Advertisement
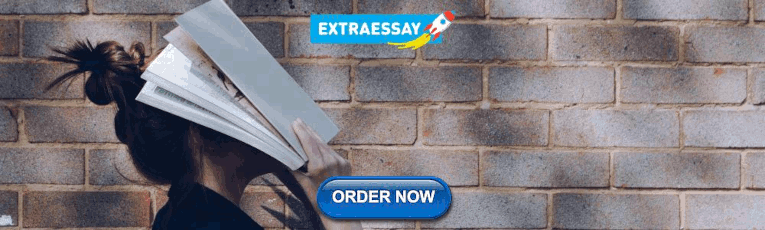
Integrating BIM and AI for Smart Construction Management: Current Status and Future Directions
- Review article
- Published: 03 November 2022
- Volume 30 , pages 1081–1110, ( 2023 )
Cite this article
- Yue Pan 1 &
- Limao Zhang 2
10k Accesses
54 Citations
3 Altmetric
Explore all metrics
At present, building information modeling (BIM) has been developed into a digital backbone of the architecture, engineering, and construction industry. Also, recent decades have witnessed the fast development of various AI techniques in reliably tackling a huge amount of data under complex and uncertain environments. Since both BIM and artificial intelligence (AI) have attracted sustainable attention, the integration of BIM and AI can demonstrate newly added value in handling construction projects with inherent complexity and uncertainty. For a clear understanding of BIM-AI integration in boosting smart construction management, the goal of this paper is to make a comprehensive investigation and summary of its potential value and practical utility to drive the construction industry to catch up with the fast pace of automation and digitalization. Through both the bibliometric analysis and information analysis, this paper provides a deep insight into the status quo and future trends for leveraging AI during the entire lifecycle of a BIM-enabled project. It is worth noting that keywords that are highly cited in the latest two years contain deep learning, internet of things, digital twin, and others, which means AI is evolving as the next frontier to accelerate the revolution in the traditional civil engineering. According to keyword clusters derived from the log-likelihood ratio, we determine six advanced research interests and discuss the state-of-the-art research, including automated design and rule checking, 3D as-built reconstruction, event log mining, building performance analysis, virtual and augmented reality, and digital twin. Besides, a growing force can be put on three potential directions to more broadly adopt the BIM-AI integration, including synthesis of human–machine intelligence, civil-level digital twin, and blockchain, aiming to make BIM and AI live up to expectations.
This is a preview of subscription content, log in via an institution to check access.
Access this article
Price includes VAT (Russian Federation)
Instant access to the full article PDF.
Rent this article via DeepDyve
Institutional subscriptions
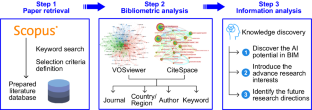
Similar content being viewed by others
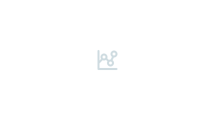
Digital twin-enabled smart facility management: A bibliometric review
Obaidullah Hakimi, Hexu Liu & Osama Abudayyeh
Generative AI Use in the Construction Industry
From BIM Towards Digital Twin: Strategy and Future Development for Smart Asset Management
Sacks R et al (2018) BIM handbook: a guide to building information modeling for owners, designers, engineers, contractors, and facility managers. John Wiley, New York
Book Google Scholar
Wong JKW, Zhou J (2015) Enhancing environmental sustainability over building life cycles through green BIM: a review. Autom Constr 57:156–165
Article Google Scholar
Jung N, Lee G (2019) Automated classification of building information modeling (BIM) case studies by BIM use based on natural language processing (NLP) and unsupervised learning. Adv Eng Inform 41:100917
Construction MH, (2014) The business value of BIM for construction in major global markets: How contractors around the world are driving innovation with building information modeling. Smart MarketReport. p. 1–60.
Jones S, et al., (2017) The business value of BIM for infrastructure 2017. SmartMarket Report.
Zhao X (2017) A scientometric review of global BIM research: analysis and visualization. Autom Constr 80:37–47
Li X et al (2017) Mapping the knowledge domains of building information modeling (BIM): a bibliometric approach. Autom Constr 84:195–206
Wen Q-J et al (2021) The progress and trend of BIM research: a bibliometrics-based visualization analysis. Autom Constr 124:103558
Lu Y et al (2017) Building information modeling (BIM) for green buildings: A critical review and future directions. Autom Constr 83:134–148
Wang H, Pan Y, Luo X (2019) Integration of BIM and GIS in sustainable built environment: a review and bibliometric analysis. Autom Constr 103:41–52
Tang S et al (2019) A review of building information modeling (BIM) and the internet of things (IoT) devices integration: present status and future trends. Autom Constr 101:127–139
Gao X, Pishdad-Bozorgi P (2019) BIM-enabled facilities operation and maintenance: a review. Adv Eng Inform 39:227–247
Yin X et al (2019) Building information modelling for off-site construction: review and future directions. Autom Constr 101:72–91
Hu Z-Z et al (2022) Knowledge extraction and discovery based on bim: a critical review and future directions. Arch Comput Methods Eng 29(1):335–356
Pan Y, Zhang L (2021) Roles of artificial intelligence in construction engineering and management: A critical review and future trends. Autom Constr 122:103517
Darko A et al (2020) Artificial intelligence in the AEC industry: scientometric analysis and visualization of research activities. Autom Constr 112:103081
Guo K, Zhang L (2022) Multi-objective optimization for improved project management: Current status and future directions. Autom Constr 139:104256
Abdirad H, Mathur P (2021) Artificial intelligence for BIM content management and delivery: case study of association rule mining for construction detailing. Adv Eng Inform 50:101414
Alizadehsalehi S, Hadavi A, Huang JC (2020) From BIM to extended reality in AEC industry. Autom Constr 116:103254
Zabin A et al (2022) Applications of machine learning to BIM: A systematic literature review. Adv Eng Inform 51:101474
Zhang L, Pan Y (2022) Information fusion for automated post-disaster building damage evaluation using deep neural network. Sustain Cities Soc 77:103574
Fathi M et al (2021) Big data analytics in weather forecasting: a systematic review. Arch Comput Methods in Eng. https://doi.org/10.1007/s11831-021-09630-6
Pan Y, Zhang L (2022) Mitigating tunnel-induced damages using deep neural networks. Autom Constr 138:104219
