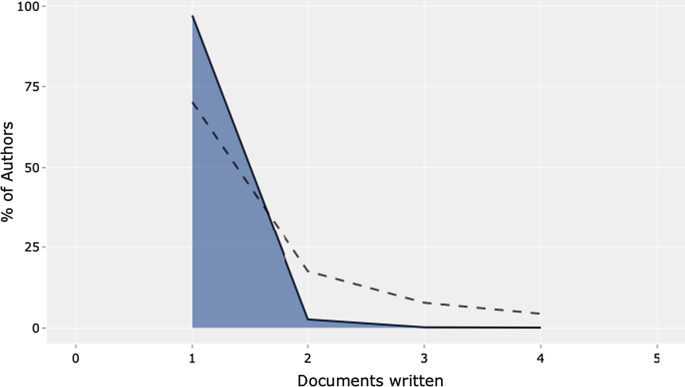
Lotka’s law.
The mathematical relationship is expressed in reverse in the following way:
where y x is equal to the number of authors producing x articles in each research field. Therefore, C and n are constants that can be estimated in the calculation.
The figure's results are in line with Lotka's results, with an average of two publications per author in a given research field. In addition, the figure shows the percentage of authors. Our results lead us to state that we are dealing with a young and growing research field, even with this analysis. Approximately 70% of the authors had published only their first research article. Only approximately 20% had published two scientific papers.
Authors’ keywords
This section provides information on the relationship between the keywords artificial intelligence and healthcare . This analysis is essential to determine the research trend, identify gaps in the discussion on AI in healthcare, and identify the fields that can be interesting as research areas [ 42 , 62 ].
Table 8 highlights the total number of keywords per author in the top 20 positions. The ranking is based on the following elements: healthcare, artificial intelligence, and clinical decision support system . Keyword analysis confirms the scientific area of reference. In particular, we deduce the definition as “Artificial intelligence is the theory and development of computer systems able to perform tasks normally requiring human intelligence, such as visual perception, speech recognition, decision-making, and translation between languages” [ 2 , 63 ]. Panch et al. [ 4 ] find that these technologies can be used in different business and management areas. After the first keyword, the analysis reveals AI applications and related research such as machine learning and deep learning.
Additionally, data mining and big data are a step forward in implementing exciting AI applications. According to our specific interest, if we applied AI in healthcare, we would achieve technological applications to help and support doctors and medical researchers in decision-making. The link between AI and decision-making is the reason why we find, in the seventh position, the keyword clinical decision support system . AI techniques can unlock clinically relevant information hidden in the massive amount of data that can assist clinical decision-making [ 64 ]. If we analyse the following keywords, we find other elements related to decision-making and support systems.
The TreeMap below (Fig. 6 ) highlights the combination of possible keywords representing AI and healthcare.
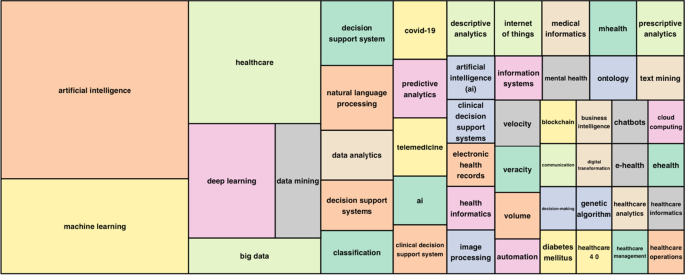
Keywords treemap.
The topic dendrogram in Fig. 7 represents the hierarchical order and the relationship between the keywords generated by hierarchical clustering [ 42 ]. The cut in the figure and the vertical lines facilitate an investigation and interpretation of the different clusters. As stated by Andrews [ 48 ], the figure is not intended to find the perfect level of associations between clusters. However, it aims to estimate the approximate number of clusters to facilitate further discussion.
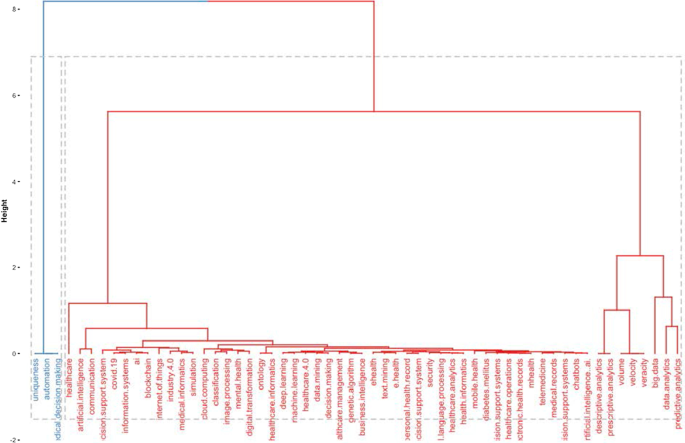
Topic dendrogram.
The research stream of AI in healthcare is divided into two main strands. The blue strand focuses on medical information systems and the internet. Some papers are related to healthcare organisations, such as the Internet of Things, meaning that healthcare organisations use AI to support health services management and data analysis. AI applications are also used to improve diagnostic and therapeutic accuracy and the overall clinical treatment process [ 2 ]. If we consider the second block, the red one, three different clusters highlight separate aspects of the topic. The first could be explained as AI and ML predictive algorithms. Through AI applications, it is possible to obtain a predictive approach that can ensure that patients are better monitored. This also allows a better understanding of risk perception for doctors and medical researchers. In the second cluster, the most frequent words are decisions , information system , and support system . This means that AI applications can support doctors and medical researchers in decision-making. Information coming from AI technologies can be used to consider difficult problems and support a more straightforward and rapid decision-making process. In the third cluster, it is vital to highlight that the ML model can deal with vast amounts of data. From those inputs, it can return outcomes that can optimise the work of healthcare organisations and scheduling of medical activities.
Furthermore, the word cloud in Fig. 8 highlights aspects of AI in healthcare, such as decision support systems, decision-making, health services management, learning systems, ML techniques and diseases. The figure depicts how AI is linked to healthcare and how it is used in medicine.
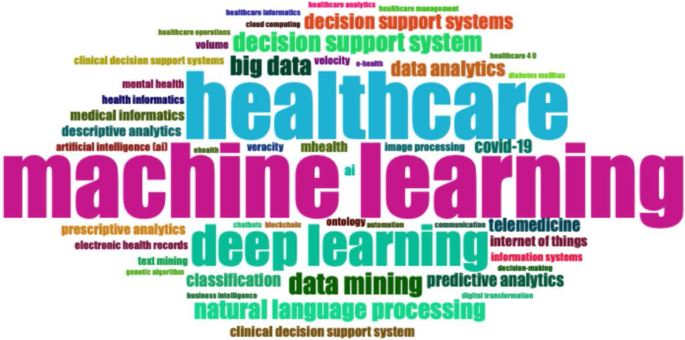
Word cloud.
Figure 9 represents the search trends based on the keywords analysed. The research started in 2012. First, it identified research topics related to clinical decision support systems. This topic was recurrent during the following years. Interestingly, in 2018, studies investigated AI and natural language processes as possible tools to manage patients and administrative elements. Finally, a new research stream considers AI's role in fighting COVID-19 [ 65 , 66 ].
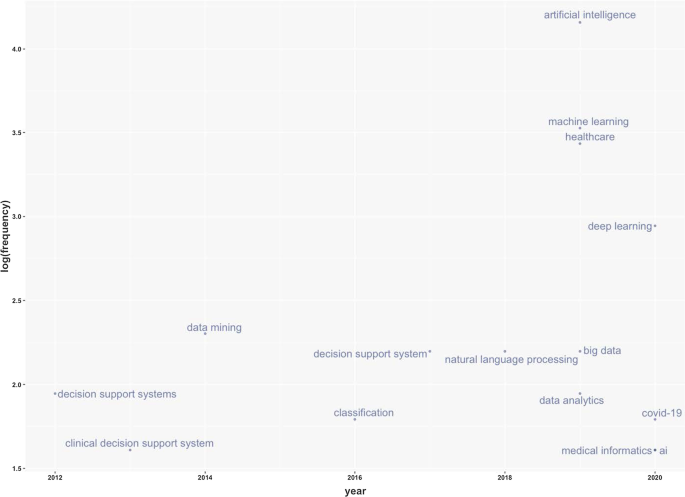
Keywords frequency.
Table 9 represents the number of citations from other articles within the top 20 rankings. The analysis allows the benchmark studies in the field to be identified [ 48 ]. For instance, Burke et al. [ 67 ] writes the most cited paper and analyses efficient nurse rostering methodologies. The paper critically evaluates tangible interdisciplinary solutions that also include AI. Immediately thereafter, Ahmed M.A.'s article proposes a data-driven optimisation methodology to determine the optimal number of healthcare staff to optimise patients' productivity [ 68 ]. Finally, the third most cited article lays the groundwork for developing deep learning by considering diverse health and administrative information [ 51 ].
This section analyses the diffusion of AI in healthcare around the world. It highlights countries to show the geographies of this research. It includes all published articles, the total number of citations, and the collaboration network. The following sub-sections start with an analysis of the total number of published articles.
Country total articles
Figure 9 and Table 10 display the countries where AI in healthcare has been considered. The USA tops the list of countries with the maximum number of articles on the topic (215). It is followed by China (83), the UK (54), India (51), Australia (54), and Canada (32). It is immediately evident that the theme has developed on different continents, highlighting a growing interest in AI in healthcare. The figure shows that many areas, such as Russia, Eastern Europe and Africa except for Algeria, Egypt, and Morocco, have still not engaged in this scientific debate.
Country publications and collaboration map
This section discusses articles on AI in healthcare in terms of single or multiple publications in each country. It also aims to observe collaboration and networking between countries. Table 11 and Fig. 10 highlight the average citations by state and show that the UK, the USA, and Kuwait have a higher average number of citations than other countries. Italy, Spain and New Zealand have the most significant number of citations.
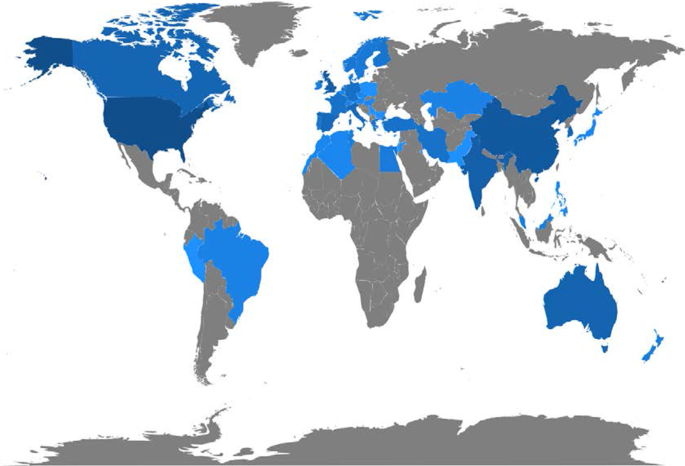
Articles per country.
Figure 11 depicts global collaborations. The blue colour on the map represents research cooperation among nations. Additionally, the pink border linking states indicates the extent of collaboration between authors. The primary cooperation between nations is between the USA and China, with two collaborative articles. Other collaborations among nations are limited to a few papers.
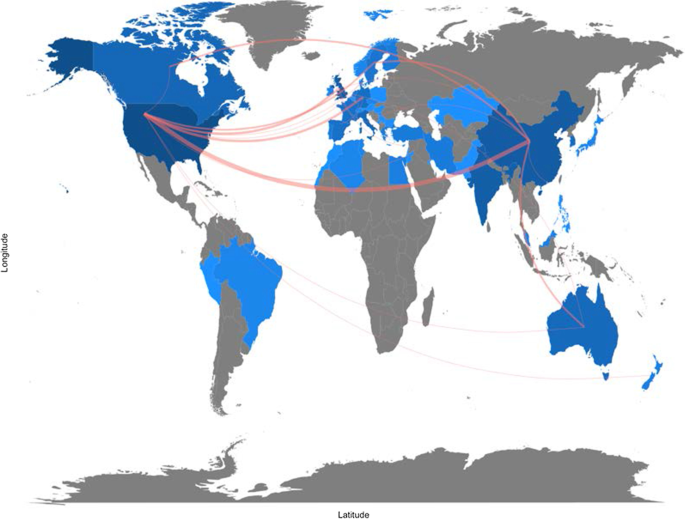
Collaboration map.
Artificial intelligence for healthcare: applications
This section aims to strengthen the research scope by answering RQ3: What are the research applications of artificial intelligence for healthcare?
Benefiting from the topical dendrogram, researchers will provide a development model based on four relevant variables [ 69 , 70 ]. AI has been a disruptive innovation in healthcare [ 4 ]. With its sophisticated algorithms and several applications, AI has assisted doctors and medical professionals in the domains of health information systems, geocoding health data, epidemic and syndromic surveillance, predictive modelling and decision support, and medical imaging [ 2 , 9 , 10 , 64 ]. Furthermore, the researchers considered the bibliometric analysis to identify four macro-variables dominant in the field and used them as authors' keywords. Therefore, the following sub-sections aim to explain the debate on applications in healthcare for AI techniques. These elements are shown in Fig. 12 .
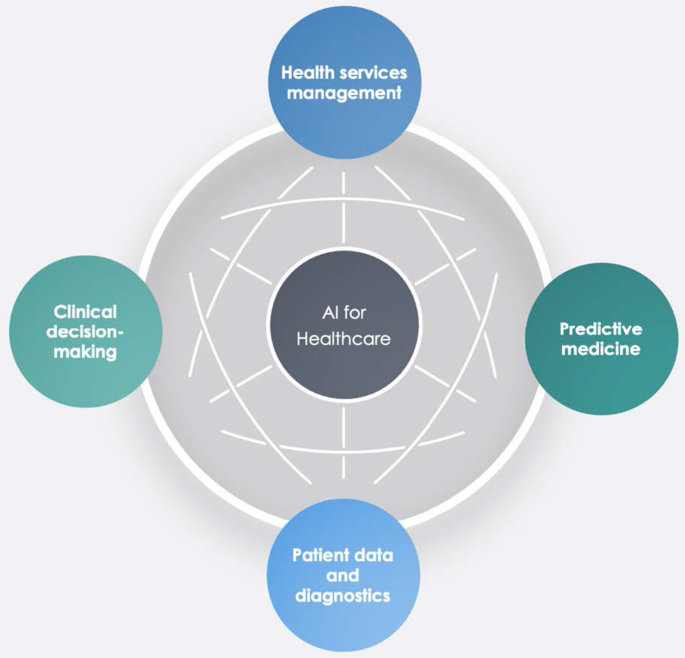
Dominant variables for AI in healthcare.
Health services management
One of the notable aspects of AI techniques is potential support for comprehensive health services management. These applications can support doctors, nurses and administrators in their work. For instance, an AI system can provide health professionals with constant, possibly real-time medical information updates from various sources, including journals, textbooks, and clinical practices [ 2 , 10 ]. These applications' strength is becoming even more critical in the COVID-19 period, during which information exchange is continually needed to properly manage the pandemic worldwide [ 71 ]. Other applications involve coordinating information tools for patients and enabling appropriate inferences for health risk alerts and health outcome prediction [ 72 ]. AI applications allow, for example, hospitals and all health services to work more efficiently for the following reasons:
Clinicians can access data immediately when they need it.
Nurses can ensure better patient safety while administering medication.
Patients can stay informed and engaged in their care by communicating with their medical teams during hospital stays.
Additionally, AI can contribute to optimising logistics processes, for instance, realising drugs and equipment in a just-in-time supply system based totally on predictive algorithms [ 73 , 74 ]. Interesting applications can also support the training of personnel working in health services. This evidence could be helpful in bridging the gap between urban and rural health services [ 75 ]. Finally, health services management could benefit from AI to leverage the multiplicity of data in electronic health records by predicting data heterogeneity across hospitals and outpatient clinics, checking for outliers, performing clinical tests on the data, unifying patient representation, improving future models that can predict diagnostic tests and analyses, and creating transparency with benchmark data for analysing services delivered [ 51 , 76 ].
Predictive medicine
Another relevant topic is AI applications for disease prediction and diagnosis treatment, outcome prediction and prognosis evaluation [ 72 , 77 ]. Because AI can identify meaningful relationships in raw data, it can support diagnostic, treatment and prediction outcomes in many medical situations [ 64 ]. It allows medical professionals to embrace the proactive management of disease onset. Additionally, predictions are possible for identifying risk factors and drivers for each patient to help target healthcare interventions for better outcomes [ 3 ]. AI techniques can also help design and develop new drugs, monitor patients and personalise patient treatment plans [ 78 ]. Doctors benefit from having more time and concise data to make better patient decisions. Automatic learning through AI could disrupt medicine, allowing prediction models to be created for drugs and exams that monitor patients over their whole lives [ 79 ].
- Clinical decision-making
One of the keyword analysis main topics is that AI applications could support doctors and medical researchers in the clinical decision-making process. According to Jiang et al. [ 64 ], AI can help physicians make better clinical decisions or even replace human judgement in healthcare-specific functional areas. According to Bennett and Hauser [ 80 ], algorithms can benefit clinical decisions by accelerating the process and the amount of care provided, positively impacting the cost of health services. Therefore, AI technologies can support medical professionals in their activities and simplify their jobs [ 4 ]. Finally, as Redondo and Sandoval [ 81 ] find, algorithmic platforms can provide virtual assistance to help doctors understand the semantics of language and learning to solve business process queries as a human being would.
Patient data and diagnostics
Another challenging topic related to AI applications is patient data and diagnostics. AI techniques can help medical researchers deal with the vast amount of data from patients (i.e., medical big data ). AI systems can manage data generated from clinical activities, such as screening, diagnosis, and treatment assignment. In this way, health personnel can learn similar subjects and associations between subject features and outcomes of interest [ 64 ].
These technologies can analyse raw data and provide helpful insights that can be used in patient treatments. They can help doctors in the diagnostic process; for example, to realise a high-speed body scan, it will be simpler to have an overall patient condition image. Then, AI technology can recreate a 3D mapping solution of a patient’s body.
In terms of data, interesting research perspectives are emerging. For instance, we observed the emergence of a stream of research on patient data management and protection related to AI applications [ 82 ].
For diagnostics, AI techniques can make a difference in rehabilitation therapy and surgery. Numerous robots have been designed to support and manage such tasks. Rehabilitation robots physically support and guide, for example, a patient’s limb during motor therapy [ 83 ]. For surgery, AI has a vast opportunity to transform surgical robotics through devices that can perform semi-automated surgical tasks with increasing efficiency. The final aim of this technology is to automate procedures to negate human error while maintaining a high level of accuracy and precision [ 84 ]. Finally, the -19 period has led to increased remote patient diagnostics through telemedicine that enables remote observation of patients and provides physicians and nurses with support tools [ 66 , 85 , 86 ].
This study aims to provide a bibliometric analysis of publications on AI in healthcare, focusing on accounting, business and management, decision sciences and health profession studies. Using the SLR method of Massaro et al. [ 11 ], we provide a reliable and replicable research protocol for future studies in this field. Additionally, we investigate the trend of scientific publications on the subject, unexplored information, future directions, and implications using the science mapping workflow. Our analysis provides interesting insights.
In terms of bibliometric variables, the four leading journals, Journal of Medical Systems , Studies in Health Technology and Informatics , IEEE Journal of Biomedical and Health Informatics , and Decision Support Systems , are optimal locations for the publication of scientific articles on this topic. These journals deal mainly with healthcare, medical information systems, and applications such as cloud computing, machine learning, and AI. Additionally, in terms of h-index, Bushko R.G. and Liu C. are the most productive and impactful authors in this research stream. Burke et al.’s [ 67 ] contribution is the most cited with an analysis of nurse rostering using new technologies such as AI. Finally, in terms of keywords, co-occurrence reveals some interesting insights. For instance, researchers have found that AI has a role in diagnostic accuracy and helps in the analysis of health data by comparing thousands of medical records, experiencing automatic learning with clinical alerts, efficient management of health services and places of care, and the possibility of reconstructing patient history using these data.
Second, this paper finds five cluster analyses in healthcare applications: health services management, predictive medicine, patient data, diagnostics, and finally, clinical decision-making. These technologies can also contribute to optimising logistics processes in health services and allowing a better allocation of resources.
Third, the authors analysing the research findings and the issues under discussion strongly support AI's role in decision support. These applications, however, are demonstrated by creating a direct link to data quality management and the technology awareness of health personnel [ 87 ].
The importance of data quality for the decision-making process
Several authors have analysed AI in the healthcare research stream, but in this case, the authors focus on other literature that includes business and decision-making processes. In this regard, the analysis of the search flow reveals a double view of the literature. On the one hand, some contributions belong to the positivist literature and embrace future applications and implications of technology for health service management, data analysis and diagnostics [ 6 , 80 , 88 ]. On the other hand, some investigations also aim to understand the darker sides of technology and its impact. For example, as Carter [ 89 ] states, the impact of AI is multi-sectoral; its development, however, calls for action to protect personal data. Similarly, Davenport and Kalakota [ 77 ] focus on the ethical implications of using AI in healthcare. According to the authors, intelligent machines raise issues of accountability, transparency, and permission, especially in automated communication with patients. Our analysis does not indicate a marked strand of the literature; therefore, we argue that the discussion of elements such as the transparency of technology for patients is essential for the development of AI applications.
A large part of our results shows that, at the application level, AI can be used to improve medical support for patients (Fig. 11 ) [ 64 , 82 ]. However, we believe that, as indicated by Kalis et al. [ 90 ] on the pages of Harvard Business Review, the management of costly back-office problems should also be addressed.
The potential of algorithms includes data analysis. There is an immense quantity of data accessible now, which carries the possibility of providing information about a wide variety of medical and healthcare activities [ 91 ]. With the advent of modern computational methods, computer learning and AI techniques, there are numerous possibilities [ 79 , 83 , 84 ]. For example, AI makes it easier to turn data into concrete and actionable observations to improve decision-making, deliver high-quality patient treatment, adapt to real-time emergencies, and save more lives on the clinical front. In addition, AI makes it easier to leverage capital to develop systems and facilities and reduce expenses at the organisational level [ 78 ]. Studying contributions to the topic, we noticed that data accuracy was included in the debate, indicating that a high standard of data will benefit decision-making practitioners [ 38 , 77 ]. AI techniques are an essential instrument for studying data and the extraction of medical insight, and they may assist medical researchers in their practices. Using computational tools, healthcare stakeholders may leverage the power of data not only to evaluate past data ( descriptive analytics ) but also to forecast potential outcomes ( predictive analytics ) and to define the best actions for the present scenario ( prescriptive analytics ) [ 78 ]. The current abundance of evidence makes it easier to provide a broad view of patient health; doctors should have access to the correct details at the right time and location to provide the proper treatment [ 92 ].
Will medical technology de-skill doctors?
Further reflection concerns the skills of doctors. Studies have shown that healthcare personnel are progressively being exposed to technology for different purposes, such as collecting patient records or diagnosis [ 71 ]. This is demonstrated by the keywords (Fig. 6 ) that focus on technology and the role of decision-making with new innovative tools. In addition, the discussion expands with Lu [ 93 ], which indicates that the excessive use of technology could hinder doctors’ skills and clinical procedures' expansion. Among the main issues arising from the literature is the possible de-skilling of healthcare staff due to reduced autonomy in decision-making concerning patients [ 94 ]. Therefore, the challenges and discussion we uncovered in Fig. 11 are expanded by also considering the ethical implications of technology and the role of skills.
Implications
Our analysis also has multiple theoretical and practical implications.
In terms of theoretical contribution, this paper extends the previous results of Connelly et al., dos Santos et al, Hao et al., Huang et al., Liao et al. and Tran et al. [ 2 , 19 , 20 , 21 , 22 , 24 ] in considering AI in terms of clinical decision-making and data management quality.
In terms of practical implications, this paper aims to create a fruitful discussion with healthcare professionals and administrative staff on how AI can be at their service to increase work quality. Furthermore, this investigation offers a broad comprehension of bibliometric variables of AI techniques in healthcare. It can contribute to advancing scientific research in this field.
Limitations
Like any other, our study has some limitations that could be addressed by more in-depth future studies. For example, using only one research database, such as Scopus, could be limiting. Further analysis could also investigate the PubMed, IEEE, and Web of Science databases individually and holistically, especially the health parts. Then, the use of search terms such as "Artificial Intelligence" OR "AI" AND "Healthcare" could be too general and exclude interesting studies. Moreover, although we analysed 288 peer-reviewed scientific papers, because the new research topic is new, the analysis of conference papers could return interesting results for future researchers. Additionally, as this is a young research area, the analysis will be subject to recurrent obsolescence as multiple new research investigations are published. Finally, although bibliometric analysis has limited the subjectivity of the analysis [ 15 ], the verification of recurring themes could lead to different results by indicating areas of significant interest not listed here.
Future research avenues
Concerning future research perspectives, researchers believe that an analysis of the overall amount that a healthcare organisation should pay for AI technologies could be helpful. If these technologies are essential for health services management and patient treatment, governments should invest and contribute to healthcare organisations' modernisation. New investment funds could be made available in the healthcare world, as in the European case with the Next Generation EU programme or national investment programmes [ 95 ]. Additionally, this should happen especially in the poorest countries around the world, where there is a lack of infrastructure and services related to health and medicine [ 96 ]. On the other hand, it might be interesting to evaluate additional profits generated by healthcare organisations with AI technologies compared to those that do not use such technologies.
Further analysis could also identify why some parts of the world have not conducted studies in this area. It would be helpful to carry out a comparative analysis between countries active in this research field and countries that are not currently involved. It would make it possible to identify variables affecting AI technologies' presence or absence in healthcare organisations. The results of collaboration between countries also present future researchers with the challenge of greater exchanges between researchers and professionals. Therefore, further research could investigate the difference in vision between professionals and academics.
In the accounting, business, and management research area, there is currently a lack of quantitative analysis of the costs and profits generated by healthcare organisations that use AI technologies. Therefore, research in this direction could further increase our understanding of the topic and the number of healthcare organisations that can access technologies based on AI. Finally, as suggested in the discussion section, more interdisciplinary studies are needed to strengthen AI links with data quality management and AI and ethics considerations in healthcare.
In pursuing the philosophy of Massaro et al.’s [ 11 ] methodological article, we have climbed on the shoulders of giants, hoping to provide a bird's-eye view of the AI literature in healthcare. We performed this study with a bibliometric analysis aimed at discovering authors, countries of publication and collaboration, and keywords and themes. We found a fast-growing, multi-disciplinary stream of research that is attracting an increasing number of authors.
The research, therefore, adopts a quantitative approach to the analysis of bibliometric variables and a qualitative approach to the study of recurring keywords, which has allowed us to demonstrate strands of literature that are not purely positive. There are currently some limitations that will affect future research potential, especially in ethics, data governance and the competencies of the health workforce.
Availability of data and materials
All the data are retrieved from public scientific platforms.
Tagliaferri SD, Angelova M, Zhao X, Owen PJ, Miller CT, Wilkin T, et al. Artificial intelligence to improve back pain outcomes and lessons learnt from clinical classification approaches: three systematic reviews. NPJ Digit Med. 2020;3(1):1–16.
Article Google Scholar
Tran BX, Vu GT, Ha GH, Vuong Q-H, Ho M-T, Vuong T-T, et al. Global evolution of research in artificial intelligence in health and medicine: a bibliometric study. J Clin Med. 2019;8(3):360.
Article PubMed Central Google Scholar
Hamid S. The opportunities and risks of artificial intelligence in medicine and healthcare [Internet]. 2016 [cited 2020 May 29]. http://www.cuspe.org/wp-content/uploads/2016/09/Hamid_2016.pdf
Panch T, Szolovits P, Atun R. Artificial intelligence, machine learning and health systems. J Glob Health. 2018;8(2):020303.
Article PubMed PubMed Central Google Scholar
Yang X, Wang Y, Byrne R, Schneider G, Yang S. Concepts of artificial intelligence for computer-assisted drug discovery | chemical reviews. Chem Rev. 2019;119(18):10520–94.
Article CAS PubMed Google Scholar
Burton RJ, Albur M, Eberl M, Cuff SM. Using artificial intelligence to reduce diagnostic workload without compromising detection of urinary tract infections. BMC Med Inform Decis Mak. 2019;19(1):171.
Meskò B, Drobni Z, Bényei E, Gergely B, Gyorffy Z. Digital health is a cultural transformation of traditional healthcare. Mhealth. 2017;3:38.
Cho B-J, Choi YJ, Lee M-J, Kim JH, Son G-H, Park S-H, et al. Classification of cervical neoplasms on colposcopic photography using deep learning. Sci Rep. 2020;10(1):13652.
Article CAS PubMed PubMed Central Google Scholar
Doyle OM, Leavitt N, Rigg JA. Finding undiagnosed patients with hepatitis C infection: an application of artificial intelligence to patient claims data. Sci Rep. 2020;10(1):10521.
Shortliffe EH, Sepúlveda MJ. Clinical decision support in the era of artificial intelligence. JAMA. 2018;320(21):2199–200.
Article PubMed Google Scholar
Massaro M, Dumay J, Guthrie J. On the shoulders of giants: undertaking a structured literature review in accounting. Account Auditing Account J. 2016;29(5):767–801.
Junquera B, Mitre M. Value of bibliometric analysis for research policy: a case study of Spanish research into innovation and technology management. Scientometrics. 2007;71(3):443–54.
Casadesus-Masanell R, Ricart JE. How to design a winning business model. Harvard Business Review [Internet]. 2011 Jan 1 [cited 2020 Jan 8]. https://hbr.org/2011/01/how-to-design-a-winning-business-model
Aria M, Cuccurullo C. bibliometrix: an R-tool for comprehensive science mapping analysis. J Informetr. 2017;11(4):959–75.
Zupic I, Čater T. Bibliometric methods in management and organization. Organ Res Methods. 2015;1(18):429–72.
Secinaro S, Calandra D. Halal food: structured literature review and research agenda. Br Food J. 2020. https://doi.org/10.1108/BFJ-03-2020-0234 .
Rialp A, Merigó JM, Cancino CA, Urbano D. Twenty-five years (1992–2016) of the international business review: a bibliometric overview. Int Bus Rev. 2019;28(6):101587.
Zhao L, Dai T, Qiao Z, Sun P, Hao J, Yang Y. Application of artificial intelligence to wastewater treatment: a bibliometric analysis and systematic review of technology, economy, management, and wastewater reuse. Process Saf Environ Prot. 2020;1(133):169–82.
Article CAS Google Scholar
Huang Y, Huang Q, Ali S, Zhai X, Bi X, Liu R. Rehabilitation using virtual reality technology: a bibliometric analysis, 1996–2015. Scientometrics. 2016;109(3):1547–59.
Hao T, Chen X, Li G, Yan J. A bibliometric analysis of text mining in medical research. Soft Comput. 2018;22(23):7875–92.
dos Santos BS, Steiner MTA, Fenerich AT, Lima RHP. Data mining and machine learning techniques applied to public health problems: a bibliometric analysis from 2009 to 2018. Comput Ind Eng. 2019;1(138):106120.
Liao H, Tang M, Luo L, Li C, Chiclana F, Zeng X-J. A bibliometric analysis and visualization of medical big data research. Sustainability. 2018;10(1):166.
Choudhury A, Renjilian E, Asan O. Use of machine learning in geriatric clinical care for chronic diseases: a systematic literature review. JAMIA Open. 2020;3(3):459–71.
Connelly TM, Malik Z, Sehgal R, Byrnes G, Coffey JC, Peirce C. The 100 most influential manuscripts in robotic surgery: a bibliometric analysis. J Robot Surg. 2020;14(1):155–65.
Guo Y, Hao Z, Zhao S, Gong J, Yang F. Artificial intelligence in health care: bibliometric analysis. J Med Internet Res. 2020;22(7):e18228.
Choudhury A, Asan O. Role of artificial intelligence in patient safety outcomes: systematic literature review. JMIR Med Inform. 2020;8(7):e18599.
Forliano C, De Bernardi P, Yahiaoui D. Entrepreneurial universities: a bibliometric analysis within the business and management domains. Technol Forecast Soc Change. 2021;1(165):120522.
Secundo G, Del Vecchio P, Mele G. Social media for entrepreneurship: myth or reality? A structured literature review and a future research agenda. Int J Entrep Behav Res. 2020;27(1):149–77.
Dal Mas F, Massaro M, Lombardi R, Garlatti A. From output to outcome measures in the public sector: a structured literature review. Int J Organ Anal. 2019;27(5):1631–56.
Google Scholar
Baima G, Forliano C, Santoro G, Vrontis D. Intellectual capital and business model: a systematic literature review to explore their linkages. J Intellect Cap. 2020. https://doi.org/10.1108/JIC-02-2020-0055 .
Dumay J, Guthrie J, Puntillo P. IC and public sector: a structured literature review. J Intellect Cap. 2015;16(2):267–84.
Dal Mas F, Garcia-Perez A, Sousa MJ, Lopes da Costa R, Cobianchi L. Knowledge translation in the healthcare sector. A structured literature review. Electron J Knowl Manag. 2020;18(3):198–211.
Mas FD, Massaro M, Lombardi R, Biancuzzi H. La performance nel settore pubblico tra misure di out-put e di outcome. Una revisione strutturata della letteratura ejvcbp. 2020;1(3):16–29.
Dumay J, Cai L. A review and critique of content analysis as a methodology for inquiring into IC disclosure. J Intellect Cap. 2014;15(2):264–90.
Haleem A, Javaid M, Khan IH. Current status and applications of Artificial Intelligence (AI) in medical field: an overview. Curr Med Res Pract. 2019;9(6):231–7.
Paul J, Criado AR. The art of writing literature review: what do we know and what do we need to know? Int Bus Rev. 2020;29(4):101717.
Liberati A, Altman DG, Tetzlaff J, Mulrow C, Gøtzsche PC, Ioannidis JPA, et al. The PRISMA statement for reporting systematic reviews and meta-analyses of studies that evaluate health care interventions: explanation and elaboration. PLoS Med. 2009;6(7):e1000100.
Biancone PP, Secinaro S, Brescia V, Calandra D. Data quality methods and applications in health care system: a systematic literature review. Int J Bus Manag. 2019;14(4):p35.
Secinaro S, Brescia V, Calandra D, Verardi GP, Bert F. The use of micafungin in neonates and children: a systematic review. ejvcbp. 2020;1(1):100–14.
Bert F, Gualano MR, Biancone P, Brescia V, Camussi E, Martorana M, et al. HIV screening in pregnant women: a systematic review of cost-effectiveness studies. Int J Health Plann Manag. 2018;33(1):31–50.
Levy Y, Ellis TJ. A systems approach to conduct an effective literature review in support of information systems research. Inf Sci Int J Emerg Transdiscipl. 2006;9:181–212.
Chen G, Xiao L. Selecting publication keywords for domain analysis in bibliometrics: a comparison of three methods. J Informet. 2016;10(1):212–23.
Falagas ME, Pitsouni EI, Malietzis GA, Pappas G. Comparison of PubMed, Scopus, Web of Science, and Google Scholar: strengths and weaknesses. FASEB J. 2007;22(2):338–42.
Article PubMed CAS Google Scholar
Sicilia M-A, Garcìa-Barriocanal E, Sànchez-Alonso S. Community curation in open dataset repositories: insights from zenodo. Procedia Comput Sci. 2017;1(106):54–60.
Secinaro S, Calandra D, Secinaro A, Muthurangu V, Biancone P. Artificial Intelligence for healthcare with a business, management and accounting, decision sciences, and health professions focus [Internet]. Zenodo; 2021 [cited 2021 Mar 7]. https://zenodo.org/record/4587618#.YEScpl1KiWh .
Elango B, Rajendran D. Authorship trends and collaboration pattern in the marine sciences literature: a scientometric Study. Int J Inf Dissem Technol. 2012;1(2):166–9.
Jacoby WG. Electoral inquiry section Loess: a nonparametric, graphical tool for depicting relationships between variables q. In 2000.
Andrews JE. An author co-citation analysis of medical informatics. J Med Libr Assoc. 2003;91(1):47–56.
PubMed PubMed Central Google Scholar
White HD, Griffith BC. Author cocitation: a literature measure of intellectual structure. J Am Soc Inf Sci. 1981;32(3):163–71.
Santosh KC. AI-driven tools for coronavirus outbreak: need of active learning and cross-population train/test models on multitudinal/multimodal data. J Med Syst. 2020;44(5):93.
Shickel B, Tighe PJ, Bihorac A, Rashidi P. Deep EHR: a survey of recent advances in deep learning techniques for electronic health record (EHR) analysis. IEEE J Biomed Health Inform. 2018;22(5):1589–604.
Baig MM, GholamHosseini H, Moqeem AA, Mirza F, Lindén M. A systematic review of wearable patient monitoring systems—current challenges and opportunities for clinical adoption. J Med Syst. 2017;41(7):115.
Kumar S, Kumar S. Collaboration in research productivity in oil seed research institutes of India. In: Proceedings of fourth international conference on webometrics, informetrics and scientometrics. p. 28–1; 2008.
Gatto A, Drago C. A taxonomy of energy resilience. Energy Policy. 2020;136:111007.
Levitt JM, Thelwall M. Alphabetization and the skewing of first authorship towards last names early in the alphabet. J Informet. 2013;7(3):575–82.
Saad G. Exploring the h-index at the author and journal levels using bibliometric data of productive consumer scholars and business-related journals respectively. Scientometrics. 2006;69(1):117–20.
Egghe L. Theory and practise of the g-index. Scientometrics. 2006;69(1):131–52.
Schreiber M. A modification of the h-index: the hm-index accounts for multi-authored manuscripts. J Informet. 2008;2(3):211–6.
Engqvist L, Frommen JG. The h-index and self-citations. Trends Ecol Evol. 2008;23(5):250–2.
London School of Economics. 3: key measures of academic influence [Internet]. Impact of social sciences. 2010 [cited 2021 Jan 13]. https://blogs.lse.ac.uk/impactofsocialsciences/the-handbook/chapter-3-key-measures-of-academic-influence/ .
Lotka A. The frequency distribution of scientific productivity. J Wash Acad Sci. 1926;16(12):317–24.
Khan G, Wood J. Information technology management domain: emerging themes and keyword analysis. Scientometrics. 2015;9:105.
Oxford University Press. Oxford English Dictionary [Internet]. 2020. https://www.oed.com/ .
Jiang F, Jiang Y, Zhi H, Dong Y, Li H, Ma S, et al. Artificial intelligence in healthcare: past, present and future. Stroke Vasc Neurol. 2017;2(4):230–43.
Calandra D, Favareto M. Artificial Intelligence to fight COVID-19 outbreak impact: an overview. Eur J Soc Impact Circ Econ. 2020;1(3):84–104.
Bokolo Anthony Jnr. Use of telemedicine and virtual care for remote treatment in response to COVID-19 pandemic. J Med Syst. 2020;44(7):132.
Burke EK, De Causmaecker P, Berghe GV, Van Landeghem H. The state of the art of nurse rostering. J Sched. 2004;7(6):441–99.
Ahmed MA, Alkhamis TM. Simulation optimization for an emergency department healthcare unit in Kuwait. Eur J Oper Res. 2009;198(3):936–42.
Forina M, Armanino C, Raggio V. Clustering with dendrograms on interpretation variables. Anal Chim Acta. 2002;454(1):13–9.
Wartena C, Brussee R. Topic detection by clustering keywords. In: 2008 19th international workshop on database and expert systems applications. 2008. p. 54–8.
Hussain AA, Bouachir O, Al-Turjman F, Aloqaily M. AI Techniques for COVID-19. IEEE Access. 2020;8:128776–95.
Agrawal A, Gans JS, Goldfarb A. Exploring the impact of artificial intelligence: prediction versus judgment. Inf Econ Policy. 2019;1(47):1–6.
Chakradhar S. Predictable response: finding optimal drugs and doses using artificial intelligence. Nat Med. 2017;23(11):1244–7.
Fleming N. How artificial intelligence is changing drug discovery. Nature. 2018;557(7707):S55–7.
Guo J, Li B. The application of medical artificial intelligence technology in rural areas of developing countries. Health Equity. 2018;2(1):174–81.
Aisyah M, Cockcroft S. A snapshot of data quality issues in Indonesian community health. Int J Netw Virtual Organ. 2014;14(3):280–97.
Davenport T, Kalakota R. The potential for artificial intelligence in healthcare. Future Healthc J. 2019;6(2):94–8.
Mehta N, Pandit A, Shukla S. Transforming healthcare with big data analytics and artificial intelligence: a systematic mapping study. J Biomed Inform. 2019;1(100):103311.
Collins GS, Moons KGM. Reporting of artificial intelligence prediction models. Lancet. 2019;393(10181):1577–9.
Bennett CC, Hauser K. Artificial intelligence framework for simulating clinical decision-making: a Markov decision process approach. Artif Intell Med. 2013;57(1):9–19.
Redondo T, Sandoval AM. Text Analytics: the convergence of big data and artificial intelligence. Int J Interact Multimed Artif Intell. 2016;3. https://www.ijimai.org/journal/bibcite/reference/2540 .
Winter JS, Davidson E. Big data governance of personal health information and challenges to contextual integrity. Inf Soc. 2019;35(1):36–51.
Novak D, Riener R. Control strategies and artificial intelligence in rehabilitation robotics. AI Mag. 2015;36(4):23–33.
Tarassoli SP. Artificial intelligence, regenerative surgery, robotics? What is realistic for the future of surgery? Ann Med Surg (Lond). 2019;17(41):53–5.
Saha SK, Fernando B, Cuadros J, Xiao D, Kanagasingam Y. Automated quality assessment of colour fundus images for diabetic retinopathy screening in telemedicine. J Digit Imaging. 2018;31(6):869–78.
Gu D, Li T, Wang X, Yang X, Yu Z. Visualizing the intellectual structure and evolution of electronic health and telemedicine research. Int J Med Inform. 2019;130:103947.
Madnick S, Wang R, Lee Y, Zhu H. Overview and framework for data and information quality research. J Data Inf Qual. 2009;1:1.
Chen X, Liu Z, Wei L, Yan J, Hao T, Ding R. A comparative quantitative study of utilizing artificial intelligence on electronic health records in the USA and China during 2008–2017. BMC Med Inform Decis Mak. 2018;18(5):117.
Carter D. How real is the impact of artificial intelligence? Bus Inf Surv. 2018;35(3):99–115.
Kalis B, Collier M, Fu R. 10 Promising AI Applications in Health Care. 2018;5.
Biancone P, Secinaro S, Brescia V, Calandra D. Management of open innovation in healthcare for cost accounting using EHR. J Open Innov Technol Market Complex. 2019;5(4):99.
Kayyali B, Knott D, Van Kuiken S. The ‘big data’ revolution in US healthcare [Internet]. McKinsey & Company. 2013 [cited 2020 Aug 14]. https://healthcare.mckinsey.com/big-data-revolution-us-healthcare/ .
Lu J. Will medical technology deskill doctors? Int Educ Stud. 2016;9(7):130–4.
Hoff T. Deskilling and adaptation among primary care physicians using two work innovations. Health Care Manag Rev. 2011;36(4):338–48.
Picek O. Spillover effects from next generation EU. Intereconomics. 2020;55(5):325–31.
Sousa MJ, Dal Mas F, Pesqueira A, Lemos C, Verde JM, Cobianchi L. The potential of AI in health higher education to increase the students’ learning outcomes. TEM J. 2021. ( In press ).
Download references
Acknowledgements
The authors are grateful to the Editor-in-Chief for the suggestions and all the reviewers who spend a part of their time ensuring constructive feedback to our research article.
This research received no specific grant from any funding agency in the public, commercial, or not-for-profit sectors.
Author information
Authors and affiliations.
Department of Management, University of Turin, Turin, Italy
Silvana Secinaro, Davide Calandra & Paolo Biancone
Ospedale Pediatrico Bambino Gesù, Rome, Italy
Aurelio Secinaro
Institute of Child Health, University College London, London, UK
Vivek Muthurangu
You can also search for this author in PubMed Google Scholar
Contributions
SS and PB, Supervision; Validation, writing, AS and VM; Formal analysis, DC and AS; Methodology, DC; Writing; DC, SS and AS; conceptualization, VM, PB; validation, VM, PB. All authors read and approved the final manuscript.
Corresponding author
Correspondence to Davide Calandra .
Ethics declarations
Ethical approval and consent to participate.
Not applicable.
Consent for publication
Competing interests.
The authors declare that they have no known competing financial interests or personal relationships that could have appeared to influence the work reported in this paper.
Additional information
Publisher's note.
Springer Nature remains neutral with regard to jurisdictional claims in published maps and institutional affiliations.
Rights and permissions
Open Access This article is licensed under a Creative Commons Attribution 4.0 International License, which permits use, sharing, adaptation, distribution and reproduction in any medium or format, as long as you give appropriate credit to the original author(s) and the source, provide a link to the Creative Commons licence, and indicate if changes were made. The images or other third party material in this article are included in the article's Creative Commons licence, unless indicated otherwise in a credit line to the material. If material is not included in the article's Creative Commons licence and your intended use is not permitted by statutory regulation or exceeds the permitted use, you will need to obtain permission directly from the copyright holder. To view a copy of this licence, visit http://creativecommons.org/licenses/by/4.0/ . The Creative Commons Public Domain Dedication waiver ( http://creativecommons.org/publicdomain/zero/1.0/ ) applies to the data made available in this article, unless otherwise stated in a credit line to the data.
