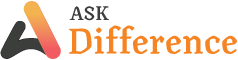
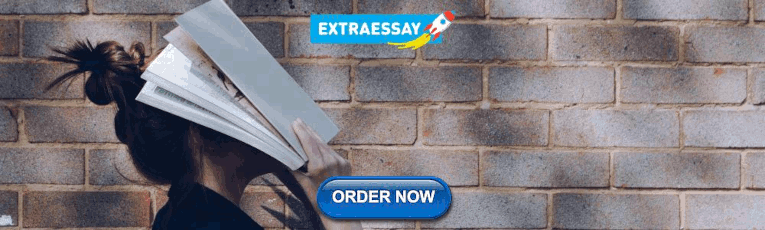
Survey vs. Research — What's the Difference?

Difference Between Survey and Research
Table of contents, key differences, comparison chart, compare with definitions, common curiosities, is research always based on surveys, what is the main goal of conducting a survey, can the results of a survey be used in research, how does research contribute to knowledge, can a survey be considered research, what makes research different from mere data collection, how important is methodology in research, can research be conducted without a survey, can a single survey answer complex research questions, what ethical considerations are important in surveys and research, is participant selection important in surveys and research, how do the outcomes of surveys and research differ, how is research validated, what role do surveys play in market research, can the findings from a survey be challenged, share your discovery.

Author Spotlight

Popular Comparisons
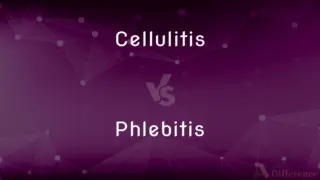
Trending Comparisons
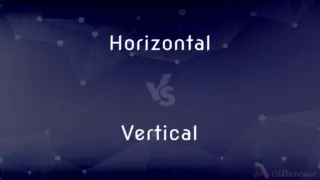
New Comparisons
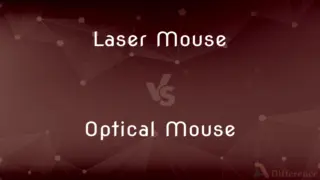
Trending Terms
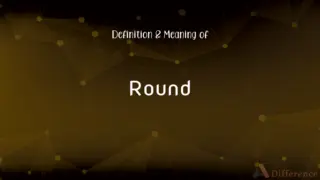

An official website of the United States government
The .gov means it’s official. Federal government websites often end in .gov or .mil. Before sharing sensitive information, make sure you’re on a federal government site.
The site is secure. The https:// ensures that you are connecting to the official website and that any information you provide is encrypted and transmitted securely.
- Publications
- Account settings
Preview improvements coming to the PMC website in October 2024. Learn More or Try it out now .
- Advanced Search
- Journal List
- J Adv Pract Oncol
- v.6(2); Mar-Apr 2015
Understanding and Evaluating Survey Research
A variety of methodologic approaches exist for individuals interested in conducting research. Selection of a research approach depends on a number of factors, including the purpose of the research, the type of research questions to be answered, and the availability of resources. The purpose of this article is to describe survey research as one approach to the conduct of research so that the reader can critically evaluate the appropriateness of the conclusions from studies employing survey research.
SURVEY RESEARCH
Survey research is defined as "the collection of information from a sample of individuals through their responses to questions" ( Check & Schutt, 2012, p. 160 ). This type of research allows for a variety of methods to recruit participants, collect data, and utilize various methods of instrumentation. Survey research can use quantitative research strategies (e.g., using questionnaires with numerically rated items), qualitative research strategies (e.g., using open-ended questions), or both strategies (i.e., mixed methods). As it is often used to describe and explore human behavior, surveys are therefore frequently used in social and psychological research ( Singleton & Straits, 2009 ).
Information has been obtained from individuals and groups through the use of survey research for decades. It can range from asking a few targeted questions of individuals on a street corner to obtain information related to behaviors and preferences, to a more rigorous study using multiple valid and reliable instruments. Common examples of less rigorous surveys include marketing or political surveys of consumer patterns and public opinion polls.
Survey research has historically included large population-based data collection. The primary purpose of this type of survey research was to obtain information describing characteristics of a large sample of individuals of interest relatively quickly. Large census surveys obtaining information reflecting demographic and personal characteristics and consumer feedback surveys are prime examples. These surveys were often provided through the mail and were intended to describe demographic characteristics of individuals or obtain opinions on which to base programs or products for a population or group.
More recently, survey research has developed into a rigorous approach to research, with scientifically tested strategies detailing who to include (representative sample), what and how to distribute (survey method), and when to initiate the survey and follow up with nonresponders (reducing nonresponse error), in order to ensure a high-quality research process and outcome. Currently, the term "survey" can reflect a range of research aims, sampling and recruitment strategies, data collection instruments, and methods of survey administration.
Given this range of options in the conduct of survey research, it is imperative for the consumer/reader of survey research to understand the potential for bias in survey research as well as the tested techniques for reducing bias, in order to draw appropriate conclusions about the information reported in this manner. Common types of error in research, along with the sources of error and strategies for reducing error as described throughout this article, are summarized in the Table .

Sources of Error in Survey Research and Strategies to Reduce Error
The goal of sampling strategies in survey research is to obtain a sufficient sample that is representative of the population of interest. It is often not feasible to collect data from an entire population of interest (e.g., all individuals with lung cancer); therefore, a subset of the population or sample is used to estimate the population responses (e.g., individuals with lung cancer currently receiving treatment). A large random sample increases the likelihood that the responses from the sample will accurately reflect the entire population. In order to accurately draw conclusions about the population, the sample must include individuals with characteristics similar to the population.
It is therefore necessary to correctly identify the population of interest (e.g., individuals with lung cancer currently receiving treatment vs. all individuals with lung cancer). The sample will ideally include individuals who reflect the intended population in terms of all characteristics of the population (e.g., sex, socioeconomic characteristics, symptom experience) and contain a similar distribution of individuals with those characteristics. As discussed by Mady Stovall beginning on page 162, Fujimori et al. ( 2014 ), for example, were interested in the population of oncologists. The authors obtained a sample of oncologists from two hospitals in Japan. These participants may or may not have similar characteristics to all oncologists in Japan.
Participant recruitment strategies can affect the adequacy and representativeness of the sample obtained. Using diverse recruitment strategies can help improve the size of the sample and help ensure adequate coverage of the intended population. For example, if a survey researcher intends to obtain a sample of individuals with breast cancer representative of all individuals with breast cancer in the United States, the researcher would want to use recruitment strategies that would recruit both women and men, individuals from rural and urban settings, individuals receiving and not receiving active treatment, and so on. Because of the difficulty in obtaining samples representative of a large population, researchers may focus the population of interest to a subset of individuals (e.g., women with stage III or IV breast cancer). Large census surveys require extremely large samples to adequately represent the characteristics of the population because they are intended to represent the entire population.
DATA COLLECTION METHODS
Survey research may use a variety of data collection methods with the most common being questionnaires and interviews. Questionnaires may be self-administered or administered by a professional, may be administered individually or in a group, and typically include a series of items reflecting the research aims. Questionnaires may include demographic questions in addition to valid and reliable research instruments ( Costanzo, Stawski, Ryff, Coe, & Almeida, 2012 ; DuBenske et al., 2014 ; Ponto, Ellington, Mellon, & Beck, 2010 ). It is helpful to the reader when authors describe the contents of the survey questionnaire so that the reader can interpret and evaluate the potential for errors of validity (e.g., items or instruments that do not measure what they are intended to measure) and reliability (e.g., items or instruments that do not measure a construct consistently). Helpful examples of articles that describe the survey instruments exist in the literature ( Buerhaus et al., 2012 ).
Questionnaires may be in paper form and mailed to participants, delivered in an electronic format via email or an Internet-based program such as SurveyMonkey, or a combination of both, giving the participant the option to choose which method is preferred ( Ponto et al., 2010 ). Using a combination of methods of survey administration can help to ensure better sample coverage (i.e., all individuals in the population having a chance of inclusion in the sample) therefore reducing coverage error ( Dillman, Smyth, & Christian, 2014 ; Singleton & Straits, 2009 ). For example, if a researcher were to only use an Internet-delivered questionnaire, individuals without access to a computer would be excluded from participation. Self-administered mailed, group, or Internet-based questionnaires are relatively low cost and practical for a large sample ( Check & Schutt, 2012 ).
Dillman et al. ( 2014 ) have described and tested a tailored design method for survey research. Improving the visual appeal and graphics of surveys by using a font size appropriate for the respondents, ordering items logically without creating unintended response bias, and arranging items clearly on each page can increase the response rate to electronic questionnaires. Attending to these and other issues in electronic questionnaires can help reduce measurement error (i.e., lack of validity or reliability) and help ensure a better response rate.
Conducting interviews is another approach to data collection used in survey research. Interviews may be conducted by phone, computer, or in person and have the benefit of visually identifying the nonverbal response(s) of the interviewee and subsequently being able to clarify the intended question. An interviewer can use probing comments to obtain more information about a question or topic and can request clarification of an unclear response ( Singleton & Straits, 2009 ). Interviews can be costly and time intensive, and therefore are relatively impractical for large samples.
Some authors advocate for using mixed methods for survey research when no one method is adequate to address the planned research aims, to reduce the potential for measurement and non-response error, and to better tailor the study methods to the intended sample ( Dillman et al., 2014 ; Singleton & Straits, 2009 ). For example, a mixed methods survey research approach may begin with distributing a questionnaire and following up with telephone interviews to clarify unclear survey responses ( Singleton & Straits, 2009 ). Mixed methods might also be used when visual or auditory deficits preclude an individual from completing a questionnaire or participating in an interview.
FUJIMORI ET AL.: SURVEY RESEARCH
Fujimori et al. ( 2014 ) described the use of survey research in a study of the effect of communication skills training for oncologists on oncologist and patient outcomes (e.g., oncologist’s performance and confidence and patient’s distress, satisfaction, and trust). A sample of 30 oncologists from two hospitals was obtained and though the authors provided a power analysis concluding an adequate number of oncologist participants to detect differences between baseline and follow-up scores, the conclusions of the study may not be generalizable to a broader population of oncologists. Oncologists were randomized to either an intervention group (i.e., communication skills training) or a control group (i.e., no training).
Fujimori et al. ( 2014 ) chose a quantitative approach to collect data from oncologist and patient participants regarding the study outcome variables. Self-report numeric ratings were used to measure oncologist confidence and patient distress, satisfaction, and trust. Oncologist confidence was measured using two instruments each using 10-point Likert rating scales. The Hospital Anxiety and Depression Scale (HADS) was used to measure patient distress and has demonstrated validity and reliability in a number of populations including individuals with cancer ( Bjelland, Dahl, Haug, & Neckelmann, 2002 ). Patient satisfaction and trust were measured using 0 to 10 numeric rating scales. Numeric observer ratings were used to measure oncologist performance of communication skills based on a videotaped interaction with a standardized patient. Participants completed the same questionnaires at baseline and follow-up.
The authors clearly describe what data were collected from all participants. Providing additional information about the manner in which questionnaires were distributed (i.e., electronic, mail), the setting in which data were collected (e.g., home, clinic), and the design of the survey instruments (e.g., visual appeal, format, content, arrangement of items) would assist the reader in drawing conclusions about the potential for measurement and nonresponse error. The authors describe conducting a follow-up phone call or mail inquiry for nonresponders, using the Dillman et al. ( 2014 ) tailored design for survey research follow-up may have reduced nonresponse error.
CONCLUSIONS
Survey research is a useful and legitimate approach to research that has clear benefits in helping to describe and explore variables and constructs of interest. Survey research, like all research, has the potential for a variety of sources of error, but several strategies exist to reduce the potential for error. Advanced practitioners aware of the potential sources of error and strategies to improve survey research can better determine how and whether the conclusions from a survey research study apply to practice.
The author has no potential conflicts of interest to disclose.
- Skip to main content
- Skip to primary sidebar
- Skip to footer
- QuestionPro

- Solutions Industries Gaming Automotive Sports and events Education Government Travel & Hospitality Financial Services Healthcare Cannabis Technology Use Case NPS+ Communities Audience Contactless surveys Mobile LivePolls Member Experience GDPR Positive People Science 360 Feedback Surveys
- Resources Blog eBooks Survey Templates Case Studies Training Help center

Home Market Research
Survey Research: Definition, Examples and Methods
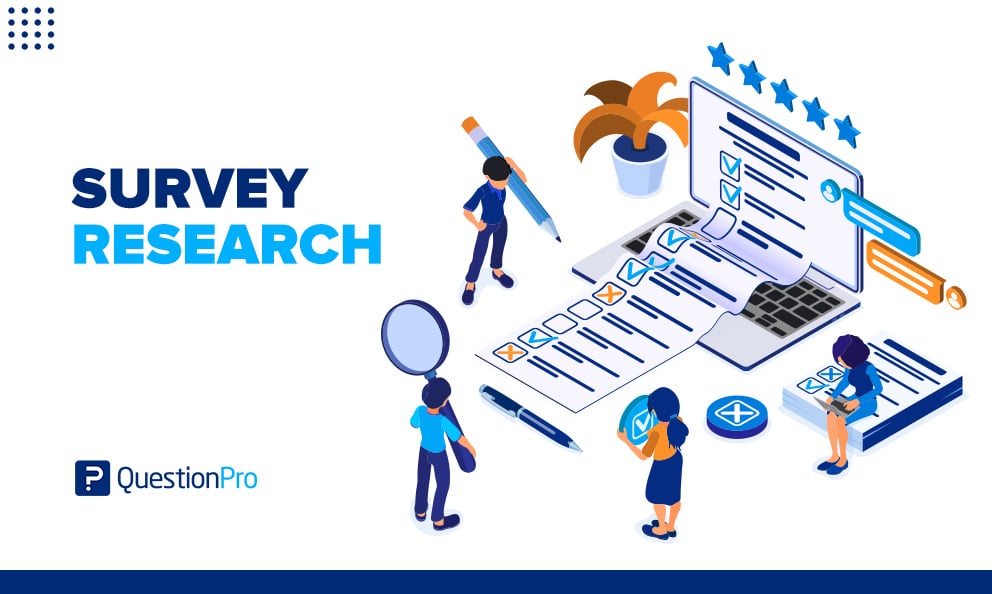
Survey Research is a quantitative research method used for collecting data from a set of respondents. It has been perhaps one of the most used methodologies in the industry for several years due to the multiple benefits and advantages that it has when collecting and analyzing data.
LEARN ABOUT: Behavioral Research
In this article, you will learn everything about survey research, such as types, methods, and examples.
Survey Research Definition
Survey Research is defined as the process of conducting research using surveys that researchers send to survey respondents. The data collected from surveys is then statistically analyzed to draw meaningful research conclusions. In the 21st century, every organization’s eager to understand what their customers think about their products or services and make better business decisions. Researchers can conduct research in multiple ways, but surveys are proven to be one of the most effective and trustworthy research methods. An online survey is a method for extracting information about a significant business matter from an individual or a group of individuals. It consists of structured survey questions that motivate the participants to respond. Creditable survey research can give these businesses access to a vast information bank. Organizations in media, other companies, and even governments rely on survey research to obtain accurate data.
The traditional definition of survey research is a quantitative method for collecting information from a pool of respondents by asking multiple survey questions. This research type includes the recruitment of individuals collection, and analysis of data. It’s useful for researchers who aim to communicate new features or trends to their respondents.
LEARN ABOUT: Level of Analysis Generally, it’s the primary step towards obtaining quick information about mainstream topics and conducting more rigorous and detailed quantitative research methods like surveys/polls or qualitative research methods like focus groups/on-call interviews can follow. There are many situations where researchers can conduct research using a blend of both qualitative and quantitative strategies.
LEARN ABOUT: Survey Sampling
Survey Research Methods
Survey research methods can be derived based on two critical factors: Survey research tool and time involved in conducting research. There are three main survey research methods, divided based on the medium of conducting survey research:
- Online/ Email: Online survey research is one of the most popular survey research methods today. The survey cost involved in online survey research is extremely minimal, and the responses gathered are highly accurate.
- Phone: Survey research conducted over the telephone ( CATI survey ) can be useful in collecting data from a more extensive section of the target population. There are chances that the money invested in phone surveys will be higher than other mediums, and the time required will be higher.
- Face-to-face: Researchers conduct face-to-face in-depth interviews in situations where there is a complicated problem to solve. The response rate for this method is the highest, but it can be costly.
Further, based on the time taken, survey research can be classified into two methods:
- Longitudinal survey research: Longitudinal survey research involves conducting survey research over a continuum of time and spread across years and decades. The data collected using this survey research method from one time period to another is qualitative or quantitative. Respondent behavior, preferences, and attitudes are continuously observed over time to analyze reasons for a change in behavior or preferences. For example, suppose a researcher intends to learn about the eating habits of teenagers. In that case, he/she will follow a sample of teenagers over a considerable period to ensure that the collected information is reliable. Often, cross-sectional survey research follows a longitudinal study .
- Cross-sectional survey research: Researchers conduct a cross-sectional survey to collect insights from a target audience at a particular time interval. This survey research method is implemented in various sectors such as retail, education, healthcare, SME businesses, etc. Cross-sectional studies can either be descriptive or analytical. It is quick and helps researchers collect information in a brief period. Researchers rely on the cross-sectional survey research method in situations where descriptive analysis of a subject is required.
Survey research also is bifurcated according to the sampling methods used to form samples for research: Probability and Non-probability sampling. Every individual in a population should be considered equally to be a part of the survey research sample. Probability sampling is a sampling method in which the researcher chooses the elements based on probability theory. The are various probability research methods, such as simple random sampling , systematic sampling, cluster sampling, stratified random sampling, etc. Non-probability sampling is a sampling method where the researcher uses his/her knowledge and experience to form samples.
LEARN ABOUT: Survey Sample Sizes
The various non-probability sampling techniques are :
- Convenience sampling
- Snowball sampling
- Consecutive sampling
- Judgemental sampling
- Quota sampling
Process of implementing survey research methods:
- Decide survey questions: Brainstorm and put together valid survey questions that are grammatically and logically appropriate. Understanding the objective and expected outcomes of the survey helps a lot. There are many surveys where details of responses are not as important as gaining insights about what customers prefer from the provided options. In such situations, a researcher can include multiple-choice questions or closed-ended questions . Whereas, if researchers need to obtain details about specific issues, they can consist of open-ended questions in the questionnaire. Ideally, the surveys should include a smart balance of open-ended and closed-ended questions. Use survey questions like Likert Scale , Semantic Scale, Net Promoter Score question, etc., to avoid fence-sitting.
LEARN ABOUT: System Usability Scale
- Finalize a target audience: Send out relevant surveys as per the target audience and filter out irrelevant questions as per the requirement. The survey research will be instrumental in case the target population decides on a sample. This way, results can be according to the desired market and be generalized to the entire population.
LEARN ABOUT: Testimonial Questions
- Send out surveys via decided mediums: Distribute the surveys to the target audience and patiently wait for the feedback and comments- this is the most crucial step of the survey research. The survey needs to be scheduled, keeping in mind the nature of the target audience and its regions. Surveys can be conducted via email, embedded in a website, shared via social media, etc., to gain maximum responses.
- Analyze survey results: Analyze the feedback in real-time and identify patterns in the responses which might lead to a much-needed breakthrough for your organization. GAP, TURF Analysis , Conjoint analysis, Cross tabulation, and many such survey feedback analysis methods can be used to spot and shed light on respondent behavior. Researchers can use the results to implement corrective measures to improve customer/employee satisfaction.
Reasons to conduct survey research
The most crucial and integral reason for conducting market research using surveys is that you can collect answers regarding specific, essential questions. You can ask these questions in multiple survey formats as per the target audience and the intent of the survey. Before designing a study, every organization must figure out the objective of carrying this out so that the study can be structured, planned, and executed to perfection.
LEARN ABOUT: Research Process Steps
Questions that need to be on your mind while designing a survey are:
- What is the primary aim of conducting the survey?
- How do you plan to utilize the collected survey data?
- What type of decisions do you plan to take based on the points mentioned above?
There are three critical reasons why an organization must conduct survey research.
- Understand respondent behavior to get solutions to your queries: If you’ve carefully curated a survey, the respondents will provide insights about what they like about your organization as well as suggestions for improvement. To motivate them to respond, you must be very vocal about how secure their responses will be and how you will utilize the answers. This will push them to be 100% honest about their feedback, opinions, and comments. Online surveys or mobile surveys have proved their privacy, and due to this, more and more respondents feel free to put forth their feedback through these mediums.
- Present a medium for discussion: A survey can be the perfect platform for respondents to provide criticism or applause for an organization. Important topics like product quality or quality of customer service etc., can be put on the table for discussion. A way you can do it is by including open-ended questions where the respondents can write their thoughts. This will make it easy for you to correlate your survey to what you intend to do with your product or service.
- Strategy for never-ending improvements: An organization can establish the target audience’s attributes from the pilot phase of survey research . Researchers can use the criticism and feedback received from this survey to improve the product/services. Once the company successfully makes the improvements, it can send out another survey to measure the change in feedback keeping the pilot phase the benchmark. By doing this activity, the organization can track what was effectively improved and what still needs improvement.
Survey Research Scales
There are four main scales for the measurement of variables:
- Nominal Scale: A nominal scale associates numbers with variables for mere naming or labeling, and the numbers usually have no other relevance. It is the most basic of the four levels of measurement.
- Ordinal Scale: The ordinal scale has an innate order within the variables along with labels. It establishes the rank between the variables of a scale but not the difference value between the variables.
- Interval Scale: The interval scale is a step ahead in comparison to the other two scales. Along with establishing a rank and name of variables, the scale also makes known the difference between the two variables. The only drawback is that there is no fixed start point of the scale, i.e., the actual zero value is absent.
- Ratio Scale: The ratio scale is the most advanced measurement scale, which has variables that are labeled in order and have a calculated difference between variables. In addition to what interval scale orders, this scale has a fixed starting point, i.e., the actual zero value is present.
Benefits of survey research
In case survey research is used for all the right purposes and is implemented properly, marketers can benefit by gaining useful, trustworthy data that they can use to better the ROI of the organization.
Other benefits of survey research are:
- Minimum investment: Mobile surveys and online surveys have minimal finance invested per respondent. Even with the gifts and other incentives provided to the people who participate in the study, online surveys are extremely economical compared to paper-based surveys.
- Versatile sources for response collection: You can conduct surveys via various mediums like online and mobile surveys. You can further classify them into qualitative mediums like focus groups , and interviews and quantitative mediums like customer-centric surveys. Due to the offline survey response collection option, researchers can conduct surveys in remote areas with limited internet connectivity. This can make data collection and analysis more convenient and extensive.
- Reliable for respondents: Surveys are extremely secure as the respondent details and responses are kept safeguarded. This anonymity makes respondents answer the survey questions candidly and with absolute honesty. An organization seeking to receive explicit responses for its survey research must mention that it will be confidential.
Survey research design
Researchers implement a survey research design in cases where there is a limited cost involved and there is a need to access details easily. This method is often used by small and large organizations to understand and analyze new trends, market demands, and opinions. Collecting information through tactfully designed survey research can be much more effective and productive than a casually conducted survey.
There are five stages of survey research design:
- Decide an aim of the research: There can be multiple reasons for a researcher to conduct a survey, but they need to decide a purpose for the research. This is the primary stage of survey research as it can mold the entire path of a survey, impacting its results.
- Filter the sample from target population: Who to target? is an essential question that a researcher should answer and keep in mind while conducting research. The precision of the results is driven by who the members of a sample are and how useful their opinions are. The quality of respondents in a sample is essential for the results received for research and not the quantity. If a researcher seeks to understand whether a product feature will work well with their target market, he/she can conduct survey research with a group of market experts for that product or technology.
- Zero-in on a survey method: Many qualitative and quantitative research methods can be discussed and decided. Focus groups, online interviews, surveys, polls, questionnaires, etc. can be carried out with a pre-decided sample of individuals.
- Design the questionnaire: What will the content of the survey be? A researcher is required to answer this question to be able to design it effectively. What will the content of the cover letter be? Or what are the survey questions of this questionnaire? Understand the target market thoroughly to create a questionnaire that targets a sample to gain insights about a survey research topic.
- Send out surveys and analyze results: Once the researcher decides on which questions to include in a study, they can send it across to the selected sample . Answers obtained from this survey can be analyzed to make product-related or marketing-related decisions.
Survey examples: 10 tips to design the perfect research survey
Picking the right survey design can be the key to gaining the information you need to make crucial decisions for all your research. It is essential to choose the right topic, choose the right question types, and pick a corresponding design. If this is your first time creating a survey, it can seem like an intimidating task. But with QuestionPro, each step of the process is made simple and easy.
Below are 10 Tips To Design The Perfect Research Survey:
- Set your SMART goals: Before conducting any market research or creating a particular plan, set your SMART Goals . What is that you want to achieve with the survey? How will you measure it promptly, and what are the results you are expecting?
- Choose the right questions: Designing a survey can be a tricky task. Asking the right questions may help you get the answers you are looking for and ease the task of analyzing. So, always choose those specific questions – relevant to your research.
- Begin your survey with a generalized question: Preferably, start your survey with a general question to understand whether the respondent uses the product or not. That also provides an excellent base and intro for your survey.
- Enhance your survey: Choose the best, most relevant, 15-20 questions. Frame each question as a different question type based on the kind of answer you would like to gather from each. Create a survey using different types of questions such as multiple-choice, rating scale, open-ended, etc. Look at more survey examples and four measurement scales every researcher should remember.
- Prepare yes/no questions: You may also want to use yes/no questions to separate people or branch them into groups of those who “have purchased” and those who “have not yet purchased” your products or services. Once you separate them, you can ask them different questions.
- Test all electronic devices: It becomes effortless to distribute your surveys if respondents can answer them on different electronic devices like mobiles, tablets, etc. Once you have created your survey, it’s time to TEST. You can also make any corrections if needed at this stage.
- Distribute your survey: Once your survey is ready, it is time to share and distribute it to the right audience. You can share handouts and share them via email, social media, and other industry-related offline/online communities.
- Collect and analyze responses: After distributing your survey, it is time to gather all responses. Make sure you store your results in a particular document or an Excel sheet with all the necessary categories mentioned so that you don’t lose your data. Remember, this is the most crucial stage. Segregate your responses based on demographics, psychographics, and behavior. This is because, as a researcher, you must know where your responses are coming from. It will help you to analyze, predict decisions, and help write the summary report.
- Prepare your summary report: Now is the time to share your analysis. At this stage, you should mention all the responses gathered from a survey in a fixed format. Also, the reader/customer must get clarity about your goal, which you were trying to gain from the study. Questions such as – whether the product or service has been used/preferred or not. Do respondents prefer some other product to another? Any recommendations?
Having a tool that helps you carry out all the necessary steps to carry out this type of study is a vital part of any project. At QuestionPro, we have helped more than 10,000 clients around the world to carry out data collection in a simple and effective way, in addition to offering a wide range of solutions to take advantage of this data in the best possible way.
From dashboards, advanced analysis tools, automation, and dedicated functions, in QuestionPro, you will find everything you need to execute your research projects effectively. Uncover insights that matter the most!
MORE LIKE THIS
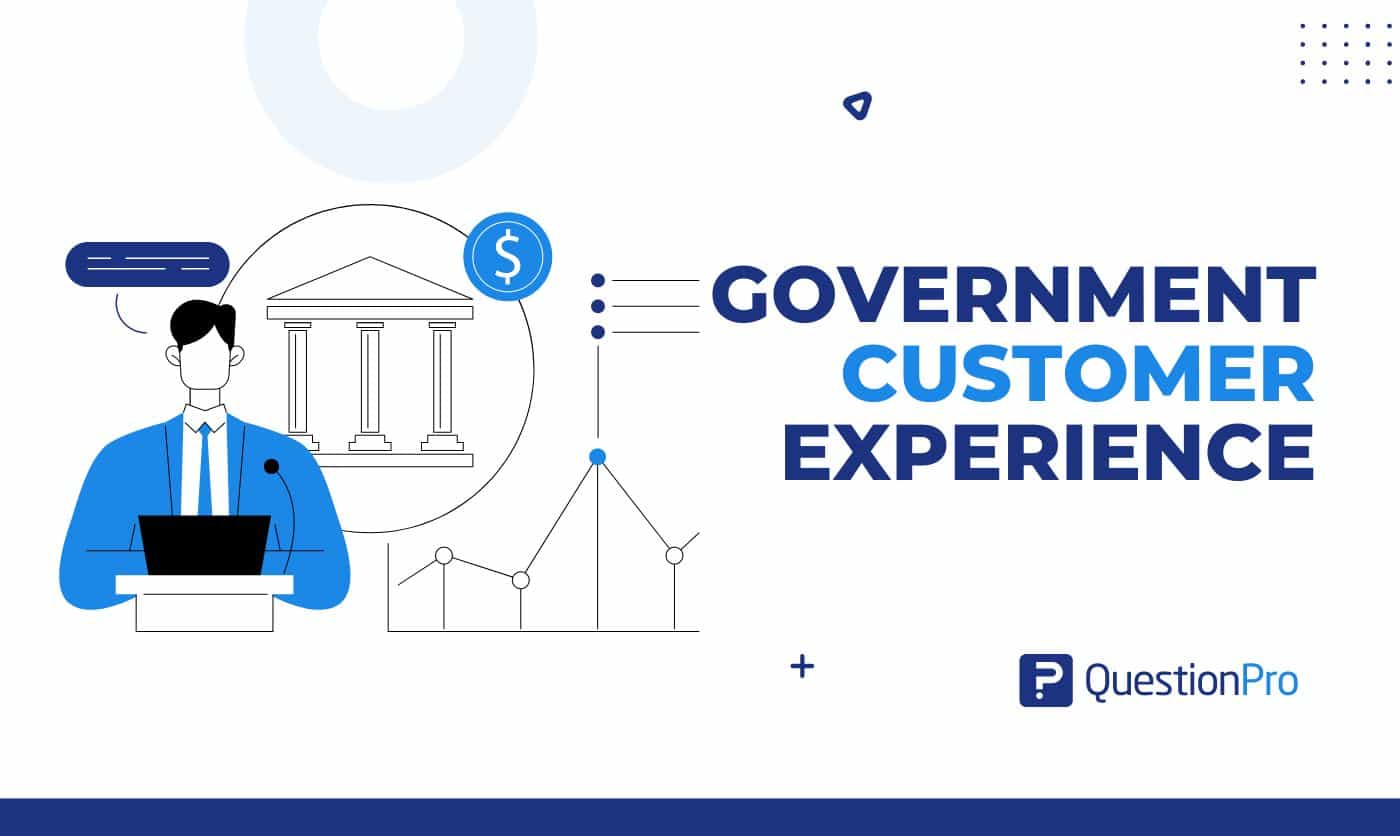
Government Customer Experience: Impact on Government Service
Apr 11, 2024
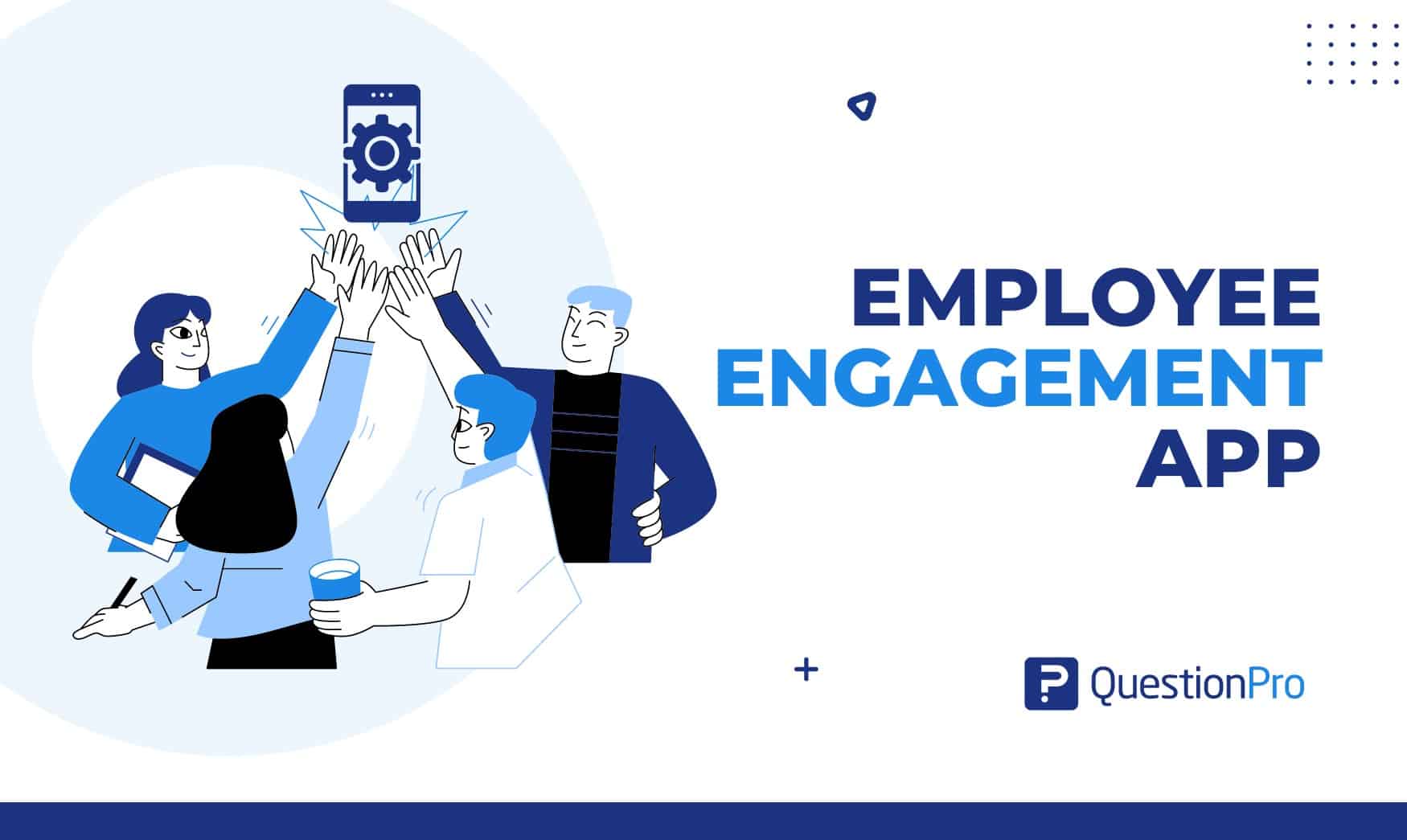
Employee Engagement App: Top 11 For Workforce Improvement
Apr 10, 2024
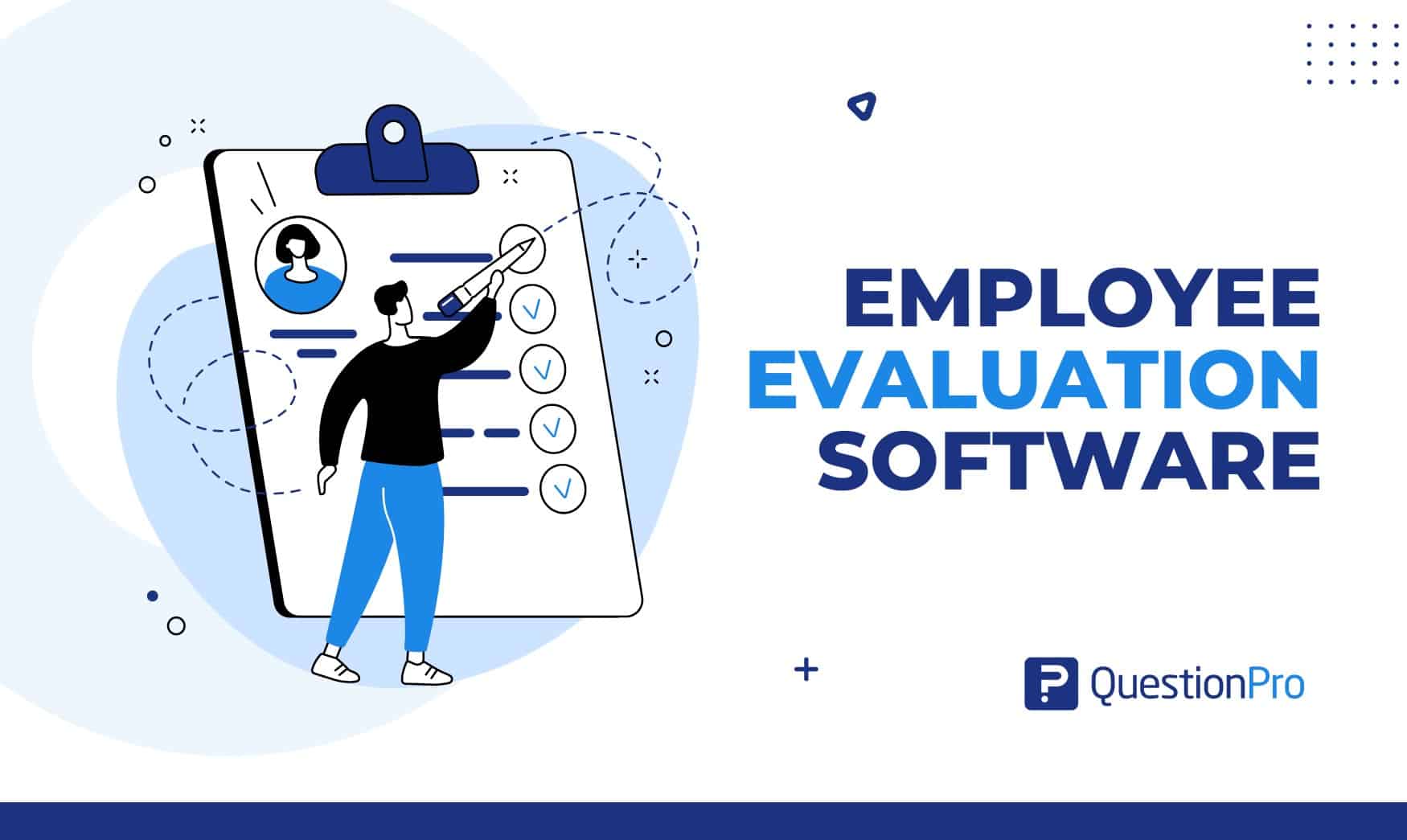
Top 15 Employee Evaluation Software to Enhance Performance
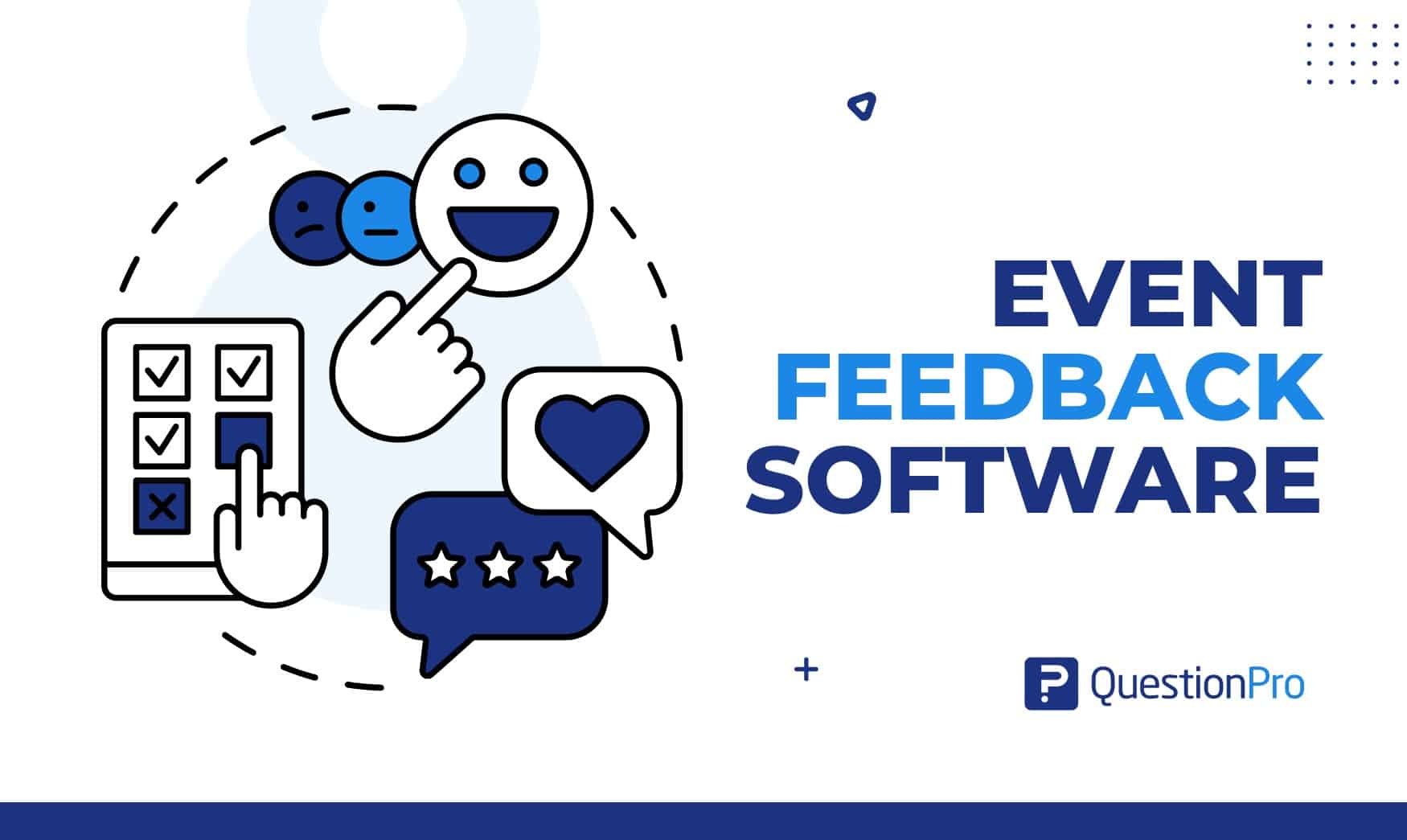
Event Feedback Software: Top 11 Best in 2024
Apr 9, 2024
Other categories
- Academic Research
- Artificial Intelligence
- Assessments
- Brand Awareness
- Case Studies
- Communities
- Consumer Insights
- Customer effort score
- Customer Engagement
- Customer Experience
- Customer Loyalty
- Customer Research
- Customer Satisfaction
- Employee Benefits
- Employee Engagement
- Employee Retention
- Friday Five
- General Data Protection Regulation
- Insights Hub
- Life@QuestionPro
- Market Research
- Mobile diaries
- Mobile Surveys
- New Features
- Online Communities
- Question Types
- Questionnaire
- QuestionPro Products
- Release Notes
- Research Tools and Apps
- Revenue at Risk
- Survey Templates
- Training Tips
- Uncategorized
- Video Learning Series
- What’s Coming Up
- Workforce Intelligence
A Comprehensive Guide to Survey Research Methodologies
For decades, researchers and businesses have used survey research to produce statistical data and explore ideas. The survey process is simple, ask questions and analyze the responses to make decisions. Data is what makes the difference between a valid and invalid statement and as the American statistician, W. Edwards Deming said:
“Without data, you’re just another person with an opinion.” - W. Edwards Deming
In this article, we will discuss what survey research is, its brief history, types, common uses, benefits, and the step-by-step process of designing a survey.
What is Survey Research
A survey is a research method that is used to collect data from a group of respondents in order to gain insights and information regarding a particular subject. It’s an excellent method to gather opinions and understand how and why people feel a certain way about different situations and contexts.
Brief History of Survey Research
Survey research may have its roots in the American and English “social surveys” conducted around the turn of the 20th century. The surveys were mainly conducted by researchers and reformers to document the extent of social issues such as poverty. ( 1 ) Despite being a relatively young field to many scientific domains, survey research has experienced three stages of development ( 2 ):
- First Era (1930-1960)
- Second Era (1960-1990)
- Third Era (1990 onwards)
Over the years, survey research adapted to the changing times and technologies. By exploiting the latest technologies, researchers can gain access to the right population from anywhere in the world, analyze the data like never before, and extract useful information.
Survey Research Methods & Types
Survey research can be classified into seven categories based on objective, data sources, methodology, deployment method, and frequency of deployment.

