- Research Outputs
- Output Types
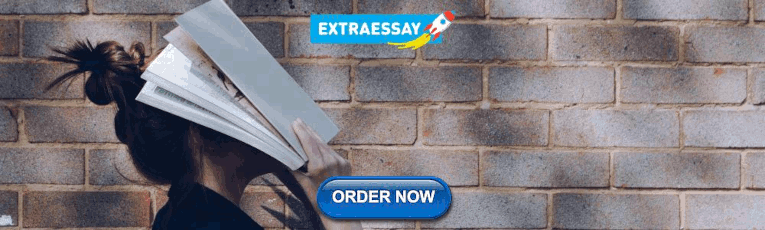
Ask a Librarian

Scholars circulate and share research in a variety of ways and in numerous genres. Below you'll find a few common examples. Keep in mind there are many other ways to circulate knowledge: factsheets, software, code, government publications, clinical guidelines, and exhibitions, just to name a few.
Outputs Defined
Original research article.
An article published in an academic journal can go by several names: original research, an article, a scholarly article, or a peer reviewed article. This format is an important output for many fields and disciplines. Original research articles are written by one or a number of authors who typically advance a new argument or idea to their field.
Conference Presentations or Proceedings
Conferences are organized events, usually centered on one field or topic, where researchers gather to present and discuss their work. Typically, presenters submit abstracts, or short summaries of their work, before a conference, and a group of organizers select a number of researchers who will present. Conference presentations are frequently transcribed and published in written form after they are given.
Books are often composed of a collection of chapters, each written by a unique author. Usually, these kinds of books are organized by theme, with each author's chapter presenting a unique argument or perspective. Books with uniquely authored chapters are often curated and organized by one or more editors, who may contribute a chapter or foreward themselves.
Often, when researchers perform their work, they will produce or work with large amounts of data, which they compile into datasets. Datasets can contain information about a wide variety of topics, from genetic code to demographic information. These datasets can then be published either independently, or as an accompaniment to another scholarly output, such as an article. Many scientific grants and journals now require researchers to publish datasets.
For some scholars, artwork is a primary research output. Scholars’ artwork can come in diverse forms and media, such as paintings, sculptures, musical performances, choreography, or literary works like poems.
Reports can come in many forms and may serve many functions. They can be authored by one or a number of people, and are frequently commissioned by government or private agencies. Some examples of reports are market reports, which analyze and predict a sector of an economy, technical reports, which can explain to researchers or clients how to complete a complex task, or white papers, which can inform or persuade an audience about a wide range of complex issues.
Digital Scholarship
Digital scholarship is a research output that significantly incorporates or relies on digital methodologies, authoring, presentation, and presentation. Digital scholarship often complements and adds to more traditional research outputs, and may be presented in a multimedia format. Some examples include mapping projects; multimodal projects that may be composed of text, visual, and audio elements; or digital, interactive archives.
Researchers from every field and discipline produce books as a research output. Because of this, books can vary widely in content, length, form, and style, but often provide a broad overview of a topic compared to research outputs that are more limited in length, such as articles or conference proceedings. Books may be written by one or many authors, and researchers may contribute to a book in a number of ways: they could author an entire book, write a forward, or collect and organize existing works in an anthology, among others.
Scholars may be called upon by media outlets to share their knowledge about the topic they study. Interviews can provide an opportunity for researchers to teach a more general audience about the work that they perform.
Article in a Newspaper or Magazine
While a significant amount of researchers’ work is intended for a scholarly audience, occasionally researchers will publish in popular newspapers or magazines. Articles in these popular genres can be intended to inform a general audience of an issue in which the researcher is an expert, or they may be intended to persuade an audience about an issue.
In addition to other scholarly outputs, many researchers also compose blogs about the work they do. Unlike books or articles, blogs are often shorter, more general, and more conversational, which makes them accessible to a wider audience. Blogs, again unlike other formats, can be published almost in real time, which can allow scholars to share current developments of their work.
- University of Colorado Boulder Libraries
- Research Guides
- Research Strategies
- Last Updated: May 14, 2024 2:33 PM
- URL: https://libguides.colorado.edu/products
- © Regents of the University of Colorado