Reprints and permissions
About this article
Cite this article.
Secinaro, S., Calandra, D., Secinaro, A. et al. The role of artificial intelligence in healthcare: a structured literature review. BMC Med Inform Decis Mak 21 , 125 (2021). https://doi.org/10.1186/s12911-021-01488-9
Download citation
Received : 24 December 2020
Accepted : 01 April 2021
Published : 10 April 2021
DOI : https://doi.org/10.1186/s12911-021-01488-9
Share this article
Anyone you share the following link with will be able to read this content:
Sorry, a shareable link is not currently available for this article.
Provided by the Springer Nature SharedIt content-sharing initiative
- Artificial intelligence
- Patient data
BMC Medical Informatics and Decision Making
ISSN: 1472-6947
- General enquiries: [email protected]
Advertisement
Mapping research on healthcare operations and supply chain management: a topic modelling-based literature review
- Original Research
- Published: 31 March 2022
- Volume 315 , pages 29–55, ( 2022 )
Cite this article
- Imran Ali 1 &
- Devika Kannan 2
10k Accesses
33 Citations
Explore all metrics
The literature on healthcare operations and supply chain management has seen unprecedented growth over the past two decades. This paper seeks to advance the body of knowledge on this topic by utilising a topic modelling-based literature review to identify the core topics, examine their dynamic changes, and identify opportunities for further research in the area. Based on an analysis of 571 articles published until 25 January 2022, we identify numerous popular topics of research in the area, including patient waiting time, COVID-19 pandemic, Industry 4.0 technologies, sustainability, risk and resilience, climate change, circular economy, humanitarian logistics, behavioural operations, service-ecosystem, and knowledge management. We reviewed current literature around each topic and offered insights into what aspects of each topic have been studied and what are the recent developments and opportunities for more impactful future research. Doing so, this review help advance the contemporary scholarship on healthcare operations and supply chain management and offers resonant insights for researchers, research students, journal editors, and policymakers in the field.
Similar content being viewed by others
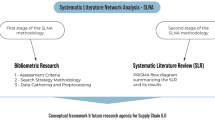
Towards Supply Chain 5.0: Redesigning Supply Chains as Resilient, Sustainable, and Human-Centric Systems in a Post-pandemic World
Alice Villar, Stefania Paladini & Oliver Buckley
Supply Chain “Flows” Management
Humanitarian supply chain management: a thematic literature review and future directions of research.
Abhishek Behl & Pankaj Dutta
Avoid common mistakes on your manuscript.
1 Introduction
Effective and efficient delivery of healthcare services is essential to counteract emergent diseases and promote healthy lives and the well-being of a population, particularly amid the COVID-19 outbreak. Many healthcare systems across the globe strive to efficiently utilise available resources and improve processes through more integrated operations involving all key stakeholders. As such, in the past few decades, research on healthcare has grown exponentially and has become a self-sustained discipline in operations and supply chain management (OSCM). Healthcare OSCM is a network of healthcare providers, pharmaceutical manufacturers, drug suppliers, distributors, and logistics service providers that are involved in the process of acquiring and transforming raw material into finished products, adding value, and facilitating the flow of products and services to the end-customer. With the rapid growth of research on healthcare OSCM, the extant studies have been conducted in various directions and expanded over a dozen of academic journals.
As the field has grown, a corresponding growth in systematic literature reviews in healthcare has occurred, especially in the past six years. For example, Narayana et al. ( 2014 ) reviewed 99 articles (published between 2000 to 2011) on the pharmaceutical supply chain and explore that most studies focus on efficiency improvement with growing interest in process analysis and technology implementation. Volland et al. ( 2017 ) presented a review of 145 articles (published between 1998 and 2014) on material logistics in hospitals and suggested further work on the concepts of supply and procurement, inventory management, and distribution and scheduling. Ali et al. ( 2018 ) conducted a review of 88 articles (published between 2009 and 2018) on cloud computing in hospitals and devised a framework on opportunities, issues, and applications of cloud computing-enabled healthcare systems. Malik et al. ( 2018 ) reviewed 22 articles (published between 2001 and 2015) on the application of data mining in healthcare service delivery and revealed that application of data mining is narrow-focused (workforce scheduling and quality of care), thereby suggesting further research on the broader application of data mining. Moons et al. ( 2019 ) presented a review of 56 articles (published between 2010 and 2016) on logistics performance measurement in the internal hospital supply chain, specifically inventory management and distribution activities in the operation theatre. Diwas Singh et al. ( 2020 ) offered a review of 70 empirical articles (published between 1999 and 2018) from three journals ( Management Science, Manufacturing and Service Operations Management, and Production and Operations Management ) and suggested the need for more empirical research on personalised medicine, value-based healthcare, and digitisation of healthcare. Keskinocak and Savva ( 2020 ) mapped the healthcare research published between 2009 and 2018 from a single journal ( Manufacturing and Service Operations Management ) and suggested the need to develop and apply new OM methods for the improvement of healthcare service.
The existing literature reviews have different scopes and emphases focusing on a specific topic (e.g., material logistics in hospitals, data mining, only empirical papers, etc.). In other words, they are not designed to capture latent topics (hidden topics) that reside in large volumes of scholarly data. Expanding upon some knowledge from the existing reviews, this paper seeks to advance our understanding of the key research topics that have been addressed in healthcare OSCM using a topic modelling-based literature review. Topic modelling with Latent Dirichlet Allocation (LDA) has been found as an effective method to algorithmically and automatically uncover the abstract topics that reside in a large and unstructured collection of articles (Antons et al., 2016 ; Blei, 2012 ; Zhang, 2012 ). LDA topic modelling builds upon rigorous statistical foundations which allows topic generation with little human intervention and/or manual processing (Blei, 2012 ; Zhang, 2012 ). Such an automatic method creates more meaningful and realistic topics and ensures the reliability and validity of outcomes as opposed to manual methods (Griffiths & Steyvers, 2004 ). LDA has been successfully applied for topic modelling in the information science field (Yan, 2014 ), marketing (Tirunillai & Tellis, 2014 ), statistics (De Battisti et al., 2015 ), tourism (Guo et al., 2017 ), decision sciences (Chae & Olson, 2018 ), computer science (Guo et al., 2017 ), and hydropower research (Jiang et al., 2016 ). However, to date, we have only seen a very limited application of topic modelling-base literature in OSCM fields (e.g., Chae & Olson, 2018 ; Lee & Kang, 2018 ), particularly in the healthcare context, to explore the significant topics hidden in a large sample of articles and to locate gaps for further research in each topic. As such, this study aims to: (1) uncover the latent topics residing in the articles published on healthcare OSCM, (2) examine the topic trend/evolution over time including hot, cold, and steady topics, and (3) find opportunities for more impactful research on each topic through a review of the literature around each topic. Driven by these core aims; the following research questions are posed:
What are the emergent topics of research in the field of healthcare OSCM?
How has research on each topic evolved over the past two decades?
What are the opportunities for more impactful future research in the area?
Our study is among the few that offers a topic modelling-based review of literature, which allows us objectively to uncover hidden patterns of research that pervade in a large collection of studies and to explore the development of literature on each topic. The paper advances our understanding of how the literature on different topics in the field of healthcare OSCM has evolved over time and what are the recent trends, knowledge flow, and opportunities for insightful research on each topic. We find an increasing focus and need for further investigation on some emergent topics, such as patient waiting time, COVID-19 pandemic, Industry 4.0 technologies, sustainability, risk and resilience, climate change, circular economy, humanitarian logistics, behavioural operations, service-ecosystem, and knowledge management. In terms of research methods, our analysis shows the increasing interest of researchers in the Markov method, mixed-integer programming, simulation, queueing model and stochastic programming in exploring multidimensional issues of healthcare OSCM. In addition, our analysis suggests that the past three years have also seen some empirical contributions in the area, including structural equation modelling, longitudinal study, and action research; thus, encouraging empirical researchers for more fruitful contributions on the topic.
Our study offers valuable research directions to researchers, journal editors, and policymakers in the field of healthcare OSCM. Researchers can observe whether their recent research topics are hot, cold, or steady, which will allow them to select appropriate topics for more productive forthcoming study in the area. Particularly, some early-career researchers find it difficult to keep up with the growing literature in their fields of research and familiarise themselves with a huge volume of literature in identifying the subject area on which they can focus or apply for research grants. The information from our topic modelling can be a useful tool for these researchers to understand recent developments in the field and then select more appropriate topics. Our findings can also be helpful to PhD students, who often face difficulties in topic selection due to time pressure. Journal editors can view if the extant research is consistent with their editorial policy, and they may even choose to launch a new editorial vision and direction. Our list of hot topics can be a valuable reference tool to launch special issues in the journals. Discovering underlying topics and tracking their evolution could also be of great interest to policymakers (e.g., government agencies) and industry. The information on which research themes and methods are growing or declining in popularity over time (Table 3 and Fig. 4 ) can support governmental funding agencies for grants allocation to promising areas. Likewise, firm managers and the industry as a whole can use this information to formulate promising R&D strategies and more informed investment decisions.
The rest of the paper is structured as follows. Section 2 elaborates on the research methods, covering data collection, screening and analysis procedures. Section 3 outlines results which include word cloud, key term matrix, topic labelling, and evolution of each topic over time. Section 4 provides discussion, while Sect. 5 offers a review of literature and opportunities for further research. The study is concluded in Sect. 6.
As mentioned earlier, this study employs the topic modelling method. The superiority of the topic modelling method has been evidenced in other fields, for example, Latent Semantic Analysis (LSA) (Deerwester et al., 1990 ), Non-Negative Matrix Factorization (NMF) (Lee & Seung, 1999 ), and probabilistic topic modelling. LSA uses singular value decomposition and a traditional matrix factorization technique to reduce the dimensionality of documents (Deerwester et al., 1990 ). Unlike singular value decomposition, in NMF, the transformed and reduced version of documents contains no negative elements (Lee & Seung, 1999 ). Probabilistic topic modelling is a recent development in topic modelling where words and documents are described through probabilistic topics, and a topic is described in terms of a probability distribution over a vocabulary of words (Hofmann, 2001 ; Lee & Kang, 2018 ). Latent Dirichlet Allocation (LDA) is the most popular probabilistic topic modelling algorithm. A comparison of LSA, NMF, and LDA suggests that LDA has the greatest ability to maintain topic coherence and to generate predominant topics that handle the issue of polysemy, the overlapping of words with multiple meanings (Stevens et al., 2012 ). LDA has been successfully applied in the information science field (Yan, 2014 ), marketing (Tirunillai & Tellis, 2014 ), statistics (De Battisti et al., 2015 ), tourism (Guo et al., 2017 ), decision sciences (Chae & Olson, 2018 ), computer science (Guo et al., 2017 ), and hydropower research (Jiang et al., 2016 ). Borrowing knowledge from adjacent areas, this study employs LDA in healthcare. The analysis is completed in four main steps: data collection; text pre-processing; word cloud; and topic modelling.
2.1 Data collection
The analysis aims to uncover the landscape of healthcare research published in good quality international journals in the field of operations and supply chains. The data were collected from Scopus on 25 January 2022, which is one of the largest abstracts and citation databases of academic papers (Ali & Gölgeci, 2019 ; Ferreira, 2018 ; Ali et al., 2021a , 2021b ). To retrieve a broad range of literature on the topic, a large set of keywords was developed based on the authors’ extensive knowledge of the field and review of the extant literature in the field. These keywords included: healthcare, AND operations AND “supply chain” OR hospital OR pharmaceutical OR drug OR medicine AND model OR framework OR Stochastic OR Simulation OR “linear programming” OR Heuristic OR mathematical OR optimization OR “numerical model” OR “nonlinear programming” OR “decision tool” OR “decision analysis” OR “discrete event” OR multi-objective OR multi-objective OR multi-attribute OR multi-criteria OR MCDM OR MCDA OR qualitative OR quantitative OR “case study” OR empirical. Quotation marks were placed on the keywords comprised of more than one word (e.g., “linear programming”) to ensure that search engines consider them as one word.
Initially, we did not limit the timespan because we sought to capture a wide range of publications. The search query generated 663 articles. We excluded the three articles published before 2000 to set the time interval between 2000 and 25 January 2022 (the date of data collection). Next, we carefully read the title and abstract of each article and excluded irrelevant articles. We also excluded articles in non-English languages. Extreme care was taken to include the articles that focus on healthcare OSCM. The inclusion and exclusion criteria resulted in the final set of 571 articles as shown in the supplementary file. Table 1 presents the distribution of these articles.
The articles selected from Scopus were validated using other databases, such as Web of Science, Google Scholar, ProQuest, and EBSCO to ensure that no important articles were missed in the review. The validation process also helped confirm that Scopus contains a greater number of related articles on the topic of healthcare OSCM compared to other databases, so it was used as a preferred database for this research.
2.2 Data pre-processing
Once the articles are collected from Scopus, some pre-processing of data collected is required to conduct further analysis. First, since our search result included the article’s title, keywords and abstract, we merged them as a single document. Second, we removed all punctuation and numbers and transformed all characters into lower-case. Third, we eliminated all “stop-words,” whose main role is to make a sentence grammatically correct, e.g., articles (a, an, the) and prepositions (of, by, from, etc.) and general words that repeatedly appear in most articles, e.g., “and,” “paper”, “find”, “effect”, and “discuss”. These words are not semantically meaningful, yet they create trouble in the analysis process. This step is important because without applying stop-word elimination, our analytical method could result in a big proportion of these unwanted words in the word cloud as well as in the topic matrix.
2.3 Word cloud
Word cloud with R-package is a text mining method to find the most frequently used keywords in sampled articles. A word cloud was designed to allow a visual interpretation of main themes and their trajectories in our review transcripts over the four different periods (2000–2004, 2005–2009, 2010–2014, 2015–2022). As mentioned earlier, we also included articles published until 25 January 2022. Data file for each period was imported into a popular R package, word cloud (Hornik & Grün, 2011 ) with default Dirichlet hyperparameters. The following functions in the R-package were applied to create word clouds: tm for text mining: snowballC for text stemming; Wordcloud2 for generating word cloud images; RColorBrewer for colour palettes.
2.4 Topics modelling
To extract the predominant topics from the 571 sampled articles, LDA’s generative process model was applied (Blei, 2012 ). Figure 1 demonstrates the process and its main variables where η is the parameter of the Dirichlet prior for the per-topic word distribution. K is the defined number of topics, while β k is a distribution of words over a topic. W represents the observable variable—the words in each article. W d,n is the n th word in article d . α is the parameter of the Dirichlet on the per-article topic distribution. θ d is a distribution of topics for the d th article. Z d,n is the topic assignment for the nth word in article d . Some variables are interdependent, that is, W (the observed word) depends on β (the distribution of topics) and Z (the topic assignment for the word), on θ (the topic proportion for the document). This process can be represented by the joint distribution of latent and observed variables through the following Figure and equations:
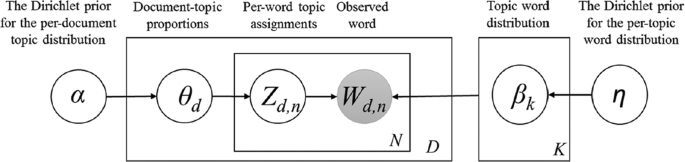
Adapted from Blei ( 2012 )
The graphical representation for LDA.
The generative process aims to explore the topic structure (θ, z , and β), which can explain W . Given the observed variable ( W ), the posterior distribution is estimated with the following equation:
The purpose of Fig. 1 and Eqs. 1 and 2 herein is to demonstrate the analysis process followed by LDA. To perform the analysis objectively and automatically, we used the R-package topic-models along with the tm package (Hornik & Grün, 2011 ). This analytical process produces a document term matrix for the desired number of topics.
3 Analysis and results
This section presents the analysis and the results of data processing, which consist of word cloud, topic modelling, and topic labelling.
3.1 Word cloud
As presented in Fig. 2 , the word clouds show some noticeable changes between 2000 and 2022 in the healthcare literature. In the first period (2000–2004), the popular terms include strategy, logistics, risk, simulation, integer, programming, operations, reengineering, information, inventory, and planning. While a few terms in the first period and second period (2005–2009) are similar (e.g., reengineering, outsourcing, supply, chains, healthcare, strategy, logistics), several new terms became popular in the second period which includes sustainability, warehousing, reverse, heuristic, stochastics, empirical, knowledge, humanitarian, among others.
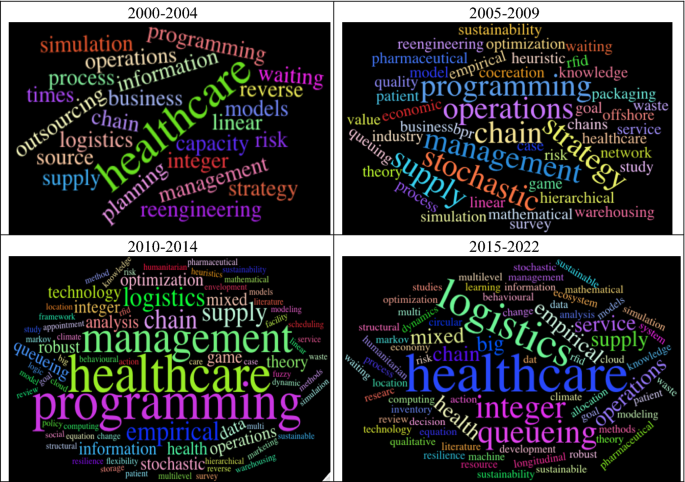
Word cloud demonstrating changing pattern of terms over time (2000–2022)
The third period (2010–2014) shows some important changes, where several new terms (e.g., behavioural, literature, reviews, multilevel, action, structural, equation, Markov) emerged, and other terms (e.g., reengineering, outsourcing, strategy) disappeared. Some other terms remain similar to the second period (e.g., sustainability, warehousing, reverse, heuristic, stochastics, empirical, knowledge, humanitarian, waiting time).
While many terms of the third period continued to appear in the fourth period (2015–2022), several new terms are generated: circular, economy, climate, change, longitudinal. Terms such as warehousing, marketing, strategy disappeared in this period. Understandably, the terms “healthcare”, “operations”, “supply”, “chain” appeared consistently across the four periods.
3.2 Topic modelling
While the visualisation of word clouds (observed words) provides a broad picture of the research dynamics in the literature motivating towards further analysis, it does not provide quantitative information on the relationship between different terms that are generated. Without having quantitative information on each key term, it would be difficult to merge the related terms and assign them into specific topics.
To derive the quantitative information on the nexus between different terms, we used LDA topic modelling that explores the hidden or latent structure and generates different topics by merging interrelated terms from the data set. Generating an appropriate number of topics ( K value) from the given sample of articles is always challenging (Roberts et al., 2014 ). Selecting too few topics would not provide a full and meaningful understanding of the field, while selecting too many topics often results in meaningless outcomes. Therefore, to select the appropriate number of topics, two measures were undertaken: (1) a different number of topics (K value) were tried and accuracies of outcome were checked for each set of topics, and (2) brainstorming among researchers was conducted to ensure topic quality and relatedness to the context of the study (Mimno & McCallum, 2012 ; Silge & Robinson, 2016 ). As a result, 34 relevant topics were produced using LDA with R-package (Fig. 3 ). For each topic, the top five terms were selected, where each term represents a distribution weight (per-topic term probability).
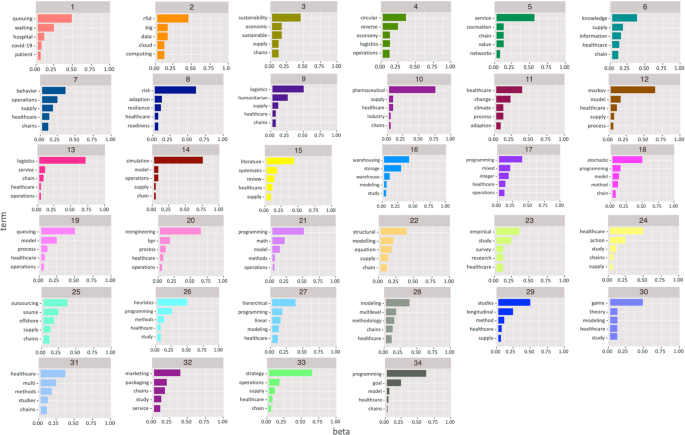
Topic matrix generated in R-package using LDA principle
3.3 Topic labelling
We conducted topic labelling based on the ‘LDA topic matrix’ that produced 34 topics (see Fig. 3 ). Each topic constitutes five terms with different distribution weights. Since the term “healthcare,” “operations,” “supply,” and “chain” appeared in most topics, we decided not to use any of them in labelling the topics. Further, while topic labelling in Table 2 , we used the numbering/sequence of topics exactly following the sequence of topics in the ‘LDA topic matrix’ (Fig. 3 ). To briefly illustrate the process of topic labelling, we discuss a few examples of topic labelling. From Fig. 3 , the top terms in Topic#1 are queuing and waiting, followed by hospital, patient, process; therefore, the topic is labelled the “patient’s waiting time”. Topic#2 includes top terms, such as RFID, big, data followed cloud, computing; therefore, we label the topic as “Industry 4.0”. Industry 4.0 refers to the fourth industrial revolution with the growing use of autonomous technologies such as cloud computing, big data analytics, internet supported RFID and others. Topic# 3 encompasses sustainability, economic, sustainable; hence, it is labelled as sustainability.
Likewise, Topic#8 is labelled “risk and resilience” since the top terms are risk, adaption, resilience supported by healthcare and readiness. Topic#18 is labelled “Stochastic programming” because its top terms are stochastics, programming, followed by model, method, and chain. We adopted a similar process for labelling all 34 topics as demonstrated in Table 2 .
3.4 Topic dynamic/evolution over time
In this section, we introduce the hot, cold, and steady topics (research themes and methods) to provide researchers with an idea of where trends are going (hot) and not going (cold). Exploring the dynamic changes in different topics over time can offer directions for more impactful research on healthcare. We identified three patterns of topic evolution including hot, cold, and steady. For this purpose, we employed a bivariate correlation between the frequency of keywords and time period using four different time periods, namely 2000–2004; 2005–2009; 2010–2014; 2015–2022 (Fig. 3 ). Scopus database was used to collect the information on the frequency of keywords (for each topic shown in Fig. 3 and Table 2 ) in each time interval.
Hot, cold, and steady topics (which include ‘research themes’ and ‘research methods’ as shown in Table 3 ) were identified based on the direction of the trendline on each topic and the value of slope (Fig. 4 ).
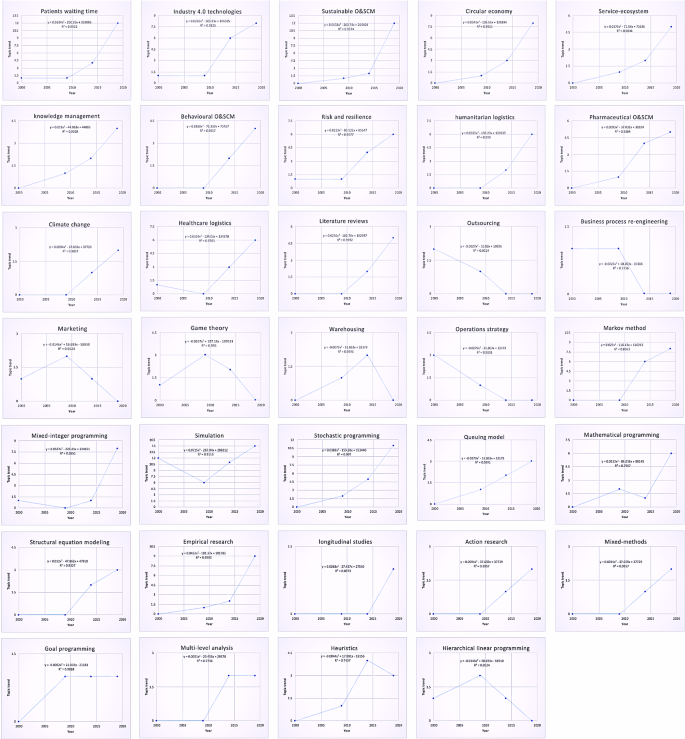
Topic trend/dynamic over time
Hot topics show a trendline moving upward and a positive slope (x-value). Examples of the hot topics related to research themes include the Patient’s waiting time, Industry 4.0 technologies, Sustainability, Circular economy, Behavioural OSCM (OSCM), Risk and resilience, Climate change, and research methods that constitute Markov method, Simulation, Stochastic programming, among others.
The number of contributions on these topics (themes and methods) increased almost continuously from 2010 to 2014, with a dramatic increase on some topics (e.g., patient waiting time, sustainability, circular economy, etc.) between 2015 and 2022.
Cold topics, on the other hand, imply the opposite pattern to these hot topics; they show declining trendline and negative slope values, perhaps due to the relative lack of interest of researchers in recent times.
Examples of cold topics associated with research themes include warehousing, marketing, outsourcing, business process reengineering, strategy, operation strategy, and research methods of heuristics and game theory model among others. These topics enjoyed significant attention between 2000 and 2015, but their proportions have been steadily decreasing over the past five years (Fig. 4 ).
Finally, steady topics show a rise in the beginning but there is no rise or fall in trendlines over the past 5 or more years; perhaps, their importance has not been increased over time.
Overall, two topics depicted a steady growth over the past 5 or more years including goal programming and multi-level analysis. As can be seen in Fig. 4 , the trendline for goal programming modelling increased up to 2009, while no remarkable increase was observed since then. Likewise, the trendline for multi-level analysis shows an upward trend until 2014; however, no growth has been demonstrated since then.
While not investigated in this study, it can be speculated that these topics may lose the interest of research and likely become a cold topic in future. Drawing upon trendline and slope for each topic in Fig. 4 , we have presented all 34 topics under three main categories: hot, cold, and steady (Table 3 ). For better clarity of expression, we have segregated the topics into themes and methods. While both themes and methods are both important, our focus is on research themes in this analysis and discussion.
4 Discussion
Over the last two decades, research on healthcare OSCM has seen spectacular growth. In light of the burgeoning literature on the topic, this study was motivated to explore recent developments, trends, and core topics of research in the area. The study was driven by three key research questions. Addressing the first research question, we explored 34 core topics on which extant research on the topic has been conducted over the past decades. For the second research question, our topic modelling analysis has resulted in the identification of hot, cold, and steady topics in empirical research healthcare OSCM (Table 3 and Fig. 4 ). Thirteen topics of research themes show an upward trend, and nine of them (Patient waiting time, Industry 4.0, Sustainable OSCM, Behavioural OSCM, Risk and resilience, Humanitarian logistics, Climate change, Healthcare logistics, and Literature reviews) start with a relatively flat value (close to 0) before showing a rising trend from around 2010. The other four topics (Circular economy, Service ecosystem, Knowledge management, and Pharmaceutical OSCM) show a gradual upward trend from the early 2000s. Five research topics belong to cold topics. Outsourcing and operations strategy shows an almost linear declining trend, while both Marketing and Warehousing show a bell-shaped trend whose popularity rose in the early 2000s but fell after 2010 and 2015, respectively. Business process reengineering was a popular topic during the early 2000s but, since 2010, shows a declining trend. It is important to note, however, that being identified as a cold topic does not suggest that the topics are not important and should be abandoned. The results provide more of an indication about the interest (trends) of researchers’ interests rather than the importance of the topics themselves. It is also interesting to see that most of the changes of the trends (either upward or downward) of the topics took place around 2010.
Finally, in response to the third research question, we offered a review of the literature and identified numerous opportunities for further research (Sect. 5). Our findings show a shift of current research from traditional topics of operations management (Business process reengineering, Marketing, Outsourcing, Heuristics) to emergent topics such as Patient waiting time, Industry 4.0 technologies, Sustainable operations, Risk and resilience, Climate change, and Circular economy. In general, the shift in topics seems to show a move away from traditional, efficiency-focused research toward broader goals of superior customer service and system’s effectiveness. While each of the three groups of topics deserves discussion, in this section, we discuss the hot research topics as well as provide recommendations of avenues for fruitful future research. It should be noted that although the topic “literature reviews” was a hot topic, we do not include it in our discussion below as the extant research related to it has already been discussed in the introduction section.
5 Literature review and opportunities for further research
The following section provides a brief synthesis of current literature around emergent research topics that are recovered through topic modelling, and it offers numerous opportunities for further research in the field.
5.1 Patients’ waiting time and COVID-19
This research topic covers two interrelated and emerging issues: patient waiting time and COVID-19 outbreak. With a rapidly growing population of aged people, healthcare systems have been facing pressure for more health services resulting in patients’ long waiting times. Therefore, determining methods to ameliorate waiting time has been high on the agenda of most developing and developed economies. Correspondingly, in recent years, mostly building upon simulation and Markov as the primary method of research, considerable studies appeared around the issues of patients’ waiting time, such as patient’s admission process (Chae, 2019 ; Vissers et al., 2007 ); surgery procedures (VanBerkel & Blake, 2007 ); patient inflow and outflow in the emergency department (Abo-Hamad & Arisha, 2013 ); no-shows of patients and overbooking (Topuz et al., 2018 ); outpatient appointment scheduling (Shehadeh et al., 2019 ); time overestimation for the emergency procedure (Gartner & Padman, 2019 ); walk-in patients treatment (Pan et al., 2019 ); patient readmission and discharge planning model (Gu et al., 2019 ); resource planning model to reduce waiting time of patients (Hejazi, 2021 ); load smoothing of scheduled admission to the reduced number of beds required and probability of delay (Asgari & Asgari, 2021 ), and modelled association between length of appointment interval and no-shows of patients (Pan et al., 2021 ).
Overall, although the existing studies have captured several important issues related to patient waiting time, we identify the need for further research on this significant topic. For example, there is potential to develop an integrated model investigating how multiple factors such as general service time, equipment breakdown, high attrition of hospital staff, variability in patient’s arrival time, and unpunctuality of already booked patients cumulatively contribute to patients’ waiting time. Further research can also devise a model at the intersection of patients’ waiting list management, operations theatre scheduling, and patient waiting time for surgery. While the literature on simulation and mathematical modelling continues to grow, there is also an opportunity to further expand empirical research for testing causal links between various factors and their combined effect on patient waiting time. For example, empirical research can test links among customers’ heterogeneous behaviour and their service time in scheduling appointments. Further, a unified model capturing non-value-added practices at both micro and meso levels would be fruitful future research in reducing patient waiting time. While research on bedding and staffing is growing, there is a need to optimise bed and staff requirements amid the global pandemic. Another potential research area is to examine the longitudinal effect of hospital staff’s training on patients’ waiting time.
Likewise, given the outbreak of COVID-19, the recent literature of healthcare OSCM has been rapidly growing on the topic. Pamucar et al. ( 2022 ) proposed a model for supplier selection amid COVID-19. Fan and Xie ( 2022 ) generated an optimization model for COVID-19 testing facility design and planning. Ghaderi ( 2022 ) offered a framework for public health intervention in the wake of the pandemic. Govindan et al. ( 2020 ) developed a decision support system and fuzzy interface system to help with demand management in the healthcare supply chain amid the COVID-19 crises. Nagurney ( 2021a ) modelled labour shortage due to COVID-19, resulting in reduced food harvest and supply. Tavana et al. ( 2021 ) presented a mixed-integer programming model for the equitable distribution of COVID-19 vaccine in developing countries. Salarpour and Nagurney ( 2021 ) constructed a stochastic model to study competition among different countries for medical supplies amid COVID-19 crises. Nagurney ( 2021b ) offered a supply chain network optimization model to manage labour in electronic commerce during the COVID-19 pandemic. Thakur ( 2021 ) developed a model to manage hospital waste during the COVID-19 outbreak. Choi ( 2021 ) used a sense-and-response specific OR model to demonstrate the specific actions needed to deal with the COVID-19 outbreak.
To sum up, while the past research continues to grow on COVID, it would be interesting to explore how to extenuate potential risks and develop shockproof healthcare systems to deal with unanticipated outbreaks such as COVID-19 and similar future incidents. In this regard, it is worth exploring how such a topic as Industry 4.0 technologies could be applied across the healthcare system to capture data from wide sources and help the decision-making process in the event of a pandemic, including contact tracing, the identification of the outbreak location. Some potential areas of research amid COVID-19 include but are not limited to patient inflow and outflow in the emergency department; outpatient appointment scheduling model; models on time estimation for the emergency procedure; walk-in patients’ treatment model; patient admission and discharge planning model; resource planning model to reduce waiting time of patients; load smoothing of scheduled admission to the reduced number of beds required and probability of delay. Besides, pharmaceutical SCM would be the ultimate hope for the recovery and possibly eradication of the virus that allows us to go back to normal life. It would be insightful to examine how pharmaceutical supply chains were affected by COVID-19 and develop a recovery model dealing with such a situation. In particular, if/when a pharmaceutical solution to COVID-19 emerges, research is needed to help understand the best ways to rapidly scale up and distribute the materials on a scale that has never been seen before. It is also of interest to study whether synergy in logistics could be achieved between the public organisations and the growing number of private companies that have entered the healthcare business. Future research should continue to incorporate the healthcare and hospital view into operations management and transfer established concepts from other industries into healthcare while accounting for industry specifics. Again, the links between this and COVID-19 are both obvious and critical.
5.2 Industry 4.0 technologies
Industry 4.0 or the fourth industrial revolution refers to fully automated and in-connected technologies such as the Internet of Things (IoT), cloud computing, big data analytics, blockchain, artificial intelligence, among others (Ali & Govindan, 2021 ; Ali et al., 2021a , 2021b ). Industry 4.0 technologies can enhance the level of service in the industry through real-time information sharing, visibility, traceability, agility, and connectivity within a firm’s boundary and across the supply chain (Govindan et al., 2022 ). Given this, growth in research on the role of Industry 4.0 technologies in healthcare service provision is evident, such as Sultan ( 2014 ) developed a conceptual framework on the potential of cloud computing in the advancement of healthcare services. Chong et al. ( 2015 ) modelled variables that influence the adoption of IoT (e.g., RFID) in healthcare. Priya and Ranjith Kumar ( 2015 ) used big data analytics to predict the progression of atherosclerotic disease, the narrowing and hardening of arteries due to the accumulation of plaque on the artery wall. Fan et al. ( 2018 ) recognised the factors affecting the adoption of artificial intelligence-based medical diagnosis support systems. Kochan et al. ( 2018 ) modelled on how cloud computing improves visibility and responsiveness in hospital SC. Galetsi et al. ( 2020 ) developed a theoretical framework for the realisation of big data analytics.
The research on Industry 4.0 is growing with the advent of new technologies and provides fruitful opportunities for further research. The models on big data should incorporate data of all inter-connected functions in healthcare, instead of just focusing on a single function or silo approach. For example, the impact of Industry 4.0 on the entire healthcare supply chain can be modelled. This is important to understand how interaction with different functions/partners through Industry 4.0 technologies influence healthcare productivity. Most of the current research focuses on big data and cloud computing which needs to be expanded to other Industry 4.0 technologies, such as blockchain, cyber-physical systems and the Internet of Things in healthcare OSCM. There is a lack of a holistic model testing the combined impact of all Industry 4.0 technologies on the healthcare system and an investigation into if there is a trade-off for investing between various digital technologies would be helpful. Future research can also investigate how machine learning techniques impact diagnostic, maintenance, and prognostics systems in healthcare settings. Research highlights the intricate issues of human–machine interaction at the workplace (Arslan et al., 2021 ); we suggest further research on how to maintain trustworthiness and to harmonise productive human–machine interactions and reduce workers’ resistance to automated machines in the healthcare settings. We also highlight the need for further research on the application of artificial intelligence in healthcare OSCM.
5.3 Sustainable operations and supply chain
Today sustainability is a growing concern of most organisations and therefore the topic of sustainability has received increasing focus from researchers from operations management. Healthcare systems worldwide face pressing challenges of service quality and cost issues as well as safe disposal of waste. As such, in recent times considerable attempts have been made to realise sustainability in the healthcare system. Chauhan and Singh ( 2016 ) employed a hybrid model for the selection of sustainable locations to dispose of healthcare waste. Anuar et al. ( 2018 ) offered a conceptual framework demonstrating the link between lean practices and a sustainable healthcare system. Malekpoor et al. ( 2018 ) modelled a sustainable healthcare treatment plan. Thorsen and McGarvey ( 2018 ) investigated the effectiveness of fixed and mobile dentistry for the financial sustainability of the healthcare system. Mousa and Othman ( 2020 ) found a positive impact of green human resource management practices on a sustainable healthcare system.
While research on healthcare sustainability is growing, there is still space for further research. For instance, more studies are needed that simultaneously investigate the impact of all three dimensions (social, environmental, economic) of sustainability through a single model. Prospective studies can also investigate the combined impact of synchronous business activities including green human resource management, green marketing, and green production on healthcare sustainability. There is also an opportunity to develop a sustainable performance measurement and management model in healthcare.
5.4 Circular economy
The circular economy is a recent concept aimed to eliminate waste through the make, use, recycle and reuse approach. It helps to overcome a take-make-dispose linear pattern of production and consumption. The healthcare industry inevitably generates waste that may become hazardous to both public health and the environment. Therefore, the circular economy has recently gained significant attention in healthcare literature. For example, Kumar and Rahman ( 2014 ) suggested RFID supported process reengineering for waste management ensuring circular economy. Jensen et al. ( 2019 ) discussed the healthcare refurbish system as a means to reduce waste. Dehghani et al. ( 2019 ) suggested that transhipment can reduce waste and save substantial costs compared to no-transhipment. Viegas et al. ( 2019 ) identified the need for research on the circularity of end-of-use medicine in the pharmaceutical supply chain.
Although research circular economy has witnessed mounting attention, there is still room for further research. For instance, there is a need to develop a model that could make the circularity of outdated medicine more visible through all stages of reverse flow and re-manufacturing. Also, healthcare literature lacks studies modelling processes and decision-making for circular economy implementation at micro and meso levels. Prospective research can also explore the healthcare staff’s perception of the circular economy. It would be interesting to investigate how healthcare professionals are prepared to deal with the circular economy and how they might contribute to reducing wastage in reverse flow.