Surveys based on Objective
Exploratory survey research.
Exploratory survey research is aimed at diving deeper into research subjects and finding out more about their context. It’s important for marketing or business strategy and the focus is to discover ideas and insights instead of gathering statistical data.
Generally, exploratory survey research is composed of open-ended questions that allow respondents to express their thoughts and perspectives. The final responses present information from various sources that can lead to fresh initiatives.
Predictive Survey Research
Predictive survey research is also called causal survey research. It’s preplanned, structured, and quantitative in nature. It’s often referred to as conclusive research as it tries to explain the cause-and-effect relationship between different variables. The objective is to understand which variables are causes and which are effects and the nature of the relationship between both variables.
Descriptive Survey Research
Descriptive survey research is largely observational and is ideal for gathering numeric data. Due to its quantitative nature, it’s often compared to exploratory survey research. The difference between the two is that descriptive research is structured and pre-planned.
The idea behind descriptive research is to describe the mindset and opinion of a particular group of people on a given subject. The questions are every day multiple choices and users must choose from predefined categories. With predefined choices, you don’t get unique insights, rather, statistically inferable data.
Survey Research Types based on Concept Testing
Monadic concept testing.
Monadic testing is a survey research methodology in which the respondents are split into multiple groups and ask each group questions about a separate concept in isolation. Generally, monadic surveys are hyper-focused on a particular concept and shorter in duration. The important thing in monadic surveys is to avoid getting off-topic or exhausting the respondents with too many questions.
Sequential Monadic Concept Testing
Another approach to monadic testing is sequential monadic testing. In sequential monadic surveys, groups of respondents are surveyed in isolation. However, instead of surveying three groups on three different concepts, the researchers survey the same groups of people on three distinct concepts one after another. In a sequential monadic survey, at least two topics are included (in random order), and the same questions are asked for each concept to eliminate bias.
Based on Data Source
Primary data.
Data obtained directly from the source or target population is referred to as primary survey data. When it comes to primary data collection, researchers usually devise a set of questions and invite people with knowledge of the subject to respond. The main sources of primary data are interviews, questionnaires, surveys, and observation methods.
Compared to secondary data, primary data is gathered from first-hand sources and is more reliable. However, the process of primary data collection is both costly and time-consuming.
Secondary Data
Survey research is generally used to collect first-hand information from a respondent. However, surveys can also be designed to collect and process secondary data. It’s collected from third-party sources or primary sources in the past.
This type of data is usually generic, readily available, and cheaper than primary data collection. Some common sources of secondary data are books, data collected from older surveys, online data, and data from government archives. Beware that you might compromise the validity of your findings if you end up with irrelevant or inflated data.
Based on Research Method
Quantitative research.
Quantitative research is a popular research methodology that is used to collect numeric data in a systematic investigation. It’s frequently used in research contexts where statistical data is required, such as sciences or social sciences. Quantitative research methods include polls, systematic observations, and face-to-face interviews.
Qualitative Research
Qualitative research is a research methodology where you collect non-numeric data from research participants. In this context, the participants are not restricted to a specific system and provide open-ended information. Some common qualitative research methods include focus groups, one-on-one interviews, observations, and case studies.
Based on Deployment Method
Online surveys.
With technology advancing rapidly, the most popular method of survey research is an online survey. With the internet, you can not only reach a broader audience but also design and customize a survey and deploy it from anywhere. Online surveys have outperformed offline survey methods as they are less expensive and allow researchers to easily collect and analyze data from a large sample.
Paper or Print Surveys
As the name suggests, paper or print surveys use the traditional paper and pencil approach to collect data. Before the invention of computers, paper surveys were the survey method of choice.
Though many would assume that surveys are no longer conducted on paper, it's still a reliable method of collecting information during field research and data collection. However, unlike online surveys, paper surveys are expensive and require extra human resources.
Telephonic Surveys
Telephonic surveys are conducted over telephones where a researcher asks a series of questions to the respondent on the other end. Contacting respondents over a telephone requires less effort, human resources, and is less expensive.
What makes telephonic surveys debatable is that people are often reluctant in giving information over a phone call. Additionally, the success of such surveys depends largely on whether people are willing to invest their time on a phone call answering questions.
One-on-one Surveys
One-on-one surveys also known as face-to-face surveys are interviews where the researcher and respondent. Interacting directly with the respondent introduces the human factor into the survey.
Face-to-face interviews are useful when the researcher wants to discuss something personal with the respondent. The response rates in such surveys are always higher as the interview is being conducted in person. However, these surveys are quite expensive and the success of these depends on the knowledge and experience of the researcher.
Based on Distribution
The easiest and most common way of conducting online surveys is sending out an email. Sending out surveys via emails has a higher response rate as your target audience already knows about your brand and is likely to engage.
Buy Survey Responses
Purchasing survey responses also yields higher responses as the responders signed up for the survey. Businesses often purchase survey samples to conduct extensive research. Here, the target audience is often pre-screened to check if they're qualified to take part in the research.
Embedding Survey on a Website
Embedding surveys on a website is another excellent way to collect information. It allows your website visitors to take part in a survey without ever leaving the website and can be done while a person is entering or exiting the website.
Post the Survey on Social Media
Social media is an excellent medium to reach abroad range of audiences. You can publish your survey as a link on social media and people who are following the brand can take part and answer questions.
Based on Frequency of Deployment
Cross-sectional studies.
Cross-sectional studies are administered to a small sample from a large population within a short period of time. This provides researchers a peek into what the respondents are thinking at a given time. The surveys are usually short, precise, and specific to a particular situation.
Longitudinal Surveys
Longitudinal surveys are an extension of cross-sectional studies where researchers make an observation and collect data over extended periods of time. This type of survey can be further divided into three types:
- Trend surveys are employed to allow researchers to understand the change in the thought process of the respondents over some time.
- Panel surveys are administered to the same group of people over multiple years. These are usually expensive and researchers must stick to their panel to gather unbiased opinions.
- In cohort surveys, researchers identify a specific category of people and regularly survey them. Unlike panel surveys, the same people do not need to take part over the years, but each individual must fall into the researcher’s primary interest category.
Retrospective Survey
Retrospective surveys allow researchers to ask questions to gather data about past events and beliefs of the respondents. Since retrospective surveys also require years of data, they are similar to the longitudinal survey, except retrospective surveys are shorter and less expensive.
Why Should You Conduct Research Surveys?
“In God we trust. All others must bring data” - W. Edwards Deming
In the information age, survey research is of utmost importance and essential for understanding the opinion of your target population. Whether you’re launching a new product or conducting a social survey, the tool can be used to collect specific information from a defined set of respondents. The data collected via surveys can be further used by organizations to make informed decisions.
Furthermore, compared to other research methods, surveys are relatively inexpensive even if you’re giving out incentives. Compared to the older methods such as telephonic or paper surveys, online surveys have a smaller cost and the number of responses is higher.
What makes surveys useful is that they describe the characteristics of a large population. With a larger sample size , you can rely on getting more accurate results. However, you also need honest and open answers for accurate results. Since surveys are also anonymous and the responses remain confidential, respondents provide candid and accurate answers.
Common Uses of a Survey
Surveys are widely used in many sectors, but the most common uses of the survey research include:
- Market research : surveying a potential market to understand customer needs, preferences, and market demand.
- Customer Satisfaction: finding out your customer’s opinions about your services, products, or companies .
- Social research: investigating the characteristics and experiences of various social groups.
- Health research: collecting data about patients’ symptoms and treatments.
- Politics: evaluating public opinion regarding policies and political parties.
- Psychology: exploring personality traits, behaviors, and preferences.
6 Steps to Conduct Survey Research
An organization, person, or company conducts a survey when they need the information to make a decision but have insufficient data on hand. Following are six simple steps that can help you design a great survey.
Step 1: Objective of the Survey
The first step in survey research is defining an objective. The objective helps you define your target population and samples. The target population is the specific group of people you want to collect data from and since it’s rarely possible to survey the entire population, we target a specific sample from it. Defining a survey objective also benefits your respondents by helping them understand the reason behind the survey.
Step 2: Number of Questions
The number of questions or the size of the survey depends on the survey objective. However, it’s important to ensure that there are no redundant queries and the questions are in a logical order. Rephrased and repeated questions in a survey are almost as frustrating as in real life. For a higher completion rate, keep the questionnaire small so that the respondents stay engaged to the very end. The ideal length of an interview is less than 15 minutes. ( 2 )
Step 3: Language and Voice of Questions
While designing a survey, you may feel compelled to use fancy language. However, remember that difficult language is associated with higher survey dropout rates. You need to speak to the respondent in a clear, concise, and neutral manner, and ask simple questions. If your survey respondents are bilingual, then adding an option to translate your questions into another language can also prove beneficial.
Step 4: Type of Questions
In a survey, you can include any type of questions and even both closed-ended or open-ended questions. However, opt for the question types that are the easiest to understand for the respondents, and offer the most value. For example, compared to open-ended questions, people prefer to answer close-ended questions such as MCQs (multiple choice questions)and NPS (net promoter score) questions.
Step 5: User Experience
Designing a great survey is about more than just questions. A lot of researchers underestimate the importance of user experience and how it affects their response and completion rates. An inconsistent, difficult-to-navigate survey with technical errors and poor color choice is unappealing for the respondents. Make sure that your survey is easy to navigate for everyone and if you’re using rating scales, they remain consistent throughout the research study.
Additionally, don’t forget to design a good survey experience for both mobile and desktop users. According to Pew Research Center, nearly half of the smartphone users access the internet mainly from their mobile phones and 14 percent of American adults are smartphone-only internet users. ( 3 )
Step 6: Survey Logic
Last but not least, logic is another critical aspect of the survey design. If the survey logic is flawed, respondents may not continue in the right direction. Make sure to test the logic to ensure that selecting one answer leads to the next logical question instead of a series of unrelated queries.
How to Effectively Use Survey Research with Starlight Analytics
Designing and conducting a survey is almost as much science as it is an art. To craft great survey research, you need technical skills, consider the psychological elements, and have a broad understanding of marketing.
The ultimate goal of the survey is to ask the right questions in the right manner to acquire the right results.
Bringing a new product to the market is a long process and requires a lot of research and analysis. In your journey to gather information or ideas for your business, Starlight Analytics can be an excellent guide. Starlight Analytics' product concept testing helps you measure your product's market demand and refine product features and benefits so you can launch with confidence. The process starts with custom research to design the survey according to your needs, execute the survey, and deliver the key insights on time.
- Survey research in the United States: roots and emergence, 1890-1960 https://searchworks.stanford.edu/view/10733873
- How to create a survey questionnaire that gets great responses https://luc.id/knowledgehub/how-to-create-a-survey-questionnaire-that-gets-great-responses/
- Internet/broadband fact sheet https://www.pewresearch.org/internet/fact-sheet/internet-broadband/
Related Articles
A guide to competitive analysis & how to outperform your competitors.
Learn all about competitive analysis and its frameworks along with a step-by-step guide to conduct competitive analysis and outperform your competitors.
Fuel your innovation with data - Custom AI-powered Research
Need fresh innovation ideas? Read more about our custom AI-powered methods that generate + prioritize new product ideas for your innovation pipeline.
What is Product Positioning? (Examples and Strategies)
Launching a new product is a long and arduous process. Learn how to define and differentiate your product for maximum success with a product positioning strategy.
The Introduction Stage of Product Life Cycle | What to Know
The introduction stage in the product life cycle is meant to build product awareness. Click here to learn more about the introduction stage and how it works.
Product Life Cycle | What is it and What are the Stages?
The product life cycle outlines a product's journey from introduction to decline. Learn all about the product life cycle, its stages, and examples here.
The Growth Stage of Product Life Cycle | What to Know
All you need to know about the growth stage of the product life cycle and how you should make your product during this stage.
Root out friction in every digital experience, super-charge conversion rates, and optimize digital self-service
Uncover insights from any interaction, deliver AI-powered agent coaching, and reduce cost to serve
Increase revenue and loyalty with real-time insights and recommendations delivered to teams on the ground
Know how your people feel and empower managers to improve employee engagement, productivity, and retention
Take action in the moments that matter most along the employee journey and drive bottom line growth
Whatever they’re are saying, wherever they’re saying it, know exactly what’s going on with your people
Get faster, richer insights with qual and quant tools that make powerful market research available to everyone
Run concept tests, pricing studies, prototyping + more with fast, powerful studies designed by UX research experts
Track your brand performance 24/7 and act quickly to respond to opportunities and challenges in your market
Explore the platform powering Experience Management
- Free Account
- For Digital
- For Customer Care
- For Human Resources
- For Researchers
- Financial Services
- All Industries
Popular Use Cases
- Customer Experience
- Employee Experience
- Employee Exit Interviews
- Net Promoter Score
- Voice of Customer
- Customer Success Hub
- Product Documentation
- Training & Certification
- XM Institute
- Popular Resources
- Customer Stories
- Market Research
- Artificial Intelligence
- Partnerships
- Marketplace
The annual gathering of the experience leaders at the world’s iconic brands building breakthrough business results, live in Salt Lake City.
- English/AU & NZ
- Español/Europa
- Español/América Latina
- Português Brasileiro
- REQUEST DEMO
- Experience Management
What is a survey?
- Survey vs Questionnaire
Try Qualtrics for free
Survey vs questionnaire: the differences for market research.
12 min read If you’re looking to gain valuable insight into markets, businesses, customer perceptions and general feelings towards events or products at scale, look no further than surveys and questionnaires. In this article, we’ll cover the differences between the two terms and how to use them to best effect.
People and businesses use and complete surveys and questionnaires all the time.
Whether it’s to plan marketing campaigns or identify areas of opportunity, surveys and questionnaires enable us to gain valuable insight into markets, businesses, customer perceptions and sentiment and much more at scale.
But more often than not, people and businesses use the terms survey and questionnaire interchangeably — when in reality, they are two different disciplines.
Especially when it comes to market research .
Now, it’s easy to see why: both ask questions and aim to gather useful insights.
However, there are several differences between the two, and the method you decide to use is ultimately determined by the information you are trying to gather. So, what are the differences?
Find out about Qualtrics CoreXM and how it can transform your research
What is a questionnaire?
A questionnaire uses a set of questions to gain answers from a set of respondents. Questionnaires are often used to understand information like customer feedback and usually include a series of closed (yes/no) questions, with the occasional open-ended question to gain qualitative insights.
You can also use a questionnaire to gather information on specific issues like customer preferences and behaviors. You can conduct questionnaires over the phone, online or in-person.
A survey can be a more in-depth form of data collection to gather data from a specific target audience and survey respondents. It’s also more complex than a questionnaire.
Researchers can use the data gathered from a survey for statistical analysis to evaluate the data and responses, and generate conclusions from the responses to the survey questions.
Now, while you can also use a questionnaire in a research project to gather information — and it still collects high-quality data — surveys have several layers to consider, including:
- The design of the survey
- What type of sampling to use to create an effective survey
- The method of data collection
- Aggregation of data
- How and what method you’ll apply to the data analysis to understand the results glean actionable insights.
Ultimately, while a questionnaire is a list of questions to gather certain information, a survey can help researchers understand the bigger picture of a topic or issue to drive business action. That is the key difference.
Survey vs questionnaire examples
Now that you understand the basics, how can we differentiate the two further? Here are few examples to give you a better understanding of what we mean:
A questionnaire is a logical list of questions that a business uses to gather specific information from many respondents (not necessarily a certain group) — we know that — but how might you use it in a business context?
For example, you might use a questionnaire to gather customer information when they need to make a payment (name, bank details etc), or you might use one to accept donations. If you’re building a brand-new marketing campaign, you might send out a questionnaire to capture new prospect names and then send out a survey — once they become customers — to determine their level of satisfaction or loyalty.
Here’s another example — doctors and nurses use questionnaires when gathering medical histories of patients. They would then use a survey to understand patient satisfaction and to determine how those patients feel about the standard of care.
To summarize: you use questionnaires to capture specific information about an individual.
So what if you wanted to use questionnaires for your recruitment process? Well, a typical recruitment questionnaire follows a logical step-by-step approach to get a ‘feel’ for a candidate — their aims and objectives, experience, what they can bring to the job and so on. It’s very much like an interview, just far less personal.
But if you wanted to understand the thoughts and feelings about your interview process as a whole, you could design a survey for candidates (and successful hires) to understand trends, including collecting personal accounts, which your hiring team can use in the future.
You could also create surveys for your own hiring team to fill out and use their responses to uncover any gaps in your process.
Remember: the main difference between a questionnaire and a survey is that a questionnaire is often used to get information from an individual, while a survey is a method of data collection targeted at a specific group.
What data can you collect using surveys?
Researchers use surveys to gather both qualitative and quantitative data. Note — qualitative surveys use open-ended questions or video response options to produce long-form written or typed responses.
The main data types you’ll gather from surveys are:
Qualitative data
Qualitative data is most typically gathered in the form of longer, more descriptive answers and responses from your target audience. It looks at the “Why” behind the “What”.
Qualitative data is gathered when you need information that’s difficult to count or measured statistically, or when you need specific insights.
The data is usually gathered through the use of open-ended questions, but there are plenty of other qualitative methods beyond open-ends in surveys, such as:
Ethnographic research
Ethnography is the study of direct observation of users in their natural environment. The objective of this type of research is to gain insights into how users normally interact. Methods include direct observation, diary studies, video recordings and much more.
Moderated focus groups
Focus groups allow researchers to generate guided discussion around topics of choice. The moderator starts the discussion, but allows respondents (or participants) to construct the conversation, providing real-time insight. The moderator’s role is to ensure the conversation stays on track and is relevant.
Moderated discussions
Similar to focus groups, discussion boards are useful for collecting dynamic data. Much like an online forum, researchers can prompt a topic for discussion. It’s a much more interactive way to generate qualitative data, and allows researchers to flex their level of input.
Video responses
Another great way to acquire good qualitative research is through video feedback. As our lives become increasingly digital and more brands and businesses move online, researchers need a way to capture qualitative feedback and at scale. Through video responses provided in surveys, researchers get more authentic, natural and insightful feedback from respondents.
Quantitative data
Quantitative data is more statistical data when research is based on numerical data. It’s more concerned with the “What”.
This type of data is used to understand “hard” facts. For example, it’s often used to assess a content strategy when you want to understand or find trends in consumer behavior, or how they’re interacting with your content.
Within the two camps of quantitative and qualitative data, there are several other types of research data that can be collected:
Nominal data is information that’s classified into specific categories, but you can’t order it or measure it in any meaningful way for data analysis.
For example, data that highlights someone’s favorite item of clothing — with the categories being t-shirts, jeans, shorts, etc.
Ordinal data is detailed data in which data values follow a natural order. It’s commonly used in surveys and questionnaires to uncover preferences or agreement levels towards certain statements.
The likert scale is an example of ordinal data in which a survey may ask a respondent to choose between “Strongly Agree”, “Agree” etc. You can use ordinal data with advanced analysis tools like hypothesis testing.
Discrete data is data that can only take particular values, but doesn’t necessarily use whole numbers. A prime example of discrete data is the amount of profit a business makes in a given month.
Continuous data is a type of numerical data that refers to the unspecified number of possible measurements (or points) between two realistic variables. Continuous data, as such, is often referred to as ‘infinite’ data. Generally, continuous data is measured using a scale. Typical examples of continuous data are temperature, distance or weight. Due to the infinite nature of continuous data, it can change over time.
Research methods for surveys and questionnaires
As well as having different data types, surveys and questionnaires also use several research methods to gather information — these include:
Qualitative research
Qualitative research is the process of obtaining non-numerical data for use in research and decision making.
Qualitative research usually involves in-depth questioning of respondents to gather detailed survey data. For example, a researcher might carry out interviews, focus groups and/or one-to-one discussions to capture data.
Quantitative research
Quantitative research is about gathering statistical (numerical) data that researchers can use to uncover trends that guide decision making and future planning. For example, customer satisfaction surveys (CSATs) or Net Promoter Score (NPS) surveys are both examples of quantitative research.
Descriptive research
Descriptive research helps researchers to understand the characteristics of a population, situation, or phenomenon that they are studying. It’s more concerned with questions that answer the who, what, where and when, rather than the why.
Analytical research
Analytical research helps researchers to understand why certain things happen and the order in which they happened. They can then apply critical thinking to get a grip on the situation. This type of research can be extremely useful for research used to lead strategic decision making.
Applied research
Applied research is a type of examination that focuses on finding practical solutions to solve real-life problems. For example, challenges in the workplace or improving employee productivity.
Exploratory research
Exploratory research uses a range of quantitative and qualitative methods to look into a topic that hasn’t already been widely investigated.
Questionnaire survey method
Ultimately, while surveys and questionnaires are often used interchangeably, for anyone looking for in-depth insights to guide decisions or improve processes, surveys are far superior.
The fact is, surveys offer far more opportunities to collect and analyze data at scale to uncover critical, business-changing insights. And with the right tools, you can create high-quality surveys that attract and engage target audiences, ensuring you get the responses you need to make changes and improve experiences.
And Qualtrics CoreXM is exactly what you need to do so.
With Qualtrics CoreXM, you can empower everyone in your organization to carry out research — at scale — and get insights faster than ever before.
Featuring a suite of best-in-class analytics tools and an intuitive drag-and-drop interface, carry out end-to-end market research projects and generate the insights you need to drive meaningful change.
And with machine learning and AI-powered analysis, you can turn everyday data into a goldmine and use our built-in tool for assessing survey methodology to ensure you get high-quality responses.
Related resources
Response bias 13 min read, double barreled question 11 min read, likert scales 14 min read, survey research 15 min read, survey bias types 24 min read, post event survey questions 10 min read, best survey software 16 min read, request demo.
Ready to learn more about Qualtrics?
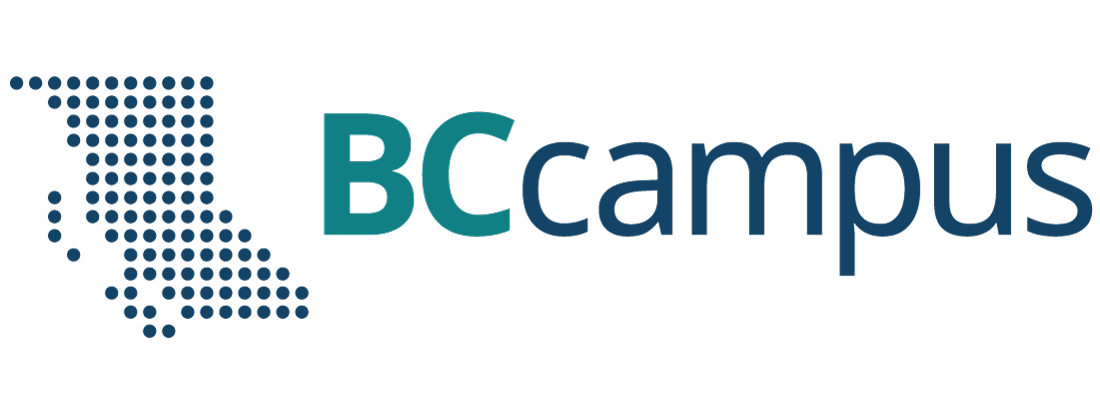
Want to create or adapt books like this? Learn more about how Pressbooks supports open publishing practices.
Chapter 8: Data Collection Methods: Survey Research
8.2 Understanding the Difference between a Survey and a Questionnaire
Before we move on to look at the strengths and weaknesses of survey research, we will take a step back to make sure you understand the difference between the concepts of surveys and questionnaires. Both surveys and questionnaires use a series of questions to gather information, however the purpose of the research and the treatment of the data after it is collected distinguish a questionnaire from a survey, e.g.:
- A questionnaire is a set of written questions used for collecting information for the benefit of one single individual.
- A survey is a process of gathering information for statistical analysis to the benefit of a group of individuals (a research method).
- A questionnaire does not aggregate data for statistical analysis after the data is collected, whereas survey responses are aggregated to draw conclusions.
A questionnaire is the set of questions that are used to gather the information, whereas a survey is a process of collecting and analyzing data. If the collected data will not be aggregated and is solely for the benefit of the respondent, then that is a questionnaire. If the data being collected with be aggregated and used for analytical purposes that is a survey (McKay, 2015). Sometimes questionnaire data is aggregated; it then becomes a survey, sometimes without the participant’s knowledge. For example, the bank where you filled in a loan application aggregates the data from all loan applications in the year 2017 and presents the information to shareholders in aggregated form at its 2018 annual general meeting. The bank has taken questionnaire data and aggregated it into survey data.
Understanding the difference between a survey and a questionnaire.
Adapted from Surbhi, S. (2016). Difference between survey and questionnaire. Retrieved from https://keydifferences.com/difference-between-survey-and-questionnaire.html
Research Methods for the Social Sciences: An Introduction Copyright © 2020 by Valerie Sheppard is licensed under a Creative Commons Attribution-NonCommercial-ShareAlike 4.0 International License , except where otherwise noted.
Share This Book
Frequently asked questions
What’s the difference between questionnaires and surveys.
A questionnaire is a data collection tool or instrument, while a survey is an overarching research method that involves collecting and analyzing data from people using questionnaires.
Frequently asked questions: Methodology
Attrition refers to participants leaving a study. It always happens to some extent—for example, in randomized controlled trials for medical research.
Differential attrition occurs when attrition or dropout rates differ systematically between the intervention and the control group . As a result, the characteristics of the participants who drop out differ from the characteristics of those who stay in the study. Because of this, study results may be biased .
Action research is conducted in order to solve a particular issue immediately, while case studies are often conducted over a longer period of time and focus more on observing and analyzing a particular ongoing phenomenon.
Action research is focused on solving a problem or informing individual and community-based knowledge in a way that impacts teaching, learning, and other related processes. It is less focused on contributing theoretical input, instead producing actionable input.
Action research is particularly popular with educators as a form of systematic inquiry because it prioritizes reflection and bridges the gap between theory and practice. Educators are able to simultaneously investigate an issue as they solve it, and the method is very iterative and flexible.
A cycle of inquiry is another name for action research . It is usually visualized in a spiral shape following a series of steps, such as “planning → acting → observing → reflecting.”
To make quantitative observations , you need to use instruments that are capable of measuring the quantity you want to observe. For example, you might use a ruler to measure the length of an object or a thermometer to measure its temperature.
Criterion validity and construct validity are both types of measurement validity . In other words, they both show you how accurately a method measures something.
While construct validity is the degree to which a test or other measurement method measures what it claims to measure, criterion validity is the degree to which a test can predictively (in the future) or concurrently (in the present) measure something.
Construct validity is often considered the overarching type of measurement validity . You need to have face validity , content validity , and criterion validity in order to achieve construct validity.
Convergent validity and discriminant validity are both subtypes of construct validity . Together, they help you evaluate whether a test measures the concept it was designed to measure.
- Convergent validity indicates whether a test that is designed to measure a particular construct correlates with other tests that assess the same or similar construct.
- Discriminant validity indicates whether two tests that should not be highly related to each other are indeed not related. This type of validity is also called divergent validity .
You need to assess both in order to demonstrate construct validity. Neither one alone is sufficient for establishing construct validity.
- Discriminant validity indicates whether two tests that should not be highly related to each other are indeed not related
Content validity shows you how accurately a test or other measurement method taps into the various aspects of the specific construct you are researching.
In other words, it helps you answer the question: “does the test measure all aspects of the construct I want to measure?” If it does, then the test has high content validity.
The higher the content validity, the more accurate the measurement of the construct.
If the test fails to include parts of the construct, or irrelevant parts are included, the validity of the instrument is threatened, which brings your results into question.
Face validity and content validity are similar in that they both evaluate how suitable the content of a test is. The difference is that face validity is subjective, and assesses content at surface level.
When a test has strong face validity, anyone would agree that the test’s questions appear to measure what they are intended to measure.
For example, looking at a 4th grade math test consisting of problems in which students have to add and multiply, most people would agree that it has strong face validity (i.e., it looks like a math test).
On the other hand, content validity evaluates how well a test represents all the aspects of a topic. Assessing content validity is more systematic and relies on expert evaluation. of each question, analyzing whether each one covers the aspects that the test was designed to cover.
A 4th grade math test would have high content validity if it covered all the skills taught in that grade. Experts(in this case, math teachers), would have to evaluate the content validity by comparing the test to the learning objectives.
Snowball sampling is a non-probability sampling method . Unlike probability sampling (which involves some form of random selection ), the initial individuals selected to be studied are the ones who recruit new participants.
Because not every member of the target population has an equal chance of being recruited into the sample, selection in snowball sampling is non-random.
Snowball sampling is a non-probability sampling method , where there is not an equal chance for every member of the population to be included in the sample .
This means that you cannot use inferential statistics and make generalizations —often the goal of quantitative research . As such, a snowball sample is not representative of the target population and is usually a better fit for qualitative research .
Snowball sampling relies on the use of referrals. Here, the researcher recruits one or more initial participants, who then recruit the next ones.
Participants share similar characteristics and/or know each other. Because of this, not every member of the population has an equal chance of being included in the sample, giving rise to sampling bias .
Snowball sampling is best used in the following cases:
- If there is no sampling frame available (e.g., people with a rare disease)
- If the population of interest is hard to access or locate (e.g., people experiencing homelessness)
- If the research focuses on a sensitive topic (e.g., extramarital affairs)
The reproducibility and replicability of a study can be ensured by writing a transparent, detailed method section and using clear, unambiguous language.
Reproducibility and replicability are related terms.
- Reproducing research entails reanalyzing the existing data in the same manner.
- Replicating (or repeating ) the research entails reconducting the entire analysis, including the collection of new data .
- A successful reproduction shows that the data analyses were conducted in a fair and honest manner.
- A successful replication shows that the reliability of the results is high.
Stratified sampling and quota sampling both involve dividing the population into subgroups and selecting units from each subgroup. The purpose in both cases is to select a representative sample and/or to allow comparisons between subgroups.
The main difference is that in stratified sampling, you draw a random sample from each subgroup ( probability sampling ). In quota sampling you select a predetermined number or proportion of units, in a non-random manner ( non-probability sampling ).
Purposive and convenience sampling are both sampling methods that are typically used in qualitative data collection.
A convenience sample is drawn from a source that is conveniently accessible to the researcher. Convenience sampling does not distinguish characteristics among the participants. On the other hand, purposive sampling focuses on selecting participants possessing characteristics associated with the research study.
The findings of studies based on either convenience or purposive sampling can only be generalized to the (sub)population from which the sample is drawn, and not to the entire population.
Random sampling or probability sampling is based on random selection. This means that each unit has an equal chance (i.e., equal probability) of being included in the sample.
On the other hand, convenience sampling involves stopping people at random, which means that not everyone has an equal chance of being selected depending on the place, time, or day you are collecting your data.
Convenience sampling and quota sampling are both non-probability sampling methods. They both use non-random criteria like availability, geographical proximity, or expert knowledge to recruit study participants.
However, in convenience sampling, you continue to sample units or cases until you reach the required sample size.
In quota sampling, you first need to divide your population of interest into subgroups (strata) and estimate their proportions (quota) in the population. Then you can start your data collection, using convenience sampling to recruit participants, until the proportions in each subgroup coincide with the estimated proportions in the population.
A sampling frame is a list of every member in the entire population . It is important that the sampling frame is as complete as possible, so that your sample accurately reflects your population.
Stratified and cluster sampling may look similar, but bear in mind that groups created in cluster sampling are heterogeneous , so the individual characteristics in the cluster vary. In contrast, groups created in stratified sampling are homogeneous , as units share characteristics.
Relatedly, in cluster sampling you randomly select entire groups and include all units of each group in your sample. However, in stratified sampling, you select some units of all groups and include them in your sample. In this way, both methods can ensure that your sample is representative of the target population .
A systematic review is secondary research because it uses existing research. You don’t collect new data yourself.
The key difference between observational studies and experimental designs is that a well-done observational study does not influence the responses of participants, while experiments do have some sort of treatment condition applied to at least some participants by random assignment .
An observational study is a great choice for you if your research question is based purely on observations. If there are ethical, logistical, or practical concerns that prevent you from conducting a traditional experiment , an observational study may be a good choice. In an observational study, there is no interference or manipulation of the research subjects, as well as no control or treatment groups .
It’s often best to ask a variety of people to review your measurements. You can ask experts, such as other researchers, or laypeople, such as potential participants, to judge the face validity of tests.
While experts have a deep understanding of research methods , the people you’re studying can provide you with valuable insights you may have missed otherwise.
Face validity is important because it’s a simple first step to measuring the overall validity of a test or technique. It’s a relatively intuitive, quick, and easy way to start checking whether a new measure seems useful at first glance.
Good face validity means that anyone who reviews your measure says that it seems to be measuring what it’s supposed to. With poor face validity, someone reviewing your measure may be left confused about what you’re measuring and why you’re using this method.
Face validity is about whether a test appears to measure what it’s supposed to measure. This type of validity is concerned with whether a measure seems relevant and appropriate for what it’s assessing only on the surface.
Statistical analyses are often applied to test validity with data from your measures. You test convergent validity and discriminant validity with correlations to see if results from your test are positively or negatively related to those of other established tests.
You can also use regression analyses to assess whether your measure is actually predictive of outcomes that you expect it to predict theoretically. A regression analysis that supports your expectations strengthens your claim of construct validity .
When designing or evaluating a measure, construct validity helps you ensure you’re actually measuring the construct you’re interested in. If you don’t have construct validity, you may inadvertently measure unrelated or distinct constructs and lose precision in your research.
Construct validity is often considered the overarching type of measurement validity , because it covers all of the other types. You need to have face validity , content validity , and criterion validity to achieve construct validity.
Construct validity is about how well a test measures the concept it was designed to evaluate. It’s one of four types of measurement validity , which includes construct validity, face validity , and criterion validity.
There are two subtypes of construct validity.
- Convergent validity : The extent to which your measure corresponds to measures of related constructs
- Discriminant validity : The extent to which your measure is unrelated or negatively related to measures of distinct constructs
Naturalistic observation is a valuable tool because of its flexibility, external validity , and suitability for topics that can’t be studied in a lab setting.
The downsides of naturalistic observation include its lack of scientific control , ethical considerations , and potential for bias from observers and subjects.
Naturalistic observation is a qualitative research method where you record the behaviors of your research subjects in real world settings. You avoid interfering or influencing anything in a naturalistic observation.
You can think of naturalistic observation as “people watching” with a purpose.
A dependent variable is what changes as a result of the independent variable manipulation in experiments . It’s what you’re interested in measuring, and it “depends” on your independent variable.
In statistics, dependent variables are also called:
- Response variables (they respond to a change in another variable)
- Outcome variables (they represent the outcome you want to measure)
- Left-hand-side variables (they appear on the left-hand side of a regression equation)
An independent variable is the variable you manipulate, control, or vary in an experimental study to explore its effects. It’s called “independent” because it’s not influenced by any other variables in the study.
Independent variables are also called:
- Explanatory variables (they explain an event or outcome)
- Predictor variables (they can be used to predict the value of a dependent variable)
- Right-hand-side variables (they appear on the right-hand side of a regression equation).
As a rule of thumb, questions related to thoughts, beliefs, and feelings work well in focus groups. Take your time formulating strong questions, paying special attention to phrasing. Be careful to avoid leading questions , which can bias your responses.
Overall, your focus group questions should be:
- Open-ended and flexible
- Impossible to answer with “yes” or “no” (questions that start with “why” or “how” are often best)
- Unambiguous, getting straight to the point while still stimulating discussion
- Unbiased and neutral
A structured interview is a data collection method that relies on asking questions in a set order to collect data on a topic. They are often quantitative in nature. Structured interviews are best used when:
- You already have a very clear understanding of your topic. Perhaps significant research has already been conducted, or you have done some prior research yourself, but you already possess a baseline for designing strong structured questions.
- You are constrained in terms of time or resources and need to analyze your data quickly and efficiently.
- Your research question depends on strong parity between participants, with environmental conditions held constant.
More flexible interview options include semi-structured interviews , unstructured interviews , and focus groups .
Social desirability bias is the tendency for interview participants to give responses that will be viewed favorably by the interviewer or other participants. It occurs in all types of interviews and surveys , but is most common in semi-structured interviews , unstructured interviews , and focus groups .
Social desirability bias can be mitigated by ensuring participants feel at ease and comfortable sharing their views. Make sure to pay attention to your own body language and any physical or verbal cues, such as nodding or widening your eyes.
This type of bias can also occur in observations if the participants know they’re being observed. They might alter their behavior accordingly.
The interviewer effect is a type of bias that emerges when a characteristic of an interviewer (race, age, gender identity, etc.) influences the responses given by the interviewee.
There is a risk of an interviewer effect in all types of interviews , but it can be mitigated by writing really high-quality interview questions.
A semi-structured interview is a blend of structured and unstructured types of interviews. Semi-structured interviews are best used when:
- You have prior interview experience. Spontaneous questions are deceptively challenging, and it’s easy to accidentally ask a leading question or make a participant uncomfortable.
- Your research question is exploratory in nature. Participant answers can guide future research questions and help you develop a more robust knowledge base for future research.
An unstructured interview is the most flexible type of interview, but it is not always the best fit for your research topic.
Unstructured interviews are best used when:
- You are an experienced interviewer and have a very strong background in your research topic, since it is challenging to ask spontaneous, colloquial questions.
- Your research question is exploratory in nature. While you may have developed hypotheses, you are open to discovering new or shifting viewpoints through the interview process.
- You are seeking descriptive data, and are ready to ask questions that will deepen and contextualize your initial thoughts and hypotheses.
- Your research depends on forming connections with your participants and making them feel comfortable revealing deeper emotions, lived experiences, or thoughts.
The four most common types of interviews are:
- Structured interviews : The questions are predetermined in both topic and order.
- Semi-structured interviews : A few questions are predetermined, but other questions aren’t planned.
- Unstructured interviews : None of the questions are predetermined.
- Focus group interviews : The questions are presented to a group instead of one individual.
Deductive reasoning is commonly used in scientific research, and it’s especially associated with quantitative research .
In research, you might have come across something called the hypothetico-deductive method . It’s the scientific method of testing hypotheses to check whether your predictions are substantiated by real-world data.
Deductive reasoning is a logical approach where you progress from general ideas to specific conclusions. It’s often contrasted with inductive reasoning , where you start with specific observations and form general conclusions.
Deductive reasoning is also called deductive logic.
There are many different types of inductive reasoning that people use formally or informally.
Here are a few common types:
- Inductive generalization : You use observations about a sample to come to a conclusion about the population it came from.
- Statistical generalization: You use specific numbers about samples to make statements about populations.
- Causal reasoning: You make cause-and-effect links between different things.
- Sign reasoning: You make a conclusion about a correlational relationship between different things.
- Analogical reasoning: You make a conclusion about something based on its similarities to something else.
Inductive reasoning is a bottom-up approach, while deductive reasoning is top-down.
Inductive reasoning takes you from the specific to the general, while in deductive reasoning, you make inferences by going from general premises to specific conclusions.
In inductive research , you start by making observations or gathering data. Then, you take a broad scan of your data and search for patterns. Finally, you make general conclusions that you might incorporate into theories.
Inductive reasoning is a method of drawing conclusions by going from the specific to the general. It’s usually contrasted with deductive reasoning, where you proceed from general information to specific conclusions.
Inductive reasoning is also called inductive logic or bottom-up reasoning.
A hypothesis states your predictions about what your research will find. It is a tentative answer to your research question that has not yet been tested. For some research projects, you might have to write several hypotheses that address different aspects of your research question.
A hypothesis is not just a guess — it should be based on existing theories and knowledge. It also has to be testable, which means you can support or refute it through scientific research methods (such as experiments, observations and statistical analysis of data).
Triangulation can help:
- Reduce research bias that comes from using a single method, theory, or investigator
- Enhance validity by approaching the same topic with different tools
- Establish credibility by giving you a complete picture of the research problem
But triangulation can also pose problems:
- It’s time-consuming and labor-intensive, often involving an interdisciplinary team.
- Your results may be inconsistent or even contradictory.
There are four main types of triangulation :
- Data triangulation : Using data from different times, spaces, and people
- Investigator triangulation : Involving multiple researchers in collecting or analyzing data
- Theory triangulation : Using varying theoretical perspectives in your research
- Methodological triangulation : Using different methodologies to approach the same topic
Many academic fields use peer review , largely to determine whether a manuscript is suitable for publication. Peer review enhances the credibility of the published manuscript.
However, peer review is also common in non-academic settings. The United Nations, the European Union, and many individual nations use peer review to evaluate grant applications. It is also widely used in medical and health-related fields as a teaching or quality-of-care measure.
Peer assessment is often used in the classroom as a pedagogical tool. Both receiving feedback and providing it are thought to enhance the learning process, helping students think critically and collaboratively.
Peer review can stop obviously problematic, falsified, or otherwise untrustworthy research from being published. It also represents an excellent opportunity to get feedback from renowned experts in your field. It acts as a first defense, helping you ensure your argument is clear and that there are no gaps, vague terms, or unanswered questions for readers who weren’t involved in the research process.
Peer-reviewed articles are considered a highly credible source due to this stringent process they go through before publication.
In general, the peer review process follows the following steps:
- First, the author submits the manuscript to the editor.
- Reject the manuscript and send it back to author, or
- Send it onward to the selected peer reviewer(s)
- Next, the peer review process occurs. The reviewer provides feedback, addressing any major or minor issues with the manuscript, and gives their advice regarding what edits should be made.
- Lastly, the edited manuscript is sent back to the author. They input the edits, and resubmit it to the editor for publication.
Exploratory research is often used when the issue you’re studying is new or when the data collection process is challenging for some reason.
You can use exploratory research if you have a general idea or a specific question that you want to study but there is no preexisting knowledge or paradigm with which to study it.
Exploratory research is a methodology approach that explores research questions that have not previously been studied in depth. It is often used when the issue you’re studying is new, or the data collection process is challenging in some way.
Explanatory research is used to investigate how or why a phenomenon occurs. Therefore, this type of research is often one of the first stages in the research process , serving as a jumping-off point for future research.
Exploratory research aims to explore the main aspects of an under-researched problem, while explanatory research aims to explain the causes and consequences of a well-defined problem.
Explanatory research is a research method used to investigate how or why something occurs when only a small amount of information is available pertaining to that topic. It can help you increase your understanding of a given topic.
Clean data are valid, accurate, complete, consistent, unique, and uniform. Dirty data include inconsistencies and errors.
Dirty data can come from any part of the research process, including poor research design , inappropriate measurement materials, or flawed data entry.
Data cleaning takes place between data collection and data analyses. But you can use some methods even before collecting data.
For clean data, you should start by designing measures that collect valid data. Data validation at the time of data entry or collection helps you minimize the amount of data cleaning you’ll need to do.
After data collection, you can use data standardization and data transformation to clean your data. You’ll also deal with any missing values, outliers, and duplicate values.
Every dataset requires different techniques to clean dirty data , but you need to address these issues in a systematic way. You focus on finding and resolving data points that don’t agree or fit with the rest of your dataset.
These data might be missing values, outliers, duplicate values, incorrectly formatted, or irrelevant. You’ll start with screening and diagnosing your data. Then, you’ll often standardize and accept or remove data to make your dataset consistent and valid.
Data cleaning is necessary for valid and appropriate analyses. Dirty data contain inconsistencies or errors , but cleaning your data helps you minimize or resolve these.
Without data cleaning, you could end up with a Type I or II error in your conclusion. These types of erroneous conclusions can be practically significant with important consequences, because they lead to misplaced investments or missed opportunities.
Data cleaning involves spotting and resolving potential data inconsistencies or errors to improve your data quality. An error is any value (e.g., recorded weight) that doesn’t reflect the true value (e.g., actual weight) of something that’s being measured.
In this process, you review, analyze, detect, modify, or remove “dirty” data to make your dataset “clean.” Data cleaning is also called data cleansing or data scrubbing.
Research misconduct means making up or falsifying data, manipulating data analyses, or misrepresenting results in research reports. It’s a form of academic fraud.
These actions are committed intentionally and can have serious consequences; research misconduct is not a simple mistake or a point of disagreement but a serious ethical failure.
Anonymity means you don’t know who the participants are, while confidentiality means you know who they are but remove identifying information from your research report. Both are important ethical considerations .
You can only guarantee anonymity by not collecting any personally identifying information—for example, names, phone numbers, email addresses, IP addresses, physical characteristics, photos, or videos.
You can keep data confidential by using aggregate information in your research report, so that you only refer to groups of participants rather than individuals.
Research ethics matter for scientific integrity, human rights and dignity, and collaboration between science and society. These principles make sure that participation in studies is voluntary, informed, and safe.
Ethical considerations in research are a set of principles that guide your research designs and practices. These principles include voluntary participation, informed consent, anonymity, confidentiality, potential for harm, and results communication.
Scientists and researchers must always adhere to a certain code of conduct when collecting data from others .
These considerations protect the rights of research participants, enhance research validity , and maintain scientific integrity.
In multistage sampling , you can use probability or non-probability sampling methods .
For a probability sample, you have to conduct probability sampling at every stage.
You can mix it up by using simple random sampling , systematic sampling , or stratified sampling to select units at different stages, depending on what is applicable and relevant to your study.
Multistage sampling can simplify data collection when you have large, geographically spread samples, and you can obtain a probability sample without a complete sampling frame.
But multistage sampling may not lead to a representative sample, and larger samples are needed for multistage samples to achieve the statistical properties of simple random samples .
These are four of the most common mixed methods designs :
- Convergent parallel: Quantitative and qualitative data are collected at the same time and analyzed separately. After both analyses are complete, compare your results to draw overall conclusions.
- Embedded: Quantitative and qualitative data are collected at the same time, but within a larger quantitative or qualitative design. One type of data is secondary to the other.
- Explanatory sequential: Quantitative data is collected and analyzed first, followed by qualitative data. You can use this design if you think your qualitative data will explain and contextualize your quantitative findings.
- Exploratory sequential: Qualitative data is collected and analyzed first, followed by quantitative data. You can use this design if you think the quantitative data will confirm or validate your qualitative findings.
Triangulation in research means using multiple datasets, methods, theories and/or investigators to address a research question. It’s a research strategy that can help you enhance the validity and credibility of your findings.
Triangulation is mainly used in qualitative research , but it’s also commonly applied in quantitative research . Mixed methods research always uses triangulation.
In multistage sampling , or multistage cluster sampling, you draw a sample from a population using smaller and smaller groups at each stage.
This method is often used to collect data from a large, geographically spread group of people in national surveys, for example. You take advantage of hierarchical groupings (e.g., from state to city to neighborhood) to create a sample that’s less expensive and time-consuming to collect data from.
No, the steepness or slope of the line isn’t related to the correlation coefficient value. The correlation coefficient only tells you how closely your data fit on a line, so two datasets with the same correlation coefficient can have very different slopes.
To find the slope of the line, you’ll need to perform a regression analysis .
Correlation coefficients always range between -1 and 1.
The sign of the coefficient tells you the direction of the relationship: a positive value means the variables change together in the same direction, while a negative value means they change together in opposite directions.
The absolute value of a number is equal to the number without its sign. The absolute value of a correlation coefficient tells you the magnitude of the correlation: the greater the absolute value, the stronger the correlation.
These are the assumptions your data must meet if you want to use Pearson’s r :
- Both variables are on an interval or ratio level of measurement
- Data from both variables follow normal distributions
- Your data have no outliers
- Your data is from a random or representative sample
- You expect a linear relationship between the two variables
Quantitative research designs can be divided into two main categories:
- Correlational and descriptive designs are used to investigate characteristics, averages, trends, and associations between variables.
- Experimental and quasi-experimental designs are used to test causal relationships .
Qualitative research designs tend to be more flexible. Common types of qualitative design include case study , ethnography , and grounded theory designs.
A well-planned research design helps ensure that your methods match your research aims, that you collect high-quality data, and that you use the right kind of analysis to answer your questions, utilizing credible sources . This allows you to draw valid , trustworthy conclusions.
The priorities of a research design can vary depending on the field, but you usually have to specify:
- Your research questions and/or hypotheses
- Your overall approach (e.g., qualitative or quantitative )
- The type of design you’re using (e.g., a survey , experiment , or case study )
- Your sampling methods or criteria for selecting subjects
- Your data collection methods (e.g., questionnaires , observations)
- Your data collection procedures (e.g., operationalization , timing and data management)
- Your data analysis methods (e.g., statistical tests or thematic analysis )
