Qualitative case study data analysis: an example from practice
Affiliation.
- 1 School of Nursing and Midwifery, National University of Ireland, Galway, Republic of Ireland.
- PMID: 25976531
- DOI: 10.7748/nr.22.5.8.e1307
Aim: To illustrate an approach to data analysis in qualitative case study methodology.
Background: There is often little detail in case study research about how data were analysed. However, it is important that comprehensive analysis procedures are used because there are often large sets of data from multiple sources of evidence. Furthermore, the ability to describe in detail how the analysis was conducted ensures rigour in reporting qualitative research.
Data sources: The research example used is a multiple case study that explored the role of the clinical skills laboratory in preparing students for the real world of practice. Data analysis was conducted using a framework guided by the four stages of analysis outlined by Morse ( 1994 ): comprehending, synthesising, theorising and recontextualising. The specific strategies for analysis in these stages centred on the work of Miles and Huberman ( 1994 ), which has been successfully used in case study research. The data were managed using NVivo software.
Review methods: Literature examining qualitative data analysis was reviewed and strategies illustrated by the case study example provided. Discussion Each stage of the analysis framework is described with illustration from the research example for the purpose of highlighting the benefits of a systematic approach to handling large data sets from multiple sources.
Conclusion: By providing an example of how each stage of the analysis was conducted, it is hoped that researchers will be able to consider the benefits of such an approach to their own case study analysis.
Implications for research/practice: This paper illustrates specific strategies that can be employed when conducting data analysis in case study research and other qualitative research designs.
Keywords: Case study data analysis; case study research methodology; clinical skills research; qualitative case study methodology; qualitative data analysis; qualitative research.
- Case-Control Studies*
- Data Interpretation, Statistical*
- Nursing Research / methods*
- Qualitative Research*
- Research Design
- AI & NLP
- Churn & Loyalty
- Customer Experience
- Customer Journeys
- Customer Metrics
- Feedback Analysis
- Product Experience
- Product Updates
- Sentiment Analysis
- Surveys & Feedback Collection
- Try Thematic
Welcome to the community
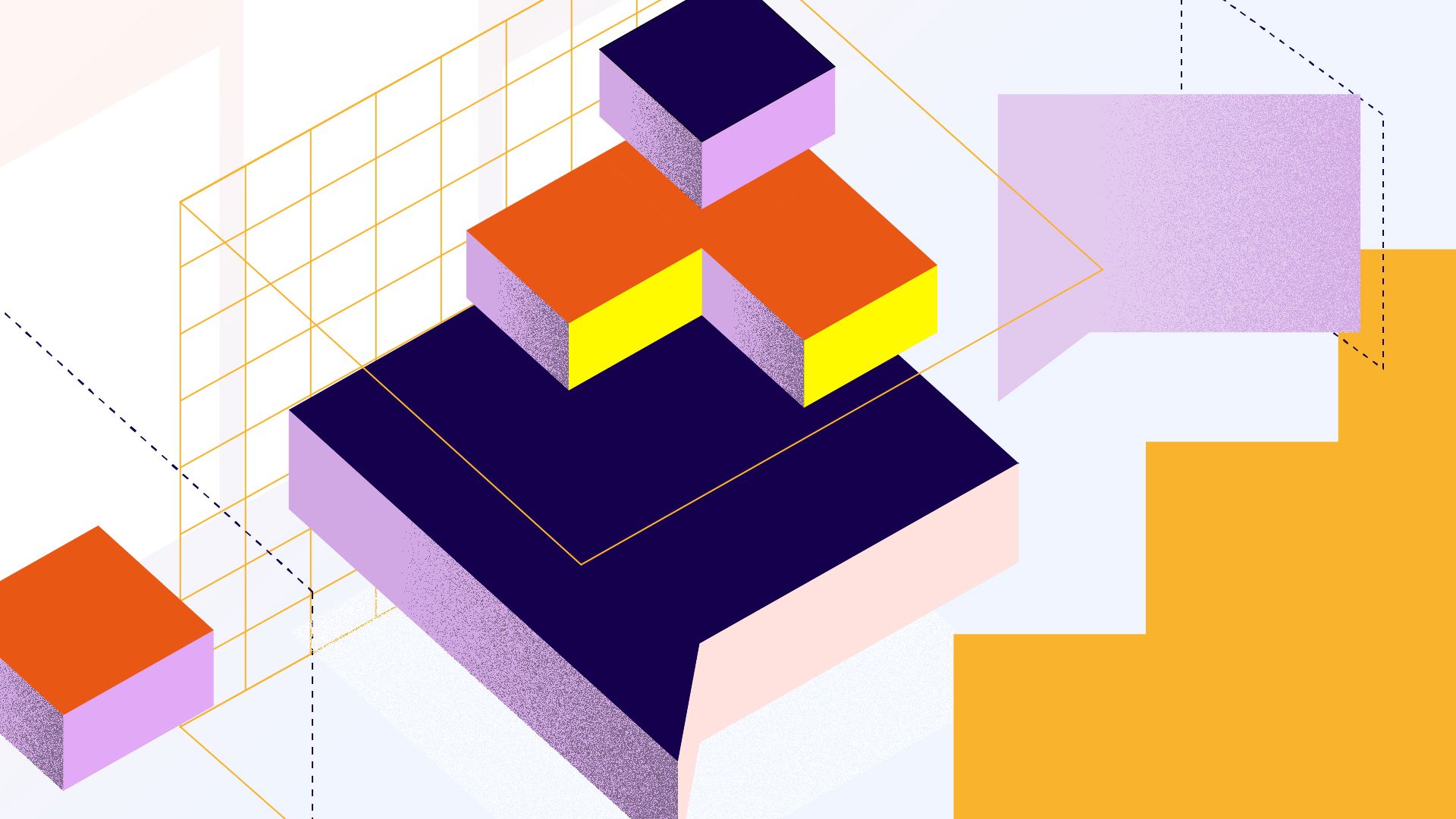
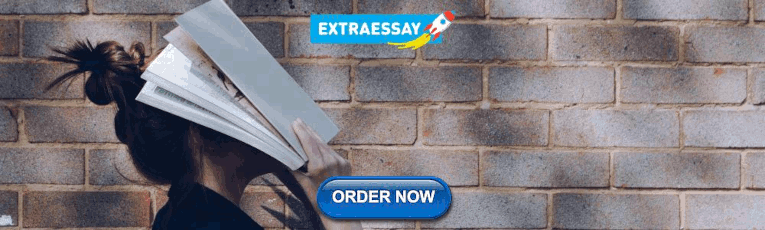
Qualitative Data Analysis: Step-by-Step Guide (Manual vs. Automatic)
When we conduct qualitative methods of research, need to explain changes in metrics or understand people's opinions, we always turn to qualitative data. Qualitative data is typically generated through:
- Interview transcripts
- Surveys with open-ended questions
- Contact center transcripts
- Texts and documents
- Audio and video recordings
- Observational notes
Compared to quantitative data, which captures structured information, qualitative data is unstructured and has more depth. It can answer our questions, can help formulate hypotheses and build understanding.
It's important to understand the differences between quantitative data & qualitative data . But unfortunately, analyzing qualitative data is difficult. While tools like Excel, Tableau and PowerBI crunch and visualize quantitative data with ease, there are a limited number of mainstream tools for analyzing qualitative data . The majority of qualitative data analysis still happens manually.
That said, there are two new trends that are changing this. First, there are advances in natural language processing (NLP) which is focused on understanding human language. Second, there is an explosion of user-friendly software designed for both researchers and businesses. Both help automate the qualitative data analysis process.
In this post we want to teach you how to conduct a successful qualitative data analysis. There are two primary qualitative data analysis methods; manual & automatic. We will teach you how to conduct the analysis manually, and also, automatically using software solutions powered by NLP. We’ll guide you through the steps to conduct a manual analysis, and look at what is involved and the role technology can play in automating this process.
More businesses are switching to fully-automated analysis of qualitative customer data because it is cheaper, faster, and just as accurate. Primarily, businesses purchase subscriptions to feedback analytics platforms so that they can understand customer pain points and sentiment.
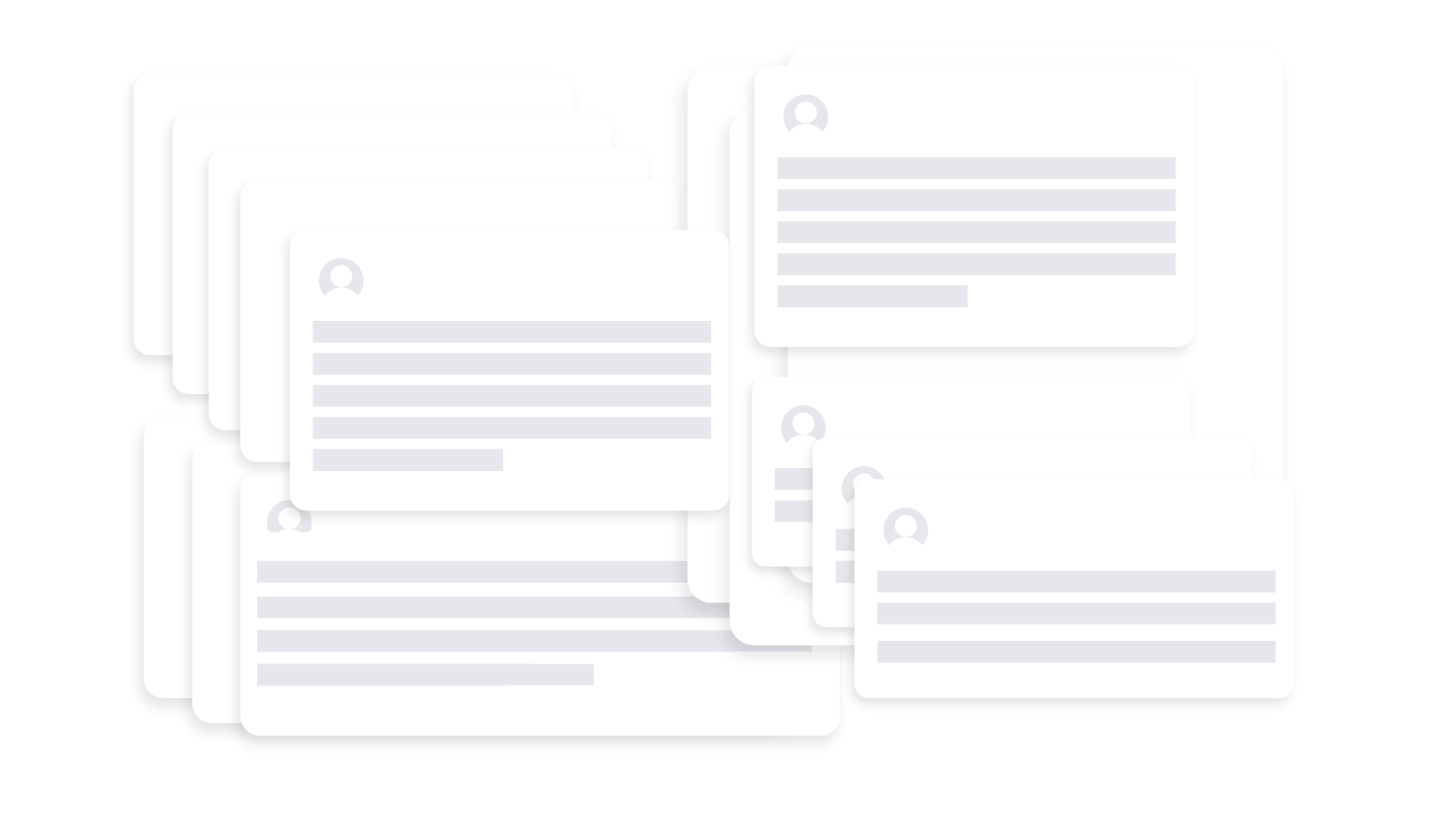
We’ll take you through 5 steps to conduct a successful qualitative data analysis. Within each step we will highlight the key difference between the manual, and automated approach of qualitative researchers. Here's an overview of the steps:
The 5 steps to doing qualitative data analysis
- Gathering and collecting your qualitative data
- Organizing and connecting into your qualitative data
- Coding your qualitative data
- Analyzing the qualitative data for insights
- Reporting on the insights derived from your analysis
What is Qualitative Data Analysis?
Qualitative data analysis is a process of gathering, structuring and interpreting qualitative data to understand what it represents.
Qualitative data is non-numerical and unstructured. Qualitative data generally refers to text, such as open-ended responses to survey questions or user interviews, but also includes audio, photos and video.
Businesses often perform qualitative data analysis on customer feedback. And within this context, qualitative data generally refers to verbatim text data collected from sources such as reviews, complaints, chat messages, support centre interactions, customer interviews, case notes or social media comments.
How is qualitative data analysis different from quantitative data analysis?
Understanding the differences between quantitative & qualitative data is important. When it comes to analyzing data, Qualitative Data Analysis serves a very different role to Quantitative Data Analysis. But what sets them apart?
Qualitative Data Analysis dives into the stories hidden in non-numerical data such as interviews, open-ended survey answers, or notes from observations. It uncovers the ‘whys’ and ‘hows’ giving a deep understanding of people’s experiences and emotions.
Quantitative Data Analysis on the other hand deals with numerical data, using statistics to measure differences, identify preferred options, and pinpoint root causes of issues. It steps back to address questions like "how many" or "what percentage" to offer broad insights we can apply to larger groups.
In short, Qualitative Data Analysis is like a microscope, helping us understand specific detail. Quantitative Data Analysis is like the telescope, giving us a broader perspective. Both are important, working together to decode data for different objectives.
Qualitative Data Analysis methods
Once all the data has been captured, there are a variety of analysis techniques available and the choice is determined by your specific research objectives and the kind of data you’ve gathered. Common qualitative data analysis methods include:
Content Analysis
This is a popular approach to qualitative data analysis. Other qualitative analysis techniques may fit within the broad scope of content analysis. Thematic analysis is a part of the content analysis. Content analysis is used to identify the patterns that emerge from text, by grouping content into words, concepts, and themes. Content analysis is useful to quantify the relationship between all of the grouped content. The Columbia School of Public Health has a detailed breakdown of content analysis .
Narrative Analysis
Narrative analysis focuses on the stories people tell and the language they use to make sense of them. It is particularly useful in qualitative research methods where customer stories are used to get a deep understanding of customers’ perspectives on a specific issue. A narrative analysis might enable us to summarize the outcomes of a focused case study.
Discourse Analysis
Discourse analysis is used to get a thorough understanding of the political, cultural and power dynamics that exist in specific situations. The focus of discourse analysis here is on the way people express themselves in different social contexts. Discourse analysis is commonly used by brand strategists who hope to understand why a group of people feel the way they do about a brand or product.
Thematic Analysis
Thematic analysis is used to deduce the meaning behind the words people use. This is accomplished by discovering repeating themes in text. These meaningful themes reveal key insights into data and can be quantified, particularly when paired with sentiment analysis . Often, the outcome of thematic analysis is a code frame that captures themes in terms of codes, also called categories. So the process of thematic analysis is also referred to as “coding”. A common use-case for thematic analysis in companies is analysis of customer feedback.
Grounded Theory
Grounded theory is a useful approach when little is known about a subject. Grounded theory starts by formulating a theory around a single data case. This means that the theory is “grounded”. Grounded theory analysis is based on actual data, and not entirely speculative. Then additional cases can be examined to see if they are relevant and can add to the original grounded theory.
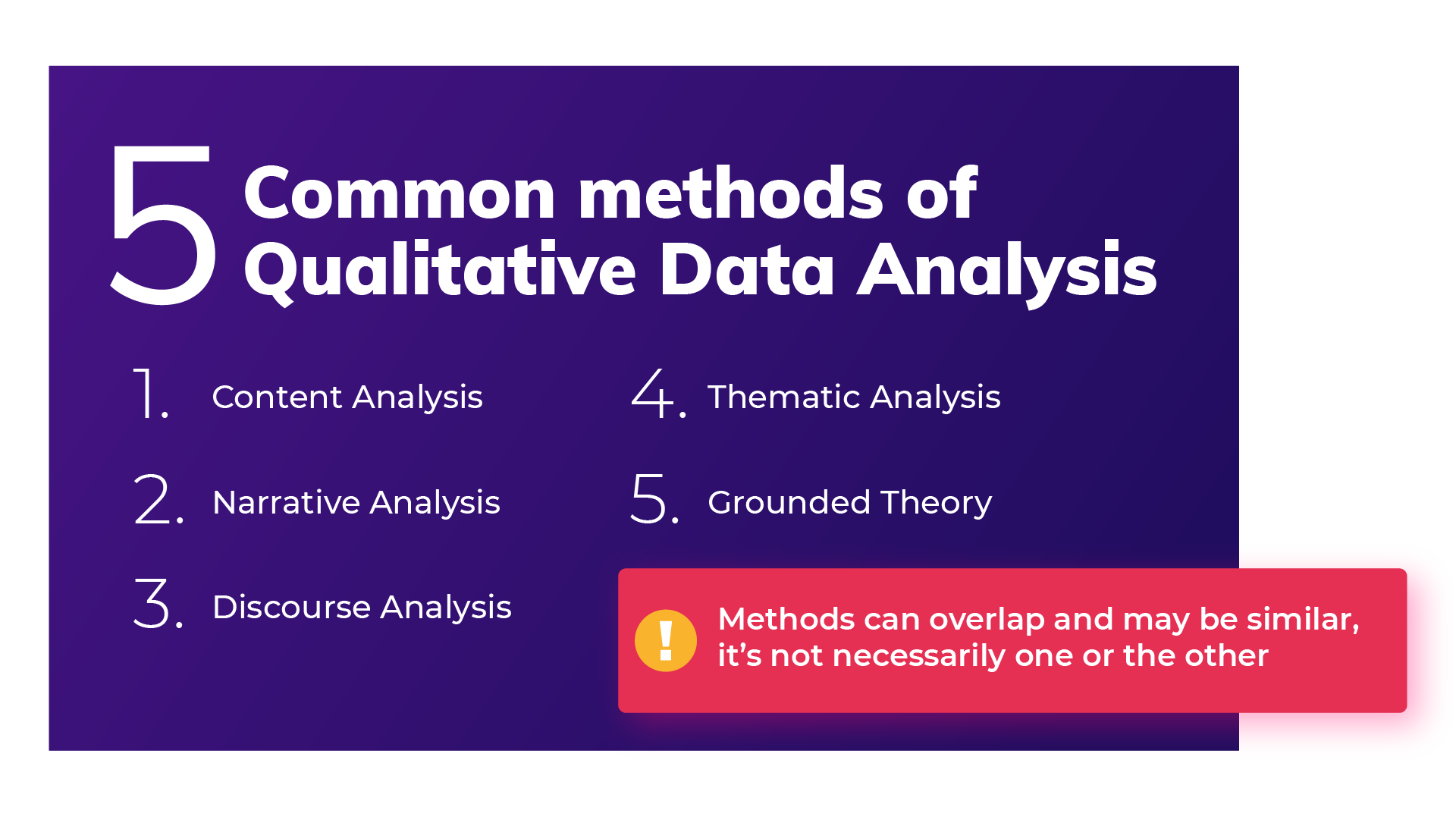
Challenges of Qualitative Data Analysis
While Qualitative Data Analysis offers rich insights, it comes with its challenges. Each unique QDA method has its unique hurdles. Let’s take a look at the challenges researchers and analysts might face, depending on the chosen method.
- Time and Effort (Narrative Analysis): Narrative analysis, which focuses on personal stories, demands patience. Sifting through lengthy narratives to find meaningful insights can be time-consuming, requires dedicated effort.
- Being Objective (Grounded Theory): Grounded theory, building theories from data, faces the challenges of personal biases. Staying objective while interpreting data is crucial, ensuring conclusions are rooted in the data itself.
- Complexity (Thematic Analysis): Thematic analysis involves identifying themes within data, a process that can be intricate. Categorizing and understanding themes can be complex, especially when each piece of data varies in context and structure. Thematic Analysis software can simplify this process.
- Generalizing Findings (Narrative Analysis): Narrative analysis, dealing with individual stories, makes drawing broad challenging. Extending findings from a single narrative to a broader context requires careful consideration.
- Managing Data (Thematic Analysis): Thematic analysis involves organizing and managing vast amounts of unstructured data, like interview transcripts. Managing this can be a hefty task, requiring effective data management strategies.
- Skill Level (Grounded Theory): Grounded theory demands specific skills to build theories from the ground up. Finding or training analysts with these skills poses a challenge, requiring investment in building expertise.
Benefits of qualitative data analysis
Qualitative Data Analysis (QDA) is like a versatile toolkit, offering a tailored approach to understanding your data. The benefits it offers are as diverse as the methods. Let’s explore why choosing the right method matters.
- Tailored Methods for Specific Needs: QDA isn't one-size-fits-all. Depending on your research objectives and the type of data at hand, different methods offer unique benefits. If you want emotive customer stories, narrative analysis paints a strong picture. When you want to explain a score, thematic analysis reveals insightful patterns
- Flexibility with Thematic Analysis: thematic analysis is like a chameleon in the toolkit of QDA. It adapts well to different types of data and research objectives, making it a top choice for any qualitative analysis.
- Deeper Understanding, Better Products: QDA helps you dive into people's thoughts and feelings. This deep understanding helps you build products and services that truly matches what people want, ensuring satisfied customers
- Finding the Unexpected: Qualitative data often reveals surprises that we miss in quantitative data. QDA offers us new ideas and perspectives, for insights we might otherwise miss.
- Building Effective Strategies: Insights from QDA are like strategic guides. They help businesses in crafting plans that match people’s desires.
- Creating Genuine Connections: Understanding people’s experiences lets businesses connect on a real level. This genuine connection helps build trust and loyalty, priceless for any business.
How to do Qualitative Data Analysis: 5 steps
Now we are going to show how you can do your own qualitative data analysis. We will guide you through this process step by step. As mentioned earlier, you will learn how to do qualitative data analysis manually , and also automatically using modern qualitative data and thematic analysis software.
To get best value from the analysis process and research process, it’s important to be super clear about the nature and scope of the question that’s being researched. This will help you select the research collection channels that are most likely to help you answer your question.
Depending on if you are a business looking to understand customer sentiment, or an academic surveying a school, your approach to qualitative data analysis will be unique.
Once you’re clear, there’s a sequence to follow. And, though there are differences in the manual and automatic approaches, the process steps are mostly the same.
The use case for our step-by-step guide is a company looking to collect data (customer feedback data), and analyze the customer feedback - in order to improve customer experience. By analyzing the customer feedback the company derives insights about their business and their customers. You can follow these same steps regardless of the nature of your research. Let’s get started.
Step 1: Gather your qualitative data and conduct research (Conduct qualitative research)
The first step of qualitative research is to do data collection. Put simply, data collection is gathering all of your data for analysis. A common situation is when qualitative data is spread across various sources.
Classic methods of gathering qualitative data
Most companies use traditional methods for gathering qualitative data: conducting interviews with research participants, running surveys, and running focus groups. This data is typically stored in documents, CRMs, databases and knowledge bases. It’s important to examine which data is available and needs to be included in your research project, based on its scope.
Using your existing qualitative feedback
As it becomes easier for customers to engage across a range of different channels, companies are gathering increasingly large amounts of both solicited and unsolicited qualitative feedback.
Most organizations have now invested in Voice of Customer programs , support ticketing systems, chatbot and support conversations, emails and even customer Slack chats.
These new channels provide companies with new ways of getting feedback, and also allow the collection of unstructured feedback data at scale.
The great thing about this data is that it contains a wealth of valubale insights and that it’s already there! When you have a new question about user behavior or your customers, you don’t need to create a new research study or set up a focus group. You can find most answers in the data you already have.
Typically, this data is stored in third-party solutions or a central database, but there are ways to export it or connect to a feedback analysis solution through integrations or an API.
Utilize untapped qualitative data channels
There are many online qualitative data sources you may not have considered. For example, you can find useful qualitative data in social media channels like Twitter or Facebook. Online forums, review sites, and online communities such as Discourse or Reddit also contain valuable data about your customers, or research questions.
If you are considering performing a qualitative benchmark analysis against competitors - the internet is your best friend. Gathering feedback in competitor reviews on sites like Trustpilot, G2, Capterra, Better Business Bureau or on app stores is a great way to perform a competitor benchmark analysis.
Customer feedback analysis software often has integrations into social media and review sites, or you could use a solution like DataMiner to scrape the reviews.
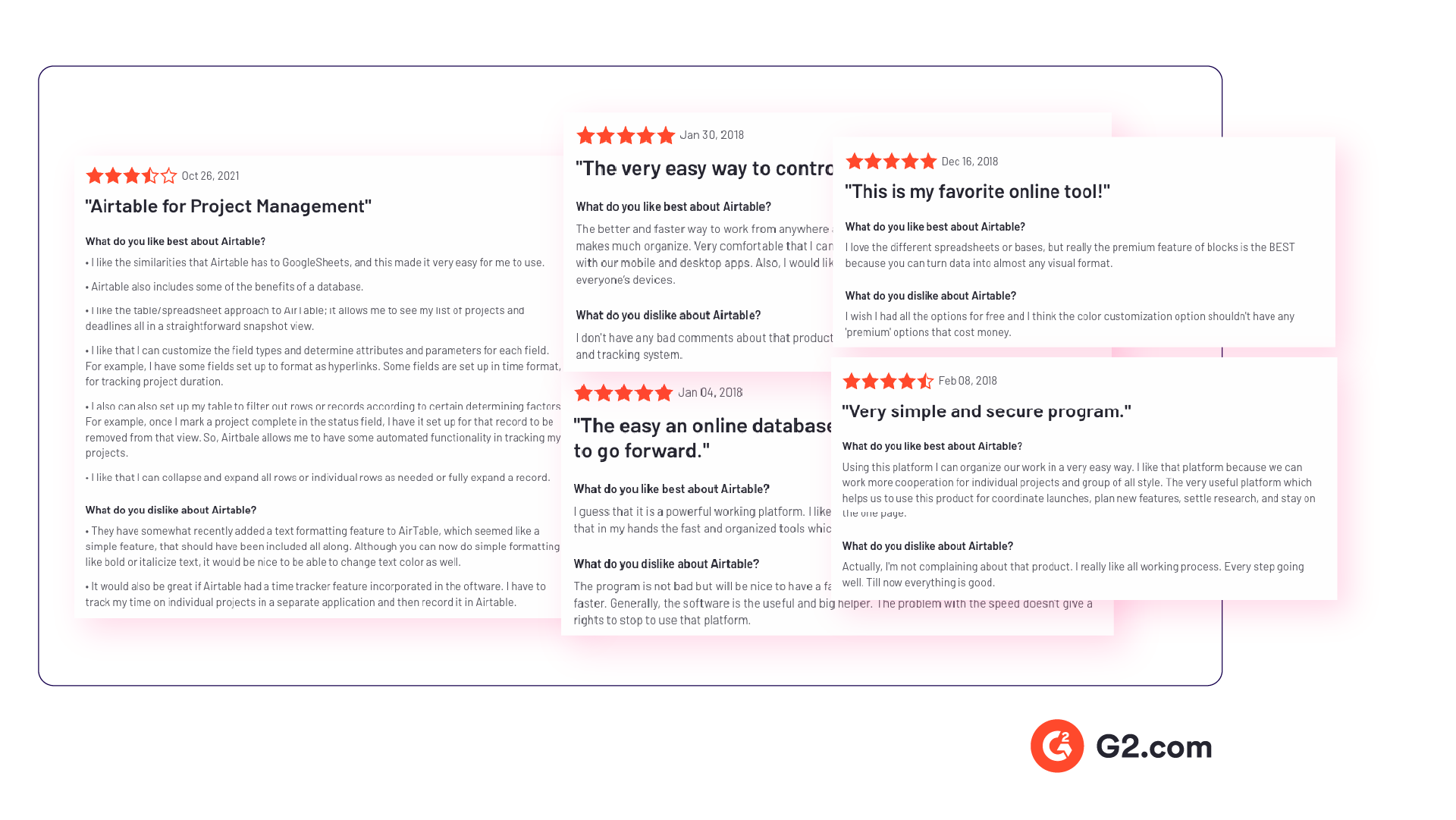
Step 2: Connect & organize all your qualitative data
Now you all have this qualitative data but there’s a problem, the data is unstructured. Before feedback can be analyzed and assigned any value, it needs to be organized in a single place. Why is this important? Consistency!
If all data is easily accessible in one place and analyzed in a consistent manner, you will have an easier time summarizing and making decisions based on this data.
The manual approach to organizing your data
The classic method of structuring qualitative data is to plot all the raw data you’ve gathered into a spreadsheet.
Typically, research and support teams would share large Excel sheets and different business units would make sense of the qualitative feedback data on their own. Each team collects and organizes the data in a way that best suits them, which means the feedback tends to be kept in separate silos.
An alternative and a more robust solution is to store feedback in a central database, like Snowflake or Amazon Redshift .
Keep in mind that when you organize your data in this way, you are often preparing it to be imported into another software. If you go the route of a database, you would need to use an API to push the feedback into a third-party software.
Computer-assisted qualitative data analysis software (CAQDAS)
Traditionally within the manual analysis approach (but not always), qualitative data is imported into CAQDAS software for coding.
In the early 2000s, CAQDAS software was popularised by developers such as ATLAS.ti, NVivo and MAXQDA and eagerly adopted by researchers to assist with the organizing and coding of data.
The benefits of using computer-assisted qualitative data analysis software:
- Assists in the organizing of your data
- Opens you up to exploring different interpretations of your data analysis
- Allows you to share your dataset easier and allows group collaboration (allows for secondary analysis)
However you still need to code the data, uncover the themes and do the analysis yourself. Therefore it is still a manual approach.
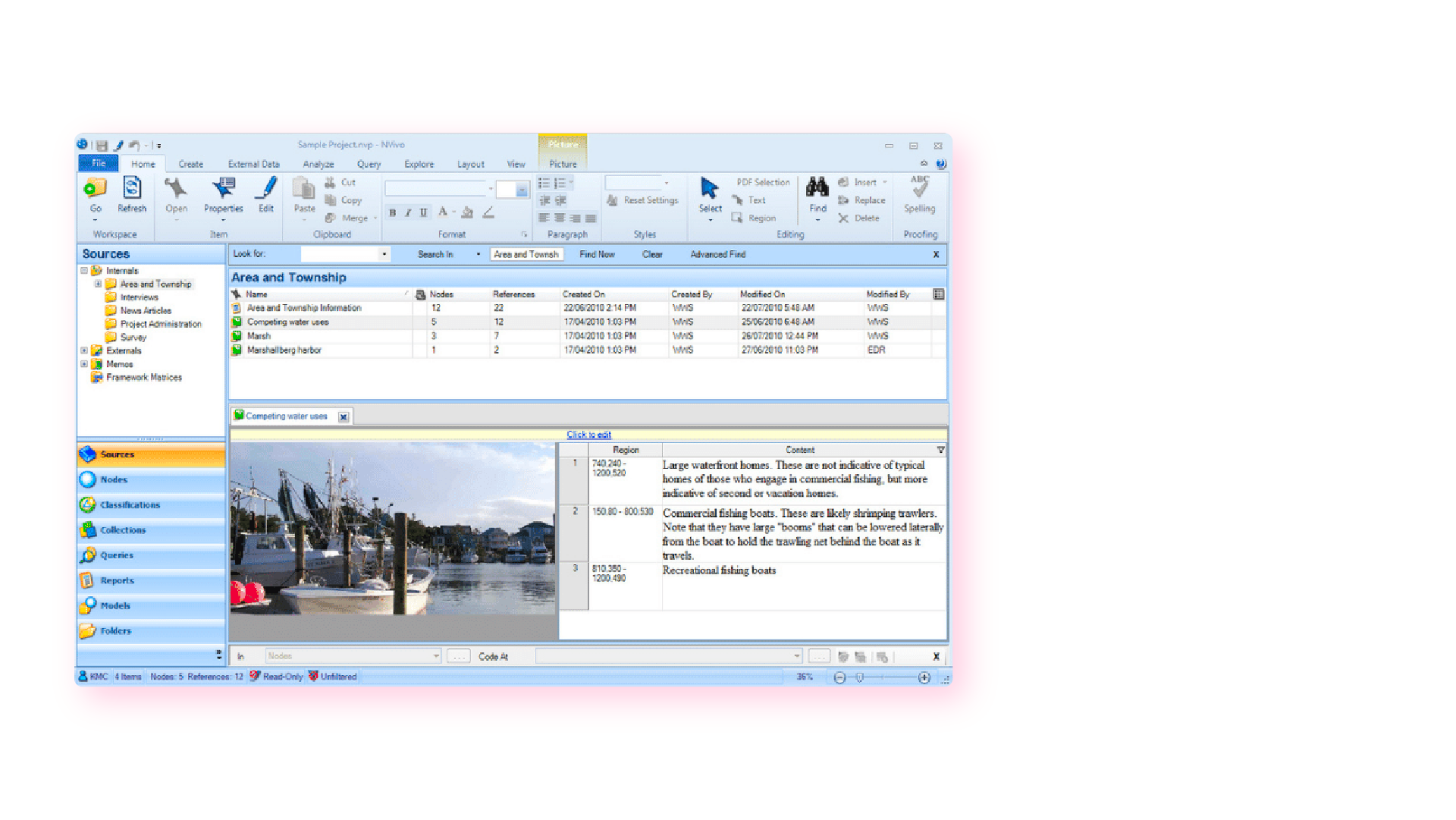
Organizing your qualitative data in a feedback repository
Another solution to organizing your qualitative data is to upload it into a feedback repository where it can be unified with your other data , and easily searchable and taggable. There are a number of software solutions that act as a central repository for your qualitative research data. Here are a couple solutions that you could investigate:
- Dovetail: Dovetail is a research repository with a focus on video and audio transcriptions. You can tag your transcriptions within the platform for theme analysis. You can also upload your other qualitative data such as research reports, survey responses, support conversations, and customer interviews. Dovetail acts as a single, searchable repository. And makes it easier to collaborate with other people around your qualitative research.
- EnjoyHQ: EnjoyHQ is another research repository with similar functionality to Dovetail. It boasts a more sophisticated search engine, but it has a higher starting subscription cost.
Organizing your qualitative data in a feedback analytics platform
If you have a lot of qualitative customer or employee feedback, from the likes of customer surveys or employee surveys, you will benefit from a feedback analytics platform. A feedback analytics platform is a software that automates the process of both sentiment analysis and thematic analysis . Companies use the integrations offered by these platforms to directly tap into their qualitative data sources (review sites, social media, survey responses, etc.). The data collected is then organized and analyzed consistently within the platform.
If you have data prepared in a spreadsheet, it can also be imported into feedback analytics platforms.
Once all this rich data has been organized within the feedback analytics platform, it is ready to be coded and themed, within the same platform. Thematic is a feedback analytics platform that offers one of the largest libraries of integrations with qualitative data sources.
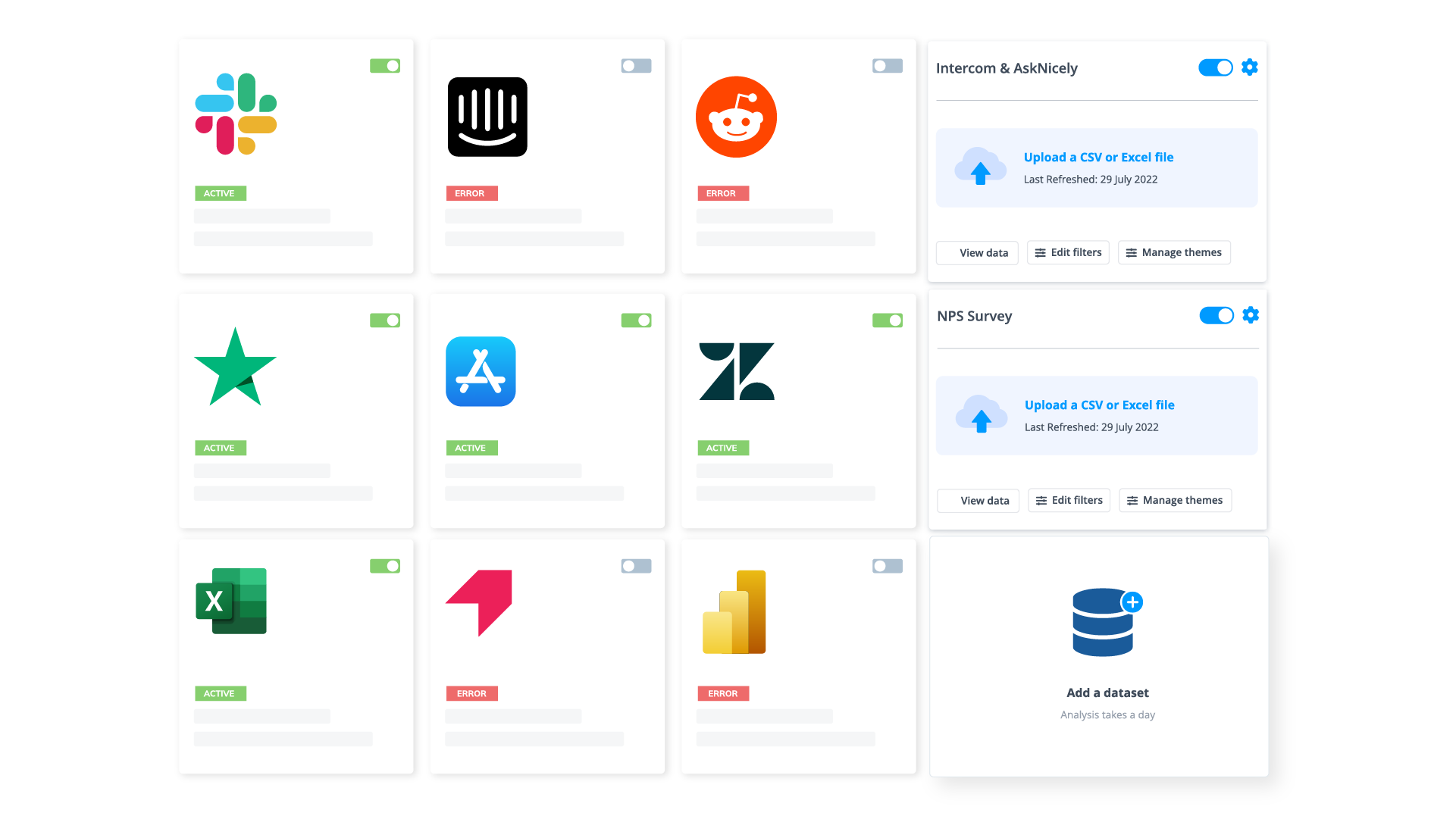
Step 3: Coding your qualitative data
Your feedback data is now organized in one place. Either within your spreadsheet, CAQDAS, feedback repository or within your feedback analytics platform. The next step is to code your feedback data so we can extract meaningful insights in the next step.
Coding is the process of labelling and organizing your data in such a way that you can then identify themes in the data, and the relationships between these themes.
To simplify the coding process, you will take small samples of your customer feedback data, come up with a set of codes, or categories capturing themes, and label each piece of feedback, systematically, for patterns and meaning. Then you will take a larger sample of data, revising and refining the codes for greater accuracy and consistency as you go.
If you choose to use a feedback analytics platform, much of this process will be automated and accomplished for you.
The terms to describe different categories of meaning (‘theme’, ‘code’, ‘tag’, ‘category’ etc) can be confusing as they are often used interchangeably. For clarity, this article will use the term ‘code’.
To code means to identify key words or phrases and assign them to a category of meaning. “I really hate the customer service of this computer software company” would be coded as “poor customer service”.
How to manually code your qualitative data
- Decide whether you will use deductive or inductive coding. Deductive coding is when you create a list of predefined codes, and then assign them to the qualitative data. Inductive coding is the opposite of this, you create codes based on the data itself. Codes arise directly from the data and you label them as you go. You need to weigh up the pros and cons of each coding method and select the most appropriate.
- Read through the feedback data to get a broad sense of what it reveals. Now it’s time to start assigning your first set of codes to statements and sections of text.
- Keep repeating step 2, adding new codes and revising the code description as often as necessary. Once it has all been coded, go through everything again, to be sure there are no inconsistencies and that nothing has been overlooked.
- Create a code frame to group your codes. The coding frame is the organizational structure of all your codes. And there are two commonly used types of coding frames, flat, or hierarchical. A hierarchical code frame will make it easier for you to derive insights from your analysis.
- Based on the number of times a particular code occurs, you can now see the common themes in your feedback data. This is insightful! If ‘bad customer service’ is a common code, it’s time to take action.
We have a detailed guide dedicated to manually coding your qualitative data .
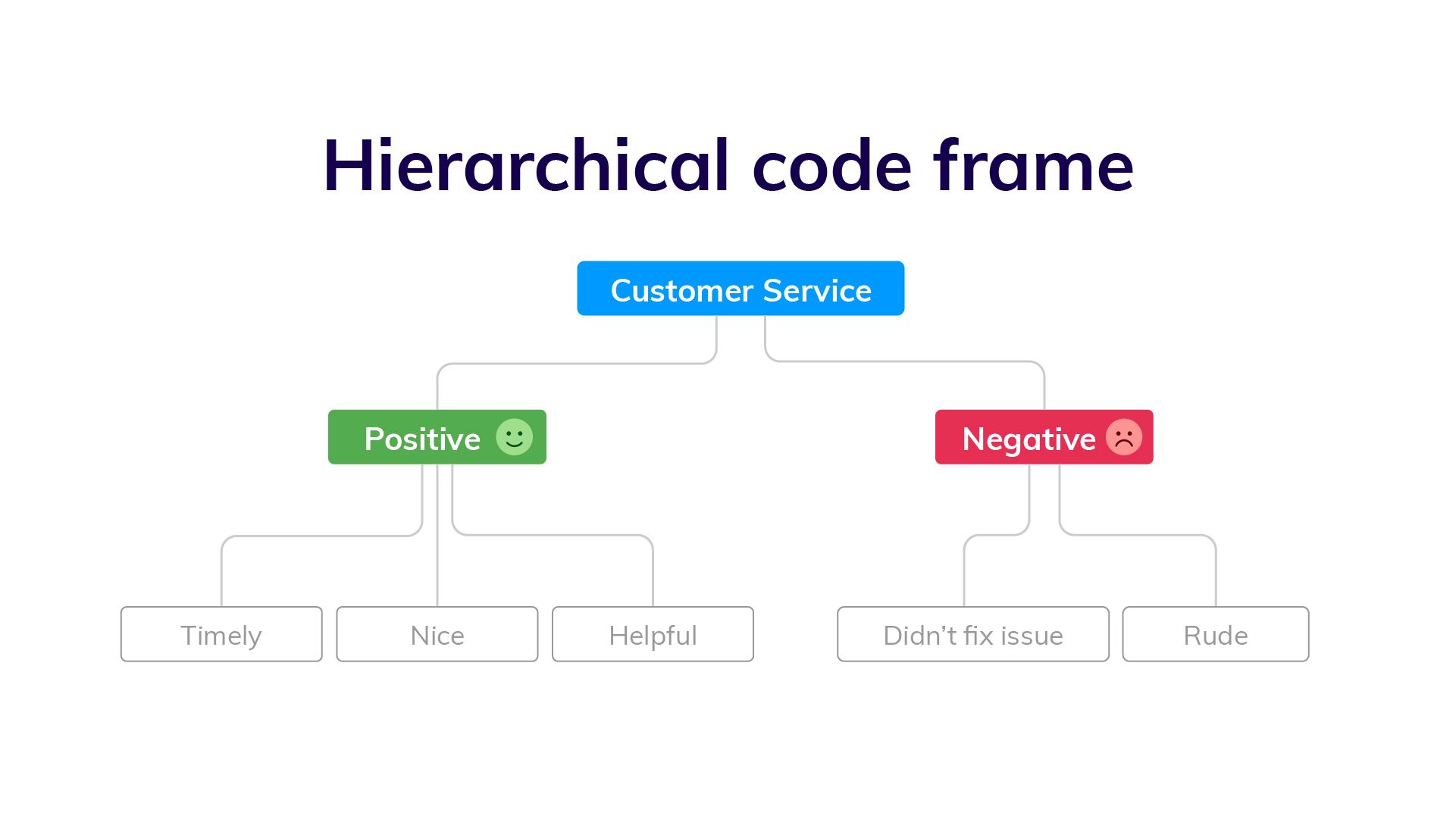
Using software to speed up manual coding of qualitative data
An Excel spreadsheet is still a popular method for coding. But various software solutions can help speed up this process. Here are some examples.
- CAQDAS / NVivo - CAQDAS software has built-in functionality that allows you to code text within their software. You may find the interface the software offers easier for managing codes than a spreadsheet.
- Dovetail/EnjoyHQ - You can tag transcripts and other textual data within these solutions. As they are also repositories you may find it simpler to keep the coding in one platform.
- IBM SPSS - SPSS is a statistical analysis software that may make coding easier than in a spreadsheet.
- Ascribe - Ascribe’s ‘Coder’ is a coding management system. Its user interface will make it easier for you to manage your codes.
Automating the qualitative coding process using thematic analysis software
In solutions which speed up the manual coding process, you still have to come up with valid codes and often apply codes manually to pieces of feedback. But there are also solutions that automate both the discovery and the application of codes.
Advances in machine learning have now made it possible to read, code and structure qualitative data automatically. This type of automated coding is offered by thematic analysis software .
Automation makes it far simpler and faster to code the feedback and group it into themes. By incorporating natural language processing (NLP) into the software, the AI looks across sentences and phrases to identify common themes meaningful statements. Some automated solutions detect repeating patterns and assign codes to them, others make you train the AI by providing examples. You could say that the AI learns the meaning of the feedback on its own.
Thematic automates the coding of qualitative feedback regardless of source. There’s no need to set up themes or categories in advance. Simply upload your data and wait a few minutes. You can also manually edit the codes to further refine their accuracy. Experiments conducted indicate that Thematic’s automated coding is just as accurate as manual coding .
Paired with sentiment analysis and advanced text analytics - these automated solutions become powerful for deriving quality business or research insights.
You could also build your own , if you have the resources!
The key benefits of using an automated coding solution
Automated analysis can often be set up fast and there’s the potential to uncover things that would never have been revealed if you had given the software a prescribed list of themes to look for.
Because the model applies a consistent rule to the data, it captures phrases or statements that a human eye might have missed.
Complete and consistent analysis of customer feedback enables more meaningful findings. Leading us into step 4.
Step 4: Analyze your data: Find meaningful insights
Now we are going to analyze our data to find insights. This is where we start to answer our research questions. Keep in mind that step 4 and step 5 (tell the story) have some overlap . This is because creating visualizations is both part of analysis process and reporting.
The task of uncovering insights is to scour through the codes that emerge from the data and draw meaningful correlations from them. It is also about making sure each insight is distinct and has enough data to support it.
Part of the analysis is to establish how much each code relates to different demographics and customer profiles, and identify whether there’s any relationship between these data points.
Manually create sub-codes to improve the quality of insights
If your code frame only has one level, you may find that your codes are too broad to be able to extract meaningful insights. This is where it is valuable to create sub-codes to your primary codes. This process is sometimes referred to as meta coding.
Note: If you take an inductive coding approach, you can create sub-codes as you are reading through your feedback data and coding it.
While time-consuming, this exercise will improve the quality of your analysis. Here is an example of what sub-codes could look like.
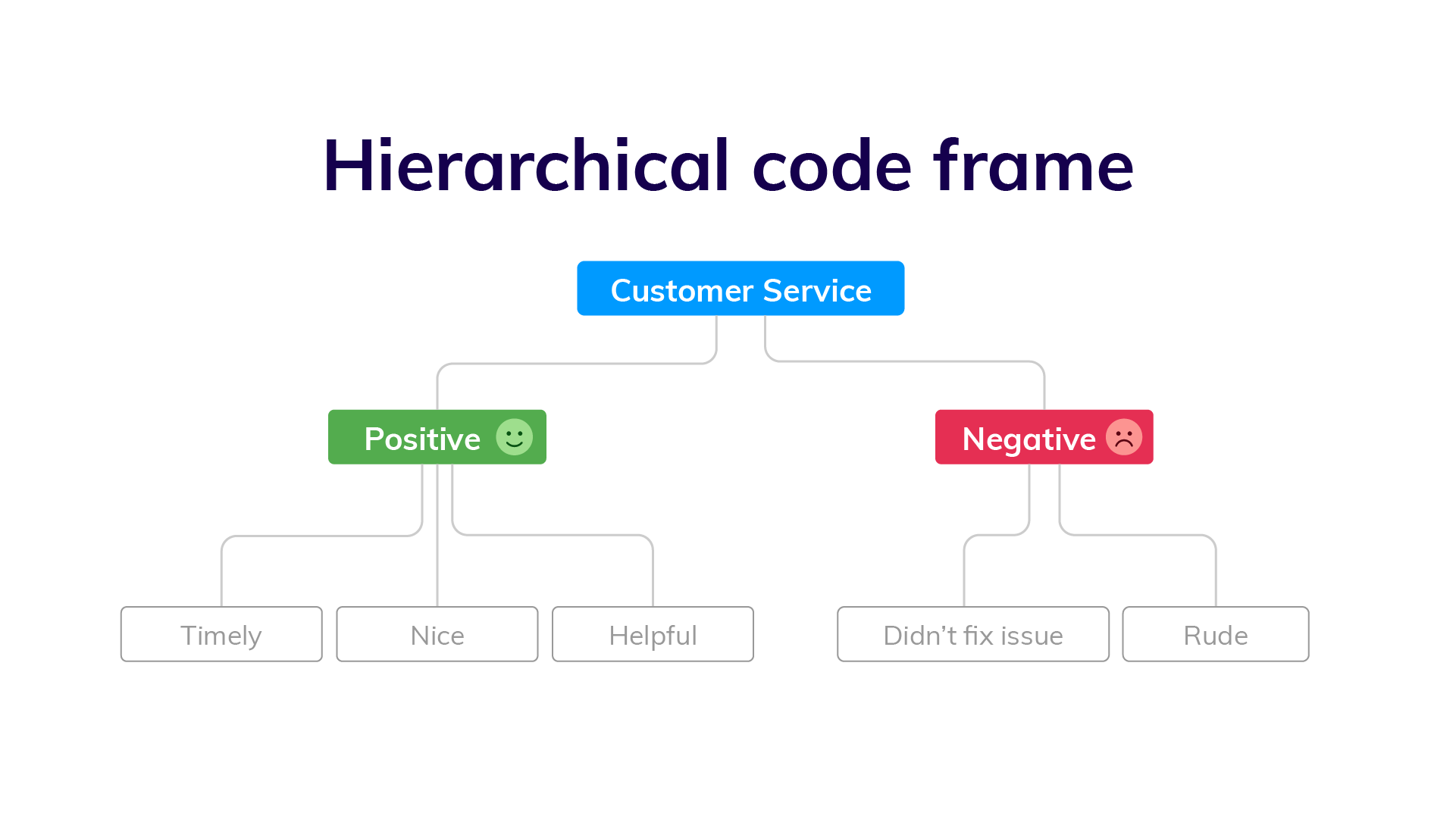
You need to carefully read your qualitative data to create quality sub-codes. But as you can see, the depth of analysis is greatly improved. By calculating the frequency of these sub-codes you can get insight into which customer service problems you can immediately address.