An official website of the United States government
The .gov means it’s official. Federal government websites often end in .gov or .mil. Before sharing sensitive information, make sure you’re on a federal government site.
The site is secure. The https:// ensures that you are connecting to the official website and that any information you provide is encrypted and transmitted securely.
- Publications
- Account settings
Preview improvements coming to the PMC website in October 2024. Learn More or Try it out now .
- Advanced Search
- Journal List
- Front Comput Neurosci
Nine Criteria for a Measure of Scientific Output
Gabriel kreiman.
1 Department of Ophthalmology, Children’s Hospital, Harvard Medical School, Boston, MA, USA
2 Department of Neurology, Children’s Hospital, Harvard Medical School, Boston, MA, USA
John H. R. Maunsell
3 Department of Neurobiology, Harvard Medical School, Boston, MA, USA
Scientific research produces new knowledge, technologies, and clinical treatments that can lead to enormous returns. Often, the path from basic research to new paradigms and direct impact on society takes time. Precise quantification of scientific output in the short-term is not an easy task but is critical for evaluating scientists, laboratories, departments, and institutions. While there have been attempts to quantifying scientific output, we argue that current methods are not ideal and suffer from solvable difficulties. Here we propose criteria that a metric should have to be considered a good index of scientific output. Specifically, we argue that such an index should be quantitative, based on robust data, rapidly updated and retrospective, presented with confidence intervals, normalized by number of contributors, career stage and discipline, impractical to manipulate, and focused on quality over quantity. Such an index should be validated through empirical testing. The purpose of quantitatively evaluating scientific output is not to replace careful, rigorous review by experts but rather to complement those efforts. Because it has the potential to greatly influence the efficiency of scientific research, we have a duty to reflect upon and implement novel and rigorous ways of evaluating scientific output. The criteria proposed here provide initial steps toward the systematic development and validation of a metric to evaluate scientific output.
Introduction
Productivity is the ratio of some output value to some input value. In some enterprises productivity can be measured with high precision. A factory can easily measure how many widgets are produced per man-hour of labor. Evaluating scientific productivity, however, is trickier. The input value for scientific productivity is tractable: it might be measured in terms of years of effort by a scientist, research team, department or program, or perhaps in terms of research dollars. It is the output value for scientific productivity that is problematic.
Scientific research produces new knowledge, some fraction of which can lead to enormous returns. In the long run, science evaluates itself. History has a particularly rigorous way of revealing the value of different scientific theories and efforts. Good science leads to novel ideas and changes the way we interpret physical phenomena and the world around us. Good science influences the direction of science itself, and the development of new technologies and social policies. Poor science leads to dead ends, either because it fails to advance understanding in useful ways or because it contains important errors. Poor science produces papers that can eventually feed the fireplace, or in a more modern and ecologically friendly version, the accumulation of electronic documents.
The process of science evaluating itself is slow. Meanwhile, we need more immediate ways of evaluating scientific output. Sorting out which scientists and research directions are currently providing the most useful output is a thorny problem, but it must be done. Scientists must be evaluated for hiring and promotion, and informed decisions need to be made about how to distribute research funding. The need for evaluation goes beyond the level of individuals. It is often important to evaluate the scientific output of groups of scientists such as laboratories, departments, centers, whole institutions, and perhaps even entire fields. Similarly, funding organizations and agencies need to evaluate the output from various initiatives and funding mechanisms.
Scientific output has traditionally been assessed using peer review in the form of evaluations from a handful of experts. Expert reviewers can evaluate the rigor, value and beauty of new findings, and gauge how they advance the field. Such peer-review constitutes an important approach to evaluating scientific output and it will continue to play a critical role in many forms of evaluation. However, peer review is limited by its subjective nature and the difficulty of obtaining comments from experts that are thorough and thoughtful, and whose comments can be compared across different evaluations. These limitations have driven institutions and agencies to seek more quantitative measures that can complement and sometimes extend thorough evaluation by peers.
In the absence of good quantitative measures of scientific output, many have settled for poor ones. For example, it is often assumed, explicitly, or implicitly, that a long list of publications indicates good output. Using the number of publications as a metric emphasizes quantity rather than quality, when it is the latter that is almost always the value of interest (Siegel and Baveye, 2010 ; Refinetti, 2011 ). In an attempt to measure something closer to quality, many turn to journal impact factors (Garfield, 2006 ). The misuse of journal impact factors in evaluating scientific output has been discussed many times (e.g., Hecht et al., 1998 ; Amin and Mabe, 2000 ; Skorka, 2003 ; Hirsch, 2005 ; Editors, 2006 ; Alberts et al., 2008 ; Castelnuovo, 2008 ; Petsko, 2008 ; Simons, 2008 ; Bollen et al., 2009 ; Dimitrov et al., 2010 ; Hughes et al., 2010 among many others). We will not repeat the problems with using the impact factors of journals to evaluate the output of individual scientists here, nor will we focus on the negative effects this use has had on the process of publishing scientific articles. Instead, we note that the persistent misuse of impact factors in the face of clear evidence of its inadequacies must reflect desperation for a quantitative measure of scientific output.
Many measures of scientific output have been devised or discussed. Because most scientific output takes the form of publication in peer-reviewed journals, these measures focus on articles and citations (Bollen et al., 2009 ). They include a broad range of approaches, such as total number of citations, journal impact factors (Garfield, 2006 ), h -factor (Hirsch, 2005 ), page ranks, article download statistics, and comments using social media (e.g., Mandavilli, 2011 ). While all these approaches have merit, we believe that no existing method captures all the criteria that are needed for a rigorous and comprehensive measure of scientific output. Here we discuss what we consider necessary (but not necessarily sufficient) criteria for a metric or index of scientific output. The goal of developing quantitative criteria to evaluate scientific output is not to replace examination by expert reviewers but rather to complement peer-review efforts. The criteria that we propose are aimed toward developing a quantitative metric that is appropriately normalized, emphasizes the quality of scientific output, and can be used for rigorous, reliable comparisons. We do not propose a specific measure, which should be based on extensive testing and comparison of candidate approaches, together with feedback from interested parties. Nevertheless, we believe that a discussion of properties that would make a suitable measure may help progress toward this goal.
We propose that a good index of scientific output will need to have nine characteristics.
Data Quality and Presentation
Quantitative.
Perhaps the most important requirement of a good measure of scientific output is that it be quantitative. The primary alternative, subjective ratings by experts will continue to be important for evaluations, but nevertheless suffers from some important limitations. Ratings by a handful of invited peers, as is normally used in hiring and promoting of scientists, provide ratings of undetermined precision. Moreover, the peers providing detailed comments on different job candidates or grant applications are typically non-overlapping, making it difficult to directly compare their comments.
A further problem with subjective comments is that they put considerable demands on reviewers’ time. This makes it impractical to overcome uncertainties about comparisons between different reviewers by reaching out to a very large pool of reviewers for detailed comments. The alternative of getting brief comments from a very large pool of reviewers is also unlikely to work. Several initiatives provide frameworks for peer commentary from large sets of commenters. Most online journals provide rapid publication of comments from readers about specific articles (e.g., electronic responses for journals hosted by HighWire Press). However, few articles attract many comments, and most get none. The comments that are posted typically come from people with interest in the specific subject of the article, which means there is little overlap in the people commenting on articles in different journals. Even with comments from many peers, it remains unclear how a large set of subjective comments should be turned into a decision about scientific output.
Based on robust data
Some ventures have sought to quantify peer commentary. For example, The Faculty of 1000 maintains a large editorial board for post-publication peer review of published articles, with numerical rating being given to each rated article. Taking another approach, WebmedCentral is a journal that publishes reviewers’ comments and quantitative ratings along with published articles. However, only a small fraction of published articles are evaluated by systems like these, and many of these are rated by one or two evaluators, limiting the value of this approach as a comprehensive tool for evaluating scientific contributions. It is difficult to know how many evaluations would be needed to provide a precise evaluation of an article, but the number is clearly more than the few that are currently received for most articles. Additionally, it is difficult to assess the accuracy of the comments (should one also evaluate the comments?).
It seems very unlikely that a sufficiently broad and homogeneous set of evaluations could be obtained to achieve uniformly widespread quantitative treatment of most scientists while avoiding being dominated by people who are most vocal or who have the most free time (as opposed to people with the most expertise). There is also reason for concern that peer-rating systems could be subject to manipulation (see below). For these reasons, we believe that a reliable measure of scientific output should be based on hard data rather than subjective ratings.
One could imagine specific historical instances where subjective peer commentary could have been (and probably was) quite detrimental to scientific progress. Imagine Galileo’s statement that the Earth moves or Darwin’s Theory of Evolution being dismissed by Twitter-like commentators.
Based on data that are rapidly updated and retrospective
While other sources might be useful and should not be excluded from consideration, the obvious choice for evaluation data is the citations of peer-reviewed articles. Publication of findings in peer-reviewed journals is the sine qua non for scientific progress, so the scientific literature is the natural place to look for a measure of scientific output. Article citations fulfill several important criteria. First, because every scientist must engage in scientific publication, a measure based on citations can be used to assess any scientist or group of scientists. Second, data on article citations are readily accessible and updated regularly, so that an index of output can be up-to-date. This may be particularly important for evaluating junior scientists, who have a short track record. Finally, publication data are available for a period that spans the lives of almost all working scientists, making it possible to track trends or monitor career trajectories. Historical data are particularly important for validating any measure of scientific output (see below), and would be impractical to obtain historical rankings using peer ratings or other subjective approaches. Because citations provide an objective, quantifiable, and available resource, different indices can be compared (see Validation below) and incremental improvements can be made based on evaluation of their relative merits.
Citations are not without weaknesses as a basis for measuring scientific output. While more-cited articles tend to correlate with important new findings, articles can also be cited more because they contain important errors. Review articles are generally cited more than original research articles, and books or chapters are generally cited less. Although articles are now identified by type in databases, how these factors should be weighted in determining an individual’s contribution would need to be carefully addressed in constructing a metric. Additionally, there will be a lag between publication and citations due to the publishing process itself and due to the time required to carry out new experiments inspired by that publication.
Citations also overlook other important components of a scientist’s contribution. Scientists mentor students and postdoctorals, teach classes and give lectures, organize workshops, courses and conferences, review manuscripts and grants, generate patents, lead clinical trials, contribute methods, algorithms and data to shared repositories and reach out to the public through journalists, books, or other efforts. For this reason, subjective evaluations by well-qualified experts are likely to remain an essential component of evaluating scientific output. Some aspects of the scientific output not involving publication might be quantified and incorporated into an index of output, but some are difficult to quantify. Because it is likely that a robust index of scientific output will depend to a large extent on citation data, in the following section we restrict our discussion to citations, but without intending to exclude other data that could contribute to an index (which might be multidimensional).
We acknowledge that there are practical issues that will need to be overcome to create even the simplest metric based on citations. In particular, to perform well it will be necessary for databases to assign a unique identifier to individual authors, without which it would be impossible to evaluate anyone with names like Smith, Martin, or Nguyen. However, efforts such as these should not be a substantial obstacle and some are already underway (e.g., Author ID by PubMed or ArXiv, see Enserink, 2009 ).
Presented with distributions and confidence intervals
An index of scientific output must be presented together with an appropriate distribution or confidence interval. Considering variation and confidence intervals is commonplace in most areas of scientific research. There is something deeply inappropriate about scientists using a measure of performance without considering its precision. A substantial component of the misuse of impact factor is the failure to consider its lack of precision (e.g., Dimitrov et al., 2010 ).
While the confidence intervals for an index of output for prolific senior investigators or large programs might be narrow, those for junior investigators will be appreciable because they have had less time to affect their field. Yet it is junior investigators who are most frequently evaluated for hiring or promotion. For example, when comparing different postdoctoral candidates for a junior faculty position, it would be desirable to know the distribution of values for a given index across a large population of individuals in the same field and at the same career stage so that differences among candidates can be evaluated in the context of this distribution. Routinely providing a confidence interval with an index of performance will reveal when individuals are statistically indistinguishable and reduce the chances of misuse.
Normalization and Fairness
Normalized by number of contributors.
When evaluating the science reported in a manuscript, the quality and significance of the work are the main consideration, and the number of authors that contributed the findings is almost irrelevant. However, the situation differs when evaluating the contributions of individuals. Clearly, if a paper has only one author, that scientist deserves more credit for the work than if that author published the same paper with 10 other authors.
Defining an appropriate way to normalize for the number of contributors is not simple. Dividing credit equally among the authors is an attractive approach, but in most cases the first author listed has contributed more to an article than other individual authors. Similarly, in some disciplines the last place in the list is usually reserved for the senior investigator, and the relative credit due to a senior investigator is not well established.
Given the importance of authorship, it would not be unreasonable to require authors to explicitly assign to each author a quantitative fractional contribution. However, divvying up author credit quantitatively would not only be extremely difficult but would also probably lead to authorship disputes on a scale well beyond those that currently occur when only the order of authors must be decided. Nevertheless, some disciplines have already taken steps in this direction, with an increasing number of journals requiring explicit statements of how each author contributed to an article.
While it seems difficult to precisely quantify how different authors contribute to a given study, if such an approach came into practice, it might not take long before disciplines established standards for assigning appropriate credit for different types of contributions. Regardless of how normalization for the number of authors is done, one likely benefit of a widely used metric normalized in this way would be the rapid elimination of honorary authorship.
Normalized by discipline
Scientists comprise overlapping but distinct communities that differ considerably in their size and publication habits. Publications in some disciplines include far more citations than others, either because the discipline is larger and produces more papers, or because it has a tradition of providing more comprehensive treatment of prior work (e.g., Jemec, 2001 ; Della Sala and Crawford, 2006 ; Bollen et al., 2009 ; Fersht, 2009 ). Other factors can affect the average number of citations in an article, such as journals that restrict the number of citations that an article may include.
A simple index based on how frequently an author is cited can make investigators working in a large field that is generous with citations appear more productive than one working in a smaller field where people save extensive references for review articles. For example, if two fields are equivalent except that articles in one field reference twice the number of articles as the other field, a simple measure based on citations could make scientists in the first field appear on average to be twice as productive as those in the second. To have maximal value, an index of output based on citations should normalize for differences in the way that citations are used in different fields (including number of people in the field, etc.). Ideally, a measure would reflect an individual’s relative contribution within his or her field. It will be challenging to produce a method to normalize for such differences between disciplines in a rigorous and automatic way. Comprehensive treatment of this issue will require simulation and experimentation. Here, we will briefly mention potential approaches to illustrate a class of solutions.