A research design is a strategy for answering your research question . It defines your overall approach and determines how you will collect and analyze data.
Questionnaires can be self-administered or researcher-administered.
Self-administered questionnaires can be delivered online or in paper-and-pen formats, in person or through mail. All questions are standardized so that all respondents receive the same questions with identical wording.
Researcher-administered questionnaires are interviews that take place by phone, in-person, or online between researchers and respondents. You can gain deeper insights by clarifying questions for respondents or asking follow-up questions.
You can organize the questions logically, with a clear progression from simple to complex, or randomly between respondents. A logical flow helps respondents process the questionnaire easier and quicker, but it may lead to bias. Randomization can minimize the bias from order effects.
Closed-ended, or restricted-choice, questions offer respondents a fixed set of choices to select from. These questions are easier to answer quickly.
Open-ended or long-form questions allow respondents to answer in their own words. Because there are no restrictions on their choices, respondents can answer in ways that researchers may not have otherwise considered.
The third variable and directionality problems are two main reasons why correlation isn’t causation .
The third variable problem means that a confounding variable affects both variables to make them seem causally related when they are not.
The directionality problem is when two variables correlate and might actually have a causal relationship, but it’s impossible to conclude which variable causes changes in the other.
Correlation describes an association between variables : when one variable changes, so does the other. A correlation is a statistical indicator of the relationship between variables.
Causation means that changes in one variable brings about changes in the other (i.e., there is a cause-and-effect relationship between variables). The two variables are correlated with each other, and there’s also a causal link between them.
While causation and correlation can exist simultaneously, correlation does not imply causation. In other words, correlation is simply a relationship where A relates to B—but A doesn’t necessarily cause B to happen (or vice versa). Mistaking correlation for causation is a common error and can lead to false cause fallacy .
Controlled experiments establish causality, whereas correlational studies only show associations between variables.
- In an experimental design , you manipulate an independent variable and measure its effect on a dependent variable. Other variables are controlled so they can’t impact the results.
- In a correlational design , you measure variables without manipulating any of them. You can test whether your variables change together, but you can’t be sure that one variable caused a change in another.
In general, correlational research is high in external validity while experimental research is high in internal validity .
A correlation is usually tested for two variables at a time, but you can test correlations between three or more variables.
A correlation coefficient is a single number that describes the strength and direction of the relationship between your variables.
Different types of correlation coefficients might be appropriate for your data based on their levels of measurement and distributions . The Pearson product-moment correlation coefficient (Pearson’s r ) is commonly used to assess a linear relationship between two quantitative variables.
A correlational research design investigates relationships between two variables (or more) without the researcher controlling or manipulating any of them. It’s a non-experimental type of quantitative research .
A correlation reflects the strength and/or direction of the association between two or more variables.
- A positive correlation means that both variables change in the same direction.
- A negative correlation means that the variables change in opposite directions.
- A zero correlation means there’s no relationship between the variables.
Random error is almost always present in scientific studies, even in highly controlled settings. While you can’t eradicate it completely, you can reduce random error by taking repeated measurements, using a large sample, and controlling extraneous variables .
You can avoid systematic error through careful design of your sampling , data collection , and analysis procedures. For example, use triangulation to measure your variables using multiple methods; regularly calibrate instruments or procedures; use random sampling and random assignment ; and apply masking (blinding) where possible.
Systematic error is generally a bigger problem in research.
With random error, multiple measurements will tend to cluster around the true value. When you’re collecting data from a large sample , the errors in different directions will cancel each other out.
Systematic errors are much more problematic because they can skew your data away from the true value. This can lead you to false conclusions ( Type I and II errors ) about the relationship between the variables you’re studying.
Random and systematic error are two types of measurement error.
Random error is a chance difference between the observed and true values of something (e.g., a researcher misreading a weighing scale records an incorrect measurement).
Systematic error is a consistent or proportional difference between the observed and true values of something (e.g., a miscalibrated scale consistently records weights as higher than they actually are).
On graphs, the explanatory variable is conventionally placed on the x-axis, while the response variable is placed on the y-axis.
- If you have quantitative variables , use a scatterplot or a line graph.
- If your response variable is categorical, use a scatterplot or a line graph.
- If your explanatory variable is categorical, use a bar graph.
The term “ explanatory variable ” is sometimes preferred over “ independent variable ” because, in real world contexts, independent variables are often influenced by other variables. This means they aren’t totally independent.
Multiple independent variables may also be correlated with each other, so “explanatory variables” is a more appropriate term.
The difference between explanatory and response variables is simple:
- An explanatory variable is the expected cause, and it explains the results.
- A response variable is the expected effect, and it responds to other variables.
In a controlled experiment , all extraneous variables are held constant so that they can’t influence the results. Controlled experiments require:
- A control group that receives a standard treatment, a fake treatment, or no treatment.
- Random assignment of participants to ensure the groups are equivalent.
Depending on your study topic, there are various other methods of controlling variables .
There are 4 main types of extraneous variables :
- Demand characteristics : environmental cues that encourage participants to conform to researchers’ expectations.
- Experimenter effects : unintentional actions by researchers that influence study outcomes.
- Situational variables : environmental variables that alter participants’ behaviors.
- Participant variables : any characteristic or aspect of a participant’s background that could affect study results.
An extraneous variable is any variable that you’re not investigating that can potentially affect the dependent variable of your research study.
A confounding variable is a type of extraneous variable that not only affects the dependent variable, but is also related to the independent variable.
In a factorial design, multiple independent variables are tested.
If you test two variables, each level of one independent variable is combined with each level of the other independent variable to create different conditions.
Within-subjects designs have many potential threats to internal validity , but they are also very statistically powerful .
Advantages:
- Only requires small samples
- Statistically powerful
- Removes the effects of individual differences on the outcomes
Disadvantages:
- Internal validity threats reduce the likelihood of establishing a direct relationship between variables
- Time-related effects, such as growth, can influence the outcomes
- Carryover effects mean that the specific order of different treatments affect the outcomes
While a between-subjects design has fewer threats to internal validity , it also requires more participants for high statistical power than a within-subjects design .
- Prevents carryover effects of learning and fatigue.
- Shorter study duration.
- Needs larger samples for high power.
- Uses more resources to recruit participants, administer sessions, cover costs, etc.
- Individual differences may be an alternative explanation for results.
Yes. Between-subjects and within-subjects designs can be combined in a single study when you have two or more independent variables (a factorial design). In a mixed factorial design, one variable is altered between subjects and another is altered within subjects.
In a between-subjects design , every participant experiences only one condition, and researchers assess group differences between participants in various conditions.
In a within-subjects design , each participant experiences all conditions, and researchers test the same participants repeatedly for differences between conditions.
The word “between” means that you’re comparing different conditions between groups, while the word “within” means you’re comparing different conditions within the same group.
Random assignment is used in experiments with a between-groups or independent measures design. In this research design, there’s usually a control group and one or more experimental groups. Random assignment helps ensure that the groups are comparable.
In general, you should always use random assignment in this type of experimental design when it is ethically possible and makes sense for your study topic.
To implement random assignment , assign a unique number to every member of your study’s sample .
Then, you can use a random number generator or a lottery method to randomly assign each number to a control or experimental group. You can also do so manually, by flipping a coin or rolling a dice to randomly assign participants to groups.
Random selection, or random sampling , is a way of selecting members of a population for your study’s sample.
In contrast, random assignment is a way of sorting the sample into control and experimental groups.
Random sampling enhances the external validity or generalizability of your results, while random assignment improves the internal validity of your study.
In experimental research, random assignment is a way of placing participants from your sample into different groups using randomization. With this method, every member of the sample has a known or equal chance of being placed in a control group or an experimental group.
“Controlling for a variable” means measuring extraneous variables and accounting for them statistically to remove their effects on other variables.
Researchers often model control variable data along with independent and dependent variable data in regression analyses and ANCOVAs . That way, you can isolate the control variable’s effects from the relationship between the variables of interest.
Control variables help you establish a correlational or causal relationship between variables by enhancing internal validity .
If you don’t control relevant extraneous variables , they may influence the outcomes of your study, and you may not be able to demonstrate that your results are really an effect of your independent variable .
A control variable is any variable that’s held constant in a research study. It’s not a variable of interest in the study, but it’s controlled because it could influence the outcomes.
Including mediators and moderators in your research helps you go beyond studying a simple relationship between two variables for a fuller picture of the real world. They are important to consider when studying complex correlational or causal relationships.
Mediators are part of the causal pathway of an effect, and they tell you how or why an effect takes place. Moderators usually help you judge the external validity of your study by identifying the limitations of when the relationship between variables holds.
If something is a mediating variable :
- It’s caused by the independent variable .
- It influences the dependent variable
- When it’s taken into account, the statistical correlation between the independent and dependent variables is higher than when it isn’t considered.
A confounder is a third variable that affects variables of interest and makes them seem related when they are not. In contrast, a mediator is the mechanism of a relationship between two variables: it explains the process by which they are related.
A mediator variable explains the process through which two variables are related, while a moderator variable affects the strength and direction of that relationship.
There are three key steps in systematic sampling :
- Define and list your population , ensuring that it is not ordered in a cyclical or periodic order.
- Decide on your sample size and calculate your interval, k , by dividing your population by your target sample size.
- Choose every k th member of the population as your sample.
Systematic sampling is a probability sampling method where researchers select members of the population at a regular interval – for example, by selecting every 15th person on a list of the population. If the population is in a random order, this can imitate the benefits of simple random sampling .
Yes, you can create a stratified sample using multiple characteristics, but you must ensure that every participant in your study belongs to one and only one subgroup. In this case, you multiply the numbers of subgroups for each characteristic to get the total number of groups.
For example, if you were stratifying by location with three subgroups (urban, rural, or suburban) and marital status with five subgroups (single, divorced, widowed, married, or partnered), you would have 3 x 5 = 15 subgroups.
You should use stratified sampling when your sample can be divided into mutually exclusive and exhaustive subgroups that you believe will take on different mean values for the variable that you’re studying.
Using stratified sampling will allow you to obtain more precise (with lower variance ) statistical estimates of whatever you are trying to measure.
For example, say you want to investigate how income differs based on educational attainment, but you know that this relationship can vary based on race. Using stratified sampling, you can ensure you obtain a large enough sample from each racial group, allowing you to draw more precise conclusions.
In stratified sampling , researchers divide subjects into subgroups called strata based on characteristics that they share (e.g., race, gender, educational attainment).
Once divided, each subgroup is randomly sampled using another probability sampling method.
Cluster sampling is more time- and cost-efficient than other probability sampling methods , particularly when it comes to large samples spread across a wide geographical area.
However, it provides less statistical certainty than other methods, such as simple random sampling , because it is difficult to ensure that your clusters properly represent the population as a whole.
There are three types of cluster sampling : single-stage, double-stage and multi-stage clustering. In all three types, you first divide the population into clusters, then randomly select clusters for use in your sample.
- In single-stage sampling , you collect data from every unit within the selected clusters.
- In double-stage sampling , you select a random sample of units from within the clusters.
- In multi-stage sampling , you repeat the procedure of randomly sampling elements from within the clusters until you have reached a manageable sample.
Cluster sampling is a probability sampling method in which you divide a population into clusters, such as districts or schools, and then randomly select some of these clusters as your sample.
The clusters should ideally each be mini-representations of the population as a whole.
If properly implemented, simple random sampling is usually the best sampling method for ensuring both internal and external validity . However, it can sometimes be impractical and expensive to implement, depending on the size of the population to be studied,
If you have a list of every member of the population and the ability to reach whichever members are selected, you can use simple random sampling.
The American Community Survey is an example of simple random sampling . In order to collect detailed data on the population of the US, the Census Bureau officials randomly select 3.5 million households per year and use a variety of methods to convince them to fill out the survey.
Simple random sampling is a type of probability sampling in which the researcher randomly selects a subset of participants from a population . Each member of the population has an equal chance of being selected. Data is then collected from as large a percentage as possible of this random subset.
Quasi-experimental design is most useful in situations where it would be unethical or impractical to run a true experiment .
Quasi-experiments have lower internal validity than true experiments, but they often have higher external validity as they can use real-world interventions instead of artificial laboratory settings.
A quasi-experiment is a type of research design that attempts to establish a cause-and-effect relationship. The main difference with a true experiment is that the groups are not randomly assigned.
Blinding is important to reduce research bias (e.g., observer bias , demand characteristics ) and ensure a study’s internal validity .
If participants know whether they are in a control or treatment group , they may adjust their behavior in ways that affect the outcome that researchers are trying to measure. If the people administering the treatment are aware of group assignment, they may treat participants differently and thus directly or indirectly influence the final results.
- In a single-blind study , only the participants are blinded.
- In a double-blind study , both participants and experimenters are blinded.
- In a triple-blind study , the assignment is hidden not only from participants and experimenters, but also from the researchers analyzing the data.
Blinding means hiding who is assigned to the treatment group and who is assigned to the control group in an experiment .
A true experiment (a.k.a. a controlled experiment) always includes at least one control group that doesn’t receive the experimental treatment.
However, some experiments use a within-subjects design to test treatments without a control group. In these designs, you usually compare one group’s outcomes before and after a treatment (instead of comparing outcomes between different groups).
For strong internal validity , it’s usually best to include a control group if possible. Without a control group, it’s harder to be certain that the outcome was caused by the experimental treatment and not by other variables.
An experimental group, also known as a treatment group, receives the treatment whose effect researchers wish to study, whereas a control group does not. They should be identical in all other ways.
Individual Likert-type questions are generally considered ordinal data , because the items have clear rank order, but don’t have an even distribution.
Overall Likert scale scores are sometimes treated as interval data. These scores are considered to have directionality and even spacing between them.
The type of data determines what statistical tests you should use to analyze your data.
A Likert scale is a rating scale that quantitatively assesses opinions, attitudes, or behaviors. It is made up of 4 or more questions that measure a single attitude or trait when response scores are combined.
To use a Likert scale in a survey , you present participants with Likert-type questions or statements, and a continuum of items, usually with 5 or 7 possible responses, to capture their degree of agreement.
In scientific research, concepts are the abstract ideas or phenomena that are being studied (e.g., educational achievement). Variables are properties or characteristics of the concept (e.g., performance at school), while indicators are ways of measuring or quantifying variables (e.g., yearly grade reports).
The process of turning abstract concepts into measurable variables and indicators is called operationalization .
There are various approaches to qualitative data analysis , but they all share five steps in common:
- Prepare and organize your data.
- Review and explore your data.
- Develop a data coding system.
- Assign codes to the data.
- Identify recurring themes.
The specifics of each step depend on the focus of the analysis. Some common approaches include textual analysis , thematic analysis , and discourse analysis .
There are five common approaches to qualitative research :
- Grounded theory involves collecting data in order to develop new theories.
- Ethnography involves immersing yourself in a group or organization to understand its culture.
- Narrative research involves interpreting stories to understand how people make sense of their experiences and perceptions.
- Phenomenological research involves investigating phenomena through people’s lived experiences.
- Action research links theory and practice in several cycles to drive innovative changes.
Hypothesis testing is a formal procedure for investigating our ideas about the world using statistics. It is used by scientists to test specific predictions, called hypotheses , by calculating how likely it is that a pattern or relationship between variables could have arisen by chance.
Operationalization means turning abstract conceptual ideas into measurable observations.
For example, the concept of social anxiety isn’t directly observable, but it can be operationally defined in terms of self-rating scores, behavioral avoidance of crowded places, or physical anxiety symptoms in social situations.
Before collecting data , it’s important to consider how you will operationalize the variables that you want to measure.
When conducting research, collecting original data has significant advantages:
- You can tailor data collection to your specific research aims (e.g. understanding the needs of your consumers or user testing your website)
- You can control and standardize the process for high reliability and validity (e.g. choosing appropriate measurements and sampling methods )
However, there are also some drawbacks: data collection can be time-consuming, labor-intensive and expensive. In some cases, it’s more efficient to use secondary data that has already been collected by someone else, but the data might be less reliable.
Data collection is the systematic process by which observations or measurements are gathered in research. It is used in many different contexts by academics, governments, businesses, and other organizations.
There are several methods you can use to decrease the impact of confounding variables on your research: restriction, matching, statistical control and randomization.
In restriction , you restrict your sample by only including certain subjects that have the same values of potential confounding variables.
In matching , you match each of the subjects in your treatment group with a counterpart in the comparison group. The matched subjects have the same values on any potential confounding variables, and only differ in the independent variable .
In statistical control , you include potential confounders as variables in your regression .
In randomization , you randomly assign the treatment (or independent variable) in your study to a sufficiently large number of subjects, which allows you to control for all potential confounding variables.
A confounding variable is closely related to both the independent and dependent variables in a study. An independent variable represents the supposed cause , while the dependent variable is the supposed effect . A confounding variable is a third variable that influences both the independent and dependent variables.
Failing to account for confounding variables can cause you to wrongly estimate the relationship between your independent and dependent variables.
To ensure the internal validity of your research, you must consider the impact of confounding variables. If you fail to account for them, you might over- or underestimate the causal relationship between your independent and dependent variables , or even find a causal relationship where none exists.
Yes, but including more than one of either type requires multiple research questions .
For example, if you are interested in the effect of a diet on health, you can use multiple measures of health: blood sugar, blood pressure, weight, pulse, and many more. Each of these is its own dependent variable with its own research question.
You could also choose to look at the effect of exercise levels as well as diet, or even the additional effect of the two combined. Each of these is a separate independent variable .
To ensure the internal validity of an experiment , you should only change one independent variable at a time.
No. The value of a dependent variable depends on an independent variable, so a variable cannot be both independent and dependent at the same time. It must be either the cause or the effect, not both!
You want to find out how blood sugar levels are affected by drinking diet soda and regular soda, so you conduct an experiment .
- The type of soda – diet or regular – is the independent variable .
- The level of blood sugar that you measure is the dependent variable – it changes depending on the type of soda.
Determining cause and effect is one of the most important parts of scientific research. It’s essential to know which is the cause – the independent variable – and which is the effect – the dependent variable.
In non-probability sampling , the sample is selected based on non-random criteria, and not every member of the population has a chance of being included.
Common non-probability sampling methods include convenience sampling , voluntary response sampling, purposive sampling , snowball sampling, and quota sampling .
Probability sampling means that every member of the target population has a known chance of being included in the sample.
Probability sampling methods include simple random sampling , systematic sampling , stratified sampling , and cluster sampling .
Using careful research design and sampling procedures can help you avoid sampling bias . Oversampling can be used to correct undercoverage bias .
Some common types of sampling bias include self-selection bias , nonresponse bias , undercoverage bias , survivorship bias , pre-screening or advertising bias, and healthy user bias.
Sampling bias is a threat to external validity – it limits the generalizability of your findings to a broader group of people.
A sampling error is the difference between a population parameter and a sample statistic .
A statistic refers to measures about the sample , while a parameter refers to measures about the population .
Populations are used when a research question requires data from every member of the population. This is usually only feasible when the population is small and easily accessible.
Samples are used to make inferences about populations . Samples are easier to collect data from because they are practical, cost-effective, convenient, and manageable.
There are seven threats to external validity : selection bias , history, experimenter effect, Hawthorne effect , testing effect, aptitude-treatment and situation effect.
The two types of external validity are population validity (whether you can generalize to other groups of people) and ecological validity (whether you can generalize to other situations and settings).
The external validity of a study is the extent to which you can generalize your findings to different groups of people, situations, and measures.
Cross-sectional studies cannot establish a cause-and-effect relationship or analyze behavior over a period of time. To investigate cause and effect, you need to do a longitudinal study or an experimental study .
Cross-sectional studies are less expensive and time-consuming than many other types of study. They can provide useful insights into a population’s characteristics and identify correlations for further research.
Sometimes only cross-sectional data is available for analysis; other times your research question may only require a cross-sectional study to answer it.
Longitudinal studies can last anywhere from weeks to decades, although they tend to be at least a year long.
The 1970 British Cohort Study , which has collected data on the lives of 17,000 Brits since their births in 1970, is one well-known example of a longitudinal study .
Longitudinal studies are better to establish the correct sequence of events, identify changes over time, and provide insight into cause-and-effect relationships, but they also tend to be more expensive and time-consuming than other types of studies.
Longitudinal studies and cross-sectional studies are two different types of research design . In a cross-sectional study you collect data from a population at a specific point in time; in a longitudinal study you repeatedly collect data from the same sample over an extended period of time.
There are eight threats to internal validity : history, maturation, instrumentation, testing, selection bias , regression to the mean, social interaction and attrition .
Internal validity is the extent to which you can be confident that a cause-and-effect relationship established in a study cannot be explained by other factors.
In mixed methods research , you use both qualitative and quantitative data collection and analysis methods to answer your research question .
The research methods you use depend on the type of data you need to answer your research question .
- If you want to measure something or test a hypothesis , use quantitative methods . If you want to explore ideas, thoughts and meanings, use qualitative methods .
- If you want to analyze a large amount of readily-available data, use secondary data. If you want data specific to your purposes with control over how it is generated, collect primary data.
- If you want to establish cause-and-effect relationships between variables , use experimental methods. If you want to understand the characteristics of a research subject, use descriptive methods.
A confounding variable , also called a confounder or confounding factor, is a third variable in a study examining a potential cause-and-effect relationship.
A confounding variable is related to both the supposed cause and the supposed effect of the study. It can be difficult to separate the true effect of the independent variable from the effect of the confounding variable.
In your research design , it’s important to identify potential confounding variables and plan how you will reduce their impact.
Discrete and continuous variables are two types of quantitative variables :
- Discrete variables represent counts (e.g. the number of objects in a collection).
- Continuous variables represent measurable amounts (e.g. water volume or weight).
Quantitative variables are any variables where the data represent amounts (e.g. height, weight, or age).
Categorical variables are any variables where the data represent groups. This includes rankings (e.g. finishing places in a race), classifications (e.g. brands of cereal), and binary outcomes (e.g. coin flips).
You need to know what type of variables you are working with to choose the right statistical test for your data and interpret your results .
You can think of independent and dependent variables in terms of cause and effect: an independent variable is the variable you think is the cause , while a dependent variable is the effect .
In an experiment, you manipulate the independent variable and measure the outcome in the dependent variable. For example, in an experiment about the effect of nutrients on crop growth:
- The independent variable is the amount of nutrients added to the crop field.
- The dependent variable is the biomass of the crops at harvest time.
Defining your variables, and deciding how you will manipulate and measure them, is an important part of experimental design .
Experimental design means planning a set of procedures to investigate a relationship between variables . To design a controlled experiment, you need:
- A testable hypothesis
- At least one independent variable that can be precisely manipulated
- At least one dependent variable that can be precisely measured
When designing the experiment, you decide:
- How you will manipulate the variable(s)
- How you will control for any potential confounding variables
- How many subjects or samples will be included in the study
- How subjects will be assigned to treatment levels
Experimental design is essential to the internal and external validity of your experiment.
I nternal validity is the degree of confidence that the causal relationship you are testing is not influenced by other factors or variables .
External validity is the extent to which your results can be generalized to other contexts.
The validity of your experiment depends on your experimental design .
Reliability and validity are both about how well a method measures something:
- Reliability refers to the consistency of a measure (whether the results can be reproduced under the same conditions).
- Validity refers to the accuracy of a measure (whether the results really do represent what they are supposed to measure).
If you are doing experimental research, you also have to consider the internal and external validity of your experiment.
A sample is a subset of individuals from a larger population . Sampling means selecting the group that you will actually collect data from in your research. For example, if you are researching the opinions of students in your university, you could survey a sample of 100 students.
In statistics, sampling allows you to test a hypothesis about the characteristics of a population.
Quantitative research deals with numbers and statistics, while qualitative research deals with words and meanings.
Quantitative methods allow you to systematically measure variables and test hypotheses . Qualitative methods allow you to explore concepts and experiences in more detail.
Methodology refers to the overarching strategy and rationale of your research project . It involves studying the methods used in your field and the theories or principles behind them, in order to develop an approach that matches your objectives.
Methods are the specific tools and procedures you use to collect and analyze data (for example, experiments, surveys , and statistical tests ).
In shorter scientific papers, where the aim is to report the findings of a specific study, you might simply describe what you did in a methods section .
In a longer or more complex research project, such as a thesis or dissertation , you will probably include a methodology section , where you explain your approach to answering the research questions and cite relevant sources to support your choice of methods.
Ask our team
Want to contact us directly? No problem. We are always here for you.
- Email [email protected]
- Start live chat
- Call +1 (510) 822-8066
- WhatsApp +31 20 261 6040
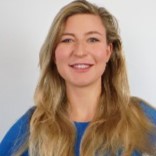
Our team helps students graduate by offering:
- A world-class citation generator
- Plagiarism Checker software powered by Turnitin
- Innovative Citation Checker software
- Professional proofreading services
- Over 300 helpful articles about academic writing, citing sources, plagiarism, and more
Scribbr specializes in editing study-related documents . We proofread:
- PhD dissertations
- Research proposals
- Personal statements
- Admission essays
- Motivation letters
- Reflection papers
- Journal articles
- Capstone projects
Scribbr’s Plagiarism Checker is powered by elements of Turnitin’s Similarity Checker , namely the plagiarism detection software and the Internet Archive and Premium Scholarly Publications content databases .
The add-on AI detector is powered by Scribbr’s proprietary software.
The Scribbr Citation Generator is developed using the open-source Citation Style Language (CSL) project and Frank Bennett’s citeproc-js . It’s the same technology used by dozens of other popular citation tools, including Mendeley and Zotero.
You can find all the citation styles and locales used in the Scribbr Citation Generator in our publicly accessible repository on Github .
Survey vs. focus group: Choosing the right research method
Focus groups, key differences between surveys and focus groups, choosing the right method: when to use surveys vs. focus groups, benefits of combining both methods, create online surveys with surveyplanet.
In the world of research, data collection plays a pivotal role in gathering insights and understanding about various phenomena. Two prominent methods that researchers turn to are surveys and focus groups. While both are powerful tools for data collection, they do possess distinct characteristics and are better suited for different research objectives.
This comprehensive exploration will delve into the realms of surveys and focus groups, highlighting the key differences between them and acting as a guide in selecting the optimal research method based on specific needs.
Understanding the difference between a survey and a focus group
The differences between surveys and focus groups are significant and revolve around issues like data type, sample size, interaction, flexibility, and data analysis. These differences highlight each method’s unique strengths and characteristics and why they are suited for different research objectives and contexts.
Surveys are structured questionnaires designed to gather quantitative data from a large and diverse group of participants. These questionnaires can be administered through various channels, such as online platforms, phone calls, paper forms, or face-to-face interviews.
Surveys are highly flexible and customizable, allowing researchers to ask a wide array of questions, both closed-ended (requiring predefined responses) and open-ended (permitting free-form answers).
They are an ideal choice for collecting demographic information , preferences, attitudes, and opinions on specific subjects. The data collected through surveys can be analyzed using statistical techniques and offer numerical insights and trends that can help researchers draw conclusions and make informed decisions.
Focus groups, on the other hand, are qualitative research sessions that involve a small, carefully selected group of participants—usually ranging from 6 to 10. In a focus group setting, participants engage in guided discussions led by a skilled moderator.
These exchanges encourage participants to share their thoughts, ideas, experiences, and opinions with one another. Group dynamics often lead to a rich and multi-layered exploration of the chosen topic. Focus groups are particularly effective when exploring complex issues, understanding participant perceptions, and uncovering nuances that might not be captured by quantitative surveys alone.
Let’s explore some key differences between surveys and focus groups.
- Surveys generate quantitative data, which can be measured, categorized, and analyzed statistically. This type of data is valuable for identifying trends and patterns in large datasets.
- Focus groups generate qualitative data, which is textual, descriptive, and narrative in nature. This information provides a deeper understanding of participants’ perspectives, motivations, and emotions.
- Surveys can accommodate a larger sample size, making them suitable for reaching a broad audience and collecting data from diverse populations.
- Focus groups involve a smaller sample size due to the demands of a group discussion. They are better suited for in-depth exploration and richer interactions among participants.
- Surveys lack the real-time interaction that focus groups offer. Participants in surveys respond individually without being influenced by the responses of others.
- Focus groups thrive on interaction. Participants can react to each other’s viewpoints, providing insights that may not emerge in isolation.
- Surveys offer flexibility in terms of timing and participation. Participants can complete surveys at their own convenience.
- Focus groups require participants to be available at specific times. This demands a commitment from them and involves scheduling challenges.
- Survey data can be quantified and analyzed statistically using software. This assessment allows for identifying correlations, trends, and statistical significance.
- Focus group data necessitates a thorough qualitative analysis involving the identification of recurring themes, patterns, and subtle insights. This analysis often involves coding and thematic analysis.
Focus groups vs. surveys: Pros and cons
Let’s explore the pros and cons of focus groups and surveys to help you decide which method best suits your needs.
- Rich qualitative insights: Focus groups offer a deep exploration of participants’ thoughts, feelings, and experiences. The interactive nature of discussions allows for the uncovering of underlying motivations and complex viewpoints.
- Group dynamics: Participants in focus groups can react to each other’s opinions, sparking new ideas and encouraging the consideration of alternate viewpoints.
- In-depth understanding: Focus groups are particularly effective for exploring complex topics where understanding participants’ perceptions, attitudes, and emotions is crucial.
- Nuanced data: The qualitative data generated in focus groups can capture nuances, subtleties, and contextual details that might be missed in quantitative surveys.
- Limited generalizability: Findings from focus groups might not be easily generalized to larger populations due to the small sample size and potential selection bias.
- Resource intensive: Conducting focus groups requires more resources, including time, a skilled moderator, and a physical or virtual space for the discussion.
- Subjectivity: Analyzing focus group data can be subjective, as interpretations of participants’ responses rely on the skills and biases of the researcher.
- Quantitative data: Surveys generate structured quantitative data that can be easily analyzed using statistical techniques, allowing for the identification of trends and patterns. Learn when surveys are qualitative or quantitative research by reading our blog.
- Large sample size: Surveys can reach a large and diverse audience, making them suitable for obtaining insights from a wide range of participants.
- Efficiency: Surveys are effective in terms of data collection and analysis since they can be administered to a large number of participants simultaneously.
- Standardization: Surveys ensure consistency in data collection, as all participants respond to the same set of questions.
- Lack of depth: Surveys might not capture the intensity of participants’ feelings, motivations, or experiences since they often rely on closed-ended questions.
- Limited interaction: Surveys do not have the interactive nature of focus group discussions, which means participants can’t react to or build upon each other’s responses.
- Potential for biased responses: Participants might provide socially desirable or biased responses, especially on sensitive or controversial topics. Learn more about biased surveys by reading our blog.
- Question wording: The phrasing of survey questions can influence responses, leading to unintended interpretations or bias.
The choice between surveys and focus groups hinges on several factors related to research goals, resources, timeline, and the depth of insights that are being sought.
- When you aim to gather data from a wide and diverse audience.
- When you want to quantify trends, relationships, and patterns.
- When you have limited resources for conducting in-depth discussions.
- When you desire to explore participants’ perceptions, emotions, and experiences more deeply.
- When you need to uncover the “why” behind quantitative data trends.
- When you can allocate the necessary time and resources for coordinating and conducting interactive sessions.
In some cases, a combination of both methods can provide a comprehensive understanding of a research topic by leveraging the strengths of each approach. Ultimately, the choice should align with research objectives, available resources, and the depth of information sought.
Explore more types of surveys and survey methods that will provide valuable insights into a particular group’s opinions, behaviors, and preferences. And learn more about research surveys and how they can help you base your next important decision on data.
While surveys and focus groups have distinct strengths, they can be used in tandem to provide a comprehensive understanding of a research topic. Combining quantitative data from surveys with qualitative insights from focus groups can offer a well-rounded perspective that captures both the breadth and depth of the subject matter. This hybrid approach can illuminate not only what participants think but also why they hold certain opinions.
In conclusion, the choice between surveys and focus groups depends on your research objectives, budget, timeline, and the depth of insights being pursued. Surveys offer scalable quantitative data collection, while focus groups provide qualitative insights through interactive discussions.
By understanding the differences between these two methods and considering the unique advantages each offers, you can select the research approach that aligns best with goals and resources. And in some cases, a combination of both methods might be the key to unlocking a comprehensive understanding of a research topic.
Are you eager to tap into the thoughts and opinions of a target audience? Are you looking to make data-driven decisions that resonate with that target demographic? Our cutting-edge online survey tool is here to empower the uncovering of valuable insights.
Tailor surveys to fit unique research goals and company branding effortlessly. With a wide array of question types and design options, have the freedom to craft surveys that capture exactly what you need.
Click here to sign up now and revolutionize your approach to decision-making with our state-of-the-art survey tool. Your target market is waiting to be heard!
Photo by Firmbee.com on Unsplash
Case Study vs. Survey
What's the difference.
Case studies and surveys are both research methods used in various fields to gather information and insights. However, they differ in their approach and purpose. A case study involves an in-depth analysis of a specific individual, group, or situation, aiming to understand the complexities and unique aspects of the subject. It often involves collecting qualitative data through interviews, observations, and document analysis. On the other hand, a survey is a structured data collection method that involves gathering information from a larger sample size through standardized questionnaires. Surveys are typically used to collect quantitative data and provide a broader perspective on a particular topic or population. While case studies provide rich and detailed information, surveys offer a more generalizable and statistical overview.
Further Detail
Introduction.
When conducting research, there are various methods available to gather data and analyze it. Two commonly used methods are case study and survey. Both approaches have their own unique attributes and can be valuable in different research contexts. In this article, we will explore the characteristics of case study and survey, highlighting their strengths and limitations.
A case study is an in-depth investigation of a particular individual, group, or phenomenon. It involves collecting detailed information about the subject of study through various sources such as interviews, observations, and document analysis. Case studies are often used in social sciences, psychology, and business research to gain a deep understanding of complex issues.
One of the key attributes of a case study is its ability to provide rich and detailed data. Researchers can gather extensive information about the subject, including their background, experiences, and perspectives. This depth of data allows for a comprehensive analysis and interpretation of the case, providing valuable insights into the phenomenon under investigation.
Furthermore, case studies are particularly useful when studying rare or unique cases. Since case studies focus on specific individuals or groups, they can shed light on situations that are not easily replicated or observed in larger populations. This makes case studies valuable in exploring complex and nuanced phenomena that may not be easily captured through other research methods.
However, it is important to note that case studies have certain limitations. Due to their in-depth nature, case studies are often time-consuming and resource-intensive. Researchers need to invest significant effort in data collection, analysis, and interpretation. Additionally, the findings of a case study may not be easily generalized to larger populations, as the focus is on a specific case rather than a representative sample.
Despite these limitations, case studies offer a unique opportunity to explore complex issues in real-life contexts. They provide a detailed understanding of individual experiences and can generate hypotheses for further research.
A survey is a research method that involves collecting data from a sample of individuals through a structured questionnaire or interview. Surveys are widely used in social sciences, market research, and public opinion studies to gather information about a larger population. They aim to provide a snapshot of people's opinions, attitudes, behaviors, or characteristics.
One of the main advantages of surveys is their ability to collect data from a large number of respondents. By reaching out to a representative sample, researchers can generalize the findings to a larger population. Surveys also allow for efficient data collection, as questionnaires can be distributed electronically or in person, making it easier to gather a wide range of responses in a relatively short period.
Moreover, surveys offer a structured approach to data collection, ensuring consistency in the questions asked and the response options provided. This allows for easy comparison and analysis of the data, making surveys suitable for quantitative research. Surveys can also be conducted anonymously, which can encourage respondents to provide honest and unbiased answers, particularly when sensitive topics are being explored.
However, surveys also have their limitations. One of the challenges is the potential for response bias. Respondents may provide inaccurate or socially desirable answers, leading to biased results. Additionally, surveys often rely on self-reported data, which may be subject to memory recall errors or misinterpretation of questions. Researchers need to carefully design the survey instrument and consider potential biases to ensure the validity and reliability of the data collected.
Furthermore, surveys may not capture the complexity and depth of individual experiences. They provide a snapshot of people's opinions or behaviors at a specific point in time, but may not uncover the underlying reasons or motivations behind those responses. Surveys also rely on predetermined response options, limiting the range of possible answers and potentially overlooking important nuances.
Case studies and surveys are both valuable research methods, each with its own strengths and limitations. Case studies offer in-depth insights into specific cases, providing rich and detailed data. They are particularly useful for exploring complex and unique phenomena. On the other hand, surveys allow for efficient data collection from a large number of respondents, enabling generalization to larger populations. They provide structured and quantifiable data, making them suitable for statistical analysis.
Ultimately, the choice between case study and survey depends on the research objectives, the nature of the research question, and the available resources. Researchers need to carefully consider the attributes of each method and select the most appropriate approach to gather and analyze data effectively.
Comparisons may contain inaccurate information about people, places, or facts. Please report any issues.
- Key Differences
Know the Differences & Comparisons
Difference Between Survey and Questionnaire
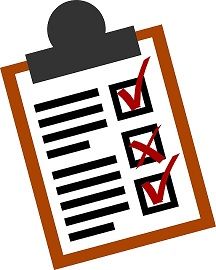
Conversely, Questionnaires are a tool of acquiring data on a particular topic, which involves distributing forms that comprise of questions relating to the topic under study. This article is presented for you to know the differences between survey and questionnaire.
Content: Survey Vs Questionnaire
Comparison chart, definition of survey.
By the term survey, we mean a research process, used for orderly collection and analysis of information, from a group of people to measure opinions, thoughts, experiences, etc. It is not confined to gathering information using questions, but it also encompasses observations, measurement, evaluation of data and judgment of the researcher.
A survey can have different forms like a survey of the whole population is known as the census, but it can also be conducted on a representative sample of a group with a view to drawing conclusions on a larger population. A sample survey is a widely used method because of its cost effectiveness, speed, and practical approach. There are many modes of carrying out surveys:
- Face to face survey (Interview)
- Questionnaire
- Telephonic survey
- Postal or mail out survey
- Email survey
- Web-based survey
Definition of Questionnaire
The term questionnaire refers to a form, which contains a set of survey questions, so designed, with a view to extracting certain information from the respondent. The instrument includes questions, instructions, and spaces for answers. The questions to be asked are so framed, to obtain straightforward information from the respondents.
A questionnaire has a written and printed format, delivered or distributed to people to provide responses to facts or opinions. The surveyor uses these responses for statistical analysis. It is mainly used for gathering factual information, with an intention to bifurcate people and their circumstances.
Key Differences Between Survey and Questionnaire
The difference between survey and questionnaire can be drawn clearly on the following grounds:
- The term survey, means the collection, recording, and analysis of information on a particular subject, an area or people’s group. Questionnaire implies a form containing a series of ready-made questions, delivered to people for to obtain statistical information.
- The survey is a process of collecting and analysing data, from the population. On the contrary, the questionnaire is an instrument used in the acquiring data.
- The survey is a time-consuming process, whereas questionnaire is the least time-consuming method of data acquisition.
- The survey is conducted while questionnaire is delivered, distributed or mailed to the respondents.
- In a survey, the questions asked in the survey can be open ended or closed ended, which depends on the topic, for which the survey is carried on. On the other hand, in the questionnaire the may include close ended questions only.
- The answer provided by the respondents during the survey can either be subjective or objective depending on the question. In contrast, the respondents provide objective answers to the questionnaire.
‘Survey’ is an umbrella term that includes a questionnaire, interview, observation method as a tool for collecting information. Although, the best, quickest and inexpensive way of conducting a survey, is the questionnaire. Surveys are usually conducted for research or studies, while questionnaire is used just to collect information such as job application or patient history form, etc.
You Might Also Like:
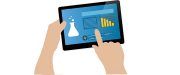
Leave a Reply Cancel reply
Your email address will not be published. Required fields are marked *
Save my name, email, and website in this browser for the next time I comment.
- Scroll to top
- Dark Light Dark Light
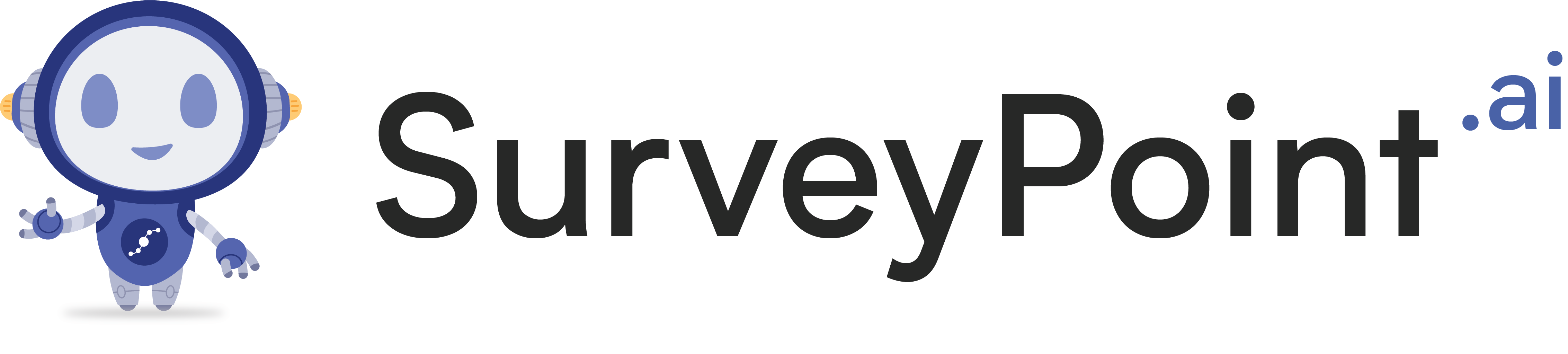
Questionnaire vs Survey in Research: Understanding the Differences
- Author Survey Point Team
- Published March 23, 2023
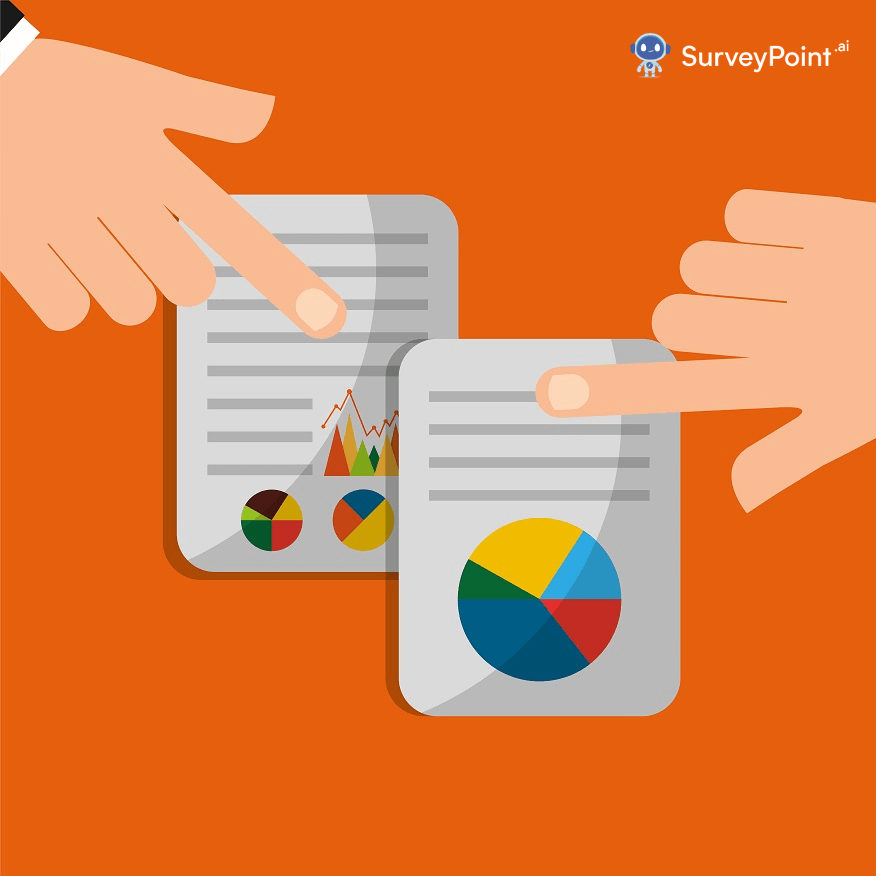
Are you trying to collect data for your research project but need help determining the best approach? Data collection is an essential phase in the research process. In most cases, questionnaires and surveys are used to collect data. But what makes questionnaire vs survey different? No need to look any further! This blog post will explore the two most commonly utilized data collection methods.
Although the terms’ questionnaire’ and ‘survey’ are sometimes used interchangeably, they have several key distinctions that can affect the methods and results of research.
For researchers to select the best tool for their research objectives, it is critical to understand the differences between surveys and questionnaires. Purpose, sample size, timeline, complexity, etc. are all things to take into account while deciding between the two.
Researchers can improve their chances of collecting and evaluating data by choosing the right method in advance.
Table of Contents
What is a Questionnaire?
Picture this: You’re handed a piece of paper with a list of questions, each neatly organized with multiple-choice answers. Congratulations! You’ve just encountered a questionnaire!
A questionnaire is a carefully designed list of questions typically distributed to a large population. The questions are usually designed to show specific information from participants and are often multiple-choice questions. You can give the participants a particular amount of time to complete questionnaires distributed online or on paper.
What is a Survey?
Imagine being handed a blank canvas and a set of paints and told to create a masterpiece. That’s what a survey can feel like – a blank slate for respondents to provide thoughtful responses.
Surveys have a more flexible approach of gathering data. They include a more comprehensive range of question types, including open-ended questions and ranking questions. You can also conduct surveys of smaller groups of people in person, over the phone, or online.
Like in a conversation, you can ask follow-up questions and go deeper into their comments. To understand this theory, let’s look at survey vs questionnaire examples .
Examples of Questionnaire Vs Survey
Now that you know the two, how can we further separate them?
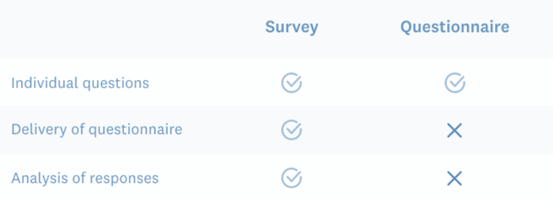
Here are some samples of a questionnaire vs survey so you can see what we mean:
Customer Satisfaction Questionnaire Vs Survey
Nowadays, you receive a customer satisfaction survey immediately after purchasing any product. It focuses more on particular areas of the customer experience, like usability, service quality, and customer assistance.
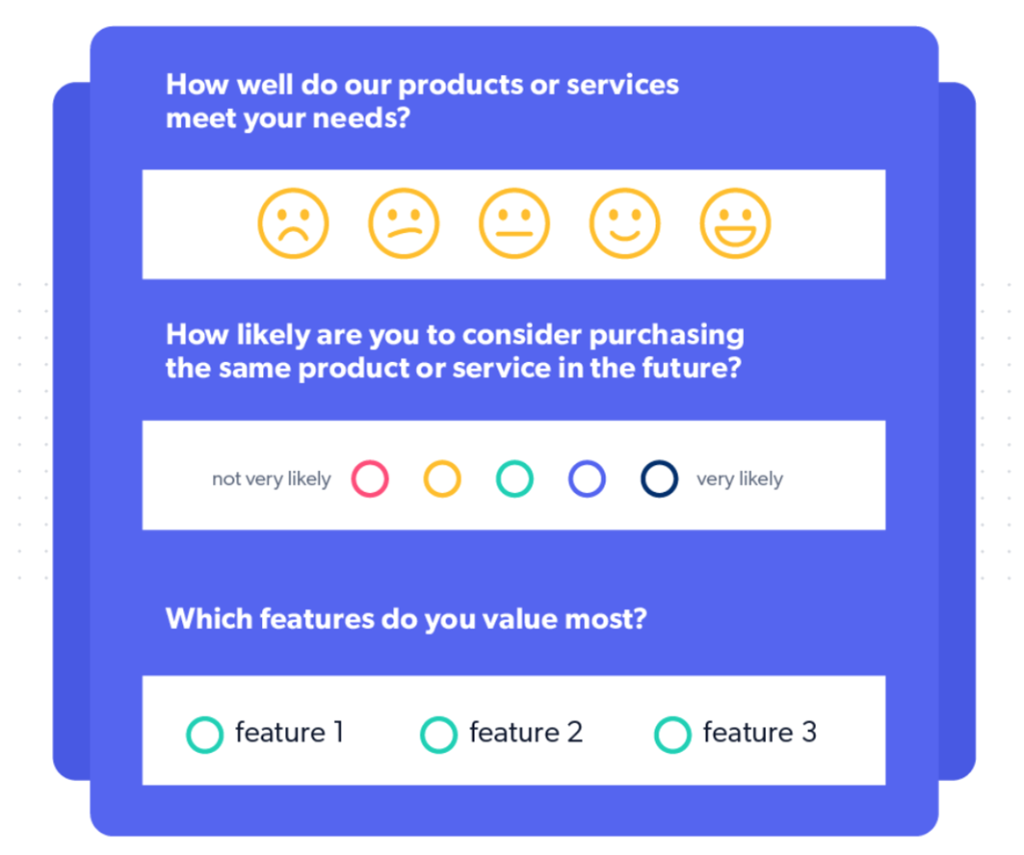
Health and Wellness Questionnaire Vs Survey
A health and wellness survey gathers data on various health attitudes and behaviors, such as food, exercise, sleep patterns, and more. Some of these questions can be:
- Are there any known medical issues with you?
- If so, do you have access to sufficient treatment and care for your conditions?
- How frequently do you work out?
- Which exercises do you find most enjoyable?
- What is the average number of hours you sleep each night?
Questions on mental and physical health, as well as lifestyle factors such as smoking and drinking, tend to be more narrowly focused in a health and wellness survey.
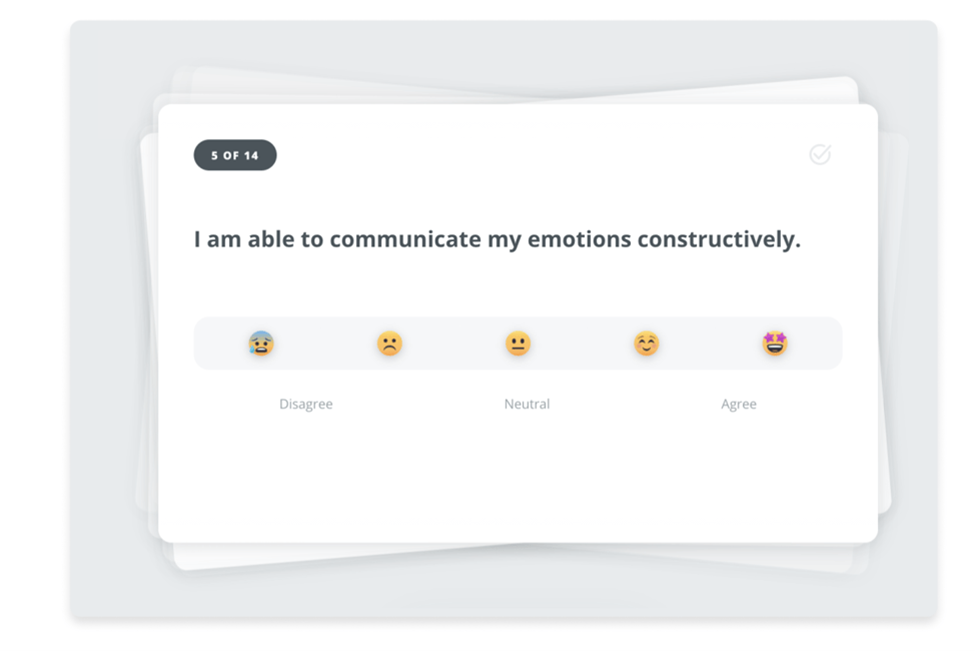
Employee Engagement Questionnaire vs Survey
An employee engagement questionnaire focuses more on specific aspects of the work environment , such as opportunities for growth and development, work-life balance, and team dynamics.
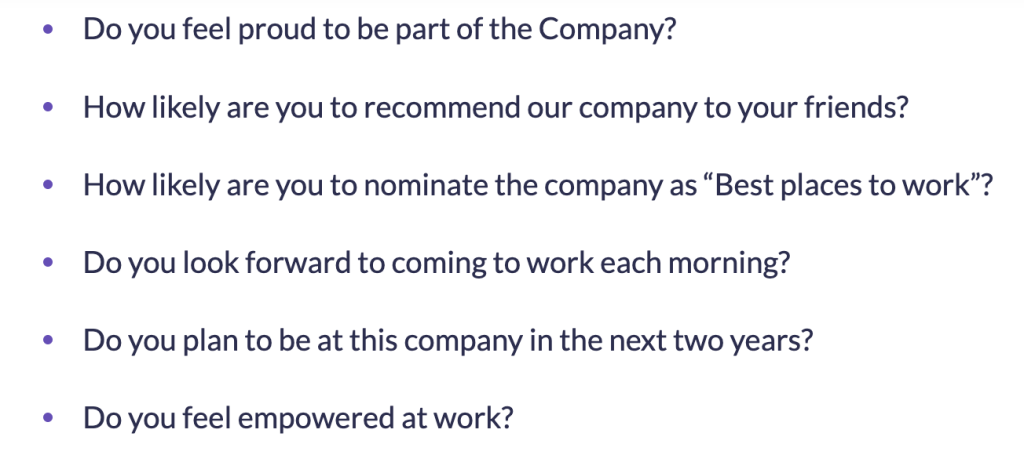
An employee engagement survey measures overall employee engagement , considering factors like motivation, dedication, and job satisfaction.
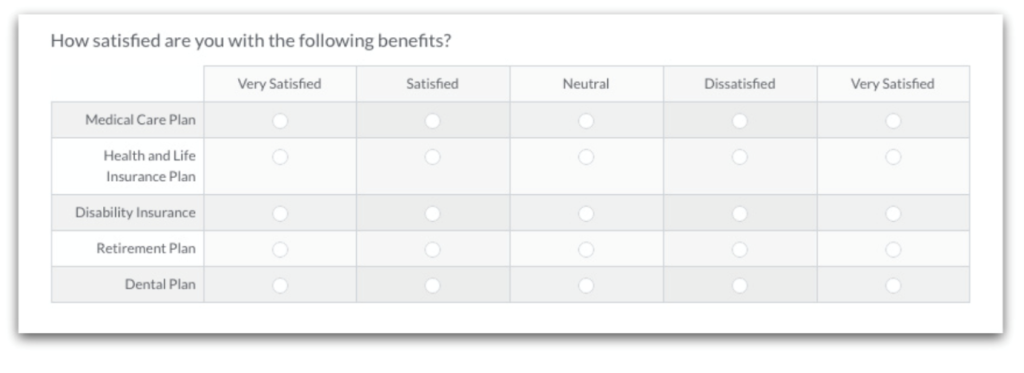
When designing research projects or gathering data about a particular objective, it’s useful to understand the various examples of questionnaire vs survey . It is common to use both approaches to collect data, but their administration, structure, and purposes differ greatly.
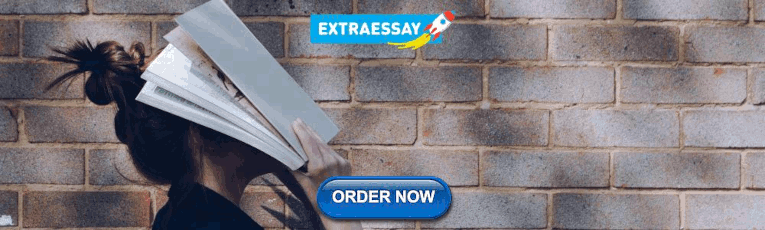
Choosing the Right Method for Your Research
Your decisions about the research methods to use can have a significant effect on the credibility and validity of your results. The research topic, the data to be collected, the individuals to be worked with, and the available resources are all factors to think about when deciding on a research strategy.
The selected approach will depend on the nature of the research being conducted and its specific goals. For instance,
- Suppose you’re interested in learning about a particular demographic’s worldview. In that case, you can choose to do a qualitative study using interviews or focus groups.
- Suppose you need to acquire numerical data to evaluate a hypothesis. In that case, one option is to employ a quantitative research approach , such as a survey or experiment.
Due to the need for unique equipment or expertise, certain research methods may be more costly or time-consuming than others. The consideration of ethics is also crucial.
Before commencing your investigation, ensure you have all the necessary permissions and followed all the correct processes.
Factors You Should Consider For Your Research
How do I choose which method works best? The following factors can decide questionnaire vs survey in research :
- Purpose: The first factor to consider is the purpose of the research. Surveys are better suited to gathering general information and opinions from a large sample of respondents than questionnaires.
- Sample Size: The sample size for questionnaires is better suited for smaller, more focused samples. Surveys typically use large-scale research with plenty of participants.
- Timeframe: The survey timeframe is quicker and more efficient, while questionnaires take longer to administer and analyze.
- Complexity: Surveys are better suited for straightforward research questions, while questionnaires are more complex and detailed.
- Resources: The availability of resources is essential. You may require more resources, such as personnel, technology, and budget, than questionnaires for surveys.
- Data Analysis: The last factor to consider is the method of data analysis. Surveys typically require more advanced data analysis techniques, while questionnaires can often be analyzed more simply.
Conclusion
It comes down to choosing the right tool for the job when choosing between questionnaires and surveys. While questionnaires are like precision knives, perfect for extracting specific information, surveys are more like fishing nets, great for casting a wide net and gathering a large sample of opinions.
A good choice depends on factors such as the purpose, the sample size, the timeframe, the complexity, the resources, and the data analysis.
Researchers can increase their chances of collecting and interpreting data successfully by selecting the appropriate tool. So, knowing the distinctions between questionnaires and surveys is essential in conducting successful research, whether you’re an experienced researcher or a curious first-timer.
Interested In Sending Your Own Surveys?
Explore our solutions that help researchers collect accurate insights, boost ROI, and retain respondents using pre-built templates that don’t require coding.
Survey Point Team
Recent posts.
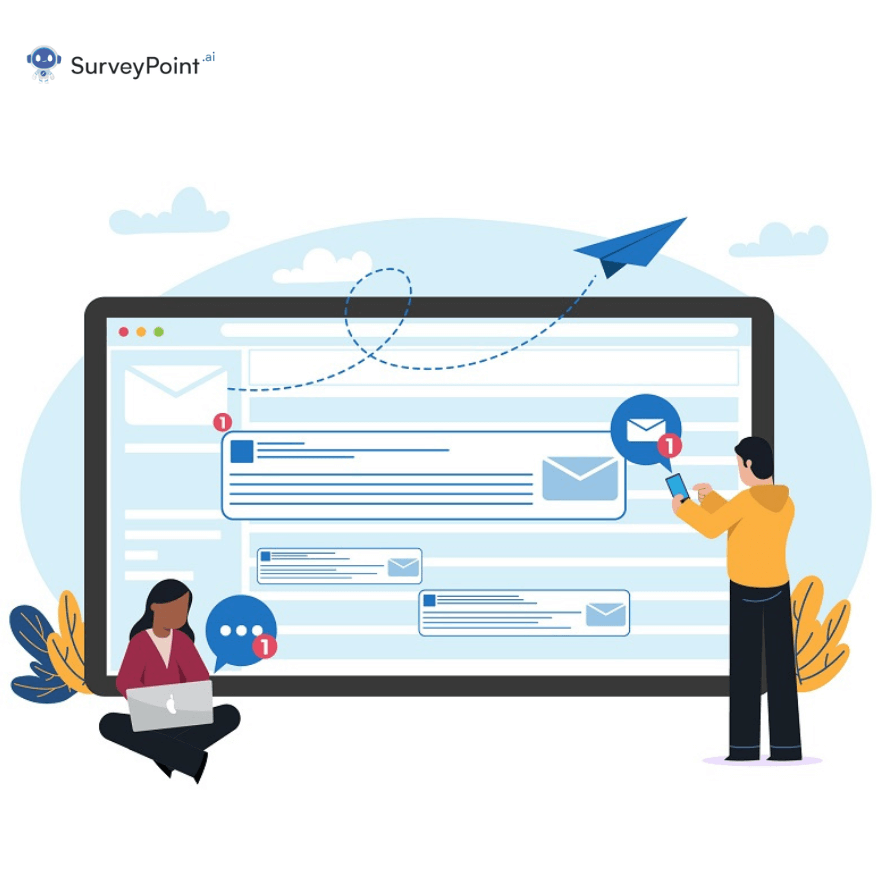
- Posted by Survey Point Team
Survey Email Examples to Improve Response Rate
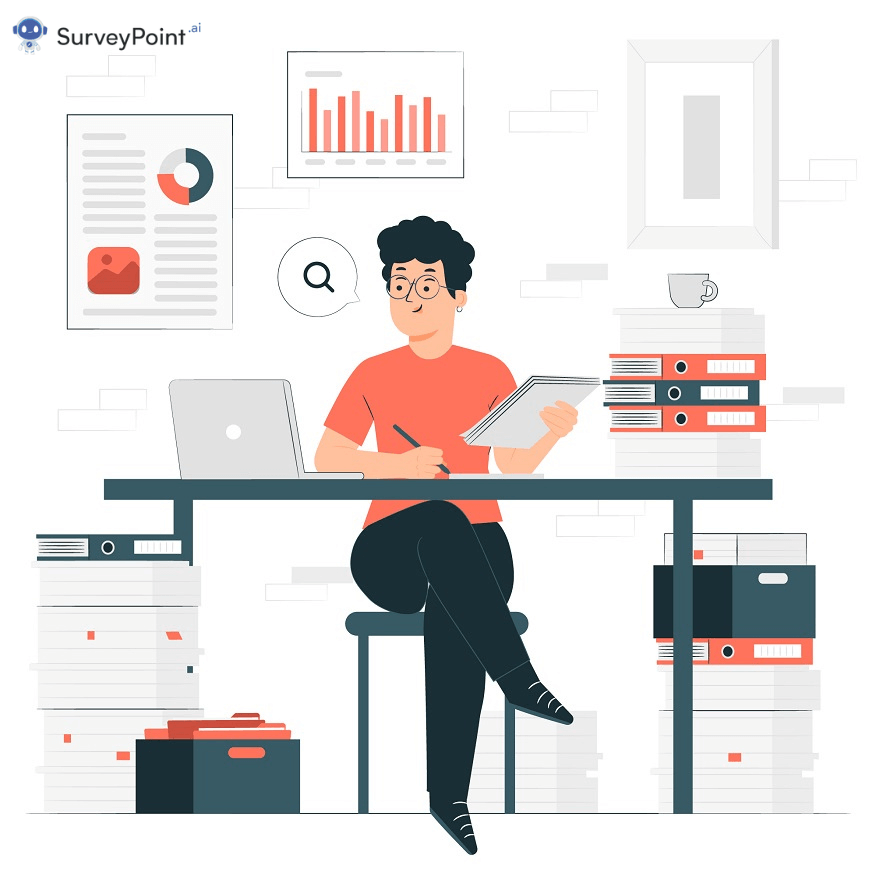
Market Research vs Marketing Research: What Are The Differences?
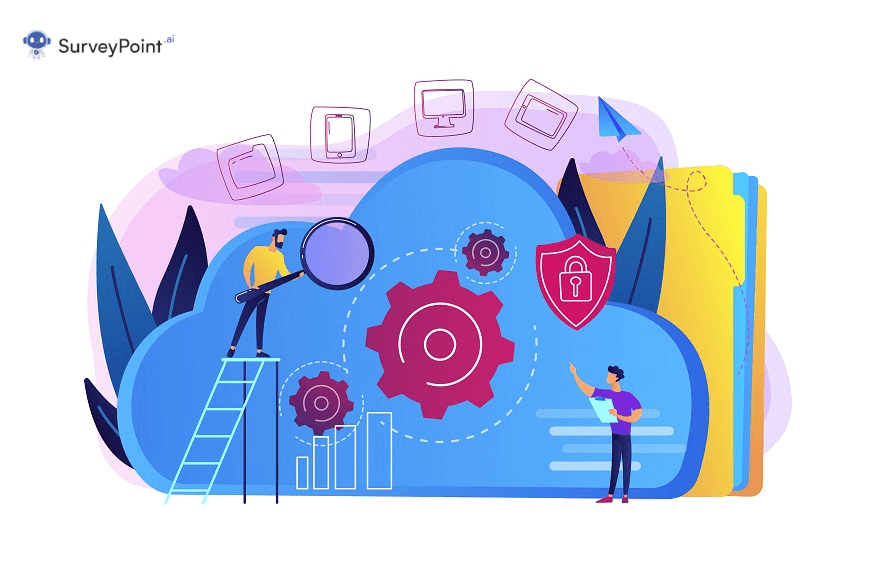
Getting to Know Research Methodology: A Quick Guide
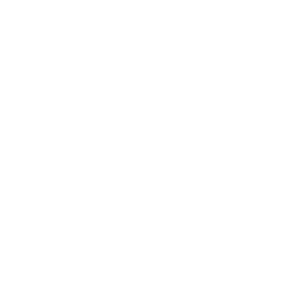
Cookie consent
We use our own and third-party cookies to show you more relevant content based on your browsing and navigation history. Please accept or manage your cookie settings below. Here's our cookie policy
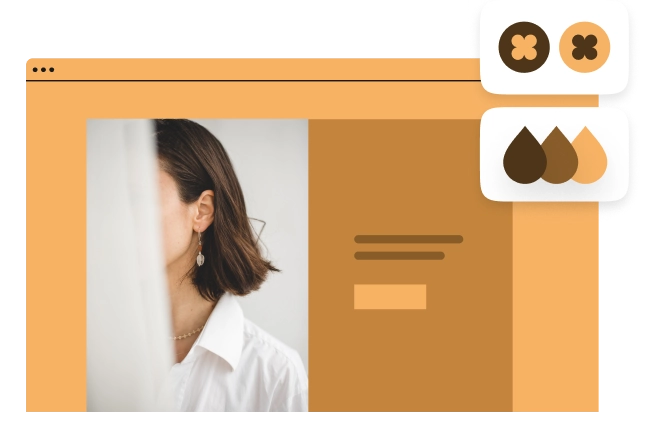
- Form builder Signups and orders
- Survey maker Research and feedback
- Quiz maker Trivia and product match
- Find customers Generate more leads
- Get feedback Discover ways to improve
- Do research Uncover trends and ideas
- Marketers Forms for marketing teams
- Product Forms for product teams
- HR Forms for HR teams
- Customer success Forms for customer success teams
- Business Forms for general business
- Form templates
- Survey templates
- Quiz templates
- Poll templates
- Order forms
- Feedback forms
- Satisfaction surveys
- Application forms
- Feedback surveys
- Evaluation forms
- Request forms
- Signup forms
- Business surveys
- Marketing surveys
- Report forms
- Customer feedback form
- Registration form
- Branding questionnaire
- 360 feedback
- Lead generation
- Contact form
- Signup sheet
- Google Sheets
- Google Analytics
- ActiveCampaign
- Help center Find quick answers
- Contact us Speak to someone
- Our blog Get inspired
- Our community Share and learn
- Our guides Tips and how-to
- Updates News and announcements
- Partners Browse or join
- Careers Join our team
- → Survey vs. questionnaire: What’s the ...
Survey vs. questionnaire: What’s the difference?
Get savvy on surveys and qualified in questionnaires. Learn the difference, boost your business.

