
An official website of the United States government
The .gov means it’s official. Federal government websites often end in .gov or .mil. Before sharing sensitive information, make sure you’re on a federal government site.
The site is secure. The https:// ensures that you are connecting to the official website and that any information you provide is encrypted and transmitted securely.
- Publications
- Account settings
Preview improvements coming to the PMC website in October 2024. Learn More or Try it out now .
- Advanced Search
- Journal List
- HHS Author Manuscripts

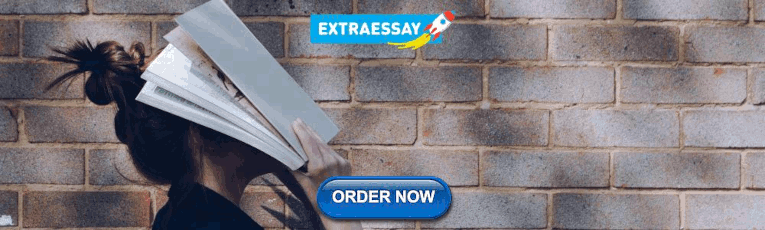
The Present and Future of Robotic Technology in Rehabilitation
Jeffrey laut.
New York University Tandon School of Engineering
Maurizio Porfiri
Preeti raghavan.
New York University School of Medicine
Robotic technology designed to assist rehabilitation can potentially increase the efficiency of and accessibility to therapy by assisting therapists to provide consistent training for extended periods of time, and collecting data to assess progress. Automatization of therapy may enable many patients to be treated simultaneously and possibly even remotely, in the comfort of their own homes, through telerehabilitation. The data collected can be used to objectively assess performance and document compliance as well as progress. All of these characteristics can make therapists more efficient in treating larger numbers of patients. Most importantly for the patient, it can increase access to therapy which is often in high demand and rationed severely in today’s fiscal climate. In recent years, many consumer grade low-cost and off-the-shelf devices have been adopted for use in therapy sessions and methods for increasing motivation and engagement have been integrated with them. This review paper outlines the effort devoted to the development and integration of robotic technology for rehabilitation.
Introduction
Disability from neurologic conditions can greatly increase health-care costs [ 1 ]. Such costs stem from inpatient care, medication cost, long-term care, and rehabilitation. Rehabilitation is critical to reduce future morbidity from immobility, depression, loss of autonomy and reduced functional independence [ 2 ], and inadequate provision of rehabilitation services, can further increase disability, leading to a vicious cycle of increasing costs [ 3 ]. Motor impairment, in particular, restricts mobility and function contributing significantly to disability and escalating costs [ 4 ] both due to the cost of long-term therapy services and that of caregiving, which are human resource intensive. While motor impairment after stroke is one of the most common reasons for rehabilitation, impairments resulting from cerebral palsy, multiple sclerosis, spinal cord injury, and Parkinson’s disease require similar interventions to improve motor function [ 5 ].
The process of rehabilitation typically involves four steps [ 6 ]. First, the patient needs to be assessed to evaluate the type and extent of impairment. Second, the therapist must set goals to direct the treatment and plan an intervention to meet the goals. Third, the intervention must be implemented, which often requires repeated assisted practice of specific movements. Finally, the patient must be reassessed after the intervention, and the next set of goals set. The potential for robotics to support and facilitate the process of rehabilitation was recognized decades ago [ 7 ]. Robots can be used to enhance each of the four processes that therapists must perform. They can be used to assess performance before, after and during an intervention, and also provide targeted intervention consistently and repetitively depending on the extent of impairment. Actuators integral to the system can move a user’s limb to assist or resist movement to varying degrees, while the sensors used for feedback to control the robotic mechanism also record the user’s performance. An early study that investigated the use of an industrial robotic arm as a therapy aid demonstrated its safety and acceptance both by patients and therapists [ 8 ]. The advantage of robotics-based rehabilitation, when compared to conventional therapy, is that it enables consistent training of the prescribed intensity for extended periods of time [ 9 ]. Robotic devices also offer flexibility in their operation, as feedback of the user’s performance based on the data from the sensors can be used to provide appropriate movements and forces during training [ 10 , 11 ].
The first robotic device designed specifically for rehabilitation, the MIT-Manus, was built in 1992 and offered two degree-of-freedom motion of the shoulder and elbow in the horizontal plane, while guiding the motion of the user’s arm along a trajectory with varying degrees of firmness [ 12 ]. The use of robots in rehabilitation has since grown substantially [ 13 – 16 ], and it has been verified that robotics-based regimens have outcomes similar to or better than those of traditional therapy [ 17 ]. However, several challenges, including cost, safety, efficacy, and ease of use for both the user and therapist, remain to be addressed to make robotic therapies more user-friendly and widely accessible. Significant research effort has also been devoted to adapting low-cost systems for rehabilitation, and assessing their ability to provide meaningful data for remote assessment in order to increase the accessibility to such devices [ 18 ]. The purpose of this review article is to describe the different types of robotic devices used for rehabilitation, how they are used to assess performance, and how the accessibility to such devices can be increased through telerehabilitation, virtual environments and the addition of gaming elements to increase adherence to the prescribed training regimens.
Types of robotic devices for rehabilitation
Devices for rehabilitation may operate passively, where the limb under treatment is constrained to a certain range of motion with no assistance or resistance; actively, where the affected limb is guided along a trajectory through the use of actuators; or interactively, with forces provided in response to the movement of the affected limb to provide an optimal assistive strategy [ 19 ]. Most of robotic the devices used in clinical settings today are active or interactive, but may also act in a passive manner. Furthermore, based on the interface of the device with the user, the devices may be classified as end-effector devices or exoskeletal devices.
End-effector robotic devices
End-effector devices for the treatment of the upper limb interface with the user through a manipulandum that is held in the hand [ 20 ]; end-effector devices for gait training interface at the foot or feet [ 21 ]. The manipulandum is connected to a robotic arm, which provides the force supplied to the user and contains the sensors that measure performance. Since the forces and measurements occur at a single interface, this type of system can easily be adapted to users of various body sizes without major modification to the system. Given this advantage, end-effector systems are popular for rehabilitation of the upper limb and for gait training. However, since the interaction with the robot is only at a single interface, the movements at each joint cannot be independently adjusted.
The MIT-Manus is an end-effector type of robot for treating the post-stroke upper limb [ 10 ]. The manipulandum, and thus the movement of the hand, is constrained to the horizontal plane with some play along the vertical direction provided by springs. Sensory-motor training using this device uses simple video games, where the input is provided through the motion of the manipulandum. The goal of training is to move a point on the screen through interaction with the manipulandum, enabling the user to draw shapes or move along a path. If the user is unable to accomplish the task, assistive force is provided by the robot through the manipulandum. A series of studies using this technology support the potential of robotics to assist and enhance traditional rehabilitation [ 10 ], and improve rehabilitation outcomes [ 22 , 23 ]. Importantly, research on the MIT-Manus also demonstrated that kinematic data collected by the robot as a result of the manipulandum’s motion may be useful in gauging post-stroke motor recovery. By shifting from traditional human-assessed metrics to metrics based on kinematic data collected by the robot, one can achieve more reliable and objective information to guide training [ 10 ]. Passive end-effector type robots have also been used to train both arms together but separately [ 24 , 25 ], or in a mirror-symmetric coupled manner [ 26 – 28 ].
End-effector robots are also used to improve lower limb function, which is typically easier to train compared to upper limb function due to the lower level of complexity associated with these movements compared with the upper limb. Hence, the development of devices for gait rehabilitation has received substantial attention, with positive results [ 21 , 29 ]. The HapticWalker is an end-effector type robot that is able to simulate walking and stair-climbing [ 30 ]. Force and torque sensors allow for an interactive control strategy, while enabling data collection to gauge the user’s progress and walking performance. The G-EO-System is a similar end-effector robot for gait training [ 31 ]. In a clinical study, users rated this system positively and experienced increased walking and stair-climbing ability when compared to a control group [ 32 ]. Such gait training robots allow for repetitive training with minimal effort from a physical therapist without increased risk of falling [ 32 ].
Exoskeletal devices
In contrast to the end-effector robots, the exoskeleton-type robots act directly upon specific joints of the user, and are often more difficult to design and construct as they must follow the design of the limb that is targeted, be adjustable to accommodate users of different anthropomorphic dimensions, and attach to the limb at multiple points. The forces at each joint can be adjusted using motors, but the joints are typically connected using rigid links attached to the arm (e.g. ARMin and MGA [ 33 , 34 ]) or leg (e.g. AnkleBot [ 35 ] and LOPES [ 36 ]. Robotic devices for bimanual training have also been tested [ 37 – 39 ].
Current designs of exoskeletons for the upper limb use rigid links that add inertia to the segments of the human arm making it 4–6 times heavier, which invariably requires users to use compensatory non-physiological muscle strategies during movement. Approaches to reduce inertia of the exoskeleton are to place the motors away from the joints and drive the joints using cables and pulleys (e.g. L-exos, CADEN-7, and MEDARM [ 40 – 42 ]). The Cable Driven Upper Arm Exoskeleton, CAREX, is a novel robot which suspends the arm using a robot-controlled cable system, making it 10 times lighter [ 43 ]. In a preliminary study, the CAREX was found to promote near-physiological muscle patterns, similar to those observed during movements without CAREX [ 44 ]. The upper limb exoskeleton robots offer: (1) assist-as-needed force fields to keep the upper limb within the desired path of motion, referred to as path-assistance, to promote higher movement accuracy, and (2) adjustable weight support to eliminate the effect of gravity, both of which can potentially assist with motor learning after a stroke. It has been shown that elimination of gravity using partial weight support can reduce abnormal motor synergies in the upper limb after stroke and improve range of motion and function [ 45 – 47 ]. Studies have also shown that subjects exhibit higher movement accuracy along the desired path with assist-as-needed force fields [ 43 ]. What is not clear is what aspects of robotic function should be applied to a given user, and how the therapeutic protocols should be adjusted for individuals with different levels of impairment.
For the lower limb, the AnkleBot is a two degree-of-freedom exoskeleton robot that directly actuates the ankle, and is capable of detecting deficiencies in gait and actively applying corrective forces to train a paretic ankle and improve both balance and walking after stroke [ 48 ]. This robot can also serve as a clinical measurement device by estimating the stiffness of the ankle joint, which can be used as a measure of rehabilitation progress [ 49 ]. Similarly, LOPES is an eight degree-of-freedom exoskeleton device for the legs designed to actuate the leg for gait training, or act passively as a measurement device [ 36 ].
Assessing performance with robotic devices
Beyond the development of interventions for rehabilitation, both the kinetic and kinematic data collected by the sensors embedded in the devices can be extremely informative. While clinical indices assessed by a therapist are based on movements that are performed only for assessment, kinematic data collected by the various sensors during a robotic intervention can inform how motor learning occurs. For example, in a path following task, movement accuracy can be scored by taking the mean of the absolute values of the distance of each point in the path generated by the user from the ideal path to be followed [ 20 ]. In such a scenario, as the user’s performance improves, the metric will decrease. Examples of performance metrics extend beyond precision of the movements and can also include movement smoothness [ 50 ], range of motion [ 20 , 51 ], and inter-limb coordination [ 52 ], with the last metric being used in bi-manual training involving both the affected and unaffected limbs [ 53 ].
In the case of end-effector type robots which interface at a single location, assessment of individual joint function is not possible [ 54 ]. For example, using an end-effector robot one can assess the range of motion of the hand, but not how much the user is moving the shoulder or the elbow during the task. This issue is minimized when using an exoskeleton type robot, where each joint of the limb that is being actuated can be assessed. However, for complex movements with many degrees of freedom, the movements with the robot must be compared with the movements performed without the robot to know if the robot-facilitated movements are physiologic and use patterns of muscle activity that are typical of healthy individuals. A comprehensive review on the use of kinematic data for movement assessment in stroke using robotic devices and how they tie in to rehabilitation progress is presented in [ 54 ].
Robot-Assisted Telerehabilitation
In contrast to traditional assessments that require one-on-one interaction with a therapist, objective assessment of motor function using data collected by rehabilitation robots can enable consistent, reliable and automatic assessment of motor function free of subjective bias from a treating therapist. Furthermore, by pairing rehabilitation devices with information technology and delivering assessment and performance data over the internet to the therapist, treatment can be moved out of specialized facilities and into patient’s homes with remote supervision by a therapist. This can allow a single therapist to treat multiple patients simultaneously greatly increasing the numbers of patients treated and increasing the efficiency of the therapist. Making this shift, from the hospital to a home-based rehabilitation program has the potential to decrease costs of the program while also making rehabilitation more accessible [ 55 , 56 ].
In general, telerehabilitation leverages telecommunications, computing technology, and remote sensing to enable both the delivery and assessment of rehabilitation services from a distance. While traditional telerehabilitation often requires a one-on-one interaction with a therapist [ 57 ], albeit from a distance, a robotics-based approach allows for a more flexible interaction. Research in robot-mediated telerehabilitation has been gaining traction, yielding a spectrum of reliable services [ 58 – 61 ]. These programs enable a trained professional to remotely verify that tasks are executed with the necessary intensity, level of precision, and posture, while supervising multiple robots and patients simultaneously and remotely [ 62 , 63 ]. A comprehensive review of this area from its inception up to 2010 has been conducted in [ 64 ].
Challenges to Adoption and Accessibility to Robot-Assisted Rehabilitation
Although robotics-based rehabilitation and telerehabilitation have both been widely demonstrated to be effective, they are not yet part of routine treatment in most settings. This is primarily due to the fact that most studies include ad-hoc robotic devices that are not mass-produced, and although commercially-available rehabilitation robots are becoming more common, their cost remains high. Coupled with the need to provide outpatient rehabilitation to greater numbers of patients [ 65 ], a considerable effort is now geared towards the development and adoption of low-cost devices that mitigate direct supervision from a therapist.
Robotic devices have been demonstrated to be effective in therapeutic settings; however, in order for a treatment to be widely accessible, providing such systems in under-supervised environments outside of treatment centers will be necessary. A major challenge to implementing robot-assisted rehabilitation in such settings is that the patient must be willing to comply with the prescribed regimen(s) [ 66 ]. In rehabilitation, patient adherence to prescribed regimens is associated with both increased satisfaction [ 67 ] and improved outcome of treatment [ 68 ]. However, lack of motivation to perform the exercises is one of the main reasons for non-compliance with therapy [ 69 – 71 ]. Supplementing rehabilitation exercises with additional motivational elements could thus indirectly improve the effectiveness of rehabilitation interventions through increased compliance. For example, including gaming consoles has been shown to increase motivation and enjoyment during exercise [ 72 , 73 ], and interactive computer play may be an effective means for bolstering engagement in children [ 74 ].
Most early robotic devices lacked engaging interfaces; however more recent efforts have begun to include interactivity and virtual reality elements in an effort to challenge patients and provide additional motivation. The GENTLE/s system, for example, provides feedback to the patient through visual, haptic, auditory, and performance cues [ 75 ]. In this system, the user sits in a chair, and the arm and wrist are suspended with support mechanisms. The wrist is connected to a haptic device via an orthosis, and the user is presented with an artificial environment selected by a therapist through a computer screen, which they navigate using the haptic device as input. The system also has several strategies for correcting the user’s motion while they move through the virtual environment, and clinical trials have demonstrated that the haptic and visual feedback provided by the system has a more positive treatment effect than treatment without feedback [ 76 ].
Java therapy is an early example of the use of a low-cost gaming device for rehabilitation of the arm and hand following stroke in an effort to increase accessibility to rehabilitation in home settings. It consists of a force-feedback joystick connected to a home computer with custom software. The system has been found to be feasible to provide therapeutic treatment over the internet and enable a therapist to assess progress [ 77 ]. Unmodified consumer grade off-the-shelf gaming systems have also been used to augment rehabilitation [ 78 , 79 ]. For example, the Nintendo Wii is a popular gaming device used in rehabilitation, with 20% of surveyed therapists having used the device for upper-limb stroke rehabilitation [ 80 ]. The system consists of hand-held sensors to interact with the game which offer some haptic feedback, or a sensorized balance board. It however lacks assistive feedback which is present with most robotic devices [ 81 ]. Nevertheless, clinical trials with the system for patients undergoing stroke rehabilitation have reported that it is both enjoyable and beneficial [ 82 ], and both patients and caregivers have reported high use rates in a home setting [ 83 ]. The PlayStation EyeToy is a similar gaming console that uses a digital webcam to track interaction. It has also been shown to be suitable for rehabilitation, and was found to elicit higher intensities of movement than the Nintendo Wii [ 84 ].
While rehabilitation systems with gaming elements can enhance user motivation and engagement [ 79 , 85 ], one major challenge lies in the nature of the video games that are commercially available. Games designed primarily for entertainment may be too fast-paced for sensorimotor-impaired individuals [ 86 ]. Serious games developed not purely for entertainment but specifically for rehabilitation [ 87 , 88 ] hold more promise in this regard [ 89 , 90 ]. These games should enable clinicians to tailor the game as rehabilitation progresses to ensure that the appropriate level of difficulty is maintained throughout the recovery process [ 91 ]. An important consideration in selecting games for rehabilitation is to examine if the games encourage or discourage the use of unwanted compensatory strategies. Structured gaming that uses available games but in a step-by-step guided manner may be one solution to using existing gaming consoles, such as the Wii [ 92 ]. Furthermore, rehabilitation games are now being designed to provide specific feedback regarding desirable and undesirable movements and forces through the use of sensors embedded in real-world objects [ 93 ] and instrumented clothing [ 94 ].
Rehabilitation gaming primarily leverages entertainment to increase engagement in exercises, but pairing participants in cooperative or competitive gameplay may serve as a means to further enhance participation in robot-mediated therapy. Such a concept was investigated using a pair of ARMin exoskeleton rehabilitation robots, where participants competed in a virtual air-hockey game in single-player competitive and cooperative gameplay conditions [ 95 ]. While subjects’ personality traits roughly dictated which gameplay mode they preferred, the study indicated that multi-player gaming can improve the enjoyability and level of intensity of rehabilitation.
The older population, which comprises the majority of patients with neurologic disability, may be more receptive to games that challenge their intellect in contrast to the typical action and reflex videogames. This population has been found to enjoy helping new players, solving puzzles, and participating in the social aspects of gaming [ 96 ]. In a departure from traditional gaming, the effect of science learning in a virtual environment has also been studied [ 97 ]. Here an off-the-shelf haptic joystick, the Novint Falcon, was used to navigate a virtual environment representing a zoo. Rather than presenting gaming or competitive elements, participants were presented with educational content about the zoo and some of its exhibits. Subjects controlled the movement of a cursor along a predefined trajectory superimposed on a map of New York City’s Bronx Zoo to learn from the zoo-related content presented, and answer scientific questions. The task engaged the participants cognitively, and simultaneously enhanced visuomotor dexterity. The findings suggest that educational content may be used to increase the level of interest in the activity, time spent in rehabilitation, and can increase participant satisfaction. Building on this infrastructure, and shifting from passive scientific learning to active science participation, another study used citizen science to boost motivation in rehabilitation [ 98 ]. Here participants used a haptic joystick to tag images of a polluted environment and contribute to the analysis of data as part of an authentic environmental monitoring project [ 99 ]. The results suggested that users were more willing to repeat their exercises when they had an authentic citizen science task to perform as part of their therapy. The Novint Falcon used in these studies [ 97 , 98 ] is a low-cost haptic device capable of both providing programmed force-feedback, and recording end-effector position data at a high rate. Importantly, it was demonstrated that performance metrics using data collected from the Novint Falcon can distinguish between participants without disabilities and those undergoing stroke rehabilitation [ 98 ], suggesting that it can measure progress during rehabilitation.
It may be reasonable to argue that rehabilitative exercises can be more easily, effectively, and routinely executed from the comfort of the home when compared to clinical environments. However substantial progress remains to be made to make robot-assisted home-based telerehabilitation an affordable reality. While some consumer grade low-cost and portable devices, such as the Novint Falcon or the Java therapy system, can easily be adopted in a confined home environment, they cannot be used to practice large shoulder and arm movements often needed during rehabilitation [ 100 ]. To address this issue, consumer motion capture systems have been adopted both for human motion analysis and rehabilitation [ 101 ]. For example, the Microsoft Kinect is a camera-based human motion tracking device that is able to estimate the coordinates of the joints of a human skeleton model in three dimensions [ 102 ]. Similar to the aforementioned gaming systems, it was originally intended to be used as an input device for videogames, but has been repurposed for use as a natural user interface [ 103 ], whereby the captured motion, often in the form of gestures [ 104 ], is used as input for human-computer applications [ 105 ]. This functionality has enabled the use of the Kinect in both human motion analysis [ 106 ] and rehabilitation [ 107 , 108 ]. Adding to the versatility of the Kinect is its ability to be paired with a personal computer rather than a gaming console, making the development of custom rehabilitation interfaces and virtual environments more straightforward. Custom game-based rehabilitation software has been developed for the Kinect, which requires the user to perform therapeutic gestures to accomplish the goals of the game [ 107 ], with most participants reporting the experience to be both enjoyable and challenging. Importantly, the Kinect can estimate joint angles with accuracy comparable to professional-grade optoelectronic systems [ 109 ], suggesting that it can also be used to accurately gauge progress during rehabilitation. Most of the studies using the Kinect however focus on the upper limb [ 110 ].
Conclusions
The use of robotic technology has the potential to transform rehabilitation from a one-on-one human resource intensive treatment that can only be provided in specialized centers, to a technology driven, remotely-supervised and widely accessible enterprise. Given the increased costs associated with long-term rehabilitation and the difficulty in providing appropriate duration and intensity of rehabilitation services required to manage disability, cost-effective development of robotic rehabilitation is greatly warranted. Many new developments in the use of robotic technology include development of light-weight devices, use of off-the shelf devices, incorporation of motivational elements such as gaming, virtual reality, and educational and scientific tasks to provide user-friendly access to technology to empower therapists to provide rehabilitation more efficiently, and to empower patients to have greater access to rehabilitation. The true transformative potential of robotic rehabilitation will require the development of telerehabilitation that can be provided via low-cost devices to effectively and efficiently rehabilitate individuals in their own homes.
Conflict of Interest
Jeffrey Laut, Maurizio Porfiri, and Preeti Raghavan declare that they have no conflict of interest.
Compliance with Ethics Guidelines
Human and Animal Rights and Informed Consent
This article does not contain any studies with human or animal subjects performed by any of the authors.
Contributor Information
Jeffrey Laut, New York University Tandon School of Engineering.
Maurizio Porfiri, New York University Tandon School of Engineering.
Preeti Raghavan, New York University School of Medicine.
A review of rehabilitation robot
Ieee account.
- Change Username/Password
- Update Address
Purchase Details
- Payment Options
- Order History
- View Purchased Documents
Profile Information
- Communications Preferences
- Profession and Education
- Technical Interests
- US & Canada: +1 800 678 4333
- Worldwide: +1 732 981 0060
- Contact & Support
- About IEEE Xplore
- Accessibility
- Terms of Use
- Nondiscrimination Policy
- Privacy & Opting Out of Cookies
A not-for-profit organization, IEEE is the world's largest technical professional organization dedicated to advancing technology for the benefit of humanity. © Copyright 2024 IEEE - All rights reserved. Use of this web site signifies your agreement to the terms and conditions.
- Open access
- Published: 30 August 2019
A Review on Lower Limb Rehabilitation Exoskeleton Robots
- Di Shi 1 , 2 ,
- Wuxiang Zhang ORCID: orcid.org/0000-0001-6141-3368 1 , 2 ,
- Wei Zhang 1 , 2 &
- Xilun Ding 1 , 2
Chinese Journal of Mechanical Engineering volume 32 , Article number: 74 ( 2019 ) Cite this article
44k Accesses
240 Citations
Metrics details
Lower limb rehabilitation exoskeleton robots integrate sensing, control, and other technologies and exhibit the characteristics of bionics, robotics, information and control science, medicine, and other interdisciplinary areas. In this review, the typical products and prototypes of lower limb exoskeleton rehabilitation robots are introduced and state-of-the-art techniques are analyzed and summarized. Because the goal of rehabilitation training is to recover patients’ sporting ability to the normal level, studying the human gait is the foundation of lower limb exoskeleton rehabilitation robot research. Therefore, this review critically evaluates research progress in human gait analysis and systematically summarizes developments in the mechanical design and control of lower limb rehabilitation exoskeleton robots. From the performance of typical prototypes, it can be deduced that these robots can be connected to human limbs as wearable forms; further, it is possible to control robot movement at each joint to simulate normal gait and drive the patient’s limb to realize robot-assisted rehabilitation training. Therefore human–robot integration is one of the most important research directions, and in this context, rigid-flexible-soft hybrid structure design, customized personalized gait generation, and multimodal information fusion are three key technologies.
1 Introduction
A rehabilitation robot, which is a robot directly serving humans, has extensive application prospects in rehabilitation therapy with high professional requirements. Therefore, it is of great importance to develop advanced rehabilitation robots.
Research on lower limb rehabilitation robots for patients with limb movement disorders is an important part of rehabilitation robot research. By 2030, 18.2% of China’s population will be over the age of 65. Due to an aging society and improving living standards, the number of people with limb dyskinesia is increasing rapidly. Limb movement disorders can lead to abnormal gait and affect normal walking. For patients with lower limb movement disorder, active rehabilitation training should be started as early as possible. In China, which has the highest stroke rate in the world [ 1 ], there are nearly 15 million disabled people with lower limb motor dysfunctions, such as cerebral palsy, hemiplegia, and paraplegia, and nearly 40 million disabled elderly people who have lost the ability to walk, due to aging. About 350,000 people are in urgent need of rehabilitation technical personnel, but less than 20,000 personnel are available. Therefore, lower limb rehabilitation robots are of great significance. The use of rehabilitation robots can reduce the burden on therapists, realize data detection during training, and aid the quantitative evaluation of recovery in a controllable and repeatable manner [ 2 ].
Lower limb rehabilitation exoskeleton robots, which are a major class of rehabilitation robots, connect with the human body in a wearable way and can control the movement of all joints in the training process. Research on lower limb rehabilitation exoskeleton robots began in the 1960s [ 3 , 4 ]. Due to technical limitations, these early robots failed to reach the expected targets, but laid the foundation for follow-up studies. In recent decades, especially after Lokomat was applied in clinical rehabilitation, lower limb rehabilitation exoskeleton robots have gradually become a major research topic. They are mainly used to provide power assistance and rehabilitation to the elderly and patients with lower limb motor dysfunctions. Exoskeleton robot technology is a comprehensive technology that integrates sensing, control, information and computer science to provide a wearable mechanical device. Many enterprises and research institutions have carried out relevant research work and achieved several milestones in the theory and application of these robots. According to their application, these robots are divided into two types, namely for treadmill-based and overground applications. Patients can receive gait training from treadmill-based exoskeleton robots on a treadmill. In these robots, in addition to the exoskeleton that is used to provide assistance to leg movement [ 3 ], a body weight support (BWS) system is required to reduce gravitational forces acting on the legs, ensure safety, and maintain balance; some examples of such robots include ALEX [ 2 ], Lokomat [ 4 ], and LOPES [ 5 ], as shown in Figure 1 . Overground exoskeleton robots help patients in regaining overground gait, as shown in Figure 2 ; examples include eLEGS (Exoskeleton Lower limb Gait System) [ 6 , 7 ], Indego [ 8 ], ReWalk [ 9 ], MINDWALKER [ 10 , 11 ], and HAL (Hybrid Assistive Limb) [ 12 ].
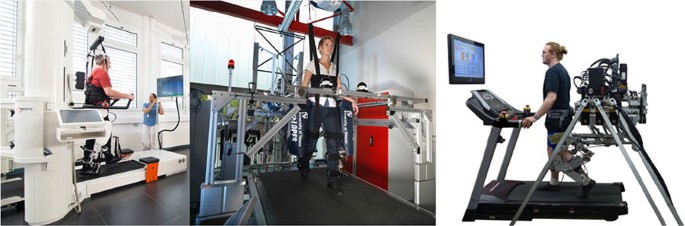
Treadmill-based lower limb rehabilitation exoskeletons
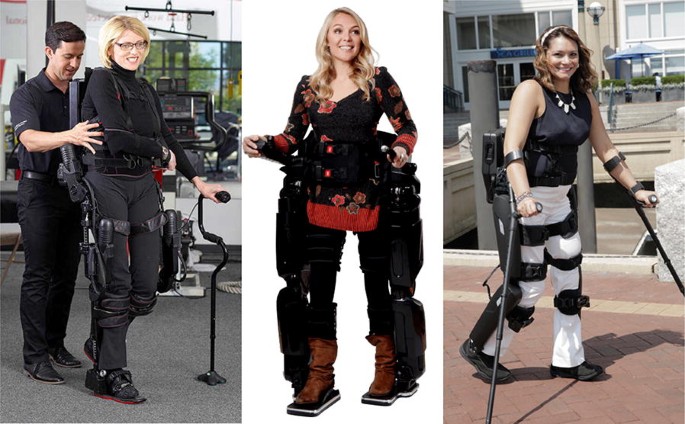
Overground lower limb rehabilitation exoskeletons
At present, a large gap exists in rehabilitation robot technology between China and the developed countries. China urgently requires rehabilitation-assistive devices and has the largest market potential in the world. By 2020, the industrial scale is expected to exceed 700 billion. Universities and institutes in China [ 13 , 14 , 15 , 16 , 17 , 18 , 19 , 20 , 21 , 22 ] have conducted a number of studies and achieved some promising results. However, deep disparities exist between Chinese and overseas research, and no systematic industry has yet been formed. Therefore, China’s lower limb rehabilitation exoskeleton robot research has the potential to produce another revolution in the robot industry.
Hence, in this paper, we present a review on lower limb rehabilitation exoskeleton robots. Research and results in mechanical design and control methods are discussed after collating a summary on human gait analysis. The state-of-the-art research on lower limb rehabilitation exoskeleton robots is described and human–robot integration, which is one of the most important research directions, is discussed.
2 Human Gait Analysis
Wearability is one of the most vital features of lower limb rehabilitation exoskeleton robots and hence such robots must have good human compatibility. Therefore, an illustration of lower limb anatomy and human gait analysis can provide the underlying basis for the design and control of lower limb exoskeleton rehabilitation robots.
2.1 Anatomy of Lower Limbs
The human walking process is mainly accomplished by lower limbs, and hence, analyzing their structure and movement characteristics is necessary.
Walking is achieved by coordination between the pelvis, hip, knee, and ankle. Their ranges of motion (ROM) are illustrated in Table 1 . The pelvis is located between the trunk and thighs. As a ball-and-socket joint, the hip is formed by the head of the femur and pelvic bone and it allows simultaneous movement between the thighs and pelvis [ 23 ]. It allows sagittal flexion/extension, frontal abduction/adduction, and transverse external/internal rotation [ 24 ]. Knee is a joint complex containing tibiofemoral and patellofemoral joints. Their movement occurs in two planes, allowing sagittal flexion/extension and transverse internal/external rotation [ 23 ]. During walking, knees perform important functions. In the swing phase, knees shorten leg length by flexion [ 25 ]. In the stance phase, they remain flexed to absorb shock and transmit forces through legs. The ankle/foot is a complex structure that absorbs this shock and imparts thrust to the body. Ankle movements mainly occur about talocrural and subtalar joints [ 24 ]. The talocrural joint is located between the talus, distal tibia, and fibula to provide plantar/dorsiflexion as a hinge joint, in which the surface of one bone is spool-like and the surface of the other bone is concave. The subtalar joint is located between the calcaneus and talus and allows eversion/inversion and internal/external rotation. The basis for the mechanical design of rehabilitation exoskeleton robots is provided by an analysis of the lower limb structure.
2.2 Analysis on Human Gait
The normal gait pattern of patients cannot be measured directly because of their impaired motor functions. Therefore, it is necessary to conduct rehabilitation training and evaluate normal gait data, which is significant in clinical applications. Patients with hemiplegia or physical disabilities often follow a predetermined trajectory in their rehabilitation. These predetermined trajectories can be obtained from normal gait data collection. Through gait analysis, the relevant characteristics of human gait can be revealed. Step length, width, and speed are all used for human walking gait characterization. Thus, human body movement parameters and structural parameters have a significant influence on human gait characteristics.
Human gait is affected by walking speed [ 26 , 27 ] as confirmed by an analysis of gait parameters [ 28 ] and joint angles [ 29 ], as confirmed by recording and analyze the gait data with different walking speeds on a walkway [ 30 ] or treadmill [ 31 ]. Because most rehabilitation robots use a body support system during rehabilitation training, analyzing human gait on a treadmill is necessary. Additionally, studies have revealed that body height, as a structural parameter, has limited effect on the human gait as compared to walking speed. This was proved by comparing the difference in the correlation between regression models when using speed and normalized speed (normalized to leg length) [ 30 , 32 ] or by using step-wise regression in regression models by including body height [ 31 ] as a parameter. Further, these studies focused on the effect of these parameters on joint angles. Studies have also outlined the relationship between gait parameters and the body mass index (BMI) [ 26 , 33 ]. These studies provide a foundation for control over lower limb rehabilitation exoskeleton robots.
3 Mechanics of Lower Limb Rehabilitation Exoskeleton Robots
Lower limb rehabilitation exoskeleton robots need a mechanical structure matching human lower limbs to realize force and energy transmission through the wearable connection. These can be achieved by designing the appropriate robot mechanism and actuation. An overview of the mechanics involved is presented in Table 2 .
3.1 Anatomy of Human Upper Limbs
The mechanism of lower limb rehabilitation exoskeleton robots should realize movement matching with human lower limbs. The mechanism design of the Berkeley exoskeleton system (BLEEX) laid a foundation for subsequently developed robots. To ensure safety and avoid collisions maximally with users, BLEEX is almost anthropomorphic but does not include all the degrees of freedom available for human legs (Figure 3 ). Additionally, BLEEX joints are purely rotary joints and hence, are different from human joints [ 34 ]. The hip is simplified as three rotatory joints to achieve flexion/extension, abduction/extension, and internal/external rotation. The knee is simplified as a rotating joint to achieve pure sagittal rotation. The ankle is simplified into three rotation joints to achieve plantar/dorsiflexion, eversion/inversion and internal/external rotation. The configuration of the current lower limb exoskeleton robots, such as ALEX [ 35 ], Lokomat [ 4 , 36 ], LOPES [ 37 , 38 ], Rewalk [ 9 ], Rex [ 39 ] and HAL [ 40 ], is mainly based on BLEEX.
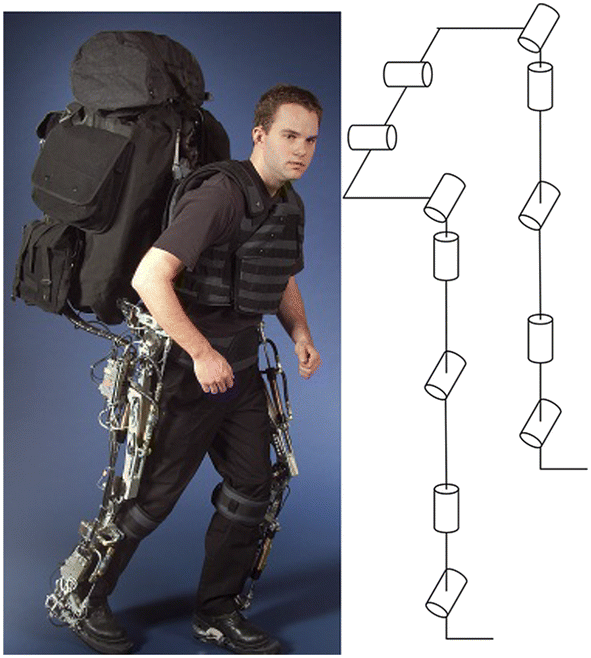
Biomechanical design of BLEEX [ 41 ]
Due to the existence of the BWS system and the fact that the robot body is often connected to a fixed platform, an important feature of treadmill-based exoskeleton robots is that the patient does not need to carry the entire weight of the robot, which complicates the mechanical structure of the robot. At the same time, rehabilitation training on a treadmill requires less room, but there is a difference between gait on the treadmill and natural gait [ 42 , 43 ], which is an important aspect in clinical evaluations [ 44 , 45 , 46 , 47 ]. Additional mechanisms were designed for pelvic movement. Pelvis motion is also being integrated into new robotic devices, such as KineAssist [ 48 , 49 , 50 ]. ALEX III can actively control motions of the pelvis [ 45 , 51 ]. The new version of Lokomat includes an optional FreeD module to improve therapy by allowing for pelvic lateral translation and transverse rotation, as shown in Figure 4 .
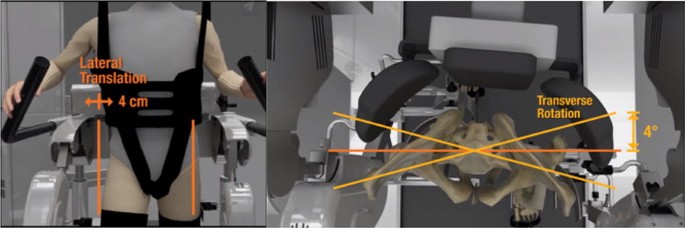
The optional FreeD module
Such simplified design means that there is a motion mismatch between the robot and human, which is manifested in the mismatch between the joint centers of the robot and human. Designing an innovative mechanism can offer a solution to this problem. For hip joints, a parallel structure is adopted to realize three rotational motions and automatic centering with the human hip [ 52 , 53 , 54 ] as shown in Figure 5 . When a 3-UPS parallel mechanism is mounted on the human waist and thigh, the thigh of the human and the mechanism are connected as a whole, which can be considered as a 3-UPS/1-S parallel mechanism [ 52 ] (Figure 5 (a)). A novel metamorphic parallel mechanism was applied for lower limb rehabilitation using two configurations, 3-UPS/S and 2-RPS/UPS/S, by taking into account the human hip joint to satisfy different demands of patients at different phases of rehabilitation therapy [ 53 ] (Figure 5 (b)). An asymmetric fully constrained parallel mechanism prototype is designed for hip joint assistance and rehabilitation and employs pantographs as three-rotation constrained legs instead of using three serial rotation joints-leg to avoid disadvantages such as singularity, uncertainty, or interference with other legs [ 54 ] (Figure 5 (c)).
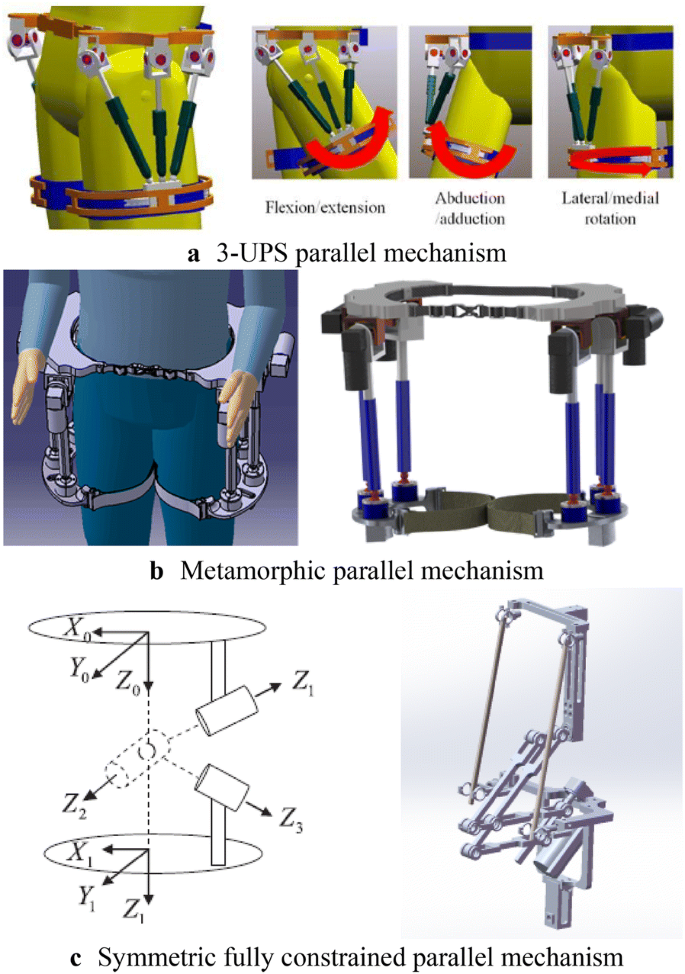
Parallel mechanisms for hip joint assistance
The knee joint is treated as a rotating joint in the simplification process and only the flexion and extension motion are considered. In fact, knee motion is relatively complex and hence different mechanisms have been designed to solve this problem, as shown in Figure 6 . For the knee joint, an adaptive knee was used and it could effectively eliminate negative effects on human knees [ 55 ] (Figure 6 (a)). Based on the knee joint complex, axial translational motion was coupled with rotational motion and a serial-parallel hybrid mechanism was designed for lower limb rehabilitation [ 17 ] (Figure 6 (b)).
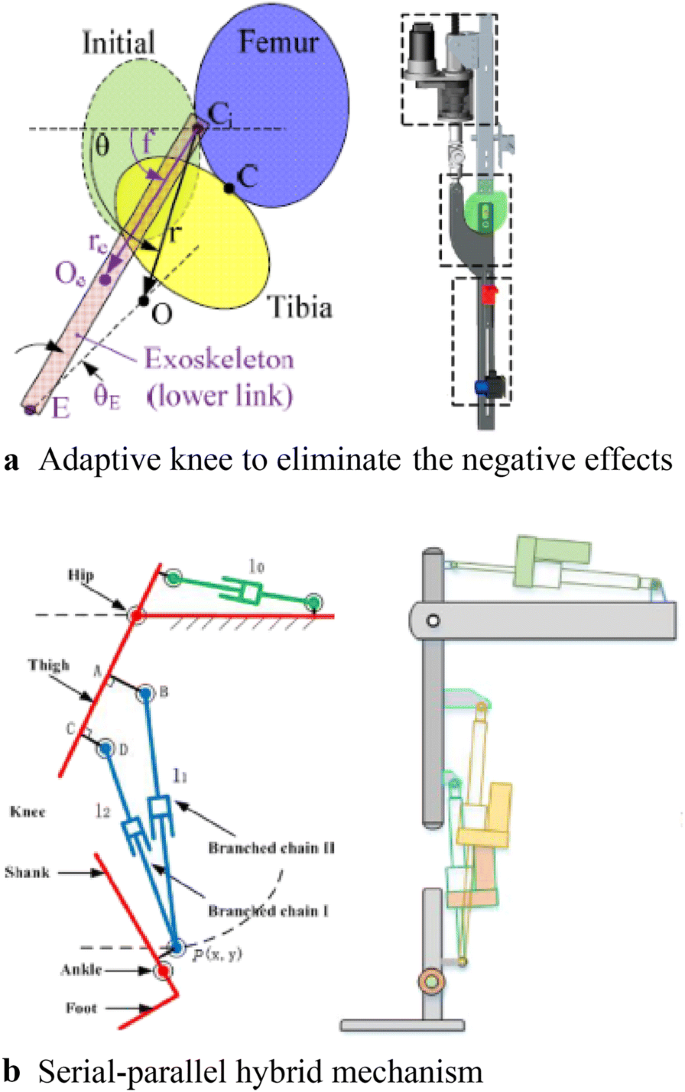
Novel mechanisms for knee joints
The human body is a coupled structure based on bone-muscle tissue structure and characteristics. According to this principle, some scholars have suggested a new kind of structure for lower limb rehabilitation robots. There is no rigid support for the mechanical structure but it includes a soft body and software structure using cables to provide power; this reduces response to muscle contractions and energy consumption of the body. This design for coupled wearable robots is expected to pave a new research direction [ 56 ] (Figure 7 ).
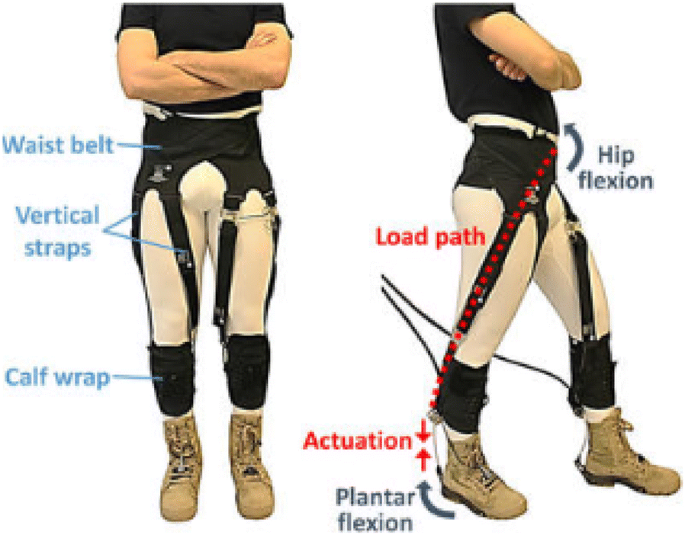
Soft exosuit
3.2 Actuation Design
Most lower limb exoskeleton rehabilitation robots are driven by electric motors. In eLEGS, only sagittal flexion/extension for the hip and knee are actuated using motors while the ankle remains passive [ 57 ]. The hip and knee of Lokomat are actuated by motors with linear ball screws [ 58 ].
A remarkable feature of the above method is that the driver is directly placed on the robot body, which increases its mass and complexity. Therefore, using cable-driven motors can reduce the mass of the exoskeleton robot itself, because the motor and driver are placed on the platform instead of directly on the exoskeleton [ 59 ].
Lower limb rehabilitation exoskeletons are mainly driven by rigid transmission without compliance. This causes a large vibration impact, difficulty in directly controlling the force, and leads to a complicated robot system. Therefore, a series elastic drive (SEA) was designed to achieve force control and enhance drive flexibility in RoboKnee [ 60 ] (Figure 8 (a)). SEA with a combination of cable-driven actuation was applied in LOPES [ 59 ] (Figure 8 (b)). A variable stiffness elastic actuator was designed for lower limb exoskeletons by adjusting the stiffness of the elastic elements driven by series elasticity [ 61 ] (Figure 8 (b)).
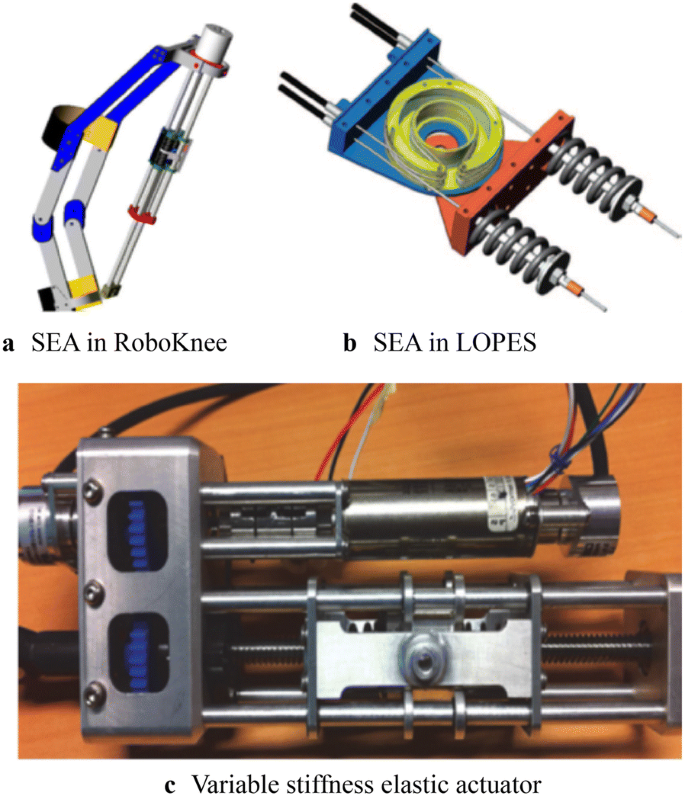
A series elastic actuator
4 Control of Lower Limb Rehabilitation Robots
4.1 trajectory planning.
The main purpose of rehabilitation training is to restore the lower limb motor functions of disabled patients to normal levels [ 62 ]; therefore, in the process of rehabilitation training, a normal gait pattern is required as a reference input to the control system, as a training goal, and as a rehabilitation evaluation standard. For patients with hemiplegia or physical disabilities, a predetermined trajectory is often used. These predetermined trajectories can be obtained from data on normal gait. However, due to the limited amount of data, it is difficult to match the obtained motion data with different human motion characteristics, and hence, a parameterized motion pattern generation method was proposed to predict data not present in the test sample.
The trajectories of Lokomat can be adjusted to a specific patient and step length [ 58 ]. In LOPES, trajectories are generated by a method based on regression analysis to reconstruct the body height and speed-dependent trajectories [ 31 ]; further, “complementary limb motion estimation” may be used to generate reference motion using the motion of healthy limbs [ 63 ]. When the collected number of samples is large enough, statistical learning techniques, such as the radial basis function neural networks (RBFs) [ 64 ] and multi-layer perceptron neural network (MLPNN) [ 65 ], are often used for motion prediction.
There is another type of gait planning that does not rely on specific data; however, it depends on the given gait parameters, such as step length and swing duration to generate gait patterns. The model predictive control (MPC) is used for online trajectory generation based on gait parameters [ 66 ].
4.2 Control System
A hierarchical control strategy is used in most lower limb rehabilitation exoskeleton robots. Generally, the control system is divided into two levels. The upper level is the decision-making layer, which realizes control decisions and trajectory generation, and the lower level is the servo layer, which realizes servo control of the drive system [ 65 ]. As for overground rehabilitation exoskeletons, they are divided into three layers due to the large number of movements involved (Figure 9 ). In particular, a human–robot interaction layer is added to adapt to the training needs of a variety of movements [ 6 ]. An overview of the control method of lower limb exoskeletons is shown in Table 3 .
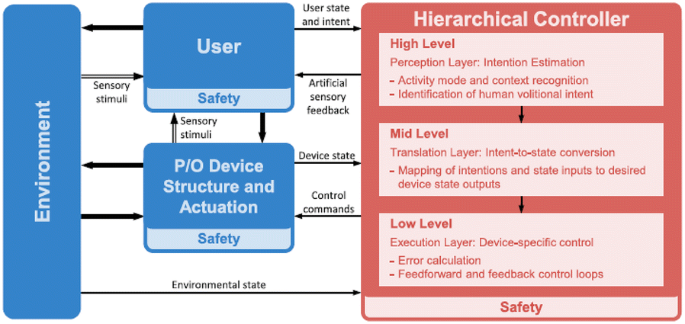
Generalized control framework for lower limbs [ 67 ]
In early rehabilitation stages, the lower limbs of patients are dragged for continuous passive motion (CPM) for passive training, which can effectively keep joints flexible for a long time. Correspondingly, position control ensures that the robot can accurately follow the desired position. In this case, movement information of the robot is measured by sensors, such as linear and rotary potentiometers [ 40 , 68 , 69 ], inertia measurement units (IMUs) [ 70 , 71 ], torque sensors to measure torque [ 70 , 71 ], and foot pressure sensors to measure the ground reaction to detect gait events [ 72 , 73 ]. Control methods widely used in servo control have also been used in lower limb rehabilitation exoskeleton robots. For example, proportional derivative control (PD control) [ 68 ], computed torque control [ 74 ], fuzzy control [ 74 ], robust variable structure control [ 75 ], fuzzy proportional integral derivative control (PID contro), and sliding mode variable structure control [ 76 ] have been used for lower limb exoskeleton robots. Such position control is actually a type of tracking control. Force-position hybrid control was also used to adjust the output force exerted on the patient. This was the first impedance or admittance control strategy ever developed. It has been applied in the Lokomat to guide patients’ legs and supply hip and knee joint torque [ 4 ]. In LOPES, the robotic support was controlled using a virtual model controller (VMC) [ 69 , 77 ].
All the control systems described above are passive in nature because the wearer is not considered in the system. By increasing active participation, the dependence of patients on robot assistance can be reduced by improving the effect of rehabilitation training. To achieve this effect, it is important to integrate people into the control system. As mentioned earlier, sensors have been used to measure human movement information and human–robot interaction information to control a robot. In ALEX a force field control is used to guide patients’ legs [ 45 , 70 ].
Another way is to measure human biological signals such as electromyography (EMG) and electroencephalogram (EEG) signals, to perceive body movement. Currently, EMG signals are being used in robots such as HAL [ 78 ]. The EMG signal to be detected is used as a trigger switch to judge the timing of assistance provided by the robot [ 78 , 79 ]. As continuous control, a proportional myoelectric control is used in the robotic ankle exoskeleton [ 80 , 81 ]. However, EMG-based robot-assisted rehabilitation is only suitable for patients who are able to produce a sufficiently high level of muscle activity. Brain-machine interfaces to restore mobility in severely paralyzed patients [ 82 ] have been applied in many lower limb rehabilitation exoskeletons [ 39 , 83 , 84 , 85 ]. However, this research is still in the early stages. All the systems described above rely on the presence of sensors and control the signals measured by sensors. Recently, robots not based on sensors have been developed. In this case, an admittance shaping controller is used [ 86 ].
5 Conclusions and Outlook
5.1 conclusions.
Lower limb rehabilitation exoskeleton robots integrate sensing, control, and other technologies and exhibit characteristics of bionics, robotics, information and control science, medicine, and other interdisciplinary fields and therefore, have become a major research hotspot. In recent years, remarkable progress has been achieved in mechanical design and control system design, based on which, several products have been commercialized. However, there is still a large research gap with respect to human–robot integration. The wearer (patient) should organically combine with the robot to form a whole. Only when true integration of the human body and robot is realized can rehabilitation training be truly effective.
5.2 Outlook
The problem of human–robot integration is a current research hot spot. The National Natural Science Foundation of China (NSFC) has launched the Tri-Co Robot (i.e., the Coexisting-Cooperative-Cognitive Robot), a major research program in 2017, with research themes of robot structure design and control, multi-mode dynamic perception, and natural interaction [ 87 ]. Human–robot integration is a key issue in the design and control of lower limb rehabilitation exoskeleton robots. In summary, such integration should include three components, namely structure, movement, and response.
Humanoid structures and flexible drive systems should be designed to achieve structural integration between robots and patients. Currently, a simplified human movement model and a rigid structural design are adopted, causing movement mismatch between a robot and human and affecting wearability and rehabilitation training. Therefore, SEA is being used to increase the flexibility of the local structure. The addition of flexibility inevitably leads to structural complexity and difficulty in control. An ideal robot structure is a rigid-flexible-soft hybrid structure. However, rigid-soft-soft coupling configurations should be designed to effectively transfer energy from a robot to a human. At the same time, modular designs for exoskeleton mechanism should be explored. In fact, many active orthosis devices can also be referred to as modular single-joint exoskeletons.
Customized and personalized gait patterns should be generated to achieve motion integration between robots and patients. A normal movement mode is often required as a reference and input to the control system as the expected robot movement, training target, and evaluation standard. For patients with hemiplegia or other physical disabilities, a predetermined trajectory is often used in rehabilitation. These predetermined trajectories can be obtained from normal gait data collection. However, due to the limited amount of data collected, it is difficult for the obtained motion data to match different motion characteristics of the human body, and hence, a parameterized motion pattern generation method has been proposed. However, the current planning and gait generation methods based on the biped robot control theory and human natural gait cannot achieve a perfect match; gait generation is mainly focused on the sagittal plane and does not account for human three-dimensional gait characteristics. The mechanism of how human gait is affected by motion and structural parameters is not yet fully understood, and hence, it is difficult to realize a perfect integration of motion levels.
Multimodal information fusion should be used to achieve motion integration between humans and machines. Current research on the design of sensing systems indicates that it is not enough to measure a robot’s movement information; instead, people also should be included in the system, not only to measure human body movement information and biological signals but also to collect interaction information between a human and the force exerted by the robot. However, it can be imagined that more information is not always better as redundant information increases the complexity of a system and affects its practical application. In the process of rehabilitation training, lower limb rehabilitation exoskeleton robots need to participate in effective dynamic interaction with the patient. Such rehabilitation training can effectively improve the level of active participation of the patient and significantly enhance the rehabilitation effect. However, the evaluation method of the robot itself lacks a set of system indicators for the adaptability and degree of matching with the human body. Hence, it is necessary to study multimodal information to realize effective human–robot integration.
G L R O Collaborators. Global, regional, and country-specific lifetime risks of stroke, 1990 and 2016 . New England Journal of Medicine , 2018, 379(25): 2429-2437.
Article Google Scholar
S K Banala, S H Kim, S K Agrawal, et al. Robot assisted gait training with active leg exoskeleton (ALEX) . IEEE Transactions on Neural Systems and Rehabilitation Engineering , 2009, 17(1): 2-8.
G Chen, C K Chan, Z Guo, et al. A review of lower extremity assistive robotic exoskeletons in rehabilitation therapy . Critical Reviews in Biomedical Engineering , 2013, 41(4-5): https://doi.org/10.1615/critrevbiomedeng.2014010453 .
M Bernhardt, M Frey, G Colombo, et al. Hybrid force-position control yields cooperative behaviour of the rehabilitation robot LOKOMAT . International Conference on Rehabilitation Robotics , 2005: 536-539.
Van Asseldonk E H, Van Der Kooij H. Robot-aided gait training with LOPES . Neurorehabilitation Technology, Springer, 2012.
Book Google Scholar
S T Alan. Control and trajectory generation of a wearable mobility exoskeleton for spinal cord injury patients . University of California, Berkeley, 2011.
Google Scholar
S K Ann. Development of a human machine interface for a wearable exoskeleton for users with spinal . University of California, Berkeley, 2011.
R J Farris, H A Quintero, M Goldfarb. Preliminary evaluation of a powered lower limb orthosis to aid walking in paraplegic individuals . IEEE Transactions on Neural Systems and Rehabilitation Engineering , 2011, 19(6): 652-659.
G Zeilig, H Weingarden, M Zwecker, et al. Safety and tolerance of the ReWalk™ exoskeleton suit for ambulation by people with complete spinal cord injury: A pilot study . The Journal of Spinal Cord Medicine , 2012, 35(2): 96-101.
J Gancet, M Ilzkovitz, E Motard, et al. MINDWALKER: going one step further with assistive lower limbs exoskeleton for SCI condition subjects . IEEE RAS & EMBS International Conference on Biomedical Robotics and Biomechatronics , Rome, Italy, June 24-27, 2012: 1794-1800.
J Gancet, M Ilzkovitz, G Cheron, et al. MINDWALKER: a brain controlled lower limbs exoskeleton for rehabilitation. Potential applications to space . 11th Symposium on Advanced Space Technologies in Robotics and Automation , Noordwijk, Netherlands, April 12-15, 2011: 12-14.
H Satoh, T Kawabata, Y Sankai. Bathing care assistance with robot suit HAL . IEEE International Conference on Robotics and Biomimetics , Guilin, China, December 18-22, 2009: 498-503.
H Yan, C Yang. Design and validation of a lower limb exoskeleton employing the recumbent cycling modality for post-stroke rehabilitation . Proceedings of the Institution of Mechanical Engineers, Part C: Journal of Mechanical Engineering Science , 2014, 228(18): 3517-3525.
Y Miao, F Gao, P Dan. Mechanical design of a hybrid leg exoskeleton to augment load-carrying for walking . International Journal of Advanced Robotic Systems , 2013, 10: https://doi.org/10.5772/57238 .
P Pei, Z Pei, Z Shi, et al. Sensorless control for joint drive unit of lower extremity exoskeleton with cascade feedback observer . Mathematical Problems in Engineering , 2018, 3029514: 1-11, https://doi.org/10.1155/2018/3029514 .
Article MathSciNet Google Scholar
D Liu, Z Tang, Z Pei. Variable structure compensation PID control of asymmetrical hydraulic cylinder trajectory tracking . Mathematical Problems in Engineering , 2015, 890704: 1-9. https://doi.org/10.1155/2015/890704 .
Article MathSciNet MATH Google Scholar
M Lyu, W Chen, X Ding, et al. Design of a biologically inspired lower limb exoskeleton for human gait rehabilitation. Review of Scientific Instruments , 2016, 87(10): 104301.
R Huang, H Cheng, H Guo, et al. Hierarchical learning control with physical human-exoskeleton interaction . Information Sciences , 2018, 432: 584-595.
R Huang, H Cheng, Y Chen, et al. Optimisation of reference gait trajectory of a lower limb exoskeleton . International Journal of Social Robotics , 2016, 8(2): 223-235.
D Wang, K Lee, J Ji. A passive gait-based weight-support lower extremity exoskeleton with compliant joints . IEEE Transactions on Robotics , 2016, 32(4): 933-942.
X Wu, D Liu, M Liu, et al. Individualized gait pattern generation for sharing lower limb exoskeleton robot . IEEE Transactions on Automation Science and Engineering , 2018, 15(4): 1459-1470.
C Chen, X Wu, D Liu, et al. Design and voluntary motion intention estimation of a novel wearable full-body flexible exoskeleton robot . Mobile Information Systems , 2017: https://doi.org/10.1155/2017/8682168 .
D A Neumann. Kinesiology of the musculoskeletal system: foundations for rehabilitation . Elsevier Health Sciences, 2013.
J E Muscolino. Kinesiology: The skeletal system and muscle function . Elsevier Medicine, 2011.
A D Kuo. The six determinants of gait and the inverted pendulum analogy: A dynamic walking perspective . Human Movement Science , 2007, 26(4): 617-656.
E F Chehab, T P Andriacchi, J Favre. Speed, age, sex, and body mass index provide a rigorous basis for comparing the kinematic and kinetic profiles of the lower extremity during walking . Journal of Biomechanics , 2017, 58: 11-20.
J W Kwon, S M Son, N K Lee. Changes of kinematic parameters of lower extremities with gait speed: a 3D motion analysis study . Journal of Physical Therapy Science , 2015, 27(2): 477-479.
S Al-Obaidi, J C Wall, A Al-Yaqoub, et al. Basic gait parameters: A comparison of reference data for normal subjects 20 to 29 years of age from Kuwait and Scandinavia . Journal of Rehabilitation Research and Development , 2003, 40(4): 361-366.
T J Cunningham. Three-dimensional quantitative analysis of the trajectory of the foot while running. University of Kentucky, 2007.
J L Lelas, G J Merriman, P O Riley, et al. Predicting peak kinematic and kinetic parameters from gait speed . Gait & Posture , 2003, 17(2): 106-112.
B Koopman, E Van Asseldonk, H Van der Kooij. Speed-dependent reference joint trajectory generation for robotic gait support . Journal of Biomechanics , 2014, 47(6): 1447-1458.
M Hanlon, R Anderson. Prediction methods to account for the effect of gait speed on lower limb angular kinematics . Gait & Posture , 2006, 24(3): 280-287.
S Ko, S Stenholm, L Ferrucci. Characteristic gait patterns in older adults with obesity - results from the baltimore longitudinal study of aging . Journal of Biomechanics , 2010, 43(6): 1104-1110.
A B Zoss, H Kazerooni, et al. Biomechanical design of the Berkeley lower extremity exoskeleton (BLEEX) . IEEE/ASME Transactions on Mechatronics , 2006, 1(2): 128-138.
S K Banala, S K Agrawal, S H Kim, et al. Novel gait adaptation and neuromotor training results using an active leg exoskeleton. Mechatronics , 2010, 15(2): 216-225.
R Riener, L Lunenburger, S Jezernik, et al. Patient-cooperative strategies for robot-aided treadmill training: first experimental results . IEEE Transactions on Neural Systems and Rehabilitation Engineering , 2005, 13(3): 380-394.
J Meuleman, E Van Asseldonk, G Van Oort, et al. LOPES II—design and evaluation of an admittance controlled gait training robot with shadow-leg approach . IEEE Transactions on Neural Systems and Rehabilitation Engineering , 2016, 24(3): 352-363.
R Ekkelenkamp, J Veneman, H Van der Kooij. LOPES: Selective control of gait functions during the gait rehabilitation of CVA patients . International Conference on Rehabilitation Robotics , Chicago, IL, USA, June 28 - July 1, 2005: 361-364.
N Kwak, K Muller, S Lee. Toward exoskeleton control based on steady state visual evoked potentials . International Winter Workshop on Brain-Computer Interface (BCI) , Gangwon Province, Korea (South), February 17-19, 2014: 1-2.
A Tsukahara, Y Hasegawa, K Eguchi, et al. Restoration of gait for spinal cord injury patients using hal with intention estimator for preferable swing speed . IEEE Transactions on Neural Systems and Rehabilitation Engineering , 2015, 23(2): 308-318.
M W Whittle. Gait analysis: an introduction . Butterworth-Heinemann, 2014.
J H Hollman, M K Watkins, A C Imhoff, et al. A comparison of variability in spatiotemporal gait parameters between treadmill and overground walking conditions . Gait & Posture , 2016, 43: 204-209.
S J Lee, J Hidler. Biomechanics of overground vs. treadmill walking in healthy individuals . Journal of Applied Physiology , 2008, 104(3): 747-755.
J Mehrholz, L A Harvey, S Thomas, et al. Is body-weight-supported treadmill training or robotic-assisted gait training superior to overground gait training and other forms of physiotherapy in people with spinal cord injury? A systematic review. Spinal Cord , 2017, 55(8): 722-729.
S Srivastava, P Kao, S H Kim, et al. Assist-as-needed robot-aided gait training improves walking function in individuals following stroke . IEEE Transactions on Neural Systems and Rehabilitation Engineering , 2015, 23(6): 956-963.
T Senthilvelkumar, H Magimairaj, J Fletcher, et al. Comparison of body weight-supported treadmill training versus body weight-supported overground training in people with incomplete tetraplegia: a pilot randomized trial. Clinical Rehabilitation , 2015, 29(1): 42-49.
M Alcobendasmaestro, A Esclarínruz, R M Casadolópez, et al. Lokomat robotic-assisted versus overground training within 3 to 6 months of incomplete spinal cord lesion: randomized controlled trial. Neurorehabilitation & Neural Repair , 2012, 26(9): 1058.
S A Graham, C P Hurt, D A Brown. Minimizing postural demands of walking while still emphasizing locomotor force generation for nonimpaired individuals. IEEE Transactions on Neural Systems and Rehabilitation Engineering: A Publication of the IEEE Engineering in Medicine and Biology Society , 2018, 26(5): 1003-1010.
V C Dionisio, C P Hurt, D A Brown. Effect of forward-directed aiding force on gait mechanics in healthy young adults while walking faster . Gait & Posture , 2018, 64: 12-17.
J Patton, D A Brown, M Peshkin, et al. KineAssist: design and development of a robotic overground gait and balance therapy device . Topics in Stroke Rehabilitation , 2008, 15(2): 131-139.
D Zanotto, P Stegall, S K Agrawal. ALEX III: A novel robotic platform with 12 DOFs for human gait training . IEEE International Conference on Robotics and Automation , Karlsruhe, Germany, 2013, 2013: 3914-3919.
Y Yu, W Liang. Manipulability inclusive principle for hip joint assistive mechanism design optimization. The International Journal of Advanced Manufacturing Technology , 2014, 70(5-8): 929-945.
W Zhang, S Zhang, M Ceccarelli, et al. Design and kinematic analysis of a novel metamorphic mechanism for lower limb rehabilitation . Springer, Cham, 2016.
W Zhang, W Zhang, D Shi, et al. Design of hip joint assistant asymmetric parallel mechanism and optimization of singularity-free workspace . Mechanism and Machine Theory , 2018, 122: 389-403.
D Wang, K Lee, J Guo, et al. Adaptive knee joint exoskeleton based on biological geometries. IEEE/ASME Transactions on Mechatronics , 2014, 19(4): 1268-1278.
B T Quinlivan, S Lee, P Malcolm, et al. Assistance magnitude versus metabolic cost reductions for a tethered multiarticular soft exosuit . Science Robotics , 2017, 2(2): 1-10.
S A Kolakowsky-Hayner, J Crew, S Moran, et al. Safety and feasibility of using the EksoTM bionic exoskeleton to aid ambulation after spinal cord injury . Journal of Spine , 2013, 4: 1-8.
J Hidler, W Wisman, N Neckel. Kinematic trajectories while walking within the Lokomat robotic gait-orthosis . Clinical Biomechanics , 2008, 23(10): 1251-1259.
H Vallery, J Veneman, E Van Asseldonk, et al. Compliant actuation of rehabilitation robots . IEEE Robotics & Automation Magazine , 2008, 15(3): 60-69.
J E Pratt, B T Krupp, C J Morse, et al. The RoboKnee: an exoskeleton for enhancing strength and endurance during walking . Proceedings of IEEE International Conference on Robotics and Automation , New Orleans, LA, USA, April 26 - May 1, 2004: 2430-2435.
H Yu, S Huang, G Chen, et al. Human–robot interaction control of rehabilitation robots with series elastic actuators . Robotics, IEEE Transactions on ., 2015, 31(5): 1089-1100.
E Sariyildiz, G Chen, H Yu. An acceleration-based robust motion controller design for a novel series elastic actuator. IEEE Transactions on Industrial Electronics , 2016, 63(3): 1900-1910.
H Vallery, R Burgkart, C Hartmann, et al. Complementary limb motion estimation for the control of active knee prostheses . Biomedizinische Technik/Biomedical Engineering , 2011, 56(1): 45-51.
K Seo, Y Park, S Yun, et al. Gait pattern generation for robotic gait rehabilitation system on treadmill . 14th International Conference on Control, Automation and Systems , KINTEX, Gyeonggi-do, Korea, October 22-25, 2014: 1090-1094.
H B Lim, T P Luu, K H Hoon, et al. Natural gait parameters prediction for gait rehabilitation via artificial neural network . IEEE/RSJ International Conference on Intelligent Robots and Systems , Taipei, Taiwan, China, October 18-22, 2010: 5398-5403.
L Wang, E H van Asseldonk, H van der Kooij. Model predictive control-based gait pattern generation for wearable exoskeletons . IEEE International Conference on Rehabilitation Robotics , ETH Zurich Science City, Switzerland, June 29 - July 1, 2011: 1-6.
M R Tucker, J Olivier, A Pagel, et al. Control strategies for active lower extremity prosthetics and orthotics: a review . Journal of Neuroengineering and Rehabilitation , 2015, 12(1): 1-30.
S Maggioni, L Lünenburger, R Riener, et al. Robot-aided assessment of walking function based on an adaptive algorithm . IEEE International Conference on Rehabilitation Robotics , Singapore, Aug. 11-14, 2015: 804-809.
B Koopman, E H van Asseldonk, H van der Kooij. Selective control of gait subtasks in robotic gait training: foot clearance support in stroke survivors with a powered exoskeleton . Journal of Neuroengineering and Rehabilitation , 2013, 10(3): 1-21.
X Jin, X Cui, S K Agrawal. Design of a cable-driven active leg exoskeleton (C-alex) and gait training experiments with human subjects . IEEE International Conference on Robotics and Automation , Seattle, Washington, USA, May 26-30, 2015: 5578-5583.
O Harib, A Hereid, A Agrawal, et al. Feedback control of an exoskeleton for paraplegics: toward robustly stable hands-free dynamic walking . IEEE Control Systems Magazine , 2018, 38(6): 61-87.
T Lenzi, M C Carrozza, S K Agrawal. Powered hip exoskeletons can reduce the user’s hip and ankle muscle activations during walking . IEEE Transactions on Neural Systems and Rehabilitation Engineering , 2013, 21(6): 938-948.