There is a well-developed field of defining areas of science based on whether pairs of authors are cited in the same articles (author co-citation analysis; Griffith et al., 1986 ). More recently, these methods have been extended by automated rating of text similarity between articles (e.g., Greene et al., 2009 ). Methods like these might be adopted to define a community for any given scientist. With this approach, an investigator might self-define their community based on the literature that they consider most relevant, as reflected by the articles they cite in their own articles. For a robust definition that could not be easily manipulated (see below), an iterative process that used articles that cite cited articles, or articles that are cited by cited articles, would probably be needed. While it is difficult to anticipate what definition of a scientist’s community might be most effective, one benefit of using objective, accessible data is that alternative definitions can be tested and refined.
Once a community of articles has been defined for an investigator, the fraction of all the citations in those articles that refer to the investigator would give a measure of the investigator’s impact within that field. This might provide a much more valuable and interpretable measure than raw counts of numbers of papers or number of citations. It is conceivable that this type of analysis could also permit deeper insights. For example, it might reveal investigators who were widely cited within multiple communities, who were playing a bridging role.
Normalized for career stage
A measure that incorporated the properties discussed so far would allow a meaningful assessment of an individual’s contribution to science. It would, however, rate senior investigators as more influential than junior investigators. This is a property of many existing measures, such as total number of citations or h -index. For some purposes this is appropriate; investigators are frequently compared against others at a similar stage of their careers, and senior scientists generally have contributed more than junior scientists. However, for some decisions, such as judging which investigators are most productive per unit time, an adjustment for seniority is needed. Additionally, it might be revealing for a search committee to compare candidates for an Assistant Professor position with well-known senior investigators when they entered the rank of Assistant Professor.
This type of normalization for stage of career would be difficult to achieve for several reasons. The explosive growth in the number of journals and scientists will make precise normalization difficult. Additionally, data for when individuals entered particular stages (postdoctoral, Assistant Professor, Associate Professor, Full Professor) are not widely available. A workable approximation might be possible based on the time since an author’s first (or first n ) papers were published. Because the size of different disciplines changes with time, and the rate at which articles are cited does not remain constant, these trends would need to be compensated in making comparisons over time.
A related issue is the effect of time itself on citation rates. An earlier publication has had more time to be cited (yet scientists tend to cite more recent work). In some sense, a publication from the year 2000 with 100 citations is less notable than a publication from the year 2010 with 100 citations. A simple way to address this is to compute the number of citations per year (yet we note that this involves arguable assumptions of stationarity in citation rates).
Fostering Great Science
Impractical to manipulate.
If a metric can be manipulated, such that it can be changed through actions that are relatively easy compared to those that it is supposed to measure, people will undoubtedly exploit that weakness. Given an index that is based on an open algorithm (and the algorithm should be open, computable and readily available), it is inevitable that scientists whose livelihoods are affected by that index will come up with ingenious ways to game the system. A good index should be impractical to game so that it encourages scientists to do good science rather than working on tactics that distort the measure.
It is for this reason that measures such as the number of times an article is downloaded cannot be used. That approach would invite the generation of an industry that would surreptitiously download specific articles many times for a fee. For the same reason, a post-publication peer-review measure that depended on evaluations from small numbers of evaluators cannot be robust when careers are at stake.
A measure that is based on the number of times an author’s articles are cited should be relatively secure from gaming, assuming that the neighborhood of articles used to normalize by discipline is sufficiently large. Even a moderate-sized cartel of scientists who agreed to cite each other gratuitously would have little impact on their metrics unless their articles were so poorly cited that any manipulation would still leave them uncompetitive. Nevertheless, it seems likely that a measure based on citations should ignore self-citations and perhaps eliminate or discount citations from recent co-authors (Sala and Brooks, 2008 ).
One would hope that a key motivation for scientific inquiry is, as Feynman put it, “the pleasure of finding things out.” Yet, any metric to evaluate scientific output establishes a certain incentive structure in the research efforts. To some extent, this is unavoidable. Ideally, the incentive structure imposed by a good metric should promote great science as opposed to incentive structures that reward (even financially in some cases) merely publishing an article in specific journals or publishing a certain number of articles. A good metric might encourage collaborative efforts, interdisciplinary efforts, and innovative approaches. It would be important to continuously monitor and evaluate the effects of incentive structures imposed by any metric to ensure that they do not discourage important scientific efforts including interdisciplinary research, collaborations, adequate training, and mentoring of students and others.
Focused on quality over quantity
Most existing metrics show a monotonic dependence on the number of publications. In other words, there are no “negative” citations (but perhaps there should be!). This monotonicity can promote quantity rather than quality. Consider the following example (real numbers but fictitious names). We compare authors Joe Doe and Jane Smith who work in the same research field. Both published his or her first scientific article 12 years ago and the most recent publication from each author was in 2011. Joe has published 45 manuscripts, which have been cited a total of 591 times (mean = 13.1 citations per article, median = 6 citations per article). Jane has published 14 manuscripts, which have been cited 1782 times (mean = 127.3 citations per article median = 57 citations per article). We argue that Jane’s work is more impactful in spite of the fact that her colleague has published three times more manuscripts in the same period of time. The process of publishing a manuscript has a cost in itself including the time required for the authors to do the research and report the results, the time spent by editors, reviewers, and readers to evaluate the manuscript.
In addressing this issue, care must be taken to avoid a measure that discourages scientists from reporting solid, but apparently unexciting, results. For example, penalizing the publication of possibly uninteresting manuscripts by using the average number of citations per article would be inappropriate because it would discourage the publication of any results of below-average interest. The h -index (and variants) constitutes an interesting attempt to emphasize quality (Hirsch, 2005 ). An extension of this notion would be to apply a threshold to the number of citations: publications that do not achieve a certain minimum number of citations would not count toward the overall measure of output. This threshold would have to be defined empirically and may itself be field-dependent. This may help encourage scientists to devote more time thinking about and creating excellence rather than wasting everyone’s time with publications that few consider valuable.
Given a metric, we must be able to ask how good it is. Intuitively, one could compare different metrics by selecting the one that provides a better assessment of excellence in scientific output. The argument, however, appears circular because it seems that we need to have a priori information about excellence to compare different possible metrics. It could be argued that the scientific community will be able to evaluate whether a metric is good or not by assessing whether it correlates well with intuitive judgments about what constitutes good science and innovative scientists. While this is probably correct to some extent, this procedure has the potential to draw the problem back to subjective measures.
To circumvent these difficulties, one could attempt to develop quantitative criteria to evaluate the metrics themselves. One possibility is to compare each proposed quantitative metric against independent evaluations of scientific output (which may not be quantitative or readily available for every scientist). For example, Hirsch ( 2005 ) attempted to validate the h -index by considering Nobel laureates and showing that they typically show a relatively large h -index. In general, one would like to observe that the metric correlates with expert evaluations across a broad range of individuals with different degrees of productivity. While this approach seems intuitive and straightforward it suffers from bringing the problem back to subjective criteria.
An alternative may be to consider historical data. A good metric could provide predictive value. Imagine a set of scientists and their corresponding productivity metric values evaluated in the year 2011. We can ask how well we can predict the productivity metric values in 2011 from their corresponding values in the year 2000 or 1990. Under the assumption that the scientific productivity of a given cohort is approximately stationary, we expect that a useful metric would show a high degree of prediction power whereas a poor metric will not. Of course, many factors influence scientific productivity over time for a given individual and these would be only correlative and probabilistic inferences. Yet, the predictive value of a given metric could help establish a quantitative validation process.
Given the importance of evaluating scientific output, the potential for a plethora of metrics and the high-dimensional parameter landscape involved, it seems worth further examining and developing different and more sophisticated ways of validating these metrics. One could consider measures of scientific influence based on the spread of citations, the number of successful trainees, etc., and compare these to different proposed metrics. Ultimately, these are empirical questions that should be evaluated with the same rigor applied to other scientific endeavors.
We describe above nine criteria that, we hope, might lead to a better way of evaluating scientific output. The development of an evaluation algorithm and metric that capture these properties is not intended to eliminate other forms of peer evaluation. Subjective peer review is valuable (both pre-publication and post-publication) despite its multiple pitfalls and occasional failures, and a combination of different assessments will provide more information than any one alone.
A metric that captured the properties discussed above could provide many benefits. It might encourage better publishing practices by discouraging publication of a large number of uneventful reports or reducing the emphasis on publishing in journals with high impact factors. By highlighting the scientific contributions of individuals within a field it might restore a more appropriate premium: providing important results that other scientists feel compelled to read, think about, act upon, and cite. Placing emphasis on how often other scientists cite work may have other beneficial effects. A long CV with many least-publishable papers would quickly become visibly inferior to a shorter one with fewer but more influential papers. As mentioned above, there may be other benefits including correcting authorship practices, accurate evaluation across disciplines, and it may even help students choose a laboratory or institution for graduate studies or postdoctoral research.
In addition to evaluating the current value of a productivity metric, it may be of interest to compute the rate of change in this metric. This might help highlight individuals, laboratories, departments, or institutions that have recently excelled. Rates should also be normalized and presented alongside distributions as discussed above for the metric itself.
Although we have cast the discussion in terms of a single metric, an index of output does not need to be scalar. No single value can capture the complexities involved in scientific output. Different aspects of an investigator’s contributions may require different indices. Additionally, evaluating a research group, a research center, or a department may be distinct from evaluating an individual and require somewhat different metrics (e.g., Hughes et al., 2010 ), but once suitable measures of output are available, productivity can be evaluated in terms of either years of effort, number of people involved, research funding, and other relevant parameters.
No calculation can take the place of a thoughtful evaluation by competent peers, and even an index that is precise and accurate can be abused. Evaluators might blindly apply an index without actually assessing papers, recommendations, and other material. Evaluators might also ignore confidence intervals and try to make unjustified distinctions between the performance of individuals or programs with different, but statistically indistinguishable, metrics.
Given current technologies, the state of information science, and the wealth of data on authors, publications and citations, useful quantification of the scientific output of individuals should be attainable. While we have avoided the challenge of defining and validating specific algorithms, there is little doubt that a superior metric could be produced. Given how much is at stake in decisions about how to allocate research support, there is no excuse for failing to try to provide a measure that could end the misdirected use of impact factor, download statistics, or similar misleading criteria for judging the contributions of individuals. While the newly developed metrics may show some degree of correlation with existing ones, we have to develop indices that are question-specific (e.g., how do we evaluate a given scientist?) as opposed to using generic indices developed for other purposes (e.g., how do we evaluate a certain web site or journal?). Because it has the potential to greatly influence the efficiency of scientific research, we have a duty to reflect upon and eventually implement novel and rigorous ways of evaluating scientific output.
Conflict of Interest Statement
The authors declare that the research was conducted in the absence of any commercial or financial relationships that could be construed as a potential conflict of interest.
Acknowledgments
We thank John Hogenesch, Martin Hemberg, Marlene Cohen, and Douglas Ruff for comments and discussions.
- Alberts B., Hanson B., Kelner K. L. (2008). Reviewing peer review . Science 321 , 15. 10.1126/science.1162115 [ PubMed ] [ CrossRef ] [ Google Scholar ]
- Amin M., Mabe M. (2000). Impact factors: use and abuse . Perspect. Publ. 1 , 1–6 [ Google Scholar ]
- Bollen J., Van de Sompel H., Hagberg A., Chute R. (2009). A principal component analysis of 39 scientific impact measures . PLoS ONE 4 , e6022. 10.1371/journal.pone.0006022 [ PMC free article ] [ PubMed ] [ CrossRef ] [ Google Scholar ]
- Castelnuovo G. (2008). Ditching impact factors: time for the single researcher impact factor . BMJ 336 , 789. 10.1136/bmj.39542.610000.3A [ PMC free article ] [ PubMed ] [ CrossRef ] [ Google Scholar ]
- Della Sala S., Crawford J. R. (2006). Impact factor as we know it handicaps neuropsychology and neuropsychologists . Cortex 42 , 1–2 10.1016/S0010-9452(08)70314-9 [ PubMed ] [ CrossRef ] [ Google Scholar ]
- Dimitrov J. D., Kaveri S. V., Bayry J. (2010). Metrics: journal’s impact factor skewed by a single paper . Nature 466 , 179. 10.1038/466179b [ PubMed ] [ CrossRef ] [ Google Scholar ]
- Editors (2006). The impact factor game. It is time to find a better way to assess the scientific literature . PLoS Med. 3 , e291. 10.1371/journal.pmed.0030291 [ PMC free article ] [ PubMed ] [ CrossRef ] [ Google Scholar ]
- Enserink M. (2009). Scientific publishing. Are you ready to become a number? Science 323 , 1662–1664 10.1126/science.323.5912.324a [ PubMed ] [ CrossRef ] [ Google Scholar ]
- Fersht A. (2009). The most influential journals: impact factor and Eigenfactor . Proc. Natl. Acad. Sci. U.S.A. 106 , 6883–6884 10.1073/pnas.0903307106 [ PMC free article ] [ PubMed ] [ CrossRef ] [ Google Scholar ]
- Garfield E. (2006). The history and meaning of the journal impact factor . JAMA 295 , 90–93 10.1001/jama.295.1.90 [ PubMed ] [ CrossRef ] [ Google Scholar ]
- Greene D., Freyne J., Smyth B., Cunningham P. (2009). An Analysis of Current Trends in CBR Research Using Multi-View Clustering Technical Report UCD-CSI-2009-03. Dublin: University College Dublin. [ Google Scholar ]
- Griffith B. C., White H. D., Drott M. C., Saye J. D. (1986). Tests of methods for evaluating bibliographic databases: an analysis of the National Library of Medicine’s handling of literatures in the medical behavioral sciences . J. Am. Soc. Inf. Sci. 37 , 261–270 10.1002/asi.4630370414 [ PubMed ] [ CrossRef ] [ Google Scholar ]
- Hecht F., Hecht B. K., Sandberg A. A. (1998). The journal “impact factor”: a misnamed, misleading, misused measure . Cancer Genet. Cytogenet. 104 , 77–81 10.1016/S0165-4608(97)00459-7 [ PubMed ] [ CrossRef ] [ Google Scholar ]
- Hirsch J. E. (2005). An index to quantify an individual’s scientific research output . Proc. Natl. Acad. Sci. U.S.A. 102 , 16569–16572 10.1073/pnas.0507655102 [ PMC free article ] [ PubMed ] [ CrossRef ] [ Google Scholar ]
- Hughes M. E., Peeler J., Hogenesch J. B. (2010). Network dynamics to evaluate performance of an academic institution . Sci. Transl. Med. 2 , 53ps49. 10.1126/scitranslmed.3001580 [ PubMed ] [ CrossRef ] [ Google Scholar ]
- Jemec G. B. (2001). Impact factor to assess academic output . Lancet 358 , 1373. 10.1016/S0140-6736(01)06443-1 [ PubMed ] [ CrossRef ] [ Google Scholar ]
- Mandavilli A. (2011). Peer review: trial by Twitter . Nature 469 , 286–287 10.1038/469286a [ PubMed ] [ CrossRef ] [ Google Scholar ]
- Petsko G. A. (2008). Having an impact (factor) . Genome Biol. 9 , 107. 10.1186/gb-2008-9-9-110 [ PMC free article ] [ PubMed ] [ CrossRef ] [ Google Scholar ]
- Refinetti R. (2011). Publish and flourish . Science 331 , 29. 10.1126/science.331.6013.29-a [ PubMed ] [ CrossRef ] [ Google Scholar ]
- Sala S. D., Brooks J. (2008). Multi-authors’ self-citation: a further impact factor bias? Cortex 44 , 1139–1145 10.1016/j.cortex.2007.12.006 [ PubMed ] [ CrossRef ] [ Google Scholar ]
- Siegel D., Baveye P. (2010). Battling the paper glut . Science 329 , 1466. 10.1126/science.329.5998.1466-b [ PubMed ] [ CrossRef ] [ Google Scholar ]
- Simons K. (2008). The misused impact factor . Science 322 , 165. 10.1126/science.1165316 [ PubMed ] [ CrossRef ] [ Google Scholar ]
- Skorka P. (2003). How do impact factors relate to the real world? Nature 425 , 661. 10.1038/425661c [ PubMed ] [ CrossRef ] [ Google Scholar ]
Wellcome Open Research
Why should i publish all my research outputs using different article types.