Correlate the frequency of codes to customer segments
Many businesses use customer segmentation . And you may have your own respondent segments that you can apply to your qualitative analysis. Segmentation is the practise of dividing customers or research respondents into subgroups.
Segments can be based on:
- Demographic
- And any other data type that you care to segment by
It is particularly useful to see the occurrence of codes within your segments. If one of your customer segments is considered unimportant to your business, but they are the cause of nearly all customer service complaints, it may be in your best interest to focus attention elsewhere. This is a useful insight!
Manually visualizing coded qualitative data
There are formulas you can use to visualize key insights in your data. The formulas we will suggest are imperative if you are measuring a score alongside your feedback.
If you are collecting a metric alongside your qualitative data this is a key visualization. Impact answers the question: “What’s the impact of a code on my overall score?”. Using Net Promoter Score (NPS) as an example, first you need to:
- Calculate overall NPS
- Calculate NPS in the subset of responses that do not contain that theme
- Subtract B from A
Then you can use this simple formula to calculate code impact on NPS .
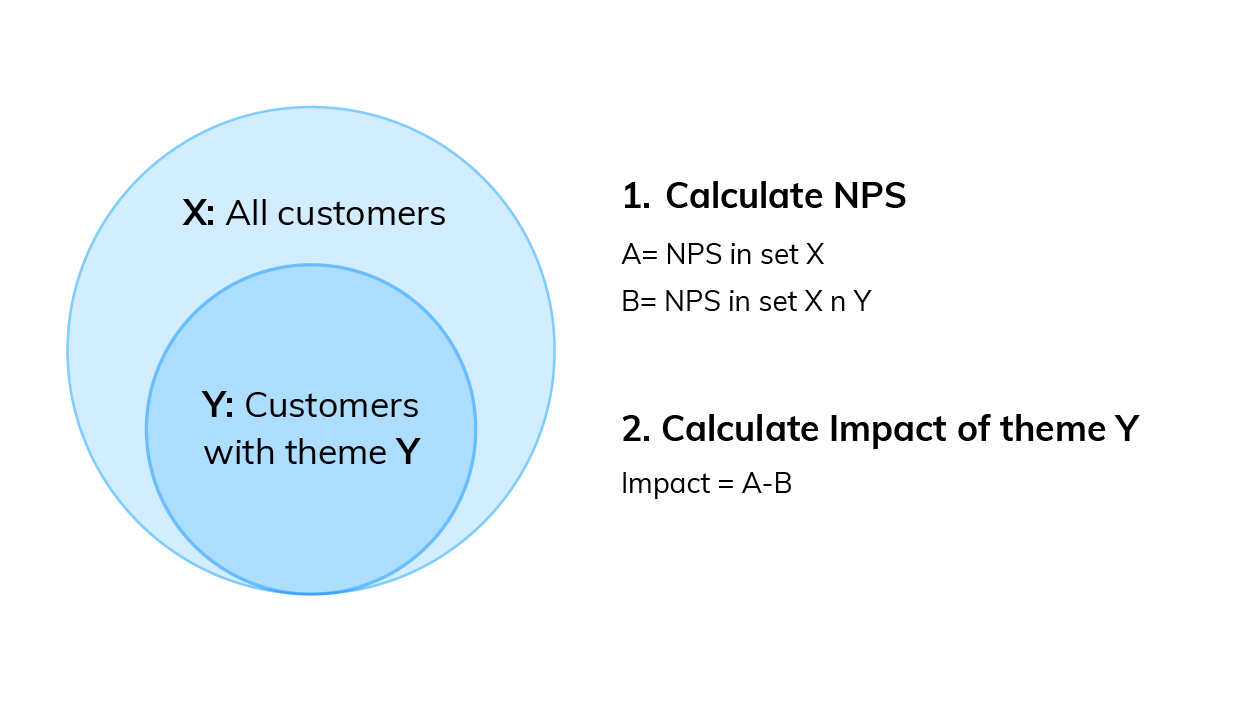
You can then visualize this data using a bar chart.
You can download our CX toolkit - it includes a template to recreate this.
Trends over time
This analysis can help you answer questions like: “Which codes are linked to decreases or increases in my score over time?”
We need to compare two sequences of numbers: NPS over time and code frequency over time . Using Excel, calculate the correlation between the two sequences, which can be either positive (the more codes the higher the NPS, see picture below), or negative (the more codes the lower the NPS).
Now you need to plot code frequency against the absolute value of code correlation with NPS. Here is the formula:
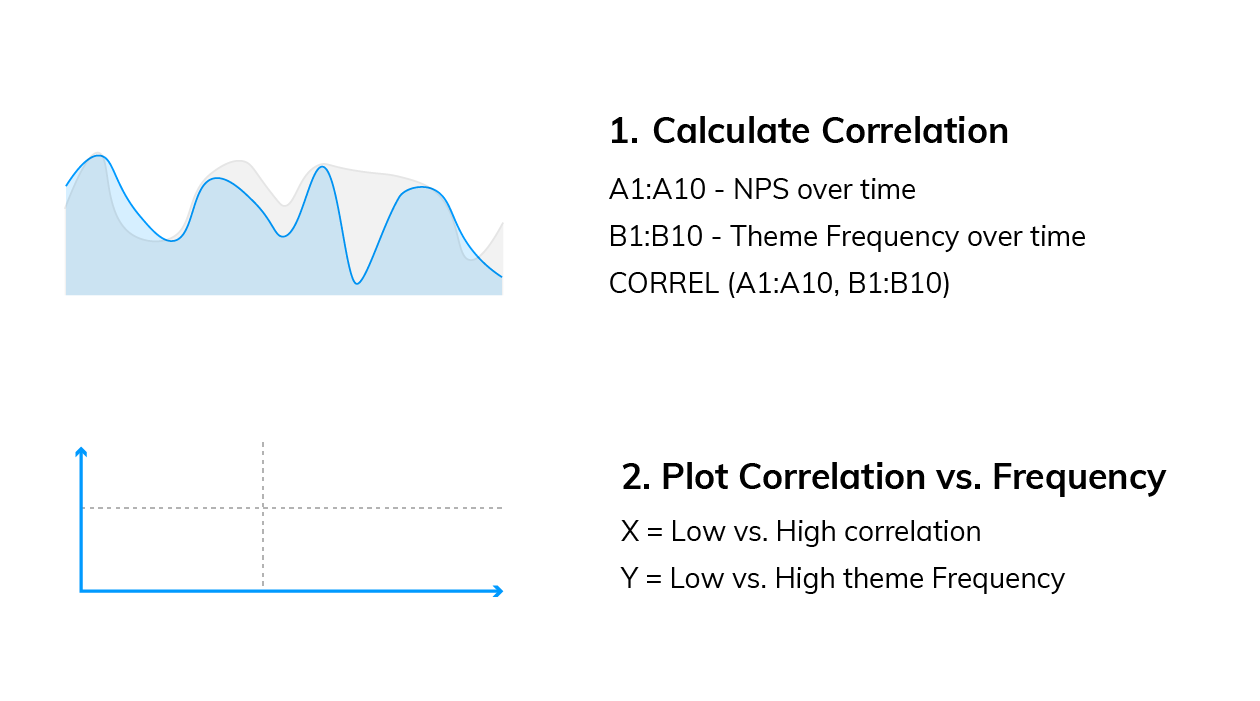
The visualization could look like this:
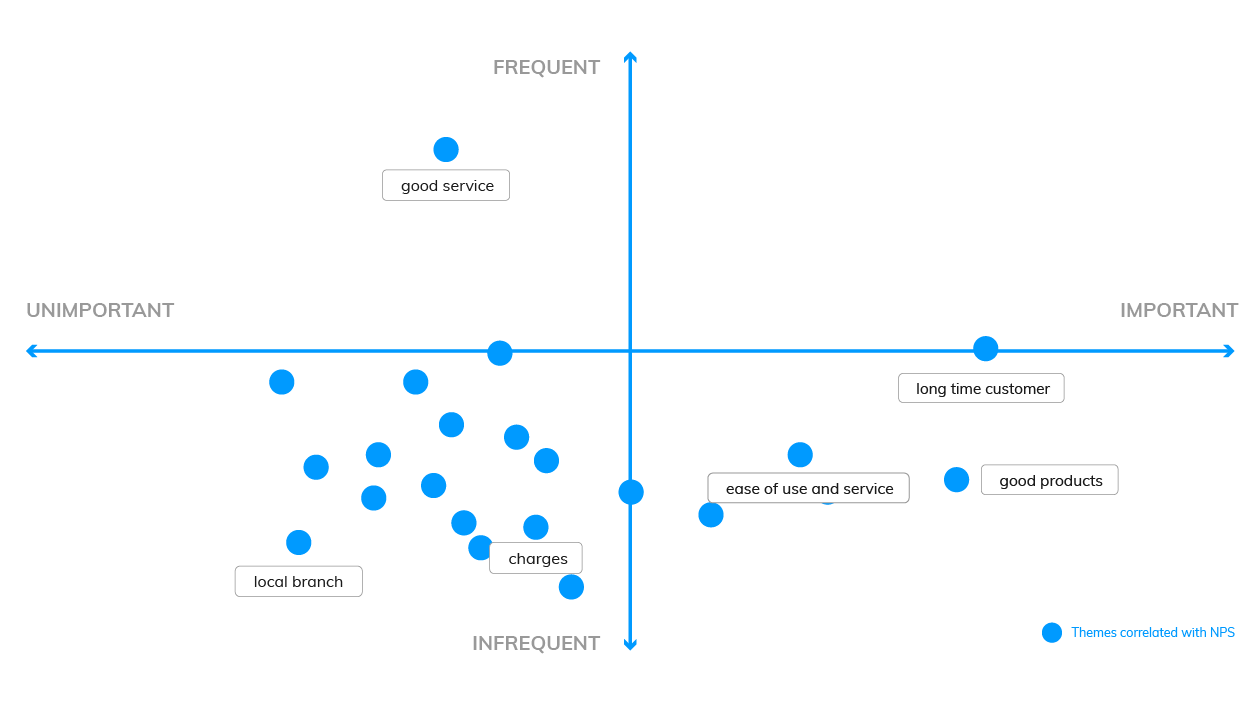
These are two examples, but there are more. For a third manual formula, and to learn why word clouds are not an insightful form of analysis, read our visualizations article .
Using a text analytics solution to automate analysis
Automated text analytics solutions enable codes and sub-codes to be pulled out of the data automatically. This makes it far faster and easier to identify what’s driving negative or positive results. And to pick up emerging trends and find all manner of rich insights in the data.
Another benefit of AI-driven text analytics software is its built-in capability for sentiment analysis, which provides the emotive context behind your feedback and other qualitative textual data therein.
Thematic provides text analytics that goes further by allowing users to apply their expertise on business context to edit or augment the AI-generated outputs.
Since the move away from manual research is generally about reducing the human element, adding human input to the technology might sound counter-intuitive. However, this is mostly to make sure important business nuances in the feedback aren’t missed during coding. The result is a higher accuracy of analysis. This is sometimes referred to as augmented intelligence .
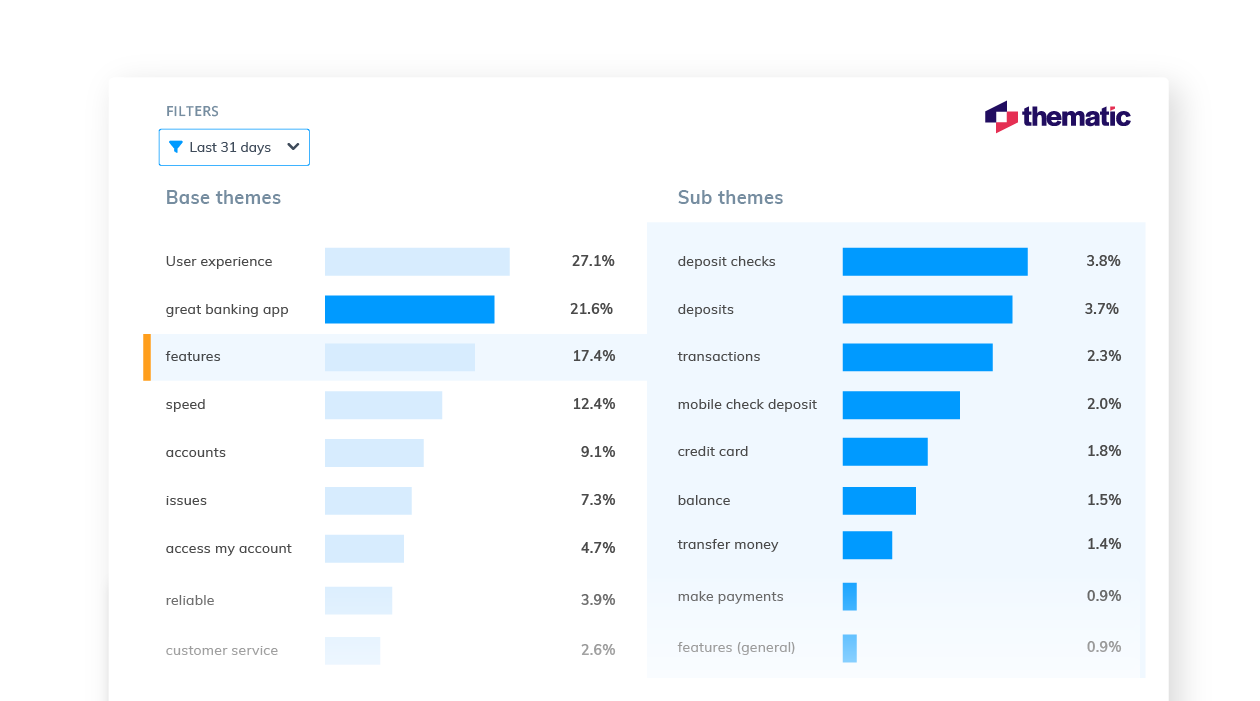
Step 5: Report on your data: Tell the story
The last step of analyzing your qualitative data is to report on it, to tell the story. At this point, the codes are fully developed and the focus is on communicating the narrative to the audience.
A coherent outline of the qualitative research, the findings and the insights is vital for stakeholders to discuss and debate before they can devise a meaningful course of action.
Creating graphs and reporting in Powerpoint
Typically, qualitative researchers take the tried and tested approach of distilling their report into a series of charts, tables and other visuals which are woven into a narrative for presentation in Powerpoint.
Using visualization software for reporting
With data transformation and APIs, the analyzed data can be shared with data visualisation software, such as Power BI or Tableau , Google Studio or Looker. Power BI and Tableau are among the most preferred options.
Visualizing your insights inside a feedback analytics platform
Feedback analytics platforms, like Thematic, incorporate visualisation tools that intuitively turn key data and insights into graphs. This removes the time consuming work of constructing charts to visually identify patterns and creates more time to focus on building a compelling narrative that highlights the insights, in bite-size chunks, for executive teams to review.
Using a feedback analytics platform with visualization tools means you don’t have to use a separate product for visualizations. You can export graphs into Powerpoints straight from the platforms.
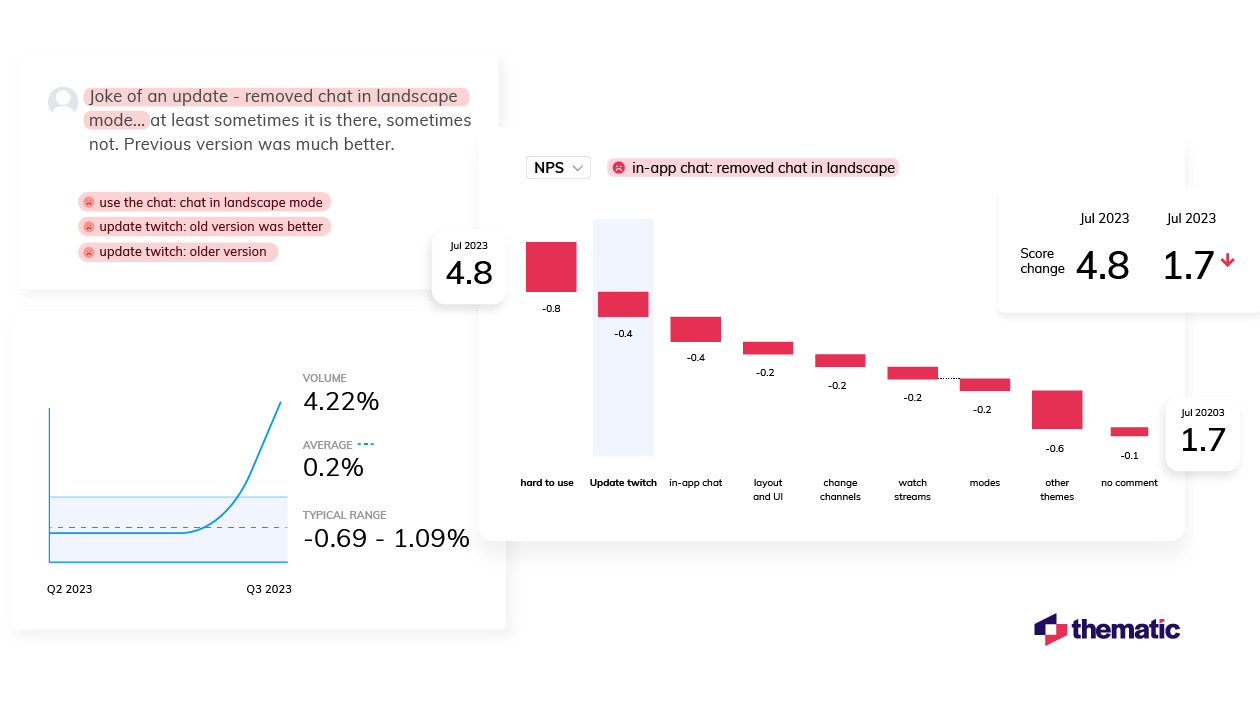
Conclusion - Manual or Automated?
There are those who remain deeply invested in the manual approach - because it’s familiar, because they’re reluctant to spend money and time learning new software, or because they’ve been burned by the overpromises of AI.
For projects that involve small datasets, manual analysis makes sense. For example, if the objective is simply to quantify a simple question like “Do customers prefer X concepts to Y?”. If the findings are being extracted from a small set of focus groups and interviews, sometimes it’s easier to just read them
However, as new generations come into the workplace, it’s technology-driven solutions that feel more comfortable and practical. And the merits are undeniable. Especially if the objective is to go deeper and understand the ‘why’ behind customers’ preference for X or Y. And even more especially if time and money are considerations.
The ability to collect a free flow of qualitative feedback data at the same time as the metric means AI can cost-effectively scan, crunch, score and analyze a ton of feedback from one system in one go. And time-intensive processes like focus groups, or coding, that used to take weeks, can now be completed in a matter of hours or days.
But aside from the ever-present business case to speed things up and keep costs down, there are also powerful research imperatives for automated analysis of qualitative data: namely, accuracy and consistency.
Finding insights hidden in feedback requires consistency, especially in coding. Not to mention catching all the ‘unknown unknowns’ that can skew research findings and steering clear of cognitive bias.
Some say without manual data analysis researchers won’t get an accurate “feel” for the insights. However, the larger data sets are, the harder it is to sort through the feedback and organize feedback that has been pulled from different places. And, the more difficult it is to stay on course, the greater the risk of drawing incorrect, or incomplete, conclusions grows.
Though the process steps for qualitative data analysis have remained pretty much unchanged since psychologist Paul Felix Lazarsfeld paved the path a hundred years ago, the impact digital technology has had on types of qualitative feedback data and the approach to the analysis are profound.
If you want to try an automated feedback analysis solution on your own qualitative data, you can get started with Thematic .
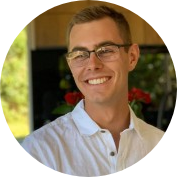
Community & Marketing
Tyler manages our community of CX, insights & analytics professionals. Tyler's goal is to help unite insights professionals around common challenges.
We make it easy to discover the customer and product issues that matter.
Unlock the value of feedback at scale, in one platform. Try it for free now!
- Questions to ask your Feedback Analytics vendor
- How to end customer churn for good
- Scalable analysis of NPS verbatims
- 5 Text analytics approaches
- How to calculate the ROI of CX
Our experts will show you how Thematic works, how to discover pain points and track the ROI of decisions. To access your free trial, book a personal demo today.
Recent posts
Watercare is New Zealand's largest water and wastewater service provider. They are responsible for bringing clean water to 1.7 million people in Tamaki Makaurau (Auckland) and safeguarding the wastewater network to minimize impact on the environment. Water is a sector that often gets taken for granted, with drainage and
Become a qualitative theming pro! Creating a perfect code frame is hard, but thematic analysis software makes the process much easier.
Qualtrics is one of the most well-known and powerful Customer Feedback Management platforms. But even so, it has limitations. We recently hosted a live panel where data analysts from two well-known brands shared their experiences with Qualtrics, and how they extended this platform’s capabilities. Below, we’ll share the
Qualitative Data Analysis Methods
In the following, we will discuss basic approaches to analyzing data in all six of the acceptable qualitative designs.
After reviewing the information in this document, you will be able to:
- Recognize the terms for data analysis methods used in the various acceptable designs.
- Recognize the data preparation tasks that precede actual analysis in all the designs.
- Understand the basic analytic methods used by the respective qualitative designs.
- Identify and apply the methods required by your selected design.
Terms Used in Data Analysis by the Six Designs
Each qualitative research approach or design has its own terms for methods of data analysis:
- Ethnography—uses modified thematic analysis and life histories.
- Case study—uses description, categorical aggregation, or direct interpretation.
- Grounded theory—uses open, axial, and selective coding (although recent writers are proposing variations on those basic analysis methods).
- Phenomenology—describes textures and structures of the essential meaning of the lived experience of the phenomenon
- Heuristics—patterns, themes, and creative synthesis along with individual portraits.
- Generic qualitative inquiry—thematic analysis, which is really a foundation for all the other analytic methods. Thematic analysis is the starting point for the other five, and the endpoint for generic qualitative inquiry. Because it is the basic or foundational method, we'll take it first.
Preliminary Tasks in Analysis in all Methods
In all the approaches—case study, grounded theory, generic inquiry, and phenomenology—there are preliminary tasks that must be performed prior to the analysis itself. For each, you will need to:
- Arrange for secure storage of original materials. Storage should be secure and guaranteed to protect the privacy and confidentiality of the participants' information and identities.
- Transcribe interviews or otherwise transform raw data into usable formats.
- Make master copies and working copies of all materials. Master copies should be kept securely with the original data. Working copies will be marked up, torn apart, and used heavily: make plenty.
- Arrange secure passwords or other protection for all electronic data and copies.
- When ready to begin, read all the transcripts repeatedly—at least three times—for a sense of the whole. Don't force it—allow the participants' words to speak to you.
These tasks are done in all forms of qualitative analysis. Now let's look specifically at generic qualitative inquiry.
Data Analysis in Generic Qualitative Inquiry: Thematic Analysis
The primary tool for conducting the analysis of data when using the generic qualitative inquiry approach is thematic analysis, a flexible analytic method for deriving the central themes from verbal data. A thematic analysis can also be used to conduct analysis of the qualitative data in some types of case study.
Thematic analysis essentially creates theme-statements for ideas or categories of ideas (codes) that the researcher extracts from the words of the participants.
There are two main types of thematic analysis:
- Inductive thematic analysis, in which the data are interpreted inductively, that is, without bringing in any preselected theoretical categories.
- Theoretical thematic analysis, in which the participants' words are interpreted according to categories or constructs from the existing literature.
Analytic Steps in Thematic Analysis: Reading
Remember that the last preliminary task listed above was to read the transcripts for a sense of the whole. In this discussion, we'll assume you're working with transcribed data, usually from interviews. You can apply each step, with changes, to any kind of qualitative data. Now, before you start analyzing, take the first transcript and read it once more, as often as necessary, for a sense of what this participant told you about the topic of your study. If you're using other sources of data, spend time with them holistically.
Thematic Analysis: Steps in the Process
When you have a feel for the data,
- Underline any passages (phases, sentences, or paragraphs) that appear meaningful to you. Don't make any interpretations yet! Review the underlined data.
- Decide if the underlined data are relevant to the research question and cross out or delete all data unrelated to the research question. Some information in the transcript may be interesting but unrelated to the research question.
- Create a name or "code" for each remaining underlined passage (expressions or meaning units) that focus on one single idea. The code should be:
- Briefer than the passage, should
- Sum up its meaning, and should be
- Supported by the meaning unit (the participant's words).
- Find codes that recur; cluster these together. Now begin the interpretation, but only with the understanding that the codes or patterns may shift and change during the process of analysis.
- After you have developed the clusters or patterns of codes, name each pattern. The pattern name is a theme. Use language supported by the original data in the language of your discipline and field.
- Write a brief description of each theme. Use brief direct quotations from the transcript to show the reader how the patterns emerged from the data.
- Compose a paragraph integrating all the themes you developed from the individual's data.
- Repeat this process for each participant, the "within-participant" analysis.
- Finally, integrate all themes from all participants in "across-participants" analysis, showing what general themes are found across all the data.
Some variation of thematic analysis will appear in most of the other forms of qualitative data analysis, but the other methods tend to be more complex. Let's look at them one at a time. If you are already clear as to which approach or design your study will use, you can skip to the appropriate section below.
Ethnographic Data Analysis
Ethnographic data analysis relies on a modified thematic analysis. It is called modified because it combines standard thematic analysis as previously described for interview data with modified thematic methods applied to artifacts, observational notes, and other non-interview data.
Depending on the kinds of data to be interpreted (for instance pictures and historical documents) Ethnographers devise unique ways to find patterns or themes in the data. Finally, the themes must be integrated across all sources and kinds of data to arrive at a composite thematic picture of the culture.
(Adapted from Bogdan and Taylor, 1975; Taylor and Bogdan, 1998; Aronson, 1994.)
Data Analysis in Grounded Theory
Going beyond the descriptive and interpretive goals of many other qualitative models, grounded theory's goal is building a theory. It seeks explanation, not simply description.
It uses a constant comparison method of data analysis that begins as soon as the researcher starts collecting data. Each data collection event (for example, an interview) is analyzed immediately, and later data collection events can be modified to seek more information on emerging themes.
In other words, analysis goes on during each step of the data collection, not merely after data collection.
The heart of the grounded theory analysis is coding, which is analogous to but more rigorous than coding in thematic analysis.
Coding in Grounded Theory Method
There are three different types of coding used in a sequential manner.
- The first type of coding is open coding, which is like basic coding in thematic analysis. During open coding, the researcher performs:
- A line-by-line analysis (or sentence or paragraph analysis) of the data.
- Labels and categorizes the dimensions or aspects of the phenomenon being studied.
- The researcher also uses memos to describe the categories that are found.
- The second type of coding is axial coding, which involves finding links between categories and subcategories found in the open coding.
- The open codes are examined for their relationships: cause and effect, co-occurrence, and so on.
- The goal here is to picture how the various dimensions or categories of data interact with one another in time and space.
- The third type of coding is selective coding, which identifies a core category and relates the categories subsidiary to this core.
- Selective coding selects the main phenomenon, (core category) around which subsidiary phenomena, (all other categories) are grouped, arranging the groupings, studying the results, and rearranging where the data require it.
The Final Stages of Grounded Theory Analysis, after Coding
From selective coding, the grounded theory researcher develops:
- A model of the process, which is the description of which actions and interactions occur in a sequence or series.
- A transactional system, which is the description of how the interactions of different events explain the phenomenon being investigated.
- Finally, A conditional matrix is diagrammed to help consider the conditions and consequences related to the phenomenon under study.
These three essentially tell the story of the outcome of the research, in other words, the description of the process by which the phenomenon seems to happen, the transactional system supporting it, and the conditional matrix that pictures the explanation of the phenomenon are the findings of a grounded theory study.
(Adapted from Corbin and Strauss, 2008; Strauss and Corbin, 1990, 1998.)
Data Analysis in Qualitative Case Study: Background
There are a few points to consider in analyzing case study data:
- Analysis can be:
- Holistic—the entire case.
- Embedded—a specific aspect of the case.
- Multiple sources and kinds of data must be collected and analyzed.
- Data must be collected, analyzed, and described about both:
- The contexts of the case (its social, political, economic contexts, its affiliations with other organizations or cases, and so on).
- The setting of the case (geography, location, physical grounds, or set-up, business organization, etc.).
Qualitative Case Study Data Analysis Methods
Data analysis is detailed in description and consists of an analysis of themes. Especially for interview or documentary analysis, thematic analysis can be used (see the section on generic qualitative inquiry). A typical format for data analysis in a case study consists of the following phases:
- Description: This entails developing a detailed description of each instance of the case and its setting. The words "instance" and "case" can be confusing. Let's say we're conducting a case study of gay and lesbian members of large urban evangelical Christian congregations in the Southeast. The case would be all such people and their congregations. Instances of the case would be any individual person or congregation. In this phase, all the congregations (the settings) and their larger contexts would be described in detail, along with the individuals who are interviewed or observed.
- Categorical Aggregation: This involves seeking a collection of themes from the data, hoping that relevant meaning about lessons to be learned about the case will emerge. Using our example, a kind of thematic analysis from all the data would be performed, looking for common themes.
- Direct Interpretation: By looking at the single instance or member of the case and drawing meaning from it without looking for multiple instances, direct interpretation pulls the data apart and puts it together in more meaningful ways. Here, the interviews with all the gay and lesbian congregation members would be subjected to thematic analysis or some other form of analysis for themes.
- Within-Case Analysis: This would identify the themes that emerge from the data collected from each instance of the case, including connections between or among the themes. These themes would be further developed using verbatim passages and direct quotation to elucidate each theme. This would serve as the summary of the thematic analysis for each individual participant.
- Cross-Case Analysis: This phase develops a thematic analysis across cases as well as assertions and interpretations of the meaning of the themes emerging from all participants in the study.
- Interpretive Phase: In the final phase, this is the creation of naturalistic generalizations from the data as a whole and reporting on the lesson learned from the case study.
(Adapted from Creswell, 1998; Stake, 1995.)
Data Analysis in Phenomenological Research
There are a few existing models of phenomenological research, and they each propose slightly different methods of data analysis. They all arrive at the same goal, however. The goal of phenomenological analysis is to describe the essence or core structures and textures of some conscious psychological experience. One such model, empirical, was developed at Duquesne University. This method of analysis consists of five essential steps and represents the other variations well. Whichever model is chosen, those wishing to conduct phenomenological research must choose a model and abide by its procedures. Empirical phenomenology is presented as an example.
- Sense of the whole. One reads the entire description in order to get a general sense of the whole statement. This often takes a few readings, which should be approached contemplatively.
- Discrimination of meaning units. Once the sense of the whole has been grasped, the researcher returns to the beginning and reads through the text once more, delineating each transition in meaning.
- The researcher adopts a psychological perspective to do this. This means that the researcher looks for shifts in psychological meaning.
- The researcher focuses on the phenomenon being investigated. This means that the researcher keeps in mind the study's topic and looks for meaningful passages related to it.
- The researcher next eliminates redundancies and unrelated meaning units.
- Transformation of subjects' everyday expressions (meaning units) into psychological language. Once meaning units have been delineated,
- The researcher reflects on each of the meaning units, which are still expressed in the concrete language of the participants, and describes the essence of the statement for the participant.
- The researcher makes these descriptions in the language of psychological science.
- Synthesis of transformed meaning units into a consistent statement of the structure of the experience.
- Using imaginative variation on these transformed meaning units, the researcher discovers what remains unchanged when variations are imaginatively applied, and
- From this develops a consistent statement regarding the structure of the participant's experience.
- The researcher completes this process for each transcript in the study.
- Final synthesis. Finally, the researcher synthesizes all of the statements regarding each participant's experience into one consistent statement that describes and captures [of] the essence of the experience being studied.
(Adapted from Giorgi, 1985, 1997; Giorgi and Giorgi, 2003.)
Data Analysis in Heuristics
Six steps typically characterize the heuristic process of data analysis, consisting of:
- Initial engagement.
- Incubation.
- Illumination.
- Explication.
To start, place all the material drawn from one participant before you (recordings, transcriptions, journals, notes, poems, artwork, and so on). This material may either be data gathered by self-search or by interviews with co-researchers.
- Immerse yourself fully in the material until you are aware of and understand everything that is before you.
- Incubate the material. Put the material aside for a while. Let it settle in you. Live with it but without particular attention or focus. Return to the immersion process. Make notes where they would enable you to remember or classify the material. Continue this rhythm of working with the data and resting until an illumination or essential configuration emerges. From your core or global sense, list the essential components or patterns and themes that characterize the fundamental nature and meaning of the experience. Reflectively study the patterns and themes, dwell inside them, and develop a full depiction of the experience. The depiction must include the essential components of the experience.
- Illustrate the depiction of the experience with verbatim samples, poems, stories, or other materials to highlight and accentuate the person's lived experience.
- Return to the raw material of your co-researcher (participant). Does your depiction of the experience fit the data from which you have developed it? Does it contain all that is essential?
- Develop a full reflective depiction of the experience, one that characterizes the participant's experience reflecting core meanings for the individuals as a whole. Include in the depiction, verbatim samples, poems, stories, and the like to highlight and accentuate the lived nature of the experience. This depiction will serve as the creative synthesis, which will combine the themes and patterns into a representation of the whole in an aesthetically pleasing way. This synthesis will communicate the essence of the lived experience under inquiry. The synthesis is more than a summary: it is like a chemical reaction, a creation anew.
- Return to the data and develop a portrait of the person in such a way that the phenomenon and the person emerge as real.
(Adapted from Douglass and Moustakas, l985; Moustakas, 1990.)
Bogdan, R., & Taylor, S. J. (1975). Introduction to qualitative research methods: A phenomenological approach (3rd ed.). New York, NY: Wiley.
Corbin, J., & Strauss, A. (2008). Basics of qualitative research: Techniques and procedures for developing grounded theory (3rd ed.). Los Angeles, CA: Sage.
Creswell, J. W. (1998). Qualitative inquiry and research design: Choosing among five traditions . Thousand Oaks, CA: Sage.
Douglass, B. G., & Moustakas, C. (1985). Heuristic inquiry: The internal search to know. Journal of Humanistic Psychology , 25(3), 39–55.
Giorgi, A. (Ed.). (1985). Phenomenology and psychological research . Pittsburgh, PA: Duquesne University Press.
Giorgi, A. (1997). The theory, practice and evaluation of phenomenological methods as a qualitative research procedure. Journal of Phenomenological Psychology , 28, 235–260.
Giorgi, A. P., & Giorgi, B. M. (2003). The descriptive phenomenological psychological method. In P. M. Camic, J. E. Rhodes, & L. Yardley (Eds.), Qualitative research in psychology: Expanding perspectives in methodology and design (pp. 243–273). Washington, DC: American Psychological Association.
Moustakas, C. (1990). Heuristic research: Design, methodology, and applications . Newbury Park, CA: Sage.
Stake, R. E. (1995). The art of case study research . Thousand Oaks, CA: Sage.
Strauss, A., & Corbin, J. (1990). Basics of qualitative research: Grounded theory procedures and techniques . Newbury Park, CA: Sage.
Strauss, A., & Corbin, J. (1998). Basics of qualitative research: Techniques and theory for developing grounded theory (2nd ed.). Thousand Oaks, CA: Sage.
Taylor, S. J., & Bogdan, R. (1998). Introduction to qualitative research methods: A guidebook and resource (3rd ed.). New York: Wiley.
Doc. reference: phd_t3_u06s6_qualanalysis.html
Have a language expert improve your writing
Run a free plagiarism check in 10 minutes, generate accurate citations for free.
- Knowledge Base
Methodology
- What Is a Case Study? | Definition, Examples & Methods
What Is a Case Study? | Definition, Examples & Methods
Published on May 8, 2019 by Shona McCombes . Revised on November 20, 2023.
A case study is a detailed study of a specific subject, such as a person, group, place, event, organization, or phenomenon. Case studies are commonly used in social, educational, clinical, and business research.
A case study research design usually involves qualitative methods , but quantitative methods are sometimes also used. Case studies are good for describing , comparing, evaluating and understanding different aspects of a research problem .
Table of contents
When to do a case study, step 1: select a case, step 2: build a theoretical framework, step 3: collect your data, step 4: describe and analyze the case, other interesting articles.
A case study is an appropriate research design when you want to gain concrete, contextual, in-depth knowledge about a specific real-world subject. It allows you to explore the key characteristics, meanings, and implications of the case.
Case studies are often a good choice in a thesis or dissertation . They keep your project focused and manageable when you don’t have the time or resources to do large-scale research.
You might use just one complex case study where you explore a single subject in depth, or conduct multiple case studies to compare and illuminate different aspects of your research problem.
Prevent plagiarism. Run a free check.
Once you have developed your problem statement and research questions , you should be ready to choose the specific case that you want to focus on. A good case study should have the potential to:
- Provide new or unexpected insights into the subject
- Challenge or complicate existing assumptions and theories
- Propose practical courses of action to resolve a problem
- Open up new directions for future research
TipIf your research is more practical in nature and aims to simultaneously investigate an issue as you solve it, consider conducting action research instead.
Unlike quantitative or experimental research , a strong case study does not require a random or representative sample. In fact, case studies often deliberately focus on unusual, neglected, or outlying cases which may shed new light on the research problem.
Example of an outlying case studyIn the 1960s the town of Roseto, Pennsylvania was discovered to have extremely low rates of heart disease compared to the US average. It became an important case study for understanding previously neglected causes of heart disease.
However, you can also choose a more common or representative case to exemplify a particular category, experience or phenomenon.
Example of a representative case studyIn the 1920s, two sociologists used Muncie, Indiana as a case study of a typical American city that supposedly exemplified the changing culture of the US at the time.
While case studies focus more on concrete details than general theories, they should usually have some connection with theory in the field. This way the case study is not just an isolated description, but is integrated into existing knowledge about the topic. It might aim to:
- Exemplify a theory by showing how it explains the case under investigation
- Expand on a theory by uncovering new concepts and ideas that need to be incorporated
- Challenge a theory by exploring an outlier case that doesn’t fit with established assumptions
To ensure that your analysis of the case has a solid academic grounding, you should conduct a literature review of sources related to the topic and develop a theoretical framework . This means identifying key concepts and theories to guide your analysis and interpretation.
There are many different research methods you can use to collect data on your subject. Case studies tend to focus on qualitative data using methods such as interviews , observations , and analysis of primary and secondary sources (e.g., newspaper articles, photographs, official records). Sometimes a case study will also collect quantitative data.
Example of a mixed methods case studyFor a case study of a wind farm development in a rural area, you could collect quantitative data on employment rates and business revenue, collect qualitative data on local people’s perceptions and experiences, and analyze local and national media coverage of the development.
The aim is to gain as thorough an understanding as possible of the case and its context.
Receive feedback on language, structure, and formatting
Professional editors proofread and edit your paper by focusing on:
- Academic style
- Vague sentences
- Style consistency
See an example
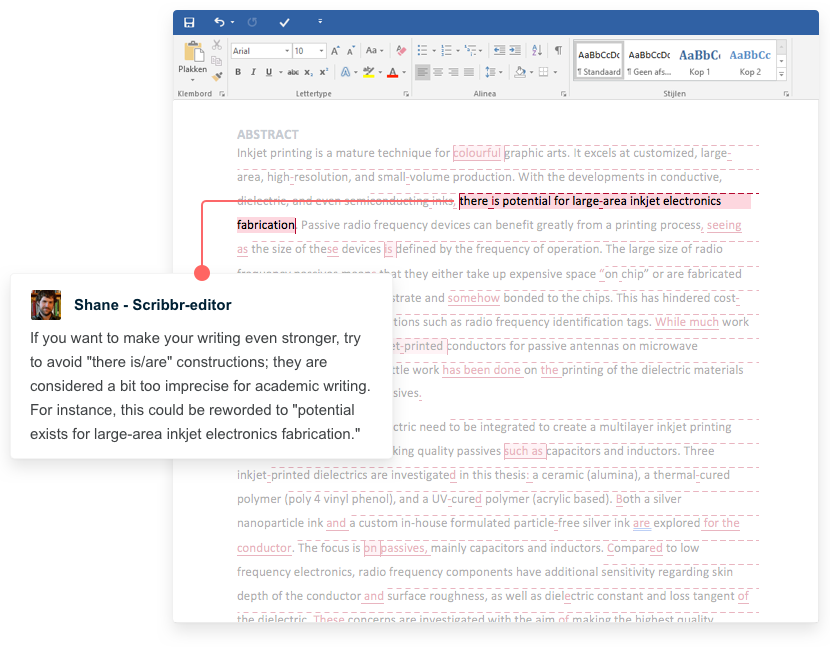
In writing up the case study, you need to bring together all the relevant aspects to give as complete a picture as possible of the subject.
How you report your findings depends on the type of research you are doing. Some case studies are structured like a standard scientific paper or thesis , with separate sections or chapters for the methods , results and discussion .
Others are written in a more narrative style, aiming to explore the case from various angles and analyze its meanings and implications (for example, by using textual analysis or discourse analysis ).
In all cases, though, make sure to give contextual details about the case, connect it back to the literature and theory, and discuss how it fits into wider patterns or debates.
If you want to know more about statistics , methodology , or research bias , make sure to check out some of our other articles with explanations and examples.
- Normal distribution
- Degrees of freedom
- Null hypothesis
- Discourse analysis
- Control groups
- Mixed methods research
- Non-probability sampling
- Quantitative research
- Ecological validity
Research bias
- Rosenthal effect
- Implicit bias
- Cognitive bias
- Selection bias
- Negativity bias
- Status quo bias
Cite this Scribbr article
If you want to cite this source, you can copy and paste the citation or click the “Cite this Scribbr article” button to automatically add the citation to our free Citation Generator.
McCombes, S. (2023, November 20). What Is a Case Study? | Definition, Examples & Methods. Scribbr. Retrieved April 9, 2024, from https://www.scribbr.com/methodology/case-study/
Is this article helpful?
Shona McCombes
Other students also liked, primary vs. secondary sources | difference & examples, what is a theoretical framework | guide to organizing, what is action research | definition & examples, what is your plagiarism score.

Qualitative Data Analysis Methods 101:
The “big 6” methods + examples.
By: Kerryn Warren (PhD) | Reviewed By: Eunice Rautenbach (D.Tech) | May 2020 (Updated April 2023)
Qualitative data analysis methods. Wow, that’s a mouthful.
If you’re new to the world of research, qualitative data analysis can look rather intimidating. So much bulky terminology and so many abstract, fluffy concepts. It certainly can be a minefield!
Don’t worry – in this post, we’ll unpack the most popular analysis methods , one at a time, so that you can approach your analysis with confidence and competence – whether that’s for a dissertation, thesis or really any kind of research project.
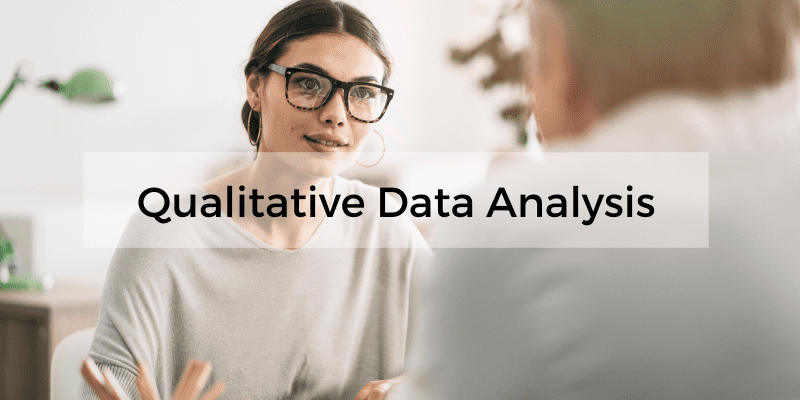
What (exactly) is qualitative data analysis?
To understand qualitative data analysis, we need to first understand qualitative data – so let’s step back and ask the question, “what exactly is qualitative data?”.
Qualitative data refers to pretty much any data that’s “not numbers” . In other words, it’s not the stuff you measure using a fixed scale or complex equipment, nor do you analyse it using complex statistics or mathematics.
So, if it’s not numbers, what is it?
Words, you guessed? Well… sometimes , yes. Qualitative data can, and often does, take the form of interview transcripts, documents and open-ended survey responses – but it can also involve the interpretation of images and videos. In other words, qualitative isn’t just limited to text-based data.
So, how’s that different from quantitative data, you ask?
Simply put, qualitative research focuses on words, descriptions, concepts or ideas – while quantitative research focuses on numbers and statistics . Qualitative research investigates the “softer side” of things to explore and describe , while quantitative research focuses on the “hard numbers”, to measure differences between variables and the relationships between them. If you’re keen to learn more about the differences between qual and quant, we’ve got a detailed post over here .
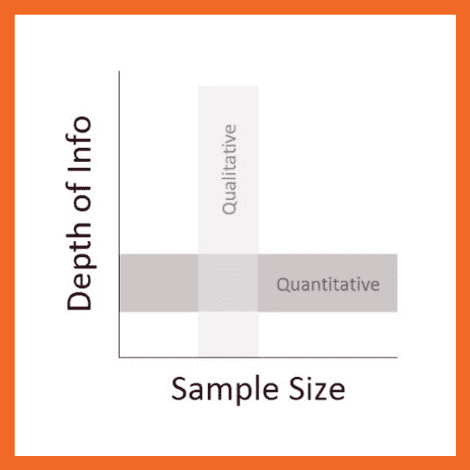
So, qualitative analysis is easier than quantitative, right?
Not quite. In many ways, qualitative data can be challenging and time-consuming to analyse and interpret. At the end of your data collection phase (which itself takes a lot of time), you’ll likely have many pages of text-based data or hours upon hours of audio to work through. You might also have subtle nuances of interactions or discussions that have danced around in your mind, or that you scribbled down in messy field notes. All of this needs to work its way into your analysis.
Making sense of all of this is no small task and you shouldn’t underestimate it. Long story short – qualitative analysis can be a lot of work! Of course, quantitative analysis is no piece of cake either, but it’s important to recognise that qualitative analysis still requires a significant investment in terms of time and effort.
Need a helping hand?
In this post, we’ll explore qualitative data analysis by looking at some of the most common analysis methods we encounter. We’re not going to cover every possible qualitative method and we’re not going to go into heavy detail – we’re just going to give you the big picture. That said, we will of course includes links to loads of extra resources so that you can learn more about whichever analysis method interests you.
Without further delay, let’s get into it.
The “Big 6” Qualitative Analysis Methods
There are many different types of qualitative data analysis, all of which serve different purposes and have unique strengths and weaknesses . We’ll start by outlining the analysis methods and then we’ll dive into the details for each.
The 6 most popular methods (or at least the ones we see at Grad Coach) are:
- Content analysis
- Narrative analysis
- Discourse analysis
- Thematic analysis
- Grounded theory (GT)
- Interpretive phenomenological analysis (IPA)
Let’s take a look at each of them…
QDA Method #1: Qualitative Content Analysis
Content analysis is possibly the most common and straightforward QDA method. At the simplest level, content analysis is used to evaluate patterns within a piece of content (for example, words, phrases or images) or across multiple pieces of content or sources of communication. For example, a collection of newspaper articles or political speeches.
With content analysis, you could, for instance, identify the frequency with which an idea is shared or spoken about – like the number of times a Kardashian is mentioned on Twitter. Or you could identify patterns of deeper underlying interpretations – for instance, by identifying phrases or words in tourist pamphlets that highlight India as an ancient country.
Because content analysis can be used in such a wide variety of ways, it’s important to go into your analysis with a very specific question and goal, or you’ll get lost in the fog. With content analysis, you’ll group large amounts of text into codes , summarise these into categories, and possibly even tabulate the data to calculate the frequency of certain concepts or variables. Because of this, content analysis provides a small splash of quantitative thinking within a qualitative method.
Naturally, while content analysis is widely useful, it’s not without its drawbacks . One of the main issues with content analysis is that it can be very time-consuming , as it requires lots of reading and re-reading of the texts. Also, because of its multidimensional focus on both qualitative and quantitative aspects, it is sometimes accused of losing important nuances in communication.