Falagas ME et al (2008) Comparison of pubmed, scopus, web of science, and google scholar: strengths and weaknesses. FASEB J 22(2):338–342
Mongeon P, Paul-Hus A (2016) The journal coverage of web of science and Scopus: a comparative analysis. Scientometrics 106(1):213–228
Waltman L, Van Eck NJ (2013) A smart local moving algorithm for large-scale modularity-based community detection. Eur Phys J B 86(11):1–14
Oraee M et al (2017) Collaboration in BIM-based construction networks: a bibliometric-qualitative literature review. Int J Project Manage 35(7):1288–1301
Miettinen R, Paavola S (2014) Beyond the BIM utopia: approaches to the development and implementation of building information modeling. Autom Constr 43:84–91
Eadie R et al (2013) BIM implementation throughout the UK construction project lifecycle: an analysis. Autom Constr 36:145–151
Gu N, London K (2010) Understanding and facilitating BIM adoption in the AEC industry. Autom Constr 19(8):988–999
Liu H et al (2018) BIM-based automated design and planning for boarding of light-frame residential buildings. Autom Constr 89:235–249
Ramaji IJ, Memari AM (2016) Product architecture model for multistory modular buildings. J Constr Eng Manag 142(10):04016047
Chen C, Tang L (2019) BIM-based integrated management workflow design for schedule and cost planning of building fabric maintenance. Autom Constr 107:102944
Sacks R et al (2019) Automating design review with artificial intelligence and BIM: State of the art and research framework. Comput Civil Eng 2019:353–360
Google Scholar
Liu Y, Van Nederveen S, Hertogh M (2017) Understanding effects of BIM on collaborative design and construction: an empirical study in China. Int J Project Manage 35(4):686–698
Hire S, Sandbhor S, Ruikar K (2022) Bibliometric Survey for Adoption of Building Information Modeling (BIM) in Construction Industry– A Safety Perspective. Archives of Computational Methods in Engineering 29(1):679–693
Bortolini R, Formoso CT, Viana DD (2019) Site logistics planning and control for engineer-to-order prefabricated building systems using BIM 4D modeling. Autom Constr 98:248–264
Whitlock K et al (2018) BIM for construction site logistics management. J Eng Proj Prod Manage 8(1):47
Jang S, Lee G (2018) Process, productivity, and economic analyses of BIM–based multi-trade prefabrication—A case study. Autom Constr 89:86–98
Zhang L, Ashuri B (2018) BIM log mining: discovering social networks. Autom Constr 91:31–43
Hu Z-Z et al (2018) BIM-based integrated delivery technologies for intelligent MEP management in the operation and maintenance phase. Adv Eng Softw 115:1–16
Deng M et al (2021) A BIM-based framework for automated generation of fabrication drawings for façade panels. Comput Ind 126:103395
Hamidavi T, Abrishami S, Hosseini MR (2020) Towards intelligent structural design of buildings: a BIM-based solution. J Build Eng 32:101685
Kumar SS, Cheng JC (2015) A BIM-based automated site layout planning framework for congested construction sites. Autom Constr 59:24–37
Wu S et al (2021) Intelligent optimal design of floor tiles: a goal-oriented approach based on BIM and parametric design platform. J Clean Prod 299:126754
Kim K, Teizer J (2014) Automatic design and planning of scaffolding systems using building information modeling. Adv Eng Inform 28(1):66–80
Amiri R, Sardroud JM, De Soto BG (2017) BIM-based applications of metaheuristic algorithms to support the decision-making process: uses in the planning of construction site layout. Procedia Eng 196:558–564
Li K, Luo H, Skibniewski MJ (2019) A non-centralized adaptive method for dynamic planning of construction components storage areas. Adv Eng Inform 39:80–94
Zhang J, El-Gohary NM (2017) Integrating semantic NLP and logic reasoning into a unified system for fully-automated code checking. Autom Constr 73:45–57
Ghannad P et al (2019) Automated BIM data validation integrating open-standard schema with visual programming language. Adv Eng Inform 40:14–28
Sydora C, Stroulia E (2020) Rule-based compliance checking and generative design for building interiors using BIM. Autom Constr 120:103368
Bloch T, Sacks R (2020) Clustering information types for semantic enrichment of building information models to support automated code compliance checking. J Comput Civ Eng 34(6):04020040
Preidel C, Borrmann A (2017) Refinement of the visual code checking language for an automated checking of building information models regarding applicable regulations. Comput Civil Eng 2017:157–165
Hu Z-Z et al (2021) Knowledge extraction and discovery based on BIM: a critical review and future directions. Arch Comput Methods Eng. https://doi.org/10.1007/s11831-021-09576-9
Zhang J et al (2019) A review of deep learning-based semantic segmentation for point cloud. IEEE Access 7:179118–179133
Xu S et al (2021) Computer vision techniques in construction: a critical review. Arch Comput Methods Eng 28(5):3383–3397
Article MathSciNet Google Scholar
Nguyen A, Le B (2013) 3D point cloud segmentation a survey. 2013 6th IEEE conference on robotics, automation and mechatronics (RAM). IEEE, Piscataway
Barazzetti L (2016) Parametric as-built model generation of complex shapes from point clouds. Adv Eng Inform 30(3):298–311
Jung J et al (2018) Automated 3D volumetric reconstruction of multiple-room building interiors for as-built BIM. Adv Eng Inform 38:811–825
Xue F et al (2019) BIM reconstruction from 3D point clouds: A semantic registration approach based on multimodal optimization and architectural design knowledge. Adv Eng Inform 42:100965
Bassier M, Vergauwen M (2020) Unsupervised reconstruction of building information modeling wall objects from point cloud data. Autom Constr 120:103338
Chen J, Kira Z, Cho YK (2019) Deep learning approach to point cloud scene understanding for automated scan to 3D reconstruction. J Comput Civ Eng 33(4):04019027
Ma JW, Czerniawski T, Leite F (2020) Semantic segmentation of point clouds of building interiors with deep learning: augmenting training datasets with synthetic BIM-based point clouds. Autom Constr 113:103144
Xu Y, Shen X, Lim S (2021) CorDet: corner-aware 3D object detection networks for automated scan-to-BIM. J Comput Civ Eng 35(3):04021002