Historically, traditional publishing venues have prioritised one research output over all else: the Research Article. Seen as the culmination of all research projects, this final paper has often taken precedent when considering publication of work.
However, this focus can undermine the value of other, less traditional outputs, and the benefits for researchers in publishing these outputs as different article types.
In this blog, we explore the importance of broadening publishable outputs, and how diverse article types on Wellcome Open Research can benefit authors.
Supporting non-traditional research outputs
To help researchers maximise the broad range of research outputs that stem from every project, Wellcome Open Research supports over 10 different article types, which enable researchers to publish results and analysis at every stage of the research lifecycle.
From Systematic Reviews and Method Articles to Study Protocols and Research Notes , these diverse article types broaden the scope of publishable work for researchers and increase visibility of these less traditional research outputs.
In fact, 53% of all articles published on Wellcome Open Research are published as a less traditional research output.

The benefits of publishing outputs as diverse article types
Broadening the scope of publishable work through different article types provides several benefits for researchers.
Increased author credit
Research projects are made up of several stages and often involve complex steps of data collection, analysis, report writing, and more. Yet, only a small amount of this is included in the final Research Article and, as a result, much of this foundational work goes unrecognised.
However, by publishing every output of a research project as its own article type, from methods in a Method Article, preliminary findings as a Research Note, or pre-research analysis as a Systematic Review, authors can get full credit for the work that goes into each research step.
Reduced research waste and improved efficiencies
A reduction in waste and an improvement in efficiency is linked to this increase in recognition.
By publishing all underlying project outputs, the research community and wider stakeholders can access this work that would otherwise be archived and, arguably, wasted.
This can help other researchers to build on the foundation of these outputs in their own studies, reduce unnecessary research repetition, and accelerate new discoveries.
Increase reproducibility and trust
By providing access to all research outputs, the entire research project becomes much more transparent. Other researchers can follow the study step-by-step – and in more depth – to reproduce and validate the original findings.
This means others can ensure that conclusions are built upon a firm foundation, boosting the research’s credibility, and building trust. This can also help to increase the chance of research being cited, replicated, and used by wider stakeholders, including policymakers.
Improve research quality
Additionally, publishing outputs earlier in the process can help authors produce higher quality work.
Every article on Wellcome Open Research is peer reviewed, which means that publishing early work, such as Study Protocols and Systematic Reviews, provides an opportunity for experts to help improve research as it evolves, as well as flag and minimize any potential issues that could become a concern further down the line.
Not only does this result in higher-quality research, but it can also save time and effort in mitigating potential issues as they arise.
Provide more in-depth analysis and enable richer peer review
Similarly, publishing research outputs as their own dedicated article allows for a much deeper analysis and discussion of each stage.
Where methods, data, software, literature reviews, and other outputs would traditionally have a small part in a final Research Article, publishing this work as an article provides space for more in-depth analysis and discussion. This can, in turn, enable much richer peer review through a more comprehensive understanding of the research.
Increase research visibility
Finally, publishing all research outputs as a dedicated article type can help boost research visibility.
Each article published on Wellcome Open Research has its own DOI and is indexed in industry databases when it passes peer review. As a result, each of these articles is discoverable, meaning readers can find a research project via many different routes rather than just through the final Research Article.
This can also lead to more citations and a potential boost in real-world impact, with an increased chance of research being discovered and used by stakeholders outside of the immediate research community.
Publishing outputs at every stage of a research project
Each article type supported by Wellcome Open Research relates to a different step of the research journey.
From Systematic Reviews, Study Protocols, and Method Articles during the concept and planning stages to Data Notes, Software Tool Articles and Research Notes during the data collection and analysis stages, the diverse article types support different outputs across the full project lifecycle.
Discover how some of the article types relate to different outputs in the infographic below.

Publish your research with Wellcome Open Research
Wellcome-funded researchers are eligible to publish their work with Wellcome Open Research at no cost to themselves, with article types available to support outputs at every stage of the journey.
Find out more about these diverse article types in our article guidelines , and submit your research for publication today .
- Sign up to our newsletter
- Follow us on Twitter
SHARE THIS POST