5.5 Service ecosystem
With its root in marketing, the service ecosystems concept is defined as “relatively self-contained, self-adjusting systems of resource-integrating actors connected by shared institutional logics and mutual value creation through service exchange” (Vargo & Akaka, 2012 , p. 207). That is, the service ecosystem generates value for all the actors in a network through resource integration, value co-creation, and co-innovation. The service ecosystem concept has received a great deal of attention in healthcare literature. Nudurupati et al. ( 2015 ) elaborate on the process of value co-creation through strategic alliance and collaboration with multi-stakeholders. Albarune et al. ( 2015 ) suggested a value-based supply chain for integrated hospital management. Chae ( 2019 ) suggested digital innovation as a promising source of resource sharing and value creation among actors in an ecosystem. Lee et al. ( 2020 ) showed a positive relationship between the previous year value-based purchasing penalty and the current year care process improvement in the hospital.
Service ecosystem research is a growing area of research in the healthcare context; thus, offering opportunities for further expansion. For example, the influence of contextual behavioural factors in value co-creation and unlocking innovation needs further investigation. Also, service ecosystem research needs to be expanded from the organisational level to the entire healthcare system. For example, how resource integration practice can be integrated into a multitude of value co-creation processes in the healthcare networks. Moreover, the role of advanced technologies and servitisation in the healthcare service ecosystem has yet to be investigated.
5.6 Knowledge management
Knowledge management refers to the process of generating, transferring, and managing knowledge and information in an organisation or network. Knowledge management becomes a major driver of performance and productivity where front-line workers are required to provide customised services tailored to the need of individual clients such as healthcare. As such, the literature has witnessed considerable growth in literature on knowledge management in healthcare settings. Gagnon et al. ( 2016 ) empirically corroborated a positive association between knowledge management on staff practices and quality of healthcare. Mura et al. ( 2016 ) found that knowledge exchange and assets have a positive impact on workers’ innovation, such as idea generation, idea promotion and idea implementation. Avgerinos and Gokpinar ( 2017 ) argued that knowledge transfer capability in a team positively influences healthcare productivity. Hiranrithikorn and Sutduean ( 2019 ) expound that access to information and knowledge has a positive influence on healthcare supply chain skills. Despite considerable contributions to knowledge management, there is still significant room for further research. A few models are investigating the impact of knowledge sharing on the quality and productivity of healthcare performance. Qualitative research can be conducted to explore the barrier and enablers of knowledge sharing in healthcare. Future research can use multi-method research investigating the relationship between social capital, culture, and knowledge management.
5.7 Behavioural operations
Research on behavioural operations is well-received, particularly from healthcare service perspectives. Healthcare systems have two main stakeholders: staff and patients. The research suggests that the findings of healthcare modelling may not be reliable without considering the behaviours of the main stakeholders (Brailsford et al., 2012 ). For example, a simulation model suggesting the effectiveness of a new drug will be unreliable if it does not consider patient behaviour; some patients may not complete the prescribed course of medication, and potential side effects of medicine should be shown. As such, healthcare literature has seen an emergence of interest in understanding both human behaviour in practice and how to capture it in operations research models. Brailsford et al. ( 2012 ) used a simulation model capturing behavioural factors of a patient while treating breast cancer. Based on a review of literature, Kunc et al. ( 2018 ) identified scarcity of research on behavioural aspects of healthcare. Harper ( 2019 ) used a queuing model to assess how healthcare workers behave and treat patients, especially when such professionals encounter changing workloads, service queues, and other factors that impact service quality.
To sum, behavioural operational research has become an important research topic in healthcare but significant room for further research in the area remains intact. Specifically, we suggest more research with detailed models on the nexus between behavioural interventions on healthcare practice. Future research can model workforce adaptive behaviours and productivity. We agree that the incorporation of behavioural factors is important to increase the reliability of the healthcare model because of the increasing influence of human behaviours in healthcare operations. Future research can also examine the behavioural consequences of inter-organisational interaction in complex healthcare systems.
5.8 Risk and resilience
Healthcare systems are susceptible to operational failures in services offered to patients when a shortage of medicine and equipment occurs, or when the execution of such systems is incorrectly managed. Given the divergent risks emerging from a variety of sources, there is an increasing focus on risk mitigation and the development of resilient healthcare. Nemeth et al. ( 2011 ) developed a model for healthcare crises management and resilience. Mahjoub et al. ( 2014 ) found risk mitigation in healthcare through a risk-sharing agreement between pharmaceutical companies and healthcare service providers. Saedi et al. ( 2016 ) suggested an optimal inventory policy to reduce drug shortage risks. Rubbio et al. ( 2019 ) examined the role of digital technologies in developing healthcare resilience. Rahimian et al. ( 2019 ) investigated an appropriate level of risk-aversion through robust optimization. Zhang et al. ( 2020 ) formulated a model which helps to reduce overtime risk during surgery in a hospital.
While research on risk and resilience in healthcare is growing, there is still substantial room for more impactful future research. Most models investigate risk and resilience in isolation. Others focus on particular functions such as hospital surgery. As such, a quantitative model that simultaneously investigates risk and resilience focusing on the key supply chain actors in healthcare is lacking. Future research can also use a mixed-methods approach where qualitative research can explore various risk factors and resilience strategies, while quantitative research can test the interrelationship between risk and resilience in explaining healthcare performance.
5.9 Humanitarian logistics
Humanitarian logistics refers to the transportation, delivery, and distribution of supplies in the event of natural disasters or another emergency to the affected area and people. Given the unprecedented incidents such as floods, cyclones, disease outbursts, the humanitarian logistics in healthcare (e.g., supplies of medicines, medical equipment, sterile items, linen, and food) has gained significant attention in recent times. For example, Naor et al. ( 2018 ) found strategies for effective humanitarian logistics in healthcare, such as quickly sending a team to the disaster area to gather firsthand information on the unique situations of disaster followed by the main delegation staff carrying effective disaster relief service. Prasad et al. ( 2018 ) suggested effective healthcare intervention to deal with disease outbreaks in a community. Cherkesly et al. ( 2019 ) assist with the design of a community healthcare network, increasing the service coverage for underserved areas in adverse situations.
Although research on humanitarian logistics has been progressing steadily, we highlight the need for further research on healthcare OSCM. A fruitful future research avenue is modelling the role of volunteers and NGOs in humanitarian logistics from a healthcare perspective. Also, interviews with disaster area patients can provide additional insights into the real-world phenomenon. Further research can also develop a model of budget allocation as the community service demand increases drastically due to a natural disaster. It would be interesting to investigate the nexus among service compliance rate, disease rate, and total cost by disaster type.
5.10 Pharmaceutical OSCM
A pharmaceutical supply chain involves firms, processes, and operations involved in the design, development, and distribution of life-saving drugs. It is one of the critical supply chains since it is related to the life and health of people. The success of healthcare services directly depends on well-managed pharmaceutical supplies. Therefore, the management of the pharmaceutical supply chain has become a popular topic in healthcare literature. Jia and Zhao ( 2017 ) highlighted the issue of drug shortage due to lack of collaboration between pharmacies and pharmaceutical manufacturing and supplier. Nematollahi et al. ( 2018 ) also found a positive link between hospital service level and efficient pharmaceutical supply chain. Viegas et al. ( 2019 ) discussed the significance of a coordinated pharmaceutical supply chain for the reverse flow of end-of-life medicine. Recently, Zandkarimkhani et al. ( 2020 ) formulated a model to redesign the pharmaceutical supply chain network in the wake of demand uncertainty.
Despite growth in literature on the pharmaceutical supply chain, significant room for further research still exists, particularly in the context of a recent pandemic. Most of the existing research lacks a holistic view that involves all key stakeholders of the supply chain. There is less focus on upstream networks and processes (Narayana et al., 2014 ). There is also limited research on integrated new product development in the pharmaceutical supply chain. There is also an opportunity for research to model examining the link between healthcare financing and pharmaceutical supply chain financing such as investment in R&D, production and distribution, between waste management, environmental and pharmaceutical supply chains. Future studies can also model the behaviour of individuals in the robustness of pharmaceutical supply chains. Given that supply chain design is critical in the planning process (Govindan et al., 2017 ), we suggest designing integrated models that encompass all stages from drug manufacturing to final consumer for robust decision-making in the pharmaceutical supply chain. Another interesting avenue is formulating a model at the intersection of supplier selection and sustainable pharmaceutical supply chain networks.
5.11 Climate change and healthcare
The increased intensity and frequency of disasters due to extreme weather conditions draw our attention to the unprecedented changes in climate which are mainly attributed to excessive greenhouse gas emissions and carbon footprints. To deal with the climate change challenges, greenhouse gas mitigation targets were set to a minimum level in the historic Paris Agreement (Bodansky, 2016 ). While a lot of research spotlights the emissions reduction in the automotive, energy, and mining industries, the carbon footprint and climate change research has recently received attention. Rezali et al. ( 2018 ) discussed the importance of a green healthcare supply chain to reduce the environmental impact of the healthcare industry. Belkhir and Elmeligi ( 2019 ) examined 15 pharmaceutical supply chains across different countries and found that the pharmaceutical industry is more emission-intensive than the automotive industry. Tanwar et al. ( 2019 ) identify the potential risks and risk mitigation measures in implementing a green pharmaceutical supply chain. Over the past five years, the topic of climate change has received increasing attention in healthcare settings. Most studies on the topic focus on factors contributing to climate change (greenhouse gas emissions, carbon footprint), thus leaving a gap for research models incorporating the business impact of climate risks when they unfold and examining specific risk mitigation measures.
5.12 Healthcare logistics
Healthcare logistics refer to the physical flow of healthcare materials from the pharmaceutical production facility to the healthcare service provider. Logistics cost has been identified as the second largest cost after personnel in the healthcare system (Ross & Jayaraman, 2009 ). As such, the importance of material flow in healthcare service is recognised important to add value and reduce the cost of services. Correspondingly, a growth in literature on healthcare logistics is evident. Oumlil and Williams ( 2011 ) suggested strategic alliance and consolidation of logistics in the healthcare sector as a strategy to reduced logistics costs. Volland et al. ( 2017 ) identified four main domains in which healthcare logistics research exists, including procurement, inventory management, distribution, and supply chain management. Al-Sharhan et al. ( 2019 ) expounded on the significance of hospital-supplier integration of inefficient material logistics. Fathollahi-Fard et al. ( 2019 ) developed a green home healthcare supply chain model to reduce emissions in logistics. Pohjosenperä et al. ( 2019 ) discussed the impact of service modularity in value-creating and improving healthcare logistics service. Zabinsky et al. ( 2020 ) developed a model through mixed-integer linear programming to address healthcare scheduling and routing problem. Further research could examine an item-level analysis of different product categories in the regional healthcare units, together with their replenishment models. Such a study is needed to show the true potential of modularity in service offerings of the logistics support organisation.
6 Conclusion
Over the last two decades, research on healthcare OSCM has been continuously advancing making it a self-sustained discipline in the OSCM field. We argue that with this unprecedented development in healthcare literature, the current status and trends in the field need to be mapped. The use of robust statistical and automatic methods for extracting key topics from a large set of articles has been increasingly used in other fields such as finance, marketing, statistics, tourism, and computer sciences. Borrowing the knowledge from these adjacent fields, this study employs probabilistic topic modelling with LDA as an automatic text analytics approach to unpack dominant topics and development trajectories in a large collection of research articles on healthcare OSCM. In doing so, we contribute to the literature on new and rigorous methods for systematic literature review in the field of OSCM. Our sample included 571 articles retrieved from 20 journals in the field of OSCM. The results produced 34 topics on healthcare research. Analysis of dynamic changes in the 34 over the past twenty years suggested 24 hot, 8 cold, and 2 steady topics (see Table 3 ).
Our findings show a shift of current research from traditional topics of operations management (Business process reengineering, Marketing, Outsourcing, Heuristics) to emergent topics such as Patient waiting for time, Industry 4.0 technologies, Sustainable operations, Risk and resilience, Climate change, and Circular economy. In general, the shift in topics seems to show a move away from traditional, efficiency-focused research toward broader goals of superior customer service and system’s effectiveness. The data on research methods shows a larger dominance of modelling research (e.g., Markov, mixed-integer programming, simulation, queueing model, and stochastic) in exploring multidimensional issues of healthcare operations. However, the past three years have also seen some empirical research, including Structural equation modelling, Longitudinal study, and Action research. This shows a positive change in operations research journals from purely quantitative modelling studies to empirical research as well. This recent shift in a strategy not only offers a great opportunity to empirical researchers for publication in some OR journals but also provides sound premises on which to expand the scope and subscription of these journals.
The findings of this research offer useful research directions to researchers, journal editors, and policymakers in the field of healthcare research. Researchers can observe whether their recent research topics are hot, cold, or steady, and accordingly select appropriate topics for more impactful future research in the area. Particularly, some early-career researchers find it difficult to keep up with the growing literature in their fields of research and familiarise themselves with a huge volume of literature in identifying the subject area on which they can focus or apply for a research grant. The information from our topic modelling can be a useful tool for these researchers to understand recent developments in the field and then select more appropriate topics. Our findings can also be helpful to PhD students, who often face difficulties in topic selection due to time pressure. Journal editors can view if the extant research is consistent with their editorial policy, and they may like to launch a new editorial vision and direction. Our list of hot topics can be a valuable reference tool to launch special issues in the journals. Discovering underlying topics and tracking their evolution could also be of great interest to policymakers (e.g., government agencies) and industry. The information on which research themes and methods are growing or declining in popularity over time (Table 3 and Fig. 4 ) can support governmental funding agencies for grants allocation to promising areas. Likewise, firm managers and the industry as a whole can use this information to formulate promising R&D strategies and to make more informed investment decisions.
We believe that topic modelling is one of the most effective and reliable approaches in revealing the latent (hidden) structure and development of research topics in a field, and recommend that future research can combine the LDA-based models with other text analytics techniques from different text data (e.g., customer feedback, medical records, research reports, business reviews, company descriptions) and interconnection among various business areas (e.g., marketing, supply chain, information systems). For example, sentiment analysis (Pang & Lee, 2008 ) is deemed a popular text analytics tool in the field of finance (Sul et al., 2017 ), marketing (Liang et al., 2015 ), and operations/supply chain (Chae, 2015 ). The sentiment analysis can be integrated with topic modelling: a two-pronged method using topic modelling followed by sentiment analysis can offer more nuanced findings by revealing both positive and negative aspects of a product and/or service.
As with several studies, there are some limitations of our study. First, given the authors’ extensive knowledge of the field and review of the extant literature, full efforts were made to guarantee that all pertinent articles were included in the analysis. However, some articles might have been ignored. Nevertheless, two measures might help mitigate this limitation: (1) the topics are generated based on the frequencies of the keywords/key terms and, therefore, missing a few articles from such a large dataset (571 articles) will not have a significant impact on overall results; (2) the dataset is validated with other databases. Second, the research is limited to healthcare research published in OSCM journals. That is, we excluded the papers and related journals containing healthcare research but not from OSCM perspectives.
Abo-Hamad, W., & Arisha, A. (2013). Simulation-based framework to improve patient experience in an emergency department. European Journal of Operational Research, 224 (1), 154–166.
Article Google Scholar
Albarune, A. R. B., Farhat, N., & Afzal, F. (2015). The valued supply chain for integrated hospital management: A conceptual framework. International Journal of Supply Chain Management, 4 (3), 39–49.
Google Scholar
Ali, I. (2019). The impact of industry 4.0 on the nexus between supply chain risks and firm performance. In A. Guclu (Ed. 2019) Academy of Management Proceedings (1–6). Boston: Academy of Management.
Ali, I., Arslan, A., Khan, Z., & Tarba, S. Y. (2021a). The role of industry 4.0 technologies in mitigating supply chain disruption: Empirical evidence from the Australian Food Processing Industry. IEEE Transactions on Engineering Management, 1–11. https://doi.org/10.1109/TEM.2021.3088518
Ali, I., & Aboelmaged, M. G. S. (2021). Implementation of supply chain 4.0 in the food and beverage industry: Perceived drivers and barriers. International Journal of Productivity and Performance Management , ahead-of-print (ahead-of-print). https://doi.org/10.1108/IJPPM-07-2020-0393
Ali, I., & Gölgeci, I. (2019). Where is supply chain resilience research heading? A systematic and co-occurrence analysis. International Journal of Physical Distribution and Logistics Management, 49 (8), 793–815.
Ali, I., & Govindan, K. (2021). Extenuating operational risks through digital transformation of agri-food supply chains. Production Planning & Control . https://doi.org/10.1080/09537287.2021.1988177
Ali, I., Sultan, P., & Aboelmaged, M. (2021b). A bibliometric analysis of academic misconduct research in higher education: Current status and future research opportunities. Accountability in Research, 28 (6), 372–393.
Ali, O., Shrestha, A., Soar, J., & Wamba, S. F. (2018). Cloud computing-enabled healthcare opportunities, issues, and applications: A systematic review. International Journal of Information Management, 43 (6), 146–158.
Al-Sharhan, S., Omran, E., & Lari, K. (2019). An integrated holistic model for an eHealth system: A national implementation approach and a new cloud-based security model. International Journal of Information Management, 47 (1), 121–130.
Antons, D., Kleer, R., & Salge, T. O. (2016). Mapping the topic landscape of JPIM, 1984–2013: In search of hidden structures and development trajectories. Journal of Product Innovation Management, 33 (6), 726–749.
Anuar, A., Saad, R., & Yusoff, R. Z. (2018). Sustainability through lean healthcare and operational performance in the private hospitals: A proposed framework. Supply Chain Management, 7 (5), 221–227.
Arslan, A., Cooper, C., Khan, Z., Golgeci, I., & Ali, I. (2021). Artificial intelligence and human workers interaction at the team level: a conceptual assessment of the challenges and potential HRM strategies. International Journal of Manpower, ahead-of-print (ahead-of-print). https://doi.org/10.1108/IJM-01-2021-0052
Asgari, F., & Asgari, S. (2021). Addressing artificial variability in patient flow. Operations Research for Health Care, 28 , 100288. https://doi.org/10.1016/j.orhc.2021.100288
Avgerinos, E., & Gokpinar, B. (2017). Team familiarity and productivity in cardiac surgery operations: The effect of dispersion, bottlenecks, and task complexity. Manufacturing & Service Operations Management, 19 (1), 19–35.
Belkhir, L., & Elmeligi, A. (2019). Carbon footprint of the global pharmaceutical industry and relative impact of its major players. Journal of Cleaner Production, 214 (1), 185–194.
Blei, D. M. (2012). Probabilistic topic models. Communications of the ACM, 55 (4), 77–84.
Bodansky, D. (2016). The Paris climate change agreement: A new hope? American Journal of International Law, 110 (2), 288–319.
Brailsford, S. C., Harper, P. R., & Sykes, J. (2012). Incorporating human behaviour in simulation models of screening for breast cancer. European Journal of Operational Research, 219 (3), 491–507.
Chae, B., & Olson, D. (2018). A topical exploration of the intellectual development of decision sciences 1975–2016. Decision Sciences, Published (online) , 1–24.
Chae, B. K. (2015). Insights from hashtag# supplychain and Twitter Analytics: Considering Twitter and Twitter data for supply chain practice and research. International Journal of Production Economics, 165 (3), 247–259.
Chae, B. K. (2019). A General framework for studying the evolution of the digital innovation ecosystem: The case of big data. International Journal of Information Management, 45 (2), 83–94.
Chauhan, A., & Singh, A. (2016). A hybrid multi-criteria decision making method approach for selecting a sustainable location of healthcare waste disposal facility. Journal of Cleaner Production, 139 (4), 1001–1010.
Cherkesly, M., Rancourt, M. È., & Smilowitz, K. R. (2019). Community healthcare network in underserved areas: Design, mathematical models, and analysis. Production and Operations Management, 28 (7), 1716–1734.
Choi, T.-M. (2021). Fighting against COVID-19: What operations research can help and the sense-and-respond framework. Annals of Operations Research . https://doi.org/10.1007/s10479-021-03973-w
Chong, A.Y.-L., Liu, M. J., Luo, J., & Keng-Boon, O. (2015). Predicting RFID adoption in healthcare supply chain from the perspectives of users. International Journal of Production Economics, 159 (5), 66–75.
De Battisti, F., Ferrara, A., & Salini, S. (2015). A decade of research in statistics: A topic model approach. Scientometrics, 103 (2), 413–433.
Deerwester, S., Dumais, S. T., Furnas, G. W., Landauer, T. K., & Harshman, R. (1990). Indexing by latent semantic analysis. Journal of the American Society for Information Science, 41 (6), 391–407.
Dehghani, M., Abbasi, B., & Oliveira, F. (2019). Proactive transshipment in the blood supply chain: A stochastic programming approach. Omega , Published (online), 102–112.
Diwas Singh, K. C., Scholtes, S., & Terwiesch, C. (2020). Empirical research in healthcare operations: Past research, present understanding, and future opportunities. Manufacturing and Service Operations Management, 22 (1), 73–83.
Fan, Z., & Xie, X. (2022). A distributionally robust optimisation for COVID-19 testing facility territory design and capacity planning. International Journal of Production Research, 1–24.
Fan, W., Liu, J., Zhu, S., & Pardalos, P. M. (2018). Investigating the impacting factors for the healthcare professionals to adopt artificial intelligence-based medical diagnosis support system (AIMDSS). Annals of Operations Research . https://doi.org/10.1007/s10479-018-2818-y
Fathollahi-Fard, A. M., Govindan, K., Hajiaghaei-Keshteli, M. and Ahmadi, A. (2019). A green home health care supply chain: New modified simulated annealing algorithms. Journal of Cleaner Production, 240, pp. ahead-of-print.
Fattahi, M., Keyvanshokooh, E., Kannan, D., & Govindan, K. (2022). Resource planning strategies for healthcare systems during a pandemic. European Journal of Operational Research .
Ferreira, F. A., Kannan, D., Meidutė-Kavaliauskienė, I., & Vale, I. M. (2022). A sociotechnical approach to vaccine manufacturer selection as part of a global immunization strategy against epidemics and pandemics. Annals of Operations Research, 1–30.
Ferreira, F. A. (2018). Mapping the field of arts-based management: Bibliographic coupling and co-citation analyses. Journal of Business Research, 85 (2), 348–357.
Gagnon, M.-P., Simonyan, D., Godin, G., Labrecque, M., Ouimet, M., & Rousseau, M. (2016). Factors influencing electronic health record adoption by physicians: A multilevel analysis. International Journal of Information Management, 36 (3), 258–270.
Galetsi, P., Katsaliaki, K., & Kumar, S. (2020). Big data analytics in health sector: Theoretical framework, techniques and prospects. International Journal of Information Management, 50 (4), 206–216.
Gartner, D., & Padman, R. (2019). Machine learning for healthcare behavioural OR: Addressing waiting time perceptions in emergency care. Journal of the Operational Research Society, 71 (7), 1081–1101.
Ghaderi, M. (2022). Public health interventions in the face of pandemics: Network structure, social distancing, and heterogeneity. European Journal of Operational Research, 298 (3), 1016–1031.
Govindan, K., Nasr, A. K., Mostafazadeh, P., & Mina, H. (2021). Medical waste management during coronavirus disease 2019 (COVID-19) outbreak: A mathematical programming model. Computers & Industrial Engineering , 162 , 107668.
Govindan, K., Nasr, A. K., Saeed Heidary, M., Nosrati-Abargooee, S., & Mina, H. (2022). Prioritizing adoption barriers of platforms based on blockchain technology from balanced scorecard perspectives in healthcare industry: A structural approach. International Journal of Production Research, 1–15.
Govindan, K., Fattahi, M., & Keyvanshokooh, E. (2017). Supply chain network design under uncertainty: A comprehensive review and future research directions. European Journal of Operational Research, 263 (1), 108–141.
Govindan, K., Mina, H., & Alavi, B. (2020). A decision support system for demand management in healthcare supply chains considering the epidemic outbreaks: A case study of coronavirus disease 2019 (COVID-19). Transportation Research Part e: Logistics and Transportation Review . https://doi.org/10.1016/j.tre.2020.101967
Griffiths, T. L., & Steyvers, M. (2004). Finding scientific topics. Proceedings of the National Academy of Sciences, 101 (1), 5228–5235.
Gu, W., Fan, N., & Liao, H. (2019). Evaluating readmission rates and discharge planning by analyzing the length-of-stay of patients. Annals of Operations Research, 276 (1–2), 89–108.
Guo, Y., Barnes, S. J., & Jia, Q. (2017). Mining meaning from online ratings and reviews: Tourist satisfaction analysis using latent dirichlet allocation. Tourism Management, 59 (2), 467–483.
Harper, P. R. (2019). Server behaviours in healthcare queueing systems. Journal of the Operational Research Society, 71 (4), 1124–1136.
Hejazi, T.-H. (2021). State-dependent resource reallocation plan for health care systems: A simulation optimization approach. Computers & Industrial Engineering, 159 , 107502. https://doi.org/10.1016/j.cie.2021.107502
Hiranrithikorn, P., & Sutduean, J. (2019). Social capital predicting the supply chain skills: Mediating role of access to information and network resources. International Journal of Supply Chain Management, 8 (5), 842–853.
Hofmann, T. (2001). Unsupervised learning by probabilistic latent semantic analysis. Machine Learning, 42 (1–2), 177–196.
Hornik, K., & Grün, B. (2011). topicmodels: An R package for fitting topic models. Journal of Statistical Software, 40 (13), 1–30.
Jensen, J. P., Prendeville, S. M., Bocken, N. M., & Peck, D. (2019). Creating sustainable value through remanufacturing: Three industry cases. Journal of Cleaner Production, 218 (4), 304–314.
Jia, J., & Zhao, H. (2017). Mitigating the US drug shortages through pareto-improving contracts. Production and Operations Management, 26 (8), 1463–1480.
Jiang, H., Qiang, M., & Lin, P. (2016). A topic modeling based bibliometric exploration of hydropower research. Renewable and Sustainable Energy Reviews, 57 (3), 226–237.
Keskinocak, P., & Savva, N. (2020). A review of the healthcare-management (Modeling) literature published in manufacturing & service operations management. Manufacturing & Service Operations Management, 22 (1), 59–72.
Kochan, C. G., Nowicki, D. R., Sauser, B., & Randall, W. S. (2018). Impact of cloud-based information sharing on hospital supply chain performance: A system dynamics framework. International Journal of Production Economics, 195 (5), 168–185.
Kumar, A., & Rahman, S. (2014). RFID-enabled process reengineering of closed-loop supply chains in the healthcare industry of Singapore. Journal of Cleaner Production, 85 (2), 382–394.
Kunc, M., Harper, P., & Katsikopoulos, K. (2018). A review of implementation of behavioural aspects in the application of OR in healthcare. Journal of the Operational Research Society, 71 (7), 1055–1072.
Lee, D. D., & Seung, H. S. (1999). Learning the parts of objects by non-negative matrix factorization. Nature, 401 (6755), 788–791.
Lee, H., & Kang, P. (2018). Identifying core topics in technology and innovation management studies: A topic model approach. The Journal of Technology Transfer, 43 (5), 1291–1317.
Lee, S. J., Venkataraman, S., Heim, G. R., Roth, A. V., & Chilingerian, J. (2020). Impact of the value-based purchasing program on hospital operations outcomes: An econometric analysis. Journal of Operations Management, 66 (1–2), 151–175.
Liang, T.-P., Li, X., Yang, C.-T., & Wang, M. (2015). What in consumer reviews affects the sales of mobile apps: A multifacet sentiment analysis approach. International Journal of Electronic Commerce, 20 (2), 236–260.
Mahjoub, R., Odegaard, F., & Zaric, G. S. (2014). Health-based pharmaceutical pay-for-performance risk-sharing agreements. Journal of the Operational Research Society, 65 (4), 588–604.
Malekpoor, H., Mishra, N., & Kumar, S. (2018). A novel TOPSIS–CBR goal programming approach to sustainable healthcare treatment. Annals of Operations Research, 1–23.
Malik, M. M., Abdallah, S., & Ala’raj, M. (2018). Data mining and predictive analytics applications for the delivery of healthcare services: A systematic literature review. Annals of Operations Research, 270 (1–2), 287–312.
Mimno, D., & McCallum, A. (2012). Topic models conditioned on arbitrary features with Dirichlet-multinomial regression. UAI, 24 , 1–8.
Moons, K., Waeyenbergh, G., & Pintelon, L. (2019). Measuring the logistics performance of internal hospital supply chains—a literature study. Omega (united Kingdom), 82 (3), 205–217.
Mousa, S. K., & Othman, M. (2020). The impact of green human resource management practices on sustainable performance in healthcare organisations: A conceptual framework. Journal of Cleaner Production, 243 (2), 118595.
Mura, M., Lettieri, E., Radaelli, G., & Spiller, N. (2016). Behavioural operations in healthcare: A knowledge sharing perspective. International Journal of Operations & Production Management, 36 (10), 1222–1246.
Nagurney, A. (2021a). Supply chain game theory network modeling under labor constraints: Applications to the Covid-19 pandemic. European Journal of Operational Research, 293 (3), 880–891.
Naor, M., Dey, A., Meyer Goldstein, S., & Rosen, Y. (2018). Civilian-military pooling of health care resources in Haiti: A theory of complementarities perspective. International Journal of Production Research, 56 (21), 6741–6757.
Narayana, S. A., Pati, R. K., & Vrat, P. (2014). Managerial research on the pharmaceutical supply chain—a critical review and some insights for future directions. Journal of Purchasing and Supply Management, 20 (1), 18–40.
Nematollahi, M., Hosseini-Motlagh, S.-M., Ignatius, J., Goh, M., & Nia, M. S. (2018). Coordinating a socially responsible pharmaceutical supply chain under periodic review replenishment policies. Journal of Cleaner Production, 172 (3), 2876–2891.
Nemeth, C., Wears, R. L., Patel, S., Rosen, G., & Cook, R. (2011). Resilience is not control: healthcare, crisis management, and ICT. Cognition, Technology & Work , 13 (3), 189–202.
Nudurupati, S. S., Bhattacharya, A., Lascelles, D., & Caton, N. (2015). Strategic sourcing with multi-stakeholders through value co-creation: An evidence from global health care company. International Journal of Production Economics, 166 (5), 248–257.
Oumlil, A. B., & Williams, A. J. (2011). Strategic alliances and organisational buying: An empirical study of the healthcare industry. International Journal of Procurement Management, 4 (6), 610–626.
Pamucar, D., Torkayesh, A. E., & Biswas, S. (2022). Supplier selection in healthcare supply chain management during the COVID-19 pandemic: A novel fuzzy rough decision-making approach. Annals of Operations Research, 1–43.
Pan, X., Geng, N., Xie, X., & Wen, J. (2019). Managing appointments with waiting time targets and random walk-ins. Omega , 95 , 102062.
Pan, X., Geng, Na., & Xie, X. (2021). Appointment scheduling and real-time sequencing strategies for patient unpunctuality. European Journal of Operational Research, 295 (1), 246–260.
Pang, B., & Lee, L. (2008). Opinion mining and sentiment analysis. Foundations and Trends® in Information Retrieval, 2 (1–2), 1–135.
Pohjosenperä, T., Kekkonen, P., Pekkarinen, S., & Juga, J. (2019). Service modularity in managing healthcare logistics. The International Journal of Logistics Management, 3 (1), 174–191.
Prasad, S., Sundarraj, R., Tata, J., & Altay, N. (2018). Action-research-based optimisation model for health care behaviour change in rural India. International Journal of Production Research, 56 (21), 6774–6792.
Priya, M., & Ranjith Kumar, P. (2015). A novel intelligent approach for predicting atherosclerotic individuals from big data for healthcare. International Journal of Production Research, 53 (24), 7517–7532.
Rahimian, H., Bayraksan, G., & Homem-de-Mello, T. (2019). Controlling risk and demand ambiguity in newsvendor models. European Journal of Operational Research, 279 (3), 854–868.
Rezali, N., Ali, M. H., & Idris, F. (2018). Empowering green healthcare supply chain management practices challenges and future research. International Journal of Supply Chain Management, 7 (5), 282–289.
Roberts, M. E., Stewart, B. M., Tingley, D., Lucas, C., Leder-Luis, J., Gadarian, S. K., et al. (2014). Structural topic models for open-ended survey responses. American Journal of Political Science , 58 (4), 1064–1082.
Ross, A. D., & Jayaraman, V. (2009). Strategic purchases of bundled products in a health care supply chain environment. Decision Sciences, 40 (2), 269–293.
Rubbio, I., Bruccoleri, M., Pietrosi, A., & Ragonese, B. (2019). Digital health technology enhances resilient behaviour: Evidence from the ward. International Journal of Operations & Production Management, 40 (1), 34–67.
Saedi, S., Erhun Kundakcioglu, O., & Henry, A. C. (2016). Mitigating the impact of drug shortages for a healthcare facility: An inventory management approach. European Journal of Operational Research, 251 (1), 107–123.
Salarpour, M., & Nagurney, A. (2021). A multicountry, multicommodity stochastic game theory network model of competition for medical supplies inspired by the Covid-19 pandemic. International Journal of Production Economics, 236 , 108074. https://doi.org/10.1016/j.ijpe.2021.108074
Shehadeh, K. S., Cohn, A. E. M., & Epelman, M. A. (2019). Analysis of models for the Stochastic Outpatient Procedure Scheduling Problem. European Journal of Operational Research, 279 (3), 721–731.
Silge, J., & Robinson, D. (2016). tidytext: Text mining and analysis using tidy data principles in R. Journal of Open Source Software, 1 (3), 37–45.
Stevens, K., Kegelmeyer, P., Andrzejewski, D., & Buttler, D. (2012). Exploring topic coherence over many models and many topics . Paper presented at the proceedings of the 2012 joint conference on empirical methods in natural language processing and computational natural language learning .
Sul, H. K., Dennis, A. R., & Yuan, L. (2017). Trading on twitter: Using social media sentiment to predict stock returns. Decision Sciences, 48 (3), 454–488.
Sultan, N. (2014). Making use of cloud computing for healthcare provision: Opportunities and challenges. International Journal of Information Management, 34 (2), 177–184.
Tanwar, T., Kumar, U. D., & Mustafee, N. (2019). Optimal package pricing in healthcare services. Journal of the Operational Research Society , published online, 1–13.
Tavana, M., Govindan, K., Nasr, A. K., Heidary, M. S., & Mina, H. (2021). A mathematical programming approach for equitable COVID-19 vaccine distribution in developing countries. Annals of Operations Research . https://doi.org/10.1007/s10479-021-04130-z
Thakur, V. (2021). Framework for PESTEL dimensions of sustainable healthcare waste management: Learnings from COVID-19 outbreak. Journal of Cleaner Production, 287 , 125562. https://doi.org/10.1016/j.jclepro.2020.125562
Thorsen, A., & McGarvey, R. G. (2018). Efficient frontiers in a frontier state: Viability of mobile dentistry services in rural areas. European Journal of Operational Research, 268 (3), 1062–1076.
Tirunillai, S., & Tellis, G. J. (2014). Mining marketing meaning from online chatter: Strategic brand analysis of big data using latent dirichlet allocation. Journal of Marketing Research, 51 (4), 463–479.
Topuz, K., Uner, H., Oztekin, A., & Yildirim, M. B. (2018). Predicting pediatric clinic no-shows: A decision analytic framework using elastic net and Bayesian belief network. Annals of Operations Research, 263 (1–2), 479–499.
VanBerkel, P. T., & Blake, J. T. (2007). A comprehensive simulation for wait time reduction and capacity planning applied in general surgery. Health Care Management Science, 10 (4), 373–385.
Vargo, S. L., & Akaka, M. A. (2012). Value cocreation and service systems (re) formation: A service ecosystems view. Service Science, 4 (3), 207–217.
Viegas, C. V., Bond, A., Vaz, C. R., & Bertolo, R. J. (2019). Reverse flows within the pharmaceutical supply chain: A classificatory review from the perspective of end-of-use and end-of-life medicines. Journal of Cleaner Production , published online, 117719.
Vissers, J. M., Adan, I. J., & Dellaert, N. P. (2007). Developing a platform for comparison of hospital admission systems: An illustration. European Journal of Operational Research, 180 (3), 1290–1301.
Volland, J., Fügener, A., Schoenfelder, J., & Brunner, J. O. (2017). Material logistics in hospitals: A literature review. Omega (united Kingdom), 69 (3), 82–101.
Yan, E. (2014). Research dynamics: Measuring the continuity and popularity of research topics. Journal of Informetrics, 8 (1), 98–110.
Zabinsky, Z. B., Dulyakupt, P., Zangeneh-Khamooshi, S., Xiao, C., Zhang, P., Kiatsupaibul, S., & Heim, J. A. (2020). Optimal collection of medical specimens and delivery to central laboratory. Annals of Operations Research, 287 (1), 537–564.
Zandkarimkhani, S., Mina, H., Biuki, M., & Govindan, K. (2020). A chance constrained fuzzy goal programming approach for perishable pharmaceutical supply chain network design. Annals of Operations Research . https://doi.org/10.1007/s10479-020-03677-7
Zhang, Y., Wang, Y., Tang, J., & Lim, A. (2020). Mitigating overtime risk in tactical surgical scheduling. Omega, 93 , online 102024.
Zhang, L. (2012). Aspect and entity extraction from opinion documents . Springer.
Download references
Author information
Authors and affiliations.
School of Business and Law, CQ University, Rockhampton North Campus, Sydney, Australia
SDU- Center for Sustainable Supply Chain Engineering, Department of Technology and Innovation, University of Southern Denmark, Campusvej 55, Odense, Denmark
Devika Kannan
You can also search for this author in PubMed Google Scholar
Corresponding author
Correspondence to Devika Kannan .
Additional information
Publisher's note.
Springer Nature remains neutral with regard to jurisdictional claims in published maps and institutional affiliations.
Rights and permissions
Reprints and permissions
About this article
Ali, I., Kannan, D. Mapping research on healthcare operations and supply chain management: a topic modelling-based literature review. Ann Oper Res 315 , 29–55 (2022). https://doi.org/10.1007/s10479-022-04596-5
Download citation
Accepted : 14 February 2022
Published : 31 March 2022
Issue Date : August 2022
DOI : https://doi.org/10.1007/s10479-022-04596-5
Share this article
Anyone you share the following link with will be able to read this content:
Sorry, a shareable link is not currently available for this article.
Provided by the Springer Nature SharedIt content-sharing initiative
- Supply chain
- Topic modelling
- Literature review
- Find a journal
- Publish with us
- Track your research