Content analysis also tends to concentrate on a very specific timeline and doesn’t take into account what happened before or after that timeline. This isn’t necessarily a bad thing though – just something to be aware of. So, keep these factors in mind if you’re considering content analysis. Every analysis method has its limitations , so don’t be put off by these – just be aware of them ! If you’re interested in learning more about content analysis, the video below provides a good starting point.
QDA Method #2: Narrative Analysis
As the name suggests, narrative analysis is all about listening to people telling stories and analysing what that means . Since stories serve a functional purpose of helping us make sense of the world, we can gain insights into the ways that people deal with and make sense of reality by analysing their stories and the ways they’re told.
You could, for example, use narrative analysis to explore whether how something is being said is important. For instance, the narrative of a prisoner trying to justify their crime could provide insight into their view of the world and the justice system. Similarly, analysing the ways entrepreneurs talk about the struggles in their careers or cancer patients telling stories of hope could provide powerful insights into their mindsets and perspectives . Simply put, narrative analysis is about paying attention to the stories that people tell – and more importantly, the way they tell them.
Of course, the narrative approach has its weaknesses , too. Sample sizes are generally quite small due to the time-consuming process of capturing narratives. Because of this, along with the multitude of social and lifestyle factors which can influence a subject, narrative analysis can be quite difficult to reproduce in subsequent research. This means that it’s difficult to test the findings of some of this research.
Similarly, researcher bias can have a strong influence on the results here, so you need to be particularly careful about the potential biases you can bring into your analysis when using this method. Nevertheless, narrative analysis is still a very useful qualitative analysis method – just keep these limitations in mind and be careful not to draw broad conclusions . If you’re keen to learn more about narrative analysis, the video below provides a great introduction to this qualitative analysis method.
QDA Method #3: Discourse Analysis
Discourse is simply a fancy word for written or spoken language or debate . So, discourse analysis is all about analysing language within its social context. In other words, analysing language – such as a conversation, a speech, etc – within the culture and society it takes place. For example, you could analyse how a janitor speaks to a CEO, or how politicians speak about terrorism.
To truly understand these conversations or speeches, the culture and history of those involved in the communication are important factors to consider. For example, a janitor might speak more casually with a CEO in a company that emphasises equality among workers. Similarly, a politician might speak more about terrorism if there was a recent terrorist incident in the country.
So, as you can see, by using discourse analysis, you can identify how culture , history or power dynamics (to name a few) have an effect on the way concepts are spoken about. So, if your research aims and objectives involve understanding culture or power dynamics, discourse analysis can be a powerful method.
Because there are many social influences in terms of how we speak to each other, the potential use of discourse analysis is vast . Of course, this also means it’s important to have a very specific research question (or questions) in mind when analysing your data and looking for patterns and themes, or you might land up going down a winding rabbit hole.
Discourse analysis can also be very time-consuming as you need to sample the data to the point of saturation – in other words, until no new information and insights emerge. But this is, of course, part of what makes discourse analysis such a powerful technique. So, keep these factors in mind when considering this QDA method. Again, if you’re keen to learn more, the video below presents a good starting point.
QDA Method #4: Thematic Analysis
Thematic analysis looks at patterns of meaning in a data set – for example, a set of interviews or focus group transcripts. But what exactly does that… mean? Well, a thematic analysis takes bodies of data (which are often quite large) and groups them according to similarities – in other words, themes . These themes help us make sense of the content and derive meaning from it.
Let’s take a look at an example.
With thematic analysis, you could analyse 100 online reviews of a popular sushi restaurant to find out what patrons think about the place. By reviewing the data, you would then identify the themes that crop up repeatedly within the data – for example, “fresh ingredients” or “friendly wait staff”.
So, as you can see, thematic analysis can be pretty useful for finding out about people’s experiences , views, and opinions . Therefore, if your research aims and objectives involve understanding people’s experience or view of something, thematic analysis can be a great choice.
Since thematic analysis is a bit of an exploratory process, it’s not unusual for your research questions to develop , or even change as you progress through the analysis. While this is somewhat natural in exploratory research, it can also be seen as a disadvantage as it means that data needs to be re-reviewed each time a research question is adjusted. In other words, thematic analysis can be quite time-consuming – but for a good reason. So, keep this in mind if you choose to use thematic analysis for your project and budget extra time for unexpected adjustments.
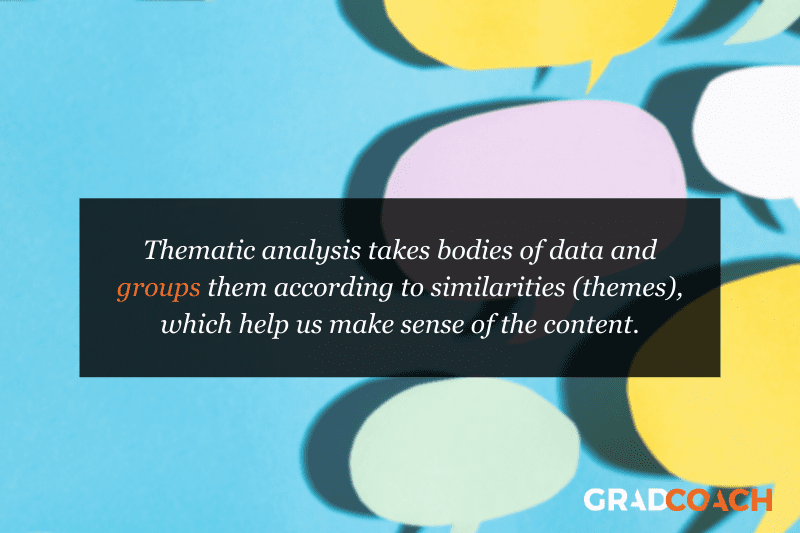
QDA Method #5: Grounded theory (GT)
Grounded theory is a powerful qualitative analysis method where the intention is to create a new theory (or theories) using the data at hand, through a series of “ tests ” and “ revisions ”. Strictly speaking, GT is more a research design type than an analysis method, but we’ve included it here as it’s often referred to as a method.
What’s most important with grounded theory is that you go into the analysis with an open mind and let the data speak for itself – rather than dragging existing hypotheses or theories into your analysis. In other words, your analysis must develop from the ground up (hence the name).
Let’s look at an example of GT in action.
Assume you’re interested in developing a theory about what factors influence students to watch a YouTube video about qualitative analysis. Using Grounded theory , you’d start with this general overarching question about the given population (i.e., graduate students). First, you’d approach a small sample – for example, five graduate students in a department at a university. Ideally, this sample would be reasonably representative of the broader population. You’d interview these students to identify what factors lead them to watch the video.
After analysing the interview data, a general pattern could emerge. For example, you might notice that graduate students are more likely to read a post about qualitative methods if they are just starting on their dissertation journey, or if they have an upcoming test about research methods.
From here, you’ll look for another small sample – for example, five more graduate students in a different department – and see whether this pattern holds true for them. If not, you’ll look for commonalities and adapt your theory accordingly. As this process continues, the theory would develop . As we mentioned earlier, what’s important with grounded theory is that the theory develops from the data – not from some preconceived idea.
So, what are the drawbacks of grounded theory? Well, some argue that there’s a tricky circularity to grounded theory. For it to work, in principle, you should know as little as possible regarding the research question and population, so that you reduce the bias in your interpretation. However, in many circumstances, it’s also thought to be unwise to approach a research question without knowledge of the current literature . In other words, it’s a bit of a “chicken or the egg” situation.
Regardless, grounded theory remains a popular (and powerful) option. Naturally, it’s a very useful method when you’re researching a topic that is completely new or has very little existing research about it, as it allows you to start from scratch and work your way from the ground up .
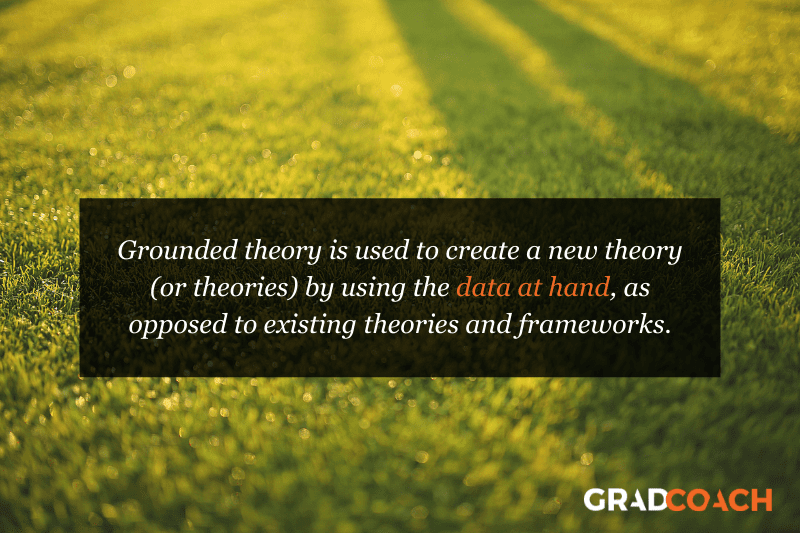
QDA Method #6: Interpretive Phenomenological Analysis (IPA)
Interpretive. Phenomenological. Analysis. IPA . Try saying that three times fast…
Let’s just stick with IPA, okay?
IPA is designed to help you understand the personal experiences of a subject (for example, a person or group of people) concerning a major life event, an experience or a situation . This event or experience is the “phenomenon” that makes up the “P” in IPA. Such phenomena may range from relatively common events – such as motherhood, or being involved in a car accident – to those which are extremely rare – for example, someone’s personal experience in a refugee camp. So, IPA is a great choice if your research involves analysing people’s personal experiences of something that happened to them.
It’s important to remember that IPA is subject – centred . In other words, it’s focused on the experiencer . This means that, while you’ll likely use a coding system to identify commonalities, it’s important not to lose the depth of experience or meaning by trying to reduce everything to codes. Also, keep in mind that since your sample size will generally be very small with IPA, you often won’t be able to draw broad conclusions about the generalisability of your findings. But that’s okay as long as it aligns with your research aims and objectives.
Another thing to be aware of with IPA is personal bias . While researcher bias can creep into all forms of research, self-awareness is critically important with IPA, as it can have a major impact on the results. For example, a researcher who was a victim of a crime himself could insert his own feelings of frustration and anger into the way he interprets the experience of someone who was kidnapped. So, if you’re going to undertake IPA, you need to be very self-aware or you could muddy the analysis.
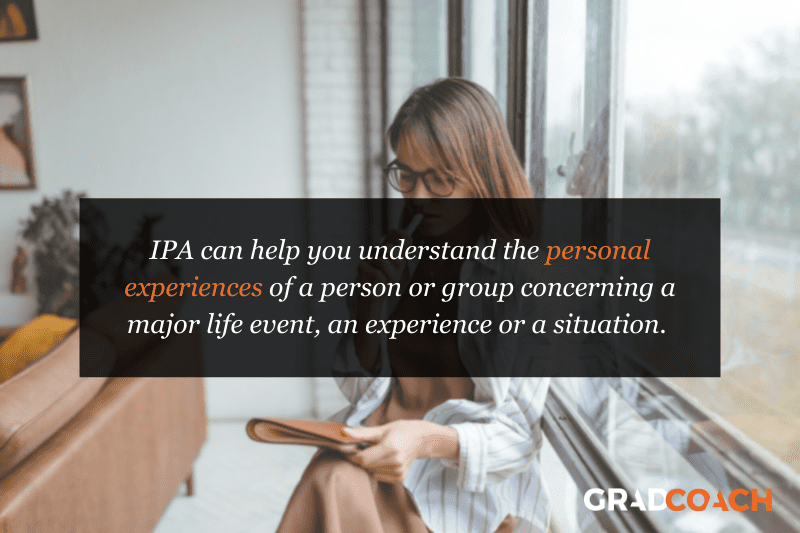
How to choose the right analysis method
In light of all of the qualitative analysis methods we’ve covered so far, you’re probably asking yourself the question, “ How do I choose the right one? ”
Much like all the other methodological decisions you’ll need to make, selecting the right qualitative analysis method largely depends on your research aims, objectives and questions . In other words, the best tool for the job depends on what you’re trying to build. For example:
- Perhaps your research aims to analyse the use of words and what they reveal about the intention of the storyteller and the cultural context of the time.
- Perhaps your research aims to develop an understanding of the unique personal experiences of people that have experienced a certain event, or
- Perhaps your research aims to develop insight regarding the influence of a certain culture on its members.
As you can probably see, each of these research aims are distinctly different , and therefore different analysis methods would be suitable for each one. For example, narrative analysis would likely be a good option for the first aim, while grounded theory wouldn’t be as relevant.
It’s also important to remember that each method has its own set of strengths, weaknesses and general limitations. No single analysis method is perfect . So, depending on the nature of your research, it may make sense to adopt more than one method (this is called triangulation ). Keep in mind though that this will of course be quite time-consuming.
As we’ve seen, all of the qualitative analysis methods we’ve discussed make use of coding and theme-generating techniques, but the intent and approach of each analysis method differ quite substantially. So, it’s very important to come into your research with a clear intention before you decide which analysis method (or methods) to use.
Start by reviewing your research aims , objectives and research questions to assess what exactly you’re trying to find out – then select a qualitative analysis method that fits. Never pick a method just because you like it or have experience using it – your analysis method (or methods) must align with your broader research aims and objectives.
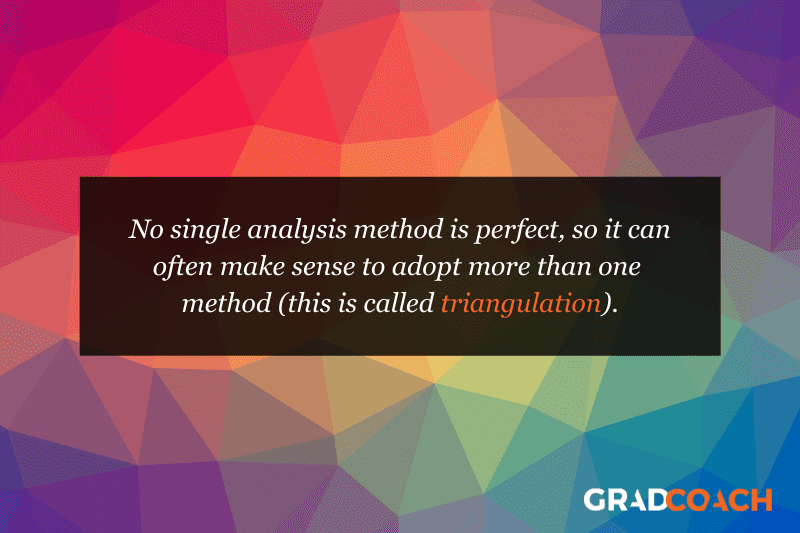
Let’s recap on QDA methods…
In this post, we looked at six popular qualitative data analysis methods:
- First, we looked at content analysis , a straightforward method that blends a little bit of quant into a primarily qualitative analysis.
- Then we looked at narrative analysis , which is about analysing how stories are told.
- Next up was discourse analysis – which is about analysing conversations and interactions.
- Then we moved on to thematic analysis – which is about identifying themes and patterns.
- From there, we went south with grounded theory – which is about starting from scratch with a specific question and using the data alone to build a theory in response to that question.
- And finally, we looked at IPA – which is about understanding people’s unique experiences of a phenomenon.
Of course, these aren’t the only options when it comes to qualitative data analysis, but they’re a great starting point if you’re dipping your toes into qualitative research for the first time.
If you’re still feeling a bit confused, consider our private coaching service , where we hold your hand through the research process to help you develop your best work.
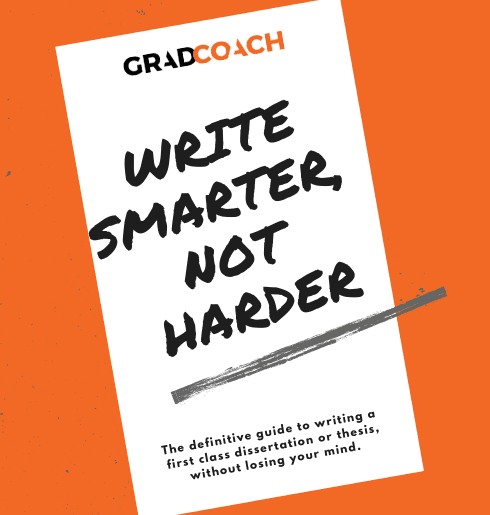
Psst… there’s more (for free)
This post is part of our dissertation mini-course, which covers everything you need to get started with your dissertation, thesis or research project.
You Might Also Like:
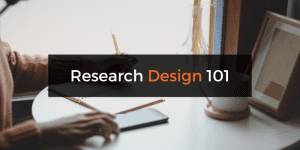
84 Comments
This has been very helpful. Thank you.
Thank you madam,
Thank you so much for this information
I wonder it so clear for understand and good for me. can I ask additional query?
Very insightful and useful
Good work done with clear explanations. Thank you.
Thanks so much for the write-up, it’s really good.
Thanks madam . It is very important .
thank you very good
This has been very well explained in simple language . It is useful even for a new researcher.
Great to hear that. Good luck with your qualitative data analysis, Pramod!
This is very useful information. And it was very a clear language structured presentation. Thanks a lot.
Thank you so much.
very informative sequential presentation
Precise explanation of method.
Hi, may we use 2 data analysis methods in our qualitative research?
Thanks for your comment. Most commonly, one would use one type of analysis method, but it depends on your research aims and objectives.
You explained it in very simple language, everyone can understand it. Thanks so much.
Thank you very much, this is very helpful. It has been explained in a very simple manner that even a layman understands
Thank nicely explained can I ask is Qualitative content analysis the same as thematic analysis?
Thanks for your comment. No, QCA and thematic are two different types of analysis. This article might help clarify – https://onlinelibrary.wiley.com/doi/10.1111/nhs.12048
This is my first time to come across a well explained data analysis. so helpful.
I have thoroughly enjoyed your explanation of the six qualitative analysis methods. This is very helpful. Thank you!
Thank you very much, this is well explained and useful
i need a citation of your book.
Thanks a lot , remarkable indeed, enlighting to the best
Hi Derek, What other theories/methods would you recommend when the data is a whole speech?
Keep writing useful artikel.
It is important concept about QDA and also the way to express is easily understandable, so thanks for all.
Thank you, this is well explained and very useful.
Very helpful .Thanks.
Hi there! Very well explained. Simple but very useful style of writing. Please provide the citation of the text. warm regards
The session was very helpful and insightful. Thank you
This was very helpful and insightful. Easy to read and understand
As a professional academic writer, this has been so informative and educative. Keep up the good work Grad Coach you are unmatched with quality content for sure.
Keep up the good work Grad Coach you are unmatched with quality content for sure.
Its Great and help me the most. A Million Thanks you Dr.
It is a very nice work
Very insightful. Please, which of this approach could be used for a research that one is trying to elicit students’ misconceptions in a particular concept ?
This is Amazing and well explained, thanks
great overview
What do we call a research data analysis method that one use to advise or determining the best accounting tool or techniques that should be adopted in a company.
Informative video, explained in a clear and simple way. Kudos
Waoo! I have chosen method wrong for my data analysis. But I can revise my work according to this guide. Thank you so much for this helpful lecture.
This has been very helpful. It gave me a good view of my research objectives and how to choose the best method. Thematic analysis it is.
Very helpful indeed. Thanku so much for the insight.
This was incredibly helpful.
Very helpful.
very educative
Nicely written especially for novice academic researchers like me! Thank you.
choosing a right method for a paper is always a hard job for a student, this is a useful information, but it would be more useful personally for me, if the author provide me with a little bit more information about the data analysis techniques in type of explanatory research. Can we use qualitative content analysis technique for explanatory research ? or what is the suitable data analysis method for explanatory research in social studies?
that was very helpful for me. because these details are so important to my research. thank you very much
I learnt a lot. Thank you
Relevant and Informative, thanks !
Well-planned and organized, thanks much! 🙂
I have reviewed qualitative data analysis in a simplest way possible. The content will highly be useful for developing my book on qualitative data analysis methods. Cheers!
Clear explanation on qualitative and how about Case study
This was helpful. Thank you
This was really of great assistance, it was just the right information needed. Explanation very clear and follow.
Wow, Thanks for making my life easy
This was helpful thanks .
Very helpful…. clear and written in an easily understandable manner. Thank you.
This was so helpful as it was easy to understand. I’m a new to research thank you so much.
so educative…. but Ijust want to know which method is coding of the qualitative or tallying done?
Thank you for the great content, I have learnt a lot. So helpful
precise and clear presentation with simple language and thank you for that.
very informative content, thank you.
You guys are amazing on YouTube on this platform. Your teachings are great, educative, and informative. kudos!
Brilliant Delivery. You made a complex subject seem so easy. Well done.
Beautifully explained.
Thanks a lot
Is there a video the captures the practical process of coding using automated applications?
Thanks for the comment. We don’t recommend using automated applications for coding, as they are not sufficiently accurate in our experience.
content analysis can be qualitative research?
THANK YOU VERY MUCH.
Thank you very much for such a wonderful content
do you have any material on Data collection
What a powerful explanation of the QDA methods. Thank you.
Great explanation both written and Video. i have been using of it on a day to day working of my thesis project in accounting and finance. Thank you very much for your support.
very helpful, thank you so much
Submit a Comment Cancel reply
Your email address will not be published. Required fields are marked *
Save my name, email, and website in this browser for the next time I comment.
- Print Friendly
Case Study Research in Software Engineering: Guidelines and Examples by Per Runeson, Martin Höst, Austen Rainer, Björn Regnell
Get full access to Case Study Research in Software Engineering: Guidelines and Examples and 60K+ other titles, with a free 10-day trial of O'Reilly.
There are also live events, courses curated by job role, and more.
DATA ANALYSIS AND INTERPRETATION
5.1 introduction.
Once data has been collected the focus shifts to analysis of data. It can be said that in this phase, data is used to understand what actually has happened in the studied case, and where the researcher understands the details of the case and seeks patterns in the data. This means that there inevitably is some analysis going on also in the data collection phase where the data is studied, and for example when data from an interview is transcribed. The understandings in the earlier phases are of course also valid and important, but this chapter is more focusing on the separate phase that starts after the data has been collected.
Data analysis is conducted differently for quantitative and qualitative data. Sections 5.2 – 5.5 describe how to analyze qualitative data and how to assess the validity of this type of analysis. In Section 5.6 , a short introduction to quantitative analysis methods is given. Since quantitative analysis is covered extensively in textbooks on statistical analysis, and case study research to a large extent relies on qualitative data, this section is kept short.
5.2 ANALYSIS OF DATA IN FLEXIBLE RESEARCH
5.2.1 introduction.
As case study research is a flexible research method, qualitative data analysis methods are commonly used [176]. The basic objective of the analysis is, as in any other analysis, to derive conclusions from the data, keeping a clear chain of evidence. The chain of evidence means that a reader ...
Get Case Study Research in Software Engineering: Guidelines and Examples now with the O’Reilly learning platform.
O’Reilly members experience books, live events, courses curated by job role, and more from O’Reilly and nearly 200 top publishers.
Don’t leave empty-handed
Get Mark Richards’s Software Architecture Patterns ebook to better understand how to design components—and how they should interact.
It’s yours, free.
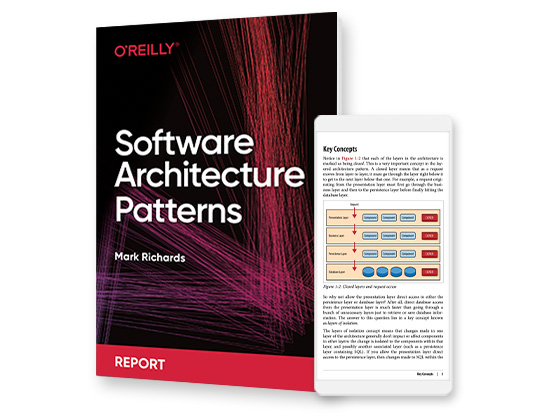
Check it out now on O’Reilly
Dive in for free with a 10-day trial of the O’Reilly learning platform—then explore all the other resources our members count on to build skills and solve problems every day.
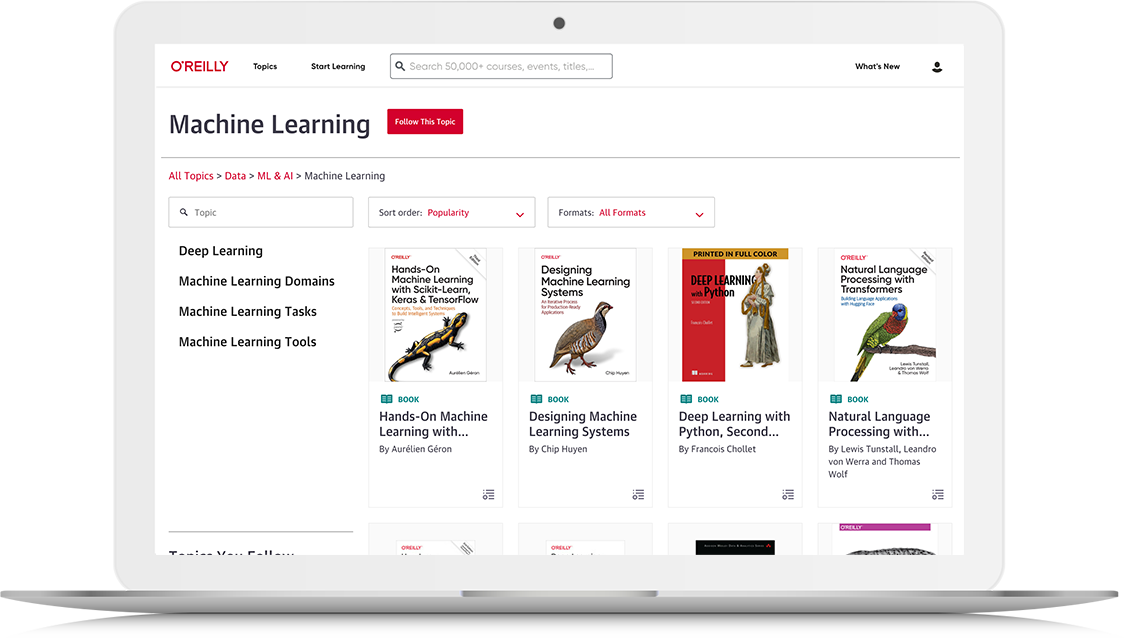
- Cancer Nursing Practice
- Emergency Nurse
- Evidence-Based Nursing
- Learning Disability Practice
- Mental Health Practice
- Nurse Researcher
- Nursing Children and Young People
- Nursing Management
- Nursing Older People
- Nursing Standard
- Primary Health Care
- RCN Nursing Awards
- Nursing Live
- Nursing Careers and Job Fairs
- CPD webinars on-demand
- --> Advanced -->

- Clinical articles
- Expert advice
- Career advice
- Revalidation
Data analysis Previous Next
Qualitative case study data analysis: an example from practice, catherine houghton lecturer, school of nursing and midwifery, national university of ireland, galway, republic of ireland, kathy murphy professor of nursing, national university of ireland, galway, ireland, david shaw lecturer, open university, milton keynes, uk, dympna casey senior lecturer, national university of ireland, galway, ireland.
Aim To illustrate an approach to data analysis in qualitative case study methodology.
Background There is often little detail in case study research about how data were analysed. However, it is important that comprehensive analysis procedures are used because there are often large sets of data from multiple sources of evidence. Furthermore, the ability to describe in detail how the analysis was conducted ensures rigour in reporting qualitative research.
Data sources The research example used is a multiple case study that explored the role of the clinical skills laboratory in preparing students for the real world of practice. Data analysis was conducted using a framework guided by the four stages of analysis outlined by Morse ( 1994 ): comprehending, synthesising, theorising and recontextualising. The specific strategies for analysis in these stages centred on the work of Miles and Huberman ( 1994 ), which has been successfully used in case study research. The data were managed using NVivo software.
Review methods Literature examining qualitative data analysis was reviewed and strategies illustrated by the case study example provided.
Discussion Each stage of the analysis framework is described with illustration from the research example for the purpose of highlighting the benefits of a systematic approach to handling large data sets from multiple sources.
Conclusion By providing an example of how each stage of the analysis was conducted, it is hoped that researchers will be able to consider the benefits of such an approach to their own case study analysis.
Implications for research/practice This paper illustrates specific strategies that can be employed when conducting data analysis in case study research and other qualitative research designs.
Nurse Researcher . 22, 5, 8-12. doi: 10.7748/nr.22.5.8.e1307
This article has been subject to double blind peer review
None declared
Received: 02 February 2014
Accepted: 16 April 2014
Case study data analysis - case study research methodology - clinical skills research - qualitative case study methodology - qualitative data analysis - qualitative research
User not found
Want to read more?
Already have access log in, 3-month trial offer for £5.25/month.
- Unlimited access to all 10 RCNi Journals
- RCNi Learning featuring over 175 modules to easily earn CPD time
- NMC-compliant RCNi Revalidation Portfolio to stay on track with your progress
- Personalised newsletters tailored to your interests
- A customisable dashboard with over 200 topics
Alternatively, you can purchase access to this article for the next seven days. Buy now
Are you a student? Our student subscription has content especially for you. Find out more
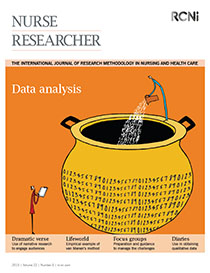
15 May 2015 / Vol 22 issue 5
TABLE OF CONTENTS
DIGITAL EDITION
- LATEST ISSUE
- SIGN UP FOR E-ALERT
- WRITE FOR US
- PERMISSIONS
Share article: Qualitative case study data analysis: an example from practice
We use cookies on this site to enhance your user experience.
By clicking any link on this page you are giving your consent for us to set cookies.
To read this content please select one of the options below:
Please note you do not have access to teaching notes, a systematic qualitative case study: questions, data collection, nvivo analysis and saturation.
Qualitative Research in Organizations and Management
ISSN : 1746-5648
Article publication date: 20 August 2020
Issue publication date: 23 February 2021
The study aims to explore the case study method with the formation of questions, data collection procedures and analysis, followed by how and on which position the saturation is achieved in developing a centralized Shariah governance framework for Islamic banks in Bangladesh.
Design/methodology/approach
Using purposive and snowball sampling procedures, data have been collected from 17 respondents who are working in the central bank and Islamic banks of Bangladesh through face-to-face and semi-structured interviews.
The study claims that researchers can form the research questions by using “what” question mark in qualitative research. Besides, the qualitative research and case study could explore the answers of “what” questions along with the “why” and “how” more broadly, descriptively and extensively about a phenomenon. Similarly, saturation can be considered attaining the ultimate point of data collection by the researchers without adding anything in the databank. Overall, this study proposes three stages of saturation: First, information redundancy. Second, referring the respondents (already considered in the study) without knowing anything about the data collection and their responses. Third, through the NVivo open coding process due to the decrease of reference or quotes in a certain position or in the saturation position as a result of fewer outcomes or insufficient information. The saturation is thus achieved in the diversified positions, i.e. three respondents for regulatory, nine for Shariah scholars and officers and five for the experts concerning the responses and respondents.
Research limitations/implications
The study has potential implications on the qualitative research method, including the case study, saturation process and points, NVivo analysis and qualitative questions formation.
Originality/value
This research defines a case study with the inclusion of “what” and illustrates the saturation process in diverse positions. The qualitative research questions can also be formed with “what” in addition “why” and “how”.
- Qualitative research
Acknowledgements
The author would like to thank both Editors, Associate Editor Dr. Amon Barros and anonymous reviewers for their valuable time, constructive comments and suggestions for the improvement of the manuscript. A special thanks to Dr. Mohd Mursyid Arshad, Senior Lecturer at Faculty of Educational Studies, Universiti Putra Malaysia, Malaysia for his suggestions and unconditional help in the NVivo data analysis process of this research project. The study does not receive any specific fund or research grant.
Alam, M.K. (2021), "A systematic qualitative case study: questions, data collection, NVivo analysis and saturation", Qualitative Research in Organizations and Management , Vol. 16 No. 1, pp. 1-31. https://doi.org/10.1108/QROM-09-2019-1825
Emerald Publishing Limited
Copyright © 2020, Emerald Publishing Limited
Related articles
We’re listening — tell us what you think, something didn’t work….
Report bugs here
All feedback is valuable
Please share your general feedback
Join us on our journey
Platform update page.
Visit emeraldpublishing.com/platformupdate to discover the latest news and updates
Questions & More Information
Answers to the most commonly asked questions here
Thank you for visiting nature.com. You are using a browser version with limited support for CSS. To obtain the best experience, we recommend you use a more up to date browser (or turn off compatibility mode in Internet Explorer). In the meantime, to ensure continued support, we are displaying the site without styles and JavaScript.
- View all journals
- My Account Login
- Explore content
- About the journal
- Publish with us
- Sign up for alerts
- Open access
- Published: 01 April 2024
Adaptive neighborhood rough set model for hybrid data processing: a case study on Parkinson’s disease behavioral analysis
- Imran Raza 1 ,
- Muhammad Hasan Jamal 1 ,
- Rizwan Qureshi 1 ,
- Abdul Karim Shahid 1 ,
- Angel Olider Rojas Vistorte 2 , 3 , 4 ,
- Md Abdus Samad 5 &
- Imran Ashraf 5
Scientific Reports volume 14 , Article number: 7635 ( 2024 ) Cite this article
151 Accesses
Metrics details
- Computational biology and bioinformatics
- Machine learning
Extracting knowledge from hybrid data, comprising both categorical and numerical data, poses significant challenges due to the inherent difficulty in preserving information and practical meanings during the conversion process. To address this challenge, hybrid data processing methods, combining complementary rough sets, have emerged as a promising approach for handling uncertainty. However, selecting an appropriate model and effectively utilizing it in data mining requires a thorough qualitative and quantitative comparison of existing hybrid data processing models. This research aims to contribute to the analysis of hybrid data processing models based on neighborhood rough sets by investigating the inherent relationships among these models. We propose a generic neighborhood rough set-based hybrid model specifically designed for processing hybrid data, thereby enhancing the efficacy of the data mining process without resorting to discretization and avoiding information loss or practical meaning degradation in datasets. The proposed scheme dynamically adapts the threshold value for the neighborhood approximation space according to the characteristics of the given datasets, ensuring optimal performance without sacrificing accuracy. To evaluate the effectiveness of the proposed scheme, we develop a testbed tailored for Parkinson’s patients, a domain where hybrid data processing is particularly relevant. The experimental results demonstrate that the proposed scheme consistently outperforms existing schemes in adaptively handling both numerical and categorical data, achieving an impressive accuracy of 95% on the Parkinson’s dataset. Overall, this research contributes to advancing hybrid data processing techniques by providing a robust and adaptive solution that addresses the challenges associated with handling hybrid data, particularly in the context of Parkinson’s disease analysis.
Similar content being viewed by others
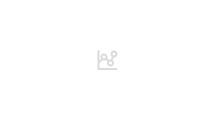
Soft ordered double quantitative approximations based three-way decisions and their applications
Gustavo Santos-García, Abbas Ali & Noor Rehman
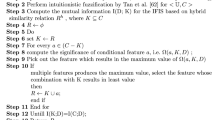
Hybrid similarity relation based mutual information for feature selection in intuitionistic fuzzy rough framework and its applications
Anoop Kumar Tiwari, Rajat Saini, … Mohd Asif Shah
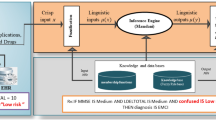
A dynamic fuzzy rule-based inference system using fuzzy inference with semantic reasoning
Nora Shoaip, Shaker El-Sappagh, … Mohammed Elmogy
Introduction
The advancement of technology has facilitated the accumulation of vast amounts of data from various sources such as databases, web repositories, and files, necessitating robust tools for analysis and decision-making 1 , 2 . Data mining, employing techniques such as support vector machine (SVM), decision trees, neural networks, clustering, fuzzy logic, and genetic algorithms, plays a pivotal role in extracting information and uncovering hidden patterns within the data 3 , 4 . However, the complexity of the data landscape, characterized by high dimensionality, heterogeneity, and non-traditional structures, renders the data mining process inherently challenging 5 , 6 . To tackle these challenges effectively, a combination of complementary and cooperative intelligent techniques, including SVM, fuzzy logic, probabilistic reasoning, genetic algorithms, and neural networks, has been advocated 7 , 8 .
Hybrid intelligent systems, amalgamating various intelligent techniques, have emerged as a promising approach to enhance the efficacy of data mining. Adaptive neuro-fuzzy inference systems (ANFIS) have laid the groundwork for intelligent systems in data mining techniques, providing a foundation for exploring complex data relationships 7 , 8 . Moreover, the theory of rough sets has found practical application in tasks such as attribute selection, data reduction, decision rule generation, and pattern extraction, contributing to the development of intelligent systems for knowledge discovery 7 , 8 . Extracting meaningful knowledge from hybrid data, which encompasses both categorical and numerical data, presents a significant challenge. Two predominant strategies have emerged to address this challenge 9 , 10 . The first strategy involves employing numerical data processing techniques such as Principal Component Analysis (PCA) 11 , 12 , Neural Networks 13 , 14 , 15 , 16 , and SVM 17 . However, this approach necessitates converting categorical data into numerical equivalents, leading to a loss of contextual meaning 18 , 19 . The second strategy leverages rough set theory alongside methods tailored for categorical data. Nonetheless, applying rough set theory to numerical data requires a discretization process, resulting in information loss 20 , 21 . Numerous hybrid data processing methods have been proposed, combining rough sets and fuzzy sets to handle uncertainty 22 , 23 , 24 , 25 , 26 , 27 , 28 , 29 , 30 , 31 , 32 , 33 , 34 , 35 , 36 , 37 , 38 , 39 , 40 , 41 . However, selecting an appropriate rough set model for a given dataset necessitates exploring the inherent relationships among existing models, presenting a challenge for users. The selection and utilization of an appropriate model in data mining thus demand qualitative and quantitative comparisons of existing hybrid data processing models.
This research endeavors to present a comprehensive analysis of hybrid data processing models, with a specific focus on those rooted in neighborhood rough sets (NRS). By investigating the inherent interconnections among these models, this study aims to elucidate their complex dynamics. To address the challenges posed by hybrid data, a novel hybrid model founded on NRS is introduced. This model enhances the efficiency of the data mining process without discretization mitigating information loss and ambiguity in data interpretation. Notably, the adaptability of the proposed model, particularly in adjusting the threshold value governing the neighborhood approximation space, ensures optimal performance aligned with dataset characteristics while maintaining high accuracy. A dedicated testbed tailored for Parkinson’s patients is developed to evaluate the real-world effectiveness of the proposed approach. Furthermore, a rigorous evaluation of the proposed model is conducted, encompassing both accuracy and overall effectiveness. Encouragingly, the results demonstrate that the proposed scheme surpasses alternative approaches, adeptly managing both numerical and categorical data through an adaptive framework.
The major contributions, listed below, collectively emphasize the innovative hybrid data processing model, the adaptive nature of its thresholding mechanism, and the empirical validation using a Parkinson’s patient testbed, underscoring the relevance and significance of the study’s findings.
Novel Hybrid Data Processing Model: This research introduces a novel hybrid data processing model based on NRS, preserving the practical meaning of both numerical and categorical data types. Unlike conventional methods, it minimizes information loss while optimizing interpretability. The proposed distance function combines Euclidean and Levenshtein distances with weighted calculations and dynamic selection mechanisms to enhance accuracy and realism in neighborhood approximation spaces.
Adaptive Thresholding Mechanism: Another key contribution is the integration of an adaptive thresholding mechanism within the hybrid model. This feature dynamically adjusts the threshold value based on dataset characteristics, ensuring optimal performance and yielding more accurate and contextually relevant results.
Empirical Validation through Parkinson’s Testbed: This research provides a dedicated testbed for analyzing behavioral data from Parkinson’s patients, allowing rigorous evaluation of the proposed hybrid data processing model. Utilizing real-world datasets enhances the model’s practical applicability and advances knowledge in medical data analysis and diagnosis.
The subsequent structure of the paper unfolds as follows: section “ Related work ” delves into the related work. The proposed model is introduced in section “ Adaptive neighborhood rough set model ”, Section “ Instrumentation ” underscores the instrumentation aspect, section “ Result and discussion ” unfolds the presentation of results and ensuing discussions, while section “ Conclusion and future work ” provides the concluding remarks for the paper. A list of notations used in this study is provided in Table 1 .
Related work
Rough set-based approaches have been utilized in various applications like bankruptcy prediction 42 , attribute/feature subset selection 43 , 44 , cancer prediction 45 , 46 , etc. In addition, recently, several innovative hybrid models have emerged, blending the realms of fuzzy logic and non-randomized systems (NRSs). One such development is presented by Yin et al. 47 , who introduce a parameterized hybrid fuzzy similarity relation. They apply this relation to the task of granulating multilabel data, subsequently extending it to the domain of multilabel learning. To construct a noise-tolerant multilabel fuzzy NRS model (NT-MLFNRS), they leverage the inclusion relationship between fuzzy neighborhood granules and fuzzy decisions. Building upon NT-MLFNRS, Yin et al. also devise a noise-resistant heuristic multilabel feature selection (NRFSFN) algorithm. To further enhance the efficiency of feature selection and address the complexities associated with handling large-scale multilabel datasets, they culminate their efforts by introducing an efficient extended version of NRFSFN known as ENFSFN.
Sang et al. 48 explore incremental feature selection methodologies, introducing a novel conditional entropy metric tailored for dynamic ordered data robustness. Their approach introduces the concept of a fuzzy dominance neighborhood rough set (FDNRS) and defines a conditional entropy metric with robustness, leveraging the FDNRS model. This metric serves as an evaluation criterion for features, and it is integrated into a heuristic feature selection algorithm. The resulting incremental feature selection algorithm is built upon this innovative model
Wang et al. 19 introduced the Fuzzy Rough Iterative Computational (FRIC) model, addressing challenges in hybrid information systems (HIS). Their framework includes a specialized distance function for object sets, enhancing object differentiation precision within HIS. Utilizing this function, they establish fuzzy symmetric relations among objects to formulate fuzzy rough approximations. Additionally, they introduce evaluation functions like fuzzy positive regions, dependency functions, and attribute importance functions to assess classification capabilities of attribute sets. They developed an attribute reduction algorithm tailored for hybrid data based on FRIC principles. This work contributes significantly to HIS analysis, providing a robust framework for data classification and feature selection in complex hybrid information systems.
Xu et al. 49 introduced a novel Fitting Fuzzy Rough Set (FRS) model enriched with relative dependency complement mutual information. This model addresses challenges related to data distribution and precision enhancement of fuzzy information granules. They utilized relative distance to mitigate the influence of data distribution on fuzzy similarity relationships and introduced a fitting fuzzy neighborhood radius optimized for enhancing the precision of fuzzy information granules. Within this model, the authors conducted a comprehensive analysis of information uncertainty, introducing definitions of relative complement information entropy and formulating a multiview uncertainty measure based on relative dependency complement mutual information. This work significantly advances our understanding of managing information uncertainty within FRS models, making a valuable contribution to computational modeling and data analysis.
Jiang et al. 50 presented an innovative approach for multiattribute decision-making (MADM) rooted in PROMETHEE II methodologies. Building upon the NRS model, they introduce two additional variants of covering-based variable precision fuzzy rough sets (CVPFRSs) by applying fuzzy logical operators, specifically type-I CVPFRSs and type-II CVPFRSs. In the context of MADM, their method entails the selection of medicines using an algorithm that leverages the identified features.
Qu et al. 51 introduced the concept of Adaptive Neighborhood Rough Sets (ANRSs), aiming for effective integration of feature separation and linkage with classification. They utilize the mRMR-based Feature Selection Algorithm (FSRMI), demonstrating outstanding performance across various selected datasets. However, it’s worth noting that FSRMI may not consistently outperform other algorithms on all datasets.
Xu et al. 52 introduced the Fuzzy Neighborhood Joint Entropy Model (FNSIJE) for feature selection, leveraging fuzzy neighborhood self-information measures and joint entropy to capture combined feature information. FNSIJE comprehensively analyzes the neighborhood decision system, considering noise, uncertainty, and ambiguity. To improve classification performance, the authors devised a new forward search method. Experimental results demonstrated the effectiveness of FNSIJE-KS, efficiently selecting fewer features for both low-dimensional UCI datasets and high-dimensional gene datasets while maintaining optimal classification performance. This approach advances feature selection techniques in machine learning and data analysis.
In 53 , the authors introduced a novel multi-label feature selection method utilizing fuzzy NRS to optimize classification performance in multi-label fuzzy neighborhood decision systems. By combining the NRS and FRS models a Multi-Label Fuzzy NRS model is introduced. They devised a fuzzy neighborhood approximation accuracy metric and crafted a hybrid metric integrating fuzzy neighborhood approximate accuracy with fuzzy neighborhood conditional entropy for attribute importance evaluation. Rigorous evaluation of their methods across ten diverse multi-label datasets showcased significant progress in multi-label feature selection techniques, promising enhanced classification performance in complex multi-label scenarios.
Sanget et al. 54 introduced the Fuzzy Dominance Neighborhood Rough Set (NRS) model for Interval-Valued Ordered Decision Systems (IvODS), along with a robust conditional entropy measure to assess monotonic consistency within IvODS. They also presented two incremental feature selection algorithms. Experimental results on nine publicly available datasets showcased the robustness of their proposed metric and the effectiveness and efficiency of the incremental algorithms, particularly in dynamic IvODS updates. This research significantly advances the application of fuzzy dominance NRS models in IvODS scenarios, providing valuable insights for data analysis and decision-making processes.
Zheng et al. 55 generalized the FRSs using axiomatic and constructive approaches. A pair of dual generalized fuzzy approximation operators is defined using arbitrary fuzzy relation in the constructive approach. Different classes of FRSs are characterized using different sets of axioms. The postulates governing fuzzy approximation operators ensure the presence of specific categories of fuzzy relations yielding identical operators. Using a generalized FRS model, Hu et al. 18 introduced an efficient algorithm for hybrid attribute reduction based on fuzzy relations constructing a forward greedy algorithm for hybrid attribute reduction resulting in optimal classification performance with lesser selected features and higher accuracy. Considering the similarity between two objects, Wang et al. 36 redefine fuzzy upper and lower approximations. The existing concepts of knowledge reduction are extending fuzzy environment resulting in a heuristic algorithm to learn fuzzy rules.
Gogoi et al. 56 use rough set theory for generating decision rules from inconsistent data. The proposed scheme uses indiscernibility relation to find inconsistencies in the data generating minimized and non-redundant rules using lower and upper approximations. The proposed scheme is based on the LEM2 algorithm 57 which performs the local covering option for generating minimum and non-redundant sets of classification rules and does not consider the global covering. The scheme is evaluated on a variety of data sets from the UCI Machine Learning Repository. All these data sets are either categorical or numerical having variable feature spaces. The proposed scheme performs consistently better for categorical data sets, as it is designed to handle inconsistencies in the data having at least one inconsistency. Results show that the proposed scheme generates minimized rule without reducing the feature space unlike other schemes, which compromise the feature space.
In 58 , the authors introduced a novel NRS model to address attribute reduction in noisy systems with heterogeneous attributes. This model extends traditional NRS by incorporating tolerance neighborhood relation and probabilistic theory, resulting in more comprehensive information granules. It evaluates the significance of heterogeneous attributes by considering neighborhood dependency and aims to maximize classification consistency within selected feature spaces. The feature space reduction algorithm employs an incremental approach, adding features while preserving maximal dependency in each round and halting when a new feature no longer increases dependency. This approach selects fewer features than other methods while achieving significantly improved classification performance, demonstrating its effectiveness in attribute reduction for noisy systems.
Zhu et al. 59 propose a fault tolerance scheme combining kernel method, NRS, and statistical features to adaptively select sensitive features. They employ a Gaussian kernel function with NRS to map fault data to a high-dimensional space. Their feature selection algorithm utilizes the hyper-sphere radius in high-dimensional feature space as the neighborhood value, selecting features based on significance measure regardless of the classification algorithm. A wrapper deploys a classification algorithm to evaluate selected features, choosing a subset for optimal classification. Experimental results demonstrate precise determination of the neighborhood value by mapping data into a high-dimensional space using the kernel function and hyper-sphere radius. This methodology proficiently selects sensitive fault features, diagnoses fault types, and identifies fault degrees in rolling bearing datasets.
A neighborhood covering a rough set model for the fuzziness of decision systems is proposed that solves the problem of hybrid decision systems having both fuzzy and numerical attributes 60 . The fuzzy neighborhood relation measures the indiscernibility relation and approximates the universe space using information granules, which deal with fuzzy attributes directly. The experimental results evaluate the influence of neighborhood operator size on the accuracy and attribute reduction of fuzzy neighborhood rough sets. The attribute reduction increases with the increase in the threshold size. A feature will not distinguish any samples and cannot reduce attributes if the neighborhood operator exceeds a certain value.
Hou et al. 61 applied NRS reduction techniques to cancer molecular classification, focusing on gene expression profiles. Their method introduced a novel perspective by using gene occurrence probability in selected gene subsets to indicate tumor classification efficacy. Unlike traditional methods, it integrated both Filters and Wrappers, enhancing classification performance while being computationally efficient. Additionally, they developed an ensemble classifier to improve accuracy and stability without overfitting. Experimental results showed the method achieved high prediction accuracy, identified potential cancer biomarkers, and demonstrated stability in performance.