Collette McKenny
Content Marketing Executive at F1000
View contributor biography
Popular tags
data sharing
open research
open peer review
Peer Review
Popular posts
Using neuroimaging to understand Borderline Personality Disorder 6 Mar 2017
We need an interdisciplinary approach to solve real world problems: a case study from the COVID-19 pandemic 18 Nov 2021
Introducing Plain Language Summaries to improve research accessibility 6 Dec 2023
How does climate change impact global health? 6 Jun 2023
A new living evidence resource for mental health research 24 Apr 2023
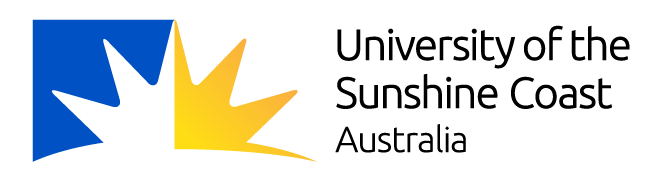
Research Output Reporting
- 2008 FoR Codes (Legacy)
Traditional Research Outputs
- Non-Traditional Research Outputs
- NTRO Research Statement
A1 - Authored Book (Research)
A2 - authored book (not scholarly), a3 - authored book (revision or new edition), a4 - edited book, b1 - book chapter (research), b2 - book chapter (not scholarly), b3 - book chapter (revision or new edition), c1 - journal article (scholarly refereed), c2 - journal article (non-refereed, scholarly journal), c3 - journal articles (other articles), c4 - edited journal issue, c5 - editorial or letter in journal issue, c6 - book reviews in journal issue, e1 - conference paper (scholarly, refereed), e2 - conference paper (full paper, non-eligible), e3 - conference abstract (published), e4 - edited conference proceedings, e5 - conference item (not meeting other criteria), d1 - reference works, f1 - audiovisual material, i - invention, g - software (computer program), n1 - newsletter, newspaper or magazine item, o1 - other output not otherwise defined, p - translation, x - externally authored publications, evidence of excellence, evidence of peer review.
- Output Weightings
Verification Material
- Category Codes
To be included in this category, the publication must meet the definition of research and must meet the Evidence of Excellence criteria below.
The types of books that may meet the criteria include:
- critical scholarly texts (e.g. music, medieval or classical texts)
- new interpretations of historical events
- new ideas or perspectives based on established research findings
- freely available research-related reports published by external institutes or public agencies and departments.
The types of books that do not meet the criteria include:
- textbooks ( possibly A2 / A2Ext)
- anthologies ( possibly A2 / A2Ext)
- edited books ( possibly A4 / A4Ext)
- creative works such as novels ( possibly J3 / J3Ext)
- translations (unless they have a major demonstrable original research component) ( possibly A2 / A2Ext)
- revisions or new editions (unless they constitute substantial new knowledge and constitutes original research) ( possibly A3 / A3Ext)
- manuals and handbooks ( possibly A2 / A2Ext)
- theses (PhD, Masters and Honours) ( possibly T1 / T1Ext).
Evidence of excellence includes:
- must be a major work of scholarship
- mainly previously unpublished material, making a substantial contribution to knowledge
- must have an ISBN
- must be offered for sale
- must be written entirely by a single author, or by joint authors who share responsibility for the whole book
- must have been published by a commercial publisher, or if not published by a commercial publisher, must have been peer reviewed.
An acceptable peer review process is one that involves impartial and independent assessment or review of the research publication in its entirety before publication, conducted by independent, qualified experts. Independent in this context means independent of the author.
For books that are not published by a commercial publisher any of the following are acceptable as evidence of peer review:
- a statement in the book which shows that contributions are peer reviewed
- a statement or acknowledgement from the publisher or editor which shows that contributions are peer reviewed
- a copy of a reviewer’s assessment relating to the book.
Standard Output
Not applicable.
Major Output
Authored books are a weighted publication category. All authored books in the A1 category are weighted 5:1 compared with the other research publication categories.
Verification material that must be provided includes:
- proof that the book was published by a commercial publisher or of peer review
- contents, preface and introduction, and pages showing all bibliographic information (ISBN, title, authors, publisher, all dates referring to copyright, publication, printing, distribution).
A book authored in its entirety by the author or authors, but does not meet the criteria for a scholarly research book.
The types of books that may meet this criteria include:
- reprints of previously published books
- books not published by a commercial publisher or peer reviewed
- books that do not meet the definition of research, and are not revised or new editions
- edited books (possibly A4 / A4Ext)
- creative published books (possibly J3 / J3Ext)
- working papers, technical reports, discussion papers (possibly O1)
- reports (possibly K1 / K1Ext / K1n)
- revisions or new editions that do not involve substantial new research (possibly A3 / A3Ext)
- proof that the book was published
This category is used for revised editions or new editions of authored books. The book must have been previously published by either the current author or some previous author.
- new editions that do not involve substantial additions or revisions
- revisions that do not involve substantial additions or revisions
- updates to previous editions that do not involve substantial additions or revisions
The types of books that may not meet the criteria include:
- revised editions that have subtantially new research (possibly A1 / A1Ext)
- contents, preface and introduction, and pages showing all bibliographic information of the new edition (ISBN, title, authors, publisher, all dates referring to copyright, publication, printing, distribution)
- table of contents from both new edition and previous edition.
This category is used for editors of a book consisting of contributions from a number of authors.
- edited journal issues ( possibly C4 )
- edited conference proceedings ( possibly E4 )
- table of contents
This category refers to a contribution consisting of substantially new material, to an edited compilation in which the material is subject to editorial scrutiny. To be included in this category, the publication must meet the definition of research and must meet the Evidence of Excellence criteria below.
The types of book chapters that may meet the criteria include:
- a scholarly introduction of chapter length to an edited volume, where the content of the introduction reports research and makes a substantial contribution to a defined area of knowledge
- a critical scholarly text of chapter length, e.g. in music, medieval or classical texts
- critical reviews of current research.
Unless they meet all of the criteria for inclusion, the following book chapters must be excluded :
- chapters in textbooks (possibly B2 / B2Ext)
- entries in reference books (possibly D1 / D1Ext)
- anthologies (possibly B2 / B2Ext)
- revisions of chapters in edited books (unless they constitute substantial new knowledge and constitutes original research) (possibly B3 / B3Ext)
- forewords (possibly B2 / B2Ext)
- brief introductions (possibly B2 / B2Ext)
- brief editorials (possibly B2 / B2Ext)
- appendices (possibly B2 / B2Ext)
- literary or creative pieces such as collections of short stories (possibly J3 / J3Ext)
- translations (unless they have a major demonstrable original research component) (possibly B2 / B2Ext)
- case studies (possibly B2 / B2Ext)
- encyclopaedia entries (possibly D1 / D1Ext).
- must have an International Standard Book Number (ISBN)
- a statement in the book which shows that contributions are peer reviewed and in the case of book chapters, which indicates which chapters are peer reviewed, if this does not apply to all content
- a copy of a reviewer’s assessment relating to the book chapter
Book chapters (research) are standard outputs.
Verification material that must be able to be provided for government reporting upon request includes:
- the chapter
- contents, preface and introduction and pages showing all bibliographic information (ISBN, title, editor, authors, publisher, all dates referring to copyright, publication, printing, distribution)
- if new chapter in revised edition, include also contents of previous edition if preface does not indicate that chapter is new
- proof that the book was published by a commercial publisher or of peer review.
This category refers to a contribution consisting of substantially new material, to an edited compilation in which the material is subject to editorial scrutiny, but does not meet the criteria for a scholarly book chapter.
The types of book chapters that may meet the criteria include:
- chapters in textbooks
- anthologies
- brief introductions
- brief editorials
- translations (unless they have a major demonstrable original research component)
- case studies
- scholarly chapters in books not published by a commercial publisher and not peer reviewed
- chapters in a report or government department publication that are not published by a commercial publisher and which appear in book format rather than report format
Unless they meet all of the criteria for inclusion, the following book chapters do not meet the criteria:
- entries in reference books (possibly D1 / D1Ext)
- revisions of chapters in edited books (unless they constitute substantial new knowledge and constitutes original research) (possibly B3 / B3Ext)
- literary or creative pieces such as collections of short stories (possibly J3 / J3Ext)
- encyclopedia entries (possibly D1 / D1Ext).
This category is for use for revised editions or new editions of chapters in an edited book. The book must have been previously published by either the current editors or some previous editor.
The types of book chapters that may meet this criteria include:
The types of book chapters that do not meet the criteria include:
- revised editions that have substantially new research (possibly B1 / B1Ext)
The types of journal articles that may meet the criteria include:
- commentaries and communications of original research
- research notes
- letters to journals, provided that the letter satisfies the definition of research and the requirements for journal articles in this section
- critical scholarly texts which appear in article form
- articles reviewing multiple works or an entire field of research
- invited papers in journals
- articles in journals which are targeted to both scholars and professionals
- articles in a stand-alone series.
The types of journal articles that do not meet the criteria include:
- letters to the editor (possibly C3 / C3Ext)
- case studies (possibly C2 / C3 / C3Ext / C2Ext )
- articles designed to inform practitioners on existing knowledge in a professional field (possibly C2 / C3 / C2Ext / C3Ext)
- articles in newspapers and popular magazines (possibly C3 / C3Ext / N1 / N1Ext)
- editorials (possibly C3 / C3Ext)
- book reviews (possibly C3 / C3Ext)
- brief commentaries and communications of original research (possibly C2 / C3 / C2Ext / C3Ext)
- reviews of art exhibitions, concerts, theatre productions (possibly C3 / J3 / C3Ext / J3Ext) .
- must be published in a scholarly journal
- must have been peer reviewed
- must have an ISSN (Note: Some journals may be regularly published as separate volumes with an ISBN rather than an ISSN. Provided that the publication is clearly identified as an edition of a journal, and not a book, articles in such publications may be eligible if they meet all other criteria)
An acceptable peer review process is one that involves an assessment or review, before publication, of the research output in its entirety by independent, qualified experts.
The term 'independent' in this context means independent of the author. A statement from an author that a research output was peer reviewed is not sufficient evidence.
For journal articles, the following are acceptable as evidence for peer review:
- listing on the ARC's Excellence in Research for Australia (ERA) latest available journal list
- classification as 'refereed' in the Ulrich's Knowledgebase
- listing in Thomson Reuters Web of Knowledge Master Journal list
- a statement in the journal which shows that contributions are peer reviewed
- a statement or acknowledgement from the journal editor which shows that contributions are peer reviewed
- a copy of a reviewer's assessment relating to the article.
Journal articles (research) are a standard output type.
Not applicable
- the article
- pages showing all bibliographic information (journal title, ISSN, dates where not provided in the article or offprint)
- proof of peer review
This category incudes journal articles in scholarly refereed publications that are not refereed.
- non-refereed journal articles
- case studies that are not refereed
- invited commentaries that are not refereed
- articles designed to inform practitioners on existing knowledge in a professional field
- brief commentaries and communications of original research
The types of journal articles that may not meet the criteria include:
- letters (possibly C3 / C3Ext)
This category may includes:
- letters to the editor
- book reviews
- editorials that do not meet the eligibility criteria for C1 journal articles
- refereed non-scholarly articles
- articles in journals without an ISSN
- short contributions (less than a page long)
Types of articles that may not be included are:
- articles in newspapers and newsletters (possibly N1 / N1Ext)
This category includes where the author is responsible for editing a complete journal issue.
- page showing editorials credit
This category includes editorials and letters within a journal issue.
- Editorials can be defined as work consisting of a statement of the opinions, beliefs, and policy of the editor or publisher of a journal.
- Letters can be defined as work addressed to the editor / editorial office, and comments on or discusses an article published earlier in the periodical, or is of interest to a specific readership.
- the editorial or letter
This category includes a written review and critical analysis of the content, scope and quality of a book or other monographic work, published in a Journal Issue.
- the book review
This category is used to claim for research contributions to publications such as a dictionary or encyclopaedia.
The types of reference works that may be include are:
- entire reference works written solely by the author/s
- single author/s contribution to an edited reference work
- series of contributions to different sections of an edited reference work
- revisions/editions of previously published reference works
Verification material that must be provided includes:
- the chapter (if a book section)
- table of contents showing all chapters/sections and page numbers
To be eligible, a conference paper must have been:
- presented at a conference, workshop or seminar of national or international significance
- peer reviewed
- published in full (the publication may appear in a number of different formats, e.g. a volume of proceedings, a special edition of a journal, a normal issue of a journal, a book, digitally on the conference or organisational website).
The types of conference publications that do not meet the criteria include:
- papers that appear only in a volume handed out to conference participants (possibly E2 / E2Ext)
- keynote addresses (possibly E5 / E5Ext)
- plenary addresses (possibly E5 / E5Ext)
- poster presentations (possibly E5 / E5Ext)
- abstracts of conference publications (possibly E3 / E3Ext) .
Evidence of excellence would include:
- be published in full. The papers may appear in any format, e.g. a volume of proceedings, a special edition of a journal, a normal issue of a journal, a book or a monograph, CD or conference or organisational web site
- be peer reviewed
- be presented at conferences, workshops or seminars of national or international significance
The term 'independent' in this context means independent of the author. A statement from an author that a research output was peer reviewed is not sufficient evidence.
For conference papers, the following are acceptable as evidence for peer review:
- a statement in the conference proceedings which shows that contributions are peer reviewed
- a statement or acknowledgement from the conference proceedings editor which shows that contributions are peer reviewed
- a copy of a reviewer’s assessment relating to the conference paper.
Conference papers are a standard output type.
- the full paper
- contents, preface and introduction and pages showing all relevant bibliographic information (ISBN, title, editor, authors, publisher, dates)
- proof that the conference is of national or international significance
This category includes papers that were delivered at a conference and may have been:
- full conference papers where no refereeing process was employed
- full conference papers that where not refereed in full (only the abstract may have been refereed)
- full conference papers that were not published in the proceedings (but may have been refereed)
- full conference papers that were not in publicly available proceedings (i.e. proceedings only available to delegates or on a restricted website)
- full conference papers where the conference is of local or state significance
The conference may be of local, state, national or international significance.
- proof of peer review (if applicable)
Conference abstracts that have been published in the conference proceedings. The abstract may or may not have been assessed by a formal peer review process.
- the abstract
Where the author is an editor of a published conference proceedings.
Conference proceedings is the official record of a conference meeting. It is a collection of documents which corresponds to the presentations given at the conference. It may include additional content.
The papers within the proceedings may or may not have been assessed by a formal peer review process.
- pages showing all relevant bibliographic information (ISBN or ISSN, title, editor, authors, publisher, dates)
Conference items not meeting other criteria, and may include:
- presentations
- keynote presentations
- workshops.
These conference items may or may not have been assessed by a formal peer review process, and may or may not have been published in the conference proceedings.
- the presentation, abstract or paper
This category includes audiovisual outputs including:
- documentaries
- non-creative works.
Outputs that may not meet this definition include:
- audiovisual that are considered a creative work (possibly J9 / J9Ext)
A computer program in source code (text) or compiled form.
This category refers to innovative software products of commercial quality and offered for sale or distributed as Shareware through a recognised publisher or distributor.
This category refers to full patents (or equivalent) on specific products or processes granted for the first time, either in Australia or in an overseas country.
This types of outputs that may meet this criteria include:
- plant breeder rights
- a product or process for which a full patent has been granted
- provisional patents
The types of outputs that may not meet this criteria include:
- applications for which no determination has been made on patent rights
- multiple rights for the same product or process
- patents that have been published but not granted
This category is defined as a representation normally to scale and on a flat medium, of a selection of material or abstract features on, or in relation to, the surface of the earth or of another celestial body.
This category may include:
- Newsletters or newsletter sections written in full by the author/s, generally referring to serial publications containing news and information of interest to the general public or to a special group
- Newspaper article are news items appearing in a general-interest newspaper or other general news periodical, containing information of current and timely interest in a field
- Magazine article consist of an article appearing in a periodical, containing information on current and timely interest in a field, targeted to a specific audience.
This category includes:
- working papers
- unpublished works
- project summaries
- discussion papers
- commentaries
- annotations
Translations of books and articles that identify modifications to the original edition, such as a new or revised preface.
All externally authored publications, published by UniSC
- << Previous: Publications Categories
- Next: Non-Traditional Research Outputs >>
- Updated: Apr 15, 2024 2:43 PM
- URL: https://libguides.usc.edu.au/HERDC-ERA
- UniSC Library YouTube
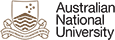
Staff Services

- Business units
- News & events
- Directories
People & culture
Research support, learning & teaching, campus environment, information technology, marketing & outreach, financial management, planning & governance.

- Respect & inclusion
- Salaries & benefits
- Supporting development
- Health & safety
- ANU Vice-Chancellor's Awards
- ANU Chancellor’s Awards
- Business travel
- Academic promotions
- Enterprise agreement
- Transition & leaving
- Funding opportunities
- Fellowships & support
- Ethics & integrity
- Research contracts
- Reporting & publications
- Research systems
- Costing & pricing
- Partner Engagement and Commercialisation
- Tools & resources
- Communities & training
- HDR supervision development
- Teaching support
- NECTAR mentoring program
- Awards & grants
- ANU Careers & Employability
- Academic integrity
- Education data
- Global programs
- Student administration
- Supporting students
- Facilities & maps
- Building management
- Transport & parking
- Safety & security
- Venues & functions
- Accommodation
- Sustainability & environment
- Campus development
- Login & access
- Print, copy, scan
- Information security
- Software & systems
- Teaching space list
- Infrastructure
- ANU Service Desk community support request form
- IT management
- ANU identity
- Digital communication
- Media engagement
- Storytelling & writing
- Events & VIP visits
- Merchandise & uniforms
- International engagements
- Ledger integrity
- Investments
- Research Financial Advisory
- Transactional services
- Procurement & contracts
- Purchase cards
- Financial frameworks
- Planning & review
- Performance measurement
- Project management framework
- Current projects
- Service improvement
- Legal services
- Risk & audit
- Recordkeeping
- Search ANU web, staff & maps
- Search current site content
Help you with all aspects of your employment including conditions, pay and other benefits, training, and wellbeing.
- Achieve & recognise

Information about research, publishing, funding, and supervision.

Information about teaching & learning, student administration and student services.
- Professional development

Information about ANU buildings, rooms, gardens, car parks, roads and more.

Technical services and infrastructure that support teaching, learning, research and administration.
- Learning space technology
- Help & support

Supporting public lectures and events, media and publications, advertising, marketing, branding and more.
Get help with financial management and administration including purchase cards.

Information relating to strategic planning and management of University business.

You are here

Publication output types & categories
There are many categories within each research output type. The Publications Collection User Guide provides a detailed explanation of each research output type and category.
The list below summarises the output types and categories and indicates those eligible for ERA.
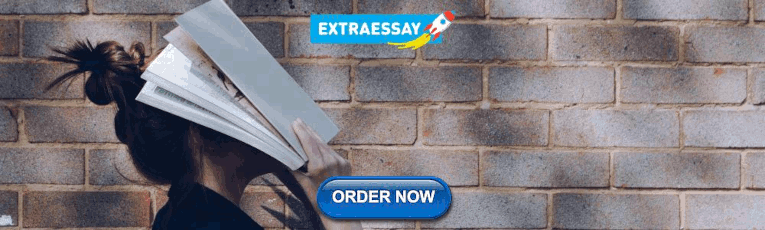
Reference documents
Use contact details to request an alternative file format.