151+ Public Health Research Topics [Updated 2024]
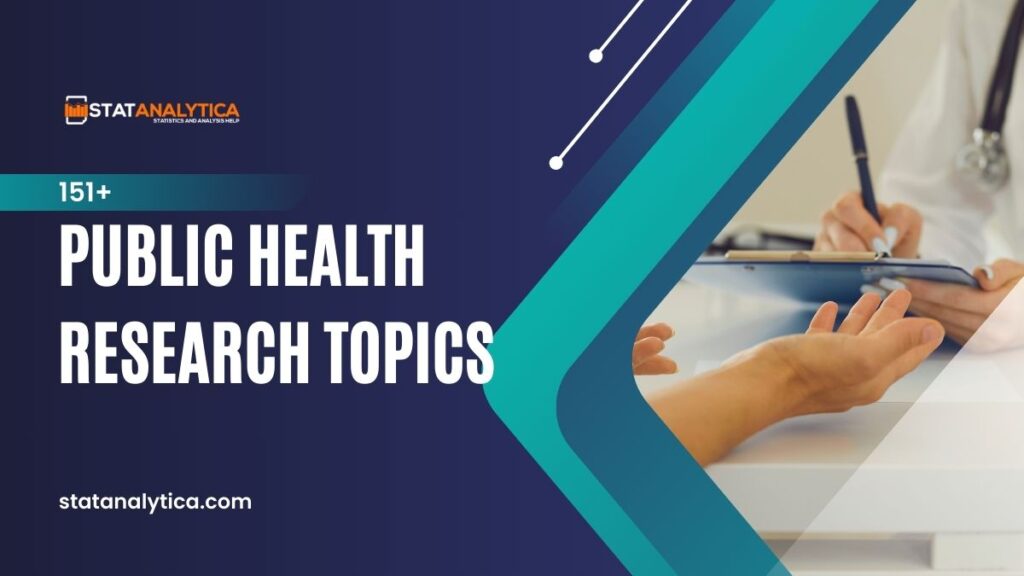
The important area of public health research is essential to forming laws, influencing medical procedures, and eventually enhancing community well-being. As we delve into the vast landscape of public health research topics, it’s essential to understand the profound impact they have on society.
This blog aims to provide a comprehensive guide to selecting and understanding the diverse array of public health research topics.
Overview of Public Health Research Topics
Table of Contents
Public health research encompasses a wide range of subjects, reflecting the interdisciplinary nature of the field. From epidemiology and health policy to environmental health and infectious diseases, researchers navigate through various dimensions to address complex health challenges.
Each category holds its own significance, contributing to the overall understanding of public health dynamics.
Key Considerations in Selecting Public Health Research Topics
- Current Relevance: Assess the timeliness of potential topics by considering recent health trends, emerging issues, and societal concerns.
- Impact on Public Health: Evaluate the potential impact of the research on improving health outcomes, addressing disparities, or influencing policy and interventions.
- Feasibility and Resources: Gauge the practicality of conducting research on a particular topic, considering available resources, data accessibility, and research infrastructure.
- Ethical Considerations: Scrutinize the ethical implications of the research, ensuring it aligns with ethical standards and guidelines, especially when dealing with vulnerable populations or sensitive topics.
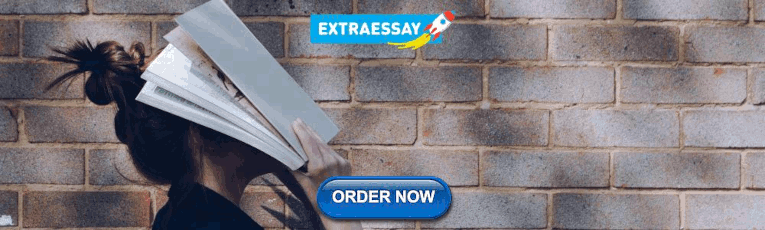
Top 151+ Public Health Research Topics
Epidemiology.
- The Impact of Social Determinants on Disease Outcomes
- Patterns and Trends in Emerging Infectious Diseases
- Investigating Health Disparities among Different Ethnic Groups
- Childhood Obesity and its Long-Term Health Consequences
- Assessing the Effectiveness of Contact Tracing in Disease Control
Health Policy
- Universal Healthcare: Comparative Analysis of Global Models
- The Role of Telemedicine in Improving Healthcare Access
- Evaluating Mental Health Policies and Their Impact on Communities
- Assessing the Impact of Affordable Care Act on Public Health
- Vaccine Policies and Public Perception: A Comprehensive Study
Environmental Health
- Climate Change and Health: Adapting to the Challenges
- Air Quality and Respiratory Health in Urban Environments
- Waterborne Diseases and Strategies for Safe Water Supply
- Occupational Health Hazards: A Comprehensive Workplace Analysis
- The Impact of Green Spaces on Mental Health in Urban Areas
Infectious Diseases
- Antimicrobial Resistance: Strategies for Mitigation
- Vaccination Strategies and Herd Immunity
- Global Health Security: Preparedness for Pandemics
- The Impact of Vector-Borne Diseases on Public Health
- Emerging Trends in Antibiotic-Resistant Infections
Chronic Diseases
- Lifestyle Interventions for Preventing Cardiovascular Diseases
- Genetic Factors in the Development of Cancer: A Comprehensive Study
- Aging and Health: Addressing the Healthcare Needs of the Elderly
- Diabetes Prevention Programs: Efficacy and Implementation
- Mental Health in Chronic Disease Patients: Bridging the Gap
Maternal and Child Health
- Maternal Mortality: Understanding Causes and Prevention
- The Impact of Breastfeeding on Infant Health and Development
- Childhood Immunization: Barriers and Strategies for Improvement
- Teenage Pregnancy and Its Long-Term Health Consequences
- Mental Health Support for Postpartum Women: Current Gaps and Solutions
Health Behavior and Promotion
- Smoking Cessation Programs: Effectiveness and Challenges
- Physical Activity Promotion in Schools: Strategies for Success
- Nutrition Education and Its Impact on Healthy Eating Habits
- Mental Health Awareness Campaigns: Assessing Public Perceptions
- The Role of Social Media in Health Promotion
Global Health
- Assessing the Impact of International Aid on Global Health
- Water, Sanitation, and Hygiene (WASH) Programs in Developing Countries
- The Role of Non-Governmental Organizations in Global Health
- Communicable Disease Control in Refugee Populations
- Global Access to Essential Medicines: Challenges and Solutions
Community Health
- Community-Based Participatory Research: Best Practices and Challenges
- The Impact of Community Health Workers on Health Outcomes
- Health Literacy and its Relationship to Health Disparities
- Assessing the Effectiveness of Mobile Health (mHealth) Interventions
- Community Resilience in the Face of Public Health Crises
Healthcare Quality and Patient Safety
- Hospital-Acquired Infections: Strategies for Prevention
- Patient Safety Culture in Healthcare Organizations
- Quality Improvement Initiatives in Primary Care Settings
- Healthcare Accreditation: Impact on Patient Outcomes
- Implementing Electronic Health Records: Challenges and Benefits
Mental Health
- Stigma Reduction Programs for Mental Health Disorders
- Integrating Mental Health into Primary Care Settings
- The Impact of COVID-19 on Mental Health: Long-Term Implications
- Mental Health in the Workplace: Strategies for Employee Well-being
- Suicide Prevention Programs: Effectiveness and Outreach
Health Disparities
- Racial Disparities in Healthcare: Addressing Systemic Inequities
- LGBTQ+ Health Disparities and Inclusive Healthcare Practices
- Socioeconomic Status and Access to Healthcare Services
- Geographical Disparities in Health: Rural vs. Urban
- The Impact of Gender on Health Outcomes and Access to Care
Public Health Education
- Evaluation of Public Health Education Programs
- Innovative Approaches to Teaching Public Health Concepts
- Online Health Education Platforms: Opportunities and Challenges
- Interdisciplinary Training in Public Health: Bridging Gaps
- Continuing Education for Public Health Professionals: Current Landscape
Digital Health
- The Role of Wearable Devices in Health Monitoring
- Telehealth Adoption: Barriers and Opportunities
- Health Apps for Chronic Disease Management: User Perspectives
- Blockchain Technology in Healthcare: Privacy and Security Implications
- Artificial Intelligence in Disease Diagnosis and Prediction
Health Economics
- Cost-Effectiveness of Preventive Health Interventions
- The Impact of Healthcare Financing Models on Access to Care
- Pharmaceutical Pricing and Access to Essential Medicines
- Economic Evaluation of Health Promotion Programs
- Health Insurance Coverage and Health Outcomes: A Global Perspective
Innovations in Public Health
- 3D Printing in Healthcare: Applications and Future Prospects
- Gene Editing Technologies and their Ethical Implications
- Smart Cities and Public Health: Integrating Technology for Well-being
- Nanotechnology in Medicine: Potential for Disease Treatment
- The Role of Drones in Public Health: Surveillance and Intervention
Food Safety and Nutrition
- Foodborne Illness Outbreaks: Investigating Causes and Prevention
- Sustainable Food Systems: Implications for Public Health
- Nutritional Interventions for Malnutrition in Developing Countries
- Food Labeling and Consumer Understanding: A Critical Review
- The Impact of Fast Food Consumption on Public Health
Substance Abuse
- Opioid Epidemic: Strategies for Prevention and Treatment
- Harm Reduction Approaches in Substance Abuse Programs
- Alcohol Consumption Patterns and Public Health Outcomes
- Smoking and Mental Health: Exploring the Connection
- Novel Psychoactive Substances: Emerging Threats and Strategies
Occupational Health
- Workplace Stress and Mental Health: Intervention Strategies
- Occupational Hazards in Healthcare Professions: A Comparative Analysis
- Ergonomics in the Workplace: Improving Worker Health and Productivity
- Night Shift Work and Health Consequences: Addressing Challenges
- Occupational Health and Safety Regulations: A Global Overview
Disaster Preparedness and Response
- Pandemic Preparedness and Lessons from COVID-19
- Natural Disasters and Mental Health: Post-Traumatic Stress
- Emergency Response Systems: Improving Timeliness and Efficiency
- Communicating Health Risks During Emergencies: Public Perception
- Collaborative Approaches to Disaster Response in Global Health
Cancer Research
- Precision Medicine in Cancer Treatment: Current Advancements
- Cancer Screening Programs: Efficacy and Challenges
- Environmental Factors and Cancer Risk: Exploring Connections
- Survivorship Care Plans: Enhancing Quality of Life after Cancer
- Integrative Therapies in Cancer Care: Complementary Approaches
Sexual and Reproductive Health
- Access to Contraception in Developing Countries: Challenges and Solutions
- Comprehensive Sex Education Programs: Impact on Teen Pregnancy
- Reproductive Health Rights: Global Perspectives and Challenges
- Infertility Treatment: Ethical Considerations and Societal Impact
- Maternal and Child Health in Conflict Zones: Addressing Challenges
Cardiovascular Health
- Hypertension Prevention Programs: Strategies and Effectiveness
- Cardiovascular Disease in Women: Gender-Specific Risk Factors
- Innovations in Cardiac Rehabilitation Programs
- Artificial Heart Technology: Advancements and Ethical Implications
- Impact of Air Pollution on Cardiovascular Health: A Global Concern
Social Determinants of Health
- Educational Attainment and Health Outcomes: Exploring Links
- Income Inequality and its Impact on Population Health
- Social Support Networks and Mental Health: A Comprehensive Study
- Neighborhood Environments and Health Disparities
- Employment and Health: The Interplay of Work and Well-being
Genomics and Public Health
- Population Genomics and its Implications for Public Health
- Genetic Counseling and Education: Empowering Individuals and Families
- Ethical Issues in Genetic Research: Privacy and Informed Consent
- Pharmacogenomics: Tailoring Drug Therapies to Individual Genotypes
- Gene-Environment Interactions in Disease Risk: Unraveling Complexities
Public Health Ethics
- Informed Consent in Public Health Research: Current Practices
- Ethical Challenges in Global Health Research: Balancing Priorities
- Confidentiality in Public Health Reporting: Striking the Right Balance
- Research with Vulnerable Populations: Ethical Considerations
- Ethical Implications of Emerging Technologies in Healthcare
Health Communication
- The Role of Media in Shaping Public Health Perceptions
- Health Literacy Interventions: Improving Understanding of Health Information
- Social Media Campaigns for Public Health Promotion: Best Practices
- Tailoring Health Messages for Diverse Audiences: Cultural Competency
- Risk Communication in Public Health Emergencies: Lessons Learned
Nutrigenomics
- Personalized Nutrition Plans based on Genetic Makeup
- Impact of Nutrigenomics on Chronic Disease Prevention
- Ethical Considerations in Nutrigenomics Research
- Public Perceptions of Nutrigenomic Testing: A Qualitative Study
- Integrating Nutrigenomics into Public Health Policies
Public Health and Artificial Intelligence
- Predictive Analytics in Disease Surveillance: Harnessing AI for Early Detection
- Ethical Considerations in AI-Driven Health Decision Support Systems
- Machine Learning in Epidemiology: Predicting Disease Outbreaks
- Natural Language Processing in Public Health: Text Mining for Insights
- Bias in AI Algorithms: Implications for Health Equity
Health Disparities in Aging
- Geriatric Health Disparities: Bridging the Gap in Elderly Care
- Ageism in Healthcare: Addressing Stereotypes and Discrimination
- Social Isolation and Health Consequences in Aging Populations
- Access to Palliative Care for Older Adults: A Global Perspective
- Alzheimer’s Disease and Ethnic Disparities in Diagnosis and Treatment
- Loneliness and Mental Health in the Elderly: Interventions and Support
Research Methodologies in Public Health
Public health research employs various methodologies, including quantitative, qualitative, and mixed-methods approaches. Each method brings its own strengths to the research process, allowing researchers to gain a comprehensive understanding of the complex issues they investigate.
Community-based participatory research is another valuable approach, emphasizing collaboration with communities to address their specific health concerns.
Challenges and Opportunities in Public Health Research
While public health research is immensely rewarding, it comes with its own set of challenges. Funding constraints, ethical dilemmas, the need for interdisciplinary collaboration, and the integration of technology pose both obstacles and opportunities.
Researchers must navigate these challenges to ensure their work has a meaningful impact on public health.
In conclusion, public health research topics are diverse and dynamic, reflecting the complex nature of the field. As researchers embark on their journeys, they must carefully consider the relevance, impact, and ethical implications of their chosen topics.
The collaborative and interdisciplinary nature of public health research positions it as a powerful tool in addressing the health challenges of our time. By exploring the depths of these topics, researchers contribute to the collective effort to build healthier and more equitable communities.
As we move forward, a continued exploration of relevant public health research topics is essential for shaping the future of healthcare and improving the well-being of populations worldwide.
Related Posts
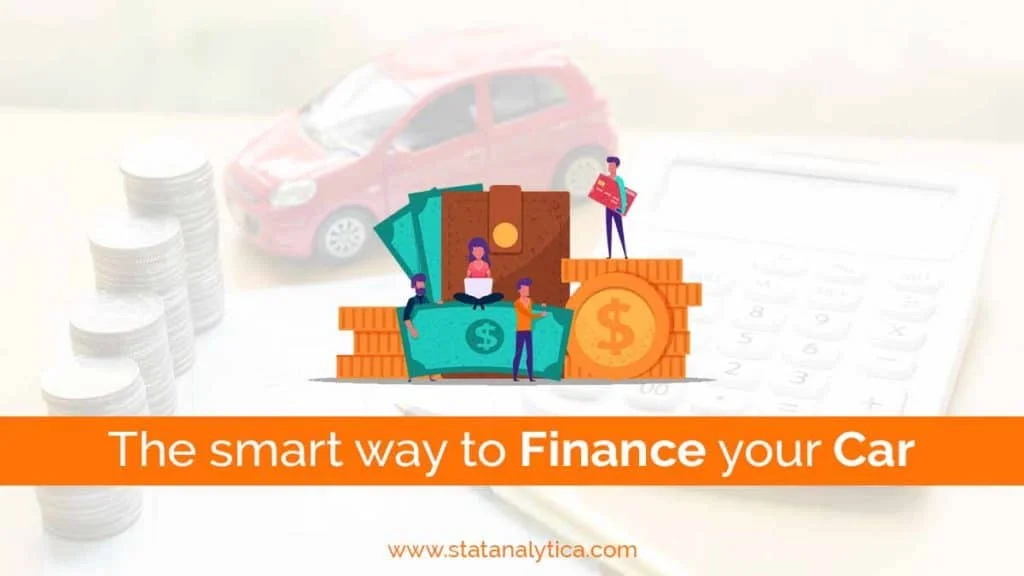
Step by Step Guide on The Best Way to Finance Car
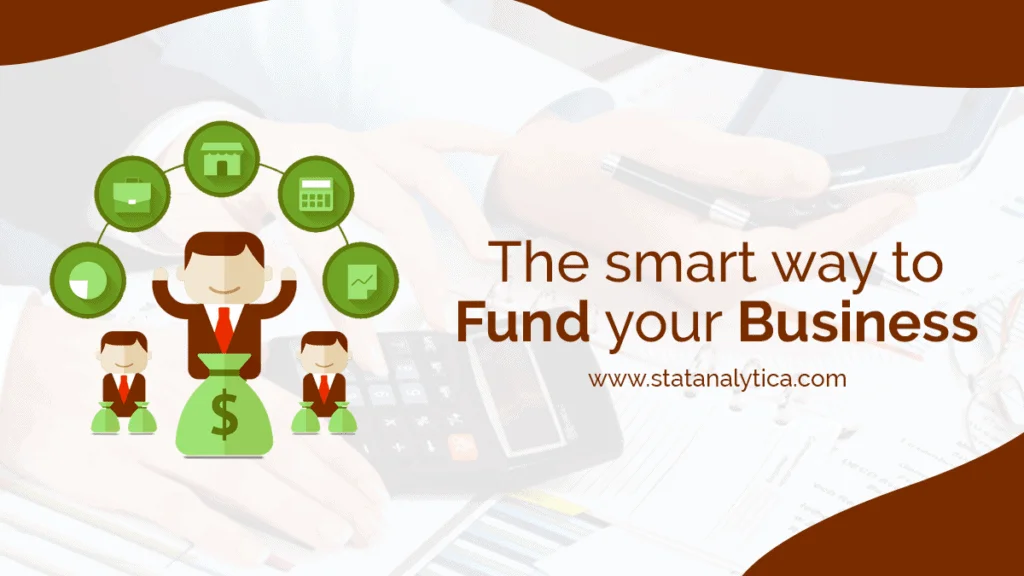
The Best Way on How to Get Fund For Business to Grow it Efficiently
- Browse All Articles
- Newsletter Sign-Up
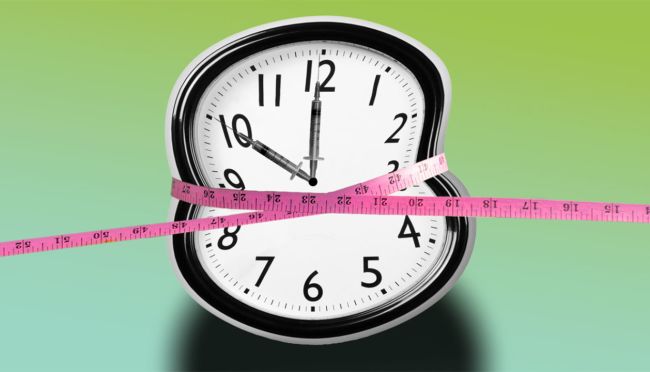
- 09 Feb 2024
Slim Chance: Drugs Will Reshape the Weight Loss Industry, But Habit Change Might Be Elusive
Medications such as Ozempic, Wegovy, and Mounjaro have upended a $76 billion industry that has long touted lifestyle shifts as a means to weight loss. Regina Herzlinger says these drugs might bring fast change, especially for busy professionals, but many questions remain unanswered.
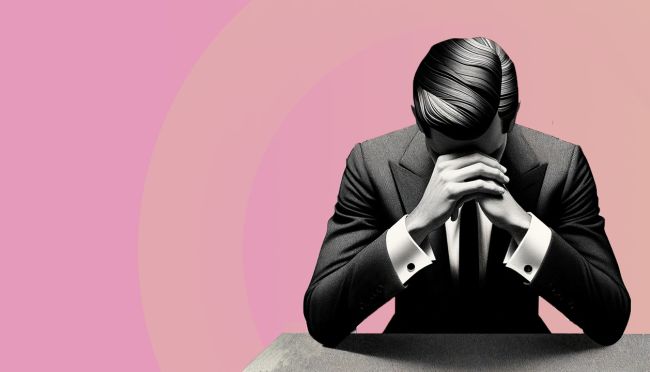
- 09 Nov 2023
What Will It Take to Confront the Invisible Mental Health Crisis in Business?
The pressure to do more, to be more, is fueling its own silent epidemic. Lauren Cohen discusses the common misperceptions that get in the way of supporting employees' well-being, drawing on case studies about people who have been deeply affected by mental illness.
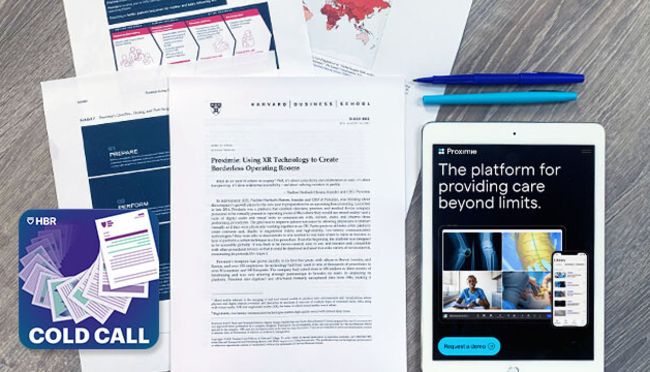
- 12 Sep 2023
- Cold Call Podcast
Can Remote Surgeries Digitally Transform Operating Rooms?
Launched in 2016, Proximie was a platform that enabled clinicians, proctors, and medical device company personnel to be virtually present in operating rooms, where they would use mixed reality and digital audio and visual tools to communicate with, mentor, assist, and observe those performing medical procedures. The goal was to improve patient outcomes. The company had grown quickly, and its technology had been used in tens of thousands of procedures in more than 50 countries and 500 hospitals. It had raised close to $50 million in equity financing and was now entering strategic partnerships to broaden its reach. Nadine Hachach-Haram, founder and CEO of Proximie, aspired for Proximie to become a platform that powered every operating room in the world, but she had to carefully consider the company’s partnership and data strategies in order to scale. What approach would position the company best for the next stage of growth? Harvard Business School associate professor Ariel Stern discusses creating value in health care through a digital transformation of operating rooms in her case, “Proximie: Using XR Technology to Create Borderless Operating Rooms.”
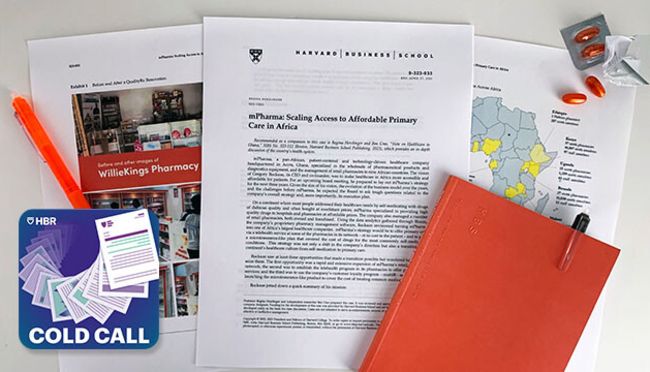
- 01 Aug 2023
Can Business Transform Primary Health Care Across Africa?
mPharma, headquartered in Ghana, is trying to create the largest pan-African health care company. Their mission is to provide primary care and a reliable and fairly priced supply of drugs in the nine African countries where they operate. Co-founder and CEO Gregory Rockson needs to decide which component of strategy to prioritize in the next three years. His options include launching a telemedicine program, expanding his pharmacies across the continent, and creating a new payment program to cover the cost of common medications. Rockson cares deeply about health equity, but his venture capital-financed company also must be profitable. Which option should he focus on expanding? Harvard Business School Professor Regina Herzlinger and case protagonist Gregory Rockson discuss the important role business plays in improving health care in the case, “mPharma: Scaling Access to Affordable Primary Care in Africa.”
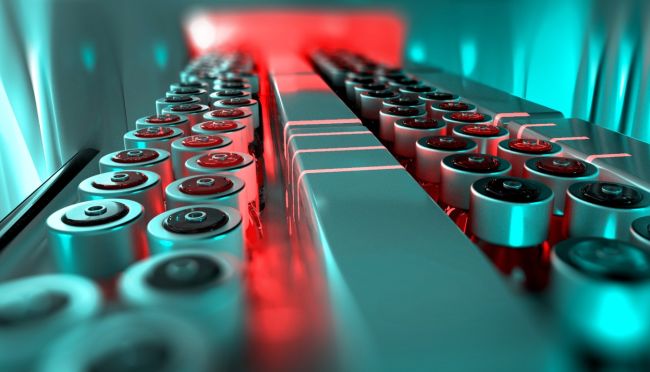
- 25 Jul 2023
- Research & Ideas
Could a Business Model Help Big Pharma Save Lives and Profit?
Gilead Sciences used a novel approach to help Egypt address a public health crisis while sustaining profits from a key product. V. Kasturi Rangan and participants at a recent seminar hosted by the Institute for the Study of Business in Global Society discussed what it would take to apply the model more widely.
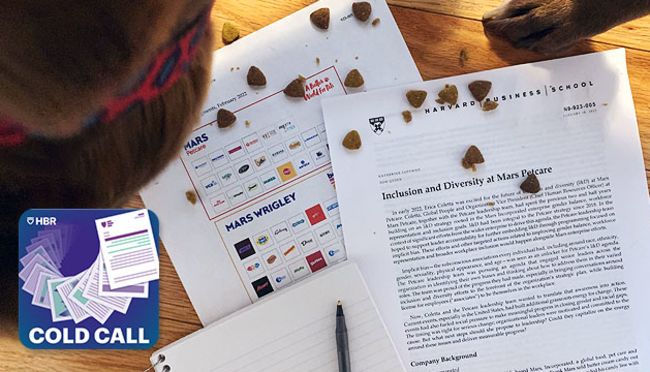
- 18 Jul 2023
Diversity and Inclusion at Mars Petcare: Translating Awareness into Action
In 2020, the Mars Petcare leadership team found themselves facing critically important inclusion and diversity issues. Unprecedented protests for racial justice in the U.S. and across the globe generated demand for substantive change, and Mars Petcare's 100,000 employees across six continents were ready for visible signs of progress. How should Mars’ leadership build on their existing diversity, equity, and inclusion efforts and effectively capitalize on the new energy for change? Harvard Business School associate professor Katherine Coffman is joined by Erica Coletta, Mars Petcare’s chief people officer, and Ibtehal Fathy, global inclusion and diversity officer at Mars Inc., to discuss the case, “Inclusion and Diversity at Mars Petcare.”
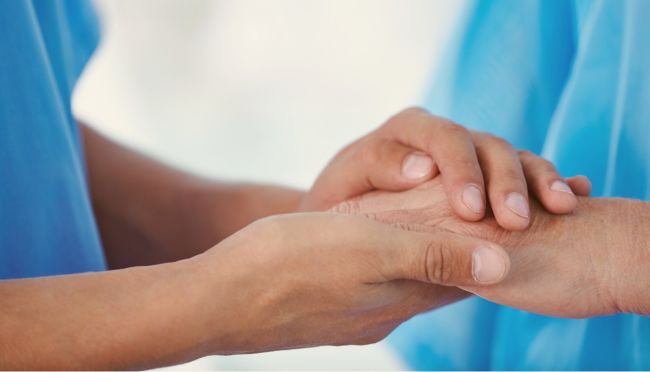
- 23 Jun 2023
This Company Lets Employees Take Charge—Even with Life and Death Decisions
Dutch home health care organization Buurtzorg avoids middle management positions and instead empowers its nurses to care for patients as they see fit. Tatiana Sandino and Ethan Bernstein explore how removing organizational layers and allowing employees to make decisions can boost performance.
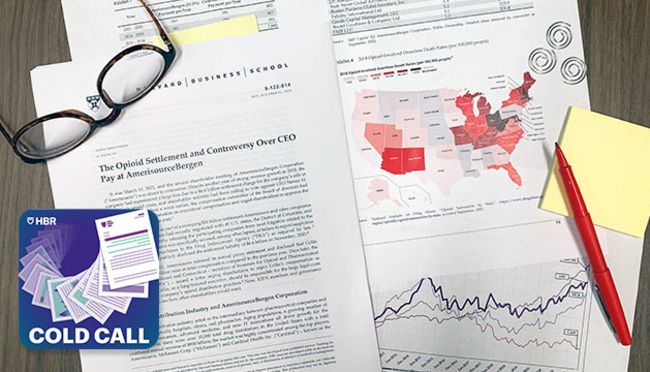
- 06 Jun 2023
The Opioid Crisis, CEO Pay, and Shareholder Activism
In 2020, AmerisourceBergen Corporation, a Fortune 50 company in the drug distribution industry, agreed to settle thousands of lawsuits filed nationwide against the company for its opioid distribution practices, which critics alleged had contributed to the opioid crisis in the US. The $6.6 billion global settlement caused a net loss larger than the cumulative net income earned during the tenure of the company’s CEO, which began in 2011. In addition, AmerisourceBergen’s legal and financial troubles were accompanied by shareholder demands aimed at driving corporate governance changes in companies in the opioid supply chain. Determined to hold the company’s leadership accountable, the shareholders launched a campaign in early 2021 to reject the pay packages of executives. Should the board reduce the executives’ pay, as of means of improving accountability? Or does punishing the AmerisourceBergen executives for paying the settlement ignore the larger issue of a business’s responsibility to society? Harvard Business School professor Suraj Srinivasan discusses executive compensation and shareholder activism in the context of the US opioid crisis in his case, “The Opioid Settlement and Controversy Over CEO Pay at AmerisourceBergen.”
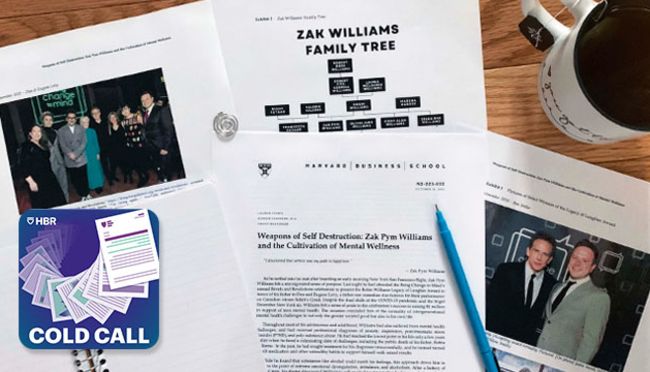
- 09 May 2023
Can Robin Williams’ Son Help Other Families Heal Addiction and Depression?
Zak Pym Williams, son of comedian and actor Robin Williams, had seen how mental health challenges, such as addiction and depression, had affected past generations of his family. Williams was diagnosed with generalized anxiety disorder, depression, and post-traumatic stress disorder (PTSD) as a young adult and he wanted to break the cycle for his children. Although his children were still quite young, he began considering proactive strategies that could help his family’s mental health, and he wanted to share that knowledge with other families. But how can Williams help people actually take advantage of those mental health strategies and services? Professor Lauren Cohen discusses his case, “Weapons of Self Destruction: Zak Pym Williams and the Cultivation of Mental Wellness.”
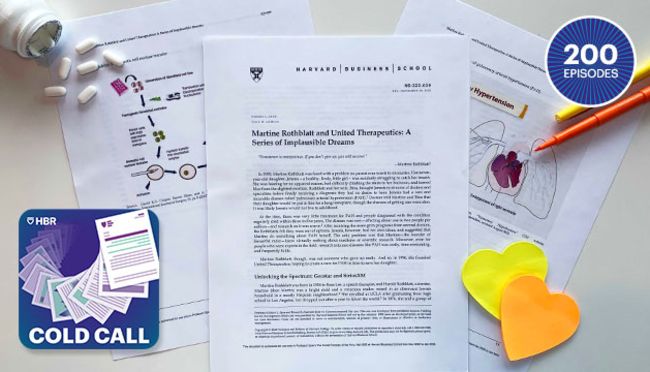
- 26 Apr 2023
How Martine Rothblatt Started a Company to Save Her Daughter
When serial entrepreneur Martine Rothblatt (founder of Sirius XM) received her seven-year-old daughter’s diagnosis of Pulmonary Arterial Hypertension (PAH), she created United Therapeutics and developed a drug to save her life. When her daughter later needed a lung transplant, Rothblatt decided to take what she saw as the logical next step: manufacturing organs for transplantation. Rothblatt’s entrepreneurial career exemplifies a larger debate around the role of the firm in creating solutions for society’s problems. If companies are uniquely good at innovating, what voice should society have in governing the new technologies that firms create? Harvard Business School professor Debora Spar debates these questions in the case “Martine Rothblatt and United Therapeutics: A Series of Implausible Dreams.” As part of a new first-year MBA course at Harvard Business School, this case examines the central question: what is the social purpose of the firm?
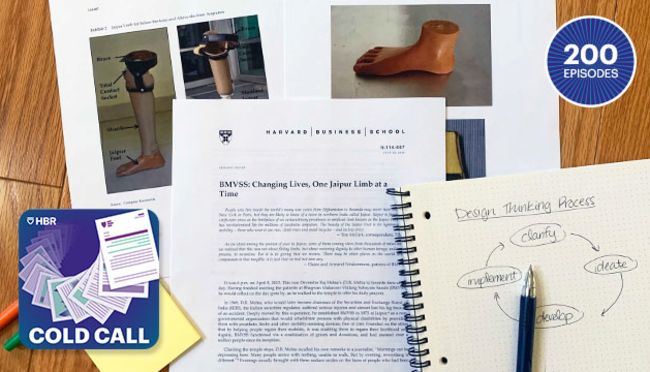
- 25 Apr 2023
Using Design Thinking to Invent a Low-Cost Prosthesis for Land Mine Victims
Bhagwan Mahaveer Viklang Sahayata Samiti (BMVSS) is an Indian nonprofit famous for creating low-cost prosthetics, like the Jaipur Foot and the Stanford-Jaipur Knee. Known for its patient-centric culture and its focus on innovation, BMVSS has assisted more than one million people, including many land mine survivors. How can founder D.R. Mehta devise a strategy that will ensure the financial sustainability of BMVSS while sustaining its human impact well into the future? Harvard Business School Dean Srikant Datar discusses the importance of design thinking in ensuring a culture of innovation in his case, “BMVSS: Changing Lives, One Jaipur Limb at a Time.”
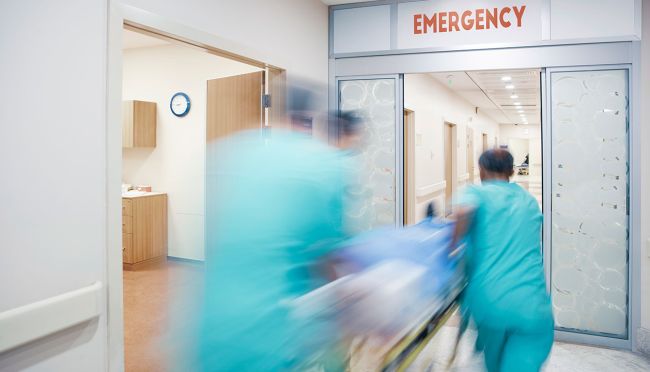
- 31 Mar 2023
Can a ‘Basic Bundle’ of Health Insurance Cure Coverage Gaps and Spur Innovation?
One in 10 people in America lack health insurance, resulting in $40 billion of care that goes unpaid each year. Amitabh Chandra and colleagues say ensuring basic coverage for all residents, as other wealthy nations do, could address the most acute needs and unlock efficiency.
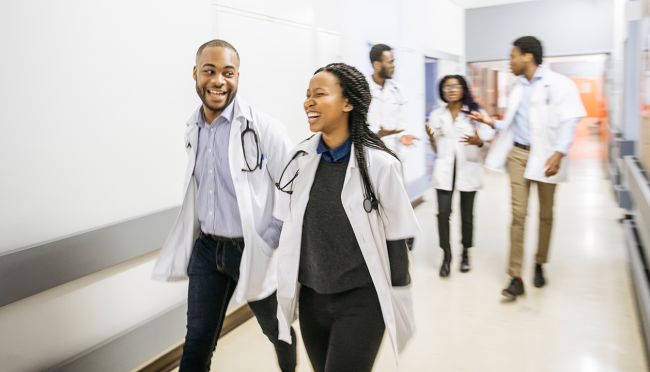
- 13 Mar 2023
The Power of Personal Connections: How Shared Experiences Boost Performance
Doctors who train together go on to provide better patient care later in their careers. What could teams in other industries learn? Research by Maximilian Pany and J. Michael McWilliams.
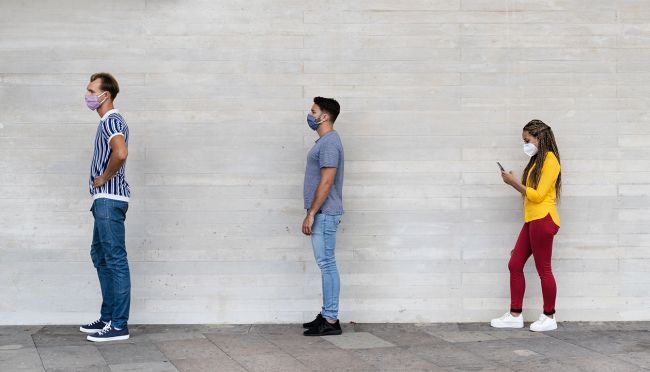
- 10 Feb 2023
COVID-19 Lessons: Social Media Can Nudge More People to Get Vaccinated
Social networks have been criticized for spreading COVID-19 misinformation, but the platforms have also helped public health agencies spread the word on vaccines, says research by Michael Luca and colleagues. What does this mean for the next pandemic?
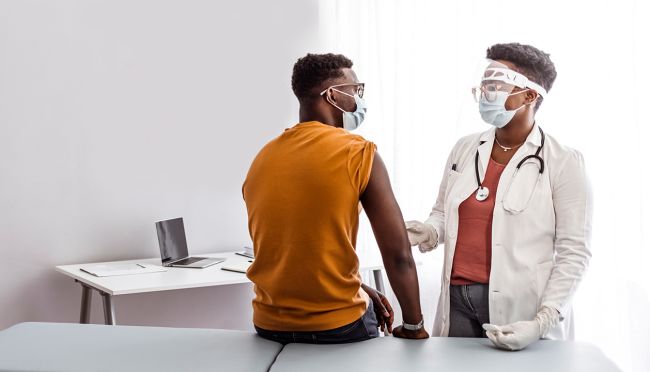
- 12 Dec 2022
Buy-In from Black Patients Suffers When Drug Trials Don’t Include Them
Diversifying clinical trials could build trust in new treatments among Black people and their physicians. Research by Joshua Schwartzstein, Marcella Alsan, and colleagues probes the ripple effects of underrepresentation in testing, and offers a call to action for drugmakers.
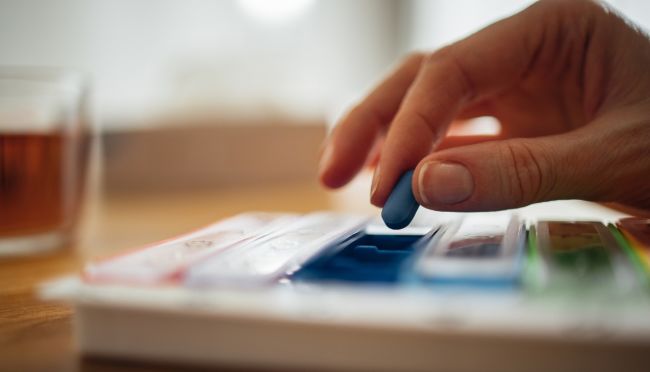
- 06 Sep 2022
Curbing an Unlikely Culprit of Rising Drug Prices: Pharmaceutical Donations
Policymakers of every leaning have vowed to rein in prescription drug costs, with little success. But research by Leemore Dafny shows how closing a loophole on drugmaker donations could eliminate one driver of rising expenses.
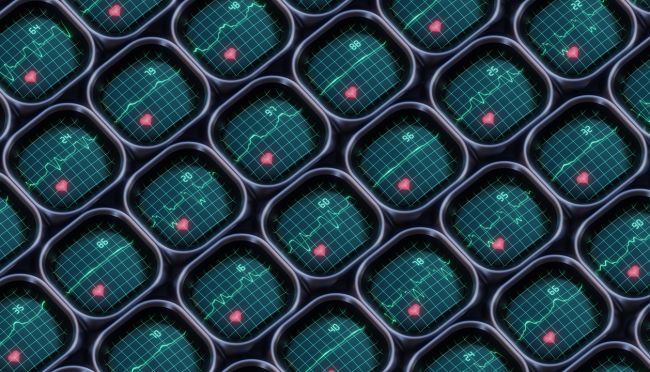
- 22 Aug 2022
Can Amazon Remake Health Care?
Amazon has disrupted everything from grocery shopping to cloud computing, but can it transform health care with its One Medical acquisition? Amitabh Chandra discusses company's track record in health care and the challenges it might face.
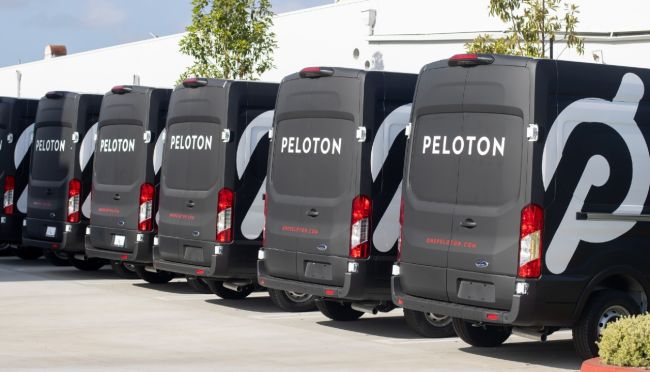
- 30 Jun 2022
Peloton Changed the Exercise Game. Can the Company Push Through the Pain?
When COVID-19 closed gyms, seemingly everyone rushed to order a Peloton bike and claim a spot on the company's signature leader board. And then things quickly went downhill. A case study by Robert Dolan looks at the tough road the exercise equipment maker faces.
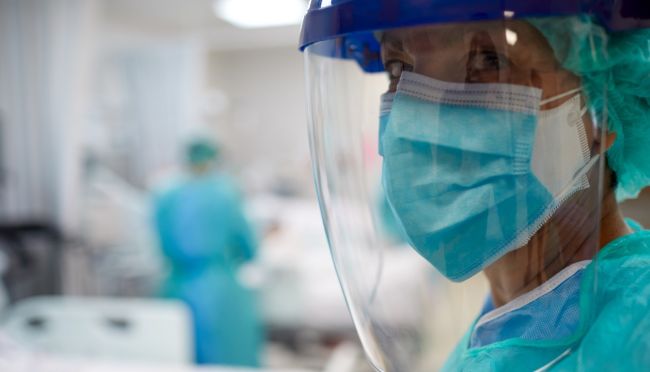
- 14 Mar 2022
Lessons from COVID-19: The Business Skills Doctors Need
The pandemic forced many physicians to become supply chain experts and strategic planners. Robert Huckman and colleagues offer a roadmap for teaching doctors the management and leadership skills they need—before the next public health crisis. Open for comment; 0 Comments.
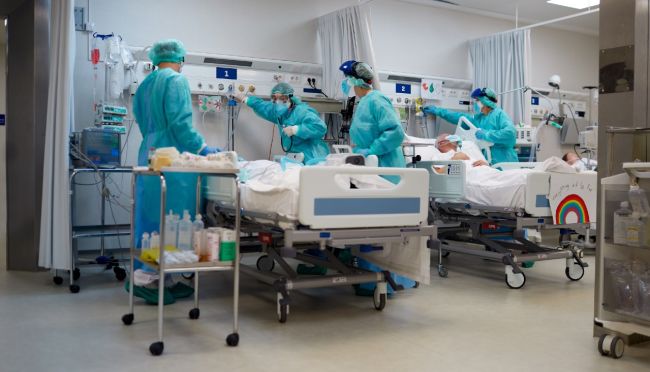
- 24 Feb 2022
Want to Prevent the Next Hospital Bed Crisis? Enlist the SEC
After two years of COVID-19, many hospitals still haven't figured out how to manage the overwhelming wave of patients that flood ICUs during each surge. Regina Herzlinger and Richard Boxer offer a novel solution. Open for comment; 0 Comments.
- Google Meet
- Mobile Dialer