Table 2 gives a comparison of existing rough set-based schemes for quantitative and qualitative analysis. The comparative parameters include handling hybrid data, generalized NRS, attribute reduction, classification, and accuracy rate. Most of the existing schemes do not handle hybrid data sets without discretization resulting in information loss and a lack of practical meanings. Another parameter to evaluate the effectiveness of the existing scheme is the ability to adapt the threshold value according to the given data sets. Most of the schemes do not adapt threshold values for neighborhood approximation space resulting in variable accuracy rates for different datasets. The end-user has to adjust the value of the threshold for different datasets without understanding its impact in terms of overfitting. Selecting a large threshold value will result in more global rules resulting in poor accuracy. There needs to be a mechanism to adaptively choose the value of the threshold considering both the global and local information without compromising on the accuracy rate. The schemes are also evaluated for their ability to attribute reduction using NRS. This can greatly improve processing time and accuracy by not considering insignificant attributes. The comparative analysis shows that most of the NRS-based existing schemes perform better than many other well-known schemes in terms of accuracy. Most of these schemes have a higher accuracy rate than CART, C4.5, and k NN. This makes the NRS-based schemes a choice for attribute reduction and classification.
Adaptive neighborhood rough set model
The detailed analysis of existing techniques highlights the need for a generalized NRS-based classification technique to handle both categorical and numerical data. The proposed NRS-based techniques not only handle the hybrid information granules but also dynamically select the threshold \(\delta \) producing optimal results with a high accuracy rate. The proposed scheme considers a hybrid tuple \(HIS=\langle U_h,\ Q_h,\ V,\ f \rangle \) , where \(U_h\) is nonempty set of hybrid records \(\{x_{h1},\ x_{h2},\ x_{h3},\ \ldots ,\ x_{hn}\}\) , \(Q_h=\left\{ q_{h1},\ q_{h2},\ \ q_{h3},\ \ldots \,\ q_{hn}\right\} \) is the non-empty set of hybrid features. \( V_{q_h}\) is the domain of attribute \(q_h\) and \(V=\ \cup _{q_h\in Q_h}V_{q_h}\) , and \(f=U_h\ x\ Q_h\rightarrow V\) is a total function such \(f\left( x_h,q_h\right) \in V_{q_h}\) for each \(q_h\in Q_h, x_h\in U_h\) , called information function. \(\langle U_h,\ Q_h,\ V,\ f\rangle \) is also known as a decision table if \(Q_h=C_h\cup D\) , where \(C_h\) is the set of hybrid condition attributes and D is the decision attribute.
A neighborhood relation N is calculated using this set of hybrid samples \(U_h\) creating the neighborhood approximation space \(\langle U_h,\ N\rangle \) which contains information granules \( \left\{ \delta ({x_h}_i)\big |{x_h}_i\in U_h\right\} \) based on some distance function \(\Delta \) . For an arbitrary sample \({x_h}_i\in U_h\) and \(B \subseteq C_h\) , the neighborhood \(\delta _B({x_h}_i)\) of \({x_h}_i\) in the subspace B is defined as \(\delta _B\left( {x_h}_i\right) =\{{x_h}_j\left| {x_h}_j\right. \in U_h,\ \Delta B(x_i,x_j) \le \delta \}\) . The scheme proposes a new hybrid distance function to handle both the categorical and numerical features in an approximation space.
The proposed distance function uses Euclidean distance for numerical features and Levenshtein distance for categorical features. The distance function also takes care of the significant features calculating weighted distance for both the categorical and numerical features. The proposed algorithm dynamically selects the distance function at the run time. The use of Levenshtein distance for categorical features provides precise distance for optimal neighborhood approximation space providing better results. Existing techniques add 1 to distance if two strings do not match in calculating the distance for categorical data and add 0 otherwise. This may not result in a realistic neighborhood approximation space.
The neighborhood size depends on the threshold \(\delta \) . The neighborhood will contain more samples if \(\delta \) is greater and results in more rules not considering the local information data. The accuracy rate of the NRS greatly depends on the selection of threshold values. The proposed scheme dynamically calculates the threshold value for any given dataset considering both local and global information. The threshold calculation formula is given below where \({min}_D\) is the minimum distance between the set of training samples and the test sample containing local information and \(R_D\) is the range of distance between the set of training samples and the test sample containing the global information.
The proposed scheme then calculates the lower and upper approximations given a neighborhood space \(\langle U_h, N\rangle \) for \(X \subseteq U_h\) , the lower and upper approximations of X are defined as:
Given a hybrid neighborhood decision table \(HNDT=\langle U_h,\ C_h\cup \ D, V, f\rangle \) , \(\{ X_{h1},X_{h2},\ \ldots ,\ X_{hN} \}\) are the sample hybrid subjects with decision 1 to N , \(\delta _B\left( x_{hi}\right) \) is the information granules generated by attributes \(B \subseteq C_h\) , then the lower and upper approximation is defined as:
and the boundary region of D is defined as:
The lower and upper approximation spaces are the set of rules, which are used to classify a test sample. A test sample forms its neighborhood using a lower approximation having all the rules with a distance less than a dynamically calculated threshold value. The majority voting is used in the neighborhood of a test sample to decide the class of a test sample. K-fold cross-validation is used to measure the accuracy of the proposed scheme where the value k is 10. The algorithm 1 of the proposed scheme has a time complexity of \(O(nm^{2})\) where n is the number of clients and m is the size of the categorial data.
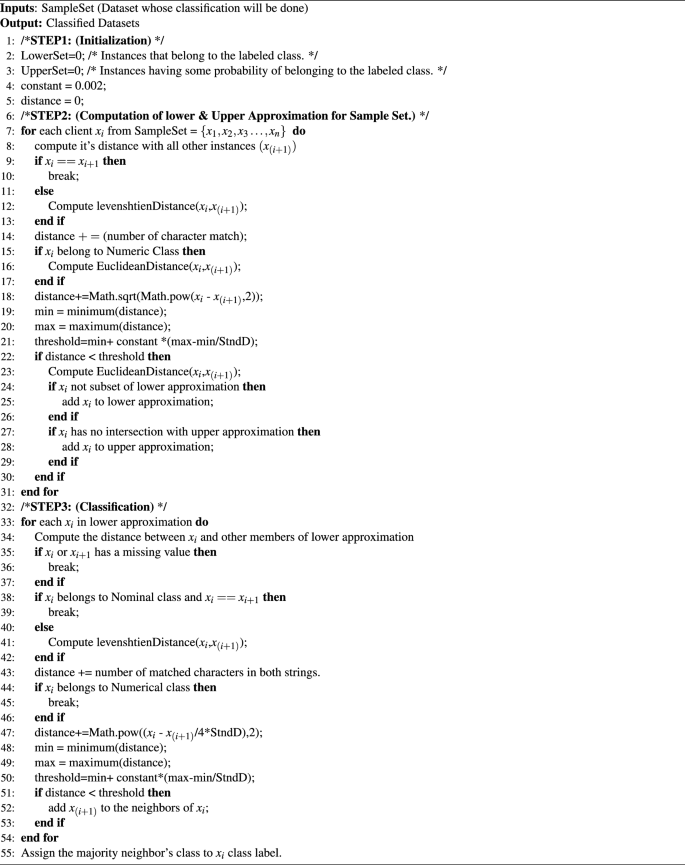
Instrumentation
The proposed generalized rough set model has been rigorously assessed through the development of a testbed designed for the classification of Parkinson’s patients. It has also been subjected to testing using various standard datasets sourced from the University of California at Irvine machine learning data repository 63 . This research underscores the increasing significance of biomedical engineering in healthcare, particularly in light of the growing prevalence of Parkinson’s disease, which ranks as the second most common neurodegenerative condition, impacting over 1% of the population aged 65 and above 64 . The disease manifests through distinct motor symptoms like resting tremors, bradykinesia (slowness of movement), rigidity, and poor balance, with medication-related side effects such as wearing off and dyskinesias 65 .
In this study, to address the need for a reliable quantitative method for assessing motor complications in Parkinson’s patients, the data collection process involves utilizing a home-monitoring system equipped with wireless wearable sensors. These sensors were specifically deployed to closely monitor Parkinson’s patients with severe tremors in real time. It’s important to note that all patients involved in the study were clinically diagnosed with Parkinson’s disease. Additionally, before data collection, proper consent was obtained from each participant, and the study protocol was approved by the ethical committee of our university. The data collected from these sensors is then analyzed, yielding reliable quantitative information that can significantly aid clinical decision-making within both routine patient care and clinical trials of innovative treatments.
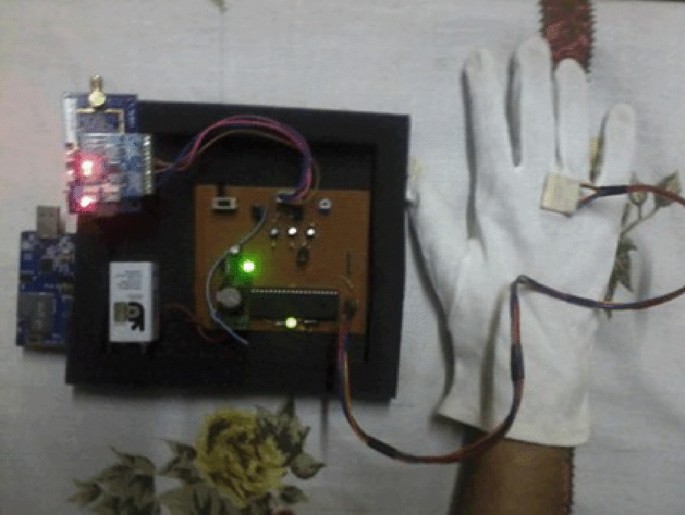
Testbed for Parkinson’s patients.
Figure 1 illustrates a real-time Testbed designed for monitoring Parkinson’s patients. This system utilizes a tri-axial accelerometer to capture three signals, one for each axis \((x,\ y,\ and\ z)\) , resulting in a total of 18 channels of data. The sensors employed in this setup employ ZigBee (IEEE 802.15.4 infrastructure) protocol to transmit data to a computer at a sampling rate of 62.5 Hz. To ensure synchronization of the transmitted signals, a transition protocol is applied. These data packets are received through the Serial Forwarder using the TinyOS platform ( http://www.tinyos.net ). The recorded acceleration data is represented as digital signals and can be visualized on an oscilloscope. The frequency domain data is obtained by applying the Fast Fourier Transform (FFT) to the signal, resulting in an ARFF file format that is then employed for classification purposes. The experimental flowchart is shown in Fig. 2 .

Experimental flowchart.
The real-time testbed includes various components to capture data using the Unified Parkinson’s Disease Rating Scale (UPDRS). TelosB MTM-CM5000-MSP and MTM-CM3000-MSP sensors are used to send and receive radio signals from the sensor to the PC. These sensors are based on an open-source TelosB/Tmote Sky platform, designed and developed by the University of California, Berkeley.
TelosB sensor uses the IEEE 802.15.4 wireless structure and the embedded sensors can measure temperature, relative humidity, and light. In CM3000, the USB connector is replaced with an ERNI connector that is compatible with interface modules. Also, the Hirose 51-pin connector makes this more versatile as it can be attachable to any sensor board family, and the coverage area is increased using SMA design by a 5dBi external antenna 66 . These components can be used for a variety of applications such as low-power Wireless Sensor Networks (WSN) platforms, network monitoring, and environment monitoring systems.
MTS-EX1000 sensor board is used for the amplification of the voltage/current value from the accelerometer. The EX1000 is an attachable board that supports the CMXXXX series of wireless sensors network Motes (Hirose 51-pin connector). The basic functionality of EX1000 is to connect the external sensors with CMXX00 communication modules to enhance the mote’s I/O capability and support different kinds of sensors based on the sensor type and its output signal. ADXL-345 Tri-accelerometer sensor is used to calculate body motion along x, y, and z-axis relative to gravity. It is a small, thin, low-power, 3-axis accelerometer that calculates high resolution (13-bit) measurements at up to ±16g. Its digital output, in 16-bit twos complement format, is accessible through either an SPI (3- or 4-wire) or I2C digital interface. A customized main circuit board is used having a programmed IC, registers, and transistors. Its basic functionality is to convert the digital data, accessed through the ADXL-345 sensor, into analog form and send it to MTS1000.
Result and discussion
The proposed generalized and ANRS is evaluated against different data sets taken from the machine learning data repository, at the University of California at Irvine. In addition to these common data sets, a real-time Testbed for Parkinson’s patients is also used to evaluate the proposed scheme. The hybrid data of 500 people was collected using the Testbed for Parkinson’s patients including 10 Parkinson’s patients, 20 people have abnormal and uncontrolled hand movements, and the rest of the samples were taken approximating the hand movements of Parkinson’s patients. The objective of this evaluation is to compare the accuracy rate of the proposed scheme with CART, k NN, and SVM having both simple and complex datasets containing numerical and hybrid features respectively. The results also demonstrate the selection of radius r for dynamically calculating the threshold value.
Table 3 provides the details of the datasets used for the evaluation of the proposed scheme including the training and test ratio used for evaluation in addition to data type, total number of instances, total feature, a feature considered for evaluation, and number of classes. The hybrid datasets are also selected to evaluate to performance of the proposed scheme against the hybrid feature space without discretization preventing information loss.
The accuracy of the NRS is greatly dependent on the threshold value. Most of the existing techniques do not dynamically adapt the threshold \(\delta \) value for different hybrid datasets. This results in the variant of NRS suitable for specific datasets with different threshold values. A specific threshold value may produce better results for one dataset and poor results for others requiring a more generic threshold value catering to different datasets with optimal results. The proposed scheme introduces an adaptable threshold calculation mechanism to achieve optimal results regardless of the datasets under evaluation. The radius value plays a pivotal role in forming a neighborhood, as the threshold values consider both the local and global information of the NRS to calculate neighborhood approximation space. Table 4 shows the accuracy rate having different values of the radius of the NRS. The proposed threshold mechanism provides better results for all datasets if the value of the radius is 0.002. Results also show that assigning no weight to the radius produces poor results, as it will then only consider the local information for the approximation space. Selecting other weights for radius may produce better results for one dataset but not for all datasets.
Table 5 presents the comparative analysis of the proposed scheme with k NN, Naive Bayes, and C45. The results show that the proposed scheme performs well against other well-known techniques for both the categorical and numerical features space. Naive Bayes and C45 also result in information loss, as these techniques cannot process the hybrid data. So the proposed scheme handles the hybrid data without compromising on the information completeness producing acceptable results. K-fold cross-validation is used to measure the accuracy of the proposed scheme. Each dataset is divided into 10 subsets to use one of the K subsets as the test set and the other K-1 subsets as training sets. Then the average accuracy of all K trials is computed with the advantage of having results regardless of the dataset division.
Conclusion and future work
This work evaluates the existing NRS-based scheme for handling hybrid data sets i.e. numerical and categorical features. The comparative analysis of existing NRS-based schemes shows that there is a need for a generic NRS-based approach to adapt the threshold selection forming neighborhood approximation space. A generalized and ANRS-based scheme is proposed to handle both the categorical and numerical features avoiding information loss and lack of practical meanings. The proposed scheme uses a Euclidean and Levenshtein distance to calculate the upper and lower approximation of NRS for numerical and categorical features respectively. Euclidean and Levenshtein distances have been modified to handle the impact of outliers in calculating the approximation spaces. The proposed scheme defines an adaptive threshold mechanism for calculating neighborhood approximation space regardless of the data set under consideration. A Testbed is developed for real-time behavioral analysis of Parkinson’s patients evaluating the effectiveness of the proposed scheme. The evaluation results show that the proposed scheme provides better accuracy than k NN, C4.5, and Naive Bayes for both the categorical and numerical feature space achieving 95% accuracy on the Parkinson’s dataset. The proposed scheme will be evaluated against the hybrid data set having more than two classes in future work. Additionally, in future work, we aim to explore the following areas; (i) conduct longitudinal studies to track the progression of Parkinson’s disease over time, allowing for a deeper understanding of how behavioral patterns evolve and how interventions may impact disease trajectory, (ii) explore the integration of additional data sources, such as genetic data, imaging studies, and environmental factors, to provide a more comprehensive understanding of Parkinson’s disease etiology and progression, (iii) validate our findings in larger and more diverse patient populations and investigate the feasibility of implementing our proposed approach in clinical settings to support healthcare providers in decision-making processes, (iv) investigate novel biomarkers or physiological signals that may provide additional insights into Parkinson’s disease progression and motor complications, potentially leading to the development of new diagnostic and monitoring tools, and (v) conduct patient-centered outcomes research to better understand the impact of Parkinson’s disease on patients’ quality of life, functional abilities, and overall well-being, with a focus on developing personalized treatment approaches.
Data availability
The datasets used in this study are publicly available at the following links:
Bupa 67 : https://doi.org/10.24432/C54G67 , Sonar 68 : https://doi.org/10.24432/C5T01Q , Mammographic Mass 69 : https://doi.org/10.24432/C53K6Z , Haberman’s Survival 70 : https://doi.org/10.24432/C5XK51 , Credit-g 71 : https://doi.org/10.24432/C5NC77 , Lymmography 73 : https://doi.org/10.24432/C54598 , Splice 74 : https://doi.org/10.24432/C5M888 , Optdigits 75 : https://doi.org/10.24432/C50P49 , Pendigits 76 : https://doi.org/10.1137/1.9781611972825.9 , Pageblocks 77 : https://doi.org/10.24432/C5J590 , Statlog 78 : https://doi.org/10.24432/C55887 , Magic04 79 : https://doi.org/10.1609/aaai.v29i1.9277 .
Gaber, M. M. Scientific Data Mining and Knowledge Discovery Vol. 1 (Springer, 2009).
Google Scholar
Hajirahimi, Z. & Khashei, M. Weighting approaches in data mining and knowledge discovery: A review. Neural Process. Lett. 55 , 10393–10438 (2023).
Article Google Scholar
Kantardzic, M. Data Mining: Concepts, Models, Methods, and Algorithms (Wiley, 2011).
Book Google Scholar
Shu, X. & Ye, Y. Knowledge discovery: Methods from data mining and machine learning. Soc. Sci. Res. 110 , 102817 (2023).
Article PubMed Google Scholar
Tan, P.-N., Steinbach, M. & Kumar, V. Introduction to Data Mining (Pearson Education India, 2016).
Khan, S. & Shaheen, M. From data mining to wisdom mining. J. Inf. Sci. 49 , 952–975 (2023).
Engelbrecht, A. P. Computational Intelligence: An Introduction (Wiley, 2007).
Bhateja, V., Yang, X.-S., Lin, J.C.-W. & Das, R. Evolution in computational intelligence. In Evolution (Springer, 2023).
Wei, W., Liang, J. & Qian, Y. A comparative study of rough sets for hybrid data. Inf. Sci. 190 , 1–16 (2012).
Article ADS MathSciNet Google Scholar
Kumari, N. & Acharjya, D. Data classification using rough set and bioinspired computing in healthcare applications—An extensive review. Multimedia Tools Appl. 82 , 13479–13505 (2023).
Martinez, A. M. & Kak, A. C. PCA versus LDA. IEEE Trans. Pattern Anal. Mach. Intell. 23 , 228–233 (2001).
Brereton, R. G. Principal components analysis with several objects and variables. J. Chemom. 37 (4), e3408 (2023).
Article CAS Google Scholar
De, R. K., Basak, J. & Pal, S. K. Neuro-fuzzy feature evaluation with theoretical analysis. Neural Netw. 12 , 1429–1455 (1999).
Talpur, N. et al. Deep neuro-fuzzy system application trends, challenges, and future perspectives: A systematic survey. Artif. Intell. Rev. 56 , 865–913 (2023).
Jang, J.-S.R., Sun, C.-T. & Mizutani, E. Neuro-fuzzy and soft computing—A computational approach to learning and machine intelligence [book review]. IEEE Trans. Autom. Control 42 , 1482–1484 (1997).
Ouifak, H. & Idri, A. Application of neuro-fuzzy ensembles across domains: A systematic review of the two last decades (2000–2022). Eng. Appl. Artif. Intell. 124 , 106582 (2023).
Jung, T. & Kim, J. A new support vector machine for categorical features. Expert Syst. Appl. 229 , 120449 (2023).
Hu, Q., Xie, Z. & Yu, D. Hybrid attribute reduction based on a novel fuzzy-rough model and information granulation. Pattern Recognit. 40 , 3509–3521 (2007).
Article ADS Google Scholar
Wang, P., He, J. & Li, Z. Attribute reduction for hybrid data based on fuzzy rough iterative computation model. Inf. Sci. 632 , 555–575 (2023).
Yeung, D. S., Chen, D., Tsang, E. C., Lee, J. W. & Xizhao, W. On the generalization of fuzzy rough sets. IEEE Trans. Fuzzy Syst. 13 , 343–361 (2005).
Gao, L., Yao, B.-X. & Li, L.-Q. L-fuzzy generalized neighborhood system-based pessimistic l-fuzzy rough sets and its applications. Soft Comput. 27 , 7773–7788 (2023).
Bhatt, R. B. & Gopal, M. On fuzzy-rough sets approach to feature selection. Pattern Recognit. Lett. 26 , 965–975 (2005).
Dubois, D. & Prade, H. Putting fuzzy sets and rough sets together. Intell. Decis. Support 23 , 203–232 (1992).
Jensen, R. & Shen, Q. Fuzzy-rough sets for descriptive dimensionality reduction. In 2002 IEEE World Congress on Computational Intelligence. 2002 IEEE International Conference on Fuzzy Systems. FUZZ-IEEE’02. Proceedings (Cat. No. 02CH37291) , vol. 1, 29–34 (IEEE, 2002).
Pedrycz, W. & Vukovich, G. Feature analysis through information granulation and fuzzy sets. Pattern Recognit. 35 , 825–834 (2002).
Jensen, R. & Shen, Q. Fuzzy-rough sets assisted attribute selection. IEEE Trans. Fuzzy Syst. 15 , 73–89 (2007).
Shen, Q. & Jensen, R. Selecting informative features with fuzzy-rough sets and its application for complex systems monitoring. Pattern Recognit. 37 , 1351–1363 (2004).
Wang, X., Tsang, E. C., Zhao, S., Chen, D. & Yeung, D. S. Learning fuzzy rules from fuzzy samples based on rough set technique. Inf. Sci. 177 , 4493–4514 (2007).
Article MathSciNet Google Scholar
Wei, W., Liang, J., Qian, Y. & Wang, F. An attribute reduction approach and its accelerated version for hybrid data. In 2009 8th IEEE International Conference on Cognitive Informatics , 167–173 (IEEE, 2009).
Yin, T., Chen, H., Li, T., Yuan, Z. & Luo, C. Robust feature selection using label enhancement and \(\beta \) -precision fuzzy rough sets for multilabel fuzzy decision system. Fuzzy Sets Syst. 461 , 108462 (2023).
Yin, T. et al. Exploiting feature multi-correlations for multilabel feature selection in robust multi-neighborhood fuzzy \(\beta \) covering space. Inf. Fusion 104 , 102150 (2024).
Yin, T. et al. A robust multilabel feature selection approach based on graph structure considering fuzzy dependency and feature interaction. IEEE Trans. Fuzzy Syst. 31 , 4516–4528. https://doi.org/10.1109/TFUZZ.2023.3287193 (2023).
Huang, W., She, Y., He, X. & Ding, W. Fuzzy rough sets-based incremental feature selection for hierarchical classification. IEEE Trans. Fuzzy Syst. https://doi.org/10.1109/TFUZZ.2023.3300913 (2023).
Dong, L., Wang, R. & Chen, D. Incremental feature selection with fuzzy rough sets for dynamic data sets. Fuzzy Sets Syst. 467 , 108503 (2023).
Chakraborty, M. K. & Samanta, P. Fuzzy sets and rough sets: A mathematical narrative. In Fuzzy, Rough and Intuitionistic Fuzzy Set Approaches for Data Handling: Theory and Applications , 1–21 (Springer, 2023).
Wang, Z., Chen, H., Yuan, Z. & Li, T. Fuzzy-rough hybrid dimensionality reduction. Fuzzy Sets Syst. 459 , 95–117 (2023).
Xue, Z.-A., Jing, M.-M., Li, Y.-X. & Zheng, Y. Variable precision multi-granulation covering rough intuitionistic fuzzy sets. Granul. Comput. 8 , 577–596 (2023).
Akram, M., Nawaz, H. S. & Deveci, M. Attribute reduction and information granulation in pythagorean fuzzy formal contexts. Expert Systems Appl. 222 , 119794 (2023).
Hu, M., Guo, Y., Chen, D., Tsang, E. C. & Zhang, Q. Attribute reduction based on neighborhood constrained fuzzy rough sets. Knowl. Based Syst. 274 , 110632 (2023).
Zhang, C., Ding, J., Zhan, J., Sangaiah, A. K. & Li, D. Fuzzy intelligence learning based on bounded rationality in IOMT systems: A case study in Parkinson’s disease. IEEE Trans. Comput. Soc. Syst. 10 , 1607–1621. https://doi.org/10.1109/TCSS.2022.3221933 (2023).
Zhang, C. & Zhang, J. Three-way group decisions with incomplete spherical fuzzy information for treating Parkinson’s disease using IOMT devices. Wireless Communications and Mobile Computing , vol. 2022 (2022).
Jain, P., Tiwari, A. K. & Som, T. Improving financial bankruptcy prediction using oversampling followed by fuzzy rough feature selection via evolutionary search. In Computational Management: Applications of Computational Intelligence in Business Management , 455–471 (Springer, 2021).
Shreevastava, S., Singh, S., Tiwari, A. & Som, T. Different classes ratio and Laplace summation operator based intuitionistic fuzzy rough attribute selection. Iran. J. Fuzzy Syst. 18 , 67–82 (2021).
MathSciNet Google Scholar
Shreevastava, S., Tiwari, A. & Som, T. Feature subset selection of semi-supervised data: an intuitionistic fuzzy-rough set-based concept. In Proceedings of International Ethical Hacking Conference 2018: eHaCON 2018, Kolkata, India , 303–315 (Springer, 2019).
Tiwari, A. K., Nath, A., Subbiah, K. & Shukla, K. K. Enhanced prediction for observed peptide count in protein mass spectrometry data by optimally balancing the training dataset. Int. J. Pattern Recognit. Artif. Intell. 31 , 1750040 (2017).
Jain, P., Tiwari, A. K. & Som, T. An intuitionistic fuzzy bireduct model and its application to cancer treatment. Comput. Ind. Eng. 168 , 108124 (2022).
Yin, T., Chen, H., Yuan, Z., Li, T. & Liu, K. Noise-resistant multilabel fuzzy neighborhood rough sets for feature subset selection. Inf. Sci. 621 , 200–226 (2023).
Sang, B., Chen, H., Yang, L., Li, T. & Xu, W. Incremental feature selection using a conditional entropy based on fuzzy dominance neighborhood rough sets. IEEE Trans. Fuzzy Syst. 30 , 1683–1697 (2021).
Xu, J., Meng, X., Qu, K., Sun, Y. & Hou, Q. Feature selection using relative dependency complement mutual information in fitting fuzzy rough set model. Appl. Intell. 53 , 18239–18262 (2023).
Jiang, H., Zhan, J. & Chen, D. Promethee ii method based on variable precision fuzzy rough sets with fuzzy neighborhoods. Artif. Intell. Rev. 54 , 1281–1319 (2021).
Qu, K., Xu, J., Han, Z. & Xu, S. Maximum relevance minimum redundancy-based feature selection using rough mutual information in adaptive neighborhood rough sets. Appl. Intell. 53 , 17727–17746 (2023).
Xu, J., Yuan, M. & Ma, Y. Feature selection using self-information and entropy-based uncertainty measure for fuzzy neighborhood rough set. Complex Intell. Syst. 8 , 287–305 (2022).
Xu, J., Shen, K. & Sun, L. Multi-label feature selection based on fuzzy neighborhood rough sets. Complex Intell. Syst. 8 , 2105–2129 (2022).
Sang, B. et al. Feature selection for dynamic interval-valued ordered data based on fuzzy dominance neighborhood rough set. Knowl. Based Syst. 227 , 107223 (2021).
Wu, W.-Z., Mi, J.-S. & Zhang, W.-X. Generalized fuzzy rough sets. Inf. Sci. 151 , 263–282 (2003).
Gogoi, P., Bhattacharyya, D. K. & Kalita, J. K. A rough set-based effective rule generation method for classification with an application in intrusion detection. Int. J. Secur. Netw. 8 , 61–71 (2013).
Grzymala-Busse, J. W. Knowledge acquisition under uncertainty—A rough set approach. J. Intell. Robot. Syst. 1 , 3–16 (1988).
Jing, S. & She, K. Heterogeneous attribute reduction in noisy system based on a generalized neighborhood rough sets model. World Acad. Sci. Eng. Technol. 75 , 1067–1072 (2011).
Zhu, X., Zhang, Y. & Zhu, Y. Intelligent fault diagnosis of rolling bearing based on kernel neighborhood rough sets and statistical features. J. Mech. Sci. Technol. 26 , 2649–2657 (2012).
Zhao, B.-T. & Jia, X.-F. Neighborhood covering rough set model of fuzzy decision system. Int. J. Comput. Sci. Issues 10 , 51 (2013).
Hou, M.-L. et al. Neighborhood rough set reduction-based gene selection and prioritization for gene expression profile analysis and molecular cancer classification. J Biomed Biotechnol. 2010 , 726413 (2010).
Article PubMed PubMed Central Google Scholar
He, M.-X. & Qiu, D.-D. A intrusion detection method based on neighborhood rough set. TELKOMNIKA Indones. J. Electr. Eng. 11 , 3736–3741 (2013).
ADS Google Scholar
Newman, D. J., Hettich, S., Blake, C. L. & Merz, C. UCI repository of machine learning databases (1998).
Aarsland, D. et al. Parkinson disease-associated cognitive impairment. Nat. Rev. Dis. Primers 7 , 47 (2021).
Lang, A. E. & Lozano, A. M. Parkinson’s disease. N. Engl. J. Med. 339 , 1130–1143 (1998).
Article CAS PubMed Google Scholar
Engin, M. et al. The classification of human tremor signals using artificial neural network. Expert Syst. Appl. 33 , 754–761 (2007).
Liver Disorders. UCI Machine Learning Repository. https://doi.org/10.24432/C54G67 (1990).
Sejnowski, T. & Gorman, R. Connectionist bench (sonar, mines vs. rocks). UCI Machine Learning Repository. https://doi.org/10.24432/C5T01Q
Elter, M. Mammographic Mass. UCI Machine Learning Repository. https://doi.org/10.24432/C53K6Z (2007).
Haberman, S. Haberman’s Survival. UCI Machine Learning Repository. https://doi.org/10.24432/C5XK51 (1999).
Hofmann, H. Statlog (German Credit Data). UCI Machine Learning Repository. https://doi.org/10.24432/C5NC77 (1994).
Kubat, M., Holte, R. C. & Matwin, S. Machine learning for the detection of oil spills in satellite radar images. Mach. Learn. 30 , 195–215 (1998).
Zwitter, M. & Soklic, M. Lymphography. UCI Machine Learning Repository. https://doi.org/10.24432/C54598 (1988).
Molecular Biology (Splice-junction Gene Sequences). UCI Machine Learning Repository. https://doi.org/10.24432/C5M888 (1992).
Alpaydin, E. & Kaynak, C. Optical Recognition of Handwritten Digits. UCI Machine Learning Repository. https://doi.org/10.24432/C50P49 (1998).
Schubert, E., Wojdanowski, R., Zimek, A. & Kriegel, H.-P. On evaluation of outlier rankings and outlier scores. In Proceedings of the 2012 SIAM International Conference on Data Mining , 1047–1058 (SIAM, 2012).
Malerba, D. Page Blocks Classification. UCI Machine Learning Repository. https://doi.org/10.24432/C5J590 (1995).
Srinivasan, A. Statlog (Landsat Satellite). UCI Machine Learning Repository. https://doi.org/10.24432/C55887 (1993).
Rossi, R. A. & Ahmed, N. K. The network data repository with interactive graph analytics and visualization. In AAAI (2015).
Download references
Acknowledgements
This research was funded by the European University of Atlantic.
Author information
Authors and affiliations.
Department of Computer Science, COMSATS University Islamabad, Lahore Campus, Lahore, 54000, Pakistan
Imran Raza, Muhammad Hasan Jamal, Rizwan Qureshi & Abdul Karim Shahid
Universidad Europea del Atlántico, Isabel Torres 21, 39011, Santander, Spain
Angel Olider Rojas Vistorte
Universidad Internacional Iberoamericana Campeche, 24560, Campeche, Mexico
Universidade Internacional do Cuanza, Cuito, Bié, Angola
Department of Information and Communication Engineering, Yeungnam University, Gyeongsan-si, Gyeongsangbuk-do, 38541, South Korea
Md Abdus Samad & Imran Ashraf
You can also search for this author in PubMed Google Scholar
Contributions
Imran Raza: Conceptualization, Formal analysis, Writing—original draft; Muhammad Hasan Jamal: Conceptualization, Data curation, Writing—original draft; Rizwan Qureshi: Data curation, Formal analysis, Methodology; Abdul Karim Shahid: Project administration, Software, Visualization; Angel Olider Rojas Vistorte: Funding acquisition, Investigation, Project administration; Md Abdus Samad: Investigation, Software, Resources; Imran Ashraf: Supervision, Validation, Writing —review and editing. All authors reviewed the manuscript and approved it.
Corresponding authors
Correspondence to Md Abdus Samad or Imran Ashraf .
Ethics declarations
Competing interests.
The authors declare no competing interests.
Additional information
Publisher's note.
Springer Nature remains neutral with regard to jurisdictional claims in published maps and institutional affiliations.
Rights and permissions
Open Access This article is licensed under a Creative Commons Attribution 4.0 International License, which permits use, sharing, adaptation, distribution and reproduction in any medium or format, as long as you give appropriate credit to the original author(s) and the source, provide a link to the Creative Commons licence, and indicate if changes were made. The images or other third party material in this article are included in the article’s Creative Commons licence, unless indicated otherwise in a credit line to the material. If material is not included in the article’s Creative Commons licence and your intended use is not permitted by statutory regulation or exceeds the permitted use, you will need to obtain permission directly from the copyright holder. To view a copy of this licence, visit http://creativecommons.org/licenses/by/4.0/ .
Reprints and permissions
About this article
Cite this article.
Raza, I., Jamal, M.H., Qureshi, R. et al. Adaptive neighborhood rough set model for hybrid data processing: a case study on Parkinson’s disease behavioral analysis. Sci Rep 14 , 7635 (2024). https://doi.org/10.1038/s41598-024-57547-4
Download citation
Received : 01 October 2023
Accepted : 19 March 2024
Published : 01 April 2024
DOI : https://doi.org/10.1038/s41598-024-57547-4
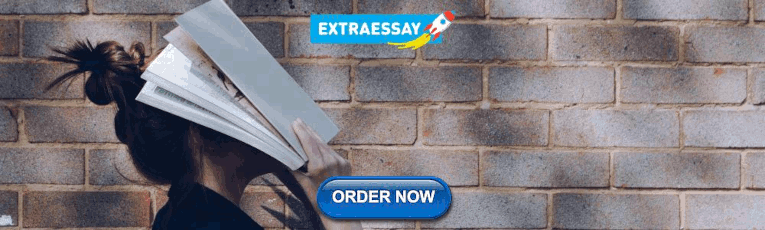
Share this article
Anyone you share the following link with will be able to read this content:
Sorry, a shareable link is not currently available for this article.
Provided by the Springer Nature SharedIt content-sharing initiative
By submitting a comment you agree to abide by our Terms and Community Guidelines . If you find something abusive or that does not comply with our terms or guidelines please flag it as inappropriate.
Quick links
- Explore articles by subject
- Guide to authors
- Editorial policies
Sign up for the Nature Briefing newsletter — what matters in science, free to your inbox daily.

- Open access
- Published: 23 September 2023
Educational interventions targeting pregnant women to optimise the use of caesarean section: What are the essential elements? A qualitative comparative analysis
- Rana Islamiah Zahroh ORCID: orcid.org/0000-0001-7831-2336 1 ,
- Katy Sutcliffe ORCID: orcid.org/0000-0002-5469-8649 2 ,
- Dylan Kneale ORCID: orcid.org/0000-0002-7016-978X 2 ,
- Martha Vazquez Corona ORCID: orcid.org/0000-0003-2061-9540 1 ,
- Ana Pilar Betrán ORCID: orcid.org/0000-0002-5631-5883 3 ,
- Newton Opiyo ORCID: orcid.org/0000-0003-2709-3609 3 ,
- Caroline S. E. Homer ORCID: orcid.org/0000-0002-7454-3011 4 &
- Meghan A. Bohren ORCID: orcid.org/0000-0002-4179-4682 1
BMC Public Health volume 23 , Article number: 1851 ( 2023 ) Cite this article
1227 Accesses
1 Citations
1 Altmetric
Metrics details
Caesarean section (CS) rates are increasing globally, posing risks to women and babies. To reduce CS, educational interventions targeting pregnant women have been implemented globally, however, their effectiveness is varied. To optimise benefits of these interventions, it is important to understand which intervention components influence success. In this study, we aimed to identify essential intervention components that lead to successful implementation of interventions focusing on pregnant women to optimise CS use.
We re-analysed existing systematic reviews that were used to develop and update WHO guidelines on non-clinical interventions to optimise CS. To identify if certain combinations of intervention components (e.g., how the intervention was delivered, and contextual characteristics) are associated with successful implementation, we conducted a Qualitative Comparative Analysis (QCA). We defined successful interventions as interventions that were able to reduce CS rates. We included 36 papers, comprising 17 CS intervention studies and an additional 19 sibling studies (e.g., secondary analyses, process evaluations) reporting on these interventions to identify intervention components. We conducted QCA in six stages: 1) Identifying conditions and calibrating the data; 2) Constructing truth tables, 3) Checking quality of truth tables; 4) Identifying parsimonious configurations through Boolean minimization; 5) Checking quality of the solution; 6) Interpretation of solutions. We used existing published qualitative evidence synthesis to develop potential theories driving intervention success.
We found successful interventions were those that leveraged social or peer support through group-based intervention delivery, provided communication materials to women, encouraged emotional support by partner or family participation, and gave women opportunities to interact with health providers. Unsuccessful interventions were characterised by the absence of at least two of these components.
We identified four key essential intervention components which can lead to successful interventions targeting women to reduce CS. These four components are 1) group-based delivery, 2) provision of IEC materials, 3) partner or family member involvement, and 4) opportunity for women to interact with health providers. Maternal health services and hospitals aiming to better prepare women for vaginal birth and reduce CS can consider including the identified components to optimise health and well-being benefits for the woman and baby.
Peer Review reports
Introduction
In recent years, caesarean section (CS) rates have increased globally [ 1 , 2 , 3 , 4 ]. CS can be a life-saving procedure when vaginal birth is not possible; however, it comes with higher risks both in the short- and long-term for women and babies [ 1 , 5 ]. Women with CS have increased risks of surgical complications, complications in future pregnancies, subfertility, bowel obstruction, and chronic pain [ 5 , 6 , 7 , 8 ]. Similarly, babies born through CS have increased risks of hypoglycaemia, respiratory problems, allergies and altered immunity [ 9 , 10 , 11 ]. At a population level, CS rates exceeding 15% are unlikely to reduce mortality rates [ 1 , 12 ]. Despite these risks, an analysis across 154 countries reported a global average CS rate of 21.1% in 2018, projected to increase to 28.5% by 2030 [ 3 ].
There are many reasons for the increasing CS rates, and these vary between and within countries. Increasingly, non-clinical factors across different societal dimensions and stakeholders (e.g. women and communities, health providers, and health systems) are contributing to this increase [ 13 , 14 , 15 , 16 , 17 ]. Women may prefer CS over vaginal birth due to fear of labour or vaginal birth, previous negative experience of childbirth, perceived increased risks of vaginal birth, beliefs about an auspicious or convenient day of birth, or beliefs that caesarean section is safer, quick, and painless compared to vaginal birth [ 13 , 14 , 15 ].
Interventions targeting pregnant women to reduce CS have been implemented globally. A Cochrane intervention review synthesized evidence from non-clinical interventions targeting pregnant women and family, providers, and health systems to reduce unnecessary CS, and identified 15 interventions targeting women [ 18 ]. Interventions targeting women primarily focused on improving women’s knowledge around birth, improving women’s ability to cope during labour, and decreasing women’s stress related to labour through childbirth education, and decision aids for women with previous CS [ 18 ]. These types of interventions aim to reduce the concerns of pregnant women and their partners around childbirth, and prepare them for vaginal birth.
The effectiveness of interventions targeting women in reducing CS is mixed [ 18 , 19 ]. Plausible explanations for this limited success include the multifactorial nature of the factors driving increases in CS, as well as the contextual characteristics of the interventions, which may include the study environment, participant characteristics, intensity of exposure to the intervention and method of implementation. Understanding which intervention components are essential influencers of the success of the interventions is conducive to optimising benefits. This study used a Qualitative Comparative Analysis (QCA) approach to re-analyse evidence from existing systematic reviews to identify essential intervention components that lead to the successful implementation of non-clinical interventions focusing on pregnant women to optimise the use of CS. Updating and re-analysing existing systematic reviews using new analytical frameworks may help to explore the heterogeneity in effects and ascertain why some studies appear to be effective while others are not.
Data sources, case selection, and defining outcomes
Developing a logic model.
We developed a logic model to guide our understanding of different pathways and intervention components potentially leading to successful implementation (Additional file 1 ). The logic model was developed based on published qualitative evidence syntheses and systematic reviews [ 18 , 20 , 21 , 22 , 23 , 24 ]. The logic model depicts the desired outcome of reduced CS rates in low-risk women (at the time of admission for birth, these women are typically represented by Robson groups 1–4 [ 25 ] and are women with term, cephalic, singleton pregnancies without a previous CS) and works backwards to understand what inputs and processes are needed to achieve the desired outcome. Our logic model shows multiple pathways to success and highlights the interactions between different levels of factors (women, providers, societal, health system) (Additional file 1 ). Based on the logic model, we have separated our QCA into two clusters of interventions: 1) interventions targeting women, and 2) interventions targeting health providers. The results of analysis on interventions targeting health providers have been published elsewhere [ 26 ]. The logic model was also used to inform the potential important components that influence success.
Identifying data sources and selecting cases
We re-analysed the systematic reviews which were used to inform the development and update of World Health Organization (WHO) guidelines. In 2018, WHO issued global guidance on non-clinical interventions to reduce unnecessary CS, with interventions designed to target three different levels or stakeholders: women, health providers, and health systems [ 27 ]. As part of the guideline recommendations, a series of systematic reviews about CS interventions were conducted: 1) a Cochrane intervention review of effectiveness by Chen et al. (2018) [ 18 ] and 2) three qualitative evidence syntheses exploring key stakeholder perspectives and experiences of interventions focusing on women and communities, health professionals, and health organisations, facilities and systems by Kingdon et al. (2018) [ 20 , 21 , 22 ]. Later on, Opiyo and colleagues (2020) published a scoping review of financial and regulatory interventions to optimise the use of CS [ 23 ].
Therefore, the primary data sources of this QCA are the intervention studies included in Chen et al. (2018) [ 18 ] and Opiyo et al. (2020) [ 23 ]. We used these two systematic reviews as not only they are comprehensive, but they were also used to inform the WHO guidelines development. A single intervention study is referred to as a “case”. Eligible cases were intervention studies focusing on pregnant women and aimed to reduce or optimise the use of CS. No restrictions on study design were imposed in the QCA. Therefore, we also assessed the eligibility of intervention studies excluded from Chen et al. (2018) [ 18 ] and Opiyo et al. (2020) [ 23 ] due to ineligible study designs (such as cohort study, uncontrolled before and after study, interrupted time series with fewer than three data points), as these studies could potentially show other pathways to successful implementation. We complemented these intervention studies with additional intervention studies published since the last review updates in 2018 and 2020, to include intervention studies that are likely to meet the review inclusion criteria for future review updates. No further search was conducted as QCA is suitable for medium-N cases, approximately around 10–50 cases, and inclusion of more studies may threaten study rigour [ 28 ].
Once eligible studies were selected, we searched for their ‘sibling studies’. Sibling studies are studies linked to the included intervention studies, such as formative research or process evaluations which may have been published separately. Sibling studies can provide valuable additional information about study context, intervention components, and implementation outcomes (e.g. acceptability, fidelity, adherence, dosage), which may not be well described in a single article about intervention effectiveness. We searched for sibling studies using the following steps: 1) reference list search of the intervention studies included in Chen et al. (2018) [ 18 ] and Opiyo et al. (2020) [ 23 ], 2) reference list search of the qualitative studies included in Kingdon et al. (2018) reviews [ 20 , 21 , 22 ]; and 3) forward reference search of the intervention studies (through “Cited by” function) in Scopus and Web of Science. Sibling studies were included if they included any information on intervention components or implementation outcomes, regardless of the methodology used. One author conducted the study screening independently (RIZ), and 10% of the screening was double-checked by a second author (MAB). Disagreements during screening were discussed until consensus, and with the rest of the author team if needed.
Defining outcomes
We assessed all outcomes related to the mode of birth in the studies included in the Chen et al. (2018) [ 18 ] and Opiyo et al. (2020) [ 23 ] reviews. Based on the consistency of outcome reporting, we selected “overall CS rate” as the primary outcome of interest due to its presence across studies. We planned to rank the rate ratio across these studies to select the 10 most successful and unsuccessful intervention studies. However, due to heterogeneity in how CS outcomes were reported across studies (e.g. odds ratios, rate ratios, percentages across different intervention stages), the final categorisation of successful or unsuccessful interventions is based on whether the CS rate decreased, based on the precision of the confidence interval or p-value (successful, coded as 1), or CS rate increased or did not change (unsuccessful, coded as 0).
Assessing risk of bias in intervention studies
All intervention studies eligible for inclusion were assessed for risk of bias. All studies included in Chen et al. (2018) and Opiyo et al. (2020) already had risk of bias assessed and reported [ 18 , 23 ], and we used these assessments. Additional intervention studies outside the included studies on these reviews were assessed using the same tools depending on the type of evidence (two randomized controlled trials and one uncontrolled before and after study), and details of the risk of bias assessment results can be found in Additional file 2 . We excluded studies with a high risk of bias to ensure that the analysis was based on high-quality studies and to enhance the ability of researchers to develop deep case knowledge by limiting the overall number of studies.
Qualitative comparative analysis (QCA)
QCA was first developed and used in political sciences and has since been extended to systematic reviews of complex health interventions [ 24 , 29 , 30 , 31 ]. Despite the term “qualitative”, QCA is not a typical qualitative analysis, and is often conceptualised as a methodology that bridges qualitative and quantitative methodologies based on its process, data used and theoretical standpoint [ 24 ]. Here, QCA is used to identify if certain configurations or combinations of intervention components (e.g. participants, types of interventions, contextual characteristics, and intervention delivery) are associated with the desired outcome [ 31 ]. These intervention components are referred to as “conditions” in the QCA methodology. Whilst statistical synthesis methods may be used to examine intervention heterogeneity in systematic reviews, such as meta-regression, QCA is a particularly suitable method to understand complex interventions like those aiming to optimise CS, as it allows for multiple overlapping pathways to causality [ 31 ]. Moreover, QCA allows the exploration of different combinations of conditions, rather than relying on a single condition leading to intervention effectiveness [ 31 ]. Although meta-regression allows for the assessment of multiple conditions, a sufficient number of studies may not be available to conduct the analysis. In complex interventions, such as interventions aiming to optimise the use of CS, single condition or standard meta-analysis may be less likely to yield usable and nuanced information about what intervention components are more or less likely to yield success [ 31 ].
QCA uses ‘set theory’ to systematically compare characteristics of the cases (e.g. intervention in the case of systematic reviews) in relation to the outcomes [ 31 , 32 ]. This means QCA compares the characteristics of the successful ‘cases’ (e.g. interventions that are effective) to those unsuccessful ‘cases’ (e.g. interventions that are not effective). The comparison is conducted using a scoring system based on ‘set membership’ [ 31 , 32 ]. In this scoring, conditions and outcomes are coded based on the extent to which a certain feature is present or absent to form set membership scores [ 31 , 32 ]. There are two scoring systems in QCA: 1) crisp set QCA (csQCA) and 2) fuzzy set QCA (fsQCA). csQCA assigns binary scores of 0 (“fully out” to set membership for cases with certain conditions) and 1 (“fully in” to set membership for cases with certain conditions), while fsQCA assigns ordinal scoring of conditions and outcomes, permitting partial membership scores between 0 and 1 [ 31 , 32 ]. For example, using fsQCA we may assign a five-level scoring system (0, 0.33, 0.5, 0.67, 1), where 0.33 would indicate “more out” than “in” to the set of membership, and 0.67 would indicate “more in” than “out”, and 0.5 would indicate ambiguity (i.e. a lack of information about whether a case was “in” or “out”) [ 31 , 32 ]. In our analysis, we used the combination of both csQCA and fsQCA to calibrate our data. This approach was necessary because some conditions were better suited to binary options using csQCA, while others were more complex, depending on the distribution of cases, and required fsQCA to capture the necessary information. In our final analysis, however, the conditions run on the final analysis were all using the csQCA scoring system.
Two relationships can be investigated using QCA [ 24 , 31 ]. First, if all instances of successful interventions share the same condition(s), this suggests these features are ‘necessary’ to trigger successful outcomes [ 24 , 31 ]. Second, if all instances of a particular condition are associated with successful interventions, this suggests these conditions are ‘sufficient’ for triggering successful outcomes [ 24 , 31 ]. In this QCA, we were interested to explore the relationship of sufficiency: that is, to assess the various combinations of intervention components that can trigger successful outcomes. We were interested in sufficiency because our logic model (explained further below) highlighted the multiple pathways that can lead to a CS and different interventions that may optimise the use of CS along those pathways, which suggested that it would be unlikely for all successful interventions to share the same conditions. We calculated the degree of sufficiency using consistency measures, which evaluate the frequency in which conditions are present when the desired outcome is achieved [ 31 , 32 ]. The conditions with a consistency score of at least 0.8 were considered sufficient in triggering successful interventions [ 31 , 32 ]. At present, there is no tool available for reporting guidelines in the re-analysis of systematic reviews using QCA, however, CARU-QCA is currently being developed for this purpose [ 33 ]. QCA was conducted using R programming software with a package developed by Thiem & Duşa (2013) and QCA with R guidebook [ 32 ]. QCA was conducted in six stages based on Thomas et al. (2014) [ 31 ] and explained below.