Latest posts on Tips
Typeform | 03.2024
Cement vs. concrete. Poisonous vs. venomous. Shrimp vs. prawn.
Survey vs. questionnaire.
There are a ton of words in English that people mistake for synonyms. Although these words have different meanings, they tend to be used interchangeably. But delve into the details, and you see that they’re actually very different.
Surveys and questionnaires are a great example of this. There are a few differences between them, such as sample sizes and whether or not you’re looking to report and analyze data . In this article, we’ll dive into the key differences between a survey and questionnaire every marketer, manager, and researcher should know.
Survey vs. questionnaire: Differences and definitions
A survey collects data about a group of people so you can analyze and forecast trends about that group. As opposed to its questionnaire cousin, the data isn’t analyzed in isolation. Surveys look for trends, behavior, or a bigger picture rather than individual insights.
A questionnaire collects data about individuals from a list of questions. It’s not used to look for trends, behavior, or a bigger picture. A questionnaire is usually limited in scope, and it isn’t used for gathering data or analyzing statistics.
Here’s another way to put it:
A questionnaire is a single-purpose data collection through a set of questions.
A survey is data collection using a set of questions for statistical analysis.
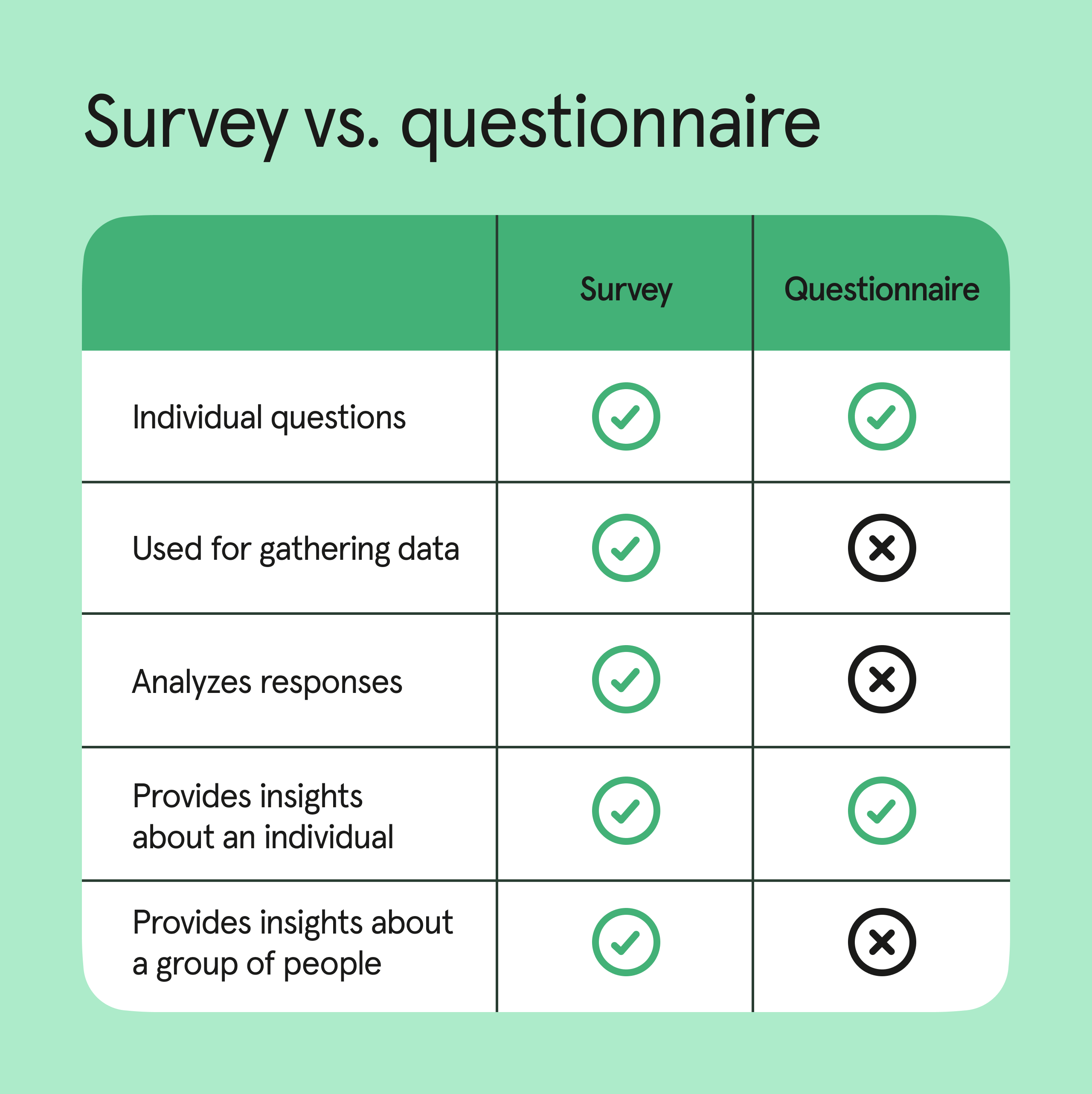
In the average person’s daily life, it’s not really a big deal to use "survey" and "questionnaire" interchangeably. But why? Well, sometimes because the context clears things up. Other times, the difference is so subtle the mishap goes unnoticed and unpunished, and so confusing the two terms doesn’t impact the message.
But these differences matter when it comes to surveys and questionnaires. Clarity is crucial if you’re the person reaching out for information. For marketers, managers, and researchers, these terms are two different beasts.
Once you have a handle on precisely what each does and doesn’t do, you’ll never mix them up again.
What is a survey?
If you’ve ever been handed a slip of paper asking for feedback after enjoying a dinner out, then you’re familiar with surveys.
Think of a survey as a major project that uses a larger dataset to analyze trends in that dataset. With a survey, you can dig deeper and find out peoples’ opinions and ideas. You can ask demographic survey questions, determine how engaged your employees are, conduct market research , and much more.
What is a questionnaire?
Flashback to the last time you joined a gym. Maybe you opted for a health check when you signed up. If so, you’d have been asked to answer a list of specific questions about your medical history.
That was a questionnaire.
The information you provide is used to assess risk, help with diagnoses, and paint a picture of your personal medical history. It’s not used to look for trends, behavior, or paint a bigger picture.
When to use a survey vs. questionnaire
So, when all is said and done, does any of this matter? Who even cares about the terminology?
Let’s go back to the gym membership example.
When you answer all those questions about heart problems, fainting, and diabetes, your answers aren’t used to assess the health of local people in the area. Unless you reveal you have a medical condition, that questionnaire is placed in your file—until it’s needed.
But what if that medical questionnaire were being used as part of a large-scale medical research program?
Simply filing that piece of paper away wouldn’t achieve anything—the answers on it would have to be collated and merged with the answers given by other people.
And this is when the questionnaire becomes part of a survey. There’s a need to turn that raw data into actionable intelligence, which requires aggregation, analysis, and the identification of statistical trends.
Now, to dial your level of confusion up to 11, there’s also such a thing as a survey questionnaire. It starts as a simple questionnaire but later transforms into a survey—mindblown.
Imagine you’re trying to gauge how your employees feel about working with you. By using Likert scale questionnaires , you can ask people to express their feelings on a scale of, say, one to five. Then, by aggregating the scores, you can get an overall picture of satisfaction levels within your organization.
Let’s talk through some common situations where you might need to gather data and how you can choose between using a survey versus a questionnaire.
Building your pipeline
Surveys and questionnaires have their own purposes, so how do you decide which is right for what you need to accomplish?
Questionnaires are better suited for when you need quick data intake. Looking to gather contact information for new clients? A questionnaire is a great way to get everything you need.
Surveys are better for gathering large sets of data to interpret and pull trends from. Looking to create a report analyzing the behaviors of your top-converting prospects for some target audience research? A survey can help you spot and analyze big-picture trends.
Collecting data
Both surveys and questionnaires work for data collection, depending on what you want to do with that data.
Surveys are typically employed when you need to collect large amounts of data about groups of people, while questionnaires are better suited for smaller groups or collecting data about individuals .
Are you trying to gather info on which new benefits your employees want the most? A survey will do. What about helping your employees set goals for the next quarter? Try a questionnaire.
Data analysis
Surveys are the clear winner if you need to conduct data analysis. Think about a medical questionnaire—it’s helpful if you’re trying to evaluate a single patient’s health history, but you can’t use it to inform public health decisions.
A survey is built to make it easier to gather data about a large group of people, relying on qualitative data so you can pull patterns from responses at a glance. Questionnaires typically gather quantitative data, so they tell you a lot about an individual, but are too complicated for data analysis.
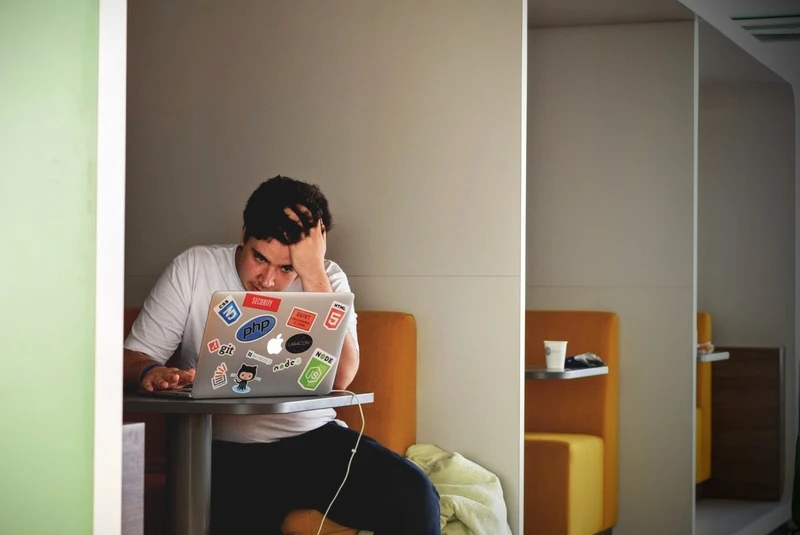
Perfect your surveys and questionnaires with Typeform
We’ve covered everything you need to know about using a survey vsersus a questionnaire to gather the data you need. But how do you get started?
You don’t need to worry. Whether you need to gather information to gather customer feedback or learn more about your customers, help is at hand. Use a simple survey maker and give your survey the best possible start.
Remember that you need to ask the right questions—and in the right way, to get the best answers.
Typeform captures your participants’ attention and keeps them engaged, guiding them through your survey or questionnaire one step at a time. Conditional logic allows a customized experience for each respondent, helping you dive deeper and gather more data—without creating more surveys. And you never have to worry about security because Typeforms are PCI, HIPAA, and WCAG 2.1 compliant.
Questionnaire or survey, out-of-the-blue conversational data collection is the best way to increase engagement rates—and give your business the information it needs to grow.
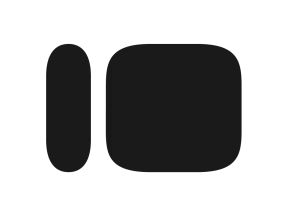
About the author
We're Typeform - a team on a mission to transform data collection by bringing you refreshingly different forms.
Liked that? Check these out:
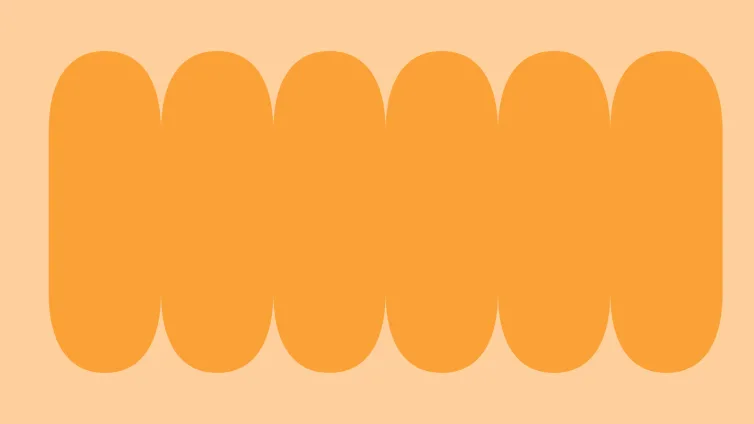
Survey School 5: Compliance considerations
Data compliance considerations don't need to be complicated. The good news is that you can gather useful data while staying on the right side of regulations and keeping your customers' trust.
Typeform | 06.2023
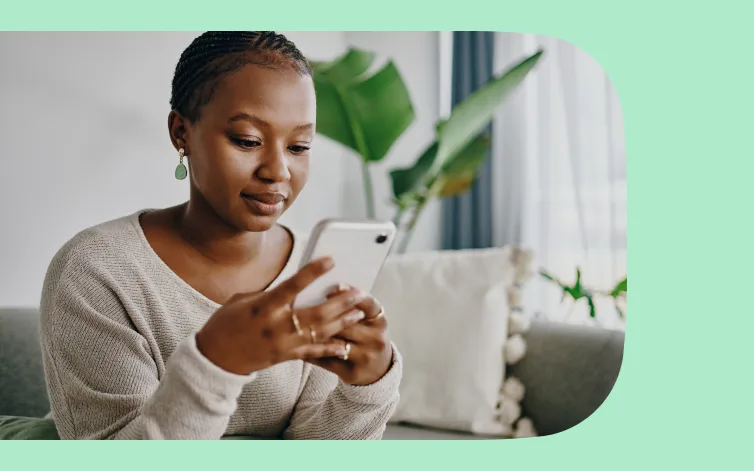
Typeform | 12.2023
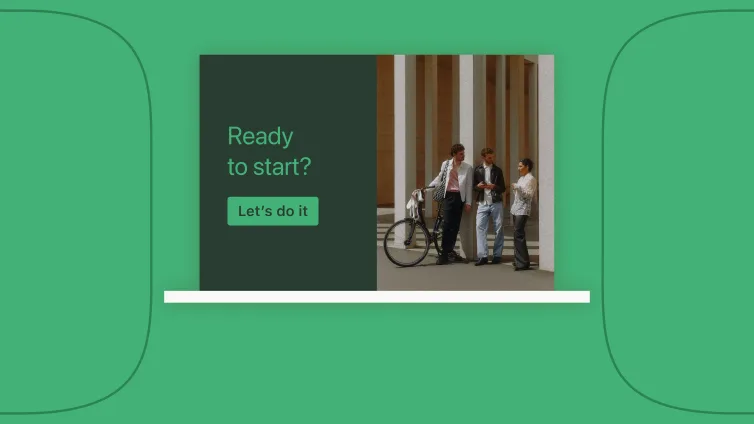
Survey School 1: Forms and questions
Dive into the first installment of our survey school, where we'll cover how to learn more about your customers by asking better questions. Follow our 10 research-backed guidelines for writing better questions to unlock more and better insights.
Typeform | 04.2023
Frequently asked questions
What’s the difference between a questionnaire and a survey.
A questionnaire is a data collection tool or instrument, while a survey is an overarching research method that involves collecting and analysing data from people using questionnaires.
Frequently asked questions: Methodology
Quantitative observations involve measuring or counting something and expressing the result in numerical form, while qualitative observations involve describing something in non-numerical terms, such as its appearance, texture, or color.
To make quantitative observations , you need to use instruments that are capable of measuring the quantity you want to observe. For example, you might use a ruler to measure the length of an object or a thermometer to measure its temperature.
Scope of research is determined at the beginning of your research process , prior to the data collection stage. Sometimes called “scope of study,” your scope delineates what will and will not be covered in your project. It helps you focus your work and your time, ensuring that you’ll be able to achieve your goals and outcomes.
Defining a scope can be very useful in any research project, from a research proposal to a thesis or dissertation . A scope is needed for all types of research: quantitative , qualitative , and mixed methods .
To define your scope of research, consider the following:
- Budget constraints or any specifics of grant funding
- Your proposed timeline and duration
- Specifics about your population of study, your proposed sample size , and the research methodology you’ll pursue
- Any inclusion and exclusion criteria
- Any anticipated control , extraneous , or confounding variables that could bias your research if not accounted for properly.
Inclusion and exclusion criteria are predominantly used in non-probability sampling . In purposive sampling and snowball sampling , restrictions apply as to who can be included in the sample .
Inclusion and exclusion criteria are typically presented and discussed in the methodology section of your thesis or dissertation .
The purpose of theory-testing mode is to find evidence in order to disprove, refine, or support a theory. As such, generalisability is not the aim of theory-testing mode.
Due to this, the priority of researchers in theory-testing mode is to eliminate alternative causes for relationships between variables . In other words, they prioritise internal validity over external validity , including ecological validity .
Convergent validity shows how much a measure of one construct aligns with other measures of the same or related constructs .
On the other hand, concurrent validity is about how a measure matches up to some known criterion or gold standard, which can be another measure.
Although both types of validity are established by calculating the association or correlation between a test score and another variable , they represent distinct validation methods.
Validity tells you how accurately a method measures what it was designed to measure. There are 4 main types of validity :
- Construct validity : Does the test measure the construct it was designed to measure?
- Face validity : Does the test appear to be suitable for its objectives ?
- Content validity : Does the test cover all relevant parts of the construct it aims to measure.
- Criterion validity : Do the results accurately measure the concrete outcome they are designed to measure?
Criterion validity evaluates how well a test measures the outcome it was designed to measure. An outcome can be, for example, the onset of a disease.
Criterion validity consists of two subtypes depending on the time at which the two measures (the criterion and your test) are obtained:
- Concurrent validity is a validation strategy where the the scores of a test and the criterion are obtained at the same time
- Predictive validity is a validation strategy where the criterion variables are measured after the scores of the test
Attrition refers to participants leaving a study. It always happens to some extent – for example, in randomised control trials for medical research.
Differential attrition occurs when attrition or dropout rates differ systematically between the intervention and the control group . As a result, the characteristics of the participants who drop out differ from the characteristics of those who stay in the study. Because of this, study results may be biased .
Criterion validity and construct validity are both types of measurement validity . In other words, they both show you how accurately a method measures something.
While construct validity is the degree to which a test or other measurement method measures what it claims to measure, criterion validity is the degree to which a test can predictively (in the future) or concurrently (in the present) measure something.
Construct validity is often considered the overarching type of measurement validity . You need to have face validity , content validity , and criterion validity in order to achieve construct validity.
Convergent validity and discriminant validity are both subtypes of construct validity . Together, they help you evaluate whether a test measures the concept it was designed to measure.
- Convergent validity indicates whether a test that is designed to measure a particular construct correlates with other tests that assess the same or similar construct.
- Discriminant validity indicates whether two tests that should not be highly related to each other are indeed not related. This type of validity is also called divergent validity .
You need to assess both in order to demonstrate construct validity. Neither one alone is sufficient for establishing construct validity.
Face validity and content validity are similar in that they both evaluate how suitable the content of a test is. The difference is that face validity is subjective, and assesses content at surface level.
When a test has strong face validity, anyone would agree that the test’s questions appear to measure what they are intended to measure.
For example, looking at a 4th grade math test consisting of problems in which students have to add and multiply, most people would agree that it has strong face validity (i.e., it looks like a math test).
On the other hand, content validity evaluates how well a test represents all the aspects of a topic. Assessing content validity is more systematic and relies on expert evaluation. of each question, analysing whether each one covers the aspects that the test was designed to cover.
A 4th grade math test would have high content validity if it covered all the skills taught in that grade. Experts(in this case, math teachers), would have to evaluate the content validity by comparing the test to the learning objectives.
Content validity shows you how accurately a test or other measurement method taps into the various aspects of the specific construct you are researching.
In other words, it helps you answer the question: “does the test measure all aspects of the construct I want to measure?” If it does, then the test has high content validity.
The higher the content validity, the more accurate the measurement of the construct.
If the test fails to include parts of the construct, or irrelevant parts are included, the validity of the instrument is threatened, which brings your results into question.
Construct validity refers to how well a test measures the concept (or construct) it was designed to measure. Assessing construct validity is especially important when you’re researching concepts that can’t be quantified and/or are intangible, like introversion. To ensure construct validity your test should be based on known indicators of introversion ( operationalisation ).
On the other hand, content validity assesses how well the test represents all aspects of the construct. If some aspects are missing or irrelevant parts are included, the test has low content validity.
- Discriminant validity indicates whether two tests that should not be highly related to each other are indeed not related
Construct validity has convergent and discriminant subtypes. They assist determine if a test measures the intended notion.
The reproducibility and replicability of a study can be ensured by writing a transparent, detailed method section and using clear, unambiguous language.
Reproducibility and replicability are related terms.
- A successful reproduction shows that the data analyses were conducted in a fair and honest manner.
- A successful replication shows that the reliability of the results is high.
- Reproducing research entails reanalysing the existing data in the same manner.
- Replicating (or repeating ) the research entails reconducting the entire analysis, including the collection of new data .
Snowball sampling is a non-probability sampling method . Unlike probability sampling (which involves some form of random selection ), the initial individuals selected to be studied are the ones who recruit new participants.
Because not every member of the target population has an equal chance of being recruited into the sample, selection in snowball sampling is non-random.
Snowball sampling is a non-probability sampling method , where there is not an equal chance for every member of the population to be included in the sample .
This means that you cannot use inferential statistics and make generalisations – often the goal of quantitative research . As such, a snowball sample is not representative of the target population, and is usually a better fit for qualitative research .
Snowball sampling relies on the use of referrals. Here, the researcher recruits one or more initial participants, who then recruit the next ones.
Participants share similar characteristics and/or know each other. Because of this, not every member of the population has an equal chance of being included in the sample, giving rise to sampling bias .
Snowball sampling is best used in the following cases:
- If there is no sampling frame available (e.g., people with a rare disease)
- If the population of interest is hard to access or locate (e.g., people experiencing homelessness)
- If the research focuses on a sensitive topic (e.g., extra-marital affairs)
Stratified sampling and quota sampling both involve dividing the population into subgroups and selecting units from each subgroup. The purpose in both cases is to select a representative sample and/or to allow comparisons between subgroups.
The main difference is that in stratified sampling, you draw a random sample from each subgroup ( probability sampling ). In quota sampling you select a predetermined number or proportion of units, in a non-random manner ( non-probability sampling ).
Random sampling or probability sampling is based on random selection. This means that each unit has an equal chance (i.e., equal probability) of being included in the sample.
On the other hand, convenience sampling involves stopping people at random, which means that not everyone has an equal chance of being selected depending on the place, time, or day you are collecting your data.
Convenience sampling and quota sampling are both non-probability sampling methods. They both use non-random criteria like availability, geographical proximity, or expert knowledge to recruit study participants.
However, in convenience sampling, you continue to sample units or cases until you reach the required sample size.
In quota sampling, you first need to divide your population of interest into subgroups (strata) and estimate their proportions (quota) in the population. Then you can start your data collection , using convenience sampling to recruit participants, until the proportions in each subgroup coincide with the estimated proportions in the population.
A sampling frame is a list of every member in the entire population . It is important that the sampling frame is as complete as possible, so that your sample accurately reflects your population.
Stratified and cluster sampling may look similar, but bear in mind that groups created in cluster sampling are heterogeneous , so the individual characteristics in the cluster vary. In contrast, groups created in stratified sampling are homogeneous , as units share characteristics.
Relatedly, in cluster sampling you randomly select entire groups and include all units of each group in your sample. However, in stratified sampling, you select some units of all groups and include them in your sample. In this way, both methods can ensure that your sample is representative of the target population .
When your population is large in size, geographically dispersed, or difficult to contact, it’s necessary to use a sampling method .
This allows you to gather information from a smaller part of the population, i.e. the sample, and make accurate statements by using statistical analysis. A few sampling methods include simple random sampling , convenience sampling , and snowball sampling .
The two main types of social desirability bias are:
- Self-deceptive enhancement (self-deception): The tendency to see oneself in a favorable light without realizing it.
- Impression managemen t (other-deception): The tendency to inflate one’s abilities or achievement in order to make a good impression on other people.
Response bias refers to conditions or factors that take place during the process of responding to surveys, affecting the responses. One type of response bias is social desirability bias .
Demand characteristics are aspects of experiments that may give away the research objective to participants. Social desirability bias occurs when participants automatically try to respond in ways that make them seem likeable in a study, even if it means misrepresenting how they truly feel.
Participants may use demand characteristics to infer social norms or experimenter expectancies and act in socially desirable ways, so you should try to control for demand characteristics wherever possible.
A systematic review is secondary research because it uses existing research. You don’t collect new data yourself.
Ethical considerations in research are a set of principles that guide your research designs and practices. These principles include voluntary participation, informed consent, anonymity, confidentiality, potential for harm, and results communication.
Scientists and researchers must always adhere to a certain code of conduct when collecting data from others .
These considerations protect the rights of research participants, enhance research validity , and maintain scientific integrity.
Research ethics matter for scientific integrity, human rights and dignity, and collaboration between science and society. These principles make sure that participation in studies is voluntary, informed, and safe.
Research misconduct means making up or falsifying data, manipulating data analyses, or misrepresenting results in research reports. It’s a form of academic fraud.
These actions are committed intentionally and can have serious consequences; research misconduct is not a simple mistake or a point of disagreement but a serious ethical failure.
Anonymity means you don’t know who the participants are, while confidentiality means you know who they are but remove identifying information from your research report. Both are important ethical considerations .
You can only guarantee anonymity by not collecting any personally identifying information – for example, names, phone numbers, email addresses, IP addresses, physical characteristics, photos, or videos.
You can keep data confidential by using aggregate information in your research report, so that you only refer to groups of participants rather than individuals.
Peer review is a process of evaluating submissions to an academic journal. Utilising rigorous criteria, a panel of reviewers in the same subject area decide whether to accept each submission for publication.
For this reason, academic journals are often considered among the most credible sources you can use in a research project – provided that the journal itself is trustworthy and well regarded.
In general, the peer review process follows the following steps:
- First, the author submits the manuscript to the editor.
- Reject the manuscript and send it back to author, or
- Send it onward to the selected peer reviewer(s)
- Next, the peer review process occurs. The reviewer provides feedback, addressing any major or minor issues with the manuscript, and gives their advice regarding what edits should be made.
- Lastly, the edited manuscript is sent back to the author. They input the edits, and resubmit it to the editor for publication.
Peer review can stop obviously problematic, falsified, or otherwise untrustworthy research from being published. It also represents an excellent opportunity to get feedback from renowned experts in your field.
It acts as a first defence, helping you ensure your argument is clear and that there are no gaps, vague terms, or unanswered questions for readers who weren’t involved in the research process.
Peer-reviewed articles are considered a highly credible source due to this stringent process they go through before publication.
Many academic fields use peer review , largely to determine whether a manuscript is suitable for publication. Peer review enhances the credibility of the published manuscript.
However, peer review is also common in non-academic settings. The United Nations, the European Union, and many individual nations use peer review to evaluate grant applications. It is also widely used in medical and health-related fields as a teaching or quality-of-care measure.
Peer assessment is often used in the classroom as a pedagogical tool. Both receiving feedback and providing it are thought to enhance the learning process, helping students think critically and collaboratively.
- In a single-blind study , only the participants are blinded.
- In a double-blind study , both participants and experimenters are blinded.
- In a triple-blind study , the assignment is hidden not only from participants and experimenters, but also from the researchers analysing the data.
Blinding is important to reduce bias (e.g., observer bias , demand characteristics ) and ensure a study’s internal validity .
If participants know whether they are in a control or treatment group , they may adjust their behaviour in ways that affect the outcome that researchers are trying to measure. If the people administering the treatment are aware of group assignment, they may treat participants differently and thus directly or indirectly influence the final results.
Blinding means hiding who is assigned to the treatment group and who is assigned to the control group in an experiment .
Explanatory research is a research method used to investigate how or why something occurs when only a small amount of information is available pertaining to that topic. It can help you increase your understanding of a given topic.
Explanatory research is used to investigate how or why a phenomenon occurs. Therefore, this type of research is often one of the first stages in the research process , serving as a jumping-off point for future research.
Exploratory research is a methodology approach that explores research questions that have not previously been studied in depth. It is often used when the issue you’re studying is new, or the data collection process is challenging in some way.
Exploratory research is often used when the issue you’re studying is new or when the data collection process is challenging for some reason.
You can use exploratory research if you have a general idea or a specific question that you want to study but there is no preexisting knowledge or paradigm with which to study it.
To implement random assignment , assign a unique number to every member of your study’s sample .
Then, you can use a random number generator or a lottery method to randomly assign each number to a control or experimental group. You can also do so manually, by flipping a coin or rolling a die to randomly assign participants to groups.
Random selection, or random sampling , is a way of selecting members of a population for your study’s sample.
In contrast, random assignment is a way of sorting the sample into control and experimental groups.
Random sampling enhances the external validity or generalisability of your results, while random assignment improves the internal validity of your study.
Random assignment is used in experiments with a between-groups or independent measures design. In this research design, there’s usually a control group and one or more experimental groups. Random assignment helps ensure that the groups are comparable.
In general, you should always use random assignment in this type of experimental design when it is ethically possible and makes sense for your study topic.
Clean data are valid, accurate, complete, consistent, unique, and uniform. Dirty data include inconsistencies and errors.
Dirty data can come from any part of the research process, including poor research design , inappropriate measurement materials, or flawed data entry.
Data cleaning takes place between data collection and data analyses. But you can use some methods even before collecting data.
For clean data, you should start by designing measures that collect valid data. Data validation at the time of data entry or collection helps you minimize the amount of data cleaning you’ll need to do.
After data collection, you can use data standardisation and data transformation to clean your data. You’ll also deal with any missing values, outliers, and duplicate values.
Data cleaning involves spotting and resolving potential data inconsistencies or errors to improve your data quality. An error is any value (e.g., recorded weight) that doesn’t reflect the true value (e.g., actual weight) of something that’s being measured.
In this process, you review, analyse, detect, modify, or remove ‘dirty’ data to make your dataset ‘clean’. Data cleaning is also called data cleansing or data scrubbing.
Data cleaning is necessary for valid and appropriate analyses. Dirty data contain inconsistencies or errors , but cleaning your data helps you minimise or resolve these.
Without data cleaning, you could end up with a Type I or II error in your conclusion. These types of erroneous conclusions can be practically significant with important consequences, because they lead to misplaced investments or missed opportunities.
Observer bias occurs when a researcher’s expectations, opinions, or prejudices influence what they perceive or record in a study. It usually affects studies when observers are aware of the research aims or hypotheses. This type of research bias is also called detection bias or ascertainment bias .
The observer-expectancy effect occurs when researchers influence the results of their own study through interactions with participants.
Researchers’ own beliefs and expectations about the study results may unintentionally influence participants through demand characteristics .
You can use several tactics to minimise observer bias .
- Use masking (blinding) to hide the purpose of your study from all observers.
- Triangulate your data with different data collection methods or sources.
- Use multiple observers and ensure inter-rater reliability.
- Train your observers to make sure data is consistently recorded between them.
- Standardise your observation procedures to make sure they are structured and clear.
Naturalistic observation is a valuable tool because of its flexibility, external validity , and suitability for topics that can’t be studied in a lab setting.
The downsides of naturalistic observation include its lack of scientific control , ethical considerations , and potential for bias from observers and subjects.
Naturalistic observation is a qualitative research method where you record the behaviours of your research subjects in real-world settings. You avoid interfering or influencing anything in a naturalistic observation.
You can think of naturalistic observation as ‘people watching’ with a purpose.
Closed-ended, or restricted-choice, questions offer respondents a fixed set of choices to select from. These questions are easier to answer quickly.
Open-ended or long-form questions allow respondents to answer in their own words. Because there are no restrictions on their choices, respondents can answer in ways that researchers may not have otherwise considered.
You can organise the questions logically, with a clear progression from simple to complex, or randomly between respondents. A logical flow helps respondents process the questionnaire easier and quicker, but it may lead to bias. Randomisation can minimise the bias from order effects.
Questionnaires can be self-administered or researcher-administered.
Self-administered questionnaires can be delivered online or in paper-and-pen formats, in person or by post. All questions are standardised so that all respondents receive the same questions with identical wording.
Researcher-administered questionnaires are interviews that take place by phone, in person, or online between researchers and respondents. You can gain deeper insights by clarifying questions for respondents or asking follow-up questions.
In a controlled experiment , all extraneous variables are held constant so that they can’t influence the results. Controlled experiments require:
- A control group that receives a standard treatment, a fake treatment, or no treatment
- Random assignment of participants to ensure the groups are equivalent
Depending on your study topic, there are various other methods of controlling variables .
An experimental group, also known as a treatment group, receives the treatment whose effect researchers wish to study, whereas a control group does not. They should be identical in all other ways.
A true experiment (aka a controlled experiment) always includes at least one control group that doesn’t receive the experimental treatment.
However, some experiments use a within-subjects design to test treatments without a control group. In these designs, you usually compare one group’s outcomes before and after a treatment (instead of comparing outcomes between different groups).
For strong internal validity , it’s usually best to include a control group if possible. Without a control group, it’s harder to be certain that the outcome was caused by the experimental treatment and not by other variables.
A Likert scale is a rating scale that quantitatively assesses opinions, attitudes, or behaviours. It is made up of four or more questions that measure a single attitude or trait when response scores are combined.
To use a Likert scale in a survey , you present participants with Likert-type questions or statements, and a continuum of items, usually with five or seven possible responses, to capture their degree of agreement.
Individual Likert-type questions are generally considered ordinal data , because the items have clear rank order, but don’t have an even distribution.
Overall Likert scale scores are sometimes treated as interval data. These scores are considered to have directionality and even spacing between them.
The type of data determines what statistical tests you should use to analyse your data.
A research hypothesis is your proposed answer to your research question. The research hypothesis usually includes an explanation (‘ x affects y because …’).
A statistical hypothesis, on the other hand, is a mathematical statement about a population parameter. Statistical hypotheses always come in pairs: the null and alternative hypotheses. In a well-designed study , the statistical hypotheses correspond logically to the research hypothesis.
A hypothesis states your predictions about what your research will find. It is a tentative answer to your research question that has not yet been tested. For some research projects, you might have to write several hypotheses that address different aspects of your research question.
A hypothesis is not just a guess. It should be based on existing theories and knowledge. It also has to be testable, which means you can support or refute it through scientific research methods (such as experiments, observations, and statistical analysis of data).
Cross-sectional studies are less expensive and time-consuming than many other types of study. They can provide useful insights into a population’s characteristics and identify correlations for further research.
Sometimes only cross-sectional data are available for analysis; other times your research question may only require a cross-sectional study to answer it.
Cross-sectional studies cannot establish a cause-and-effect relationship or analyse behaviour over a period of time. To investigate cause and effect, you need to do a longitudinal study or an experimental study .
Longitudinal studies and cross-sectional studies are two different types of research design . In a cross-sectional study you collect data from a population at a specific point in time; in a longitudinal study you repeatedly collect data from the same sample over an extended period of time.
Longitudinal studies are better to establish the correct sequence of events, identify changes over time, and provide insight into cause-and-effect relationships, but they also tend to be more expensive and time-consuming than other types of studies.
The 1970 British Cohort Study , which has collected data on the lives of 17,000 Brits since their births in 1970, is one well-known example of a longitudinal study .
Longitudinal studies can last anywhere from weeks to decades, although they tend to be at least a year long.
A correlation reflects the strength and/or direction of the association between two or more variables.
- A positive correlation means that both variables change in the same direction.
- A negative correlation means that the variables change in opposite directions.
- A zero correlation means there’s no relationship between the variables.
A correlational research design investigates relationships between two variables (or more) without the researcher controlling or manipulating any of them. It’s a non-experimental type of quantitative research .
A correlation coefficient is a single number that describes the strength and direction of the relationship between your variables.
Different types of correlation coefficients might be appropriate for your data based on their levels of measurement and distributions . The Pearson product-moment correlation coefficient (Pearson’s r ) is commonly used to assess a linear relationship between two quantitative variables.
Controlled experiments establish causality, whereas correlational studies only show associations between variables.
- In an experimental design , you manipulate an independent variable and measure its effect on a dependent variable. Other variables are controlled so they can’t impact the results.
- In a correlational design , you measure variables without manipulating any of them. You can test whether your variables change together, but you can’t be sure that one variable caused a change in another.
In general, correlational research is high in external validity while experimental research is high in internal validity .
The third variable and directionality problems are two main reasons why correlation isn’t causation .
The third variable problem means that a confounding variable affects both variables to make them seem causally related when they are not.
The directionality problem is when two variables correlate and might actually have a causal relationship, but it’s impossible to conclude which variable causes changes in the other.
As a rule of thumb, questions related to thoughts, beliefs, and feelings work well in focus groups . Take your time formulating strong questions, paying special attention to phrasing. Be careful to avoid leading questions , which can bias your responses.
Overall, your focus group questions should be:
- Open-ended and flexible
- Impossible to answer with ‘yes’ or ‘no’ (questions that start with ‘why’ or ‘how’ are often best)
- Unambiguous, getting straight to the point while still stimulating discussion
- Unbiased and neutral
Social desirability bias is the tendency for interview participants to give responses that will be viewed favourably by the interviewer or other participants. It occurs in all types of interviews and surveys , but is most common in semi-structured interviews , unstructured interviews , and focus groups .
Social desirability bias can be mitigated by ensuring participants feel at ease and comfortable sharing their views. Make sure to pay attention to your own body language and any physical or verbal cues, such as nodding or widening your eyes.
This type of bias in research can also occur in observations if the participants know they’re being observed. They might alter their behaviour accordingly.
A focus group is a research method that brings together a small group of people to answer questions in a moderated setting. The group is chosen due to predefined demographic traits, and the questions are designed to shed light on a topic of interest. It is one of four types of interviews .
The four most common types of interviews are:
- Structured interviews : The questions are predetermined in both topic and order.
- Semi-structured interviews : A few questions are predetermined, but other questions aren’t planned.
- Unstructured interviews : None of the questions are predetermined.
- Focus group interviews : The questions are presented to a group instead of one individual.
An unstructured interview is the most flexible type of interview, but it is not always the best fit for your research topic.
Unstructured interviews are best used when:
- You are an experienced interviewer and have a very strong background in your research topic, since it is challenging to ask spontaneous, colloquial questions
- Your research question is exploratory in nature. While you may have developed hypotheses, you are open to discovering new or shifting viewpoints through the interview process.
- You are seeking descriptive data, and are ready to ask questions that will deepen and contextualise your initial thoughts and hypotheses
- Your research depends on forming connections with your participants and making them feel comfortable revealing deeper emotions, lived experiences, or thoughts
A semi-structured interview is a blend of structured and unstructured types of interviews. Semi-structured interviews are best used when:
- You have prior interview experience. Spontaneous questions are deceptively challenging, and it’s easy to accidentally ask a leading question or make a participant uncomfortable.
- Your research question is exploratory in nature. Participant answers can guide future research questions and help you develop a more robust knowledge base for future research.
The interviewer effect is a type of bias that emerges when a characteristic of an interviewer (race, age, gender identity, etc.) influences the responses given by the interviewee.
There is a risk of an interviewer effect in all types of interviews , but it can be mitigated by writing really high-quality interview questions.
A structured interview is a data collection method that relies on asking questions in a set order to collect data on a topic. They are often quantitative in nature. Structured interviews are best used when:
- You already have a very clear understanding of your topic. Perhaps significant research has already been conducted, or you have done some prior research yourself, but you already possess a baseline for designing strong structured questions.
- You are constrained in terms of time or resources and need to analyse your data quickly and efficiently
- Your research question depends on strong parity between participants, with environmental conditions held constant
More flexible interview options include semi-structured interviews , unstructured interviews , and focus groups .
When conducting research, collecting original data has significant advantages:
- You can tailor data collection to your specific research aims (e.g., understanding the needs of your consumers or user testing your website).
- You can control and standardise the process for high reliability and validity (e.g., choosing appropriate measurements and sampling methods ).
However, there are also some drawbacks: data collection can be time-consuming, labour-intensive, and expensive. In some cases, it’s more efficient to use secondary data that has already been collected by someone else, but the data might be less reliable.
Data collection is the systematic process by which observations or measurements are gathered in research. It is used in many different contexts by academics, governments, businesses, and other organisations.
A mediator variable explains the process through which two variables are related, while a moderator variable affects the strength and direction of that relationship.
A confounder is a third variable that affects variables of interest and makes them seem related when they are not. In contrast, a mediator is the mechanism of a relationship between two variables: it explains the process by which they are related.
If something is a mediating variable :
- It’s caused by the independent variable
- It influences the dependent variable
- When it’s taken into account, the statistical correlation between the independent and dependent variables is higher than when it isn’t considered
Including mediators and moderators in your research helps you go beyond studying a simple relationship between two variables for a fuller picture of the real world. They are important to consider when studying complex correlational or causal relationships.
Mediators are part of the causal pathway of an effect, and they tell you how or why an effect takes place. Moderators usually help you judge the external validity of your study by identifying the limitations of when the relationship between variables holds.
You can think of independent and dependent variables in terms of cause and effect: an independent variable is the variable you think is the cause , while a dependent variable is the effect .
In an experiment, you manipulate the independent variable and measure the outcome in the dependent variable. For example, in an experiment about the effect of nutrients on crop growth:
- The independent variable is the amount of nutrients added to the crop field.
- The dependent variable is the biomass of the crops at harvest time.
Defining your variables, and deciding how you will manipulate and measure them, is an important part of experimental design .
Discrete and continuous variables are two types of quantitative variables :
- Discrete variables represent counts (e.g., the number of objects in a collection).
- Continuous variables represent measurable amounts (e.g., water volume or weight).
Quantitative variables are any variables where the data represent amounts (e.g. height, weight, or age).
Categorical variables are any variables where the data represent groups. This includes rankings (e.g. finishing places in a race), classifications (e.g. brands of cereal), and binary outcomes (e.g. coin flips).
You need to know what type of variables you are working with to choose the right statistical test for your data and interpret your results .
Determining cause and effect is one of the most important parts of scientific research. It’s essential to know which is the cause – the independent variable – and which is the effect – the dependent variable.
You want to find out how blood sugar levels are affected by drinking diet cola and regular cola, so you conduct an experiment .
- The type of cola – diet or regular – is the independent variable .
- The level of blood sugar that you measure is the dependent variable – it changes depending on the type of cola.
No. The value of a dependent variable depends on an independent variable, so a variable cannot be both independent and dependent at the same time. It must be either the cause or the effect, not both.
Yes, but including more than one of either type requires multiple research questions .
For example, if you are interested in the effect of a diet on health, you can use multiple measures of health: blood sugar, blood pressure, weight, pulse, and many more. Each of these is its own dependent variable with its own research question.
You could also choose to look at the effect of exercise levels as well as diet, or even the additional effect of the two combined. Each of these is a separate independent variable .
To ensure the internal validity of an experiment , you should only change one independent variable at a time.
To ensure the internal validity of your research, you must consider the impact of confounding variables. If you fail to account for them, you might over- or underestimate the causal relationship between your independent and dependent variables , or even find a causal relationship where none exists.
A confounding variable is closely related to both the independent and dependent variables in a study. An independent variable represents the supposed cause , while the dependent variable is the supposed effect . A confounding variable is a third variable that influences both the independent and dependent variables.
Failing to account for confounding variables can cause you to wrongly estimate the relationship between your independent and dependent variables.
There are several methods you can use to decrease the impact of confounding variables on your research: restriction, matching, statistical control, and randomisation.
In restriction , you restrict your sample by only including certain subjects that have the same values of potential confounding variables.
In matching , you match each of the subjects in your treatment group with a counterpart in the comparison group. The matched subjects have the same values on any potential confounding variables, and only differ in the independent variable .
In statistical control , you include potential confounders as variables in your regression .
In randomisation , you randomly assign the treatment (or independent variable) in your study to a sufficiently large number of subjects, which allows you to control for all potential confounding variables.
In scientific research, concepts are the abstract ideas or phenomena that are being studied (e.g., educational achievement). Variables are properties or characteristics of the concept (e.g., performance at school), while indicators are ways of measuring or quantifying variables (e.g., yearly grade reports).
The process of turning abstract concepts into measurable variables and indicators is called operationalisation .
In statistics, ordinal and nominal variables are both considered categorical variables .
Even though ordinal data can sometimes be numerical, not all mathematical operations can be performed on them.
A control variable is any variable that’s held constant in a research study. It’s not a variable of interest in the study, but it’s controlled because it could influence the outcomes.
Control variables help you establish a correlational or causal relationship between variables by enhancing internal validity .
If you don’t control relevant extraneous variables , they may influence the outcomes of your study, and you may not be able to demonstrate that your results are really an effect of your independent variable .
‘Controlling for a variable’ means measuring extraneous variables and accounting for them statistically to remove their effects on other variables.
Researchers often model control variable data along with independent and dependent variable data in regression analyses and ANCOVAs . That way, you can isolate the control variable’s effects from the relationship between the variables of interest.
An extraneous variable is any variable that you’re not investigating that can potentially affect the dependent variable of your research study.
A confounding variable is a type of extraneous variable that not only affects the dependent variable, but is also related to the independent variable.
There are 4 main types of extraneous variables :
- Demand characteristics : Environmental cues that encourage participants to conform to researchers’ expectations
- Experimenter effects : Unintentional actions by researchers that influence study outcomes
- Situational variables : Eenvironmental variables that alter participants’ behaviours
- Participant variables : Any characteristic or aspect of a participant’s background that could affect study results
The difference between explanatory and response variables is simple:
- An explanatory variable is the expected cause, and it explains the results.
- A response variable is the expected effect, and it responds to other variables.
The term ‘ explanatory variable ‘ is sometimes preferred over ‘ independent variable ‘ because, in real-world contexts, independent variables are often influenced by other variables. This means they aren’t totally independent.
Multiple independent variables may also be correlated with each other, so ‘explanatory variables’ is a more appropriate term.
On graphs, the explanatory variable is conventionally placed on the x -axis, while the response variable is placed on the y -axis.
- If you have quantitative variables , use a scatterplot or a line graph.
- If your response variable is categorical, use a scatterplot or a line graph.
- If your explanatory variable is categorical, use a bar graph.
A correlation is usually tested for two variables at a time, but you can test correlations between three or more variables.
An independent variable is the variable you manipulate, control, or vary in an experimental study to explore its effects. It’s called ‘independent’ because it’s not influenced by any other variables in the study.
Independent variables are also called:
- Explanatory variables (they explain an event or outcome)
- Predictor variables (they can be used to predict the value of a dependent variable)
- Right-hand-side variables (they appear on the right-hand side of a regression equation)
A dependent variable is what changes as a result of the independent variable manipulation in experiments . It’s what you’re interested in measuring, and it ‘depends’ on your independent variable.
In statistics, dependent variables are also called:
- Response variables (they respond to a change in another variable)
- Outcome variables (they represent the outcome you want to measure)
- Left-hand-side variables (they appear on the left-hand side of a regression equation)
Deductive reasoning is commonly used in scientific research, and it’s especially associated with quantitative research .
In research, you might have come across something called the hypothetico-deductive method . It’s the scientific method of testing hypotheses to check whether your predictions are substantiated by real-world data.
Deductive reasoning is a logical approach where you progress from general ideas to specific conclusions. It’s often contrasted with inductive reasoning , where you start with specific observations and form general conclusions.
Deductive reasoning is also called deductive logic.
Inductive reasoning is a method of drawing conclusions by going from the specific to the general. It’s usually contrasted with deductive reasoning, where you proceed from general information to specific conclusions.
Inductive reasoning is also called inductive logic or bottom-up reasoning.
In inductive research , you start by making observations or gathering data. Then, you take a broad scan of your data and search for patterns. Finally, you make general conclusions that you might incorporate into theories.
Inductive reasoning is a bottom-up approach, while deductive reasoning is top-down.
Inductive reasoning takes you from the specific to the general, while in deductive reasoning, you make inferences by going from general premises to specific conclusions.
There are many different types of inductive reasoning that people use formally or informally.
Here are a few common types:
- Inductive generalisation : You use observations about a sample to come to a conclusion about the population it came from.
- Statistical generalisation: You use specific numbers about samples to make statements about populations.
- Causal reasoning: You make cause-and-effect links between different things.
- Sign reasoning: You make a conclusion about a correlational relationship between different things.
- Analogical reasoning: You make a conclusion about something based on its similarities to something else.
It’s often best to ask a variety of people to review your measurements. You can ask experts, such as other researchers, or laypeople, such as potential participants, to judge the face validity of tests.
While experts have a deep understanding of research methods , the people you’re studying can provide you with valuable insights you may have missed otherwise.
Face validity is important because it’s a simple first step to measuring the overall validity of a test or technique. It’s a relatively intuitive, quick, and easy way to start checking whether a new measure seems useful at first glance.
Good face validity means that anyone who reviews your measure says that it seems to be measuring what it’s supposed to. With poor face validity, someone reviewing your measure may be left confused about what you’re measuring and why you’re using this method.
Face validity is about whether a test appears to measure what it’s supposed to measure. This type of validity is concerned with whether a measure seems relevant and appropriate for what it’s assessing only on the surface.
Statistical analyses are often applied to test validity with data from your measures. You test convergent validity and discriminant validity with correlations to see if results from your test are positively or negatively related to those of other established tests.
You can also use regression analyses to assess whether your measure is actually predictive of outcomes that you expect it to predict theoretically. A regression analysis that supports your expectations strengthens your claim of construct validity .
When designing or evaluating a measure, construct validity helps you ensure you’re actually measuring the construct you’re interested in. If you don’t have construct validity, you may inadvertently measure unrelated or distinct constructs and lose precision in your research.
Construct validity is often considered the overarching type of measurement validity , because it covers all of the other types. You need to have face validity , content validity, and criterion validity to achieve construct validity.
Construct validity is about how well a test measures the concept it was designed to evaluate. It’s one of four types of measurement validity , which includes construct validity, face validity , and criterion validity.
There are two subtypes of construct validity.
- Convergent validity : The extent to which your measure corresponds to measures of related constructs
- Discriminant validity: The extent to which your measure is unrelated or negatively related to measures of distinct constructs
Attrition bias can skew your sample so that your final sample differs significantly from your original sample. Your sample is biased because some groups from your population are underrepresented.
With a biased final sample, you may not be able to generalise your findings to the original population that you sampled from, so your external validity is compromised.
There are seven threats to external validity : selection bias , history, experimenter effect, Hawthorne effect , testing effect, aptitude-treatment, and situation effect.
The two types of external validity are population validity (whether you can generalise to other groups of people) and ecological validity (whether you can generalise to other situations and settings).
The external validity of a study is the extent to which you can generalise your findings to different groups of people, situations, and measures.
Attrition bias is a threat to internal validity . In experiments, differential rates of attrition between treatment and control groups can skew results.
This bias can affect the relationship between your independent and dependent variables . It can make variables appear to be correlated when they are not, or vice versa.
Internal validity is the extent to which you can be confident that a cause-and-effect relationship established in a study cannot be explained by other factors.
There are eight threats to internal validity : history, maturation, instrumentation, testing, selection bias , regression to the mean, social interaction, and attrition .
A sampling error is the difference between a population parameter and a sample statistic .
A statistic refers to measures about the sample , while a parameter refers to measures about the population .
Populations are used when a research question requires data from every member of the population. This is usually only feasible when the population is small and easily accessible.
Systematic sampling is a probability sampling method where researchers select members of the population at a regular interval – for example, by selecting every 15th person on a list of the population. If the population is in a random order, this can imitate the benefits of simple random sampling .
There are three key steps in systematic sampling :
- Define and list your population , ensuring that it is not ordered in a cyclical or periodic order.
- Decide on your sample size and calculate your interval, k , by dividing your population by your target sample size.
- Choose every k th member of the population as your sample.
Yes, you can create a stratified sample using multiple characteristics, but you must ensure that every participant in your study belongs to one and only one subgroup. In this case, you multiply the numbers of subgroups for each characteristic to get the total number of groups.
For example, if you were stratifying by location with three subgroups (urban, rural, or suburban) and marital status with five subgroups (single, divorced, widowed, married, or partnered), you would have 3 × 5 = 15 subgroups.
You should use stratified sampling when your sample can be divided into mutually exclusive and exhaustive subgroups that you believe will take on different mean values for the variable that you’re studying.
Using stratified sampling will allow you to obtain more precise (with lower variance ) statistical estimates of whatever you are trying to measure.
For example, say you want to investigate how income differs based on educational attainment, but you know that this relationship can vary based on race. Using stratified sampling, you can ensure you obtain a large enough sample from each racial group, allowing you to draw more precise conclusions.
In stratified sampling , researchers divide subjects into subgroups called strata based on characteristics that they share (e.g., race, gender, educational attainment).
Once divided, each subgroup is randomly sampled using another probability sampling method .
Multistage sampling can simplify data collection when you have large, geographically spread samples, and you can obtain a probability sample without a complete sampling frame.
But multistage sampling may not lead to a representative sample, and larger samples are needed for multistage samples to achieve the statistical properties of simple random samples .