Resent Search

Management Assignment Writing

Technical Assignment Writing

Finance Assignment Writing

Medical Nursing Writing

Resume Writing

Civil engineering writing

Mathematics and Statistics Projects

CV Writing Service

Essay Writing Service

Online Dissertation Help

Thesis Writing Help

RESEARCH PAPER WRITING SERVICE

Case Study Writing Service

Electrical Engineering Assignment Help

IT Assignment Help

Mechanical Engineering Assignment Help

Homework Writing Help

Science Assignment Writing

Arts Architecture Assignment Help

Chemical Engineering Assignment Help
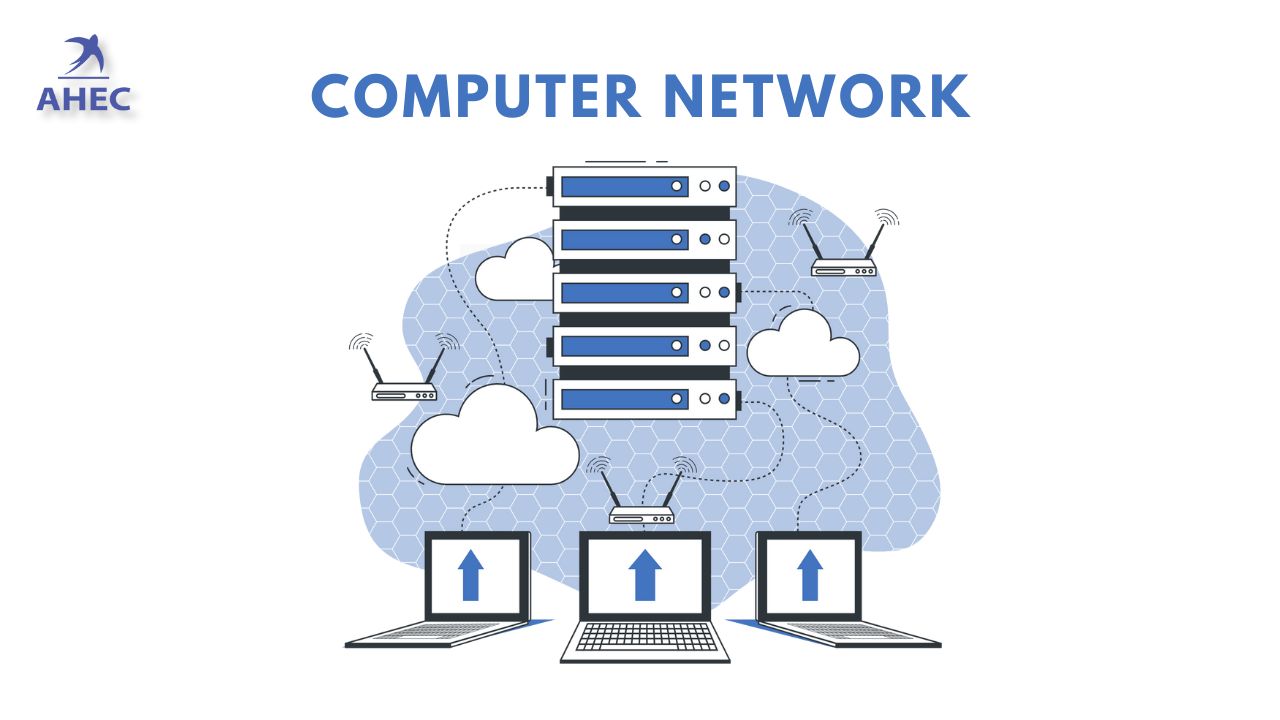
Computer Network Assignment Help

Arts Assignment Help
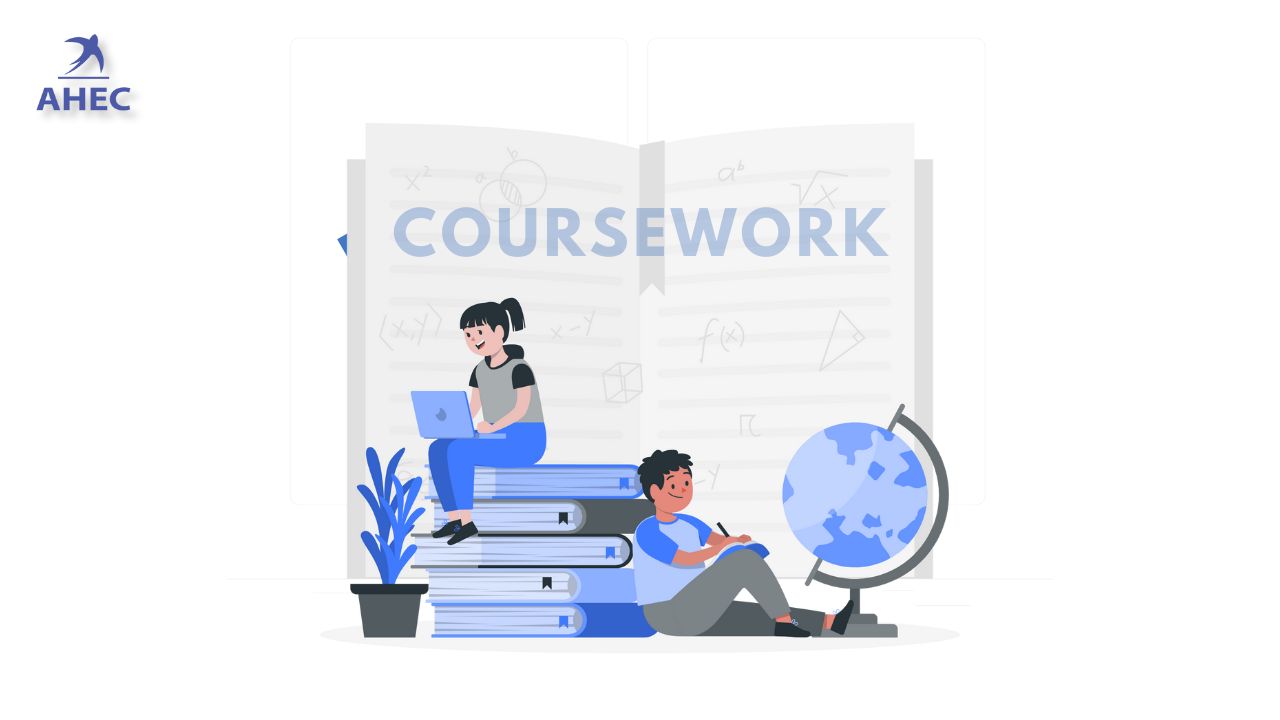
Coursework Writing Help

Custom Paper Writing Services

Personal Statement Writing

Biotechnology Assignment Help
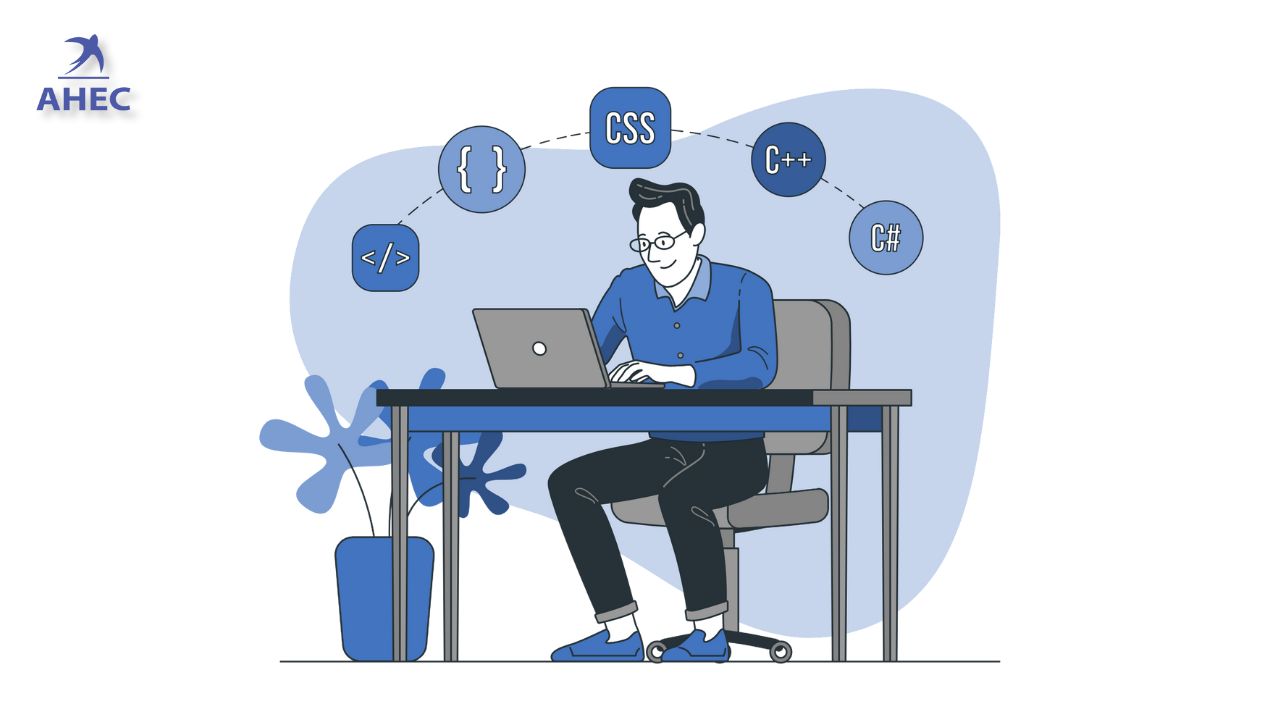
C Programming Assignment Help

MBA Assignment Help

English Essay Writing

MATLAB Assignment Help

Narrative Writing Help
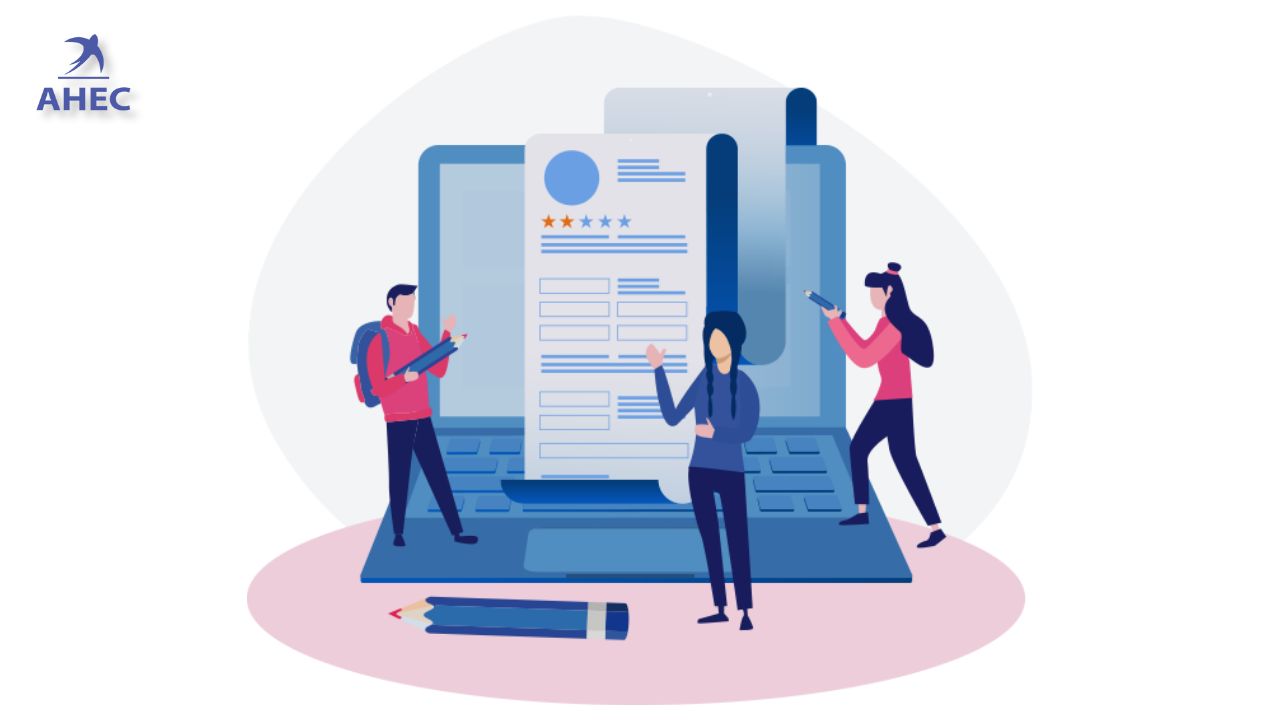
Report Writing Help

Get Top Quality Assignment Assistance

Online Exam Help

Macroeconomics Homework Help
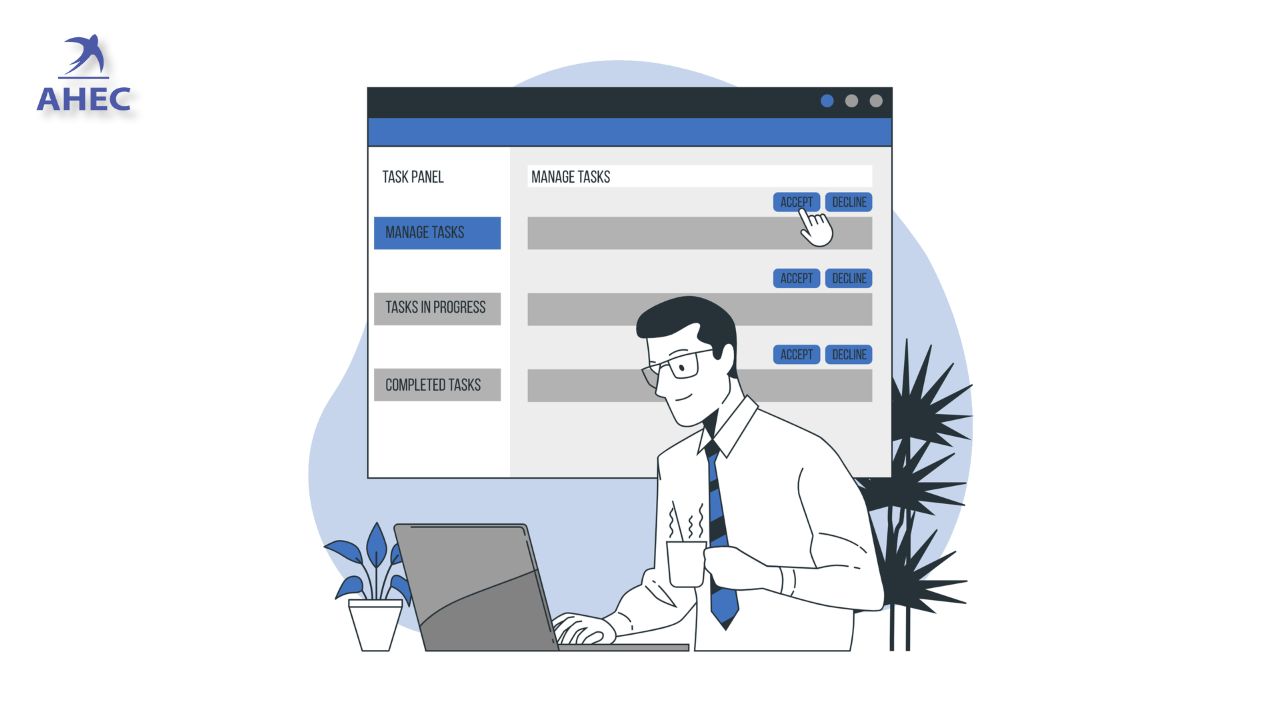
Change Management Assignment Help

Operation management Assignment Help

Strategy Assignment Help

Human Resource Management Assignment Help

Psychology Assignment Writing Help
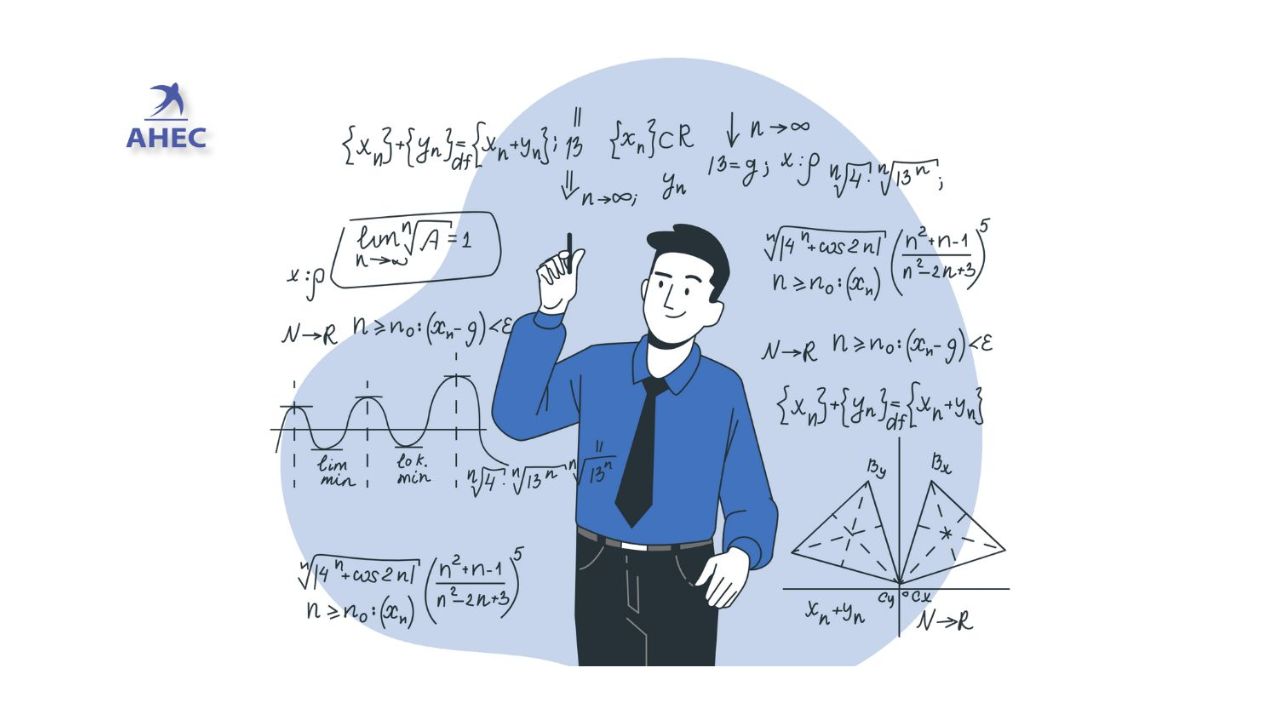
Algebra Homework Help

Best Assignment Writing Tips

Statistics Homework Help
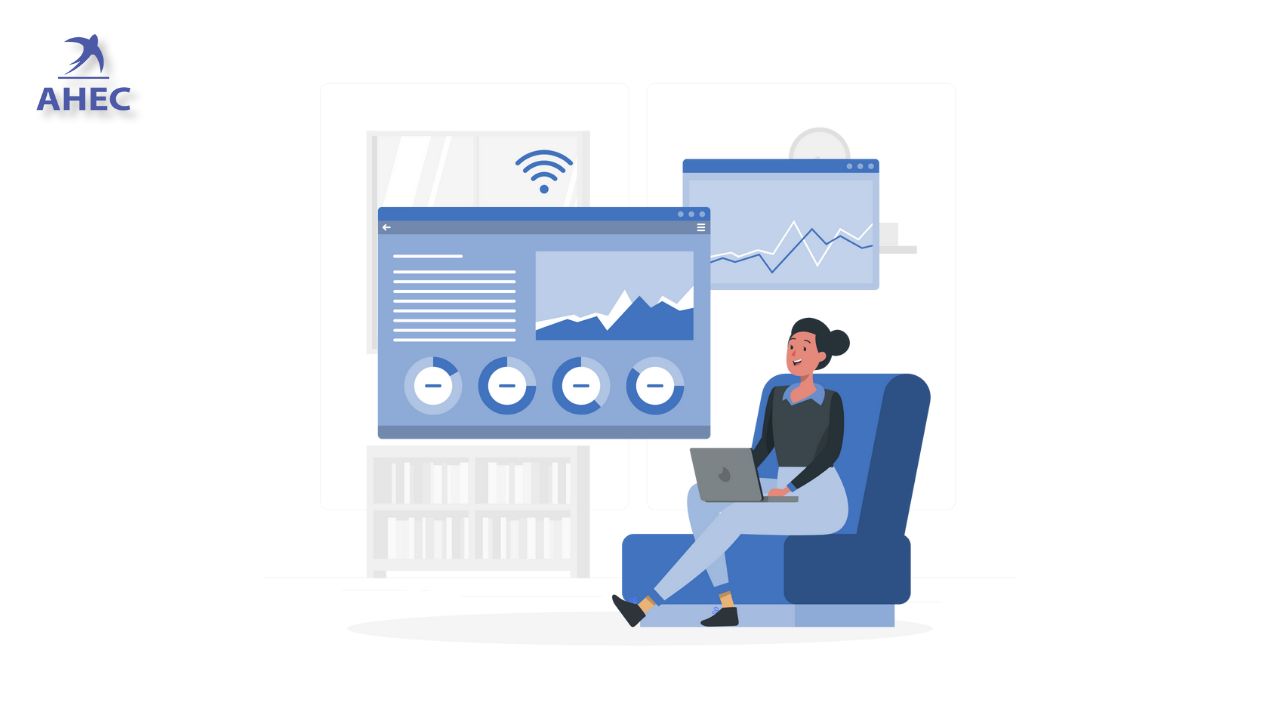
CDR Writing Services

TAFE Assignment Help

Auditing Assignment Help

Literature Essay Help

Online University Assignment Writing

Economics Assignment Help

Programming Language Assignment Help

Political Science Assignment Help

Marketing Assignment Help

Project Management Assignment Help

Geography Assignment Help

Do My Assignment For Me

Business Ethics Assignment Help

Pricing Strategy Assignment Help
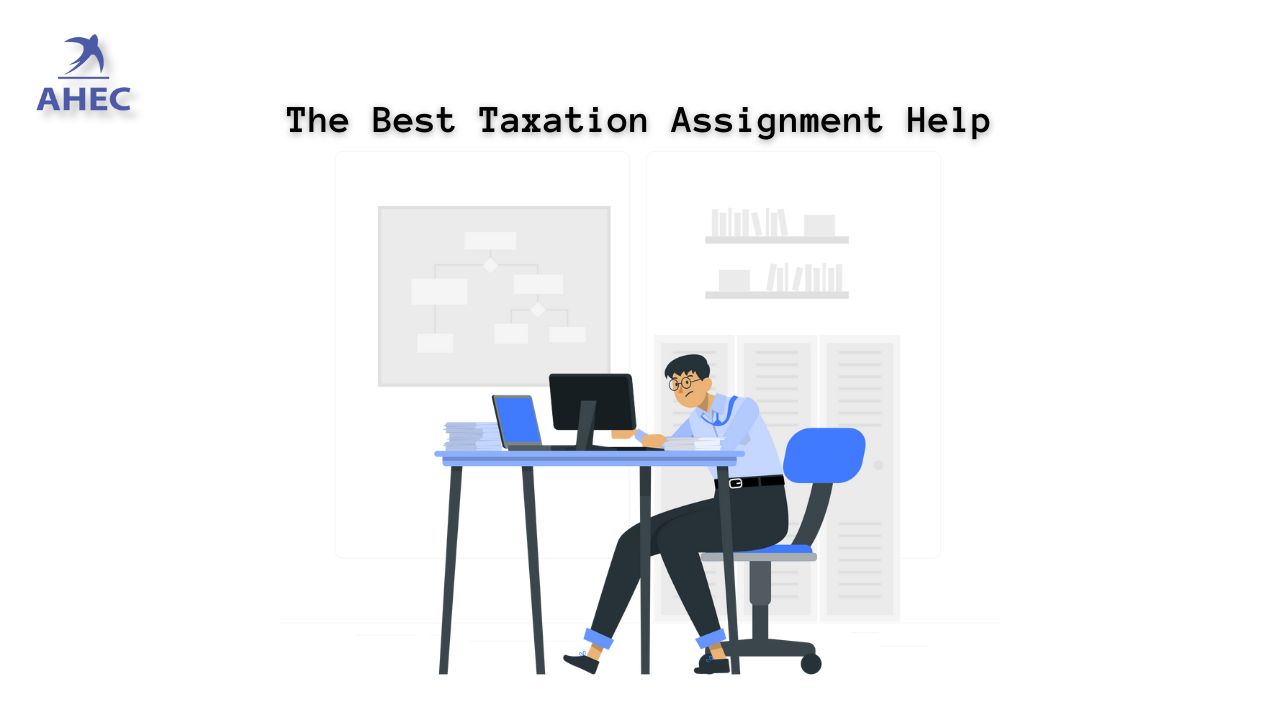
The Best Taxation Assignment Help

Finance Planning Assignment Help

Solve My Accounting Paper Online

Market Analysis Assignment
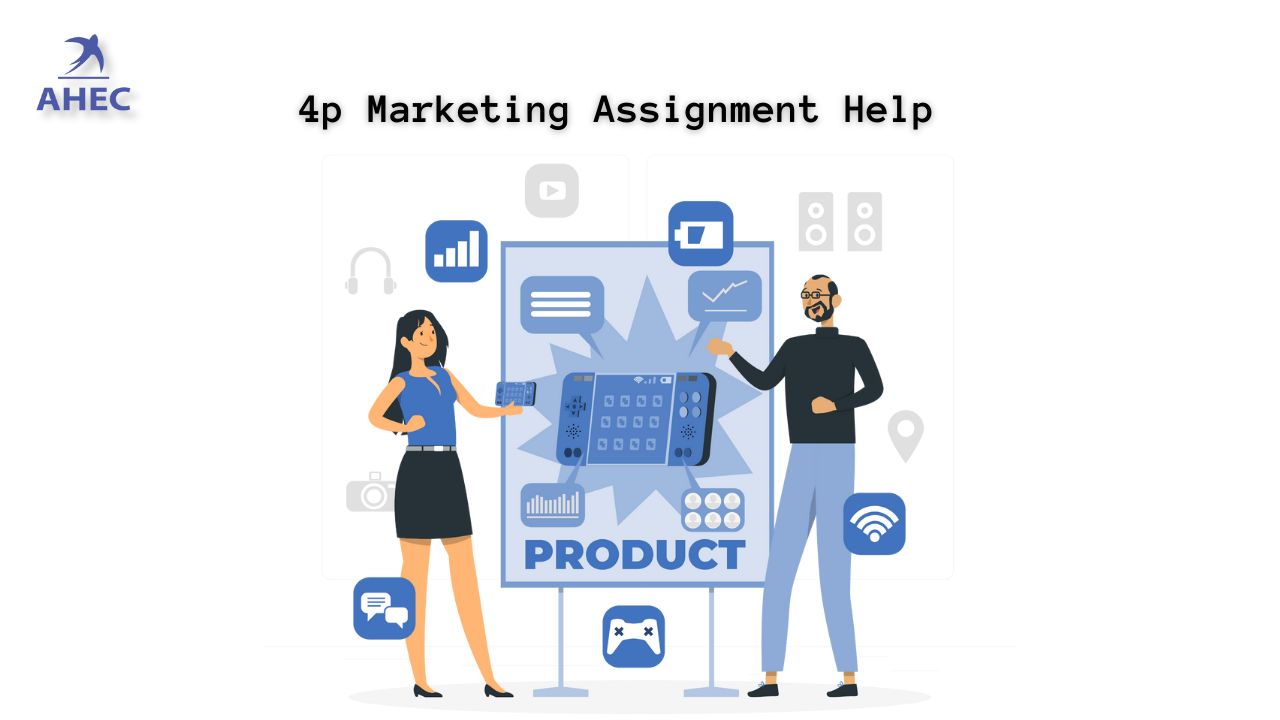
4p Marketing Assignment Help
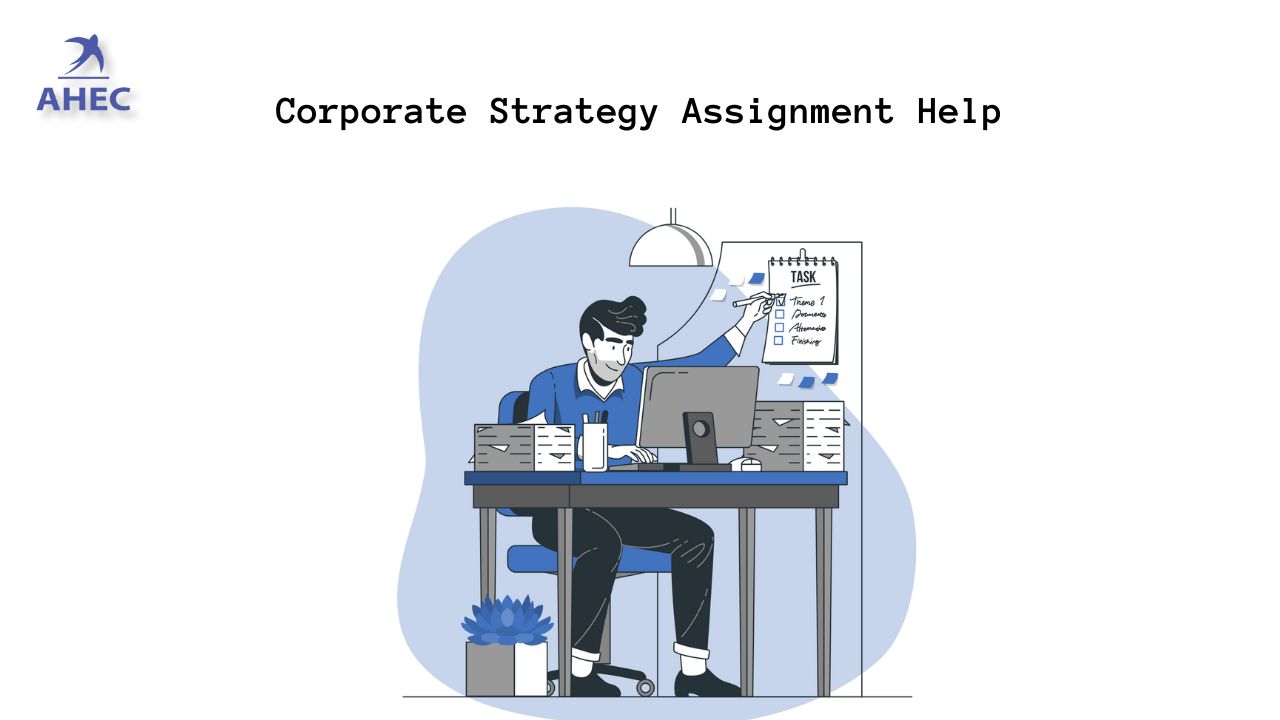
Corporate Strategy Assignment Help