Kim H, Kim C (2021) 3D as-built modeling from incomplete point clouds using connectivity relations. Autom Constr 130:103855
Qi, C.R., et al. (2017) Pointnet: Deep learning on point sets for 3d classification and segmentation. in Proceedings of the IEEE conference on computer vision and pattern recognition
Yarmohammadi S, Pourabolghasem R, Castro-Lacouture D (2017) Mining implicit 3D modeling patterns from unstructured temporal BIM log text data. Autom Constr 81:17–24
Zhang L, Wen M, Ashuri B (2018) BIM log mining: measuring design productivity. J Comput Civ Eng 32(1):04017071
Pan Y, Zhang L (2020) BIM log mining: Exploring design productivity characteristics. Autom Constr 109:102997
Pan Y, Zhang L, Li Z (2020) Mining event logs for knowledge discovery based on adaptive efficient fuzzy Kohonen clustering network. Knowl-Based Syst 209:106482
Pan, Y. and L. Zhang. Sequential Design Command Prediction Using BIM Event Logs. in Construction Research Congress 2020: Computer Applications. 2020. American Society of Civil Engineers Reston
Pan Y, Zhang L (2020) BIM log mining: Learning and predicting design commands. Autom Constr 112:103107
Pan Y, Zhang L, Skibniewski MJ (2020) Clustering of designers based on building information modeling event logs. Computer-Aided Civil Infrastruct Eng 35(7):701–718
Bernstein H, Jones S, Russo M (2015) Green BIM—How Building Information Modeling is contributing to green design and construction. J Inf Technol Civ Eng Arch 2:20–36
Ramesh T, Prakash R, Shukla K (2010) Life cycle energy analysis of buildings: An overview. Energy Build 42(10):1592–1600
Jin R et al (2019) Integrating BIM with building performance analysis in project life-cycle. Autom Constr 106:102861
Chong A et al (2019) Continuous-time Bayesian calibration of energy models using BIM and energy data. Energy Build 194:177–190
Singh, M.M. and P. Geyer. (2019) Statistical decision assistance for determining energy-efficient options in building design under uncertainty. in 26th International Workshop on Intelligent Computing in Engineering.. CEUR Workshop Proceedings.
Geyer P, Singaravel S (2018) Component-based machine learning for performance prediction in building design. Appl Energy 228:1439–1453
Hammad AW (2019) Minimising the deviation between predicted and actual building performance via use of neural networks and BIM. Buildings 9(5):131
Peng Y et al (2017) A hybrid data mining approach on BIM-based building operation and maintenance. Build Environ 126:483–495
Tushar Q et al (2021) An integrated approach of BIM-enabled LCA and energy simulation: The optimized solution towards sustainable development. J Clean Prod 289:125622
Chen B et al (2021) Multiobjective optimization of building energy consumption based on BIM-DB and LSSVM-NSGA-II. J Clean Prod 294:126153
Dong Y et al (2021) Intelligent optimization: a novel framework to automatize multi-objective optimization of building daylighting and energy performances. J Buil Eng. https://doi.org/10.1016/j.jobe.2021.102804
Singh MM et al (2020) Quick energy prediction and comparison of options at the early design stage. Adv Eng Inform 46:101185
Sidani A et al (2021) Recent tools and techniques of bim-based virtual reality: a systematic review. Arch Comput Methods Eng 28(2):449–462
Schiavi B et al (2022) BIM data flow architecture with AR/VR technologies: Use cases in architecture, engineering and construction. Autom Constr 134:104054
Du J et al (2018) Zero latency: Real-time synchronization of BIM data in virtual reality for collaborative decision-making. Autom Constr 85:51–64
Chen K et al (2020) Developing Efficient Mechanisms for BIM-to-AR/VR data transfer. J Comput Civ Eng 34(5):04020037
Wolfartsberger J (2019) Analyzing the potential of Virtual Reality for engineering design review. Autom Constr 104:27–37
Shiratuddin, M.F. and W. Thabet, (2011) Utilizing a 3D game engine to develop a virtual design review system
Garbett J, Hartley T, Heesom D (2021) A multi-user collaborative BIM-AR system to support design and construction. Autom Constr 122:103487
Meža S, Turk Ž, Dolenc M (2015) Measuring the potential of augmented reality in civil engineering. Adv Eng Softw 90:1–10
Khalek IA, Chalhoub JM, Ayer SK (2019) Augmented reality for identifying maintainability concerns during design. Adv Civil Eng. https://doi.org/10.1155/2019/8547928
Lin Y-C et al (2018) Integrated BIM, game engine and VR technologies for healthcare design: a case study in cancer hospital. Adv Eng Inform 36:130–145
Boton C (2018) Supporting constructability analysis meetings with Immersive Virtual Reality-based collaborative BIM 4D simulation. Autom Constr 96:1–15
Getuli V et al (2020) BIM-based immersive Virtual Reality for construction workspace planning: a safety-oriented approach. Autom Constr 114:103160
Jiao Y et al (2013) Towards cloud augmented reality for construction application by BIM and SNS integration. Autom Constr 33:37–47
Park C-S et al (2013) A framework for proactive construction defect management using BIM, augmented reality and ontology-based data collection template. Autom Constr 33:61–71
Chen H et al (2021) Development of BIM, IoT and AR/VR technologies for fire safety and upskilling. Autom Constr 125:103631
Negri E, Fumagalli L, Macchi M (2017) A review of the roles of digital twin in CPS-based production systems. Procedia Manuf 11:939–948
Jiang F et al (2021) Digital twin and its implementations in the civil engineering sector. Autom Constr 130:103838
Ma Z et al (2020) Data-driven decision-making for equipment maintenance. Autom Constr 112:103103
Cheng JC et al (2020) Data-driven predictive maintenance planning framework for MEP components based on BIM and IoT using machine learning algorithms. Autom Constr 112:103087
Lu R, Brilakis I (2019) Digital twinning of existing reinforced concrete bridges from labelled point clusters. Autom Constr 105:102837
Lydon GP et al (2019) Coupled simulation of thermally active building systems to support a digital twin. Energy Build 202:109298
Chen, L. and J. Whyte, (2021) Understanding design change propagation in complex engineering systems using a digital twin and design structure matrix. Engineering, Construction and Architectural Management.