In multistage sampling , you can use probability or non-probability sampling methods.
For a probability sample, you have to probability sampling at every stage. You can mix it up by using simple random sampling , systematic sampling , or stratified sampling to select units at different stages, depending on what is applicable and relevant to your study.
Cluster sampling is a probability sampling method in which you divide a population into clusters, such as districts or schools, and then randomly select some of these clusters as your sample.
The clusters should ideally each be mini-representations of the population as a whole.
There are three types of cluster sampling : single-stage, double-stage and multi-stage clustering. In all three types, you first divide the population into clusters, then randomly select clusters for use in your sample.
- In single-stage sampling , you collect data from every unit within the selected clusters.
- In double-stage sampling , you select a random sample of units from within the clusters.
- In multi-stage sampling , you repeat the procedure of randomly sampling elements from within the clusters until you have reached a manageable sample.
Cluster sampling is more time- and cost-efficient than other probability sampling methods , particularly when it comes to large samples spread across a wide geographical area.
However, it provides less statistical certainty than other methods, such as simple random sampling , because it is difficult to ensure that your clusters properly represent the population as a whole.
If properly implemented, simple random sampling is usually the best sampling method for ensuring both internal and external validity . However, it can sometimes be impractical and expensive to implement, depending on the size of the population to be studied,
If you have a list of every member of the population and the ability to reach whichever members are selected, you can use simple random sampling.
The American Community Survey is an example of simple random sampling . In order to collect detailed data on the population of the US, the Census Bureau officials randomly select 3.5 million households per year and use a variety of methods to convince them to fill out the survey.
Simple random sampling is a type of probability sampling in which the researcher randomly selects a subset of participants from a population . Each member of the population has an equal chance of being selected. Data are then collected from as large a percentage as possible of this random subset.
Sampling bias occurs when some members of a population are systematically more likely to be selected in a sample than others.
In multistage sampling , or multistage cluster sampling, you draw a sample from a population using smaller and smaller groups at each stage.
This method is often used to collect data from a large, geographically spread group of people in national surveys, for example. You take advantage of hierarchical groupings (e.g., from county to city to neighbourhood) to create a sample that’s less expensive and time-consuming to collect data from.
In non-probability sampling , the sample is selected based on non-random criteria, and not every member of the population has a chance of being included.
Common non-probability sampling methods include convenience sampling , voluntary response sampling, purposive sampling , snowball sampling , and quota sampling .
Probability sampling means that every member of the target population has a known chance of being included in the sample.
Probability sampling methods include simple random sampling , systematic sampling , stratified sampling , and cluster sampling .
Samples are used to make inferences about populations . Samples are easier to collect data from because they are practical, cost-effective, convenient, and manageable.
While a between-subjects design has fewer threats to internal validity , it also requires more participants for high statistical power than a within-subjects design .
Advantages:
- Prevents carryover effects of learning and fatigue.
- Shorter study duration.
Disadvantages:
- Needs larger samples for high power.
- Uses more resources to recruit participants, administer sessions, cover costs, etc.
- Individual differences may be an alternative explanation for results.
In a factorial design, multiple independent variables are tested.
If you test two variables, each level of one independent variable is combined with each level of the other independent variable to create different conditions.
Yes. Between-subjects and within-subjects designs can be combined in a single study when you have two or more independent variables (a factorial design). In a mixed factorial design, one variable is altered between subjects and another is altered within subjects.
Within-subjects designs have many potential threats to internal validity , but they are also very statistically powerful .
- Only requires small samples
- Statistically powerful
- Removes the effects of individual differences on the outcomes
- Internal validity threats reduce the likelihood of establishing a direct relationship between variables
- Time-related effects, such as growth, can influence the outcomes
- Carryover effects mean that the specific order of different treatments affect the outcomes
Quasi-experimental design is most useful in situations where it would be unethical or impractical to run a true experiment .
Quasi-experiments have lower internal validity than true experiments, but they often have higher external validity as they can use real-world interventions instead of artificial laboratory settings.
In experimental research, random assignment is a way of placing participants from your sample into different groups using randomisation. With this method, every member of the sample has a known or equal chance of being placed in a control group or an experimental group.
A quasi-experiment is a type of research design that attempts to establish a cause-and-effect relationship. The main difference between this and a true experiment is that the groups are not randomly assigned.
In a between-subjects design , every participant experiences only one condition, and researchers assess group differences between participants in various conditions.
In a within-subjects design , each participant experiences all conditions, and researchers test the same participants repeatedly for differences between conditions.
The word ‘between’ means that you’re comparing different conditions between groups, while the word ‘within’ means you’re comparing different conditions within the same group.
A confounding variable , also called a confounder or confounding factor, is a third variable in a study examining a potential cause-and-effect relationship.
A confounding variable is related to both the supposed cause and the supposed effect of the study. It can be difficult to separate the true effect of the independent variable from the effect of the confounding variable.
In your research design , it’s important to identify potential confounding variables and plan how you will reduce their impact.
Triangulation can help:
- Reduce bias that comes from using a single method, theory, or investigator
- Enhance validity by approaching the same topic with different tools
- Establish credibility by giving you a complete picture of the research problem
But triangulation can also pose problems:
- It’s time-consuming and labour-intensive, often involving an interdisciplinary team.
- Your results may be inconsistent or even contradictory.
There are four main types of triangulation :
- Data triangulation : Using data from different times, spaces, and people
- Investigator triangulation : Involving multiple researchers in collecting or analysing data
- Theory triangulation : Using varying theoretical perspectives in your research
- Methodological triangulation : Using different methodologies to approach the same topic
Experimental designs are a set of procedures that you plan in order to examine the relationship between variables that interest you.
To design a successful experiment, first identify:
- A testable hypothesis
- One or more independent variables that you will manipulate
- One or more dependent variables that you will measure
When designing the experiment, first decide:
- How your variable(s) will be manipulated
- How you will control for any potential confounding or lurking variables
- How many subjects you will include
- How you will assign treatments to your subjects
Exploratory research explores the main aspects of a new or barely researched question.
Explanatory research explains the causes and effects of an already widely researched question.
The key difference between observational studies and experiments is that, done correctly, an observational study will never influence the responses or behaviours of participants. Experimental designs will have a treatment condition applied to at least a portion of participants.
An observational study could be a good fit for your research if your research question is based on things you observe. If you have ethical, logistical, or practical concerns that make an experimental design challenging, consider an observational study. Remember that in an observational study, it is critical that there be no interference or manipulation of the research subjects. Since it’s not an experiment, there are no control or treatment groups either.
These are four of the most common mixed methods designs :
- Convergent parallel: Quantitative and qualitative data are collected at the same time and analysed separately. After both analyses are complete, compare your results to draw overall conclusions.
- Embedded: Quantitative and qualitative data are collected at the same time, but within a larger quantitative or qualitative design. One type of data is secondary to the other.
- Explanatory sequential: Quantitative data is collected and analysed first, followed by qualitative data. You can use this design if you think your qualitative data will explain and contextualise your quantitative findings.
- Exploratory sequential: Qualitative data is collected and analysed first, followed by quantitative data. You can use this design if you think the quantitative data will confirm or validate your qualitative findings.
Triangulation in research means using multiple datasets, methods, theories and/or investigators to address a research question. It’s a research strategy that can help you enhance the validity and credibility of your findings.
Triangulation is mainly used in qualitative research , but it’s also commonly applied in quantitative research . Mixed methods research always uses triangulation.
Operationalisation means turning abstract conceptual ideas into measurable observations.
For example, the concept of social anxiety isn’t directly observable, but it can be operationally defined in terms of self-rating scores, behavioural avoidance of crowded places, or physical anxiety symptoms in social situations.
Before collecting data , it’s important to consider how you will operationalise the variables that you want to measure.
Hypothesis testing is a formal procedure for investigating our ideas about the world using statistics. It is used by scientists to test specific predictions, called hypotheses , by calculating how likely it is that a pattern or relationship between variables could have arisen by chance.
There are five common approaches to qualitative research :
- Grounded theory involves collecting data in order to develop new theories.
- Ethnography involves immersing yourself in a group or organisation to understand its culture.
- Narrative research involves interpreting stories to understand how people make sense of their experiences and perceptions.
- Phenomenological research involves investigating phenomena through people’s lived experiences.
- Action research links theory and practice in several cycles to drive innovative changes.
There are various approaches to qualitative data analysis , but they all share five steps in common:
- Prepare and organise your data.
- Review and explore your data.
- Develop a data coding system.
- Assign codes to the data.
- Identify recurring themes.
The specifics of each step depend on the focus of the analysis. Some common approaches include textual analysis , thematic analysis , and discourse analysis .
In mixed methods research , you use both qualitative and quantitative data collection and analysis methods to answer your research question .
Methodology refers to the overarching strategy and rationale of your research project . It involves studying the methods used in your field and the theories or principles behind them, in order to develop an approach that matches your objectives.
Methods are the specific tools and procedures you use to collect and analyse data (e.g. experiments, surveys , and statistical tests ).
In shorter scientific papers, where the aim is to report the findings of a specific study, you might simply describe what you did in a methods section .
In a longer or more complex research project, such as a thesis or dissertation , you will probably include a methodology section , where you explain your approach to answering the research questions and cite relevant sources to support your choice of methods.
The research methods you use depend on the type of data you need to answer your research question .
- If you want to measure something or test a hypothesis , use quantitative methods . If you want to explore ideas, thoughts, and meanings, use qualitative methods .
- If you want to analyse a large amount of readily available data, use secondary data. If you want data specific to your purposes with control over how they are generated, collect primary data.
- If you want to establish cause-and-effect relationships between variables , use experimental methods. If you want to understand the characteristics of a research subject, use descriptive methods.
Ask our team
Want to contact us directly? No problem. We are always here for you.
- Chat with us
- Email [email protected]
- Call +44 (0)20 3917 4242
- WhatsApp +31 20 261 6040
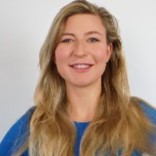
Our support team is here to help you daily via chat, WhatsApp, email, or phone between 9:00 a.m. to 11:00 p.m. CET.
Our APA experts default to APA 7 for editing and formatting. For the Citation Editing Service you are able to choose between APA 6 and 7.
Yes, if your document is longer than 20,000 words, you will get a sample of approximately 2,000 words. This sample edit gives you a first impression of the editor’s editing style and a chance to ask questions and give feedback.
How does the sample edit work?
You will receive the sample edit within 24 hours after placing your order. You then have 24 hours to let us know if you’re happy with the sample or if there’s something you would like the editor to do differently.
Read more about how the sample edit works
Yes, you can upload your document in sections.
We try our best to ensure that the same editor checks all the different sections of your document. When you upload a new file, our system recognizes you as a returning customer, and we immediately contact the editor who helped you before.
However, we cannot guarantee that the same editor will be available. Your chances are higher if
- You send us your text as soon as possible and
- You can be flexible about the deadline.
Please note that the shorter your deadline is, the lower the chance that your previous editor is not available.
If your previous editor isn’t available, then we will inform you immediately and look for another qualified editor. Fear not! Every Scribbr editor follows the Scribbr Improvement Model and will deliver high-quality work.
Yes, our editors also work during the weekends and holidays.
Because we have many editors available, we can check your document 24 hours per day and 7 days per week, all year round.
If you choose a 72 hour deadline and upload your document on a Thursday evening, you’ll have your thesis back by Sunday evening!
Yes! Our editors are all native speakers, and they have lots of experience editing texts written by ESL students. They will make sure your grammar is perfect and point out any sentences that are difficult to understand. They’ll also notice your most common mistakes, and give you personal feedback to improve your writing in English.
Every Scribbr order comes with our award-winning Proofreading & Editing service , which combines two important stages of the revision process.
For a more comprehensive edit, you can add a Structure Check or Clarity Check to your order. With these building blocks, you can customize the kind of feedback you receive.
You might be familiar with a different set of editing terms. To help you understand what you can expect at Scribbr, we created this table:
View an example
When you place an order, you can specify your field of study and we’ll match you with an editor who has familiarity with this area.
However, our editors are language specialists, not academic experts in your field. Your editor’s job is not to comment on the content of your dissertation, but to improve your language and help you express your ideas as clearly and fluently as possible.
This means that your editor will understand your text well enough to give feedback on its clarity, logic and structure, but not on the accuracy or originality of its content.
Good academic writing should be understandable to a non-expert reader, and we believe that academic editing is a discipline in itself. The research, ideas and arguments are all yours – we’re here to make sure they shine!
After your document has been edited, you will receive an email with a link to download the document.
The editor has made changes to your document using ‘Track Changes’ in Word. This means that you only have to accept or ignore the changes that are made in the text one by one.
It is also possible to accept all changes at once. However, we strongly advise you not to do so for the following reasons:
- You can learn a lot by looking at the mistakes you made.
- The editors don’t only change the text – they also place comments when sentences or sometimes even entire paragraphs are unclear. You should read through these comments and take into account your editor’s tips and suggestions.
- With a final read-through, you can make sure you’re 100% happy with your text before you submit!
You choose the turnaround time when ordering. We can return your dissertation within 24 hours , 3 days or 1 week . These timescales include weekends and holidays. As soon as you’ve paid, the deadline is set, and we guarantee to meet it! We’ll notify you by text and email when your editor has completed the job.
Very large orders might not be possible to complete in 24 hours. On average, our editors can complete around 13,000 words in a day while maintaining our high quality standards. If your order is longer than this and urgent, contact us to discuss possibilities.
Always leave yourself enough time to check through the document and accept the changes before your submission deadline.
Scribbr is specialised in editing study related documents. We check:
- Graduation projects
- Dissertations
- Admissions essays
- College essays
- Application essays
- Personal statements
- Process reports
- Reflections
- Internship reports
- Academic papers
- Research proposals
- Prospectuses
Calculate the costs
The fastest turnaround time is 24 hours.
You can upload your document at any time and choose between three deadlines:
At Scribbr, we promise to make every customer 100% happy with the service we offer. Our philosophy: Your complaint is always justified – no denial, no doubts.
Our customer support team is here to find the solution that helps you the most, whether that’s a free new edit or a refund for the service.
Yes, in the order process you can indicate your preference for American, British, or Australian English .
If you don’t choose one, your editor will follow the style of English you currently use. If your editor has any questions about this, we will contact you.
- Open access
- Published: 10 April 2024
An investigation into the present status and influencing factors of nurse retention in grade-a tertiary general hospitals in Shanxi Province within the framework of the magnet hospital concept
- Li-Hong Yue 1 ,
- Lin-Ying Wang 2 ,
- Jin-Li Guo 3 ,
- Wan-Ling Li 2 , 4 &
- Jian-Wei Zhang 1
BMC Health Services Research volume 24 , Article number: 452 ( 2024 ) Cite this article
Metrics details
The attrition of nursing staff significantly contributes to the shortage of healthcare professionals. This study entailed an examination of the propensity of nurses to sustain employment within Grade-A tertiary general hospitals and the various influencing factors.
A total of 2,457 nurses from three grade-A tertiary general hospitals were surveyed. The survey instruments included a general information questionnaire, a scale measuring their willingness to continue working, and a Chinese version of the Magnet Hospital Factor scale.
The scores of the willingness to continue working scale and the Magnet Hospital Factor scale were 21.53 ± 4.52 and 145.46 ± 25.82, respectively. There were statistically significant differences in the scores of willingness of nurses to continue working across various factors, including the department, age, marital status, family location, length of service as nurses, professional title, position, and employment type, upon comparison ( P < 0.001). The correlation analysis showed that there was a positive correlation between the willingness of nurses to continue working and the magnet hospital factors, with a correlation coefficient of 0.523 ( P < 0.01). Regression analysis showed that department, length of service as nurses, professional title, position, average monthly income, number of night shifts, medical care relationship, educational support, and nursing manager support among the magnet hospital factors were important predictors of willingness to continue working ( P < 0.001).
The willingness of nurses to continue working in grade-A tertiary general hospitals in Shanxi Province was determined to be at an upper-middle level. The magnet status of grade-A tertiary general hospitals needs to be improved, and there are many factors that influenced willingness of nurses to continue working. To cultivate a more favorable environment and bolster nurse recruitment and retention, all healthcare institutions should strive to establish a magnet nursing environment, thereby fostering the robust development of the nursing team.
Peer Review reports
As of the conclusion of 2020, China’s ratio of registered nurses per 1,000 individuals stood at 3.34, a figure that remains below the World Health Organization’s 2014 recommendation of maintaining a ratio exceeding 5 registered nurses per 1,000 people. In Norway, the ratio of registered nurses per 1,000 individuals was 17.27, in Japan, it was 11.49, and in the United States, it was 9.8, as reported by Cn-healthcare in 2016 [ 1 ]. The World Health Care 2020 report indicates an anticipated nurse shortage of 5.7 million by the year 2030 [ 2 ]. The shortage of nurses has multifaceted implications, impacting not only the quality of nursing care and patient safety but also the physical and mental well-being of nurses. Nurse resignation stands out as a significant contributor to this shortage. The turnover rate among nurses in China’s tertiary hospitals ranges from 5.8 to 12%. Findings from a survey conducted by China’s Nursing Quality Control Center reveal that 17.86% of nurses express an intention to leave their current positions, with 83% of them considering leaving the nursing profession altogether. Approximately 27% of nurses who have left their positions have transitioned to non-nursing professions, and only 67.89% of nursing graduates choose to pursue a career in nursing [ 3 ], underscoring the need for robust strategies in recruiting and retaining nurses.
The willingness of nurses to continue working was initially introduced by Mowday et al. [ 4 ] in 1979. In 2001, Price standardized this concept, defining it as the inclination of nurses to remain in their roles after a comprehensive evaluation of their present work conditions and future professional development prospects [ 5 ]. Studies have confirmed that increasing the willingness to continue working can increase the retention of nurses and reduce the turnover rate [ 6 , 7 , 8 , 9 ]. Currently, there is a dearth of large-sample empirical studies on the willingness of nurses to continue working in Shanxi Province. Existing research on the influencing factors of this willingness predominantly revolves around demographic, psychological, and organizational factors [ 10 , 11 , 12 , 13 , 14 , 15 , 16 , 17 , 18 , 19 , 20 ]. The concept of a ‘magnet hospital’ was first introduced by McClure et al. in 1981 [ 21 ]. This term was coined to characterize hospitals that demonstrated proficiency in attracting and retaining nursing staff, particularly in the face of elevated nurse turnover rates. Findings from various studies suggest that magnet hospitals have the potential to enhance the practical experience of nurses and mitigate turnover rates [ 22 ]. Therefore, in this study we investigated the willingness of nurses to continue working in grade-A tertiary general hospitals, the highest tier of hospitals in China, located in Shanxi Province. We further explored the influencing factors from the perspective of magnet hospitals, with the objective of fostering nurses’ sustained commitment to their roles. We also sought to delineate the contours of a magnet nursing work environment suitable for Shanxi Province, providing a foundation for enhancing the practical experience of nurses.
Participants and methods
Participants.
The participants in this study were nurses from three grade-A tertiary general hospitals in Shanxi Province. Inclusion criteria: ① A practicing nurse certificate from the People’s Republic of China (PRC); ② Worked in a registered medical and health institution for at least 1 year; ③ No history of severe physical and mental illness or psychological disorder; ④ Informed consent and voluntary participation in the study. Exclusion criteria: ① Under training nurses; ② Pregnant nurses; ③ Absent nurses due to personal leave, sick leave, public holiday, etc.; ④ Rehired nurses after retirement. Nurses were excluded from the study based on specific criteria, including being training nurses who sought professional knowledge and practice without the primary aim of employment, and thus experiencing lower work and psychological pressures than unit employees. Additionally, pregnant nurses, given the physical demands of their work, were excluded due to increased fatigue, which could elevate the likelihood of considering leaving their jobs. Throughout the survey period, the occurrence of factors such as accidental leave, sick leave, and sabbatical leave resulted in the absence of nurses, potentially impacting their responses to the survey questionnaires. The study underwent review by the Hospital Ethics Committee.
Investigation tool
The investigation was carried out through the administration of an electronic questionnaire. The data collection occurred at Shanxi Baiqun Hospital from January 24th to 28th, 2022, at the People’s Hospital of Xinzhou City from February 21st to 22nd, 2022, and at the Second Hospital of Shanxi Medical University from May 17th to May 21st, 2022. The survey design drew significant inspiration from previous studies. In a departmental meeting, the head of the Nursing Department outlined the study’s objectives, content, and provided instructions for completing the questionnaire. The survey QR code was distributed, and participants were informed of the questionnaire deadline. Ensuring anonymity was a paramount consideration in this survey, achieved by omitting any fields for participant names in the questionnaire. ① General information questionnaire: Mainly included age, gender, marital status, educational background, professional title, and working life. ② The scale measuring the willingness of nurses to continue working was translated and revised by Tao and Wang [ 23 ]. Comprising 6 items, the scale utilized Likert’s 5-level scale, incorporating questions such as contemplating leaving the current job, frequency of job-seeking activities, and willingness to quit nursing. A reverse scoring system was applied, and the total score for the scale was calculated as the sum of individual item scores. A higher total score indicated a greater inclination to continue working. The Cronbach’s α coefficient for the scale ranges from 0.742 to 0.759, indicating a high level of reliability and validity [ 20 ]. ③ Chinese version of the magnet factor scale: The scale utilized in this study was initially developed by American scholar Kramer and subsequently revised for localization by Pan et al. [ 24 , 25 ] It encompasses 7 dimensions and comprises 45 items. Employing Likert’s 4-level scale, ranging from “strongly disagree” to “strongly agree,” with corresponding scores of 1–4, the total score for the scale falls within the range of 45–180. A higher score indicates a greater level of the hospital’s magnet factor. The validity of the scale ranges between 0.743 and 0.906, and its internal consistency reliability is reported to be between 0.831 and 0.972.
Investigation methods
For this study, the convenience sampling method was employed to select Shanxi Bethune Hospital, the Second Hospital of Shanxi Medical University, and Xinzhou People’s Hospital. The research group initiated contact with the head of the nursing department at each selected hospital. Following a detailed explanation of the research purpose, content, and questionnaire filling method using standardized instructions, a questionnaire link was sent to the participants through the WJX platform. The designated individual in charge of each hospital disseminated the questionnaire link among the nursing staff, clarifying that the link to the informed consent form could be accessed on the questionnaire homepage. To commence the questionnaire, participants were required to click on the “Informed Consent” section. Throughout the survey, submission of completed questionnaires was limited to a single instance from each unique IP address. All collected information remained confidential, visible solely to the data analyst. A total of 2,464 questionnaires were collected, and following the exclusion of questionnaires demonstrating apparent patterns or logical inconsistencies in responses, 2,457 valid questionnaires were retained. This resulted in an effective recovery rate of 99.72%.
Statistical methods
The data obtained from the WJX platform, were analyzed using SPSS 22.0 statistical software. Measurement data are presented as mean ± standard deviation, and t -tests were employed. Count data are expressed as percentages and analyzed using the chi-squared test. Pearson’s correlation analysis, one-way analysis of variance (ANOVA), and binary logistic regression analysis were utilized. A significance level of P < 0.05 was deemed statistically significant.
General information
There were 103 males and 2,354 females among the 2,457 nurses; ages ranged from 21 to 30 (33.18 ± 6.83) years. In terms of work experience, 123 nurses had worked for ≤ 1 year, 539 nurses had worked for 1–5 years, 841 nurses had worked for 6–10 years, 542 nurses had worked for 11–15 years, 167 nurses had worked for 16–20 years, 83 nurses had worked for 21–25 years, and 162 nurses had worked for ≥ 26 years; There were 1,845 contract nurses and 612 nurses on regular payroll. There were 552 nurses who had no night shift every month, 678 nurses had 1–5 night shifts, 1,075 nurses had 6–10 night shifts, and 152 nurses who had 11 night shifts or more.
Willingness of nurses to continue working and magnet hospital factors score
The total score on the willingness of nurses to continue working scale was 21.53 ± 4.52, with an average item score of 3.59 ± 0.75. The highest individual item score was observed for the question “How often do you seek a new job,” with a score of 4.43 ± 0.82. The detailed scores for each item are presented in Table 1 .
Regarding the magnet hospital factor scale, the total score was 145.46 ± 25.82, and the average score for each item was 3.23 ± 0.11, corresponding to a score rate of 80.81%. Among the dimensions, “cultural values” had the highest score rate at 83.40%, while “nursing practice management” had the lowest score rate at 76.44%. The scores for each dimension are outlined in Table 2 .
Factors affecting willingness of nurses to continue working
Comparison scores of willingness of nurses to continue working with different demographic characteristics.
Significant differences were found in the scores of willingness of nurses to continue working with respect to departments, age, marital status, family location, service experience as nurses, professional title, position, employment method, average monthly income, and the number of night shifts per month (Table 3 ).
Correlation analysis between the score of willingness of nurses to continue working and the score of magnet hospital factors
Pearson’s correlation analysis was performed between scores of the willingness to continue working and the scores of the magnet hospital factors. The results showed a positive correlation, with the correlation coefficient r = 0.523, P < 0.01. There was a positive correlation between the dimensions of the magnet hospital factors and the willingness to continue working, as shown in Table 4 .
Binary logistic regression analysis of willingness of nurses to continue working
In the binary logistic regression analysis, the grouping of scores indicating willingness to continue working was considered the dependent variable. The variables identified as statistically significant in the single-factor analysis of general information and each dimension in the magnet hospital factor scale were utilized as independent variables. The variable assignments are delineated in Table 5 . Dummy variables were established during the analysis, designating the item with assignment = 1 as the control. The finally obtained logistic model was statistically significant. The model could explain 27.9% variation in willingness of nurses to continue working. The results of multivariate analysis are shown in Table 6 .
Status of willingness of nurses to continue working in grade-a tertiary general hospitals in Shanxi Province
In this study, the willingness of nurses to continue working scale exhibited an overall score and average item score of 21.53 ± 4.52 and 3.59 ± 0.75, respectively. These scores surpassed those reported in grade-A tertiary general hospitals in Shanghai and Hangzhou [ 10 , 26 ], as well as those documented in grade-A tertiary general hospitals across China by Zhang et al. [ 11 ] and tertiary traditional Chinese medicine (TCM) hospitals in China by Liu [ 16 ] Furthermore, the scores were higher than those found in male nurses in Shenzhen as investigated by Xu et al. [ 20 ] Nevertheless, the scores were lower than the reported scores of willingness of nurses to continue working during the COVID-19 epidemic investigated by Li [ 27 ] and those in military hospitals in island areas investigated by Wang et al. [ 17 ]. This suggests that the willingness of nurses to continue working in grade-A tertiary general hospitals in Shanxi Province is positioned at an upper-middle level. This observation could be attributed to the consistent advancement of nursing science and the escalating attention from the state, government, and medical institutions toward nurses. Alternatively, it may be associated with the heightened professional pride among the majority of nurses who have been at the forefront, especially during the COVID-19 epidemic. It is advisable for nursing managers to leverage both external and internal motivating factors as foundational elements, drawing insights from advanced practices both within China and internationally. Exploring affirmative strategies to foster the retention of nurses should be a priority in this endeavor.
Magnet level of the grade-A tertiary general hospitals in Shanxi Province
In this study, the total score on the magnet factor scale for Grade-A tertiary general hospitals in the Shanxi area was 145.46 ± 25.82, with a score rate of 80.81%. This score exceeded those reported for Grade-A tertiary general hospitals in the Xinjiang area investigated by Lu et al. [ 28 ]., Grade-A tertiary general hospitals in Bengbu City examined by Lin et al. [ 29 ]., and Grade-A tertiary general hospitals explored by Liu [ 30 ], where new nurses were the research participants. Additionally, it surpassed the scores in a study conducted by Pan et al. [ 25 ] The findings indicated that the score rates for the management dimension of nursing practice and the rational allocation dimension of human resources were low. This aligns with the research outcomes reported by Lu et al. [ 28 ]. It is advisable for nursing managers to prioritize nursing practice, consolidating basic nursing care, and enhancing nurses’ proficiency in risk prediction and specialized nursing skills. Establishing open communication channels, implementing a shared governance model, and ensuring adequate human resources are essential for nursing safety. Medical institutions should strategically allocate human resources based on evaluation criteria and the specific context of Grade-A tertiary general hospitals. Promoting a magnet culture can contribute to the high-quality development of hospitals.
Factors affecting the willingness of nurses to continue working in grade-A tertiary general hospitals in Shanxi Province
Effect of department on nurses’ willingness to continue working.
The study results indicated that departments were significant factors influencing the willingness of nurses to continue working. Nurses in pediatrics and critical care departments exhibited lower willingness to continue working, whereas nurses in other departments demonstrated higher willingness, ranging from 1.5 to 2.2 times that of pediatric nurses ( P < 0.001). This observation may be attributed to the nature of the work within each department and the allocation of human resources. The specific regulations governing the implementation of evaluation standards in Grade-A tertiary general hospitals in Jiangxi Province stipulate that the ratio of pediatric nurses to the actual number of open beds should not be less than 0.6:1. Similarly, the ratio of nurses in intensive medical care departments to the actual number of open beds should be no less than 2.5–3:1. Presently however, many medical institutions face challenges in meeting these requirements, and the bed-to-nursing ratio does not align with the actual clinical conditions [ 31 ], including factors such as bed utilization rates and the number of nurses engaged in non-nursing tasks. The inadequacy of human resources, high workload, responsibilities for pediatric and critically ill patients, and substantial work pressure have contributed to a diminished willingness to continue working to some extent. Hence, it is recommended that nursing managers proactively allocate nurses, tailor the working environment to the specific needs of departments, and implement incentive measures to attract and retain nursing staff.
Longer the working experience as a nurse, the higher the willingness of nurses to continue working
The univariate analysis results revealed significant differences in nurses’ willingness to continue working based on their work experience, indicating a gradual increase in willingness with extended service as nurses. However, the multivariate analysis indicated no significant difference in willingness to continue working between nurses with ≥ 26 years of nursing experience and those with ≤ 1 year of nursing experience. The research results were in line with those of Liu [ 30 ] and Zhuang [ 10 ]. This observation may be associated with the limited professional knowledge of junior nurses and their potential lack of a sense of belonging during rotation training. Conversely, senior nurses may have already acquired substantial subject knowledge and professional skills, along with robust interpersonal communication abilities and enhanced post competency. Research indicates that new nurses experience the greatest work pressure and highest turnover rates within their initial year of employment [ 32 – 33 ]. The research data also indicated that nurses with greater work experience tended to have clearer career planning [ 34 , 35 , 36 ], which positively influenced their willingness to continue working. It is recommended that nursing managers prioritize the professional development of junior nurses and devise personalized retention strategies tailored to their unique characteristics to enhance their willingness to continue working.
High professional title and high position can promote the willingness of nurses to continue working
The study results indicated that the willingness to continue working in the positions of deputy director of senior nurses and director of the nursing division was 1.95 times higher than that of a nurse ( P < 0.001). These findings align with similar research conducted by Xu [ 20 ] on the influencing factors of willingness of male nurses to continue working in Shenzhen and by Li [ 27 ] on the influencing factors of willingness of nurses to continue working during the COVID-19 epidemic. The study revealed that the willingness of teaching nurses to continue working was 1.137 times that of common nurses, while the willingness of head nurses or nursing department directors to continue working was 1.81 times that of common nurses. These results mirror findings reported by Zhang [ 11 ] and Zhuang [ 10 ]. This phenomenon may be attributed to the fact that nurses with high professional titles or positions tend to possess specific professional and academic achievements, enjoy relatively strong work autonomy, and have increased opportunities for training and further education. It is recommended that government bodies and medical and health institutions study and promote exemplary experiences and practices. They should develop a scheme for professional title evaluation and appointment tailored to local conditions, address issues related to the professional titles of nurses, enhance the quality of professional training for junior nurses, demonstrate care for nurses, and create customized career development plans for nurses. This approach aims to facilitate nurse retention based on emotional and visionary considerations.
Income level is an important factor for predicting the willingness of nurses to continue working
The findings indicated that a higher income was associated with a stronger willingness to continue working, aligning with results from various studies, including those by Zhang [ 11 ] and Wei [ 37 ]. This implies that income level is a significant factor in predicting willingness of nurses to continue working. When nurses perceive a lack of proportionality between their efforts and income, they may be inclined to consider leaving their jobs, and a sustained disparity could contribute to higher turnover rates. Medical institutions and nursing managers should adopt a scientific, fair, and impartial approach in developing performance assessment programs. These programs should comprehensively consider factors such as workload, work quality, and work effects, reflecting a principle of rewarding more for higher productivity and outstanding performance. Implementing effective performance and rewards policies can contribute to a more equitable and motivating work environment for nurses.
The lesser the number of night shifts, the higher the willingness of nurses to continue working
The study results demonstrated a negative correlation between the number of night shifts and the willingness of nurses to continue working. Specifically, a smaller number of night shifts was associated with a higher willingness of nurses to continue working. Previous research has shown that involving nurses in the weekly shift arrangement for their department can enhance their working enthusiasm and autonomy [ 38 ]. In light of these findings, nursing managers should consider exploring department-specific night shift patterns. Scientifically determining the number and duration of night shifts and designating a day for nurses working at night as a “nurse sleep day (SD)” without additional requirements for participation in learning and training can contribute to enhancing nurses’ willingness to continue working.
Establishing a magnet nursing workplace can foster the willingness of nurses to continue working
The data presented in Table 4 illustrate that all dimensions of the magnet hospital factor scale exhibit a positive correlation with the willingness to continue working. The correlation coefficient between the magnet hospital level and the willingness to continue working is 0.523. The regression results further indicate that for every 1-point increase in dimensions such as healthcare relationship, education support, nursing manager support, rational allocation of human resources, and cultural values on the magnet hospital factor scale, the willingness of nurses to continue working nearly doubles. The dimension of nursing autonomy exhibited a negative correlation with the willingness to continue working, suggesting a relationship between nursing autonomy and willingness of nurses to continue working, albeit without a regression effect. This may be attributed to the presence of intermediary or confounding variables [ 39 , 40 ]. Further research is needed to explore the interactions between variables and delve into potential mediating or moderating factors.
A magnet hospital is characterized as a facility that has the ability to attract high-quality nurses, akin to a magnet, and offers a practice environment that aligns with both professional and personal values [ 41 ]. The outcomes of this study suggest that the working environment in magnet hospitals positively influences the willingness of nurses to continue working, aligning with the findings of the majority of studies in this area [ 42 , 43 , 44 , 45 , 46 , 47 , 48 ]. Promoting equality, trust, and fostering positive collaborative relationships between doctors and nurses contributes to a favorable working atmosphere and environment for nurses. Departments, nursing departments, and hospitals should actively encourage nurses to engage in continuing education, participate in academic exchanges, and pursue further studies. Providing comprehensive support to nurses in these endeavors can significantly contribute to enhancing their professional quality and confidence. Nursing managers play a pivotal role as the “backbone” of the nursing team. Their focus should be on team building, safeguarding the interests of nurses, making informed decisions through scientific methods, ensuring optimal working conditions, and actively listening to the concerns of nurses. A judicious allocation of human resources is a fundamental prerequisite to ensure the delivery of high-quality care. Currently, in most Grade-A tertiary general hospitals, the proportion of nurses in wards and special departments falls short of established standards. This deficiency inevitably raises the workload for nurses, contributing to an elevated risk of occupational injuries, reduced job satisfaction, heightened job burnout, and an increased turnover rate among nursing staff [ 49 ]. The rational allocation of human resources should take into account the workload, considering factors such as professional titles and job levels. Utilizing flexible scheduling is essential to ensure that nurses have the necessary energy to effectively carry out clinical work. Hospital culture is a composite of widely acknowledged ideologies, value systems, and cultural forms. It encompasses the collective values, beliefs, and behavioral norms shared by the staff within the hospital [ 50 ]. An exemplary hospital culture can significantly bolster the sense of belonging and cohesion among employees, holding profound significance for the sustained development of the hospital. Medical institutions and nursing managers should prioritize the strengthening of cultural development, disseminate the values of the hospital and nursing culture, and integrate these cultural elements into the personal values of nurses.
Research limitations and deficiencies
The study participants were drawn from Taiyuan and Xinzhou City, and it is acknowledged that there may be limitations in the representation and homogeneity of the sample. This potential lack of diversity could introduce a certain degree of bias to the research results, consequently limiting the generalizability and applicability of the findings. Furthermore, among the influencing factors considered, the analysis was limited to basic information and the impact of the magnet hospital level on the willingness of nurses to continue working. Factors such as psychological resilience, psychological pressure, and job embeddedness were not included in the analysis, and there was no in-depth exploration of how these factors might influence the willingness of nurses to continue working. This suggests a potential gap in the comprehensive understanding of the various elements influencing the willingness of nurses to continue working.
Given the ongoing shortage of nurses, their willingness to continue working in Grade-A tertiary general hospitals in Shanxi Province was found to be at an upper-middle level. Various factors influenced the willingness of nurses to continue working, with the magnet hospital level showing a positive correlation with this willingness. To elevate the magnet status of these hospitals, initiatives should prioritize optimizing income structures, nurturing medical care relationships, and enhancing educational and managerial support systems. Additionally, strategic emphasis should be placed on the prudent allocation of human resources, the promotion of cultural values, and the establishment of customized magnet nursing environments. Innovative adaptations to the nursing working environment, tailored to the distinctive characteristics of the region, are recommended to effectively enhance the willingness of nurses to continue working, thereby ensuring the stability of the nursing team.
Data availability
All data generated or analysed during this study are included in this article. Further enquiries can be directed to the corresponding author.
Abbreviations
People’s Republic of China
One-way analysis of variance
Traditional Chinese medicine
CN-HEALTHCARE. China has a shortage of one million nurses, accounting for only one-fifth of the world’s total population. [EB/OL]. https://www.cn-healthcare.com/article/20160520/content-483148.html
Malone B. A perspective on the state of the World’s nursing report. Nurs Adm Q. 2021;45(1):6–12. https://doi.org/10.1097/NAQ.0000000000000443
Article PubMed Google Scholar
Leng Changyu. Reasons and countermeasures for nursing talent loss in China. Health Career Educ. 2017;35(3):149–50.
Google Scholar
Mowday RT, Steers RM, Porter LW. The measurement of organizational commitment. J Vocat Behav. 1979;14(2):224–47.
Article Google Scholar
Price JL. Reflections on the determinants of voluntary turnover. Int J Manpow. 2001;22(7):600–24.
Wang L, Tao H, Bowers BJ, Brown R, Zhang Y. When nurse emotional intelligence matters: how transformational leadership influences intent to stay. J Nurs Manag. 2018;26(4):358–65. https://doi.org/10.1111/jonm.12509
Brown P, Fraser K, Wong CA, Muise M, Cummings G. Factors influencing intentions to stay and retention of nurse managers: a systematic review. J Nurs Manag. 2013;21(3):459–72. https://doi.org/10.1111/j.1365-2834.2012.01352.x
Kaewboonchoo O, Yingyuad B, Rawiworrakul T, Jinayon A. Job stress and intent to stay at work among registered female nurses working in Thai hospitals. J Occup Health. 2014;56(2):93–9. https://doi.org/10.1539/joh.12-0204-oa
Vian T, White EE, Biemba G, Mataka K, Scott N. Willingness to pay for a maternity Waiting Home stay in Zambia. J Midwifery Womens Health. 2017;62(2):155–62. https://doi.org/10.1111/jmwh.12528
Zhuang Y, Tian BJ, Wang Q, Yang XL. The level and determinants of nurse retention in first-class tertiary hospitals in Shanghai. J Nurs Sci. 2020;05:9–12.
Zhang XM, Zhang YQ, Wen FL, Li XY, Fang ZY. Investigation on nurses’ willingness to stay in Tertiary hospitals. Military Nurs. 2019;11:46–9.
Xue T, Jiang WB, Lu XH, Chen Q, Wang SY, Ma MD, Jiang YM. The influence of humanistic management atmosphere on nurses′ intention to stay. Chinese Journal of Hospital Administration. 2020,02:160-161-162-163-164.
Tang LM, Jiang QQ, He Y, Tian SZ. Correlations among perceived professional benefits,job embeddedness and intent to stay in nurses of a Class III hospital. Chin J Mod Nurs. 2019;32:4203–8.
Wang H, Ye ZH, Pan HY. Investigation of relationships among nurse work environment, organizational commitment and intent to stay. Chin J Hosp Adm. 2019;05:420–5.
Wang JJ, Fu NN, Wang JW, Bu XM. Status quo of research on the influence of nurses’willingness to stay from the perspective of psychological empowerment. Chin J Mod Nurs. 2020;29:4118–22.
Liu J, Wen FL, Zhang YQ. Investigation and influencing factors of nurses’ willingness to stay at work in Class III hospitals of Chinese medicine. Chin J Mod Nurs. 2021;11:1458–63.
Wang Q, Wang L. Relationships among Perceived Organizational Support, Psychological Capital and Intention to stay of nurses in a Military Hospital in Island Area. Military Nurs. 2021;05:21–4.
Wang XF, Xu MJ, Di J. Survey on the status of ICU nurses’ intention to stay and analysis of its influencing factors. Chin J Emerg Crit Care Nurs. 2021;03:257–62.
Xue T, Jiang WB, Chen Q, Cui MY, Liu MY, Jiang YM. Mediating Effect of Sub-health on Occupational Adaptation and Retention Intention in New Nurse. J Nursing(China). 2021;09:48–53.
Xu SH, Wu HP, Wu QP, Zhu YF, Song HZ. Current status and influencing factors of male nurses’ intention to stay in Shenzhen. J Qilu Nurs. 2020;09:74–7.
Hoffman M. Magnet hospitals: Attraction and Retention of Professional nurses. Aorn J ournal. 1983;38(3):456.
Yang J, Chen F, Li LT, Cheng L. The application status of the advantages and concepts of magnet hospitals in the domestic hospital. J Nurs Adm. 2019;05:309–13.
Tao H, Wang L. Establishment of questionnaire for nurse intention to remain employed: the Chinese version. Acad J Naval Med Univ. 2010;08:925–7.
Pan YS. To translate and apply the Chinese version of the magnetic elements Scale. Journal of Qingdao University(Medical Sciences); 2019.
Pan YS, Yin CL, Jiang WB, Song L, Zhang Y, Wu Y, Wang Q, Wei LL, Chen K, Wang SY. The current status and influencing factors of magnetic level in tertiary general hospitals. Chin Nurs Manage. 2019;02:225–30.
Qian Y. Study on retention strategies of nurses in tertiary hospitals. Journal of Zhejiang University(Medical Sciences); 2017.
Li DM, Zhao XM. Study on present situation and influencing factors of nurses’intent to stay during the COVID-19 epidemic. Chin J Hosp Stat. 2021;28(1):4.
Lu CC, Zuo PX, Nu Y. Hospital Magnet status and its influencing factors in first-class tertiary hospitals in Xinjiang. J Nurs Sci. 2021;14:73–5.
CAS Google Scholar
Lin X, Wang F. Current situation and influencing factors of magnetic level in a Class III Grade A hospital in Bengbu. J Mudanjiang Med Univ. 2020;02:165–9.
Liu WJ. Current status and influencing factors of new nurses’ intention to stay during role transition from the perspective of magnetic hospital. J Qingdao University(Medical Sciences). 2020.
Park SH, Gass S, Boyle DK. Comparison of reasons for nurse turnover in Magnet® and Non-magnet hospitals. J Nurs Adm. 2016;46(5):284–90. https://doi.org/10.1097/NNA.0000000000000344
Zhang Y, Wu J, Fang Z, Zhang Y, Wong FK. Newly graduated nurses’ intention to leave in their first year of practice in Shanghai: a longitudinal study [published correction appears in Nurs Outlook. 2018]. Nurs Outlook. 2017;65(2):202–11. https://doi.org/10.1016/j.outlook.2016.10.007
Zhang YY, Zhang YQ, Qian P, et al. Occupational stress among new graduate nurses during the first year of transition:a longitudinal study. Chin Nurs Manage. 2017;17(11):1517–21.
Cai TT. Investigation and intervention study on role adaptation of new nurses from the perspective of organizational socialization. J Hangzhou Normal Univ. 2017.
Duchscher JB. A process of becoming: the stages of new nursing graduate professional role transition. J Contin Educ Nurs. 2008;39(10):441–80. https://doi.org/10.3928/00220124-20081001-03
ten Hoeve Y, Jansen G, Roodbol P. The nursing profession: public image, self-concept and professional identity. A discussion paper. J Adv Nurs. 2014;70(2):295–309. https://doi.org/10.1111/jan.12177
Wei W, Zheng Q, Zhou GH, Liu L, Lu CX. Investigation of influencing factors and turnover intervention of Chief Resident Nurse. Military Nurs. 2015;32(21):4.
Wright C, McCartt P, Raines D, Oermann MH. Implementation and evaluation of self-scheduling in a Hospital System. J Nurses Prof Dev. 2017;33(1):19–24. https://doi.org/10.1097/NND.0000000000000324
Hu JR. The relation of Socioeconomic Status and Functional Health of Chinese Elderly people: Physic Exercises and Health Insurance as Mediator. J Univ Sci Technol Beijing(Social Sci Edition). 2019;35(4):9.
Liu W, Shen HY, Wang XY, Pan Y. Relationship between attachment and emotional experience in psychological counselors:Empathy as a Mediator. Chin J Clin Psychol. 2014;03:552–4.
Lasater KB, Richards MR, Dandapani NB, Burns LR, McHugh MD. Magnet hospital recognition in hospital systems over time. Health Care Manage Rev. 2019;44(1):19–29. https://doi.org/10.1097/HMR.0000000000000167
Article PubMed PubMed Central Google Scholar
Zhao J, Jiang X. Application of magnetic hospital management concept in neurosurgery operating room management. J Nurs Adm. 2020;10:753–6.
Liu ZY, Zhu YL, Mao YN, Yin HM. To explore the effects of magnetic hospital management mode on job burnout, coping style and nursing quality of nurses in operating room. J Qilu Nurs. 2021;12:166–7.
Wu YW, Mao JF, Wang L, et al. Study of nurses’ evaluation of the magnetism nursing work environment and their quit intention in Tertiary hospitals. Chin Hosp Manage. 2018;05:64–6.
Li HH, Xing ZJ, Li Y, et al. Research progress on intervention of nurses’ intention to stay at work. Chin J Nurs. 2017;08:1007–9.
Wang Y, Zeng TY, Liu Y, et al. Construction and practice of magnetic nursing workplace. J Nurs Sci. 2019;01:52–6.
Stone L, Arneil M, Coventry L, et al. Benchmarking nurse outcomes in Australian Magnet® hospitals: cross-sectional survey. BMC Nurs. 2019;18:62. https://doi.org/10.1186/s12912-019-0383-6 . Published 2019 Dec 3.
Article CAS PubMed PubMed Central Google Scholar
Budin WC, Brewer CS, Chao YY, Kovner C. Verbal abuse from nurse colleagues and work environment of early career registered nurses. J Nurs Scholarsh. 2013;45(3):308–16. https://doi.org/10.1111/jnu.12033
Marć M, Bartosiewicz A, Burzyńska J, Chmiel Z, Januszewicz P. A nursing shortage - a prospect of global and local policies. Int Nurs Rev. 2019;66(1):9–16. https://doi.org/10.1111/inr.12473
Yin L, Lu QJ, Peng LL, et al. Bibliometric analysis of research hotspots and evolution trends of public hospital culture in China. Chin Hosp. 2022;26(06):68–70.
Download references
Acknowledgements
We are particularly grateful to all the people who have given us help on our article.
This study was funded by the Shanxi Bethune Hospital 2021 Hospital-level Nursing Research Project(2021YH05).
Author information
Authors and affiliations.
Department of Infection, Tongji Shanxi Hospital, Shanxi Bethune Hospital, Shanxi Academy of Medical Sciences, Third Hospital of Shanxi Medical University, 030032, Taiyuan, China
Li-Hong Yue & Jian-Wei Zhang
Department of Nursing, Tongji Shanxi Hospital, Shanxi Bethune Hospital, Shanxi Academy of Medical Sciences, Third Hospital of Shanxi Medical University, No.99 of Longcheng Street, Xiaodian District, 030032, Taiyuan, China
Lin-Ying Wang & Wan-Ling Li
Department of Nursing, Second Hospital of Shanxi Medical University, 030001, Taiyuan, China
Department of Geriatrics, Tongji Hospital, Tongji Medical College, Huazhong University of Science and Technology, No.1095 of Jiefang Avenue, Qiaokou District, 430030, Wuhan, China
Wan-Ling Li
You can also search for this author in PubMed Google Scholar
Contributions
Lin-Ying Wang, Wan-Ling Li conceived the idea and conceptualised the study. Jin-Li Guo, Jian-Wei Zhang, Wan-Ling Li collected the data. Lin-Ying Wang, Jin-Li Guo analysed the data. Li-Hong Yue, Lin-Ying Wang drafted the manuscript. Li-Hong Yue, Wan-Ling Li reviewed the manuscript. All authors read and approved the final draft.
Corresponding author
Correspondence to Wan-Ling Li .
Ethics declarations
Ethics approval and consent to participate.
The study was conducted in accordance with the Declaration of Helsinki(as was revised in 2013). The study was approved by Ethics Committee of the Shanxi Bethune Hospital (NO.YXLL-2022-015). Written informed consent was obtained from all participants.
Consent to publish
Not applicable.
Competing interests
The authors declare no competing interests.
Additional information
Publisher’s note.
Springer Nature remains neutral with regard to jurisdictional claims in published maps and institutional affiliations.
Rights and permissions
Open Access This article is licensed under a Creative Commons Attribution 4.0 International License, which permits use, sharing, adaptation, distribution and reproduction in any medium or format, as long as you give appropriate credit to the original author(s) and the source, provide a link to the Creative Commons licence, and indicate if changes were made. The images or other third party material in this article are included in the article’s Creative Commons licence, unless indicated otherwise in a credit line to the material. If material is not included in the article’s Creative Commons licence and your intended use is not permitted by statutory regulation or exceeds the permitted use, you will need to obtain permission directly from the copyright holder. To view a copy of this licence, visit http://creativecommons.org/licenses/by/4.0/ . The Creative Commons Public Domain Dedication waiver ( http://creativecommons.org/publicdomain/zero/1.0/ ) applies to the data made available in this article, unless otherwise stated in a credit line to the data.
Reprints and permissions
About this article
Cite this article.
Yue, LH., Wang, LY., Guo, JL. et al. An investigation into the present status and influencing factors of nurse retention in grade-a tertiary general hospitals in Shanxi Province within the framework of the magnet hospital concept. BMC Health Serv Res 24 , 452 (2024). https://doi.org/10.1186/s12913-024-10945-w
Download citation
Received : 22 March 2023
Accepted : 02 April 2024
Published : 10 April 2024
DOI : https://doi.org/10.1186/s12913-024-10945-w
Share this article
Anyone you share the following link with will be able to read this content:
Sorry, a shareable link is not currently available for this article.
Provided by the Springer Nature SharedIt content-sharing initiative
- Grade-A tertiary general hospitals
- Influencing factor
- Magnet hospital
- Willingness to continue working
BMC Health Services Research
ISSN: 1472-6963
- General enquiries: [email protected]