D B Fineberg, P Asselin, N Y Harel, et al. Vertical ground reaction force-based analysis of powered exoskeleton-assisted walking in persons with motor-complete paraplegia . The Journal of Spinal Cord Medicine , 2013, 36(4): 313-321.
P K Jamwal, S Q Xie, S Hussain, et al. An adaptive wearable parallel robot for the treatment of ankle injuries . IEEE/ASME Transactions on Mechatronics , 2014, 19(1): 64-75.
S Hussain, S Q Xie, P K Jamwal. Robust nonlinear control of an intrinsically compliant robotic gait training orthosis . IEEE Transactions on Systems, Man, and Cybernetics: Systems , 2013, 43(3): 655-665.
Z Tang, D Shi, D Liu, et al. Electro-hydraulic servo system for Human Lower-limb Exoskeleton based on sliding mode variable structure control . Information and Automation (ICIA), 2013 IEEE International Conference on , 2013: 559-563.
J Pratt, C Chew, A Torres, et al. Virtual model control: An intuitive approach for bipedal locomotion . The International Journal of Robotics Research , 2001, 20(2): 129-143.
T Lenzi, S M De Rossi, N Vitiello, et al. Intention-based EMG control for powered exoskeletons . IEEE Trans. Biomed Eng ., 2012, 59(8): 2180-2190.
H He, K Kiguchi. A study on EMG-based control of exoskeleton robots for human lower-limb motion assist . Information Technology Applications in Biomedicine, 2007. ITAB 2007. 6th International Special Topic Conference on , 2007: 292-295.
P Kao, C L Lewis, D P Ferris. Invariant ankle moment patterns when walking with and without a robotic ankle exoskeleton . Journal of Biomechanics , 2010, 43(2): 203-209.
D P Ferris, K E Gordon, G S Sawicki, et al. An improved powered ankle–foot orthosis using proportional myoelectric control . Gait & Posture , 2006, 23(4): 425-428.
A R Donati, S Shokur, E Morya, et al. Long-term training with a brain-machine interface-based gait protocol induces partial neurological recovery in paraplegic patients . Scientific Reports , 2016, 6: 30383.
D Liu, W Chen, Z Pei, et al. A brain-controlled lower-limb exoskeleton for human gait training . Review of Scientific Instruments , 2017, 88(10): 104302.
N Kwak, K Müller, S Lee. A lower limb exoskeleton control system based on steady state visual evoked potentials . Journal of Neural Engineering , 2015, 12(5): 56009.
R Xu, N Jiang, N Mrachacz-Kersting, et al. A closed-loop brain-computer interface triggering an active ankle-foot orthosis for inducing cortical neural plasticity. IEEE Transactions on Biomedical Engineering , 2014, 61(7): 2092-2101.
G Aguirre-Ollinger, U Nagarajan, A Goswami. An admittance shaping controller for exoskeleton assistance of the lower extremities . Autonomous Robots , 2016, 40(4): 701-728.
H Ding, X Yang, N Zheng, et al. Tri-Co Robot: a Chinese robotic research initiative for enhanced robot interaction capabilities. National Science Review , 2017, 5(6): 799-801.
Download references
Authors’ Contributions
XD was in charge of the whole trial; DS wrote the manuscript; WXZ and WZ assisted with structure and language of the manuscript. All authors read and approved the final manuscript.
Authors’ Information
Di Shi, born in 1988, is currently a PhD candidate at Robotics Institute, Beihang University, China . He received his bachelor degree from Wuhan University of Technology, China , in 2011. And he received his master degree from Beihang University, China , in 2014. His research interests include wearable lower limb rehabilitation robot control.
Wuxiang Zhang, born in 1978, is currently an associate professor at School of Mechanical Engineering and Automation, Beihang University, China . He received his PhD degree from Beihang University, China , in 2009. His research interests include the dynamics of compliant mechanical systems and robots, intelligent device and detection technology.
Wei Zhang, born in 1986, is currently a PhD candidate at Robotics Institute, Beihang University, China . He received his bachelor’s degree from Northwestern Polytechnical University, China , in 2009. His research interests include mechanical design of the wearable robot.
Xilun Ding, born in 1967, is currently a professor and a PhD candidate supervisor at School of Mechanical Engineering and Automation, Beihang University, China . He received his PhD degree from Harbin Institute of Technology, China , in 1997. His research interests include the dynamics of compliant mechanical systems and robots, nonholonomic control of space robots, dynamics and control of aerial robots, and biomimetic robots.
Acknowledgements
The authors sincerely thank Mr. Chong Qi, and Yixin Shao of Beihang University for their critical discussion and reading during manuscript preparation.
Competing Interests
The authors declare that they have no competing interests.
Supported by National Key R&D Program of China (Grant No. 2016YFE0105000) and National Natural Science Foundation of China (Grant No. 91848104).
Author information
Authors and affiliations.
School of Mechanical Engineering and Automation, Beihang University, Beijing, 100191, China
Di Shi, Wuxiang Zhang, Wei Zhang & Xilun Ding
Beijing Advanced Innovation Center for Biomedical Engineering, Beihang University, Beijing, 100191, China
You can also search for this author in PubMed Google Scholar
Corresponding author
Correspondence to Wuxiang Zhang .
Rights and permissions
Open Access This article is distributed under the terms of the Creative Commons Attribution 4.0 International License ( http://creativecommons.org/licenses/by/4.0/ ), which permits unrestricted use, distribution, and reproduction in any medium, provided you give appropriate credit to the original author(s) and the source, provide a link to the Creative Commons license, and indicate if changes were made.
Reprints and permissions
About this article
Cite this article.
Shi, D., Zhang, W., Zhang, W. et al. A Review on Lower Limb Rehabilitation Exoskeleton Robots. Chin. J. Mech. Eng. 32 , 74 (2019). https://doi.org/10.1186/s10033-019-0389-8
Download citation
Received : 05 March 2019
Revised : 30 July 2019
Accepted : 14 August 2019
Published : 30 August 2019
DOI : https://doi.org/10.1186/s10033-019-0389-8
Share this article
Anyone you share the following link with will be able to read this content:
Sorry, a shareable link is not currently available for this article.
Provided by the Springer Nature SharedIt content-sharing initiative
- Control method
- Lower limb exoskeleton
- Mechanical design
- Rehabilitation robot
- Reference Manager
- Simple TEXT file
People also looked at
Original research article, robotic home-based rehabilitation systems design: from a literature review to a conceptual framework for community-based remote therapy during covid-19 pandemic.
- Department of Mechanical Engineering, Isfahan University of Technology, Isfahan, Iran
During the COVID-19 pandemic, the higher susceptibility of post-stroke patients to infection calls for extra safety precautions. Despite the imposed restrictions, early neurorehabilitation cannot be postponed due to its paramount importance for improving motor and functional recovery chances. Utilizing accessible state-of-the-art technologies, home-based rehabilitation devices are proposed as a sustainable solution in the current crisis. In this paper, a comprehensive review on developed home-based rehabilitation technologies of the last 10 years (2011–2020), categorizing them into upper and lower limb devices and considering both commercialized and state-of-the-art realms. Mechatronic, control, and software aspects of the system are discussed to provide a classified roadmap for home-based systems development. Subsequently, a conceptual framework on the development of smart and intelligent community-based home rehabilitation systems based on novel mechatronic technologies is proposed. In this framework, each rehabilitation device acts as an agent in the network, using the internet of things (IoT) technologies, which facilitates learning from the recorded data of the other agents, as well as the tele-supervision of the treatment by an expert. The presented design paradigm based on the above-mentioned leading technologies could lead to the development of promising home rehabilitation systems, which encourage stroke survivors to engage in under-supervised or unsupervised therapeutic activities.
Introduction
Coronavirus disease 2019 (COVID-19) is an infectious disease with serious public health risk declared a global pandemic by WHO on March 11, 2020. In the present time of the COVID-19 pandemic, the lives of individuals have been drastically affected due to the imposed restrictions such as social distancing, curfews, and travel restrictions. This situation has had a considerable impact on the lives of certain more vulnerable groups on a larger scale, namely people living with chronic diseases such as stroke. In a pooled data analysis published in the International Journal of Stroke (IJS), Aggarwal et al. emphasized that COVID-19 puts post-stroke patients at a greater risk of developing complications and death. In fact, the odds of severe COVID-19 infection increase by 2.5 times in patients with a history of cerebrovascular disease ( Aggarwal et al., 2020 ). This calls for consideration of extra safety precautions for this vulnerable population to protect them from infected environments, namely strictly abiding by the quarantine rules and social distancing, as breaking them could prove fatal ( Markus and Brainin, 2020 ).
Stroke is accounted one of the dominant causes of severe and long-term disability. It leads to a total or partial loss of aptitude to trigger muscle activation to perform any activity ( Borboni et al., 2016 ). The broad spectrum of induced disabilities includes a reduced range of motion (ROM), strength of the affected limb, and abnormal inter-joint coordination ( Chen J. et al., 2017 ). The sensitive/motor deficits impede the performance of activities of daily living (ADLs), such as reaching, grasping, and lifting objects, as well as walking, etc., in the surviving individuals ( Chen J. et al., 2017 ; Heung et al., 2019 ). Patients must be subjected to rehabilitative treatment to help them regain the ability to perform daily activities independently. As Martinez-Martin et al. stated, “Rehabilitation can be defined as the step-by-step process designed to reduce disability and to optimize functioning in individuals with health conditions, enabling them to better interact with their environment.” ( Martinez-Martin and Cazorla, 2019 ). In the post-stroke rehabilitation process, the period between the first and the sixth months after stroke, known as the post-stroke sensitive period, has been proved to bear the maximum recovery impact, both spontaneous and intervention-mediated. Indeed, according to the statistics presented in Krakauer et al. article, during the first four weeks of rehabilitation, failure of reaching an arm Fugl-Meyer score of at least 11 would indicate only a 6% possibility of regaining dexterity at six months ( Krakauer 2006 ). Regarding the aforementioned issues, in the current restrictive climate of COVID-19, the crucial question to be addressed for post-stroke patients would be how to use this critical and limited window of time to achieve the best possible recovery.
Robot-mediated therapy for post-stroke rehabilitation offers highly repetitive, high-dosage, and high-intensity alternatives, while reducing labor intensity and the manual burden on therapists. Hence, myriads of studies have focused on exploring robotics technologies for post-stroke rehabilitation. An increasing amount of research has investigated the efficiency of different types of robotic rehabilitation systems and found that these interventions can effectively complement conventional physical therapy, e.g., Mehrholz et al. and Bertani et al. investigated the effects of robot-assisted gait and upper-limb training, respectively, and both concluded that using robotic technologies positively affects post-stroke recovery ( Bertani et al., 2017 ; Mehrholz et al., 2020 ). To tackle the recent rising issues associated with restrictions caused by the pandemic, there is a need to speed up the process of providing autonomous and affordable care that can be transferred out of inpatient or out-patient facilities into home environments. This study reviews the existing home-based robotic rehabilitation interventions and proposes reliable concepts that can be used to confront the discussed problems.
Home-based rehabilitation systems can be considered viable options capable of promoting care delivery while adhering to physical distancing measures and reducing the potential exposure to the infectious virus along with protecting vulnerable stroke survivors. Besides, even prior to this pandemic, the demand for home-based rehabilitation far exceeded its availability, further emphasizing the importance of this form of rehabilitation as a sustainable solution. According to WHO, demand for rehabilitation is approximately ten times that of the capacity of the service that the current healthcare system can provide, in terms of both rehabilitation professionals and rehabilitative tools ( Gupta et al., 2011 ; World Health Organization, 2017 ). This poses a clear priority on further extension of home-based rehabilitation, which could prove to be a better alternative than conventional care by maintaining physical distancing and ameliorating the saturated health service.
Home-based systems offer a platform for unsupervised or under-supervised therapy, in which the need for the physical presence of a therapist is reduced. Rehabilitative treatments need to be intensive with long duration to improve functional outcomes and motor recovery ( Bütefisch et al., 1995 ). Compared to clinical therapy, home therapy potentially augments standard care and enables consistent treatment by increasing the frequency and duration of training sessions. Performing rehabilitation at home provides patients with a comfortable setting. It gives them a sense of control of therapy as it reduces their reliance on external assistance ( Chen et al., 2019 ). This can result in the patients demonstrating enhanced motivation and engagement ( Borghese et al., 2013 ). In terms of the associated costs, home-based therapy reduces the expenses compared with clinical-based therapy; for example, in statistics provided by Housley et al., a saving of $2,352 (64.97%) was reported ( Housley et al., 2016 ).
However, deployment in the unsupervised context of home-based rehabilitation poses risks to patients. Therapists perceive risks to patients regarding the training/acquisition of harmful movements when unsupervised at home—abnormal movement can be damaging or slow recovery ( Borghese et al., 2014 ). Careful system design and deployment measures need to be taken, such as providing adequate feedback on proper task execution, to preclude these movements. On the other hand, in robotic medical devices the occurrence of errors that cannot be accounted for or predicted during device design leads to patient injuries and more severe incidents in some cases ( Kim, 2020 ), e.g., crashes in device operability, both in hardware and software, and errors induced by contextual barriers in patients home environment. In clinical settings, in case of such incidents, the physical presence of healthcare professionals could mitigate the risk, yet such an option is not available at home.
Due to the benefits mentioned above, robot-mediated home therapy has gained attraction in recent years. Its feasibility has been evaluated through several studies using state-of-the-art home robots. The literature surveys indicated the feasibility of self-administered treatment at home using rehabilitation robots in terms of functional outputs, training duration, user acceptance, motivation, and safety concerns. Remotely supervised participants of these studies exhibited increased motivation and autonomy in completing the prescribed task with no adverse events or edema. They also self-reported increased mobility, improved mood, and an outlet for physical and mental tension and anxiety ( Sivan et al., 2014 ; Nijenhuis et al., 2015 ; Cherry et al., 2017 ; Bernocchi et al., 2018 ; Catalan et al., 2018 ). Catalan et al. compared the performance of a commercialized clinical upper-limb rehabilitation device and its newly developed home-based counterpart, which showed that functional outcomes of treatment are similar for home users and clinic patients ( Catalan et al., 2018 ). This suggests that, although both groups would reach the task’s goals similarly in terms of session numbers, due to the higher frequency of home-based therapy, users are able to master tasks in a shorter timespan ( Godlove et al., 2019 ).
This article is intended to be used as a general guideline for developing robotic home-based rehabilitation systems. For this purpose, first, the authors conducted a comprehensive review on developed home-based rehabilitation technologies of the last 10 years (2011–2020), categorizing them into upper and lower limb devices and considering both commercialized and state-of-the-art realms. The literature review analyzes and synthesizes the current knowledge of home-based robotic systems. This aims to provide a categorized comparison among reviewed literature leading to a classified roadmap to help guide current research and propose recommendations for advancing research development in this field. By addressing current challenges and shortcomings, three main aspects are considered in the proposed design paradigm, i.e., mechatronics, control, and user interface. While existing reviews take a generalized approach on home-based rehabilitation solutions—e.g., Chen et al. provided a systematic review based on the utilized technology types ( Chen et al., 2019 )—we focus and expand on robotic devices. In contrast to solutions relying solely on VR and game-based technologies, this paper addresses robotics-based technologies to cover the need for a significantly wider range of post-stroke patients, including patients who require external assistance reflecting the therapist’s role in unsupervised settings. In the end, a conceptual community-based robotic rehabilitation framework, offering smart rehabilitation, is also introduced.
Home-Based Rehabilitation Systems
Over the last decade, researchers have been addressing existing challenges and requirements to design and develop rehabilitation robots suitable for home therapy. As a result, many at-home rehabilitation devices have been designed within the research realm, and some have been commercialized ( Tables 1 – 4 ). In the following subsections, a comprehensive review of developed home-based rehabilitation technologies of the last ten years (2011–2020) is presented.
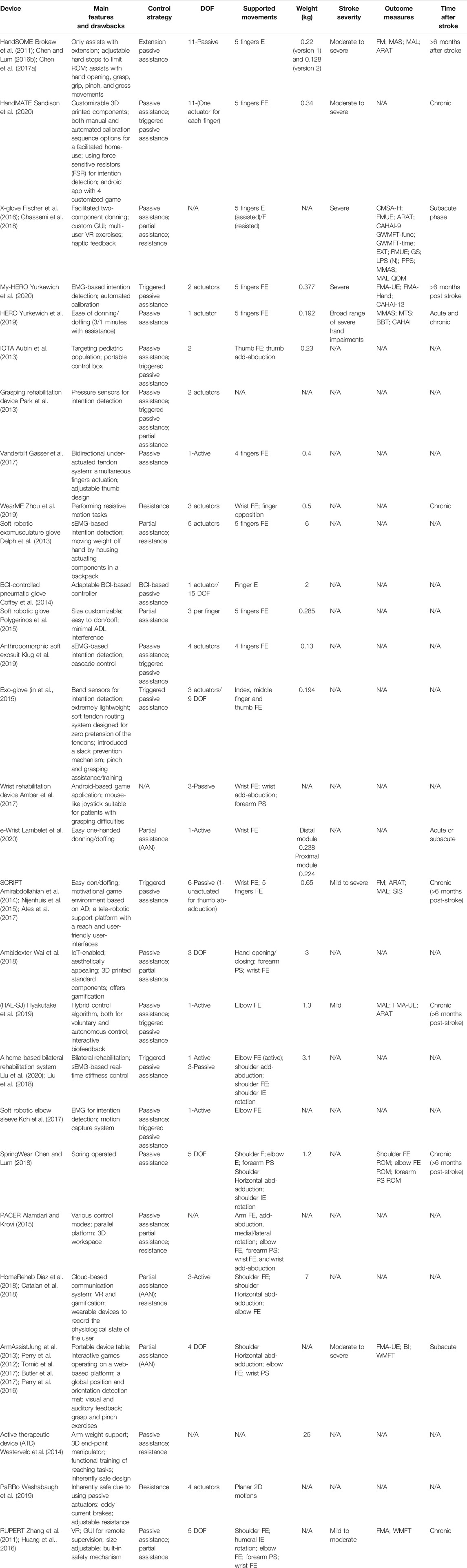
TABLE 1 . Summary of state-of-the-art robotic systems for home-based upper-limb rehabilitation.
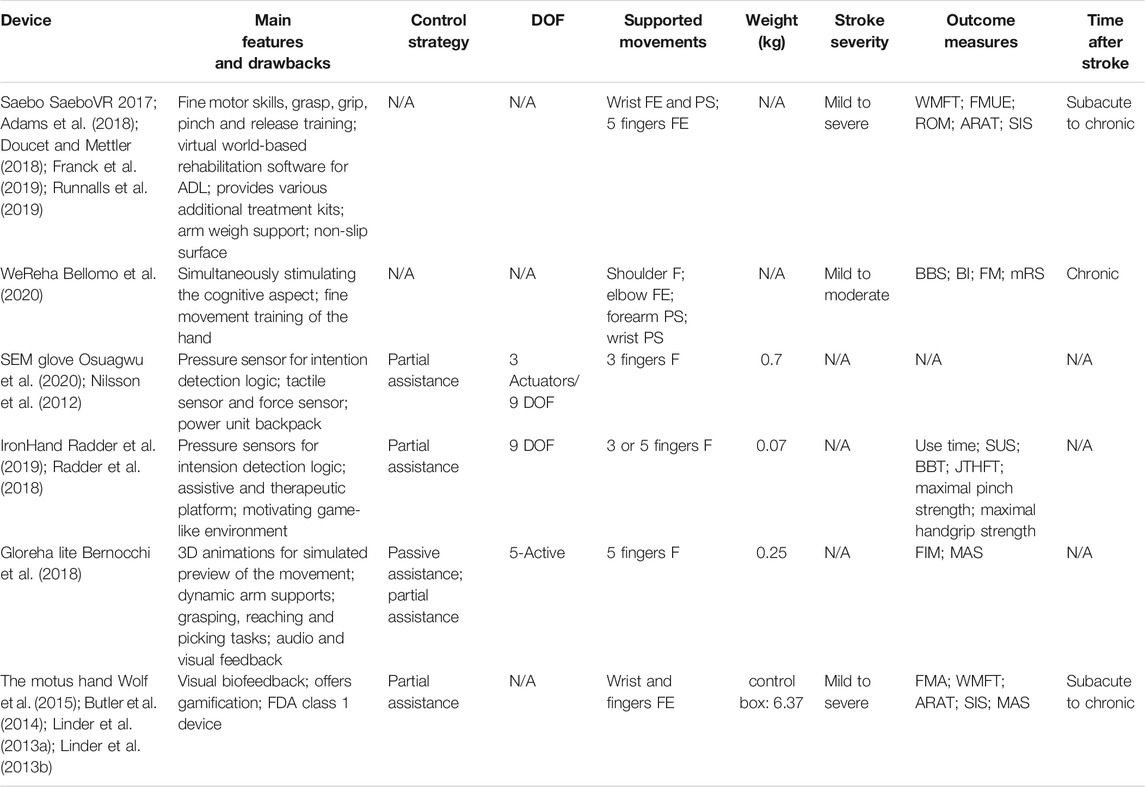
TABLE 2 . Summary of commercialized robotic systems for home-based upper-limb rehabilitation.
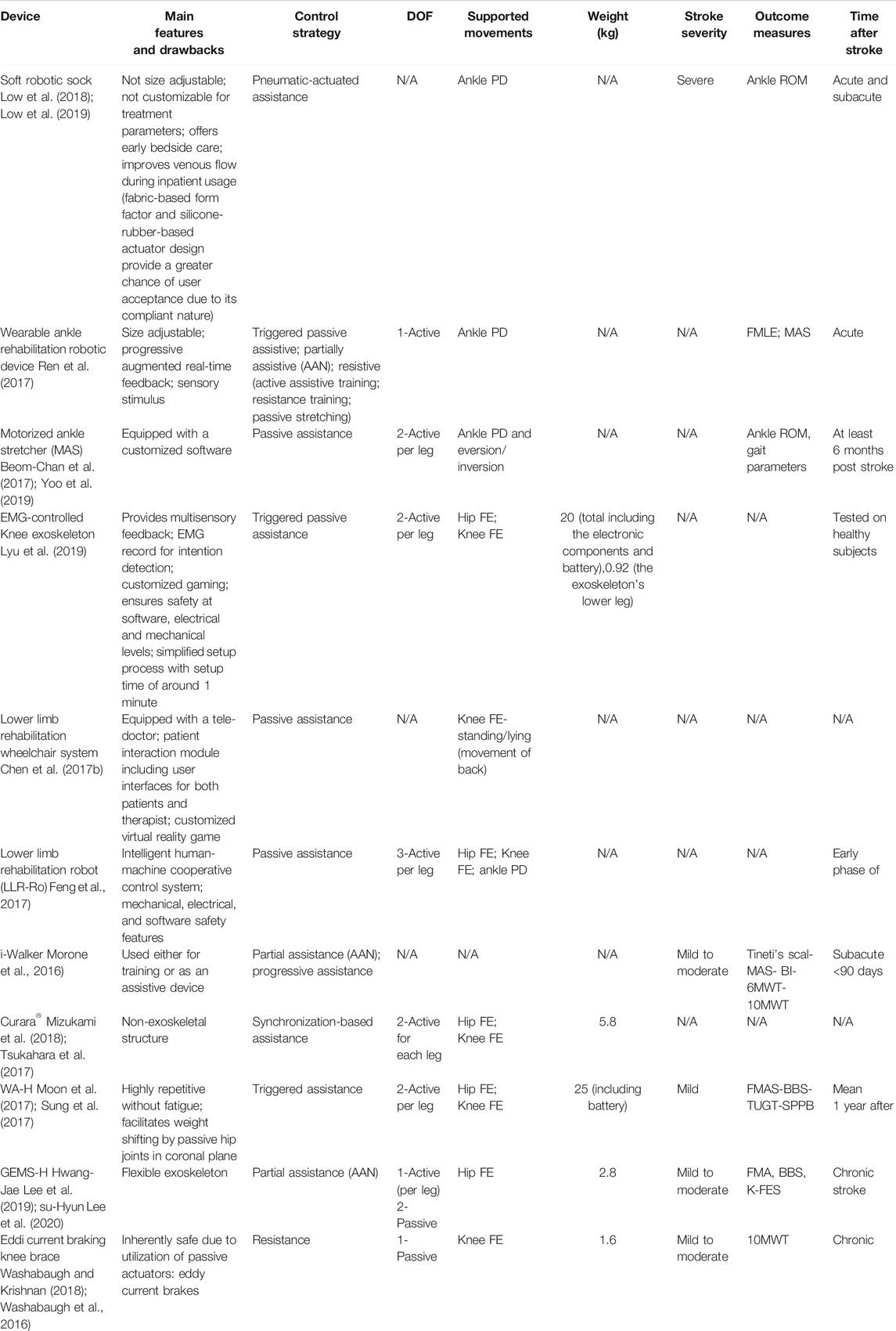
TABLE 3 . Summary of state-of-the-art robotic systems for home-based lower-limb rehabilitation.
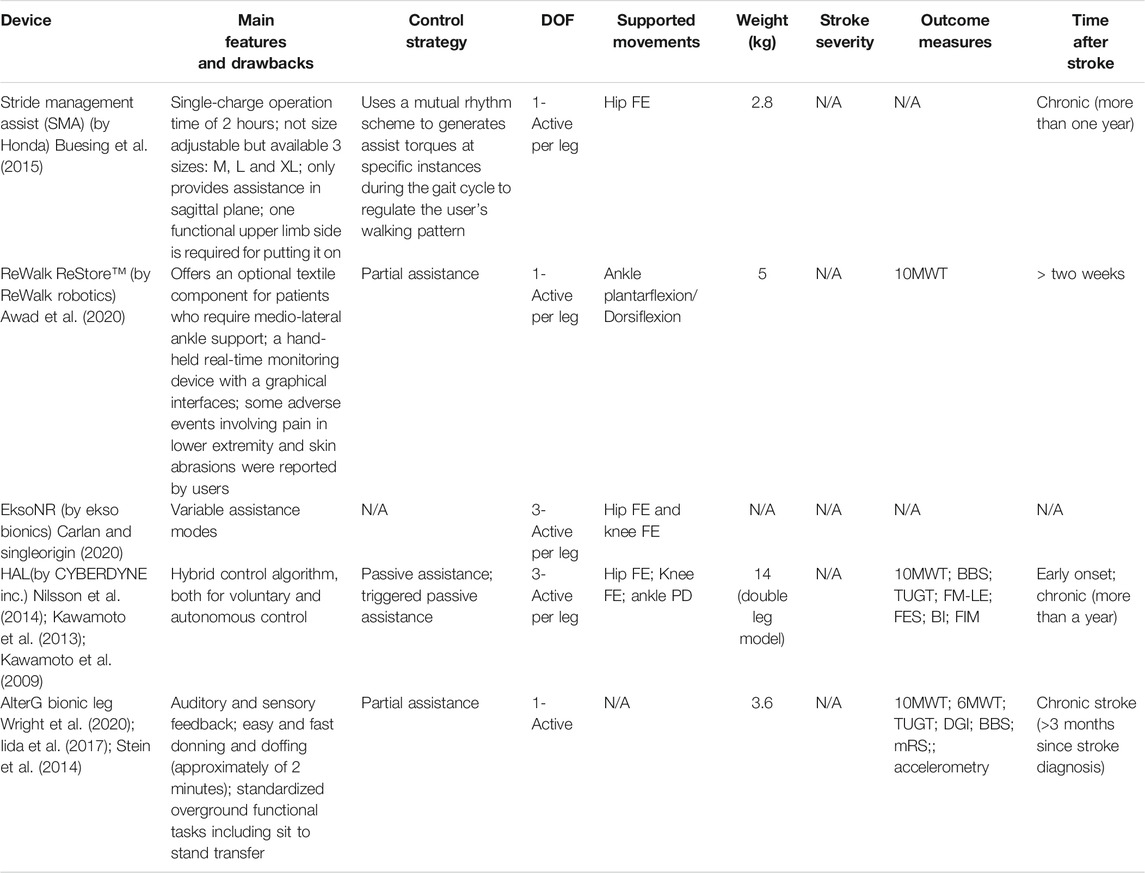
TABLE 4 . Summary of commercialized robotic systems for home-based lower-limb rehabilitation.
We conducted a literature review in the PubMed search engine. The search included the following terms: “Rehabilitation Robotics,” “Home-Based Rehabilitation,” and “Stroke Rehabilitation.” Secondary references and citations of the resultant articles were checked to further identify relevant literature and other available sources providing information on commercially available solutions. Reviewers screened the abstracts of the collected articles for extracting those satisfying the eligibility criteria. Studies were excluded if they were not implemented and/or did not demonstrate implementation potential in home settings, based on the criteria introduced by authors in the following subsections.
Approximately 70% of stroke patients experience impaired arm function ( Intercollegiate Working Party for Stroke, 2012 ). Hemiparesis is prevalent in up to 88% of post-stroke patients, which mostly leads to gait and balance disorders, that even persists in almost one-third of patients even after rehabilitation interventions, leaving them with the inability in independent walking ( Gresham et al., 1995 ; Duncan et al., 2005 ; Díaz et al., 2011 ; Morone et al., 2016 ). Lower-limb devices face critical challenges for home use, making the upper-limb the primary focus of early efforts in this field. The following subsections were set to cover both state-of-the-art and commercialized devices by categorizing based on the upper or lower targeted limb.
State-of-the-Art: Systems in the Literature
Due to the significant results of active participation between patients and robots in functional improvement, the majority of current rehabilitative robots are equipped with electrical or pneumatic motors ( Pehlivan et al., 2011 ). However, the inherent considerable weight of mounted motors precludes the device from being used during daily activities, since proximal arm weakness is prevalent among individuals with stroke. Thus, to allow hand rehabilitation during the performance of ADLs, HandSOME ( Brokaw et al., 2011 ), a passive lightweight wearable device has been developed. The design is based on the concepts of patient-initiated repetitive tasks to rehabilitate and assist during ADL performance by increasing assistive torque with increasing extension angle. To help with opening the patient’s hand and assisting with finger and thumb extension movements, HandSOME uses a series of elastic cords, as springs, to apply extension torques to the finger joint. For safety precautions, adjustable hard stops are used to control the ROM. Studies demonstrated that HandSOME could benefit stroke patients with ROM improvement. While patients commented that the device was generally comfortable for use at home ( Chen J. et al., 2017 ), there is a need to develop a remote communication system instead of weekly clinical visits. Yet, one of the disadvantages of the device is its inability of assistance level adaptation to patient performance. Addressing this issue, Sandison et al. built a wearable motorized hand exoskeleton, HandMATE, upon HandSOME. This device benefits from 3D printing technology for manufacturing the components; hence it can be optimally adjustable and customizable to fit the patients’ physiological parameters ( Sandison et al., 2020 ). Combining hand orthosis with serious gaming, Ghasemi et al. have integrated eXtention Glove (X-Glove) actuated glove orthosis with a VR system to augment home-based hand therapy. For a facilitated donning, the device design allows for being put on by two separate components ( Ghassemi et al., 2018 ). The glove provides both stretching therapy and extension assistance for each digit independently while allowing free movements and interaction with real-world objects ( Fischer et al., 2016 ). Gasser et al. presented compact and lightweight hand exoskeleton Vanderbilt intended to facilitate ADLs for post-stroke hand paresis. The design includes an embedded system and onboard battery to provide a single degree of freedom (DOF) actuation that assists with both opening and closing of a power grasp ( Gasser et al., 2017 ).
The Hand Extension Robot Orthosis (HERO) Glove is another wearable rehabilitation system that provides mechanical assistance to the index and middle fingers and thumb ( Yurkewich et al., 2019 ). Linear actuators control the artificial tendons embedded into the batting glove’s fingers for finger extension and grip assistance. Yurkewich et al. proceeded with their research by introducing My-HERO, a battery-powered, myoelectric untethered robotic glove. The new glove benefits from forearm electromyography for sensing the user’s intent to grasp or release objects and provides assistance to all five fingers ( Yurkewich et al., 2020 ). Addressing the pediatric disorders, such as stroke, causing thumb deformation, lightweight hand-mounted rehabilitation exoskeleton, the Isolated Orthosis for Thumb Actuation (IOTA), offers 2 degrees of freedom thumb rehabilitation at home while allowing for significant flexibility in the patient’s wrist ( Aubin et al., 2013 ). The device is patient-specific and can be securely aligned and customized to the patient’s hand. The portable control box of the design enhances user freedom and allows rehabilitation exercises to be executed virtually anywhere. For recovering hand grasp function, Park et al. developed a robotic grasp rehabilitation device integrated with patient intention detection utilizing handle-embedded pressure sensors ( Park et al., 2013 ). The device was designed for home use by being small in size, portable, and inexpensive. As one of the first wearable robots performing resistive training, Wearable Mechatronics-Enabled (WearME) glove was developed coupled with an associated control system for enabling the execution of functional resistive training. The soft-actuated cable-driven mechanism of the power actuation allows for applying resistive torque to the index finger, thumb, and wrist independently ( Zhou et al., 2019 ).
Targeting individuals with functional grasp pathologies, Delph et al. developed an sEMG-based cable-driven soft robotic glove that can independently actuate all five fingers to any desired position between open and closed grip using position or force control and simultaneously regulate grip force using motor current ( Delph et al., 2013 ). Coffey et al. integrated a soft pneumatic glove with a novel EEG-based BCI controller for an increased motor-neurorehabilitation during hand therapy at home ( Coffey et al., 2014 ). In contrast to clinical BCI-mediated solutions, it is an inexpensive and simplified alternative for training the subject’s wrist and fingers at home together with a haptic feedback system. Polygerinos et al. presented a portable soft robotic glove that combines assistance with ADL and at-home rehabilitation ( Polygerinos et al., 2015 ). Hydraulically actuated multi-segment soft actuators using elastomers with fiber reinforcements induce specific bending, twisting, and extending trajectories when pressurized. The soft actuators are able to replicate the finger and thumb motions suitable for many typical grasping motions, to match and support the range of motion of individual fingers. Furthermore, the device has an open palm design in which the actuators are mounted to the dorsal side of the hand. This provides an open-palm interface, potentially increasing user freedom as it does not impede object interaction. The entire compact system can be packaged into one portable waist belt pack that can be operated for several hours on a single battery charge. Gross and fine functional grasping abilities of the robotic glove in free-space and interaction with daily life objects were qualitatively evaluated on healthy subjects. Compared to other robotic rehabilitation devices, the soft robotic glove potentially increases independence, as it is lightweight and portable. An electrically actuated tendon-driven soft exosuit was developed for supporting and training hand’s grasp function. The device offers versatile rehabilitation exercises covering motion patterns, including both power and precision grip, on each independently actuated finger ( Klug et al., 2019 ). The Exo-Glove, a soft wearable robot using a glove interface for hand and finger assistance, developed by In et al., employs a soft tendon routing system and an underactuated adaptive mechanism ( In et al., 2015 ). Inspired by the human musculoskeletal system, Exo-Glove transmits the tension of the tendons routed around the index and middle finger at the palm and back sides to the body to induce flexion and extension motions of the fingers.
The majority of upper-limb devices are dedicated to wrist rehabilitation due to its importance for peoples’ daily work and life ( Wang and Xu, 2019 ). By combining sensing technology with an interactive computer game, Ambar et al. aimed at developing a portable device for wrist rehabilitation ( Ambar et al., 2017 ). To consider difficulties of stroke patients in firm grasping, the design was based on a single-person mouse-like joystick. The third DOF is considered for forearm pronation/supination, adding to standard flexion/extension and adduction-abduction movements for wrist rehabilitation. Clinical trials on healthy subjects using the device have shown task completion through a smooth recorded trajectory. In 2020, they also developed an android-based game application to enable patients to use the rehabilitation device at home or anywhere while making the therapy systematic and enjoyable ( Ail et al., 2020 ). Lambelet et al. developed a fully portable sEMG-based force-controlled wrist exoskeleton offering extension/flexion assistance, eWrist. Given the prominence of the donning aspects of rehabilitation robots in unsupervised settings, the device was iteratively designed emphasizing attachment mechanism and distal weight reduction to enable one-hand and independent donning of the device ( Lambelet et al., 2020 ).
One of the first projects dedicated to enabling home rehabilitation is the SCRIPT project. SCRIPT project—Supervised Care, and Rehabilitation Involving Personal Tele-robotics—is based on designing a passive finger, thumb, and wrist orthosis for stroke rehabilitation ( Amirabdollahian et al., 2014 ). SPO-F, the final design, is equipped with novel actuation mechanisms at the fingers and wrist and a motivational game environment based on ADL, combined with remotely monitored consistent interfaces ( Ates et al., 2017 ). To make the devices inherently safe and integrable to home environment, a dynamic but passive mechanism is implemented, providing adaptable and compliant extension assistance. The device is targeted at patients who are able to generate some residual muscle control. Also, it utilizes physical interfaces developed by Saebo Inc. due to its proven track record in providing safe and comfortable interaction. An evaluation study on post-stroke patients using the device training with virtual reality games indicated the feasibility of home training using SPO-F, providing reports on the compliance and improvement of hand function after training ( Nijenhuis et al., 2015 ) ( Figure 1 ).
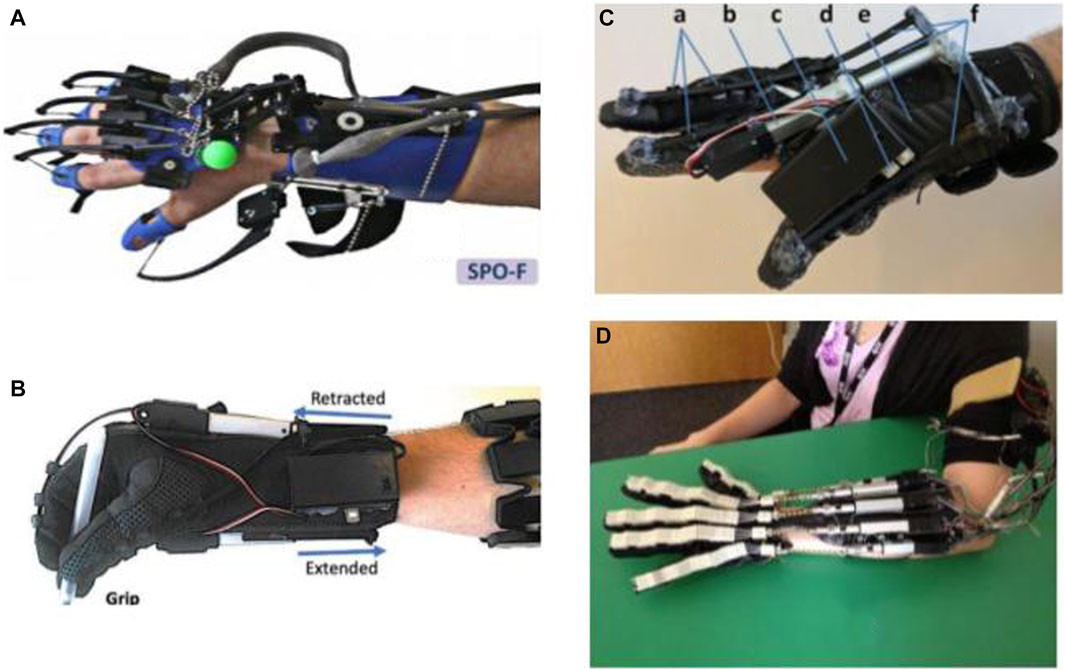
FIGURE 1 . Robotic gloves and exoskeleton devices for home-based hand rehabilitation: (A) SCRIPT project ( Ates et al., 2017 ), (B) My-HERO ( Yurkewich et al., 2020 ), (C) HERO ( Yurkewich et al., 2019 ), and (D) X-Glove ( Triandafilou, 2014 ).
Liu et al. integrated a powered variable-stiffness elbow exoskeleton device with an sEMG-based real-time joint stiffness control to offer bilateral rehabilitation to patients suffering from hemiparesis ( Liu et al., 2021 ). For a patient-specific approach ensuring human-like behavior patterns and facilitated coordinated movements, the device mirrors the dynamic movement captured from the unaffected side to generate stiffness-adapted motion to the contralateral side ( Liu et al., 2018 ). The device also benefits from five passive DOFs for providing natural range of motion and minimizing misalignments between the robot and hand joints. Koh et al. introduced a soft robotic elbow sleeve for enabling flexion and extension of the elbow through passive and intent-based assisted movement execution. Further investigation is required to assess the efficiency of the device in neuro-muscular training ( Koh et al., 2017 ).
Motivated by their prior research with HandSOME, Chen et al. attempted to target another population of patients with arm weakness instead of grasping impairment and developed a spring-operated wearable upper limb exoskeleton, called SpringWear, for potential at-home arm rehabilitation ( Chen and Lum, 2018 ). With a total of five DOFs, SpringWear applies angle-dependent assistance to the forearm supination, elbow extension, and shoulder extension while incorporating passive joints for two other shoulder movements to allow complex and lifelike multi-joint movement patterns ( Chen J. P. S. and Lum P. S., 2016 ). Over a ten-year iterative research cycle, Zhang et al. developed the wearable exoskeleton RUPERT—Robotic Upper Extremity Repetitive Trainer—for both clinical and in-home post-stroke upper-extremity therapy that incorporates five degrees of freedom of shoulder, humeral, elbow, forearm, and wrist ( Zhang et al., 2011 ). Each DOF is supported by a compliant and safe pneumatic muscle ( Huang et al., 2016 ). The device employs adaptive sensory feedback control algorithms with associated safety mechanisms. The developers claim that easiness in donning and operating the device and its graphic user interface excludes the need for the presence of a physical therapist.
As the end-effector of the human body, the hand takes the lead of ADL ( Ates et al., 2017 ). Hence, improving the ability to perform ADLs is regarded as one of the main goals of physical/occupational therapy. To this end, Ambidexter, an end-effector type three DOF robotic device, has been developed for training hand opening/closing, forearm pronation/supination, and wrist flexion/extension ( Wai et al., 2018 ). To meet the essential requirements for home systems, the cost was reduced while maintaining the effectiveness, the set-up process is easy and fast—taking less than one minute—and compactness and aesthetics were practiced carefully during the design process. A universal and ambidextrous grip is utilized to allow both left- and right-hand usage and to be adjustable to different hand sizes without changing the attachment. Both active and passive motion assistance in a game context is provided based on the ability of the user. Also, automatic customization is allowed by receiving user information from a mobile interfacethrough an integrated internet of things (IoT) network to monitor and communicate with the therapist. Ambidexter is lighter and smaller compared to its commercial counterparts, ReachMan and ReachMan2.
A novel home-based End-effector-based Cable-articulated Parallel Robot, PACER—Parallel articulated-cable exercise robot—, was developed by Alamdari et al. for post-stroke rehabilitation ( Alamdari and Krovi, 2015 ). The device features a modular and reconfigurable design and is easy to assemble/disassemble. The device is able to assist and train the muscles involved in arm, forearm, and wrist motions. In 2018, Díaz et al. (2018) conducted research on converting a large pneumatic commercialized device, which had been designed for clinical therapy, into an electric and compact system for home rehabilitation by making it smaller, lighter, and cheaper, but maintaining the functionality ( Díaz et al., 2018 ). It resulted in the development of HomeRehab, a desktop-type 2DOF robotic system intended to improve ROM and strength of the paretic hand in stroke patients. Designed for the home environment, all the components are concentrated in a small portable box, and the device can easily be placed on a home table. VR and gamification are also enabled through a standard PC communication and a novel low-cost force sensor. Force feedback allowed both assistive—the assist-as-needed approach—and resistive scenarios to be implemented during the therapy, depending on the therapist’s assigned task. Remote management is enabled by using wearable devices to record patient’s biosignals and providing a cloud-based communication system. A comparison between PupArm, a commercialized rehabilitation device for clinical settings, and HomeRehab indicated that, while offering similar outcomes, the latter is significantly lighter; 80 vs. 7 kg ( Catalan et al., 2018 ) ( Figure 2 ).
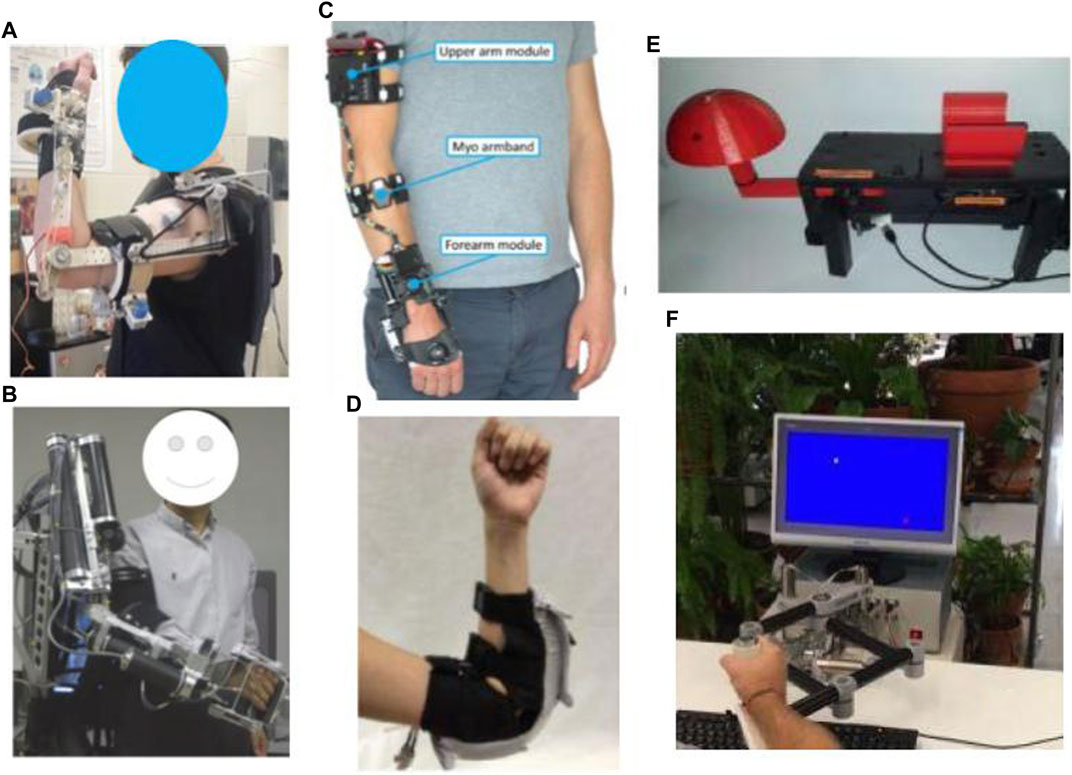
FIGURE 2 . Robotic exoskeleton and end-effector devices for home-based upper-limb rehabilitation: (A) SpringWear ( Chen and Lum, 2018 ), (B) RUPERT ( Tu et al., 2017 ), (C) eWrist ( Lambelet et al., 2020 ), (D) Soft robotic elbow sleeve ( Koh et al., 2017 ), (E) Portable device for wrist rehabilitation ( Ambar et al., 2017 ), and (F) HomeRehab ( Díaz et al., 2018 ).
ArmAssist (Tecnalia R&I, Spain) is a portable, modular, easy-to-use, low-cost robotic system consisting of a tabletop module using omni-wheels, an arm, and hand gravity compensator orthosis aimed at post-stroke shoulder and elbow rehabilitation. Also, at the University of Idaho, add-on modules for wrist prono-supination and hand grasping training have been presented. Over the years, the device has been iteratively redesigned based on the updated requirements gained from clinical interviews, expert focus groups, and pilot tests with patients and therapists ( Perry et al., 2012 ; Jung et al., 2013 ; Perry et al., 2016 ; Butler et al., 2017 ; Tomić et al., 2017 ).
As an intermediate step between high-power active and passive assistive robots, Westerveld et al. developed a low-power three-dimensional damper-driven end-point robotic manipulator, called active therapeutic device (ATD) ( Westerveld et al., 2014 ). ATD provides a combination of passive arm weight support and assistance for functional reaching training. Increasing its potential for home-based therapy, the device deployed an inherently safe and compact system design. Washabaugh et al. designed a planar passive rehabilitation robot, PaRRo, that is fully passive yet provides multi-directional functional resistance training for the upper-limb ( Washabaugh et al., 2019 ). This happens through integrating eddy current brakes with a portable mechanical layout that incorporates a large reachable workspace for a patient’s planar movements. The considered kinematic redundancies of the layout allow for posing direct resistance to the patients’ trajectories.
Commercially Available Devices
Based on the premise that the best way to reacquire the capability to perform a task is to practice that task repeatedly, Saebo Inc. proposed SaeboVR, a non-immersive VR rehabilitation system incorporating motivating games. These games are designed to simulate activities of daily living (ADL) and engage patient’s impaired arm in meaningful tasks aiming to evoke functional movements ( Recover From Your Stroke With Saebo, 2017 ). The goal of the customizable tasks is to test and train user’s cognitive and motor skills such as endurance, speed, range of motion, coordination, timing, and cognitive demand, e.g., visual-spatial planning, attention, or memory, under the supervision of a medical professional in a home setting. The device includes a Provider Dashboard application that enables the medical professional to view patient performance metrics and participation history while providing audiovisual feedback and graphic movement representation for patients.
To target a specific group of patients with various treatment options, this device can be upgraded with additional technologies, e.g., SaeboMas, SaeboRejoyce, or SaeboGlove, which can be integrated into the virtual environment. SaeboGlove, a functional hand orthosis, combined with electrical stimulation, has been shown to be beneficial for functional use of moderately to severely impaired hands in sub-acute stroke patients ( Franck et al., 2019 ). SaeboMAS, a zero-gravity upper extremity dynamic mobile arm support device, provides the necessary weight support in a customizable manner, facilitating exercise drills and functional tasks for the individuals who have arm weaknesses ( Runnalls et al., 2019 ). The usage of this technology will potentially allow patients with proximal weakness to reach a larger anterior workspace and engage in more versatile functional tasks and exercises that would have otherwise been difficult or impossible. The SaeboReJoyce is an upper extremity rehabilitation computerized workstation that includes pre-installed neurogaming software and offers task-oriented and customizable games. The workstation is composed of two components. A lightweight height-adjustable and portable gross motor component makes the device useful for sitting, standing, and lying positions and enables the execution of exercises and tasks in all directions and planes. A fine motor component aims at improving necessary dexterity for daily tasks by incorporating various grip and pinch patterns, such as spherical grasp, grip strength, wrist flexion/extension, tip to tip pinch, and pronation/supination, among others. Several studies have been conducted to prove the efficacy and results of Saebo products ( Adams et al., 2018 ; Doucet and Mettler, 2018 ; Runnalls et al., 2019 ).
Another home rehabilitation device for post-stroke patients is WeReha, which is intended to be used for hand impairments with the possible remote supervision of physiotherapists ( Bellomo et al., 2020 ). The primary purpose of this device’s invention is to train the fine movements of the hand and simultaneously stimulate the cognitive aspect, exploiting the biofeedback technology. It can track patient movements with the 3D printed “smart” objects equipped with inertial sensors while transmitting data via Bluetooth to the software. The software processes the data and produces visual-auditory feedback to guide the patient. Such guidance enables the patients to correctly execute various motor tasks through the exercises in the specially designed and studied video games that allow them to exercise the grip of finer or larger objects. Moreover, simple and effective gamification is based on indexes of rotation, flexion-extension, and prono-supination of the upper-limb.
The soft extra muscle (SEM) Glove by Bioservo Technologies AB, Sweden ( BIOSERVO, n.d. ), was developed to improve the hand’s grasping capacity by providing additional finger flexion strength. This novel technical solution simultaneously mimics a biological solution and functions in symbiosis with the biological system that excludes the need for an external mechanical structure to achieve controlling and strengthening effects. The servo device uses artificial tendons connected to electrical motors that actuate finger movements by creating pulling forces. The device features intention detection to apply proportional finger flexion strength facilitating grip or object manipulation, benefitting from control algorithms that are based on tactile sensor signals located on the tip of fingers. The efficacy of SEM Glove has been evaluated for improving gross and fine hand motor functions for at-home rehabilitation for people with impaired hand function after high-level spinal cord injury, yet further investigation is required for its efficiency for post-stroke rehabilitation ( Nilsson et al., 2012 ; Osuagwu et al., 2020 ). Based on the SEM Glove technology and with the aim of extending its application for stroke rehabilitation at home, Radder et al. designed IronHand, a lightweight and easy-to-use soft robotic glove that thanks to the soft and flexible materials used for its fabrication, accommodates wearable applications ( Radder et al., 2019 ). The IronHand, formerly known as HandinMind (HiM) project, offers an easy-to-use combination of assistive functionality during ADL and therapeutic functionality through a training context within a motivating game-like environment ( Prange-Lasonder et al., 2017 ). They allow individuals with reduced hand function to use their hand(s) during a large variety of functional activities. The therapeutic functionality of the device incorporates a therapeutic software platform for patient assessment and database and it covers therapy goals of simultaneous finger coordination, hand strength and sequential finger coordination ( Radder et al., 2018 ). The assistive functionality provides extra strength to the grip of fingers after the active contribution of the user’s grip force, and an intention detection logic ensures that extra force proportional to that of the user is activated. IronHand is one of the first user trials that applied and tested a fully wearable robotic system in an unsupervised home setting to support hand function during an extended period of multiple weeks. Findings from this extensive trial indicated improvements in unsupported handgrip strength and pinch strength ( Radder et al., 2019 ).
In an attempt to transfer Gloreha—Hand Rehabilitation Glove—Professional, a wearable hand rehabilitation hospital device, to a home setting, Gloreha Lite has been miniaturized and specifically designed for home use in a safe and feasible way for hand rehabilitation ( Bernocchi et al., 2018 ). Gloreha Lite is a portable, lightweight, and space-saving glove-brace ( Aggogeri et al., 2019 ). The robotic glove represents a relevant adjunct intervention to intensify activity-based therapy, integrating the principle of neuroplasticity with the intensity of treatment ( Proulx et al., 2020 ). It provides computer-controlled passive mobilization of the fingers. Before each exercise drill, a 3D-simulated preview of the movement is presented on the monitor, and during the performance of the movement, a simultaneous 3D simulation of the movement is displayed as it is being performed. Bernocchi et al. evaluated and demonstrated the feasibility and safety of the device for in-home therapy and indicated improvement of functional capacity of the paretic hand. They also demonstrated that the acquired benefits on strength and dexterity were maintained over time. The majority of patients completed the entire course of the program while performing all the prescribed home exercises.
The Motus Hand ( Motus Nova, n.d. ) ( https://motusnova.com/hand ), previously known as Hand Mentor Pro, is a portable robotic device designed to enhance active flexion and extension movements of wrist and fingers along with motor control of the distal upper limb. The device deploys pneumatic artificial muscles for simulating dorsal muscle contraction and relaxation. The Motus Hand has been classified as an FDA class 1 device presenting non-significant risk (NSR). Several clinical trials investigated and supported the clinical efficiency, feasibility, and user-friendliness of the Motus Hand for in-home telerehabilitation among subacute to chronic post-stroke ( Linder et al., 2013a ; Linder et al., 2013b ; Butler et al., 2014 ; Wolf et al., 2015 ) ( Figure 3 ).
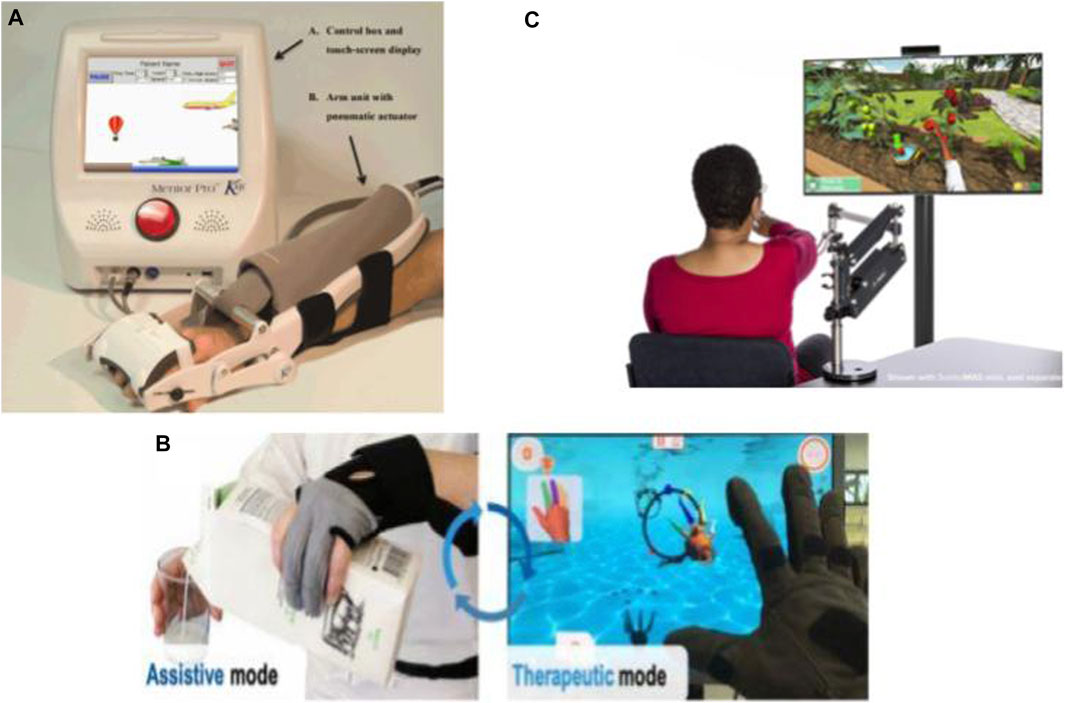
FIGURE 3 . Commercialized robotic devices for home-based upper-limb rehabilitation: (A) The Motus Hand ( Butler et al., 2014 ), (B) IronHand ( Radder et al., 2019 ), and (C) SaeboVR ( Recover From Your Stroke With Saebo 2017 ).
Hyakutake et al. investigated the efficiency and feasibility of home-based rehabilitation involving the single-joint hybrid assistive limb (HAL-SJ) ( Hyakutake et al., 2019 ). Drawing on the “interactive biofeedback” theory, HAL-SJ is a lightweight power-assisted exoskeleton on the elbow joint triggered by biofeedback for assisting the patient in the voluntary movements of the affected upper limb.
Many developed lower-limb robotic systems offer rehabilitation in sitting/lying positions for stroke patients who cannot stand or walk safely ( Eiammanussakul and Sangveraphunsiri, 2018 ). In this approach, the patients may exercise more independently with no safety concerns like falling. Therefore, compared to the other lower-limb rehabilitation principles, such as treadmill gait trainers, this kind of lower-limb device shows considerable potential for in-home therapy. Moreover, these robots are potentially suitable for home environments, as they can be smaller, lighter, and portable.
Spasticity of the limbs is one of the most common impairments ensuing onset of stroke. It puts patients at a high risk of developing foot deformity. Hence, treating the spasticity of lower extremities to prevent any deformity and facilitate ankle muscle activities during the acute phase and even after the long bedridden period is of utmost importance. In order to reduce or prevent the occurrence of spasticity at later stages, Low et al. developed a soft robotic sock, which can provide compliant actuation to simulate natural ankle movements in the early stage of stroke recovery. The soft robotic sock controls the internal pneumatic pressure of the soft extension actuators to assist the patient in ankle dorsiflexion and plantarflexion ( Low et al., 2018 ; Low et al., 2019 ). For the same purpose, Ren et al. also developed a wearable ankle rehabilitation robotic device capable of delivering in-bed stroke rehabilitation in three training modes, active assistive training, resistance training, and passive stretching ( Ren et al., 2017 ). These aforementioned devices mainly target people with acute stroke; Nonetheless, to treat chronic stroke survivors who have already developed ankle-foot deformities or imbalanced ankle muscles, Lee et al. developed a relatively small, lightweight, and user-friendly rehabilitative system, called Motorized Ankle Stretcher (MAS) ( Beom-Chan et al., 2017 ; Yoo et al., 2019 ). The MAS consists of two linear in-line actuators, each connected to a platform for generating ankle dorsiflexion and eversion. The system requires patients to perform exercises in the standing position, with a walker positioned in front of them for safety concerns. The above-mentioned devices have not yet been implemented in home-setting but are deemed potentially feasible by the authors in terms of safety, size, portability, complexity, and user-friendliness. However, the clinical efficiency of these devices for in-home treatment requires further investigation.
As one of the first home-based EMG-controlled systems, Lyu et al. (2019) developed a knee exoskeleton within a game context to be used while the subject was seated on the chair wearing the exoskeleton. Utilizing four active DOF at both hip and knee, the exoskeleton improves knee joint movement stability and accuracy by strengthening anti-gravity knee extensor muscles. The robot can be generally considered a successful effort at designing a home-based system by being adjustable to the wearer’s leg length, with a quick setup time of approximately 1 min, and considering safety cautions in all stages of software, electrical, and mechanical. Initial testing on healthy subjects represented promising results on the possibility of carrying out early rehabilitation by this device, the amount of muscle activation by the participants, and the timing of that activation.
Another example of lower-limb devices is a multi-posture electric wheelchair developed by Chen et al. with a lower-limb training function. Combined with virtual reality games and a tele-doctor–patient interaction, this device forms an intelligent rehabilitation system suitable for home therapy ( Chen S. et al., 2017 ). Apart from rehabilitation training, it can be used as an everyday wheelchair. This hybrid nature of the design has made it economically efficient. The wheelchair is equipped with four linear motors to carry out the training function and the lying/standing process, and is controlled by a cell phone interface. The system also benefits from a communication platform through web-based interfaces for patients and doctors. As another genre of lower-limb rehabilitation robots seemingly viable for home implementation, a sitting/lying Lower Limb Rehabilitation Robot (LLR-Ro) was developed containing a moveable seat and bilaterally symmetrical right and left leg mechanism modules, each comprising the hip, knee, and ankle joints. This device benefits from mechanical, electrical, and software safety features and an amendment impedance control strategy to realize good compliance ( Feng et al., 2017 ) ( Figure 4 ).
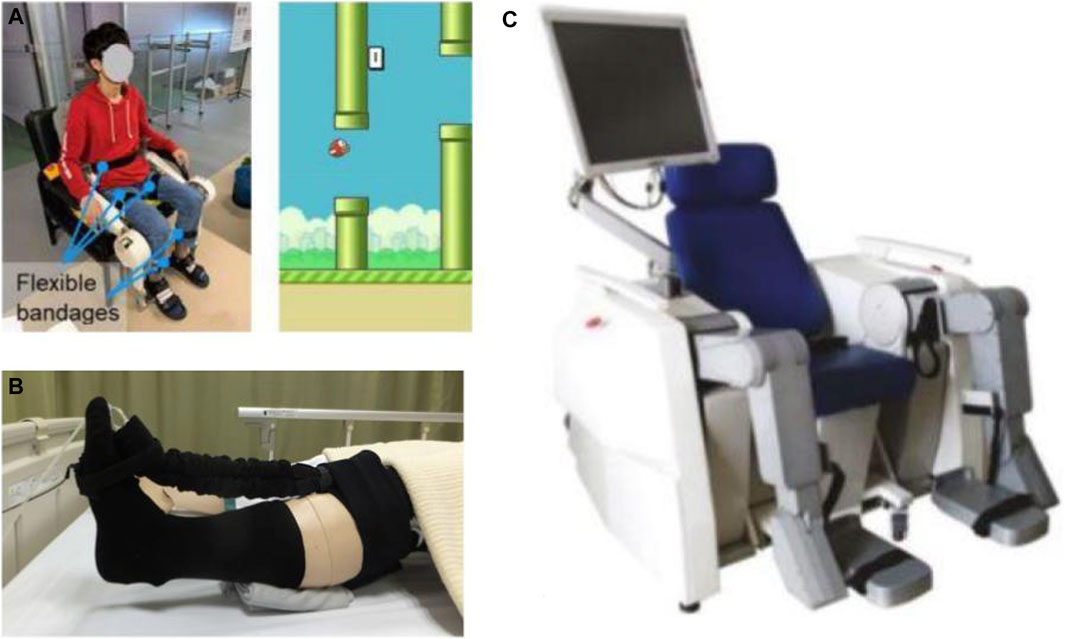
FIGURE 4 . Robotic devices for sitting/lying home-based lower-limb rehabilitation: (A) EMG-Controlled Knee Exoskeleton ( Lyu et al., 2019 ), (B) Soft Robotic Sock ( Low et al., 2019 ), and (C) LLR-Ro ( Feng et al., 2017 ).
One of the most prevalent lower-limb impairments following hemiplegia in post-stroke patients is asymmetric gait patterns and balance dysfunction. These impairments can adversely affect the quality of life as they lead to compensatory movement patterns, slowed gait speed, limited functional mobility, which results in reduced performance of the activities of daily living and increased risk of experiencing falls. Therefore, regaining autonomous gait and improving independent walking ability should be among the top priorities of rehabilitation interventions post-stroke. Aimed at improving stability and walking capacity, Morone et al. introduced i-Walker, a robotic walker for overground training with embedded intelligence that provides asymmetrical assistance as needed by detecting the imposed force by the user to adjust the amount of help to the impaired side ( Morone et al., 2016 ).
Developing wearable robots for lower-limb treatments has increasingly gained traction for their capability to facilitate ambulatory rehabilitation delivery. Among them, Gait Exercise Assist Robot (GEAR), proposed by Hirano et al. in collaboration with Toyota Motor Corporation, is a wearable knee-ankle-foot robot (only for the paralyzed leg) integrated with a low floor treadmill and a safety suspending device ( Hirano et al., 2017 ; Tomida et al., 2019 ). The Lokomat (Hocoma AG, Volketswil, Switzerland) is a commercial widely used exoskeleton-type robot for gait training worn over both lower extremities, consisting of a combination of adjustable orthoses, a dynamic bodyweight support system, and virtual reality for providing sensory-motor stimulation ( van Kammen et al., 2017 ). Although the clinical efficacy of wearable devices for lower extremity is supported by a growing body of evidence ( Hidler et al., 2009 ; Tomida et al., 2019 ; Mehrholz et al., 2020 ), only a number of them are realizable in a home setting with modifications addressing various factors, e.g., safety issues, size, weight, portability, complexity, and cost. Among robotic rehabilitation devices of the past ten years, those provisioned for home therapy only requiring further investigation validating their clinical efficiency at home are presented.
Walking Assist for Hemiplegia (WA-H) is a portable, lightweight, modular, and wearable exoskeletal robot supporting the hip and knee joint movements that, by providing customized gait training, can be used in various environments depending on the degree of impairment in patients. WA-H has an inherently safe design in which all robot joints mechanically limit the movements occurring beyond the natural range of motion. The device features a passive joint simulating the weight shift occurring during walking in the hip joint in the coronal plane ( Moon et al., 2017 ; Sung et al., 2017 ).
As both rehabilitation and welfare robot, Curara ® is a wear robot that assists hip and knee joints in both impaired and unaffected legs simultaneously with no rigid connection between joint frames, resulting in a higher degree of freedom. Prioritizing user-friendliness in terms of ease in don/doffing and minimizing the restraining stress against the natural human movement, Mizukami et al. adopted a non-exoskeletal structure coupled with a synchronization-based control system, introducing the ability to feel what natural movement would be like. Due to the absence of any rigid connection between joint frames, the device provides a high degree of freedom for patient movement ( Tsukahara and Hashimoto, 2016 ; Tsukahara et al., 2017 ; Mizukami et al., 2018 ). Lee et al. developed a smart wearable hip-assist robot for restoring the locomotor function, the Gait Enhancing and Motivating System (GEMS, Samsung Advanced Institute of Technology, Suwon, South Korea). GEMS is equipped with an assist-as-needed algorithm for delivering active-assistance in hip extension and flexion ( Lee et al., 2019 ; Lee et al., 2020 ) ( Figure 5 ).
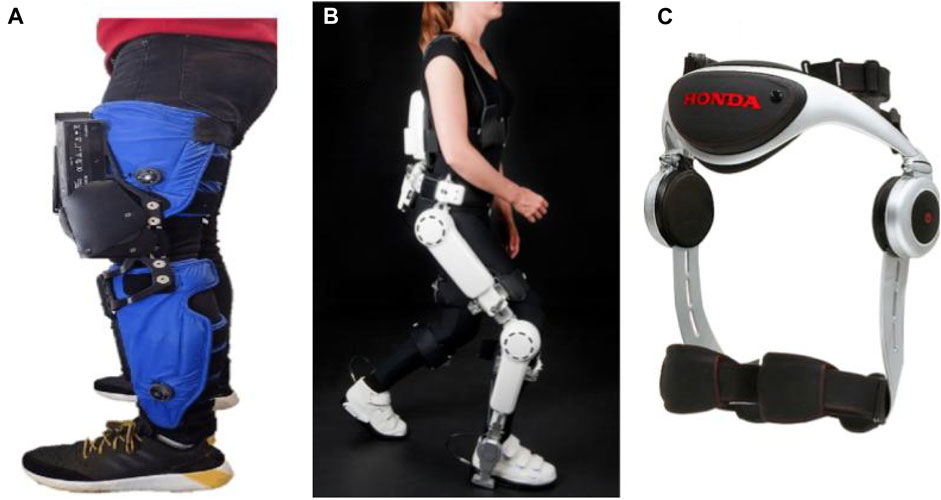
FIGURE 5 . Robotic devices for in-home walking training: (A) AlterG Bionic Leg ( Wright et al., 2020 ), (B) HAL lower-limb exoskeleton (Anneli Nilsson et al., 2014 ), and (C) SMA ( Buesing et al., 2015 ).
Interposed between active and passive training robots, Washabaugh et al. proposed their eddy current braking device on a knee brace as a wearable passive alternative that provides functional resisted gait training while adhering to features required for home-based devices ( Washabaugh et al., 2016 ; Washabaugh and Krishnan, 2018 ).
There are several commercially available lower-extremity rehabilitation robots, and those exhibiting potential for home therapy are presented. The wear overground robotic Stride Management Assist (SMA ® ) is developed and available for purchase ( Honda Global, 2020 ) by Honda that assists hip joint movements for increasing walking performance independence ( Buesing et al., 2015 ). The ReWalk ReStore™ is a soft exosuit designed and introduced by ReWalk (2019) for actively assisting paretic ankle plantarflexion and dorsiflexion for the propulsion and ground clearance walking subtasks ( Awad et al., 2020 ). ReWalk Robotics also proposed the ReWalk Personal 6.0 System as a customizable exoskeleton with motors at the hip and knee joints specifically designed for all day home-use ( ReWalk, 2015 ). EksoNR is an FDA-cleared ambulatory exoskeleton featuring adaptive gait training and posture support, yet it is currently being tested in a clinical trial with stroke patients for evaluating its clinical efficiency ( Carlan and singleorigin, 2020 ) ( https://eksobionics.com/eksonr/ ).
Different exoskeletons have been developed based on the HAL’s technology to offer active motion support systems with a hybrid control algorithm, Cybernic Voluntary Control for providing physical support associated with the patients’ voluntary muscles activity and Cybernic Autonomous Control that utilizes characterized movements of healthy subjects and adopts the motion patterns in accordance. One such device has been described in the prior section for elbow rehabilitation. Kawamoto et al. also, based on this technology, developed the single-leg version of the HAL, an exoskeleton-based robotic suit for independent supporting of the ankle, knee and hip joints ( Kawamoto et al., 2009 ). Kawamoto et al. and then Nilsson et al. investigated the efficiency of this exoskeleton for intensive gait training for chronic and acute, respectively, hemiparetic patients ( Kawamoto et al., 2013 ; Nilsson et al., 2014 ). The device is commercially available in Japan ( Cyberoyne, 2021 ).