Pan Y, Zhang L (2021) A BIM-data mining integrated digital twin framework for advanced project management. Autom Constr 124:103564
Matthews J et al (2015) Real time progress management: Re-engineering processes for cloud-based BIM in construction. Autom Constr 58:38–47
Lu Q et al (2020) Developing a digital twin at building and city levels: case study of West Cambridge campus. J Manag Eng 36(3):05020004
Kang J-S, Chung K, Hong EJ (2021) Multimedia knowledge-based bridge health monitoring using digital twin. Multimedia Tools Appl 80(26):1–16
Ye, C., et al., (2019) A digital twin of bridges for structural health monitoring
Zhang P, Yin Z-Y, Jin Y-F (2021) State-of-the-art review of machine learning applications in constitutive modeling of soils. Arch Comput Methods Eng 28(5):3661–3686
Zhang L, Lin P (2021) Multi-objective optimization for limiting tunnel-induced damages considering uncertainties. Reliab Eng Syst Saf 216:107945
Zhang C et al (2017) Human-centered automation for resilient nuclear power plant outage control. Autom Constr 82:179–192
Schirner G et al (2013) The future of human-in-the-loop cyber-physical systems. Computer 46(1):36–45
Eskandar S, Wang J, Razavi S (2020) Human-in-the-Loop Cyber-Physical Systems for Construction Safety. Cyber-physical systems in the built environment. Springer, pp 161–173
Chapter Google Scholar
Agnisarman S et al (2019) A survey of automation-enabled human-in-the-loop systems for infrastructure visual inspection. Autom Constr 97:52–76
Ford DN, Wolf CM (2020) Smart cities with digital twin systems for disaster management. J Manag Eng 36(4):04020027
Shahat E, Hyun CT, Yeom C (2021) City digital twin potentials: a review and research agenda. Sustainability 13(6):3386
Ham Y, Kim J (2020) Participatory sensing and digital twin city: updating virtual city models for enhanced risk-informed decision-making. J Manag Eng 36(3):04020005
Fan C et al (2021) Disaster city digital twin: a vision for integrating artificial and human intelligence for disaster management. Int J Inf Manage 56:102049
Francisco A, Mohammadi N, Taylor JE (2020) Smart city digital twin-enabled energy management: toward real-time urban building energy benchmarking. J Manag Eng 36(2):04019045
Schrotter G, Hürzeler C (2020) The digital twin of the city of Zurich for urban planning. PFG–J Photogramm Remote Sensing Geoinform Sci 88(1):99–112
Xue F, Lu W (2020) A semantic differential transaction approach to minimizing information redundancy for BIM and blockchain integration. Autom Constr 118:103270
Das M, Tao X, Cheng JC (2021) BIM security: A critical review and recommendations using encryption strategy and blockchain. Autom Constr 126:103682
Kinnaird C, Geipel M, Bew M (2017) Blockchain technology: how the inventions behind bitcoin are enabling a network of trust for the built environment. Arup, London
Hunhevicz JJ, Hall DM (2020) Do you need a blockchain in construction? Use case categories and decision framework for DLT design options. Adv Eng Inform 45:101094
Zheng R et al (2019) bcBIM: a blockchain-based big data model for BIM modification audit and provenance in mobile cloud. Math Probl Eng 2019:5349538
Nawari NO, Ravindran S (2019) Blockchain and building information modeling (BIM): Review and applications in post-disaster recovery. Buildings 9(6):149
Liu Z et al (2019) Building information management (BIM) and blockchain (BC) for sustainable building design information management framework. Electronics 8(7):724
Download references
The work was supported in part by the National Natural Science Foundation of China (Nos. 72201171, 72271101), National Key Research and Development Plan (No. 2022YFC3802205), and Shanghai Sailing Program, China (No. 22YF1419100).
Author information
Authors and affiliations.
Shanghai Key Laboratory for Digital Maintenance of Buildings and Infrastructure, Department of Civil Engineering, Shanghai Jiao Tong University, 800 Dongchuan Road, Shanghai, China
School of Civil and Hydraulic Engineering, Huazhong University of Science and Technology, 1037 Luoyu Road, Hongshan District, Wuhan, 430074, Hubei, China
Limao Zhang
You can also search for this author in PubMed Google Scholar
Corresponding author
Correspondence to Limao Zhang .
Ethics declarations
Conflict of interest.
The authors declare that they have no known competing financial interests or personal relationships that could have appeared to influence the work reported in this paper.
Additional information
Publisher's note.
Springer Nature remains neutral with regard to jurisdictional claims in published maps and institutional affiliations.
Rights and permissions
Springer Nature or its licensor (e.g. a society or other partner) holds exclusive rights to this article under a publishing agreement with the author(s) or other rightsholder(s); author self-archiving of the accepted manuscript version of this article is solely governed by the terms of such publishing agreement and applicable law.
Reprints and permissions
About this article
Pan, Y., Zhang, L. Integrating BIM and AI for Smart Construction Management: Current Status and Future Directions. Arch Computat Methods Eng 30 , 1081–1110 (2023). https://doi.org/10.1007/s11831-022-09830-8
Download citation
Received : 05 March 2022
Accepted : 08 October 2022
Published : 03 November 2022
Issue Date : March 2023
DOI : https://doi.org/10.1007/s11831-022-09830-8
Share this article
Anyone you share the following link with will be able to read this content:
Sorry, a shareable link is not currently available for this article.
Provided by the Springer Nature SharedIt content-sharing initiative
- Find a journal
- Publish with us
- Track your research
Subscribe to the PwC Newsletter
Join the community, edit social preview.

Add a new code entry for this paper
Remove a code repository from this paper, mark the official implementation from paper authors, add a new evaluation result row, remove a task, add a method, remove a method, edit datasets, embodied neuromorphic artificial intelligence for robotics: perspectives, challenges, and research development stack.