QCA stage 1: Identifying conditions, building data tables and calibration
We used a deductive and inductive process to determine the potential conditions (intervention components) that may trigger successful implementation. Conditions were first derived deductively using the developed logic model (Additional file 1 ). We then added additional conditions inductively using Intervention Component Analysis from the intervention studies [ 34 ], and qualitative evidence (“view”) synthesis [ 22 ] using Melendez-Torres’s (2018) approach [ 35 ]. Intervention Component Analysis is a methodological approach that examines factors affecting implementation through reflections from the trialist, which is typically presented in the discussion section of a published trial [ 34 ]. Examples of conditions identified in the Intervention Component Analysis include using an individualised approach, interaction with health providers, policies that encourage CS and acknowledgement of women’s previous birth experiences. After consolidating or merging similar conditions, a total of 52 conditions were selected and extracted from each included intervention and analysed in this QCA (Details of conditions and definitions generated for this study can be found in Additional files 3 and 4 ). We adapted the coding framework from Harris et al. (2019) [ 24 ] by adapting coding rules and six domains that were used, to organize the 52 conditions and make more sense of the data. These six domains are broadly classified as 1) context and participants, 2) intervention design, 3) program content, 4) method of engagement, 5) health system factors, and 6) process outcomes.
One author (RIZ) extracted data relevant to the conditions for each included study into a data table, which was then double-reviewed by two other authors (MVC, MAB). The data table is a matrix in which each case is represented in a row, and columns are used to represent the conditions. Following data extraction, calibration rules using either csQCA or fsQCA (e.g. group-based intervention delivery condition: yes = 1 (present), no = 0 (absent)) were developed through consultation with all authors. We developed a table listing the conditions and rules of coding the conditions, by either direct or transformational assignment of quantitative and qualitative data [ 24 , 32 ] (Additional file 3 depicts the calibration rules). The data tables were then calibrated by applying scores, to explore the extent to which interventions have ‘set membership’ with the outcome or conditions of interest. During this iterative process, the calibration criteria were explicitly defined, emerging from the literature and the cases themselves. It is important to note, that maximum ambiguity is typically scored as 0.5 in QCA, however, we decided it would be more appropriate to assume that if a condition was not reported it was unlikely to be a feature of the intervention, so we treated not reported as “absence” that is we coded it 0.
QCA stage 2: Constructing truth tables
Truth tables are an analytical tool used in QCA to analyse associations between configurations of conditions and outcomes. Whereas the data table represents individual cases (rows) and individual conditions (columns) – the truth table synthesises this data to examine configurations – with each row representing a different configuration of the conditions. The columns indicate a) which conditions are featured in the configuration in that row, b) how many of the cases are represented by that configuration, and c) their association with the outcome.
We first constructed the truth tables based on context and participants, intervention designs, program content, and method of engagement; however, no configurations to trigger successful interventions were observed. Instead, we observed limited diversity, meaning there were many instances in which the configurations were unsupported by cases, likely due to the presence of too many conditions in the truth tables. We used the learning from these truth tables to return to the literature to explore potential explanatory theories about what conditions are important from the perspectives of participants and trialists to trigger successful interventions (adhering to the ‘utilisation of view’ perspective [ 35 ]). Through this process, we found that women and communities liked to learn new information about childbirth, and desired emotional support from partners and health providers while learning [ 22 ]. They also appreciated educational interventions that provide opportunities for discussion and dialogue with health providers and align with current clinical practice and advice from health providers [ 22 ]. Therefore, three models of truth tables were iteratively constructed and developed based on three important hypothesised theories about how the interventions should be delivered: 1) how birth information was provided to women, 2) emotional support was provided to women (including interactions between women and providers), and 3) a consolidated model examining the interactions of important conditions identified from model 1 and 2. We also conducted a sub-analysis of interventions targeting both women and health providers or systems (‘multi-target interventions’). This sub-analysis was conducted to explore if similar conditions were observed in triggering successful interventions in multi-target interventions, among the components for women only. Table 1 presents the list of truth tables that were iteratively constructed and refined.
QCA stage 3: Checking quality of truth tables
We iteratively developed and improved the quality of truth tables by checking the configurations of successful and unsuccessful interventions, as recommended by Thomas et al. (2014) [ 31 ]. This includes by assessing the number of studies clustering to each configuration, and exploring the presence of any contradictory results between successful and unsuccessful interventions. We found contradictory configurations across the five truth tables, which were resolved by considering the theoretical perspectives and iteratively refining the truth tables.
QCA stage 4: Identifying parsimonious configurations through Boolean minimization
Once we determined that the truth tables were suitable for further analysis, we used Boolean minimisation to explore pathways resulting in successful intervention through the configurations of different conditions [ 31 ]. We simplified the “complex solution” of the pathways to a “parsimonious solution” and an “intermediate solution” by incorporating logical remainders (configurations where no cases were observed) [ 36 ].
QCA stage 5: Checking the quality of the solution
We presented the intermediate solution as the final solution instead of the most parsimonious solution, as it is most closely aligned with the underlying theory. We checked consistency and coverage scores to assess if the pathways identified were sufficient to trigger success. We also checked the intermediate solution by negating the outcome to see if it predicts the observed solutions.
QCA stage 6: Interpretation of solutions
We iteratively interpreted the results of the findings through discussions among the QCA team. This reflexive approach ensured that the results of the analysis considered the perspectives from the literature discourse, methodological approach, and that the results were coherent with the current understanding of the phenomenon.
Overview of included studies
Out of 79 intervention studies assessed by Chen et al. (2018) [ 18 ] and Opiyo et al. (2020) [ 23 ], 17 intervention studies targeted women and are included, comprising 11 interventions targeting only women [ 37 , 38 , 39 , 40 , 41 , 42 , 43 ] and six interventions targeting both women and health providers or systems [ 44 , 45 , 46 , 47 , 48 , 49 ]. From 17 included studies, 19 sibling studies were identified [ 43 , 49 , 50 , 51 , 52 , 53 , 54 , 55 , 56 , 57 , 58 , 59 , 60 , 61 , 62 , 63 , 64 , 65 , 66 , 67 ]. Thus, a total of 36 papers from 17 intervention studies are included in this QCA (See Fig. 1 : PRISMA Flowchart).
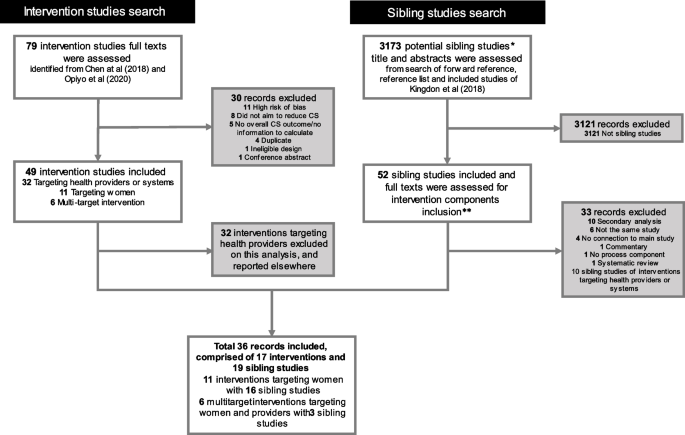
PRISMA flowchart. *Sibling studies: studies that were conducted in the same settings, participants, and timeframe; **Intervention components: information on intervention input, activities, and outputs, including intervention context and other characteristics
The 11 interventions targeting women comprised of five successful interventions [ 37 , 68 , 69 , 70 , 71 ] and six unsuccessful interventions [ 37 , 38 , 39 , 40 , 41 , 42 , 43 ] in reducing CS. Sixteen sibling studies were identified, from five out of 11 included interventions [ 37 , 41 , 43 , 70 , 71 ]. Included studies were conducted in six countries across North America (2 from Canada [ 38 ] and 1 from United States of America [ 71 ]), Asia–Pacific (1 from Australia [ 41 ]), 5 from Iran [ 39 , 40 , 68 , 69 , 70 ]), Europe (2 from Finland [ 37 , 42 ], 1 from United Kingdom [ 43 ]). Six studies were conducted in high-income countries, while five studies were conducted in upper-middle-income countries (all from Iran). All 11 studies targeted women, with three studies also explicitly targeting women’s partners [ 68 , 69 , 71 ]. One study delivering psychoeducation allowed women to bring any family members to accompany them during the intervention but did not specifically target partners [ 37 ]. All 11 studies delivered childbirth education, with four delivering general antenatal education [ 38 , 40 , 68 , 69 ], six delivering psychoeducation [ 37 , 39 , 41 , 42 , 70 , 71 ], and one implementing decision aids [ 43 ]. All studies were included in Chen et al. (2018), and some risks of bias were identified [ 18 ] (Additional file 2).
The multi-target interventions consisted of five successful interventions [ 44 , 45 , 46 , 47 , 48 ] and one unsuccessful intervention [ 49 ]. Sibling studies were only identified from one study [ 48 ]. The interventions were delivered in five countries across: South America (1 from Brazil [ 46 ]), Asia–Pacific (4 from China [ 44 , 45 , 47 , 49 ]), Europe (1 from Italy [ 48 ], 1 from Ireland [ 48 ], and 1 from Germany [ 48 ]). Three studies were conducted in high-income countries and five studies in upper middle-income countries. The multi-target interventions targeted women, health providers and health organisations. For this analysis, however, we only consider the components of the intervention that targeted women, which was typically childbirth education. One study came from Chen et al. (2018) [ 18 ] and was graded as having some concerns [ 47 ], two studies from Opiyo et al. (2020) [ 23 ] were graded as having no serious concerns [ 45 , 46 ], and three studies are newly published studies assessed as low [ 44 ] and some concerns about risk of bias [ 48 , 49 ] Table 2 and 3 show characteristics of included studies.
The childbirth education interventions included information about mode of birth, birth process, mental health and coping strategies, pain relief methods, and partners’ roles in birth. Most interventions were delivered in group settings, and only in three studies they were delivered on a one-to-one basis [ 38 , 41 , 42 ]. Only one study explicitly stated that the intervention was individualised to a woman’s unique needs and experiences [ 38 ].
Overall, there was limited theory used to design interventions among the included studies: less than half of interventions (7/17) explicitly used theory in designing the intervention. Among the seven interventions that used theory in intervention development, the theories included the health promotion-disease prevention framework [ 38 ], midwifery counselling framework [ 41 ], cognitive behavioural therapy [ 42 ], Ost’s applied relaxation [ 70 ], conceptual model of parenting [ 71 ], attachment and social cognitive theories [ 37 ], and healthcare improvement scale-up framework [ 46 ]. The remaining 10 studies only relied on previously published studies to design the interventions. We identified very limited process evaluation or implementation outcome evidence related to the included interventions, which is a limitation of the field of CS and clinical interventions more broadly.
- Qualitative comparative analysis
Model 1 – How birth information was provided to women
Model 1 is constructed based on the finding from Kingdon et al. (2018) [ 22 ] that women and communities enjoy learning new birth information, as it opens up new ways of thinking about vaginal birth and CS. Learning new information allows them to understand better the benefits and risks of CS and vaginal births, as well as increase their knowledge about CS [ 22 ].
We used four conditions in constructing model 1 truth table: 1) the provision of information, education, and communication (IEC) materials on what to expect during labour and birth, 2) type of education delivered (antenatal education or psychoeducation), and 3) group-based intervention delivery. We explored this model considering other conditions, such as type of information provided (e.g. information about mode of birth including birth process, mental health and coping strategies, pain relief), delivery technique (e.g. didactic, practical) and frequency and duration of intervention delivery; however these additional conditions did not result in configurations.
Of 16 possible configurations, we identified seven configurations (Table 4 ). The first two row shows perfect consistency of configurations (inclusion = 1) in five studies [ 37 , 68 , 69 , 70 , 71 ] in which all conditions are present, except antenatal education or psychoeducation. The remaining configurations are unsuccessful interventions. Interestingly, when either IEC materials or group-based intervention delivery are present (but not both), implementation is likely to be unsuccessful (rows 3–7).
Boolean minimisation identified two intermediate pathways to successful interventions (Fig. 2 ). The two pathways are similar, except for one condition: type of education. The antenatal education or psychoeducation materials is the content tailored to the type of women they target. Therefore, from the two pathways, we can see that the presence of distribution of IEC materials on birth information and group-based intervention delivery of either antenatal education to the general population of women (e.g. not groups of women with specific risks or conditions) or psychoeducation to women with fear of birth trigger successful interventions. From this solution, we can see that the successful interventions are consistently characterised by the presence of both IEC materials and group-based intervention delivery.
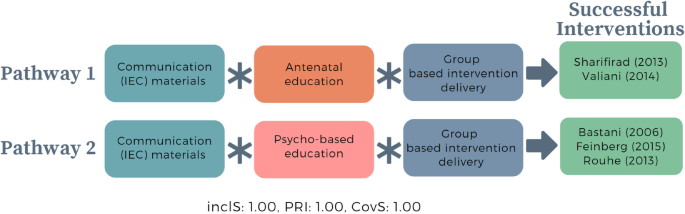
Intermediate pathways from model 1 that trigger successful interventions targeting pregnant women to optimise CS. In QCA, asterisk (*) denotes an ‘AND’ relationship; Inclusion score (InclS), also known as consistency, indicates the degree to which the evidence is consistent with the hypothesis that there is sufficient relation between the configuration and the outcome; Proportional Reduction in Inconsistency (PRI) refers to the extent in which a configuration is sufficient in triggering successful outcome as well as the negation of the outcome; Coverage score (CovS) refers to percentage of cases in which the configuration is valid
Model 2 – Emotional support was provided to women
Model 2 was constructed based on the theory that women desire emotional support alongside the communication of information about childbirth [ 22 ]. This includes emotional support from husbands or partners, health professional, or doulas [ 22 ]. Furthermore, Kingdon et al. (2018) describe the importance of two-way conversation and dialogue between women and providers during pregnancy care, particularly to ensure the opportunity for discussion [ 22 ]. Interventions may generate more questions than they answered, creating the need and desire of women to have more dialogue with health professionals [ 22 ]. Women considered intervention content to be most useful when it complements clinical care, is consistent with advice from health professionals and provides a basis for more informed, meaningful dialogue between women and care providers [ 22 ].
Based on this underlying theory, we constructed model 3 truth table by considering three conditions representative of providing emotional support to women, including partner or family member involvement, group-based intervention delivery which provide social or peer support to women, and opportunity for women to interact with health providers. Of 8 possible configurations, we identified six configurations (Table 5 ). The first three rows represent successful interventions with perfect consistency (inclusion = 1). The first row shows successful interventions with all conditions present. The second and third row shows successful interventions with all conditions except partner or family member involvement or interaction with health providers. The remaining rows represent unsuccessful interventions, where at least two conditions are absent.
Boolean minimisation identified two intermediate pathways to successful interventions (Fig. 3 ). In the first pathway, the partner or family members involvement and group-based intervention delivery enable successful interventions. In the second pathway, however, when partner or family members are not involved, successful interventions can happen only when interaction with health providers is included alongside group-based intervention. From these two pathways, we can see that group-based intervention, involvement of partner and family member, and opportunity for women to interact with providers seem to be important in driving intervention success.
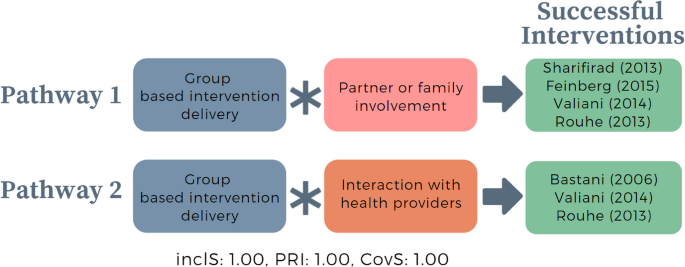
Intermediate pathways from model 2 that trigger successful interventions targeting pregnant women to optimise CS. In QCA, asterisk (*) denotes an ‘AND’ relationship; Inclusion score (InclS), also known as consistency, indicates the degree to which the evidence is consistent with the hypothesis that there is sufficient relation between the configuration and the outcome; Proportional Reduction in Inconsistency (PRI) refers to the extent in which a configuration is sufficient in triggering successful outcome as well as the negation of the outcome; Coverage score (CovS) refers to percentage of cases in which the configuration is valid
Consolidated model – Essential conditions to prompt successful interventions focusing on women
Using the identified important conditions observed in models 1 and 2, we constructed a consolidated model to examine the final essential conditions which could prompt successful educational interventions targeting women. We merged and tested four conditions: the provision of IEC materials on what to expect during labour and birth, group-based intervention delivery, partner or family member involvement, and opportunity for interaction between women and health providers.
Of the 16 possible configurations, we identified six configurations (Table 6 ). The first three rows show configurations resulting in successful interventions with perfect consistency (inclusion = 1). The first row shows successful interventions with all conditions present; the second and third rows show successful interventions with all conditions present except interaction with health providers or partner or family member involvement. The remaining three rows are configurations of unsuccessful interventions, missing at least two conditions, including the consistent absence of partner or family member involvement.
Boolean minimisation identified two intermediate pathways to successful intervention (Fig. 4 ). The first pathway shows that the opportunity for women to interact with health providers, provision of IEC materials, and group-based intervention delivery prompts successful interventions. The second pathway, however, shows that when there is no opportunity for women to interact with health providers, it is important to have partner or family member involvement alongside group-based intervention delivery and provision of IEC materials. These two pathways suggest that the delivery of educational interventions accompanied by provision of IEC materials and presence of emotional support for women during the intervention is important to trigger successful interventions. These pathways also emphasise that emotional support for women during the intervention can come from either partner, family member, or health provider. For the consolidated model, we did not simplify the solution further, as the intermediate solution is more theoretically sound compared to the most parsimonious solution.
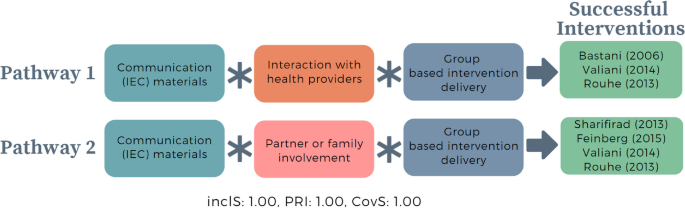
Intermediate pathways from consolidated model that trigger successful interventions targeting pregnant women to optimise CS. In QCA, asterisk (*) denotes an ‘AND’ relationship; Inclusion score (InclS), also known as consistency, indicates the degree to which the evidence is consistent with the hypothesis that there is sufficient relation between the configuration and the outcome; Proportional Reduction in Inconsistency (PRI) refers to the extent in which a configuration is sufficient in triggering successful outcome as well as the negation of the outcome; Coverage score (CovS) refers to percentage of cases in which the configuration is valid.
Sub-analysis – Interventions targeting both women and health providers or systems
In this sub-analysis, we run the important conditions identified from the consolidated model, added condition of multi-target intervention, and applied it to 17 interventions: 11 interventions targeting women, and six interventions targeting both women and health providers or systems (multi-target interventions).
Of 32 possible configurations, we identified eight configurations (Table 7 ). The first four rows show configurations with successful interventions with perfect consistency (inclusion = 1). The first row is where all the multi-target interventions are clustered, except the unsuccessful intervention Zhang (2020) [ 49 ], and where all the conditions are present. All the conditions in the second to fourth rows are present, except multi-target interventions (all rows), interaction with health providers (third row) and partner and family member involvement (fourth row). The remaining rows are all configurations to unsuccessful interventions, where at least three conditions are missing, except row 8, which is a single case row. This case is the only multi-target intervention that is unsuccessful and in which partner or family members were not involved.
The Boolean minimisation identified two intermediate pathways (Fig. 5 ). The first pathway shows that partner or family involvement, provision of IEC materials, and group-based intervention delivery prompt successful interventions. The first pathway is comprised of all five successful multi-target interventions [ 44 , 45 , 46 , 47 , 48 ] and four of 11 interventions targeting only women [ 37 , 68 , 69 , 71 ]. The second pathway shows that when multi-target interventions are absent, but when interaction with health providers is present, alongside provision of IEC materials and group-based intervention delivery, it prompts successful interventions (3/11 interventions targeting women only [ 37 , 69 , 70 ]). The first pathway shows that there are successful configurations with and without multi-target interventions. Therefore, similar to the interventions targeting women, when implementing multi-target interventions, intervention components targeting women are more likely to be successful when partners or family members are involved, interventions are implemented through group-based intervention delivery, IEC materials were provided, and there is an opportunity for women to interact with health providers.
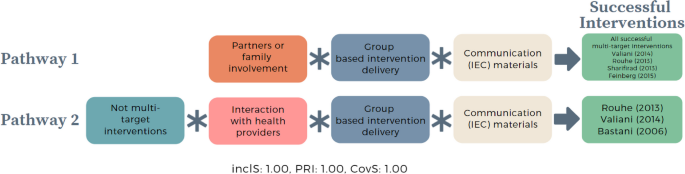
Intermediate pathways from multi-target interventions sub-analysis that trigger successful interventions targeting pregnant women to optimise CS. In QCA, asterisk (*) denotes an ‘AND’ relationship; Inclusion score (InclS), also known as consistency, indicates the degree to which the evidence is consistent with the hypothesis that there is sufficient relation between the configuration and the outcome; Proportional Reduction in Inconsistency (PRI) refers to the extent in which a configuration is sufficient in triggering successful outcome as well as the negation of the outcome; Coverage score (CovS) refers to percentage of cases in which the configuration is valid
To summarise, there are four essential intervention components which trigger successful educational interventions focusing on pregnant women to reduce CS, this includes 1) group-based intervention delivery, 2) provision of IEC materials on what to expect during labour and birth, 3) partner or family member involvement on the intervention, and 4) opportunity for women to interact with health providers. These conditions do not work in siloed or independently but instead work jointly as parts of configurations to enable successful interventions.
Our extensive QCA identified configurations of essential intervention components which are sufficient to trigger successful interventions to optimised CS. Educational interventions focusing on women were successful by: 1) leveraging social or peer support through group-based intervention delivery, 2) improving women’s knowledge and awareness of what to expect during labour and birth, 3) ensuring women have emotional support through partner or family participation in the intervention, and 4) providing opportunities for women to interact with health providers. We found that the absence of two or more of the above characteristics in an intervention result in unsuccessful interventions. Unlike our logic model, which predicted engagement strategies (i.e. intensity, frequency, technique, recruitment, incentives) to be essential to intervention success, we found that “support” seems to be central in maximising benefits of interventions targeting women.
Group-based intervention delivery is present across all four truth tables and eight pathways leading to successful intervention implementation, suggesting that group-based intervention delivery is an essential component of interventions targeting women. Despite this, we cannot conclude that group-based intervention delivery is a necessary condition, as there may be other pathways not captured in this QCA. The importance of group-based intervention delivery may be due to the group setting providing women with a sense of confidence through peer support and engagement. In group-based interventions, women may feel more confident when learning with others and peer support may motivate women. Furthermore, all group-based interventions in our included studies are conducted at health facilities, which may provide women with more confidence that information is aligned with clinical recommendations. Evidence on benefits of group-based interventions involving women who are pregnant has been demonstrated previously [ 72 , 73 ]. Women reported that group-based interventions reduce their feelings of isolation, provide access to group support, and allow opportunities for them to share their experiences [ 72 , 74 , 75 , 76 ]. This is aligned with social support theory, in which social support through a group or social environment may provide women with feelings of reassurance, compassion, reduce feelings of uncertainty, increase sense of control, access to new contacts to solve problems, and provision of instrumental support, which eventually influence positive health behaviours [ 72 , 77 ]. Women may resolve their uncertainties around mode of birth by sharing their concerns with others and learning at the same time how others cope with it. These findings are consistent with the benefits associated with group-based antenatal care, which is recommended by WHO [ 78 , 79 ].
Kingdon et al. (2018) reported that women and communities liked learning new birth information, as it opens new ways of thinking about vaginal birth and CS, and educates about benefits of different modes of birth, including risks of CS. Our QCA is aligned with this finding where provision of information about birth through education delivery leads to successful interventions but with certain caveats. That is, provision of birth information should be accompanied by IEC materials and through group-based intervention delivery. There is not enough information to distinguish what type of IEC materials lead to successful intervention; however, it is important to note that the format of the IEC materials (such as paper-based or mobile application) may affect success. More work is needed to understand how women and families react to format of IEC materials; for example, will paper-based IEC materials be relegated over more modern methods of reaching women with information through digital applications? The QUALI-DEC (Quality decision-making (QUALI-DEC) by women and healthcare providers for appropriate use of caesarean section) study is currently implementing a decision-analysis tool to help women make an informed decision on preferred mode of birth using both a paper-based and mobile application that may shed some light on this [ 80 ].
Previous research has shown that women who participated in interventions aiming to reduce CS desired emotional support (from partners, doulas or health providers) alongside the communication about childbirth [ 22 ]. Our QCA is aligned with this finding in which emotional support from partners or family members is highly influential in leading to successful interventions. Partner involvement in maternity care has been extensively studied and has been demonstrated to improve maternal health care utilisation and outcomes [ 81 ]. Both women and their partners perceived that partner involvement is crucial as it facilitates men to learn directly from providers, thus promoting shared decision-making among women and partners and enabling partners to reinforce adherence to any beneficial suggestions [ 82 , 83 , 84 , 85 , 86 ]. Partners provide psychosocial support to women, for example through being present during pregnancy and the childbirth process, as well as instrumental support, which includes supporting women financially [ 82 , 83 , 84 ]. Despite the benefits of partner involvement, partner's participation in maternity care is still low [ 82 ], as reflected in this study where only four out of 11 included interventions on this study involved partner or family member involvement. Reasons for this low participation, which include unequal gender norms and limited health system capability [ 82 , 84 , 85 , 86 ], should be explored and addressed to ensure the benefits of the interventions.
Furthermore, our QCA demonstrates the importance of interaction with health providers to trigger successful interventions. The interaction of women with providers in CS decision-making, however, is on a “nexus of power, trust, and risk”, where it may be beneficial but can also reinforce the structural oppression of women [ 13 ]. A recent study on patient-provider interaction in CS decision-making concluded that the interaction between providers who are risk-averse, and women who are cautious about their pregnancies in the health system results in discouragement of vaginal births [ 87 ]. However, this decision could be averted by meaningful communication between women and providers where CS risks and benefits are communicated in an environment where vaginal birth is encouraged [ 87 ]. Furthermore, the reasons women desire interaction with providers can come from opposite directions. Some women see providers as the most trusted and knowledgeable source, in which women can trust the judgement and ensure that the information learned is reliable and evidenced-based [ 22 ]. On the other hand, some women may have scepticism towards providers where women understand that providers’ preference may negatively influence their preferred mode of birth [ 22 ]. Therefore, adequate, two-way interaction is important for women to build a good rapport with providers.
It is also important to note that we have limited evidence (3/17 intervention studies) involving women with previous CS. Vaginal birth after previous CS (VBAC) can be a safe and positive experience for some women, but there are also potential risks depending on their obstetric history [ 88 , 89 , 90 ]. Davis (2020) found that women were motivated to have VBAC due to negative experiences of CS, such as the difficult recovery, and that health providers' roles served as pivotal drivers in motivating women towards VBAC [ 91 ]. Other than this, VBAC also requires giving birth in a suitably staffed and equipped maternity unit, with staff trained on VBAC, equipment for labour monitoring, and resources for emergency CS if needed [ 89 , 90 ]. There is comparatively less research conducted on VBAC and trial of labour after CS [ 88 ]. Therefore, more work is needed to explore if there are potentially different pathways that lead to successful intervention implementation for women with previous CS. It may be more likely that interventions targeting various stakeholders are more crucial in this group of women. For example, both education for women and partners or families, as well as training to upskill health providers might be needed to support VBAC.
Strength and limitations
We found many included studies had poor reporting of the interventions, including the general intervention components (e.g. presence of policies that may support interventions) and process evaluation components, which is reflective of the historical approach to reporting trial data. This poor reporting means we could not engage further in the interventions and thus may have missed important conditions that were not reported. However, we have attempted to compensate for limited process evaluation components by identifying all relevant sibling studies that could contribute to a better understanding of context. Furthermore, there are no studies conducted in low-income countries, despite rapidly increasing CS rates in these settings. Lastly, we were not able to conduct more nuanced analyses about CS, such as exploring how CS interventions impacted changes to emergency versus elective CS, VBAC, or instrumental birth, due to an insufficient number of studies and heterogeneity in outcome measurements. Therefore, it is important to note that we are not necessarily measuring the optimal outcome of interest—reducing unnecessary CS. However, it is unlikely that these non-clinical interventions will interfere with a decision of CS based on clinical indications.
Despite these limitations, this is the first study aiming to understand how certain interventions can be successful in targeting women to optimise CS use. We used the QCA approach and new analytical frameworks to re-analyse existing systematic review evidence to generate new knowledge. We ensure robustness through the use of a logic model and worked backwards in understanding what aspects are different in the intervention across different outcomes. The use of QCA and qualitative evidence synthesis ensured that the results are theory-driven, incorporate participants’ perspectives into the analysis, and explored iteratively to find the appropriate configurations, reducing the risk of data fishing. Lastly, this QCA extends the understanding of effectiveness review conducted by Chen et al. (2018) [ 18 ] by explaining the potential intervention components which may influence heterogeneity.
Implications for practice and research
To aid researchers and health providers to reduce CS in their contexts and designing educational interventions targeting women during pregnancy, we have developed a checklist of key components or questions to consider when designing the interventions that may help lead to successful implementation:
Is the intervention delivered in a group setting?
Are IEC materials on what to expect during labour and birth disseminated to women?
Are women’s partners or families involved in the intervention?
Do women have opportunities to interact with health providers?
We have used this checklist to explore the extent to which the included interventions in our QCA include these components using a matrix model (Fig. 6 ).
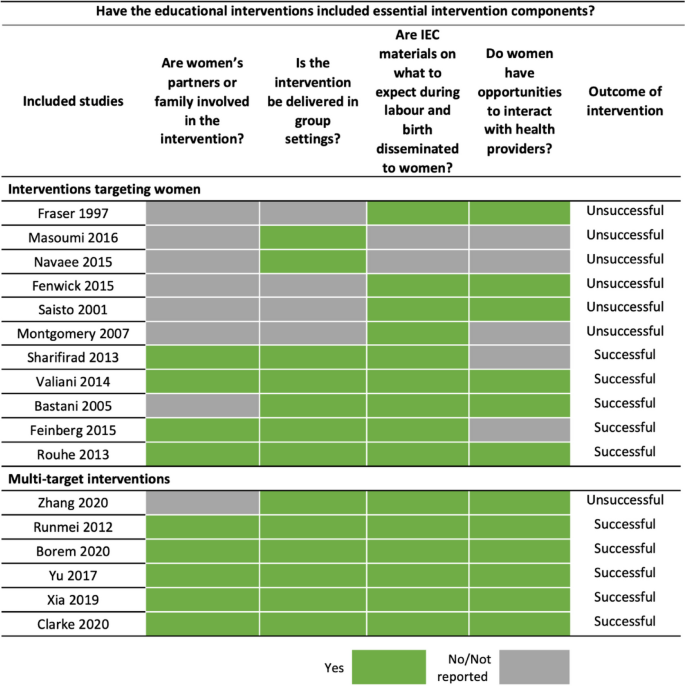
Matrix model assessing the extent to which the included intervention studies have essential intervention components identified in the QCA
Additionally, future research on interventions to optimise the use of CS should report the intervention components implemented, including process outcomes such as fidelity, attrition, contextual factors (e.g. policies, details of how the intervention is delivered), and stakeholder factors (e.g. women’s perceptions and satisfaction). These factors are important in not just evaluating whether the intervention is successful or not, but also in exploring why similar interventions can work in one but not in another context. There is also a need for more intervention studies implementing VBAC to reduce CS, to understand how involving women with previous CS may result in successful interventions. Furthermore, more studies understanding impact of the interventions targeting women in LMICs are needed.
This QCA illustrates crucial intervention components and potential pathways that can trigger successful educational interventions to optimise CS, focusing on pregnant women. The following intervention components are found to be sufficient in triggering successful outcomes: 1) group-based delivery, 2) provision of IEC materials, 3) partner or family member involvement, and 4) opportunity for women to interact with health providers. These intervention components do not work in siloed or independently but instead work jointly as parts of configurations to enable successful interventions. Researchers, trialists, hospitals, or other institutions and stakeholders planning interventions focusing on pregnant women can consider including these components to ensure benefits. More studies understanding impact of the interventions targeting women to optimise CS are needed from LMICs. Researchers should clearly describe and report intervention components in trials, and consider how process evaluations can help explain why trials were successful or not. More robust trial reporting and process evaluations can help to better understand mechanisms of action and why interventions may work in one context yet not another.
Availability of data and materials
Additional information files have been provided and more data may be provided upon request to [email protected].
Abbreviations
Coverage score
- Caesarean section
Crisp set qualitative comparative analysis
Fuzzy set qualitative comparative analysis
Information, education, and communication
Inclusion score
Low- and middle-income countries
Proportional reduction in inconsistency
Quality decision-making by women and healthcare providers for appropriate use of caesarean section
Vaginal birth after previous caesarean section
World Health Organization
World Health Organization. WHO statement on caesarean section rates. Available from: https://www.who.int/publications/i/item/WHO-RHR-15.02 . Cited 20 Sept 2023.
Zahroh RI, Disney G, Betrán AP, Bohren MA. Trends and sociodemographic inequalities in the use of caesarean section in Indonesia, 1987–2017. BMJ Global Health. 2020;5:e003844. https://doi.org/10.1136/bmjgh-2020-003844 .
Article PubMed PubMed Central Google Scholar
Betran AP, Ye J, Moller A-B, Souza JP, Zhang J. Trends and projections of caesarean section rates: global and regional estimates. BMJ Global Health. 2021;6:e005671. https://doi.org/10.1136/bmjgh-2021-005671 .
Boerma T, Ronsmans C, Melesse DY, Barros AJD, Barros FC, Juan L, et al. Global epidemiology of use of and disparities in caesarean sections. The Lancet. 2018;392:1341–8. https://doi.org/10.1016/S0140-6736(18)31928-7 .
Article Google Scholar
Sandall J, Tribe RM, Avery L, Mola G, Visser GH, Homer CS, et al. Short-term and long-term effects of caesarean section on the health of women and children. Lancet. 2018;392:1349–57. https://doi.org/10.1016/S0140-6736(18)31930-5 .
Article PubMed Google Scholar
Abenhaim HA, Tulandi T, Wilchesky M, Platt R, Spence AR, Czuzoj-Shulman N, et al. Effect of Cesarean Delivery on Long-term Risk of Small Bowel Obstruction. Obstet Gynecol. 2018;131:354–9. https://doi.org/10.1097/AOG.0000000000002440 .
Gurol-Urganci I, Bou-Antoun S, Lim CP, Cromwell DA, Mahmood TA, Templeton A, et al. Impact of Caesarean section on subsequent fertility: a systematic review and meta-analysis. Hum Reprod. 2013;28:1943–52. https://doi.org/10.1093/humrep/det130 .
Article CAS PubMed Google Scholar
Hesselman S, Högberg U, Råssjö E-B, Schytt E, Löfgren M, Jonsson M. Abdominal adhesions in gynaecologic surgery after caesarean section: a longitudinal population-based register study. BJOG: An Int J Obstetrics Gynaecology. 2018;125:597–603. https://doi.org/10.1111/1471-0528.14708 .
Article CAS Google Scholar
Tita ATN, Landon MB, Spong CY, Lai Y, Leveno KJ, Varner MW, et al. Timing of elective repeat cesarean delivery at term and neonatal outcomes. N Engl J Med. 2009;360:111–20. https://doi.org/10.1056/NEJMoa0803267 .
Article CAS PubMed PubMed Central Google Scholar
Wilmink FA, Hukkelhoven CWPM, Lunshof S, Mol BWJ, van der Post JAM, Papatsonis DNM. Neonatal outcome following elective cesarean section beyond 37 weeks of gestation: a 7-year retrospective analysis of a national registry. Am J Obstet Gynecol. 2010;202(250):e1-8. https://doi.org/10.1016/j.ajog.2010.01.052 .
Keag OE, Norman JE, Stock SJ. Long-term risks and benefits associated with cesarean delivery for mother, baby, and subsequent pregnancies: Systematic review and meta-analysis. PLoS Med. 2018;15:e1002494. https://doi.org/10.1371/journal.pmed.1002494 .
Ye J, Betrán AP, Guerrero Vela M, Souza JP, Zhang J. Searching for the optimal rate of medically necessary cesarean delivery. Birth. 2014;41:237–44. https://doi.org/10.1111/birt.12104 .
Eide KT, Morken N-H, Bærøe K. Maternal reasons for requesting planned cesarean section in Norway: a qualitative study. BMC Pregnancy Childbirth. 2019;19:102. https://doi.org/10.1186/s12884-019-2250-6 .
Long Q, Kingdon C, Yang F, Renecle MD, Jahanfar S, Bohren MA, et al. Prevalence of and reasons for women’s, family members’, and health professionals’ preferences for cesarean section in China: A mixed-methods systematic review. PLoS Med. 2018;15. https://doi.org/10.1371/journal.pmed.1002672 .
McAra-Couper J, Jones M, Smythe L. Caesarean-section, my body, my choice: The construction of ‘informed choice’ in relation to intervention in childbirth. Fem Psychol. 2012;22:81–97. https://doi.org/10.1177/0959353511424369 .
Panda S, Begley C, Daly D. Clinicians’ views of factors influencing decision-making for caesarean section: A systematic review and metasynthesis of qualitative, quantitative and mixed methods studies. PLoS One 2018;13. https://doi.org/10.1371/journal.pone.0200941 .
Takegata M, Smith C, Nguyen HAT, Thi HH, Thi Minh TN, Day LT, et al. Reasons for increased Caesarean section rate in Vietnam: a qualitative study among Vietnamese mothers and health care professionals. Healthcare. 2020;8:41. https://doi.org/10.3390/healthcare8010041 .
Chen I, Opiyo N, Tavender E, Mortazhejri S, Rader T, Petkovic J, et al. Non-clinical interventions for reducing unnecessary caesarean section. Cochrane Database Syst Rev. 2018. https://doi.org/10.1002/14651858.CD005528.pub3 .
Catling-Paull C, Johnston R, Ryan C, Foureur MJ, Homer CSE. Non-clinical interventions that increase the uptake and success of vaginal birth after caesarean section: a systematic review. J Adv Nurs. 2011;67:1662–76. https://doi.org/10.1111/j.1365-2648.2011.05662.x .
Kingdon C, Downe S, Betran AP. Non-clinical interventions to reduce unnecessary caesarean section targeted at organisations, facilities and systems: Systematic review of qualitative studies. PLOS ONE. 2018;13:e0203274. https://doi.org/10.1371/journal.pone.0203274 .
Kingdon C, Downe S, Betran AP. Interventions targeted at health professionals to reduce unnecessary caesarean sections: a qualitative evidence synthesis. BMJ Open. 2018;8:e025073. https://doi.org/10.1136/bmjopen-2018-025073 .
Kingdon C, Downe S, Betran AP. Women’s and communities’ views of targeted educational interventions to reduce unnecessary caesarean section: a qualitative evidence synthesis. Reprod Health. 2018;15:130. https://doi.org/10.1186/s12978-018-0570-z .
Opiyo N, Young C, Requejo JH, Erdman J, Bales S, Betrán AP. Reducing unnecessary caesarean sections: scoping review of financial and regulatory interventions. Reprod Health. 2020;17:133. https://doi.org/10.1186/s12978-020-00983-y .
Harris K, Kneale D, Lasserson TJ, McDonald VM, Grigg J, Thomas J. School-based self-management interventions for asthma in children and adolescents: a mixed methods systematic review. Cochrane Database Syst Rev. 2019. https://doi.org/10.1002/14651858.CD011651.pub2 .
World Health Organization. Robson Classifcation: Implementation Manual. 2017. Available from: https://www.who.int/publications/i/item/9789241513197 . Cited 20 Sept 2023.
Zahroh RI, Kneale D, Sutcliffe K, Vazquez Corona M, Opiyo N, Homer CSE, et al. Interventions targeting healthcare providers to optimise use of caesarean section: a qualitative comparative analysis to identify important intervention features. BMC Health Serv Res. 2022;22:1526. https://doi.org/10.1186/s12913-022-08783-9 .
World Health Organization. WHO recommendations: non-clinical interventions to reduce unnecessary caesarean sections. 2018. Available from: https://www.who.int/publications/i/item/9789241550338 . Cited 20 Sept 2023.
Hanckel B, Petticrew M, Thomas J, Green J. The use of Qualitative Comparative Analysis (QCA) to address causality in complex systems: a systematic review of research on public health interventions. BMC Public Health. 2021;21:877. https://doi.org/10.1186/s12889-021-10926-2 .
Melendez-Torres GJ, Sutcliffe K, Burchett HED, Rees R, Richardson M, Thomas J. Weight management programmes: Re-analysis of a systematic review to identify pathways to effectiveness. Health Expect. 2018;21:574–84. https://doi.org/10.1111/hex.12667 .
Chatterley C, Javernick-Will A, Linden KG, Alam K, Bottinelli L, Venkatesh M. A qualitative comparative analysis of well-managed school sanitation in Bangladesh. BMC Public Health. 2014;14:6. https://doi.org/10.1186/1471-2458-14-6 .
Thomas J, O’Mara-Eves A, Brunton G. Using qualitative comparative analysis (QCA) in systematic reviews of complex interventions: a worked example. Syst Rev. 2014;3:67. https://doi.org/10.1186/2046-4053-3-67 .
Dușa A. QCA with R: A Comprehensive Resource. 2021. Available from: https://bookdown.org/dusadrian/QCAbook/ . Cited 20 Sept 2023.
Kneale D, Sutcliffe K, Thomas J. Critical Appraisal of Reviews Using Qualitative Comparative Analyses (CARU-QCA): a tool to critically appraise systematic reviews that use qualitative comparative analysis. In: Abstracts of the 26th Cochrane Colloquium, Santiago, Chile. Cochrane Database of Systematic Reviews 2020;(1 Suppl 1). https://doi.org/10.1002/14651858.CD201901 .
Sutcliffe K, Thomas J, Stokes G, Hinds K, Bangpan M. Intervention Component Analysis (ICA): a pragmatic approach for identifying the critical features of complex interventions. Syst Rev. 2015;4:140. https://doi.org/10.1186/s13643-015-0126-z .
Melendez-Torres GJ, Sutcliffe K, Burchett HED, Rees R, Thomas J. Developing and testing intervention theory by incorporating a views synthesis into a qualitative comparative analysis of intervention effectiveness. Res Synth Methods. 2019;10:389–97. https://doi.org/10.1002/jrsm.1341 .
Thomas J, Harden A. Methods for the thematic synthesis of qualitative research in systematic reviews. BMC Med Res Methodol. 2008;8:45. https://doi.org/10.1186/1471-2288-8-45 .
Rouhe H, Salmela-Aro K, Toivanen R, Tokola M, Halmesmäki E, Saisto T. Obstetric outcome after intervention for severe fear of childbirth in nulliparous women – randomised trial. BJOG: An Int J Obstetrics Gynaecology. 2013;120:75–84. https://doi.org/10.1111/1471-0528.12011 .
Fraser W, Maunsell E, Hodnett E, Moutquin JM. Randomized controlled trial of a prenatal vaginal birth after cesarean section education and support program Childbirth alternatives Post-Cesarean study group. Am J Obstet Gynecol. 1997;176:419–25. https://doi.org/10.1016/s0002-9378(97)70509-x .
Masoumi SZ, Kazemi F, Oshvandi K, Jalali M, Esmaeili-Vardanjani A, Rafiei H. Effect of training preparation for childbirth on fear of normal vaginal delivery and choosing the type of delivery among pregnant women in Hamadan, Iran: a randomized controlled trial. J Family Reprod Health. 2016;10:115–21.
PubMed PubMed Central Google Scholar
Navaee M, Abedian Z. Effect of role play education on primiparous women’s fear of natural delivery and their decision on the mode of delivery. Iran J Nurs Midwifery Res. 2015;20:40–6.
Fenwick J, Toohill J, Gamble J, Creedy DK, Buist A, Turkstra E, et al. Effects of a midwife psycho-education intervention to reduce childbirth fear on women’s birth outcomes and postpartum psychological wellbeing. BMC Pregnancy Childbirth. 2015;15:284. https://doi.org/10.1186/s12884-015-0721-y .
Saisto T, Salmela-Aro K, Nurmi J-E, Könönen T, Halmesmäki E. A randomized controlled trial of intervention in fear of childbirth. Obstet Gynecol. 2001;98:820–6. https://doi.org/10.1016/S0029-7844(01)01552-6 .
Montgomery AA, Emmett CL, Fahey T, Jones C, Ricketts I, Patel RR, et al. Two decision aids for mode of delivery among women with previous Caesarean section: randomised controlled trial. BMJ: British Medic J. 2007;334:1305–9.
Xia X, Zhou Z, Shen S, Lu J, Zhang L, Huang P, et al. Effect of a two-stage intervention package on the cesarean section rate in Guangzhou, China: A before-and-after study. PLOS Medicine. 2019;16:e1002846. https://doi.org/10.1371/journal.pmed.1002846 .
Yu Y, Zhang X, Sun C, Zhou H, Zhang Q, Chen C. Reducing the rate of cesarean delivery on maternal request through institutional and policy interventions in Wenzhou. China PLoS ONE. 2017;12:1–12. https://doi.org/10.1371/journal.pone.0186304 .
Borem P, de Cássia SR, Torres J, Delgado P, Petenate AJ, Peres D, et al. A quality improvement initiative to increase the frequency of Vaginal delivery in Brazilian hospitals. Obstet Gynecol. 2020;135:415–25. https://doi.org/10.1097/AOG.0000000000003619 .
Ma R, Lao Terence T, Sun Y, Xiao H, Tian Y, Li B, et al. Practice audits to reduce caesareans in a tertiary referral hospital in south-western China. Bulletin World Health Organiz. 2012;90:488–94. https://doi.org/10.2471/BLT.11.093369 .
Clarke M, Devane D, Gross MM, Morano S, Lundgren I, Sinclair M, et al. OptiBIRTH: a cluster randomised trial of a complex intervention to increase vaginal birth after caesarean section. BMC Pregnancy Childbirth. 2020;20:143. https://doi.org/10.1186/s12884-020-2829-y .
Zhang L, Zhang L, Li M, Xi J, Zhang X, Meng Z, et al. A cluster-randomized field trial to reduce cesarean section rates with a multifaceted intervention in Shanghai. China BMC Medicine. 2020;18:27. https://doi.org/10.1186/s12916-020-1491-6 .
Fenwick J, Gamble J, Creedy DK, Buist A, Turkstra E, Sneddon A, et al. Study protocol for reducing childbirth fear: a midwife-led psycho-education intervention. BMC Pregnancy Childbirth. 2013;13:190. https://doi.org/10.1186/1471-2393-13-190 .
Toohill J, Fenwick J, Gamble J, Creedy DK, Buist A, Turkstra E, et al. A randomized controlled trial of a psycho-education intervention by midwives in reducing childbirth fear in pregnant women. Birth. 2014;41:384–94. https://doi.org/10.1111/birt.12136 .
Toohill J, Callander E, Gamble J, Creedy D, Fenwick J. A cost effectiveness analysis of midwife psycho-education for fearful pregnant women – a health system perspective for the antenatal period. BMC Pregnancy Childbirth. 2017;17:217. https://doi.org/10.1186/s12884-017-1404-7 .
Turkstra E, Mihala G, Scuffham PA, Creedy DK, Gamble J, Toohill J, et al. An economic evaluation alongside a randomised controlled trial on psycho-education counselling intervention offered by midwives to address women’s fear of childbirth in Australia. Sex Reprod Healthc. 2017;11:1–6. https://doi.org/10.1016/j.srhc.2016.08.003 .
Emmett CL, Shaw ARG, Montgomery AA, Murphy DJ, DiAMOND study group. Women’s experience of decision making about mode of delivery after a previous caesarean section: the role of health professionals and information about health risks. BJOG 2006;113:1438–45. https://doi.org/10.1111/j.1471-0528.2006.01112.x .
Emmett CL, Murphy DJ, Patel RR, Fahey T, Jones C, Ricketts IW, et al. Decision-making about mode of delivery after previous caesarean section: development and piloting of two computer-based decision aids. Health Expect. 2007;10:161–72. https://doi.org/10.1111/j.1369-7625.2006.00429.x .
Hollinghurst S, Emmett C, Peters TJ, Watson H, Fahey T, Murphy DJ, et al. Economic evaluation of the DiAMOND randomized trial: cost and outcomes of 2 decision aids for mode of delivery among women with a previous cesarean section. Med Decis Making. 2010;30:453–63. https://doi.org/10.1177/0272989X09353195 .
Frost J, Shaw A, Montgomery A, Murphy D. Women’s views on the use of decision aids for decision making about the method of delivery following a previous caesarean section: Qualitative interview study. BJOG : An Int J Obstetrics Gynaecology. 2009;116:896–905. https://doi.org/10.1111/j.1471-0528.2009.02120.x .
Rees KM, Shaw ARG, Bennert K, Emmett CL, Montgomery AA. Healthcare professionals’ views on two computer-based decision aids for women choosing mode of delivery after previous caesarean section: a qualitative study. BJOG. 2009;116:906–14. https://doi.org/10.1111/j.1471-0528.2009.02121.x .