The AlterG Bionic Leg ( AlterG, 2015 ; AlterG, n.d ), a portable dynamic battery-operated over-ground wearable lower extremity orthosis, provides adjustable progressive mobility training by supplementing existing muscle strength, providing audio-sensory feedback and mobility assistance during rehabilitation ( Stein et al., 2014 ; Iida et al., 2017 ; Wright et al., 2020 ). This dynamic orthosis supports knee mobility when standing or walking by providing external support to the lower extremity and assisting the patient in aid of weight shifts and knee movement and can be worn in a manner similar to an orthopedic knee brace.
Design Paradigm
The interdisciplinary field of rehabilitation requires the simultaneous employment of a range of expertise, including engineering, medicine, occupational therapy, and neuroscience, especially due to the lack of enriched research in motor learning principles for optimized post-stroke motor recovery ( Krakauer, 2006 ; Brewer et al., 2007 ). A successful home rehabilitation device can be designed within a certain sequence of steps that incorporate all of the aforementioned expertise, as skipping one could prevent achieving optimum outcomes.
Mechatronic home-based systems for post-stroke therapy are based on four basic components: 1) a mechatronic device delivering rehabilitation intervention, 2) a control system ensuring proper performance of the system, 3) interactive interfaces for patients and medical professionals who provide remotely supervised therapy, 4) a communication system gluing the whole system together.
To provide a classified roadmap for assisting researchers who aim at further developing this field, a design paradigm is proposed to form a guideline on developing each component based on the engineering design process.
Post-Stroke Rehabilitation and Treatment Interventions
Among different post-stroke symptoms, motor deficits are the most commonly recognized impairments that affect the face, arm, and leg motor functions. These impairments result in various manifestations, including impaired motor control, muscle weakness or contracture, changes in muscle tone, joint laxity, spasticity, increased reflexes, loss of coordination, and apraxia ( Basteris et al., 2014 ; Hatem et al., 2016 ). To recover lost function and aid motor recovery, many rehabilitation interventions have been developed based on neurorehabilitation principles ( Basteris et al., 2014 ). So as to develop a robot-assisted therapy that offers maximal motor function recovery, it is essential to employ interdisciplinary research on the broad spectrum of post-stroke disabilities and their corresponding rehabilitation protocols. The outcomes could help create a clear picture of the target users, establish target and rehabilitation program specifications, and derive requirements from the needs of all stakeholders, the largest being the therapists and the patients. Note that it is essential for the device to be accepted by medical professionals. From the therapists’ point of view, the crucial features for iterative design and modification of each rehabilitation robot must be surveyed and practiced carefully.
Engineers should develop the rehabilitation system based on multiple contributing factors, including the part of the limb being trained, the targeted stage of recovery, the severity of initial motor deficit, range of movements in the paretic limb, grade of spasticity, age, and individual patient’s characteristics. Depending on the patient, it is known that motor impairments can induce disabilities in several functions, such as range of motion, speed, coordination, cadence (steps/minute), balance, precision, the ability to regulate forces, muscle strength, and energy efficiency ( Perry et al., 2011 ). Physiological measurements during rehabilitation, i.e., heart rate, blood pressure, body temperature, etc., assist in sensing the patient’s status during therapy and their capability to do exercise, and in turn, offer a foundation for determining the dosage of assigned tasks based on one’s capability ( Solanki et al., 2020 ). Monitoring physiological parameters could also be utilized for detecting the user’s psychological state, in terms of mood, motivation, engagement, etc., and lead to modification of the course of therapy accordingly ( Novak et al., 2010 ). It is important to tailor treatment strategies to the goals of improving one or a combination of these functional disabilities. Current robotic rehabilitation systems incorporate a variety of neurorehabilitation strategies. These strategies include constraint-induced movement therapy (CIMT), repetitive movement training, impairment-oriented training, explicit learning paradigms such as bilateral training, implicit training, and functional task paradigms ( Brewer et al., 2007 ). Given training goals as guidelines, a combination of these interventions can be utilized in order to develop a reasonable user-specific program with respect to the aforementioned critical factors ( Hatem et al., 2016 ), as different types of tasks are necessary to retrain all lost function.
Recovery would benefit if scientific principles behind post-stroke motor learning were incorporated into the design of the rehabilitation device. Under the assumption that performance improvement is dependent on the amount of practice, most current mechatronic devices for post-stroke therapy are solely based on the repetition of a single task, termed “massed practice” ( Brewer et al., 2007 ). It is important to note that, although repetition is the key to improving within-session performance, other critical factors must be considered while scheduling rehabilitation protocols. As Krakour et al. state, there are two crucial questions to ask before developing any rehabilitation system: “whether gains persist for a significant period after training and whether they generalize to untrained tasks” ( Krakauer, 2006 ; Kitago and Krakauer, 2013 ). There is evidence that “distributed practice,” which means inserting more extended rest periods between repetitions, e.g., increasing the number of sessions while decreasing session duration, promotes retention ( Krakauer, 2006 ; Kitago and Krakauer, 2013 ). While limitations of clinical therapy preclude the proper implementation of this method, home-based therapy provides the opportunity of distributed task scheduling in a way that it can always be at the patients’ disposal.
For rehabilitation interventions to be meaningful, learned tasks must generalize to new tasks or contexts, especially real-world tasks. Introducing variability to training sessions, though worsening the patients’ performance in the short term, improves their performance in retention sessions and also increases generalization by representing each task as a problem to be solved rather than just memorized and repeated ( Krakauer, 2006 ; Kitago and Krakauer, 2013 ). Contextual interference is a concept used to introduce variability to the task by random ordering between several existing tasks. Moreover, recovery of function to increase patient autonomy is another important aspect of rehabilitation. It seeks to consider training for true recovery, as well as, compensatory mechanisms—respectively accomplishing task goals by recruiting the affected muscles or alternative muscles ( Krakauer, 2006 ; Kitago and Krakauer, 2013 ). Nevertheless, when establishing goals for rehabilitation interventions, there has to be a clear distinction in mind between these two, true or compensatory recovery, as they may make differential contributions to the treatment plan.
Mechatronic System
Once the target group and treatment plan have been identified, the design criteria, including requirements and constraints, need to be established and prioritized to fit the need. Since the device is being designed for the home setting, certain factors are introduced, and some others become more prominent—safety, adaptability to the home setting, the autonomy of patients, aesthetic appeal, affordability, to name a few ( Carbone et al., 2018 ; Chen et al., 2019 ).
Design Criteria for Home-Use
In addition to the general criteria, the adoption of each home rehabilitation solution requests specific features of the device itself. For example, in the case of exoskeletons, besides absolute safety when worn, lightness, wearing ease, comfortability, and smoothness, there should be an absence of friction and allergenic factors as it is in contact with the skin. So the device should guarantee a high tunability and reliability ( Borboni et al., 2016 ). Considering all of the factors and criteria, a solution has to be adopted, which offers a satisfactory compromise to each of the existing issues and requests of all parties involved, as all of the requirements affect the device’s structure. Also, there are some optional and preferable criteria, among which expandability and upgradability are favorable to cover a wide range of disabilities and possible treatment methods. To this end, modularization benefits both manufacturers and customers for it increases diversity and a variety of available options and enables interchangeability and compatibility. This way, various gadgets could be developed to be integrated into a wide range of existing home-based devices, which in turn enhances both acceptability and functionality. For example, Amirabdollahian et al. in the SCRIPT project and Kutlu et al. in their home-based FES rehabilitation system utilized commercialized Saebo module, SaeboMAS arm support ( Amirabdollahian et al., 2014 ; Kutlu et al., 2017 ). Moreover, the donning aspect of robotic devices in an unsupervised context should be underlined. Therefore, attachment mechanisms should be designed to enable facilitated and independent donning and doffing of the device by the patient. In this regard, Lambelet et al. designed the eWrist to offer easy and fast donning/doffing to enable single-handed mounting of the device for hemiparetic patients, significantly promoting the autonomy of the patient were they to train independently ( Lambelet et al., 2020 ). Equivalently, Fischer et al. realized facilitated donning of X-Glove by means of adopting a two-separate component design, a zipper on the palmar side, and a flexed wrist posture ( Fischer et al., 2016 ). It should be flexible to be used in different positions, such as sitting/lying for bedridden or chaired individuals, and also light enough to be easily transportable. Another criterion that needs to be taken into account is that the device’s functioning noise must be as low as possible to be acceptable by the patient ( Borboni et al., 2016 ).
Mechanism Type
Having design criteria and target functionality in mind, the designer has to decide the mechanism type. The type of the mechanism and the treatment options are correlated; for example, additional movement protocols can be utilized based on the number of arms. In general, human limb rehabilitation robots are divided into two groups based on the target limbs: upper-limb rehabilitation devices and lower-limb rehabilitation devices, each divided into several subgroups. Based on motion systems, upper-limbs are categorized into exoskeletons or end-effector devices. Based on the patient’s posture, lower-limb devices can be designed to be used in sitting/lying positions or standing positions with the help of body and robot weight support.
In his study in 2019, Aggogeri et al. categorize robotic rehabilitation technologies into end-effector or exoskeleton devices based on design concepts ( Aggogeri et al., 2019 ). End-effector devices, also known as endpoint control, determine the joint level movements by recreating dynamic environments corresponding to ADL. End-effector devices may be dedicated to hand rehabilitation or integrated into more complex structures for arm recovery. Attached to the user’s limbs, exoskeletons are wearable robots aiming at enhancing their movements. Focusing on the patient’s anatomy, each degree of freedom of the device is aligned with the corresponding human joint. Exoskeletons should be compliant with the user’s movements and deliver part of the power required by the movements. Therefore, the mechanical axes of exoskeleton joints and anatomical joints should be aligned to prevent patient discomfort and not obstruct natural limb movement ( Fischer et al., 2016 ). In designing exoskeletons, the high sensitivity of stroke survivors to the applied mechanical load on their paretic limb makes the weight of the device an important factor to be considered. Reducing the applied load on the impaired limb can be achieved through locating the components not directly involved in actuation—e.g., battery and controller—on more proximal rather than distal body parts. For example, Fischer et al. provided an upper arm module for locating the battery and electronics, resulting in the reduced weight of the forearm module located on the distal part of the arm ( Fischer et al., 2016 ). Equivalently, in the ReWalk Restore lower-limb exosuit, the actuation pack is worn at the waist so that the larger proportion of device weight is located proximally ( Awad et al., 2020 ).
Comparing these two different approaches, end-effector robots are more flexible than exoskeleton devices in fitting the different sizes, require less setup time, and increase the usability for new patients. Besides, end-effector mechanisms are also generally ambidextrous. On the contrary, exoskeletons should be fully user-adjustable and therefore require more complex control systems. While both distal and proximal joints are constrained in exoskeleton devices, end-effector robots merely constrain the distal joints ( Aubin et al., 2013 ). Therefore, explicit control of each individual joint is only possible with exoskeleton devices. The limited control of end-effector robots could result in abnormal movement patterns in patients. In contrast, due to the direct controllability of individual joints in exoskeletons, these abnormal postures or movements are minimized ( Aggogeri et al., 2019 ).
In conventional exoskeleton mechanisms, the rigidity of the frames and fixed straps poses an issue on their wearability and usability. The heaviness and bulkiness of such frames result in high energy cost and also affects the natural gait dynamic and kinematics of the patient. Hence, soft orthotic systems have been developed as an alternative to traditional rigid exoskeletons ( Lee et al., 2019 ). In this regard, soft robots have shown promising potential to be adopted for at-home rehabilitation. In their study, Polygerinos et al. argue that soft wearables could further advance home-based rehabilitation in that they provide safer human-robot interaction due to the use of soft and compliant materials, a larger range of motion and degrees of freedom, and increased portability. The materials used for the fabrication of these robots are inexpensive, making these devices affordable. Also, soft material makes these devices inherently lighter and, therefore, more suitable for rehabilitation purposes. Another advantage of soft robotic devices over conventional rehabilitation robots is that they can be fully adapted to the patient’s anatomy offering a more customizable actuation ( Polygerinos et al., 2015 ).
In rehabilitation devices, it is essential to improve physical human-robot interaction (pHRI). For each type of rehabilitation device, the recruitment of different engineering methods is required for such improvements. This interaction is fundamentally affected by the mechanisms that should be designed by taking sophisticated biological features and activities into account. By considering the compliance/stiffness factor, modes of actuation and transmission need to be selected in a systematic way. Control methods also affect pHRI ( Gull et al., 2020 ).
Degrees of Freedom
The number of active and passive DOFs determines the system’s functionality. They condition the workspace in which the joints are capable of moving, indicating the assistance/rehabilitation, which is needed to be delivered to each joint ( Shen et al., 2020 ). Patients’ anatomy should be incorporated into the design when determining a reachable workspace based on anthropometric norms of the end-user ( Washabaugh et al., 2019 ). By reviews on upper and lower-limb devices, it can be figured out that the majority of the developed devices profit from certain degrees of freedom that are compatible with the human body’s anatomy. Anatomically speaking, in the upper extremity, often simplified to have seven degrees of freedom, the shoulder is simplified as three rotary joints achieving extension/flexion, adduction/abduction, and internal/external rotation. Elbow and forearm are simplified to provide extension/flexion and pronation/supination movements, respectively. Lastly, the wrist achieves extension/flexion and radial/ulnar deviations. The seventh DOF in the upper extremity’s joint space poses challenging complications since the maximum DOFs in the task space is six. Not only does this require us to have a firm understanding of how a human resolves this redundancy issue, but also such understanding must be taken into consideration when designing rehabilitation device mechanisms ( Shen et al., 2020 ). Furthermore, fingers are simplified as joints capable of achieving flexion/extension and abduction/adduction movements.
In the lower extremity, the hip is simplified as three rotary joints to achieve flexion/extension, abduction/extension, and internal/external rotation. The knee achieves pure sagittal rotation and flexion/extension. And finally, the ankle, simplified into three rotation joints, achieves plantar/dorsiflexion, eversion/inversion, and internal/external rotation ( Shi et al., 2019 ). The kinematic models should be developed by considering the anthropometric and morphology of human body structure in accordance with the command-and-control possibilities of actuated joints ( Dumitru et al., 2018 ; Cardona and Garcia Cena, 2019 ). Joint movements during upper and lower-limb movements cause misalignments between the exoskeletal and human joint axes that need to be adjusted and fit to the position of joints. Some exoskeletal devices have addressed this issue by providing passive joints to trace the joint movements to enable of the wearer’s natural range of motion. For example, Sung et al. equipped WA-H with passive hip joints in the coronal plane to enable weight shifting during walking ( Sung et al., 2017 ). Also, Liu et al. incorporated three passive shoulder joints in their design to minimize the misalignments ( Liu et al., 2021 ).
Modeling Tools
Modeling tools are of paramount importance in the design of rehabilitation devices for both robot and musculoskeletal modeling. Rigid body simulation programs or general-purpose simulation software such as Adams, Matlab, and Modelica can be used to evaluate the mechanics and control aspects. Also, computer-aided design software, such as CATIA and SOLIDWORKS, could be used to design, simulate, and analyze these robotic mechanisms. To simulate and control soft robots, SOFA, an open-source framework, can be used. It provides an interactive simulation of the mechanical behavior of the robot and its interactive control. It is also possible to model a robot’s environment to be able to simulate their mechanical interaction.
On the other hand, the heavy dependence of design parameters upon the targeted application requires careful analysis of the human body anatomy to design the device by considering the end-user application ( Gull et al., 2020 ). In turn, programs such as OpenSim and AnyBody can evaluate and predict the effect of the device on the human musculoskeletal system for any given motion. For example, in order to generate a digital exoskeleton model, Bai et al. exported the designed exoskeleton in CAD, SolidWorks, to AnyBody ( Bai and Rasmussen, 2011 ). When it comes to musculoskeletal systems, software tools can significantly facilitate the process of derivation of the motion equations to model muscle force and path. Various musculoskeletal software packages are commercially available such as SIMM, OpenSim, AnyBody, and MSMS. Among these software packages, OpenSim is free and open-source software, and MSMS is a free software ( Cardona and Garcia Cena, 2019 ). Muscle activation and muscular contraction dynamics of musculoskeletal models can also be used as a reference input signal for real-time controlling methods. For example, Liu et al. utilized an s-EMG-driven musculoskeletal model to adjust the stiffness control based on the patient’s physical status and assigned task requirements ( Liu et al., 2018 ).
Two commonly used dynamic modeling methods are Newton-Euler and Lagrange’s methods. In the Newton Euler method, by solving the Newton-Euler equation, the robot’s internal and external forces are extracted. In Lagrange’s method, which is based on the system’s energy, the external driving force/torque of the system can be calculated. In 2019 Zhang et al. drew a comparison between these two methods and provided a table to demonstrate the differences between these methods. Derivation analysis in the Newton-Euler method is more complicated than the Lagrange method, but calculations in the Newton-Euler method are large and heavier to compile, while in the Lagrange method, are easily compiled. In the Newton-Euler method, in addition to the driving force/moment, internal forces can be obtained ( Zhang et al., 2019 ). Dynamic simulation can be carried out in Adams environment while theoretical calculations are performed in MATLAB. Zhang et al. use these simulations to provide a basis for the optimal design of the structure and the selection of the motor ( Zhang et al., 2019 ).
Actuation and Transmission
The design of the device, further completed and integrated with the detailed design of chosen actuators, the transmission system, and the sensing system, should be presented at the next step. As there are myriad options to choose from, the designer has to weigh his/her options against the design criteria and their allocated importance and priority.
Efficient actuator design is important for home-based rehabilitation systems since these systems should be compact. Therefore, as the main powering elements, small-sized actuators that have a high power-to-weight ratio are required as they are capable of producing high torques with precise movement ( Gull et al., 2020 ). Actuators should be chosen based on the target application. Three main categories of actuators are electric motors, hydraulic/pneumatic actuators, and linear actuators.
Electric motors are used for their quick responses and capability of providing high controllability and controlled precision. However, they have a low power-to-mass ratio and are usually expensive. Pneumatic actuators can yield high torques but could save self-weight. Nevertheless, by using these types of actuators, the portability of the system is compromised due to the accompanying inherent components such as pump, regulators, valves, and reservoirs. Another factor that makes these types of actuators unsuitable for home-based systems is that they require maintenance since lubricant/oil leakages could be problematic for users. In hydraulic and pneumatic actuators, control is less precise, and hence the safety cannot be ensured. According to Shen et al. and Gul et al., these actuators are not suitable for providing assistance or therapy or for rehabilitation purposes due to their possessing high impedances; however, some studies utilize them because of their ability to provide high power. Ultimately, based on the studies done by Gull et al., Series Elastic Actuators (SEAs) by reducing inertia and user interface offer a safe pHRI and can achieve stable force control ( Gull et al., 2020 ). Tuning the stiffness of the transmission system is one of the approaches to achieve a specific level of compliance. In this regard, Jamwal et al. suggest using variable/adjustable stiffness actuators for rehabilitation purposes since these actuators offer safer human-robot interaction due to their ability to minimize large forces caused by shocks ( Jamwal et al., 2015 ). Especially, employing SEA in lower-limb robots offers the advantage of a facilitated control-based disturbance rejection by improving tolerance to mechanical shocks, e.g., resulting from foot-ground impacts ( Simonetti et al., 2018 ). According to Hussain et al., compliant actuators enable lightweight design with low endpoint impedance for wrist rehabilitation, while electromagnetic actuators are bulky and have high endpoint impedance ( Hussain et al., 2020 ). Also, based on the study done by Chen et al., in 2016, compliant actuators are regarded as safe and human-friendly. These actuators are preferable over stiff actuators for various reasons. For example, such systems can deliver controlled force with back-drivability and low output impedance and are tolerant against shock and impacts. Chen et al. suggest using SEAs for assistive and rehabilitation robots ( Chen et al., 2016 ).
On the other hand, passive robots must be equipped with passive actuators to enable scalable resistance and assistance based on the patient’s mobility status. There are various types of passive actuators such as friction brakes, viscous dampers, and elastic springs to name a few ( Washabaugh et al., 2019 ).
Power transmission may be realized through the utilization of direct drive, gear, linkages, or cable-driven methods. Cable-driven transmission means allow for a more lightweight and compliant design. Backlash and transmission losses render the control of such systems challenging ( Fischer et al., 2016 ). Sanjuan et al. classify cable-driven transmission into two categories of open-ended cables and closed-loop cables ( Sanjuan et al., 2020 ). According to this study, open-ended cable systems exert forces in one direction, while close-ended cable systems exert friction forces ( Sanjuan et al., 2020 ).
In order to provide proper guidance for the device’s movement to execute the required tasks, the system should utilize sensing methods as input signals. Generally, four main sensors are used in rehabilitation devices, namely Motion and Position sensors, Force/Torque sensors, Electromyograms (EMG), and Electroencephalogram (EEG) ( Shen et al., 2020 ). Since the rehabilitation devices are directly in contact with the human body, they should be reliable and highly accurate to provide the control system with real-time feedback of moving components ( Zheng et al., 2005 ; Porciuncula et al., 2018 ).
The spatial configuration of the device is needed in order to analyze its kinematics and dynamics. For this purpose, position sensors are used to measure and indicate this spatial configuration. Among the sensors used for this purpose are: encoders, potentiometers, flex sensors, and transducers. For haptic applications like rehabilitation in VR, force/torque sensors are required. Usually, extra force/torque sensors are added to the system to provide additional safety levels. Gyro and acceleration sensors can be mounted on the mechanical structure for measuring the patient’s posture, for example, in HAL ( Kawamoto et al., 2013 ).
Moreover, EMG and EEG could be used for measurements in noninvasive ways. The received signals in these two methods are often noisy and thus require further processing. Also, sensor fusion, in which the data from multiple sensors are merged, could provide a safer and more stable intention detection for the system.
Safety Measures
Last but not least, in the unsupervised context of home-based rehabilitation, safety is the most crucial criterion to be considered. Multiple redundant safety features must be incorporated in different modules of the device. In designing the mechanism stage, the device could be designed to offer intrinsic mechanical safety so as not to transfer excessive force to the patient’s body. Also, abnormal reactions of the patient should not be transferred to the actuator. For example, in Gloreha, these eventual reactions are absorbed by the mechanical transmission ( Borboni et al., 2016 ). In “Design Issues for an Inherently Safe Robotic Rehabilitation Device,” Carbone et al. maintain that there are three major aspects that significantly affect the safety of the rehabilitation device, namely operation ranges, operation modes (speed, accelerations, paths), and operation force/torque ( Carbone et al., 2018 ). Other measures could be taken to ensure safety; for example, Kim et al. utilized emergency stop buttons for both the user and the operator, hard stops at every joint, and also at the software level added safety features limiting the ROM and joint velocity and stopping the robot in case of excessive force/torque interaction ( Kim and Deshpande, 2017 ). Feng et al. designed LLR-Ro to tackle the safety issue in three levels, i.e., mechanical limit, the electrical limit, and the software protection, to fully guarantee the limb safety of the patient in the whole training process ( Feng et al., 2017 ). The device took advantage of inherent mechanical joint range of motion limits and limit switches placed on joint extreme positions to effectuate electrical limits and realizes the software protection according to the recorded patient information. Biomechanical, physiological, and even psychological information of patients can be utilized at software level safety checks to limit forces, motion, speed, and user adjustments to control parameters ( Van der Loos and Reinkensmeyer, 2008 ).
Ultimately, at the end of each step, following the suit of the engineering design process, iterative optimization is needed to redefine the design process based on the predefined criteria. All of these design steps are highly interconnected and, therefore, should be considered from different aspects ( Kruif et al., 2017 ). The HERO Glove was iteratively modified and redesigned based on evaluations and feedbacks from occupational therapists, specialized in stroke therapy, engineers, and stroke survivors ( Yurkewich et al., 2019 ).
Control System
After considering the mechatronic aspects, the control system has to be developed to ensure the proper behavior of the device ( Desplenter et al., 2020 ). From the hierarchy point of view, it is suggested to group the control system into three levels, mission planning, trajectory planning, and state space control, where the state space control can itself be in two levels of supervisory control and low-level control. The mission planning level is responsible for task assignment and completion. Task assignment includes enabling the therapist or task planning algorithms on the therapy control unit to customize task details such as treatment strategy, initial angles and positions, movement period and dwell, movement velocities, motion patterns, number of repetitions, range of motion, to name a few. At this level, IoT technology can be utilized to provide proper feedback from the treatment and identification of muscloskeletal parameters for the therapist, as well as therapist supervision. To this end, a mission planning module must be developed proposing a wide variety of options applicable to the mechatronic design; including multiple modalities and training protocols can increase the chance of adoption by medical professionals ( Desplenter and Trejos, 2020 ). According to the specified task, the trajectory planning level plans the kinematic and kinetic parameters of the motion to meet the objectives of the planned mission. For example, if the robot is supposed to provide a motion from an initial to a final configuration, the trajectory planning level designs the detailed variation of position, velocity and acceleration of the joints at each moment. If a force control mission is given, the force trajectory to be given to the control level is designed in the trajectory planning level. In the last level, the planned mechanical motion has to be implemented via the state space control level. Since the robot dynamic equations are normally nonlinear with unknown parameters and considerable uncertainties, a high-level supervisory control may be required to adaptively tune the control parameters recursively. This requirement can be handled easily by fuzzy logic or neural network schemes ( Lin and Lee, 1991 ; Bai et al., 2018 ). The low-level control can then be a model-based control, where the computed torque method (CTM) is one of the most effective and convenient method for this level ( Yıldırım, 2008 ).
During the last two decades, many solutions have been proposed to provide motion tasks suitable for post-stroke motor recovery. Priotteti et al. categorized existing global strategies for robotic-mediated rehabilitation into three groups of assistive, corrective, and resistive controllers ( Proietti et al., 2016 ).
Passive assistance, where the device moves a muscle rigidly along the desired path by adopting different techniques, is the most common treatment strategy for acute patients due to the unresponsiveness of the paretic limb at early post-stroke stages. As soon as patients regain some degrees of mobilization, switching to other assistive solutions, such as triggered passive, where the patient initiates the assistance, or partial assistance, where the device assists the patient as needed (AAN), as utilized by Díaz et al. in HomoRehab ( Díaz et al., 2018 ), may lead to better functional outcomes. Wai et al. provided a combination of the two mentioned assistive strategies in Ambidexter ( Wai et al., 2018 ). Active initiation and execution of movements are the cornerstones of these strategies due to their proven effectiveness in stimulating neuroplasticity to enhance functional therapy.
The corrective mode is linked to the rehabilitation situation in which the patient is not performing the movement correctly, and the robot intervenes by forcing the impaired limb to the correct orthogonal direction. However, it is not easy to clearly differentiate assistive and corrective techniques. Hence, combined assistive-corrective controllers are mostly recurrent among existing controllers ( Proietti et al., 2016 ).
When patients have recovered enough motor capacity, undergoing resistive therapies, which make tasks more difficult in some ways, may result in more significant rehabilitation gains ( Ali et al., 2016 ). Added to the assistive controller, HomoRehab is also equipped with a resistive controller ( Díaz et al., 2018 ). Resistive control, also termed as “challenge-based control,” can be achieved by merely applying constant resistance to the movement or error-based methods such as error augmenting and error amplification strategies ( Ali et al., 2016 ). Existing devices mostly provide a combination of these three categories to offer a span variety of motion tasks adaptable to the patient’s degree of rehabilitation and the targeted impairment ( Proietti et al., 2016 ). The control system of HAL exoskeletons is based on a hybrid control algorithm, consisting of two subsystems; one for voluntary control according to the patient’s voluntary muscles activity and another for autonomous control, which provides predefined physical passive assistance when patients are not able to generate required voluntary signals ( Kawamoto et al., 2013 ). For asymmetrical gait rehabilitation, Curara ® employed a synchronization-based assistance control strategy using neural oscillators based on a central pattern generator network. The synchronization-based controller detects the user’s movement and measures the mutual gap between the user and the device, and then achieves a desired joint angle trajectory to be synchronized with human movements ( Mizukami et al., 2018 ). Ren et al. proposed three different control strategies in their in-bed wearable ankle rehabilitation robotic device, namely triggered passive assistance for the early stages of recovery, and partial assistance and resistance when the patient gained some mobility ( Ren et al., 2017 ). Their robot-guided rehabilitation protocol is capable of automatically switching between the control modes based on the patient situation and recovery status during the therapy.
Different control strategies can be realized by different methods and algorithms, such as position control, force control, force/position hybrid control, impedance/admittance control, or other control methods ( Zhang et al., 2018 ). Due to their ability to provide natural, comfortable, and safe interaction between robots and patients, impedance control and its dual admittance control are two of the most common control algorithms currently used for rehabilitation exoskeletons, mostly for active training approaches ( Proietti et al., 2016 ).
The difficult task of translating physiotherapist’s experience in manipulating the paretic limb into the desired trajectory for rehabilitative devices has made trajectory planning one of the issues for researchers in this field ( Proietti et al., 2016 ). Proietti et al. summarized three main methods for defining reference trajectories, teach/record-and play ( Pignolo et al., 2012 ), motion intention detection ( Lyu et al., 2019 ), and optimization algorithms ( Su et al., 2014 ).
Considering the type of human-machine interaction, control system inputs can be divided into bioelectric signals, e.g., EMG, and biomechanical signals, e.g., joint position, or a combination of these two types of signals ( Desplenter et al., 2020 ). Biomechanical signals provide an accurate and stable interaction, especially for patients with high-level impairment, while biosignals are used for their potential in intention detection and neurological clinical applications due to their favorable effects on nerve rehabilitation treatment ( Zhang et al., 2018 ).
Given the underlying significance of the control system in motor control recovery, some characteristics must be carefully practiced during design steps. Especially when designing for the home setting, providing an appropriately shared control between the device and the patient is of paramount importance. Such control enables a favorable autonomous therapy, including predictable behaviors for patients and never taking control when undesired by the user ( Beckerle et al., 2017 ). It is also required for addressing neurorehabilitation issues not to suppress or hinder patients’ motor capabilities ( Jarrassé et al., 2014 ).
Regarding the safety concerns of home therapy due to the absence of the therapist, highly adaptive control systems need to be developed to consider the differences of the human bodies, the motion tasks during the recovery process for each patient, and the nature of the injury between patients ( Desplenter and Trejos, 2020 ). The control solution has to show an adequate amount of active compliance to avoid hurting the patient in the case of trajectory errors due to abnormal or excessive muscle contraction, spasticity, or other pathological synergies ( Proietti et al., 2016 ). Impedance-based control schemes provide a dynamic relationship between force and position and could be utilized for adopting a safe, compliant, and flexible human-machine relationship. In this regard, LLR-Lo benefits from an amendment impedance control based on position control to realize motion compliance for patients in case of patient’s discomfort, which leads to aching movement of their leg while the mechanism leg is still moving ( Feng et al., 2017 ). Analyzing the kinesthetic biomechanical capabilities of the target limb is also necessary for developing safe human-robot interaction ( Atashzar et al., 2017 ). Compared to conventional therapy, relying on time or position-dependent trajectories can restrict rehabilitation therapy from the generalization of learning to new tasks. Thus, targeting goal-independent strategies would increase therapy’s efficacy, as patients are not constrained by following rigidly planned trajectories ( Proietti et al., 2016 ).
As control system development tools, there are software frameworks designed to provide developers with a platform for the design and implementation of the control system, (e.g., Tekin et al., 2009 ; Coevoet et al., 2017 ; Desplenter and Trejos, 2020 ). The use of such frameworks supports the idea of standardization across different studies and devices. This enables a more efficient evolution and comparison of control systems, reduces the current ambiguity in classification, and neuters existing poor attention in documenting the control strategy of developed devices ( Basteris et al., 2014 ), all assisting in identifying which strategy provides better results. WearMECS is a recently developed control software design and implementation tool available as an open-source software library. The framework provides a foundation for regulating the motion behavior of therapeutic mechatronic devices by considering three main control functionality groups, i.e., task-level control, estimation-level control, and actuation-level control ( Desplenter and Trejos, 2020 ).
User-Interfaces
The concept of remote supervision is entangled in home-rehabilitation. Developing a platform for tele-patient-doctor interaction is the key enabling factor for home-based therapy, in which the interaction of three parties of the patient, the therapist, and the device is provided ( Desplenter et al., 2019 ). Harmonious completion of the rehabilitation program relies on the interaction between each of these parties, which takes place through two main user interfaces of the therapist and the patient. Therapists need to have access to the interface to prescribe treatment regimens and monitor patient’s progress in a scheduled plan through detailed reports containing the qualitative and quantitative evaluation of exercises. Patients also would have access to the prescriptions in their interface and move forward through the exercises. In the interface, the correct execution of motions should be displayed ( Pereira et al., 2019 ). Before each exercise, visual and auditory descriptions need to be provided for the patient regarding how he/she is supposed to carry out the activity. The patient’s interface must provide feedback on the correctness of the performed activities, as well as a qualitative evaluation of his/her movement. All measurements and relevant data are collected, processed, and stored during each session and are remotely available. For example, in PAMAP the data is stored in the user’s electronic health record (EHR) ( Martinez-Martin and Cazorla, 2019 ). In the end, IoT technologies can be utilized to connect the entire system together. These IoT-enabled software interfaces enable the therapist to remotely monitor a patient’s progress and tailor a customized treatment plan for him/her ( Agyeman and Al-Mahmood, 2019 ).
Due to differences in patient demographics, needs, and characteristics of the target group must be carefully mapped to a set of general design principles. So as for considering the common cognitive impairments resulting from stroke or normal aging, there are certain factors to have in mind. These factors include ensuring clear directions, providing larger letters and numbering for improved readability, and avoiding vagueness, complexity, and involvement of what Egglestone et al. introduce as abstract thought ( Egglestone et al., 2009 ; Cavuoto et al., 2018 ). Also, people with other pre-existing disabilities should also be taken into account, e.g., a text to speech component could be added to the interface for people with vision problems and sign language for people with hearing problems ( Gomez-Donoso et al., 2017b ).
To successfully replace the direct involvement of a therapist with a virtual one, the interfaces should provide options resembling those of traditional methods. Each interface has to be designed to replicate the traditional rehabilitation in a clinical setting, which means the therapist should be able to track the patient’s movements, assess his/her performance and analyze his/her progress to be able to prescribe the right treatment plan. Therefore, there is a need for careful evaluation of all of the steps that a therapist takes in a traditional setting to identify the parameters he needs to access. Once these parameters have been identified, user interface software through graphical interfaces (GUIs) provides access for displaying and editing stored data. This provides a base for therapists upon which they can make more educated decisions and choose the best treatment plan for their patients. Also, it is possible to add reminders to the system for notifying the patient to do his/her daily practices. In the end, the system uses this information to adapt the treatment program focusing on the parts that require more recovery to increase therapeutic value.
There are some other crucial principles to be considered for offering software interfaces that assist home-based systems in providing structured training sessions. A user-friendly interface in terms of environment and layout of features and components is the starting point of having a well-established interaction between patients and the device. This friendliness arises from patients’, as well as their caregivers’, ability to interact easily with the device in a familiar environment and to have quick and straightforward access to the training and monitoring components ( Amirabdollahian et al., 2014 ). Enabling the patient to personalize the user interface environment, for example, in terms of shape or size of components or layout of different parts and modules, may increase the user-friendliness and create a sense of comfort and control.
For patients to be engaged and challenged during home rehabilitation in the physical absence of the therapist, there is a significant need for careful study and practice of motivational scenarios and features that ensure patient’s adherence to the treatment program. The most commonly identified and mentioned barriers to motivation are that rehabilitation scenarios are inherently repetitive and boring, having in mind that stroke patients themselves are prone to depression due to several factors such as social isolation and fatigue that is common among them ( Egglestone et al., 2009 ; Borghese et al., 2013 ). The intrinsic entertaining characteristic of Virtual/Augmented Reality (VR, AR) and gaming present an alternative world in which mundane rehabilitation exercises are disguised in the shape of appealing fantasies. Integrating these methods into home rehabilitation is a common practice aimed at encouraging motivation in patients. The score-based nature of gaming further motivates patients—by rewarding points, coins, and badges as immediate feedback—and reinforces confidence and positivity through achievements ( Hatem et al., 2016 ). VR, AR, and gaming offer plausible environments for versatile game design. Tuning the game content to the interests and needs of users ( Egglestone et al., 2009 ), introducing variability and unpredictability to the tasks, e.g., by contextual interface concept, and programming real-world tasks by simulating real-life objects and events ( Martinez-Martin and Cazorla, 2019 ), may encourage motivation even further. Another great feature of these methods, which makes them preferable to traditional ones, is that during therapy, the enjoyable experience distracts the patients from the prevalent potential pain induced by stroke ( Gama et al., 2016 ). However, an important question remains regarding the efficacy and generalization of this virtual recovery to real world activities. Several studies have been conducted addressing this issue and found that training in a virtual environment offers equal, if not better, motor recovery compared to real-life training ( Rose et al., 2005 ). According to Gama et al. (2016) , AR might be more effective than VR, for it provides auto visualization during task execution and this, in turn, can improve patient corporal conscience and potentially engage additional visuospatial networks of the cortex; hence, it leads to more precision and accuracy respectively during interactive exercises and path traveling.
Designing games specifically for rehabilitation purposes, serious games, requires the incorporation of both entertaining and therapeutic goals. Games should offer fully customizable task options and hierarchical order of difficulty for each task. During the recovery process, patients’ mental and functional abilities improve, and tasks must be adapted to this variability to keep patients motivated by challenging yet achievable tasks at each stage of the recovery. In other words, by careful modification of the current skill level of the patient and challenge posed by the game, e.g., tuning assistance level and therapy intensity and difficulty, patients may go through a flow-like experience which in turn could potentially increase their active participation ( Egglestone et al., 2009 ). In order to create a unified experience for the patient, it is preferable to develop games around a resonant theme, like Egglstone et al. approach, rather than unrelated minigames. In this approach, the systems do not merely offer video games but complete environments ( Agyeman, Al-Mahmood, et al., 2019 ; Egglestone et al., 2009 ). It is also suggested to design flexible systems to have the capacity to integrate and support various devices. For example, audio-visual and haptic interfaces will enable the therapists to customize interaction mechanisms and to choose the proper device to deliver the best treatment regime with optimum efficacy for covering the wide range of disabilities, be it upper or lower. In this regard, Egglestone et al. developed a game that could be played by feet, hands, or whole body ( Egglestone et al., 2009 ). In this way, a standardized game platform could be proposed to the entire community of therapists.
Training in home settings requires interface developers to design meaningful and consistent feedback, which should be similar to therapists’ clinical feedback—both on the quality of movement and their progress in performing tasks and achieving long-term and personal goals. Providing clear and customized feedback is another way of increasing engagement and motivation in patients, positively affecting retention and recovery ( Kitago and Krakauer, 2013 ). Indeed, creating awareness in patients of their progress and offering a progress tracking module is one crucial approach toward stimulating internal motivation ( Chen et al., 2019 ). Designing both immediate feedback during the therapy and after-session/long-term feedback must be taken into consideration as well. According to Guadagnoli et al., the immediacy of the feedback should be proportional to the task difficulty, i.e., the easier the task, the less frequent the feedback ( Guadagnoli et al., 1996 ). Most current devices, as seen in the previous section, provided immediate visual, auditory, and haptic feedback to the patient ( Lyu et al., 2019 ), which showed improved motivation, for example, by congratulation alarms after each correctly performed task, as well as educating and correcting task performances. Should the patient execute an exercise incorrectly, the system warns the patient and provides graphical help to guide the patient to perform it correctly. For instance, Gloreha provides a 3D simulated preview of the movement on the monitor as well as when the exercise is being performed ( Bernocchi et al., 2018 ), PHAROS provides audio-visual descriptions ( Martinez-Martin and Cazorla, 2019 ), and Lyu et al. generated haptic feedback through vibrating motors as punishment ( Bernocchi et al., 2018 ; Lyu et al., 2019 ).
Daily and long-term summaries of patient records are another critical form of feedback, termed “knowledge of results” by Schmidt and Lee (1999) , and it is a major source of motivation for patients. According to Chen et al. review study, a number of developers of home-based devices described how patients came motivated to proceed with therapy when they found themselves recovering ( Chen et al., 2019 ). Progress tracking and visual feedback are better provided by graphical representation such as bar or pie charts, rather than numerical or analytical representation, to make a clearer sense of change at first glance. This can be particularly useful when the patients are mostly among elderlies, and it may be difficult for them to analyze the results. Note that patients’ dependence on feedback is not favorable, and to avoid that, it is suggested to lower the feedback frequency over time ( Kitago and Krakauer, 2013 ).
When designing for home use, particularly due to the absence of a medical professional, it is essential to consider therapeutic and clinical requirements from the therapists’ perspective. Based on the premise that adoption of mechatronic rehabilitation devices heavily relies on the acceptability of the device from the therapist’s point of view, Despleneter et al.surveyed Canadian therapists to extract design features that successfully convey the needs of the therapists. Then they mapped the derived data into software requirements for an enhanced therapist-device relationship ( Desplenter et al., 2019 ). A good therapist-device relationship is desirable for equipping therapists with an extensive data set of patient history to be assessed and analyzed toward optimized and educated planning of treatment strategies. Participants mentioned the five areas of patient history, pain, motion, activities, or strength as their most favorable data to be tracked over time for patients’ assessment. Desplenter et al. concluded that the designed software system should have features such as standard data records, treatment plan templates, and patient evaluation scales. The user should also be able to design and customize these templates and scales to meet the needs of a particular treatment strategy, both qualitative and quantitative. For this purpose, time-stamped data should be collected, and numerical analyses and visualizations should be generated based on the quantitative data to provide reports. Visualization, particularly in graphs and tables, facilitates data assessment, and puts them into perspective for the therapists. Eventually, a cumulative report must be provided in the therapist’s interface in which session history, the patient’s history, and progress tracker are among its most important components.
Conceptual Framework
In the previous sections, some of the existing home-based rehabilitation devices were briefly reviewed, and after a general analysis of current challenges and shortcomings, a guideline for designing the components of these systems, in particular for utilizing new advanced technologies, was discussed. As described before, there is a need for a telerehabilitation platform that concerns remote supervision implementation by interconnecting all described modules. To this end, reviewed home-based systems have leveraged different approaches. For example, HomeRehab benefited from a cloud service for exchanging performance and therapy information between the patient and the therapist ( Díaz et al., 2018 ). Amirabdollahian et al. utilized a tele-robotic support platform in their SCRIPT project consisting of a replicated database, a healthcare professional web portal, and a decision support system ( Amirabdollahian et al., 2014 ). Chen et al. also benefited from a web server for the Tele-Doctor-Patient Interaction module in their proposed rehabilitation wheelchair system ( Chen S. et al., 2017 ). Ambidexter provides remote rehabilitation through IoT technologies ( Wai et al., 2018 ). While the aforementioned systems undoubtedly help with remote supervision and patient-therapist interaction, most of them are case-specific for their device and do not offer an interoperable platform allowing for supporting other devices. On the other hand, telehealth platforms for in-home interventions, which need more supervision, information exchange, and online communication, have gained significant traction over the recent years, (e.g., Wang et al., 2017 ; Kouris et al., 2018 ; Hilty et al., 2019 ; Tun et al., 2020 ; Tsiouris et al., 2020 ). Among existing telehealth frameworks, they either do not specifically and comprehensively address post-stroke robotic rehabilitation interventions or do not cover the beyond-physical-rehabilitation demands of this population. Therefore, to address the aforementioned limitations as well as to cover the real-life needs of post-stroke individuals, we propose a versatile conceptual framework for community-based robotic in-home rehabilitation. Our proposed framework conceptually provides a unified interoperable solution for interconnecting various rehabilitation robots, lays a foundation for in-home rehabilitation and supports beyond-rehabilitation needs of the post-stroke community—like the need for socialization and assisting in gaining independence for daily activities like shopping, especially during pandemics. Our framework offers a comprehensive solution addressing the multi-faceted needs of involved parties in post-stroke rehabilitation, including patients, healthcare professionals and researchers. To this end, some existing telehealth frameworks, e.g., HOLOBALANCE ( Tsiouris et al., 2020 ), could potentially be modified and adapted for implementing our conceptual framework.
This framework’s central concept is based on the development of a multi-agent IoT communication system in which patients, therapists, and administrators each represent an agent of their community interacting with each other over the provided platform ( Figure 6 ). This interaction utilizes cloud-based methods for computation, storage and analysis of data collected from each agent. The acquired data is initially processed before being transmitted to cloud and fog servers, where they form a large data set. According to Sahu et al. (2020) , due to the sensitive nature of medical data, security, privacy, and confidentiality, as well as the integrity of these data, are of critical importance. Hence, to monitor these concerns, precautionary safety measures must be developed in advance. Also, policies and rules, defining and authorizing the access level of each agent, need to be carefully established and implemented in order to ensure and enhance the security and privacy of the system ( Sahu et al., 2020 ).
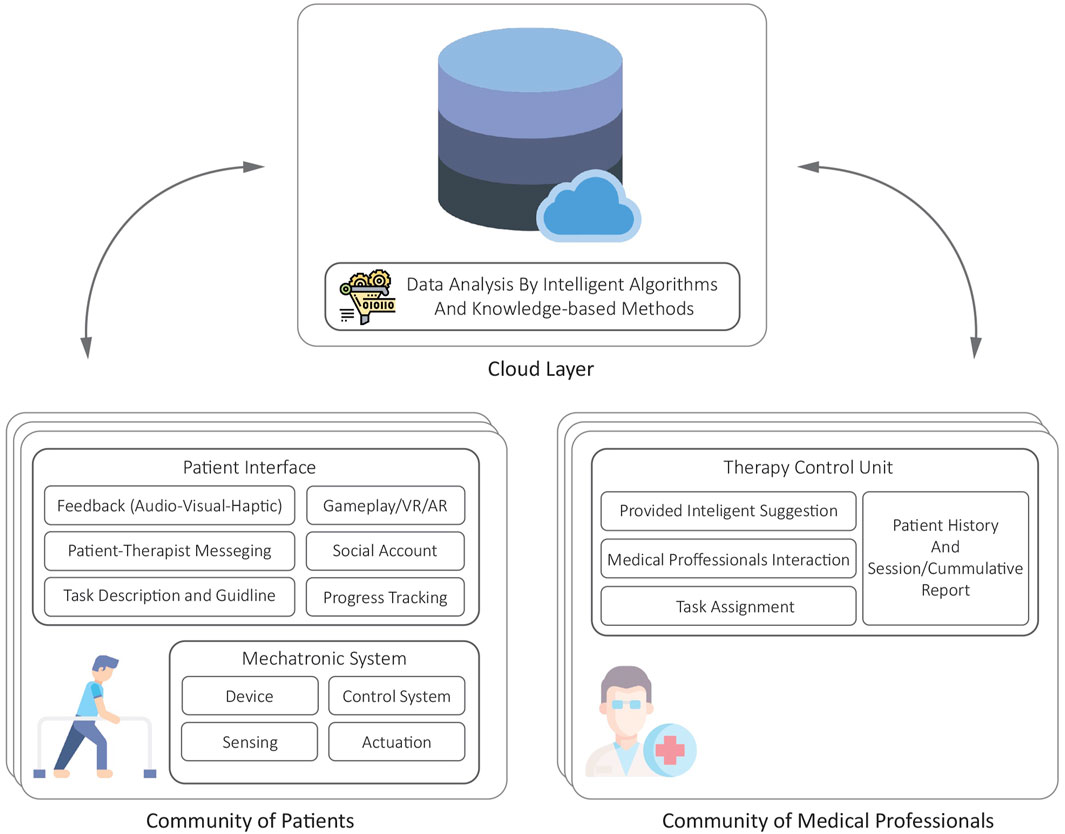
FIGURE 6 . The proposed conceptual framework for community-based home rehabilitation.
The acquired data can be initially processed before being transmitted to cloud and fog servers, where they form a large data set. For example, online identification of musculoskeletal parameters and their variation during treatment can be conducted and plotted in the smartphone, PC, or any other digital processor which is connected to the rehabilitation robot. These preprocessing features can be based on either model-based approaches, or artificial intelligence (AI) algorithms such as artificial neural networks. Although training of such systems can be computationally very demanding for these processors, the training process is performed mostly offline. Applying the trained AI feature and even its required recursive update does not involve significant computation, and can be easily handled on the processor of a smartphone, PC, or any other available digital processor.
Acquired data from agents should be comparable with each other, and therefore it is critical to bring the collected data into a common format which occurs through data standardization. By providing internally consistent data with the same content and format, any confusion and ambiguity are avoided. This ensures that all parties involved have mutual understanding, and view the results in the same way which leads to reliable measurements and decision plans. Standardized quantitative and qualitative data should be collected for reliable and educated assessment of patients’ performance through evaluation forms and outcome measurements. From a therapist’s point of view, the prescribed tasks, reports, and evaluations should be offered in a standard format. To do so, there needs to be a predetermined data extraction on which the community of healthcare professionals has reached a consensus. However, in case of any ambiguity or to provide further details and explanations, therapists should have the option of attaching notes to the data ( Desplenter et al., 2019 ).
The proposed community-based rehabilitation framework facilitates knowledge sharing and provides comprehensive data to ensure educated judgment regarding the best treatment regimen yielding the best recovery outcome. This massive pool of information—both from recorded data and also normalized datasets from already existing medical center repositories—generates big data. By applying machine learning, meaningful information from these large data sets can be extracted. This extensive database can be beneficial for various applications, one of which could be providing a research base enabling further studies in fields of neurorehabilitation, engineering, and occupational/physical therapists. Thus, in this IoT-enabled rehabilitation system, data analysis is performed by intelligent algorithms and knowledge-based methods to provide intelligent suggestions to the therapists, creating a basis for the best treatment plan assignment.
In the framework, the capability of communication among agents of each community is also feasibly provided. The interaction of healthcare professionals gives them the opportunity to clarify any issues or ambiguities, gain additional expertise, discuss possible treatments, and share their knowledge. The provided platform can also enable socialization among patients. Social restrictions imposed by stroke and exacerbated by the COVID-19 pandemic, have adversely affected patients’ lives. Therefore, any means of social interaction could potentially improve their quality of life, which in turn could further motivate them to adhere to the therapy ( Egglestone et al., 2009 ). This community-based rehabilitation allows the possibility of performing rehabilitation in the connected groups, for example, through multiplayer gaming. By optional assignment of a social media profile to each patient ID, they could also share their achievements and support each other.
We suggest an alternative approach in which the purpose of the framework goes beyond rehabilitation to contribution to society and household chores. The virtual environment tasks should be designed so that, along with rehabilitation, they can offer patients the opportunity to learn real-life and daily living skills—cooking, gardening, and housekeeping, to name a few. For example, learning the cooking skill takes place in a virtual kitchen where all of the ingredients and utensils are available along with cooking instructions in the form of video and audio to enable the patient to learn and practice simultaneously. The theme-based free world gameplay would be one of the best options as it can be flexible enough to reflect various aspects of daily life. For example, Saebo Inc. has developed a virtual reality environment in which in the cooking scenario, should the virtual kitchen lack any of the ingredients or utensils required to complete the task, the game enables the patient to go grocery shopping; this way through a comprehensive experience, patient not only goes through therapy but gets to enhance his/her life skills. Integrating this free world environment with our suggested community-based framework adds an additional social layer where patients can teach and learn these skills from each other. For instance, if a patient knows a particular recipe, she/he can teach and share it with the patient community. This provides the patients with a sense of control over their treatment and fosters a sense of usefulness, purpose, and contribution to society. Also, in this time of COVID-19, where everybody, especially elders, feels disconnected from the world, this will excite the feelings of inclusion and connection, which in turn will further motivate the patients to partake more frequently and actively in their treatment sessions.
To go one step further, this virtual environment could be linked to existing online shops allowing the patient to contribute to household chores by purchasing the required and essential items for the house and facilitating the acquisition of his/her own necessities. According to Brenner and Clarke (2019) , shopping difficulty is one of the most common self-reported difficulties with daily activities among individuals with disabilities. Thus, the enhanced autonomy and independence in patients alleviates the prevalent feeling of being a burden to their family which in turn promotes a sense of achievement and self-worth. The suggested alternative approaches and rehabilitation are not mutually exclusive, as maintaining engagement in daily life activities is critical for functional and motor recovery ( Brenner and Clarke, 2019 ). As of 2020, due to restrictions caused by the COVID-19 Pandemic and the importance of adhering to social distancing measures, online shopping has become ubiquitous, making this option ever-increasingly plausible.
Finally, the framework has the capacity to incorporate an ambient assisted living ecosystem that can provide patients, specifically elders, with personalized options. These options include remote monitoring, assessment, and support based on their unique profiles and surrounding context. The purpose of such options is to enhance the independence of elderly or disabled individuals in their own secure and convenient space of living ( Al-Shaqi et al., 2016 ; Sahu et al., 2020 ; Stavropoulos et al., 2020 ).
Rehabilitation programs, even in the clinical setting, are often not fully supported by public health systems, and hence not all post-stroke patients can afford this option, and due to the enormous socioeconomic impact and high costs, not just on the patient but also on their families, patients opt to drop out ( Borghese et al., 2014 ). Additionally, implementing the proposed framework for post-stroke telerehabilitation, imposes extra costs to the patients for providing the required hardware and software basis of the telehealth framework. For home-based rehabilitation to be acceptable by all end-users involved (patients, clinicians, therapists, and health administrators), these challenges and limitations need to be addressed both by healthcare providers and technology developers. Burden and strain potentially imposed on caregivers are other limiting concerns. However, implementation of the proposed telerehabilitation framework could offer valuable functional outcomes for motor recovery of the wide range of the post-stroke population. Future work should focus on evaluating the proposed telerehabilitation framework for community-based remote therapy during and beyond the pandemic, in terms of different use dimensions, technological acceptance by the stakeholders, clinical efficacy of the system and its economic efficiency.
Early-stage post-stroke rehabilitation, a crucial phase of rehabilitation, could be a direct indicator of the possibility of motor recovery. In the current restrictive climate of the COVID-19, due to the restrictions associated with social distancing and limitations for commuting, it has become more difficult for patients to use this sensitive window of time for rehabilitation treatments. In this context, home-based rehabilitation can be a solution.
Several home-based rehabilitation devices have been developed in recent years, both at the research and commercial levels. Although there are some challenges in the research and development of these devices, based on a technical evaluation of aspects such as mechatronics, the control system, and software, these devices can be tuned to be suitable for home-based rehabilitation therapy. Eventually, such systems can be utilized in a framework aiming at creating a comprehensive network for therapists, patients, engineers, and researchers. To this end, standardization in every aspect of home-based systems, from design criteria to performance evaluation, is required.
The COVID-19 pandemic has proved the necessity of further deploying the power of the internet and computers, not only for the purpose of communication but also for big data analysis. Such technologies can be feasibly integrated with mechatronic systems such as rehabilitation robots to enable new applications such as remote home-based therapies. The outcome of such an interconnected framework would be significantly vital for the recovery of post-stroke patients, promoting the quality of their lives, and eventually reducing the associated burdens on the healthcare system in the long term.
Data Availability Statement
The original contributions presented in the study are included in the article/Supplementary Material, further inquiries can be directed to the corresponding author.
Author Contributions
FH and AA equally performed the reviewing of the literature, analyzing the reviewed studies, and writing the draft of the manuscript. SB performed the conception of the order of the paper. All authors contributed to manuscript revision, read, and approved the submitted version.
Conflict of Interest
The authors declare that the research was conducted in the absence of any commercial or financial relationships that could be construed as a potential conflict of interest.
Adams, R. J., Lichter, M. D., Ellington, A., White, M., Armstead, K., Patrie, J. T., et al. (2018). Virtual Activities of Daily Living for Recovery of Upper Extremity Motor Function. IEEE Trans. Neural Syst. Rehabil. Eng. 26 (1), 252–260. doi:10.1109/tnsre.2017.2771272
PubMed Abstract | CrossRef Full Text | Google Scholar
Aggarwal, G., Lippi, G., and Michael Henry, B. (2020). Cerebrovascular Disease Is Associated with an Increased Disease Severity in Patients with Coronavirus Disease 2019 (COVID-19): A Pooled Analysis of Published Literature. Int. J. Stroke 15 (4), 385–389. doi:10.1177/1747493020921664
Aggogeri, F., Mikolajczyk, T., and O’Kane, J. (2019). Robotics for Rehabilitation of Hand Movement in Stroke Survivors. Adv. Mech. Eng. 11 (4), 168781401984192. doi:10.1177/1687814019841921
CrossRef Full Text | Google Scholar
Agyeman, M. O., and Al-Mahmood, A. (2019). “Design and Implementation of a Wearable Device for Motivating Patients with Upper And/or Lower Limb Disability via Gaming and Home Rehabilitation,” in 2019 Fourth International Conference on Fog and Mobile Edge Computing (FMEC) . doi:10.1109/fmec.2019.8795317
Agyeman, M. O., Al-Mahmood, A., and Hoxha, I. (2019). “A Home Rehabilitation System Motivating Stroke Patients with Upper And/or Lower Limb Disability,” in Proceedings of the 2019 3rd International Symposium on Computer Science and Intelligent Control . doi:10.1145/3386164.3386168
Ail, N. L. M., Ambar, R., Wahab, M. H. A., Idrus, S. Z. S., and Jamil, M. M. A. (2020). Development of a Wrist Rehabilitation Device with Android-Based Game Application. J. Phys. Conf. Ser. 1529, 022110. doi:10.1088/1742-6596/1529/2/022110
Al-Shaqi, R., Mourshed, M., and Rezgui, Y. (2016). Progress in Ambient Assisted Systems for Independent Living by the Elderly. Springer Plus 5, 624. doi:10.1186/s40064-016-2272-8
Alamdari, A., and Krovi, V. (2015). “Modeling and Control of a Novel Home-Based Cable-Driven Parallel Platform Robot: PACER,” in 2015 IEEE/RSJ International Conference on Intelligent Robots and Systems (IROS) . doi:10.1109/iros.2015.7354281
Ali, A., Ahmed, S. F., Joyo, M. K., Malik, A., Ali, M., Kadir, K., et al. (2016). Control Strategies for Robot Therapy . Sindh University Research Journal (Science Series) SURJ .
AlterG (2015). AlterG Bionic Leg User Manual. https://www.who.int/news-room/fact-sheets/detail/e-coli (Accessed April 10, 2021).
Google Scholar
AlterG (n.d). https://www.alterg.com/ (Accessed May 5, 2021).
Ambar, R., Yusof, Y., Jamil, M. M. A., Sharif, J. M., and Ngadi, M. A. (2017). Design of Accelerometer Based Wrist Rehabilitation Device . International Journal of Electrical and Computer Engineering (IJECE) . doi:10.1109/ict-ispc.2017.8075342
CrossRef Full Text
Amirabdollahian, F., Ates, S., Basteris, A., Cesario, A., Buurke, J., Hermens, H., et al. (2014). Design, Development and Deployment of a Hand/wrist Exoskeleton for Home-Based Rehabilitation after Stroke - SCRIPT Project . Robotica .
Atashzar, S. F., Shahbazi, M., Tavakoli, M., and Patel, R. V. (2017). A Grasp-Based Passivity Signature for Haptics-Enabled Human-Robot Interaction: Application to Design of a New Safety Mechanism for Robotic Rehabilitation. Int. J. Robotics Res. 36 (5–7), 778–799. doi:10.1177/0278364916689139
Ates, S., Haarman, C. J. W., and Stienen, A. H. A. (2017). SCRIPT Passive Orthosis: Design of Interactive Hand and Wrist Exoskeleton for Rehabilitation at Home after Stroke . Autonomous Robots .
Aubin, P. M., Sallum, H., Walsh, C., Stirling, L., and Correia, A. (2013). A Pediatric Robotic Thumb Exoskeleton for at-home Rehabilitation: the Isolated Orthosis for Thumb Actuation (IOTA). IEEE Int. Conf. Rehabil. Robot 2013, 6650500. doi:10.1109/ICORR.2013.6650500
Awad, L. N., Esquenazi, A., Francisco, G. E., Nolan, K. J., and Jayaraman, A. (2020). The ReWalk ReStore Soft Robotic Exosuit: a Multi-Site Clinical Trial of the Safety, Reliability, and Feasibility of Exosuit-Augmented post-stroke Gait Rehabilitation. J. Neuroengineering Rehabil. 17 (1), 80. doi:10.1186/s12984-020-00702-5
Bai, S., and Rasmussen, J. (2011). “Modelling of Physical Human-Robot Interaction for Exoskeleton Designs,” in Proceedings of the ECCOMAS Thematic Conference on Multibody Dynamics , Belgium .
Bai, S., Virk, G. S., and Sugar, T. G. (2018). Wearable Exoskeleton Systems: Design, Control and Applications, Control . Robotics and Sensors , 408.
Basteris, A., Nijenhuis, S. M., Stienen, A. H., Buurke, J. H., Prange, G. B., and Amirabdollahian, F. (2014). Training Modalities in Robot-Mediated Upper Limb Rehabilitation in Stroke: A Framework for Classification Based on a Systematic Review. J. NeuroEngineering Rehabil. 11, 111. doi:10.1186/1743-0003-11-111
Beckerle, P., Salvietti, G., Unal, R., Prattichizzo, D., Rossi, S., Castellini, C., et al. (2017). A Human-Robot Interaction Perspective on Assistive and Rehabilitation Robotics. Front. Neurorobot. 11, 24. doi:10.3389/fnbot.2017.00024
Bellomo, R. G., Paolucci, T., Saggino, A., Pezzi, L., Bramanti, A., Cimino, V., et al. (2020). The WeReha Project for an Innovative Home-Based Exercise Training in Chronic Stroke Patients: A Clinical Study. J. Cent. Nerv Syst. Dis. 12, 117957352097986. doi:10.1177/1179573520979866
Beom-Chan, L., Dae-Hee, K., Younsun, S., Kap-Ho, S., Sung Ho, P., Dongyual, Y., et al. (2017). Development and Assessment of a Novel Ankle Rehabilitation System for Stroke Survivors. IEEE Eng. Med. Biol. Soc. 2017, 3773–3776. doi:10.1109/EMBC.2017.8037678
Bernocchi, P., Mulè, C., Vanoglio, F., Taveggia, G., Luisa, A., and Scalvini, S. (2018). Home-based Hand Rehabilitation with a Robotic Glove in Hemiplegic Patients after Stroke: A Pilot Feasibility Study. Top. Stroke Rehabil. 25 (2), 114–119. doi:10.1080/10749357.2017.1389021
Bertani, R., Melegari, C., De Cola, M. C., Bramanti, A., Bramanti, P., and Calabrò, R. S. (2017). Effects of Robot-Assisted Upper Limb Rehabilitation in Stroke Patients: A Systematic Review with Meta-Analysis. Neurol. Sci. 38 (9), 1561–1569. doi:10.1007/s10072-017-2995-5
BIOSERVO (). Keeping People Strong Healthy and Motivated. https://www.bioservo.com (Accessed May 5, 2021).
Borboni, A., Mor, M., and Faglia, R. (2016). Gloreha—Hand Robotic Rehabilitation: Design, Mechanical Model, and Experiments. J. Dynamic Syst. Meas. Control. 138, 11. doi:10.1115/1.4033831
Borghese, N. A., Alberto Borghese, N., Murray, D., Paraschiv-Ionescu, A., Bruin, E. D., Bulgheroni, M., et al. (2014). Rehabilitation at Home: A Comprehensive Technological Approach . Virtual, Augmented Reality and Serious Games for Healthcare , 1.
Borghese, N. A., Mainetti, R., Pirovano, M., Lanzi, P. L., and Lanzi, P. L. (2013). “An Intelligent Game Engine for the at-Home Rehabilitation of Stroke Patients,” in 2013 IEEE 2nd International Conference on Serious Games and Applications for Health (SeGAH) . doi:10.1109/segah.2013.6665318
Brenner, A. B., and Clarke, P. J. (2019). Difficulty and Independence in Shopping Among Older Americans: More Than Just Leaving the House. Disabil. Rehabil. 41 (2), 191–200. doi:10.1080/09638288.2017.1398785
Brewer, B. R., McDowell, S. K., and Worthen-Chaudhari, L. C. (2007). Poststroke Upper Extremity Rehabilitation: A Review of Robotic Systems and Clinical Results. Top. Stroke Rehabil. 14 (6), 22–44. doi:10.1310/tsr1406-22
Brokaw, E. B., Black, I., Holley, R. J., and Lum, P. S. (2011). Hand Spring Operated Movement Enhancer (HandSOME): A Portable, Passive Hand Exoskeleton for Stroke Rehabilitation. IEEE Trans. Neural Syst. Rehabil. Eng. 19 (4), 391–399. doi:10.1109/tnsre.2011.2157705
Buesing, C., Fisch, G., O’Donnell, M., Shahidi, I., Thomas, L., Mummidisetty, C. K., et al. (2015). Effects of a Wearable Exoskeleton Stride Management Assist System (SMA) on Spatiotemporal Gait Characteristics in Individuals after Stroke: a Randomized Controlled Trial. J. Neuroengineering Rehabil. 12, 69. doi:10.1186/s12984-015-0062-0
Bütefisch, C., Hummelsheim, H., Denzler, P., and Mauritz, K.-H. (1995). Repetitive Training of Isolated Movements Improves the Outcome of Motor Rehabilitation of the Centrally Paretic Hand. J. Neurol. Sci. 130 (1), 59–68. doi:10.1016/0022-510x(95)00003-k
Butler, A. J., Bay, C., Wu, D., Richards, K. M., and Buchanan, S. (2014). Expanding Tele-Rehabilitation of Stroke through in-home Robot- Assisted Therapy. Int. J. Phys. Med. Rehabil. 2, 184. doi:10.4172/2329-9096.1000184
Butler, N. R., Goodwin, S. A., and Perry, J. C. (2017). “Design Parameters and Torque Profile Modification of a Spring-Assisted Hand-Opening Exoskeleton Module, IEEE,” in International Conference on Rehabilitation Robotics: [proceedings] , 591–596.
Carbone, G., Gherman, B., Ulinici, I., Vaida, C., and Pisla, D. (2018). Design Issues for an Inherently Safe Robotic Rehabilitation Device . Advances in Service and Industrial Robotics .
Cardona, M., and Garcia Cena, C. E. (2019). Biomechanical Analysis of the Lower Limb: A Full-Body Musculoskeletal Model for Muscle-Driven Simulation . IEEE Access .
Carlan, A.Singleorigin (2020). EksoNR - the Next Step in NeuroRehabilitation - Ekso Bionics. https://eksobionics.com/eksonr/ (Accessed May 5, 2021).
Catalan, J. M., Garcia, J. V., Lopez, D., Diez, J., Blanco, A., Lledo, L. D., Badesa, F. J., Ugartemendia, A., Diaz, I., Neco, R., and Garcia-Aracil, N. (2018). “Patient Evaluation of an Upper-Limb Rehabilitation Robotic Device for Home Use,” in 2018 7th IEEE International Conference on Biomedical Robotics and Biomechatronics (Biorob) . doi:10.1109/biorob.2018.8487201
Cavuoto, L. A., Subryan, H., Stafford, M., Yang, Z., Bhattacharjya, S., Xu, W., et al. (2018). “Understanding User Requirements for the Design of a Home-Based Stroke Rehabilitation System,” in Proceedings of the Human Factors and Ergonomics Society Annual Meeting .
Chen, G., Qi, P., Guo, Z., and Yu, H. (2016). Mechanical Design and Evaluation of a Compact Portable Knee–Ankle–Foot Robot for Gait Rehabilitation . Mechanism and Machine Theory .
Chen, J., and Lum, P. S. (2018). Pilot Testing of the Spring Operated Wearable Enhancer for Arm Rehabilitation (SpringWear). J. Neuroengineering Rehabil. 15 (1), 13. doi:10.1186/s12984-018-0352-4
Chen, J., Nichols, D., Brokaw, E. B., and Lum, P. S. (2017). Home-based Therapy after Stroke Using the Hand Spring Operated Movement Enhancer (HandSOME). IEEE Trans. Neural Syst. Rehabil. Eng. 25 (12), 2305–2312. doi:10.1109/tnsre.2017.2695379
Chen, J. P. S., and Lum, P. S. (2016a). Spring Operated Wearable Enhancer for Arm Rehabilitation (SpringWear) after Stroke. Annu. Int. Conf. IEEE Eng. Med. Biol. Soc. 2016, 4893–4896. doi:10.1109/EMBC.2016.7591824
Chen, S., Fu, F.-F., Meng, Q.-L., and Yu, H.-L. (2017). Development of a Lower Limb Rehabilitation Wheelchair System Based on Tele-Doctor–Patient Interaction . Wearable Sensors and Robots .
Chen, T. P. S., and Lum, P. S. (2016b). “Hand Rehabilitation after Stroke Using a Wearable, High DOF, Spring Powered Exoskeleton,” in Annual International Conference of the IEEE Engineering in Medicine and Biology Society , 578–581. doi:10.1109/EMBC.2016.7590768
Chen, Y., Abel, K. T., Janecek, J. T., Chen, Y., Zheng, K., and Cramer, S. C. (2019). Home-based Technologies for Stroke Rehabilitation: A Systematic Review. Int. J. Med. Inform. 123, 11–22. doi:10.1016/j.ijmedinf.2018.12.001
Cherry, C. O. B., Chumbler, N. R., Richards, K., Huff, A., Wu, D., Tilghman, L. M., et al. (2017). Expanding Stroke Telerehabilitation Services to Rural Veterans: a Qualitative Study on Patient Experiences Using the Robotic Stroke Therapy Delivery and Monitoring System Program. Disabil. Rehabil. Assistive Tech. 12 (1), 21–27. doi:10.3109/17483107.2015.1061613
Coevoet, E., Morales-Bieze, T., Largilliere, F., Zhang, Z., Thieffry, M., Sanz-Lopez, M., et al. (2017). Software Toolkit for Modeling, Simulation, and Control of Soft Robots . Advanced Robotics .
Coffey, A. L., Leamy, D. J., and Ward, T. E. (2014). “A Novel BCI-Controlled Pneumatic Glove System for home-based Neurorehabilitation,” in Conference Proceedings: IEEE Engineering in Medicine and Biology Society. Conference , 3622–3625. doi:10.1109/EMBC.2014.6944407
Cyberoyne (2021). Hall for Well Being (Lower Limb Type). https://www.cyberdyne.jp/english/products/fl05.html (Accessed April 10, 2021).
Delph, M. A., Fischer, S. A., Gauthier, P. W., Luna, C. H. M., Clancy, E. A., and Fischer, G. S. (2013). A Soft Robotic Exomusculature Glove with Integrated sEMG Sensing for Hand Rehabilitation. IEEE Int. Conf. Rehabil. Robot 2013, 6650426. doi:10.1109/ICORR.2013.6650426
Desplenter, T., Chinchalkar, S., and Trejos, A. L. (2019). “Enhancing the Therapist-Device Relationship: Software Requirements for Digital Collection and Analysis of Patient Data,” in 2019 IEEE 16th International Conference on Rehabilitation Robotics (ICORR) . doi:10.1109/icorr.2019.8779528
Desplenter, T., and Trejos, A. L. (2020). A Control Software Framework for Wearable Mechatronic Devices . Journal of Intelligent & Robotic Systems .