Project Risk Management Assignment Help

Environmental Law Assignment Help
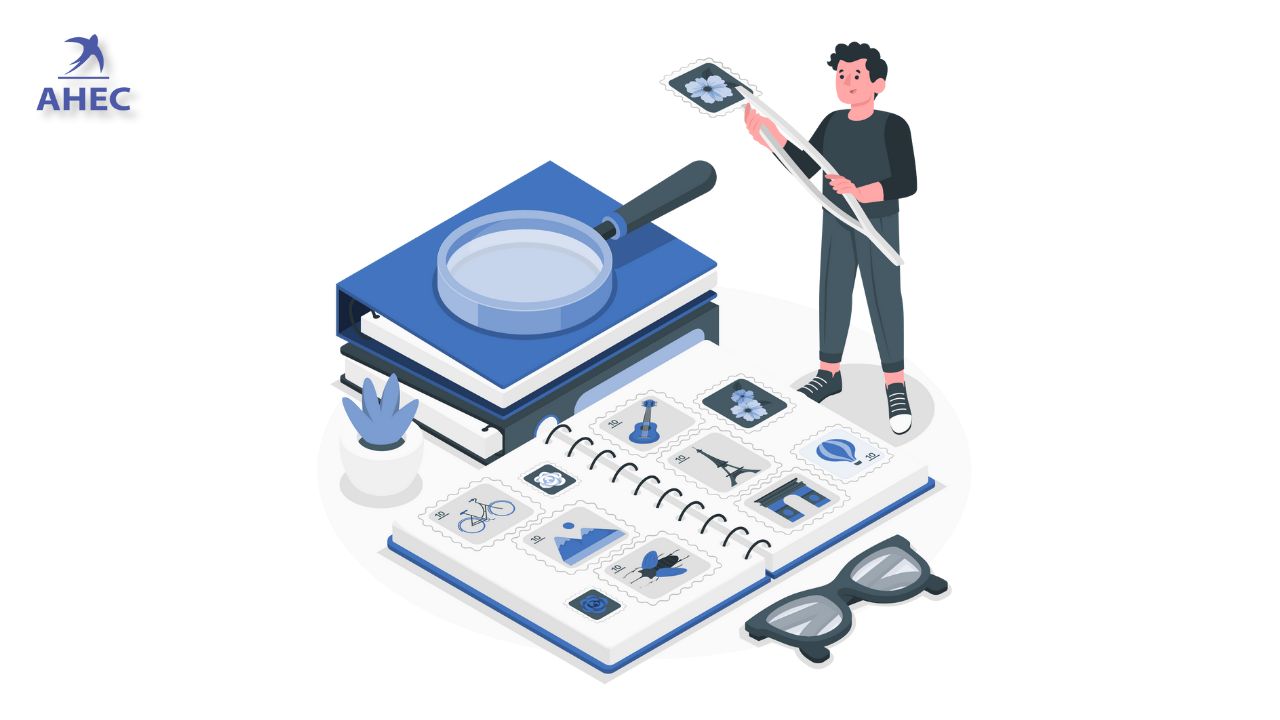
History Assignment Help

Geometry Assignment Help

Physics Assignment Help

Clinical Reasoning Cycle

Forex Assignment Help

Python Assignment Help

Behavioural Finance Assignment Help

PHP Assignment Help
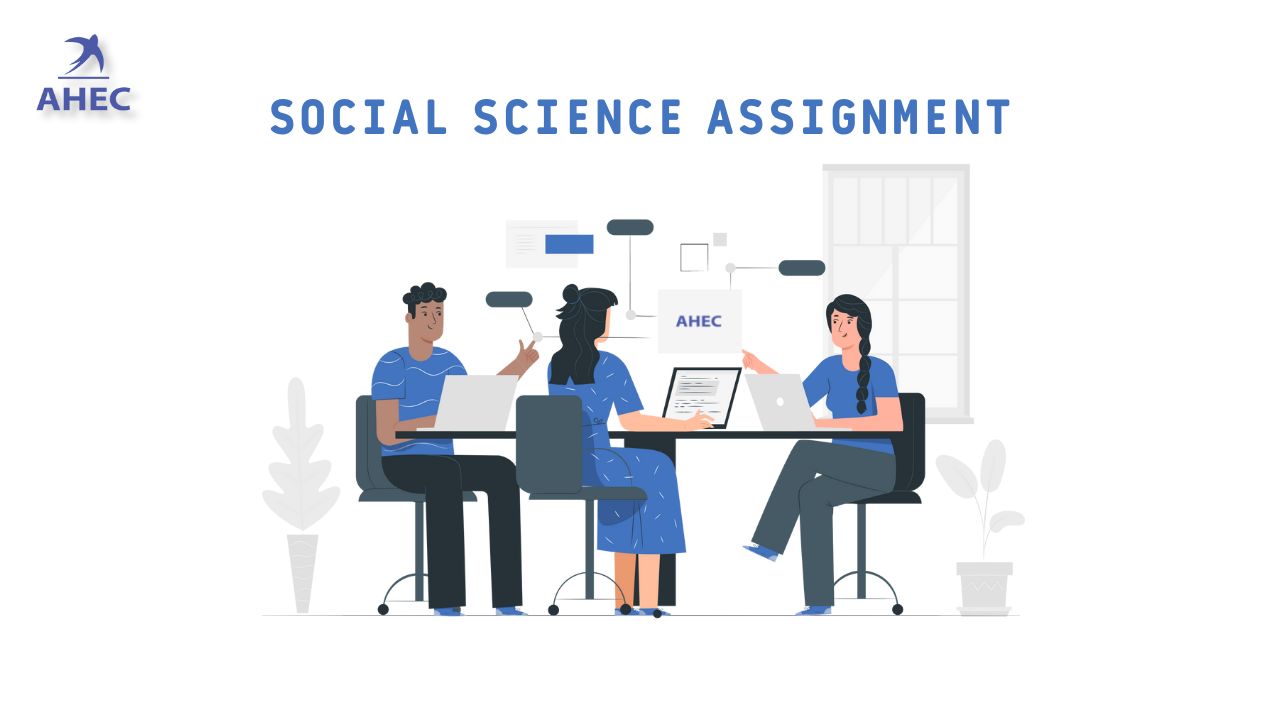
Social Science Assignment Help

Capital Budgeting Assignment Help

Trigonometry Assignment Help

Java Programming Assignment Help

Corporate Finance Planning Help

Sports Science Assignment Help

Accounting For Financial Statements Assignment Help

Robotics Assignment Help

Cost Accounting Assignment Help
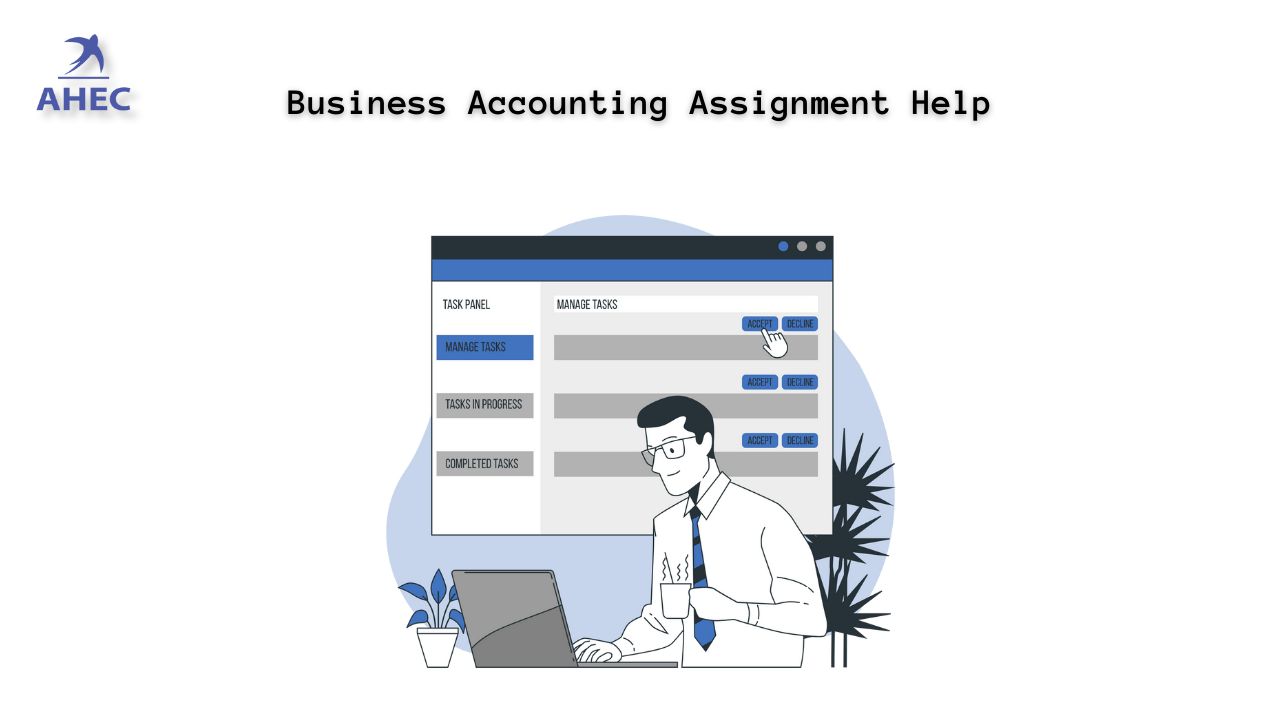
Business Accounting Assignment Help

Activity Based Accounting Assignment Help

Econometrics Assignment Help

Managerial Accounting Assignment Help

R Studio Assignment Help
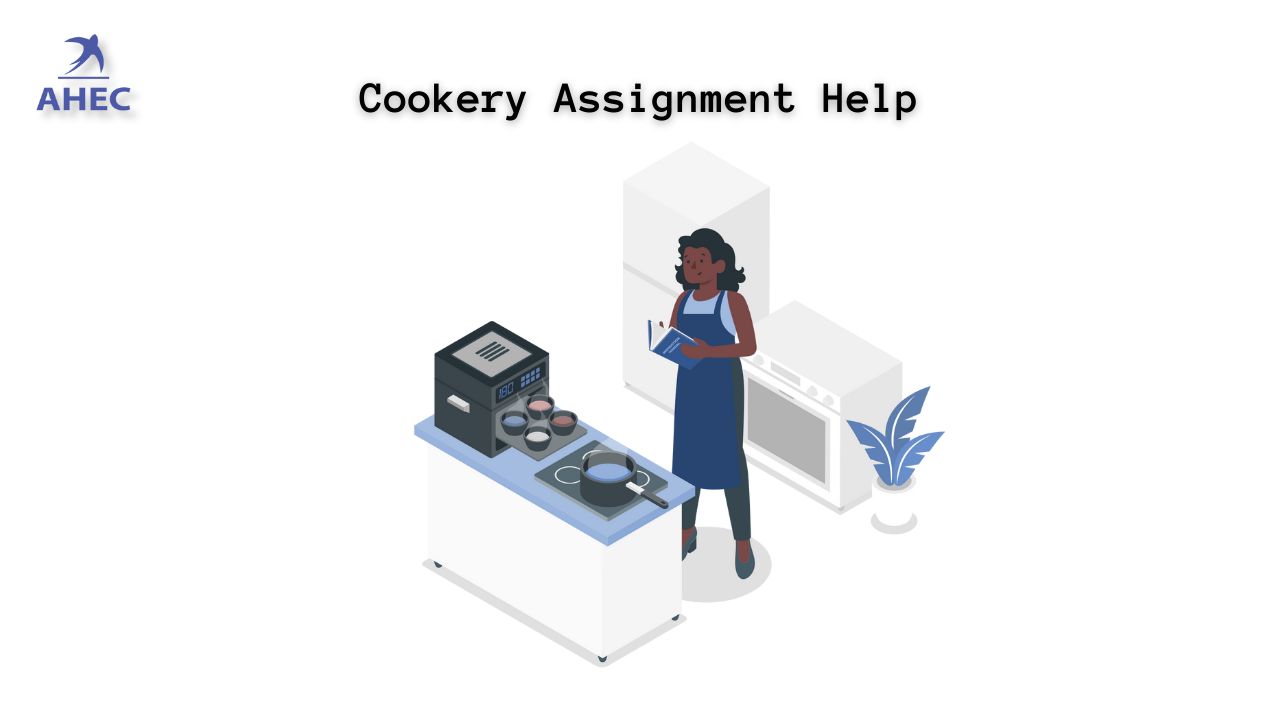
Cookery Assignment Help

Solidworks assignment Help
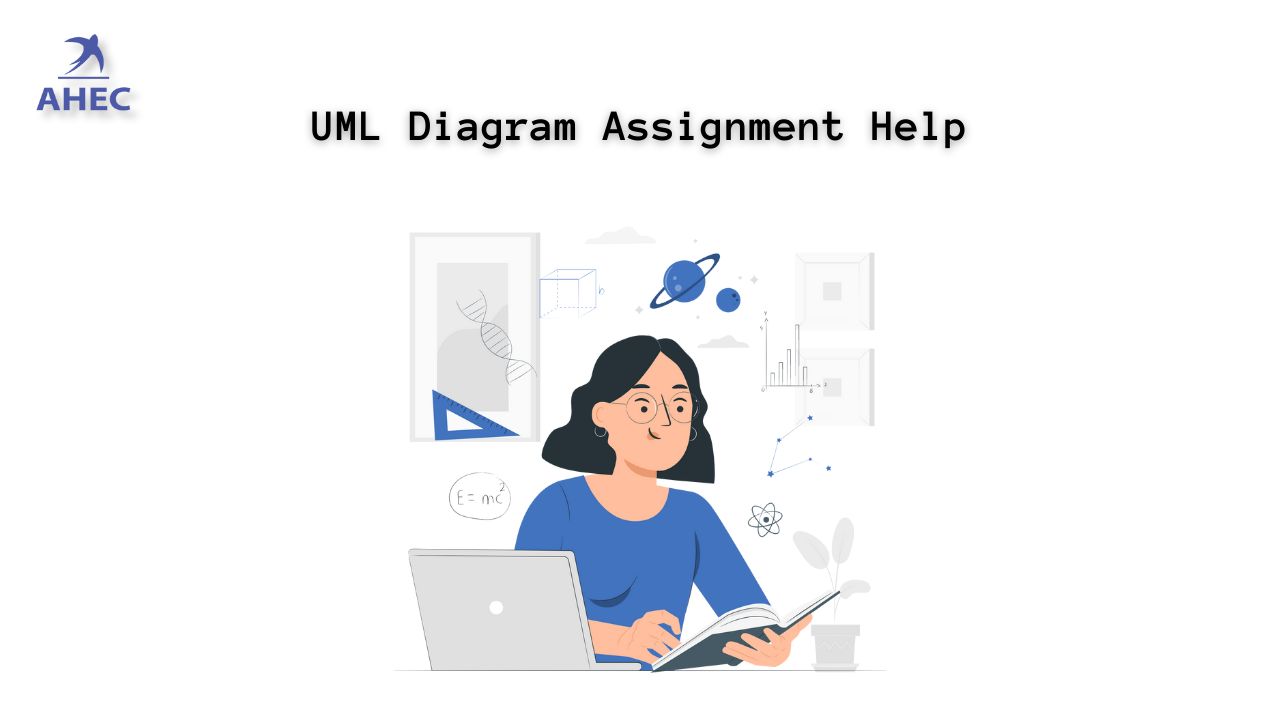
UML Diagram Assignment Help

Data Flow Diagram Assignment Help

Employment Law Assignment Help

Calculus Assignment Help

Arithmetic Assignment Help

Write My Assignment

Business Intelligence Assignment Help
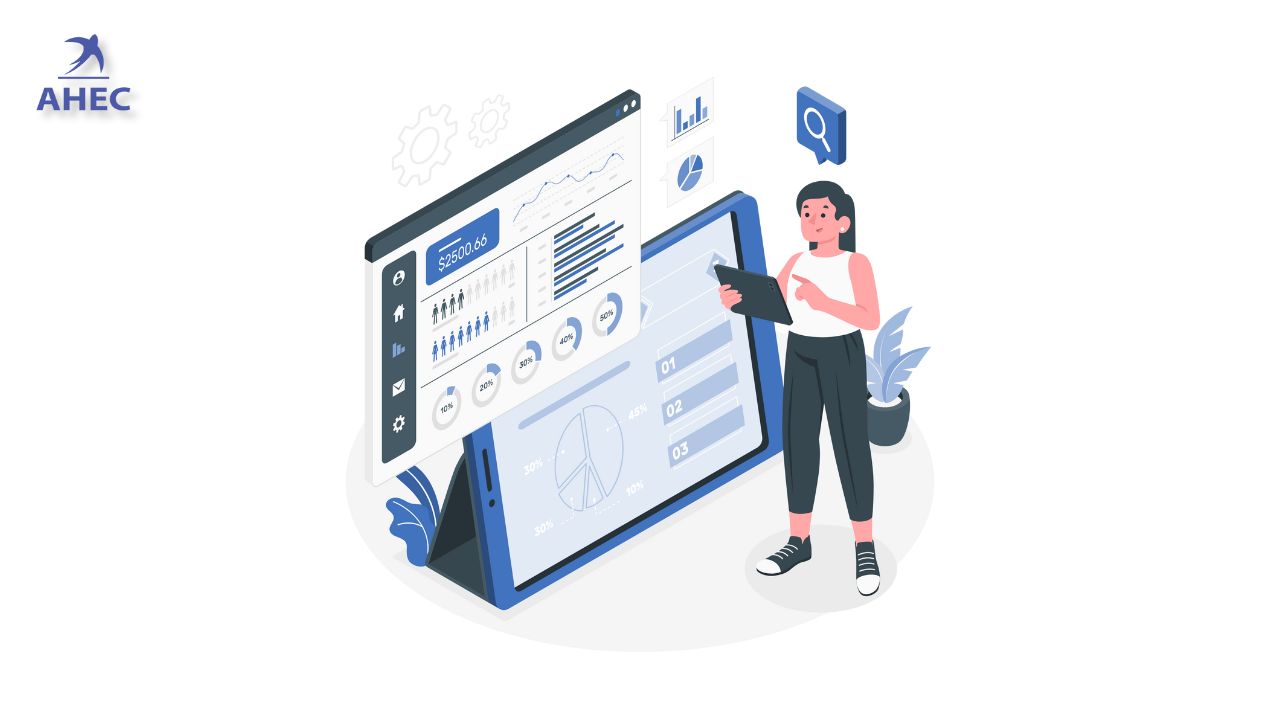
Database Assignment Help

Fluid Mechanics Assignment Help

Web Design Assignment Help

Student Assignment Help

Online CPM Homework Help

Chemistry Assignment Help

Biology Assignment Help

Corporate Governance Law Assignment Help
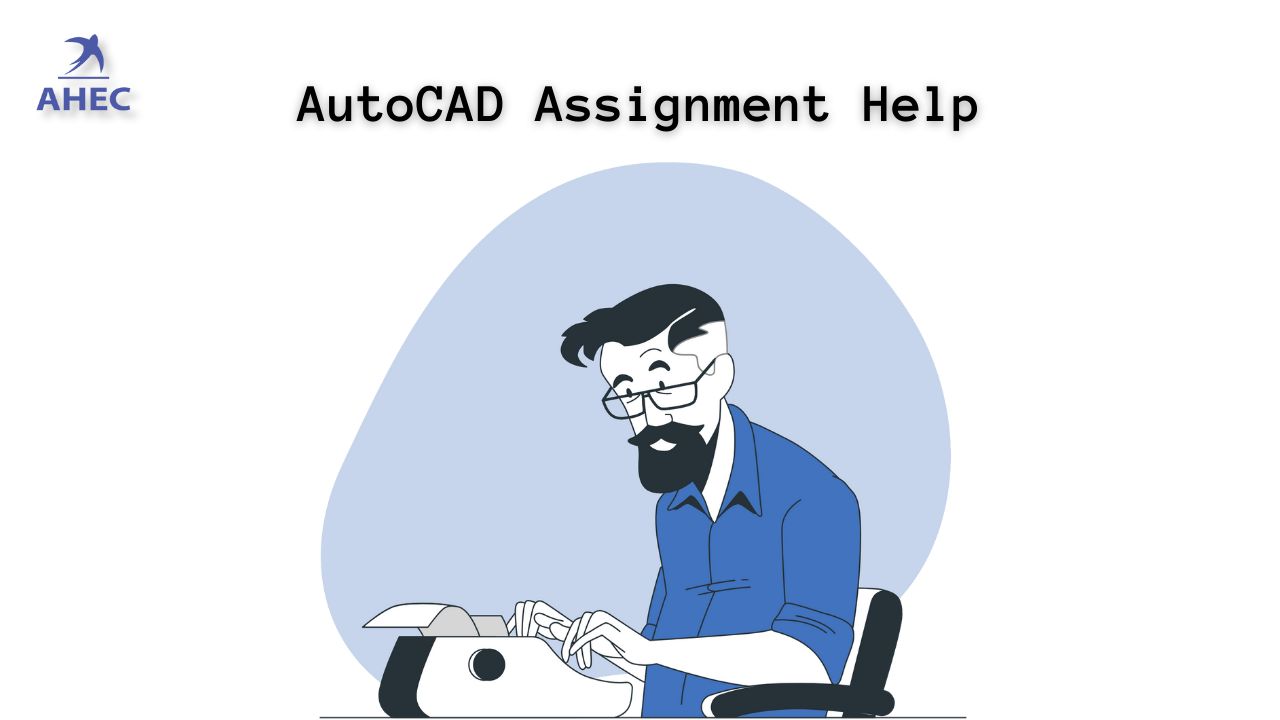
Auto CAD Assignment Help

Public Relations Assignment Help

Bioinformatics Assignment Help
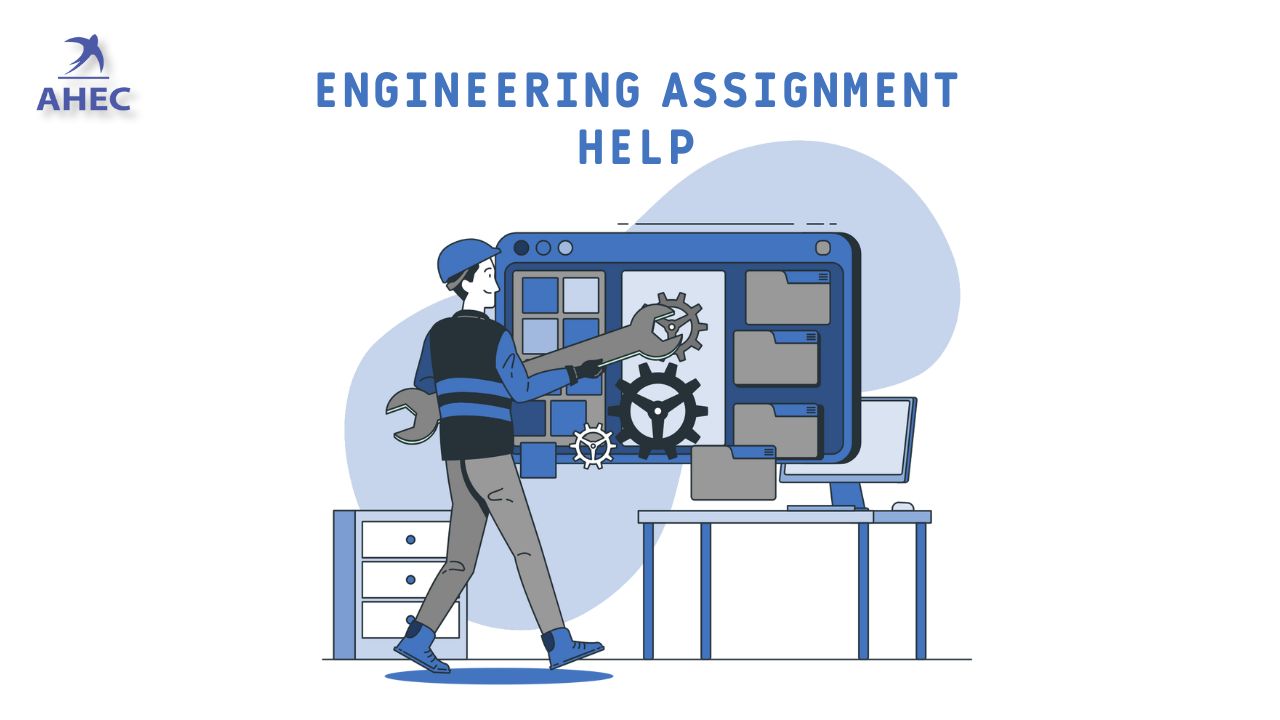
Engineering Assignment Help
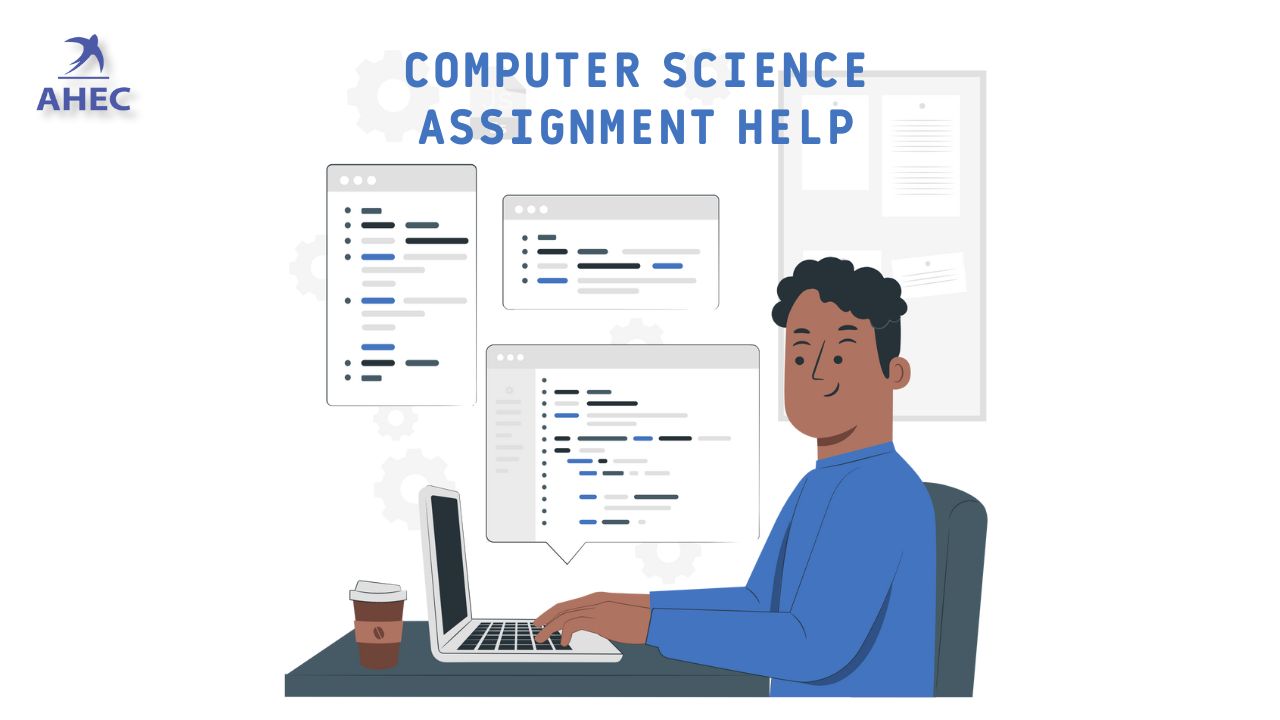
Computer Science Assignment Help

C++ Programming Assignment Help

Aerospace Engineering Assignment Help

Agroecology Assignment Help

Finance Assignment Help

Conflict Management Assignment Help

Paleontology Assignment Help

Commercial Law Assignment Help

Criminal Law Assignment Help

Anthropology Assignment Help

Biochemistry Assignment Help

Get the best cheap assignment Help

Online Pharmacology Course Help

Urgent Assignment Help

Paying For Assignment Help

HND Assignment Help

Legitimate Essay Writing Help
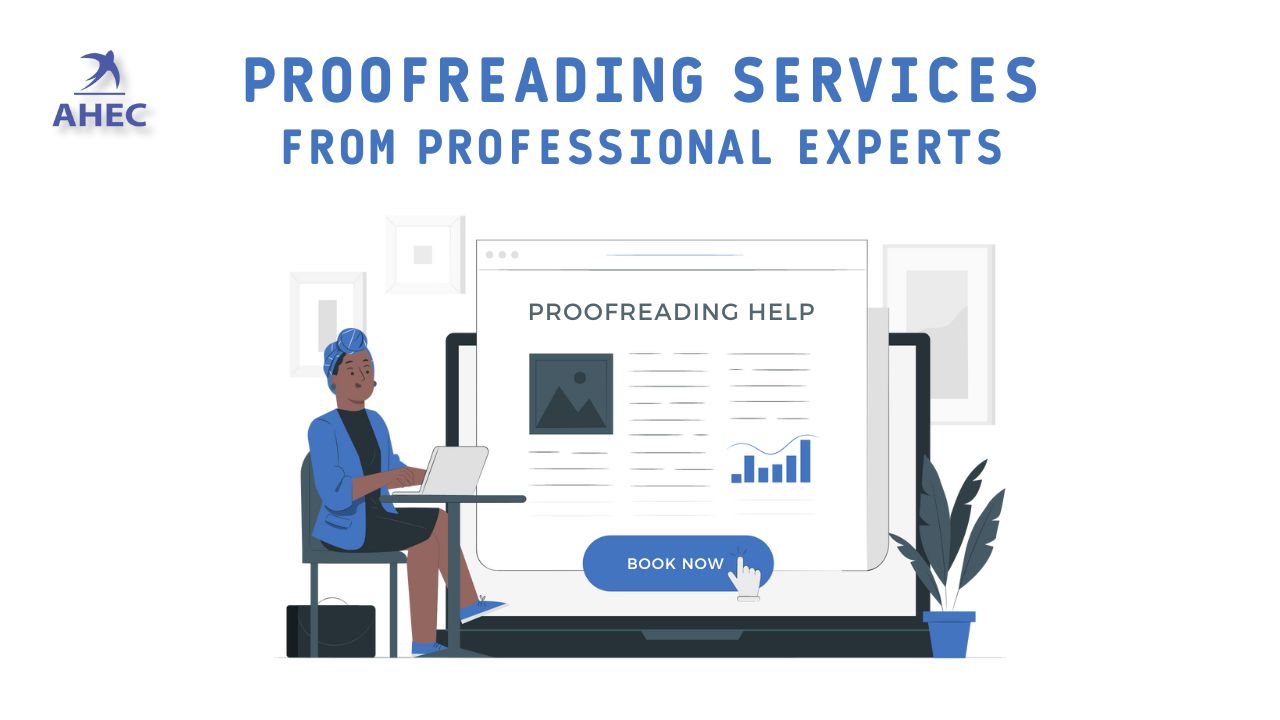
Best Online Proofreading Services

Need Help With Your Academic Assignment
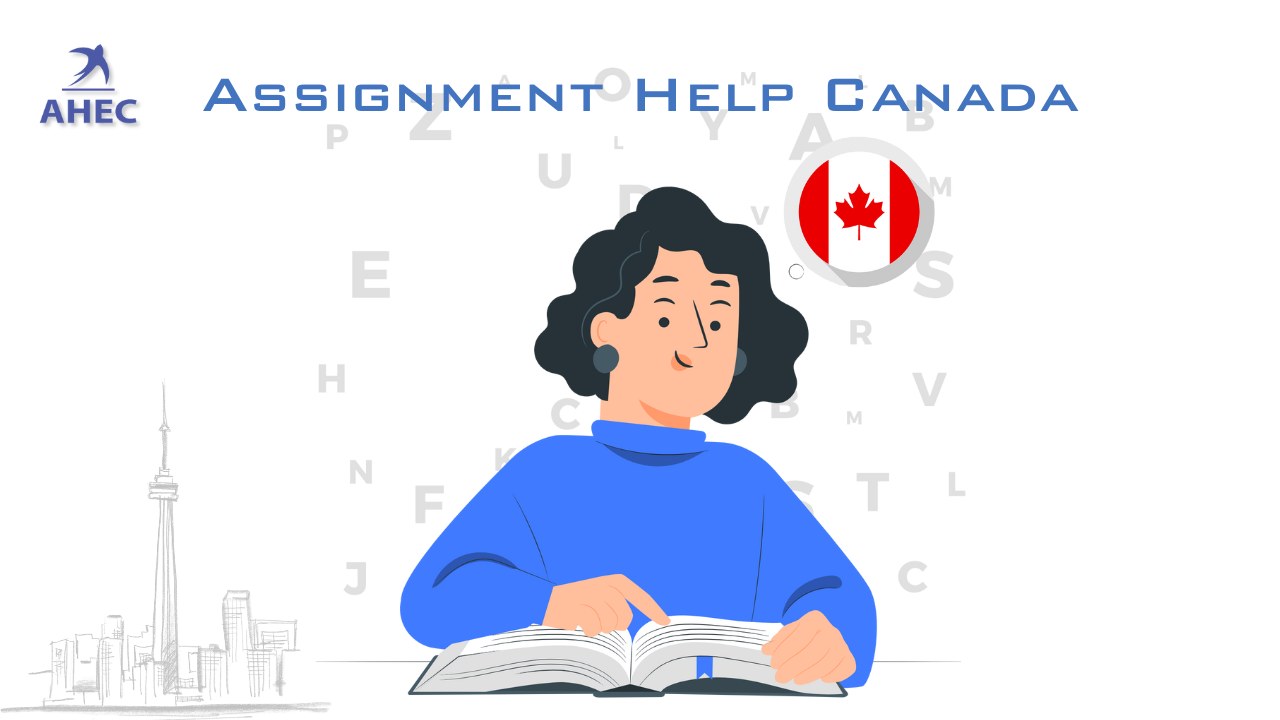
Assignment Writing Help In Canada