Emmett CL, Montgomery AA, Murphy DJ. Preferences for mode of delivery after previous caesarean section: what do women want, what do they get and how do they value outcomes? Health Expect. 2011;14:397–404. https://doi.org/10.1111/j.1369-7625.2010.00635.x .
Bastani F, Hidarnia A, Montgomery KS, Aguilar-Vafaei ME, Kazemnejad A. Does relaxation education in anxious primigravid Iranian women influence adverse pregnancy outcomes?: a randomized controlled trial. J Perinat Neonatal Nurs. 2006;20:138–46. https://doi.org/10.1097/00005237-200604000-00007 .
Feinberg ME, Kan ML. Establishing Family Foundations: Intervention Effects on Coparenting, Parent/Infant Well-Being, and Parent-Child Relations. J Fam Psychol. 2008;22:253–63. https://doi.org/10.1037/0893-3200.22.2.253 .
Me F, Ml K, Mc G. Enhancing coparenting, parenting, and child self-regulation: effects of family foundations 1 year after birth. Prevention Science: Official J Soc Prevention Res. 2009;10. https://doi.org/10.1007/s11121-009-0130-4 .
Rouhe H, Salmela-Aro K, Toivanen R, Tokola M, Halmesmäki E, Saisto T. Life satisfaction, general well-being and costs of treatment for severe fear of childbirth in nulliparous women by psychoeducative group or conventional care attendance. Acta Obstet Gynecol Scand. 2015;94:527–33. https://doi.org/10.1111/aogs.12594 .
Rouhe H, Salmela-Aro K, Toivanen R, Tokola M, Halmesmäki E, Ryding E-L, et al. Group psychoeducation with relaxation for severe fear of childbirth improves maternal adjustment and childbirth experience–a randomised controlled trial. J Psychosom Obstet Gynaecol. 2015;36:1–9. https://doi.org/10.3109/0167482X.2014.980722 .
Healy P, Smith V, Savage G, Clarke M, Devane D, Gross MM, et al. Process evaluation for OptiBIRTH, a randomised controlled trial of a complex intervention designed to increase rates of vaginal birth after caesarean section. Trials. 2018;19:9. https://doi.org/10.1186/s13063-017-2401-x .
Clarke M, Savage G, Smith V, Daly D, Devane D, Gross MM, et al. Improving the organisation of maternal health service delivery and optimising childbirth by increasing vaginal birth after caesarean section through enhanced women-centred care (OptiBIRTH trial): study protocol for a randomised controlled trial (ISRCTN10612254). Trials. 2015;16:542. https://doi.org/10.1186/s13063-015-1061-y .
Lundgren I, Healy P, Carroll M, Begley C, Matterne A, Gross MM, et al. Clinicians’ views of factors of importance for improving the rate of VBAC (vaginal birth after caesarean section): a study from countries with low VBAC rates. BMC Pregnancy Childbirth. 2016;16:350. https://doi.org/10.1186/s12884-016-1144-0 .
Sharifirad G, Rezaeian M, Soltani R, Javaheri S, Mazaheri MA. A survey on the effects of husbands’ education of pregnant women on knowledge, attitude, and reducing elective cesarean section. J Educ Health Promotion. 2013;2:50. https://doi.org/10.4103/2277-9531.119036 .
Valiani M, Haghighatdana Z, Ehsanpour S. Comparison of childbirth training workshop effects on knowledge, attitude, and delivery method between mothers and couples groups referring to Isfahan health centers in Iran. Iran J Nurs Midwifery Res. 2014;19:653–8.
Bastani F, Hidarnia A, Kazemnejad A, Vafaei M, Kashanian M. A randomized controlled trial of the effects of applied relaxation training on reducing anxiety and perceived stress in pregnant women. J Midwifery Womens Health. 2005;50:e36-40. https://doi.org/10.1016/j.jmwh.2004.11.008 .
Feinberg ME, Roettger ME, Jones DE, Paul IM, Kan ML. Effects of a psychosocial couple-based prevention program on adverse birth outcomes. Matern Child Health J. 2015;19:102–11. https://doi.org/10.1007/s10995-014-1500-5 .
Evans K, Spiby H, Morrell CJ. Developing a complex intervention to support pregnant women with mild to moderate anxiety: application of the medical research council framework. BMC Pregnancy Childbirth. 2020;20:777. https://doi.org/10.1186/s12884-020-03469-8 .
Rising SS. Centering pregnancy. An interdisciplinary model of empowerment. J Nurse Midwifery. 1998;43:46–54. https://doi.org/10.1016/s0091-2182(97)00117-1 .
Breustedt S, Puckering C. A qualitative evaluation of women’s experiences of the Mellow Bumps antenatal intervention. British J Midwife. 2013;21:187–94. https://doi.org/10.12968/bjom.2013.21.3.187 .
Evans K, Spiby H, Morrell JC. Non-pharmacological interventions to reduce the symptoms of mild to moderate anxiety in pregnant women a systematic review and narrative synthesis of women’s views on the acceptability of and satisfaction with interventions. Arch Womens Ment Health. 2020;23:11–28. https://doi.org/10.1007/s00737-018-0936-9 .
Hoddinott P, Chalmers M, Pill R. One-to-one or group-based peer support for breastfeeding? Women’s perceptions of a breastfeeding peer coaching intervention. Birth. 2006;33:139–46. https://doi.org/10.1111/j.0730-7659.2006.00092.x .
Heaney CA, Israel BA. Social networks and social support. In Glanz K, Rimer BK, Viswanath K (Eds.), Health behavior and health education: Theory, research, and practice. Jossey-Bass; 2008. pp. 189–210. https://psycnet.apa.org/record/2008-17146-009 .
World Health Organization. WHO recommendations on antenatal care for a positive pregnancy experience. 2016. Available from: https://www.who.int/publications/i/item/9789241549912 . Cited 20 Sept 2023.
World Health Organization. WHO recommendation on group antenatal care. WHO - RHL. 2021. Available from: https://srhr.org/rhl/article/who-recommendation-on-group-antenatal-care . Cited 20 Sept 2023.
Dumont A, Betrán AP, Kabore C, de Loenzien M, Lumbiganon P, Bohren MA, et al. Implementation and evaluation of nonclinical interventions for appropriate use of cesarean section in low- and middle-income countries: protocol for a multisite hybrid effectiveness-implementation type III trial. Implementation Science 2020. https://doi.org/10.21203/rs.3.rs-35564/v2 .
Tokhi M, Comrie-Thomson L, Davis J, Portela A, Chersich M, Luchters S. Involving men to improve maternal and newborn health: A systematic review of the effectiveness of interventions. PLOS ONE. 2018;13:e0191620. https://doi.org/10.1371/journal.pone.0191620 .
Gibore NS, Bali TAL. Community perspectives: An exploration of potential barriers to men’s involvement in maternity care in a central Tanzanian community. PLOS ONE. 2020;15:e0232939. https://doi.org/10.1371/journal.pone.0232939 .
Galle A, Plaieser G, Steenstraeten TV, Griffin S, Osman NB, Roelens K, et al. Systematic review of the concept ‘male involvement in maternal health’ by natural language processing and descriptive analysis. BMJ Global Health. 2021;6:e004909. https://doi.org/10.1136/bmjgh-2020-004909 .
Ladur AN, van Teijlingen E, Hundley V. Male involvement in promotion of safe motherhood in low- and middle-income countries: a scoping review. Midwifery. 2021;103:103089. https://doi.org/10.1016/j.midw.2021.103089 .
Comrie-Thomson L, Tokhi M, Ampt F, Portela A, Chersich M, Khanna R, et al. Challenging gender inequity through male involvement in maternal and newborn health: critical assessment of an emerging evidence base. Cult Health Sex. 2015;17:177–89. https://doi.org/10.1080/13691058.2015.1053412 .
Article PubMed Central Google Scholar
Comrie-Thomson L, Gopal P, Eddy K, Baguiya A, Gerlach N, Sauvé C, et al. How do women, men, and health providers perceive interventions to influence men’s engagement in maternal and newborn health? A qualitative evidence synthesis. Soc Scie Medic. 2021;291:114475. https://doi.org/10.1016/j.socscimed.2021.114475 .
Doraiswamy S, Billah SM, Karim F, Siraj MS, Buckingham A, Kingdon C. Physician–patient communication in decision-making about Caesarean sections in eight district hospitals in Bangladesh: a mixed-method study. Reprod Health. 2021;18:34. https://doi.org/10.1186/s12978-021-01098-8 .
Dodd JM, Crowther CA, Huertas E, Guise J-M, Horey D. Planned elective repeat caesarean section versus planned vaginal birth for women with a previous caesarean birth. Cochrane Database Syst Rev. 2013. https://doi.org/10.1002/14651858.CD004224.pub3 .
Royal College of Obstetricians and Gynaecologists. Birth After Previous Caesarean Birth:Green-top Guideline No. 45. 2015. Available from: https://www.rcog.org.uk/globalassets/documents/guidelines/gtg_45.pdf . Cited 20 Sept 2023.
Royal Australian and New Zealand College of Obstetricians and Gynaecologists. Birth after previous caesarean section. 2019. Available from: https://ranzcog.edu.au/RANZCOG_SITE/media/RANZCOG-MEDIA/Women%27s%20Health/Statement%20and%20guidelines/Clinical-Obstetrics/Birth-after-previous-Caesarean-Section-(C-Obs-38)Review-March-2019.pdf?ext=.pdf . Cited 20 Sept 2023.
Davis D, Homer CS, Clack D, Turkmani S, Foureur M. Choosing vaginal birth after caesarean section: Motivating factors. Midwifery. 2020;88:102766. https://doi.org/10.1016/j.midw.2020.102766 .
Download references
Acknowledgements
We extend our thanks to Jim Berryman (Brownless Medical Library, Faculty of Medicine, Dentistry and Health Sciences, The University of Melbourne) for his help in refining the search strategy for sibling studies.
This research was made possible with the support of UNDP/UNFPA/UNICEF/WHO/World Bank Special Programme of Research, Development and Research Training in Human Reproduction (HRP), a co-sponsored programme executed by the World Health Organization (WHO). RIZ is supported by Melbourne Research Scholarship and Human Rights Scholarship from The University of Melbourne. CSEH is supported by a National Health and Medical Research Council (NHMRC) Principal Research Fellowship. MAB’s time is supported by an Australian Research Council Discovery Early Career Researcher Award (DE200100264) and a Dame Kate Campbell Fellowship (University of Melbourne Faculty of Medicine, Dentistry, and Health Sciences). The funders had no role in the study design, data collection and analysis, decision to publish, or preparation of the manuscript. The contents of this publication are the responsibility of the authors and do not reflect the views of the UNDP/UNFPA/UNICEF/WHO/World Bank Special Programme of Research, Development and Research Training in Human Reproduction (HRP), World Health Organization.
Author information
Authors and affiliations.
Gender and Women’s Health Unit, Nossal Institute for Global Health, School of Population and Global Health, University of Melbourne, Melbourne, VIC, Australia
Rana Islamiah Zahroh, Martha Vazquez Corona & Meghan A. Bohren
EPPI Centre, UCL Social Research Institute, University College London, London, UK
Katy Sutcliffe & Dylan Kneale
Department of Sexual and Reproductive Health and Research, UNDP/UNFPA/UNICEF/WHO/World Bank Special Programme of Research, Development and Research Training in Human Reproduction (HRP), World Health Organization, Geneva, Switzerland
Ana Pilar Betrán & Newton Opiyo
Maternal, Child, and Adolescent Health Programme, Burnet Institute, Melbourne, VIC, Australia
Caroline S. E. Homer
You can also search for this author in PubMed Google Scholar
Contributions
- Conceptualisation and study design: MAB, APB, RIZ
- Funding acquisition: MAB, APB
- Data curation: RIZ, MAB, MVC
- Investigation, methodology and formal analysis: all authors
- Visualisation: RIZ, MAB
- Writing – original draft preparation: RIZ, MAB
- Writing – review and editing: all authors
Corresponding author
Correspondence to Rana Islamiah Zahroh .
Ethics declarations
Ethics approval and consent to participate.
This study utilised published and openly available data, and thus ethics approval is not required.
Consent for publication
No direct individual contact is involved in this study, therefore consent for publication is not needed.
Competing interests
The authors declare no competing interests.
Additional information
Publisher's note.
Springer Nature remains neutral with regard to jurisdictional claims in published maps and institutional affiliations.
Supplementary Information
Additional file 1..
Logic model in optimizing CS use.
Additional file 2.
Risk of bias assessments.
Additional file 3.
Coding framework and calibration rules.
Additional file 4.
Coding framework as applied to each intervention (data table).
Rights and permissions
Open Access This article is licensed under a Creative Commons Attribution 4.0 International License, which permits use, sharing, adaptation, distribution and reproduction in any medium or format, as long as you give appropriate credit to the original author(s) and the source, provide a link to the Creative Commons licence, and indicate if changes were made. The images or other third party material in this article are included in the article's Creative Commons licence, unless indicated otherwise in a credit line to the material. If material is not included in the article's Creative Commons licence and your intended use is not permitted by statutory regulation or exceeds the permitted use, you will need to obtain permission directly from the copyright holder. To view a copy of this licence, visit http://creativecommons.org/licenses/by/4.0/ . The Creative Commons Public Domain Dedication waiver ( http://creativecommons.org/publicdomain/zero/1.0/ ) applies to the data made available in this article, unless otherwise stated in a credit line to the data.
Reprints and permissions
About this article
Cite this article.
Zahroh, R.I., Sutcliffe, K., Kneale, D. et al. Educational interventions targeting pregnant women to optimise the use of caesarean section: What are the essential elements? A qualitative comparative analysis. BMC Public Health 23 , 1851 (2023). https://doi.org/10.1186/s12889-023-16718-0
Download citation
Received : 07 March 2022
Accepted : 07 September 2023
Published : 23 September 2023
DOI : https://doi.org/10.1186/s12889-023-16718-0
Share this article
Anyone you share the following link with will be able to read this content:
Sorry, a shareable link is not currently available for this article.
Provided by the Springer Nature SharedIt content-sharing initiative
- Maternal health
- Complex intervention
- Intervention implementation
BMC Public Health
ISSN: 1471-2458
- Submission enquiries: [email protected]
- General enquiries: [email protected]
- Open access
- Published: 05 April 2024
“It is very hard to just accept this” – a qualitative study of palliative care teams’ ethical reasoning when patients do not want information
- Joar Björk 1 , 2 , 3
BMC Palliative Care volume 23 , Article number: 91 ( 2024 ) Cite this article
217 Accesses
13 Altmetric
Metrics details
The aim of this study was to explore how palliative care staff reason about the autonomy challenge that arises when a patient who has first said he wants full information appears to change his mind and rejects being informed.
The study had a qualitative and exploratory design. Participants (physicians, registred nurses, social workers, physiotherapists and occupational therapists) were recruited from palliative care teams in southern Sweden. Six separate focus group interviews with a total number of 33 participants were conducted. The teams were asked to discuss a fictional case of a man who first wants, then rejects, information about his situation. The interviews were audiotaped and transcribed verbatim. Reflexive thematic analysis following Braun and Clarke was undertaken to analyse data.
The analysis resulted in three themes: Patients have a right to reject information , Questioning whether this patient WANTS to reject information and There are other values at stake, too . Although participants endorsed a right to reject information, they were unsure whether this right was relevant in this situation, and furthermore felt that it should be balanced against counteracting factors. The effect of such balancing was that participants would aim to find a way to present relevant information to the patient, but in a probing and flexible way.
Conclusions
In their work with dying patients, palliative care staff meet many autonomy challenges. When faced with a choice to withhold information as per a patient’s wishes, or to provide information with the patient’s best interest in mind, staff find it hard to balance competing values. Staff also find it hard to balance their own interests against a purely professional stance. The overall strategy seems to be to look for caring ways to impart the information.
Peer Review reports
Respect for patient autonomy is one of the central ethical principles or ideals in modern health care [ 1 ]. What it means to respect patients’ autonomy is a complex question, and the concept and implications of autonomy are much contended in contemporary bioethics [ 2 , 3 , 4 , 5 ]. Nonetheless, most agree that patients should be invited to participate as far as possible in medical decision-making and that medical information should be tailored according to the patient’s previous knowledge and expressed information preferences. The same consideration is manifest in many national healthcare jurisdictions. For instance, the Swedish Patient law stipulates that the patient should be given information about his/her medical situation and the expected future health trajectory, but also that if patients do not wish such information this should be respected [ 6 ].
Palliative care (PC) is the subdivision of care which is focused on patients with life threatening illness [ 7 ]. The World Health Organization emphasises that PC ”should be provided through person-centred and integrated health services that pay special attention to the specific needs and preferences of individuals” [ibid]. Many publications in and on PC stress the profound importance of autonomy as a guiding ethical ideal in this context [ 8 , 9 ], although some also point out that ”standard” accounts of autonomy may not be suitably nuanced for PC [ 10 ]. Reasons for suggesting a special understanding of autonomy within PC include that PC patients are particularly ill, that families’ preferences play an especially important role in PC, and that some understandings of autonomy posit a relation of distrust between patient and healthcare provider [ 11 , 12 , 13 ].
Despite the great importance of patient autonomy as an ethical ideal in PC and elsewhere, it may sometimes be difficult to tease out which action would in fact best respect autonomy in a particular clinical situation [ 14 , 15 ]. Practical and theoretical challenges regarding how to best respect autonomy may arise, for instance, in cases of patient ambivalence and intra-personal conflicts of interest [ 16 , 17 , 18 ], autonomy-promoting withholding of information [ 19 ] and advance directives [ 20 ]. Add to this that autonomy is not the only aspect to account for in the ethical calculus. Indeed, healthcare staff must seek to balance autonomy considerations with (at least) considerations about potential beneficence and harm, as well as potential direct and indirect effects on other patients [ 21 ].
There is to date very little understanding of how PC staff reason about and deal with tricky autonomy cases. A limited number of qualitative studies have been undertaken which shine a light on some aspects [see for instance 22 – 25 ], but much remains unclear. As part of a larger project on patient autonomy in PC Footnote 1 , the present study aimed to expand this knowledge by investigating how PC teams reflect upon a clinical situation where a patient first claims to want information about his likely health trajectory, but then reports he has changed his mind so that he no longer wishes to be informed. To gain an in-depth understanding of the ethical as well as practical aspects of such a situation, the study aimed to elucidate both how PC teams reason about the ethics, and how they claim they would usually act in such a situation.
The study had a qualitative, exploratory and descriptive intent. Data was collected by focus group interviews, as this is a suitable way of getting information about factors which may influence motivation, opinions and behaviour in complex social settings [ 26 ]. Reflexive thematic analysis according to Braun and Clarke was chosen as method for data analysis as it may be used to understand how persons make sense of the world, which is relevant for a study involving ethical attitudes [ 27 , 28 , 29 ]. The method does not obscure but rather emphasises the creative input of the researcher, whereby both coding and the construction of themes is seen as a partly subjective process. In terms of Byrne’s four theoretical assumptions [ 27 ], this project was informed by a constructionist epistemology, meaning that language is seen not merely as an expression of experienced reality but also plays a role in constructing it. The orientation to data interpretation was mainly experiential as the study aimed to examine how participants understand certain professional challenges. Data analysis was inductive, data-driven and utilised open coding. In coding and analysis both semantic and latent content was interrogated.
Participants
Participants were drawn from six specialized PC teams in three southern Swedish regions (Region Jönköping, Region Halland and Region Blekinge). By international standards these regions are sparsely populated, with some mid-sized urban areas, encompassing a total population of approximately 850 000 inhabitants. The PC is organized similarly in the three regions, with mobile teams attending to patients in their homes and in qualified nursing homes, as well as in hospitals. Driving times are long, up to 90 min one-way. The teams are multiprofessional, consisting of 10–20 members (typically physicians, nurses and social workers, although some teams also have physiotherapists and occupational therapists). Staff may or may not have formalized PC specialist qualification. For a fuller description about the PC services in this geographical area, see [ 30 ] where the teams studied here correspond to the “less developed PC” category in that article. The “less developed PC” setting was chosen for this study as the aim was to understand an everyday ethical approach to the challenge.
Recruitment started with an informative letter to the head of each PC team. The head of each team was asked to put together a group for interview with suitable variation regarding professional background, working experience, gender and age. Prior to commencing each interview the composition of the groups was checked to see that there was sufficient variation.
Data collection
The vignette and the interview agenda (see below) were developed for this study. They were pilot tested in the author’s own work-place in Region Kronoberg (results not included in the study, but congruent with the results).
The focus group interviews were conducted in undisturbed rooms at the participants’ work places. JB was the sole interviewer. Each focus group interview commenced with JB reading a vignette to introduce the kind of challenge to be discussed:
Mr B, a man with disseminated prostate cancer, is cared for by the specialized palliative care team. He has repeatedly stated to the team that it is vital to him to have full knowledge about his situation, including what his death may come to look like. As there have been many bodily symptoms to deal with, his questions about death and dying have not yet been answered. Now, the team has set aside time for the conversation Mr B has asked for. But as soon as they start talking, Mr B states that he does not want any more information and that this issue should not be brought up again. He provides no reason. Nothing in the situation appears to have changed. He is perceived to be of sound mind, now as before.
A semi-structured interview agenda was used to make sure key topics were covered (do you recognise situations like this one; how do you as individuals and teams usually deal with situations like this; what are your feelings and reflections about the situation). (See also Supplementary file). During the interview, open questions were used to encourage participants to develop their answers, and participants were urged to discuss among themselves [ 26 ]. Field notes were taken immediately after each interview to aid in the analytical process. Interviews were audio recorded and transcribed verbatim.
Notions of saturation are contested within this field of qualitative research [ 31 ], where a pragmatic view of sample sizing is advocated. Instead a provisional sample size of six focus group interviews was estimated based on previous recommendations [see for instance 29 , 32 ], and after having conducted six interviews the study was discontinued as it was felt the gathered data enabled the researcher to give a rich presentation of the studied phenomenon.
Data analysis
In accordance with suggestions by Braun and Clarke, data analysis consisted of six phases [ 27 , 28 ]. In Phase 1 (Familiarisation) , the entirety of the transcribed data corpus was read and reread with a curious mind and pen in hand to get a thorough understanding of the whole material. In Phase 2 (Generation of codes) , short labels summarizing relevant meaning units were created and refined. Data obviously not relevant to the research questions was left un-coded, whereas attention to both semantic and latent meaning meant that some data segments were double-coded. In Phase 3 (Construction of candidate themes) codes were grouped by identification of central organising concepts. Here, preliminary thematic maps were drawn to organise candidate themes as well as identify relations between them. In Phase 4 (Review of candidate themes) the suggested way of organizing information was reviewed and revised. Themes should be comprehensive as well as non-overlapping, and capture relevant meanings within the data. In Phase 5 (Naming and defining of themes) themes were given preliminary names, which should be evocative as well as descriptive, and a short presentation. In Phase 6 (Writing the report) the results section was drafted. Although Braun and Clarke recommend an “analytical” style of reporting whereby the results and discussion sections are intermingled [ 33 ], this study stuck to reporting consensus and left the part of the analytical work which looked beyond the dataset for a stand-alone discussion section.
Six focus group interviews were performed, with four to six participants per group. The total number of participants was 33. For participant characteristics, see Table 1 . Interviews lasted from 52 to 81 min (median: 69 min).
The analysis resulted in three themes: Patients have a right to reject information , Questioning whether this patient WANTS to reject information and There are other values at stake, too . All themes contained subthemes so that there were a total of seven subthemes – see Table 2 as well as the rest of the results section.
Patients have a right to reject information
The core meaning of this theme was that participants looked beyond the case at hand to an abstract, general right applicable to any patient to reject information. The first subtheme “A simple and self-evident right” focused on this right itself, whereas the second subtheme “The right must be contextualized” described contextual aspects which might or might not impact on this right. Hence both subthemes indicated that patients such as Mr B have or may have a right such that when he says he does not want to hear more, the PC team should abide by this.
A simple and self-evident right
In each focus group interview in this study, there was early and emphatic mention that Mr B has the right to say no to information, or to decide what information to receive. Consider this quote:
Well I guess that I try to be clear in our conversations, but still never… force anybody to take information they don’t want. To show, like, you have the right to know all that I know, but you also have the right to avoid it. (Interview V)
The right to reject unwanted information was often mentioned alongside other alleged negative rights, such as rejecting medical treatment, visits by the PC team etc. The right was generally stated as being self-evident, strong and irrefutable. True to its supposedly “self-evident” nature, the right was never further explained. In the few cases where external support was given for the right, this was provided by brief mention of healthcare law and/or coherence with bioethical norms. Mentions of a right to reject unwanted information always met general approval and was never interrogated or directly contradicted by other participants.
Sometimes, Mr B’s alleged right was mentioned in a way which highlighted the team’s responsibilities to honour the right. For instance, a common expression was that staff must “meet the patient where he is at”. This means that his desire not to talk about his health situation is not seen as merely neutral information, but that it implies tangible restrictions on the team’s further communication:
Perhaps what you need to do is to stop and back up, and then come back. It’s hard to see that you should… what do you call it… go over his defences, force your way, push it. Surely, that must be avoided. (Interview II)
The right must be contextualized
In addition to the self-evident and general right to reject unwanted information discussed above, some participants suggested a right to reject unwanted information which was both contingent and restricted. Many stated that they needed more information about Mr B and his decision to know whether such a right applied. For instance, there were questions about Mr B’s decision making competence (despite the case description stating that Mr B was unequivocally decision competent). Also, there were lively discussions about what might have made Mr B change his mind. It was clear that Mr B’s reasons for changing his mind affected the participants’ views of the ethical challenge here, although participants rarely articulated which reasons would be seen as more conducive to a right to remain uninformed or not. The one exception was that if he had been pressurized by somebody else to remain uniformed, rather than changed his own mind, participants suggested this detracted substantively from the alleged right to remain uninformed. Participants’ provided many and wide ranging speculations about why a patient may be pressurized to change his mind. One participant saw the matter through a cultural lens which affected this participant’s dealing with the matter:
[If they used joint decisionmaking] this would be easier to handle in a foreign family because we know that, and we have had that before, all of us… but since that’s not really the way Swedes usually behave it would challenge me more [if the family was from a traditional Swedish background]. I’d be more baffled about it (…) I mean it is easier to understand what we don’t understand, right? When we don’t understand a culture it is easier to understand what we don’t understand and accept it. (Interview III)
Some discussions also situated the specific question of a right to reject unwanted information within the larger framework of patients’ right to be involved in decision-making. According to this view, heeding any particular patient preference was seen as less important than working in a general spirit of shared decision making and balancing decision-making mandates between patient and the PC team. On this view, the relevant right was to be included in the decision-making process along with members of the PC team:
That they get to say no to something, that’s what’s important. Of course I have a decision at the back of my mind that I’ll make sure to get across later (…) sometimes it’s getting to decide about some little things which makes it feel good [for the patient] (Interview II).
Questioning whether this patient WANTS to reject information
This theme explored the way that some arguments did not contradict or balance out a possible right to reject information, but rather questioned whether Mr B did indeed want to reject information. The first subtheme “Staff must probe patients’ preferences” dealt with staff’s difficulty of knowing this, whereas the second subtheme “Preferences may change” instead dealt with, as it were, Mr B’s own difficulty of knowing whether he wanted or did not want information.
Staff must probe patients’ preferences
Rather than informing or not informing Mr B, participants stressed that in a case like this the team ought to “probe”, “investigate”, “explore” or “dig deeper”. One thing that they hoped to find by probing was Mr B’s real wishes, which they stated might not at all correspond to the lexical message he had conveyed. The participants asserted that it is not at all uncommon for patients to say one thing and mean another. Suggested reasons for this included that perhaps Mr B did not ever want information, even when he said he did, but rather only said so to please the team or appear brave, or that he still wanted information but now sought to protect somebody else from possibly disheartening information.
Another rationale for probing was the belief that most patients really want to know at least some things about their situation, implying that if a patient claims he wants to know nothing, then probing is required to elucidate what, in fact, he wants to know. The preference for probing over just taking things at face value is expressed as a general rule for communicating in this quote:
I think it’s pretty dangerous to think you understand everything… you have to kind of ask the question “Is this what you mean?” (Interview IV).
However, there were also cautionary comments about probing, including that this must never become heavy-handed and that too much curiosity should be kept at bay.
Preferences may change
While the previous subtheme dealt with reasons having to do with staff’s difficulty of knowing a patient’s (true) preferences, another factor which made participants cautious to let Mr Br remain uniformed was that Mr B himself might not know what he wanted. Many participants claimed having met patients whose information preferences changed drastically over time, which led participants to stress that information strategies must also be flexible in order to honour changing preferences. Having continuous personal contact with the patient was highlighted as an important advantage in this context. One benefit of continuous contact, to the participants, was that it allowed them to bring the matter up again at a later stage. This was predominantly seen not as a violation of Mr B’s expressed wish, but rather as a communicative tool to make sure that the patient got the information he currently desired:
Like we already said, it’s a process, so you have to continue later and take it again and adapt to the level where the patient is currently at (Interview I).
In contrast to the quote above, some participants felt that the team’s previous interaction with Mr B amounted to something akin to a promise to provide him with information. They thus suggested that the previous promise and the present protestation were separate matters which should be balanced against each other.
There are other values at stake, too
The last theme captured the rich array of reasons and strategies presented by participants, which would go against any possible right to remain uninformed. These reasons for providing information even in the face of Mr B’s protestations were grounded in different things: an ideal (“Striving for the good death”), staff’s interests (“Staff’s own interests”) and third party considerations (“Others’ rights are also relevant”).
Striving for the good death
One consideration that seemed to weigh on the participants was that by rejecting information, Mr B might jeopardize his chances of having a good death. There seemed to be consensus among participants across the teams that it was desirable, from the point of view of dying well, that the patient should have understood at least the superficial facts about his situation:
[Being informed] is a kind of security which increases the possibility of a dignified, calm and good death (…) that you’re not deprived of the time you could have had to think about whether you want to sell your paintings or not, because you didn’t understand how close it was… (Interview II).
Having a clear picture of the good death, and striving for it, meant the PC teams do not come to the patient’s bedside as blank slates. Instead they have an agenda and goals of care they strive for. The stress on providing sufficient information to the patient was sometimes tied to the patient’s possibility of properly understanding his situation, as in the quote above. Indeed, having the chance to really prepare for death, in terms of saying goodbyes and making final wishes etc., was sometimes expressed as being a bona fide right of its own, which was explicitly pitted against the alleged right of remaining uninformed. On the other hand, providing sufficient information was also tied to the necessity of making medical plans. In this quote, where a participant tries to distinguish the information that should be given from that which could be left out, the desire to make a good plan looms large:
So I think these are, somehow, different aspects. I’m thinking about how we deal with prognosis, being a palliative team. We may not have an interest, for our sake, to speak about his prognosis and how long time he has left, but we do have an interest in preparing for different things that may happen. So I make a difference there. There are some things that we perhaps don’t push so hard, but other things we do [push] (Interview VI).
Staff’s own interests
It was frequently acknowledged that individual team members and/or the team itself have more personal interests, which may clash with a patient such as Mr B’s expressed wishes. One prominent interest was that the team desired to have done a good job by having helped the patient. This desire was expressed not only in altruistic terms but also in terms of private experience and satisfaction. Helping the patient was largely described in the team’s own terms, in the sense that participants wanted to have provided the help they felt was necessary. Relatedly, the participants expressed a wish for a substantial professional mandate and the manoeuvring space to act as they saw fit. In all interviews, the word “frustrating” or synonyms came up to describe situations such as this, as the patient’s attempt to block information limited staff’s possibility to do their job properly:
There is a frustration to feel that we cannot do our very best, or we, we could do our best but we aren’t allowed to do our best, what we think is the best. So it’s like a… not that it becomes… you’re not less inclined to help because of it, but it makes it harder, I think. (Interview II)
Participants wished to keep the flow of communication as free from overt blockages as possible. They expressed a desire to be able to share the information they had and perceived as important. Not being able to share information was described as uncomfortable or that one was being “dishonest” for knowing and not telling.
When the team’s own interests were discussed, there was often hesitation as though the subject was controversial. For instance, there were mixed feelings about staff’s curiosity and their desire to really understand what motivated Mr B to change his mind. Some saw this curious interest as a legitimate aspect of being truly engaged in PC work, whereas others saw over-curious “probing” as something to avoid. Participants often found it challenging to disentangle their private feelings from their professional mandate. Consider this quote where a participant speaks of “converting” patients:
It is very hard to just accept this, I think… I mean you want to convert them… (…) You don’t want to tell them what to do, neither, you don’t want to do that. But I want to convert them so they have the possibility to… but is that just because I think for myself that’s what would have been good? If he doesn’t think that’s good, like… then it is just what I think he should want to know… sometimes it feels a little like that (Interview II).
Others’ rights are also relevant
As mentioned above, participants worried that others might have influenced Mr B’s decision-making, in a way which might detract from his alleged right to remain uninformed. Additionally, participants expressed concerns that Mr B’s decision might, in turn, affect others. If so, they argued, the effect on others could outweigh his right to remain uninformed. To this participant, the presence of children in the dilemma could make a great difference:
But I do think that what makes the situation easier, well not easier really but… is if they have small children. Because then there is a responsibility that people have as adults, to protect the children. That is, giving the children what they need. So then you don’t always have the right to say ”No, I don’t want information”, because it also affects someone who cannot govern their own fate (Interview IV).
The main finding in this qualitative study is that members of PC teams in Sweden find it hard to deal with situations where a patient (“Mr B”) who previously said he wanted information suddenly wishes to remain uninformed. Participants describe this situation as emotionally frustrating, as they perceive the provision of information as a crucial part of their work and they fear that the patient’s attitudes may lead to them not being able to carry out their task as well as they would wish. In this sense, the participants conceive of the patient’s present rejection of information as ill advised. They further describe the situation as a challenging balance where the patient’s right to govern the flow of information is pitted against strong counteracting rationales in favour of giving the information.
The participants in this study strongly endorse, and never straight out question, that patients have a right to choose whether to be informed or not. The possibility of staff ”forcing their way” with information is empathetically rejected, and instead “being flexible” and ”meeting the patient where he is at” were cited as exemplary strategies. This result is well aligned with ambitions in current PC [ 8 , 9 , 34 ]. Yet as participants discuss the alleged right to remain uninformed, they struggle to identify necessary and sufficient conditions for this right. This comes as no surprise, as contemporary bioethics struggles with the same questions. For instance, the issue of how and when family may influence patients’ decision-making without threatening autonomy is much debated [ 2 , 3 , 22 ]. As the participants stress a contextual understanding of autonomy which includes careful attention to Mr B’s decision-making competence and to understanding his real wishes rather than merely what he is saying, they are again mirroring live discussions in contemporary bioethics [ 11 , 35 , 36 ].
For all their endorsement of aspects of autonomy, the participants stated that they would not “just accept” a patient’s plea not to be informed nor pressed on the matter. Counteracting rationales in favour of disclosure weigh heavily in this study. For instance, participants express a desire to be honest to the patient and hide no information. This is in line with ethical recommendations in palliative care [ 37 , 38 ]. Another important rationale is to be able to make good medical plans, as well as help the patient understand and deal with his situation. Many authors have noted that working for patient preparedness or acceptance of death is a central commitment to PC teams [ 39 , 40 ]. In ethical parlance, this is a commitment to promoting beneficence, which may tip over into paternalism [ 41 , 42 ]. This is resonant with findings from other qualitative studies with palliative care personnel, where personnel for instance report struggling to find a balance between “leading and following” [ 43 ]. It is in this light the cautiously self-critical comment on “converting” patients in the present study should be read. Participants here communicate a clear picture of the good death, but simultaneously realize they run the risk of sometimes using the patient as a canvas to paint this picture on. Striving for the good death may flow from beneficence concerns as well as self-serving interests, as indicated by results in this material. Thus, there seems to be a risk of staff being smitten by the ”substitute success syndrome” [ 42 ], whereby staff may steer a patient in desired directions partly to make themselves feel good.
Another topic where participants self-reflected and expressed self-criticism concerned staff’s own needs and desires. For instance, feeling and expressing curiosity about why Mr B changed his mind was controversial in this study. Many professed their strong desire to explore Mr B’s motivations for changing his mind, and apart from autonomy-honouring reasons to do so there were also self-regarding reasons such as a personal desire to understand other persons. Participants repeatedly pointed out that this may be problematic. Indeed, the recurring concept of “probing” was also invoked as a cautionary note in discussions about understanding Mr B, in the sense of overstepping the boundary of professionalism. All in all, then, it seems to be a slight discord between participants’ ethical reasoning and how they report they would act in a case like Mr B’s. As for professional curiosity, this has been recommended in previous literature as an ideal for PC [ 44 , 45 ], and a conceptual link between curiosity and empathy has been described [ 46 ]. At the same time, the thought that the professional must keep his/her curiosity at bay is commonplace in manuals of professional caring [e.g. 47 ]. As expressed in this study, the red line between the right and wrong kinds of curiosity in PC may be both important and hard to pick out. This topic clearly merits further investigations.
Participants claimed that the scenario depicted in the case description demanded that staff be “flexible”. Indeed “flexibility” has been described as an ideal in PC [ 48 , 49 ], and is frequently discussed in relation to practical issues such as scheduling visits [see for instance 50 ]. Interestingly, participants in this study instead used the notion of “flexibility” to discuss the approach to Mr B’s wish to remain uninformed. As noted above, being “flexible” in the sense of abandoning one’s own agenda and uncritically taking the patient’s perspective was not advocated in this material. Instead, the “flexible” attitudes evidenced here were, first, one where staff reported to “accept” Mr B’s preference while holding on to their conviction that he ought to have agreed to being informed, and second one where staff aim to provide information, but in an underhand or cautious way. Again, the notion of “probing” captures a salient aspect of this study. In regards to the probing approach, participants report consciously using the continuing relations within PC to achieve their goal. The possibility to come back and make a new attempt at providing the information later on eases participants’ stress about the patient’s refusal. In this sense, PC work is conceptualized longitudinally with the passing of time as one tool among many, and relations are seen as unfolding not only in discrete interactions but also over series of interactions. It is in this light that participants’ stress on the importance of building and maintaining an open relationship with patients should be seen [compare 51 ]. That the slow probing approach would be in violation of Mr B’s desire not to have the issue brought up again was no big source of worry. Instead the sense was that both the medical situation and Mr B’s preferences might very well change over time. A possible topic for future research would be how PC staff understand the notion of “flexibility” and the ways that this ideal informs professional approaches beyond merely practical issues.
Beyond what was said, it is interesting to note also what was not said. Although the case involves a clear ethical dilemma, the word “ethics” was rarely mentioned and no participant or group attempted to make a structured analysis of the ethical dilemma. Nonetheless, the participants’ discussions about the right to refuse information and the balancing of this right against counteracting factors is bona fide ethics [ 52 ]. Similarly, and perhaps surprisingly, the word “autonomy” was scarce. This could be due to a lack of training in formal ethics, but another interpretation is that the participants did not conceptualize the challenge here as weighing autonomy against beneficence (or similar), but rather as different ways of understanding what autonomy entails. As discussed in the introduction, autonomy is a complex concept and may mean different things in different contexts and according to different people. Indeed, participants explored several autonomy-related questions which are also discussed in theoretical bioethics, such as what makes a decision autonomous [ 21 ]. A final possibility is that within PC, the provision of information is commonly seen as a core feature of respecting a patients’ autonomy [ 53 ], which may make the thought that not providing information could be mandated by autonomy considerations somewhat of a nonstarter.
Although the teams describe the situation as very difficult, the dominant sense in the material is rather that participants are unhappy about Mr B’s preferences than clueless about how to deal with them. For instance, there is no mention of the situation giving rise to moral distress. One reason may be that participants count on time as working in their favour. The participants were self-confident that Mr B would eventually accept information. Hence, awareness of time here seemed to attenuate potential moral distress. This interpretation was supported by the fact that teams admitted there would be greater ethical tension if Mr B had only little time left. Whatever the reason, it would be wrong to construe the ethical qualms witnessed in this material as mere charade. Instead, the recommendations of treading softly and checking and re-checking Mr B’s preferences are motivated by true concern, and they likely represent the participants’ desire to strike a balance between counteracting goals here.
Methodological considerations
An advantage of the study set-up is that participants were recruited from several regions and belonged to various professions, which likely increased variation in answering patterns. Further advantages included using a well described methodological approach with clear and clearly articulated theoretical assumptions. Furthermore, the results were member checked with members from two groups, which makes for increased credibility.
In this as other focus group interview hierarchies and existing power differentials within groups may hamper participants’ communication [ 26 ]. For this particular study, interviewing physicians together with nurses and social workers was nonetheless chosen as this reflected the working circumstances of the PC teams, whose reality the study sought to capture. It should also be pointed out that the study focuses on staff’s ethical reasoning , which may not reflect how they in fact act [ 54 ]. Another aspect of studying ethical reasoning is that participants’ language may sometimes obstruct as much as it elucidates. For instance, participants in this study placed great weight on a perceived difference between “digging” into Mr B’s reasons for changing his mind, which was scorned, and “probing” the same, which was considered praiseworthy. Although this distinction makes intuitive sense, it is an open question whether it corresponds to any difference to a patient such as Mr B.
The author (JB) being himself an ethicist as well as PC physician, having presuppositions about the topic was unavoidable. More precisely, the author entered the project with the following assumptions: that the vignette case raises ethically difficult questions about autonomy, which different ethical theories would read and resolve differently; that such situations are difficult to manage in the clinic, as the patient’s request creates a self-awareness about the exchange of information; and that staff react to a wish such as Mr B’s in a number of different ways. In addition, self-reflexion before and during this project revealed that the author is ideologically convinced about the value of autonomy as an inspirational ideal in PC, yet still struggle to disentangle the different implications of the ideal. There was (only) one aspect of the analytical work that really triggered the author’s own feelings and that was when staff appeared to simplify the complexity in the situation. Whether this coloured the analysis is difficult to judge.
A potential set-back for this study is that the single author is both interviewer and analyst. Although such studies are acceptable within the tradition of reflexive thematic analysis [ 27 ], there is a risk that the single analyst becomes blind to potentially relevant features of the data. During the analysis phase the author used another researcher (Niklas Juth) as a “critical friend”, asking for Juth’s view on some interpretative matters regarding the transcripts and how to analyse the relationships among themes. His input was no substitute for real co-authorship but nevertheless valueable in the process.
In this qualitative study of how PC staff reason about a fictional case in which a patient first expresses a wish to be informed, but then says he does not want information, the main finding is that staff find it hard to “just accept” his preference to remain uninformed. Participants express support for an abstract or contextualized right to remain uninformed, yet pit this against countervailing considerations that, ultimately, are seen as overriding. Through the aid of time and flexibility, participants state that they will likely be able to communicate relevant information without “forcing their way”. The results are instructive in understanding the views of PC staff in regards to autonomy, changing patient preferences, the value of information and professional attitudes. As the themes were engaging as well as challenging to participants in this study, PC teams may do well to discuss the implications of patient autonomy more. Further studies on the topic could explore the meaning of “flexibility” within palliative care, as well as the observed controversies surrounding staff’s private interests, including curiosity and the wish to do a good job.
Data availability
The data generated and analyzed in this study is not publicly available due to ethics restrictions, but is available from the corresponding author on reasonable request.
Forthcoming from the same author is ”Autonomy of the dying – charting the palliative care ethos”.
Abbreviations
- Palliative care
Pugh J. Autonomy, rationality, and contemporary bioethics. New York: Oxford University Press; 2020.
Book Google Scholar
Mackenzie C, Stoljar N. Introduction: autonomy refigured. In: Mackenzie C, Stoljar N, editors. Relational autonomy: Feminist perspectives on autonomy, agency, and the social self. New York: Oxford University Press; 2000.
Chapter Google Scholar
O’Neill O. Autonomy and trust in bioethics. Cambridge: Cambridge University Press; 2002.
Fan R. Self-determination vs. Family-Determination: two Incommensurable principles of Autonomy. Bioethics. 2003;11:309–22.
Article Google Scholar
Lim MYH. Patient autonomy in an East-Asian cultural milieu: a critique of the individualism-collectivism model. J Med Ethics. 2022. https://doi.org/10.1136/medethics-2022-108123 .
Article PubMed Google Scholar
Socialdepartementet. Patientlag (2014:821) [The Patient Law]. Socialdepartementet. Stockholm; 2014.
World Health Organization. WHO Fact sheets: Palliative care. In.; 2020.
Lavoie M, Blondeau D, Martineau I. The integration of a person-centered approach in palliative care. Palliat Support Care. 2013;11(6):453–64.
Cherny NI, Fallon M, Kaasa S, Portenoy RK, Currow D. Oxford Textbook of Palliative Medicine. 5th ed. Oxford: Oxford University Press; 2015.
Wilson F, Ingleton C, Gott M, Gardiner C. Autonomy and choice in palliative care: time for a new model? J Adv Nurs. 2014;70(5):1020–9.
Woods S. Respect for persons, autonomy and palliative care. Med Health Care Philos. 2005;8(2):243–53.
Houska A, Loučka M. Patients’ autonomy at the end of life: a critical review. J Pain Symptom Manage. 2019;57(4):835–45.
Janssens RM, Zylicz Z, Ten Have HA. Articulating the concept of palliative care: philosophical and theological perspectives. J Palliat Care. 1999;15(2):38–44.
Article CAS PubMed Google Scholar
Stoljar N. Autonomy and the feminist intuition. In: Mackenzie C, Stoljar N, editors. Relational autonomy: Feminist perspectives on autonomy, agency, and the social self. New York: Oxford University Press; 2000.
Google Scholar
Johnson SB, Butow PN, Kerridge I, Tattersall MH. Patient autonomy and advance care planning: a qualitative study of oncologist and palliative care physicians’ perspectives. Support Care Cancer. 2018;26(2):565–74.
Owen GS, Szmukler G, Richardson G, David AS, Raymont V, Freyenhagen F, Martin W, Hotopf M. Decision-making capacity for treatment in psychiatric and medical in-patients: cross-sectional, comparative study. Br J Psychiatry. 2013;203(6):461–7.
Article PubMed PubMed Central Google Scholar
Maio GR, Haddock G, Verplanken B. The psychology of attitudes and attitude change, Third edition edn. London: Sage; 2019.
Kjelsvik M, Tveit Sekse RJ, Moi AL, Aasen EM, Gjengedal E. Walking on a tightrope—caring for ambivalent women considering abortions in the first trimester. J Clin Nurs. 2018;27(21–22):4192–202.
Rees M. Patient autonomy and withholding information. Bioethics. 2023;3:256–64.
Schneider CE. After autonomy. Wake for L Rev. 2006;41:411.
Beauchamp TL, Childress JF. Principles of biomedical ethics, Eighth Edition Edn. New York, NY: Oxford University Press; 2019.
Rejnö Å, Ternestedt B-M, Nordenfelt L, Silfverberg G, Godskesen TE. Dignity at stake: caring for persons with impaired autonomy. Nurs Ethics. 2020;27(1):104–15.
Lavoie M, Godin G, Vézina-Im L-A, Blondeau D, Martineau I, Roy L. Psychosocial determinants of physicians’ intention to practice euthanasia in palliative care. BMC Med Ethics. 2015;16(1):6.
Kurkowski S, Heckel M, Pfaller L, Peters J, Bazata J, Schildmann E, Ostgathe C. Possible age-related differences in healthcare professionals’ perspectives on younger and older patients’ autonomy and decision-making in the context of sedation in specialised palliative care: exploratory secondary qualitative content and linguistic conversation analysis of interviews with healthcare professionals. BMC Palliat Care. 2022;21(1):71.
Bekkema N, de Veer AJE, Hertogh CMPM, Francke AL. Respecting autonomy in the end-of-life care of people with intellectual disabilities: a qualitative multiple-case study. J Intellect Disabil Res. 2014;58(4):368–80.
Krueger RA, Casey MA. Focus groups: a practical guide for applied research. 5 ed. Sage; 2014.
Byrne D. A worked example of Braun and Clarke’s approach to reflexive thematic analysis. Qual Quant. 2022;56(3):1391–412.
Braun V, Clarke V. Using thematic analysis in psychology. Qualitative Res Psychol. 2006;3(2):77–101.
Terry G, Hayfield N, Clarke V, Braun V. Thematic analysis. The SAGE handbook of qualitative research in psychology. 2017; 2:17–37.
Schelin ME, Sallerfors B, Rasmussen BH, Fürst CJ. Quality of care for the dying across different levels of palliative care development: a population-based cohort study. Palliat Med. 2018;32(10):1596–604.
Braun V, Clarke V. To saturate or not to saturate? Questioning data saturation as a useful concept for thematic analysis and sample-size rationales. Qualitative Res Sport Exerc Health. 2019:1–16.
Guest G, Namey E, McKenna K. How many focus groups are enough? Building an evidence base for nonprobability sample sizes. Field Methods. 2017;29(1):3–22.
Braun V, Clarke V. Is thematic analysis used well in health psychology? A critical review of published research, with recommendations for quality practice and reporting. Health Psychol Rev. 2023;17(4):695–718.
Baik D, Cho H, Masterson Creber RM. Examining interventions designed to support shared decision making and subsequent patient outcomes in palliative care: a systematic review of the literature. Am J Hosp Palliat Care. 2019;36(1):76–88.
Dive L, Newson AJ. Reconceptualizing autonomy for bioethics. Kennedy Inst Ethics J. 2018;28(2):171–203.
Decision making and consent. [ https://www.gmc-uk.org/-/media/documents/gmc-guidance-for-doctors---decision-making-and-consent-english_pdf-84191055.pdf ] Accessed: 2023-10-15.
Ferrell BR, Paice JA. Oxford textbook of palliative nursing. Oxford University Press; 2019.