Desplenter, T., Zhou, Y., Edmonds, B. P., Lidka, M., Goldman, A., and Trejos, A. L. (2020). Rehabilitative and Assistive Wearable Mechatronic Upper-Limb Devices: A Review. J. Rehabil. Assistive Tech. Eng. 7, 205566832091787. doi:10.1177/2055668320917870
Díaz, I., Catalan, J. M., Badesa, F. J., Justo, X., Lledo, L. D., Ugartemendia, A., et al. (2018). Development of a Robotic Device for Post-Stroke Home Tele-Rehabilitation. Adv. Mech. Eng. 10 (1), 168781401775230. doi:10.1177/1687814017752302
Díaz, I., Gil, J. J., and Sánchez, E. (2011). Lower-Limb Robotic Rehabilitation: Literature Review and Challenges. J. Robotics 2011, 1. doi:10.1155/2011/759764
Doucet, B. M., and Mettler, J. A. (2018). Pilot Study Combining Electrical Stimulation and a Dynamic Hand Orthosis for Functional Recovery in Chronic Stroke . American Journal of Occupational Therapy .
Dumitru, S., Copilusi, C., and Dumitru, N. (2018). A Leg Exoskeleton Command Unit for Human Walking Rehabilitation . Advanced Engineering Forum . doi:10.1109/aqtr.2018.8402732
Duncan, P. W., Zorowitz, R., Bates, B., Choi, J. Y., Glasberg, J. J., Graham, G. D., et al. (2005). Management of Adult Stroke Rehabilitation Care. Stroke 36 (9), e100–143. doi:10.1161/01.str.0000180861.54180.ff
Egglestone, S. R., Axelrod, L., Nind, T., Turk, R., Wilkinson, A., Burridge, J., Fitzpatrick, G., Mawson, S., Robertson, Z., Hughes, A. M., Ng, K. H., Pearson, W., Shublaq, N., Probert-Smith, P., Rickets, I., and Rodden, T. (2009). “A Design Framework for a Home-Based Stroke Rehabilitation System: Identifying the Key Components,” in Proceedings of the 3d International ICST Conference on Pervasive Computing Technologies for Healthcare . doi:10.4108/icst.pervasivehealth2009.6049
Eiammanussakul, T., and Sangveraphunsiri, V. (2018). A Lower Limb Rehabilitation Robot in Sitting Position with a Review of Training Activities. J. Healthc. Eng. 2018, 1–18. doi:10.1155/2018/1927807
Feng, Y., Wang, H., Yan, H., Wang, X., Jin, Z., and Vladareanu, L. (2017). Research on Safety and Compliance of a New Lower Limb Rehabilitation Robot. J. Healthc. Eng. doi:10.1155/2017/1523068
Fischer, H. C., Triandafilou, K. M., Thielbar, K. O., Ochoa, J. M., Lazzaro, E. D. C., Pacholski, K. A., et al. (2016). Use of a Portable Assistive Glove to Facilitate Rehabilitation in Stroke Survivors with Severe Hand Impairment. IEEE Trans. Neural Syst. Rehabil. Eng. 24 (3), 344–351. doi:10.1109/tnsre.2015.2513675
Franck, J. A., Smeets, R. J. E. M., and Seelen, H. A. M. (2019). Evaluation of a Functional Hand Orthosis Combined with Electrical Stimulation Adjunct to Arm-Hand Rehabilitation in Subacute Stroke Patients with a Severely to Moderately Affected Hand Function. Disabil. Rehabil. 41 (10), 1160–1168. doi:10.1080/09638288.2017.1423400
Gama, A. E. F. D., Da Gama, A. E. F., Chaves, T. M., Figueiredo, L. S., Baltar, A., Meng, M., et al. (2016). MirrARbilitation: A Clinically-Related Gesture Recognition Interactive Tool for an AR Rehabilitation System. Comp. Methods Programs Biomed. 135, 105–114. doi:10.1016/j.cmpb.2016.07.014
Gasser, B. W., Bennett, D. A., Durrough, C. M., and Goldfarb, M. (2017). “Design and Preliminary Assessment of Vanderbilt Hand Exoskeleton,” in IEEE International Conference on Rehabilitation Robotics: [proceedings] , 1537–1542.
Ghassemi, M., Ochoa, J. M., Yuan, N., Tsoupikova, D., and Kamper, D. (2018). “Development of an Integrated Actuated Hand Orthosis and Virtual Reality System for Home-Based Rehabilitation,” in Conference Proceedings: IEEE Engineering in Medicine and Biology Society. Conference , 1689–1692. doi:10.1109/EMBC.2018.8512704
Godlove, J., Anantha, V., Advani, M., Des Roches, C., and Kiran, S. (2019). Comparison of Therapy Practice at Home and in the Clinic: A Retrospective Analysis of the Constant Therapy Platform Data Set. Front. Neurol. 10, 140. doi:10.3389/fneur.2019.00140
Gomez-Donoso, F., Orts-Escolano, S., Garcia-Garcia, A., Garcia-Rodriguez, J., Castro-Vargas, J. A., and Ovidiu-Oprea, S. (2017b). A Robotic Platform for Customized and Interactive Rehabilitation of Persons with Disabilities . Pattern Recognition Letters , 105–113.
Gresham, G. E., Duncan, P. W., Stason, W. B., Adams, H. P., Adelman, A. M., Alexander, D. N., et al. (1995). Post-stroke Rehabilitation: Clinical Practice Guidelines . Rockville: U.S. Department of Health and Human Services .
Guadagnoli, M. A., Dornier, L. A., and Tandy, R. D. (1996). Optimal Length for Summary Knowledge of Results: The Influence of Task-Related Experience and Complexity. Res. Q. Exerc. Sport 67 (2), 239–248. doi:10.1080/02701367.1996.10607950
Gull, M. A., Bai, S., and Bak, T. (2020). A Review on Design of Upper Limb Exoskeletons . Robotics .
Gupta, N., Castillo-Laborde, C., and Landry, M. D. (2011). Health-Related Rehabilitation Services: Assessing the Global Supply of and Need for Human Resources . BMC Health Services Research .
Hang Zhang, H., Austin, H., Buchanan, S., Herman, R., Koeneman, J., and Jiping He, J. (2011). Feasibility Studies of Robot-Assisted Stroke Rehabilitation at Clinic and Home Settings Using RUPERT. IEEE Int. Conf. Rehabil. Robot 2011, 5975440. doi:10.1109/ICORR.2011.5975440
Hatem, S. M., Saussez, G., Della Faille, M., Prist, V., Zhang, X., Dispa, D., et al. (2016). Rehabilitation of Motor Function after Stroke: A Multiple Systematic Review Focused on Techniques to Stimulate Upper Extremity Recovery. Front. Hum. Neurosci. 10, 442. doi:10.3389/fnhum.2016.00442
Heung, K. H. L., Tang, Z. Q., Ho, L., Tung, M., Li, Z., and Tong, R. K. Y. (2019). Design of a 3D Printed Soft Robotic Hand for Stroke Rehabilitation and Daily Activities Assistance. IEEE Int. Conf. Rehabil. Robot 2019, 65–70. doi:10.1109/ICORR.2019.8779449
Hidler, J., Nichols, D., Pelliccio, M., Brady, K., Campbell, D. D., Kahn, J. H., et al. (2009). Multicenter Randomized Clinical Trial Evaluating the Effectiveness of the Lokomat in Subacute Stroke. Neurorehabil. Neural Repair 23 (15–13), 5–13. doi:10.1177/1545968308326632
Hilty, D. M., Chan, S., Torous, J., Luo, J., and Boland, R. J. (2019). A Telehealth Framework for Mobile Health, Smartphones, and Apps: Competencies, Training, and Faculty Development. J. Tech. Behav. Sci. 4, 1. doi:10.1007/s41347-019-00091-0
Hirano, S., Saitoh, E., Tanabe, S., Tanikawa, H., Sasaki, S., Kato, D., et al. (2017). The Features of Gait Exercise Assist Robot: Precise Assist Control and Enriched Feedback. Nre 41 (1), 77–84. doi:10.3233/nre-171459
Honda Global (2020). Honda Walking Assist Device. https://global.honda/products/power/walkingassist.html (Accessed May 5, 2020).
Housley, S. N., Garlow, A. R., Ducote, K., Howard, A., Thomas, T., Wu, D., et al. (2016). Increasing Access to Cost Effective Home-Based Rehabilitation for Rural Veteran Stroke Survivors. Austin J. Cerebrovasc. Dis. Stroke 3 (21–11), 1–11. 28018979
PubMed Abstract | Google Scholar
Huang, J., Tu, X., and He, J. (2016). Design and Evaluation of the RUPERT Wearable Upper Extremity Exoskeleton Robot for Clinical and in-Home Therapies. IEEE Trans. Syst. Man. Cybern, Syst. 46 (7), 926–935. doi:10.1109/tsmc.2015.2497205
Hussain, S., Jamwal, P. K., Van Vliet, P., and Ghayesh, M. H. (2020). State-of-the-Art Robotic Devices for Wrist Rehabilitation: Design and Control Aspects. IEEE Trans. Human-Machine Syst. 99, 1–12. doi:10.1109/THMS.2020.2976905
Hyakutake, K., Morishita, T., Saita, K., Fukuda, H., Shiota, E., Higaki, Y., et al. (2019). Effects of Home-Based Robotic Therapy Involving the Single-Joint Hybrid Assistive Limb Robotic Suit in the Chronic Phase of Stroke: A Pilot Study. Biomed. Res. Int. 2019, 1–9. doi:10.1155/2019/5462694
Iida, S., Kawakita, D., Fujita, T., Uematsu, H., Kotaki, T., Ikeda, K., et al. (2017). Exercise Using a Robotic Knee Orthosis in Stroke Patients with Hemiplegia. J. Phys. Ther. Sci. 29, 1920–1924. doi:10.1589/jpts.29.1920
In, H., Kang, B. B., Sin, M., and Cho, K.-J. (2015). Exo-glove: A Wearable Robot for the Hand with a Soft Tendon Routing System. IEEE Robot. Automat. Mag. 22, 97–105. doi:10.1109/mra.2014.2362863
Intercollegiate Working Party for Stroke (2012). Stroke Association (Great Britain) and Royal College of Physicians of London, National Clinical Guideline for Stroke , 208.
Jamwal, P. K., Hussain, S., and Xie, S. Q. (2015). Review on Design and Control Aspects of Ankle Rehabilitation Robots. Disabil. Rehabil. Assistive Tech. 10 (2), 93–101. doi:10.3109/17483107.2013.866986
Jarrassé, N., Proietti, T., Crocher, V., Robertson, J., Sahbani, A., Morel, G., et al. (2014). Robotic Exoskeletons: A Perspective for the Rehabilitation of Arm Coordination in Stroke Patients. Front. Hum. Neurosci. 8, 947. doi:10.3389/fnhum.2014.00947
Je Hyung Jung, J. H., Valencia, D. B., Rodríguez-de-Pablo, C., Keller, T., and Perry, J. C. (2013). Development of a Powered Mobile Module for the ArmAssist Home-Based Telerehabilitation Platform. IEEE Int. Conf. Rehabil. Robot 2013, 6650424. doi:10.1109/ICORR.2013.6650424
Jian, H., Tu, X., and He, j. (2016). Design and Evaluation of the RUPERT Wearable Upper Extremity Exoskeleton Robot for Clinical and in-Home Therapies. IEEE Trans. Syst. Man, Cybernetics: Syst. 46 (7), 926–935. doi:10.1109/tsmc.2016.2645026
Kawamoto, H., Hayashi, T., Sakurai, T., Eguchi, K., and Sankai, Y. (2009). Development of Single Leg Version of HAL for Hemiplegia. Conf. Proc. IEEE Eng. Med. Biol. Soc. 2009, 5038–5043. doi:10.1109/IEMBS.2009.5333698
Kawamoto, H., Kamibayashi, K., Nakata, Y., Yamawaki, K., Ariyasu, R., Sankai, Y., et al. (2013). Pilot Study of Locomotion Improvement Using Hybrid Assistive Limb in Chronic Stroke Patients. BMC Neurol. 13, 141. doi:10.1186/1471-2377-13-141
Kim, B., and Deshpande, A. D. (2017). An Upper-Body Rehabilitation Exoskeleton Harmony with an Anatomical Shoulder Mechanism: Design, Modeling, Control, and Performance Evaluation. Int. J. Robotics Res. 36, 414–435. doi:10.1177/0278364917706743
Kim, T. (2020). Factors Influencing Usability of Rehabilitation Robotic Devices for Lower Limbs . Sustainability .
Kitago, T., and Krakauer, J. W. (2013). Motor Learning Principles for Neurorehabilitation. Neurol. Rehabil. 110, 93–103. doi:10.1016/B978-0-444-52901-5.00008-3
Klug, F., Hessinger, M., Koka, T., Witulla, P., Will, C., Schlichting, T., et al. (2019). An Anthropomorphic Soft Exosuit for Hand Rehabilitation. IEEE Int. Conf. Rehabil. Robot 2019, 1121–1126. doi:10.1109/ICORR.2019.8779481
Koh, T. H., Cheng, N., Yap, H. K., and Yeow, C.-H. (2017). Design of a Soft Robotic Elbow Sleeve with Passive and Intent-Controlled Actuation. Front. Neurosci. 11, 597. doi:10.3389/fnins.2017.00597
Kouris, I., Sarafidis, M., Androutsou, T., and Koutsouris, D. (2018). HOLOBALANCE: An Augmented Reality Virtual Trainer Solution Forbalance Training and Fall Prevention. Annu. Int. Conf. IEEE Eng. Med. Biol. Soc. 2018, 4233–4236. doi:10.1109/EMBC.2018.8513357
Krakauer, J. W. (2006). Motor Learning: Its Relevance to Stroke Recovery and Neurorehabilitation. Curr. Opin. Neurol. 19 (1), 84–90. doi:10.1097/01.wco.0000200544.29915.cc
Kruif, B. J., Schmidhauser, E., Stadler, K. S., and O’Sullivan, L. W. (2017). Simulation Architecture for Modelling Interaction between User and Elbow-Articulated Exoskeleton. J. Bionic Eng. 14, 706–715. doi:10.1016/S1672-6529(16)60437-7
Kutlu, M., Freeman, C., Hughes, A.-M., and Spraggs, M. (2017). A Home-Based FES System for Upper-Limb Stroke Rehabilitation with Iterative Learning Control. IFAC-PapersOnLine 50, 12089–12094. doi:10.1016/j.ifacol.2017.08.2153
Lambelet, C., Temiraliuly, D., Siegenthaler, M., Wirth, M., Woolley, D. G., Lambercy, O., et al. (2020). Characterization and Wearability Evaluation of a Fully Portable Wrist Exoskeleton for Unsupervised Training after Stroke. J. Neuroengineering Rehabil. 17 (1), 132. doi:10.1186/s12984-020-00749-4
Lee, H.-J., Lee, S.-H., Seo, K., Lee, M., Chang, W. H., Choi, B.-O., et al. (2019). Training for Walking Efficiency with a Wearable Hip-Assist Robot in Patients with Stroke. Stroke 50 (12), 3545–3552. doi:10.1161/strokeaha.119.025950
Lee, S.-H., Lee, H.-J., Shim, Y., Chang, W. H., Choi, B.-O., Ryu, G.-H., et al. (2020). Wearable Hip-Assist Robot Modulates Cortical Activation during Gait in Stroke Patients: A Functional Near-Infrared Spectroscopy Study. J. Neuroengineering Rehabil. 17 (1), 145. doi:10.1186/s12984-020-00777-0
Lin, C.-T., and Lee, C. S. G. (1991). Neural-Network-Based Fuzzy Logic Control and Decision System . IEEE Transactions on Computers .
Linder, S. M., Reiss, A., Buchanan, S., Sahu, K., Rosenfeldt, A. B., Clark, C., et al. (2013a). Incorporating Robotic-Assisted Telerehabilitation in a Home Program to Improve Arm Function Following Stroke. J. Neurol. Phys. Ther. 37, 125–132. doi:10.1097/NPT.0b013e31829fa808
Linder, S. M., Rosenfeldt, A. B., Reiss, A., Buchanan, S., Sahu, K., Bay, C. R., et al. (2013b). The Home Stroke Rehabilitation and Monitoring System Trial: A Randomized Controlled Trial. Int. J. Stroke 8, 46–53. doi:10.1111/j.1747-4949.2012.00971.x
Liu, Y., Guo, S., Hirata, H., Ishihara, H., and Tamiya, T. (2018). Development of a Powered Variable-Stiffness Exoskeleton Device for Elbow Rehabilitation. Biomed. Microdevices 20 (3), 64. doi:10.1007/s10544-018-0312-6
Liu, Y., Guo, S., Yang, Z., Hirata, H., and Tamiya, T. (2021). A Home-Based Bilateral Rehabilitation System with sEMG-Based Real-Time Variable Stiffness. IEEE J. Biomed. Health Inform. 25, 1529–1541. doi:10.1109/JBHI.2020.3027303,
Low, F.-Z., Lim, J. H., Kapur, J., and Yeow, R. C.-H. (2019). Effect of a Soft Robotic Sock Device on Lower Extremity Rehabilitation Following Stroke: A Preliminary Clinical Study with Focus on Deep Vein Thrombosis Prevention. IEEE J. Transl. Eng. Health Med. 7, 1–6. doi:10.1109/JTEHM.2019.2894753
Low, F.-Z., Lim, J. H., and Yeow, C.-H. (2018). Design, Characterisation and Evaluation of a Soft Robotic Sock Device on Healthy Subjects for Assisted Ankle Rehabilitation. J. Med. Eng. Tech. 42 (1), 26–34. doi:10.1080/03091902.2017.1411985
Lyu, M., Chen, W.-H., Ding, X., Wang, J., Pei, Z., and Zhang, B. (2019). Development of an EMG-Controlled Knee Exoskeleton to Assist Home Rehabilitation in a Game Context. Front. Neurorobotics 13, 67. doi:10.3389/fnbot.2019.00067
Markus, H. S., and Brainin, M. (2020). COVID-19 and Stroke-A Global World Stroke Organization Perspective. Int. J. Stroke 15 (4), 361–364. doi:10.1177/1747493020923472
Martinez-Martin, E., and Cazorla, M. (2019). Rehabilitation Technology: Assistance from Hospital to Home. Comput. Intell. Neurosci. 2019, 1431509. doi:10.1155/2019/1431509
Mehrholz, J., Thomas, S., Kugler, J., Pohl, M., and Elsner, B. (2020). Electromechanical-Assisted Training for Walking after Stroke. Cochrane Database Syst. Rev. 10, CD006185. doi:10.1002/14651858.CD006185.pub5
Mizukami, N., Takeuchi, S., Tetsuya, M., Tsukahara, A., Yoshida, K., Matsushima, A., et al. (2018). Effect of the Synchronization-Based Control of a Wearable Robot Having a Non-exoskeletal Structure on the Hemiplegic Gait of Stroke Patients. IEEE Trans. Neural Syst. Rehabil. Eng. 26 (5), 1011–1016. doi:10.1109/tnsre.2018.2817647
Moon, S. B., Ji, Y.-H., Jang, H.-Y., Hwang, S.-H., Shin, D.-B., Lee, S.-C., et al. (2017). Gait Analysis of Hemiplegic Patients in Ambulatory Rehabilitation Training Using a Wearable Lower-Limb Robot: A Pilot Study . International Journal of Precision Engineering and Manufacturing .
Morone, G., Annicchiarico, R., Iosa, M., Federici, A., Paolucci, S., Cortés, U., et al. (2016). Overground Walking Training with the I-Walker, a Robotic Servo-Assistive Device, Enhances Balance in Patients with Subacute Stroke: A Randomized Controlled Trial. J. Neuroengineering Rehabil. 13 (1), 47. doi:10.1186/s12984-016-0155-4
Motus Nova (n.d.). Motus Nova Stroke Rehab Recovery at Home. https://motusnova.com/hand (Accessed April 10, 2021).
National University of Singapore (2015). Robotic Sock Designed to Prevent Blood Clots .
Nijenhuis, S. M., Prange, G. B., Amirabdollahian, F., Sale, P., Infarinato, F., Nasr, N., et al. (2015). Feasibility Study into Self-Administered Training at Home Using an Arm and Hand Device with Motivational Gaming Environment in Chronic Stroke. J. Neuroengineering Rehabil. 12, 89. doi:10.1186/s12984-015-0080-y
Nilsson, A., Vreede, K. S., Häglund, V., Kawamoto, H., Sankai, Y., and Borg, J. (2014). Gait Training Early after Stroke with a New Exoskeleton – the Hybrid Assistive Limb: a Study of Safety and Feasibility. J. Neuroengineering Rehabil. 11, 92. doi:10.1186/1743-0003-11-92
Nilsson, M., Ingvast, J., Wikander, J., and von Holst, H. (2012). “The Soft Extra Muscle System for Improving the Grasping Capability in Neurological Rehabilitation,” in 2012 IEEE-EMBS Conference on Biomedical Engineering and Sciences . doi:10.1109/iecbes.2012.6498090
Novak, D., Ziherl, J., Olenšek, A., Milavec, M., Podobnik, J., Mihelj, M., et al. (2010). Psychophysiological Responses to Robotic Rehabilitation Tasks in Stroke. IEEE Trans. Neural Syst. Rehabil. Eng. 18 (4), 351–361. doi:10.1109/tnsre.2010.2047656
Osuagwu, B. A. C., Timms, S., Peachment, R., Dowie, S., Thrussell, H., Cross, S., et al. (2020). Home-based Rehabilitation Using a Soft Robotic Hand Glove Device Leads to Improvement in Hand Function in People with Chronic Spinal Cord Injury:a Pilot Study. J. Neuroengineering Rehabil. 17 (1), 40. doi:10.1186/s12984-020-00660-y
Pehlivan, A. U., Celik, O., and O'Malley, M. K. (2011). Mechanical Design of a Distal Arm Exoskeleton for Stroke and Spinal Cord Injury Rehabilitation. IEEE Int. Conf. Rehabil. Robot 2011, 5975428. doi:10.1109/ICORR.2011.5975428
Pereira, A., Folgado, D., Nunes, F., Almeida, J., and Sousa, I. (2019). “Using Inertial Sensors to Evaluate Exercise Correctness in Electromyography-Based Home Rehabilitation Systems,” in 2019 IEEE International Symposium on Medical Measurements and Applications (MeMeA) . doi:10.1109/memea.2019.8802152
Perry, J. C., Trimble, S., Castilho Machado, L. G., Schroeder, J. S., Belloso, A., Rodriguez-de-Pablo, C., et al. (2016). Design of a Spring-Assisted Exoskeleton Module for Wrist and Hand Rehabilitation. Annu. Int. Conf. IEEE Eng. Med. Biol. Soc. 2016, 594–597. doi:10.1109/EMBC.2016.7590772
Perry, J. C., Ruiz-Ruano, J. A., and Keller, T. (2011). Telerehabilitation: Toward a Cost-Efficient Platform for Post-Stroke Neurorehabilitation. IEEE Int. Conf. Rehabil. Robotics 2011. doi:10.1109/icorr.2011.5975413
Perry, J. C., Zabaleta, H., Belloso, A., Rodriguez-de-Pablo, C., Cavallaro, F. I., and Keller, T. (2012). “ArmAssist: Development of a Functional Prototype for at-Home Telerehabilitation of Post-Stroke Arm Impairment,” in 2012 4th IEEE RAS & EMBS International Conference on Biomedical Robotics and Biomechatronics (BioRob) . doi:10.1109/biorob.2012.6290858
Pignolo, L., Dolce, G., Basta, G., Lucca, L. F., Serra, S., and Sannita, W. G. (2012). “Upper Limb Rehabilitation after Stroke: ARAMIS a “Robo-mechatronic” Innovative Approach and Prototype,” in 2012 4th IEEE RAS & EMBS International Conference on Biomedical Robotics and Biomechatronics (BioRob) . doi:10.1109/biorob.2012.6290868
Polygerinos, P., Galloway, K. C., Sanan, S., Herman, M., and Walsh, C. J. (2015). EMG Controlled Soft Robotic Glove for Assistance during Activities of Daily Living . Robotics and Autonomous Systems . doi:10.1109/icorr.2015.7281175
Porciuncula, F., Roto, A. V., Kumar, D., Davis, I., Roy, S., Walsh, C. J., et al. (2018). Wearable Movement Sensors for Rehabilitation: A Focused Review of Technological and Clinical Advances. PM&R 10, S220–S232. doi:10.1016/j.pmrj.2018.06.013
Prange-Lasonder, G. B., Radder, B., Kottink, A. I. R., Melendez-Calderon, A., Buurke, J. H., and Rietman, J. S. (2017). Applying a Soft-Robotic Glove as Assistive Device and Training Tool with Games to Support Hand Function after Stroke: Preliminary Results on Feasibility and Potential Clinical Impact. IEEE Int. Conf. Rehabil. Robot 2017, 1401–1406. doi:10.1109/ICORR.2017.8009444
Proietti, T., Crocher, V., Roby-Brami, A., and Jarrasse, N. (2016). Upper-Limb Robotic Exoskeletons for Neurorehabilitation: A Review on Control Strategies. IEEE Rev. Biomed. Eng. 9, 4–14. doi:10.1109/rbme.2016.2552201
Proulx, C. E., Beaulac, M., David, M., Deguire, C., Haché, C., Klug, F., et al. (2020). Review of the Effects of Soft Robotic Gloves for Activity-Based Rehabilitation in Individuals with Reduced Hand Function and Manual Dexterity Following a Neurological Event. J. Rehabil. Assistive Tech. Eng. 7, 205566832091813. doi:10.1177/2055668320918130
Radder, B., Prange-Lasonder, G. B., Kottink, A. I. R., Holmberg, J., Sletta, K., Dijk, M. V., et al. (2019). Home Rehabilitation Supported by a Wearable Soft-Robotic Device for Improving Hand Function in Older Adults: A Pilot Randomized Controlled Trial. PLOS ONE 14, e0220544. doi:10.1371/journal.pone.0220544
Radder, B., Prange-Lasonder, G., Kottink, A., Melendez-Calderon, A., Buurke, J., and Rietman, J. (2018). Feasibility of a Wearable Soft-Robotic Glove to Support Impaired Hand Function in Stroke Patients. J. Rehabil. Med. 50 (7), 598–606. doi:10.2340/16501977-2357
Recover From Your Stroke With Saebo (2017). https://www.saebo.com (Accessed May 5, 2021).
Ren, Y., Wu, Y.-N., Yang, C.-Y., Xu, T., Harvey, R. L., and Zhang, L.-Q. (2017). Developing a Wearable Ankle Rehabilitation Robotic Device for In-Bed Acute Stroke Rehabilitation. IEEE Trans. Neural Syst. Rehabil. Eng. 25 (6), 589–596. doi:10.1109/tnsre.2016.2584003
ReWalk (2019). ReStore TM Soft Exo-Suit for Stroke Rehabilitation - ReWalk Robotics. https://rewalk.com/restore-exo-suit/ (Accessed May 5, 2021).
ReWalk (2015). ReWalk TM Personal 6.0 Exoskeleton. https://rewalk.com/rewalk-personal-3/ (Accessed May 5, 2021).
Rose, F. D., David Rose, F., Brooks, B. M., and Rizzo, A. A. (2005). Virtual Reality in Brain Damage Rehabilitation: Review . CyberPsychology & Behavior .
Runnalls, K. D., Ortega-Auriol, P., McMorland, A. J. C., Anson, G., and Byblow, W. D. (2019). Effects of Arm Weight Support on Neuromuscular Activation during Reaching in Chronic Stroke Patients. Exp. Brain Res. 237 (12), 3391–3408. doi:10.1007/s00221-019-05687-9
Sahu, M. L., Atulkar, M., and Ahirwal, M. K. (2020). “Comprehensive Investigation on IoT Based Smart HealthCare System,” in 2020 First International Conference on Power, Control and Computing Technologies (ICPC2T) . doi:10.1109/icpc2t48082.2020.9071442
Sandison, M., Phan, K., Casas, R., Nguyen, L., Lum, M., Pergami-Peries, M., et al. (2020). HandMATE: Wearable Robotic Hand Exoskeleton and Integrated Android App for at Home Stroke Rehabilitation. Annu. Int. Conf. IEEE Eng. Med. Biol. Soc. 2020, 4867–4872. doi:10.1109/EMBC44109.2020.9175332
Sanjuan, J. D., Castillo, A. D., Padilla, M. A., Quintero, M. C., Gutierrez, E. E., Sampayo, I. P., et al. (2020). Cable Driven Exoskeleton for Upper-Limb Rehabilitation: A Design Review . Robotics and Autonomous Systems .
Schmidt, R. A., and Lee, T. D. (1999). Motor Control and Learning: A Behavioral Emphasis . Human Kinetics Publishers , 495.
Shen, Y., Ferguson, P. W., and Rosen, J. (2020). Upper Limb Exoskeleton Systems—Overview . Wearable Robotics .
Shi, D., Zhang, W., Zhang, W., and Ding, X. (2019). A Review on Lower Limb Rehabilitation Exoskeleton Robots. Chin. J. Mech. Eng. 32, 74. doi:10.1186/s10033-019-0389-8
Simonetti, D., Tagliamonte, N. L., Zollo, L., Accoto, D., and Guglielmelli, E. (2018). Biomechatronic Design Criteria of Systems for Robot-Mediated Rehabilitation Therapy . Rehabilitation Robotics , 29–46. doi:10.1016/b978-0-12-811995-2.00032-1
Sivan, M., Gallagher, J., Makower, S., Keeling, D., Bhakta, B., O’Connor, R. J., et al. (2014). Home-based Computer Assisted Arm Rehabilitation (hCAAR) Robotic Device for Upper Limb Exercise after Stroke: Results of a Feasibility Study in Home Setting. J. NeuroEngineering Rehabil. 11, 163. doi:10.1186/1743-0003-11-163
Solanki, D., Kumar, S., Shubha, B., and Lahiri, U. (2020). Implications of Physiology-Sensitive Gait Exercise on the Lower Limb Electromyographic Activity of Hemiplegic Post-Stroke Patients: A Feasibility Study in Low Resource Settings. IEEE J. Transl. Eng. Health Med. 8, 12100709–9. doi:10.1109/JTEHM.2020.3006181
Stavropoulos, T. G., Papastergiou, A., Mpaltadoros, L., Nikolopoulos, S., and Kompatsiaris, I. (2020). IoT Wearable Sensors and Devices in Elderly Care: A Literature Review. Sensors 20, 2826. doi:10.3390/s20102826
Stein, J., Bishop, L., Stein, D. J., and Wong, C. K. (2014). Gait Training with a Robotic Leg Brace after Stroke. Am. J. Phys. Med. Rehabil./ Assoc. Acad. Physiatrists 93 (11), 987–994. doi:10.1097/phm.0000000000000119
Su, C.-J., Chiang, C.-Y., and Huang, J.-Y. (2014). Kinect-Enabled Home-Based Rehabilitation System Using Dynamic Time Warping and Fuzzy Logic . Applied Soft Computing .
Sung, J., Choi, S., Kim, H., Lee, G., Han, C., Ji, Y., et al. (2017). Feasibility of Rehabilitation Training with a Newly Developed, Portable, Gait Assistive Robot for Balance Function in Hemiplegic Patients. Ann. Rehabil. Med. 41 (2), 178–187. doi:10.5535/arm.2017.41.2.178
Tekin, O. A., Babuska, R., Tomiyama, T., De Schutter, B., and De Schutter, B. (2009). “Toward a Flexible Control Design Framework to Automatically Generate Control Code for Mechatronic Systems,” in 2009 American Control Conference . doi:10.1109/acc.2009.5160184
Tomić, T. J. D., Savić, A. M., Vidaković, A. S., Rodić, S. Z., Isaković, M. S., Rodríguez-de-Pablo, C., et al. (2017). ArmAssist Robotic System versus Matched Conventional Therapy for Poststroke Upper Limb Rehabilitation: A Randomized Clinical Trial. Biomed. Res. Int. 2017, 1–7. doi:10.1155/2017/7659893
Tomida, K., Sonoda, S., Hirano, S., Suzuki, A., Tanino, G., Kawakami, K., et al. (2019). Randomized Controlled Trial of Gait Training Using Gait Exercise Assist Robot (GEAR) in Stroke Patients with Hemiplegia. J. Stroke Cerebrovasc. Dis. 28 (9), 2421–2428. doi:10.1016/j.jstrokecerebrovasdis.2019.06.030
Triandafilou, K. M. (2014). Effect of Static versus Cyclical Stretch on Hand Motor Control in Subacute Stroke . International Journal of Neurorehabilitation .
Tsiouris, K. M., Gatsios, D., Tsakanikas, V., Pardalis, A. A., Kouris, I., Androutsou, T., et al. (2020). Designing Interoperable Telehealth Platforms: Bridging IoT Devices with Cloud Infrastructures . Enterprise Information Systems . doi:10.1109/embc44109.2020.9176082
Tsukahara, A., and Hashimoto, M. (2016). “Pilot Study of Single-Legged Walking Support Using Wearable Robot Based on Synchronization Control for Stroke Patients,” in 2016 IEEE International Conference on Robotics and Biomimetics (ROBIO) . doi:10.1109/robio.2016.7866436
Tsukahara, A., Yoshida, K., Matsushima, A., Ajima, K., Kuroda, C., Mizukami, N., et al. (2017). Evaluation of Walking Smoothness Using Wearable Robotic System Curara for Spinocerebellar Degeneration Patients. IEEE Int. Conf. Rehabil. Robot 2017, 1494–1499. doi:10.1109/ICORR.2017.8009459
Tu, X., Han, H., Huang, J., Li, J., Su, C., Jiang, X., et al. (2017). Upper Limb Rehabilitation Robot Powered by PAMs Cooperates with FES Arrays to Realize Reach-To-Grasp Trainings. J. Healthc. Eng. 2017. doi:10.1155/2017/1282934
Tun, S. Y. Y., Madanian, S., and Mirza, F. (2020). Internet of Things (IoT) Applications for Elderly Care: A Reflective Review. Aging Clin. Exp. Res. 33, 855–867. doi:10.1007/s40520-020-01545-9
Van der Loos, H. F. M., and Reinkensmeyer, D. J. (2008). “Rehabilitation and Health Care Robotics,” in Springer Handbook of Robotics (Berlin, Heidelberg: Springer Berlin Heidelberg ), 1223–1251. doi:10.1007/978-3-540-30301-5_54
van Kammen, K., Boonstra, A. M., van der Woude, L. H. V., Reinders-Messelink, H. A., and den Otter, R. (2017). Differences in Muscle Activity and Temporal Step Parameters between Lokomat Guided Walking and Treadmill Walking in Post-Stroke Hemiparetic Patients and Healthy Walkers. J. Neuroengineering Rehabil. 14 (1), 32. doi:10.1186/s12984-017-0244-z
Wai, C. C., Leong, T. C., Gujral, M., Hung, J., Hui, T. S., and Wen, K. K. (2018). “Ambidexter: A Low Cost Portable Home-Based Robotic Rehabilitation Device for Training Fine Motor Skills,” in 2018 7th IEEE International Conference on Biomedical Robotics and Biomechatronics (Biorob) . doi:10.1109/biorob.2018.8487204
Wang, J., Qiu, M., and Guo, B. (2017). Enabling Real-Time Information Service on Telehealth System over Cloud-Based Big Data Platform. J. Syst. Architecture 72. doi:10.1016/j.sysarc.2016.05.003
Wang, Y., and Xu, Q. (2019). “Design of a New Wrist Rehabilitation Robot Based on Soft Fluidic Muscle,” in IEEE/ASME International Conference on Advanced Intelligent Mechatronics (AIM) . doi:10.1109/aim.2019.8868626
Wanjoo Park, W., Wookjin Jeong, W., Gyu-Hyun Kwon, G. H., Yun-Hee Kim, Y. H., and Laehyun Kim, L. (2013). A Rehabilitation Device to Improve the Hand Grasp Function of Stroke Patients Using a Patient-Driven Approach. IEEE Int. Conf. Rehabil. Robot 2013, 6650482. doi:10.1109/ICORR.2013.6650482
Washabaugh, E. P., Claflin, E. S., Gillespie, R. B., and Krishnan, C. (2016). A Novel Application of Eddy Current Braking for Functional Strength Training during Gait. Ann. Biomed. Eng. 44 (9), 2760–2773. doi:10.1007/s10439-016-1553-2
Washabaugh, E. P., Guo, J., Chang, C.-K., Remy, C. D., and Krishnan, C. (2019). A Portable Passive Rehabilitation Robot for Upper-Extremity Functional Resistance Training. IEEE Trans. Biomed. Eng. 66 (2), 496–508. doi:10.1109/tbme.2018.2849580
Washabaugh, E. P., and Krishnan, C. (2018). A Wearable Resistive Robot Facilitates Locomotor Adaptations during Gait. Rnn 36 (2), 215–223. doi:10.3233/rnn-170782
Westerveld, A. J., Aalderink, B. J., Hagedoorn, W., Buijze, M., Schouten, A. C., and Kooij, H. v. d. (2014). A Damper Driven Robotic End-Point Manipulator for Functional Rehabilitation Exercises after Stroke. IEEE Trans. Biomed. Eng. 61 (10), 2646–2654. doi:10.1109/tbme.2014.2325532
Wolf, S. L., Sahu, K., Curtis Bay, R., Buchanan, S., Reiss, A., Linder, S., et al. (2015). The HAAPI (Home Arm Assistance Progression Initiative) Trial. Neurorehabil. Neural Repair 29 (10), 958–968. doi:10.1177/1545968315575612
World Health Organization (2017). The Need to Scale up Rehabilitation . World Health Organization . WHO/NMH/NVI/17.1.
Wright, A., Stone, K., Martinelli, L., Fryer, S., Smith, G., Lambrick, D., et al. (2020). Effect of Combined Home-Based, Overground Robotic-Assisted Gait Training and Usual Physiotherapy on Clinical Functional Outcomes in People with Chronic Stroke: A Randomized Controlled Trial. Clin. Rehabil. 2020, 026921552098413. doi:10.1177/0269215520984133
Yoo, D., Kim, D.-H., Seo, K.-H., and Lee, B.-C. (2019). The Effects of Technology-Assisted Ankle Rehabilitation on Balance Control in Stroke Survivors. IEEE Trans. Neural Syst. Rehabil. Eng. 27 (9), 1817–1823. doi:10.1109/tnsre.2019.2934930
Yurkewich, A., Hebert, D., Wang, R. H., and Mihailidis, A. (2019). Hand Extension Robot Orthosis (HERO) Glove: Development and Testing with Stroke Survivors with Severe Hand Impairment. IEEE Trans. Neural Syst. Rehabil. Eng. 27 (5), 916–926. doi:10.1109/tnsre.2019.2910011
Yurkewich, A., Kozak, I. J., Ivanovic, A., Rossos, D., Wang, R. H., Hebert, D., et al. (2020). Myoelectric Untethered Robotic Glove Enhances Hand Function and Performance on Daily Living Tasks after Stroke. J. Rehabil. Assistive Tech. Eng. 7, 205566832096405. doi:10.1177/2055668320964050
Yıldırım, Ş. (2008). A Proposed Hybrid Neural Network for Position Control of a Walking Robot . Nonlinear Dynamics .
Zhang, K., Chen, X., Liu, F., Tang, H., Wang, J., and Wen, W. (2018). System Framework of Robotics in Upper Limb Rehabilitation on Poststroke Motor Recovery. Behav. Neurol. 2018, 1–14. doi:10.1155/2018/6737056
Zhang, M., Guo, Y., and He, M. (2019). “Dynamic Analysis of Lower Extremity Exoskeleton of Rehabilitation Robot,” in Proceedings of the 2019 4th International Conference on Robotics, Control and Automation - ICRCA 2019 . doi:10.1145/3351180.3351207
Zheng, H., Black, N. D., and Harris, N. D. (2005). Position-Sensing Technologies for Movement Analysis in Stroke Rehabilitation. Med. Biol. Eng. Comput. 43 (4), 413–420. doi:10.1007/bf02344720
Zhou, Y., Desplenter, T., Chinchalkar, S., and Trejos, A. L. (2019). A Wearable Mechatronic Glove for Resistive Hand Therapy Exercises. IEEE Int. Conf. Rehabil. Robot 2019, 1097–1102. doi:10.1109/ICORR.2019.8779502
MAL Motor activity log
MAL QOM Motor activity log (quality of movement)
BBS Berg balance scale
FMAS Fugl-Meyer assessment scale
FMUE or FMA-UE The Fugl-Meyer assessment for motor recovery after stroke for the upper extremity
FMA-Hand The Fugl-Meyer assessment for hand
FMLE or FM-LE The Fugl-Meyer assessment for motor recovery after stroke for the lower extremity
FM Fugl-Meyer assessment of motor recovery
TUGT Timed up and go test
SPPB Short physical performance battery
DGI Dynamic Gait index
mRS Modified Rankin scale
CMSA-H Chedoke McMaster stroke assessment stage
FES(S) Falls-efficacy scale Swedish version
BI Barthel index
FIM Functional independence measure
ARAT Action research arm test
WMFT Wolf motor function test
GWMFT or GWMFT-func The graded wolf motor function test
GWMFT-time Graded wolf motor function test (completion time)
CAHAI The Chedoke arm and hand inventory
MAS The motor assessment scale
MMAS The modified Modified ashworth scale
EXT Finger extension force
GS Grip strength
LPS Lateral pinch strength
PPS Palmar pinch strength
MTS Modified Tardieu scale
SIS Stroke impact scale
SUS System usability scale
BBT Box and blocks test
JTHFT Jebsen-Taylor hand function test
10MWT 10 minute walk test
6MWT 6 minute walk test
K-FES Korean falls-efficiency scale
FE Flexion/Extension
E Extension
PS Pronation/Supination
IE Inversion/Eversion
PD Plantarflexion/Dorsiflexion
Keywords: home based rehabilitation, stroke rehabilitaiton, COVID 19 pandemic, conceptual framework, rehabilitation robotics
Citation: Akbari A, Haghverd F and Behbahani S (2021) Robotic Home-Based Rehabilitation Systems Design: From a Literature Review to a Conceptual Framework for Community-Based Remote Therapy During COVID-19 Pandemic. Front. Robot. AI 8:612331. doi: 10.3389/frobt.2021.612331
Received: 30 September 2020; Accepted: 01 June 2021; Published: 22 June 2021.
Reviewed by:
Copyright © 2021 Akbari, Haghverd and Behbahani. This is an open-access article distributed under the terms of the Creative Commons Attribution License (CC BY). The use, distribution or reproduction in other forums is permitted, provided the original author(s) and the copyright owner(s) are credited and that the original publication in this journal is cited, in accordance with accepted academic practice. No use, distribution or reproduction is permitted which does not comply with these terms.
*Correspondence: Saeed Behbahani, [email protected]
† These authors have contributed equally to this work and share first authorship
This article is part of the Research Topic
Robotics, Autonomous Systems and AI for Nonurgent/Nonemergent Healthcare Delivery During and After the COVID-19 Pandemic
- Systematic Review
- Open access
- Published: 08 May 2023
Overview of the role of robots in upper limb disabilities rehabilitation: a scoping review
- Khadijeh Moulaei 1 ,
- Kambiz Bahaadinbeigy 1 ,
- Ali Akbar Haghdoostd 2 ,
- Mansour Shahabi Nezhad 3 &
- Abbas Sheikhtaheri 4
Archives of Public Health volume 81 , Article number: 84 ( 2023 ) Cite this article
3535 Accesses
4 Citations
4 Altmetric
Metrics details
Neuromotor rehabilitation and improvement of upper limb functions are necessary to improve the life quality of patients who have experienced injuries or have pathological outcomes. Modern approaches, such as robotic-assisted rehabilitation can help to improve rehabilitation processes and thus improve upper limb functions. Therefore, the aim of this study was to investigate the role of robots in upper limb disability improvement and rehabilitation.
This scoping review was conducted by search in PubMed, Web of Science, Scopus, and IEEE (January 2012– February 2022). Articles related to upper limb rehabilitation robots were selected. The methodological quality of all the included studies will be appraised using the Mixed Methods Appraisal Tool (MMAT). We used an 18-field data extraction form to extract data from articles and extracted the information such as study year, country, type of study, purpose, illness or accident leading to disability, level of disability, assistive technologies, number of participants in the study, sex, age, rehabilitated part of the upper limb using a robot, duration and frequency of treatment, methods of performing rehabilitation exercises, type of evaluation, number of participants in the evaluation process, duration of intervention, study outcomes, and study conclusions. The selection of articles and data extraction was made by three authors based on inclusion and exclusion criteria. Disagreements were resolved through consultation with the fifth author. Inclusion criteria were articles involving upper limb rehabilitation robots, articles about upper limb disability caused by any illness or injury, and articles published in English. Also, articles involving other than upper limb rehabilitation robots, robots related to rehabilitation of diseases other than upper limb, systematic reviews, reviews, and meta-analyses, books, book chapters, letters to the editor, and conference papers were also excluded. Descriptive statistics methods (frequency and percentage) were used to analyses the data.
We finally included 55 relevant articles. Most of the studies were done in Italy (33.82%). Most robots were used to rehabilitate stroke patients (80%). About 60.52% of the studies used games and virtual reality rehabilitate the upper limb disabilities using robots. Among the 14 types of applied evaluation methods, “evaluation and measurement of upper limb function and dexterity” was the most applied evaluation method. “Improvement in musculoskeletal functions”, “no adverse effect on patients”, and “Safe and reliable treatment” were the most cited outcomes, respectively.
Conclusions
Our findings show that robots can improve musculoskeletal functions (musculoskeletal strength, sensation, perception, vibration, muscle coordination, less spasticity, flexibility, and range of motion) and empower people by providing a variety of rehabilitation capabilities.
Peer Review reports
Introduction
Impaired upper limb (UL) functions to restrict the performance of activities of daily living, limit social participation [ 1 ], specifically decrease the independence of affected individuals and reduce patients’ quality of life [ 2 , 3 ]. In upper limb disabilities, patients develop musculoskeletal problems such as paresis, pain, loss of sensation, and spasticity in different parts of the upper limb and so these problems can have manifold consequences in the daily lives of those impacted. These include a decreased capacity to carry out primary self-care tasks and to accomplish life-roles, which can affect emotional, mental, and psychological wellbeing [ 4 ]. Patients with upper limb disabilities need rehabilitation to improve their musculoskeletal status [ 5 ].
Neuromotor rehabilitation and recovery of upper extremity functions are necessary to improve the life quality of individuals who have suffered injuries, disabilities or have pathological outcomes, where it is favorable to raise the development of activities of daily living [ 6 ]. However, the conventional rehabilitations done by one manual-assisted movement training created by physiotherapists suffers from a lot of inherent restrictions, such as heavy labor severity, long-time consumption, lack of repeatability, low patients participation, and their low motivation to perform rehabilitation exercises [ 7 ]. Also, in conventional therapy, the accessibility of therapists, the duration of therapy sessions, and the high cost of rehabilitation tools are all considered parameters that impact on both therapists and patients [ 8 ].
New therapeutic methods have been presented to rehabilitate and improve upper limb function, and such methods are robotic rehabilitation [ 1 ]. Severe repetitions of harmonized motor activities by robots establish an important burden for the therapists who help patients. Moreover and due to economic reasons, the duration of preliminary rehabilitation is getting shorter and shorter [ 9 ]. However, some studies have pointed out that repetitive and high-severity exercises can specifically contribute to the functional recovery of the affected upper limb movement [ 10 ]. Rehabilitation robots are able of decreasing the burden on therapists by replace human intervention and preparing desire therapies that accomplish the following primary principles of upper limb rehabilitation: iteration, high severity, and task particularly [ 11 ]. Moreover, the functional impetus of a patient can be activated to raise strenuous participation and improve cognitive functions. The physical factors and treatment data can be stored and analyzed by the sensing system, and that can provide a realistic basis for optimization training technique and speed up the recovery process [ 7 ]. In addition, major boost has been given by the extension of such devices to clinical care medical and rehabilitation centers [ 1 , 2 ].
Therefore, multiple benefits can be considered for rehabilitation robots : they can produce high-quality repetitive movements and increase rehabilitation strength and intensity; they can offer many types of exercises for therapists to choose from; they supply a man-machine interaction that allows for objective measurement of advancement, which itself can status modifications in interaction by changing control parameters [ 12 ]. On the other hand, task-oriented training is one of the other capabilities of rehabilitation robots, which is known as the dominant and most effective method for motor rehabilitation of upper limb function [ 13 ]. Task-based approaches in which the patient is assisted to perform a specific prescribed movement, such as lifting an object with the hand, show promising results compared to conventional exercises based on passive movement of the impaired joints in the restriction of their range of motion [ 14 ].
Robots can also be used in homes, clinics and medical centers. Patients can do the rehabilitation exercises prescribed by the therapist with the help of the robot at home without having to visit the treatment center frequently. Successful robot-assisted rehabilitation at home can facilitate intensive therapy, facilitate in-person or virtual therapy visits, preferably at low cost, and motivate patients to participate in supervised or unsupervised therapeutic activities at necessary levels for motor learning and generalization to occur [ 15 ]. Moreover, in clinics, rehabilitation robots can decrease the burden of therapists by automating tedious and labor-intensive treatment and by adapting to the particularized needs of targeted individuals [ 16 ]. For example, the hand robot skeleton designed by Wege et al., [ 17 ] can move patients’ fingers skillfully and repeatedly compared to a tired therapist training patients with manual labor.
To our knowledge, no systematic or scoping review has been performed on robots to rehabilitate upper limb disabilities. Only a few systematic reviews have been done in the areas of classification of interactive wearable systems for monitoring body movement and posture during upper body rehabilitation, estimating the wearability of the wearable devices [ 18 ], presenting state of the art in sensor fusion applied in applications for hand rehabilitation [ 19 ], evaluating the role of serious games in upper limb rehabilitation, identifying common procedures and exercises as well as technology patterns [ 20 ], evaluating the effectiveness of upper limb wearable technology to improve activity and engagement in stroke survivors [ 21 ] and determining the effects of robot-assisted treatment on motor-functional improvement in stroke patients [ 22 ]. So, the aim of the present study was to investigate the role of robots in upper limb disability improvement and rehabilitation. In this study, we identify the most common diseases or complications leading to upper limb disability for which robots have been used for rehabilitation. Moreover, we identify the most important technologies that can be used along with rehabilitation robots, the types of evaluation methods of rehabilitation robots and the outcomes of robot use for individuals with upper limb disabilities.
Materials and methods
In the current study, we used the PRISMA scoping reviews checklist for selecting studies and reporting the results [ 23 ].
Search strategy and information sources
To find articles related to the rehabilitation of upper limb disabilities using robots, four databases, PubMed, Web of Science, Scopus, and IEEE were searched. In order to search these databases, the keywords related to the upper limb, rehabilitation, and robots were used. Relevant Medical Subject Heading (MeSH) Keywords, spelling differences and synonyms were included and altered as suitable for each database. Then, the below search strategy was developed by KB, ASH, and KHM and finally approved by AH.
((upper extremity disability OR upper limb disability) AND (rehabilitation OR telerehabilitation) AND (robot OR robotics))
Articles addressing upper limb rehabilitation robots were included in to study. The PRISMA diagram is demonstrated in Fig. 1 .
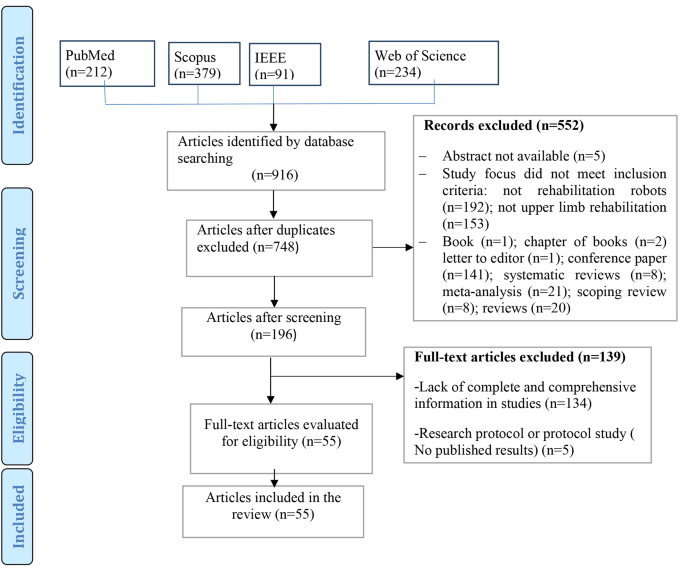
Study selection process
Eligibility criteria.
Inclusion and exclusion criteria are presented below.
Inclusion criteria
Articles involving upper limb rehabilitation robots.
Articles about upper limb disability caused by any illness or injury.
Studies involving rehabilitation robots designed for all ages.
Robotic devices used with any assistive technologies (such as telerehabilitation systems and games based on virtual reality, etc.)
Articles published in English.
Time of publication between January 2012– February 2022.
It should be noted due to the rapid development of technologies and rehabilitation robots, we limited our investigation to the last ten years. We wanted to identify the latest and newest technologies that can be used along with rehabilitation robots, evaluation methods and the outcomes of these robots in recent years. Xue et al. [ 24 ], believed that rehabilitation robotics is a relatively young field growing rapidly with increasing penetration into therapeutic environments. Therefore, the newer dimensions of this rehabilitation tool should always be considered. In addition, the number of articles published in the last ten years was sufficient to answer the questions of this research. Therefore, this scoping review included articles published from January 2012– February 2022.
Exclusion criteria
Articles involving other than upper limb rehabilitation robots.
Robots related to rehabilitation of diseases other than upper limb.
Systematic reviews, reviews, and meta-analysis.
Book chapters.
Letter to the editor.
Conference abstracts.
Research protocol or protocol study.
Study selection
First, abstracts of all related articles were retrieved from four databases: PubMed, Web of Science, Scopus, and IEEE, and imported into EndNote software by KHM. Duplicate articles were removed. After studying the title, and abstract, the relevant articles were selected according to the inclusion and exclusion criteria by KHM and ASH. Then, all studies included in the study were reviewed and finalized by KB and AH. In disagreement, the final decision on each article is decided by discussion among the study team members. Finally, the full text of the articles was studied to extract data and information from KHM and ASH.
Critical appraisal of individual sources of evidence
Critical evaluation of data was done by ASH and KHM independently using Mixed Methods Appraisal Tool (MMAT, version 2018: Hong et al., 2018). Disagreement between authors (n = 4 articles) was resolved by discussion between two other authors (KB and AH) until reaching an agreement. We evaluated studies according to the MMAT criteria according to the selected category. The latest version of the MMAT offers a descriptive quality appraisal instead of a summative numerical score. Answer options in all study categories include “yes”, “no” and “can’t tell”. The answer “can’t tell” indicates that not enough information was reported in the study for a “yes” or “no” answer. A “can’t tell” rating may indicate a need to search for companion studies or contact study authors for more information [ 25 ].
Data charting process and data items
Data were extracted using a predetermined form. The validity of this form was confirmed by two specialists in medical informatics and software engineer, a physiotherapist, and a specialist in health information management. This data extraction form also includes fields such as year, country, type of study, purpose, illness or accident leading to disability (Table 1 ), level of disability (Table 2 ), assistive technologies (telerehabilitation, virtual reality or games) (Table 1 and more details in Appendix B ), number of participants in the study, sex, age (Table 3 and more details in B)), rehabilitated part of the upper limb using a robot (Table 4 ), duration and frequency of treatment, methods of performing rehabilitation exercises, type of evaluation, number of participants in the evaluation process (Table 5 and more details in Appendix B ), study outcomes (Table 6 and more details in Appendix B ) and study conclusions (more details in Appendix B ).
Data collation process
The information extracted from the articles was re-examined by KB and finally approved by AH. In case of disagreement, the consensus was achieved by the review of the members of the study team. It should be noted that for articles with missing data and information, we emailed the corresponding author and asked them to send us the necessary information. Finally, two authors extracted all data from eligible full-text documents through Excel.
Synthesis of results
After the data were stored and managed in MS Excel for processing, to synthesize data, one author (KHM) checks all imported data (e.g., spell check, cell formatting). Then, descriptive statistics (frequency and percentage) were used to summarize the collected data. Descriptive data obtained from the findings of the included articles were organized into tables and figures based on themes to present the findings of this review, which guided the study aims by (KB, AH, and ASH).
Ethical considerations
The protocol of this study was approved by the ethical committee of Kerman University of Medical Sciences) IR.KMU.REC.1400.606).
From the 348 non-duplicate articles found using the search strategy, 55 articles were selected for inclusion (Fig. 1 ). Summarized findings from selected articles are included in Table 1 .
The findings of the quality assessment of studies based on MMAT are presented in Appendix A .
Results of the reviewed studies
Most of the studies were done in Italy (n = 19, 34.55%) [ 26 , 27 , 28 , 31 , 33 , 36 , 37 , 43 , 46 , 47 , 48 , 49 , 57 , 62 , 63 , 68 , 76 , 79 ]. After Italy, 14.24% and 8.9% of the studies were conducted in the USA (n = 8) [ 29 , 42 , 50 , 51 , 56 , 60 , 61 , 72 ] and China (n = 5) [ 32 , 54 , 59 , 65 , 73 ], respectively. (More details in Table 1 ). Also, most of the studies were RCT (n = 26) [ 28 , 29 , 30 , 31 , 35 , 36 , 40 , 41 , 42 , 47 , 48 , 50 , 53 , 54 , 55 , 63 , 66 , 68 , 70 , 72 , 73 , 75 , 76 , 78 , 79 , 80 ].
As shown in Fig. 2 , most articles on robots for upper limb rehabilitation were published in 2017 (n = 9) [ 33 , 34 , 35 , 60 , 61 , 67 , 72 , 79 , 80 ], 2020 (n = 7) [ 46 , 47 , 48 , 49 , 50 , 63 , 64 , 69 ], and 2021 (n = 7) [ 51 , 52 , 53 , 54 , 55 , 65 , 73 ]. (More details in Table 1 )
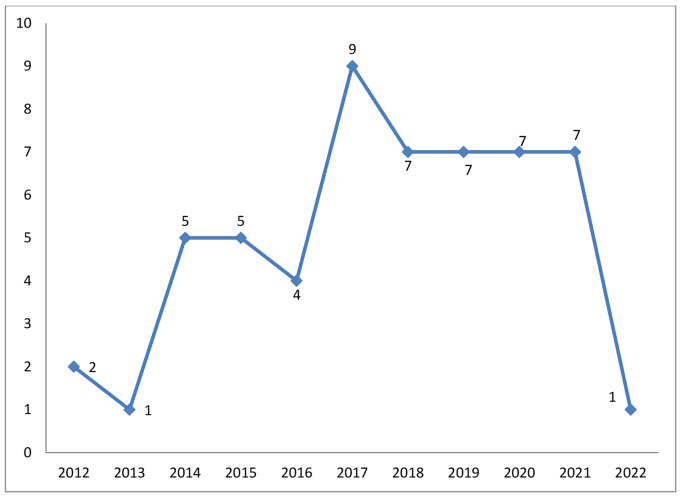
Distribution of the studies in terms of publication year
Robots were used to rehabilitate seven categories of diseases or complications leading to upper limb disability (Fig. 3 ). Most robots were used for patients with stroke (n = 44, 80%) [ 28 , 29 , 31 , 32 , 33 , 34 , 35 , 37 , 38 , 39 , 40 , 41 , 42 , 44 , 45 , 48 , 49 , 50 , 51 , 52 , 53 , 54 , 55 , 56 , 57 , 58 , 59 , 60 , 61 , 62 , 64 , 65 , 66 , 68 , 70 , 72 , 73 , 74 , 75 , 76 , 77 , 78 , 79 , 80 ]. Other diseases with frequencies and percentages are shown in Fig. 3 .
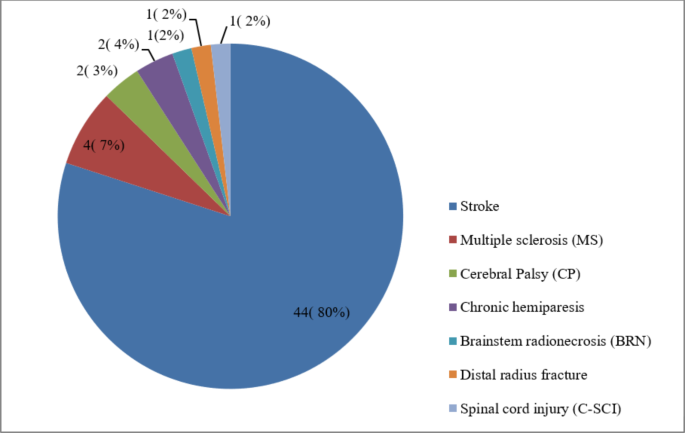
The distribution of the studies based on diseases and injuries leading to upper limb disabilities
Eight different levels of upper limb disability were identified (Table 2 ). The highest frequency of disabilities was related to the entire upper limb (n = 30). Wrist (n = 10), fingers (n = 7) and arm (n = 7) were other parts of the upper limb that had the highest level of disabilities. (More details in Table 1 )
Table 4 shows that some robots were designed to be used to rehabilitate the entire upper limb (n = 15). Then, robots were mostly used for wrist (n = 14), finger (n = 12), and shoulder (n = 11) rehabilitation, respectively. (More details in Appendix B )
Use of telerehabilitation and virtual reality in robots.
60.52% of the studies used games and virtual reality to rehabilitate the upper extremities in robots [ 26 , 30 , 31 , 32 , 36 , 38 , 40 , 42 , 43 , 44 , 45 , 46 , 47 , 48 , 49 , 50 , 51 , 54 , 55 , 57 , 59 , 60 , 61 , 62 , 64 , 66 , 67 , 68 , 69 , 70 , 71 , 72 , 73 , 75 ](n = 34). Moreover, 3.56% of studies had used telerehabilitation in their robots (n = 2) [ 32 , 35 ].
Evaluations in upper limb rehabilitation robots
The number of participants in the evaluation of upper limb rehabilitation robots varied from one [ 43 , 46 , 59 , 64 , 77 ] to 224 [ 48 ]. According to Tables 3 , 89% of the studies used both males and females in the robot evaluation process(n = 49) [ 26 , 27 , 28 , 29 , 31 , 32 , 33 , 34 , 35 , 36 , 37 , 38 , 39 , 40 , 41 , 42 , 44 , 45 , 47 , 48 , 49 , 50 , 51 , 52 , 53 , 54 , 55 , 56 , 57 , 58 , 60 , 61 , 62 , 63 , 65 , 66 , 67 , 68 , 69 , 70 , 71 , 72 , 73 , 74 , 75 , 76 , 78 , 79 , 80 ]. Most of the participants were over 18 years old (n = 47, 85%) [ 26 , 27 , 28 , 29 , 31 , 33 , 34 , 35 , 36 , 37 , 38 , 39 , 40 , 41 , 42 , 43 , 44 , 45 , 46 , 47 , 48 , 49 , 50 , 51 , 53 , 54 , 55 , 56 , 57 , 58 , 59 , 60 , 62 , 63 , 65 , 66 , 67 , 68 , 70 , 71 , 72 , 73 , 75 , 78 , 79 , 80 ]. Moreover, in some studies, the age of the participants was less than or equal to 18 years (n = 4, 7%) [ 30 , 67 , 69 , 76 ]. The minimum and maximum duration of evaluation processes were 30–45 min [ 26 ] to 12 months [ 52 ], respectively. Also, 83% of the studies included both male and female patients in the robot evaluation process. One study included only women [ 43 ], and two studies focused on men [ 46 , 77 ] (More details in Appendix B ).
The duration of treatment of patients in 38% of studies was less than one month (n = 21) [ 26 , 28 , 32 , 33 , 35 , 39 , 47 , 49 , 51 , 54 , 56 , 58 , 60 , 62 , 63 , 64 , 66 , 68 , 72 , 73 , 75 ]. In 41% of the studies (n = 23) [ 29 , 30 , 31 , 34 , 36 , 40 , 43 , 44 , 45 , 46 , 50 , 53 , 55 , 57 , 61 , 65 , 67 , 69 , 71 , 76 , 78 , 79 , 80 ], the duration of treatment of patients was between one and two months. In other studies, the duration of treatment of patients was more than three months [ 27 , 42 , 48 , 52 , 70 ](n = 5, 9%) (More details in Appendix B).
According to Tables 5 , 14 types of evaluation were performed for upper limb rehabilitation robots. “Evaluation and measurement of upper limb function and dexterity” was the most common type of evaluation (n = 52). “Range and motor skills and functional strength of the hand” were ranked next (n = 12). Then, “Neuropsychological assessment” was the most common type of evaluation (n = 11). As shown in Tables 5 , 85 different evaluation methods were used. “Fugl-Meyer Upper Extremity score” was the most used evaluation method in studies (n = 30). Then, “Barthel Index (BI)” (n = 11), “Modified Ashworth Scale (MAS)” (n = 11), and “Box and Block Test (BBT)” (n = 11) were the most widely used evaluation methods.
Outcomes of using upper limb rehabilitation robots
The most important outcomes of upper limb rehabilitation robots were “Improvement in musculoskeletal functions”, “No adverse effect on patients”, and “Safe and reliable treatment”, respectively. Other outcomes are listed in Table 6 . (More details in Appendix B ).
In this scoping review, outcomes of rehabilitation robots for upper limb disabilities and different methods for evaluating rehabilitation robots were identified. Most robots were used for the rehabilitation of patients with stroke. Along with robots, telerehabilitation and virtual reality, technologies were also used for upper limb disabilities rehabilitation. “Evaluation and measurement of upper limb function and dexterity” was the most common type of evaluation among the 14 types performed for upper limb rehabilitation robots. Also, we identified different outcomes of using rehabilitation robots for upper limb disabilities.
As discussed above, telerehabilitation and virtual reality were two types of technology used along with rehabilitation robots. Some studies [ 3 , 82 , 83 ] showed that if special interventions such as game-based virtual reality, and telerehabilitation with rehabilitation robots are used, upper limb function, mental health and patients’ participation in rehabilitation exercises can be improved. Moulaei et al. [ 3 ], showed in a scoping review that telerehabilitation could lead to “improving in musculoskeletal functions”, “increasing patients’ interest and motivation to perform rehabilitation exercises”, “increasing adherence to rehabilitation exercises and more participation in treatment processes”, “remote monitoring and control of patients”, “reducing or relieved pain” and “improving quality of life”. Fernández-Vázquez et al. [ 84 ], also pointed out that virtual reality as a very interesting tool in treating upper limb disorders along with rehabilitation robots can improve the motor function of the upper limb, increase users’ motivation and enjoyment, a large number of repetitions or high intensity rehabilitation. Repetitive and long exercises become easy and reduce the time needed to perform therapeutic exercises. Some studies [ 85 , 86 , 87 ] stated that for telerehabilitation systems and virtual reality to be as effective as robots, they should be easy to use, user-friendly, and have a beautiful and appropriate user interface. Zanatta et al. [ 87 ] pointed out that when telerehabilitation systems, robots and virtual reality are usable and attractive, patients’ motivation to perform rehabilitation exercises increases, their satisfaction with treatment processes increases, and efficiency and effectiveness increase. Therefore, to combine the robot with telerehabilitation or virtual reality to be effective, various factors such as usability, suitable user interface and even technical support should be considered in design, development and implementation.
The findings of this study and other reviews [ 2 , 22 , 88 , 89 ] have shown that rehabilitation robots have been able to improve the musculoskeletal status of patients with upper limb disabilities. FMA-UE scores obtained from different studies showed that robot-based treatments can improve upper limb musculoskeletal function [ 1 , 90 , 91 , 92 ]. In a review of the literature on therapeutic robotics in chronic stroke survivors and individuals with hemiparesis, the FMA total score improved from 2.1 to 15.1 and the ARAT improved to 11.1 [ 1 , 90 , 91 , 92 ]. Milot et al. [ 91 ], tested 20 volunteers with mild to moderate chronic stroke to investigate the robot’s functional outcomes on the upper limb. Their study showed that training with the robot led to significant improvements in FMA, BBT, MAL, WMFT, and quantitative amounts of strength and speed, and these statuses were maintained in the 3-month follow-up [ 91 ]. Cimolin et al., [ 93 ] conducted a clinical trial using the Armeo Spring device on children aged 4 to 17 years with cerebral palsy. The subjects of this study showed promising improvements in different movement patterns and hand functions. It seems that to increase the effect of robots on improving upper limb disabilities, it is better to consider a number of factors. For example, the mechanical characteristics of rehabilitation robots may affect outcomes. Exoskeleton robots can control several joints simultaneously, leading to tight physical interaction between humans and robots, which may increase the patient burden.
Additionally, the exoskeleton robot’s high inertia to its complex structure can intervene with manipulation. Or, in the mechanical dimensions of the robot, the degree of freedom should be considered an important factor in the design. For example, intervention with six degrees of freedom in a three-dimensional space is very challenging for stroke patients with moderate to severe motor impairment [ 1 ]. The term “paradox of reduced number of degrees of freedom” states that to train a patient with a severe movement disorder, one should first use the lowest number of degrees of freedom, then gradually increase the number of degrees of freedom as improvements are achieved [ 94 ]. On the other hand, if the robots are not light, they can put pressure on different organs of patients during exercise. Also, rehabilitation robots can be costly, except for people who might profit from using them for training. As a result, efforts should be made to make rehabilitation robots as cost-effective as possible. Finally, these devices should be easily available to people, and awareness of them as an effective and helpful alternative treatment should also continuously increase.
Although, in this review we observed that rehabilitation robots have no adverse effect on patients, in some studies, the complications of rehabilitation robots have been mentioned [ 89 ]. Bessler et al. [ 95 ], believed that the safety of using rehabilitation robots in clinical trials should be confirmed for monitoring and reporting adverse events. In a systematic literature review, Bessler et al. [ 89 ], gathered information on the type of adverse events associated with the training of static robotic gait trainers. They identified about 17 adverse events per 100 people trained on a stationary robotic gait trainer. The most common types of side effects were classified into two categories: side effects related to soft tissue and musculoskeletal side effects. The third category included physiological adverse events (such as sudden changes in blood pressure) that seem to be unrelated to the mechanical settings of the robotic device in most cases, but are generally considered to be related to the activity engaged. Side effects associated to soft tissue in trainers also included things such as skin redness, skin irritation, open skin lesions and bruising, skin abrasion, as well as hurt and pain in soft tissue areas. Musculoskeletal side effects also included tendinopathy, muscle pain, tibial fracture, back and malleolus pain, and joint discomfort and pain [ 89 ]. Therefore, rehabilitation robots should be designed to minimize the side effects related to all three dimensions, i.e., soft tissue, musculoskeletal, and physiological. First of all, it can be said that the designers of these systems must ensure that there are no side effects before using robots by patients or therapists, so that they can be used safely for rehabilitation. Then, the therapists should set the rehabilitation exercises in terms of dosage and duration in such a way that the side effects caused by the low or high use of the robot do not occur for the patients.