4 Apr 2024 · Rachmad Vidya Wicaksana Putra , Alberto Marchisio , Fakhreddine Zayer , Jorge Dias , Muhammad Shafique · Edit social preview
Robotic technologies have been an indispensable part for improving human productivity since they have been helping humans in completing diverse, complex, and intensive tasks in a fast yet accurate and efficient way. Therefore, robotic technologies have been deployed in a wide range of applications, ranging from personal to industrial use-cases. However, current robotic technologies and their computing paradigm still lack embodied intelligence to efficiently interact with operational environments, respond with correct/expected actions, and adapt to changes in the environments. Toward this, recent advances in neuromorphic computing with Spiking Neural Networks (SNN) have demonstrated the potential to enable the embodied intelligence for robotics through bio-plausible computing paradigm that mimics how the biological brain works, known as "neuromorphic artificial intelligence (AI)". However, the field of neuromorphic AI-based robotics is still at an early stage, therefore its development and deployment for solving real-world problems expose new challenges in different design aspects, such as accuracy, adaptability, efficiency, reliability, and security. To address these challenges, this paper will discuss how we can enable embodied neuromorphic AI for robotic systems through our perspectives: (P1) Embodied intelligence based on effective learning rule, training mechanism, and adaptability; (P2) Cross-layer optimizations for energy-efficient neuromorphic computing; (P3) Representative and fair benchmarks; (P4) Low-cost reliability and safety enhancements; (P5) Security and privacy for neuromorphic computing; and (P6) A synergistic development for energy-efficient and robust neuromorphic-based robotics. Furthermore, this paper identifies research challenges and opportunities, as well as elaborates our vision for future research development toward embodied neuromorphic AI for robotics.
Code Edit Add Remove Mark official
Tasks edit add remove, datasets edit, results from the paper edit, methods edit add remove.
Help | Advanced Search
Computer Science > Robotics
Title: embodied neuromorphic artificial intelligence for robotics: perspectives, challenges, and research development stack.
Abstract: Robotic technologies have been an indispensable part for improving human productivity since they have been helping humans in completing diverse, complex, and intensive tasks in a fast yet accurate and efficient way. Therefore, robotic technologies have been deployed in a wide range of applications, ranging from personal to industrial use-cases. However, current robotic technologies and their computing paradigm still lack embodied intelligence to efficiently interact with operational environments, respond with correct/expected actions, and adapt to changes in the environments. Toward this, recent advances in neuromorphic computing with Spiking Neural Networks (SNN) have demonstrated the potential to enable the embodied intelligence for robotics through bio-plausible computing paradigm that mimics how the biological brain works, known as "neuromorphic artificial intelligence (AI)". However, the field of neuromorphic AI-based robotics is still at an early stage, therefore its development and deployment for solving real-world problems expose new challenges in different design aspects, such as accuracy, adaptability, efficiency, reliability, and security. To address these challenges, this paper will discuss how we can enable embodied neuromorphic AI for robotic systems through our perspectives: (P1) Embodied intelligence based on effective learning rule, training mechanism, and adaptability; (P2) Cross-layer optimizations for energy-efficient neuromorphic computing; (P3) Representative and fair benchmarks; (P4) Low-cost reliability and safety enhancements; (P5) Security and privacy for neuromorphic computing; and (P6) A synergistic development for energy-efficient and robust neuromorphic-based robotics. Furthermore, this paper identifies research challenges and opportunities, as well as elaborates our vision for future research development toward embodied neuromorphic AI for robotics.
Submission history
Access paper:.
- HTML (experimental)
- Other Formats
References & Citations
- Google Scholar
- Semantic Scholar
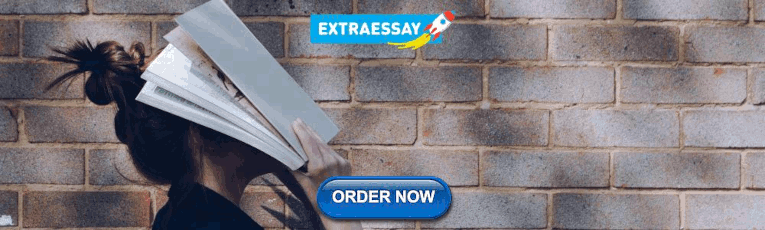
BibTeX formatted citation

Bibliographic and Citation Tools
Code, data and media associated with this article, recommenders and search tools.
- Institution
arXivLabs: experimental projects with community collaborators
arXivLabs is a framework that allows collaborators to develop and share new arXiv features directly on our website.
Both individuals and organizations that work with arXivLabs have embraced and accepted our values of openness, community, excellence, and user data privacy. arXiv is committed to these values and only works with partners that adhere to them.
Have an idea for a project that will add value for arXiv's community? Learn more about arXivLabs .
Suggestions or feedback?
MIT News | Massachusetts Institute of Technology
- Machine learning
- Social justice
- Black holes
- Classes and programs
Departments
- Aeronautics and Astronautics
- Brain and Cognitive Sciences
- Architecture
- Political Science
- Mechanical Engineering
Centers, Labs, & Programs
- Abdul Latif Jameel Poverty Action Lab (J-PAL)
- Picower Institute for Learning and Memory
- Lincoln Laboratory
- School of Architecture + Planning
- School of Engineering
- School of Humanities, Arts, and Social Sciences
- Sloan School of Management
- School of Science
- MIT Schwarzman College of Computing
Large language models use a surprisingly simple mechanism to retrieve some stored knowledge
Press contact :.