García-Baquero Merino MT. Palliative care: taking the long view. Front Pharmacol. 2018;9:1140.
Seow H, Bainbridge D. A review of the essential components of Quality Palliative Care in the home. J Palliat Med. 2018;21(S1):S37–44.
Zimmermann C. Acceptance of dying: a discourse analysis of palliative care literature. Soc Sci Med. 2012;75(1):217–24.
Ågren A, Krevers B, Cedersund E, Nedlund A-C. Policy narratives on Palliative Care in Sweden 1974–2018. Health Care Anal. 2023:1–15.
Slote M. Paternalism and sentimentalism. In: Grill K, Hanna J, editors. The Routledge Handbook of the philosophy of Paternalism. Abingdon: Routledge; 2018.
Hilding U, Allvin R, Blomberg K. Striving for a balance between leading and following the patient and family – nurses’ strategies to facilitate the transition from life-prolonging care to palliative care: an interview study. BMC Palliat Care. 2018;17(1):55.
Ferrell B. Curiosity and Compassion. J Hospice Palliat Nurs. 2018;20(5):427–8.
Rosenberg LB, Brenner KO, Jackson VA, Jacobsen JC, Shalev D, Byrne-Martelli S, Cramer MA. The meaning of together: exploring transference and countertransference in Palliative Care settings. J Palliat Med. 2021;24(11):1598–602.
Halpern J. From idealized clinical empathy to empathic communication in medical care. Med Health Care Philos. 2014;17(2):301–11.
Katz RS, Johnson TA. When professionals weep: emotional and countertransference responses in Palliative and End-of-Life Care. Taylor & Francis; 2016.
Carduff E, Johnston S, Winstanley C, Morrish J, Murray SA, Spiller J, Finucane A. What does ‘complex’ mean in palliative care? Triangulating qualitative findings from 3 settings. BMC Palliat Care. 2018;17(1):12.
Philip JA, Komesaroff P. Ideals and compromises in palliative care. J Palliat Med. 2006;9(6):1339–47.
Danielsen BV, Sand AM, Rosland JH, Førland O. Experiences and challenges of home care nurses and general practitioners in home-based palliative care–a qualitative study. BMC Palliat Care. 2018;17:1–13.
Hawthorne DL, Yurkovich NJ. Human relationship: the forgotten dynamic in palliative care. Palliat Support Care. 2003;1(3):261–5.
Scher S, Kozlowska K. Rethinking health care ethics. Singapore: Palgrave Pivot; 2018.
Zalonis R, Slota M. The use of palliative care to promote autonomy in decision making. Clin J Oncol Nurs. 2014;18(6):707–11.
Ellemers N, van der Toorn J, Paunov Y, van Leeuwen T. Personality Social Psychol Rev. 2019;23(4):332–66. The Psychology of Morality: A Review and Analysis of Empirical Studies Published From 1940 Through 2017.
World Medical Association. Declaration of Helsinki: ethical principles for medical research involving human subjects. JAMA. 2013;310(20):2191–4.
Download references
Acknowledgements
The generous help from Niklas Juth in critically assessing some aspects of the research material and the analysis, and from Ingela Björck with transcription of the focus group interviews is gratefully acknowledged.
Open access funding provided by Uppsala University.
Author information
Authors and affiliations.
Centre for Research Ethics and Bioethics (CRB), Department of Public Health and Caring Sciences, Uppsala University, Uppsala, Sweden
Department of Research and Development, Region Kronoberg, Växjö, Sweden
Stockholm Centre for Healthcare Ethics (CHE), LIME, Karolinska Institutet, Stockholm, Sweden
You can also search for this author in PubMed Google Scholar
Contributions
JB is the sole author of this manuscript.
Corresponding author
Correspondence to Joar Björk .
Ethics declarations
Ethics approval and consent to participate.
All methods were performed in accordance with the Declaration of Helsinki [ 55 ]. Ethics approval for the study was sought from the Swedish Ethical Review Authority (registration number 2022-00142-01). In their response, the authority stated that a study such as this does not need formal approval. All participants were informed about the study orally and in writing in advance, including that participation was voluntary. All participants gave their oral informed consent to participate and publish anonymised data. As some interviewed teams were small and might have only one team member of a particular professional background, anonymisation of quotes entailed giving interviews arbitrary numbers rather than stating where interviews were held, and not stating the quoted participant’s professional background.
Consent for publication
Competing interests.
The author declares no competing interests.
Additional information
Publisher’s note.
Springer Nature remains neutral with regard to jurisdictional claims in published maps and institutional affiliations.
Electronic supplementary material
Below is the link to the electronic supplementary material.
Supplementary Material 1
Rights and permissions.
Open Access This article is licensed under a Creative Commons Attribution 4.0 International License, which permits use, sharing, adaptation, distribution and reproduction in any medium or format, as long as you give appropriate credit to the original author(s) and the source, provide a link to the Creative Commons licence, and indicate if changes were made. The images or other third party material in this article are included in the article’s Creative Commons licence, unless indicated otherwise in a credit line to the material. If material is not included in the article’s Creative Commons licence and your intended use is not permitted by statutory regulation or exceeds the permitted use, you will need to obtain permission directly from the copyright holder. To view a copy of this licence, visit http://creativecommons.org/licenses/by/4.0/ . The Creative Commons Public Domain Dedication waiver ( http://creativecommons.org/publicdomain/zero/1.0/ ) applies to the data made available in this article, unless otherwise stated in a credit line to the data.
Reprints and permissions
About this article
Cite this article.
Björk, J. “It is very hard to just accept this” – a qualitative study of palliative care teams’ ethical reasoning when patients do not want information. BMC Palliat Care 23 , 91 (2024). https://doi.org/10.1186/s12904-024-01412-8
Download citation
Received : 05 November 2023
Accepted : 15 March 2024
Published : 05 April 2024
DOI : https://doi.org/10.1186/s12904-024-01412-8
Share this article
Anyone you share the following link with will be able to read this content:
Sorry, a shareable link is not currently available for this article.
Provided by the Springer Nature SharedIt content-sharing initiative
- Health care staff
- Qualitative research
- Informed consent
- Beneficence
BMC Palliative Care
ISSN: 1472-684X
- Submission enquiries: [email protected]
- General enquiries: [email protected]
- Open access
- Published: 10 April 2024
“So at least now I know how to deal with things myself, what I can do if it gets really bad again”—experiences with a long-term cross-sectoral advocacy care and case management for severe multiple sclerosis: a qualitative study
- Anne Müller ORCID: orcid.org/0000-0002-2456-2492 1 ,
- Fabian Hebben ORCID: orcid.org/0009-0003-6401-3433 1 ,
- Kim Dillen 1 ,
- Veronika Dunkl 1 ,
- Yasemin Goereci 2 ,
- Raymond Voltz 1 , 3 , 4 ,
- Peter Löcherbach 5 ,
- Clemens Warnke ORCID: orcid.org/0000-0002-3510-9255 2 &
- Heidrun Golla ORCID: orcid.org/0000-0002-4403-630X 1
on behalf of the COCOS-MS trial group represented by Martin Hellmich
BMC Health Services Research volume 24 , Article number: 453 ( 2024 ) Cite this article
Metrics details
Persons with severe Multiple Sclerosis (PwsMS) face complex needs and daily limitations that make it challenging to receive optimal care. The implementation and coordination of health care, social services, and support in financial affairs can be particularly time consuming and burdensome for both PwsMS and caregivers. Care and case management (CCM) helps ensure optimal individual care as well as care at a higher-level. The goal of the current qualitative study was to determine the experiences of PwsMS, caregivers and health care specialists (HCSs) with the CCM.
In the current qualitative sub study, as part of a larger trial, in-depth semi-structured interviews with PwsMS, caregivers and HCSs who had been in contact with the CCM were conducted between 02/2022 and 01/2023. Data was transcribed, pseudonymized, tested for saturation and analyzed using structuring content analysis according to Kuckartz. Sociodemographic and interview characteristics were analyzed descriptively.
Thirteen PwsMS, 12 caregivers and 10 HCSs completed interviews. Main categories of CCM functions were derived deductively: (1) gatekeeper function, (2) broker function, (3) advocacy function, (4) outlook on CCM in standard care. Subcategories were then derived inductively from the interview material. 852 segments were coded. Participants appreciated the CCM as a continuous and objective contact person, a person of trust (92 codes), a competent source of information and advice (on MS) (68 codes) and comprehensive cross-insurance support (128 codes), relieving and supporting PwsMS, their caregivers and HCSs (67 codes).
Conclusions
Through the cross-sectoral continuous support in health-related, social, financial and everyday bureaucratic matters, the CCM provides comprehensive and overriding support and relief for PwsMS, caregivers and HCSs. This intervention bears the potential to be fine-tuned and applied to similar complex patient groups.
Trial registration
The study was approved by the Ethics Committee of the University of Cologne (#20–1436), registered at the German Register for Clinical Studies (DRKS00022771) and in accordance with the Declaration of Helsinki.
Peer Review reports
Introduction
Multiple sclerosis (MS) is the most frequent and incurable chronic inflammatory and degenerative disease of the central nervous system (CNS). Illness awareness and the number of specialized MS clinics have increased since the 1990s, paralleled by the increased availability of disease-modifying therapies [ 1 ]. There are attempts in the literature for the definition of severe MS [ 2 , 3 ]. These include a high EDSS (Expanded disability Status Scale [ 4 ]) of ≥ 6, which we took into account in our study. There are also other factors to consider, such as a highly active disease course with complex therapies that are associated with side effects. These persons are (still) less disabled, but may feel overwhelmed with regard to therapy, side effects and risk monitoring of therapies [ 5 , 6 ].
Persons with severe MS (PwsMS) develop individual disease trajectories marked by a spectrum of heterogeneous symptoms, functional limitations, and uncertainties [ 7 , 8 ] manifesting individually and unpredictably [ 9 ]. This variability can lead to irreversible physical and mental impairment culminating in complex needs and daily challenges, particularly for those with progressive and severe MS [ 5 , 10 , 11 ]. Such challenges span the spectrum from reorganizing biographical continuity and organizing care and everyday live, to monitoring disease-specific therapies and integrating palliative and hospice care [ 5 , 10 ]. Moreover, severe MS exerts a profound of social and economic impact [ 9 , 12 , 13 , 14 ]. PwsMS and their caregivers (defined in this manuscript as relatives or closely related individuals directly involved in patients’ care) often find themselves grappling with overwhelming challenges. The process of organizing and coordinating optimal care becomes demanding, as they contend with the perceived unmanageability of searching for, implementing and coordinating health care and social services [ 5 , 15 , 16 , 17 ].
Case management (CM) proved to have a positive effect on patients with neurological disorders and/or patients with palliative care needs [ 17 , 18 , 19 , 20 , 21 , 22 , 23 , 24 ]. However, a focus on severe MS has been missed so far Case managers primarily function as: (1) gatekeeper involving the allocation of necessary and available resources to a case, ensuring the equitable distribution of resources; as (2) broker assisting clients in pursuing their interests, requiring negotiation to provide individualized assistance that aligns as closely as possible with individual needs and (3) advocate working to enhance clients’ individual autonomy, to advocate for essential care offers, and to identify gaps in care [ 25 , 26 , 27 , 28 , 29 ].
Difficulties in understanding, acting, and making decisions regarding health care-related aspects (health literacy) poses a significant challenge for 54% of the German population [ 30 ]. Additionally acting on a superordinate level as an overarching link, a care and case management (CCM) tries to reduce disintegration in the social and health care system [ 31 , 32 ]. Our hypothesis is that a CCM allows PwsMS and their caregivers to regain time and resources outside of disease management and to facilitate the recovery and establishment of biographical continuity that might be disrupted due to severe MS [ 33 , 34 ].
Health care specialists (HCSs) often perceive their work with numerous time and economic constraints, especially when treating complex and severely ill individuals like PwsMS and often have concerns about being blamed by patients when expectations could not be met [ 35 , 36 ]. Our hypothesis is that the CCM will help to reduce time constraints and free up resources for specialized tasks.
To the best of our knowledge there is no long-term cross-sectoral and outreaching authority or service dedicated to assisting in the organization and coordination of the complex care concerns of PwsMS within the framework of standard care addressing needs in health, social, financial, every day and bureaucratic aspects. While some studies have attempted to design and test care programs for persons with MS (PwMS), severely affected individuals were often not included [ 37 , 38 , 39 ]. They often remain overlooked by existing health and social care structures [ 5 , 9 , 15 ].
The COCOS-MS trial developed and applied a long-term cross-sectoral CCM intervention consisting of weekly telephone contacts and monthly re-assessments with PwsMS and caregivers, aiming to provide optimal care. Their problems, resources and (unmet) needs were assessed holistically including physical health, mental health, self-sufficiency and social situation and participation. Based on assessed (unmet) needs, individual care plans with individual actions and goals were developed and constantly adapted during the CCM intervention. Contacts with HCSs were established to ensure optimal care. The CCM intervention was structured through and documented in a CCM manual designed for the trial [ 40 , 41 ].
Our aim was to find out how PwsMS, caregivers and HCSs experienced the cross-sectoral long-term, outreaching patient advocacy CCM.
This study is part of a larger phase II, randomized, controlled clinical trial “Communication, Coordination and Security for people with severe Multiple Sclerosis (COCOS-MS)” [ 41 ]. This explorative clinical trial, employing a mixed-method design, incorporates a qualitative study component with PwsMS, caregivers and HCSs to enrich the findings of the quantitative data. This manuscript focuses on the qualitative data collected between February 2022 and January 2023, following the Consolidated Criteria for Reporting Qualitative Research (COREQ) guidelines [ 42 ].
Research team
Three trained authors AM, KD and FH (AM, female, research associate, M.A. degree in Rehabilitation Sciences; KD, female, researcher, Dr. rer. medic.; FH, male, research assistant, B.Sc. degree in Health Care Management), who had no prior relationship with patients, caregivers or HCSs conducted qualitative interviews. A research team, consisting of clinical experts and health services researchers, discussed the development of the interview guides and the finalized category system.
Theoretical framework
Interview data was analyzed with the structuring content analysis according to Kuckartz. This method enables a deductive structuring of interview material, as well as the integration of new aspects found in the interview material through the inductive addition of categories in an iterative analysis process [ 43 ].
Sociodemographic and interview characteristics were analyzed descriptively (mean, median, range, SD). PwsMS, caregivers and HCSs were contacted by the authors AM, KD or FH via telephone or e-mail after providing full written informed consent. Participants had the option to choose between online interviews conducted via the GoToMeeting 10.19.0® Software or face-to-face. Peasgood et al. (2023) found no significant differences in understanding questions, engagement or concentration between face-to-face and online interviews [ 44 , 45 ]. Digital assessments were familiar to participants due to pandemic-related adjustments within the trial.
Out of 14 PwsMS and 14 caregivers who were approached to participate in interviews, three declined to complete interviews, resulting in 13 PwsMS (5 male, 8 female) and 12 caregiver (7 male, 5 female) interviews, respectively (see Fig. 1 ). Thirty-one HCSs were contacted of whom ten (2 male, 8 female) agreed to be interviewed (see Fig. 2 ).
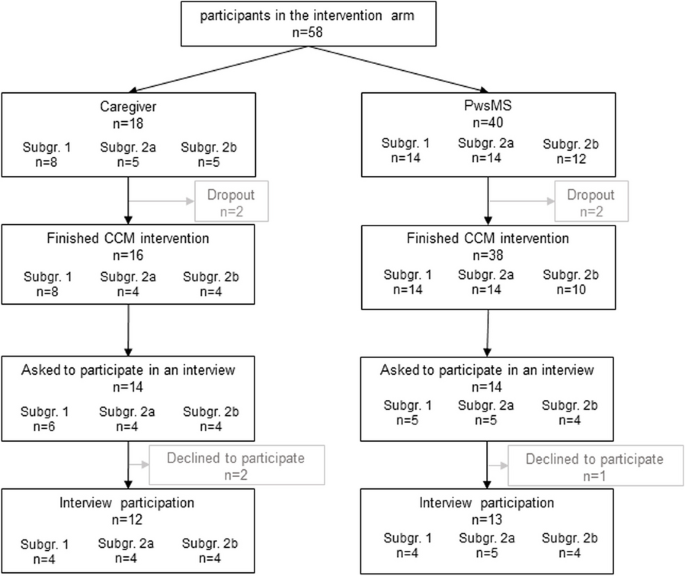
Flowchart of PwsMS and caregiver participation in the intervention group of the COCOS-MS trial. Patients could participate with and without a respective caregiver taking part in the trial. Therefore, number of caregivers does not correspond to patients. For detailed inclusion criteria see also Table 1 in Golla et al. [ 41 ]
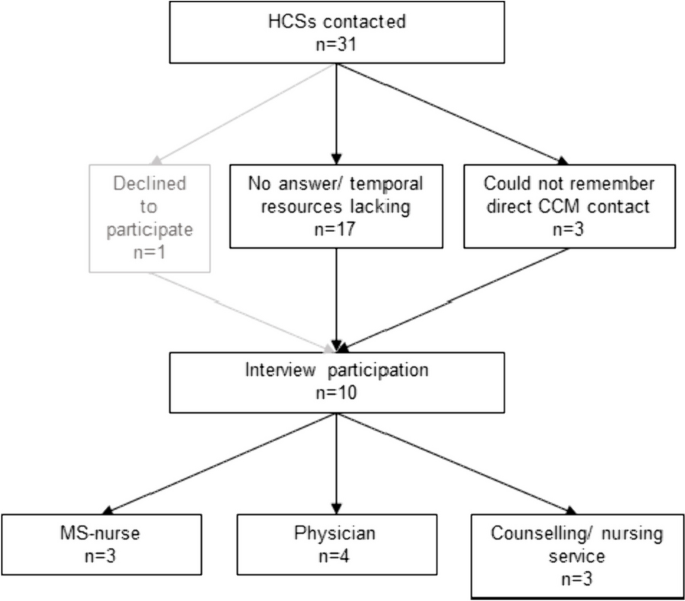
Flowchart of HCSs interview participation
Setting and data collection
Interviews were carried out where participants preferred, e.g. at home, workplace, online, and no third person being present. In total, we conducted 35 interviews whereof 7 interviews face-to-face (3 PwsMS, 3 caregivers, 1 HCS).
The research team developed a topic guide which was meticulously discussed with research and clinical staff to enhance credibility. It included relevant aspects for the evaluation of the CCM (see Tables 1 and 2 , for detailed topic guides see Supplementary Material ). Patient and caregiver characteristics (covering age, sex, marital status, living situation, EDSS (patients only), subgroup) were collected during the first assessment of the COCOS-MS trial and HCSs characteristics (age, sex, profession) as well as interview information (length and setting) were collected during the interviews. The interview guides developed for this study addressed consistent aspects both for PwsMS and caregivers (see Supplementary Material ):
For HCSs it contained the following guides:
Probing questions were asked to get more specific and in-depth information. Interviews were carried out once and recorded using a recording device or the recording function of the GoToMeeting 10.19.0® Software. Data were pseudonymized (including sensitive information, such as personal names, dates of birth, or addresses), audio files were safely stored in a data protection folder. The interview duration ranged from 11 to 56 min (mean: 23.9 min, SD: 11.1 min). Interviews were continued until we found that data saturation was reached. Audio recordings were transcribed verbatim by an external source and not returned to participants.
Data analysis
Two coders (AM, FH) coded the interviews. Initially, the first author (AM) thoroughly reviewed the transcripts to gain a sense of the interview material. Using the topic guide and literature, she deductively developed a category system based on the primary functions of CM [ 25 , 26 , 27 , 28 , 29 ]. Three interviews were coded repeatedly for piloting, and inductive subcategories were added when new themes emerged in the interview material. This category system proved suitable for the interview material. The second coder (FH) familiarized himself with the interview material and category system. Both coders (AM, FH) independently coded all interviews, engaging in discussions and adjusting codes iteratively. The finalized category system was discussed and consolidated in a research workshop and within the COCOS-MS trial group and finally we reached an intercoder agreement of 90% between the two coders AM and FH, computed by the MAXQDA Standard 2022® software.
We analyzed sociodemographic and interview characteristics using IBM SPSS Statistics 27® and Excel 2016®. Transcripts were managed and analyzed using MAXQDA Standard 2022®.
Participants were provided with oral and written information about the trial and gave written informed consent. Ethical approvals were obtained from the Ethics Committee of the University of Cologne (#20–1436). The trial is registered in the German Register for Clinical Studies (DRKS) (DRKS00022771) and is conducted under the Declaration of Helsinki.
Characteristics of participants and interviews
PwsMS participating in an interview were mainly German (84.6%), had a mean EDSS of 6.8 (range: 6–8) and MS for 13.5 years (median: 14; SD: 8.1). For detailed characteristics see Table 3 .
Most of the interviewed caregivers (9 caregivers) were the partners of the PwsMS with whom they lived in the same household. For further details see Table 3 .
HCSs involved in the study comprised various professions, including MS-nurse (3), neurologist (2), general physician with further training in palliative care (1), physician with further training in palliative care and pain therapist (1), housing counselling service (1), outpatient nursing service manager (1), participation counselling service (1).
Structuring qualitative content analysis
The experiences of PwsMS, caregivers and HCSs were a priori deductively assigned to four main categories: (1) gatekeeper function, (2) broker function, (3) advocacy function [ 25 , 26 , 27 , 28 , 29 ] and (4) Outlook on CCM in standard care, whereas the subcategories were developed inductively (see Fig. 3 ).
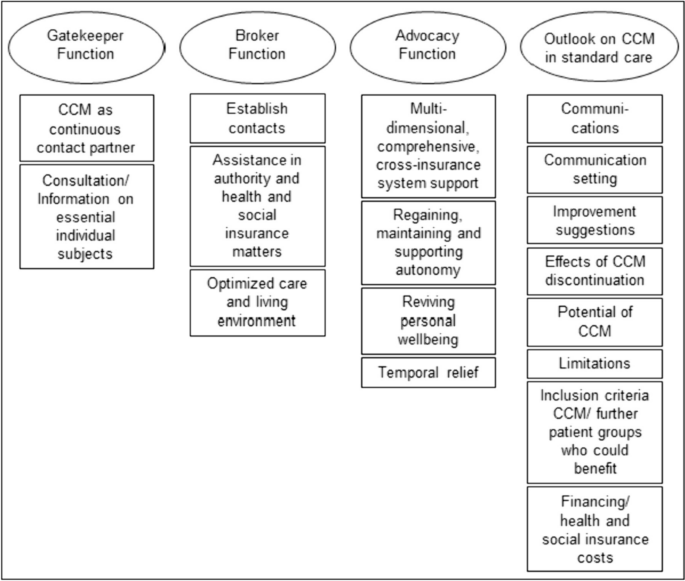
Category system including main and subcategories of the qualitative thematic content analysis
The most extensive category, housing the highest number of codes and subcodes, was the “ Outlook on CCM in standard care ” (281 codes). Following this, the category “ Advocacy Function ” contained 261 codes. The “ Broker Function ” (150 codes) and the “ Gatekeeper Function ” (160 codes) constituted two smaller categories. The majority of codes was identified in the caregivers’ interviews, followed by those of PwsMS (see Table 4 ). Illustrative quotes for each category and subcategory can be found in Table 5 .
Persons with severe multiple sclerosis
In the gatekeeper function (59 codes), PwsMS particularly valued the CCM as a continuous contact person . They appreciated the CCM as a person of trust who was reliably accessible throughout the intervention period. This aspect, with 41 codes, held significant importance for PwsMS.
Within the broker function (44 codes), establishing contact was most important for PwsMS (22 codes). This involved the CCM as successfully connecting PwsMS and caregivers with physicians and therapists, as well as coordinating and arranging medical appointments, which were highly valued. Assistance in authority and health and social insurance matters (10 codes) was another subcategory, where the CCM encompassed support in communication with health insurance companies, such as improving the level of care, assisting with retirement pension applications, and facilitating rehabilitation program applications. Optimized care (12 codes) resulted in improved living conditions and the provision of assistive devices through the CCM intervention.
The advocacy function (103 codes) emerged as the most critical aspect for PwsMS, representing the core of the category system. PwsMS experienced multidimensional, comprehensive, cross-insurance system support from the CCM. This category, with 43 statements, was the largest within all subcategories. PwsMS described the CCM as addressing their concerns, providing help, and assisting with the challenges posed by the illness in everyday life. The second-largest subcategory, regaining, maintaining and supporting autonomy (25 codes), highlighted the CCM’s role in supporting self-sufficiency and independence. Reviving personal wellbeing (17 codes) involved PwsMSs’ needs of regaining positive feelings, improved quality of life, and a sense of support and acceptance, which could be improved by the CCM. Temporal relief (18 codes) was reported, with the CCM intervention taking over or reducing tasks.
Within the outlook on CCM in standard care (84 codes), eight subcategories were identified. Communications was described as friendly and open (9 codes), with the setting of communication (29 codes) including the frequency of contacts deemed appropriate by the interviewed PwsMS, who preferred face-to-face contact over virtual or telephone interactions. Improvement suggestions for CCM (10 codes) predominantly revolved around the desire for the continuation of the CCM beyond the trial, expressing intense satisfaction with the CCM contact person and program. PwsMS rarely wished for better cooperation with the CCM. With respect to limitations (7 codes), PwsMS distinguished between individual limitations (e.g. when not feeling ready for using a wheelchair) and overriding structural limitations (e.g. unsuccessful search for an accessible apartment despite CCM support). Some PwsMS mentioned needing the CCM earlier in the course of the disease and believed it would beneficial for anyone with a chronic illness (6 codes).
In the gatekeeper function (75 codes), caregivers highly valued the CCM as a continuous contact partner (33 codes). More frequently than among the PwsMS interviewed, caregivers valued the CCM as a source of consultation/ information on essential individual subjects (42 codes). The need for basic information about the illness, its potential course, treatment and therapy options, possible supportive equipment, and basic medical advice/ information could be met by the CCM.
Within the broker function (63 codes), caregivers primarily experienced the subcategory establish contacts (24 codes). They found the CCM as helpful in establishing and managing contact with physicians, therapists and especially with health insurance companies. In the subcategory assistance in authority and health and social insurance matters (22 codes), caregivers highlighted similar aspects as the PwsMS interviewed. However, there was a particular emphasis on assistance with patients' retirement matters. Caregivers also valued the optimization of patients’ care and living environment (17 codes) in various life areas during the CCM intervention, including improved access to assistive devices, home modification, and involvement of a household support and/ or nursing services.
The advocacy function, with 115 codes, was by far the broadest category . The subcategory multidimensional, comprehensive, cross-insurance system support represented the largest subcategory of caregivers, with 70 statements. In summary, caregivers felt supported by the CCM in all domains of life. Regaining, maintaining and supporting autonomy (11 codes) and reviving personal wellbeing (8 codes) in the form of an improved quality of life played a role not only for patients but also for caregivers, albeit to a lower extend. Caregivers experienced temporal relief (26 codes) as the CCM undertook a wide range of organizational tasks, freeing up more needed resources for their own interests.
For the Outlook on CCM in standard care , caregivers provided various suggestions (81 codes). Similar to PwsMS, caregivers felt that setting (home based face-to-face, telephone, virtual) and frequency of contact were appropriate (10 codes, communication setting ) and communications (7 codes) were recognized as open and friendly. However, to avoid conflicts between caregiver and PwsMS, caregivers preferred meeting the CCM separately from the PwsMS in the future. Some caregivers wished the CCM to specify all services it might offer at the beginning, while others emphasized not wanting this. Like PwsMS, caregivers criticized the CCM intervention being (trial-related) limited to one year, regardless of whether further support was needed or processes being incomplete (13 codes, improvement suggestions ). After the CCM intervention time had expired, the continuous contact person and assistance were missed and new problems had arisen and had to be managed with their own resources again (9 codes, effects of CCM discontinuation ), which was perceived as an exhausting or unsolvable endeavor. Caregivers identified analogous limitations (8 codes), both individual and structural. However, the largest subcategory, was the experienced potential of CCM (27 codes), reflected in extremely high satisfaction with the CCM intervention. Like PwsMS, caregivers regarded severe chronically ill persons in general as target groups for a CCM (7 codes) and would implement it even earlier, starting from the time of diagnosis. They considered a CCM to be particularly helpful for patients without caregivers or for caregivers with limited (time) resources, as it was true for most caregivers.
Health care specialists
In the gatekeeper function (26 codes) HCSs particularly valued the CCM as a continuous contact partner (18 codes). They primarily described their valuable collaboration with the CCM, emphasizing professional exchange between the CCM and HCSs.
Within the broker function (43 codes), the CCM was seen as a connecting link between patients and HCSs, frequently establishing contacts (18 codes). This not only improved optimal care on an individual patient level (case management) but also at a higher, superordinate care level (care management). HCSs appreciated the optimized care and living environment (18 codes) for PwsMS, including improved medical and therapeutic access and the introduction of new assistive devices. The CCM was also recognized as providing assistance in authority and health and social matters (7 codes) for PwsMS and their caregivers.
In the advocacy function (43 codes), HCSs primarily reported temporal relief through CCM intervention (23 codes). They experienced this relief, especially as the CCM provided multidimensional, comprehensive, and cross-insurance system support (15 codes) for PwsMS and their caregivers. Through this support, HCSs felt relieved from time intensive responsibilities that may not fall within their area of expertise, freeing up more time resources for their actual professional tasks.
The largest category within the HCSs interviews was the outlook on CCM in standard care (116 codes). In the largest subcategory, HCSs made suggestions for further patient groups who could benefit (38 codes) from a CCM. Chronic neurological diseases like neurodegenerative diseases (e.g. amyotrophic lateral sclerosis), typical and atypical Parkinson syndromes were mentioned. HCSs considered the enrollment of the CCM directly after the diagnosis of these complex chronic diseases. Additionally, chronic progressive diseases in general or oncological diseases, which may also run chronically, were regarded worthwhile for this approach. HCSs also provided suggestions regarding improvement (21 codes). They wished e.g. for information or contact when patients were enrolled to the CCM, regular updates, exchange and collaborative effort. On the other hand, HCSs reported, that their suggestions for improvement would hardly be feasible due to their limited time resources. Similar to patients and caregivers, HCSs experienced structural limits (13 codes), which a CCM could not exceed due to overriding structural limitations (e.g. insufficient supply of (household) aids, lack of outreach services like psychotherapists, and long processing times on health and pension insurers' side). HCSs were also asked about their opinions on financial resources (14 codes) of a CCM in standard care. All interviewed HCSs agreed that CCM would initially cause more costs for health and social insurers, but they were convinced of cost savings in the long run. HCSs particularly perceived the potential of the CCM (20 codes) through the feedback of PwsMS, highlighting the trustful relationship enabling individualized help for PwsMS and their caregivers.
Persons with severe multiple sclerosis and their caregivers
The long-term cross-sectoral CCM intervention implemented in the COCOS-MS trial addressed significant unmet needs of PwsMS and their caregivers which previous research revealed as burdensome and hardly or even not possible to improve without assistance [ 5 , 6 , 9 , 10 , 33 , 35 , 46 ]. Notably, the CCM service met the need for a reliable, continuous contact partner, guiding patients through the complexities of regulations, authorities and the insurance system. Both, PwsMS and their caregivers highly valued the professional, objective perspective provided by the CCM, recognizing it as a source of relief, support and improved care in line with previous studies [ 37 , 47 ]. Caregivers emphasized the CCM’s competence in offering concrete assistance and information on caregiving and the fundamentals of MS, including bureaucratic, authority and insurances matters. On the other hand, PwsMS particularly appreciated the CCMs external reflective and advisory function, along with empathic social support tailored to their individual concerns. Above all, the continuous partnership of trust, available irrespective of the care sector, was a key aspect that both PwsMS and their caregivers highlighted. This consistent support was identified as one of the main components in the care of PwsMS in previous studies [ 5 , 33 , 35 ].
As the health literacy is inadequate or problematic for 54% of the German population and disintegration in the health and social care system is high [ 30 , 31 , 32 ], the CCM approach serves to enhance health literacy and reduce disintegration of PwsMS and their caregivers by providing cross-insurance navigational guidance in the German health and social insurance sector on a superordinate level. Simultaneously PwsMS and caregivers experienced relief and gained more (time) resources for all areas of life outside of the disease and its management, including own interests and establishing biographical continuity. This empowerment enables patients to find a sense of purpose beyond their illness, regain autonomy, and enhance social participation, reducing the feeling of being a burden to those closest to them. Such feelings are often experienced as burdensome and shameful by PwsMS [ 6 , 48 , 49 , 50 ]. Finding a sense of purpose beyond the illness also contributes to caregivers perceiving their loved ones not primarily as patient but as individuals outside of the disease, reinforcing valuable relationships such as partners, siblings, or children, strengthening emotional bonds. These factors are also highly relevant and well-documented in a suicide-preventive context, as the suicide rate is higher in persons diagnosed with neurological disorders [ 19 , 51 , 52 , 53 , 54 , 55 , 56 , 57 , 58 , 59 , 60 ] and the feeling of being a burden to others, loss of autonomy, and perceived loss of dignity are significant factors in patients with severe chronic neurological diseases for suicide [ 50 , 57 ].
The temporal relief experienced by the CCM was particularly significant for HCSs and did not only improve the satisfaction of HCSs but also removed unfulfilled expectations and concerns about being blamed by patients when expectations could not be met, which previous studied elaborated [ 35 , 36 ]. Moreover, the CCM alleviated the burden on HCSs by addressing patients’ concerns, allowing them to focus on their own medical responsibilities. This aspect probably reduced the dissatisfaction that arises when HCSs are expected to address issues beyond their medical expertise, such as assistive devices, health and social insurance, and the organization and coordination of supplementary therapies, appointments, and contacts [ 35 , 36 , 61 ]. Consequently, the CCM reduced difficulties of HCSs treating persons with neurological or chronical illnesses, which previous research identified as problematic.
HCSs perceive their work as increasingly condensed with numerous time and economic constraints, especially when treating complex and severely ill individuals like PwsMS [ 36 ]. This constraint was mentioned by HCSs in the interviews and was one of the main reasons why they were hesitant to participate in interviews and may also be an explanation for a shorter interview duration than initially planned in the interview guides. The CCM’s overarching navigational competence in the health and social insurance system was particularly valued by HCSs. The complex and often small-scale specialties in the health and social care system are not easily manageable or well-known even for HCSs, and dealing with them can exceed their skills and time capacities [ 61 ]. The CCM played a crucial role in keeping (temporal) resources available for what HCSs are professionally trained and qualified to work on. However, there remains a challenge in finding solutions to the dilemma faced by HCSs regarding their wish to be informed about CCM procedures and linked with each other, while also managing the strain of additional requests and contact with the CCM due to limited (time) resources [ 62 ]. Hudon et al. (2023) suggest that optimizing time resources and improving exchange could involve meetings, information sharing via fax, e-mail, secure online platforms, or, prospectively, within the electronic patient record (EPR). The implementation of an EPR has shown promise in improving the quality of health care and time resources, when properly implemented [ 63 , 64 ]. The challenge lies ineffective information exchange between HCSs and CCM for optimal patient care. The prospect of time saving in the long run and at best for a financial incentive, e.g., when anchoring in the Social Security Code, will help best to win over the HCSs.If this crucial factor can be resolved, there is a chance that HCSs will thoroughly accept the CCM as an important pillar, benefiting not only PwsMS but also other complex patient groups, especially those with long-term neurological or complex oncological conditions that might run chronically.
Care and case management and implications for the health care system
The results of our study suggest that the cross-sectoral long-term advocacy CCM in the COCOS-MS trial, with continuous personal contacts at short intervals and constant reevaluation of needs, problems, resources and goals, is highly valued by PwsMS, caregivers, and HCSs. The trial addresses several key aspects that may have been overlooked in previous studies which have shown great potential for the integration of case management [ 17 , 47 , 62 , 65 , 66 ]. However, they often excluded the overriding care management, missed those patient groups with special severity and complexity who might struggle to reach social and health care structures independently or the interventions were not intended for long-term [ 22 , 37 ]. Our results indicate that the CCM intervention had a positive impact on PwsMS and caregivers as HCSs experienced them with benefits such as increased invigoration, reduced demands, and enhanced self-confidence. However, there was a notable loss experienced by PwsMS and caregivers after the completion of the CCM intervention, even if they had stabilized during the intervention period. The experiences of optimized social and health care for the addressed population, both at an individual and superordinate care level, support the integration of this service into standard care. Beyond the quantitatively measurable outcomes and economic considerations reported elsewhere [ 16 , 20 , 21 ], our results emphasize the importance of regaining control, self-efficacy, self-worth, dignity, autonomy, and social participation. These aspects are highlighted as preventive measures in suicidal contexts, which is particularly relevant for individuals with severe and complex illnesses [ 19 , 50 , 53 , 54 , 55 , 56 , 57 , 58 , 59 , 60 ]. Our findings further emphasize the societal responsibilities to offer individuals with severe and complex illnesses the opportunity to regain control and meaningful aspects of life, irrespective of purely economic considerations. This underscores the need for a comprehensive evaluation that not only takes into account quantitative measures but also the qualitative aspects of well-being and quality of life when making recommendations of a CCM in standard care.
The study by J. Y. Joo and Huber (2019) highlighted that CM interventions aligned with the standards of the Case Management Society of America varied in duration, ranging from 1 month to 15.9 years, and implemented in community- or hospital-based settings. However, they noted a limitation in understanding how CM processes unfold [ 67 ]. In contrast, our trial addressed this criticism by providing transparent explanations of the CCM process, which also extends to a superordinate care management [ 40 , 41 ]. Our CCM manual [ 40 ] outlines a standardized and structured procedure for measuring and reevaluating individual resources, problems, and unmet needs on predefined dimensions. It also identifies goals and actions at reducing unmet needs and improving the individual resources of PwsMS and caregivers. Importantly, the CCM manual demonstrates that the CCM process can be structured and standardized, while accounting for the unique aspects of each individual’s serious illness, disease courses, complex needs, available resources, and environmental conditions. Furthermore, the adaptability of the CCM manual to other complex chronically ill patient groups suggests the potential for a standardized approach in various health care settings. This standardized procedure allows for consistency in assessing and addressing the individual needs of patients, ensuring that the CCM process remains flexible while maintaining a structured and goal-oriented framework.
The discussion about the disintegration in the social and health care system and the increasing specialization dates back to 2009 [ 31 , 32 ]. Three strategies were identified to address this issue: (a) “driver-minimizing” [Treiberminimierende], (b) “effect-modifying” [Effektmodifizierende] and (c) “disintegration-impact-minimizing” [Desintegrationsfolgenminimierende] strategies. “Driver-minimizing strategies” involve comprehensive and radical changes within the existing health and social care system, requiring political and social pursuit. “Disintegration-impact-minimizing strategies” are strategies like quality management or tele-monitoring, which are limited in scope and effectiveness. “Effect-modifying strategies”, to which CCM belongs, acknowledges the segmentation within the system but aims to overcome it through cooperative, communicative, and integrative measures. CCM, being an “effect-modifying strategy”, operates the “integrated segmentation model” [Integrierte Segmentierung] rather than the “general contractor model” [Generalunternehmer-Modell] or “total service provider model” [Gesamtdienstleister-Modell] [ 31 , 32 ]. In this model, the advantage lies in providing an overarching and coordinating service to link different HCSs and services cross-sectorally. The superordinate care management aspect of the CCM plays a crucial role in identifying gaps in care, which is essential for future development strategies within the health and social care system. It aims to find or develop (regional) alternatives to ensure optimal care [ 17 , 23 , 24 , 68 , 69 ], using regional services of existing health and social care structures. Therefore, superordinate care management within the CCM process is decisive for reducing disintegration in the system.
Strengths and limitations
The qualitative study results of the explorative COCOS-MS clinical trial, which employed an integrated mixed-method design, provide valuable insights into the individual experiences of three leading stakeholders: PwsMS, caregivers and HCSs with a long-term cross-sectoral CCM. In addition to in-depth interviews, patient and caregiver reported outcome measurements were utilized and will be reported elsewhere. The qualitative study’s strengths include the inclusion of patients who, due to the severity of their condition (e.g. EDSS mean: 6.8, range: 6–8, highly active MS), age (mean: 53.9 years, range: 36–73 years) family constellations, are often underrepresented in research studies and often get lost in existing social and health care structures. The study population is specific to the wider district region of Cologne, but the broad inclusion criteria make it representative of severe MS in Germany. The methodological approach of a deductive and inductive structuring content analysis made it possible to include new findings into an existing theoretical framework.
However, the study acknowledges some limitations. While efforts were made to include more HCSs, time constraints on their side limited the number of interviews conducted and might have biased the results. Some professions are underrepresented in the interviews. Complex symptoms (e.g. fatigue, ability to concentrate), medical or therapeutic appointments and organization of the everyday live may have been reasons for the patients’ and caregivers’ interviews lasting shorter than initially planned.
The provision of functions of a CCM, might have pre-structured the answers of the participants.
At current, there is no support system for PwsMS, their caregivers and HCSs that addresses their complex and unmet needs comprehensively and continuously. There are rare qualitative insights of the three important stakeholders: PwsMS, caregivers and HCSs in one analysis about a supporting service like a CCM. In response to this gap, we developed and implemented a long-term cross-sectoral advocacy CCM and analyzed it qualitatively. PwsMS, their caregivers and HCSs expressed positive experiences, perceiving the CCM as a source of relief and support that improved care across various aspects of life. For patients, the CCM intervention resulted in enhanced autonomy, reviving of personal wellbeing and new established contacts with HCSs. Caregivers reported a reduced organizational burden and felt better informed, and HCSs experienced primarily temporal relief, allowing them to concentrate on their core professional responsibilities. At a higher level of care, the study suggests that the CCM contributed to a reduction in disintegration within the social and health care system.
The feedback from participants is seen as valuable for adapting the CCM intervention and the CCM manual for follow-up studies, involving further complex patient groups such as neurological long-term diseases apart from MS and tailoring the duration of the intervention depending on the complexity of evolving demands.
Availability of data and materials
Generated and/or analyzed datasets of participants are available from the corresponding author on reasonable request to protect participants. Preliminary partial results have been presented as a poster during the EAPC World Congress in June 2023 and the abstract has been published in the corresponding abstract booklet [ 70 ].
Abbreviations
Amyotrophic lateral sclerosis
- Care and case management
Case management
Central nervous system
Communication, Coordination and security for people with multiple sclerosis
Consolidated criteria for reporting qualitative research
German register for clinical studies
Extended disability status scale
Electronic patient record
Quality of life
Multiple sclerosis
Koch-Henriksen N, Magyari M. Apparent changes in the epidemiology and severity of multiple sclerosis. Nat Rev Neurol. 2021;17:676–88. https://doi.org/10.1038/s41582-021-00556-y .
Article PubMed Google Scholar
Ellenberger D, Flachenecker P, Fneish F, Frahm N, Hellwig K, Paul F, et al. Aggressive multiple sclerosis: a matter of measurement and timing. Brain. 2020;143:e97. https://doi.org/10.1093/brain/awaa306 .
Article PubMed PubMed Central Google Scholar
Edmonds P, Vivat B, Burman R, Silber E, Higginson IJ. Loss and change: experiences of people severely affected by multiple sclerosis. Palliat Med. 2007;21:101–7. https://doi.org/10.1177/0269216307076333 .
Kurtzke JF. Rating neurologic impairment in multiple rating neurologic impairment in multiple sclerosis: An expanded disability status scale (EDSS). Neurology. 1983;33(11):1444–52.
Article CAS PubMed Google Scholar
Galushko M, Golla H, Strupp J, Karbach U, Kaiser C, Ernstmann N, et al. Unmet needs of patients feeling severely affected by multiple sclerosis in Germany: a qualitative study. J Palliat Med. 2014;17:274–81. https://doi.org/10.1089/jpm.2013.0497 .
Borreani C, Bianchi E, Pietrolongo E, Rossi I, Cilia S, Giuntoli M, et al. Unmet needs of people with severe multiple sclerosis and their carers: qualitative findings for a home-based intervention. PLoS One. 2014:e109679. https://doi.org/10.1371/journal.pone.0109679 .
Yamout BI, Alroughani R. Multiple Sclerosis. Semin Neurol. 2018;38:212–25. https://doi.org/10.1055/s-0038-1649502 .
Nissen N, Lemche J, Reestorff CM, Schmidt M, Skjerbæk AG, Skovgaard L, et al. The lived experience of uncertainty in everyday life with MS. Disabil Rehabil. 2022;44:5957–63. https://doi.org/10.1080/09638288.2021.1955302 .
Strupp J, Hartwig A, Golla H, Galushko M, Pfaff H, Voltz R. Feeling severely affected by multiple sclerosis: what does this mean? Palliat Med. 2012;26:1001–10. https://doi.org/10.1177/0269216311425420 .
Strupp J, Voltz R, Golla H. Opening locked doors: Integrating a palliative care approach into the management of patients with severe multiple sclerosis. Mult Scler J. 2016;22:13–8.
Article CAS Google Scholar
Kraft AK, Berger K. Kernaspekte einer bedarfsgerechten Versorgung von Patienten mit Multipler Sklerose : Inanspruchnahme ambulanter Leistungen und „shared decision making“ [Core aspects of a needs-conform care of patients with multiple sclerosis : Utilization of outpatient services and shared decision making]. Nervenarzt. 2020;91:503–10. https://doi.org/10.1007/s00115-020-00906-z .
Doshi A, Chataway J. Multiple sclerosis, a treatable disease. Clin Med (Lond). 2017;17:530–6. https://doi.org/10.7861/clinmedicine.17-6-530 .
Kobelt G, Thompson A, Berg J, Gannedahl M, Eriksson J. New insights into the burden and costs of multiple sclerosis in Europe. Mult Scler. 2017;23:1123–36. https://doi.org/10.1177/1352458517694432 .
Conradsson D, Ytterberg C, Engelkes C, Johansson S, Gottberg K. Activity limitations and participation restrictions in people with multiple sclerosis: a detailed 10-year perspective. Disabil Rehabil. 2021;43:406–13. https://doi.org/10.1080/09638288.2019.1626919 .
Sorensen PS, Giovannoni G, Montalban X, Thalheim C, Zaratin P, Comi G. The Multiple Sclerosis Care Unit. Mult Scler J. 2019;5:627–36.
Article Google Scholar
Tan H, Yu J, Tabby D, Devries A, Singer J. Clinical and economic impact of a specialty care management program among patients with multiple sclerosis: a cohort study. Mult Scler. 2010;16:956–63. https://doi.org/10.1177/1352458510373487 .
Article CAS PubMed PubMed Central Google Scholar
Oeseburg B, Wynia K, Middel B, Reijneveld SA. Effects of case management for frail older people or those with chronic illness: a systematic review. Nurs Res. 2009;58:201–10.
Aiken LS, Butner J, Lockhart CA, Volk-Craft BE, Hamilton G, Williams FG. Outcome evaluation of a randomized trial of the PhoenixCare intervention: program of case management and coordinated care for the seriously chronically ill. J Palliat Med. 2006;9:111–26. https://doi.org/10.1089/jpm.2006.9.111 .
Kuhn U, Düsterdiek A, Galushko M, Dose C, Montag T, Ostgathe C, Voltz R. Identifying patients suitable for palliative care—a descriptive analysis of enquiries using a Case Management Process Model approach. BMC Res Notes. 2012;5:611. https://doi.org/10.1186/1756-0500-5-611 .
Leary A, Quinn D, Bowen A. Impact of proactive case management by multiple sclerosis specialist nurses on use of unscheduled care and emergency presentation in multiple sclerosis: a case study. Int J MS Care. 2015;17:159–63. https://doi.org/10.7224/1537-2073.2014-011 .
Strupp J, Dose C, Kuhn U, Galushko M, Duesterdiek A, Ernstmann N, et al. Analysing the impact of a case management model on the specialised palliative care multi-professional team. Support Care Cancer. 2018;26:673–9. https://doi.org/10.1007/s00520-017-3893-3 .
Wynia K, Annema C, Nissen H, de Keyser J, Middel B. Design of a Randomised Controlled Trial (RCT) on the effectiveness of a Dutch patient advocacy case management intervention among severely disabled Multiple Sclerosis patients. BMC Health Serv Res. 2010;10:142. https://doi.org/10.1186/1472-6963-10-142 .
Ewers M, Schaeffer D, editors. Case Management in Theorie und Praxis. Bern: Huber; 2005.
Google Scholar
Neuffer M. Case Management: Soziale Arbeit mit Einzelnen und Familien. 5th ed. Weinheim, Basel: Beltz Juventa; 2013.
Case Management Society of America. The standards of practice for case management. 2022.
Deutsche Gesellschaft für Care und Case Management e.V., editor. Case Management Leitlinien: Rahmenempfehlung, Standards und ethische Grundlagen. 2nd ed. Heidelberg: Medhochzwei; 2020.
Monzer M. Case Management Grundlagen. 2nd ed. Heidelberg: Medhochzwei; 2018.
Wissert M. Grundfunktionen und fachliche Standards des Unterstützungsmanagements. Z Gerontol Geriat. 1998;31(5):331–7.
Wissert M. Tools und Werkzeuge beim Case Management: Die Hilfeplanung. Case Manag. 2007;1:35–7.
Schaeffer D, Berens E-M, Vogt D. Health literacy in the German population. Dtsch Arztebl Int. 2017;114:53–60. https://doi.org/10.3238/arztebl.2017.0053 .
Pfaff H, Schulte H. Der onkologische Patient der Zukunft. Onkologe. 2012;18:127–33. https://doi.org/10.1007/s00761-011-2201-y .
Pfaff H, Kowalski C, Ommen O. Modelle zur Analyse von Integration und Koordination im Versorgungssystem. In: Ameldung, Sydow, Windeler, editor. Vernetzung im Gesundheitswesen: Wettbewerb und Kooperation. Stuttgart: Kohlhammer Verlag; 2009. p. 75–90.