- Contact ANU
- Freedom of Information
+61 2 6125 5111 The Australian National University, Canberra TEQSA Provider ID: PRV12002 (Australian University) CRICOS Provider : 00120C ABN : 52 234 063 906
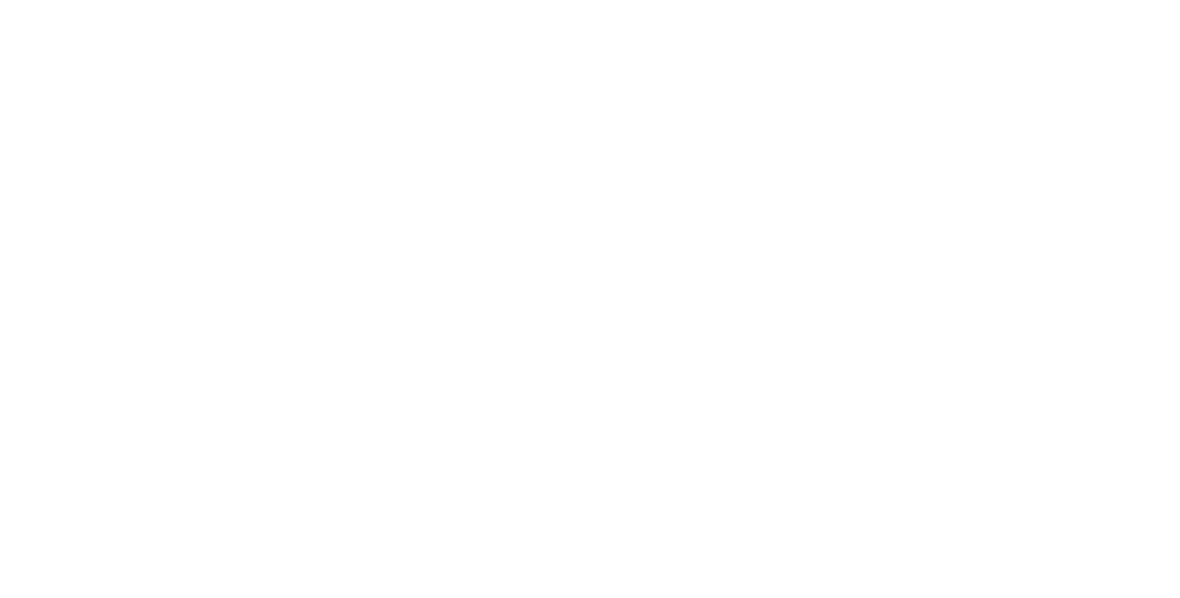
Are Markups Driving the Ups and Downs of Inflation?
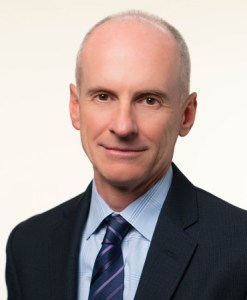
Download PDF (158 KB)
FRBSF Economic Letter 2024-12 | May 13, 2024
How much impact have price markups for goods and services had on the recent surge and the subsequent decline of inflation? Since 2021, markups have risen substantially in a few industries such as motor vehicles and petroleum. However, aggregate markups—which are more relevant for overall inflation—have generally remained flat, in line with previous economic recoveries over the past three decades. These patterns suggest that markup fluctuations have not been a main driver of the ups and downs of inflation during the post-pandemic recovery.
In the recovery from the pandemic, U.S. inflation surged to a peak of over 7% in June 2022 and has since declined to 2.7% in March 2024, as measured by the 12-month change in the personal consumption expenditures (PCE) price index. What factors have been driving the ups and downs of inflation? Production costs are traditionally considered a main contributor, particularly costs stemming from fluctuations in demand for and supply of goods and services. As demand for their products rises, companies need to hire more workers and buy more intermediate goods, pushing up production costs. Supply chain disruptions can also push up the cost of production. Firms may pass on all or part of the cost increases to consumers by raising prices. Thus, an important theoretical linkage runs from cost increases to inflation. Likewise, decreases in costs should lead to disinflation.
Labor costs are an important factor of production costs and are often useful for gauging inflationary pressures. However, during the post-pandemic surge in inflation, nominal wages rose more slowly than prices, such that real labor costs were falling until early 2023. By contrast, disruptions to global supply chains pushed up intermediate goods costs, contributing to the surge in inflation (see, for example, Liu and Nguyen 2023). However, supply chains have more direct impacts on goods inflation than on services inflation, which also rose substantially.
In this Economic Letter , we consider another factor that might drive inflation fluctuations: changes in firms’ pricing power and markups. An increase in pricing power would be reflected in price-cost markups, leading to higher inflation; likewise, a decline in pricing power and markups could alleviate inflation pressures. We use industry-level measures of markups to trace their evolving impact on inflation during the current expansion. We find that markups rose substantially in some sectors, such as the motor vehicles industry. However, the aggregate markup across all sectors of the economy, which is more relevant for inflation, has stayed essentially flat during the post-pandemic recovery. This is broadly in line with patterns during previous business cycle recoveries. Overall, our analysis suggests that fluctuations in markups were not a main driver of the post-pandemic surge in inflation, nor of the recent disinflation that started in mid-2022.
Potential drivers of inflation: Production costs and markups
To support households and businesses during the pandemic, the Federal Reserve lowered the federal funds rate target to essentially zero, and the federal government provided large fiscal transfers and increased unemployment benefits. These policies boosted demand for goods and services, especially as the economy recovered from the depth of the pandemic.
The increase in overall demand, combined with supply shortages, boosted the costs of production, contributing to the surge in inflation during the post-pandemic recovery. Although labor costs account for a large part of firms’ total production costs, real labor costs were falling between early 2021 and mid-2022 such that the increases in prices outpaced those in nominal wages. This makes it unlikely that labor costs were driving the surge in inflation.
Instead, we focus on another potential alternative driver of inflation that resulted from firms’ ability to adjust prices, known as pricing power. As demand for goods surged early in the post-pandemic recovery, companies may have had a greater ability to raise their prices above their production costs, a gap known as markups. Following a sharp drop in spending at the height of the pandemic, people may have become eager to resume normal spending patterns and hence more tolerant to price increases than in the past. In fact, growth of nonfinancial corporate profits accelerated in the early part of the recovery (see Figure 1), suggesting that companies had increased pricing power. Some studies have pointed to the strong growth in nonfinancial corporate profits in 2021 as evidence that increased markups have contributed to inflation (see, for example, Weber and Wasmer 2023). However, the figure also shows that growth in corporate profits is typically volatile. Corporate profits tend to rise in the early stages of economic recoveries. Data for the current recovery show that the increase in corporate profits is not particularly pronounced compared with previous recoveries.
Figure 1 Profit growth for nonfinancial businesses
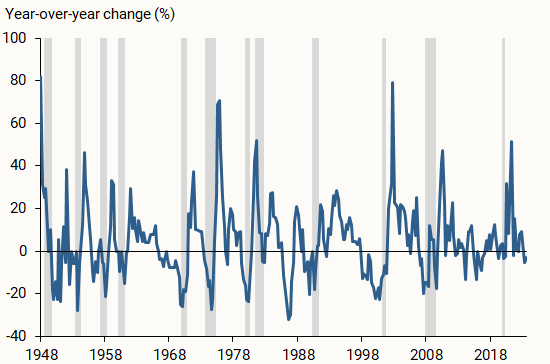
More importantly, corporate profits are an imperfect measure of a firm’s pricing power because several other factors can drive changes in profitability. For instance, much of the recent rise in corporate profits can be attributed to lower business taxes and higher subsidies from pandemic-related government support, as well as lower net interest payments due to monetary policy accommodation (Pallazzo 2023).
Instead of relying on profits as a measure of pricing power, we construct direct measures of markups based on standard economic models. Theory suggests that companies set prices as a markup over variable production costs, and that markup can be inferred from the share of a firm’s revenue spent on a given variable production factor, such as labor or intermediate goods. Over the period of data we use, we assume that the specific proportion of a company’s production costs going toward inputs does not change. If the share of a firm’s revenue used for inputs falls, it would imply a rise in the firm’s price-cost margin or markup. In our main analysis, we use industry-level data from the Bureau of Economic Analysis (BEA) to compute markups based on the share of revenue spent on intermediate inputs. Our results are similar if we instead use the share of revenue going toward labor costs.
We compare the evolution of markups to that of prices, as measured by the PCE price index, since the recovery from the pandemic. In constructing this price index, the BEA takes into account changes in product characteristics (for instance, size) that could otherwise bias the inflation measure by comparing the prices of inherently different products over time. Similarly, based upon standard economic theory, our markup measure implicitly captures changes in those characteristics (see, for example, Aghion et al. 2023).
The post-pandemic evolution of markups
We examine the evolution of markups in each industry since the third quarter of 2020, the start of the post-pandemic recovery. Figure 2 shows that some sectors, such as the motor vehicles and petroleum industries, experienced large cumulative increases in markups during the recovery. Markups also rose substantially in general merchandise, such as department stores, and for other services, such as repair and maintenance, personal care, and laundry services. Since the start of the expansion, markups in those industries rose by over 10%—comparable in size to the cumulative increases over the same period in the core PCE price index, which excludes volatile food and energy components. However, the surge in inflation through June 2022 was broad based, with prices also rising substantially outside of these sectors. Thus, understanding the importance of markups for driving inflation requires a macroeconomic perspective that examines the evolution of aggregate markups across all sectors of the economy.
Figure 2 Cumulative changes in markups for salient industries
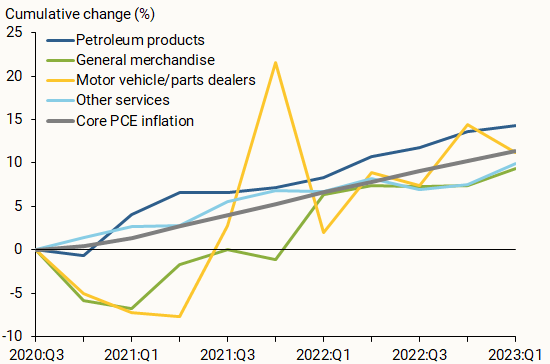
The role of aggregate markups in the economy
To assess how much markup changes contribute to movements in inflation more broadly, we use our industry-level measurements to calculate an aggregate markup at the macroeconomic level. We aggregate the cumulative changes in industry markups, applying two different weighting methods, as displayed in Figure 3. In the first method (green line), we match our industry categories to the spending categories in the core PCE price index for ease of comparison; we then use the PCE weights for each category to compute the aggregate markup. Alternatively, we use each industry’s cost weights to compute the aggregate markup (blue line). Regardless of the weighting method, Figure 3 shows that aggregate markups have stayed essentially flat since the start of the recovery, while the core PCE price index (gray line) rose by more than 10%. Thus, changes in markups are not likely to be the main driver of inflation during the recovery, which aligns with results from Glover, Mustre-del-Río, and von Ende-Becker (2023) and Hornstein (2023) using different methodologies or data. Markups also have not played much of a role in the slowing of inflation since the summer of 2022.
Figure 3 Cumulative changes in aggregate markups and prices
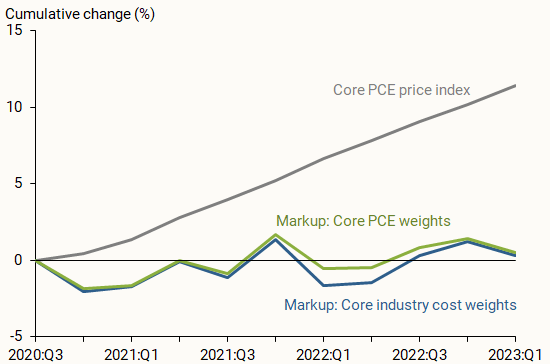
Moreover, the path of aggregate markups over the past three years is not unusual compared with previous recoveries. Figure 4 shows the cumulative changes in aggregate markups since the start of the current recovery (dark blue line), alongside aggregate markups following the 1991 (green line), 2001 (yellow line), and 2008 (light blue line) recessions. Aggregate markups have stayed roughly constant throughout all four recoveries.
Figure 4 Cumulative changes of aggregate markups in recoveries
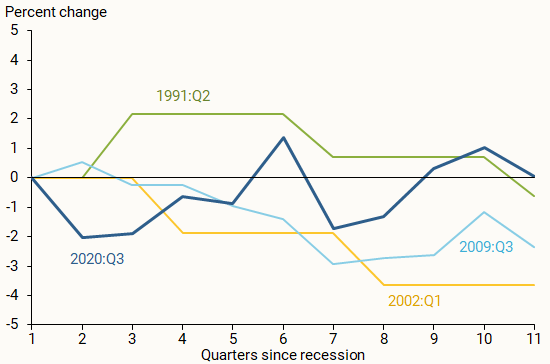
Firms’ pricing power may change over time, resulting in markup fluctuations. In this Letter , we examine whether increases in markups played an important role during the inflation surge between early 2021 and mid-2022 and if declines in markups have contributed to disinflation since then. Using industry-level data, we show that markups did rise substantially in a few important sectors, such as motor vehicles and petroleum products. However, aggregate markups—the more relevant measure for overall inflation—have stayed essentially flat since the start of the recovery. As such, rising markups have not been a main driver of the recent surge and subsequent decline in inflation during the current recovery.
Aghion, Philippe, Antonin Bergeaud, Timo Boppart, Peter J. Klenow, and Huiyu Li. 2023. “A Theory of Falling Growth and Rising Rents.” Review of Economic Studies 90(6), pp.2,675-2,702.
Glover, Andrew, José Mustre-del-Río, and Alice von Ende-Becker. 2023. “ How Much Have Record Corporate Profits Contributed to Recent Inflation? ” FRB Kansas City Economic Review 108(1).
Hornstein, Andreas. 2023. “ Profits and Inflation in the Time of Covid .” FRB Richmond Economic Brief 23-38 (November).
Liu, Zheng, and Thuy Lan Nguyen. 2023. “ Global Supply Chain Pressures and U.S. Inflation .” FRBSF Economic Letter 2023-14 (June 20).
Palazzo, Berardino. 2023. “ Corporate Profits in the Aftermath of COVID-19 .” FEDS Notes , Federal Reserve Board of Governors, September 8.
Weber, Isabella M. and Evan Wasner. 2023. “Sellers’ Inflation, Profits and Conflict: Why Can Large Firms Hike Prices in an Emergency?” Review of Keynesian Economics 11(2), pp. 183-213.
Opinions expressed in FRBSF Economic Letter do not necessarily reflect the views of the management of the Federal Reserve Bank of San Francisco or of the Board of Governors of the Federal Reserve System. This publication is edited by Anita Todd and Karen Barnes. Permission to reprint portions of articles or whole articles must be obtained in writing. Please send editorial comments and requests for reprint permission to [email protected]

Journal of Materials Chemistry C
Functionalised al(iii) metal organic frameworks for fluorescence sensing of nitroaromatic vapours.
The employment of fluorescence sensors provides a platform for rapid and efficient in-field detection of nitroaromatic compounds and is gaining increasing research ground. Herein, we report the synthesis and characterisation of three new fluorescent Al(ΙΙΙ) MOFs, structurally analogous to MIL–53, with the assigned formula {Al(OH)(bdc) 1-n (L–1) n }·xsolv (bdc 2- = terephthalate; L–1 = 2–((benzyl)amino)-terephthalate). L–1 is a strongly fluorescent dicarboxylic ligand with a pendant π–electron rich aromatic group suitable for donor-acceptor interactions with electron–deficient nitroaromatic guests. Our MOFs show strong fluorescence quenching upon exposure to vapours of nitrobenzene, 1,3-dinitrobenzene, 4-nitrotoluene, 2,4-dinitrotoluene. Additionally, we prepare and study MOF-polymer composites in the form of thin films that are strongly quenched in the presence of nitrobenzene vapours.
Supplementary files
- Supplementary information PDF (4230K)
Article information

Download Citation
Permissions.