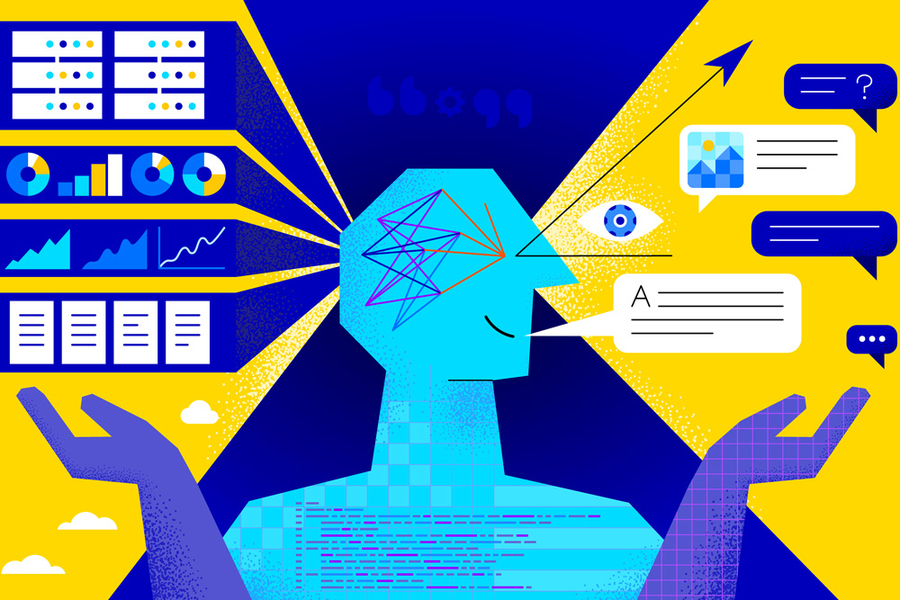
Previous image Next image
Large language models, such as those that power popular artificial intelligence chatbots like ChatGPT, are incredibly complex. Even though these models are being used as tools in many areas, such as customer support, code generation, and language translation, scientists still don’t fully grasp how they work.
In an effort to better understand what is going on under the hood, researchers at MIT and elsewhere studied the mechanisms at work when these enormous machine-learning models retrieve stored knowledge.
They found a surprising result: Large language models (LLMs) often use a very simple linear function to recover and decode stored facts. Moreover, the model uses the same decoding function for similar types of facts. Linear functions, equations with only two variables and no exponents, capture the straightforward, straight-line relationship between two variables.
The researchers showed that, by identifying linear functions for different facts, they can probe the model to see what it knows about new subjects, and where within the model that knowledge is stored.
Using a technique they developed to estimate these simple functions, the researchers found that even when a model answers a prompt incorrectly, it has often stored the correct information. In the future, scientists could use such an approach to find and correct falsehoods inside the model, which could reduce a model’s tendency to sometimes give incorrect or nonsensical answers.
“Even though these models are really complicated, nonlinear functions that are trained on lots of data and are very hard to understand, there are sometimes really simple mechanisms working inside them. This is one instance of that,” says Evan Hernandez, an electrical engineering and computer science (EECS) graduate student and co-lead author of a paper detailing these findings .
Hernandez wrote the paper with co-lead author Arnab Sharma, a computer science graduate student at Northeastern University; his advisor, Jacob Andreas, an associate professor in EECS and a member of the Computer Science and Artificial Intelligence Laboratory (CSAIL); senior author David Bau, an assistant professor of computer science at Northeastern; and others at MIT, Harvard University, and the Israeli Institute of Technology. The research will be presented at the International Conference on Learning Representations.
Finding facts
Most large language models, also called transformer models, are neural networks . Loosely based on the human brain, neural networks contain billions of interconnected nodes, or neurons, that are grouped into many layers, and which encode and process data.
Much of the knowledge stored in a transformer can be represented as relations that connect subjects and objects. For instance, “Miles Davis plays the trumpet” is a relation that connects the subject, Miles Davis, to the object, trumpet.
As a transformer gains more knowledge, it stores additional facts about a certain subject across multiple layers. If a user asks about that subject, the model must decode the most relevant fact to respond to the query.
If someone prompts a transformer by saying “Miles Davis plays the. . .” the model should respond with “trumpet” and not “Illinois” (the state where Miles Davis was born).
“Somewhere in the network’s computation, there has to be a mechanism that goes and looks for the fact that Miles Davis plays the trumpet, and then pulls that information out and helps generate the next word. We wanted to understand what that mechanism was,” Hernandez says.
The researchers set up a series of experiments to probe LLMs, and found that, even though they are extremely complex, the models decode relational information using a simple linear function. Each function is specific to the type of fact being retrieved.
For example, the transformer would use one decoding function any time it wants to output the instrument a person plays and a different function each time it wants to output the state where a person was born.
The researchers developed a method to estimate these simple functions, and then computed functions for 47 different relations, such as “capital city of a country” and “lead singer of a band.”
While there could be an infinite number of possible relations, the researchers chose to study this specific subset because they are representative of the kinds of facts that can be written in this way.
They tested each function by changing the subject to see if it could recover the correct object information. For instance, the function for “capital city of a country” should retrieve Oslo if the subject is Norway and London if the subject is England.
Functions retrieved the correct information more than 60 percent of the time, showing that some information in a transformer is encoded and retrieved in this way.
“But not everything is linearly encoded. For some facts, even though the model knows them and will predict text that is consistent with these facts, we can’t find linear functions for them. This suggests that the model is doing something more intricate to store that information,” he says.
Visualizing a model’s knowledge
They also used the functions to determine what a model believes is true about different subjects.
In one experiment, they started with the prompt “Bill Bradley was a” and used the decoding functions for “plays sports” and “attended university” to see if the model knows that Sen. Bradley was a basketball player who attended Princeton.
“We can show that, even though the model may choose to focus on different information when it produces text, it does encode all that information,” Hernandez says.
They used this probing technique to produce what they call an “attribute lens,” a grid that visualizes where specific information about a particular relation is stored within the transformer’s many layers.
Attribute lenses can be generated automatically, providing a streamlined method to help researchers understand more about a model. This visualization tool could enable scientists and engineers to correct stored knowledge and help prevent an AI chatbot from giving false information.
In the future, Hernandez and his collaborators want to better understand what happens in cases where facts are not stored linearly. They would also like to run experiments with larger models, as well as study the precision of linear decoding functions.
“This is an exciting work that reveals a missing piece in our understanding of how large language models recall factual knowledge during inference. Previous work showed that LLMs build information-rich representations of given subjects, from which specific attributes are being extracted during inference. This work shows that the complex nonlinear computation of LLMs for attribute extraction can be well-approximated with a simple linear function,” says Mor Geva Pipek, an assistant professor in the School of Computer Science at Tel Aviv University, who was not involved with this work.
This research was supported, in part, by Open Philanthropy, the Israeli Science Foundation, and an Azrieli Foundation Early Career Faculty Fellowship.
Share this news article on:
Press mentions.