Besides, we should be able to improve the design, development and evaluation of rehabilitation robots so that they have the necessary quality to provide rehabilitation services. Abu-Dakka et al. [ 96 ] believed that rehabilitation robots should be designed in a way that can be easily used by patients, therapists, and clinicians, increase the effectiveness of doctors’ treatments and make patients’ daily activities easier. To achieve these goals, rehabilitation robots must have some functional requirements, including: stability, safety, adaptability to patient needs, accept a wide range of patients, providing a full Range of Motion (ROM), being equipped with the necessary sensors for haptic and visual feedback, etc. [ 97 , 98 ]. Also, in the design and evaluation of rehabilitation robots, we must consider the views and opinions of users (patients and therapists), because these people are the end users of these rehabilitation tools. Considering the perspectives and opinions of patients and therapists in the design process also makes the device develop according to the patients’ needs and preferences. As a result, their level of satisfaction and continued use of this device improves. Zanatta et al., [ 87 ] believed that considering the patient’s perspective when designing or evaluating a rehabilitation device is essential to ensure adequate engagement and adherence to treatment. Or when the usability of a rehabilitation robot is suitable for users, according to Nielsen [ 99 ], learnability, efficiency, memorability and satisfaction of users improve, while the rate of errors also decreases.
Accordingly, a patient who perceives a device as usable is likely also report a positive perception as a concern: ease of learning the system’s function and behavior, effort expended to reach a goal, ease of remembering the system’s performance for any further use, the system’s ability to easily support and recover the case of errors during use and the pleasant design of the system. Thus, assessing such aspects provides important insights into the acceptability and perceived usefulness of devices, thereby allowing an understanding of how to improve patient motivation during treatment. As emphasized in Monardo’s study [ 100 ], motivation plays an essential role during the rehabilitation process because it helps create a sense of patient competence and satisfaction. In addition, patient satisfaction with treatment-rehabilitation processes performed with the help of rehabilitation devices was associated with stronger treatment compliance [ 101 ]. As a result, both factors can be considered key factors for the effectiveness of treatment-rehabilitation processes. On the other hand, the anatomical structure of the upper limb, the severity and degree of the patients’ disabilities, and the age group of the patients (for example, children or the elderly) should also be considered when designing and developing a rehabilitation tool. For example, a rehabilitation robot may only be used by children and not by elderly persons [ 102 ] or a robot may be designed for finger rehabilitation-only [ 103 ].
According to the findings of the studies, we found that robots were a safe and reliable treatment for patients. Therefore, it is necessary to consider a set of factors for the rehabilitation robot to guarantee patient safety and maintain its reliability for patients. Sampson et al. [ 104 ], evaluated the BUiLT + VR system in hemiparetic patients with upper extremity disability and found that this system is reliable for treating patients and can be administered safely. They believed that through Rests between games, ramping of the time spent playing in the early sessions, and adjustments to seating, unit height and angle, it could overcome the challenges related to patient safety and increase its reliability for users. Other studies show that the designed rehabilitation robot should ensure the safety of the patients from the aspects of using the common sensor to monitor and manage the force information of the patients during movement, when the reaction force made by the muscle tension is very high. Also, when the patient experiences a muscle spasm, the robot should automatically stop the current movement so that the muscle is not strained. Therefore, to prevent repeated injury to patients, the space and working environment should be restricted by limiting the switch within a reasonable range. The rehabilitation robot’s speed of displacement and movement should also be limited by the software used to control the robot. The operator or therapists should be able to rationally adjust the parameters of the driving device, and control and monitor the movement status of the robot in real time. Pressing the stop button is very important to avoid accidents. Also, to avoid damage created by the rotation of the joints due to gravity, the rehabilitation robot’s ability to self-lock should be considered [ 105 ].
Reduction or relief of pain was another outcome of this review. Some studies [ 75 , 95 ] have shown that robots can reduce or relieve pain in patients, but factors must be considered when designing or using robots so that the robot can reduce pain more effectively. For example, exceeding forces on the human musculoskeletal system, at the same time misalignment will make high pressure and shear forces via slipping at the cuffs or straps [ 106 , 107 ]. Therefore, forces that are not considered or compensated for in the design of the robot or its interface are easily transmitted to the musculoskeletal systems. On the other hand, if the torques and forces are too high or act in arbitrary directions on the musculoskeletal system, they can create an additional load, resulting in pain and damage to the bones, joints, and muscles [ 95 , 106 ]. Therefore, when designing robots, pain thresholds should be considered for pressures and forces applied during accidental contact such as collisions or closing situations with a robot [ 108 ]. Different limit values for many different parts of the human body are accepted by ISO/TS 15,066 [ 95 ]. The patient’s position to perform therapeutic exercises with the help of the robot is also very effective in reducing pain. The robot developed in Kim et al.‘s [ 75 ] study allows patients to be comfortable and supported in a supine position. This way can easily be linked to other pain therapeutic methods, such as interferential current therapy or hot pack application. For example, installing a friction plate to forbid the compensation of the scapula and to increase the therapy’s effectiveness and adjust the robotic arm’s height using a linear actuator can be very helpful [ 75 ].
Duration and intensity of the therapy through the robot are two other factors that effect on the robots in motor control and improving pains. By examining the effectiveness of robots on function and structure in patients with limited upper limb function, Ferreira et al., [ 109 ] showed that when robot-assisted therapy is used with conventional treatment at the same dose and duration, robot-assisted therapy has a significant effect motor control. The group’s analysis also proposed an effect of the number of sessions and duration of treatment on some estimated impacts. The greater number of sessions and the volume of treatment affect motor control [ 109 ]. The more significant treatment dose effect was proposed by Lohse et al. [ 110 ]; however, time as a representation of dose is a rather rough estimate and supplies no evidence of the actual amount of movement or types of movement, nor does it account for periods of inactivity or rest [ 111 ]. In this respect, a previous study showed that, although there is no agreement, the minimum dose should be at least 16 h of exercise [ 112 ]. Poor methodological quality and lower dose and duration of treatment may negatively affect the estimated effects. So, therapists usually consider this approach because it has very few or no side effects [ 109 ]. Moreover, some studies have shown that low or high intensity exercise therapy can increase the risk of injury and worsening pain in the hemiplegic shoulder [ 113 ]. In the study by Kim et al. [ 75 ], participants in the intervention or control groups performed stretching exercises for 30 min per day, five times per week for four weeks; Shoulder pain in the intervention group (they used the robot) was worse than the control group. The authors of this research concluded that this stretching exercise was performed for patients with excessive intensity and more than 30 min of stretching exercise caused pain in some patients even when it was performed with low intensity.
As our study showed, robots can increase patients’ interest and motivation to perform rehabilitation exercises. Therefore, one of the other factors that can be considered to increase patients’ use of robots and their interest and motivation to perform rehabilitation exercises is the use of games and virtual reality. Creating a degree of entertainment through games and virtual reality can improve patients’ adherence to rehabilitation exercises and increase patients’ attention span to spend more time on their rehabilitation program [ 114 ]. Kafri et al. [ 115 ], investigated the effect of virtual reality on patients after a stroke. Patients showed an improvement in activity and reported that they enjoyed the game. Virtual reality also supplies direct visual feedback and can empower patients with a sense and awareness of control over their recovery. Consequently, it is imaginable that people who train with virtual reality and games will enhance their performance when interacting with an avatar presented on the screen [ 114 ]. In another study, Gomez et al., [ 116 ] evaluated patients with multiple sclerosis using games, in which the experimental group received both game sessions and conventional treatment. This study showed that after using the games, interest and motivation to perform rehabilitation exercises improved in the patients.
Efficiency and effectiveness of rehabilitation robots, increasing the patient’s independence in performing rehabilitation exercises, rehabilitation robots’ feasibility for training patients, performing repetitive and long exercises very easily with the help of the robot, reducing the duration of rehabilitation exercises, increasing adherence to rehabilitation exercises and more participation in treatment processes, and improving quality of life and rehabilitation process were other outcomes related to rehabilitation robots identified in this study. But it must be said that these outcomes or other mentioned outcomes will not be created spontaneously due to the use of rehabilitation robots, but a series of main factors or capabilities must be included in the robots so that these outcomes can be created and have a and beneficial effect in rehabilitation. For example, Laut et al. [ 117 ] believed that rehabilitation robots could potentially increase efficiency and access to treatment by having capabilities such as providing continuous training for a long period of time and collecting data to evaluate progress. Furthermore, by pairing rehabilitation robots with health information technologies and presenting assessment and performance data all over the internet to the therapist, different treatment processes can be moved out of specific facilities and into patients’ homes with remote management by a therapist. This can let a therapist treat several patients simultaneously, greatly enhancing the numbers of patients treated and the efficiency of the rehabilitation robot and therapist.
In other words, changing from a hospital to a home rehabilitation program can reduce costs while making rehabilitation more accessible [ 117 ]. Francisco et al. [ 118 ] also stated that by providing frequent and intensive training through rehabilitation robotics, the effectiveness of conventional occupational therapy or physiotherapy can be improved by providing more coherent, accurate, and precise treatment. Therefore, it should be said that for robots to be able to teach patients better or be more efficient, they must be able to provide frequent and intensive training. Also, in order to improve the efficiency and effectiveness of a rehabilitation device, the satisfaction of patients should be maximized. According to Tousignant et al.‘s study [ 119 ], satisfaction is an important indicator and factor for the degree of efficiency and effectiveness. Its high level can increase patient motivation, and adherence to treatment, and improve compliance with treatment. Also, it should be noted that satisfaction is one of the important factors in the quality of health care; it can affect adherence to treatment plans, improve clinical outcomes [ 120 ], and motivation of patients to perform rehabilitation exercises [ 121 ]. In order to maximize satisfaction, the robot must be designed according to the needs and preferences of its users, be usable and easy to use, and have no side effects [ 122 , 123 ].
In addition, concerning adherence to rehabilitation exercises, it can be said that considering technologies such as sensors and computer game technologies for home rehabilitation or mRehab (mobile rehabilitation) systems in robots greatly increases the possibility of objective quantification of adherence [ 124 ]. Also, for patients to engage and be challenged while performing rehabilitation exercises at home in the physical absence of the therapist, there is a need to carefully design scenarios and motivational features that guarantee the patient’s adherence to the treatment plan [ 125 ]. Increasing the quality of life was another important outcome of using rehabilitation robots identified in our study. In the study by Kutner et al. [ 126 ], it was shown that patients who had suffered a stroke experienced a faster increase in quality of life (in physical, mental and social dimensions) after participating in targeted interventions that focused on improving upper limb strength. Mohammed et al. [ 127 ], in their study of patients with upper and lower limb amputation, defined the quality of life in three dimensions physical, mental and social health. Therefore, it should be said that when a rehabilitation robot is designed, in order to increase the quality of life of the patients, it should be focused on all three dimensions of the patients’ health, physical, psychological and social.
In general, it can be said that although the findings of the present study showed that rehabilitation robots could lead to positive outcomes for people with upper limb disabilities with various diseases or injuries, but if the factors that lead to these outcomes in If these robots are not considered, they will never have the necessary efficiency, effectiveness and quality.
Prospects of rehabilitation robots
It is predictable that rehabilitation robots will be applied much more than before in an era where the cost of manual therapy is becoming more expensive. To better popularize and commercialize this goal, future development needs to increase the universality of rehabilitation robots, focusing on portable, lightweight, reconfigurable, smart, and equipment based on artificial intelligence and machine learning techniques, new methods of treatment and thinking to the field of rehabilitation under the hypothesis of guaranteeing safety, mass production and development in a cheap direction [ 24 ]. For example, machine learning techniques may ,allow a robot to independently adapt to the changing needs of each customer over time and perform specific tasks, such as autonomous gaming [ 128 ]. Another example is the portability of the robot, which allows users to carry the robot system for deployment inside homes or medical centers [ 129 ]. People can use portable rehabilitation robots not only at home and in the treatment center, but also in nature and parks. On the other hand, Smart rehabilitation is the future development trend. It should be broken through the human-machine interface barrier, develop multidisciplinary and interdisciplinary collaboration and communication, improve and strengthen cooperation with rehabilitation medicine and artificial intelligence, actively participate in the design, development and evaluation of rehabilitation robots, and improve rehabilitation robots for providing better and more quality service to people.
Moreover, in the future, there should be more focus on “human nature” in the design and development of rehabilitation robots. Human nature means that robots have capabilities such as “perception, thinking, action and cooperation, expression and communication, and learning and adaptability and complete training and operations independently or with the assistance of an automatic machine” [ 24 ]. At present, rehabilitation robots cannot perform all the tasks of professional rehabilitation personnel; especially, the ability of self-learning, adaptability, flexibility and creativity of robots needs many theoretical developments. The most challenging and most difficult thing for rehabilitation robots is the benevolence and moral sense of medical staff [ 130 ]. Since in the future software packages and powerful hardware equipment will be produced, many of these problems can be easily solved.
Limitations of the study
There are a few limitations in this study. In the present study, only articles in English were reviewed; it is better to include articles published in non-English languages in future studies. Also, to find related studies, we searched four databases, Scopus, IEEE, PubMed, and Web of Science. It is suggested that more studies be done in more databases to obtain more comprehensive results.
This scoping review indicates that upper limb rehabilitation robots can improve musculoskeletal functions (Musculoskeletal strength, sensation, perception, vibration, muscle coordination, less spasticity, flexibility, and range of motion); avoid serious side effects or adverse effects on the patient, provide safe and reliable treatment, reduce pain, increase the patient’s independence in performing rehabilitation exercises, reduce the duration of rehabilitation exercises, increase adherence to rehabilitation exercises and treatment processes, and quality of life and rehabilitation processes. The use of rehabilitation robots is growing strongly, especially in developed countries, and it seems that this new rehabilitation technology has had significant effects in helping to improve upper limb disabilities.
Also, rehabilitation robots could provide a platform for motivating people with upper limb disabilities to carry out more rehabilitation exercises without a therapist, which could maximize recovery.
Data Availability
The datasets used and analyzed during the current study are available from the corresponding author on reasonable request.
Abbreviations
Nine-Hole Peg Test
Assisting Hand Assessment
Arm-Hand Function
Arm Motor Ability Test
Action Research Arm Test
Box and Block Test
Barthel Index
Behavioral Inattention Test
Brainstem radionecrosis
Spinal cord injury
Catherine Bergego Scale
Credibility/Expectancy Questionnaire
Cerebral Palsy
Disability Assessment Scale
Disabilities of Arm, Shoulder, and Hand
Douleur Neuropathique 4
Dynamic Surface Electromyography
Quality of Life
Functional Indipendence Measure
Fugl-Meyer Upper Extremity score
Functional magnetic resonance imaging
Functional near infrared spectroscopy
Grooved Pegboard Test
Intrinsic Motivation Inventory
Jebsen-Taylor Hand Function Test
Shoulder Disability Questionnaire
Motor Activity Log
Modified Ashworth Scale
Mental health Composite Score
Medical Subject Heading
Manual Function Test
Motricity Index
Mini-Mental State Examination
Magnetic Resonance Imaging
Modified Rankin Scale
Multiple sclerosis
Multiple Sclerosis Quality of Life-54
Nottingham Extended Activities of Daily Living
National Institutes of Health Stroke Scale
Numeric Rating Pain Scale
Numerical Rating Scale
Oxford Cognitive Screen
Physical health Composite Score
Pediatric Evaluation of Disability Inventory
Performance Oriented Mobility Assessment
Patient-Rated Wrist and Hand Evaluation
Quality of Movement
Quality of Upper Extremity Skills Test
Quick version of the Disabilities of the Arm, Shoulder, and Hand
Reliable Change Index
Randomized controlled trials
Rey Osterrieth complex figure test
Active or Passive Range Of Motion
Stroop Color and Word Test
Symbol Digit Modalities Test
Self-Depression Scale
Short Form Health Survey
Stroke Impact Scale
Total active mobility
Trail making test
Tremor Severity Scale
Usefulness, Satisfaction and Ease-of-use
Visual analog scale
Visual analogue scale
World Health Organization Disability Assessment Schedule
Wolf Motor Function
Lee SH, Park G, Cho DY, Kim HY, Lee J-Y, Kim S, Park S-B, Shin J-H. Comparisons between end-effector and exoskeleton rehabilitation robots regarding upper extremity function among chronic stroke patients with moderate-to-severe upper limb impairment. Sci Rep. 2020;10(1):1806.
Article CAS PubMed PubMed Central Google Scholar
Vélez-Guerrero MA, Callejas-Cuervo M, Mazzoleni S. Artificial Intelligence-Based Wearable Robotic Exoskeletons for Upper Limb Rehabilitation: a review. Sens (Basel). 2021;21(6):2146.
Article Google Scholar
Moulaei K, Sheikhtaheri A, Nezhad MS, Haghdoost A, Gheysari M, Bahaadinbeigy K. Telerehabilitation for upper limb disabilities: a scoping review on functions, outcomes, and evaluation methods. Archives of Public Health. 2022;80(1):196.
Article PubMed PubMed Central Google Scholar
Poltawski L, Allison R, Briscoe S, Freeman J, Kilbride C, Neal D, Turton AJ, Dean SJD. rehabilitation: Assessing the impact of upper limb disability following stroke: a qualitative enquiry using internet-based personal accounts of stroke survivors. 2016, 38(10):945–51.
Maciejasz P, Eschweiler J, Gerlach-Hahn K, Jansen-Troy A, Leonhardt S. A survey on robotic devices for upper limb rehabilitation. J Neuroeng Rehabil. 2014;11(1):3.
Vélez-Guerrero MA, Callejas-Cuervo M, Mazzoleni S. Design, Development, and testing of an Intelligent Wearable Robotic Exoskeleton Prototype for Upper Limb Rehabilitation. Sens (Basel). 2021;21(16):5411.
Wu Q, Wu H. Development, dynamic modeling, and Multi-Modal Control of a therapeutic exoskeleton for Upper Limb Rehabilitation Training. Sens (Basel). 2018;18(11):3611.
Qassim HM, Wan Hasan WZ. A Review on Upper Limb Rehabilitation Robots. Appl Sci 2020, 10(19).
Richards L, Hanson C, Wellborn M, Sethi A. Driving motor recovery after stroke. Top Stroke Rehabil. 2008;15(5):397–411.
Article PubMed Google Scholar
Qian Q, Nam C, Guo Z, Huang Y, Hu X, Ng SC, Zheng Y, Poon W. Distal versus proximal - an investigation on different supportive strategies by robots for upper limb rehabilitation after stroke: a randomized controlled trial. J Neuroeng Rehabil. 2019;16(1):64–4.
Langhorne P, Bernhardt J, Kwakkel GJTL. Stroke rehabilitation. 2011, 377(9778):1693–1702.
Qian Z, Bi ZJAiME. Recent development of rehabilitation robots. 2015, 7(2):563062.
Schweighofer N, Choi Y, Winstein C. Gordon JJAJoPM, Rehabilitation: Task-oriented rehabilitation robotics. 2012, 91(11):S270-S279.
Ennaiem F, Chaker A, Laribi MA, Sandoval J, Bennour S, Mlika A, Romdhane L, Zeghloul SJAS. Task-Based design approach: development of a planar cable-driven parallel robot for upper limb rehabilitation. 2021, 11(12):5635.
Johnson MJ, Loureiro RC, Harwin WSJISR. Collaborative tele-rehabilitation and robot-mediated therapy for stroke rehabilitation at home or clinic. 2008, 1(2):109–21.
Nizamis K, Athanasiou A, Almpani S, Dimitrousis C, Astaras AJS. Converging robotic technologies in targeted neural rehabilitation: A review of emerging solutions and challenges. 2021, 21(6):2084.
Wege A, Zimmermann A. Electromyography sensor based control for a hand exoskeleton. In: 2007 IEEE International Conference on Robotics and Biomimetics (ROBIO) : 2007: IEEE; 2007: 1470–1475.
Wang Q, Markopoulos P, Yu B, Chen W, Timmermans A. Interactive wearable systems for upper body rehabilitation: a systematic review. J Neuroeng Rehabil. 2017;14(1):20.
Herrera-Luna I, Rechy-Ramirez EJ, Rios-Figueroa HV, Marin-Hernandez AJISJ. Sensor fusion used in applications for hand rehabilitation: A systematic review. 2019, 19(10):3581–3592.
Koutsiana E, Ladakis I, Fotopoulos D, Chytas A, Kilintzis V, Chouvarda I. Serious Gaming Technology in Upper Extremity Rehabilitation: scoping review. JMIR Serious Games. 2020;8(4):e19071–1.
Parker J, Powell L, Mawson S. Effectiveness of Upper Limb Wearable Technology for improving activity and participation in adult stroke survivors: systematic review. J Med Internet Res. 2020;22(1):e15981–1.
Kwakkel G, Kollen BJ, Krebs HIJN. repair n: Effects of robot-assisted therapy on upper limb recovery after stroke: a systematic review. 2008, 22(2):111–21.
PRISMA for Scoping Reviews. [ https://prisma-statement.org/Extensions/ScopingReviews ].
Xue X, Yang X, Deng Z, Tu H, Kong D, Li N, Xu F. Global Trends and hotspots in Research on Rehabilitation Robots: a bibliometric analysis from 2010 to 2020. Front Public Health. 2021;9:806723.
Hong QN, Pluye P, Fàbregues S, Bartlett G, Boardman F, Cargo M, Dagenais P, Gagnon M-P, Griffiths F. Nicolau BJRoc: Mixed methods appraisal tool (MMAT), version 2018. 2018, 1148552(10).
Carpinella I, Cattaneo D, Bertoni R, Ferrarin M. Robot training of upper limb in multiple sclerosis: comparing protocols with or without manipulative task components. IEEE Trans neural Syst rehabilitation engineering: publication IEEE Eng Med Biology Soc. 2012;20(3):351–60.
Sale P, Bovolenta F, Agosti M, Clerici P, Franceschini M. Short-term and long-term outcomes of serial robotic training for improving upper limb function in chronic stroke. Int J rehabilitation Res Int Z fur Rehabilitationsforschung Revue Int de recherches de readaptation. 2014;37(1):67–73.
Google Scholar
Pennati GV, Da Re C, Messineo I, Bonaiuti D. How could robotic training and botolinum toxin be combined in chronic post stroke upper limb spasticity? A pilot study. Eur J Phys Rehabil Med. 2015;51(4):381–7.
CAS PubMed Google Scholar
McCabe J, Monkiewicz M, Holcomb J, Pundik S, Daly JJ. Comparison of robotics, functional electrical stimulation, and motor learning methods for treatment of persistent upper extremity dysfunction after stroke: a randomized controlled trial. Arch Phys Med Rehabil. 2015;96(6):981–90.
Gilliaux M, Renders A, Dispa D, Holvoet D, Sapin J, Dehez B, Detrembleur C, Lejeune TM, Stoquart G. Upper Limb Robot-Assisted therapy in cerebral palsy: a single-blind randomized controlled trial. Neurorehabilit Neural Repair. 2015;29(2):183–92.
Taveggia G, Borboni A, Salvi L, Mulé C, Fogliaresi S, Villafañe JH, Casale R. Efficacy of robot-assisted rehabilitation for the functional recovery of the upper limb in post-stroke patients: a randomized controlled study. Eur J Phys Rehabil Med. 2016;52(6):767–73.
PubMed Google Scholar
Song AG, Wu CC, Ni DJ, Li HJ, Qin HY. One-therapist to three-patient Telerehabilitation Robot System for the Upper Limb after Stroke. Int J Social Robot. 2016;8(2):319–29.
Trujillo P, Mastropietro A, Scano A, Chiavenna A, Mrakic-Sposta S, Caimmi M, Molteni F, Rizzo G. Quantitative EEG for Predicting Upper Limb Motor Recovery in Chronic Stroke Robot-Assisted Rehabilitation. IEEE Trans Neural Syst Rehabil Eng. 2017;25(7):1058–67.
Saita K, Morishita T, Hyakutake K, Fukuda H, Shiota E, Sankai Y, Inoue T. Combined therapy using botulinum toxin A and single joint hybrid assistive limb for upper-limb disability due to spastic hemiplegia. J Neurol Sci. 2017;373:182–7.
Article CAS PubMed Google Scholar
Hsieh YW, Wu CY, Wang WE, Lin KC, Chang KC, Chen CC, Liu CT. Bilateral robotic priming before task-oriented approach in subacute stroke rehabilitation: a pilot randomized controlled trial. Clin Rehabil. 2017;31(2):225–33.
Gandolfi M, Vale N, Dimitrova EK, Mazzoleni S, Battini E, Benedetti MD, Gajofatto A, Ferraro F, Castelli M, Camin M et al. Effects of High-intensity Robot-assisted Hand Training on Upper Limb Recovery and Muscle Activity in Individuals With Multiple Sclerosis: A Randomized, Controlled, Single-Blinded Trial. Front Neurol 2018, 9.
Germanotta M, Cruciani A, Pecchioli C, Loreti S, Spedicato A, Meotti M, Mosca R, Speranza G, Cecchi F, Giannarelli G et al. Reliability, validity and discriminant ability of the instrumental indices provided by a novel planar robotic device for upper limb rehabilitation. J Neuroeng Rehabil 2018, 15.
Kim GW, Won YH, Seo JH, Ko MH, EFFECTS OF NEWLY DEVELOPED COMPACT ROBOT-AIDED UPPER EXTREMITY TRAINING SYSTEMS (NEURO-X. (R)) IN PATIENTS WITH STROKE: A PILOT STUDY. J Rehabil Med. 2018;50(7):607–12.
Iwamoto Y, Imura T, Suzukawa T, Fukuyama H, Ishii T, Taki S, Imada N, Shibukawa M, Inagawa T, Araki H, et al. Combination of Exoskeletal Upper Limb Robot and Occupational Therapy improve activities of Daily living function in Acute Stroke Patients. J stroke Cerebrovasc diseases: official J Natl Stroke Association. 2019;28(7):2018–25.
Dehem S, Gilliaux M, Stoquart G, Detrembleur C, Jacquemin G, Palumbo S, Frederick A, Lejeune T. Effectiveness of upper-limb robotic-assisted therapy in the early rehabilitation phase after stroke: a single-blind, randomised, controlled trial. Annals of Physical and Rehabilitation Medicine. 2019;62(5):313–20.
Hung CS, Lin KC, Chang WY, Huang WC, Chang YJ, Chen CL, Grace Yao K, Lee YY. Unilateral vs bilateral hybrid approaches for Upper Limb Rehabilitation in Chronic Stroke: a Randomized Controlled Trial. Arch Phys Med Rehabil. 2019;100(12):2225–32.
Conroy SS, Wittenberg GF, Krebs HI, Zhan M, Bever CT, Whitall J. Robot-Assisted arm training in chronic stroke: addition of transition-to-Task Practice. Neurorehabilit Neural Repair. 2019;33(9):751–61.
Bonanno L, Russo M, Bramanti A, Calabrò RS, Marino S. Functional connectivity in multiple sclerosis after robotic rehabilitative treatment: a case report. Medicine. 2019;98(17):e15047.
Leem MJ, Kim GS, Kim KH, Yi TI, Moon HI. Predictors of functional and motor outcomes following upper limb robot-assisted therapy after stroke. Int J rehabilitation Res Int Z fur Rehabilitationsforschung Revue Int de recherches de readaptation. 2019;42(3):223–8.
Kim DH, Kim KH, Lee SM. The effects of virtual reality training with Upper Limb sensory Exercise Stimulation on the AROM of Upper Limb joints, function, and concentration in chronic stroke patients. Phys Medizin Rehabilitationsmedizin Kurortmedizin. 2020;30(2):86–94.
Tartamella F, Chillura A, Pisano MF, Cacioppo A, Licari S, Caradonna D, Portaro S, Calabr RS, Bramanti P, Naro A. A case report on intensive, robot-assisted rehabilitation program for brainstem radionecrosis. Medicine 2020, 99(10).
Solaro C, Cattaneo D, Basteris A, Carpinella I, De Luca A, Mueller M, Bertoni R, Ferrarin M, Sanguineti V. Haptic vs sensorimotor training in the treatment of upper limb dysfunction in multiple sclerosis: A multi-center, randomised controlled trial. J Neurol Sci 2020, 412.
Aprile I, Germanotta M, Cruciani A, Pecchioli C, Loreti S, Papadopoulou D, Montesano A, Galeri S, Diverio M, Falsini C et al. Poststroke shoulder pain in subacute patients and its correlation with upper limb recovery after robotic or conventional treatment: A secondary analysis of a multicenter randomized controlled trial. Int J stroke: official J Int Stroke Soc 2020:1747493020937192.
Aprile I, Guardati G, Cipollini V, Papadopoulou D, Mastrorosa A, Castelli L, Monteleone S, Redolfi A, Galeri S, Germanotta M. Robotic Rehabilitation: An Opportunity to Improve Cognitive Functions in Subjects With Stroke. An Explorative Study. Front Neurol 2020, 11.
Kim GJ, Chen P. Role of instruction adherence during highly structured robotic arm training on Motor Outcomes for individuals after chronic stroke. Am J Phys Med Rehabil. 2020;99(4):353–6.
Bui KD, Wamsley CA, Shofer FS, Kolson DL, Johnson MJ. Robot-Based Assessment of HIV-Related Motor and Cognitive Impairment for Neurorehabilitation. IEEE Trans Neural Syst Rehabil Eng. 2021;29:576–86.
Flynn N, Froude E, Cooke D, Dennis J, Kuys S. The sustainability of upper limb robotic therapy for stroke survivors in an inpatient rehabilitation setting. Disabil Rehabil 2021.
Terranova TT, Simis M, Santos ACA, Alfieri FM, Imamura M, Fregni F, Battistella LR. Robot-Assisted Therapy and Constraint-Induced Movement Therapy for Motor Recovery in Stroke: Results From a Randomized Clinical Trial. Front Neurorobotics 2021, 15.
Qu QM, Lin YN, He ZJ, Fu JH, Zou F, Jiang ZW, Guo FX, Jia J. The Effect of Applying Robot-Assisted Task-Oriented Training Using Human-Robot Collaborative Interaction Force Control Technology on Upper Limb Function in Stroke Patients: Preliminary Findings. BioMed research international 2021, 2021.
Abd El-Kafy EM, Alshehri MA, El-Fiky AAR, Guermazi MA, Mahmoud HM. The Effect of Robot-Mediated Virtual Reality Gaming on Upper Limb Spasticity Poststroke: A Randomized-Controlled Trial. Games for health journal .
Hu XL, Tong KY, Wei XJ, Rong W, Susanto EA, Ho SK. The effects of post-stroke upper-limb training with an electromyography (EMG)-driven hand robot. J Electromyogr Kinesiol. 2013;23(5):1065–74.
Squeri V, Masia L, Giannoni P, Sandini G, Morasso P. Wrist rehabilitation in chronic stroke patients by means of adaptive, progressive robot-aided therapy. IEEE Trans neural Syst rehabilitation engineering: publication IEEE Eng Med Biology Soc. 2014;22(2):312–25.
Article CAS Google Scholar
Hsieh YW, Lin KC, Wu CY, Lien HY, Chen JL, Chen CC, Chang WH. Predicting clinically significant changes in motor and functional outcomes after robot-assisted stroke rehabilitation. Arch Phys Med Rehabil. 2014;95(2):316–21.
Chen W, Cui X, Zhang J, Wang J. A cable-driven wrist robotic rehabilitator using a novel torque-field controller for human motion training. Rev Sci Instrum. 2015;86(6):065109.
McKenzie A, Dodakian L, See J, Le V, Quinlan EB, Bridgford C, Head D, Han VL, Cramer SC. Validity of Robot-Based assessments of Upper extremity function. Arch Phys Med Rehabil. 2017;98(10):1969–76.
Housley SN, Wu D, Richards K, Belagaje S, Ghovanloo M, Butler AJ. Improving Upper Extremity Function and Quality of Life with a Tongue Driven Exoskeleton: A Pilot Study Quantifying Stroke Rehabilitation. Stroke Res Treat 2017, 2017.
Palermo E, Hayes DR, Russo EF, Calabro RS, Pacilli A, Filoni S. Translational effects of robot-mediated therapy in subacute stroke patients: an experimental evaluation of upper limb motor recovery. Peerj 2018, 6.
Picelli A, Munari D, Modenese A, Filippetti M, Saggioro G, Gandolfi M, Corain M, Smania N. Robot-assisted arm training for treating adult patients with distal radius fracture: a proof-of-concept pilot study. Eur J Phys Rehabil Med. 2020;56(4):444–50.
Bouteraa Y, Ben Abdallah I, Elmogy A. Design and control of an exoskeleton robot with EMG-driven electrical stimulation for upper limb rehabilitation. Industrial Robot-the International Journal of Robotics Research and Application. 2020;47(4):489–501.
Shi XQ, Heung HL, Tang ZQ, Li Z, Tong KY. Effects of a Soft Robotic Hand for Hand Rehabilitation in Chronic Stroke Survivors. J stroke Cerebrovasc diseases: official J Natl Stroke Association. 2021;30(7):105812.
Hwang CH, Seong JW, Son DS. Individual finger synchronized robot-assisted hand rehabilitation in subacute to chronic stroke: a prospective randomized clinical trial of efficacy. Clin Rehabil. 2012;26(8):696–704.
Bishop L, Gordon AM, Kim H. Hand Robotic Therapy in Children with Hemiparesis: a pilot study. Am J Phys Med Rehabil. 2017;96(1):1–7.
Villafañe JH, Taveggia G, Galeri S, Bissolotti L, Mullè C, Imperio G, Valdes K, Borboni A, Negrini S. Efficacy of short-term Robot-Assisted Rehabilitation in patients with Hand Paralysis after Stroke: a Randomized Clinical Trial. Hand (New York NY). 2018;13(1):95–102.
Kuo FL, Lee HC, Hsiao HY, Lin JC. Robotic-assisted hand therapy for improvement of hand function in children with cerebral palsy: a case series study. Eur J Phys Rehabil Med. 2020;56(2):237–42.
Klamroth-Marganska V, Blanco J, Campen K, Curt A, Dietz V, Ettlin T, Felder M, Fellinghauer B, Guidali M, Kollmar A, et al. Three-dimensional, task-specific robot therapy of the arm after stroke: a multicentre, parallel-group randomised trial. Lancet Neurol. 2014;13(2):159–66.
Vanmulken D, Spooren AIF, Bongers HMH, Seelen HAM. Robot-assisted task-oriented upper extremity skill training in cervical spinal cord injury: a feasibility study. Spinal Cord. 2015;53(7):547–51.
Kim GJ, Hinojosa J, Rao AK, Batavia M, O’Dell MW. Randomized Trial on the Effects of Attentional Focus on Motor Training of the Upper Extremity Using Robotics With Individuals After Chronic Stroke. Arch Phys Med Rehabil 2017, 98(10):1924–31.
Chen ZJ, Gu MH, He C, Xiong CH, Xu J, Huang XL. Robot-Assisted Arm Training in Stroke Individuals With Unilateral Spatial Neglect: A Pilot Study. Front Neurol 2021, 12.
Lee KS, Park JH, Beom J, Park HS. Design and Evaluation of Passive Shoulder Joint Tracking Module for Upper-Limb Rehabilitation Robots. Front Neurorobotics 2018, 12.
Kim MS, Kim SH, Noh SE, Bang HJ, Lee KM. Robotic-assisted Shoulder Rehabilitation Therapy effectively improved Poststroke Hemiplegic Shoulder Pain: a Randomized Controlled Trial. Arch Phys Med Rehabil. 2019;100(6):1015–22.
Sale P, Mazzoleni S, Lombardi V, Galafate D, Massimiani MP, Posteraro F, Damiani C, Franceschini M. Recovery of hand function with robot-assisted therapy in acute stroke patients: a randomized-controlled trial. Int J rehabilitation Res Int Z fur Rehabilitationsforschung Revue Int de recherches de readaptation. 2014;37(3):236–42.
Biggar S, Yao W. Design and evaluation of a Soft and Wearable Robotic Glove for Hand Rehabilitation. IEEE Trans neural Syst rehabilitation engineering: publication IEEE Eng Med Biology Soc. 2016;24(10):1071–80.
Orihuela-Espina F, Roldán GF, Sánchez-Villavicencio I, Palafox L, Leder R, Sucar LE, Hernández-Franco J. Robot training for hand motor recovery in subacute stroke patients: a randomized controlled trial. J hand therapy: official J Am Soc Hand Therapists. 2016;29(1):51–7. quiz 57.
Vanoglio F, Bernocchi P, Mule C, Garofali F, Mora C, Taveggia G, Scalvini S, Luisa A. Feasibility and efficacy of a robotic device for hand rehabilitation in hemiplegic stroke patients: a randomized pilot controlled study. Clin Rehabil. 2017;31(3):351–60.
Nam C, Rong W, Li W, Xie Y, Hu X, Zheng Y. The Effects of Upper-Limb Training assisted with an Electromyography-Driven Neuromuscular Electrical Stimulation Robotic Hand on Chronic Stroke. Front Neurol. 2017;8:679.
Aprile I, Germanotta M, Cruciani A, Pecchioli C, Loreti S, Papadopoulou D, Montesano A, Galeri S, Diverio M, Falsini C, et al. Poststroke shoulder pain in subacute patients and its correlation with upper limb recovery after robotic or conventional treatment: a secondary analysis of a multicenter randomized controlled trial. Int J stroke: official J Int Stroke Soc. 2021;16(4):396–405.
Shin JH, Bog Park S, Ho Jang S. Effects of game-based virtual reality on health-related quality of life in chronic stroke patients: a randomized, controlled study. Comput Biol Med. 2015;63:92–8.
Pulman J, Buckley E. Assessing the efficacy of different upper limb hemiparesis interventions on improving health-related quality of life in stroke patients: a systematic review. Top Stroke Rehabil. 2013;20(2):171–88.
Fernández-Vázquez D, Cano-de-la-Cuerda R, Navarro-López V. Haptic Glove Systems in Combination with Semi-Immersive Virtual Reality for Upper Extremity Motor Rehabilitation after Stroke: A Systematic Review and Meta-Analysis. Int J Environ Res Public Health 2022, 19(16).
Wonsick M, Padir TJAS. A systematic review of virtual reality interfaces for controlling and interacting with robots. 2020, 10(24):9051.
Guillén-Climent S, Garzo A, Muñoz-Alcaraz MN, Casado-Adam P, Arcas-Ruiz-Ruano J, Mejías-Ruiz M. Mayordomo-Riera FJJJon, rehabilitation: A usability study in patients with stroke using MERLIN, a robotic system based on serious games for upper limb rehabilitation in the home setting. 2021, 18(1):1–16.
Zanatta F, Giardini A, Pierobon A, D’Addario M, Steca P. A systematic review on the usability of robotic and virtual reality devices in neuromotor rehabilitation: patients’ and healthcare professionals’ perspective. BMC Health Serv Res. 2022;22(1):523.
Babaiasl M, Mahdioun SH, Jaryani P, Yazdani MJD, Technology RA. A review of technological and clinical aspects of robot-aided rehabilitation of upper-extremity after stroke. 2016, 11(4):263–80.
Bessler J, Prange-Lasonder GB, Schulte RV, Schaake L, Prinsen EC, Buurke JH. Occurrence and type of adverse events during the Use of Stationary Gait Robots-A systematic literature review. Front Rob AI. 2020;7:557606.
Housman SJ, Scott KM, Reinkensmeyer DJ. A randomized controlled trial of gravity-supported, computer-enhanced arm exercise for individuals with severe hemiparesis. Neurorehabilit Neural Repair. 2009;23(5):505–14.
Milot MH, Spencer SJ, Chan V, Allington JP, Klein J, Chou C, Bobrow JE, Cramer SC, Reinkensmeyer DJ. A crossover pilot study evaluating the functional outcomes of two different types of robotic movement training in chronic stroke survivors using the arm exoskeleton BONES. J Neuroeng Rehabil. 2013;10:112.
Page SJ, Hill V, White S. Portable upper extremity robotics is as efficacious as upper extremity rehabilitative therapy: a randomized controlled pilot trial. Clin Rehabil. 2013;27(6):494–503.
Cimolin V, Germiniasi C, Galli M, Condoluci C, Beretta E, Piccinini LJJoD, Disabilities P. Robot-Assisted upper limb training for hemiplegic children with cerebral palsy. 2019, 31(1):89–101.
Krebs HI, Saitoh E, Hogan N. Robotic therapy and the Paradox of the diminishing number of degrees of Freedom. Phys Med Rehabil Clin N Am. 2015;26(4):691–702.
Bessler J, Prange-Lasonder GB, Schaake L, Saenz JF, Bidard C, Fassi I, Valori M, Lassen AB, Buurke JH. Safety Assessment of Rehabilitation Robots: a review identifying Safety Skills and current knowledge gaps. Front Rob AI. 2021;8:602878.
Abu-Dakka FJ, Valera A, Escalera JA, Abderrahim M, Page A, Mata V. Passive Exercise Adaptation for Ankle Rehabilitation Based on Learning Control Framework. Sens (Basel) 2020, 20(21).
Martí Carrillo F, Butchart J, Knight S, Scheinberg A, Wise L, Sterling L. McCarthy CJAToH-RI: Adapting a general-purpose social robot for paediatric rehabilitation through in situ design. 2018, 7(1):1–30.
Chisholm KJ, Klumper K, Mullins A, Ahmadi M. A task oriented haptic gait rehabilitation robot. Mechatronics. 2014;24(8):1083–91.
Nielsen J. Usability 101: introduction to usability. Fremont, CA: Nielsen Norman Group. In.; 2012.
Monardo G, Pavese C, Giorgi I, Godi M, Colombo R. Evaluation of patient motivation and satisfaction during technology-assisted Rehabilitation: an Experiential Review. Games for health journal. 2021;10(1):13–27.
Tousignant M, Boissy P, Moffet H, Corriveau H, Cabana F, Marquis F, Simard JJT. e-Health: Patients’ satisfaction of healthcare services and perception with in-home telerehabilitation and physiotherapists’ satisfaction toward technology for post-knee arthroplasty: an embedded study in a randomized trial. 2011, 17(5):376–82.
Andrade RM, Sapienza S, Bonato P. Development of a “transparent operation mode” for a lower-limb exoskeleton designed for children with cerebral palsy. In: 2019 IEEE 16th international conference on rehabilitation robotics (ICORR): 2019 : IEEE; 2019: 512–517.
Wang J, Li J, Zhang Y, Wang S. Design of an exoskeleton for index finger rehabilitation. In: 2009 Annual International Conference of the IEEE Engineering in Medicine and Biology Society: 2009 : IEEE; 2009: 5957–5960.
Sampson M, Shau YW, King MJ. Bilateral upper limb trainer with virtual reality for post-stroke rehabilitation: case series report. Disabil rehabilitation Assist Technol. 2012;7(1):55–62.
Zhang K, Chen X, Liu F, Tang H, Wang J, Wen W. System Framework of Robotics in Upper Limb Rehabilitation on Poststroke Motor Recovery. Behavioural neurology 2018, 2018:6737056.
He Y, Eguren D, Luu TP, Contreras-Vidal JL. Risk management and regulations for lower limb medical exoskeletons: a review. Med devices (Auckland NZ). 2017;10:89–107.
Akiyama Y, Yamada Y, Ito K, Oda S, Okamoto S, Hara S. Test method for contact safety assessment of a wearable robot -analysis of load caused by a misalignment of the knee joint. In: 2012 IEEE RO-MAN: The 21st IEEE International Symposium on Robot and Human Interactive Communication: 9–13 Sept. 2012 2012 ; 2012: 539–544.
Melia M, Geissler B, König J, Ottersbach HJ, Umbreit M, Letzel S, Muttray A. Pressure pain thresholds: subject factors and the meaning of peak pressures. Eur J Pain. 2019;23(1):167–82.
Ferreira F, Chaves MEA, Oliveira VC, Van Petten A, Vimieiro CBS. Effectiveness of robot therapy on body function and structure in people with limited upper limb function: a systematic review and meta-analysis. PLoS ONE. 2018;13(7):e0200330.
Lohse KR, Lang CE, Boyd LA. Is more better? Using metadata to explore dose-response relationships in stroke rehabilitation. Stroke. 2014;45(7):2053–8.
Hayward KS, Brauer SG. Dose of arm activity training during acute and subacute rehabilitation post stroke: a systematic review of the literature. Clin Rehabil. 2015;29(12):1234–43.
Kwakkel, GJp. Intensity of practice after stroke: More is better. 2009, 7:24.
Wilson RD, Chae J. Hemiplegic Shoulder Pain. Phys Med Rehabil Clin North Am. 2015;26(4):641–55.
Tran DA, Pajaro-Blazquez M, Daneault JF, Gallegos JG, Pons J, Fregni F, Bonato P, Zafonte R. Combining Dopaminergic Facilitation with Robot-Assisted Upper Limb Therapy in Stroke Survivors: a focused review. Am J Phys Med Rehabil. 2016;95(6):459–74.
Kafri M, Myslinski MJ, Gade VK, Deutsch JE. Energy expenditure and exercise intensity of interactive video gaming in individuals poststroke. Neurorehabil Neural Repair. 2014;28(1):56–65.
Cuesta-Gómez A, Sánchez-Herrera-Baeza P, Oña-Simbaña ED, Martínez-Medina A, Ortiz-Comino C, Balaguer-Bernaldo-de-Quirós C, Jardón-Huete A. Cano-de-la-Cuerda RJJon, rehabilitation: Effects of virtual reality associated with serious games for upper limb rehabilitation in patients with multiple sclerosis: Randomized controlled trial. 2020, 17(1):1–10.
Laut J, Porfiri M, Raghavan P. The Present and Future of Robotic Technology in Rehabilitation. Curr Phys Med Rehabilitation Rep. 2016;4(4):312–9.
Francisco GE, Yozbatiran N, Berliner J, OʼMalley MK, Pehlivan AU, Kadivar Z, Fitle K, Boake C. Robot-Assisted training of arm and Hand Movement shows functional improvements for incomplete cervical spinal cord Injury. Am J Phys Med Rehabil. 2017;96(10 Suppl 1):171–s177.
Tousignant M, Boissy P, Moffet H, Corriveau H, Cabana F, Marquis F, Simard J. Patients’ satisfaction of healthcare services and perception with in-home telerehabilitation and physiotherapists’ satisfaction toward technology for post-knee arthroplasty: an embedded study in a randomized trial. Telemedicine J e-health: official J Am Telemedicine Association. 2011;17(5):376–82.
Shirley ED, Sanders JO. Patient satisfaction: implications and predictors of success. J bone joint Surg Am volume. 2013;95(10):e69.
Monardo G, Pavese C, Giorgi I, Godi M, Colombo RJGfhj. Evaluation of patient motivation and satisfaction during technology-assisted rehabilitation: an experiential review. 2021, 10(1):13–27.
Kwon SH, Lee BS, Lee HJ, Kim EJ, Lee JA, Yang SP, Kim TY, Pak HR, Kim HK, Kim HYJAoRM. Energy efficiency and patient satisfaction of gait with knee-ankle-foot orthosis and robot (ReWalk)-assisted gait in patients with spinal cord injury. 2020, 44(2):131–41.
Kim DJ, Hazlett-Knudsen R, Culver-Godfrey H, Rucks G, Cunningham T, Portee D, Bricout J, Wang Z, Behal A. How autonomy impacts performance and satisfaction: results from a study with spinal cord injured subjects using an Assistive Robot. IEEE Trans Syst Man Cybernetics - Part A: Syst Hum. 2012;42(1):2–14.
Ramos Muñoz EJ, Swanson VA, Johnson C, Anderson RK, Rabinowitz AR, Zondervan DK, Collier GH, Reinkensmeyer DJ. Using large-scale Sensor Data to test factors predictive of perseverance in Home Movement Rehabilitation: optimal challenge and steady Engagement. Front Neurol. 2022;13:896298.
Akbari A, Haghverd F, Behbahani S. Robotic home-based Rehabilitation Systems Design: from a literature review to a conceptual Framework for Community-Based remote therapy during COVID-19 pandemic. Front Rob AI. 2021;8:612331.
Kutner NG, Zhang R, Butler AJ, Wolf SL, Alberts JL. Quality-of-life change Associated with robotic-assisted therapy to Improve Hand Motor function in patients with Subacute Stroke: a Randomized Clinical Trial. Phys Ther. 2010;90(4):493–504.
Mohammed SA, Shebl AM. Quality of life among egyptian patients with Upper and Lower Limb Amputation: sex differences. Adv Med. 2014;2014:674323.
Rasouli S, Gupta G, Nilsen E, Dautenhahn K. Potential applications of Social Robots in Robot-Assisted interventions for social anxiety. Int J Social Robot. 2022;14(5):1–32.
Kawatsuma S, Mimura R, Asama H. Unitization for portability of emergency response surveillance robot system: experiences and lessons learned from the deployment of the JAEA-3 emergency response robot at the Fukushima Daiichi Nuclear Power plants. ROBOMECH J. 2017;4(1):6.
Treviño LR, Roberge P, Auer ME, Morales A, Torres-Reveron A. Predictors of functional outcome in a cohort of hispanic patients using Exoskeleton Rehabilitation for Cerebrovascular Accidents and Traumatic Brain Injury. Front Neurorobotics. 2021;15:682156.
Download references
Acknowledgements
The authors thank the Central Library and Documentation Center of Kerman University of Medical Sciences for providing access to the knowledge base references required for this study.
This study was supported by the Medical Informatics Research Center of Kerman University of Medical Sciences (Code: 400000856). The funder had no roles in study design, data gathering and analysis.
Author information
Authors and affiliations.
Medical Informatics Research Center, Institute for Futures Studies in Health, Kerman University of Medical Sciences, Kerman, Iran
Khadijeh Moulaei & Kambiz Bahaadinbeigy
HIV/STI Surveillance Research Center, WHO Collaborating Center for HIV Surveillance, Institute for Futures Studies in Health, Kerman University of Medical Sciences, Kerman, Iran
Ali Akbar Haghdoostd
Department of Physical Therapy, Faculty of Allied Medicine, Kerman University of Medical Sciences, Kerman, Iran
Mansour Shahabi Nezhad
Department of Health Information Management, School of Health Management and Information Sciences, Iran University of Medical Sciences, Tehran, Iran
Abbas Sheikhtaheri
You can also search for this author in PubMed Google Scholar
Contributions
Authors’ contributions: KHM and ASH have designed the study, finalized the search strategy and implemented the thematic analysis, AH and KB have searched and screened the articles and extracted the initial codes for data charting. ASH has supervised the whole review process, KHM has contributed in revising the finalizing data analysis and KB and ASH have technically edited and finalized the article. The authors read and approved the final manuscript.
Corresponding author
Correspondence to Abbas Sheikhtaheri .
Ethics declarations
Ethical approval, consent for publication.
Not applicable.
Competing interests
The authors declare that there are no conflicts of interest.
Additional information
Publisher’s note.
Springer Nature remains neutral with regard to jurisdictional claims in published maps and institutional affiliations.
Electronic supplementary material
Below is the link to the electronic supplementary material.
Supplementary Material 1 Aims and scope statement
Supplementary material 2: appendix a, supplementary material 3: appendix b, supplementary material 4: appendix c, rights and permissions.
Open Access This article is licensed under a Creative Commons Attribution 4.0 International License, which permits use, sharing, adaptation, distribution and reproduction in any medium or format, as long as you give appropriate credit to the original author(s) and the source, provide a link to the Creative Commons licence, and indicate if changes were made. The images or other third party material in this article are included in the article’s Creative Commons licence, unless indicated otherwise in a credit line to the material. If material is not included in the article’s Creative Commons licence and your intended use is not permitted by statutory regulation or exceeds the permitted use, you will need to obtain permission directly from the copyright holder. To view a copy of this licence, visit http://creativecommons.org/licenses/by/4.0/ . The Creative Commons Public Domain Dedication waiver ( http://creativecommons.org/publicdomain/zero/1.0/ ) applies to the data made available in this article, unless otherwise stated in a credit line to the data.
Reprints and permissions
About this article
Cite this article.
Moulaei, K., Bahaadinbeigy, K., Haghdoostd, A.A. et al. Overview of the role of robots in upper limb disabilities rehabilitation: a scoping review. Arch Public Health 81 , 84 (2023). https://doi.org/10.1186/s13690-023-01100-8
Download citation
Received : 25 November 2022
Accepted : 29 April 2023
Published : 08 May 2023
DOI : https://doi.org/10.1186/s13690-023-01100-8
Share this article
Anyone you share the following link with will be able to read this content:
Sorry, a shareable link is not currently available for this article.
Provided by the Springer Nature SharedIt content-sharing initiative
- Rehabilitation
- Disabilities
- Digital Health
Archives of Public Health
ISSN: 2049-3258
- General enquiries: [email protected]
An Exploratory Literature Review of Robots and Their Interaction as Assistive Technology for Persons with Disabilities: Focus on Promoting Activity and Participation
- Conference paper
- First Online: 15 March 2024
- Cite this conference paper
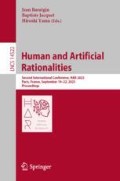
- Kai Seino ORCID: orcid.org/0000-0002-9359-4279 10
Part of the book series: Lecture Notes in Computer Science ((LNCS,volume 14522))
Included in the following conference series:
- International Conference on Human and Artificial Rationalities
53 Accesses
According to the United Nations, approximately 15% of the world’s population has some form of disability. Although the development of service robots to support those people with disabilities is increasing, there has not been enough comprehensive discussion on the research and effectiveness of robots as assistive technology for people with disabilities. This study conducted an exploratory literature review to determine the current status of previous research on robots to assist people with disabilities and to provide a basis for research on robots to assist people with disabilities. A literature review was conducted using PubMed as the database to identify current research trends. The search was conducted on August 1, 2021, using the keywords “disabled” and “robotics” and 49 articles were identified. After an in-depth screening process, 43 studies were deemed eligible for further analysis. As a result, the previous studies covered a variety of robot applications, including robot-assisted gait training, robot-assisted play activities, and robot-assisted task-oriented upper limb skills training. Physical disabilities accounted for the majority of the disability types in the previous study (81.4%), of which 94.3% were targeted for movement disorders. Therefore, the results of this study suggest that research on robots for the disabled mainly targets movement disorders such as limb inconvenience caused by physical disabilities. Regarding the purpose of using robots to overcome these motor impairments, most of the research was on support and training for upper and lower limb movements. Effects on participation or activity were found in 54.5% of the articles dealing with movement disorders. It was suggested that robots can support activity and participation for movement disorders of people with disabilities and have some effectiveness. On the other hand, 11.6% of the previous studies dealing with cognitive aspects of disability were less common than those dealing with motor aspects. In conclusion, robots hold great promise in improving the quality of life of people with disabilities, especially in promoting activity and participation.
This is a preview of subscription content, log in via an institution to check access.
Access this chapter
- Available as PDF
- Read on any device
- Instant download
- Own it forever
- Available as EPUB and PDF
- Compact, lightweight edition
- Free shipping worldwide - see info
Tax calculation will be finalised at checkout
Purchases are for personal use only
Institutional subscriptions
Hirokawa, M., Funahashi, A., Suzuki, K.: A doll-type interface for real-time humanoid teleoperation in robot-assisted activity: a case study. In: Proceedings of the 2014 ACM/IEEE International Conference on Human-Robot Interaction, pp. 174–175 (2014). https://doi.org/10.1145/2559636.2563680
Hirokawa, M., Funahashi, A., Itoh, Y., Suzuki, K.: Design of affective robot-assisted activity for children with autism spectrum disorders. In: Proceedings of the 23rd IEEE International Symposium on Robot and Human Interactive Communication, pp. 365–370 (2014). https://doi.org/10.1109/ROMAN.2014.6926280
Jain, S., Thiagarajan, B., Shi, A., Clabaughand, C., Matarić, M.J.: Modeling engagement in long-term, in-home socially assistive robot interventions for children with autism spectrum disorders. Sci. Robot. 5 (39), eaaz3791 (2020). https://doi.org/10.1126/scirobotics.aaz3791
Shizume, C.: Enabling technology at robot cafe redefines work for disability. Zenbird (2019). https://zenbird.media/enabling-technology-at-robot-cafe-redefines-work-for-disability/ . Accessed 01 Aug 2021
WHO: International Classification of Functioning, Disability and Health. World Health Organization, Geneva (2002)
Google Scholar
Gimigliano F, et al.: Robot-assisted arm therapy in neurological health conditions: rationale and methodology for the evidence synthesis in the CICERONE Italian Consensus Conference. Eur. J. Phys. Rehabil. Med. (2021). https://doi.org/10.23736/S1973-9087.21.07011-8 . Italian consensus conference on robotics in neurorehabilitation (CICERONE)
Aliaj, K., et al.: Replicating dynamic humerus motion using an industrial robot. PLoS One 15 (11), e0242005 (2020). https://doi.org/10.1371/journal.pone.0242005
Buitrago, J.A., Bolaños, A.M., Caicedo, B.E.: A motor learning therapeutic intervention for a child with cerebral palsy through a social assistive robot. Disabil. Rehabil. Assist. Technol. 15 (3), 357–362 (2020). https://doi.org/10.1080/17483107.2019.1578999
Article Google Scholar
Kostrubiec, V., Kruck, J.: Collaborative research project: developing and testing a robot-assisted intervention for children with autism. Front. Rob. AI 7 , 37 (2020). https://doi.org/10.3389/frobt.2020.00037
Mohammadi, M., Knoche, H., Gaihede, M., Bentsen, B., Andreasen Struijk, L.N.S.: A high-resolution tongue-based joystick to enable robot control for individuals with severe disabilities. In: 16th IEEE International Conference on Rehabilitation Robotics, pp. 1043–1048 (2019). https://doi.org/10.1109/ICORR.2019.8779434
Sattelmayer, M., Chevalley, O., Steuri, R., Hilfiker, R.: Over-ground walking or robot-assisted gait training in people with multiple sclerosis: does the effect depend on baseline walking speed and disease related disabilities? A systematic review and meta-regression. BMC Neurol. 19 (1), 93 (2019). https://doi.org/10.1186/s12883-019-1321-7
Zhang, J., Wang, B., Zhang, C., Xiao, Y., Wang, M.Y.: An EEG/EMG/EOG-based multimodal human-machine interface to real-time control of a soft robot hand. Front. Neurorobot. 29 (13), 7 (2019). https://doi.org/10.3389/fnbot.2019.00007
Ricklin, S., Meyer-Heim, A., van Hedel, H.J.A.: Dual-task training of children with neuromotor disorders during robot-assisted gait therapy: prerequisites of patients and influence on leg muscle activity. J. Neuroeng. Rehabil. 15 (1), 82 (2018). https://doi.org/10.1186/s12984-018-0426-3
Sakamaki, I., et al.: Preliminary testing by adults of a haptics-assisted robot platform designed for children with physical impairments to access play. Assist. Technol. 30 (5), 242–250 (2018). https://doi.org/10.1080/10400435.2017.1318974
Guo, C., Guo, S., Ji, J., Xi, F.: Iterative learning impedance for lower limb rehabilitation robot. J. Healthc. Eng. 2017 , 6732459 (2017). https://doi.org/10.1155/2017/6732459
Straudi, S., et al.: The effectiveness of robot-assisted gait training versus conventional therapy on mobility in severely disabled progressive MultiplE sclerosis patients (RAGTIME): study protocol for a randomized controlled trial. Trials 18 (1), 88 (2017). https://doi.org/10.1186/s13063-017-1838-2
Tidoni, E., Gergondet, P., Fusco, G., Kheddar, A., Aglioti, S.M.: The role of audio-visual feedback in a thought-based control of a humanoid robot: a BCI study in healthy and spinal cord injured people. IEEE Trans. Neural Syst. Rehabil. Eng. 25 (6), 772–781 (2017). https://doi.org/10.1109/TNSRE.2016.2597863
van den Heuvel, R.J.F., Lexis, M.A.S., de Witte, L.P.: Robot ZORA in rehabilitation and special education for children with severe physical disabilities: a pilot study. Int. J. Rehabil. Res. 40 (4), 353–359 (2017). https://doi.org/10.1097/MRR.0000000000000248
Ríos-Rincón, A.M., Adams, K., Magill-Evans, J., Cook, A.: Playfulness in children with limited motor abilities when using a robot. Phys. Occup. Ther. Pediatr. 36 (3), 232–246 (2016). https://doi.org/10.3109/01942638.2015.1076559
Ferm, U.M., Claesson, B.K., Ottesjö, C., Ericsson, S.: Participation and enjoyment in play with a robot between children with cerebral palsy who use AAC and their peers. Augment. Altern. Commun. 31 (2), 108–123 (2015). https://doi.org/10.3109/07434618.2015.1029141
Feys, P., et al.: Robot-supported upper limb training in a virtual learning environment: a pilot randomized controlled trial in persons with MS. J. Neuroeng. Rehabil. 12 , 60 (2015). https://doi.org/10.1186/s12984-015-0043-3
Peri, E., et al.: An ecological evaluation of the metabolic benefits due to robot-assisted gait training. In: 37th Annual International Conference of the IEEE Engineering in Medicine and Biology Society, pp. 3590–3593 (2015). https://doi.org/10.1109/EMBC.2015.7319169
Phelan, S.K., Gibson, B.E., Wright, F.V.: What is it like to walk with the help of a robot? Children’s perspectives on robotic gait training technology. Disabil. Rehabil. 37 (24), 2272–2281 (2015). https://doi.org/10.3109/09638288.2015.1019648
Vanmulken, D.A., Spooren, A.I., Bongers, H.M., Seelen, H.A.: Robot-assisted task-oriented upper extremity skill training in cervical spinal cord injury: a feasibility study. Spinal Cord. 53 (7), 547–551 (2015). https://doi.org/10.1038/sc.2014.250
Yamaguchi, J., Parone, C., Di Federico, D., Beomonte Zobel, P., Felzani, G.: Measuring benefits of telepresence robot for individuals with motor impairments. In: Sik-Lányi, C., Hoogerwerf, E.J., Miesenberger, K., Cudd, P. (eds.) Studies in Health Technology and Informatics, Volume 2017: Assistive Technology, pp. 703–709. IOS Press (2015). https://doi.org/10.3233/978-1-61499-566-1-703
Encarnação, P., Alvarez, L., Rios, A., Maya, C., Adams, K., Cook, A.: Using virtual robot-mediated play activities to assess cognitive skills. Disabil. Rehabil. Assist. Technol. 9 (3), 231–241 (2014). https://doi.org/10.3109/17483107.2013.782577
Pearson, Y., Borenstein, J.: The intervention of robot caregivers and the cultivation of children’s capability to play. Sci. Eng. Ethics 19 (1), 123–137 (2013). https://doi.org/10.1007/s11948-011-9309-8
Lebec, O., et al.: High level functions for the intuitive use of an assistive robot. In: 2013 IEEE International Conference on Rehabilitation Robotics, pp. 1–6 (2013). https://doi.org/10.1109/ICORR.2013.6650374
Fasoli, S.E., Ladenheim, B., Mast, J., Krebs, H.I.: New horizons for robot-assisted therapy in pediatrics. Am. J. Phys. Med. Rehabil. 91 (11 Suppl 3), S280-289 (2012). https://doi.org/10.1097/PHM.0b013e31826bcff4
Reinkensmeyer, D.J., Wolbrecht E.T., Chan, V., Chou, C., Cramer, S.C., Bobrow, J.E.: Comparison of 3D, assist-as-needed robotic arm/hand movement training provided with Pneu-WREX to conventional table top therapy following chronic stroke. Am. J. Phys. Med. Rehabil. 91 (11 Suppl 3), S232–S241 (2012). https://doi.org/10.1097/PHM.0b013e31826bce79
Swinnen, E., Beckwée, D., Pinte, D., Meeusen, R., Baeyens, J.P., Kerckhofs, E.: Treadmill training in multiple sclerosis: can body weight support or robot assistance provide added value? A systematic review. Mult. Scler. Int. 2012 , 240274 (2012). https://doi.org/10.1155/2012/240274
Carrera, I., Moreno, H.A., Saltarén, R., Pérez, C., Puglisi, L., Garcia, C.: ROAD: domestic assistant and rehabilitation robot. Med. Biol. Eng. Comput. 49 (10), 1201 (2011). https://doi.org/10.1007/s11517-011-0805-4
Jardón, A., Gil, Á.M., de la Peña, A.I., Monje, C.A., Balaguer, C.: Usability assessment of ASIBOT: a portable robot to aid patients with spinal cord injury. Disabil. Rehabil. Assist. Technol. 6 (4), 320–330 (2011). https://doi.org/10.3109/17483107.2010.528144
Tonin, L., Carlson, T., Leeb, R., del R Millán, J.: Brain-controlled telepresence robot by motor-disabled people. In: 2011 Annual International Conference of the IEEE Engineering in Medicine and Biology Society, pp. 4227–4230 (2011). https://doi.org/10.1109/IEMBS.2011.6091049
Micera, S., et al.: On the control of a robot hand by extracting neural signals from the PNS: preliminary results from a human implantation. In: 2009 Annual International Conference of the IEEE Engineering in Medicine and Biology Society, pp. 4586–4589 (2009). https://doi.org/10.1109/IEMBS.2009.5332764
Kulyukin, V., Gharpure, C., Coster, D.: Robot-assisted shopping for the visually impaired: proof-of-concept design and feasibility evaluation. Assist. Technol. 20 (2), 86–98 (2008). https://doi.org/10.1080/10400435.2008.10131935
Billard, A., Robins, B., Nadel, J., Dautenhahn, K.: Building Robota, a mini-humanoid robot for the rehabilitation of children with autism. Assist. Technol. 19 (1), 37–49 (2007). https://doi.org/10.1080/10400435.2007.10131864
Kamnik, R., Bajd, T.: Human voluntary activity integration in the control of a standing-up rehabilitation robot: a simulation study. Med. Eng. Phys. 29 (9), 1019–1029 (2007). https://doi.org/10.1016/j.medengphy.2006.09.012
Parsons, B., White, A., Prior, S., Warner, P.: The Middlesex university rehabilitation robot. J. Med. Eng. Technol. 29 (4), 151–162 (2005). https://doi.org/10.1080/03091900412331298898
Brisben, A.J., Lockerd, A.D., Lathan, C.: Design evolution of an interactive robot for therapy. Telemed. J. E Health 10 (2), 252–259 (2004). https://doi.org/10.1089/tmj.2004.10.252
Hesse, S., Schulte-Tigges, G., Konrad, M., Bardeleben, A., Werner, C.: Robot-assisted arm trainer for the passive and active practice of bilateral forearm and wrist movements in hemiparetic subjects. Arch. Phys. Med. Rehabil. 84 (6), 915–920 (2003). https://doi.org/10.1016/S0003-9993(02)04954-7
Bai, O., Nakamura, M., Shibasaki, H.: Compensation of hand movement for patients by assistant force: relationship between human hand movement and robot arm motion. IEEE Trans. Neural Syst. Rehabil. Eng. 9 (3), 302–307 (2001). https://doi.org/10.1109/7333.948459
Driessen, B.J., Evers, H.G., van Woerden, J.A.: MANUS–a wheelchair-mounted rehabilitation robot. Proc. Inst. Mech. Eng. H 215 (3), 285–290 (2001). https://doi.org/10.1243/0954411011535876
Hoppenot, P., Colle, E.: Localization and control of a rehabilitation mobile robot by close human-machine cooperation. IEEE Trans. Neural Syst. Rehabil. Eng. 9 (2), 181–190 (2001). https://doi.org/10.1109/7333.928578
Chen, S., Rahman, T., Harwin, W.: Performance statistics of a head-operated force-reflecting rehabilitation robot system. IEEE Trans. Rehabil. Eng. 6 (4), 406–414 (1998). https://doi.org/10.1109/86.736155
Morvan, J.S., Guichard, J.P., Torossian, V.: Technical aids for the physically handicapped: a psychological study of the master robot. Int. J. Rehabil. Res. 20 (2), 193–197 (1997). https://doi.org/10.1097/00004356-199706000-00009
Lancioni, G.E., Oliva, D., Signorino, M.: Promoting ambulation and object manipulation in persons with multiple handicaps through the use of a robot. Percept. Mot. Skills 79 (2), 843–848 (1994). https://doi.org/10.2466/pms
Harwin, W.S., Ginige, A., Jackson, R.D.: A robot workstation for use in education of the physically handicapped. IEEE Trans. Biomed. Eng. 35 (2), 127–131 (1988). https://doi.org/10.1109/10.1350
Papadopoulou, M.T., et al.: Efficacy of a robot-assisted intervention in improving learning performance of elementary school children with specific learning disorders. Child. (Basel) 9 (8), 1155 (2022). https://doi.org/10.3390/children9081155
Download references
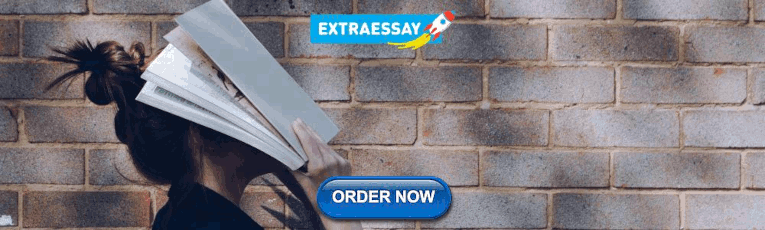
Author information
Authors and affiliations.
National Rehabilitation Center for Persons With Disabilities, Namiki 4-1, Tokorozawa, Japan
You can also search for this author in PubMed Google Scholar
Corresponding author
Correspondence to Kai Seino .
Editor information
Editors and affiliations.
Université Paris 8, Saint Denis, France
Jean Baratgin
Université Paris 8, Saint-Denis, France
Baptiste Jacquet
Osaka City University, Osaka, Japan
Hiroshi Yama
Rights and permissions
Reprints and permissions
Copyright information
© 2024 The Author(s), under exclusive license to Springer Nature Switzerland AG
About this paper
Cite this paper.
Seino, K. (2024). An Exploratory Literature Review of Robots and Their Interaction as Assistive Technology for Persons with Disabilities: Focus on Promoting Activity and Participation. In: Baratgin, J., Jacquet, B., Yama, H. (eds) Human and Artificial Rationalities. HAR 2023. Lecture Notes in Computer Science, vol 14522. Springer, Cham. https://doi.org/10.1007/978-3-031-55245-8_21
Download citation
DOI : https://doi.org/10.1007/978-3-031-55245-8_21
Published : 15 March 2024
Publisher Name : Springer, Cham
Print ISBN : 978-3-031-55244-1
Online ISBN : 978-3-031-55245-8
eBook Packages : Computer Science Computer Science (R0)
Share this paper
Anyone you share the following link with will be able to read this content:
Sorry, a shareable link is not currently available for this article.
Provided by the Springer Nature SharedIt content-sharing initiative
- Publish with us
Policies and ethics
- Find a journal
- Track your research
- Open access
- Published: 15 February 2023
Literature review of stroke assessment for upper-extremity physical function via EEG, EMG, kinematic, and kinetic measurements and their reliability
- Rene M. Maura ORCID: orcid.org/0000-0001-6023-9038 1 ,
- Sebastian Rueda Parra 4 ,
- Richard E. Stevens 2 ,
- Douglas L. Weeks 3 ,
- Eric T. Wolbrecht 1 &
- Joel C. Perry 1
Journal of NeuroEngineering and Rehabilitation volume 20 , Article number: 21 ( 2023 ) Cite this article
5987 Accesses
15 Citations
Metrics details
Significant clinician training is required to mitigate the subjective nature and achieve useful reliability between measurement occasions and therapists. Previous research supports that robotic instruments can improve quantitative biomechanical assessments of the upper limb, offering reliable and more sensitive measures. Furthermore, combining kinematic and kinetic measurements with electrophysiological measurements offers new insights to unlock targeted impairment-specific therapy. This review presents common methods for analyzing biomechanical and neuromuscular data by describing their validity and reporting their reliability measures.