A. Haj-Yahya, D. Kouskouki, A. G. Margellou, E. K. Andreou, G. S. Armatas and T. Lazarides, J. Mater. Chem. C , 2024, Accepted Manuscript , DOI: 10.1039/D4TC00862F
This article is licensed under a Creative Commons Attribution-NonCommercial 3.0 Unported Licence . You can use material from this article in other publications, without requesting further permission from the RSC, provided that the correct acknowledgement is given and it is not used for commercial purposes.
To request permission to reproduce material from this article in a commercial publication , please go to the Copyright Clearance Center request page .
If you are an author contributing to an RSC publication, you do not need to request permission provided correct acknowledgement is given.
If you are the author of this article, you do not need to request permission to reproduce figures and diagrams provided correct acknowledgement is given. If you want to reproduce the whole article in a third-party commercial publication (excluding your thesis/dissertation for which permission is not required) please go to the Copyright Clearance Center request page .
Read more about how to correctly acknowledge RSC content .
Social activity
Search articles by author.
This article has not yet been cited.
Advertisements
Research fund evaluation based on academic publication output analysis: the case of Chinese research fund evaluation
- Published: 04 March 2019
- Volume 119 , pages 959–972, ( 2019 )
Cite this article
- Gao Ji-ping ORCID: orcid.org/0000-0003-4965-7065 1 ,
- Su Cheng 1 ,
- Wang Hai-yan 1 ,
- Zhai Li-hua 1 &
- Pan Yun-tao 1
1296 Accesses
13 Citations
Explore all metrics
Government funding is a key scientific research resource, and it has made a concrete contribution to the world’s science and technology development. But these funds come from common taxpayers, so we need to evaluate the effectiveness of these funds. Generally speaking, policymakers adopt the method of peer review to make assessments. Compared to kinds of shortcomings of peer review, the paper here proposed the benchmarking evaluation method based on the academic publication outputs of supporting funds, mainly guiding indicators from scientometrics. At first, with the academic publication output extracted from the concluding report project manager submitted to the government after the fund finished, we designed the analysis framework to search and define the research field the fund belonged to. And then from the following three perspectives, including quantity, quality and relative influence, we compared the research fund output to the field output. Later, we took one fund program from national program on key basic research project of China (973 Program) in the field of quantum physics as an example to make an empirical analysis to demonstrate its effectiveness. At last, we found that the funded program performance was superior to the field, and even about 11.65% of the research achievement reaches the top 1/1000 of the world, but the research was lacking in remarkable papers, so it needs further improvement.
This is a preview of subscription content, log in via an institution to check access.
Access this article
Price includes VAT (Russian Federation)
Instant access to the full article PDF.
Rent this article via DeepDyve
Institutional subscriptions
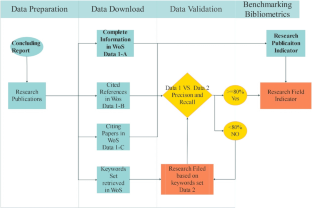
Similar content being viewed by others
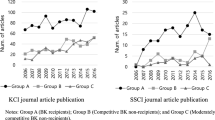
Performance-Based Research Funding and Its Impacts on Academics’ Publication Patterns in South Korea
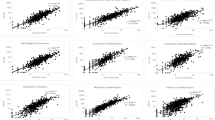
What is the relationship between research funding and citation-based performance? A comparative analysis between critical disciplines
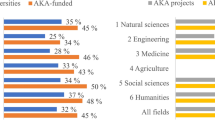
Research performance and scholarly communication profile of competitive research funding: the case of Academy of Finland
Bo, Y., & Zeng, J. (2011). Metrological analysis of national social sciences funding annual projects (2000–2009). Science and Technology Management Research, 31, 45–48.
Google Scholar
Bonzi, S., & Snyder, H. W. (1991). Motivations for citation: A comparison of self citation and citation to others. Scientometrics, 21, 245–254.
Article Google Scholar
Boyack, K. W., & Jordan, P. (2011). Metrics associated with NIH funding: A high-level view. Journal of the American Medical Informatics Association, 18, 423–431.
Cao, H., Chen, G., & Dong, E. (2013). Progress of basic research in Parkinson’s disease in China: Data mini-review from the National Natural Science Foundation. Translational neurodegeneration, 2, 18.
Chen, H., Roco, M. C., Son, J., Jiang, S., Larson, C. A., & Gao, Q. (2013). Global nanotechnology development from 1991 to 2012: Patents, scientific publications, and effect of NSF funding. Journal of Nanoparticle Research, 15, 1–21.
Costas, R., & Leeuwen, T. N. (2012). Approaching the “reward triangle”: General analysis of the presence of funding acknowledgments and “peer interactive communication” in scientific publications. Journal of the American Society for Information Science and Technology, 63, 1647–1661.
Feng, M., & Yishan, W. (2009). A survey study on motivations for citation: A case study on periodicals research and library and information science community in China. Journal of Data and Information, 2, 28–43.
Garfield, E. (1972). Citation analysis as a tool in journal evaluation. Science, 178, 471–479.
Gök, A., Rigby, J., & Shapira, P. (2016). The impact of research funding on scientific outputs: Evidence from six smaller European countries. Journal of the Association for Information Science and Technology, 67, 715–730.
Grassano, N., Rotolo, D., Hutton, J., Lang, F., & Hopkins, M. M. (2017). Funding data from publication acknowledgments: Coverage, uses, and limitations. Journal of the Association for Information Science and Technology, 68, 999–1017.
Guo, H., Pan, Y., Ma, Z., Su, C., Yu, Z., & Xu, B. (2011). Quantitative analysit of papers sponsored by Natural Science Foundation of China. Science & Technology Review, 29, 61–66.
Handley, I. M., Brown, E. R., Moss-Racusin, C. A., & Smith, J. L. (2015). Quality of evidence revealing subtle gender biases in science is in the eye of the beholder. Proceedings of the National Academy of Sciences, 112, 13201.
Hu, Z., & Wu, Y. (2014). Regularity in the time-dependent distribution of the percentage of never-cited papers: An empirical pilot study based on the six journals. Journal of Informetrics, 8, 136–146.
Hu, Z., & Wu, Y. (2017). A probe into causes of non-citation based on survey data. Social Science Information, 56, 1–13.
Huang, Z., Chen, H., Li, X., & Roco, M. C. (2006). Connecting NSF funding to patent innovation in nanotechnology (2001–2004). Journal of Nanoparticle Research, 8, 859–879.
Huang, Y., Zhang, Y., Youtie, J., Porter, A. L., & Wang, X. (2016). How does national scientific funding support emerging interdisciplinary research: A comparison study of big data research in the US and China. PLoS ONE, 11, e154509.
Khan, S., & Hanjra, M. A. (2009). Footprints of water and energy inputs in food production—global perspectives. Food Policy, 34, 130–140.
Leeuwen, T. N. V., & Moed, H. F. (2005). Characteristics of journal impact factors: The effects of uncitedness and citation distribution on the understanding of journal impact factors. Scientometrics, 63, 357–371.
Lewison, G. (1998). Gastroenterology research in the United Kingdom: funding sources and impact. Gut, 43, 288–293.
LI, G. (2004) Improving the efficiency of scientific research depends on the ouput impact. Invention and Innovation , 1–5.
Moed, H. F. (2009). New developments in the use of citation analysis in research evaluation. Archivum immunologiae et therapiae experimentalis, 57, 13.
Rigby, J. (2013). Looking for the impact of peer review: Does count of funding acknowledgements really predict research impact? Scientometrics, 94, 57–73.
Siyao, L., & Yongxin, Z. (2016). Basic research is the supply side of innovation—an interview with Yang Wei, director of the National Natural Science Foundation of China . Beijing: People’s Daily.
Tang, L., Hu, G., & Liu, W. (2017). Funding acknowledgment analysis: Queries and caveats. Journal of the Association for Information Science and Technology, 68, 790–794.
Wang, X., Liu, D., Ding, K., & Wang, X. (2011). Science funding and research output: a study on 10 countries. Scientometrics, 91, 591–599.
Wang, J., & Shapira, P. (2011). Funding acknowledgement analysis: An enhanced tool to investigate research sponsorship impacts: The case of nanotechnology. Scientometrics, 87, 563–586.
Wang, J., & Shapira, P. (2015). Is there a relationship between research sponsorship and publication impact? An analysis of funding acknowledgments in nanotechnology papers. PLoS ONE, 10, 555–582.
Wikipedia (2018) Benchmarking. https://en.wikipedia.org/wiki/Benchmarking . Accessed 24 November 2018.
Xu, X., Tan, A. M., & Zhao, S. X. (2015). Funding ratios in social science: the perspective of countries/territories level and comparison with natural sciences. Scientometrics, 104, 673–684.
Yu, Z., Ma, Z., Guo, H., Wang, N., & Jia, J. (2013). A quantitative analysis of papers sponsored by National Science and Technology programs. Journal of Intelligence, 32, 1–5.
Zhang, A., Gao, P., & Liu, S. (2010). Analysis on the output of social science paper subsidized by science foundations of countries. Information Science, 28, 705–708.
Zhao, D. (2010). Characteristics and impact of grant-funded research: A case study of the library and information science field. Scientometrics, 84, 293–306.
Zou, G., & Tang, Y. (2011). Survey and analysis on input and output of major Projects of Natural Science Fund in China. Science and Technology Management Research, 31, 32–35.
Download references
Acknowledgements
This work is supported by National Social Science Foundation of China (No. 17CTQ029). Also we would like to thank anonymous reviewers for their helpful comments.
Author information
Authors and affiliations.
Center of Scientometrics and Research Evaluation, Institute of Scientific and Technical Information of China (ISTIC), No.15 Fuxing Road, Haidian, China
Gao Ji-ping, Su Cheng, Wang Hai-yan, Zhai Li-hua & Pan Yun-tao
You can also search for this author in PubMed Google Scholar
Corresponding author
Correspondence to Gao Ji-ping .
Rights and permissions
Reprints and permissions
About this article
Gao, Jp., Su, C., Wang, Hy. et al. Research fund evaluation based on academic publication output analysis: the case of Chinese research fund evaluation. Scientometrics 119 , 959–972 (2019). https://doi.org/10.1007/s11192-019-03073-4
Download citation
Received : 03 September 2018
Published : 04 March 2019
Issue Date : 15 May 2019
DOI : https://doi.org/10.1007/s11192-019-03073-4
Share this article
Anyone you share the following link with will be able to read this content:
Sorry, a shareable link is not currently available for this article.
Provided by the Springer Nature SharedIt content-sharing initiative
- Research fund evaluation
- Academic publication output analysis
- Benchmarking bibliometrics
- Research achievement
- Scientometrics
- Find a journal
- Publish with us
- Track your research
Thank you for visiting nature.com. You are using a browser version with limited support for CSS. To obtain the best experience, we recommend you use a more up to date browser (or turn off compatibility mode in Internet Explorer). In the meantime, to ensure continued support, we are displaying the site without styles and JavaScript.
- View all journals
- Explore content
- About the journal
- Publish with us
- Sign up for alerts
- Correspondence
- Published: 15 May 2024
Science–policy research collaborations need philosophers
- Mike D. Schneider ORCID: orcid.org/0000-0002-3303-3664 1 ,
- Temitope O. Sogbanmu ORCID: orcid.org/0000-0002-8913-267X 2 , 3 ,
- Hannah Rubin ORCID: orcid.org/0000-0002-0270-9182 1 ,
- Alejandro Bortolus ORCID: orcid.org/0000-0003-3035-315X 4 ,
- Emelda E. Chukwu ORCID: orcid.org/0000-0002-3110-1678 5 ,
- Remco Heesen 6 , 7 ,
- Chad L. Hewitt ORCID: orcid.org/0000-0002-6859-6512 8 , 9 ,
- Ricardo Kaufer 10 ,
- Hanna Metzen ORCID: orcid.org/0009-0000-9116-3750 11 ,
- Veli Mitova ORCID: orcid.org/0000-0002-4181-8170 12 ,
- Anne Schwenkenbecher ORCID: orcid.org/0000-0003-2207-3043 9 , 13 ,
- Evangelina Schwindt ORCID: orcid.org/0000-0001-6693-9828 14 ,
- Helena Slanickova ORCID: orcid.org/0009-0003-5557-9654 15 ,
- Katie Woolaston ORCID: orcid.org/0000-0001-7652-9347 16 &
- Li-an Yu 17
Nature Human Behaviour ( 2024 ) Cite this article
14 Altmetric
Metrics details
- Interdisciplinary studies
First, not all philosophers are ethicists. Philosophical expertise includes expertise in conceptual work: drawing out the necessary and sufficient conditions to secure desired conclusions given the concepts introduced in the statement of an argument. This work is especially valuable in teasing apart solvable — albeit difficult — problems in evidence-based policymaking from those that are impossible to solve. For instance, as part of an international collaboration on the epistemology of evidence-based policy 6 , we targeted the basic mechanics of decision-making during ongoing empirical research to cut to the heart of what it means for the policymaking process to proceed in light of current science. This enabled us to identify root causes of disagreement in policymaking: for example, placing different importance on different kinds of evidence, miscommunication or misinterpretation of evidence, or misunderstanding of the policy process. We concluded that without a particular type of transparency — transparency of reasoning — it is impossible to determine whether anything has gone wrong in specific episodes of evidence-based policymaking based only on studying outcomes of the policymaking process (R.H. et al., unpublished).
We also identified upcoming challenges in research within the biosecurity space by teasing out which residual questions for future research extracted during a survey of the literature were themselves formulated in such a way as will require philosophical expertise to solve. For instance, many of the extracted questions were identified as involving a give-and-take between values (for example, principles, ideals or morals). Merely allocating funding towards additional empirical research is insufficient in these cases, as answering questions concerning a give-and-take of values involves some amount of reasonable disagreement, must be negotiated among relevant parties, and cannot be settled by any empirical method. How to identify when disagreement is reasonable and how to properly incorporate values into science and policy are questions discussed by philosophers, generally in the research subfield known as ‘values in science’. Importantly, the philosophical work to be done is only effectively integrated with empirical research through active collaboration: scientific research and philosophical analysis can only adequately answer crucial questions at the science–policy interface in tandem.
This is a preview of subscription content, access via your institution
Access options
Access Nature and 54 other Nature Portfolio journals
Get Nature+, our best-value online-access subscription
24,99 € / 30 days
cancel any time
Subscribe to this journal
Receive 12 digital issues and online access to articles
111,21 € per year
only 9,27 € per issue
Buy this article
- Purchase on Springer Link
- Instant access to full article PDF
Prices may be subject to local taxes which are calculated during checkout
Rittel, H. W. J. & Webber, M. M. Policy Sci. 4 , 155–169 (1973).
Article Google Scholar
Waddock, S., Meszoely, G. M., Waddell, S. & Dentoni, D. J. Organ. Change Manage. 28 , 993–1012 (2015).
Humphreys, S. Nature 619 , 35–36 (2023).
Article CAS PubMed Google Scholar
Bortolus, A. & Schwindt, E. Ecologia Austral. 32 , 767–783 (2022).
Schwenkenbecher, A. et al. Front. Mar. Sci. 10 , 1178949 (2023).
The epistemology of evidence-based policy: how philosophy can facilitate the science-policy interface. Center for Interdisciplinary Research (ZiF), Bielefeld University , https://www.uni-bielefeld.de/einrichtungen/zif/groups/previous/evidence_based_policy/ (2023).
McLevey, J. et al. Scientometrics 117 , 331–349 (2018).
Plaisance, K. S. & Elliott, K. C. Philos. Sci. 88 , 594–615 (2021).
Download references
Acknowledgements
As fellows in ‘The Epistemology of Evidence-Based Policy’ research group at the Zentrum für Interdisziplinäre Forschung (ZiF) at Bielefeld University, all authors acknowledge funding from the ZiF under grant no. RG2023/1. Additional funding to individuals comes from Volkswagen Foundation Norbert Elias Fellowships (T.O.S. and E.E.C.); National Science Foundation grant 2045007 (H.R.); Deutsche Forschungsgemeinschaft Project 254954344/GRK2073 (H.M.); Dutch Research Council and Dutch Ministry of Education, Culture and Science grant 024.003.025 (H.S.), and Proyectos de Investigación Plurianuales, Consejo Nacional de Investigaciones Científicas y Técnicas (PIP-CONICET) grant 11220210100507CO (E.S.).
Author information
Authors and affiliations.
Department of Philosophy, University of Missouri, Columbia, MO, USA
Mike D. Schneider & Hannah Rubin
Department of Zoology, University of Lagos, Lagos, Nigeria
Temitope O. Sogbanmu
Environmental Evidence Synthesis and Knowledge Translation (EESKT) Research Group, University of Lagos, Lagos, Nigeria
Instituto Patagónico para el Estudio de los Ecosistemas Continentales (IPEEC-CONICET), Puerto Madryn, Argentina
Alejandro Bortolus
Center for Infectious Diseases Research, Nigerian Institute of Medical Research, Lagos, Nigeria
Emelda E. Chukwu
Department of Philosophy, Logic and Scientific Method, London School of Economics and Political Science, London, UK
Remco Heesen
School of Humanities, Arts & Social Sciences, University of Western Australia, Perth, Western Australia, Australia
Centre for One Biosecurity Research Analysis and Synthesis, Lincoln University, Lincoln, New Zealand
Chad L. Hewitt
Centre for Biosecurity and One Health, Harry Butler Institute at Murdoch University, Perth, Western Australia, Australia
Chad L. Hewitt & Anne Schwenkenbecher
Center for Interdisciplinary Research (ZiF), Bielefeld University, Bielefeld, Germany
Ricardo Kaufer
Department of Philosophy, Bielefeld University, Bielefeld, Germany
Hanna Metzen
African Centre for Epistemology and Philosophy of Science, University of Johannesburg, Johannesburg, South Africa
Veli Mitova
School of Humanities, Arts and Social Science, Murdoch University, Perth, Western Australia, Australia
Anne Schwenkenbecher
Instituto de Biología de Organismos Marinos (IBIOMAR-CONICET), Puerto Madryn, Argentina
Evangelina Schwindt
Faculty of Philosophy, University of Groningen, Groningen, the Netherlands
Helena Slanickova
School of Law, Queensland University of Technology, Brisbane, Queensland, Australia
Katie Woolaston
Institute of European and American Studies, Academia Sinica, Taipei, Taiwan
You can also search for this author in PubMed Google Scholar
Corresponding author
Correspondence to Mike D. Schneider .
Ethics declarations
Competing interests.
The authors declare no competing interests.
Rights and permissions
Reprints and permissions
About this article
Cite this article.
Schneider, M.D., Sogbanmu, T.O., Rubin, H. et al. Science–policy research collaborations need philosophers. Nat Hum Behav (2024). https://doi.org/10.1038/s41562-024-01892-x
Download citation
Published : 15 May 2024
DOI : https://doi.org/10.1038/s41562-024-01892-x
Share this article
Anyone you share the following link with will be able to read this content:
Sorry, a shareable link is not currently available for this article.
Provided by the Springer Nature SharedIt content-sharing initiative
Quick links
- Explore articles by subject
- Guide to authors
- Editorial policies
Sign up for the Nature Briefing newsletter — what matters in science, free to your inbox daily.