Researchers at MIT have found that large language models mimic intelligence using linear functions, reports Kyle Wiggers for TechCrunch . “Even though these models are really complicated, nonlinear functions that are trained on lots of data and are very hard to understand, there are sometimes really simple mechanisms working inside them,” writes Wiggers.
Previous item Next item
Related Links
- Evan Hernandez
- Jacob Andreas
- Language and Intelligence Group
- Computer Science and Artificial Intelligence Laboratory
- Department of Electrical Engineering and Computer Science
Related Topics
- Computer science and technology
- Artificial intelligence
- Human-computer interaction
- Computer Science and Artificial Intelligence Laboratory (CSAIL)
- Electrical Engineering & Computer Science (eecs)
Related Articles
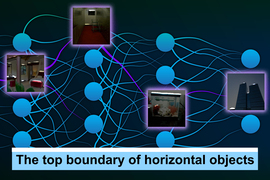
Demystifying machine-learning systems
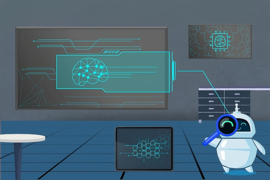
AI agents help explain other AI systems
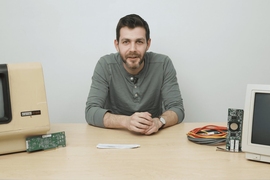
3 Questions: Jacob Andreas on large language models
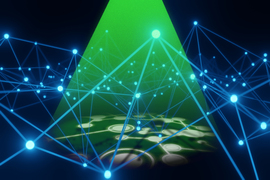
Solving a machine-learning mystery
More mit news.
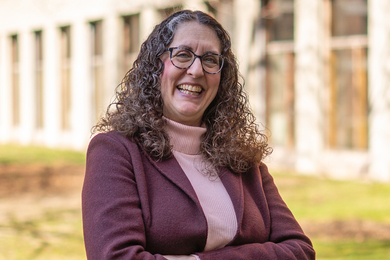
For Julie Greenberg, a career of research, mentoring, and advocacy
Read full story →
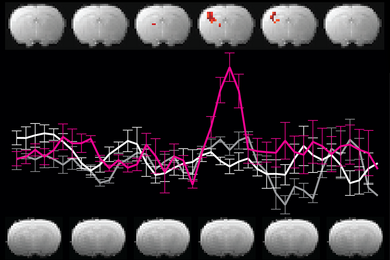
Reevaluating an approach to functional brain imaging
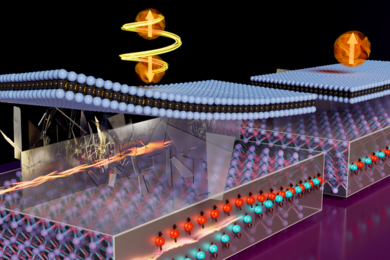
Propelling atomically layered magnets toward green computers
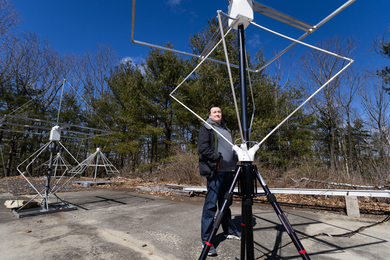
MIT Haystack scientists prepare a constellation of instruments to observe the solar eclipse’s effects
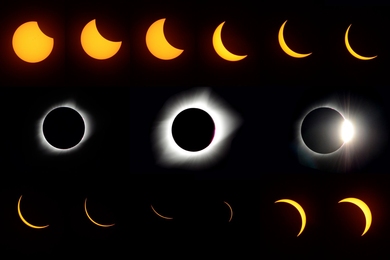
Q&A: Tips for viewing the 2024 solar eclipse
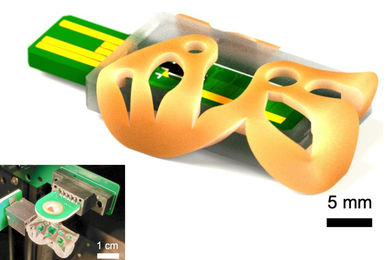
Researchers 3D print key components for a point-of-care mass spectrometer
- More news on MIT News homepage →
Massachusetts Institute of Technology 77 Massachusetts Avenue, Cambridge, MA, USA
- Map (opens in new window)
- Events (opens in new window)
- People (opens in new window)
- Careers (opens in new window)
- Accessibility
- Social Media Hub
- MIT on Facebook
- MIT on YouTube
- MIT on Instagram
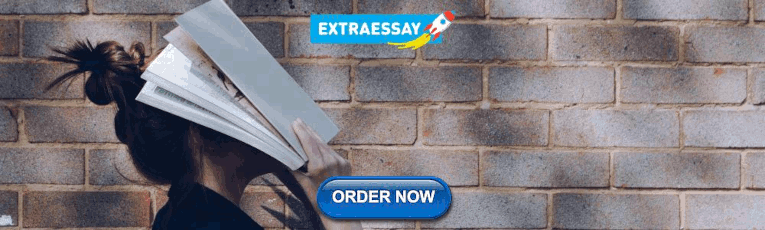
IMAGES
VIDEO
COMMENTS
The idea of developing machines exhibiting intelligence like humans traced back to several fields, which include philosophy, fiction, imagination, computer science, electronics and engineering inventions [22].Alan Turing's test for intelligence [23] was a turning point in the field of AI as the test exceeds the traditional theological positions and mathematical conclusions about the ...
This paper provides an overvie w of the recent and current. uses of AI in construct ion projects, through a descriptive anal ysis of the characteristics and contents of 86 peer-reviewed. articles ...
The development and application of generative AI in structural design can significantly enhance the efficiency and accuracy of the design process, leading to more sustainable and safer building structures. 2. Introduction to artificial intelligence (AI) methods and applications in building structural design. 2.1.
Abstract. Artificial intelligence (AI) is crucial in promoting Industry 4.0 worldwide. AI has the potential to revolutionize the engineering and construction industry by automating tasks ...
Upon scrutinizing the geographic dispersion of academic papers related to artificial intelligence research applied in the construction/execution lifecycle of the construction value chain, it was discovered that researchers in the Republic of Korea and China emerge as primary contributors to scholarly inquiry within this domain (4 articles each ...