This paper reviews literature (2000–2021) on sensor-based measures and metrics for upper-limb biomechanical and electrophysiological (neurological) assessment, which have been shown to correlate with clinical test outcomes for motor assessment. The search terms targeted robotic and passive devices developed for movement therapy. Journal and conference papers on stroke assessment metrics were selected using PRISMA guidelines. Intra-class correlation values of some of the metrics are recorded, along with model, type of agreement, and confidence intervals, when reported.
A total of 60 articles are identified. The sensor-based metrics assess various aspects of movement performance, such as smoothness, spasticity, efficiency, planning, efficacy, accuracy, coordination, range of motion, and strength. Additional metrics assess abnormal activation patterns of cortical activity and interconnections between brain regions and muscle groups; aiming to characterize differences between the population who had a stroke and the healthy population.
Range of motion, mean speed, mean distance, normal path length, spectral arc length, number of peaks, and task time metrics have all demonstrated good to excellent reliability, as well as provide a finer resolution compared to discrete clinical assessment tests. EEG power features for multiple frequency bands of interest, specifically the bands relating to slow and fast frequencies comparing affected and non-affected hemispheres, demonstrate good to excellent reliability for populations at various stages of stroke recovery. Further investigation is needed to evaluate the metrics missing reliability information. In the few studies combining biomechanical measures with neuroelectric signals, the multi-domain approaches demonstrated agreement with clinical assessments and provide further information during the relearning phase. Combining the reliable sensor-based metrics in the clinical assessment process will provide a more objective approach, relying less on therapist expertise. This paper suggests future work on analyzing the reliability of metrics to prevent biasedness and selecting the appropriate analysis.
Stroke is one of the leading causes of death and disability in developed countries. In the United States, a stroke occurs every 40 s, ranking stroke as the fifth leading cause of death and the first leading cause of disability in the country [ 1 ]. The high prevalence of stroke, coupled with increasing stroke survival rates, puts a growing strain on already limited healthcare resources; the cost of therapy is elevated [ 2 ] and restricted mostly to a clinical setting [ 3 ], leading to 50% of survivors that reach the chronic stage experiencing severe motor disability for upper extremities [ 4 ]. This highlights the need for refined (improved) assessment which can help pair person-specific impairment with appropriately targeted therapeutic strategies.
Rehabilitation typically starts with a battery of standardized tests to assess impairment and function. This initial evaluation serves as a baseline of movement capabilities and usually includes assessment of function during activities of daily living (ADL). Because these clinical assessments rely on trained therapists as raters, the scoring scale is designed to be discrete and, in some cases, bounded. While this improves the reliability of the metric [ 5 ] (i.e., raters more likely to agree), it also reduces the sensitivity of the scale. Furthermore, those assessment scales that are bounded, such as the Fugl-Meyer Assessment (FMA) [ 6 ], Ashworth or Modified Ashworth (MA) Scale [ 7 ], and Barthel Index [ 8 ], suffer from floor/ceiling effects where the limits of the scales become insensitive to the extremes of impairment and function. It is therefore important to develop new clinical assessment methods that are objective, quantifiable, reliable, and sensitive to change over the full range of function and impairment.
Over the last several decades, robotic devices have been designed and studied for administering post-stroke movement therapy. These devices have begun being adopted into clinical rehabilitation practice. More recently, researchers have proposed and studied the use of robotic devices to assess stroke-related impairments as an approach to overcome the limitations of existing clinical measures previously discussed [ 9 , 10 , 11 , 12 ]. Robots may be equipped with sensitive measurement devices that can be used to rate the person’s performance in a predefined task. These devices can include measuring kinematic (position/velocity), kinetic (force/torque), and/or neuromuscular (electromyography/electroencephalography) output from the subject during the task. Common sensor-based robotic metrics for post-stroke assessment included speed of response, planning time, movement planning, smoothness, efficiency, range, and efficacy [ 13 , 14 ]. Figure 1 demonstrates an example method for comprehensive assessment of a person who has suffered a stroke with data acquired during robotically administered tests. Furthermore, there is potential for new and more comprehensive knowledge to be gained from a wider array of assessment methods and metrics that combine the benefits of biomechanical (e.g., kinematic and kinetic) and neurological (e.g., electromyographic and electroencephalographic) measures [ 15 , 16 , 17 , 18 , 19 , 20 , 21 , 22 ].
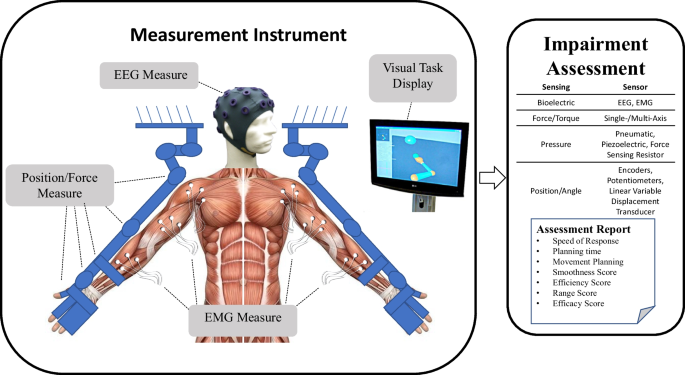
Example of instrument for upper extremities bilateral biomechanical and neuromuscular assessment. From this data, a wide variety of measures and metrics for assessment of upper-extremity impairment and function may be reported
- Biomechanical assessment
Many classical methods of assessing impairment or function involve manual and/or instrumented quantification of performance through measures of motion (i.e., kinematic) and force (i.e., kinetic) capabilities. These classical methods rely on the training of the therapist to evaluate the capabilities of the person through keen observation (e.g., FMA [ 6 ] and MA [ 7 ]). The quality of kinematic and kinetic measures can be improved with the use of electronic-based measurements [ 23 ]. Robotic devices equipped with electronic sensors have the potential to improve the objectivity, sensitivity, and reliability of the assessment process by providing a means for more quantitative, precise, and accurate information [ 9 , 10 , 11 , 12 , 24 , 25 , 26 , 27 , 28 ]. Usually, the electronic sensors on a rehabilitation robotic device are used for control purposes [ 29 , 30 , 31 ]. Robotics can also measure movement outputs, such as force or joint velocities, which the clinician may not be able to otherwise measure as accurately (or simultaneously) using existing clinical assessment methods [ 23 ]. With accurate and repeatable measurement of forces and joint velocities, sensor-based assessments have the potential to assess the person’s movement in an objective and quantifiable way. This article reviews validity and reliability of biomechanical metrics in relationship to assessment of motor function for upper extremities.
Electrophysiological features for assessment
Neural signals that originate from the body can be measured using non-invasive methods. Among others, electroencephalograms (EEG) measure cortical electrical activity, and electromyograms (EMG) measure muscle electrical activity. The relative low cost, as well as the noninvasive nature of these technologies make them suitable for studying changes in cortical or muscle activation caused by conditions or injuries of the brain, such as the ones elicited by stroke lesions [ 32 ].
Initially, EMG/EEG were used strictly as clinical diagnostic tools [ 33 , 34 ]. Recent improvements in signal acquisition hardware and computational processing methods have increased their use as viable instruments for understanding and treating neuromuscular diseases and neural conditions [ 32 ]. Features extracted from these signals are being researched to assess their relationship to motor and cognitive deficits [ 35 , 36 , 37 , 38 , 39 , 40 , 41 , 42 ] and delayed ischemia [ 34 , 43 ], as well as to identify different uses of the signals that could aid rehabilitation [ 44 ]. Applications of these features in the context of stroke include: (1) commanding robotic prostheses [ 45 , 46 ], exoskeletons [ 21 , 47 , 48 ], and brain-machine interfaces [ 44 , 49 , 50 , 51 ]; and (2) bedside monitoring for sub-acute patients and thrombolytic therapy [ 52 , 53 , 54 ]. Here we review the validity and reliability of metrics derived from electrophysiological signals in relationship to stroke motor assessment for upper extremity.
Reliability of metrics
Robotic or sensor-based assessment tools have not gained widespread clinical acceptance for stroke assessment. Numerous barriers to their clinical adoption remain, including demonstrating their reliability and providing sufficient validation of robotic metrics with respect to currently accepted assessment techniques [ 55 ]. In the assessment of motor function with sensor-based systems, several literature reviews reveal a wide spectrum of sensor-based metrics to use for stroke rehabilitation and demonstrate their validity [ 13 , 42 , 56 , 57 , 58 , 59 , 63 , 64 ]. However, in addition to demonstrating validity, new clinical assessments must also demonstrate good or excellent reliability in order to support their adoption in the clinical field. This is achieved by: (1) comparing multiple measurements on the same subject (test–retest reliability), and (2) checking agreement between multiple raters of the same subject (inter-rater reliability). Reliability quantifies an assessment’s ability to deliver scores that are free from measurement error [ 65 ]. Previous literature reviews have presented limited, if any, information on the reliability of the biomechanical robotic metrics. Murphy and Häger [ 66 ], Wang et al. [ 56 ], and Shishov et al. [ 67 ] reviewed reliability, but omitted some important aspects of intra-class correlation methods used in the study (e.g., the model type and/or the confidence interval), which are required when analyzing intra-class correlation methods for reliability [ 68 ]. If the reliability is not properly analyzed and reported, the study runs the risk of having a biased result. Murphy and Häger [ 66 ] also found a lack of studies determining the reliability of metrics in 2015. Since electronic-based assessments require the use of a therapist or an operator to administer the test, an inter-observer reliability test should be investigated to observe the effect of the test administrators on the assessment process. Therefore, both test–retest and inter-observer reliability in biomechanical and electrophysiological metrics are reviewed to provide updated information on the current findings of the metrics’ reliability.
Integrated metrics
Over the past 50 years, numerous examples of integrated metrics have provided valuable insight into the inner workings of human arm function. In the 1970s EMG was combined with kinematic data in patients with spasticity to understand muscle patterns during ballistic arm reach movements [ 69 ], the affects of pharmacological intervention on spastic stretch reflexes during passive vs. voluntary movement [ 70 ], and in the 1990s EMG was combined with kinetic data to understand the effects of abnormal synergy patterns on reach workspace when lifting the arm against gravity [ 71 ]. This work dispelled long-standing theories of muscular weakness and spasticity alone being the major contributors to arm impairment. More recently, quantified aspects of processed EEG and EMG signals are being combined with kinematic data to investigate the compensatory role, and relation to shoulder-related abnormal muscle synergies of the contralesional secondary sensorimotor cortex, in a group of chronic stroke survivors [ 72 ]. These and other works demonstrate convincingly the value of combined metrics and the insights they can uncover that isolated metrics cannot discover alone.
To provide further information on the stroke severity and the relearning process during stroke therapy, researchers are investigating a multi-modal approach using biomechanical and neuromuscular features [ 15 , 16 , 18 , 19 , 21 , 22 ]. Combining both neuromuscular and biomechanical metrics will provide a comprehensive assessment of the person’s movement starting from motor planning to the end of motor execution. Neuromuscular output provides valuable information on the feedforward control and the movement planning phase [ 22 ]. However, neuromuscular signals provides little information on the movement quality that is often investigated with movement function tests or biomechanical output [ 21 ]. Also, using neuromuscular data will provide information to therapist on the neurological status and nervous system reorganization of the person that biomechanical information cannot provide [ 73 ]. The additional information can assist in developing more personalized care for the person with stroke, as well as offer considerable information on the changes that occur at the physiological level.
Paper overview
This paper reviews published sensor-based methods, for biomechanical and neuromuscular assessment of impairment and function after neurological damage, and how the metrics resulting from the assessments, both alone and in combination, may be able to provide further information on the recovery process. Specifically, methods and metrics utilizing digitized kinematic, kinetic, EEG, and EMG data were considered. The “Methods” section explains how the literature review was performed. In “Measures and methods based on biomechanical performance” section, prevailing robotic assessment metrics are identified and categorized including smoothness, resistance, efficiency, accuracy, efficacy, planning, range-of-motion, strength, inter-joint coordination, and intra-joint coordination. In “Measures and methods based on neural activity using EEG/EMG” section, EEG- and EMG-derived measures are discussed by the primary category of analysis performed to obtain them, including frequency power and coherence analyses. The relationship of each method and metric to stroke impairment and/or function is also discussed. Section “Reliability of measures” discusses the reliability of sensor-based metrics and some of the complications in demonstrating the effectiveness of the metrics. Section “Integrated metrics” reviews previous studies on combining biomechanical and neuromuscular data to provide further information on the changes occurring during assessment and training. Finally, Section “Discussions and conclusions” concludes the paper with a discussion on the advantages of combining multi-domain data, which of the metrics from the earlier sections should be considered in future robotic applications, as well as the ones that still require more investigation for either validity and/or reliability.
A literature review was performed following PRISMA guidelines [ 74 ] on biomechanical and neuromuscular assessment in upper-limb stroke rehabilitation. The review was composed of two independent searches on (1) biomechanical robotic devices, and (2) electrophysiological digital signal processing. Figures 2 and 3 show the selection process of the electrophysiological and biomechanical papers, respectively. Each of these searches applied the following steps: In step 1, each researcher searched in Google Scholar for papers between 2000 and 2021 (see Table 1 for search terms and prompts). In step 2, resulting titles and abstracts were screened to remove duplicates, articles in other languages, and articles not related to the literature review. In step 3, researchers read the full texts of articles screened in step 2, papers qualifying for inclusion using the Literature Review Criteria in Table 1 were selected. Finally, in step 4, selected articles from independent review process were read by the other researcher. Uncertainties in determining if a paper should be included/excluded were discussed with the whole research group. Twenty-four papers focus on biomechanical measures (kinematic and kinetic), thirty-three focus on electrophysiological measures (EEG/EMG), and six papers on multimodal approaches combining biomechanical and neuromuscular measures to assess stroke. Three of the six multimodal papers are also reported in the biomechanical section and 3 papers were hand-picked. A total of 60 papers are reviewed and reported.
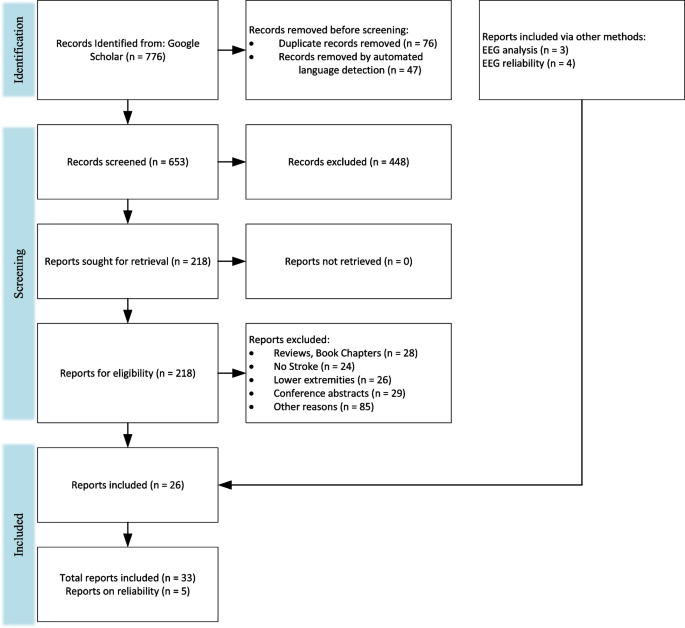
PRISMA flowchart on the selection for electrophysiological papers
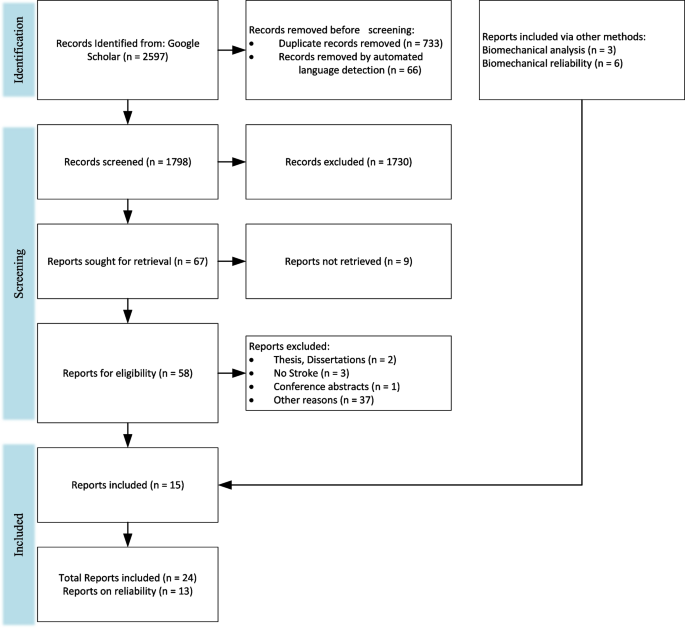
PRISMA flow chart for the selection for biomechanical papers
Measures and methods based on biomechanical performance
This review presents common robotic metrics which have been previously used to assess impairment and function after stroke. Twenty-five biomechanical papers are reviewed, which used both sensor-based and traditional clinical metrics to assess upper-extremity impairment and function. The five common metrics included in the reviewed studies measured the number of velocity peaks (~ 9 studies), path-length ratio (~ 8 studies), the max speed of the arm (~ 7 studies), active range of motion (~ 7 studies), and movement time (~ 7 studies). The metrics are often compared to an established clinical assessment to determine validity of the metric. The sensor-based metrics can be categorized by the aspect in which they evaluate movement quality similar to De Los Reyes-Guzmán et al.: smoothness, efficiency, efficacy, accuracy, coordination, or range of motion [ 14 ]. Resistance, Movement Planning, Coordination, and Strength are included as additional categories since some of the reviewed sensor-based metrics best evaluate those movement aspects. Examples of common evaluation activities and specific metrics that have been computed to quantify movement quality are outlined in Table 2 .
Lack of arm movement smoothness is a key indicator of underlying impairment [ 79 ]. Traditional therapist-administered assessments do not computationally measure smoothness leaving therapists unable to determine the degree to which disruption to movement smoothness is compromising motor function and, therefore, ADL. Most metrics that have been developed to quantify smoothness are based on features of the velocity profile of an arm movement, such as speed [ 80 , 81 ], speed arc length [ 79 ], local minima of velocity [ 10 ], velocity peaks [ 75 , 76 , 81 ], tent [ 80 ], spectral [ 25 ], spectral arc length [ 25 , 81 ], modified spectral arc length [ 79 ], and mean arrest period ratio [ 76 ]. Table 3 summarizes the smoothness metrics and their corresponding equations with equation numbers for reference. The speed metric is expressed as a ratio between the mean speed and the peak speed (Eq. 1). The speed arc length is the temporal length of the velocity profile (Eq. 2). Local minima of velocity and the velocity peaks metrics are measured by counting the number of minimum (Eq. 3) or maximum (Eq. 4) peaks in the velocity profile, respectively. The tent metric is a graphical approach that divides the area under the velocity curve by the area of a single peak velocity curve (Eq. 5). The spectral metric is the summation of the maximal Fourier transformed velocity vector (Eq. 6). The spectral arc-length metric is calculated from the frequency spectrum of the velocity profile by performing a fast Fourier transform operation and then computing the length (Eq. 7). The modified spectral arc length adapts the cutoff frequency according to a given threshold velocity and an upper-bound cutoff frequency (Eq. 8). The modified spectral arc length is then independent of temporal movement scaling. The mean arrest period ratio is the time portion that movement speed exceeds a given percentage of peak speed (Eq. 9).
Another commonly used approach is to analyze the jerk (i.e., the derivative of acceleration) profile. The common ways to assess smoothness using the jerk profile are root mean square jerk, mean rectified jerk, normalized jerk, and the logarithm of dimensionless jerk. The root mean square jerk takes the root-mean-square of the jerk that is then normalized by the movement duration [ 82 ] (Eq. 10). The mean rectified jerk (normalized mean absolute jerk) is the mean of the magnitude jerk normalized or divided by the peak velocity [ 80 , 82 ] (Eq. 11). The normalized jerk (dimensionless-squared jerk) is the square of the jerk times the duration of the movement to the fifth power over the length squared (Eq. 12). It is then integrated over the duration and square rooted. The normalized jerk can be normalized by mean speed, max speed, or mean jerk [ 80 ]. The logarithm of dimensionless jerk (Eq. 13) is the logarithm of normalized jerk defined in Eq. 12 [ 81 ].
It has yet to be determined which smoothness metric is more effective for characterizing recovery of smooth movement. According to Rohrer et al. [ 80 ], the metrics of speed, local minima of velocity, peaks, tent, and mean arrest period ratio showed increases in smoothness for inpatient recovery from stroke, but the mean rectified jerk metric seemed to show a decrease in smoothness as survivors of stroke recovered. Rohrer et al. warned that a low smoothness factor in jerk does not always mean the person is highly impaired. The spectral arc-length metric showed a consistent increase in smoothness as the number of sub-movements decreased [ 25 ], whereas the other metrics showed sudden changes in smoothness. For example, the mean arrest period ratio and the speed metric showed an increase in smoothness with two or more sub-movements, but when two sub-movements started to merge, the smoothness decreased. As a result, the spectral arc-length metric appears to capture change over a wider range of movement conditions in recovery in comparison to other metrics.
The presence of a velocity-dependent hyperactive stretch reflex is referred to as spasticity [ 83 ]. Spasticity results in a lack of smoothness during both passive and active movements and is more pronounced with activities that involve simultaneous shoulder abduction loading and extension of the elbow, wrist, or fingers [ 83 ], which are unfortunately quite common in ADL. A standard approach to assessing spasticity by a therapist involves moving a subject’s passive arm at different velocities and checking for the level of resistance. While this manual approach is subjective, electronic sensors have the potential to assess severity of spasticity in much more objective ways. Centen et al. report a method to assess the spasticity of the elbow using an upper-limb exoskeleton [ 84 ] involving the measurement of peak velocity, final angle, and creep. Sin et al., similarly performed a comparison study between a therapist moving the arm versus a robot moving the arm. An EMG sensor was used to detect the catch and compared with a torque sensor to detect catch angle for the robotic motion [ 85 ]. The robot moving the arm seemed to perform better with the inclusion of either an EMG or a torque sensor than with the therapist moving the arm and the robot simply recording the movement. A related measure that may be correlated with spasticity is the assessment of joint resistance torques during passive movement [ 76 ]. This can provide an assessment of the velocity-dependent resistance to movement that arises following stroke.
Efficiency measures movement fluency in terms of both task completion times and spatial trajectories. In point-to-point reaching, people who have suffered a stroke commonly display inefficient paths in comparison to their healthy side or compared to subjects who are unimpaired [ 10 ]. During the early phases of recovery after stroke, subjects may show slow overall movement speed resulting in longer task times. As recovery progresses, overall speed tends to increase and task times decrease, indicating more effective and efficient motor planning and path execution. Therapists usually observe the person’s efficiency in completing a task and then rate the person’s ability in completing a task in a timely manner. Therefore, both task time (or movement time) [ 10 , 76 , 77 , 86 , 87 ] and mean speed [ 25 , 75 , 77 , 81 , 86 ] are effective ways to assess temporal efficiency. Similar measures used by Wagner et al. include peak-hand velocity and time to peak-hand velocity [ 87 ]. To measure spatial efficiency of movement, both Colombo et al. [ 75 ], Mostafavi [ 77 ], and Germanotta [ 86 ] calculated the movement path length and divided it by the straight-line distance between the start and end points. This is known as the path-length ratio.
Movement planning
Movement planning is associated with feedforward sensorimotor control, elements that occur before the initial phase of movement. A common approach is to use reaction time to assess the duration of the planning phase. In a typical clinical assessment, a therapist can only observe/quantify whether movement can be initiated or not, but has no way to quantify the lag between the signal to initiate movement and initiation of movement. Keller et al., Frisoli et al., and Mostafavi et al. quantified the reaction time to assess movement planning [ 10 , 76 , 77 ] in subjects who have suffered a stroke. Mostafavi assessed movement planning in three additional ways by assessing characteristics of the actual movement: change in direction, movement distance ratio, and maximum speed ratio [ 77 ]. The change in direction is the angular deviation between the initial movement vector and the straight line between the start and end points. The first-movement-distance ratio is the ratio between the distance the hand traveled during the initial movement and the total distance between start and end points. The first-movement-maximum speed ratio is the ratio of the maximum hand speed during the initial phase of the movement divided by the global hand speed for the entire movement task.
Movement efficacy
Movement efficacy measures the person’s ability to achieve the desired task without assistance. While therapists can assess the number of completed repetitions, they have no means to kinetically quantify amount of assistance required to perform a given task. Movement efficacy is quantified by robot sensor systems that can measure: (a) person-generated movement, and/or (b) the amount of work performed by the robot to complete the movement (e.g., when voluntary person-generated movement fails to achieve a target). Hence, movement efficacy can involve both kinematic and kinetic measures. A kinematic metric that can be used to represent movement efficacy is the active movement index, which is calculated by dividing the portion of the distance the person is to complete by the total target distance for the task [ 75 ]. An example metric based on kinetic data is the amount of assistance metric, proposed by Balasubramanian et al. [ 25 ]. It is calculated by estimating the work performed by the robot to assist voluntary movement, and then dividing it by the work performed by the robot as if the person performs the task without assistance from the robot. A similar metric obtained by Germanotta et al. calculates the total work by using the movement’s path length, but Germanotta et al. also calculate the work generated towards the target [ 86 ].
Movement accuracy
Movement accuracy has been characterized by the error in the end-effector trajectory compared to a theoretical trajectory. It measures the person’s ability to follow a prescribed path, whereas movement efficiency assesses the person’s ability to find the most ideal path to reach a target. Colombo et al. measured movement accuracy in people after stroke by calculating the mean-absolute value of the distance, which is the mean absolute value of the distance between each point on the person’s path and the theoretical path [ 75 ]. Figure 4 demonstrates the difference between path-length ratio and mean-absolute value of the distance. The mean-absolute value of the distance computes the error between a desired trajectory and the actual, and the path-length ratio computes the total path length the person’s limb has traveled. Another similar metric is the average inter-quartile range, which quantifies the average “spread” among several trajectories [ 15 ]. Balasubramanian et al. characterized movement accuracy as a measure of the subject’s ability to achieve a target during active reaching. They refer to the metric as movement synergy [ 25 ], and calculate it by finding the distance between the end-effector’s final location and the target location.
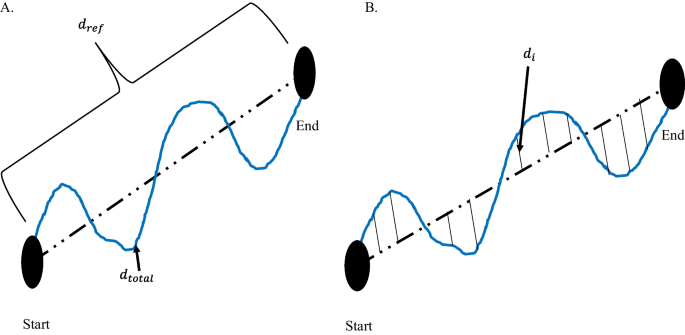
Difference between path-length ratio and mean absolute value of the distance. A Path-length ratio. \(d_{ref}\) is the theoretical distance the hand should travel between the start and end point. \(d_{total}\) is the total distance the hand travelled from Start to End. B Mean absolute value of the distance. \(d_{i}\) is the distance between the theoretical path and the actual hand path
Intra-limb coordination
Intra-limb (inter-joint) coordination is a measure of the level of coordination achieved by individual joints of a limb or between multiple joints of the same limb (i.e., joint synergy) when performing a task. Since the upper limb consists of kinematic redundancies, the human arm can achieve a desired outcome in multiple ways. For example, a person might choose to move an atypical joint in order to compensate for a loss of mobility in another joint. Frisoli et al. and Bosecker et al. used the shoulder and elbow angle to find a linear correlation between the two angles in a movement task that required multi-joint movement [ 10 , 78 ]. In terms of clinical assessment, joint angle correlations can illustrate typical or atypical contribution of a joint while performing a multi-joint task.
Inter-limb coordination
Inter-limb coordination refers to a person’s ability to appropriately perform bilateral movements with affected and unaffected arms. Therapists observe the affected limb by often comparing to the unaffected limb during a matching task, such as position matching. Matching can either be accomplished with both limbs moving simultaneously or sequentially, and typically without the use of vision. Dukelow et al. used position matching to obtain measures of inter-limb coordination [ 24 ], including trial-to-trial variability, spatial contraction/expansion, and systematic shifts. Trial-to-trial variability is the standard deviation of the matching hand’s position for each location in the x (distal/proximal), y (anterior/posterior), and both in x and y in the transverse plane. Spatial contraction/expansion is the ratio of the 2D work area of the target hand to the 2D work area of the matching hand during a matching task. Systematic shifts were found by calculating the mean absolute position error between the target and matching hand for each target location.
Semrau et al. analyzed the performance of subjects in their ability to match their unaffected arm with the location of their affected arm [ 88 ]. In the experiment, a robot moved the affected arm to a position and the person then mirrored the position with the unaffected side. The researchers compared the data when the person was able to see the driven limb versus when they were unable to see the driven limb. The initial direction error, path length ratio, response latency, peak speed ratio, and their variabilities were calculated to assess the performance of the person’s ability to perform the task.
Range of motion
Range of motion is a measure of the extent of mobility in one or multiple joints. Traditionally, range of motion can be measured with the use of a goniometer [ 89 ]. The goniometer measures the individual joint range of motion, which takes considerable time. Range of motion can be expressed as a 1-DOF angular measure [ 76 , 89 ], a 2-DOF planar measure (i.e., work area) [ 82 ], or a 3-DOF spatial measure (i.e., workspace) [ 77 ]. Individual joints are commonly measured in joint space, whereas measures of area or volume are typically given in Cartesian space. In performing an assessment of work area or workspace with a robotic device, the measure can be estimated either by: (a) measuring individual joint angles with an exoskeleton device and then using these angles to compute the region swept out by the hand, or (b) directly measuring the hand or fingertips with a Cartesian (end-effector) device. The measurement of individual joint range of motion (ROM) as well as overall workspace have significant clinical importance in assessing both passive (pROM) and active (aROM) range of motion. To measure pROM, the robot drives arm movement while the person remains passive. The pROM is the maximum range of motion the person has with minimal or no pain. For aROM, a robot may place the arm in an initial position/orientation from which the person performs unassisted joint movements to determine the ROM of particular joints [ 76 ], or the area or volume swept by multiple joints. Lin et al. quantified the work area of the elbow and shoulder using potentiometers and derived test–retest reliability [ 89 ]. The potentiometer measurements were then compared to therapist measurements to determine validity.
Measures of strength evaluate a person’s ability to generate a force in a direction or a torque about a joint. Strength measurements may involve single or multiple joints. At the individual joint level, strength is typically measured from a predefined position of a person’s arm and/or hand. The person then applies a contraction to produce a torque at the assessed joint [ 76 , 78 ]. Multi-joint strength may also be measured by assessing strength and/or torque in various directions at distal locations along the arm, such as the hand. Lin et al. compared the grip strength obtained from load cells to a clinical method using precise weights, which showed excellent concurrent validity [ 89 ].
Measures and methods based on neural activity using EEG/EMG
Although much information can be captured and analyzed using the kinematic and kinetic measures listed above, their purview is limited. These measures provide insight into the functional outcomes of neurological system performance but provide limited perspective on potential contributing sources of measured impairment [ 90 ]. For a deeper look into the neuromuscular system, measures based on neurological activation are often pursued. As a complement to biomechanical measures, methods based on quantization of neural activity like EEG and EMG have been used to characterize the impact of stroke and its underlying mechanisms of impairments [ 91 , 92 ]. Over the past 20 years, numerous academic research studies have used these measures to explore the effects of stroke, therapeutic interventions, or time on the evolution of abnormal neural activity [ 91 ]. Groups with different levels of neurological health are commonly compared (e.g., chronic/acute/subacute stroke vs. non-impaired, or impairment level) or other specific experimental characteristics (e.g., different rehabilitation paradigms [ 93 , 94 ]). With this evidence, the validity of these metrics has been tested; however, the study of reliability of these metrics is needed to complete the jump from academic to clinical settings.
Extracting biomarkers from non-invasive neural activity requires careful decomposition and processing of raw EEG and EMG recordings [ 32 ]. Various methods have been used, and the results have produced a growing body of evidence for the validity of these biomarkers in providing insight on the current and future state of motor, cognitive, and language skills in people after stroke [ 38 , 95 ]. Some of the biomarkers derived from EEG signals include: power-related band-specific information [ 34 , 35 , 43 , 47 , 53 , 54 , 96 , 97 , 98 , 99 , 100 , 101 ], band frequency event-related synchronization and desynchronization (ERS/ERD) [ 22 , 51 , 102 , 103 ], intra-cortical coherence or functional connectivity [ 39 , 59 , 73 , 94 , 104 , 105 , 106 , 107 , 108 , 109 ], corticomuscular coherence (CMC) [ 37 , 110 , 111 , 112 , 113 ], among others [ 114 , 115 ]. Biomarkers extracted from EEG can be used to assess residual functional ability [ 38 , 54 , 73 , 97 , 98 , 99 ], derive prognostic indicators [ 34 , 43 , 104 ], or categorize people into groups (e.g., to better match impairments with therapeutic strategies) [ 39 , 47 , 58 , 116 ].
In the following subsections, valid biomarkers derived mostly from EEG signal features (relationship with motor outcome for a person after stroke) will be discussed and introduced theoretically. Distinctions will be made about the stage after stroke when signals were taken. Findings are reported from 33 studies that have examined the relationship between extracted neural features and motor function for different groups of people after stroke. These records are grouped by quantization methods used including approaches based on measures of frequency spectrum power (n = 9), inter-regional coherence (n = 10 for cortical coherence and n = 9 for CMC), and reliability (n = 5).
Frequency spectrum power
Power measures the amount of activity within a signal that occurs at a specific frequency or range of frequencies. Power can be computed in absolute or relative terms (i.e., with respect to other signals). It is often displayed as a power density spectrum where the magnitudes of signal power can be seen across a range of frequencies. In electro-cognitive research, the representation of power within specific frequency bands has been useful to explain brain activity and to characterize abnormal oscillatory activity due to regional neurological damage [ 32 , 117 ].
Frequency bands in EEG content
Electrical activity in the brain is dominated primarily by frequencies from 0–100 Hz where different frequency bands correspond with different states of activity: Delta (0–4 Hz) is associated with deep sleep, Theta (4–8 Hz) with drowsiness, Alpha (8–13 Hz) with relaxed alertness and important motor activity [ 117 ], and Beta (13–31 Hz) with focused alertness. Gamma waves (> 32 Hz) are also seen in EEG activity; however, their specific relationship to level of alertness or consciousness is still debated [ 32 , 117 ]. Important cognitive tasks have been found to trigger activity in these bands in different ways. Levels of both Alpha and Delta activity have also been shown to be affected by stroke and can therefore be examined as indicators of prognosis or impairment in sub-acute and chronic stroke [ 52 , 100 , 118 ].
Power in acute and sub-acute stroke
For individuals in the early post-stroke (i.e., sub-acute) phase, abnormal power levels can be an indicator of neurological damage [ 98 ]. Attenuation of activity in Alpha and Beta bands have been observed in the first hours after stroke [ 100 ] preceding the appearance of abnormally high Delta activity. Tolonen et al. reported a high correlation between Delta power and regional Cerebral Blood Flow (rCBF). This relationship appears during the sub-acute stroke phase and has been used to predict clinical, cognitive, and functional outcomes [ 119 ]. Delta activity has also been shown to positively correlate with 1-month National Institutes of Health Stroke Scale (NIHSS) [ 52 ] and 3-month Rankin scale [ 36 ] assessments.
Based on these findings, several QEEG (Quantitative Electroencephalography) metrics involving ratios of abnormal slow (Delta) and abnormal fast (Alpha and Beta) activity have been developed. The Delta-Alpha Ratio (DAR), Delta-Theta Ratio (DTR), and (Delta + Theta)/(Alpha + Beta) Ratio (DTABR also known as PRI for Power Ratio Index) relate amount of abnormal slow activity with the activity from faster bands and have been shown to provide valuable insight into prognosis of stroke outcome and thrombolytic therapy monitoring [ 98 ]. Increased DAR and DTABR have been repeatedly found to be the QEEG indices that best predict worse outcome for the following: comparing with the Functional Independence Measure and Functional Assessment Measure (FIM-FAM) at 105 days [ 53 ], Montreal Cognitive Assessment (MoCa) at 90 days [ 54 ], NIHSS at 1 month [ 35 ], modified ranking scale (mRS) at 6 months [ 105 ], NIHSS evolution at multiple times [ 120 ], and NIHSS at 12 months [ 96 ]. DAR was also used to classify people in the acute phase and healthy subjects with an accuracy of 100% [ 58 ].
The ability of basic EEG monitoring to derive useful metrics during the early stage of stroke has made EEG collection desirable for people who have suffered a stroke in intensive care settings. The derived QEEG indices have proven to be helpful to determine Delayed Cerebral Ischemia (DCI), increased DAR [ 43 ], and increased Delta power [ 34 , 118 ]. However, finding the electrode montage with the least number of electrodes that still reveals the necessary information for prognoses is one of the biggest challenges for this particular use of EEG. Comparing DAR from 19 electrodes on the scalp with 4 electrodes on the frontal cortex suggests that DAR from 4 frontal electrodes may be enough to detect early cognitive and functional deficits [ 53 ]. Studies explored the possibility of a single-electrode montage over the Fronto-Parietal area (FP1); the DAR and DTR from this electrode might be a valid predictor of cognitive function after stroke when correlated with the MoCA [ 54 ], relative power in Theta band correlated with mRS and modified Barthel Index (mBI) 30 and 90 days after stroke [ 121 ].
Power in chronic stroke
The role of power-related QEEG indices during chronic stroke and progression of motor functional performance have been examined with respect to rehabilitation therapies, since participants have recovered their motion to a certain degree [ 4 ]. Studies have shown that therapy and functional activity improvements correlate with changes of the shape and delay of event-related desynchronization and synchronization (ERD-ERS) for time–frequency power features when analyzing Alpha and Beta bands on the primary motor cortex for ipsilesional and contralesional hemispheres [ 21 , 22 , 122 ]. Therapies with better outcome tend to have reduced Delta rhythms and increased Alpha rhythms [ 122 ].
Bertolucci [ 47 ] compared starting power spectrum density in different bands for both hemispheres with changes in WMFT and FMA over time. Increased global Alpha and Beta activity was shown to correlate with better WMFT evolution while, increase in contralesional Beta activity was shown to be correlated with FMA evolution. Metrics combining slow and fast activity have also been tested in the chronic stage of stroke, significant negative correlation between DTABR (PRI) at the start of therapy was related to FMA change during robotic therapy [ 99 ]. This finding suggests that DTABR may have promise as prognostic indicators for all stages of stroke.
Brain Symmetry Index (BSI) is a generalized measure of “left to right” (affected to non-affected) power symmetry of mean spectral power per hemisphere. These inter-hemispheric relationships of power have been used as prognostic measures during all stages of stroke. Baseline BSI (during the sub-acute stage) was found to correlate with the FMA at 2 months [ 73 ], mRS at 6 months [ 123 ], and FM-UE predictor when using only theta band BSI for patients in the chronic stage [ 124 ]. BSI can be modified to account for the direction of asymmetry, the directed BSI at Delta and Theta bands proved meaningful to describe evolution from acute to chronic stages of upper limb impairment as measured by FM-UE [ 120 , 125 ]. Table 4 and Table 11 in Appendix 1 communicate power-derived metrics across different stages of stroke documented in this section and their main reported relationships with motor function. Findings are often reported in terms of correlation with clinical tests of motor function.
Brain connectivity (cortical coherence)
Brain connectivity is a measure of interaction and synchronization between distributed networks of the brain and allows for a clearer understanding of brain function. Although cortical damage from ischemic stroke is focal, cortical coherence can explain abnormalities in functionality of remote zones that share functional connections to the stroke-affected zone [ 59 ].
Several estimators of connectivity have been proposed in the literature. Coherency, partial coherence (pCoh) [ 125 ], multiple coherence (mCoh), imaginary part of coherence (iCoh) [ 126 ], Phase Lagged Index (PLI), weighted Phase Lagged Index (wPLI) [ 127 ], and simple ratios of power at certain frequency bands [ 73 ] describe synchronic symmetric activity between ROIs and are referred to as non-directed or functional connectivity [ 128 ]. Estimators based on Granger’s prediction such as partial directed coherence (PDC) [ 129 , 130 , 131 ], or directed transfer Function (DTF) [ 132 , 133 ] and any of their normalizations describe causal relationships between variables and are referred to as directed or effective connectivity [ 134 ]. Connectivity also allows the analysis of brain activity as network topologies, borrowing methods from graph theory [ 32 , 134 ]. Network features such as complexity, linearity, efficiency, clustering, path length, node hubs, and more can be derived from graphs [ 128 ]. Comparisons of these network features among groups with impairment and healthy controls have proven to be interesting tools to understand and characterize motor and functional deficits after stroke [ 108 ].
Studies have used intra- and inter-cortical coherence to expand the clinical understanding of the neural reorganization process [ 59 , 106 , 107 , 108 , 109 ], as a clinical motor and cognitive predictor [ 38 , 94 , 104 , 135 , 136 ], and as a tool to predict the efficacy of rehabilitation therapy [ 94 ]. Table 5 and Table 12 in Appendix 2 briefly summarize the main metrics discussed in this section and their results that are related with motor function assessment. In general, studies have shown that motor deficits in stroke survivors are related to less connectivity to main sensory motor areas [ 38 , 94 , 104 , 137 ], weak interhemispheric sensorimotor connectivity [ 109 , 138 ], less efficient networks [ 106 , 135 ], with less “small world” network patterns [ 108 , 134 ] (small-world networks are optimized to integrate specialized processes in the whole network and are known as an important feature of healthy brain networks).
Survivors of stroke tend to exhibit more modular (i.e., more clustered, less integrated) and less efficient networks than non-impaired controls with the biggest difference occurring in the Beta and Gamma bands [ 106 ]. Modular networks are less “small-world” [ 134 ]; small-world networks are optimized to integrate specialized processes in the whole network and are known as an important feature of healthy brain networks. Such a transition to a less small-world network was observed during the acute stage of stroke (first hours after stroke) and documented to be bilaterally decreased in the Delta band and bilaterally increased in the high Alpha band (also known as Alpha2: 10.5–13 Hz) [ 108 ].
Global connectivity with the ipsilesional primary motor cortex (M1) is the most researched biomarker derived from connectivity and has been studied in longitudinal experiments as a plasticity indicator leading to future outcome improvement [ 38 ], motor and therapy gains [ 94 ], upper limb gains during the sub-acute stage [ 137 ], and as a feature that characterizes stroke survivors’ cognitive deficits [ 104 ]. Pietro [ 38 ] used iCoh to test the weighted node degree (WND), a measure that quantifies the importance of a ROI in the brain, for M1 and reported that Beta-band features are linearly related with motor improvement as measured by FM-UE and Nine-Hole-Peg Test. Beta-band connectivity to ipsilesional M1, as measured by spectral coherence, can be used as a therapy outcome predictor, and more than that, results point heavily toward connectivity between M1 and ipsilesional frontal premotor area (PM) to be the most important variable as a therapy gain predictor; predictions can be further improved by using lesion-related information such as CST or MRI to yield more accurate results [ 94 ]. Comparisons between groups of people with impairment and controls showed significant differences on Alpha connectivity involving ipsilesional M1, this value showed a relation with FMA 3 months for the group with impairment due to stroke [ 104 ].
The relationship between interhemispheric ROI connectivity and motor impairment has been studied. The normalized interhemispheric strength (nIHS) from PDC was used to quantify the coupling between structures in the brain, Beta- and lower Gamma-band features of this quantity in sensorimotor areas exhibited linear relationships with the degree of motor impairment measured by CST [ 136 ]. A similar measure, also derived from PDC used to measure ROI interhemispheric importance named EEG-PDC was used in [ 109 ]; here the results show that Mu-band (10–12 Hz) and Beta-band features could be used to explain results for hand motor function from FM-UE. In another study, Beta debiased weighted phase lag index (dwPLI), correlated with outcome measured by Action Research Arm Test (ARAT) and FM-UE [ 138 ].
Global and local network efficiency for Beta and Gamma bands seem to be significantly decreased in the population who suffered from a stroke compared to healthy controls as reported in [ 106 ]. Newer results, such as the ones pointed out by [ 135 ] found statistically significant relationships between Beta network efficiency, network intradensity derived using a non-parametric method (named Generalized Measure of Association), and functional recovery results given by FM-UE. Global maximal coherence features in the Alpha band have been recently recognized as FM-UE predictors, where coherence was computed using PLI and related to motor outcome by means of linear regression [ 139 ].
Corticomuscular coherence
Corticomuscular coherence (CMC) is a measure of the amount of synchronous activity between signals in the brain (i.e., EEG or MEG) and associated musculature (i.e., EMG) of the body [ 92 ]. Typically measured during voluntary contractions [ 110 ], the presence of coherence demonstrates a direct relationship between cortical rhythms in the efferent motor commands and the discharge of neurons in the motor cortex [ 140 ]. CMC is computed as correlation between EEG and EMG signals at a given frequency. Early CMC research found synchronous (correlated) activity in Beta and low Gamma bands [ 40 , 41 , 42 ]. CMC is strongest in the contralateral motor cortex [ 141 ]. This metric seems to be affected by stroke-related lesions, and thus provides an interesting tool to assess motor recovery [ 111 , 142 , 143 , 144 ]. The level of CMC is lower in the chronic stage of stroke than in healthy subjects [ 112 , 145 ], with chronic stroke survivors showing lower peak CMC frequency [ 146 ], and topographical patterns that are more widespread than in healthy people; highlighting a connection to muscle synergies [ 142 , 147 , 148 ]. CMC has been shown to increase with training [ 37 , 112 , 144 ].
Corticomuscular coherence has been proposed as a tool to: (a) identify the functional contribution of reorganized cortical areas to motor recovery [ 37 , 112 , 141 , 144 , 146 ]; (b) understand functional remapping [ 93 , 142 , 145 ]; and (c) study the mechanisms underlying synergies [ 147 , 148 ]. CMC has shown increased abnormal correlation with deltoid EMG during elbow flexion for people who have motor impairment [ 147 ], and the best muscles to target with rehabilitative interventions [ 148 ]. Changes in CMC have been shown to correlate with motor improvement for different stages of stroke, although follow-up scores based on CMC have not shown statistically significant correlations when compared to clinical metrics [ 37 , 93 ]. Results summarizing CMC on stroke can be found in Table 6 and Table 13 in Appendix 3.
Reliability of measures
Each of the aforementioned measures have the potential to be integrated into robotic devices for upper-limb assessment. However, to improve the clinical acceptability of robotic-assisted assessment, the measurements and derived metrics must meet reliability standards in a clinical setting [ 55 ]. Reliability can be defined as the degree of consistency between measurements or the degree to which a measurement is free of error. A common method to represent the relative reliability of a measurement process is the intraclass correlation coefficient (ICC) [ 150 ]. Koo and Li suggest a guideline on reporting ICC values for reliability that includes the ICC value, analysis model (one-way random effects, two-way random effects, two-way fixed effects, or two-way mixed effects), the model type per Shrout and Fleiss (individual trials or mean of k trials), model definition (absolute agreement or consistency), and confidence interval [ 68 ]. Koo and Li also provide a flowchart in selecting the appropriate ICC based on the type of reliability and rater information. An ICC value below 0.5 indicates poor reliability, 0.5 to 0.75 moderate reliability, 0.75 to 0.9 good reliability, and above 0.9 excellent reliability. The reviewed papers will be evaluated based on these guidelines. For reporting the ICC, the Shrout and Fleiss convention is used [ 68 ]. The chosen reliability studies are included in the tables if the chosen ICC model, type, definition, and confidence interval are identifiable, and the metrics have previously been used in electronic-based metrics. For studies that report multiple ICC scores due to assessment of test–retest reliability for multiple raters, the lowest ICC reported is included to avoid bias in the reported results.
In the assessment of reliability of data from robotic sensors, common ways to assess reliability are to correlate multiple measurements in a single session (intra-session) and correlate multiple measurements between different sessions (inter-session) measurements (i.e., test–retest reliability) [ 151 ]. Checking for test–retest reliability determines the repeatability of the robotic metric. The repeatability is the ability to reproduce the same measurements under the same conditions. Table 7 shows the test–retest reliability of several robotic metrics. For metrics checking for test–retest reliability, a two-way mixed-effects model with either single or multiple measurements may be used [ 68 ]. Since the same set of sensors will be used to assess subjects, the two-way mixed model is used. The test–retest reliability should be checking for absolute agreement. Checking for absolute agreement (y = x) rather than consistency (y = x + b) determines the reliability without a bias or systematic error. For example, in Fig. 5 , for a two-way random effect with a single measurement checking for agreement gives a score of 0.18. When checking for consistency, the ICC score reaches to 1.00. In other words, the bias has no effect on the ICC score when checking for consistency. Therefore, when performing test–retest reliability, it is important to check for absolute agreement to prevent bias in the test–retest result.
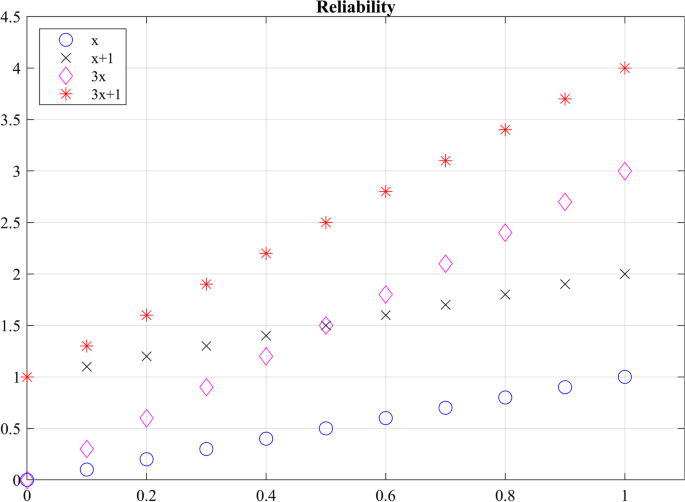
Checking agreement versus consistency among ratings. For y = x, the absolute ICC score is 1 and the consistency ICC score is 1.00. For y = x + 1, the agreement ICC score is 0.18 and the consistency ICC score is 1.00. For y = 3x, the absolute ICC score is 0.32 and the consistency ICC score is 0.60. For y = 3x + 1, the absolute ICC score is 0.13 and the consistency ICC score is 0.60
Not only should a robotic metric demonstrate repeatability, it should also be reproducible when different operators are using the same device. Reproducibility evaluates the change in measurements when conditions have changed. Inter-rater reliability tests have been performed to determine the effect raters have when collecting measurements when two or more raters perform the same experimental protocol [ 68 ]. To prevent a biased result, raters should have no knowledge of the evaluations given by other raters, ensuring that raters’ measurements are independent from one another. Table 8 shows the reproducibility of several robotic biomechanical metrics. All the included studies have used two raters to check for reproducibility. The researchers performed a two-way random effects analysis with either a single measurement or multiple measurements to check for agreement.
Measurement reliability of robotic biomechanical assessment
Of the 24 papers reviewed for biomechanical metrics, 13 papers reported on reliability. 6 papers reported reproducibility and 9 papers reported on repeatability. Overall, the metrics seem to demonstrate good to moderate reliability for both repeatability and reproducibility. However, caution should be exercised in determining which robotic metric is more effective in assessing movement quality based on reliability studies. The quality of measurements is highly dependent on the quality of the robotic device and sensors [ 85 ]. Having a completely transparent robot with a sensitive and accurate sensor will further improve assessment of reliability. Also, the researchers have used different versions of the ICC, as seen in Tables 7 and 8 , which complicates direct comparisons of the metrics.
Reliability of electrophysiological signal features
Of the 33 papers reviewed for electrophysiological metrics, 5 papers reported on reliability. 6 papers reported on repeatability. Convenience of acquiring electrophysiological signals non-invasively is relatively new. Metrics for assessment of upper limb motor impairment in stroke, derived from these signals have shown to be valid in academic settings, but most of these valid metrics have yet to be tested for intra- and inter-session reliability to be used in clinical and rehabilitation settings. Few studies found as a result of our systematic search have looked at test–retest reliability of these metrics. Therefore, we found and manually added records reporting on intra- and inter-session reliability on metrics based on electrophysiological features described in section “Measures and methods based on neural activity using EEG/EMG”, even if reliability was not assessed on people with stroke. Relevant results are illustrated in Table 9 .
Spectral power features of EEG signals have been tested during rest [ 153 , 154 ] and task (cognitive and motor) conditions for different cohorts of subjects [ 102 , 103 ]. Some of the spectral features observed during these experiments are related to timed behavior of oscillatory activity due to cued experiments, such as event-related desynchronization of the Beta band (ERD and Beta rebound) [ 102 ] and topographical patterns of Alpha activity R = 0.9302, p < 0.001 [ 103 ].
Test–retest reliability for rest EEG functional connectivity has been explored for few of the estimators listed in section “Measures and methods based on neural activity using EEG/EMG”: (1) for a cohort of people with Alzheimer by means of the amplitude envelope correlation (AEC), phase lag index (PLI) and weighted phase lag index (wPLI) [ 155 ]; (2) in healthy subjects using iCoh and PLI [ 156 ]; and (3) in infants, by studying differences of inter-session PLI graph metrics such as path length, cluster coefficient, and network “small-worldness” [ 60 ]. Reliability for upper limb CMC has not yet been documented (at least to our knowledge). However, an experiment involving testing reliability of CMC for gait reports low CMC reliability in groups with different ages [ 61 ].
EEG and EMG measurements could be combined with kinematic and kinetic measurements to provide additional information about the severity of impairment and decrease the number of false positives from individual measurements [ 21 ]. This could further be used to explain abnormal relationships between brain activation, muscle activation and movement kinematics, as well as provide insight about subject motor performance during therapy [ 15 ]. The availability of EEG and EMG measures can also enhance aspects of biofeedback given during tests or be used to complement other assessments to provide a more holistic picture of an individual’s neurological function.
It has been shown that combining EEG, EMG, and kinematic data using a multi-domain approach can produce correlations to traditional clinical assessments, a summary of some of the reviewed studies is presented in Table 10 . Belfatto et al. have assessed people’s ROM for shoulder and elbow flexion, task time, and computed jerk to measure people’s smoothness, while the EMG was used to measure muscle synergies, and EEG detected ERD and a lateralization coefficient [ 21 ]. Comani et al. used task time, path length, normalized jerk, and speed to measure motor performance while observing ERD and ERS during motor training [ 22 ]. Pierella et al. gathered kinematic data from an upper-limb exoskeleton, which assessed the mean tangential velocity, path-length ratio, the number of speed peaks, spectral arc length, the amount of assistance, task time, and percentage of workspace, while observing EEG and EMG activity [ 18 ]. Mazzoleni et al. used the InMotion2 robot system to capture the movement accuracy, movement efficiency, mean speed, and the number of velocity peaks, while measuring brain activity with EEG [ 16 ]. However, further research is necessary to determine the effectiveness of the chosen metrics and methods compared to other more promising methods to assess function. Furthermore, greater consensus in literature is needed to support the clinical use of more reliable metrics. For example, newer algorithms to estimate smoothness such as spectral arc length have been shown to provide greater validity and reliability than the commonly used normalized jerk metric. Despite this evidence, normalized jerk remains a widely accepted measure of movement smoothness.
Discussions and conclusions
In this paper we reviewed studies that used different sensor-acquired biomechanical and electrophysiological signals to derive metrics related to neuromuscular impairment for stroke survivors; such metrics are of interest for robotic therapy and assessment applications. To assess the ability of a given measure to relate with impairment or motor outcome, we looked for metrics where results have been demonstrated to correlate or predict scores from established clinical assessment metrics for impairment and function (validity). Knowing that a metric has some relationship with impairment and function (i.e., that it is valid) is not enough for it to be used in clinical settings if those results are not repeatable (reliable). Thus, we also reviewed the reliability of metrics and related signal features looking for metrics which produce similar results for the same subject during different test sessions and for different raters. With this information, researchers can aim to use metrics that not only seem to be related with stroke, but also can be trusted, with less bias, and with a simpler interpretation. The main conclusions of this review paper are presented as answers to the following research questions.
Which biomechanical-based metrics show promise for valid assessment of function and impairment?
Metrics derived from kinematic (e.g., position & velocity) and kinetic (e.g., force & torque) sensors affixed to robotic and passive mechanical devices have successfully been used to measure biomechanical aspects of upper-extremity function and impairment in people after stroke. The five common metrics included in the reviewed studies measured the number of velocity peaks (~ 9 studies), path-length ratio (~ 8 studies), the maximum speed of the arm (~ 7 studies), active range of motion (~ 7 studies), and movement time (~ 7 studies). The metrics are often compared to an established clinical assessment to determine validity of the metric. According to the review study by Murphy and Häger, the Fugl-Meyer Assessment for Upper Extremity had significant correlation with movement time, movement smoothness, peak velocity, elbow extension, and shoulder flexion [ 66 ]. The movement time and smoothness showed strong correlation with the Action Research Arm Test, whereas speed, path-length ratio, and end-point error showed moderate correlation. Tran et al. reviewed specifically validation of robotic metrics with clinical assessments [ 57 ]. The review found mean speed, number of peak velocities, movement accuracy, and movement duration to be most promising metrics based on validation with clinical assessments. However, the review mentioned that some studies seem to conflict on the correlation between the robotic metric and clinical measures, which could be due to assessment task, subject characteristics, type of intervention, and robotic device. For further information about the validation of sensor-based metrics, please refer to the previously mentioned literature reviews [ 57 , 66 ].
Which biomechanical-based metrics show promise for repeatable assessment?
Repeatable measures, in which measurement taken by a single instrument and/or person produce low variation within a single task, are a critical requirement for assessment of impairment and function. The biomechanical based metrics that show the most promise for repeatability are range of motion, mean speed, mean distance, normal path length, spectral arc length, number of peaks, and task time. Two or more studies used these metrics and demonstrated good and excellent reliability, which implies the metric is robust against measurement noise and/or disturbances. Since the metrics have been used on different measuring instruments, the sensors’ resolution and signal-to-noise ratio appear to have a minimal impact on the reliability. However, more investigation is needed to confirm this robustness. In lieu of more evidence, it is recommended that investigators choose sensors similar or superior in quality to those used in the measuring devices presented in Tables 7 and 8 to achieve the same level of reliability.
What aspects of biomechanical-based metrics lack evidence or require more investigation?
Although many metrics (see previous section) demonstrate good or excellent repeatability across multiple studies, the evidence for reproducibility is limited to single studies. When developing a novel device capable of robotic assistance and assessment, researchers have typically focused their efforts to create a device capable of repeatable and reliable measurements. However, since the person administering the test is using the device to measure the subject’s performance, the reproducibility of the metric must also be considered. The reproducibility of a metric is affected by the ease-of-use of the device; if the device is too complicated to setup and use, there is an increased probability that different operators will observe different measurements. Also, the operator’s instructions to the subject affects the reproducibility, especially in the initial sessions, which may lead to different learning effects, and different assessment results. More studies are needed across multiple sites and operators to determine the reproducibility of the biomechanical metrics reviewed in this paper.
Which neural activity-based metrics (EEG & EMG) show the most promise for reliable assessment?
Electrical neurological signals such as EEG and EMG have successfully been used to understand changes in motor performance and outcome variability across all stages of post-stroke recovery including the first few hours after onset. Experimental results have shown that metrics derived from slow frequency power (delta power, relative delta power, and theta power), and power ratio between slow and fast EEG frequency bands like DAR and DTABR convey useful information both about current and future motor capabilities, as presented in Table 4 and Table 11 in Appendix 1. Multimodal studies using robotic tools for assessment of motor performance have expanded the study of power signal features in people who suffered a stroke in the chronic recovery stage by studying not only rest EEG activity but also task-related activity [ 19 , 21 , 122 ]; ERD-ERS features like amplitude and latency along with biomechanical measures have been shown to correlate with clinical measures of motor performance and to predict a person’s response to movement therapies. EEG power features in general have been found to have good to excellent reliability for test–retest conditions among different populations, across all frequency bands of interest (see Table 9 ).
Functional connectivity (i.e., non-directed connectivity) expands the investigative capacity of EEG measurements, enabling analyzing the brain as a network system by investigating the interactions between regions of interest in the brain while resting or during movement tasks. Inter-hemispheric interactions (interactions between the same ROI in both hemispheres) and global interactions (interactions between the entire brain and an ROI) reported as power or graph indices in Beta and Gamma bands have fruitfully been used to explain motor outcome scores. Although results seem promising, connectivity reliability is still debated with results ranging mostly between moderate to good reliability only for a few connectivity estimators ( PLI, wPLI and iCoh ).
Which neural activity-based metrics (EEG and EMG) lack evidence or require more investigation?
EEG and EMG provide useful non-invasive insight into the human neuromuscular system allowing researchers to make conjectures about its function and structure; however, interpretation of results based on these measures solely must be carefully analyzed within the frame of experimental conditions. Overall, the field needs more studies involving cohorts of stroke survivors to determine the reliability (test–retest) of metrics derived from EEG and EMG signal features that have already shown validity in academic studies.
Metrics calculated from power imbalance between interhemispheric activity like BSI , pwBSI and PRI [ 62 , 73 , 124 ] are a great premise to measure how the brain relies on foreign regions to accomplish tasks related with affected areas. A battery of diverse estimators for connectivity, especially those of effective (directed) connectivity, open the door to investigations into the relationship between abnormal communication of regions of interest and impairment (see Table 5 and Table 12 in Appendix 2). These metrics, although valid have yet to be tested in terms of reliability in clinical use. Reliability for connectivity metrics should specify which estimator was used to derive the metric.
CMC is another exciting neural-activity-based metric lacking sufficient evidence to support its significance. CMC considers and bridges two of the most affected domains for motor execution in neuromuscular system, making it a good candidate for robotic-based therapy and assessment of survivors of stroke [ 147 ]. Although features in the Beta and Gamma bands seem to be related to motor impairment, there is still not agreement about which one is most closely related to motor outcomes. Studies reviewed in this paper considered cortical spatial patterns of maximum coherence, peak frequency shift when compared to healthy controls, latency for peak coherence, among others (see Table 6 and Table 13 in Appendix 3). However, when comparing to motor outcomes, results are not always significant, and test–retest reliability for this metric is yet (to our knowledge) to be documented for the upper extremity (see [ 61 ] for a lower-extremity study).
What standards should be adopted for reporting biomechanical and neural activity-based metrics and their reliability?
For metrics to be accepted as reliable in the clinical field, researchers are asked to follow the guidelines presented in Koo and Li [ 68 ], which provide guidance on which ICC model to use depending on the type of reliability study and what should be reported (e.g., the software they used to compute the ICC and confidence interval). In the papers reviewed, some investigated the learning effects of the assessment task and checked for consistency rather than agreement (see Table 7 ). However, the learning effects should be minimal in a clinical setting between each session, and potential effects should be taken into consideration during protocol design; common practices to minimize the implications of learning effects is to allow practice runs by the patients [ 99 , 122 ] and to remove the first experimental runs [ 81 , 85 ]. By removing this information, signal analysis focuses performance of learned tasks with similar associated behaviors. Therefore, to demonstrate test–retest reliability (i.e., repeatability), the researcher should be checking for absolute agreement. Also, as can be seen in Tables 7 and 8 , there does not seem to be a standard on reporting ICC values. Some researchers report the confidence interval of the ICC value, while others do not. It was also difficult to determine the ICC model used in some of the studies. Therefore, a standard on reporting ICC values is needed to help readers understand the ICC used and prevent bias (see [ 68 ] for suggestive guideline on how to report ICC scores). Also, authors are asked to include the means of each individual session or rater would provide additional information on the variation of the means between the groups. The variation between groups can be shown with Bland–Altman plot, but readers are unable to perform other forms of analysis. To help with this, data from studies should be made publicly available to allow results to be verified and enable further analysis in the future.
When is it advantageous to combine biomechanical and neural activity-based metrics for assessment?
Biomechanical and neural activity provide distinct but complementary information about the neuro-musculoskeletal system, potentially offering a more complete picture of impairment and function after stroke. Metrics derived from kinematic/kinetic information assess motor performance based on motor execution; however, compensatory strategies related to stroke may mask underlying neural deficits (i.e., muscle synergies line up to complete a given task) [ 18 , 21 , 69 , 70 , 71 , 72 , 122 ]. Information relevant to these compensatory strategies can be obtained when analyzing electrophysiological activity, as has been done using connectivity [ 59 , 107 ], CMC [ 147 , 148 ] and brain cortical power [ 91 ].
Combining signals from multiple domains, although beneficial in the sense that it would allow a deeper understanding of a subject’s motor ability, is still a subject of exploration. Experimental paradigms play an important role that influences the decision of feature selection; increasing the dimensionality of signals may provide more useful information for analysis, but comes at the expense of experimental costs (e.g., hardware) and time (e.g., subject setup). With all this in mind, merging information from different domains in the hierarchy of the neuro-musculoskeletal system may provide a more comprehensive quantitative profile of a person’s impairment and performance. Examples of robotic multidomain methods such as the ones in [ 18 , 21 ], highlight the importance of this type of assessment for monitoring and understanding the impact of rehabilitation in chronic stroke survivors. In both cases, these methodologies allowed pairing of observed behavioral changes in task execution (i.e., biomechanical data) with corresponding functional recovery, instead of adopted compensation strategies.
What should be the focus of future investigations of biomechanical and/or neural activity-based metrics?
Determining the reliability and validity of sensor-based metrics requires carefully designed experiments. In future investigations, experiments should be conducted that calculate multiple metrics from multiple sensors and device combinations, allowing the effect of sensor type and quality on the measure’s reliability to be quantified. After the conclusion of such experiments, researchers are strongly encouraged to make their anonymized raw data public to allow other researchers to compute different ICCs. Performing comparison studies on the reliability of metrics will produce reliability data to expand Tables 7 , 8 , 9 and improve our ability to compare similar sensor-based metrics. Additional reliability studies should also be performed that include neural features of survivors of stroke, with increased focus on modeling the interactions between these domains (biomechanical and neural activity). It is also important to understand how to successfully combine data from multimodal experiments; many of the studies reviewed in this paper recorded multidimensional data, but performed analysis for each domain separately.
Availability of data and materials
Not applicable.
Abbreviations
Activities of daily living
Amplitude envelope correlation
Action research arm test
Active range of motion
Autism spectrum disorder
Box and Blocks test
Brain Symmetry Index
Canonical correlation analysis
Cortico-spinal tract
Delta-alpha ratio
Delayed cerebral ischemia
Direct directed transfer function
Degree of freedom
(Delta + Theta)/(Alpha + Beta)
Directed transfer function
Delta-theta ratio
- Electroencephalography
Electromyography
Event related desynchronization
Event related synchronization
Full frequency directed transfer function
Functional independence measure and functional assessment measure
Fugl-Meyer assessment for upper extremity
Generalized Measure of Association
Generalized partial directed coherence
Intra-class correlations
Imaginary part of coherence
Primary motor cortex
Modified Ashworth
Modified Barthel Index
Multiple coherence
Motricity Index
Montreal Cognitive Assessment
Movement related beta desynchronization
Magnetic resonance imaging
Modified Ranking Scale
Normalized interhemispheric strength
National Institutes of Health Stroke Scale
Non-negative matrix factorization algorithm
Principal component analysis
Partial coherence
Partial directed coherence
Phase lag index, weight phase lag index, debiased weighted phase lag index
Premotor area
Post movement beta rebound
Power Ratio Index
Passive range of motion
Quantitative EEG
Regional cerebral blood flow
Region of interest
Renormalized partial directed coherence
Singular value decomposition
Wolf motor function
Weighted Node Degree Index
Stroke Facts. 2020. https://www.cdc.gov/stroke/facts.htm . Accessed 26 Mar 2020.
Ottenbacher KJ, Smith PM, Illig SB, Linn RT, Ostir GV, Granger CV. Trends in length of stay, living setting, functional outcome, and mortality following medical rehabilitation. JAMA. 2004;292(14):1687–95. https://doi.org/10.1001/jama.292.14.1687 .
Article CAS PubMed Google Scholar
Lang CE, MacDonald JR, Gnip C. Counting repetitions: an observational study of outpatient therapy for people with hemiparesis post-stroke. J Neurol Phys Ther. 2007;31(1). https://journals.lww.com/jnpt/Fulltext/2007/03000/Counting_Repetitions__An_Observational_Study_of.4.aspx .
Gresham GE, Phillips TF, Wolf PA, McNamara PM, Kannel WB, Dawber TR. Epidemiologic profile of long-term stroke disability: the Framingham study. Arch Phys Med Rehabil. 1979;60(11):487–91.
CAS PubMed Google Scholar
Duncan EA, Murray J. The barriers and facilitators to routine outcome measurement by allied health professionals in practice: a systematic review. BMC Health Serv Res. 2012;12(1):96.
Article PubMed PubMed Central Google Scholar
Sullivan KJ, Tilson JK, Cen SY, Rose DK, Hershberg J, Correa A, et al. Fugl-meyer assessment of sensorimotor function after stroke: standardized training procedure for clinical practice and clinical trials. Stroke. 2011;42(2):427–32.
Article PubMed Google Scholar
Ansari NN, Naghdi S, Arab TK, Jalaie S. The interrater and intrarater reliability of the Modified Ashworth Scale in the assessment of muscle spasticity: limb and muscle group effect. NeuroRehabilitation. 2008;23:231–7.
Wade DT, Collin C. The Barthel ADL Index: a standard measure of physical disability? Int Disabil Stud. 1988;10(2):64–7.
Maggioni S, Melendez-Calderon A, van Asseldonk E, Klamroth-Marganska V, Lünenburger L, Riener R, et al. Robot-aided assessment of lower extremity functions: a review. J Neuroeng Rehabil. 2016;13(1):72. https://doi.org/10.1186/s12984-016-0180-3 .
Frisoli A, Procopio C, Chisari C, Creatini I, Bonfiglio L, Bergamasco M, et al. Positive effects of robotic exoskeleton training of upper limb reaching movements after stroke. J Neuroeng Rehabil. 2012;9(1):36. https://doi.org/10.1186/1743-0003-9-36 .
Groothuis-Oudshoorn CGM, Prange GB, Hermens HJ, Ijzerman MJ, Jannink MJA. Systematic review of the effect of robot-aided therapy on recovery of the hemiparetic arm after stroke. J Rehabil Res Dev. 2006;43(2):171.
Harwin WS, Murgia A, Stokes EK. Assessing the effectiveness of robot facilitated neurorehabilitation for relearning motor skills following a stroke. Med Biol Eng Comput. 2011;49(10):1093–102.