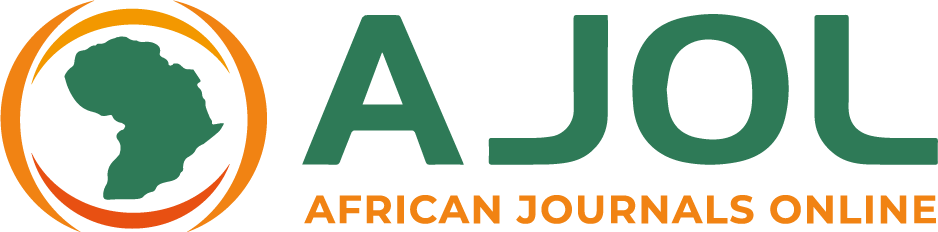
Journal of Research in Forestry, Wildlife and Environment Journal / Journal of Research in Forestry, Wildlife and Environment / Vol. 16 No. 1 (2024) / Articles (function() { function async_load(){ var s = document.createElement('script'); s.type = 'text/javascript'; s.async = true; var theUrl = 'https://www.journalquality.info/journalquality/ratings/2405-www-ajol-info-jrfwe'; s.src = theUrl + ( theUrl.indexOf("?") >= 0 ? "&" : "?") + 'ref=' + encodeURIComponent(window.location.href); var embedder = document.getElementById('jpps-embedder-ajol-jrfwe'); embedder.parentNode.insertBefore(s, embedder); } if (window.attachEvent) window.attachEvent('onload', async_load); else window.addEventListener('load', async_load, false); })();
Article sidebar.
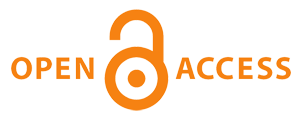
Article Details
Main article content, assessment of <i>leucaena leucocephala</i> (lam) de wit agroforestry technology adoption among smallholder farmers in benue state nigeria, t. t. torhemen, i. verinumbe, e.t. ikyaagba, g.a. ahungwa, m.a. aondoakaa, e. igbaukum.
This study was conducted to assess Leucaena leucocephala agroforestry technology adoption among smallholder farmers in Benue state. The stratified systematic random sampling technique was adopted for data collection. The state was stratified into three zones based on the senatorial districts. Respondents were stratified into three categories these were Extension agents, benefitting smallholder farmers (BSF) neighboring farmers (NF). structured questionnaire was used for data collection. Data collected were analyzed using descriptive statistic and a five-point Likert scale rating format. The study revealed that 53.3% benefitting smallholder farmers were females, 52.3% of neighboring farmers were males. Most (66.6%) of BSF were below 60 years, 74.7% of NF were below 50years. Furthermore, 51.7% of BSF had primary education while 59.3% of NF had secondary education. Hundred percent of smallholder farmers were females, 52.3% of neighboring farmers were males. All BSFs were aware of Leucaena leucocephala agroforestry technology, while 66.0% of neighboring farmers were not aware of the technology. Leucaena AF technology adoption was rated very high WMS=4.9. Farmers perceived that agroforestry significantly increase their farm output MWS=4.5, AF technology serve as fertilizer to crops on the farmland MWS=4.4 AF as staking materials MWS=4.6, windbreaks MWS=3.9, used as fuel wood MWS=4.3, treatment of disease MWS=3.8. it was shown that 60% of the farmers cultivate yam crop alongside Leucaena species, while 44.3% of farmers Combined animal and crops alongside Leucaena sp. There is the need for the Government of Benue state to renew Leucaena leucocephala agroforestry technology project in the study area; since neighboring farmers are willing to practice AF technology due to its numerous benefits. Also, there is the need for awareness creation and involvement of private or non-governmental organizations to intervene in funding and monitoring the success of this project in the study area.
AJOL is a Non Profit Organisation that cannot function without donations. AJOL and the millions of African and international researchers who rely on our free services are deeply grateful for your contribution. AJOL is annually audited and was also independently assessed in 2019 by E&Y.
Your donation is guaranteed to directly contribute to Africans sharing their research output with a global readership.
- For annual AJOL Supporter contributions, please view our Supporters page.
Journal Identifiers
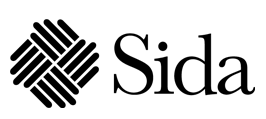
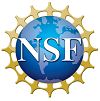
SCIENCE & ENGINEERING INDICATORS
Publications output: u.s. trends and international comparisons.
- Report PDF (816 KB)
- Report - All Formats .ZIP (4.0 MB)
- Supplemental Materials - All Formats .ZIP (25.0 MB)
- MORE DOWNLOADS OPTIONS
- Share on X/Twitter
- Share on Facebook
- Share on LinkedIn
- Send as Email
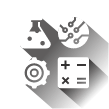
Publication Output, by Region, Country, or Economy
Publication output reached 2.6 million in 2018 ( Table 5a-1 ). Indicators 2018 : Bibliometric Data Filters sidebar )." data-bs-content="Publication output only includes those indexed in the Scopus database. The publication output discussion uses fractional counting, which credits coauthored publications according to the collaborating institutions or countries, based on the proportion of their participating authors. Country assignments refer to the institutional address of authors, with partial credit given for each international coauthorship. As part of our data analysis, we employ filters on the raw Scopus S&E publication data to remove publications with questionable quality, which appear in what are sometimes called “predatory” journals (NSB Indicators 2018 : Bibliometric Data Filters sidebar )." data-endnote-uuid="6927a2b1-65ce-4dcd-988b-175051041485"> Publication output only includes those indexed in the Scopus database. The publication output discussion uses fractional counting, which credits coauthored publications according to the collaborating institutions or countries, based on the proportion of their participating authors. Country assignments refer to the institutional address of authors, with partial credit given for each international coauthorship. As part of our data analysis, we employ filters on the raw Scopus S&E publication data to remove publications with questionable quality, which appear in what are sometimes called “predatory” journals (NSB Indicators 2018 : Bibliometric Data Filters sidebar ). The high-income economies (the United States, Germany, Japan, and other similar countries) produced 56% of S&E articles in 2018, upper-middle-income economies (China, Russia, Brazil, and other similar countries) produced 34%, and lower-middle-income economies (India, Indonesia, Pakistan, and other similar countries) produced 9% ( Figure 5a-1 and Table S5a-2 ). https://datahelpdesk.worldbank.org/knowledgebase/articles/906519-world-bank-country-and-lending-groups ." data-bs-content="This report uses the World Bank’s country income classifications issued on July 1, 2018. The World Bank updates the classifications each year on July 1. The World Bank income classifications are assigned using the gross national income per capita as measured in current U.S. dollars. This report uses the rankings. More information is available at https://datahelpdesk.worldbank.org/knowledgebase/articles/906519-world-bank-country-and-lending-groups ." data-endnote-uuid="7cc63503-e661-4f95-b85e-77d094b3b85f"> This report uses the World Bank’s country income classifications issued on July 1, 2018. The World Bank updates the classifications each year on July 1. The World Bank income classifications are assigned using the gross national income per capita as measured in current U.S. dollars. This report uses the rankings. More information is available at https://datahelpdesk.worldbank.org/knowledgebase/articles/906519-world-bank-country-and-lending-groups .
S&E articles in all fields, for 15 largest producing regions, countries, or economies: 2008 and 2018
EU = European Union.
The countries or economies are ranked based on the 2018 total. Article counts refer to publications from peer-reviewed journals and conference proceedings in S&E and indexed in Scopus (see Technical Appendix for more details). Articles are classified by their year of publication and are assigned to a region, country, or economy on the basis of the institutional address(es) of the author(s) listed in the article. Articles are credited on a fractional-count basis (i.e., for articles from multiple countries or economies, each country or economy receives fractional credit on the basis of the proportion of its participating authors). Detail may not add to total because of countries or economies that are not shown. Proportions are based on the world total excluding unclassified addresses (data not presented). Data were extracted in June 2019. Average annual change, or compound annual growth rate, is average growth rate = (year 2 publications/year 1 publications) (1/number of years) -1. See Table S5a-1 for groupings of regions, countries, or economies. Detail and other countries are available in Table S5a-2 .
National Center for Science and Engineering Statistics, National Science Foundation; Science-Metrix; Elsevier, Scopus abstract and citation database, accessed June 2019.
Science and Engineering Indicators
- For grouped bar charts, Tab to the first data element (bar/line data point) which will bring up a pop-up with the data details
- To read the data in all groups Arrow-Down will go back and forth
- For bar/line chart data points are linear and not grouped, Arrow-Down will read each bar/line data points in order
- For line charts, Arrow-Left and Arrow-Right will move to the next set of data points after Tabbing to the first data point
- For stacked bars use the Arrow-Down key again after Tabbing to the first data bar
- Then use Arrow-Right and Arrow-Left to navigate the stacked bars within that stack
- Arrow-Down to advance to the next stack. Arrow-Up reverses
Global share of S&E articles by income group: 2000–18
Article counts refer to publications from a selection of peer-reviewed journals and conference proceedings in S&E fields from Scopus. Articles are classified by their year of publication and are assigned to a region, country, or economy on the basis of the institutional address(es) of the author(s) listed in the article. Articles are credited on a fractional-count basis (i.e., for articles produced by authors from different countries, each country receives fractional credit on the basis of the proportion of its participating authors). Data are not directly comparable to Science and Engineering Indicators 2018 ; see the Technical Appendix for information on data filters. Low-income economies are not included in this figure because of their low publication output. For more information on the 2019 World Bank Country and Lending Groups classification of income groups, see https://datahelpdesk.worldbank.org/knowledgebase/articles/906519-world-bank-country-and-lending-groups , accessed January 2019. Data by country and income groups are available in Table S5a-2 .
The last decade has seen a difference in the publication growth rates of middle- and high-income economies. The number of articles from upper-middle- and lower-middle-income economies combined grew 9% per year while the output of high-income countries grew 1% per year from 2008 to 2018 ( Table S5a-2 ). The world’s average annual growth rate from 2008 to 2018 slowed to 4% compared to 6% for the 2000 to 2008 period ( Table 5a-1 and Table S5a-2 ). Indicators reports are not possible because the bibliometric database constantly updates as new articles enter the database and additional information becomes available. The publication output trends and year-to-year comparisons are valid within all the 2020 Indicators reports because they all rely upon the June 2019 Scopus database download." data-bs-content="Year-to-year comparisons across Indicators reports are not possible because the bibliometric database constantly updates as new articles enter the database and additional information becomes available. The publication output trends and year-to-year comparisons are valid within all the 2020 Indicators reports because they all rely upon the June 2019 Scopus database download." data-endnote-uuid="70b33af4-c1d9-475e-9519-c71db10e3c58"> Year-to-year comparisons across Indicators reports are not possible because the bibliometric database constantly updates as new articles enter the database and additional information becomes available. The publication output trends and year-to-year comparisons are valid within all the 2020 Indicators reports because they all rely upon the June 2019 Scopus database download.
The contribution to the world’s increasing publication output varies across countries and economies. Viewing each country’s or economy’s publication output as a share of the total global output shows that the historically large producers—United States, EU countries (combined), and Japan—have declining shares of the world’s increasing output since 2000 ( Figure 5a-2 and Figure 5a-3 ). Meanwhile China and India have increased their share of the growing world output. China produced 5% of global output in 2000 and grew to 21% in 2018; India’s share rose from 2% to 5% during this period ( Figure 5a-3 ). Among the 15 largest publication producers, countries with higher than average growth rates include South Korea (4%), Brazil (5%), China (8%), Russia (10%), India (11%), and Iran (11%) ( Table 5a-1 ).
S&E articles in all fields, for selected regions, countries, and economies and rest of world: 1996–2018
Article counts refer to publications from a selection of peer-reviewed journals and conference proceedings in S&E fields from Scopus. Articles are classified by their year of publication and are assigned to a region, country, or economy on the basis of the institutional address(es) of the author(s) listed in the article. Articles are credited on a fractional-count basis (i.e., for articles produced by authors from different countries, each country receives fractional credit on the basis of the proportion of its participating authors). Data are not directly comparable to Science and Engineering Indicators 2018 ; see Technical Appendix for information on data filters. For more information on the 2019 World Bank Country and Lending Groups classification of income groups, see https://datahelpdesk.worldbank.org/knowledgebase/articles/906519-world-bank-country-and-lending-groups , accessed January 2019. Data by country are available in Table S5a-2 .
S&E articles, by global share of selected region, country, or economy: 1996–2018
Articles are classified by their year of publication and are assigned to a region, country, or economy on the basis of the institutional address(es) of the author(s) listed in the article. Articles are credited on a fractional-count basis (i.e., for articles produced by authors from different countries, each country receives fractional credit on the basis of the proportion of its participating authors). Data are not directly comparable to Science and Engineering Indicators 2018 ; see Technical Appendix for information on data filters. For more information on the 2019 World Bank Country and Lending Groups classification of income groups, see https://datahelpdesk.worldbank.org/knowledgebase/articles/906519-world-bank-country-and-lending-groups , accessed January 2019. Data by country are available in Table S5a-2 .
The U.S. annual publication growth rate of 1% fell below the world's average annual growth (4%) ( Table 5a-1 ). EU countries that ranked among the world’s largest 15 producers had annual growth rates below the world average from 2008 to 2018: Italy (2%), Spain (2%), Germany (1%), the UK (1%), and France (0.0%) ( Table 5a-1 ). The three largest contributors to the growth in the world’s publication output—from 1.8 million in 2008 to 2.6 million in 2018—were China (35%), the EU (12%), and India (11%) ( Table 5a-1 ). The United States contributed 4% to the world’s publication research growth from 2008 to 2018.
In terms of the absolute number of publications, researchers in China and the United States produced the most S&E publications, producing 528,263 (21%) and 422,808 (17%) articles, respectively, of the 2.6 million world 2018 total ( Table 5a-1 ). The next closest countries by research publication count were India (135,788), Germany (104,396), Japan (98,793), and the UK (97,681) ( Table 5a-1 ). Nine other countries complete the list of the largest 15 countries by output: Russia, Italy, South Korea, France, Brazil, Canada, Spain, Australia, and Iran. Together, these 15 countries produced just over 75% of the world’s publication output in 2018 ( Table 5a-1 ). Figure 5a-3 and Table 5a-1 or whole counting as in Table S5a-2 . There is a slight difference between the United States and China when looking at the whole counting total production numbers. Using whole counting for 2018, the United States had 548,847 articles while China had 584,407. A whole counting measure allocates one full count to each country with an author contributing to the paper, rather than fractional counting where each country only receives a fraction. For example, if an article had four authors with two from the United States, one from China, and one from Brazil, the fractional scores would be 2/4 for the United States, 1/4 for China, and 1/4 for Brazil. The difference between whole and fractional counting indicates that the United States has more authors working with Chinese authors than China has working with U.S. authors." data-bs-content="The trends are consistent whether using fractional counting as in Figure 5a-3 and Table 5a-1 or whole counting as in Table S5a-2 . There is a slight difference between the United States and China when looking at the whole counting total production numbers. Using whole counting for 2018, the United States had 548,847 articles while China had 584,407. A whole counting measure allocates one full count to each country with an author contributing to the paper, rather than fractional counting where each country only receives a fraction. For example, if an article had four authors with two from the United States, one from China, and one from Brazil, the fractional scores would be 2/4 for the United States, 1/4 for China, and 1/4 for Brazil. The difference between whole and fractional counting indicates that the United States has more authors working with Chinese authors than China has working with U.S. authors." data-endnote-uuid="cfc5e200-65ce-494d-86a3-dee2833ef923"> The trends are consistent whether using fractional counting as in Figure 5a-3 and Table 5a-1 or whole counting as in Table S5a-2 . There is a slight difference between the United States and China when looking at the whole counting total production numbers. Using whole counting for 2018, the United States had 548,847 articles while China had 584,407. A whole counting measure allocates one full count to each country with an author contributing to the paper, rather than fractional counting where each country only receives a fraction. For example, if an article had four authors with two from the United States, one from China, and one from Brazil, the fractional scores would be 2/4 for the United States, 1/4 for China, and 1/4 for Brazil. The difference between whole and fractional counting indicates that the United States has more authors working with Chinese authors than China has working with U.S. authors. The top 15 producers of S&E articles have been stable over the last 10 years with the exception of Iran replacing Taiwan ( Table 5a-1 and Table S5a-2 ).
Related Content
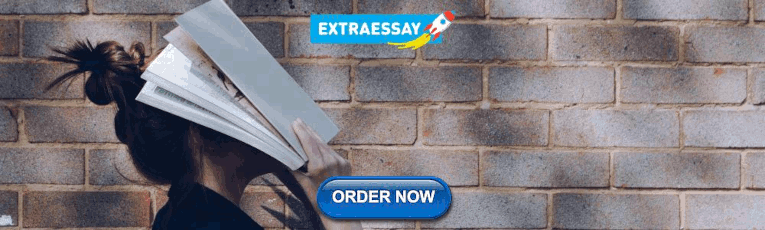
COMMENTS
This report presents data on conference proceedings and peer-reviewed science and engineering journals that reflect the expanding volume of research activity, the involvement and scientific capabilities of different countries, and the growing research ecosystem demonstrated through international collaborations. Publication output grew 5% over the past 4 years, led by China and other middle ...
An article published in an academic journal can go by several names: original research, an article, a scholarly article, or a peer reviewed article. This format is an important output for many fields and disciplines. Original research articles are written by one or a number of authors who typically advance a new argument or idea to their field.
Data on publication output indicate an increase in global research activity, a growth in the involvement and scientific capabilities of middle-income countries, and an internationally connected research ecosystem. Publication output reached 2.9 million articles in 2020, based on data from the Scopus database of S&E publications.
Total worldwide S&E publication output reached 3.3 million articles in 2022, based on entries in the Scopus database. Approximately 86% of publications in 2022 came from regions, countries, or economies with high-income and upper-middle-income economies ( Figure PBS-1 ). The number of publications from all income-level groups grew between 2003 ...
Future improvements in research evaluation strategies can incentivize Open Science and the greater dissemination of research outputs. 48 , 49 Ultimately, the considered and transparent application and interpretation of publication metrics may help address some of the social inequities in science, provide more opportunity for under‐represented ...
The Nature Index provides absolute Count and fractional Share counts of article publication at the institutional and national level and, ... is an indicator of global high-quality research output ...
Established concepts used in research evaluation such as "impact factor" and "high impact research" refer to measures of publication and citations of research, but do not measure actual ...
They include a broad range of approaches, such as total number of citations, journal impact factors (Garfield, 2006 ), h -factor (Hirsch, 2005 ), page ranks, article download statistics, and comments using social media (e.g., Mandavilli, 2011 ). While all these approaches have merit, we believe that no existing method captures all the criteria ...
Historically, traditional publishing venues have prioritised one research output over all else: the Research Article. Seen as the culmination of all research projects, this final paper has often taken precedent when considering publication of work. However, this focus can undermine the value of other, less traditional outputs, and the benefits ...
The Nature Index provides absolute and fractional counts of article publication at the institutional and national level and, as such, is an indicator of global high-quality research output and ...
Altmetrics are sourced from the Web tell about how often journal articles and other scholarly outputs like datasets are discussed and used worldwide. 2.1.3. Author-level metrics. a) Scholarly Output, Journal Count, and Journal Category Count: Journal-ranking lists have a significant influence on academics' publication strategies. Thus ...
The ever-increasing evaluation of science has led to the development of indicators at different levels. Our objective is to describe and analyze the publication output of those countries that were most active in the field between 2011 and 2020 according to the data retrieved for this category in Web of Science Core Collection. To this purpose, we are using Garfield's Impact Factor and ...
These databases provide a wealth of information about the research output of authors and institutions, including information on the number of publications, citations, and other research metrics. Web of Science is a database that provides access to scientific literature in the natural sciences, social sciences, and humanities. It covers a broad ...
First, the research output (e.g., journal articles) should be available for audiences in different countries. Nowadays, for an article to have global visibility, it should be published in a journal indexed in major databases, such as Web of Science or Scopus, and ideally be well-ranked in these databases. ...
Provided that the publication is clearly identified as an edition of a journal, and not a book, articles in such publications may be eligible if they meet all other criteria) Evidence of Peer Review An acceptable peer review process is one that involves an assessment or review, before publication, of the research output in its entirety by ...
This report utilizes data from the Scopus database of global S&E publications and finds that worldwide S&E publication output continues to grow on average at nearly 4% per year; from 2008 to 2018, output grew from 1.8 million to 2.6 million articles. In 2018, China (with a share of 21%) and the United States (with a share of 17%) were the ...
Publication output types & categories. There are many categories within each research output type. The Publications Collection User Guide provides a detailed explanation of each research output type and category. The list below summarises the output types and categories and indicates those eligible for ERA. Research output type.
The most straightforward research output measure is the number of (peer-reviewed) articles. ... In particular, this includes their previous research track record (publication experience and ...
The empirical results on the relationship between academic knowledge/research output and economic growth is far from clear-cut or conclusive. The empirical literature can be divided into two main set of studies (see Table 1).The first includes studies that consider global research output, without distinguishing research fields (De Moya-Anegón and Herrero-Solana 1999; Lee et al. 2011; Inglesi ...
These patterns suggest that markup fluctuations have not been a main driver of the ups and downs of inflation during the post-pandemic recovery. In the recovery from the pandemic, U.S. inflation surged to a peak of over 7% in June 2022 and has since declined to 2.7% in March 2024, as measured by the 12-month change in the personal consumption ...
Electrochemical energy conversion and storage systems have become an integral part towards a sustainable future, where the goal is to achieve high energy efficiency for each targeted application. The output of those devices is governed by the material design and the underlying interfacial chemistry at the ju Journal of Materials Chemistry A Recent Review Articles
For example, astronomy and astrophysics articles comprised 0.8% of the U.S. S&E publication output ( Table S5a-17 ). Normalizing the 0.8% by the world average of 0.5% in that field shows the U.S. specialization index for astronomy and astrophysics is 1.6, which is above the world average of 1.0 ( Table S5a-17 and Table S5a-19 ).
May 14, 2024 8:00 am ET. Text. Fake studies have flooded the publishers of top scientific journals, leading to thousands of retractions and millions of dollars in lost revenue. The biggest hit has ...
The employment of fluorescence sensors provides a platform for rapid and efficient in-field detection of nitroaromatic compounds and is gaining increasing research ground. Herein, we report the synthesis and characterisation of three new fluorescent Al(ΙΙΙ) MOFs, structurally analogous to MIL-53, with the as
General anesthetics disrupt brain network dynamics through multiple pathways, in part through post-synaptic potentiation of inhibitory ion channels as well as pre-synaptic inhibition of neuroexocytosis. Common clinical general anesthetic drugs, such as propofol and isoflurane, have been shown to interact and interfere with core components of the exocytic release machinery to cause impaired ...
Government funding is a key scientific research resource, and it has made a concrete contribution to the world's science and technology development. But these funds come from common taxpayers, so we need to evaluate the effectiveness of these funds. Generally speaking, policymakers adopt the method of peer review to make assessments. Compared to kinds of shortcomings of peer review, the ...
At the science-policy interface, all problems can look wicked: research exposes the complexity that is relevant to designing, executing and implementing policy fit for ambitious human needs 3, 4 ...
Journal of Research in Forestry, Wildlife and Environment ... Farmers perceived that agroforestry significantly increase their farm output MWS=4.5, AF technology serve as fertilizer to crops on the farmland MWS=4.4 AF as staking materials MWS=4.6, windbreaks MWS=3.9, used as fuel wood MWS=4.3, treatment of disease MWS=3.8. it was shown that 60% ...
The three largest contributors to the growth in the world's publication output—from 1.8 million in 2008 to 2.6 million in 2018—were China (35%), the EU (12%), and India (11%) ( Table 5a-1 ). The United States contributed 4% to the world's publication research growth from 2008 to 2018. In terms of the absolute number of publications ...