An official website of the United States government, Department of Justice.
Here's how you know
Official websites use .gov A .gov website belongs to an official government organization in the United States.
Secure .gov websites use HTTPS A lock ( Lock A locked padlock ) or https:// means you’ve safely connected to the .gov website. Share sensitive information only on official, secure websites.
Examining Financial Fraud Against Older Adults
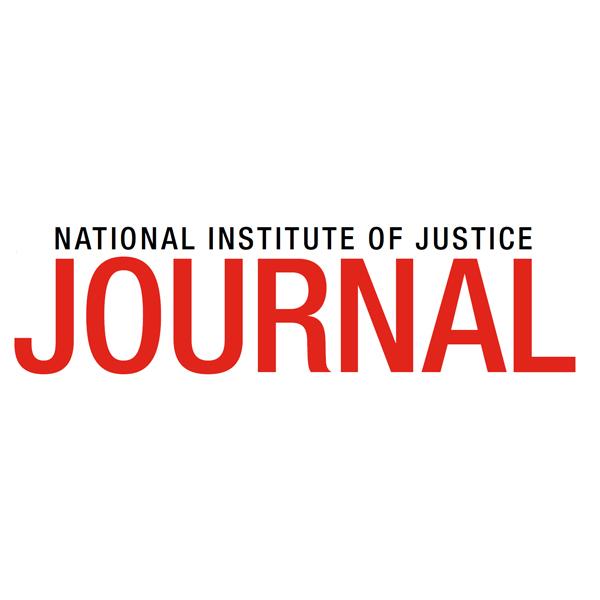
The population of older adults has expanded. The percentage of persons age 60 or older in the United States increased by 33% from 2010 to 2020 and is expected to continue to grow. [1]
The Better Business Bureau reports that older adults [2] lose more than $36 billion to financial fraud every year. [3] According to the FBI’s Internet Crime Complaint Center, 105,301 cases of fraud against persons age 60 or older were reported in 2020. [4] In 2021, 128,216 offenses against persons age 65 or older were reported through the National Incident-Based Reporting System. [5] The actual number of fraud cases is unknown as many people do not report their victimization, and underreporting is especially high for older adults. [6]
Despite these facts, there is a lack of research on fraud victimization of older adults. To date, studies that examine financial fraud have not been nationally representative, suffer from small sample sizes, or only include victims who make a formal report. Some studies use varying definitions of fraud, which may or may not include identity theft. Additionally, these studies may have other methodological limitations that lead to a wide range in prevalence estimates.
See sidebar, “What Is the Difference Between Financial Fraud and Identity Theft?”
To help fill this gap in the literature, this article presents findings from a nationally representative sample of persons age 60 or older who experienced personal financial fraud. Data came from the 2017 National Crime Victimization Survey (NCVS) Supplemental Fraud Survey (SFS).
Review of the Literature
Financial exploitation of older adults — which generally includes improper use of funds, property, or resources of another individual — can be divided into two main categories: financial abuse and financial fraud. Individuals who know the victim and are in positions of trust (for example, family members or paid caregivers) commit financial abuse of older adults, which is also referred to as elder financial abuse. Strangers mainly commit financial fraud of older adults. [7] Most research to date has focused on financial abuse rather than financial fraud, [8] although some studies include both forms of financial exploitation. This article looks only at financial fraud.
Changes in the aging brain and declines in cognitive functioning (ranging from mild impairments to Alzheimer’s disease and dementia) make older adults more susceptible to scam and fraud. [9] Other risk factors include a lack of financial literacy, [10] social isolation, and loneliness. [11] Older adults also tend to be more trusting than younger adults and less able to recognize deceitful individuals. [12]
Research has consistently found that older adults are more likely to be targets of fraud than younger adults. [13] However, this does not necessarily mean a greater number of older adults are victims of financial fraud. [14] The 2016 Health and Retirement Study found that 34.8% of persons age 50 or older had been targeted by or had been the victim of a fraud or investment scam in the past five years. [15] Estimates of financial fraud victimization of older adults differ by the population studied, the definition of fraud used, [16] and the time frame considered; however, across studies, between 2.7% and 6.6% of older adults reported experiencing financial fraud in the past 12 months. [17]
The consequences of fraud victimization may be more severe for older adults than younger adults. Research found that they lose more money, on average, than younger victims. [18] Financial fraud of older adults is rarely handled through the criminal justice system. Older fraud victims are unlikely to report the incident to the police. Prosecutors and law enforcement may be less interested in pursuing legal action when the victim is an older adult, especially one who has cognitive difficulties. [19] Other correlates of financial victimization include poor psychological well-being, [20] depression, post-traumatic stress disorder, generalized anxiety disorder, poor overall health, [21] and lower quality of life, [22] although the directionality of these relationships is not always known. Although higher rates of fraud are correlated with mental and physical health problems, experiencing fraud does not necessarily cause negative health outcomes. However, issues with physical and mental health could make individuals more vulnerable to fraud victimization.
Data and Methods
This analysis used data from the Bureau of Justice Statistics’ (BJS) 2017 NCVS SFS. BJS is the nation’s primary source for criminal justice statistics, and the NCVS is the nation’s primary source of information on criminal victimization. Each year, the NCVS collects data from a nationally representative sample of approximately 240,000 persons in about 150,000 households. The NCVS collects information on nonfatal personal and property crimes reported and not reported to police, including data on the victim, the person who perpetrated the crime, [23] and incident characteristics. [24]
From October through December 2017, BJS administered the SFS to a nationally representative sample of persons age 18 or older in NCVS-sampled households. All NCVS and SFS interviews used computer-assisted personal interviewing, either by telephone or in person. Of the 66,200 NCVS-eligible respondents age 18 or older, approximately 51,200 completed the SFS questionnaire, representing a response rate of 77.3%.
The SFS collected individual-level data on the prevalence of seven types of fraud victimization: charity, consumer investment, consumer products and services, employment, phantom debt, prize and grant, and relationship and trust. Prevalence is defined as the number or percentage of unique persons who were victims of fraud at least once during the reference period. The SFS asked respondents whether they experienced the different types of fraud in the 12 months prior to the interview. [25]
The SFS instrument began with a series of questions on these seven types of fraud; these questions screened the respondent into the survey if they reported experiencing one or more eligible types of fraud victimization. Once a respondent screened in, the interviewer administered the SFS incident instrument to collect detailed information about the type of fraud victimization experienced. The incident instrument also collected data on the characteristics of victims and their patterns of reporting to the police and other authorities.
In addition to reporting a fraud victimization in the screener questions, respondents were classified as fraud victims if they reported that they did not get their money back in the transaction. This criterion fits the legal definition of fraud and provided sufficient sample sizes to produce statistical estimates.
If respondents reported experiencing more than one incident of the same type of fraud, the SFS asked them to think about the most recent incident that occurred in the last 12 months. This article defines older adults as persons age 60 or older, which is consistent with the Elder Abuse Prevention and Prosecution Act of 2017 and Older Americans Act of 1965. [26]
In 2017, about 1.33% (929,570) of persons age 60 or older experienced at least one incident of fraud ( see exhibit 1 ). There were no statistically significant differences between the percentage of persons age 60 or older and persons age 59 or younger who experienced fraud. This pattern held when examining by fraud type both for persons age 60 or older and for persons age 59 or younger.
Exhibit 1. Percentage of persons who experienced at least one incident of personal financial fraud in the past 12 months, by type of fraud and age of person, 2017.
Source: Bureau of Justice Statistics, National Crime Victimization Survey, Supplemental Fraud Survey, 2017.
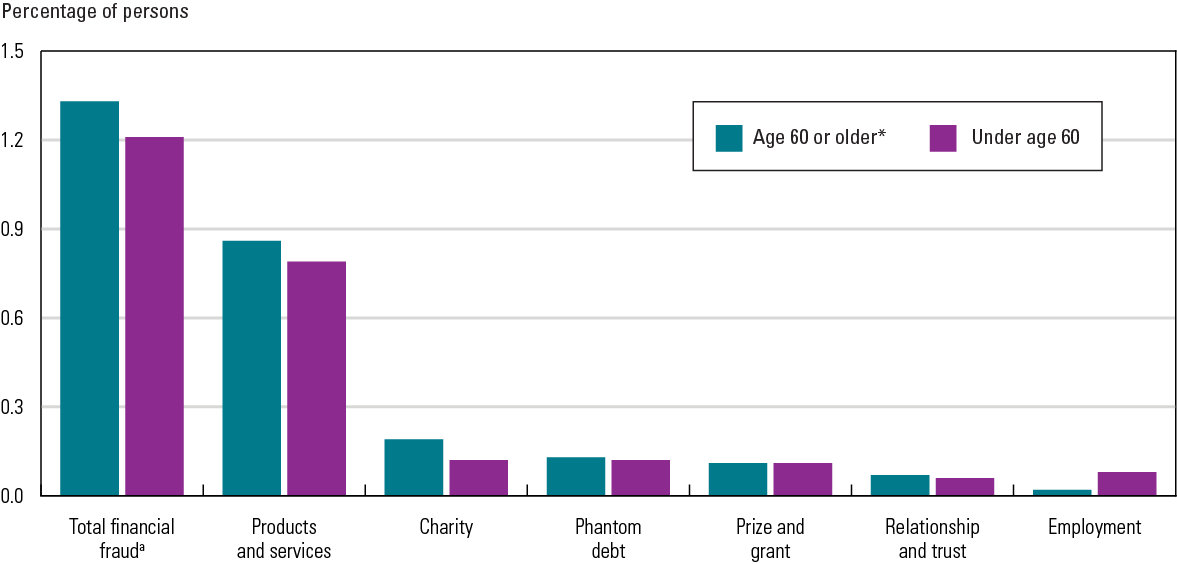
Note: Estimates are based on the most recent incident for that fraud type. * Comparison group. a Consumer investment fraud is excluded due to too few sample cases, but it is included in total financial fraud.
For estimates and standard errors, see appendix tables 1a and 1b .
Regardless of victim age, the most common type of fraud was consumer products and services fraud; about 65% of all fraud victims experienced it ( see exhibit 2 ). Technology support scams, automotive repair scams, weight-loss product scams, and online marketplace scams are common examples of this fraud type.
Exhibit 2. Number and percentage of victims who experienced personal financial fraud, by type of fraud and age of victim, 2017.
Note: Estimates are based on the most recent incident for that fraud type. Numbers and percentages of victims do not sum to totals because persons could experience multiple types of fraud. * Comparison group. † Significant difference from comparison group at the 95% confidence level. ‡ Significant difference from comparison group at the 90% confidence level. ! Interpret estimate with caution. Estimate is based on 10 or fewer sample cases, or coefficient of variation is greater than 50%. a Consumer investment fraud is excluded due to too few sample cases, but it is included in total financial fraud.
For standard errors, see appendix table 2 .
Data from the 2017 SFS show that 79.7% of fraud victims age 60 or older were non-Hispanic white persons, a significantly higher percentage than the 62.0% of all fraud victims who were non-Hispanic white persons ( see exhibit 3 ). The percentage of victims age 60 or older who were never married (8.1%) was lower than the percentage of all victims who were never married (31.1%). However, the percentage of victims age 60 or older who were widowed (22.0%) was significantly higher than the percentage of all victims who were widowed (8.0%). There were no statistically significant differences by victim sex, household income, or location of residence in the percentage of victims of financial fraud of any age and those age 60 or older.
Exhibit 3. Percentage of all victims and victims age 60 or older who experienced personal financial fraud, by demographic characteristics, 2017.
Notes: Estimates are based on the most recent incident of fraud. Details may not sum to totals due to rounding. * Comparison group. † Significant difference from comparison group at the 95% confidence level. ‡ Significant difference from comparison group at the 90% confidence level. a There were no victims of personal financial fraud age 60 or older who were American Indian or Alaska Native and Native Hawaiian or Other Pacific Islander. b Excludes persons of Hispanic origin (e.g., “white” refers to non-Hispanic white persons and “Black” refers to non-Hispanic Black persons). c Includes persons who were Asian; Native Hawaiian or Other Pacific Islander; American Indian or Alaska Native; and two or more races. Categories are not shown separately due to small numbers of sample cases. d Within the principal city of a Metropolitan Statistical Area (MSA). e Within an MSA but not in a principal city of the MSA. f Not within an MSA.
For standard errors, see appendix table 3 .
Victims may not report a crime for a variety of reasons, including fear of reprisal or getting the person who perpetrated the crime in trouble, believing that nothing could or would be done to help, and believing the crime to be a personal issue or too trivial to report. About 1 in 5 (19%) fraud victims age 60 or older reported the incident to the police, and 84% of victims age 60 or older reported the incident to another person or group ( see exhibit 4 ). Other people or groups that the victim may report the incident to include their family or friends; a bank, credit card company, or other payment provider; a state or local consumer agency; a lawyer; or a federal consumer agency.
Exhibit 4. Percentage of financial fraud victims age 60 or older who reported to police or other persons or groups, 2017.
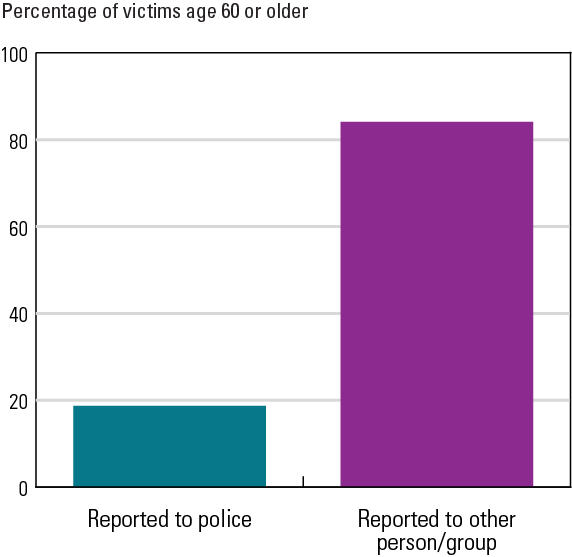
For standard errors and confidence intervals, see appendix tables 4a and 4b .
In total, fraud victims age 60 or older lost nearly $1.2 billion in 2017, and they lost an average of $1,270 ( see exhibit 5 ). More than half (65%) of the total losses resulted from consumer products and services fraud. On average, consumer products and services fraud victims age 60 or older lost about $1,190, which was significantly more money than the amount lost by victims of charity fraud ($60).
Exhibit 5. Financial losses among victims age 60 or older who experienced at least one incident of personal financial fraud in the past 12 months, by type of fraud, 2017.
Note: Estimates are based on the most recent incident of that fraud type. Details may not sum to totals due to rounding. * Comparison group. † Significant difference from comparison group at the 95% confidence level. a The percentage of victims who experienced one type of fraud multiple times during the reference period varied from 1% to 6%. To account for these losses, the average loss for each type of fraud was added to the amount lost by the victim in the most recent incident and then added to total losses. b Total financial losses are expected to be greater than the amounts shown in this table due to top coding, a procedure used to protect respondents from disclosure risk. c Employment and investment fraud are excluded due to too few sample cases, but they are included in total financial fraud. Relationship and trust fraud is excluded due to unreliability of the estimate, but it is included in total financial fraud.
For standard errors, see appendix table 5 .
Those who lost the average amount of $1,270 or less were significantly less likely to report the incident to the police (13%) than those who lost more than $1,270 (47%). Victims who lost $1,270 or less were also significantly less likely to report the fraud to another person or group (82%) compared to victims who lost more than $1,270 (95%).
Fraud victims experience different socioemotional consequences. About a third (31%) of victims age 60 or older experienced moderate emotional distress ( see exhibit 6 ). About 29% experienced mild distress and 27% experienced severe distress. One in 20 (5%) fraud victims age 60 or older reported experiencing relationship problems with friends or family because of the incident.
Exhibit 6. Percentage of victims age 60 or older who experienced socioemotional problems as a result of personal financial fraud, 2017.
Note: Details may not sum to total because victims could experience emotional distress and family/friend relationship problems. Excludes missing data, which accounted for 2% of fraud incidents. a Includes experiencing significant problems with family or friends, such as having more arguments than before the victimization, an inability to trust, or not feeling as close after victimization.
For standard errors and confidence intervals, see appendix table 6 .
Implications and Conclusions
Prior research and data on fraud are limited by issues such as small sample sizes, nonrepresentative samples, and variations in the definition of fraud and types of crimes included. To date, many of the data have relied on statistics collected by the FBI. Despite limitations, these data are a useful source of information because the FBI consistently collects them, which provides an opportunity to report on trends over time. The FBI also collects data on the severity of the problem and types of fraud targeting older adults. Data from the FBI Internet Crime Complaint Center, for example, show that financial fraud of older adults is a growing problem both in terms of number of incidents reported and total dollars lost. [27]
However, based on the SFS data analyzed in this article, we know that statistics collected by law enforcement do not capture the complete picture. The SFS aims to address the need for nationally representative estimates of fraud, both reported and not reported to the police. The SFS complements the FBI data sources by including victims who do not report to the police. Additionally, the SFS reveals that the demographic profile of financial fraud victims age 60 or older differs from the profile of fraud victims age 18 or older. Future research on older adults who do not report their victimizations will also provide a more comprehensive picture of fraud in the United States.
Additional areas of research offer opportunities to examine the evolving nature of fraud victimization, including the intersection of fraud and cybercrime, and types of fraud that target older adults. These gaps in knowledge about financial fraud of older adults should be addressed through research in the future.
Sidebar: What Is the Difference Between Financial Fraud and Identity Theft?
The Bureau of Justice Statistics defines and measures financial fraud and identity theft separately and collects data on each crime through separate National Crime Victimization Survey supplemental surveys. The primary distinction between the two crimes is whether respondents willingly provided personal information to the person who perpetrated the crime.
In the case of identity theft, victims’ personal information (for example, bank account information or Social Security number) is obtained and used without permission. For an incident to be classified as identity theft, victims must experience the misuse of an existing account, opening of a new account, or the misuse of personal information. Identity theft is like other types of theft, whereby victims’ information is taken without their knowledge, consent, or control. For more information, see https://bjs.ojp.gov/data-collection/identity-theft-supplement-its .
For personal financial fraud — the focus of this article — victims willingly provide personal information but are deceived about what they will receive in return for that information. For an incident to be classified as a personal financial fraud, victims must be knowingly and intentionally deceived and lose money in the transaction. For more information, see https://bjs.ojp.gov/data-collection/supplemental-fraud-survey-sfs .
Return to text.
Appendix Tables
Appendix tables 1a and 1b. estimates and standard errors for exhibit 1..
Note: Estimates are based on the most recent incident for that fraud type. Numbers and percentages of victims do not sum to totals because persons could experience multiple types of fraud. Standard errors were generated using generalized variance function parameters. * Comparison group. ! Interpret with caution. Estimate is based on 10 or fewer sample cases, or coefficient of variation is greater than 50. a Consumer investment fraud is excluded from this table due to too few sample cases but is included in total financial fraud.
Appendix table 2. Standard errors for Exhibit 2.
Note: Standard errors were generated using generalized variance function parameters. ~ Not applicable. Source: Bureau of Justice Statistics, National Crime Victimization Survey, Supplemental Fraud Survey, 2017.
Appendix table 3. Standard errors for Exhibit 3.
Note: Standard errors were generated using generalized variance function parameters. Source: Bureau of Justice Statistics, National Crime Victimization Survey, Supplemental Fraud Survey, 2017.
Appendix tables 4a and 4b for Exhibit 4.
Note: Estimates are based on the most recent incident of fraud. Percentages do not sum to totals because persons could report to both police and another person or group. Standard errors were generated using generalized variance function parameters.
Appendix table 5. Standard errors for Exhibit 5.
Note: Standard errors for means and total losses were calculated using direct variance estimation methods.
Appendix table 6. Estimates and standard errors for Exhibit 6.
Note: Standard errors were generated using generalized variance function parameters.
[note 1] Administration on Aging (AoA), 2021 Profile of Older Americans , Washington, DC: U.S. Department of Health and Human Services, 2022.
[note 2] Ages of older adults in the research range from a low of 50 (AARP) to a high of 65 (Census). This report defines older adults as persons age 60 or older. This is consistent with the Elder Abuse Prevention and Prosecution Act of 2017 and Older Americans Act of 1965 [42 USC §3002(38)].
[note 3] American Advisors Group (AAG), “ AAG and Better Business Bureau Expand Fight Against Senior Targeted Financial Fraud ,” March 21, 2019, Cision .
[note 4] Internet Crime Complaint Center, Elder Fraud Report: 2020 , Washington, DC: U.S. Department of Justice, Federal Bureau of Investigation, Internet Crime Complaint Center, 2021.
[note 5] Bureau of Justice Statistics, based on data from the Federal Bureau of Investigation, National Incident-Based Reporting System, “ Data year: 2021, Offense category: Fraud offenses, Unit of analysis: Count, Victim age: Age 65 or older ,” accessed April 7, 2023.
[note 6] Jingjin Shao et al., “ Why Are Older Adults Victims of Fraud? Current Knowledge and Prospects Regarding Older Adults’ Vulnerability to Fraud ,” Journal of Elder Abuse & Neglect 31 no. 3 (2019): 225-243.
[note 7] David Burnes et al., “ Prevalence of Financial Fraud and Scams Among Older Adults in the United States: A Systematic Review and Meta-Analysis ,” American Journal of Public Health 107 no. 8 (2017): 1193-1340; and Marti DeLiema, “ Fraud Versus Financial Abuse and the Influence of Social Relationships ,” National Adult Protective Services Association, Research to Practice Series, 2018.
[note 8] Burnes et al., “ Prevalence of Financial Fraud and Scams .”
[note 9] Burnes et al., “ Prevalence of Financial Fraud and Scams ”; and R. Nathan Spreng et al., “ Aging and Financial Exploitation Risk ,” in Aging and Money, 2nd ed., ed. Ronan M. Factora (Springer, 2021), 55-73.
[note 10] Michael S. Finke, John S. Howe, and Sandra J. Huston, “ Old Age and the Decline in Financial Literacy ,” Management Science 63 no. 1 (2016): 213-230.
[note 11] DeLiema, “ Fraud Versus Financial Abuse .”
[note 12] Elizabeth Castle et al., “ Neural and Behavioral Bases of Age Differences in Perceptions of Trust ,” Proceedings of the National Academy of Sciences 109 no. 51 (2012): 20848-20852.
[note 13] Joanna Bieda and Soo Park, “ Affluent Senior Citizens and Telemarketing Fraud ,” Journal of Student Research 10 no. 1 (2021): 1-10.
[note 14] Marguerite DeLiema et al., “ Financial Fraud Among Older Americans: Evidence and Implications ,” The Journals of Gerontology: Series B 75 no. 4 (2020): 861-868; and Michael Ross, Igor Grossmann, and Emily Schryer, “Contrary to Psychological and Popular Opinion, There Is No Compelling Evidence That Older Adults Are Disproportionately Victimized by Consumer Fraud,” Perspectives on Psychological Science 9 no. 4 (2014): 427-442.
[note 15] DeLiema et al., “ Financial Fraud Among Older Americans .”
[note 16] Refer to individual studies for the definition of financial fraud used.
[note 17] Stephen Deane, Elder Financial Exploitation: Why It Is a Concern, What Regulators Are Doing About It, and Looking Ahead , Washington, DC: U.S. Securities and Exchange Commission, Office of the Investor Advocate, 2018.
[note 18] Federal Trade Commission, Protecting Older Consumers 2021-2022: A Report of the Federal Trade Commission , Washington, DC: Federal Trade Commission, 2022.
[note 19] Donna J. Rabiner, Janet O’Keeffe, and David Brown, “ A Conceptual Framework of Financial Exploitation of Older Persons , ” Journal of Elder Abuse & Neglect 16 no. 2 (2004): 53-73.
[note 20] Spreng et al., “ Aging and Financial Exploitation Risk .”
[note 21] Ron Acierno et al., “ Mental Health Correlates of Financial Mistreatment in the National Elder Mistreatment Study Wave II ,” Journal of Aging and Health 31 no. 7 (2019): 1196-1211.
[note 22] Burnes et al., “ Prevalence of Financial Fraud and Scams .”
[note 23] The actual wording of the questions from which these data were derived used the term “offender.”
[note 24] See the BJS website for more information on the National Crime Victimization Survey, https://bjs.ojp.gov/programs/ncvs .
[note 25] See the 2017 Supplemental Fraud Survey instrument for question wording, https://bjs.ojp.gov/data-collection/supplemental-fraud-survey-sfs#surveys-0 .
[note 26] 42 U.S.C. § 3002(38).
[note 27] Internet Crime Complaint Center, 2020 Elder Fraud Report .
About the author
Rachel E. Morgan, Ph.D., and Susannah N. Tapp, Ph.D., are statisticians in the Victimization Statistics Unit at the Bureau of Justice Statistics, U.S. Department of Justice.
Cite this Article
Read more about:.
Research uncovers differences between the sexes in sleep, circadian rhythms and metabolism
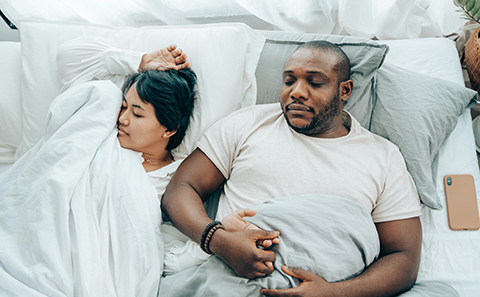
A new review of research evidence has explored the key differences in how women and men sleep, variations in their body clocks, and how this affects their metabolism.
Published in Sleep Medicine Reviews , the paper highlights the crucial role sex plays in understanding these factors and suggests a person’s biological sex should be considered when treating sleep, circadian rhythm and metabolic disorders.
Differences in sleep
The review found women rate their sleep quality lower than men’s and report more fluctuations in their quality of sleep, corresponding to changes throughout the menstrual cycle.
“Lower sleep quality is associated with anxiety and depressive disorders, which are twice as common in women as in men,” says Dr Sarah L. Chellappa from the University of Southampton and senior author of the paper. “Women are also more likely than men to be diagnosed with insomnia, although the reasons are not entirely clear. Recognising and comprehending sex differences in sleep and circadian rhythms is essential for tailoring approaches and treatment strategies for sleep disorders and associated mental health conditions.”
The paper’s authors also found women have a 25 to 50 per cent higher likelihood of developing restless legs syndrome and are up to four times as likely to develop sleep-related eating disorder, where people eat repeatedly during the night.
Meanwhile, men are three times more likely to be diagnosed with obstructive sleep apnoea (OSA). OSA manifests differently in women and men, which might explain this disparity. OSA is associated with a heightened risk of heart failure in women, but not men.
Sleep lab studies found women sleep more than men, spending around 8 minutes longer in non-REM (Rapid Eye Movement) sleep, where brain activity slows down. While the time we spend in NREM declines with age, this decline is more substantial in older men. Women also entered REM sleep, characterised by high levels of brain activity and vivid dreaming, earlier than men.
Variations in body clocks
The team of all women researchers from the University of Southampton in the UK, and Stanford University and Harvard University in the United States, found differences between the sexes are also present in our circadian rhythms.
They found melatonin, a hormone that helps with the timing of circadian rhythms and sleep, is secreted earlier in women than men. Core body temperature, which is at its highest before sleep and its lowest a few hours before waking, follows a similar pattern, reaching its peak earlier in women than in men.
Corresponding to these findings, other studies suggest women’s intrinsic circadian periods are shorter than men’s by around six minutes.
Dr Renske Lok from Stanford University, who led the review, says: “While this difference may be small, it is significant. The misalignment between the central body clock and the sleep/wake cycle is approximately five times larger in women than in men. Imagine if someone's watch was consistently running six minutes faster or slower. Over the course of days, weeks, and months, this difference can lead to a noticeable misalignment between the internal clock and external cues, such as light and darkness.
“Disruptions in circadian rhythms have been linked to various health problems, including sleep disorders, mood disorders and impaired cognitive function. Even minor differences in circadian periods can have significant implications for overall health and well-being.”
Men tend to be later chronotypes, preferring to go to bed and wake up later than women. This may lead to social jet lag, where their circadian rhythm doesn’t align with social demands, like work. They also have less consistent rest-activity schedules than women on a day-to-day basis.
Impact on metabolism
The research team also investigated if the global increase in obesity might be partially related to people not getting enough sleep - with 30 per cent of 30- to 64-year-olds sleeping less than six hours a night in the United States, with similar numbers in Europe.
There were big differences between how women’s and men’s brains responded to pictures of food after sleep deprivation. Brain networks associated with cognitive (decision making) and affective (emotional) processes were twice as active in women than in men. Another study found women had a 1.5 times higher activation in the limbic region (involved in emotion processing, memory formation, and behavioural regulation) in response to images of sweet food compared to men.
Despite this difference in brain activity, men tend to overeat more than women in response to sleep loss. Another study found more fragmented sleep, taking longer to get to sleep, and spending more time in bed trying to get to sleep were only associated with more hunger in men.
Both women and men nightshift workers are more likely to develop type 2 diabetes, but this risk is higher in men. Sixty-six per cent of women nightshift workers experienced emotional eating and another study suggests they are around 1.5 times more likely to be overweight or obese compared to women working day shifts.
The researchers also found emerging evidence on how women and men respond differently to treatments for sleep and circadian disorders. For example, weight loss was more successful in treating women with OSA than men, while women prescribed zolpidem (an insomnia medication) may require a lower dosage than men to avoid lingering sleepiness the next morning.
Dr Chellappa added: “Most of sleep and circadian interventions are a newly emerging field with limited research on sex differences. As we understand more about how women and men sleep, differences in their circadian rhythms and how these affect their metabolism, we can move towards more precise and personalised healthcare which enhances the likelihood of positive outcomes.”
Sex differences in sleep, circadian rhythms, and metabolism: Implications for precision medicine is published in Sleep Medicine Reviews and is available online.
The research was funded by the Alexander Von Humboldt Foundation, the US Department of Defense and the National Institute of Health.
Articles that may also interest you
Study explores homeless women’s experiences of ‘period poverty’, adhd medication can be lifesaving, scientists find, understanding the relationship between our sleep, body clock and mental health.
Read our research on: Gun Policy | International Conflict | Election 2024
Regions & Countries
Changing partisan coalitions in a politically divided nation, party identification among registered voters, 1994-2023.
Pew Research Center conducted this analysis to explore partisan identification among U.S. registered voters across major demographic groups and how voters’ partisan affiliation has shifted over time. It also explores the changing composition of voters overall and the partisan coalitions.
For this analysis, we used annual totals of data from Pew Research Center telephone surveys (1994-2018) and online surveys (2019-2023) among registered voters. All telephone survey data was adjusted to account for differences in how people respond to surveys on the telephone compared with online surveys (refer to Appendix A for details).
All online survey data is from the Center’s nationally representative American Trends Panel . The surveys were conducted in both English and Spanish. Each survey is weighted to be representative of the U.S. adult population by gender, age, education, race and ethnicity and other categories. Read more about the ATP’s methodology , as well as how Pew Research Center measures many of the demographic categories used in this report .
The contours of the 2024 political landscape are the result of long-standing patterns of partisanship, combined with the profound demographic changes that have reshaped the United States over the past three decades.
Many of the factors long associated with voters’ partisanship remain firmly in place. For decades, gender, race and ethnicity, and religious affiliation have been important dividing lines in politics. This continues to be the case today.
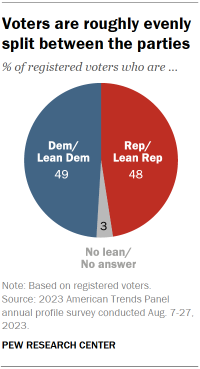
Yet there also have been profound changes – in some cases as a result of demographic change, in others because of dramatic shifts in the partisan allegiances of key groups.
The combined effects of change and continuity have left the country’s two major parties at virtual parity: About half of registered voters (49%) identify as Democrats or lean toward the Democratic Party, while 48% identify as Republicans or lean Republican.
In recent decades, neither party has had a sizable advantage, but the Democratic Party has lost the edge it maintained from 2017 to 2021. (Explore this further in Chapter 1 . )
Pew Research Center’s comprehensive analysis of party identification among registered voters – based on hundreds of thousands of interviews conducted over the past three decades – tracks the changes in the country and the parties since 1994. Among the major findings:
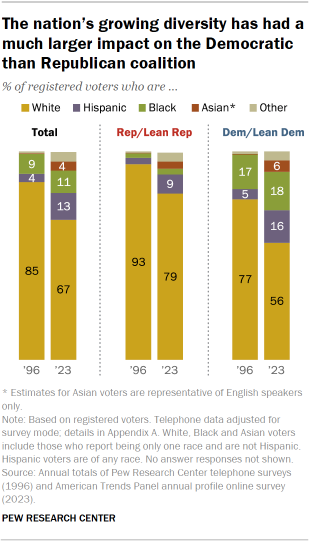
The partisan coalitions are increasingly different. Both parties are more racially and ethnically diverse than in the past. However, this has had a far greater impact on the composition of the Democratic Party than the Republican Party.
The share of voters who are Hispanic has roughly tripled since the mid-1990s; the share who are Asian has increased sixfold over the same period. Today, 44% of Democratic and Democratic-leaning voters are Hispanic, Black, Asian, another race or multiracial, compared with 20% of Republicans and Republican leaners. However, the Democratic Party’s advantages among Black and Hispanic voters, in particular, have narrowed somewhat in recent years. (Explore this further in Chapter 8 .)
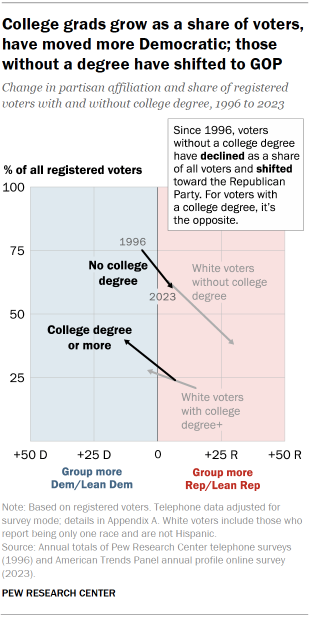
Education and partisanship: The share of voters with a four-year bachelor’s degree keeps increasing, reaching 40% in 2023. And the gap in partisanship between voters with and without a college degree continues to grow, especially among White voters. More than six-in-ten White voters who do not have a four-year degree (63%) associate with the Republican Party, which is up substantially over the past 15 years. White college graduates are closely divided; this was not the case in the 1990s and early 2000s, when they mostly aligned with the GOP. (Explore this further in Chapter 2 .)
Beyond the gender gap: By a modest margin, women voters continue to align with the Democratic Party (by 51% to 44%), while nearly the reverse is true among men (52% align with the Republican Party, 46% with the Democratic Party). The gender gap is about as wide among married men and women. The gap is wider among men and women who have never married; while both groups are majority Democratic, 37% of never-married men identify as Republicans or lean toward the GOP, compared with 24% of never-married women. (Explore this further in Chapter 3 .)
A divide between old and young: Today, each younger age cohort is somewhat more Democratic-oriented than the one before it. The youngest voters (those ages 18 to 24) align with the Democrats by nearly two-to-one (66% to 34% Republican or lean GOP); majorities of older voters (those in their mid-60s and older) identify as Republicans or lean Republican. While there have been wide age divides in American politics over the last two decades, this wasn’t always the case; in the 1990s there were only very modest age differences in partisanship. (Explore this further in Chapter 4 .)
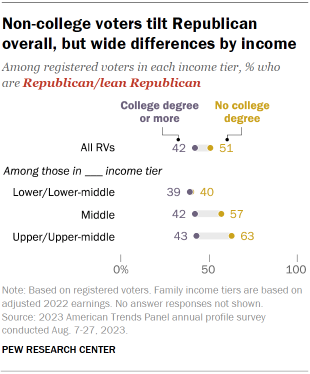
Education and family income: Voters without a college degree differ substantially by income in their party affiliation. Those with middle, upper-middle and upper family incomes tend to align with the GOP. A majority with lower and lower-middle incomes identify as Democrats or lean Democratic. There are no meaningful differences in partisanship among voters with at least a four-year bachelor’s degree; across income categories, majorities of college graduate voters align with the Democratic Party. (Explore this further in Chapter 6 .)
Rural voters move toward the GOP, while the suburbs remain divided: In 2008, when Barack Obama sought his first term as president, voters in rural counties were evenly split in their partisan loyalties. Today, Republicans hold a 25 percentage point advantage among rural residents (60% to 35%). There has been less change among voters in urban counties, who are mostly Democratic by a nearly identical margin (60% to 37%). The suburbs – perennially a political battleground – remain about evenly divided. (Explore this further in Chapter 7 . )
Growing differences among religious groups: Mirroring movement in the population overall, the share of voters who are religiously unaffiliated has grown dramatically over the past 15 years. These voters, who have long aligned with the Democratic Party, have become even more Democratic over time: Today 70% identify as Democrats or lean Democratic. In contrast, Republicans have made gains among several groups of religiously affiliated voters, particularly White Catholics and White evangelical Protestants. White evangelical Protestants now align with the Republican Party by about a 70-point margin (85% to 14%). (Explore this further in Chapter 5 .)
What this report tells us – and what it doesn’t
In most cases, the partisan allegiances of voters do not change a great deal from year to year. Yet as this study shows, the long-term shifts in party identification are substantial and say a great deal about how the country – and its political parties – have changed since the 1990s.