Nordin N, Xie SQ, Wünsche B. Assessment of movement quality in robot- assisted upper limb rehabilitation after stroke: a review. J NeuroEngineering Rehabil. 2014;11:137. https://doi.org/10.1186/1743-0003-11-137 .
Article Google Scholar
De Los Reyes-Guzman A, Dimbwadyo-Terrer I, Trincado-Alonso F, Monasterio-Huelin F, Torricelli D, Gil-Agudo A. Quantitative assessment based on kinematic measures of functional impairments during upper extremity movements: a review. Clin Biomech. 2014;29(7):719–27. https://doi.org/10.1016/j.clinbiomech.2014.06.013 .
Molteni E, Preatoni E, Cimolin V, Bianchi AM, Galli M, Rodano R. A methodological study for the multifactorial assessment of motor adaptation: integration of kinematic and neural factors. 2010 Annu Int Conf IEEE Eng Med Biol Soc EMBC’10. 2010;4910–3.
Mazzoleni S, Coscia M, Rossi G, Aliboni S, Posteraro F, Carrozza MC. Effects of an upper limb robot-mediated therapy on paretic upper limb in chronic hemiparetic subjects: a biomechanical and EEG-based approach for functional assessment. 2009 IEEE Int Conf Rehabil Robot ICORR 2009. 2009;92–7.
Úbeda A, Azorín JM, Chavarriaga R, Millán JdR. Classification of upper limb center-out reaching tasks by means of EEG-based continuous decoding techniques. J Neuroeng Rehabil. 2017;14(1):1–14.
Pierella C, Pirondini E, Kinany N, Coscia M, Giang C, Miehlbradt J, et al. A multimodal approach to capture post-stroke temporal dynamics of recovery. J Neural Eng. 2020;17(4): 045002.
Steinisch M, Tana MG, Comani S. A post-stroke rehabilitation system integrating robotics, VR and high-resolution EEG imaging. IEEE Trans Neural Syst Rehabil Eng. 2013;21(5):849–59. https://doi.org/10.1596/978-1-4648-1002-2_Module14 .
Úbeda A, Hortal E, Iáñez E, Perez-Vidal C, Azorín JM. Assessing movement factors in upper limb kinematics decoding from EEG signals. PLoS ONE. 2015;10(5):1–12.
Belfatto A, Scano A, Chiavenna A, Mastropietro A, Mrakic-Sposta S, Pittaccio S, et al. A multiparameter approach to evaluate post-stroke patients: an application on robotic rehabilitation. Appl Sci. 2018;8(11):2248.
Comani S, Schinaia L, Tamburro G, Velluto L, Sorbi S, Conforto S, et al. Assessing Neuromotor Recovery in a stroke survivor with high resolution EEG, robotics and virtual reality. In: Annual International Conference of the IEEE Engineering in Medicine and Biology Society (EMBC). 2015. p. 3925–8.
Kwon HM, Yang IH, Lee WS, Yu ARL, Oh SY, Park KK. Reliability of intraoperative knee range of motion measurements by goniometer compared with robot-assisted arthroplasty. J Knee Surg. 2019;32(3):233–8.
Dukelow SP, Herter TM, Moore KD, Demers MJ, Glasgow JI, Bagg SD, et al. Quantitative assessment of limb position sense following stroke. Neurorehabil Neural Repair. 2010;24(2):178–87.
Balasubramanian S, Wei R, Herman R, He J. Robot-measured performance metrics in stroke rehabilitation. In: 2009 ICME International Conference on Complex Medical Engineering, CME 2009. 2009.
Otaka E, Otaka Y, Kasuga S, Nishimoto A, Yamazaki K, Kawakami M, et al. Clinical usefulness and validity of robotic measures of reaching movement in hemiparetic stroke patients. J Neuroeng Rehabil. 2015;12(1):66.
Singh H, Unger J, Zariffa J, Pakosh M, Jaglal S, Craven BC, et al. Robot-assisted upper extremity rehabilitation for cervical spinal cord injuries: a systematic scoping review. Disabil Rehabil Assist Technol. 2018;13(7):704–15. https://doi.org/10.1080/17483107.2018.1425747 .
Molteni F, Gasperini G, Cannaviello G, Guanziroli E. Exoskeleton and end-effector robots for upper and lower limbs rehabilitation: narrative review. PMR. 2018;10(9):174–88.
Jutinico AL, Jaimes JC, Escalante FM, Perez-Ibarra JC, Terra MH, Siqueira AAG. Impedance control for robotic rehabilitation: a robust markovian approach. Front Neurorobot. 2017;11(AUG):1–16.
Google Scholar
Li Z, Huang Z, He W, Su CY. Adaptive impedance control for an upper limb robotic exoskeleton using biological signals. IEEE Trans Ind Electron. 2017;64(2):1664–74.
Marchal-Crespo L, Reinkensmeyer DJ. Review of control strategies for robotic movement training after neurologic injury. J NeuroEngineering Rehabil. 2009;6:20. https://doi.org/10.1186/1743-0003-6-20 .
Cohen MX. Analyzing neural time series data: theory and practice. Cambridge: MIT Press; 2014.
Book Google Scholar
Stafstrom CE, Carmant L. Seizures and epilepsy: an overview. Cold Spring Harb Perspect Med. 2015;5(6):65–77.
Machado C, Cuspineda E, Valdãs P, Virues T, Liopis F, Bosch J, et al. Assessing acute middle cerebral artery ischemic stroke by quantitative electric tomography. Clin EEG Neurosci. 2004;35(3):116–24.
Finnigan SP, Walsh M, Rose SE, Chalk JB. Quantitative EEG indices of sub-acute ischaemic stroke correlate with clinical outcomes. Clin Neurophysiol. 2007;118(11):2525–31.
Cuspineda E, Machado C, Galán L, Aubert E, Alvarez MA, Llopis F, et al. QEEG prognostic value in acute stroke. Clin EEG Neurosci. 2007;38(3):155–60.
Belardinelli P, Laer L, Ortiz E, Braun C, Gharabaghi A. Plasticity of premotor cortico-muscular coherence in severely impaired stroke patients with hand paralysis. NeuroImage Clin. 2017;14:726–33.
Di PM, Schnider A, Nicolo P, Rizk S, Guggisberg AG. Coherent neural oscillations predict future motor and language improvement after stroke. Brain. 2015;138(10):3048–60.
Chen CC, Lee SH, Wang WJ, Lin YC, Su MC. EEG-based motor network biomarkers for identifying target patients with stroke for upper limb rehabilitation and its construct validity. PLoS ONE. 2017;12(6):1–20. https://doi.org/10.1371/journal.pone.0178822 .
Article CAS Google Scholar
Conway BA, Halliday DM, Farmer SF, Shahani U, Maas P, Weir AI, et al. Synchronization between motor cortex and spinal motoneuronal pool during the performance of a maintained motor task in man. J Physiol. 1995;489(3):917–24.
Article CAS PubMed PubMed Central Google Scholar
Salenius S, Portin K, Kajola M, Salmelin R, Hari R. Cortical control of human motoneuron firing during isometric contraction. J Neurophysiol. 1997;77(6):3401–5.
Mima T, Hallett M. Electroencephalographic analysis of cortico-muscular coherence: reference effect, volume conduction and generator mechanism. Clin Neurophysiol. 1999;110(11):1892–9.
Claassen J, Hirsch LJ, Kreiter KT, Du EY, Sander Connolly E, Emerson RG, et al. Quantitative continuous EEG for detecting delayed cerebral ischemia in patients with poor-grade subarachnoid hemorrhage. Clin Neurophysiol. 2004;115(12):2699–710.
Sullivan JL, Bhagat NA, Yozbatiran N, Paranjape R, Losey CG, Grossman RG, et al. Improving robotic stroke rehabilitation by incorporating neural intent detection: preliminary results from a clinical trial. In: 2017 International Conference on Rehabilitation Robotics (ICORR). IEEE; 2017. p. 122–7.
Muralidharan A, Chae J, Taylor DM. Extracting attempted hand movements from EEGs in people with complete hand paralysis following stroke. Front Neurosci. 2011. https://doi.org/10.3389/fnins.2011.00039 .
Nam C, Rong W, Li W, Xie Y, Hu X, Zheng Y. The effects of upper-limb training assisted with an electromyography-driven neuromuscular electrical stimulation robotic hand on chronic stroke. Front Neurol. 2017. https://doi.org/10.3389/fneur.2017.00679 .
Bertolucci F, Lamola G, Fanciullacci C, Artoni F, Panarese A, Micera S, et al. EEG predicts upper limb motor improvement after robotic rehabilitation in chronic stroke patients. Ann Phys Rehabil Med. 2018;61:e200–1.
Cantillo-Negrete J, Carino-Escobar RI, Carrillo-Mora P, Elias-Vinas D, Gutierrez-Martinez J. Motor imagery-based brain-computer interface coupled to a robotic hand orthosis aimed for neurorehabilitation of stroke patients. J Healthc Eng. 2018;3(2018):1–10.
Bhagat NA, Venkatakrishnan A, Abibullaev B, Artz EJ, Yozbatiran N, Blank AA, et al. Design and optimization of an EEG-based brain machine interface (BMI) to an upper-limb exoskeleton for stroke survivors. Front Neurosci. 2016;10(MAR):122.
PubMed PubMed Central Google Scholar
Biasiucci A, Leeb R, Iturrate I, Perdikis S, Al-Khodairy A, Corbet T, et al. Brain-actuated functional electrical stimulation elicits lasting arm motor recovery after stroke. Nat Commun. 2018;9(1):1–13. https://doi.org/10.1038/s41467-018-04673-z .
Ang KK, Guan C, Chua KSG, Ang BT, Kuah C, Wang C, et al. Clinical study of neurorehabilitation in stroke using EEG-based motor imagery brain-computer interface with robotic feedback. Annu Int Conf IEEE Eng Med Biol. 2010. pp. 5549–52.
Finnigan SP, Rose SE, Walsh M, Griffin M, Janke AL, Mcmahon KL, et al. Correlation of quantitative EEG in acute ischemic stroke with 30-day NIHSS score: comparison with diffusion and perfusion MRI. Stroke. 2004;35(4):899–903.
Schleiger E, Sheikh N, Rowland T, Wong A, Read S, Finnigan S. Frontal EEG delta / alpha ratio and screening for post-stroke cognitive de fi cits: the power of four electrodes. Int J Psychophysiol. 2014;94(1):19–24. https://doi.org/10.1016/j.ijpsycho.2014.06.012 .
Aminov A, Rogers JM, Johnstone SJ, Middleton S, Wilson PH. Acute single channel EEG predictors of cognitive function after stroke. PLoS ONE. 2017;12(10): e0185841.
Andresen EM. Criteria for assessing the tools of disability outcomes research. Arch Phys Med Rehabil. 2000. https://doi.org/10.1053/apmr.2000.20619 .
Wang Q, Markopoulos P, Yu B, Chen W, Timmermans A. Interactive wearable systems for upper body rehabilitation: a systematic review. J Neuroeng Rehabil. 2017;14(1):1–21.
Tran VD, Dario P, Mazzoleni S. Kinematic measures for upper limb robot-assisted therapy following stroke and correlations with clinical outcome measures: a review. Med Eng Phys. 2018;53:13–31. https://doi.org/10.1016/j.medengphy.2017.12.005 .
Finnigan S, Wong A, Read S. Defining abnormal slow EEG activity in acute ischaemic stroke: Delta/alpha ratio as an optimal QEEG index. Clin Neurophysiol. 2016;127(2):1452–9. https://doi.org/10.1016/j.clinph.2015.07.014 .
Carter AR, Shulman GL, Corbetta M. Why use a connectivity-based approach to study stroke and recovery of function? Neuroimage. 2012;62(4):2271–80.
van der Velde B, Haartsen R, Kemner C. Test-retest reliability of EEG network characteristics in infants. Brain Behav. 2019;9(5):1–10.
Gennaro F, de Bruin ED. A pilot study assessing reliability and age-related differences in corticomuscular and intramuscular coherence in ankle dorsiflexors during walking. Physiol Rep. 2020;8(4):1–12.
Brihmat N, Loubinoux I, Castel-Lacanal E, Marque P, Gasq D. Kinematic parameters obtained with the ArmeoSpring for upper-limb assessment after stroke: a reliability and learning effect study for guiding parameter use. J Neuroeng Rehabil. 2020;17(1):130. https://doi.org/10.1186/s12984-020-00759-2 .
Dewald JPA, Ellis MD, Acosta AM, McPherson JG, Stienen AHA. Implementation of impairment- based neurorehabilitation devices and technologies following brain injury. Neurorehabilitation technology, 2nd edn. 2016. 375–392 p.
Subramanian SK, Yamanaka J, Chilingaryan G, Levin MF. Validity of movement pattern kinematics as measures of arm motor impairment poststroke. Stroke. 2010;41(10):2303–8.
Fayers PM, Machin D. Quality of life: the assessment, analysis and reporting of patient‐reported outcomes . John Wiley & Sons, Incorporated. 2016;3:89-124.
Alt Murphy M, Häger CK. Kinematic analysis of the upper extremity after stroke—how far have we reached and what have we grasped? Phys Ther Rev. 2015;20(3):137–55.
Shishov N, Melzer I, Bar-Haim S. Parameters and measures in assessment of motor learning in neurorehabilitation; a systematic review of the literature. Front Hum Neurosci. 2017. https://doi.org/10.3389/fnhum.2017.00082 .
Koo TK, Li MY. A guideline of selecting and reporting intraclass correlation coefficients for reliability research. J Chiropr Med. 2016;15(2):155–63. https://doi.org/10.1016/j.jcm.2016.02.012 .
Angel RW. Electromyographic patterns during ballistic movement of normal and spastic limbs. Brain Res. 1975;99(2):387–92.
McLellan DL. C0-contraction and stretch reflexes in spasticity during treatment with baclofen. J Neurol Neurosurg Psychiatry. 1977;40(1):30–8.
Dewald JPA, Pope PS, Given JD, Buchanan TS, Rymer WZ. Abnormal muscle coactivation patterns during isometric torque generation at the elbow and shoulder in hemiparetic subjects. Brain. 1995;118(2):495–510. https://doi.org/10.1093/brain/118.2.495 .
Wilkins KB, Yao J, Owen M, Karbasforoushan H, Carmona C, Dewald JPA. Limited capacity for ipsilateral secondary motor areas to support hand function post-stroke. J Physiol. 2020;598(11):2153–67. https://doi.org/10.1113/JP279377 .
Agius Anastasi A, Falzon O, Camilleri K, Vella M, Muscat R. Brain symmetry index in healthy and stroke patients for assessment and prognosis. Stroke Res Treat. 2017;30(2017):1–9.
Liberati A, Altman DG, Tetzlaff J, Mulrow C, Gøtzsche PC, Ioannidis JPA, et al. The PRISMA statement for reporting systematic reviews and meta-analyses of studies that evaluate healthcare interventions: explanation and elaboration. BMJ. 2009;339: b2700.
Colombo R, Pisano F, Micera S, Mazzone A, Delconte C, Carrozza MC, et al. Assessing mechanisms of recovery during robot-aided neurorehabilitation of the upper limb. Neurorehabil Neural Repair. 2008;22(1):50–63. https://doi.org/10.1177/1545968307303401 .
Keller U, Schölch S, Albisser U, Rudhe C, Curt A, Riener R, et al. Robot-assisted arm assessments in spinal cord injured patients: a consideration of concept study. PLoS One. 2015;10(5):e0126948. https://doi.org/10.1371/journal.pone.0126948 .
Mostafavi SM. Computational models for improved diagnosis and prognosis of stroke using robot-based biomarkers. 2016. http://hdl.handle.net/1974/14563 .
Bosecker C, Dipietro L, Volpe B, Krebs HI. Kinematic robot-based evaluation scales and clinical counterparts to measure upper limb motor performance in patients with chronic stroke. Neurorehabil Neural Repair. 2010;24(1):62–9.
Balasubramanian S, Melendez-Calderon A, Roby-Brami A, Burdet E. On the analysis of movement smoothness. J Neuroeng Rehabil. 2015;12(1):112. https://doi.org/10.1186/s12984-015-0090-9 .
Rohrer B, Fasoli S, Krebs HI, Hughes R, Volpe B, Frontera WR, et al. Movement smoothness changes during stroke recovery. J Neurosci. 2002;22(18):8297–304.
Mobini A, Behzadipour S, Saadat M. Test-retest reliability of Kinect’s measurements for the evaluation of upper body recovery of stroke patients. Biomed Eng Online. 2015;14(1):1–14.
Zariffa J, Myers M, Coahran M, Wang RH. Smallest real differences for robotic measures of upper extremity function after stroke: implications for tracking recovery. J Rehabil Assist Technol Eng. 2018;5:205566831878803. https://doi.org/10.1177/2055668318788036 .
Elovic E, Brashear A. Spasticity : diagnosis and management. New York: Demos Medical; 2011. http://ida.lib.uidaho.edu:2048/login?url=http://search.ebscohost.com/login.aspx?direct=true&db=e000xna&AN=352265&site=ehost-live&scope=site .
Centen A, Lowrey CR, Scott SH, Yeh TT, Mochizuki G. KAPS (kinematic assessment of passive stretch): a tool to assess elbow flexor and extensor spasticity after stroke using a robotic exoskeleton. J Neuroeng Rehabil. 2017;14(1):1–13.
Sin M, Kim WS, Cho K, Cho S, Paik NJ. Improving the test-retest and inter-rater reliability for stretch reflex measurements using an isokinetic device in stroke patients with mild to moderate elbow spasticity. J Electromyogr Kinesiol. 2017;2018(39):120–7. https://doi.org/10.1016/j.jelekin.2018.01.012 .
Germanotta M, Cruciani A, Pecchioli C, Loreti S, Spedicato A, Meotti M, et al. Reliability, validity and discriminant ability of the instrumental indices provided by a novel planar robotic device for upper limb rehabilitation. J Neuroeng Rehabil. 2018;15(1):1–14.
Wagner JM, Rhodes JA, Patten C. Reproducibility and minimal detectable change of three-dimensional kinematic analysis of reaching tasks in people with hemiparaesis after stroke. 2008. https://doi.org/10.2522/ptj.20070255 .
Semrau JA, Herter TM, Scott SH, Dukelow SP. Inter-rater reliability of kinesthetic measurements with the KINARM robotic exoskeleton. J Neuroeng Rehabil. 2017;14(1):1–10.
Lin CH, Chou LW, Wei SH, Lieu FK, Chiang SL, Sung WH. Validity and reliability of a novel device for bilateral upper extremity functional measurements. Comput Methods Programs Biomed. 2014;114(3):315–23. https://doi.org/10.1016/j.cmpb.2014.02.012 .
Wolf S, Butler A, Alberts J, Kim M. Contemporary linkages between EMG, kinetics and stroke. J Electromyogr Kinesiol. 2005;15(3):229–39.
Iyer KK. Effective assessments of electroencephalography during stroke recovery : contemporary approaches and considerations. J Neurophysiol. 2017;118(5):2521–5.
Liu J, Sheng Y, Liu H. Corticomuscular coherence and its applications: a review. Front Hum Neurosci. 2019;13(March):1–16.
Pan LLH, Yang WW, Kao CL, Tsai MW, Wei SH, Fregni F, et al. Effects of 8-week sensory electrical stimulation combined with motor training on EEG-EMG coherence and motor function in individuals with stroke. Sci Rep. 2018;8(1):1–10.
Wu J, Quinlan EB, Dodakian L, McKenzie A, Kathuria N, Zhou RJ, et al. Connectivity measures are robust biomarkers of cortical function and plasticity after stroke. Brain. 2015;138(8):2359–69.
Mrachacz-Kersting N, Jiang N, Thomas Stevenson AJ, Niazi IK, Kostic V, Pavlovic A, et al. Efficient neuroplasticity induction in chronic stroke patients by an associative brain-computer interface. J Neurophysiol. 2016;115(3):1410–21.
Bentes C, Peralta AR, Viana P, Martins H, Morgado C, Casimiro C, et al. Quantitative EEG and functional outcome following acute ischemic stroke. Clin Neurophysiol. 2018;129(8):1680–7.
Leon-carrion J, Martin-rodriguez JF, Damas-lopez J, Manuel J, Dominguez-morales MR. Delta–alpha ratio correlates with level of recovery after neurorehabilitation in patients with acquired brain injury. Clin Neurophysiol. 2009;120(6):1039–45. https://doi.org/10.1016/j.clinph.2009.01.021 .
Finnigan S, van Putten MJAM. EEG in ischaemic stroke: qEEG can uniquely inform (sub-)acute prognoses and clinical management. Clin Neurophysiol. 2013;124(1):10–9.
Trujillo P, Mastropietro A, Scano A, Chiavenna A, Mrakic-Sposta S, Caimmi M, et al. Quantitative EEG for predicting upper limb motor recovery in chronic stroke robot-assisted rehabilitation. IEEE Trans Neural Syst Rehabil Eng. 2017;25(7):1058–67.
Jordan K. Emergency EEG and continuous EEG monitoring in acute ischemic stroke. Clin Neurophysiol. 2004;21(5):341–52.
Comani S, Velluto L, Schinaia L, Cerroni G, Serio A, Buzzelli S, et al. Monitoring neuro-motor recovery from stroke with high-resolution EEG, robotics and virtual reality: a proof of concept. IEEE Trans Neural Syst Rehabil Eng. 2015;23(6):1106–16.
Espenhahn S, de Berker AO, van Wijk BCM, Rossiter HE, Ward NS. Movement-related beta oscillations show high intra-individual reliability. Neuroimage. 2017;147:175–85. https://doi.org/10.1016/j.neuroimage.2016.12.025 .
Vázquez-Marrufo M, Galvao-Carmona A, Benítez Lugo ML, Ruíz-Peña JL, Borges Guerra M, Izquierdo AG. Retest reliability of individual alpha ERD topography assessed by human electroencephalography. PLoS ONE. 2017;12(10):1–16.
Dubovik S, Ptak R, Aboulafia T, Magnin C, Gillabert N, Allet L, et al. EEG alpha band synchrony predicts cognitive and motor performance in patients with ischemic stroke. In: Behavioural Neurology. Hindawi Limited; 2013. p. 187–9.
Sheorajpanday RVAA, Nagels G, Weeren AJTMTM, Putten MJAMV, Deyn PPD, van Putten MJAM, et al. Quantitative EEG in ischemic stroke: correlation with functional status after 6 months. Clin Neurophysiol. 2011;122(5):874–83. https://doi.org/10.1016/j.clinph.2010.07.028 .
De Vico Fallani F, Astolfi L, Cincotti F, Mattia D, La Rocca D, Maksuti E, et al. Evaluation of the brain network organization from EEG signals: a preliminary evidence in stroke patient. In: Anatomical Record. 2009. p. 2023–31.
Westlake KP, Nagarajan SS. Functional connectivity in relation to motor performance and recovery after stroke. Front Syst Neurosci. 2011;18(5):8.
Caliandro P, Vecchio F, Miraglia F, Reale G, Della Marca G, La Torre G, et al. Small-world characteristics of cortical connectivity changes in acute stroke. Neurorehabil Neural Repair. 2017;31(1):81–94.
Eldeeb S, Akcakaya M, Sybeldon M, Foldes S, Santarnecchi E, Pascual-Leone A, et al. EEG-based functional connectivity to analyze motor recovery after stroke: a pilot study. Biomed Signal Process Control. 2019;49:419–26.
Myers LJ, O’Malley M. The relationship between human cortico-muscular coherence and rectified EMG. In: International IEEE/EMBS Conference on Neural Engineering, NER. IEEE Computer Society; 2003. p. 289–92.
Braun C, Staudt M, Schmitt C, Preissl H, Birbaumer N, Gerloff C. Crossed cortico-spinal motor control after capsular stroke. Eur J Neurosci. 2007;25(9):2935–45.
Larsen LH, Zibrandtsen IC, Wienecke T, Kjaer TW, Christensen MS, Nielsen JB, et al. Corticomuscular coherence in the acute and subacute phase after stroke. Clin Neurophysiol. 2017;128(11):2217–26.
Ang KK, Chua KSG, Phua KS, Wang C, Chin ZY, Kuah CWK, et al. A randomized controlled trial of EEG-based motor imagery brain-computer interface robotic rehabilitation for stroke. Clin EEG Neurosci. 2015;46(4):310–20.
Liu S, Guo J, Meng J, Wang Z, Yao Y, Yang J, et al. Abnormal EEG complexity and functional connectivity of brain in patients with acute thalamic ischemic stroke. Comput Math Methods Med. 2016;14(2016):1–9.
CAS Google Scholar
Sun R, Wong W, Wang J, Tong RK. Changes in electroencephalography complexity using a brain computer interface-motor observation training in chronic stroke patients : a fuzzy approximate entropy analysis. Front Hum Neurosci. 2017;5(11):444.
Auriat AM, Neva JL, Peters S, Ferris JK, Boyd LA. A review of transcranial magnetic stimulation and multimodal neuroimaging to characterize post-stroke neuroplasticity. Front Neurol. 2015;6:1–20.
Niedermeyer E, Schomer DL, Lopes da Silva FH. Niedermeyer’s electroencephalography: basic principles, clinical applications, and related fields, 6th edn. Philadelphia: Lippincott Williams & Wilkins.; 2011.
Foreman B, Claasen J. Update in intensive care and emergency medicine. Update in intensive care and emergency medicine. Springer Berlin Heidelberg; 2012.
Tolonen U, Ahonen A, Kallanranta T, Hokkanen E. Non-invasive external regional measurement of cerebral circulation time changes in supratentorial infarctions using pertechnetate. Stroke. 1981;12(4):437–44.
Saes M, Zandvliet SB, Andringa AS, Daffertshofer A, Twisk JWR, Meskers CGM, et al. Is resting-state EEG longitudinally associated with recovery of clinical neurological impairments early poststroke? A prospective cohort study. Neurorehabil Neural Repair. 2020;34(5):389–402.
Rogers J, Middleton S, Wilson PH, Johnstone SJ. Predicting functional outcomes after stroke: an observational study of acute single-channel EEG. Top Stroke Rehabil. 2020;27(3):161–72. https://doi.org/10.1080/10749357.2019.1673576 .
Sale P, Infarinato F, Lizio R, Babiloni C. Electroencephalographic markers of robot-aided therapy in stroke patients for the evaluation of upper limb rehabilitation. Rehabil Res. 2015;38(4):294–305.
Sheorajpanday RVA, Nagels G, Weeren AJTM, De Surgeloose D, De Deyn PP, De DPP. Additional value of quantitative EEG in acute anterior circulation syndrome of presumed ischemic origin. Clin Neurophysiol. 2010;121(10):1719–25.
Saes M, Meskers CGM, Daffertshofer A, van Wegen EEH, Kwakkel G. Are early measured resting-state EEG parameters predictive for upper limb motor impairment six months poststroke? Clin Neurophysiol. 2021;132(1):56–62. https://doi.org/10.1016/j.clinph.2020.09.031 .
Saes M, Meskers CGM, Daffertshofer A, de Munck JC, Kwakkel G, van Wegen EEH. How does upper extremity Fugl-Meyer motor score relate to resting-state EEG in chronic stroke? A power spectral density analysis. Clin Neurophysiol. 2019;130(5):856–62. https://doi.org/10.1016/j.clinph.2019.01.007 .
Nolte G, Bai O, Mari Z, Vorbach S, Hallett M. Identifying true brain interaction from EEG data using the imaginary part of coherency. Clin Neurophysiol. 2004;115(10):2292–307.
Stam CJ, Nolte G, Daffertshofer A. Phase lag index: assessment of functional connectivity from multi channel EEG and MEG with diminished bias from common sources. Hum Brain Mapp. 2007;28(11):1178–93.
Bullmore E, Sporns O. Complex brain networks: graph theoretical analysis of structural and functional systems. Nat Rev Neurosci. 2009;10(3):186–98.
Baccalá LA, Sameshima K. Partial directed coherence: a new concept in neural structure determination. Biol Cybern. 2001;84(6):463–74.
Baccalá LA, Sameshima K, Takahashi D. Generalized partial directed coherence. Int Conf Digit Signal Process. 2007;3:163–6.
Schelter B, Timmer J, Eichler M. Assessing the strength of directed influences among neural signals using renormalized partial directed coherence. J Neurosci Methods. 2009;179(1):121–30.
Kamiński M, Ding M, Truccolo WA, Bressler SL. Evaluating causal relations in neural systems: Granger causality, directed transfer function and statistical assessment of significance. Biol Cybern. 2001;85(2):145–57.
Korzeniewska A, Mańczak M, Kamiński M, Blinowska KJ, Kasicki S. Determination of information flow direction among brain structures by a modified directed transfer function (dDTF) method. J Neurosci Methods. 2003;125(1–2):195–207.
Fornito A, Bullmore ET, Zalesky A. Fundamentals of brain network analysis. Cambridge: Academic Press; 2016.
Philips GR, Daly JJ, Príncipe JC. Topographical measures of functional connectivity as biomarkers for post-stroke motor recovery. J Neuroeng Rehabil. 2017;14(1):67.
Pichiorri F, Petti M, Caschera S, Astolfi L, Cincotti F, Mattia D. An EEG index of sensorimotor interhemispheric coupling after unilateral stroke: clinical and neurophysiological study. Eur J Neurosci. 2018;47(2):158–63.
Hoshino T, Oguchi K, Inoue K, Hoshino A, Hoshiyama M. Relationship between upper limb function and functional neural connectivity among motor related-areas during recovery stage after stroke. Top Stroke Rehabil. 2020;27(1):57–66. https://doi.org/10.1080/10749357.2019.1658429 .
Hordacre B, Goldsworthy MR, Welsby E, Graetz L, Ballinger S, Hillier S. Resting state functional connectivity is associated with motor pathway integrity and upper-limb behavior in chronic stroke. Neurorehabil Neural Repair. 2020;34(6):547–57.
Riahi N, Vakorin VA, Menon C. Estimating Fugl-Meyer upper extremity motor score from functional-connectivity measures. IEEE Trans Neural Syst Rehabil Eng. 2020;28(4):860–8.
Gwin JT, Ferris DP. Beta- and gamma-range human lower limb corticomuscular coherence. Front Hum Neurosci. 2012;11(6):258.
Zheng Y, Peng Y, Xu G, Li L, Wang J. Using corticomuscular coherence to reflect function recovery of paretic upper limb after stroke: a case study. Front Neurol. 2018;10(8):728.
Rossiter HE, Eaves C, Davis E, Boudrias MH, Park CH, Farmer S, et al. Changes in the location of cortico-muscular coherence following stroke. NeuroImage Clin. 2013;2(1):50–5.
Mima T, Toma K, Koshy B, Hallett M. Coherence between cortical and muscular activities after subcortical stroke. Stroke. 2001;32(11):2597–601.
Krauth R, Schwertner J, Vogt S, Lindquist S, Sailer M, Sickert A, et al. Cortico-muscular coherence is reduced acutely post-stroke and increases bilaterally during motor recovery: a pilot study. Front Neurol. 2019;20(10):126.
Bao SC, Wong WW, Leung TW, Tong KY. Low gamma band cortico-muscular coherence inter-hemisphere difference following chronic stroke. In: Proceedings of the Annual International Conference of the IEEE Engineering in Medicine and Biology Society, EMBS. Institute of Electrical and Electronics Engineers Inc.; 2018. p. 247–50.
von Carlowitz-Ghori K, Bayraktaroglu Z, Hohlefeld FU, Losch F, Curio G, Nikulin VV. Corticomuscular coherence in acute and chronic stroke. Clin Neurophysiol. 2014;125(6):1182–91.
Chen X, Xie P, Zhang Y, Chen Y, Cheng S, Zhang L. Abnormal functional corticomuscular coupling after stroke. NeuroImage Clin. 2018;19:147–59. https://doi.org/10.1016/j.nicl.2018.04.004 .
Curado MR, Cossio EG, Broetz D, Agostini M, Cho W, Brasil FL, et al. Residual upper arm motor function primes innervation of paretic forearm muscles in chronic stroke after brain-machine interface (BMI) training. PLoS ONE. 2015;10(10):1–18.
Guo Z, Qian Q, Wong K, Zhu H, Huang Y, Hu X, et al. Altered corticomuscular coherence (CMCoh) pattern in the upper limb during finger movements after stroke. Front Neurol. 2020. https://doi.org/10.3389/fneur.2020.00410 .
Bruton A, Conway JH, Holgate ST. Reliability: what is it, and how is it measured? Physiotherapy. 2000;86(2):94–9.
Colombo R, Cusmano I, Sterpi I, Mazzone A, Delconte C, Pisano F. Test-retest reliability of robotic assessment measures for the evaluation of upper limb recovery. IEEE Trans Neural Syst Rehabil Eng. 2014;22(5):1020–9.
Costa V, Ramírez Ó, Otero A, Muñoz-García D, Uribarri S, Raya R. Validity and reliability of inertial sensors for elbow and wrist range of motion assessment. PeerJ. 2020;8: e9687.
Gasser T, Bächer P, Steinberg H. Test-retest reliability of spectral parameters of the EEG. Electroencephalogr Clin Neurophysiol. 1985;60(4):312–9.
Levin AR, Naples AJ, Scheffler AW, Webb SJ, Shic F, Sugar CA, et al. Day-to-day test-retest reliability of EEG profiles in children with autism spectrum disorder and typical development. Front Integr Neurosci. 2020;14:1–12.
Briels CT, Briels CT, Schoonhoven DN, Schoonhoven DN, Stam CJ, De Waal H, et al. Reproducibility of EEG functional connectivity in Alzheimer’s disease. Alzheimer’s Res Ther. 2020;12(1):1–14.
Marquetand J, Vannoni S, Carboni M, Li Hegner Y, Stier C, Braun C, et al. Reliability of magnetoencephalography and high-density electroencephalography resting-state functional connectivity metrics. Brain Connect. 2019;9(7):539–53.
Lowrey CR, Blazevski B, Marnet J-L, Bretzke H, Dukelow SP, Scott SH. Robotic tests for position sense and movement discrimination in the upper limb reveal that they each are highly reproducible but not correlated in healthy individuals. J Neuroeng Rehabil. 2020;17(1):103. https://doi.org/10.1186/s12984-020-00721-2 .
Simmatis LER, Early S, Moore KD, Appaqaq S, Scott SH. Statistical measures of motor, sensory and cognitive performance across repeated robot-based testing. J Neuroeng Rehabil. 2020;17(1):86. https://doi.org/10.1186/s12984-020-00713-2 .
Download references
Acknowledgements
The authors would like to thank Stephen Goodwin and Aaron I. Feinstein for their contributions to the collection and organization of references on robotic systems, measurements, and metrics.
This work was funded by the National Science Foundation (Award#1532239) and the Eunice Kennedy Shriver National Institute of Child Health & Human Development of the National Institutes of Health (Award#K12HD073945). The content is solely the responsibility of the authors and does not necessarily represent the official views of the National Science Foundation nor the National Institutes of Health.
Author information
Authors and affiliations.
Mechanical Engineering Department, University of Idaho, Moscow, ID, USA
Rene M. Maura, Eric T. Wolbrecht & Joel C. Perry
Engineering and Physics Department, Whitworth University, Spokane, WA, USA
Richard E. Stevens
College of Medicine, Washington State University, Spokane, WA, USA
Douglas L. Weeks
Electrical Engineering Department, University of Idaho, ID, Moscow, USA
Sebastian Rueda Parra
You can also search for this author in PubMed Google Scholar
Contributions
RM, and SRP drafted the manuscript and performed the literature search. EW, JP, RS, and DW provided concepts, edited, and revised the manuscript. All authors read and approved the final manuscript.
Corresponding author
Correspondence to Rene M. Maura .
Ethics declarations
Ethics approval and consent to participate, consent for publication, competing interests.
The authors declare that they have no competing interests.
Additional information
Publisher's note.
Springer Nature remains neutral with regard to jurisdictional claims in published maps and institutional affiliations.
See Table 11 .
See Table 12 .
See Table 13 .
Rights and permissions
Open Access This article is licensed under a Creative Commons Attribution 4.0 International License, which permits use, sharing, adaptation, distribution and reproduction in any medium or format, as long as you give appropriate credit to the original author(s) and the source, provide a link to the Creative Commons licence, and indicate if changes were made. The images or other third party material in this article are included in the article's Creative Commons licence, unless indicated otherwise in a credit line to the material. If material is not included in the article's Creative Commons licence and your intended use is not permitted by statutory regulation or exceeds the permitted use, you will need to obtain permission directly from the copyright holder. To view a copy of this licence, visit http://creativecommons.org/licenses/by/4.0/ . The Creative Commons Public Domain Dedication waiver ( http://creativecommons.org/publicdomain/zero/1.0/ ) applies to the data made available in this article, unless otherwise stated in a credit line to the data.
Reprints and permissions
About this article
Cite this article.
Maura, R.M., Rueda Parra, S., Stevens, R.E. et al. Literature review of stroke assessment for upper-extremity physical function via EEG, EMG, kinematic, and kinetic measurements and their reliability. J NeuroEngineering Rehabil 20 , 21 (2023). https://doi.org/10.1186/s12984-023-01142-7
Download citation
Received : 27 May 2021
Accepted : 19 January 2023
Published : 15 February 2023
DOI : https://doi.org/10.1186/s12984-023-01142-7
Share this article
Anyone you share the following link with will be able to read this content:
Sorry, a shareable link is not currently available for this article.
Provided by the Springer Nature SharedIt content-sharing initiative
- Reliability
- Robot-assisted therapy
- Exoskeleton
- Neurological assessment
- Rehabilitation
- Motor function
Journal of NeuroEngineering and Rehabilitation
ISSN: 1743-0003
- Submission enquiries: [email protected]
Thank you for visiting nature.com. You are using a browser version with limited support for CSS. To obtain the best experience, we recommend you use a more up to date browser (or turn off compatibility mode in Internet Explorer). In the meantime, to ensure continued support, we are displaying the site without styles and JavaScript.
- View all journals
- My Account Login
- Explore content
- About the journal
- Publish with us
- Sign up for alerts
- Open access
- Published: 08 April 2024
A systematic review and multivariate meta-analysis of the physical and mental health benefits of touch interventions
- Julian Packheiser ORCID: orcid.org/0000-0001-9805-6755 2 na1 nAff1 ,
- Helena Hartmann 2 , 3 , 4 na1 ,
- Kelly Fredriksen 2 ,
- Valeria Gazzola ORCID: orcid.org/0000-0003-0324-0619 2 ,
- Christian Keysers ORCID: orcid.org/0000-0002-2845-5467 2 &
- Frédéric Michon ORCID: orcid.org/0000-0003-1289-2133 2
Nature Human Behaviour ( 2024 ) Cite this article
21k Accesses
1923 Altmetric
Metrics details
- Human behaviour
- Paediatric research
- Randomized controlled trials
Receiving touch is of critical importance, as many studies have shown that touch promotes mental and physical well-being. We conducted a pre-registered (PROSPERO: CRD42022304281) systematic review and multilevel meta-analysis encompassing 137 studies in the meta-analysis and 75 additional studies in the systematic review ( n = 12,966 individuals, search via Google Scholar, PubMed and Web of Science until 1 October 2022) to identify critical factors moderating touch intervention efficacy. Included studies always featured a touch versus no touch control intervention with diverse health outcomes as dependent variables. Risk of bias was assessed via small study, randomization, sequencing, performance and attrition bias. Touch interventions were especially effective in regulating cortisol levels (Hedges’ g = 0.78, 95% confidence interval (CI) 0.24 to 1.31) and increasing weight (0.65, 95% CI 0.37 to 0.94) in newborns as well as in reducing pain (0.69, 95% CI 0.48 to 0.89), feelings of depression (0.59, 95% CI 0.40 to 0.78) and state (0.64, 95% CI 0.44 to 0.84) or trait anxiety (0.59, 95% CI 0.40 to 0.77) for adults. Comparing touch interventions involving objects or robots resulted in similar physical (0.56, 95% CI 0.24 to 0.88 versus 0.51, 95% CI 0.38 to 0.64) but lower mental health benefits (0.34, 95% CI 0.19 to 0.49 versus 0.58, 95% CI 0.43 to 0.73). Adult clinical cohorts profited more strongly in mental health domains compared with healthy individuals (0.63, 95% CI 0.46 to 0.80 versus 0.37, 95% CI 0.20 to 0.55). We found no difference in health benefits in adults when comparing touch applied by a familiar person or a health care professional (0.51, 95% CI 0.29 to 0.73 versus 0.50, 95% CI 0.38 to 0.61), but parental touch was more beneficial in newborns (0.69, 95% CI 0.50 to 0.88 versus 0.39, 95% CI 0.18 to 0.61). Small but significant small study bias and the impossibility to blind experimental conditions need to be considered. Leveraging factors that influence touch intervention efficacy will help maximize the benefits of future interventions and focus research in this field.
Similar content being viewed by others
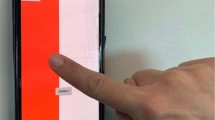
Touching the social robot PARO reduces pain perception and salivary oxytocin levels
Nirit Geva, Florina Uzefovsky & Shelly Levy-Tzedek
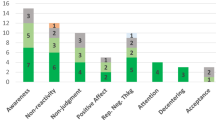
The impact of mindfulness apps on psychological processes of change: a systematic review
Natalia Macrynikola, Zareen Mir, … John Torous
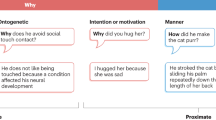
The why, who and how of social touch
Juulia T. Suvilehto, Asta Cekaite & India Morrison
The sense of touch has immense importance for many aspects of our life. It is the first of all the senses to develop in newborns 1 and the most direct experience of contact with our physical and social environment 2 . Complementing our own touch experience, we also regularly receive touch from others around us, for example, through consensual hugs, kisses or massages 3 .
The recent coronavirus pandemic has raised awareness regarding the need to better understand the effects that touch—and its reduction during social distancing—can have on our mental and physical well-being. The most common touch interventions, for example, massage for adults or kangaroo care for newborns, have been shown to have a wide range of both mental and physical health benefits, from facilitating growth and development to buffering against anxiety and stress, over the lifespan of humans and animals alike 4 . Despite the substantial weight this literature gives to support the benefits of touch, it is also characterized by a large variability in, for example, studied cohorts (adults, children, newborns and animals), type and duration of applied touch (for example, one-time hug versus repeated 60-min massages), measured health outcomes (ranging from physical health outcomes such as sleep and blood pressure to mental health outcomes such as depression or mood) and who actually applies the touch (for example, partner versus stranger).
A meaningful tool to make sense of this vast amount of research is through meta-analysis. While previous meta-analyses on this topic exist, they were limited in scope, focusing only on particular types of touch, cohorts or specific health outcomes (for example, refs. 5 , 6 ). Furthermore, despite best efforts, meaningful variables that moderate the efficacy of touch interventions could not yet be identified. However, understanding these variables is critical to tailor touch interventions and guide future research to navigate this diverse field with the ultimate aim of promoting well-being in the population.
In this Article, we describe a pre-registered, large-scale systematic review and multilevel, multivariate meta-analysis to address this need with quantitative evidence for (1) the effect of touch interventions on physical and mental health and (2) which moderators influence the efficacy of the intervention. In particular, we ask whether and how strongly health outcomes depend on the dynamics of the touching dyad (for example, humans or robots/objects, familiarity and touch directionality), demographics (for example, clinical status, age or sex), delivery means (for example, type of touch intervention or touched body part) and procedure (for example, duration or number of sessions). We did so separately for newborns and for children and adults, as the health outcomes in newborns differed substantially from those in the other age groups. Despite the focus of the analysis being on humans, it is widely known that many animal species benefit from touch interactions and that engaging in touch promotes their well-being as well 7 . Since animal models are essential for the investigation of the mechanisms underlying biological processes and for the development of therapeutic approaches, we accordingly included health benefits of touch interventions in non-human animals as part of our systematic review. However, this search yielded only a small number of studies, suggesting a lack of research in this domain, and as such, was insufficient to be included in the meta-analysis. We evaluate the identified animal studies and their findings in the discussion.
Touch interventions have a medium-sized effect
The pre-registration can be found at ref. 8 . The flowchart for data collection and extraction is depicted in Fig. 1 .
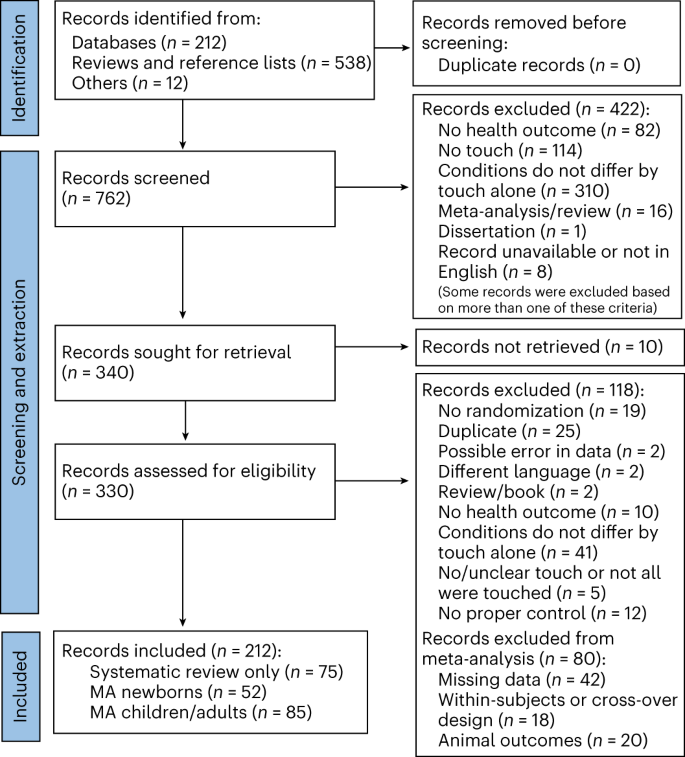
Animal outcomes refer to outcomes measured in non-human species that were solely considered as part of a systematic review. Included languages were French, Dutch, German and English, but our search did not identify any articles in French, Dutch or German. MA, meta-analysis.
For adults, a total of n = 2,841 and n = 2,556 individuals in the touch and control groups, respectively, across 85 studies and 103 cohorts were included. The effect of touch overall was medium-sized ( t (102) = 9.74, P < 0.001, Hedges’ g = 0.52, 95% confidence interval (CI) 0.42 to 0.63; Fig. 2a ). For newborns, we could include 63 cohorts across 52 studies comprising a total of n = 2,134 and n = 2,086 newborns in the touch and control groups, respectively, with an overall effect almost identical to the older age group ( t (62) = 7.53, P < 0.001, Hedges’ g = 0.56, 95% CI 0.41 to 0.71; Fig. 2b ), suggesting that, despite distinct health outcomes, touch interventions show comparable effects across newborns and adults. Using these overall effect estimates, we conducted a power sensitivity analysis of all the included primary studies to investigate whether such effects could be reliably detected 9 . Sufficient power to detect such effect sizes was rare in individual studies, as investigated by firepower plots 10 (Supplementary Figs. 1 and 2 ). No individual effect size from either meta-analysis was overly influential (Cook’s D < 0.06). The benefits were similar for mental and physical outcomes (mental versus physical; adults: t (101) = 0.79, P = 0.432, Hedges’ g difference of −0.05, 95% CI −0.16 to 0.07, Fig. 2c ; newborns: t (61) = 1.08, P = 0.284, Hedges’ g difference of −0.19, 95% CI −0.53 to 0.16, Fig. 2d ).
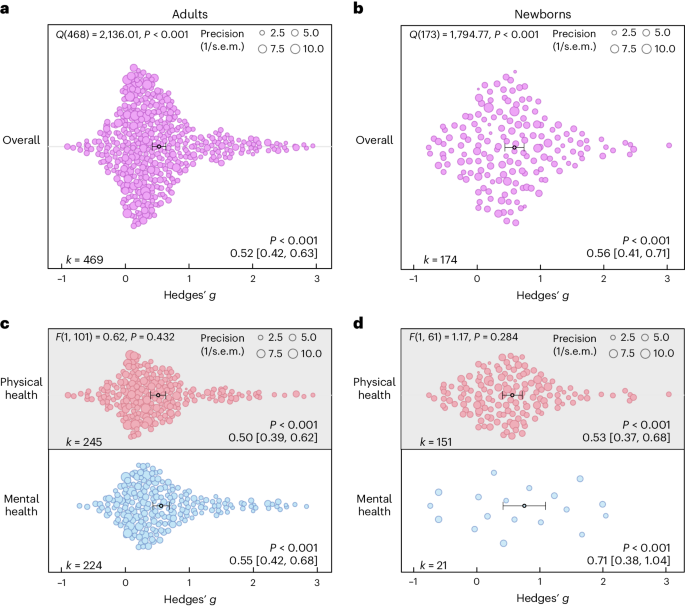
a , Orchard plot illustrating the overall benefits across all health outcomes for adults/children across 469 in part dependent effect sizes from 85 studies and 103 cohorts. b , The same as a but for newborns across 174 in part dependent effect sizes from 52 studies and 63 cohorts. c , The same as a but separating the results for physical versus mental health benefits across 469 in part dependent effect sizes from 85 studies and 103 cohorts. d , The same as b but separating the results for physical versus mental health benefits across 172 in part dependent effect sizes from 52 studies and 63 cohorts. Each dot reflects a measured effect, and the number of effects ( k ) included in the analysis is depicted in the bottom left. Mean effects and 95% CIs are presented in the bottom right and are indicated by the central black dot (mean effect) and its error bars (95% CI). The heterogeneity Q statistic is presented in the top left. Overall effects of moderator impact were assessed via an F test, and post hoc comparisons were done using t tests (two-sided test). Note that the P values above the mean effects indicate whether an effect differed significantly from a zero effect. P values were not corrected for multiple comparisons. The dot size reflects the precision of each individual effect (larger indicates higher precision). Small-study bias for the overall effect was significant ( F test, two-sided test) in the adult meta-analysis ( F (1, 101) = 21.24, P < 0.001; Supplementary Fig. 3 ) as well as in the newborn meta-analysis ( F (1, 61) = 5.25, P = 0.025; Supplementary Fig. 4 ).
Source data
On the basis of the overall effect of both meta-analyses as well as their median sample sizes, the minimum number of studies necessary for subgroup analyses to achieve 80% power was k = 9 effects for adults and k = 8 effects for newborns (Supplementary Figs. 5 and 6 ). Assessing specific health outcomes with sufficient power in more detail in adults (Fig. 3a ) revealed smaller benefits to sleep and heart rate parameters, moderate benefits to positive and negative affect, diastolic blood and systolic blood pressure, mobility and reductions of the stress hormone cortisol and larger benefits to trait and state anxiety, depression, fatigue and pain. Post hoc tests revealed stronger benefits for pain, state anxiety, depression and trait anxiety compared with respiratory, sleep and heart rate parameters (see Fig. 3 for all post hoc comparisons). Reductions in pain and state anxiety were increased compared with reductions in negative affect ( t (83) = 2.54, P = 0.013, Hedges’ g difference of 0.31, 95% CI 0.07 to 0.55; t (83) = 2.31, P = 0.024, Hedges’ g difference of 0.27, 95% CI 0.03 to 0.51). Benefits to pain symptoms were higher compared with benefits to positive affect ( t (83) = 2.22, P = 0.030, Hedges’ g difference of 0.29, 95% CI 0.04 to 0.54). Finally, touch resulted in larger benefits to cortisol release compared with heart rate parameters ( t (83) = 2.30, P = 0.024, Hedges’ g difference of 0.26, 95% CI 0.04–0.48).
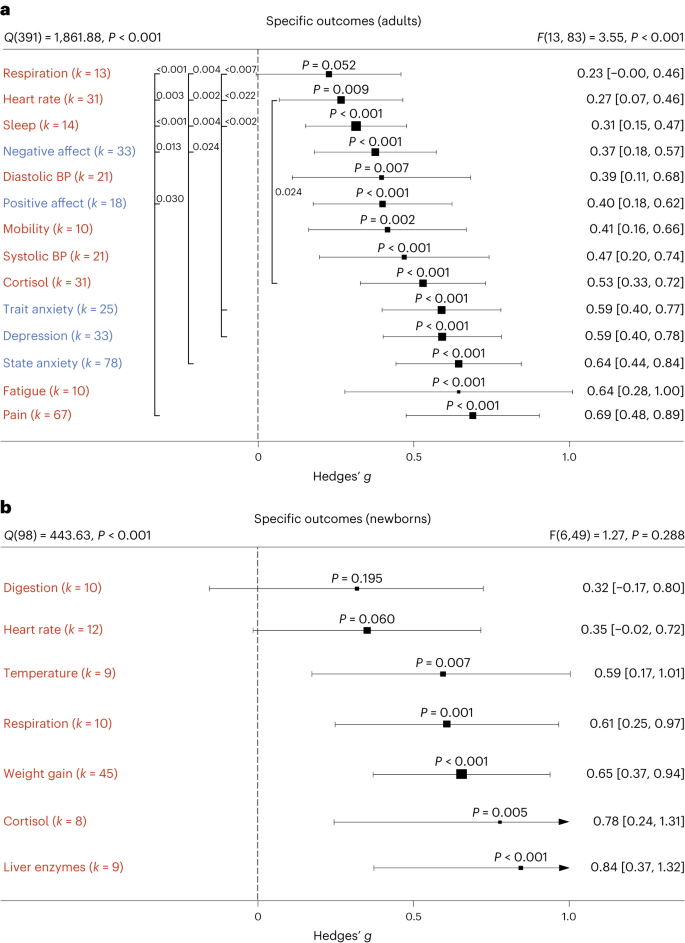
a , b , Health outcomes in adults analysed across 405 in part dependent effect sizes from 79 studies and 97 cohorts ( a ) and in newborns analysed across 105 in part dependent effect sizes from 46 studies and 56 cohorts ( b ). The type of health outcomes measured differed between adults and newborns and were thus analysed separately. Numbers on the right represent the mean effect with its 95% CI in square brackets and the significance level estimating the likelihood that the effect is equal to zero. Overall effects of moderator impact were assessed via an F test, and post hoc comparisons were done using t tests (two-sided test). The F value in the top right represents a test of the hypothesis that all effects within the subpanel are equal. The Q statistic represents the heterogeneity. P values of post hoc tests are depicted whenever significant. P values above the horizontal whiskers indicate whether an effect differed significantly from a zero effect. Vertical lines indicate significant post hoc tests between moderator levels. P values were not corrected for multiple comparisons. Physical outcomes are marked in red. Mental outcomes are marked in blue.
In newborns, only physical health effects offered sufficient data for further analysis. We found no benefits for digestion and heart rate parameters. All other health outcomes (cortisol, liver enzymes, respiration, temperature regulation and weight gain) showed medium to large effects (Fig. 3b ). We found no significant differences among any specific health outcomes.
Non-human touch and skin-to-skin contact
In some situations, a fellow human is not readily available to provide affective touch, raising the question of the efficacy of touch delivered by objects and robots 11 . Overall, we found humans engaging in touch with other humans or objects to have medium-sized health benefits in adults, without significant differences ( t (99) = 1.05, P = 0.295, Hedges’ g difference of 0.12, 95% CI −0.11 to 0.35; Fig. 4a ). However, differentiating physical versus mental health benefits revealed similar benefits for human and object touch on physical health outcomes, but larger benefits on mental outcomes when humans were touched by humans ( t (97) = 2.32, P = 0.022, Hedges’ g difference of 0.24, 95% CI 0.04 to 0.44; Fig. 4b ). It must be noted that touching with an object still showed a significant effect (see Supplementary Fig. 7 for the corresponding orchard plot).
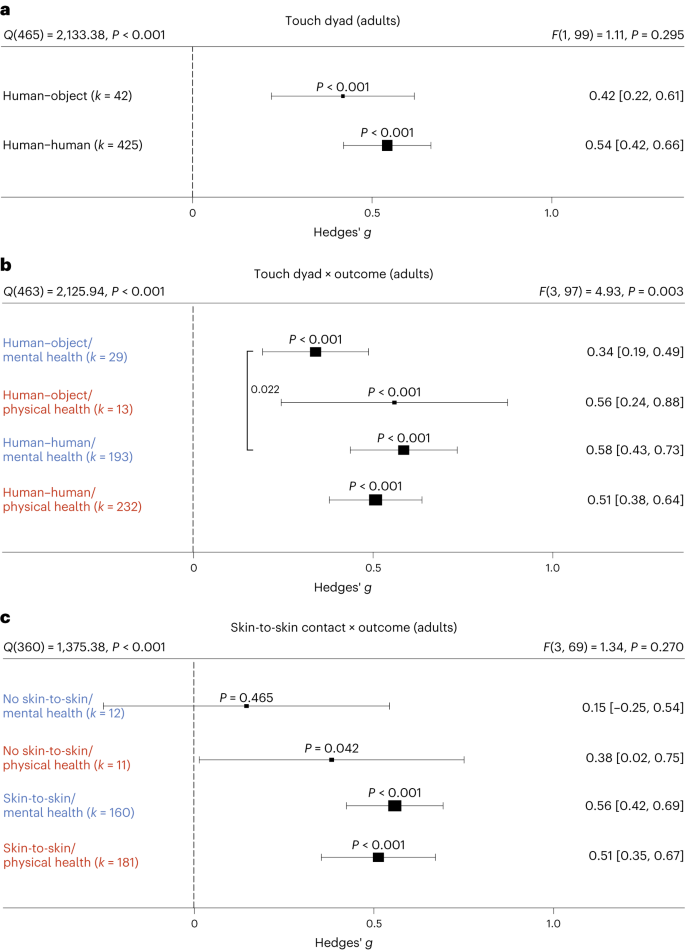
a , Forest plot comparing humans versus objects touching a human on health outcomes overall across 467 in part dependent effect sizes from 85 studies and 101 cohorts. b , The same as a but separately for mental versus physical health outcomes across 467 in part dependent effect sizes from 85 studies and 101 cohorts. c , Results with the removal of all object studies, leaving 406 in part dependent effect sizes from 71 studies and 88 cohorts to identify whether missing skin-to-skin contact is the relevant mediator of higher mental health effects in human–human interactions. Numbers on the right represent the mean effect with its 95% CI in square brackets and the significance level estimating the likelihood that the effect is equal to zero. Overall effects of moderator impact were assessed via an F test, and post hoc comparisons were done using t tests (two-sided test). The F value in the top right represents a test of the hypothesis that all effects within the subpanel are equal. The Q statistic represents the heterogeneity. P values of post hoc tests are depicted whenever significant. P values above the horizontal whiskers indicate whether an effect differed significantly from a zero effect. Vertical lines indicate significant post hoc tests between moderator levels. P values were not corrected for multiple comparisons. Physical outcomes are marked in red. Mental outcomes are marked in blue.
We considered the possibility that this effect was due to missing skin-to-skin contact in human–object interactions. Thus, we investigated human–human interactions with and without skin-to-skin contact (Fig. 4c ). In line with the hypothesis that skin-to-skin contact is highly relevant, we again found stronger mental health benefits in the presence of skin-to-skin contact that however did not achieve nominal significance ( t (69) = 1.95, P = 0.055, Hedges’ g difference of 0.41, 95% CI −0.00 to 0.82), possibly because skin-to-skin contact was rarely absent in human–human interactions, leading to a decrease in power of this analysis. Results for skin-to-skin contact as an overall moderator can be found in Supplementary Fig. 8 .
Influences of type of touch
The large majority of touch interventions comprised massage therapy in adults and kangaroo care in newborns (see Supplementary Table 1 for a complete list of interventions across studies). However, comparing the different types of touch explored across studies did not reveal significant differences in effect sizes based on touch type, be it on overall health benefits (adults: t (101) = 0.11, P = 0.916, Hedges’ g difference of 0.02, 95% CI −0.32 to 0.29; Fig. 5a ) or comparing different forms of touch separately for physical (massage therapy versus other forms: t (99) = 0.99, P = 0.325, Hedges’ g difference 0.16, 95% CI −0.15 to 0.47) or for mental health benefits (massage therapy versus other forms: t (99) = 0.75, P = 0.458, Hedges’ g difference of 0.13, 95% CI −0.22 to 0.48) in adults (Fig. 5c ; see Supplementary Fig. 9 for the corresponding orchard plot). A similar picture emerged for physical health effects in newborns (massage therapy versus kangaroo care: t (58) = 0.94, P = 0.353, Hedges’ g difference of 0.15, 95% CI −0.17 to 0.47; massage therapy versus other forms: t (58) = 0.56, P = 0.577, Hedges’ g difference of 0.13, 95% CI −0.34 to 0.60; kangaroo care versus other forms: t (58) = 0.07, P = 0.947, Hedges’ g difference of 0.02, 95% CI −0.46 to 0.50; Fig. 5d ; see also Supplementary Fig. 10 for the corresponding orchard plot). This suggests that touch types may be flexibly adapted to the setting of every touch intervention.
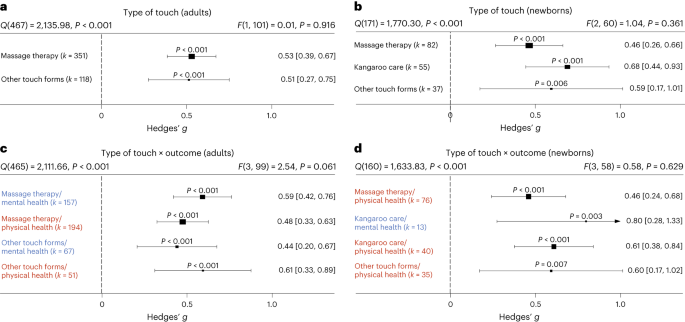
a , Forest plot of health benefits comparing massage therapy versus other forms of touch in adult cohorts across 469 in part dependent effect sizes from 85 studies and 103 cohorts. b , Forest plot of health benefits comparing massage therapy, kangaroo care and other forms of touch for newborns across 174 in part dependent effect sizes from 52 studies and 63 cohorts. c , The same as a but separating mental and physical health benefits across 469 in part dependent effect sizes from 85 studies and 103 cohorts. d , The same as b but separating mental and physical health outcomes where possible across 164 in part dependent effect sizes from 51 studies and 62 cohorts. Note that an insufficient number of studies assessed mental health benefits of massage therapy or other forms of touch to be included. Numbers on the right represent the mean effect with its 95% CI in square brackets and the significance level estimating the likelihood that the effect is equal to zero. Overall effects of moderator impact were assessed via an F test, and post hoc comparisons were done using t tests (two-sided test). The F value in the top right represents a test of the hypothesis that all effects within the subpanel are equal. The Q statistic represents heterogeneity. P values of post hoc tests are depicted whenever significant. P values above the horizontal whiskers indicate whether an effect differed significantly from a zero effect. Vertical lines indicate significant post hoc tests between moderator levels. P values were not corrected for multiple comparisons. Physical outcomes are marked in red. Mental outcomes are marked in blue.
The role of clinical status
Most research on touch interventions has focused on clinical samples, but are benefits restricted to clinical cohorts? We found health benefits to be significant in clinical and healthy populations (Fig. 6 ), whether all outcomes are considered (Fig. 6a,b ) or physical and mental health outcomes are separated (Fig. 6c,d , see Supplementary Figs. 11 and 12 for the corresponding orchard plots). In adults, however, we found higher mental health benefits for clinical populations compared with healthy ones (Fig. 6c ; t (99) = 2.11, P = 0.037, Hedges’ g difference of 0.25, 95% CI 0.01 to 0.49).
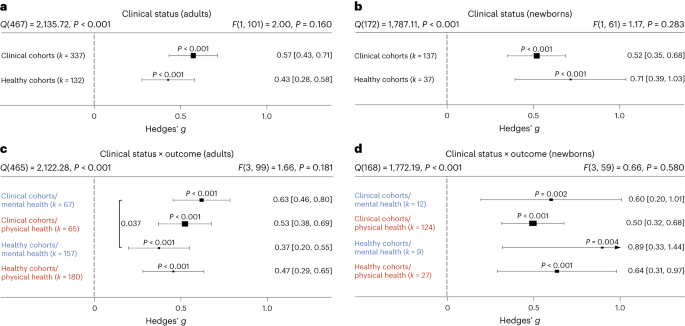
a , Health benefits for clinical cohorts of adults versus healthy cohorts of adults across 469 in part dependent effect sizes from 85 studies and 103 cohorts. b , The same as a but for newborn cohorts across 174 in part dependent effect sizes from 52 studies and 63 cohorts. c , The same as a but separating mental versus physical health benefits across 469 in part dependent effect sizes from 85 studies and 103 cohorts. d , The same as b but separating mental versus physical health benefits across 172 in part dependent effect sizes from 52 studies and 63 cohorts. Numbers on the right represent the mean effect with its 95% CI in square brackets and the significance level estimating the likelihood that the effect is equal to zero. Overall effects of moderator impact were assessed via an F test, and post hoc comparisons were done using t tests (two-sided test).The F value in the top right represents a test of the hypothesis that all effects within the subpanel are equal. The Q statistic represents the heterogeneity. P values of post hoc tests are depicted whenever significant. P values above the horizontal whiskers indicate whether an effect differed significantly from a zero effect. Vertical lines indicate significant post hoc tests between moderator levels. P values were not corrected for multiple comparisons. Physical outcomes are marked in red. Mental outcomes are marked in blue.
A more detailed analysis of specific clinical conditions in adults revealed positive mental and physical health benefits for almost all assessed clinical disorders. Differences between disorders were not found, with the exception of increased effectiveness of touch interventions in neurological disorders (Supplementary Fig. 13 ).
Familiarity in the touching dyad and intervention location
Touch interventions can be performed either by familiar touchers (partners, family members or friends) or by unfamiliar touchers (health care professionals). In adults, we did not find an impact of familiarity of the toucher ( t (99) = 0.12, P = 0.905, Hedges’ g difference of 0.02, 95% CI −0.27 to 0.24; Fig. 7a ; see Supplementary Fig. 14 for the corresponding orchard plot). Similarly, investigating the impact on mental and physical health benefits specifically, no significant differences could be detected, suggesting that familiarity is irrelevant in adults. In contrast, touch applied to newborns by their parents (almost all studies only included touch by the mother) was significantly more beneficial compared with unfamiliar touch ( t (60) = 2.09, P = 0.041, Hedges’ g difference of 0.30, 95% CI 0.01 to 0.59) (Fig. 7b ; see Supplementary Fig. 15 for the corresponding orchard plot). Investigating mental and physical health benefits specifically revealed no significant differences. Familiarity with the location in which the touch was applied (familiar being, for example, the participants’ home) did not influence the efficacy of touch interventions (Supplementary Fig. 16 ).
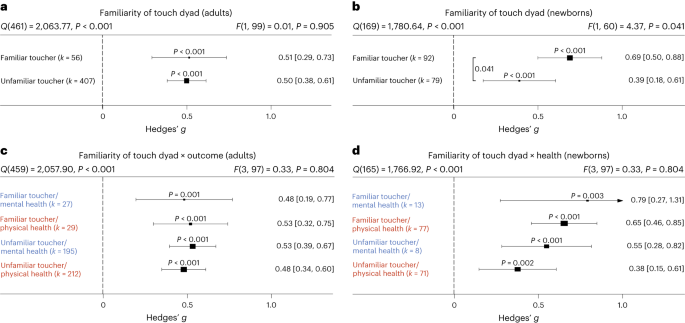
a , Health benefits for being touched by a familiar (for example, partner, family member or friend) versus unfamiliar toucher (health care professional) across 463 in part dependent effect sizes from 83 studies and 101 cohorts. b , The same as a but for newborn cohorts across 171 in part dependent effect sizes from 51 studies and 62 cohorts. c , The same as a but separating mental versus physical health benefits across 463 in part dependent effect sizes from 83 studies and 101 cohorts. d , The same as b but separating mental versus physical health benefits across 169 in part dependent effect sizes from 51 studies and 62 cohorts. Numbers on the right represent the mean effect with its 95% CI in square brackets and the significance level estimating the likelihood that the effect is equal to zero. Overall effects of moderator impact were assessed via an F test, and post hoc comparisons were done using t tests (two-sided test). The F value in the top right represents a test of the hypothesis that all effects within the subpanel are equal. The Q statistic represents the heterogeneity. P values of post hoc tests are depicted whenever significant. P values above the horizontal whiskers indicate whether an effect differed significantly from a zero effect. Vertical lines indicate significant post hoc tests between moderator levels. P values were not corrected for multiple comparisons. Physical outcomes are marked in red. Mental outcomes are marked in blue.
Frequency and duration of touch interventions
How often and for how long should touch be delivered? For adults, the median touch duration across studies was 20 min and the median number of touch interventions was four sessions with an average time interval of 2.3 days between each session. For newborns, the median touch duration across studies was 17.5 min and the median number of touch interventions was seven sessions with an average time interval of 1.3 days between each session.
Delivering more touch sessions increased benefits in adults, whether overall ( t (101) = 4.90, P < 0.001, Hedges’ g = 0.02, 95% CI 0.01 to 0.03), physical ( t (81) = 3.07, P = 0.003, Hedges’ g = 0.02, 95% CI 0.01–0.03) or mental benefits ( t (72) = 5.43, P < 0.001, Hedges’ g = 0.02, 95% CI 0.01–0.03) were measured (Fig. 8a ). A closer look at specific outcomes for which sufficient data were available revealed that positive associations between the number of sessions and outcomes were found for trait anxiety ( t (12) = 7.90, P < 0.001, Hedges’ g = 0.03, 95% CI 0.02–0.04), depression ( t (20) = 10.69, P < 0.001, Hedges’ g = 0.03, 95% CI 0.03–0.04) and pain ( t (37) = 3.65, P < 0.001, Hedges’ g = 0.03, 95% CI 0.02–0.05), indicating a need for repeated sessions to improve these adverse health outcomes. Neither increasing the number of sessions for newborns nor increasing the duration of touch per session in adults or newborns increased health benefits, be they physical or mental (Fig. 8b–d ). For continuous moderators in adults, we also looked at specific health outcomes as sufficient data were generally available for further analysis. Surprisingly, we found significant negative associations between touch duration and reductions of cortisol ( t (24) = 2.71, P = 0.012, Hedges’ g = −0.01, 95% CI −0.01 to −0.00) and heart rate parameters ( t (21) = 2.35, P = 0.029, Hedges’ g = −0.01, 95% CI −0.02 to −0.00).