Assignment Writing Help In UAE

Online Assignment Writing Help in the USA

Assignment Writing Help In Australia

Assignment Writing Help In the UK

Scholarship Essay Writing Help

University of Huddersfield Assignment Help

Ph.D. Assignment Writing Help

Law Assignment Writing Help

Website Design and Development Assignment Help

200 Research paper topics for Healthcare
In such a complicated and vast field of study as medicine, writing a unique and convincing research paper is an overwhelming endeavor. From researching public health concerns to studying cancer treatments, Each student chooses which areas of interest are most important to him. Our aim is to assist students in finding new avenues to research and concentrate on pertinent topics. With our tools, you can compose an engaging and thorough essay.
How to Choose Medical Research Paper Topics
The process of selecting a topic for your research paper is usually more difficult than writing the paper itself. It is essential to choose an intriguing topic that will draw the attention of readers, demonstrate your expertise in a particular subject, aid you in progressing through your education, and maybe even inspire your future research.
In order to accomplish the best results, start with brainstorming, then an exhaustive study. Here are some helpful suggestions to follow:
- Choose a topic that is interesting. It is important to choose a topic that you are interested in but make sure it's not overly broad or too specific. You should be able to dig deep into the subject and demonstrate that you're an expert who is willing to tackle any difficult task in the field you've chosen to specialize in.
- You can narrow your focus once you've got an idea of topics to consider by looking through the most recent medical research papers in order to stay up-to-date with the latest developments, trends, and challenges in healthcare and medicine . Explore books, news articles, and other pertinent sources for further information about your possible topics. If a specific issue or illness interests you (perhaps one that has drawn you to the field of medicine), then you're in the right place to narrow down your subject.
- Find the "why," "how," and "what" - Whether you're researching nutrition research paper issues, controversial medical subjects or nursing research topics , or any other topic, think about why each of them is relevant. What are they that they can contribute to the existing research in medical science or research? What new knowledge could be gained to help improve the field of medicine? Answering these questions can aid you in selecting the best research paper in medical science that you like and will help you achieve your goals.
New Medical Research Paper Topics
Are you looking for the latest and most intriguing advancements in the field of medicine? We have put hours into identifying current developments in research in health so that we can offer the following examples of subjects. If you are hiring the services of writing service for research for students or writing your own paper on your own, you will require a captivating subject to write about.
- Epidemics versus pandemics
- Health care for children
- Medical humanitarian missions in the developing world
- Homeopathic remedies - - the placebo effect
- Infections caused by viruses - causes and treatment
- Are animal research studies in medical science ethical?
- Vaccination - dangers versus benefits
- Organs and artificial tissues
- Rare genetic disorders
- Brain injuries
Medical Research Topics for College Students
Are you unsure of where to begin with your medical research article? It's so overwhelming with all the subjects you can write about that the biggest challenge is narrowing them down. That's why we've decided to offer assistance.
- Treatments for antibiotics
- Chronic illnesses
- Palliative care
- Combating Alzheimer's disease
- The impact of modern life on the public health
- Professional illnesses
- Sleep disorders
- Physical and mental health as a result of the aging process
- Eating disorders
- End-of-life illnesses
Controversial Medical Topics for Research Paper
In the field of healthcare, breakthroughs can transform lives within the quick blink of an eye. This is why there are so many controversial issues in the field of medicine. These topics cover everything from ethics to religion or social obligation. Find out more about the most controversial research topics.
- Implementing food safety standards
- Gluten allergy
- Aided suicide for terminally ill patients
- Animal testing for vaccines - ethical issues
- Moral responsibilities in relation to the process of cloning
- Legalization of Marijuana for medical use
- Abortion - Medical Solutions
- Vegan diets: benefits and risks
- A higher life expectancy can be a burden for the health system.
- Circumcision effects
Health Research Topics
Students who conduct health research have trouble coming up with ideas that are relevant to their interests in medicine. If you are looking to write interesting college essays, then you should pick the right topic from our list.
- How do environmental changes affect the health of humans?
- Deafness: communication disorders
- Air pollution in the household
- A public health risk is a diabetes.
- Coronaviruses
- Health assessment of the oral cavity
- Control of alcohol and tobacco
- Health problems caused by the inactivity of people
- How can urban pollution impact respiratory ailments?
- Healthy diets
Medicine Research Topics
No matter what the requirements for your research project are, you are able to write about something interesting and beneficial to your career. Select a topic below.
- What are the causes of the rising cancer cases?
- Insulin resistance
- How does terrorism impact mental health?
- AIDS/HIV - latest developments
- Treating pregnant women versus non-pregnant women
- The latest innovations in medical instruments
- Genetic engineering
- Treatment of mental illnesses that is successful
- Does autism cause a problem?
- Natural coma versus artificial coma
Healthcare Research Topics
Healthcare research encompasses social and political aspects in addition to medical. For students at college who want to learn more about how the medical profession is affected by the social values or principles of society, We provide examples of subjects for research papers. Select one of them from the options below.
- Healthcare services are a priority for the government in the EU in comparison to the USA.
- Healthcare assistance and other services
- Health records electronic systems have pros and pros and
- Can asylums treat mental disorders?
- Healthcare services for prisoners
- Equipment for improving the treatment of AIDS
- Correlation between the development of economies and health care services across nations
- Smoking can cause organ damage.
- Heart attacks - their causes and consequences
- Breast cancer - recent developments
Public Health Research Topics
For the most current examples of public health-related topics, check out our collection. We offer only authentic, researched examples, and you'll be able to locate the evidence and data to back them up.
- Hospitals that are private and public
- Health care professionals - management principles
- Failures of surgery - who's the person responsible?
- What legal responsibilities does the hospital administration been entrusted with?
- The quality of care provided to patients in public contrasts with private hospitals.
- What advantages can national health systems offer?
- Cost estimates for cancer treatment
- Public health in developing countries
- Eliminating tobacco advertising is important to public health.
- Government solutions to the anti-vaccine movement
Mental Health Research Paper Topics
Mental health is among the most complicated areas of medicine, in which there isn't a lot of clarity like other medical concerns. This makes it more difficult to research the potential of the field, which has a wide range of issues to discuss.
- Anxiety disorders are a result of a variety of causes.
- Anorexia and Bulimia
- Childhood trauma
- Public health policies for mental health
- Postpartum Depression
- Posttraumatic Stress Disorder
- Seasonal Affective Disorder
- Schizophrenia
Anatomy Research Topics
Anatomy is a comprehensive study of our body as well as how it functions. If you are interested and are willing to pay for medical research papers, begin by choosing a subject.
- Chemotherapy: how does it affect the body
- Thyroid glands play a role in the body.
- Human endocrine system
- Heart disease
- Does the human muscle system develop?
- The lymphatic system is important.
- Examining genetic causes for diseases
- Digestive system
Biomedical Research Topics
Medicine and biology frequently are inextricably linked. For the most recent developments in biomedical science, look up our topic.
- Alzheimer's disease: paths to treatment
- Development of vaccines and drugs to treat Ebola
- Resistance to antibiotics
- The biological effects of the process of aging
- Health effects of air pollution
- Infectious disease past versus present
- Regenerative medicine
- Diagnostics for biomedical diseases
- Biomedical technology
Bioethics Research Topics
A controversial area in medicine, bioethics can be a place where you can have the chance to contribute your personal perspective to a topic of study and come up with fresh perspectives. These subjects are worth considering.
- Organ donation
- Alternative or complementary medicines
- Affirmative suicide, or the right to die
- Artificial insemination, also known as surrogacy or artificial insemination.
- Chemical and biological conflict
- Contraception
- Bioethics and environmental bioethics
- In Vitro Fertilization
Cancer Research Topics
Do you have a piece that deals with cancer causes, treatment, diagnosis, or effects? Check out the following hot subject that's simple to investigate and vital for advancement in medical research.
- Immun system cells' ability to fight against cancer
- Computational Oncology
- Metastasis is influenced by resistance to drugs.
- Stem cells can be used to treat cancer.
- Tumor microenvironment
- Age and obesity in the development of cancer
- Early cancer detection - benefits
- Artificial intelligence can detect cancer.
- Hematologic malignancies
- Cancers caused by pathogens
Clinical Research Topics
Find out more about the field of clinical medicine by conducting a more thorough study. We have prepared a list of important topics to be discussed.
- Concerns about ethics in research conducted on human subjects
- Subject recruitment
- Budget preparation
- Human subject protection
- Financial support for clinical trials
- Health professionals should be aware of clinical practices.
- The use of patients at risk in clinical research
- Clinical research quality assurance
- Clinical trials units
- Management and collection of data
Critical Care Research Topics
Critical care is an important aspect of medical studies. Examine these areas within your study paper in order to acquire additional knowledge in the field. You can also make contact with nurses who write nursing research papers.
- Asthma and obesity - signs and symptoms
- Chronic obstructive lung disease
- A Rhythm Analysis to detect cardiac arrest
- Traumatic brain injury and fluid Resuscitation
- Hydrocortisone is a treatment for multiple trauma patients.
- Nutrition and care for seriously disabled adults
- The diagnosis of hypersensitivity pneumonitis
- Scales for sedation and coma
- Artificial airways sucking
- The arterial line and puncture of the arterial
Pediatric Research Topics
Anything that concerns pediatric health or pregnant women, mothers, and adolescents is covered under the umbrella of pediatric health treatment.
- ADHD is a form of attention deficit hyperactivity disorder. (ADHD)
- The congenital heart condition is common in infants.
- Adolescent medication
- Neonatal medicine
- Rare diseases that affect adolescents and children
- Weight fluctuations and obesity
- Sleep problems with children that are a result of behavioral issues
- Children suffering from anemia
Dental Research Topics Ideas
Pick a topic about dental health or oral hygiene from this selection of fascinating subjects in the area.
- What effects does smoking have on oral health?
- Children's risk for dental caries
- Dental Anxiety
- Dental materials of various types - the latest advances
- Bad breath bacteria
- How does diabetes impact oral health?
- Oral cancer
- Dental pain can be caused by a variety of causes.
- Dental implants
- Oral health-related quality of living
Dermatology Research Topics
Find the most relevant research topic for your dermatology research paper from our many examples.
- Atopic dermatitis
- Contact dermatitis
- Epidemiology behind uncommon skin disorders
- The process of aging is akin to a cutaneous one.
- Melanoma risk factors for skin cancer
- Acne versus rosacea
- Testing for genetics in skin problems
- The effects of cosmetics on the health of the skin
- Enhancing skin barrier using pharmaceutical agents
- The skin manifestations of autoimmune disorders
Primary Care Research Topics
Create a primary-care paper that demonstrates your skills in research and your passion for powerful scientific findings.
- Primary care for the most vulnerable or uninsured
- The continuity of care in the form of interpersonal relationships
- Primary care is a key component of health systems.
- Primary care models for the delivery of healthcare
- New developments in family medicine
- Occupational/environmental health
- Pharmacotherapy methods
- Tests for formal allergies
- Oral contraception side effects
- The use of behavioral or dietary interventions to aid in managing obesity
Pharmaceutical Research Topics
Students in the pharmacy field who require writing topics for their papers can choose one of our suggestions. We cover everything associated with the world of pharmacy.
- The drugs that treat cancer
- Drug excretion
- Elimination rate constant
- Treatment for stress-related inflammation with drugs
- Aspirin poising
- Ibuprofen - dangers versus benefits
- Toxicodynamics
- Opioid use disorder
- Treatment for schizophrenia with pharmacotherapy
- Ketamine as a treatment for depression
Medical Anthropology Research Topics
Medical anthropology is a fusion of different fields of human understanding. Get ideas for an effective research paper below.
- The cultural contexts that influence reproductive health
- Women sexuality
- Anthropological components of the health system
- Social science contributions to public health
- The ethics of medical and euthanasia in different cultures
- Health-related behaviors in adults from different the different cultures
- Transcultural nursing
- Forensic psychiatrist
- Signs and symptoms associated with Celiac Disease - a disease that is not accompanied by symptoms
- Ethics in nursing

Top 10 Best Universities Ranking list in India 2022

Generic Conventions: Assignment Help Services

Research Paper Topics For Medical

Top 5 Resources for Writing Excellent Academic Assignments

How to Write a Literature Review for Academic Purposes

Tips for Writing a killer introduction to your assignment

How To Write A Compelling Conclusion For Your University Assignment

Research Papers Topics For Social Science

Best 150 New Research Paper Ideas For Students

7 Best Plagiarism Checkers for Students And Teachers in 2024
Enquiry form.
What to expect in US healthcare in 2024 and beyond
The acute strain from labor shortages, inflation, and endemic COVID-19 on the healthcare industry’s financial health in 2022 is easing. Much of the improvement is the result of transformation efforts undertaken over the last year or two by healthcare delivery players, with healthcare payers acting more recently. Even so, health-system margins are lagging behind their financial performance relative to prepandemic levels. Skilled nursing and long-term-care profit pools continue to weaken. Eligibility redeterminations in a strong employment economy have hurt payers’ financial performance in the Medicaid segment. But Medicare Advantage and individual segment economics have held up well for payers.
As we look to 2027, the growth of the managed care duals population (individuals who qualify for both Medicaid and Medicare) presents one of the most substantial opportunities for payers. On the healthcare delivery side, financial performance will continue to rebound as transformation efforts, M&A, and revenue diversification bear fruit. Powered by adoption of technology, healthcare services and technology (HST) businesses, particularly those that offer measurable near-term improvements for their customers, will continue to grow, as will pharmacy services players, especially those with a focus on specialty pharmacy.
Below, we provide a perspective on how these changes have affected payers, health systems, healthcare services and technology, and pharmacy services, and what to expect in 2024 and beyond.
The fastest growth in healthcare may occur in several segments
We estimate that healthcare profit pools will grow at a 7 percent CAGR, from $583 billion in 2022 to $819 billion in 2027. Profit pools continued under pressure in 2023 due to high inflation rates and labor shortages; however, we expect a recovery beginning in 2024, spurred by margin and cost optimization and reimbursement-rate increases.
Several segments can expect higher growth in profit pools:
- Within payer, Medicare Advantage, spurred by the rapid increase in the duals population; the group business, due to recovery of margins post-COVID-19 pandemic; and individual
- Within health systems, outpatient care settings such as physician offices and ambulatory surgery centers, driven by site-of-care shifts
- Within HST, the software and platforms businesses (for example, patient engagement and clinical decision support)
- Within pharmacy services, with specialty pharmacy continuing to experience rapid growth
On the other hand, some segments will continue to see slow growth, including general acute care and post-acute care within health systems, and Medicaid within payers (Exhibit 1).
Several factors will likely influence shifts in profit pools. Two of these are:
Change in payer mix. Enrollment in Medicare Advantage, and particularly the duals population, will continue to grow. Medicare Advantage enrollment has grown historically by 9 percent annually from 2019 to 2022; however, we estimate the growth rate will reduce to 5 percent annually from 2022 to 2027, in line with the latest Centers for Medicare & Medicaid Services (CMS) enrollment data. 1 Congressional Budget Office Baseline Projections, May 2023; Medicare Advantage penetration was increasing by less than 2 percent annually from 2016 to 2019 but increased by about 3 percent annually in 2020 and 2021—for further information, see “Medicare Advantage/Part D contract and enrollment data,” Centers for Medicare & Medicaid Services, US Government. Finally, the duals population enrolled in managed care is estimated to grow at more than a 9 percent CAGR from 2022 through 2027.
We also estimate commercial segment profit pools to rebound as EBITDA margins likely return to historical averages by 2027. Growth is likely to be partially offset by enrollment changes in the segment, prompted by a shift from fully insured to self-insured businesses that could accelerate as employers seek to cut costs if the economy slows. Individual segment profit pools are estimated to expand at a 27 percent CAGR from 2022 to 2027 as enrollment rises, propelled by enhanced subsidies, Medicaid redeterminations, and other potential favorable factors (for example, employer conversions through the Individual Coverage Health Reimbursement Arrangement offered by the Affordable Care Act); EBITDA margins are estimated to improve from 2 percent in 2022 to 5 to 7 percent in 2027. On the other hand, Medicaid enrollment could decline by about ten million lives over the next five years based on our estimates, given recent legislation allowing states to begin eligibility redeterminations (which were paused during the federal public health emergency declared at the start of the COVID-19 pandemic 2 Meghana Ammula and Jennifer Tolbert, “10 things to know about the unwinding of the Medicaid Continuous Enrollment requirement,” Kaiser Family Foundation, October 25, 2022. ).
Accelerating value-based care (VBC). Based on our estimates, 90 million lives will be in VBC models by 2027, from 43 million in 2022. This expansion will be fueled by an increase in commercial VBC adoption, greater penetration of Medicare Advantage, and the Medicare Shared Savings Program (MSSP) model in Medicare fee-for-service. Also, substantial growth is expected in the specialty VBC model, where penetration in areas like orthopedics and nephrology could more than double in the next five years.
VBC models are undergoing changes as CMS updates its risk adjustment methodology and as models continue to expand beyond primary care to other specialties (for example, nephrology, oncology, and orthopedics). We expect established models that offer improvements in cost and quality to continue to thrive. The transformation of VBC business models in response to pressures from the current changes could likely deliver outsized improvement in cost and quality outcomes. The penetration of VBC business models is likely to lead to shifts in health delivery profit pools, from acute-care settings to other sites of care such as ambulatory surgical centers, physician offices, and home settings.
Payers: Government segments are expected to be 65 percent larger than commercial segments by 2027
In 2022, overall payer profit pools were $60 billion. Looking ahead, we estimate EBITDA to grow to $78 billion by 2027, a 5 percent CAGR, as the market recovers and approaches historical trends. Drivers are likely to be margin recovery of the commercial segment, inflation-driven incremental premium rate rises, and increased participation in managed care by the duals population. This is likely to be partially offset by margin compression in Medicare Advantage due to regulatory pressures (for example, risk adjustment, decline in the Stars bonus, and technical updates) and membership decline in Medicaid resulting from the expiration of the public health emergency.
We estimate increased labor costs and administrative expenses to reduce payer EBITDA by about 60 basis points in 2023. In addition, health systems are likely to push for reimbursement rate increases (up to about 350 to 400 basis-point incremental rate increases from 2023 to 2027 for the commercial segment and about 200 to 250 basis points for the government segment), according to McKinsey analysis and interviews with external experts. 3 For further information on the government segment, see “Medicare Payment Advisory Commission public meeting,” Medicare Payment Advisory Commission, December 8, 2022.
Our estimates also suggest that the mix of payer profit pools is likely to shift further toward the government segment (Exhibit 2). Overall, the profit pools for this segment are estimated to be about 65 percent greater than the commercial segment by 2027 ($36 billion compared with $21 billion). This shift would be a result of increasing Medicare Advantage penetration, estimated to reach 52 percent in 2027, and likely continued growth in the duals segment, expanding EBITDA from $7 billion in 2022 to $12 billion in 2027.
Profit pools for the commercial segment declined from $18 billion in 2019 to $15 billion in 2022. We now estimate the commercial segment’s EBITDA margins to regain historical levels by 2027, and profit pools to reach $21 billion, growing at a 7 percent CAGR from 2022 to 2027. Within this segment, a shift from fully insured to self-insured businesses could accelerate in the event of an economic slowdown, which prompts employers to pay greater attention to costs. The fully insured group enrollment could drop from 50 million in 2022 to 46 million in 2027, while the self-insured segment could increase from 108 million to 113 million during the same period.
Health systems: Transformation efforts help accelerate EBITDA recovery
In 2023, health-system profit pools continued to face substantial pressure due to inflation and labor shortages. Estimated growth was less than 5 percent from 2022 to 2023, remaining below prepandemic levels. Health systems have undertaken major transformation and cost containment efforts, particularly within the labor force, helping EBITDA margins recover by up to 100 basis points; some of this recovery was also volume-driven.
Looking ahead, we estimate an 11 percent CAGR from 2023 to 2027, or total EBITDA of $366 billion by 2027 (Exhibit 3). This reflects a rebound from below the long-term historical average in 2023, spurred by transformation efforts and potentially higher reimbursement rates. We anticipate that health systems will likely seek reimbursement increases in the high single digits or higher upon contract renewals (or more than 300 basis points above previous levels) in response to cost inflation in recent years.
Measures to tackle rising costs include improving labor productivity and the application of technological innovation across both administration and care delivery workflows (for example, further process standardization and outsourcing, increased use of digital care, and early adoption of AI within administrative workflows such as revenue cycle management). Despite these measures, 2027 industry EBITDA margins are estimated to be 50 to 100 basis points lower than in 2019, unless there is material acceleration in performance transformation efforts.
There are some meaningful exceptions to this overall outlook for health systems. Although post-acute-care profit pools could be severely affected by labor shortages (particularly nurses), other sites of care might grow (for example, non-acute and outpatient sites such as physician offices and ambulatory surgery centers). We expect accelerated adoption of VBC to drive growth.
HST profit pools will grow in technology-based segments
HST is estimated to be the fastest-growing sector in healthcare. In 2021, we estimated HST profit pools to be $51 billion. In 2022, according to our estimates, the HST profit pool shrank to $49 billion, reflecting a contracting market, wage inflation pressure, and the drag of fixed-technology investment that had not yet fulfilled its potential. Looking ahead, we estimate a 12 percent CAGR in 2022–27 due to the long-term underlying growth trend and rebound from the pandemic-related decline (Exhibit 4). With the continuing technology adoption in healthcare, the greatest acceleration is likely to happen in software and platforms as well as data and analytics, with 15 percent and 22 percent CAGRs, respectively.
In 2023, we observed an initial recovery in the HST market, supported by lower HST wage pressure and continued adoption of technology by payers and health systems searching for ways to become more efficient (for example, through automation and outsourcing).
Three factors account for the anticipated recovery and growth in HST. First, we expect continued demand from payers and health systems searching to improve efficiency, address labor challenges, and implement new technologies (for example, generative AI). Second, payers and health systems are likely to accept vendor price increases for solutions delivering measurable improvements. Third, we expect HST companies to make operational changes that will improve HST efficiency through better technology deployment and automation across services.
Pharmacy services will continue to grow
The pharmacy market has undergone major changes in recent years, including the impact of the COVID-19 pandemic, the establishment of partnerships across the value chain, and an evolving regulatory environment. Total pharmacy dispensing revenue continues to increase, growing by 9 percent to $550 billion in 2022, 4 This metric excludes rebates and discounts paid by manufacturers to pharmacy benefit managers and plan sponsors; it includes pharmacy benefit drugs only. with projections of a 5 percent CAGR, reaching $700 billion in 2027. 5 Adam J. Fein, “The 2023 economic report on US pharmacies and pharmacy benefit managers,” Drug Channels Institute, 2023. Specialty pharmacy is one of the fastest growing subsegments within pharmacy services and accounts for 40 percent of prescription revenue 6 This figure excludes rebates and does not represent payers' net spending. ; this subsegment is expected to reach nearly 50 percent of prescription revenue in 2027 (Exhibit 5). We attribute its 8 percent CAGR in revenue growth to increases in utilization and pricing as well as the continued expansion of pipeline therapies (for example, cell and gene therapies and oncology and rare disease therapies) and expect that the revenue growth will be partially offset by reimbursement pressures, specialty generics, and increased adoption of biosimilars. Specialty pharmacy dispensers are also facing an evolving landscape with increased manufacturer contract pharmacy pressures related to the 340B Drug Pricing Program. With restrictions related to size and location of contract pharmacies that covered entities can use, the specialty pharmacy subsegment has seen accelerated investment in hospital-owned pharmacies.
Retail and mail pharmacies continue to face margin pressure and a contraction of profit pools due to reimbursement pressure, labor shortages, inflation, and a plateauing of generic dispensing rates. 7 ” Meeting changing consumer needs: The US retail pharmacy of the future ,” McKinsey, March 17, 2023. Many chains have recently announced 8 Pamela N. Danziger, “Drugstore downsizing: CVS, Walgreens and Rite Aid to close nearly 1,500 stores,” Forbes, September 27, 2023; Jin Yu Young, “Rite Aid is closing 154 stores as it starts to shed debt,” New York Times, October 19, 2023. efforts to rationalize store footprints while continuing to augment additional services, including the provision of healthcare services.
Over the past year, there has also been increased attention to broad-population drugs such as GLP-1s (indicated for diabetes and obesity). The number of patients meeting clinical eligibility criteria for these drugs is among the largest of any new drug class in the past 20 to 30 years. The increased focus on these drugs has amplified conversations about care and coverage decisions, including considerations around demonstrated adherence to therapy, utilization management measures, and prescriber access points (for example, digital and telehealth services). As we look ahead, patient affordability, cost containment, and predictability of spending will likely remain key themes in the sector. The Inflation Reduction Act is poised to change the Medicare prescription Part D benefit, with a focus on reducing beneficiary out-of-pocket spending, negotiating prices for select drugs, and incentivizing better management of high-cost drugs. These changes, coupled with increased attention to broad-population drugs and the potential of high-cost therapies (such as cell and gene therapies), have set the stage for a shift in care and financing models.
The US healthcare industry faced demanding conditions in 2023, including continuing high inflation rates, labor shortages, and endemic COVID-19. However, the industry has adapted. We expect accelerated improvement efforts to help the industry address its challenges in 2024 and beyond, leading to an eventual return to historical-average profit margins.
Neha Patel is a partner in McKinsey’s New York office, and Shubham Singhal is the global leader of McKinsey’s Social, Healthcare, and Public Entities (SHaPE) Practice in the Detroit office.
The authors wish to thank Shahed Al-Haque, Maciej Biernacki, Claudia Castelino, Zachary Greenberg, Scott Heim, Ankit Jain, Gaurav Kohli, and Siddharth Manot for their contributions to this article.
Explore a career with us
Related articles.
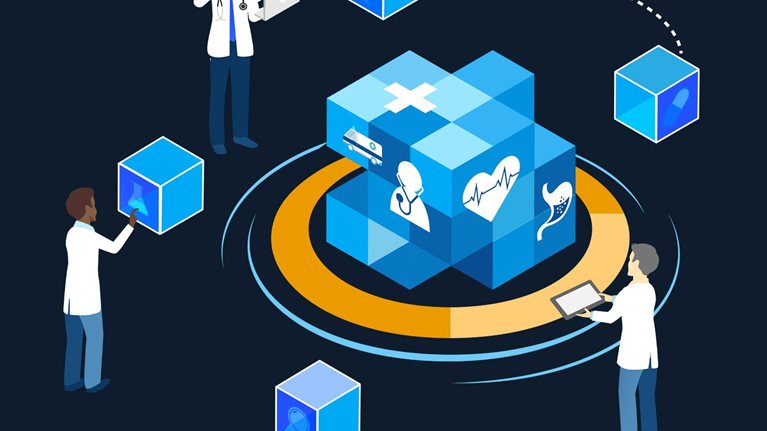
Investing in the new era of value-based care
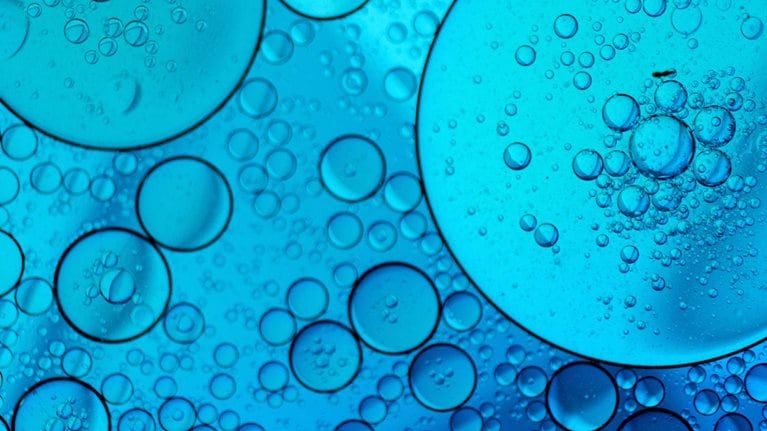
What to expect in US healthcare in 2023 and beyond
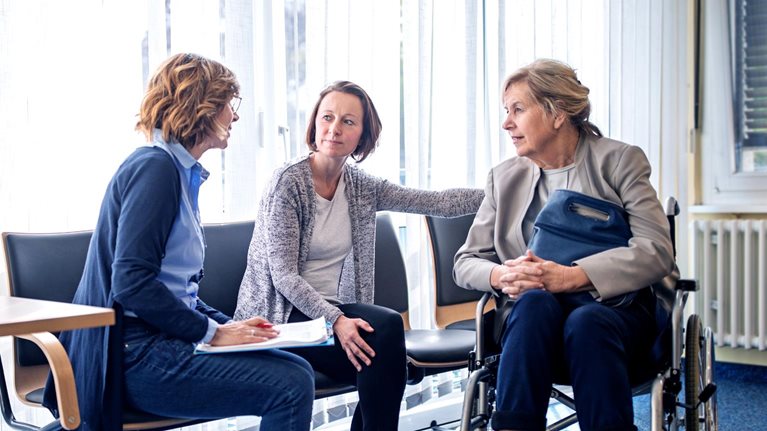
The future of Medicare Advantage

An official website of the United States government
The .gov means it’s official. Federal government websites often end in .gov or .mil. Before sharing sensitive information, make sure you’re on a federal government site.
The site is secure. The https:// ensures that you are connecting to the official website and that any information you provide is encrypted and transmitted securely.
- Publications
- Account settings
Preview improvements coming to the PMC website in October 2024. Learn More or Try it out now .
- Advanced Search
- Journal List
- Springer Nature - PMC COVID-19 Collection

The Potential of Big Data Research in HealthCare for Medical Doctors’ Learning
Manuel au-yong-oliveira.
1 INESC TEC, GOVCOPP, Department of Economics, Management, Industrial Engineering and Tourism, University of Aveiro, Aveiro, Portugal
Antonio Pesqueira
2 Bavarian Nordic A/S, Hellerup, Denmark
Maria José Sousa
3 ISCTE Instituto Universitário de Lisboa, Lisbon, Portugal
Francesca Dal Mas
4 Lincoln International Business School, University of Lincoln, Lincoln, UK
Mohammad Soliman
5 University of Technology and Applied Sciences, Salalah CAS, Salalah, Oman
6 Faculty of Tourism & Hotels, Fayoum University, Fayoum, Egypt
The main goal of this article is to identify the main dimensions of a model proposal for increasing the potential of big data research in Healthcare for medical doctors’ (MDs’) learning, which appears as a major issue in continuous medical education and learning. The paper employs a systematic literature review of main scientific databases (PubMed and Google Scholar), using the VOSviewer software tool, which enables the visualization of scientific landscapes. The analysis includes a co-authorship data analysis as well as the co-occurrence of terms and keywords. The results lead to the construction of the learning model proposed, which includes four health big data key areas for MDs’ learning: 1) data transformation is related to the learning that occurs through medical systems; 2) health intelligence includes the learning regarding health innovation based on predictions and forecasting processes; 3) data leveraging regards the learning about patient information; and 4) the learning process is related to clinical decision-making, focused on disease diagnosis and methods to improve treatments. Practical models gathered from the scientific databases can boost the learning process and revolutionise the medical industry, as they store the most recent knowledge and innovative research.
Introduction and conceptualisation
The new technologies and the consequent increasing scale of data being generated in the healthcare sector are forcing healthcare professionals, and especially medical doctors (MDs), to understand how to connect data for new growth opportunities, enabling greater efficiency in diagnosis and research activities, resulting in better addressing the patients’ needs [ 1 ].
The current literature is highlighting how healthcare analytics and data decision processes are becoming more and more critical [ 2 ]. Data allows healthcare organisations like hospitals and clinics, to maximise innovation and transformation in several different ways, affecting the decision-making process of healthcare practitioners, including MDs. Technologies like Big Data analytics and Artificial Intelligence (AI) are supporting the management of complex situations, like new treatment options for chronic diseases [ 3 , 4 ], less available human resources in hospitals [ 5 ] and a growing set of uncertain outcomes from viruses and bacterial phenomena, including the recent COVID-19 pandemic [ 6 – 9 ].
Even before the current pandemic crisis, the system was witnessing an escalating pressure on hospitals, healthcare facilities, and practitioners to be successively cost-effective [ 10 ], with increasing difficulty to justify any additional spending or investment in medical education or MD training [ 8 , 11 ].
While the industry still tries to understand the best investments in medical education and clinical training, there are growing investments in new skill development around Big Data, Data Science, AI, and Machine Learning [ 12 , 13 ]. Without exception, a growing need is emerging from healthcare professionals, especially MDs, to be better being prepared for new systems, challenges, and demands from different spectrums of healthcare organisations (HCOs) and all their related key stakeholders.
Even though HCO decision-makers, administrators, and department heads recognise the importance of appropriate education in terms of technology and data management for MDs [ 14 ], continuing education and skills development in Big Data is often forgotten. While some professions call for continued education to meet Big Data needs and requirements, MDs are still not in the spotlight in terms of receiving that advanced training that can play a decisive role in earlier disease intervention, reduced probability of adverse reactions to drugs, less medical errors, determination of causalities, understanding of co-morbidity, and many other challenges [ 9 ]. Still, new complex situations call for new competencies and tools to support those in charge in the management of everyday medical practice. The literature has identified several issues and opportunities related to Big Data and learning for MDs and other healthcare professionals. While theoretical contributions may offer pioneering insights, they often fail in suggesting implications for practice.
Following these premises, there is a call to understand the state-of-the-art of the literature, but also how MDs learning about Big Data can be practically fostered and promoted to lead to better outcomes from the whole medical system. Therefore, starting from the results of a systematic literature review, our Research Question (RQ) is:
RQ: Which are the main dimensions of a model proposal for increasing the potential of Big Data research in Healthcare for MDs’ learning?
Big data research in healthcare
Big Data refers to the mass of structured and unstructured data generated worldwide [ 15 ]. In healthcare, this encompasses everything from electronic medical records, to internet-connected (loT) devices like medical wearables or augmented reality diagnostic tools [ 16 , 17 ].
In general concepts and according to the broader literature, Big Data spans four dimensions that usually are called the 4 V’s. The 4 V’s are as follows: Volume, Veracity, Velocity, and Variety [ 18 ].
For any HCO, several different processes are being built on data and analytics to maintain a competitive and profitable edge in the face of all of the new regulations and governmental pressures for the maximisation of costs and results, but also given a growing need to improve performance, reduce errors in medical practice and discover new pathways for improved health outcomes [ 19 ].
Medical technologies and clinical activities are extremely complex and diverse. Big Data algorithms and data collection models are becoming more challenging than ever, where new wearables, data sources, and mobile apps that are being used by patients are increasing tremendously [ 17 , 20 ]. That exponential growth of data will allow MDs to improve diagnoses, prognoses, and therapy through a better understanding of their patients’ condition [ 11 ]. The impressive signs of progress of Big Data in the medical field [ 21 ] will lead to a reassessing of the worldwide view of healthcare systems and organisations [ 22 ]. Although there is still an open debate on the cost of health, it seems that only human intelligence can understand today’s dispersal of data.
Continuous medical education and learning
Nowadays MDs are also being required to support healthcare businesses to learn and quickly distil new information from masses of data [ 23 ] and transform them into actionable insights regarding cost reduction, maximisation of treatment options, diagnostic pathways, or new solutions to various problems impacting financial and human resources. The ability to extract these insights will empower the future of health and become a differentiator for companies and organiations to thrive and stay ahead of emerging competitors [ 24 – 26 ]. Continuous medical education allows for the improving of MDs’ knowledge, preparing healthcare professionals to care for and diagnose in a very demanding and highly technological, multidisciplinary environment [ 23 , 27 ].
In attempts to provide better Big Data knowledge and technical expertise to operate with different data sets, databases and insight query to MDs both efficiently and cost-effectively, hospitals and HCOs often implement online modules, hire external third-party vendors or sponsor specific academic courses. For example, most MDs are familiar with diagnostic tools and electronic medical record systems, but advanced systems that use AI or data science algorithms are still an area open to much improvement [ 28 – 31 ]. Although these concrete needs require knowledge and data, MDs are often too busy and too tired to go through the material and retain information consciously. Moreover, the lack of clinical professionals does not allow much time for MDs to concentrate on training.
Not only do professionals need a constantly updated curriculum, but they also need to have a focus on inter-professional learning in the context of continuing professional development. The entry-level healthcare curriculum should introduce to all employees the concept of lifelong learning [ 32 ]. Healthcare professionals at every stage of their career must continue to learn about advances in research, treatments, knowledge, and skills required to provide safe and effective patient care [ 33 ]. It is the responsibility of the professional to keep abreast of technological changes and relevant medical knowledge [ 16 , 22 ]. In this perspective, more and more e-learning options are available, with an increasing number of attendees, especially during the COVID pandemic crisis [ 14 , 34 ].
Most of the worldwide HCOs are adopting robust data strategy [ 35 ] relying on Big Data techniques and concepts [ 36 – 39 ], where MDs are playing a decisive role to manage internal procedures and lead advanced diagnostics, treatments, medical prescriptions and consultations as well as hospital discharges/admissions more effectively. According to the literature, some of those activities and key topics can be divided into four major areas: (1) Data transformation, (2) health intelligence, (3) data leveraging, and (4) decision making, as reported in Table Table1 1 .
Four Big Data key areas for MDs
After a first approach to identify the main concepts and issues related to Big Data and MDs’ learning, the paper employs a systematic literature review, conducted in prestigious scientific databases, to identify the main topics and research avenues, to later define a tentative model as a practical implication of the study.
The paper develops as follows: the following section explains the methodology, followed by the results gathered in the literature; a discussion of our findings leads to a tentative practical model to implement MD learning taking into account the Big Data issues and potential. A conclusion section ends the paper.
Methodology
A systematic literature review, in academia, is done primarily in order to identify where we currently stand on a specific academic topic or regarding a specific academic research question – identifying what has been discovered (trends, patterns, lines of research, new theory, prominent researchers) and what remains to be discovered (including gaps in the literature). For this purpose we used VOSviewer software [ 49 ], which is good for “visualizing scientific landscapes” [ 50 ]. VOSviewer represents an evidence-based technique that uses a reliable and structured method to perform the identification, analysis, and interpretation of several relevant academic papers related to the selected topic. The topic herein is Big Data research in the healthcare context and applied to MDs’ learning and training [ 51 ]. On the VOSviewer software tool one may add the following:
“VOSviewer is a software tool for constructing and visualizing bibliometric networks. These networks may for instance include journals, researchers, or individual publications, and they can be constructed based on citation, bibliographic coupling, co-citation, or co-authorship relations. VOSviewer also offers text mining functionality that can be used to construct and visualize co-occurrence networks of important terms extracted from a body of scientific literature.” [ 52 ].
The literature review aims to identify possible missing connections between the study of Big Data processes, technology, and procedures with continuous medical education, learning, and training in the healthcare context.
Examples of Big Data in healthcare and MDs’ education were identified by an initial literature review, after which the added value of 215 items was evaluated, based on the assessment of the added value and the quality of the pieces of evidence.
The selected search terms were derived from the keywords from our research question and also from all the previous literature review exercise where we were able to identify the key terms for the systematic literature review exercise. To better understand some of the key concepts and future network of terms, we also performed a preliminary literature analysis of the most relevant search terms, which will be further explained in the following paragraphs.
Additionally, to minimize the probability of missing relevant articles and publications, a combined search strategy was used with the preliminary literature review, involving the free resource PubMed search engine (accessing biomedical literature primarily from the bibliographic database MEDLINE) and the free Google Scholar search engine. The searches involved snowballing (backward and forwards). The snowballing approach was initially performed on the set of papers identified through the database searches. The approach included using the necessary basic criteria in which the selection applied and without major limitations (e.g. year, content) to identify the title and abstract of the papers, citing our set of selected papers.
After the preliminary and basic literature review of the most relevant publications and articles from an original initial selection of 758 items, we defined a search string used to search databases containing scientific papers in the context of Big Data research applied to medical education and learning. After applying the essential criteria exclusion, the resulting papers were defined as the starting set for the snowballing process. After executing the snowballing iterations, we used the advanced criteria exclusion, which is related to the actual data extraction and quality assessment. The whole process is reported in Fig. 1 .

Overview of the applied systematic literature review
The search terms were based on the research questions using synonyms and related terms. The following keywords were used to formulate the search string: a) Big Data Research; b) HealthCare learning and education c) continuous Medical Education; d) decision making MDS; e) Medical Doctors.
The above-selected search terms are derived from the keywords from our research question and also from all the previous literature review exercise where we were able to identify the key terms for the systematic literature review exercise. Additionally, the previously presented search terms were based on the areas that we want to further investigate the connections but avoiding terms and relationships that might go outside the keywords selected.
Performing the necessary searches with a restricted list of keywords also allowed a better quality and control of the selected relevant publications and more targeted content in terms of the necessary advanced criteria exclusions based on the VOSViewer requirements.
Our target population was all relevant papers in a healthcare context with medical, clinical, or biology-related activities or processes, where papers outside the healthcare industry were automatically removed as we intended to capture papers in that context regardless of the type of research performed.
Regarding the data sources, the goal was to cover the literature published about Big Data research applied to MDS learning, education and training, so the PubMed and Google Scholar digital databases were selected for data retrieval.
Before applying the selection criteria given the topic of the review, generic exclusion criteria were defined: a) published in non-peer-reviewed publication channels such as books, thesis or dissertations, tutorials, keynotes, and others. OR; b) Not available in English OR; c) A duplicate OR; d) published before 2010. The first two criteria were implemented in the search strings executed in the selected digital library, and the remaining papers were evaluated through two sets of selection criteria: a) basic and b) advanced. Figure Figure2 2 summarises the process.