The steadily growing alignment between demographics and partisanship reveals an important aspect of steadily growing partisan polarization. Republicans and Democrats do not just hold different beliefs and opinions about major issues , they are much more different racially, ethnically, geographically and in educational attainment than they used to be.
Yet over this period, there have been only modest shifts in overall partisan identification. Voters remain evenly divided, even as the two parties have grown further apart. The continuing close division in partisan identification among voters is consistent with the relatively narrow margins in the popular votes in most national elections over the past three decades.
Partisan identification provides a broad portrait of voters’ affinities and loyalties. But while it is indicative of voters’ preferences, it does not perfectly predict how people intend to vote in elections, or whether they will vote. In the coming months, Pew Research Center will release reports analyzing voters’ preferences in the presidential election, their engagement with the election and the factors behind candidate support.
Next year, we will release a detailed study of the 2024 election, based on validated voters from the Center’s American Trends Panel. It will examine the demographic composition and vote choices of the 2024 electorate and will provide comparisons to the 2020 and 2016 validated voter studies.
The partisan identification study is based on annual totals from surveys conducted on the Center’s American Trends Panel from 2019 to 2023 and telephone surveys conducted from 1994 to 2018. The survey data was adjusted to account for differences in how the surveys were conducted. For more information, refer to Appendix A .
Previous Pew Research Center analyses of voters’ party identification relied on telephone survey data. This report, for the first time, combines data collected in telephone surveys with data from online surveys conducted on the Center’s nationally representative American Trends Panel.
Directly comparing answers from online and telephone surveys is complex because there are differences in how questions are asked of respondents and in how respondents answer those questions. Together these differences are known as “mode effects.”
As a result of mode effects, it was necessary to adjust telephone trends for leaned party identification in order to allow for direct comparisons over time.
In this report, telephone survey data from 1994 to 2018 is adjusted to align it with online survey responses. In 2014, Pew Research Center randomly assigned respondents to answer a survey by telephone or online. The party identification data from this survey was used to calculate an adjustment for differences between survey mode, which is applied to all telephone survey data in this report.
Please refer to Appendix A for more details.
Add Pew Research Center to your Alexa
Say “Alexa, enable the Pew Research Center flash briefing”
Report Materials
Table of contents, behind biden’s 2020 victory, a voter data resource: detailed demographic tables about verified voters in 2016, 2018, what the 2020 electorate looks like by party, race and ethnicity, age, education and religion, interactive map: the changing racial and ethnic makeup of the u.s. electorate, in changing u.s. electorate, race and education remain stark dividing lines, most popular.
About Pew Research Center Pew Research Center is a nonpartisan fact tank that informs the public about the issues, attitudes and trends shaping the world. It conducts public opinion polling, demographic research, media content analysis and other empirical social science research. Pew Research Center does not take policy positions. It is a subsidiary of The Pew Charitable Trusts .
- Alzheimer's disease & dementia
- Arthritis & Rheumatism
- Attention deficit disorders
- Autism spectrum disorders
- Biomedical technology
- Diseases, Conditions, Syndromes
- Endocrinology & Metabolism
- Gastroenterology
- Gerontology & Geriatrics
- Health informatics
- Inflammatory disorders
- Medical economics
- Medical research
- Medications
- Neuroscience
- Obstetrics & gynaecology
- Oncology & Cancer
- Ophthalmology
- Overweight & Obesity
- Parkinson's & Movement disorders
- Psychology & Psychiatry
- Radiology & Imaging
- Sleep disorders
- Sports medicine & Kinesiology
- Vaccination
- Breast cancer
- Cardiovascular disease
- Chronic obstructive pulmonary disease
- Colon cancer
- Coronary artery disease
- Heart attack
- Heart disease
- High blood pressure
- Kidney disease
- Lung cancer
- Multiple sclerosis
- Myocardial infarction
- Ovarian cancer
- Post traumatic stress disorder
- Rheumatoid arthritis
- Schizophrenia
- Skin cancer
- Type 2 diabetes
- Full List »
share this!
April 10, 2024
This article has been reviewed according to Science X's editorial process and policies . Editors have highlighted the following attributes while ensuring the content's credibility:
fact-checked
trusted source
Research uncovers differences between the sexes in sleep, circadian rhythms and metabolism
by University of Southampton
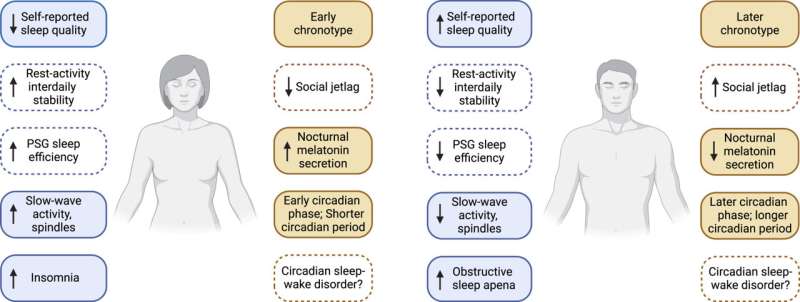
A new review of research evidence has explored the key differences in how women and men sleep, variations in their body clocks, and how this affects their metabolism.
Published in Sleep Medicine Reviews , the paper highlights the crucial role sex plays in understanding these factors and suggests a person's biological sex should be considered when treating sleep, circadian rhythm and metabolic disorders .
Differences in sleep
The review found women rate their sleep quality lower than men's and report more fluctuations in their quality of sleep, corresponding to changes throughout the menstrual cycle.
"Lower sleep quality is associated with anxiety and depressive disorders, which are twice as common in women as in men," says Dr. Sarah L. Chellappa from the University of Southampton and senior author of the paper.
"Women are also more likely than men to be diagnosed with insomnia, although the reasons are not entirely clear. Recognizing and comprehending sex differences in sleep and circadian rhythms is essential for tailoring approaches and treatment strategies for sleep disorders and associated mental health conditions."
The paper's authors also found women have a 25% to 50% higher likelihood of developing restless legs syndrome and are up to four times as likely to develop sleep-related eating disorder, where people eat repeatedly during the night.
Meanwhile, men are three times more likely to be diagnosed with obstructive sleep apnea (OSA). OSA manifests differently in women and men, which might explain this disparity. OSA is associated with a heightened risk of heart failure in women, but not men.
Sleep lab studies found women sleep more than men, spending around 8 minutes longer in non-REM (Rapid Eye Movement) sleep, where brain activity slows down. While the time we spend in NREM declines with age, this decline is more substantial in older men. Women also entered REM sleep, characterized by high levels of brain activity and vivid dreaming, earlier than men.
Variations in body clocks
The team of all women researchers from the University of Southampton in the UK, and Stanford University and Harvard University in the United States, found differences between the sexes are also present in our circadian rhythms.
They found melatonin, a hormone that helps with the timing of circadian rhythms and sleep, is secreted earlier in women than men. Core body temperature, which is at its highest before sleep and its lowest a few hours before waking, follows a similar pattern, reaching its peak earlier in women than in men.
Corresponding to these findings, other studies suggest women's intrinsic circadian periods are shorter than men's by around six minutes.
Dr. Renske Lok from Stanford University, who led the review, says, "While this difference may be small, it is significant. The misalignment between the central body clock and the sleep/wake cycle is approximately five times larger in women than in men. Imagine if someone's watch was consistently running six minutes faster or slower. Over the course of days, weeks, and months, this difference can lead to a noticeable misalignment between the internal clock and external cues, such as light and darkness.
"Disruptions in circadian rhythms have been linked to various health problems, including sleep disorders , mood disorders and impaired cognitive function. Even minor differences in circadian periods can have significant implications for overall health and well-being."
Men tend to be later chronotypes, preferring to go to bed and wake up later than women. This may lead to social jet lag, where their circadian rhythm doesn't align with social demands, like work. They also have less consistent rest-activity schedules than women on a day-to-day basis.
Impact on metabolism
The research team also investigated if the global increase in obesity might be partially related to people not getting enough sleep—with 30 percent of 30- to 64-year-olds sleeping less than 6 hours a night in the United States, with similar numbers in Europe.
There were big differences between how women's and men's brains responded to pictures of food after sleep deprivation. Brain networks associated with cognitive (decision-making) and affective (emotional) processes were twice as active in women than in men. Another study found women had a 1.5 times higher activation in the limbic region (involved in emotion processing, memory formation, and behavioral regulation) in response to images of sweet food compared to men.
Despite this difference in brain activity , men tend to overeat more than women in response to sleep loss. Another study found more fragmented sleep, taking longer to get to sleep, and spending more time in bed trying to get to sleep were only associated with more hunger in men.
Both women and men nightshift workers are more likely to develop type 2 diabetes, but this risk is higher in men. Of women nightshift workers, 66% experienced emotional eating and another study suggests they are around 1.5 times more likely to be overweight or obese compared to women working day shifts.
The researchers also found emerging evidence on how women and men respond differently to treatments for sleep and circadian disorders. For example, weight loss was more successful in treating women with OSA than men, while women prescribed zolpidem (an insomnia medication) may require a lower dosage than men to avoid lingering sleepiness the next morning.
Dr. Chellappa added, "Most of sleep and circadian interventions are a newly emerging field with limited research on sex differences. As we understand more about how women and men sleep, differences in their circadian rhythms and how these affect their metabolism, we can move towards more precise and personalized health care which enhances the likelihood of positive outcomes."
Explore further
Feedback to editors