Golla H, Mammeas S, Galushko M, Pfaff H, Voltz R. Unmet needs of caregivers of severely affected multiple sclerosis patients: A qualitative study. Palliat Support Care. 2015;13(6):1685–93.
Golla H, Galushko M, Pfaff H, Voltz R. Multiple sclerosis and palliative care - perceptions of severely affected multiple sclerosis patients and their health professionals: a qualitative study. BMC Palliat Care. 2014;13:11. https://doi.org/10.1186/1472-684x-13-11 .
Golla H, Galushko M, Pfaff H, Voltz R. Unmet needs of severely affected multiple sclerosis patients: the health professionals’ view. Palliat Med. 2012;26:139–51. https://doi.org/10.1177/0269216311401465 .
Methley AM, Chew-Graham CA, Cheraghi-Sohi S, Campbell SM. A qualitative study of patient and professional perspectives of healthcare services for multiple sclerosis: implications for service development and policy. Health Soc Care Community. 2017;25:848–57. https://doi.org/10.1111/hsc.12369 .
Kalb R, Costello K, Guiod L. Case management services to meet the complex needs of patients with multiple sclerosis in the community—the successes and challenges of a unique program from the national multiple sclerosis society. US Neurology. 2019;15:27–31.
Krüger K, Fricke LM, Dilger E-M, Thiele A, Schaubert K, Hoekstra D, et al. How is and how should healthcare for people with multiple sclerosis in Germany be designed?-The rationale and protocol for the mixed-methods study Multiple Sclerosis-Patient-Oriented Care in Lower Saxony (MS-PoV). PLoS One. 2021;16:e0259855. https://doi.org/10.1371/journal.pone.0259855 .
Ivancevic S, Weegen L, Korff L, Jahn R, Walendzik A, Mostardt S, et al. Effektivität und Kosteneffektivät von Versorgungsmanagement-Programmen bei Multipler Sklerose in Deutschland – Eine Übersichtsarbeit. Akt Neurol. 2015;42:503–8. https://doi.org/10.1055/s-0035-1564111 .
Müller A, Dillen K, Dojan T, Ungeheuer S, Goereci Y, Dunkl V, et al. Development of a long-term cross-sectoral case and care management manual for patients with severe multiple sclerosis and their caregivers. Prof Case Manag. 2023;28:183–93. https://doi.org/10.1097/NCM.0000000000000608 .
Golla H, Dillen K, Hellmich M, Dojan T, Ungeheuer S, Schmalz P, et al. Communication, Coordination, and Security for People with Multiple Sclerosis (COCOS-MS): a randomised phase II clinical trial protocol. BMJ Open. 2022;12:e049300. https://doi.org/10.1136/bmjopen-2021-049300 .
Tong A, Sainsbury P, Craig J. Consolidated criteria for reporting qualitative research (COREQ): a 32-item checklist for interviews and focus groups. Int J Qual Health Care. 2007;19:349–57. https://doi.org/10.1093/intqhc/mzm042 .
Kuckartz U. Qualitative Inhaltsanalyse: Methoden, Praxis, Computerunterstützung. 4th ed. Weinheim: Beltz Juventa; 2018.
Akyirem S, Ekpor E, Aidoo-Frimpong GA, Salifu Y, Nelson LE. Online interviews for qualitative health research in Africa: a scoping review. Int Health. 2023. https://doi.org/10.1093/inthealth/ihad010 .
Peasgood T, Bourke M, Devlin N, Rowen D, Yang Y, Dalziel K. Randomised comparison of online interviews versus face-to-face interviews to value health states. Soc Sci Med. 2023;323:115818. https://doi.org/10.1016/j.socscimed.2023.115818 .
Giordano A, Cimino V, Campanella A, Morone G, Fusco A, Farinotti M, et al. Low quality of life and psychological wellbeing contrast with moderate perceived burden in carers of people with severe multiple sclerosis. J Neurol Sci. 2016;366:139–45. https://doi.org/10.1016/j.jns.2016.05.016 .
Joo JY, Liu MF. Experiences of case management with chronic illnesses: a qualitative systematic review. Int Nurs Rev. 2018;65(1):102–1113.
Freeman J, Gorst T, Gunn H, Robens S. “A non-person to the rest of the world”: experiences of social isolation amongst severely impaired people with multiple sclerosis. Disabil Rehabil. 2020;42:2295–303. https://doi.org/10.1080/09638288.2018.1557267 .
National Institute for Health and Care Excellence. Multiple sclerosis: Management of multiple sclerosis in primary and secondary care. 2014.
Erdmann A, Spoden C, Hirschberg I, Neitzke G. The wish to die and hastening death in amyotrophic lateral sclerosis: A scoping review. BMJ Support Palliat Care. 2021;11:271–87. https://doi.org/10.1136/bmjspcare-2020-002640 .
Erlangsen A, Stenager E, Conwell Y, Andersen PK, Hawton K, Benros ME, et al. Association between neurological disorders and death by suicide in Denmark. JAMA. 2020;323:444–54. https://doi.org/10.1001/jama.2019.21834 .
Kalb R, Feinstein A, Rohrig A, Sankary L, Willis A. Depression and suicidality in multiple sclerosis: red flags, management strategies, and ethical considerations. Curr Neurol Neurosci Rep. 2019;19:77. https://doi.org/10.1007/s11910-019-0992-1 .
Feinstein A, Pavisian B. Multiple sclerosis and suicide. Mult Scler. 2017;23:923–7. https://doi.org/10.1177/1352458517702553 .
Marrie RA, Salter A, Tyry T, Cutter GR, Cofield S, Fox RJ. High hypothetical interest in physician-assisted death in multiple sclerosis. Neurology. 2017;88:1528–34. https://doi.org/10.1212/WNL.0000000000003831 .
Gauthier S, Mausbach J, Reisch T, Bartsch C. Suicide tourism: a pilot study on the Swiss phenomenon. J Med Ethics. 2015;41:611–7. https://doi.org/10.1136/medethics-2014-102091 .
Fischer S, Huber CA, Imhof L, MahrerImhof R, Furter M, Ziegler SJ, Bosshard G. Suicide assisted by two Swiss right-to-die organisations. J Med Ethics. 2008;34:810–4. https://doi.org/10.1136/jme.2007.023887 .
Strupp J, Ehmann C, Galushko M, Bücken R, Perrar KM, Hamacher S, et al. Risk factors for suicidal ideation in patients feeling severely affected by multiple sclerosis. J Palliat Med. 2016;19:523–8. https://doi.org/10.1089/jpm.2015.0418 .
Spence RA, Blanke CD, Keating TJ, Taylor LP. Responding to patient requests for hastened death: physician aid in dying and the clinical oncologist. J Oncol Pract. 2017;13:693–9. https://doi.org/10.1200/JOP.2016.019299 .
Monforte-Royo C, Villavicencio-Chávez C, Tomás-Sábado J, Balaguer A. The wish to hasten death: a review of clinical studies. Psychooncology. 2011;20:795–804. https://doi.org/10.1002/pon.1839 .
Blanke C, LeBlanc M, Hershman D, Ellis L, Meyskens F. Characterizing 18 years of the death with dignity act in Oregon. JAMA Oncol. 2017;3:1403–6. https://doi.org/10.1001/jamaoncol.2017.0243 .
Methley A, Campbell S, Cheraghi-Sohi S, Chew-Graham C. Meeting the mental health needs of people with multiple sclerosis: a qualitative study of patients and professionals. Disabil Rehab. 2017;39(11):1097-105. https://doi.org/10.1080/09638288.2016.1180547 .
Hudon C, Bisson M, Chouinard M-C, Delahunty-Pike A, Lambert M, Howse D, et al. Implementation analysis of a case management intervention for people with complex care needs in primary care: a multiple case study across Canada. BMC Health Serv Res. 2023;23:377. https://doi.org/10.1186/s12913-023-09379-7 .
Beckmann M, Dittmer K, Jaschke J, Karbach U, Köberlein-Neu J, Nocon M, et al. Electronic patient record and its effects on social aspects of interprofessional collaboration and clinical workflows in hospitals (eCoCo): a mixed methods study protocol. BMC Health Serv Res. 2021;21:377. https://doi.org/10.1186/s12913-021-06377-5 .
Campanella P, Lovato E, Marone C, Fallacara L, Mancuso A, Ricciardi W, Specchia ML. The impact of electronic health records on healthcare quality: a systematic review and meta-analysis. Eur J Public Health. 2016;26:60–4. https://doi.org/10.1093/eurpub/ckv122 .
García-Hernández M, González de León B, Barreto-Cruz S, Vázquez-Díaz JR. Multicomponent, high-intensity, and patient-centered care intervention for complex patients in transitional care: SPICA program. Front Med (Lausanne). 2022;9:1033689. https://doi.org/10.3389/fmed.2022.1033689 .
Meisinger C, Stollenwerk B, Kirchberger I, Seidl H, Wende R, Kuch B, Holle R. Effects of a nurse-based case management compared to usual care among aged patients with myocardial infarction: results from the randomized controlled KORINNA study. BMC Geriatr. 2013. https://doi.org/10.1186/1471-2318-13-115 .
Joo JY, Huber DL. Case management effectiveness on health care utilization outcomes: a systematic review of reviews. West J Nurs Res. 2019;41:111–33. https://doi.org/10.1177/0193945918762135 .
Stergiopoulos V, Gozdzik A, Misir V, Skosireva A, Connelly J, Sarang A, et al. Effectiveness of housing first with intensive case management in an ethnically diverse sample of homeless adults with mental illness: a randomized controlled trial. PLoS One. 2015;10:e0130281. https://doi.org/10.1371/journal.pone.0130281 .
Löcherbach P, Wendt R, editors. Care und Case Management: Transprofessionelle Versorgungsstrukturen und Netzwerke. 1st ed. Stuttgart: Kohlhammer; 2020.
EAPC2023 Abstract Book. Palliat Med. 2023;37:1–302. https://doi.org/10.1177/02692163231172891 .
Download references
Acknowledgements
We would like to thank all the patients, caregivers and health care specialists who volunteered their time to participate in an interview and the trial, Carola Janßen for transcribing the interviews, Fiona Brown for translating the illustrative quotes and Beatrix Münzberg, Kerstin Weiß and Monika Höveler for data collection in the quantitative study part.
COCOS-MS Trial Group
Anne Müller 1 , Fabian Hebben 1 , Kim Dillen 1 , Veronika Dunkl 1 , Yasemin Goereci 2 , Raymond Voltz 1,3,4 , Peter Löcherbach 5 , Clemens Warnke 2 , Heidrun Golla 1 , Dirk Müller 6 , Dorthe Hobus 1 , Eckhard Bonmann 7 , Franziska Schwartzkopff 8 , Gereon Nelles 9 , Gundula Palmbach 8 , Herbert Temmes 10 , Isabel Franke 1 , Judith Haas 10 , Julia Strupp 1 , Kathrin Gerbershagen 7 , Laura Becker-Peters 8 , Lothar Burghaus 11 , Martin Hellmich 12 , Martin Paus 8 , Solveig Ungeheuer 1 , Sophia Kochs 1 , Stephanie Stock 6 , Thomas Joist 13 , Volker Limmroth 14
1 Department of Palliative Medicine, Faculty of Medicine and University Hospital, University of Cologne, Cologne, Germany
2 Department of Neurology, Faculty of Medicine and University Hospital, University of Cologne, Cologne, Germany
3 Center for Integrated Oncology Aachen Bonn Cologne Düsseldorf (CIO ABCD), University of Cologne, Cologne, Germany
4 Center for Health Services Research (ZVFK), University of Cologne, Cologne, Germany
5 German Society of Care and Case Management e.V. (DGCC), Münster, Germany
6 Institute for Health Economics and Clinical Epidemiology (IGKE), Faculty of Medicine and University Hospital, University of Cologne, Cologne, Germany
7 Department of Neurology, Klinikum Köln, Cologne, Germany
8 Clinical Trials Centre Cologne (CTCC), Faculty of Medicine and University Hospital, University of Cologne, Cologne, Germany
9 NeuroMed Campus, MedCampus Hohenlind, Cologne, Germany
10 German Multiple Sclerosis Society Federal Association (DMSG), Hannover, Germany
11 Department of Neurology, Heilig Geist-Krankenhaus Köln, Cologne, Germany
12 Institute of Medical Statistics and Computational Biology (IMSB), Faculty of Medicine and University Hospital, University of Cologne, Cologne, Germany
13 Academic Teaching Practice, University of Cologne, Cologne, Germany
14 Department of Neurology, Klinikum Köln-Merheim, Cologne, Germany
Open Access funding enabled and organized by Projekt DEAL. This work was supported by the Innovation Funds of the Federal Joint Committee (G-BA), grant number: 01VSF19029.
Author information
Authors and affiliations.
Department of Palliative Medicine, Faculty of Medicine and University Hospital, University of Cologne, Cologne, Germany
Anne Müller, Fabian Hebben, Kim Dillen, Veronika Dunkl, Raymond Voltz & Heidrun Golla
Department of Neurology, Faculty of Medicine and University Hospital, University of Cologne, Cologne, Germany
Yasemin Goereci & Clemens Warnke
Center for Integrated Oncology Aachen Bonn Cologne Düsseldorf (CIO ABCD), University of Cologne, Cologne, Germany
Raymond Voltz
Center for Health Services Research, University of Cologne, Cologne, Germany
German Society of Care and Case Management E.V. (DGCC), Münster, Germany
Peter Löcherbach
You can also search for this author in PubMed Google Scholar
- Anne Müller
- , Fabian Hebben
- , Kim Dillen
- , Veronika Dunkl
- , Yasemin Goereci
- , Raymond Voltz
- , Peter Löcherbach
- , Clemens Warnke
- , Heidrun Golla
- , Dirk Müller
- , Dorthe Hobus
- , Eckhard Bonmann
- , Franziska Schwartzkopff
- , Gereon Nelles
- , Gundula Palmbach
- , Herbert Temmes
- , Isabel Franke
- , Judith Haas
- , Julia Strupp
- , Kathrin Gerbershagen
- , Laura Becker-Peters
- , Lothar Burghaus
- , Martin Hellmich
- , Martin Paus
- , Solveig Ungeheuer
- , Sophia Kochs
- , Stephanie Stock
- , Thomas Joist
- & Volker Limmroth
Contributions
HG, KD, CW designed the trial. HG, KD obtained ethical approvals. HG, KD developed the interview guidelines with help of the CCM (SU). AM was responsible for collecting qualitative data, developing the code system, coding, analysis of the data and writing the first draft of the manuscript, thoroughly revised and partly rewritten by HG. FH supported in collecting qualitative data, coding and analysis of the interviews. KD supported in collecting qualitative data. AM, FH, KD, VD, YG, RV, PL, CW, HG discussed and con-solidated the finalized category system. AM, FH, KD, VD, YG, RV, PL, CW, HG read and commented on the manuscript and agreed to the final version.
Authors’ information
Not applicable.
Corresponding author
Correspondence to Anne Müller .
Ethics declarations
Ethics approval and consent to participate.
Participants were provided with oral and written information about the trial and provided written informed consent. Ethical approval was obtained from the Ethics Committee of the University of Cologne (#20–1436). The trial is registered in the German Register for Clinical Studies (DRKS) (DRKS00022771) and is conducted under the Declaration of Helsinki.
Consent for publication
Competing interests.
Clemens Warnke has received institutional support from Novartis, Alexion, Sanofi Genzyme, Janssen, Biogen, Merck and Roche. The other authors declare that they have no competing interests.
Additional information
Publisher’s note.
Springer Nature remains neutral with regard to jurisdictional claims in published maps and institutional affiliations.
Supplementary Information
Supplementary material 1., rights and permissions.
Open Access This article is licensed under a Creative Commons Attribution 4.0 International License, which permits use, sharing, adaptation, distribution and reproduction in any medium or format, as long as you give appropriate credit to the original author(s) and the source, provide a link to the Creative Commons licence, and indicate if changes were made. The images or other third party material in this article are included in the article's Creative Commons licence, unless indicated otherwise in a credit line to the material. If material is not included in the article's Creative Commons licence and your intended use is not permitted by statutory regulation or exceeds the permitted use, you will need to obtain permission directly from the copyright holder. To view a copy of this licence, visit http://creativecommons.org/licenses/by/4.0/ . The Creative Commons Public Domain Dedication waiver ( http://creativecommons.org/publicdomain/zero/1.0/ ) applies to the data made available in this article, unless otherwise stated in a credit line to the data.
Reprints and permissions
About this article
Cite this article.
Müller, A., Hebben, F., Dillen, K. et al. “So at least now I know how to deal with things myself, what I can do if it gets really bad again”—experiences with a long-term cross-sectoral advocacy care and case management for severe multiple sclerosis: a qualitative study. BMC Health Serv Res 24 , 453 (2024). https://doi.org/10.1186/s12913-024-10851-1
Download citation
Received : 23 November 2023
Accepted : 11 March 2024
Published : 10 April 2024
DOI : https://doi.org/10.1186/s12913-024-10851-1
Share this article
Anyone you share the following link with will be able to read this content:
Sorry, a shareable link is not currently available for this article.
Provided by the Springer Nature SharedIt content-sharing initiative
- Cross-sectoral
- Qualitative research
- Health care specialist
- Severe multiple sclerosis
BMC Health Services Research
ISSN: 1472-6963
- General enquiries: [email protected]

An official website of the United States government
The .gov means it’s official. Federal government websites often end in .gov or .mil. Before sharing sensitive information, make sure you’re on a federal government site.
The site is secure. The https:// ensures that you are connecting to the official website and that any information you provide is encrypted and transmitted securely.
- Publications
- Account settings
Preview improvements coming to the PMC website in October 2024. Learn More or Try it out now .
- Advanced Search
- Journal List
- Can J Hosp Pharm
- v.68(3); May-Jun 2015

Qualitative Research: Data Collection, Analysis, and Management
Introduction.
In an earlier paper, 1 we presented an introduction to using qualitative research methods in pharmacy practice. In this article, we review some principles of the collection, analysis, and management of qualitative data to help pharmacists interested in doing research in their practice to continue their learning in this area. Qualitative research can help researchers to access the thoughts and feelings of research participants, which can enable development of an understanding of the meaning that people ascribe to their experiences. Whereas quantitative research methods can be used to determine how many people undertake particular behaviours, qualitative methods can help researchers to understand how and why such behaviours take place. Within the context of pharmacy practice research, qualitative approaches have been used to examine a diverse array of topics, including the perceptions of key stakeholders regarding prescribing by pharmacists and the postgraduation employment experiences of young pharmacists (see “Further Reading” section at the end of this article).
In the previous paper, 1 we outlined 3 commonly used methodologies: ethnography 2 , grounded theory 3 , and phenomenology. 4 Briefly, ethnography involves researchers using direct observation to study participants in their “real life” environment, sometimes over extended periods. Grounded theory and its later modified versions (e.g., Strauss and Corbin 5 ) use face-to-face interviews and interactions such as focus groups to explore a particular research phenomenon and may help in clarifying a less-well-understood problem, situation, or context. Phenomenology shares some features with grounded theory (such as an exploration of participants’ behaviour) and uses similar techniques to collect data, but it focuses on understanding how human beings experience their world. It gives researchers the opportunity to put themselves in another person’s shoes and to understand the subjective experiences of participants. 6 Some researchers use qualitative methodologies but adopt a different standpoint, and an example of this appears in the work of Thurston and others, 7 discussed later in this paper.
Qualitative work requires reflection on the part of researchers, both before and during the research process, as a way of providing context and understanding for readers. When being reflexive, researchers should not try to simply ignore or avoid their own biases (as this would likely be impossible); instead, reflexivity requires researchers to reflect upon and clearly articulate their position and subjectivities (world view, perspectives, biases), so that readers can better understand the filters through which questions were asked, data were gathered and analyzed, and findings were reported. From this perspective, bias and subjectivity are not inherently negative but they are unavoidable; as a result, it is best that they be articulated up-front in a manner that is clear and coherent for readers.
THE PARTICIPANT’S VIEWPOINT
What qualitative study seeks to convey is why people have thoughts and feelings that might affect the way they behave. Such study may occur in any number of contexts, but here, we focus on pharmacy practice and the way people behave with regard to medicines use (e.g., to understand patients’ reasons for nonadherence with medication therapy or to explore physicians’ resistance to pharmacists’ clinical suggestions). As we suggested in our earlier article, 1 an important point about qualitative research is that there is no attempt to generalize the findings to a wider population. Qualitative research is used to gain insights into people’s feelings and thoughts, which may provide the basis for a future stand-alone qualitative study or may help researchers to map out survey instruments for use in a quantitative study. It is also possible to use different types of research in the same study, an approach known as “mixed methods” research, and further reading on this topic may be found at the end of this paper.
The role of the researcher in qualitative research is to attempt to access the thoughts and feelings of study participants. This is not an easy task, as it involves asking people to talk about things that may be very personal to them. Sometimes the experiences being explored are fresh in the participant’s mind, whereas on other occasions reliving past experiences may be difficult. However the data are being collected, a primary responsibility of the researcher is to safeguard participants and their data. Mechanisms for such safeguarding must be clearly articulated to participants and must be approved by a relevant research ethics review board before the research begins. Researchers and practitioners new to qualitative research should seek advice from an experienced qualitative researcher before embarking on their project.
DATA COLLECTION
Whatever philosophical standpoint the researcher is taking and whatever the data collection method (e.g., focus group, one-to-one interviews), the process will involve the generation of large amounts of data. In addition to the variety of study methodologies available, there are also different ways of making a record of what is said and done during an interview or focus group, such as taking handwritten notes or video-recording. If the researcher is audio- or video-recording data collection, then the recordings must be transcribed verbatim before data analysis can begin. As a rough guide, it can take an experienced researcher/transcriber 8 hours to transcribe one 45-minute audio-recorded interview, a process than will generate 20–30 pages of written dialogue.
Many researchers will also maintain a folder of “field notes” to complement audio-taped interviews. Field notes allow the researcher to maintain and comment upon impressions, environmental contexts, behaviours, and nonverbal cues that may not be adequately captured through the audio-recording; they are typically handwritten in a small notebook at the same time the interview takes place. Field notes can provide important context to the interpretation of audio-taped data and can help remind the researcher of situational factors that may be important during data analysis. Such notes need not be formal, but they should be maintained and secured in a similar manner to audio tapes and transcripts, as they contain sensitive information and are relevant to the research. For more information about collecting qualitative data, please see the “Further Reading” section at the end of this paper.
DATA ANALYSIS AND MANAGEMENT
If, as suggested earlier, doing qualitative research is about putting oneself in another person’s shoes and seeing the world from that person’s perspective, the most important part of data analysis and management is to be true to the participants. It is their voices that the researcher is trying to hear, so that they can be interpreted and reported on for others to read and learn from. To illustrate this point, consider the anonymized transcript excerpt presented in Appendix 1 , which is taken from a research interview conducted by one of the authors (J.S.). We refer to this excerpt throughout the remainder of this paper to illustrate how data can be managed, analyzed, and presented.
Interpretation of Data
Interpretation of the data will depend on the theoretical standpoint taken by researchers. For example, the title of the research report by Thurston and others, 7 “Discordant indigenous and provider frames explain challenges in improving access to arthritis care: a qualitative study using constructivist grounded theory,” indicates at least 2 theoretical standpoints. The first is the culture of the indigenous population of Canada and the place of this population in society, and the second is the social constructivist theory used in the constructivist grounded theory method. With regard to the first standpoint, it can be surmised that, to have decided to conduct the research, the researchers must have felt that there was anecdotal evidence of differences in access to arthritis care for patients from indigenous and non-indigenous backgrounds. With regard to the second standpoint, it can be surmised that the researchers used social constructivist theory because it assumes that behaviour is socially constructed; in other words, people do things because of the expectations of those in their personal world or in the wider society in which they live. (Please see the “Further Reading” section for resources providing more information about social constructivist theory and reflexivity.) Thus, these 2 standpoints (and there may have been others relevant to the research of Thurston and others 7 ) will have affected the way in which these researchers interpreted the experiences of the indigenous population participants and those providing their care. Another standpoint is feminist standpoint theory which, among other things, focuses on marginalized groups in society. Such theories are helpful to researchers, as they enable us to think about things from a different perspective. Being aware of the standpoints you are taking in your own research is one of the foundations of qualitative work. Without such awareness, it is easy to slip into interpreting other people’s narratives from your own viewpoint, rather than that of the participants.
To analyze the example in Appendix 1 , we will adopt a phenomenological approach because we want to understand how the participant experienced the illness and we want to try to see the experience from that person’s perspective. It is important for the researcher to reflect upon and articulate his or her starting point for such analysis; for example, in the example, the coder could reflect upon her own experience as a female of a majority ethnocultural group who has lived within middle class and upper middle class settings. This personal history therefore forms the filter through which the data will be examined. This filter does not diminish the quality or significance of the analysis, since every researcher has his or her own filters; however, by explicitly stating and acknowledging what these filters are, the researcher makes it easer for readers to contextualize the work.
Transcribing and Checking
For the purposes of this paper it is assumed that interviews or focus groups have been audio-recorded. As mentioned above, transcribing is an arduous process, even for the most experienced transcribers, but it must be done to convert the spoken word to the written word to facilitate analysis. For anyone new to conducting qualitative research, it is beneficial to transcribe at least one interview and one focus group. It is only by doing this that researchers realize how difficult the task is, and this realization affects their expectations when asking others to transcribe. If the research project has sufficient funding, then a professional transcriber can be hired to do the work. If this is the case, then it is a good idea to sit down with the transcriber, if possible, and talk through the research and what the participants were talking about. This background knowledge for the transcriber is especially important in research in which people are using jargon or medical terms (as in pharmacy practice). Involving your transcriber in this way makes the work both easier and more rewarding, as he or she will feel part of the team. Transcription editing software is also available, but it is expensive. For example, ELAN (more formally known as EUDICO Linguistic Annotator, developed at the Technical University of Berlin) 8 is a tool that can help keep data organized by linking media and data files (particularly valuable if, for example, video-taping of interviews is complemented by transcriptions). It can also be helpful in searching complex data sets. Products such as ELAN do not actually automatically transcribe interviews or complete analyses, and they do require some time and effort to learn; nonetheless, for some research applications, it may be a valuable to consider such software tools.
All audio recordings should be transcribed verbatim, regardless of how intelligible the transcript may be when it is read back. Lines of text should be numbered. Once the transcription is complete, the researcher should read it while listening to the recording and do the following: correct any spelling or other errors; anonymize the transcript so that the participant cannot be identified from anything that is said (e.g., names, places, significant events); insert notations for pauses, laughter, looks of discomfort; insert any punctuation, such as commas and full stops (periods) (see Appendix 1 for examples of inserted punctuation), and include any other contextual information that might have affected the participant (e.g., temperature or comfort of the room).
Dealing with the transcription of a focus group is slightly more difficult, as multiple voices are involved. One way of transcribing such data is to “tag” each voice (e.g., Voice A, Voice B). In addition, the focus group will usually have 2 facilitators, whose respective roles will help in making sense of the data. While one facilitator guides participants through the topic, the other can make notes about context and group dynamics. More information about group dynamics and focus groups can be found in resources listed in the “Further Reading” section.
Reading between the Lines
During the process outlined above, the researcher can begin to get a feel for the participant’s experience of the phenomenon in question and can start to think about things that could be pursued in subsequent interviews or focus groups (if appropriate). In this way, one participant’s narrative informs the next, and the researcher can continue to interview until nothing new is being heard or, as it says in the text books, “saturation is reached”. While continuing with the processes of coding and theming (described in the next 2 sections), it is important to consider not just what the person is saying but also what they are not saying. For example, is a lengthy pause an indication that the participant is finding the subject difficult, or is the person simply deciding what to say? The aim of the whole process from data collection to presentation is to tell the participants’ stories using exemplars from their own narratives, thus grounding the research findings in the participants’ lived experiences.
Smith 9 suggested a qualitative research method known as interpretative phenomenological analysis, which has 2 basic tenets: first, that it is rooted in phenomenology, attempting to understand the meaning that individuals ascribe to their lived experiences, and second, that the researcher must attempt to interpret this meaning in the context of the research. That the researcher has some knowledge and expertise in the subject of the research means that he or she can have considerable scope in interpreting the participant’s experiences. Larkin and others 10 discussed the importance of not just providing a description of what participants say. Rather, interpretative phenomenological analysis is about getting underneath what a person is saying to try to truly understand the world from his or her perspective.
Once all of the research interviews have been transcribed and checked, it is time to begin coding. Field notes compiled during an interview can be a useful complementary source of information to facilitate this process, as the gap in time between an interview, transcribing, and coding can result in memory bias regarding nonverbal or environmental context issues that may affect interpretation of data.
Coding refers to the identification of topics, issues, similarities, and differences that are revealed through the participants’ narratives and interpreted by the researcher. This process enables the researcher to begin to understand the world from each participant’s perspective. Coding can be done by hand on a hard copy of the transcript, by making notes in the margin or by highlighting and naming sections of text. More commonly, researchers use qualitative research software (e.g., NVivo, QSR International Pty Ltd; www.qsrinternational.com/products_nvivo.aspx ) to help manage their transcriptions. It is advised that researchers undertake a formal course in the use of such software or seek supervision from a researcher experienced in these tools.
Returning to Appendix 1 and reading from lines 8–11, a code for this section might be “diagnosis of mental health condition”, but this would just be a description of what the participant is talking about at that point. If we read a little more deeply, we can ask ourselves how the participant might have come to feel that the doctor assumed he or she was aware of the diagnosis or indeed that they had only just been told the diagnosis. There are a number of pauses in the narrative that might suggest the participant is finding it difficult to recall that experience. Later in the text, the participant says “nobody asked me any questions about my life” (line 19). This could be coded simply as “health care professionals’ consultation skills”, but that would not reflect how the participant must have felt never to be asked anything about his or her personal life, about the participant as a human being. At the end of this excerpt, the participant just trails off, recalling that no-one showed any interest, which makes for very moving reading. For practitioners in pharmacy, it might also be pertinent to explore the participant’s experience of akathisia and why this was left untreated for 20 years.
One of the questions that arises about qualitative research relates to the reliability of the interpretation and representation of the participants’ narratives. There are no statistical tests that can be used to check reliability and validity as there are in quantitative research. However, work by Lincoln and Guba 11 suggests that there are other ways to “establish confidence in the ‘truth’ of the findings” (p. 218). They call this confidence “trustworthiness” and suggest that there are 4 criteria of trustworthiness: credibility (confidence in the “truth” of the findings), transferability (showing that the findings have applicability in other contexts), dependability (showing that the findings are consistent and could be repeated), and confirmability (the extent to which the findings of a study are shaped by the respondents and not researcher bias, motivation, or interest).
One way of establishing the “credibility” of the coding is to ask another researcher to code the same transcript and then to discuss any similarities and differences in the 2 resulting sets of codes. This simple act can result in revisions to the codes and can help to clarify and confirm the research findings.
Theming refers to the drawing together of codes from one or more transcripts to present the findings of qualitative research in a coherent and meaningful way. For example, there may be examples across participants’ narratives of the way in which they were treated in hospital, such as “not being listened to” or “lack of interest in personal experiences” (see Appendix 1 ). These may be drawn together as a theme running through the narratives that could be named “the patient’s experience of hospital care”. The importance of going through this process is that at its conclusion, it will be possible to present the data from the interviews using quotations from the individual transcripts to illustrate the source of the researchers’ interpretations. Thus, when the findings are organized for presentation, each theme can become the heading of a section in the report or presentation. Underneath each theme will be the codes, examples from the transcripts, and the researcher’s own interpretation of what the themes mean. Implications for real life (e.g., the treatment of people with chronic mental health problems) should also be given.
DATA SYNTHESIS
In this final section of this paper, we describe some ways of drawing together or “synthesizing” research findings to represent, as faithfully as possible, the meaning that participants ascribe to their life experiences. This synthesis is the aim of the final stage of qualitative research. For most readers, the synthesis of data presented by the researcher is of crucial significance—this is usually where “the story” of the participants can be distilled, summarized, and told in a manner that is both respectful to those participants and meaningful to readers. There are a number of ways in which researchers can synthesize and present their findings, but any conclusions drawn by the researchers must be supported by direct quotations from the participants. In this way, it is made clear to the reader that the themes under discussion have emerged from the participants’ interviews and not the mind of the researcher. The work of Latif and others 12 gives an example of how qualitative research findings might be presented.
Planning and Writing the Report
As has been suggested above, if researchers code and theme their material appropriately, they will naturally find the headings for sections of their report. Qualitative researchers tend to report “findings” rather than “results”, as the latter term typically implies that the data have come from a quantitative source. The final presentation of the research will usually be in the form of a report or a paper and so should follow accepted academic guidelines. In particular, the article should begin with an introduction, including a literature review and rationale for the research. There should be a section on the chosen methodology and a brief discussion about why qualitative methodology was most appropriate for the study question and why one particular methodology (e.g., interpretative phenomenological analysis rather than grounded theory) was selected to guide the research. The method itself should then be described, including ethics approval, choice of participants, mode of recruitment, and method of data collection (e.g., semistructured interviews or focus groups), followed by the research findings, which will be the main body of the report or paper. The findings should be written as if a story is being told; as such, it is not necessary to have a lengthy discussion section at the end. This is because much of the discussion will take place around the participants’ quotes, such that all that is needed to close the report or paper is a summary, limitations of the research, and the implications that the research has for practice. As stated earlier, it is not the intention of qualitative research to allow the findings to be generalized, and therefore this is not, in itself, a limitation.
Planning out the way that findings are to be presented is helpful. It is useful to insert the headings of the sections (the themes) and then make a note of the codes that exemplify the thoughts and feelings of your participants. It is generally advisable to put in the quotations that you want to use for each theme, using each quotation only once. After all this is done, the telling of the story can begin as you give your voice to the experiences of the participants, writing around their quotations. Do not be afraid to draw assumptions from the participants’ narratives, as this is necessary to give an in-depth account of the phenomena in question. Discuss these assumptions, drawing on your participants’ words to support you as you move from one code to another and from one theme to the next. Finally, as appropriate, it is possible to include examples from literature or policy documents that add support for your findings. As an exercise, you may wish to code and theme the sample excerpt in Appendix 1 and tell the participant’s story in your own way. Further reading about “doing” qualitative research can be found at the end of this paper.
CONCLUSIONS
Qualitative research can help researchers to access the thoughts and feelings of research participants, which can enable development of an understanding of the meaning that people ascribe to their experiences. It can be used in pharmacy practice research to explore how patients feel about their health and their treatment. Qualitative research has been used by pharmacists to explore a variety of questions and problems (see the “Further Reading” section for examples). An understanding of these issues can help pharmacists and other health care professionals to tailor health care to match the individual needs of patients and to develop a concordant relationship. Doing qualitative research is not easy and may require a complete rethink of how research is conducted, particularly for researchers who are more familiar with quantitative approaches. There are many ways of conducting qualitative research, and this paper has covered some of the practical issues regarding data collection, analysis, and management. Further reading around the subject will be essential to truly understand this method of accessing peoples’ thoughts and feelings to enable researchers to tell participants’ stories.
Appendix 1. Excerpt from a sample transcript
The participant (age late 50s) had suffered from a chronic mental health illness for 30 years. The participant had become a “revolving door patient,” someone who is frequently in and out of hospital. As the participant talked about past experiences, the researcher asked:
- What was treatment like 30 years ago?
- Umm—well it was pretty much they could do what they wanted with you because I was put into the er, the er kind of system er, I was just on
- endless section threes.
- Really…
- But what I didn’t realize until later was that if you haven’t actually posed a threat to someone or yourself they can’t really do that but I didn’t know
- that. So wh-when I first went into hospital they put me on the forensic ward ’cause they said, “We don’t think you’ll stay here we think you’ll just
- run-run away.” So they put me then onto the acute admissions ward and – er – I can remember one of the first things I recall when I got onto that
- ward was sitting down with a er a Dr XXX. He had a book this thick [gestures] and on each page it was like three questions and he went through
- all these questions and I answered all these questions. So we’re there for I don’t maybe two hours doing all that and he asked me he said “well
- when did somebody tell you then that you have schizophrenia” I said “well nobody’s told me that” so he seemed very surprised but nobody had
- actually [pause] whe-when I first went up there under police escort erm the senior kind of consultants people I’d been to where I was staying and
- ermm so er [pause] I . . . the, I can remember the very first night that I was there and given this injection in this muscle here [gestures] and just
- having dreadful side effects the next day I woke up [pause]
- . . . and I suffered that akathesia I swear to you, every minute of every day for about 20 years.
- Oh how awful.
- And that side of it just makes life impossible so the care on the wards [pause] umm I don’t know it’s kind of, it’s kind of hard to put into words
- [pause]. Because I’m not saying they were sort of like not friendly or interested but then nobody ever seemed to want to talk about your life [pause]
- nobody asked me any questions about my life. The only questions that came into was they asked me if I’d be a volunteer for these student exams
- and things and I said “yeah” so all the questions were like “oh what jobs have you done,” er about your relationships and things and er but
- nobody actually sat down and had a talk and showed some interest in you as a person you were just there basically [pause] um labelled and you
- know there was there was [pause] but umm [pause] yeah . . .
This article is the 10th in the CJHP Research Primer Series, an initiative of the CJHP Editorial Board and the CSHP Research Committee. The planned 2-year series is intended to appeal to relatively inexperienced researchers, with the goal of building research capacity among practising pharmacists. The articles, presenting simple but rigorous guidance to encourage and support novice researchers, are being solicited from authors with appropriate expertise.
Previous articles in this series:
Bond CM. The research jigsaw: how to get started. Can J Hosp Pharm . 2014;67(1):28–30.
Tully MP. Research: articulating questions, generating hypotheses, and choosing study designs. Can J Hosp Pharm . 2014;67(1):31–4.
Loewen P. Ethical issues in pharmacy practice research: an introductory guide. Can J Hosp Pharm. 2014;67(2):133–7.
Tsuyuki RT. Designing pharmacy practice research trials. Can J Hosp Pharm . 2014;67(3):226–9.
Bresee LC. An introduction to developing surveys for pharmacy practice research. Can J Hosp Pharm . 2014;67(4):286–91.
Gamble JM. An introduction to the fundamentals of cohort and case–control studies. Can J Hosp Pharm . 2014;67(5):366–72.
Austin Z, Sutton J. Qualitative research: getting started. C an J Hosp Pharm . 2014;67(6):436–40.
Houle S. An introduction to the fundamentals of randomized controlled trials in pharmacy research. Can J Hosp Pharm . 2014; 68(1):28–32.
Charrois TL. Systematic reviews: What do you need to know to get started? Can J Hosp Pharm . 2014;68(2):144–8.
Competing interests: None declared.
Further Reading
Examples of qualitative research in pharmacy practice.
- Farrell B, Pottie K, Woodend K, Yao V, Dolovich L, Kennie N, et al. Shifts in expectations: evaluating physicians’ perceptions as pharmacists integrated into family practice. J Interprof Care. 2010; 24 (1):80–9. [ PubMed ] [ Google Scholar ]
- Gregory P, Austin Z. Postgraduation employment experiences of new pharmacists in Ontario in 2012–2013. Can Pharm J. 2014; 147 (5):290–9. [ PMC free article ] [ PubMed ] [ Google Scholar ]
- Marks PZ, Jennnings B, Farrell B, Kennie-Kaulbach N, Jorgenson D, Pearson-Sharpe J, et al. “I gained a skill and a change in attitude”: a case study describing how an online continuing professional education course for pharmacists supported achievement of its transfer to practice outcomes. Can J Univ Contin Educ. 2014; 40 (2):1–18. [ Google Scholar ]
- Nair KM, Dolovich L, Brazil K, Raina P. It’s all about relationships: a qualitative study of health researchers’ perspectives on interdisciplinary research. BMC Health Serv Res. 2008; 8 :110. [ PMC free article ] [ PubMed ] [ Google Scholar ]
- Pojskic N, MacKeigan L, Boon H, Austin Z. Initial perceptions of key stakeholders in Ontario regarding independent prescriptive authority for pharmacists. Res Soc Adm Pharm. 2014; 10 (2):341–54. [ PubMed ] [ Google Scholar ]
Qualitative Research in General
- Breakwell GM, Hammond S, Fife-Schaw C. Research methods in psychology. Thousand Oaks (CA): Sage Publications; 1995. [ Google Scholar ]
- Given LM. 100 questions (and answers) about qualitative research. Thousand Oaks (CA): Sage Publications; 2015. [ Google Scholar ]
- Miles B, Huberman AM. Qualitative data analysis. Thousand Oaks (CA): Sage Publications; 2009. [ Google Scholar ]
- Patton M. Qualitative research and evaluation methods. Thousand Oaks (CA): Sage Publications; 2002. [ Google Scholar ]
- Willig C. Introducing qualitative research in psychology. Buckingham (UK): Open University Press; 2001. [ Google Scholar ]
Group Dynamics in Focus Groups
- Farnsworth J, Boon B. Analysing group dynamics within the focus group. Qual Res. 2010; 10 (5):605–24. [ Google Scholar ]
Social Constructivism
- Social constructivism. Berkeley (CA): University of California, Berkeley, Berkeley Graduate Division, Graduate Student Instruction Teaching & Resource Center; [cited 2015 June 4]. Available from: http://gsi.berkeley.edu/gsi-guide-contents/learning-theory-research/social-constructivism/ [ Google Scholar ]
Mixed Methods
- Creswell J. Research design: qualitative, quantitative, and mixed methods approaches. Thousand Oaks (CA): Sage Publications; 2009. [ Google Scholar ]
Collecting Qualitative Data
- Arksey H, Knight P. Interviewing for social scientists: an introductory resource with examples. Thousand Oaks (CA): Sage Publications; 1999. [ Google Scholar ]
- Guest G, Namey EE, Mitchel ML. Collecting qualitative data: a field manual for applied research. Thousand Oaks (CA): Sage Publications; 2013. [ Google Scholar ]
Constructivist Grounded Theory
- Charmaz K. Grounded theory: objectivist and constructivist methods. In: Denzin N, Lincoln Y, editors. Handbook of qualitative research. 2nd ed. Thousand Oaks (CA): Sage Publications; 2000. pp. 509–35. [ Google Scholar ]
- Study Guides
- Homework Questions
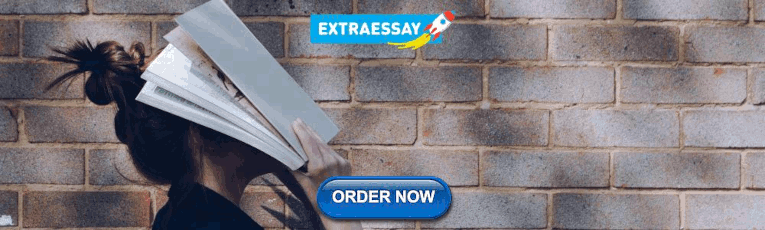
IMAGES
VIDEO
COMMENTS
A case study is one of the most commonly used methodologies of social research. This article attempts to look into the various dimensions of a case study research strategy, the different epistemological strands which determine the particular case study type and approach adopted in the field, discusses the factors which can enhance the effectiveness of a case study research, and the debate ...
Furthermore, the ability to describe in detail how the analysis was conducted ensures rigour in reporting qualitative research. Data sources: The research example used is a multiple case study that explored the role of the clinical skills laboratory in preparing students for the real world of practice. Data analysis was conducted using a ...
Qualitative case study methodology is an appropriate strategy for exploring phenomena such as lived experiences, events, and the contexts in which they occur (Houghton et al. 2014;Miles and ...
Step 1: Gather your qualitative data and conduct research (Conduct qualitative research) The first step of qualitative research is to do data collection. Put simply, data collection is gathering all of your data for analysis. A common situation is when qualitative data is spread across various sources.
McMaster University, West Hamilton, Ontario, Canada. Qualitative case study methodology prov ides tools for researchers to study. complex phenomena within their contexts. When the approach is ...
Data analysis in qualitative research is defined as the process of systematically searching and arranging the interview transcripts, observation notes, ... Attributes: An attribute is a property of a node, case or document. It is equivalent to a variable in quantitative analysis. An attribute (e.g. ethnicity) may have several values (e.g. Malay ...
According to the book Understanding Case Study Research, case studies are "small scale research with meaning" that generally involve the following: The study of a particular case, or a number of cases. That the case will be complex and bounded. That it will be studied in its context. That the analysis undertaken will seek to be holistic.
Each qualitative research approach or design has its own terms for methods of data analysis: Ethnography—uses modified thematic analysis and life histories. Case study—uses description, categorical aggregation, or direct interpretation. Grounded theory—uses open, axial, and selective coding (although recent writers are proposing ...
Definitions of qualitative case study research. Case study research is an investigation and analysis of a single or collective case, intended to capture the complexity of the object of study (Stake, 1995).Qualitative case study research, as described by Stake (), draws together "naturalistic, holistic, ethnographic, phenomenological, and biographic research methods" in a bricoleur design ...
There are many different research methods you can use to collect data on your subject. Case studies tend to focus on qualitative data using methods such as interviews, observations, and analysis of primary and secondary sources (e.g., newspaper articles, photographs, official records). Sometimes a case study will also collect quantitative data.
Qualitative Case Study. Although case studies have long been conducted in various academic fields, in the 1980s , they began to be recognized as a qualitative research method with the case study publications by researchers such as Merriam , Stake , Yin , and Hays . Case studies include both quantitative and qualitative strategies and can also ...
Therefore, the purpose of this paper is to provide a concise explanation of four common qualitative approaches, case study, ethnography, narrative, and phenomenology, demonstrating how each approach is linked to specific types of data collection and analysis. ... Qualitative data analysis: conceptual and practical considerations. Health ...
Learn the basics of qualitative data analysis (QDA) plus the most popular approaches, including content, discourse and narrative analysis. About Us; Services. 1-On-1 Coaching. ... Clear explanation on qualitative and how about Case study. Reply. Ogobuchi Otuu on January 31, 2023 at 5:40 pm This was helpful. Thank you. Reply.
As case study research is a flexible research method, qualitative data analysis methods are commonly used [176]. The basic objective of the analysis is, as in any other analysis, to derive conclusions from the data, keeping a clear chain of evidence.
Data sources The research example used is a multiple case study that explored the role of the clinical skills laboratory in preparing students for the real world of practice. Data analysis was conducted using a framework guided by the four stages of analysis outlined by Morse ( 1994 ): comprehending, synthesising, theorising and recontextualising.
This paper illustrates specific strategies that can be employed when conducting data analysis in case study research and other qualitative research designs with an example of how each stage of the analysis was conducted. AIM To illustrate an approach to data analysis in qualitative case study methodology. BACKGROUND There is often little detail in case study research about how data were ...
The study aims to explore the case study method with the formation of questions, data collection procedures and analysis, followed by how and on which position the saturation is achieved in developing a centralized Shariah governance framework for Islamic banks in Bangladesh.,Using purposive and snowball sampling procedures, data have been ...
Table 2 gives a comparison of existing rough set-based schemes for quantitative and qualitative analysis. The comparative parameters include handling hybrid data, generalized NRS, attribute ...
In the qualitative data analysis process outlined in this article, I break the coding process into five distinct phases. ... (1995) to conduct an analysis of case study data, the phases offered in this paper - particularly phases 3 through 5 - provide a more structured presentation of the stages of direct interpretation, categorical ...
This study used a Qualitative Comparative Analysis (QCA) approach to re-analyse evidence from existing systematic reviews to identify essential intervention components that lead to the successful implementation of non-clinical interventions focusing on pregnant women to optimise the use of CS. ... The data table is a matrix in which each case ...
Using multiple data collection methods is a key characteristic of all case study methodology; it enhances the credibility of the findings by allowing different facets and views of the phenomenon to be explored. 23 Common methods include interviews, focus groups, observation, and document analysis. 5,37 By seeking patterns within and across data ...
The literature review and case study analysis underscore that implementing vernacular solutions enhances a project's identity and resident satisfaction, fostering affordability. ... Qualitative data collection and analysis: Industrialized building systems : Analysis of the housing shortage strategies:
Design. The study had a qualitative, exploratory and descriptive intent. Data was collected by focus group interviews, as this is a suitable way of getting information about factors which may influence motivation, opinions and behaviour in complex social settings [].Reflexive thematic analysis according to Braun and Clarke was chosen as method for data analysis as it may be used to understand ...
Further, often the contribution of a qualitative case study research (QCSR) emerges from the 'extension of a theory' or 'developing deeper understanding—fresh meaning of a phenomenon'. ... Thematic analysis of qualitative data: AMEE Guide No. 131. Medical Teacher. Crossref. PubMed. Google Scholar. Ryan G. W., & Bernard H. R. (2003 ...
This study is part of a larger phase II, randomized, controlled clinical trial "Communication, Coordination and Security for people with severe Multiple Sclerosis (COCOS-MS)" [].This explorative clinical trial, employing a mixed-method design, incorporates a qualitative study component with PwsMS, caregivers and HCSs to enrich the findings of the quantitative data.
INTRODUCTION. In an earlier paper, 1 we presented an introduction to using qualitative research methods in pharmacy practice. In this article, we review some principles of the collection, analysis, and management of qualitative data to help pharmacists interested in doing research in their practice to continue their learning in this area.
Case Study: Data Sources, Data Analysis, Alignment, and Rationale Grand Canyon University RES 843: Designing a Qualitative Study 2 Dr. Patrick Jefferson January 10, 2024, 1. Problem Statement It is not known how millennial leaders describe their abilities, personal traits, and motivators to bring about performance and productivity in the work ...
Methods. Using a population of 136 interactions involving suspected violations of COVID-19 ordinance violations between March 2020 and November 2020, this study uses convergent holistic triangulation within a mixed-method research design to extract data for quantitative analysis paired with qualitative analysis of transcripts and BWC footage.