Overview of the database search and selection criteria
Search and selection
The first criteria were based on the titles, content, abstract, language, year, and presented results of the papers, articles, or publications. These criteria were applied to the articles that passed a generic exclusion criterion and were identified through the database search or snowballing as described in the section above. In this context, the items included were related to big data research, healthcare learning, continuous medical education, decision making MDS, big data healthcare, and medical doctors.
Following the procedure presented [ 15 ], the papers were classified as: a) Relevant; b) Irrelevant; or c) Uncertain (in this case, the available information in the title and abstract is inconclusive).
For a profound understanding of all the results, we selected two types of analysis: (1) co-authorship analysis and (2) co-occurrence.
- This type of analysis presented the relatedness of all selected authors and co-authors and was determined based on their number of documents and networks of relationships. In this paper and terms of the advanced search criteria, the type of analysis selected for the co-authorship followed the unit of analysis of authors with a full counting method and applying a minimum number of documents of an author to one, whereof the 757 authors all met the selection criteria and threshold. For each of the 757 authors from the 215 publications, the total strength of the co-authorship links with other authors was also calculated, and the authors with the greatest total link of strength were selected.
- Co-occurrence analysis: the relationship and relatedness of all presented items and selection have a main determination of the number of documents and all related search terms and keywords in which they occur together.
In most of the presented analysis, the counting of the terms and keywords were presented as a full count and instead of fractional count analysis, where the full counting represents that each of the co-authorship or co-occurrence link has the same weight. In the fractional counting, we should understand the counting method as the weight of a link, where for instance if an author co-authors a document with 10 other authors, each of the 20-co-authorship links weigh 0.10 or 1/10.
The advanced criteria were also related to the actual data extraction from the Mendeley platform and upload into VOSViewer, in which some of the additional criteria for the data analysis were thoroughly applied. Some of those additional and final options were: (1) minimum occurrence of a keyword from the total of keywords; (2) total of the strength of the co-occurrence with other keywords calculations followed a pre-defined selection of the number of keywords to be selected for all of the presented analysis (3) weight of occurrences for an effective data visualisation; (4) analysis and validation of all of the selected cluster of analysed items from the VOSViewer algorithm; (5) usage of default values in association with the normalisation for data layout visualisation (6) merging of small clusters for an easy to understand visualisation of all the presented figures in this paper.
In this section, the findings of the systematic review process are presented. All presented results had as the main basis the bibliometric indicators from the 215 selected articles. As explained in the previous point, the whereof regarding the statistical analysis allowed us to better understand the results with concrete data and measurable indicators from the scientific network of all of the co-authorship analysis as well as of the co-occurrence analysis.
Publication year
Figure Figure3 3 visualises the distribution of all 215 items since 2010 and until today. From the total number of the studied items, 88 were from the current year, showing a clear reflection of the importance of the research question to the entire community and research world.

distribution per year.
Since 2015, a growing trend of the number of publications related to the selected keywords can be observed, while in the last 3 years the growth appears as exponential.
Co-authorship data analysis
VosViewer is used by the academic community to create co-authorship, keyword co-occurences, citations, bibliographic coupling or co-citation maps based on bibliographic data. In this section, we will be presenting co-authorship analysis based on network graphs where the association strength network has been applied while constructing the network graph.
This type of analysis presents the relatedness of all selected authors and co-authors and was determined based on their number of documents and networks of relationships. In this paper and terms of the advanced search criteria, the type of analysis selected for the co-authorship followed the unit of analysis of authors with a full counting method and applying a minimum number of documents of an author to one, whereof the 757 authors all met the selection criteria and threshold. For each of the 757 authors from the 215 publications, the total strength of the co-authorship links with other authors was also calculated, and the authors with the greatest total link of strength were selected.
The strength of a link may, for example, indicate the number of cited references two publications have in common, the number of publications two researchers have co-authored, or the number of publications in which two terms occur together. For example, in the case of co-authorship links between researchers and authors, the links attribute indicates the number of co-authorship links of a given author, researcher, or publication with others. Therefore the Total link strength attribute indicates the total strength of the co-authorship links of a given researcher with other researchers.
From the previously explained overview of the database search and selection criteria, the final number of the verified selected authors from the 215 items was 757. As some of the 757 authors in the selected network were not connected as should also be expected, the largest set of connected authors consisted of 15 authors that showed connections between all of them. For the benefit of our readers, we also kept the analysis of all sets of networks and showing the different amounts of clusters from the selected analysis. While running the layout algorithm, we understood that from all 757 authors or full datasets, we needed to make 209 clusters from all the different connections of authors. As explained in the above paragraph, only 15 authors from the 757 had connections between them, and as we can see in fig. 5 . For each of the authors from the 215 publications, the total strength of the co-authorship links with other authors was also calculated, and the authors with the greatest total link of strength were selected. Figs. 4 and and5 5 report the results of our analysis in terms of coauthorships and networks.

a high-level overview of all co-authorship relationship networks from the 575 verified authors.

Fifteen authors with a relationship
Co-occurrence of terms and keywords - data analysis
The type of analysis for the co-occurrence had as the main basis the keywords as the unit of analysis and the full counting as the selected method. From the 569 keywords, only 379 of the keywords were part of the largest set of the connected items, according to Fig. 6 .

The largest set of the connected items. The set of color range overlay was not normalised and as we can see from the different colored scheme and legend
The mapping technique from the VOSViewer software of the keywords allowed us to understand that Big Data, continuous medical education, MDs, have a close relationship with a high number of incidence and good relationship quality.
Figure Figure7 7 is also part of the co-occurrence analysis. 53 keywords present a concrete strength of the co-occurrence links with other keywords and allowing us to better understand the total link strength from all 569 keywords and where the minimum of occurrences were 2 keywords as the threshold requirement for the analysis. From the verified selected keywords, the ones with more occurrences were big data ( n = 30, total link of strength = 47), healthcare ( n = 17, total link of strength = 36), and machine learning ( n = 14, total link of strength = 20).

Minimum of occurrences were 2 keywords as the threshold requirement for the analysis
From the current literature, we can also conclude that the connections and linkages between big data, continuous medical education, and medical doctors’ learning and training are not clear.
Model proposal for MDs’ learning based on big data research in health
In trying to address our research question: “ Which are the main dimensions of a model proposal for increasing the potential of Big Data research in Healthcare for MDs’ learning?,” we tried to develop a learning model proposal based on Big Data research in healthcare for MDs. The prosed model is supported by the results of the systematic literature review. It includes the Big Data key-areas in health identified in our research as being fundamental for MDs’ learning processes: data transformation; health intelligence, data leveraging, and decision-making; associated to each one of these key-areas emerge the learning categories as presented in Table Table2 2 .
Model Proposal for MDs’ Learning based on Big Data key-areas
Each of the model dimensions allows MDs to develop new skills and competencies to address better the challenges posed by the modern healthcare ecosystem [ 34 , 53 ], that needs to review its global strategies because of the technological shifts.
The first dimension is connected to data transformation. Four learning categories have been identified. Health information services allow MDs to use the new technologies in assessing the patients’ data and history, including lab results, X-rays, clinical information, demographic information, and notes. The automatic management of such data can offer immediate insights and practical working support to MDs. Still, the creation and consultation of such databases may not look immediate, if competencies are not empowered. New health applications may allow possibilities for MDs and patients to manage their relations in a different way. The Covid-19 pandemic has boosted the interest towards e-health and remote visits, to cope with the enforced social distancing measures and the hospital disruptions worldwide [ 54 – 56 ]. Again, such powerful tools and technologies must be understood and properly used by both MDs and patients. A co-learning approach is highly recommended to make the best possible use of the modern solutions available to all the stakeholders involved. Medical images represent today a frontier approach to diagnosis and treatment, including surgery [ 14 , 57 ]. Again, the understanding of medical images requires dedicated training. Last but not least, AI represents one of the most promising technologies for healthcare applications, able to disrupt the system in terms of diagnosis, treatment, and follow-ups of several clinical disciplines, including oncology, radiology, and surgery [ 28 , 31 , 58 ]. Still, AI and its related technologies like machine learning and deep learning are not included in medical curricula, both undergraduate or postgraduate.
The second dimension concerns health intelligence, which includes the learning regarding epidemic outbreak forecasting, drug discovery, big data analytics to predict diseases, and genome data. The man sources of data are health systems, communities, media and numerous other informal outlets gather epidemiological and other data to predict diseases and to discover new treatments and drugs. The goal is to learn how to gather and to make the interpretation, review, processing and generation of useful information for public health professionals, clinicians, decision-makers and policymakers to achieve a higher level of knowledge and define the appropriate health strategies [ 59 – 61 ].
The third dimension, data leveraging regards the learning about patient information, clinical records, operational data, and public health data, covering information specific to patients, such as diagnosis notes, doctor’s prescription, medical photographs, pharmacy records, most of which are supported in research data. This process of learning can also help to improve the decision-making process, including disease diagnosis, real-time monitoring patient methodologies, methods to improve treatments and patient-centred care. It can also be a framework for enhancing healthcare efficiency and productivity using big data analytics, reflecting the development of new clinical models to face problems such as how to determine the most appropriate disease treatment or which medication is the best to apply to a particular case.
A structured learning model can help to improve the quality of care, to avoid risk factors in the early diagnosis of diseases and to better manage the hospital information system. Healthcare big data helps to define emerging sources of data such as social media platforms, connected devices, and others, where MDs can study new medical situation or find a solution already discovered by other colleagues and accessible by technology which links several sources including patient medical history, data from diagnostic and clinical trials, and index of drug efficacy. It offers a valuable source of knowledge for MDs to achieve innovative healthcare solutions when the mixture of these data sources and analytics are combined together.
The main areas of learning for MDs are researched in a very significant way and are present in scientific databases, where the most recent studies are stored and constitute a knowledge bank to develop specific competencies. The scientific databases are repositories of big data in healthcare and they can be used to support the medical industry helping to provide for better health services based on cutting-edge knowledge. In this regard, the proposed model can help the MDs to develop specific and updated skills and competencies and to be up-to-date regarding health knowledge.
Discussions and conclusions
The current paper has sought to specify the key dimensions to propose a model for strengthening the likely adaptation of healthcare big data research for medical education and MDs’ learning and training. In doing so, this study employed a methodology involving the conducting of a rigorous systematic bibliometric literature analysis and review with large-scale data research within the healthcare setting (i.e., medical, clinical, or biology-related activities or processes). More specifically, the present study conducted two types of bibliometric analysis on the associated literature, namely co-authorship analysis and co-occurrence analysis. The collected data involved 215 peer-reviewed articles published in the PubMed and Google Scholar databases during the period from 2010 to 2020. VOSviewer software was used to construct and view bibliometric maps on the subject of this study.
Overall, it is evident that this area of research has experienced a considerable expansion in popularity in the last three years (from 2018 to 2020). According to co-authorship relationships network analysis, out of 757 researchers, there are only fifteen authors who had collaborations on some joint research in this field. With respect to keyword co-occurrence analysis, the results indicated that there are 379 keywords, out of 569 keywords, that were part of the greatest collection of the associated items. In conclusion, it is apparent that, based on the selected and analysed data, no obvious associations have been substantially shown between healthcare big data and continuous medical education, learning and training.
This study has several contributions and implications for practice. Due to the increasing significance of big data among different contexts, studying, systematically reviewing and critically analysing large-scale data are attracting scholars’ attention from various disciplines, including in the healthcare field. In this vein, a number of literature reviews on healthcare big data have been published. However, this fundamental work expands prior literature sources on healthcare big data by presenting novel insights and guides for scholars and healthcare stakeholders around the world to support the further production of work in this area of research, along with its implementation among medical education and MDs’ learning and training. The mapping technique via VOSViewer software of the keywords allowed us to understand that Big Data, continuous medical education, MDs, have a good and close relationship. In addition, this paper adds to the existing body of knowledge concerning bibliometric studies on large-scale data in the healthcare context. Although there are some bibliometric studies that reviewed and analysed the previous publications in healthcare, learning and training aspects were not really made clear, hence this study provides valuable findings related to the potential of healthcare Big Data for MDs’ learning and training: 1) data transformation is related to the learning that occurs through medical systems; 2) health intelligence includes the learning regarding health innovation based on predictions and forecasting processes; 3) data leveraging regards the learning about patient information; and 4) the learning process is related to the decision-making process, focused on disease diagnosis and methods to improve treatments. The above can facilitate a deeper understanding of the debates in this field as well as contributing to the existing literature by further mapping the connections among the retrieved publications within this domain. Furthermore, this research objectively and analytically outlined the selected literature with respect to healthcare large-scale data in order to present a crucial and valuable source for concerned scholars to better understand the overall evolution and trends of this area of research. The obvious implication of the existing study is that the systematic bibliometric literature review analysis of healthcare large-scale data by different computational methods can be practiced to obtain significant interpretations and outlines for improving the quality of medical education, learning and training.
Moreover, this paper sought to determine the missing gaps and links between studying and analysing big data processes, technology, and procedures and ongoing MDs’ learning and training within the healthcare sector including hospitals and clinics. As a result, the findings of this research provide a clear overview of the current status on the effective utilisation of healthcare big data for continuing medical education and MDs’ learning and training. In addition, the findings of this work can produce outstanding academic and practical lines and paths for healthcare stakeholders and professionals, including MDs, to be quick to pick up new information and technical experience and to be eager to learn from this large-scale of data regarding healthcare. This will result in improving their knowledge, professional skills, decision-making ability, and personal qualifications in particular and supporting the healthcare sector they are involved in, in general.
Additionally, one particularly interesting fact highlighted by the results of co-authorship analysis is that collaborations between scholars are limited amongst this area of research. To this end, this paper contributes to knowledge in the healthcare setting by addressing the substantial lack and absence of a cooperative research community and considerable collaboration among authors. Thus, this paper provides explicit insights and directions to effectively enhance the collaboration between researchers from different institutions and countries. This will aid in presenting more valuable cooperation and comprehensive sources regarding healthcare big data research and utilising them for medical education and MDs’ learning and training.
Finally, and in sum, professionals need a constantly updated curriculum, they need to have a focus on inter-professional learning, and the entry-level healthcare curriculum must introduce to all employees the concept of lifelong learning – as continuing education and skills development in Big Data is often forgotten.
Limitations and future research
Even though this study provides several useful insights, a number of limitations have to be acknowledged, leading to further research avenues and opportunities. First, the current paper has conducted two patterns of bibliometric analyses which are: co-authorship analysis and co-occurrence. Thus, future studies are suggested to employ different series and metrics of bibliometric analyses on large-scale data research within the healthcare setting, including, prolific authors, journals’ production trends, citation and H-index analysis, distribution of the most productive countries and institutions, countries’ and/or institutions’ co-authorship analysis and co-citation analysis on the published work in the topic. Additionally, future research is recommended to analyse the research methods, data collection and analysis procedures applied in previous studies of this domain. Additional in-depth analysis may be conducted, even employing other types of literature reviews such as more structured ones [ 62 ].
This paper employed VOSviewer software to construct and visualise bibliometric networks on the topic. Further studies can therefore adopt other software programs such as CiteSpace, BibExcel, Eigenfactor Score, R package, among others, which are common and efficient software programs employed to gain quantitative and visual information and mapping in a certain domain.
Furthermore, the focus of this study was on peer-reviewed articles published in the PubMed and Google Scholar digital databases, thus focusing on more clinical-related journals or publications. Future studies may concentrate on more sources published in other databases such as Elsevier’s Scopus and/or Web of Science (SCIE, SSCI, and/or ESCI), as these datasets include journals, reviews, and books of other scientific fields, which may create synergies on the topic, like Social Sciences, Business and Management, Engineering, or Education. As recent studies have reported, practical and innovative inputs and solutions about healthcare-related matters may be gathered and applied from other domains, especially Business and Management [ 41 ].
In addition, future research may also expand to other non-peer-reviewed publications, including books and book chapters, proceedings papers, reports, guidelines, book reviews, among others to provide a broader overview. Such sources may report pioneering and promising early-stage ideas and concepts, which may not yet be published in ranked journals. Relevant publications written in other languages, other than English, may also be targeted in future work.
This study was funded by Fundação para a Ciência e Tecnologia, Grant: UIDB/00315/2020.
Compliance with ethical standards
The authors declare that they have no conflict of interest.
All procedures performed in studies involving human participants were in accordance with the ethical standards of the institutional and/or national research committee and with the 1964 Helsinki declaration and its later amendments or comparable ethical standards.
Informed consent was obtained from all individual participants included in the study.
This article is part of the Topical Collection on Education & Training
Publisher’s Note
Springer Nature remains neutral with regard to jurisdictional claims in published maps and institutional affiliations.
Contributor Information
Manuel Au-Yong-Oliveira, Email: tp.au@oam .
Antonio Pesqueira, Email: [email protected] .
Maria José Sousa, Email: [email protected] .
Francesca Dal Mas, Email: ku.ca.nlocnil@samladf .
Mohammad Soliman, Email: [email protected] .
Read our research on: Gun Policy | International Conflict | Election 2024
Regions & Countries
9 facts about americans and marijuana.
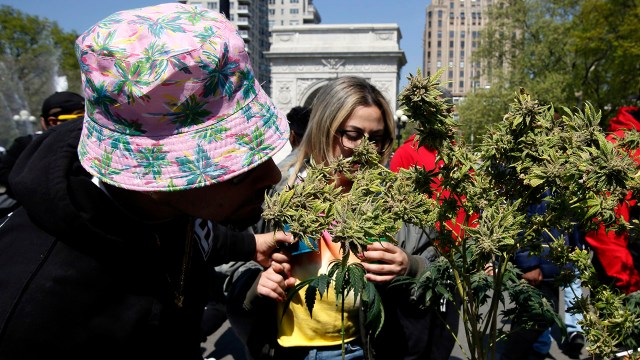
The use and possession of marijuana is illegal under U.S. federal law, but about three-quarters of states have legalized the drug for medical or recreational purposes. The changing legal landscape has coincided with a decades-long rise in public support for legalization, which a majority of Americans now favor.
Here are nine facts about Americans’ views of and experiences with marijuana, based on Pew Research Center surveys and other sources.
As more states legalize marijuana, Pew Research Center looked at Americans’ opinions on legalization and how these views have changed over time.
Data comes from surveys by the Center, Gallup , and the 2022 National Survey on Drug Use and Health from the U.S. Substance Abuse and Mental Health Services Administration. Information about the jurisdictions where marijuana is legal at the state level comes from the National Organization for the Reform of Marijuana Laws .
More information about the Center surveys cited in the analysis, including the questions asked and their methodologies, can be found at the links in the text.
Around nine-in-ten Americans say marijuana should be legal for medical or recreational use, according to a January 2024 Pew Research Center survey . An overwhelming majority of U.S. adults (88%) say either that marijuana should be legal for medical use only (32%) or that it should be legal for medical and recreational use (57%). Just 11% say the drug should not be legal in any form. These views have held relatively steady over the past five years.
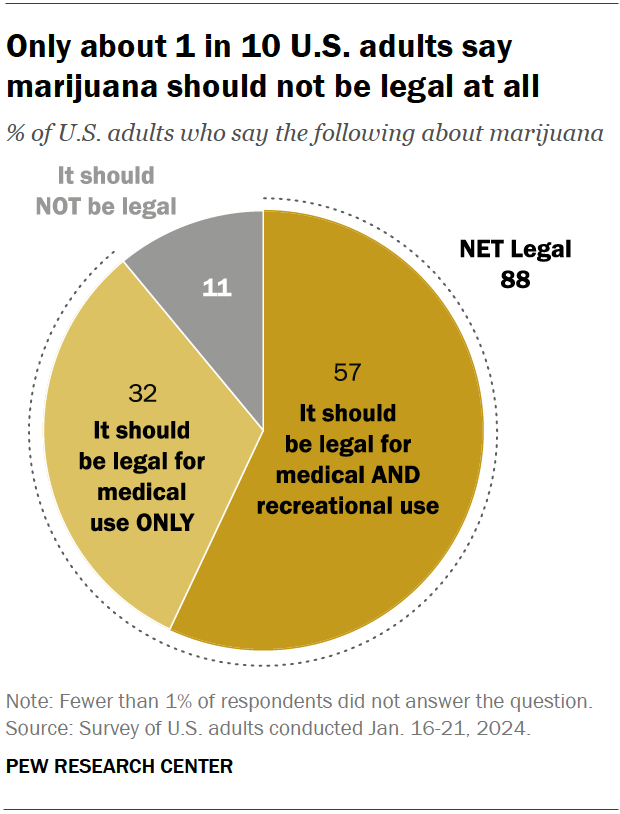
Views on marijuana legalization differ widely by age, political party, and race and ethnicity, the January survey shows.
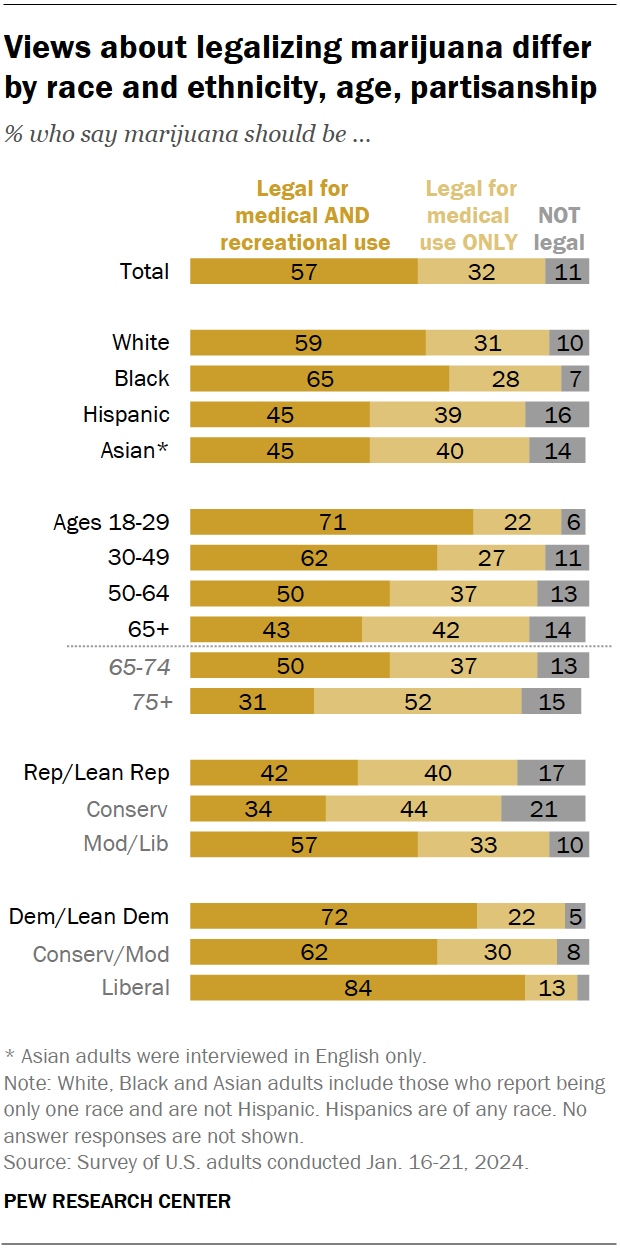
While small shares across demographic groups say marijuana should not be legal at all, those least likely to favor it for both medical and recreational use include:
- Older adults: 31% of adults ages 75 and older support marijuana legalization for medical and recreational purposes, compared with half of those ages 65 to 74, the next youngest age category. By contrast, 71% of adults under 30 support legalization for both uses.
- Republicans and GOP-leaning independents: 42% of Republicans favor legalizing marijuana for both uses, compared with 72% of Democrats and Democratic leaners. Ideological differences exist as well: Within both parties, those who are more conservative are less likely to support legalization.
- Hispanic and Asian Americans: 45% in each group support legalizing the drug for medical and recreational use. Larger shares of Black (65%) and White (59%) adults hold this view.
Support for marijuana legalization has increased dramatically over the last two decades. In addition to asking specifically about medical and recreational use of the drug, both the Center and Gallup have asked Americans about legalizing marijuana use in a general way. Gallup asked this question most recently, in 2023. That year, 70% of adults expressed support for legalization, more than double the share who said they favored it in 2000.
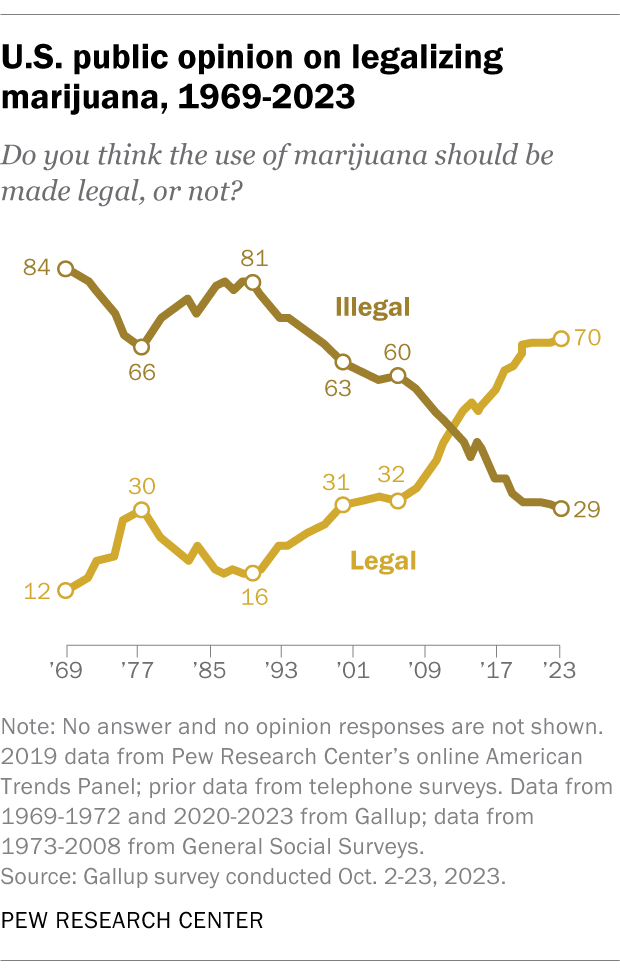
Half of U.S. adults (50.3%) say they have ever used marijuana, according to the 2022 National Survey on Drug Use and Health . That is a smaller share than the 84.1% who say they have ever consumed alcohol and the 64.8% who have ever used tobacco products or vaped nicotine.
While many Americans say they have used marijuana in their lifetime, far fewer are current users, according to the same survey. In 2022, 23.0% of adults said they had used the drug in the past year, while 15.9% said they had used it in the past month.
While many Americans say legalizing recreational marijuana has economic and criminal justice benefits, views on these and other impacts vary, the Center’s January survey shows.
- Economic benefits: About half of adults (52%) say that legalizing recreational marijuana is good for local economies, while 17% say it is bad. Another 29% say it has no impact.
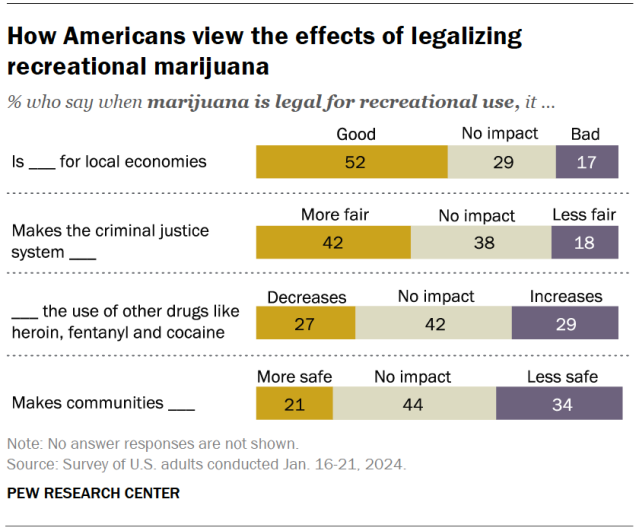
- Criminal justice system fairness: 42% of Americans say legalizing marijuana for recreational use makes the criminal justice system fairer, compared with 18% who say it makes the system less fair. About four-in-ten (38%) say it has no impact.
- Use of other drugs: 27% say this policy decreases the use of other drugs like heroin, fentanyl and cocaine, and 29% say it increases it. But the largest share (42%) say it has no effect on other drug use.
- Community safety: 21% say recreational legalization makes communities safer and 34% say it makes them less safe. Another 44% say it doesn’t impact safety.
Democrats and adults under 50 are more likely than Republicans and those in older age groups to say legalizing marijuana has positive impacts in each of these areas.
Most Americans support easing penalties for people with marijuana convictions, an October 2021 Center survey found . Two-thirds of adults say they favor releasing people from prison who are being held for marijuana-related offenses only, including 41% who strongly favor this. And 61% support removing or expunging marijuana-related offenses from people’s criminal records.
Younger adults, Democrats and Black Americans are especially likely to support these changes. For instance, 74% of Black adults favor releasing people from prison who are being held only for marijuana-related offenses, and just as many favor removing or expunging marijuana-related offenses from criminal records.
Twenty-four states and the District of Columbia have legalized small amounts of marijuana for both medical and recreational use as of March 2024, according to the National Organization for the Reform of Marijuana Laws (NORML), an advocacy group that tracks state-level legislation on the issue. Another 14 states have legalized the drug for medical use only.

Of the remaining 12 states, all allow limited access to products such as CBD oil that contain little to no THC – the main psychoactive substance in cannabis. And 26 states overall have at least partially decriminalized recreational marijuana use , as has the District of Columbia.
In addition to 24 states and D.C., the U.S. Virgin Islands , Guam and the Northern Mariana Islands have legalized marijuana for medical and recreational use.
More than half of Americans (54%) live in a state where both recreational and medical marijuana are legal, and 74% live in a state where it’s legal either for both purposes or medical use only, according to a February Center analysis of data from the Census Bureau and other outside sources. This analysis looked at state-level legislation in all 50 states and the District of Columbia.
In 2012, Colorado and Washington became the first states to pass legislation legalizing recreational marijuana.
About eight-in-ten Americans (79%) live in a county with at least one cannabis dispensary, according to the February analysis. There are nearly 15,000 marijuana dispensaries nationwide, and 76% are in states (including D.C.) where recreational use is legal. Another 23% are in medical marijuana-only states, and 1% are in states that have made legal allowances for low-percentage THC or CBD-only products.
The states with the largest number of dispensaries include California, Oklahoma, Florida, Colorado and Michigan.
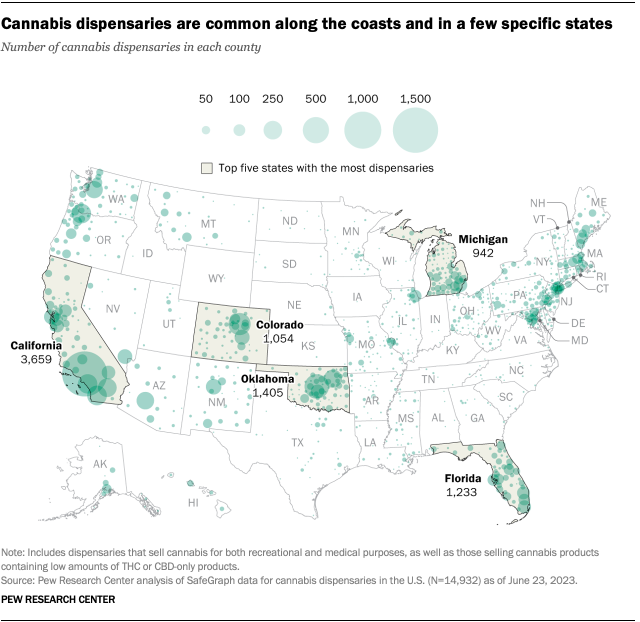
Note: This is an update of a post originally published April 26, 2021, and updated April 13, 2023.
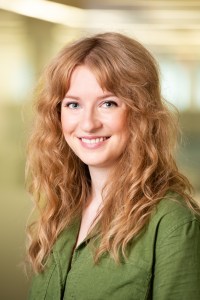
Sign up for our weekly newsletter
Fresh data delivered Saturday mornings
Americans overwhelmingly say marijuana should be legal for medical or recreational use
Religious americans are less likely to endorse legal marijuana for recreational use, four-in-ten u.s. drug arrests in 2018 were for marijuana offenses – mostly possession, two-thirds of americans support marijuana legalization, most popular.
About Pew Research Center Pew Research Center is a nonpartisan fact tank that informs the public about the issues, attitudes and trends shaping the world. It conducts public opinion polling, demographic research, media content analysis and other empirical social science research. Pew Research Center does not take policy positions. It is a subsidiary of The Pew Charitable Trusts .
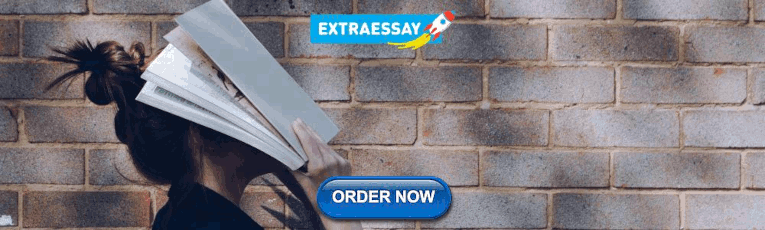
IMAGES
VIDEO
COMMENTS
Here, we'll explore a variety of healthcare-related research ideas and topic thought-starters across a range of healthcare fields, including allopathic and alternative medicine, dentistry, physical therapy, optometry, pharmacology and public health. NB - This is just the start….
Since 2020, COVID-19 has been a hot-button topic in medicine, along with the long-term symptoms in those with a history of COVID-19. Examples of COVID-19-related research topics worth exploring include: The long-term impact of COVID-19 on cardiac and respiratory health. COVID-19 vaccination rates.
Introduction. Particularly since the last decade, IT has opened up new opportunities for 'ehealth' through telemedicine and remote patient monitoring, alongside potential improvements in the cost-effectiveness and accessibility of health care (Chiasson & Davidson, 2004).Accordingly, health information systems (HIS) research has come to focus on how healthcare organizations invest in and ...
Artificial intelligence (AI) is a powerful and disruptive area of computer science, with the potential to fundamentally transform the practice of medicine and the delivery of healthcare. In this review article, we outline recent breakthroughs in the application of AI in healthcare, describe a roadmap to building effective, reliable and safe AI ...
100 Healthcare Research Paper Topics. The field of healthcare research encompasses a vast array of topics that are crucial for understanding, improving, and transforming healthcare practices. As students in the health sciences, you have the opportunity to explore these diverse areas and contribute to the knowledge base of healthcare research.
The literature on healthcare operations and supply chain management has seen unprecedented growth over the past two decades. This paper seeks to advance the body of knowledge on this topic by utilising a topic modelling-based literature review to identify the core topics, examine their dynamic changes, and identify opportunities for further research in the area.
Background Accelerated by the coronavirus disease 2019 (Covid-19) pandemic, major and lasting changes are occuring in healthcare structures, impacting people's experiences and value creation in all aspects of their lives. Information systems (IS) research can support analysing and anticipating resulting effects. Aim The purpose of this study is to examine in what areas health information ...
This paper evaluated AI in healthcare research streams using the SLR method [].As suggested by Massaro et al. [], an SLR enables the study of the scientific corpus of a research field, including the scientific rigour, reliability and replicability of operations carried out by researchers.As suggested by many scholars, the methodology allows qualitative and quantitative variables to highlight ...
The literature on healthcare operations and supply chain management has seen unprecedented growth over the past two decades. This paper seeks to advance the body of knowledge on this topic by utilising a topic modelling-based literature review to identify the core topics, examine their dynamic changes, and identify opportunities for further research in the area. Based on an analysis of 571 ...
Preprints.org. is a multidiscipline platform providing preprint service that is dedicated to sharing your research from the start and empowering your research journey.. MDPI Topics is cooperating with Preprints.org and has built a direct connection between MDPI journals and Preprints.org.Authors are encouraged to enjoy the benefits by posting a preprint at Preprints.org prior to publication:
Abstract. The emergence of quality as a driving force in healthcare now requires innovative management. techniques such as project management (PM) to attain quality management outcomes. This ...
151+ Public Health Research Topics [Updated 2024] The important area of public health research is essential to forming laws, influencing medical procedures, and eventually enhancing community well-being. As we delve into the vast landscape of public health research topics, it's essential to understand the profound impact they have on society.
by Kasandra Brabaw. One in 10 people in America lack health insurance, resulting in $40 billion of care that goes unpaid each year. Amitabh Chandra and colleagues say ensuring basic coverage for all residents, as other wealthy nations do, could address the most acute needs and unlock efficiency. 13 Mar 2023.
Most interestingly, researchers in the biomedical fields have been actively trying to apply AI to help improve analysis and treatment outcomes and, consequently, increase the efficacy of the overall healthcare industry [35], [36], [37]. Fig. 1 shows the number of publications in this area in the last 20 years, from 1999 to 2018. The growth of ...
Topics in Health Affairs journal, Forefront, briefs and other content cover a wide swath of health policy matters and health services research. Fields range from economics to political science to ...
Dermatology Research Topics. Find the most relevant research topic for your dermatology research paper from our many examples. Atopic dermatitis. Contact dermatitis. Epidemiology behind uncommon skin disorders. The process of aging is akin to a cutaneous one. Melanoma risk factors for skin cancer.
The healthcare market has been changing rapidly since the new millennium, creating a need for a new, integrated perspective on consumer relevant healthcare topics through the lens of psychology, marketing, and economics (Wood 2018; Iacobucci 2019).Even though marketing and consumer researchers with both quantitative and qualitative orientations have recently joined forces to tackle these ...
The purpose of this paper is to systematically review the literature published on the various aspects of service quality in the healthcare industry. The study is based on secondary information ...
The fastest growth in healthcare may occur in several segments. We estimate that healthcare profit pools will grow at a 7 percent CAGR, from $583 billion in 2022 to $819 billion in 2027. Profit pools continued under pressure in 2023 due to high inflation rates and labor shortages; however, we expect a recovery beginning in 2024, spurred by ...
Good Health Research Paper Topics. When you are writing a research paper on the healthcare sector, the possibilities are endless. It can be about anything from lifestyle to social issues to different syndromes. With constant improvement in the information sector as well as the technological one, the industry is witnessing a lot more innovative ...
VOSviewer represents an evidence-based technique that uses a reliable and structured method to perform the identification, analysis, and interpretation of several relevant academic papers related to the selected topic. The topic herein is Big Data research in the healthcare context and applied to MDs' learning and training . On the VOSviewer ...
Abstract. The purpose of this this paper is to review multiple research papers related to the topic 'Cybersecurity Threats and Attacks in Health care'. A total of 9 papers were shortlisted for a ...
While many Americans say they have used marijuana in their lifetime, far fewer are current users, according to the same survey. In 2022, 23.0% of adults said they had used the drug in the past year, while 15.9% said they had used it in the past month. While many Americans say legalizing recreational marijuana has economic and criminal justice ...
Abstract. A well-functioning health-care system can make a considerable contribution to a country's economic growth, development, and industrialization. Health care is traditionally thought to be ...