Pandemic drinking hit middle-aged women hardest, study finds
5 minutes ago

Researchers shed light on the molecular causes of different functions of opioid receptors
10 minutes ago

First clinical trial of vosoritide for children with hypochondroplasia shows increased growth
25 minutes ago

Study of data from thousands of women suggests ovarian cycle is regulated by circadian rhythm
39 minutes ago

First national study of Dobbs ruling's effect on permanent contraception among young adults
49 minutes ago

Genomic deletions explain why some types of melanoma resist targeted therapies

Male infertility genetic study improves molecular diagnostics and personalized management of patients

How the inflamed brain becomes disconnected after a stroke

Using AI to spot parasites in stool samples

New study probes macrophages' role in developing pulmonary fibrosis
2 hours ago
Related Stories

Sleep and circadian rhythm problems linked with poor mental health—new research
Mar 2, 2024

Understanding the relationship between our sleep, body clock and mental health
Feb 19, 2024

Obstructive sleep apnea linked to bladder pain / interstitial cystitis
Mar 7, 2024

Study of circadian rhythm reveals differences in men and women
Sep 4, 2020

Turning back the clock for daylight saving time can impact brain and body functions
Nov 2, 2022

Working night shifts is associated with sleep disorders in more than half of workers, study suggests
Dec 7, 2023
Recommended for you

Softer tumors fuel more aggressive spread of triple-negative breast cancer, research shows

Presence of specific lipids indicate tissue aging and can be decreased through exercise, study shows
6 hours ago

Scientists say outdated diabetes drug still has something to offer
23 hours ago

Case study of 4-year-old with Down syndrome and sleep apnea suggests procedure can be effective at young ages
Apr 11, 2024

How the body switches out of 'fight' mode: Study shows hormones reprogram metabolism of immune cells
Apr 10, 2024

Researchers explore role of androgens in shaping sex differences
Let us know if there is a problem with our content.
Use this form if you have come across a typo, inaccuracy or would like to send an edit request for the content on this page. For general inquiries, please use our contact form . For general feedback, use the public comments section below (please adhere to guidelines ).
Please select the most appropriate category to facilitate processing of your request
Thank you for taking time to provide your feedback to the editors.
Your feedback is important to us. However, we do not guarantee individual replies due to the high volume of messages.
E-mail the story
Your email address is used only to let the recipient know who sent the email. Neither your address nor the recipient's address will be used for any other purpose. The information you enter will appear in your e-mail message and is not retained by Medical Xpress in any form.
Newsletter sign up
Get weekly and/or daily updates delivered to your inbox. You can unsubscribe at any time and we'll never share your details to third parties.
More information Privacy policy
Donate and enjoy an ad-free experience
We keep our content available to everyone. Consider supporting Science X's mission by getting a premium account.
E-mail newsletter
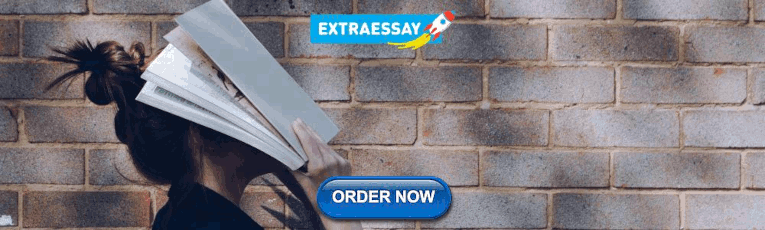
IMAGES
VIDEO
COMMENTS
Now that we have explored the differences between surveys and research, it is time to put that knowledge into practice. Below are some exercises to help you improve your understanding and use of these terms in sentences. Exercise 1: Identify The Method. Read the following scenarios and identify whether the method used is a survey or research.
Survey research means collecting information about a group of people by asking them questions and analyzing the results. To conduct an effective survey, follow these six steps: Determine who will participate in the survey. Decide the type of survey (mail, online, or in-person) Design the survey questions and layout.
Actually we should know three basic terms: Statistics is the field of study or we can say a science and art of collecting, organizing, presenting and interpreting data for exposing a problem and finding solutions.. Research is a systematic process used by statisticians to achieve the aforementioned goals.. Research has different types and tools. A survey is a kind of research or we can say a ...
Replicable: applying the same methods more than once should achieve similar results. Types: surveys can be exploratory, descriptive, or casual used in both online and offline forms. Data: survey research can generate both quantitative and qualitative data. Impartial: sampling is randomized to avoid bias.
A survey can be a component of research, providing data that can be analyzed and interpreted, while research encompasses the entire process of inquiry from hypothesis formulation to conclusion. Research can involve multiple methods of data collection and analysis, of which surveys might be just one part. The design and implementation of surveys ...
As nouns the difference between survey and research is that survey is the act of surveying; a general view, as from above while research is diligent inquiry or examination to seek or revise facts, principles, theories, applications, etc.; laborious or continued search after truth. As verbs the difference between survey and research is that survey is to inspect, or take a view of; to view with ...
Survey research is defined as "the collection of information from a sample of individuals through their responses to questions" ( Check & Schutt, 2012, p. 160 ). This type of research allows for a variety of methods to recruit participants, collect data, and utilize various methods of instrumentation. Survey research can use quantitative ...
Survey Research Definition. Survey Research is defined as the process of conducting research using surveys that researchers send to survey respondents. The data collected from surveys is then statistically analyzed to draw meaningful research conclusions. In the 21st century, every organization's eager to understand what their customers think ...
Type of research What's the difference? What to consider; Primary research vs secondary research: Primary data is collected directly by the researcher (e.g., through interviews or experiments), while secondary data has already been collected by someone else (e.g., in government surveys or scientific publications).: How much data is already available on your topic?
For decades, researchers and businesses have used survey research to produce statistical data and explore ideas. The survey process is simple, ask questions and analyze the responses to make decisions. Data is what makes the difference between a valid and invalid statement and as the American statistician, W. Edwards Deming said:
A survey can be a more in-depth form of data collection to gather data from a specific target audience and survey respondents. It's also more complex than a questionnaire. Researchers can use the data gathered from a survey for statistical analysis to evaluate the data and responses, and generate conclusions from the responses to the survey ...
Both surveys and questionnaires use a series of questions to gather information, however the purpose of the research and the treatment of the data after it is collected distinguish a questionnaire from a survey, e.g.: A questionnaire is a set of written questions used for collecting information for the benefit of one single individual.
A scientific procedure wherein the factor under study is isolated to test hypothesis is called an experiment. Surveys are performed when the research is of descriptive nature, whereas in the case of experiments are conducted in experimental research. The survey samples are large as the response rate is low, especially when the survey is ...
Attrition refers to participants leaving a study. It always happens to some extent—for example, in randomized controlled trials for medical research. Differential attrition occurs when attrition or dropout rates differ systematically between the intervention and the control group.As a result, the characteristics of the participants who drop out differ from the characteristics of those who ...
Interviews are more resource-intensive but can provide great depth to your research. Meanwhile, surveys are quick and easy to get off the ground and to analyse—especially with SurveyMonkey's survey templates and suite of tools—and bring an excellent breadth to your findings. Create a successful survey in 10 easy steps.
The differences between surveys and focus groups are significant and revolve around issues like data type, sample size, interaction, flexibility, and data analysis. These differences highlight each method's unique strengths and characteristics and why they are suited for different research objectives and contexts.
Ultimately, the choice between case study and survey depends on the research objectives, the nature of the research question, and the available resources. Researchers need to carefully consider the attributes of each method and select the most appropriate approach to gather and analyze data effectively.
The difference between survey and questionnaire can be drawn clearly on the following grounds: The term survey, means the collection, recording, and analysis of information on a particular subject, an area or people's group. ... Difference Between Research Method and Research Methodology Difference Between Structured and Unstructured ...
Complexity: Surveys are better suited for straightforward research questions, while questionnaires are more complex and detailed. Resources: The availability of resources is essential. You may require more resources, such as personnel, technology, and budget, than questionnaires for surveys.
A survey collects data about a group of people so you can analyze and forecast trends about that group. As opposed to its questionnaire cousin, the data isn't analyzed in isolation. Surveys look for trends, behavior, or a bigger picture rather than individual insights. A questionnaire collects data about individuals from a list of questions.
Face validity and content validity are similar in that they both evaluate how suitable the content of a test is. The difference is that face validity is subjective, and assesses content at surface level.. When a test has strong face validity, anyone would agree that the test's questions appear to measure what they are intended to measure.. For example, looking at a 4th grade math test ...
Background The attrition of nursing staff significantly contributes to the shortage of healthcare professionals. This study entailed an examination of the propensity of nurses to sustain employment within Grade-A tertiary general hospitals and the various influencing factors. Methods A total of 2,457 nurses from three grade-A tertiary general hospitals were surveyed. The survey instruments ...
The percentage of persons age 60 or older in the United States increased by 33% from 2010 to 2020 and is expected to continue to grow. [1] The Better Business Bureau reports that older adults [2] lose more than $36 billion to financial fraud every year. [3] According to the FBI's Internet Crime Complaint Center, 105,301 cases of fraud against ...
The difference between gen AI summarization and real AI insights The ability to create a summary out of a body of text, audio or video is a core gen AI capability that an LLM enables.
A new review of research evidence has explored the key differences in how women and men sleep, variations in their body clocks, and how this affects their metabolism. Published in Sleep Medicine Reviews , the paper highlights the crucial role sex plays in understanding these factors and suggests a person's biological sex should be considered ...
In 2014, Pew Research Center randomly assigned respondents to answer a survey by telephone or online. The party identification data from this survey was used to calculate an adjustment for differences between survey mode, which is applied to all telephone survey data in this report. Please refer to Appendix A for more details.
Conceptual framework of sex differences in sleep and circadian rhythms. Credit: Sleep Medicine Reviews (2024). DOI: 10.1016/j.smrv.2024.101926
Accuracy of antenatal visits plays a crucial role in reducing the risk of maternal death during pregnancy. West Java is a province in Indonesia that has the highest maternal mortality cases. An analysis of the accuracy of antenatal visits using a spatial approach, involving the distribution of villages and cities, was conducted to understand the interaction between humans and the environment ...