This research also emphasises and covers the opportunities and limitations of using artificial intelligence in construction. This study explores the most significant AI applications connected to the construction industry, as well as the challenges and road to achieving the potential advantages of AI in the area.
Artificial Intelligence (AI) is fast becoming the most influential tool in the construction industry. It is the backbone of 4IR, which establishes a link between the physical and virtual (digital) worlds and uses intelligent systems and technologies [].AI is increasingly being adopted worldwide to promote the efficiency of sustainable construction management [].
This research presents an exhaustive systematic review of seventy articles toward AI applicability to the entire lifecycle of the construction value chain identified via the guidelines outlined by the Preferred Reporting Items for Systematic Reviews and Meta-Analyses (PRISMA). In recent years, there has been a surge in the global digitization of corporate processes and concepts such as digital ...
Artificial Intelligence (AI) has a great impact on increas-ing productivity and economic growth in many sectors.However, in the construction industry, AI is still limitedto research and few pilot ...
technologies in the construction industry with a specific goal (e.g., Parveen [39] focused on AI's legal issues and regulatory challenges, Schia [40] focused on AI's impact on human behavior, and Abioye [41] focused on the current state, prospects, and forthcoming challenges in the field of artificial intelligence).
being replaced by Artificial Neural Networks (ANN). While some early pioneers con-ducted relevant studies in the construction industry as early as the 1960s, e.g., Architec-ture Machine [5], this cycle of AI research in the construction industry ended with the second AI winter in the 1980s; however, studies and interest in this topic never stopped.
Construction 4.0, i.e. IDCM, includes four modules: (1) Intelligent planning and design: relying on artificial intelligence, mathematical optimization, and computer simulation of the human brain to carry out the intelligent architectural and engineering design that can meet the needs of user-friendliness characteristics; (2) Intelligent equipment and construction: with the development of heavy ...
The construction industry plays a vital role in the urbanization process and global economy, and there is a growing interest in utilizing artificial intelligence (AI) technologies to improve sustainability, productivity, and efficiency. However, there is a lack of comprehensive analysis regarding the progression of AI in the construction context, particularly from the sustainability angle.
The sources analysed categorize the relationship between Building Information Modelling (BIM) and Artificial Intelligence (AI) into four levels of integration, from no involvement to AI revolutionizing construction, with varied emphasis on their collaboration, where 50% of the database indicates negligence of integration; however, some sources ...
While there are research papers that use bibliometric software to analyze AI in construction, there is no research that manually reviews the challenges and opportunities based on AI in the planning, design, and constriction phases of construction activities. ... Potentials of artificial intelligence in construction management. organization ...
The object of research is the process of using information technology in the construction industry. ... Using these links will ensure access to this page indefinitely. Copy URL. Implementation of Artificial Intelligence in the Construction Industry and Analysis of Existing Technologies. Technology Audit and Production Reserves, 2(2(58), 12-15 ...
A theoretical framework based on the research findings is developed which illustrates the application of AI technologies across the project lifecycle and the results of each application. The aim of this paper is to develop a framework for AI in construction management, to achieve this, a sequential literature review as the research methodology shall be used. Key words such as AI construction ...
In recent times, the construction industry has experienced notable progress, especially through the amalgamation of Building Information Modelling (BIM) and Artificial Intelligence (AI). This integration holds significant potential for transforming the management of construction schedules, costs, quality, and safety, thus amplifying the overall ...
The Artificial Intelligence Ontology (AIO) is a systematization of artificial intelligence (AI) concepts, methodologies, and their interrelations. Developed via manual curation, with the additional assistance of large language models (LLMs), AIO aims to address the rapidly evolving landscape of AI by providing a comprehensive framework that encompasses both technical and ethical aspects of AI ...
This paper analyzes the application of artificial intelligence (AI) techniques in lean construction (LC) and their potential to enhance project management (PM) for improved cost and schedule ...
This study delves into the application of artificial intelligence in the stability analysis of loess tunnels, with a focus on the principles, structure, advantages, and disadvantages of the BP neural network model and the optimization of this network using genetic algorithms. The research employs a genetic algorithm-optimized BP neural network method, utilizing numerical simulation results as ...
At present, building information modeling (BIM) has been developed into a digital backbone of the architecture, engineering, and construction industry. Also, recent decades have witnessed the fast development of various AI techniques in reliably tackling a huge amount of data under complex and uncertain environments. Since both BIM and artificial intelligence (AI) have attracted sustainable ...
The convergence of materials science and artificial intelligence has unlocked new opportunities for gathering, analyzing, and generating novel materials sourced from extensive scientific literature. Despite the potential benefits, persistent challenges such as manual annotation, precise extraction, and traceability issues remain. Large language models have emerged as promising solutions to ...
To address these challenges, this paper will discuss how we can enable embodied neuromorphic AI for robotic systems through our perspectives: (P1) Embodied intelligence based on effective learning rule, training mechanism, and adaptability; (P2) Cross-layer optimizations for energy-efficient neuromorphic computing; (P3) Representative and fair ...
Artificial intelligence (AI) is a powerful technology that can be utilized throughout a construction project lifecycle. Transition to incorporate AI technologies in the construction industry has been delayed due to the lack of know-how and research.
View a PDF of the paper titled Embodied Neuromorphic Artificial Intelligence for Robotics: Perspectives, Challenges, and Research Development Stack, by Rachmad Vidya Wicaksana Putra and 4 other authors ... known as "neuromorphic artificial intelligence (AI)". However, the field of neuromorphic AI-based robotics is still at an early stage ...
1. Applications of Artificial Intelligence in Construction. Management. Ar. Gayatri Patil. Allana College of Architecture, Pune India. Abstract. Digital technology is changing the construction ...
Hernandez wrote the paper with co-lead author Arnab Sharma, a computer science graduate student at Northeastern University; his advisor, Jacob Andreas, an associate professor in EECS and a member of the Computer Science and Artificial Intelligence Laboratory (CSAIL); senior author David Bau, an assistant professor of computer science at ...
Apple researchers have developed a new artificial intelligence system that can understand ambiguous references to on-screen entities as well as conversational and background context, enabling more ...