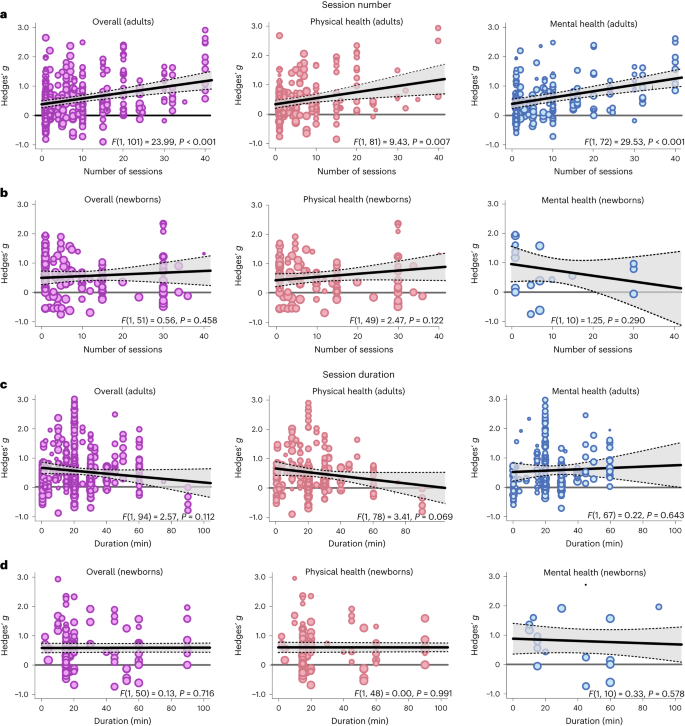
a , Meta-regression analysis examining the association between the number of sessions applied and the effect size in adults, either on overall health benefits (left, 469 in part dependent effect sizes from 85 studies and 103 cohorts) or for physical (middle, 245 in part dependent effect sizes from 69 studies and 83 cohorts) or mental benefits (right, 224 in part dependent effect sizes from 60 studies and 74 cohorts) separately. b , The same as a for newborns (overall: 150 in part dependent effect sizes from 46 studies and 53 cohorts; physical health: 127 in part dependent effect sizes from 44 studies and 51 cohorts; mental health: 21 in part dependent effect sizes from 11 studies and 12 cohorts). c , d the same as a ( c ) and b ( d ) but for the duration of the individual sessions. For adults, 449 in part dependent effect sizes across 80 studies and 96 cohorts were included in the overall analysis. The analysis of physical health benefits included 240 in part dependent effect sizes across 67 studies and 80 cohorts, and the analysis of mental health benefits included 209 in part dependent effect sizes from 56 studies and 69 cohorts. For newborns, 145 in part dependent effect sizes across 45 studies and 52 cohorts were included in the overall analysis. The analysis of physical health benefits included 122 in part dependent effect sizes across 43 studies and 50 cohorts, and the analysis of mental health benefits included 21 in part dependent effect sizes from 11 studies and 12 cohorts. Each dot represents an effect size. Its size indicates the precision of the study (larger indicates better). Overall effects of moderator impact were assessed via an F test (two-sided test). The P values in each panel represent the result of a regression analysis testing the hypothesis that the slope of the relationship is equal to zero. P values are not corrected for multiple testing. The shaded area around the regression line represents the 95% CI.
Demographic influences of sex and age
We used the ratio between women and men in the single-study samples as a proxy for sex-specific effects. Sex ratios were heavily skewed towards larger numbers of women in each cohort (median 83% women), and we could not find significant associations between sex ratio and overall ( t (62) = 0.08, P = 0.935, Hedges’ g = 0.00, 95% CI −0.00 to 0.01), mental ( t (43) = 0.55, P = 0.588, Hedges’ g = 0.00, 95% CI −0.00 to 0.01) or physical health benefits ( t (51) = 0.15, P = 0.882, Hedges’ g = −0.00, 95% CI −0.01 to 0.01). For specific outcomes that could be further analysed, we found a significant positive association of sex ratio with reductions in cortisol secretion ( t (18) = 2.31, P = 0.033, Hedges’ g = 0.01, 95% CI 0.00 to 0.01) suggesting stronger benefits in women. In contrast to adults, sex ratios were balanced in samples of newborns (median 53% girls). For newborns, there was no significant association with overall ( t (36) = 0.77, P = 0.447, Hedges’ g = −0.01, 95% CI −0.02 to 0.01) and physical health benefits of touch ( t (35) = 0.93, P = 0.359, Hedges’ g = −0.01, 95% CI −0.02 to 0.01). Mental health benefits did not provide sufficient data for further analysis.
The median age in the adult meta-analysis was 42.6 years (s.d. 21.16 years, range 4.5–88.4 years). There was no association between age and the overall ( t (73) = 0.35, P = 0.727, Hedges’ g = 0.00, 95% CI −0.01 to 0.01), mental ( t (53) = 0.94, P = 0.353, Hedges’ g = 0.01, 95% CI −0.01 to 0.02) and physical health benefits of touch ( t (60) = 0.16, P = 0.870, Hedges’ g = 0.00, 95% CI −0.01 to 0.01). Looking at specific health outcomes, we found significant positive associations between mean age and improved positive affect ( t (10) = 2.54, P = 0.030, Hedges’ g = 0.01, 95% CI 0.00 to 0.02) as well as systolic blood pressure ( t (11) = 2.39, P = 0.036, Hedges’ g = 0.02, 95% CI 0.00 to 0.04).
A list of touched body parts can be found in Supplementary Table 1 . For the touched body part, we found significantly higher health benefits for head touch compared with arm touch ( t (40) = 2.14, P = 0.039, Hedges’ g difference of 0.78, 95% CI 0.07 to 1.49) and torso touch ( t (40) = 2.23, P = 0.031; Hedges’ g difference of 0.84, 95% CI 0.10 to 1.58; Supplementary Fig. 17 ). Touching the arm resulted in lower mental health compared with physical health benefits ( t (37) = 2.29, P = 0.028, Hedges’ g difference of −0.35, 95% CI −0.65 to −0.05). Furthermore, we found a significantly increased physical health benefit when the head was touched as opposed to the torso ( t (37) = 2.10, P = 0.043, Hedges’ g difference of 0.96, 95% CI 0.06 to 1.86). Thus, head touch such as a face or scalp massage could be especially beneficial.
Directionality
In adults, we tested whether a uni- or bidirectional application of touch mattered. The large majority of touch was applied unidirectionally ( k = 442 of 469 effects). Unidirectional touch had higher health benefits ( t (101) = 2.17, P = 0.032, Hedges’ g difference of 0.30, 95% CI 0.03 to 0.58) than bidirectional touch. Specifically, mental health benefits were higher in unidirectional touch ( t (99) = 2.33, P = 0.022, Hedges’ g difference of 0.46, 95% CI 0.06 to 0.66).
Study location
For adults, we found significantly stronger health benefits of touch in South American compared with North American cohorts ( t (95) = 2.03, P = 0.046, Hedges’ g difference of 0.37, 95% CI 0.01 to 0.73) and European cohorts ( t (95) = 2.22, P = 0.029, Hedges’ g difference of 0.36, 95% CI 0.04 to 0.68). For newborns, we found weaker effects in North American cohorts compared to Asian ( t (55) = 2.28, P = 0.026, Hedges’ g difference of −0.37, 95% CI −0.69 to −0.05) and European cohorts ( t (55) = 2.36, P = 0.022, Hedges’ g difference of −0.40, 95% CI −0.74 to −0.06). Investigating the interaction with mental and physical health benefits did not reveal any effects of study location in both meta-analyses (Supplementary Fig. 18 ).
Systematic review of studies without effect sizes
All studies where effect size data could not be obtained or that did not meet the meta-analysis inclusion criteria can be found on the OSF project 12 in the file ‘Study_lists_final_revised.xlsx’ (sheet ‘Studies_without_effect_sizes’). Specific reasons for exclusion are furthermore documented in Supplementary Table 2 . For human health outcomes assessed across 56 studies and n = 2,438 individuals, interventions mostly comprised massage therapy ( k = 86 health outcomes) and kangaroo care ( k = 33 health outcomes). For datasets where no effect size could be computed, 90.0% of mental health and 84.3% of physical health parameters were positively impacted by touch. Positive impact of touch did not differ between types of touch interventions. These results match well with the observations of the meta-analysis of a highly positive benefit of touch overall, irrespective of whether a massage or any other intervention is applied.
We also assessed health outcomes in animals across 19 studies and n = 911 subjects. Most research was conducted in rodents. Animals that received touch were rats (ten studies, k = 16 health outcomes), mice (four studies, k = 7 health outcomes), macaques (two studies, k = 3 health outcomes), cats (one study, k = 3 health outcomes), lambs (one study, k = 2 health outcomes) and coral reef fish (one study, k = 1 health outcome). Touch interventions mostly comprised stroking ( k = 13 health outcomes) and tickling ( k = 10 health outcomes). For animal studies, 71.4% of effects showed benefits to mental health-like parameters and 81.8% showed positive physical health effects. We thus found strong evidence that touch interventions, which were mostly conducted by humans (16 studies with human touch versus 3 studies with object touch), had positive health effects in animal species as well.
The key aim of the present study was twofold: (1) to provide an estimate of the effect size of touch interventions and (2) to disambiguate moderating factors to potentially tailor future interventions more precisely. Overall, touch interventions were beneficial for both physical and mental health, with a medium effect size. Our work illustrates that touch interventions are best suited for reducing pain, depression and anxiety in adults and children as well as for increasing weight gain in newborns. These findings are in line with previous meta-analyses on this topic, supporting their conclusions and their robustness to the addition of more datasets. One limitation of previous meta-analyses is that they focused on specific health outcomes or populations, despite primary studies often reporting effects on multiple health parameters simultaneously (for example, ref. 13 focusing on neck and shoulder pain and ref. 14 focusing on massage therapy in preterms). To our knowledge, only ref. 5 provides a multivariate picture for a large number of dependent variables. However, this study analysed their data in separate random effects models that did not account for multivariate reporting nor for the multilevel structure of the data, as such approaches have only become available recently. Thus, in addition to adding a substantial amount of new data, our statistical approach provides a more accurate depiction of effect size estimates. Additionally, our study investigated a variety of moderating effects that did not reach significance (for example, sex ratio, mean age or intervention duration) or were not considered (for example, the benefits of robot or object touch) in previous meta-analyses in relation to touch intervention efficacy 5 , probably because of the small number of studies with information on these moderators in the past. Owing to our large-scale approach, we reached high statistical power for many moderator analyses. Finally, previous meta-analyses on this topic exclusively focused on massage therapy in adults or kangaroo care in newborns 15 , leaving out a large number of interventions that are being carried out in research as well as in everyday life to improve well-being. Incorporating these studies into our study, we found that, in general, both massages and other types of touch, such as gentle touch, stroking or kangaroo care, showed similar health benefits.
While it seems to be less critical which touch intervention is applied, the frequency of interventions seems to matter. More sessions were positively associated with the improvement of trait outcomes such as depression and anxiety but also pain reductions in adults. In contrast to session number, increasing the duration of individual sessions did not improve health effects. In fact, we found some indications of negative relationships in adults for cortisol and blood pressure. This could be due to habituating effects of touch on the sympathetic nervous system and hypothalamic–pituitary–adrenal axis, ultimately resulting in diminished effects with longer exposure, or decreased pleasantness ratings of affective touch with increasing duration 16 . For newborns, we could not support previous notions that the duration of the touch intervention is linked to benefits in weight gain 17 . Thus, an ideal intervention protocol does not seem to have to be excessively long. It should be noted that very few interventions lasted less than 5 min, and it therefore remains unclear whether very short interventions have the same effect.
A critical issue highlighted in the pandemic was the lack of touch due to social restrictions 18 . To accommodate the need for touch in individuals with small social networks (for example, institutionalized or isolated individuals), touch interventions using objects/robots have been explored in the past (for a review, see ref. 11 ). We show here that touch interactions outside of the human–human domain are beneficial for mental and physical health outcomes. Importantly, object/robot touch was not as effective in improving mental health as human-applied touch. A sub-analysis of missing skin-to-skin contact among humans indicated that mental health effects of touch might be mediated by the presence of skin-to-skin contact. Thus, it seems profitable to include skin-to-skin contact in future touch interventions, in line with previous findings in newborns 19 . In robots, recent advancements in synthetic skin 20 should be investigated further in this regard. It should be noted that, although we did not observe significant differences in physical health benefits between human–human and human–object touch, the variability of effect sizes was higher in human–object touch. The conditions enabling object or robot interactions to improve well-being should therefore be explored in more detail in the future.
Touch was beneficial for both healthy and clinical cohorts. These data are critical as most previous meta-analytic research has focused on individuals diagnosed with clinical disorders (for example, ref. 6 ). For mental health outcomes, we found larger effects in clinical cohorts. A possible reason could relate to increased touch wanting 21 in patients. For example, loneliness often co-occurs with chronic illnesses 22 , which are linked to depressed mood and feelings of anxiety 23 . Touch can be used to counteract this negative development 24 , 25 . In adults and children, knowing the toucher did not influence health benefits. In contrast, familiarity affected overall health benefits in newborns, with parental touch being more beneficial than touch applied by medical staff. Previous studies have suggested that early skin-to-skin contact and exposure to maternal odour is critical for a newborn’s ability to adapt to a new environment 26 , supporting the notion that parental care is difficult to substitute in this time period.
With respect to age-related effects, our data further suggest that increasing age was associated with a higher benefit through touch for systolic blood pressure. These findings could potentially be attributed to higher basal blood pressure 27 with increasing age, allowing for a stronger modulation of this parameter. For sex differences, our study provides some evidence that there are differences between women and men with respect to health benefits of touch. Overall, research on sex differences in touch processing is relatively sparse (but see refs. 28 , 29 ). Our results suggest that buffering effects against physiological stress are stronger in women. This is in line with increased buffering effects of hugs in women compared with men 30 . The female-biased primary research in adults, however, begs for more research in men or non-binary individuals. Unfortunately, our study could not dive deeper into this topic as health benefits broken down by sex or gender were almost never provided. Recent research has demonstrated that sensory pleasantness is affected by sex and that this also interacts with the familiarity of the other person in the touching dyad 29 , 31 . In general, contextual factors such as sex and gender or the relationship of the touching dyad, differences in cultural background or internal states such as stress have been demonstrated to be highly influential in the perception of affective touch and are thus relevant to maximizing the pleasantness and ultimately the health benefits of touch interactions 32 , 33 , 34 . As a positive personal relationship within the touching dyad is paramount to induce positive health effects, future research applying robot touch to promote well-being should therefore not only explore synthetic skin options but also focus on improving robots as social agents that form a close relationship with the person receiving the touch 35 .
As part of the systematic review, we also assessed the effects of touch interventions in non-human animals. Mimicking the results of the meta-analysis in humans, beneficial effects of touch in animals were comparably strong for mental health-like and physical health outcomes. This may inform interventions to promote animal welfare in the context of animal experiments 36 , farming 37 and pets 38 . While most studies investigated effects in rodents, which are mostly used as laboratory animals, these results probably transfer to livestock and common pets as well. Indeed, touch was beneficial in lambs, fish and cats 39 , 40 , 41 . The positive impact of human touch in rodents also allows for future mechanistic studies in animal models to investigate how interventions such as tickling or stroking modulate hormonal and neuronal responses to touch in the brain. Furthermore, the commonly proposed oxytocin hypothesis can be causally investigated in these animal models through, for example, optogenetic or chemogenetic techniques 42 . We believe that such translational approaches will further help in optimizing future interventions in humans by uncovering the underlying mechanisms and brain circuits involved in touch.
Our results offer many promising avenues to improve future touch interventions, but they also need to be discussed in light of their limitations. While the majority of findings showed robust health benefits of touch interventions across moderators when compared with a null effect, post hoc tests of, for example, familiarity effects in newborns or mental health benefit differences between human and object touch only barely reached significance. Since we computed a large number of statistical tests in the present study, there is a risk that these results are false positives. We hope that researchers in this field are stimulated by these intriguing results and target these questions by primary research through controlled experimental designs within a well-powered study. Furthermore, the presence of small-study bias in both meta-analyses is indicative that the effect size estimates presented here might be overestimated as null results are often unpublished. We want to stress however that this bias is probably reduced by the multivariate reporting of primary studies. Most studies that reported on multiple health outcomes only showed significant findings for one or two among many. Thus, the multivariate nature of primary research in this field allowed us to include many non-significant findings in the present study. Another limitation pertains to the fact that we only included articles in languages mostly spoken in Western countries. As a large body of evidence comes from Asian countries, it could be that primary research was published in languages other than specified in the inclusion criteria. Thus, despite the large and inclusive nature of our study, some studies could have been missed regardless. Another factor that could not be accounted for in our meta-analysis was that an important prerequisite for touch to be beneficial is its perceived pleasantness. The level of pleasantness associated with being touched is modulated by several parameters 34 including cultural acceptability 43 , perceived humanness 44 or a need for touch 45 , which could explain the observed differences for certain moderators, such as human–human versus robot–human interaction. Moreover, the fact that secondary categorical moderators could not be investigated with respect to specific health outcomes, owing to the lack of data points, limits the specificity of our conclusions in this regard. It thus remains unclear whether, for example, a decreased mental health benefit in the absence of skin-to-skin contact is linked mostly to decreased anxiolytic effects, changes in positive/negative affect or something else. Since these health outcomes are however highly correlated 46 , it is likely that such effects are driven by multiple health outcomes. Similarly, it is important to note that our conclusions mainly refer to outcomes measured close to the touch intervention as we did not include long-term outcomes. Finally, it needs to be noted that blinding towards the experimental condition is essentially impossible in touch interventions. Although we compared the touch intervention with other interventions, such as relaxation therapy, as control whenever possible, contributions of placebo effects cannot be ruled out.
In conclusion, we show clear evidence that touch interventions are beneficial across a large number of both physical and mental health outcomes, for both healthy and clinical cohorts, and for all ages. These benefits, while influenced in their magnitude by study cohorts and intervention characteristics, were robustly present, promoting the conclusion that touch interventions can be systematically employed across the population to preserve and improve our health.
Open science practices
All data and code are accessible in the corresponding OSF project 12 . The systematic review was registered on PROSPERO (CRD42022304281) before the start of data collection. We deviated from the pre-registered plan as follows:
Deviation 1: During our initial screening for the systematic review, we were confronted with a large number of potential health outcomes to look at. This observation of multivariate outcomes led us to register an amendment during data collection (but before any effect size or moderator screening). In doing so, we aimed to additionally extract meta-analytic effects for a more quantitative assessment of our review question that can account for multivariate data reporting and dependencies of effects within the same study. Furthermore, as we noted a severe lack of studies with respect to health outcomes for animals during the inclusion assessment for the systematic review, we decided that the meta-analysis would only focus on outcomes that could be meaningfully analysed on the meta-analytic level and therefore only included health outcomes of human participants.
Deviation 2: In the pre-registration, we did not explicitly exclude non-randomized trials. Since an explicit use of non-randomization for group allocation significantly increases the risk of bias, we decided to exclude them a posteriori from data analysis.
Deviation 3: In the pre-registration, we outlined a tertiary moderator level, namely benefits of touch application versus touch reception. This level was ignored since no included study specifically investigated the benefits of touch application by itself.
Deviation 4: In the pre-registration, we suggested using the RoBMA function 47 to provide a Bayesian framework that allows for a more accurate assessment of publication bias beyond small-study bias. Unfortunately, neither multilevel nor multivariate data structures are supported by the RoBMA function, to our knowledge. For this reason, we did not further pursue this analysis, as the hierarchical nature of the data would not be accounted for.
Deviation 5: Beyond the pre-registered inclusion and exclusion criteria, we also excluded dissertations owing to their lack of peer review.
Deviation 6: In the pre-registration, we stated to investigate the impact of sex of the person applying the touch. This moderator was not further analysed, as this information was rarely given and the individuals applying the touch were almost exclusively women (7 males, 24 mixed and 85 females in studies on adults/children; 3 males, 17 mixed and 80 females in studied on newborns).
Deviation 7: The time span of the touch intervention as assessed by subtracting the final day of the intervention from the first day was not investigated further owing to its very high correlation with the number of sessions ( r (461) = 0.81 in the adult meta-analysis, r (145) = 0.84 in the newborn meta-analysis).
Inclusion and exclusion criteria
To be included in the systematic review, studies had to investigate the relationship between at least one health outcome (physical and/or mental) in humans or animals and a touch intervention, include explicit physical touch by another human, animal or object as part of an intervention and include an experimental and control condition/group that are differentiated by touch alone. Of note, as a result of this selection process, no animal-to-animal touch intervention study was included, as they never featured a proper no-touch control. Human touch was always explicit touch by a human (that is, no brushes or other tools), either with or without skin-to-skin contact. Regarding the included health outcomes, we aimed to be as broad as possible but excluded parameters such as neurophysiological responses or pleasantness ratings after touch application as they do not reflect health outcomes. All included studies in the meta-analysis and systematic review 48 , 49 , 50 , 51 , 52 , 53 , 54 , 55 , 56 , 57 , 58 , 59 , 60 , 61 , 62 , 63 , 64 , 65 , 66 , 67 , 68 , 69 , 70 , 71 , 72 , 73 , 74 , 75 , 76 , 77 , 78 , 79 , 80 , 81 , 82 , 83 , 84 , 85 , 86 , 87 , 88 , 89 , 90 , 91 , 92 , 93 , 94 , 95 , 96 , 97 , 98 , 99 , 100 , 101 , 102 , 103 , 104 , 105 , 106 , 107 , 108 , 109 , 110 , 111 , 112 , 113 , 114 , 115 , 116 , 117 , 118 , 119 , 120 , 121 , 122 , 123 , 124 , 125 , 126 , 127 , 128 , 129 , 130 , 131 , 132 , 133 , 134 , 135 , 136 , 137 , 138 , 139 , 140 , 141 , 142 , 143 , 144 , 145 , 146 , 147 , 148 , 149 , 150 , 151 , 152 , 153 , 154 , 155 , 156 , 157 , 158 , 159 , 160 , 161 , 162 , 163 , 164 , 165 , 166 , 167 , 168 , 169 , 170 , 171 , 172 , 173 , 174 , 175 , 176 , 177 , 178 , 179 , 180 , 181 , 182 , 183 , 184 , 185 , 186 , 187 , 188 , 189 , 190 , 191 , 192 , 193 , 194 , 195 , 196 , 197 , 198 , 199 , 200 , 201 , 202 , 203 , 204 , 205 , 206 , 207 , 208 , 209 , 210 , 211 , 212 , 213 , 214 , 215 , 216 , 217 , 218 , 219 , 220 , 221 , 222 , 223 , 224 , 225 , 226 , 227 , 228 , 229 , 230 , 231 , 232 , 233 , 234 , 235 , 236 , 237 , 238 , 239 , 240 , 241 , 242 , 243 , 244 , 245 , 246 , 247 , 248 , 249 , 250 , 251 , 252 , 253 , 254 , 255 , 256 , 257 , 258 , 259 , 260 , 261 , 262 , 263 are listed in Supplementary Table 2 . All excluded studies are listed in Supplementary Table 3 , together with a reason for exclusion. We then applied a two-step process: First, we identified all potential health outcomes and extracted qualitative information on those outcomes (for example, direction of effect). Second, we extracted quantitative information from all possible outcomes (for example, effect sizes). The meta-analysis additionally required a between-subjects design (to clearly distinguish touch from no-touch effects and owing to missing information about the correlation between repeated measurements 264 ). Studies that explicitly did not apply a randomized protocol were excluded before further analysis to reduce risk of bias. The full study lists for excluded and included studies can be found in the OSF project 12 in the file ‘Study_lists_final_revised.xlsx’. In terms of the time frame, we conducted an open-start search of studies until 2022 and identified studies conducted between 1965 and 2022.
Data collection
We used Google Scholar, PubMed and Web of Science for our literature search, with no limitations regarding the publication date and using pre-specified search queries (see Supplementary Information for the exact keywords used). All procedures were in accordance with the updated Preferred Reporting Items for Systematic Reviews and Meta-Analyses guidelines 265 . Articles were assessed in French, Dutch, German or English. The above databases were searched from 2 December 2021 until 1 October 2022. Two independent coders evaluated each paper against the inclusion and exclusion criteria. Inconsistencies between coders were checked and resolved by J.P. and H.H. Studies excluded/included for the review and meta-analysis can be found on the OSF project.
Search queries
We used the following keywords to search the chosen databases. Agents (human versus animal versus object versus robot) and touch outcome (physical versus mental) were searched separately together with keywords searching for touch.
TOUCH: Touch OR Social OR Affective OR Contact OR Tactile interaction OR Hug OR Massage OR Embrace OR Kiss OR Cradling OR Stroking OR Haptic interaction OR tickling
AGENT: Object OR Robot OR human OR animal OR rodent OR primate
MENTAL OUTCOME: Health OR mood OR Depression OR Loneliness OR happiness OR life satisfaction OR Mental Disorder OR well-being OR welfare OR dementia OR psychological OR psychiatric OR anxiety OR Distress
PHYSICAL OUTCOME: Health OR Stress OR Pain OR cardiovascular health OR infection risk OR immune response OR blood pressure OR heart rate
Data extraction and preparation
Data extraction began on 10 October 2022 and was concluded on 25 February 2023. J.P. and H.H. oversaw the data collection process, and checked and resolved all inconsistencies between coders.
Health benefits of touch were always coded by positive summary effects, whereas adverse health effects of touch were represented by negative summary effects. If multiple time points were measured for the same outcome on the same day after a single touch intervention, we extracted the peak effect size (in either the positive or negative direction). If the touch intervention occurred multiple times and health outcomes were assessed for each time point, we extracted data points separately. However, we only extracted immediate effects, as long-term effects not controlled through the experimental conditions could be due to influences other than the initial touch intervention. Measurements assessing long-term effects without explicit touch sessions in the breaks were excluded for the same reason. Common control groups for touch interventions comprised active (for example, relaxation therapy) as well as passive control groups (for example, standard medical care). In the case of multiple control groups, we always contrasted the touch group to the group that most closely matched the touch condition (for example, relaxation therapy was preferred over standard medical care). We extracted information from all moderators listed in the pre-registration (Supplementary Table 4 ). A list of included and excluded health outcomes is presented in Supplementary Table 5 . Authors of studies with possible effects but missing information to calculate those effects were contacted via email and asked to provide the missing data (response rate 35.7%).
After finalizing the list of included studies for the systematic review, we added columns for moderators and the coding schema for our meta-analysis per our updated registration. Then, each study was assessed for its eligibility in the meta-analysis by two independent coders (J.P., H.H., K.F. or F.M.). To this end, all coders followed an a priori specified procedure: First, the PDF was skimmed for possible effects to extract, and the study was excluded if no PDF was available or the study was in a language different from the ones specified in ‘ Data collection ’. Effects from studies that met the inclusion criteria were extracted from all studies listing descriptive values or statistical parameters to calculate effect sizes. A website 266 was used to convert descriptive and statistical values available in the included studies (means and standard deviations/standard errors/confidence intervals, sample sizes, F values, t values, t test P values or frequencies) into Cohen’s d , which were then converted in Hedges’ g . If only P value thresholds were reported (for example, P < 0.01), we used this, most conservative, value as the P value to calculate the effect size (for example, P = 0.01). If only the total sample size was given but that number was even and the participants were randomly assigned to each group, we assumed equal sample sizes for each group. If delta change scores (for example, pre- to post-touch intervention) were reported, we used those over post-touch only scores. In case frequencies were 0 when frequency tables were used to determine effect sizes, we used a value of 0.5 as a substitute to calculate the effect (the default setting in the ‘metafor’ function 267 ). From these data, Hedges’ g and its variance could be derived. Effect sizes were always computed between the experimental and the control group.
Statistical analysis and risk of bias assessment
Owing to the lack of identified studies, health benefits to animals were not included as part of the statistical analysis. One meta-analysis was performed for adults, adolescents and children, as outcomes were highly comparable. We refer to this meta-analysis as the adult meta-analysis, as children/adolescent cohorts were only targeted in a minority of studies. A separate meta-analysis was performed for newborns, as their health outcomes differed substantially from any other age group.
Data were analysed using R (version 4.2.2) with the ‘rma.mv’ function from the ‘metafor’ package 267 in a multistep, multivariate and multilevel fashion.
We calculated an overall effect of touch interventions across all studies, cohorts and health outcomes. To account for the hierarchical structure of the data, we used a multilevel structure with random effects at the study, cohort and effects level. Furthermore, we calculated the variance–covariance matrix of all data points to account for the dependencies of measured effects within each individual cohort and study. The variance–covariance matrix was calculated by default with an assumed correlation of effect sizes within each cohort of ρ = 0.6. As ρ needed to be assumed, sensitivity analyses for all computed effect estimates were conducted using correlations between effects of 0, 0.2, 0.4 and 0.8. The results of these sensitivity analyses can be found in ref. 12 . No conclusion drawn in the present manuscript was altered by changing the level of ρ . The sensitivity analyses, however, showed that higher assumed correlations lead to more conservative effect size estimates (see Supplementary Figs. 19 and 20 for the adult and newborn meta-analyses, respectively), reducing the type I error risk in general 268 . In addition to these procedures, we used robust variance estimation with cluster-robust inference at the cohort level. This step is recommended to more accurately determine the confidence intervals in complex multivariate models 269 . The data distribution was assumed to be normal, but this was not formally tested.
To determine whether individual effects had a strong influence on our results, we calculated Cook’s distance D . Here, a threshold of D > 0.5 was used to qualify a study as influential 270 . Heterogeneity in the present study was assessed using Cochran’s Q , which determines whether the extracted effect sizes estimate a common population effect size. Although the Q statistic in the ‘rma.mv’ function accounts for the hierarchical nature of the data, we also quantified the heterogeneity estimator σ ² for each random-effects level to provide a comprehensive overview of heterogeneity indicators. These indicators for all models can be found on the OSF project 12 in the Table ‘Model estimates’. To assess small study bias, we visually inspected the funnel plot and used the standard error as a moderator in the overarching meta-analyses.
Before any sub-group analysis, the overall effect size was used as input for power calculations. While such post hoc power calculations might be limited, we believe that a minimum number of effects to be included in subgroup analyses was necessary to allow for meaningful conclusions. Such medium effect sizes would also probably be the minimum effect sizes of interest for researchers as well as clinical practitioners. Power calculation for random-effects models further requires a sample size for each individual effect as well as an approximation of the expected heterogeneity between studies. For the sample size input, we used the median sample size in each of our studies. For heterogeneity, we assumed a value between medium and high levels of heterogeneity ( I ² = 62.5% 271 ), as moderator analyses typically aim at reducing heterogeneity overall. Subgroups were only further investigated if the number of observed effects achieved ~80% power under these circumstances, to allow for a more robust interpretation of the observed effects (see Supplementary Figs. 5 and 6 for the adult and newborn meta-analysis, respectively). In a next step, we investigated all pre-registered moderators for which sufficient power was detected. We first looked at our primary moderators (mental versus physical health) and how the effect sizes systematically varied as a function of our secondary moderators (for example, human–human or human–object touch, duration, skin-to-skin presence, etc.). We always included random slopes to allow for our moderators to vary with the random effects at our clustering variable, which is recommended in multilevel models to reduce false positives 272 . All statistical tests were performed two-sided. Significance of moderators was determined using omnibus F tests. Effect size differences between moderator levels and their confidence intervals were assessed via t tests.
Post hoc t tests were performed comparing mental and physical health benefits within each interacting moderator (for example, mental versus physical health benefits in cancer patients) and mental or physical health benefits across levels of the interacting moderator (for example, mental health benefits in cancer versus pain patients). The post hoc tests were not pre-registered. Data were visualized using forest plots and orchard plots 273 for categorical moderators and scatter plots for continuous moderators.
For a broad overview of prior work and their biases, risk of bias was assessed for all studies included in both meta-analyses and the systematic review. We assessed the risk of bias for the following parameters:
Bias from randomization, including whether a randomization procedure was performed, whether it was a between- or within-participant design and whether there were any baseline differences for demographic or dependent variables.
Sequence bias resulting from a lack of counterbalancing in within-subject designs.
Performance bias resulting from the participants or experiments not being blinded to the experimental conditions.
Attrition bias resulting from different dropout rates between experimental groups.
Note that four studies in the adult meta-analysis did not explicitly mention randomization as part of their protocol. However, since these studies never showed any baseline differences in all relevant variables (see ‘Risk of Bias’ table on the OSF project ) , we assumed that randomization was performed but not mentioned. Sequence bias was of no concern for studies for the meta-analysis since cross-over designs were excluded. It was, however, assessed for studies within the scope of the systematic review. Importantly, performance bias was always high in the adult/children meta-analysis, as blinding of the participants and experimenters to the experimental conditions was not possible owing to the nature of the intervention (touch versus no touch). For studies with newborns and animals, we assessed the performance bias as medium since neither newborns or animals are likely to be aware of being part of an experiment or specific group. An overview of the results is presented in Supplementary Fig. 21 , and the precise assessment for each study can be found on the OSF project 12 in the ‘Risk of Bias’ table.
Reporting summary
Further information on research design is available in the Nature Portfolio Reporting Summary linked to this article.
Data availability
All data are available via Open Science Framework at https://doi.org/10.17605/OSF.IO/C8RVW (ref. 12 ). Source data are provided with this paper.
Code availability
All code is available via Open Science Framework at https://doi.org/10.17605/OSF.IO/C8RVW (ref. 12 ).
Fulkerson, M. The First Sense: a Philosophical Study of Human Touch (MIT Press, 2013).
Farroni, T., Della Longa, L. & Valori, I. The self-regulatory affective touch: a speculative framework for the development of executive functioning. Curr. Opin. Behav. Sci. 43 , 167–173 (2022).
Article Google Scholar
Ocklenburg, S. et al. Hugs and kisses—the role of motor preferences and emotional lateralization for hemispheric asymmetries in human social touch. Neurosci. Biobehav. Rev. 95 , 353–360 (2018).
Ardiel, E. L. & Rankin, C. H. The importance of touch in development. Paediatr. Child Health 15 , 153–156 (2010).
Article PubMed PubMed Central Google Scholar
Moyer, C. A., Rounds, J. & Hannum, J. W. A meta-analysis of massage therapy research. Psychol. Bull. 130 , 3–18 (2004).
Article PubMed Google Scholar
Lee, S. H., Kim, J. Y., Yeo, S., Kim, S. H. & Lim, S. Meta-analysis of massage therapy on cancer pain. Integr. Cancer Ther. 14 , 297–304 (2015).
LaFollette, M. R., O’Haire, M. E., Cloutier, S. & Gaskill, B. N. A happier rat pack: the impacts of tickling pet store rats on human–animal interactions and rat welfare. Appl. Anim. Behav. Sci. 203 , 92–102 (2018).
Packheiser, J., Michon, F. Eva, C., Fredriksen, K. & Hartmann H. The physical and mental health benefits of social touch: a comparative systematic review and meta-analysis. PROSPERO https://www.crd.york.ac.uk/prospero/display_record.php?ID=CRD42022304281 (2023).
Lakens, D. Sample size justification. Collabra. Psychol. 8 , 33267 (2022).
Quintana, D. S. A guide for calculating study-level statistical power for meta-analyses. Adv. Meth. Pract. Psychol. Sci. https://doi.org/10.1177/25152459221147260 (2023).
Eckstein, M., Mamaev, I., Ditzen, B. & Sailer, U. Calming effects of touch in human, animal, and robotic interaction—scientific state-of-the-art and technical advances. Front. Psychiatry 11 , 555058 (2020).
Packheiser, J. et al. The physical and mental health benefits of affective touch: a comparative systematic review and multivariate meta-analysis. Open Science Framework https://doi.org/10.17605/OSF.IO/C8RVW (2023).
Kong, L. J. et al. Massage therapy for neck and shoulder pain: a systematic review and meta-analysis. Evid. Based Complement. Altern. Med. 2013 , 613279 (2013).
Wang, L., He, J. L. & Zhang, X. H. The efficacy of massage on preterm infants: a meta-analysis. Am. J. Perinatol. 30 , 731–738 (2013).
Field, T. Massage therapy research review. Complement. Ther. Clin. Pract. 24 , 19–31 (2016).
Bendas, J., Ree, A., Pabel, L., Sailer, U. & Croy, I. Dynamics of affective habituation to touch differ on the group and individual level. Neuroscience 464 , 44–52 (2021).
Article CAS PubMed Google Scholar
Charpak, N., Montealegre‐Pomar, A. & Bohorquez, A. Systematic review and meta‐analysis suggest that the duration of Kangaroo mother care has a direct impact on neonatal growth. Acta Paediatr. 110 , 45–59 (2021).
Packheiser, J. et al. A comparison of hugging frequency and its association with momentary mood before and during COVID-19 using ecological momentary assessment. Health Commun. https://doi.org/10.1080/10410236.2023.2198058 (2023).
Whitelaw, A., Heisterkamp, G., Sleath, K., Acolet, D. & Richards, M. Skin to skin contact for very low birthweight infants and their mothers. Arch. Dis. Child. 63 , 1377–1381 (1988).
Article CAS PubMed PubMed Central Google Scholar
Yogeswaran, N. et al. New materials and advances in making electronic skin for interactive robots. Adv. Robot. 29 , 1359–1373 (2015).
Durkin, J., Jackson, D. & Usher, K. Touch in times of COVID‐19: touch hunger hurts. J. Clin. Nurs. https://doi.org/10.1111/jocn.15488 (2021).
Rokach, A., Lechcier-Kimel, R. & Safarov, A. Loneliness of people with physical disabilities. Soc. Behav. Personal. Int. J. 34 , 681–700 (2006).
Palgi, Y. et al. The loneliness pandemic: loneliness and other concomitants of depression, anxiety and their comorbidity during the COVID-19 outbreak. J. Affect. Disord. 275 , 109–111 (2020).
Heatley-Tejada, A., Dunbar, R. I. M. & Montero, M. Physical contact and loneliness: being touched reduces perceptions of loneliness. Adapt. Hum. Behav. Physiol. 6 , 292–306 (2020).
Article CAS Google Scholar
Packheiser, J. et al. The association of embracing with daily mood and general life satisfaction: an ecological momentary assessment study. J. Nonverbal Behav. 46 , 519–536 (2022).
Porter, R. The biological significance of skin-to-skin contact and maternal odours. Acta Paediatr. 93 , 1560–1562 (2007).
Hawkley, L. C., Masi, C. M., Berry, J. D. & Cacioppo, J. T. Loneliness is a unique predictor of age-related differences in systolic blood pressure. Psychol. Aging 21 , 152–164 (2006).
Russo, V., Ottaviani, C. & Spitoni, G. F. Affective touch: a meta-analysis on sex differences. Neurosci. Biobehav. Rev. 108 , 445–452 (2020).
Schirmer, A. et al. Understanding sex differences in affective touch: sensory pleasantness, social comfort, and precursive experiences. Physiol. Behav. 250 , 113797 (2022).
Berretz, G. et al. Romantic partner embraces reduce cortisol release after acute stress induction in women but not in men. PLoS ONE 17 , e0266887 (2022).
Gazzola, V. et al. Primary somatosensory cortex discriminates affective significance in social touch. Proc. Natl Acad. Sci. USA 109 , E1657–E1666 (2012).
Sorokowska, A. et al. Affective interpersonal touch in close relationships: a cross-cultural perspective. Personal. Soc. Psychol. Bull. 47 , 1705–1721 (2021).
Ravaja, N., Harjunen, V., Ahmed, I., Jacucci, G. & Spapé, M. M. Feeling touched: emotional modulation of somatosensory potentials to interpersonal touch. Sci. Rep. 7 , 40504 (2017).
Saarinen, A., Harjunen, V., Jasinskaja-Lahti, I., Jääskeläinen, I. P. & Ravaja, N. Social touch experience in different contexts: a review. Neurosci. Biobehav. Rev. 131 , 360–372 (2021).
Huisman, G. Social touch technology: a survey of haptic technology for social touch. IEEE Trans. Haptics 10 , 391–408 (2017).
Lewejohann, L., Schwabe, K., Häger, C. & Jirkof, P. Impulse for animal welfare outside the experiment. Lab. Anim. https://doi.org/10.17169/REFUBIUM-26765 (2020).
Sørensen, J. T., Sandøe, P. & Halberg, N. Animal welfare as one among several values to be considered at farm level: the idea of an ethical account for livestock farming. Acta Agric. Scand. A 51 , 11–16 (2001).
Google Scholar
Verga, M. & Michelazzi, M. Companion animal welfare and possible implications on the human–pet relationship. Ital. J. Anim. Sci. 8 , 231–240 (2009).
Coulon, M. et al. Do lambs perceive regular human stroking as pleasant? Behavior and heart rate variability analyses. PLoS ONE 10 , e0118617 (2015).
Soares, M. C., Oliveira, R. F., Ros, A. F. H., Grutter, A. S. & Bshary, R. Tactile stimulation lowers stress in fish. Nat. Commun. 2 , 534 (2011).
Gourkow, N., Hamon, S. C. & Phillips, C. J. C. Effect of gentle stroking and vocalization on behaviour, mucosal immunity and upper respiratory disease in anxious shelter cats. Prev. Vet. Med. 117 , 266–275 (2014).
Oliveira, V. E. et al. Oxytocin and vasopressin within the ventral and dorsal lateral septum modulate aggression in female rats. Nat. Commun. 12 , 2900 (2021).
Burleson, M. H., Roberts, N. A., Coon, D. W. & Soto, J. A. Perceived cultural acceptability and comfort with affectionate touch: differences between Mexican Americans and European Americans. J. Soc. Personal. Relatsh. 36 , 1000–1022 (2019).
Wijaya, M. et al. The human ‘feel’ of touch contributes to its perceived pleasantness. J. Exp. Psychol. Hum. Percept. Perform. 46 , 155–171 (2020).
Golaya, S. Touch-hunger: an unexplored consequence of the COVID-19 pandemic. Indian J. Psychol. Med. 43 , 362–363 (2021).
Ng, T. W. H., Sorensen, K. L., Zhang, Y. & Yim, F. H. K. Anger, anxiety, depression, and negative affect: convergent or divergent? J. Vocat. Behav. 110 , 186–202 (2019).
Maier, M., Bartoš, F. & Wagenmakers, E.-J. Robust Bayesian meta-analysis: addressing publication bias with model-averaging. Psychol. Methods 28 , 107–122 (2022).
Ahles, T. A. et al. Massage therapy for patients undergoing autologous bone marrow transplantation. J. Pain. Symptom Manag. 18 , 157–163 (1999).
Albert, N. M. et al. A randomized trial of massage therapy after heart surgery. Heart Lung 38 , 480–490 (2009).
Ang, J. Y. et al. A randomized placebo-controlled trial of massage therapy on the immune system of preterm infants. Pediatrics 130 , e1549–e1558 (2012).
Arditi, H., Feldman, R. & Eidelman, A. I. Effects of human contact and vagal regulation on pain reactivity and visual attention in newborns. Dev. Psychobiol. 48 , 561–573 (2006).
Arora, J., Kumar, A. & Ramji, S. Effect of oil massage on growth and neurobehavior in very low birth weight preterm neonates. Indian Pediatr. 42 , 1092–1100 (2005).
PubMed Google Scholar
Asadollahi, M., Jabraeili, M., Mahallei, M., Asgari Jafarabadi, M. & Ebrahimi, S. Effects of gentle human touch and field massage on urine cortisol level in premature infants: a randomized, controlled clinical trial. J. Caring Sci. 5 , 187–194 (2016).
Basiri-Moghadam, M., Basiri-Moghadam, K., Kianmehr, M. & Jani, S. The effect of massage on neonatal jaundice in stable preterm newborn infants: a randomized controlled trial. J. Pak. Med. Assoc. 65 , 602–606 (2015).
Bauer, B. A. et al. Effect of massage therapy on pain, anxiety, and tension after cardiac surgery: a randomized study. Complement. Ther. Clin. Pract. 16 , 70–75 (2010).
Beijers, R., Cillessen, L. & Zijlmans, M. A. C. An experimental study on mother-infant skin-to-skin contact in full-terms. Infant Behav. Dev. 43 , 58–65 (2016).
Bennett, S. et al. Acute effects of traditional Thai massage on cortisol levels, arterial blood pressure and stress perception in academic stress condition: a single blind randomised controlled trial. J. Bodyw. Mov. Therapies 20 , 286–292 (2016).
Bergman, N., Linley, L. & Fawcus, S. Randomized controlled trial of skin-to-skin contact from birth versus conventional incubator for physiological stabilization in 1200- to 2199-gram newborns. Acta Paediatr. 93 , 779–785 (2004).
Bigelow, A., Power, M., MacLellan‐Peters, J., Alex, M. & McDonald, C. Effect of mother/infant skin‐to‐skin contact on postpartum depressive symptoms and maternal physiological stress. J. Obstet. Gynecol. Neonatal Nurs. 41 , 369–382 (2012).
Billhult, A., Bergbom, I. & Stener-Victorin, E. Massage relieves nausea in women with breast cancer who are undergoing chemotherapy. J. Altern. Complement. Med. 13 , 53–57 (2007).
Billhult, A., Lindholm, C., Gunnarsson, R. & Stener-Victorin, E. The effect of massage on cellular immunity, endocrine and psychological factors in women with breast cancer—a randomized controlled clinical trial. Auton. Neurosci. 140 , 88–95 (2008).
Braun, L. A. et al. Massage therapy for cardiac surgery patients—a randomized trial. J. Thorac. Cardiovasc. Surg. 144 , 1453–1459 (2012).
Cabibihan, J.-J. & Chauhan, S. S. Physiological responses to affective tele-touch during induced emotional stimuli. IEEE Trans. Affect. Comput. 8 , 108–118 (2017).
Campeau, M.-P. et al. Impact of massage therapy on anxiety levels in patients undergoing radiation therapy: randomized controlled trial. J. Soc. Integr. Oncol. 5 , 133–138 (2007).
Can, Ş. & Kaya, H. The effects of yakson or gentle human touch training given to mothers with preterm babies on attachment levels and the responses of the baby: a randomized controlled trial. Health Care Women Int. 43 , 479–498 (2021).
Carfoot, S., Williamson, P. & Dickson, R. A randomised controlled trial in the north of England examining the effects of skin-to-skin care on breast feeding. Midwifery 21 , 71–79 (2005).
Castral, T. C., Warnock, F., Leite, A. M., Haas, V. J. & Scochi, C. G. S. The effects of skin-to-skin contact during acute pain in preterm newborns. Eur. J. Pain. 12 , 464–471 (2008).
Cattaneo, A. et al. Kangaroo mother care for low birthweight infants: a randomized controlled trial in different settings. Acta Paediatr. 87 , 976–985 (1998).
Charpak, N., Ruiz-Peláez, J. G. & Charpak, Y. Rey-Martinez kangaroo mother program: an alternative way of caring for low birth weight infants? One year mortality in a two cohort study. Pediatrics 94 , 804–810 (1994).
Chermont, A. G., Falcão, L. F. M., de Souza Silva, E. H. L., de Cássia Xavier Balda, R. & Guinsburg, R. Skin-to-skin contact and/or oral 25% dextrose for procedural pain relief for term newborn infants. Pediatrics 124 , e1101–e1107 (2009).
Chi Luong, K., Long Nguyen, T., Huynh Thi, D. H., Carrara, H. P. O. & Bergman, N. J. Newly born low birthweight infants stabilise better in skin-to-skin contact than when separated from their mothers: a randomised controlled trial. Acta Paediatr. 105 , 381–390 (2016).
Cho, E.-S. et al. The effects of kangaroo care in the neonatal intensive care unit on the physiological functions of preterm infants, maternal–infant attachment, and maternal stress. J. Pediatr. Nurs. 31 , 430–438 (2016).
Choi, H. et al. The effects of massage therapy on physical growth and gastrointestinal function in premature infants: a pilot study. J. Child Health Care 20 , 394–404 (2016).
Choudhary, M. et al. To study the effect of Kangaroo mother care on pain response in preterm neonates and to determine the behavioral and physiological responses to painful stimuli in preterm neonates: a study from western Rajasthan. J. Matern. Fetal Neonatal Med. 29 , 826–831 (2016).
Christensson, K. et al. Temperature, metabolic adaptation and crying in healthy full-term newborns cared for skin-to-skin or in a cot. Acta Paediatr. 81 , 488–493 (1992).
Cloutier, S. & Newberry, R. C. Use of a conditioning technique to reduce stress associated with repeated intra-peritoneal injections in laboratory rats. Appl. Anim. Behav. Sci. 112 , 158–173 (2008).
Cloutier, S., Wahl, K., Baker, C. & Newberry, R. C. The social buffering effect of playful handling on responses to repeated intraperitoneal injections in laboratory rats. J. Am. Assoc. Lab. Anim. Sci. 53 , 168–173 (2014).
CAS PubMed PubMed Central Google Scholar
Cloutier, S., Wahl, K. L., Panksepp, J. & Newberry, R. C. Playful handling of laboratory rats is more beneficial when applied before than after routine injections. Appl. Anim. Behav. Sci. 164 , 81–90 (2015).
Cong, X. et al. Effects of skin-to-skin contact on autonomic pain responses in preterm infants. J. Pain. 13 , 636–645 (2012).
Cong, X., Ludington-Hoe, S. M., McCain, G. & Fu, P. Kangaroo care modifies preterm infant heart rate variability in response to heel stick pain: pilot study. Early Hum. Dev. 85 , 561–567 (2009).
Cong, X., Ludington-Hoe, S. M. & Walsh, S. Randomized crossover trial of kangaroo care to reduce biobehavioral pain responses in preterm infants: a pilot study. Biol. Res. Nurs. 13 , 204–216 (2011).
Costa, R. et al. Tactile stimulation of adult rats modulates hormonal responses, depression-like behaviors, and memory impairment induced by chronic mild stress: role of angiotensin II. Behav. Brain Res. 379 , 112250 (2020).
Cutshall, S. M. et al. Effect of massage therapy on pain, anxiety, and tension in cardiac surgical patients: a pilot study. Complement. Ther. Clin. Pract. 16 , 92–95 (2010).
Dalili, H., Sheikhi, S., Shariat, M. & Haghnazarian, E. Effects of baby massage on neonatal jaundice in healthy Iranian infants: a pilot study. Infant Behav. Dev. 42 , 22–26 (2016).
Diego, M. A., Field, T. & Hernandez-Reif, M. Vagal activity, gastric motility, and weight gain in massaged preterm neonates. J. Pediatr. 147 , 50–55 (2005).
Diego, M. A., Field, T. & Hernandez-Reif, M. Temperature increases in preterm infants during massage therapy. Infant Behav. Dev. 31 , 149–152 (2008).
Diego, M. A. et al. Preterm infant massage elicits consistent increases in vagal activity and gastric motility that are associated with greater weight gain. Acta Paediatr. 96 , 1588–1591 (2007).
Diego, M. A. et al. Spinal cord patients benefit from massage therapy. Int. J. Neurosci. 112 , 133–142 (2002).
Diego, M. A. et al. Aggressive adolescents benefit from massage therapy. Adolescence 37 , 597–607 (2002).
Diego, M. A. et al. HIV adolescents show improved immune function following massage therapy. Int. J. Neurosci. 106 , 35–45 (2001).
Dieter, J. N. I., Field, T., Hernandez-Reif, M., Emory, E. K. & Redzepi, M. Stable preterm infants gain more weight and sleep less after five days of massage therapy. J. Pediatr. Psychol. 28 , 403–411 (2003).
Ditzen, B. et al. Effects of different kinds of couple interaction on cortisol and heart rate responses to stress in women. Psychoneuroendocrinology 32 , 565–574 (2007).
Dreisoerner, A. et al. Self-soothing touch and being hugged reduce cortisol responses to stress: a randomized controlled trial on stress, physical touch, and social identity. Compr. Psychoneuroendocrinol. 8 , 100091 (2021).
Eaton, M., Mitchell-Bonair, I. L. & Friedmann, E. The effect of touch on nutritional intake of chronic organic brain syndrome patients. J. Gerontol. 41 , 611–616 (1986).
Edens, J. L., Larkin, K. T. & Abel, J. L. The effect of social support and physical touch on cardiovascular reactions to mental stress. J. Psychosom. Res. 36 , 371–382 (1992).
El-Farrash, R. A. et al. Longer duration of kangaroo care improves neurobehavioral performance and feeding in preterm infants: a randomized controlled trial. Pediatr. Res. 87 , 683–688 (2020).
Erlandsson, K., Dsilna, A., Fagerberg, I. & Christensson, K. Skin-to-skin care with the father after cesarean birth and its effect on newborn crying and prefeeding behavior. Birth 34 , 105–114 (2007).
Escalona, A., Field, T., Singer-Strunck, R., Cullen, C. & Hartshorn, K. Brief report: improvements in the behavior of children with autism following massage therapy. J. Autism Dev. Disord. 31 , 513–516 (2001).
Fattah, M. A. & Hamdy, B. Pulmonary functions of children with asthma improve following massage therapy. J. Altern. Complement. Med. 17 , 1065–1068 (2011).
Feldman, R. & Eidelman, A. I. Skin-to-skin contact (kangaroo care) accelerates autonomic and neurobehavioural maturation in preterm infants. Dev. Med. Child Neurol. 45 , 274–281 (2003).
Feldman, R., Eidelman, A. I., Sirota, L. & Weller, A. Comparison of skin-to-skin (kangaroo) and traditional care: parenting outcomes and preterm infant development. Pediatrics 110 , 16–26 (2002).
Feldman, R., Singer, M. & Zagoory, O. Touch attenuates infants’ physiological reactivity to stress. Dev. Sci. 13 , 271–278 (2010).
Feldman, R., Weller, A., Sirota, L. & Eidelman, A. I. Testing a family intervention hypothesis: the contribution of mother–infant skin-to-skin contact (kangaroo care) to family interaction, proximity, and touch. J. Fam. Psychol. 17 , 94–107 (2003).
Ferber, S. G. et al. Massage therapy by mothers and trained professionals enhances weight gain in preterm infants. Early Hum. Dev. 67 , 37–45 (2002).
Ferber, S. G. & Makhoul, I. R. The effect of skin-to-skin contact (kangaroo care) shortly after birth on the neurobehavioral responses of the term newborn: a randomized, controlled trial. Pediatrics 113 , 858–865 (2004).
Ferreira, A. M. & Bergamasco, N. H. P. Behavioral analysis of preterm neonates included in a tactile and kinesthetic stimulation program during hospitalization. Rev. Bras. Fisioter. 14 , 141–148 (2010).
Fidanza, F., Polimeni, E., Pierangeli, V. & Martini, M. A better touch: C-tactile fibers related activity is associated to pain reduction during temporal summation of second pain. J. Pain. 22 , 567–576 (2021).
Field, T. et al. Leukemia immune changes following massage therapy. J. Bodyw. Mov. Ther. 5 , 271–274 (2001).
Field, T. et al. Benefits of combining massage therapy with group interpersonal psychotherapy in prenatally depressed women. J. Bodyw. Mov. Ther. 13 , 297–303 (2009).
Field, T., Delage, J. & Hernandez-Reif, M. Movement and massage therapy reduce fibromyalgia pain. J. Bodyw. Mov. Ther. 7 , 49–52 (2003).
Field, T. et al. Fibromyalgia pain and substance P decrease and sleep improves after massage therapy. J. Clin. Rheumatol. 8 , 72–76 (2002).
Field, T., Diego, M., Gonzalez, G. & Funk, C. G. Neck arthritis pain is reduced and range of motion is increased by massage therapy. Complement. Ther. Clin. Pract. 20 , 219–223 (2014).
Field, T., Diego, M., Hernandez-Reif, M., Deeds, O. & Figueiredo, B. Pregnancy massage reduces prematurity, low birthweight and postpartum depression. Infant Behav. Dev. 32 , 454–460 (2009).
Field, T. et al. Insulin and insulin-like growth factor-1 increased in preterm neonates following massage therapy. J. Dev. Behav. Pediatr. 29 , 463–466 (2008).
Field, T. et al. Yoga and massage therapy reduce prenatal depression and prematurity. J. Bodyw. Mov. Ther. 16 , 204–209 (2012).
Field, T., Diego, M., Hernandez-Reif, M., Schanberg, S. & Kuhn, C. Massage therapy effects on depressed pregnant women. J. Psychosom. Obstet. Gynecol. 25 , 115–122 (2004).
Field, T., Diego, M., Hernandez-Reif, M. & Shea, J. Hand arthritis pain is reduced by massage therapy. J. Bodyw. Mov. Ther. 11 , 21–24 (2007).
Field, T., Gonzalez, G., Diego, M. & Mindell, J. Mothers massaging their newborns with lotion versus no lotion enhances mothers’ and newborns’ sleep. Infant Behav. Dev. 45 , 31–37 (2016).
Field, T. et al. Children with asthma have improved pulmonary functions after massage therapy. J. Pediatr. 132 , 854–858 (1998).
Field, T., Hernandez-Reif, M., Diego, M. & Fraser, M. Lower back pain and sleep disturbance are reduced following massage therapy. J. Bodyw. Mov. Ther. 11 , 141–145 (2007).
Field, T. et al. Effects of sexual abuse are lessened by massage therapy. J. Bodyw. Mov. Ther. 1 , 65–69 (1997).
Field, T. et al. Pregnant women benefit from massage therapy. J. Psychosom. Obstet. Gynecol. 20 , 31–38 (1999).
Field, T. et al. Juvenilerheumatoid arthritis: benefits from massage therapy. J. Pediatr. Psychol. 22 , 607–617 (1997).
Field, T., Hernandez-Reif, M., Taylor, S., Quintino, O. & Burman, I. Labor pain is reduced by massage therapy. J. Psychosom. Obstet. Gynecol. 18 , 286–291 (1997).
Field, T. et al. Massage therapy reduces anxiety and enhances EEG pattern of alertness and math computations. Int. J. Neurosci. 86 , 197–205 (1996).
Field, T. et al. Brief report: autistic children’s attentiveness and responsivity improve after touch therapy. J. Autism Dev. Disord. 27 , 333–338 (1997).
Field, T. M. et al. Tactile/kinesthetic stimulation effects on preterm neonates. Pediatrics 77 , 654–658 (1986).
Field, T. et al. Massage reduces anxiety in child and adolescent psychiatric patients. J. Am. Acad. Child Adolesc. Psychiatry 31 , 125–131 (1992).
Field, T. et al. Burn injuries benefit from massage therapy. J. Burn Care Res. 19 , 241–244 (1998).
Filho, F. L. et al. Effect of maternal skin-to-skin contact on decolonization of methicillin-oxacillin-resistant Staphylococcus in neonatal intensive care units: a randomized controlled trial. BMC Pregnancy Childbirth https://doi.org/10.1186/s12884-015-0496-1 (2015).
Forward, J. B., Greuter, N. E., Crisall, S. J. & Lester, H. F. Effect of structured touch and guided imagery for pain and anxiety in elective joint replacement patients—a randomized controlled trial: M-TIJRP. Perm. J. 19 , 18–28 (2015).
Fraser, J. & Ross Kerr, J. Psychophysiological effects of back massage on elderly institutionalized patients. J. Adv. Nurs. 18 , 238–245 (1993).
Frey Law, L. A. et al. Massage reduces pain perception and hyperalgesia in experimental muscle pain: a randomized, controlled trial. J. Pain. 9 , 714–721 (2008).
Gao, H. et al. Effect of repeated kangaroo mother care on repeated procedural pain in preterm infants: a randomized controlled trial. Int. J. Nurs. Stud. 52 , 1157–1165 (2015).
Garner, B. et al. Pilot study evaluating the effect of massage therapy on stress, anxiety and aggression in a young adult psychiatric inpatient unit. Aust. N. Z. J. Psychiatry 42 , 414–422 (2008).
Gathwala, G., Singh, B. & Singh, J. Effect of kangaroo mother care on physical growth, breastfeeding and its acceptability. Trop. Dr. 40 , 199–202 (2010).
Geva, N., Uzefovsky, F. & Levy-Tzedek, S. Touching the social robot PARO reduces pain perception and salivary oxytocin levels. Sci. Rep. 10 , 9814 (2020).
Gitau, R. et al. Acute effects of maternal skin-to-skin contact and massage on saliva cortisol in preterm babies. J. Reprod. Infant Psychol. 20 , 83–88 (2002).
Givi, M. Durability of effect of massage therapy on blood pressure. Int. J. Prev. Med. 4 , 511–516 (2013).
PubMed PubMed Central Google Scholar
Glover, V., Onozawa, K. & Hodgkinson, A. Benefits of infant massage for mothers with postnatal depression. Semin. Neonatol. 7 , 495–500 (2002).
Gonzalez, A. et al. Weight gain in preterm infants following parent-administered vimala massage: a randomized controlled trial. Am. J. Perinatol. 26 , 247–252 (2009).
Gray, L., Watt, L. & Blass, E. M. Skin-to-skin contact is analgesic in healthy newborns. Pediatrics 105 , e14 (2000).
Grewen, K. M., Anderson, B. J., Girdler, S. S. & Light, K. C. Warm partner contact is related to lower cardiovascular reactivity. Behav. Med. 29 , 123–130 (2003).
Groër, M. W., Hill, J., Wilkinson, J. E. & Stuart, A. Effects of separation and separation with supplemental stroking in BALB/c infant mice. Biol. Res. Nurs. 3 , 119–131 (2002).
Gürol, A. P., Polat, S. & Nuran Akçay, M. Itching, pain, and anxiety levels are reduced with massage therapy in burned adolescents. J. Burn Care Res. 31 , 429–432 (2010).
Haley, S. et al. Tactile/kinesthetic stimulation (TKS) increases tibial speed of sound and urinary osteocalcin (U-MidOC and unOC) in premature infants (29–32 weeks PMA). Bone 51 , 661–666 (2012).
Harris, M., Richards, K. C. & Grando, V. T. The effects of slow-stroke back massage on minutes of nighttime sleep in persons with dementia and sleep disturbances in the nursing home: a pilot study. J. Holist. Nurs. 30 , 255–263 (2012).
Hart, S. et al. Anorexia nervosa symptoms are reduced by massage therapy. Eat. Disord. 9 , 289–299 (2001).
Hattan, J., King, L. & Griffiths, P. The impact of foot massage and guided relaxation following cardiac surgery: a randomized controlled trial. Issues Innov. Nurs. Pract. 37 , 199–207 (2002).
Haynes, A. C. et al. A calming hug: design and validation of a tactile aid to ease anxiety. PLoS ONE 17 , e0259838 (2022).
Henricson, M., Ersson, A., Määttä, S., Segesten, K. & Berglund, A.-L. The outcome of tactile touch on stress parameters in intensive care: a randomized controlled trial. Complement. Ther. Clin. Pract. 14 , 244–254 (2008).
Hernandez-Reif, M., Diego, M. & Field, T. Preterm infants show reduced stress behaviors and activity after 5 days of massage therapy. Infant Behav. Dev. 30 , 557–561 (2007).
Hernandez-Reif, M., Dieter, J. N. I., Field, T., Swerdlow, B. & Diego, M. Migraine headaches are reduced by massage therapy. Int. J. Neurosci. 96 , 1–11 (1998).
Hernandez-Reif, M. et al. Natural killer cells and lymphocytes increase in women with breast cancer following massage therapy. Int. J. Neurosci. 115 , 495–510 (2005).
Hernandez-Reif, M. et al. Children with cystic fibrosis benefit from massage therapy. J. Pediatr. Psychol. 24 , 175–181 (1999).
Hernandez-Reif, M., Field, T., Krasnegor, J. & Theakston, H. Lower back pain is reduced and range of motion increased after massage therapy. Int. J. Neurosci. 106 , 131–145 (2001).
Hernandez-Reif, M. et al. High blood pressure and associated symptoms were reduced by massage therapy. J. Bodyw. Mov. Ther. 4 , 31–38 (2000).
Hernandez-Reif, M. et al. Parkinson’s disease symptoms are differentially affected by massage therapy vs. progressive muscle relaxation: a pilot study. J. Bodyw. Mov. Ther. 6 , 177–182 (2002).
Hernandez-Reif, M., Field, T. & Theakston, H. Multiple sclerosis patients benefit from massage therapy. J. Bodyw. Mov. Ther. 2 , 168–174 (1998).
Hernandez-Reif, M. et al. Breast cancer patients have improved immune and neuroendocrine functions following massage therapy. J. Psychosom. Res. 57 , 45–52 (2004).
Hertenstein, M. J. & Campos, J. J. Emotion regulation via maternal touch. Infancy 2 , 549–566 (2001).
Hinchcliffe, J. K., Mendl, M. & Robinson, E. S. J. Rat 50 kHz calls reflect graded tickling-induced positive emotion. Curr. Biol. 30 , R1034–R1035 (2020).
Hodgson, N. A. & Andersen, S. The clinical efficacy of reflexology in nursing home residents with dementia. J. Altern. Complement. Med. 14 , 269–275 (2008).
Hoffmann, L. & Krämer, N. C. The persuasive power of robot touch. Behavioral and evaluative consequences of non-functional touch from a robot. PLoS ONE 16 , e0249554 (2021).
Holst, S., Lund, I., Petersson, M. & Uvnäs-Moberg, K. Massage-like stroking influences plasma levels of gastrointestinal hormones, including insulin, and increases weight gain in male rats. Auton. Neurosci. 120 , 73–79 (2005).
Hori, M. et al. Tickling during adolescence alters fear-related and cognitive behaviors in rats after prolonged isolation. Physiol. Behav. 131 , 62–67 (2014).
Hori, M. et al. Effects of repeated tickling on conditioned fear and hormonal responses in socially isolated rats. Neurosci. Lett. 536 , 85–89 (2013).
Hucklenbruch-Rother, E. et al. Delivery room skin-to-skin contact in preterm infants affects long-term expression of stress response genes. Psychoneuroendocrinology 122 , 104883 (2020).
Im, H. & Kim, E. Effect of yakson and gentle human touch versus usual care on urine stress hormones and behaviors in preterm infants: a quasi-experimental study. Int. J. Nurs. Stud. 46 , 450–458 (2009).
Jain, S., Kumar, P. & McMillan, D. D. Prior leg massage decreases pain responses to heel stick in preterm babies. J. Paediatr. Child Health 42 , 505–508 (2006).
Jane, S.-W. et al. Effects of massage on pain, mood status, relaxation, and sleep in Taiwanese patients with metastatic bone pain: a randomized clinical trial. Pain 152 , 2432–2442 (2011).
Johnston, C. C. et al. Kangaroo mother care diminishes pain from heel lance in very preterm neonates: a crossover trial. BMC Pediatr. 8 , 13 (2008).
Johnston, C. C. et al. Kangaroo care is effective in diminishing pain response in preterm neonates. Arch. Pediatr. Adolesc. Med. 157 , 1084–1088 (2003).
Jung, M. J., Shin, B.-C., Kim, Y.-S., Shin, Y.-I. & Lee, M. S. Is there any difference in the effects of QI therapy (external QIGONG) with and without touching? a pilot study. Int. J. Neurosci. 116 , 1055–1064 (2006).
Kapoor, Y. & Orr, R. Effect of therapeutic massage on pain in patients with dementia. Dementia 16 , 119–125 (2017).
Karagozoglu, S. & Kahve, E. Effects of back massage on chemotherapy-related fatigue and anxiety: supportive care and therapeutic touch in cancer nursing. Appl. Nurs. Res. 26 , 210–217 (2013).
Karbasi, S. A., Golestan, M., Fallah, R., Golshan, M. & Dehghan, Z. Effect of body massage on increase of low birth weight neonates growth parameters: a randomized clinical trial. Iran. J. Reprod. Med. 11 , 583–588 (2013).
Kashaninia, Z., Sajedi, F., Rahgozar, M. & Noghabi, F. A. The effect of kangaroo care on behavioral responses to pain of an intramuscular injection in neonates . J. Pediatr. Nurs. 3 , 275–280 (2008).
Kelling, C., Pitaro, D. & Rantala, J. Good vibes: The impact of haptic patterns on stress levels. In Proc. 20th International Academic Mindtrek Conference 130–136 (Association for Computing Machinery, 2016).
Khilnani, S., Field, T., Hernandez-Reif, M. & Schanberg, S. Massage therapy improves mood and behavior of students with attention-deficit/hyperactivity disorder. Adolescence 38 , 623–638 (2003).
Kianmehr, M. et al. The effect of massage on serum bilirubin levels in term neonates with hyperbilirubinemia undergoing phototherapy. Nautilus 128 , 36–41 (2014).
Kim, I.-H., Kim, T.-Y. & Ko, Y.-W. The effect of a scalp massage on stress hormone, blood pressure, and heart rate of healthy female. J. Phys. Ther. Sci. 28 , 2703–2707 (2016).
Kim, M. A., Kim, S.-J. & Cho, H. Effects of tactile stimulation by fathers on physiological responses and paternal attachment in infants in the NICU: a pilot study. J. Child Health Care 21 , 36–45 (2017).
Kim, M. S., Sook Cho, K., Woo, H.-M. & Kim, J. H. Effects of hand massage on anxiety in cataract surgery using local anesthesia. J. Cataract Refr. Surg. 27 , 884–890 (2001).
Koole, S. L., Tjew A Sin, M. & Schneider, I. K. Embodied terror management: interpersonal touch alleviates existential concerns among individuals with low self-esteem. Psychol. Sci. 25 , 30–37 (2014).
Krohn, M. et al. Depression, mood, stress, and Th1/Th2 immune balance in primary breast cancer patients undergoing classical massage therapy. Support. Care Cancer 19 , 1303–1311 (2011).
Kuhn, C. et al. Tactile-kinesthetic stimulation effects sympathetic and adrenocortical function in preterm infants. J. Pediatr. 119 , 434–440 (1991).
Kumar, J. et al. Effect of oil massage on growth in preterm neonates less than 1800 g: a randomized control trial. Indian J. Pediatr. 80 , 465–469 (2013).
Lee, H.-K. The effects of infant massage on weight, height, and mother–infant interaction. J. Korean Acad. Nurs. 36 , 1331–1339 (2006).
Leivadi, S. et al. Massage therapy and relaxation effects on university dance students. J. Dance Med. Sci. 3 , 108–112 (1999).
Lindgren, L. et al. Touch massage: a pilot study of a complex intervention. Nurs. Crit. Care 18 , 269–277 (2013).
Lindgren, L. et al. Physiological responses to touch massage in healthy volunteers. Auton. Neurosci. Basic Clin. 158 , 105–110 (2010).
Listing, M. et al. Massage therapy reduces physical discomfort and improves mood disturbances in women with breast cancer. Psycho-Oncol. 18 , 1290–1299 (2009).
Ludington-Hoe, S. M., Cranston Anderson, G., Swinth, J. Y., Thompson, C. & Hadeed, A. J. Randomized controlled trial of kangaroo care: cardiorespiratory and thermal effects on healthy preterm infants. Neonatal Netw. 23 , 39–48 (2004).
Lund, I. et al. Corticotropin releasing factor in urine—a possible biochemical marker of fibromyalgia. Neurosci. Lett. 403 , 166–171 (2006).
Ma, Y.-K. et al. Lack of social touch alters anxiety-like and social behaviors in male mice. Stress 25 , 134–144 (2022).
Massaro, A. N., Hammad, T. A., Jazzo, B. & Aly, H. Massage with kinesthetic stimulation improves weight gain in preterm infants. J. Perinatol. 29 , 352–357 (2009).
Mathai, S., Fernandez, A., Mondkar, J. & Kanbur, W. Effects of tactile-kinesthetic stimulation in preterms–a controlled trial. Indian Pediatr. 38 , 1091–1098 (2001).
CAS PubMed Google Scholar
Matsunaga, M. et al. Profiling of serum proteins influenced by warm partner contact in healthy couples. Neuroenocrinol. Lett. 30 , 227–236 (2009).
CAS Google Scholar
Mendes, E. W. & Procianoy, R. S. Massage therapy reduces hospital stay and occurrence of late-onset sepsis in very preterm neonates. J. Perinatol. 28 , 815–820 (2008).
Mirnia, K., Arshadi Bostanabad, M., Asadollahi, M. & Hamid Razzaghi, M. Paternal skin-to-skin care and its effect on cortisol levels of the infants. Iran. J. Pediatrics 27 , e8151 (2017).
Mitchell, A. J., Yates, C., Williams, K. & Hall, R. W. Effects of daily kangaroo care on cardiorespiratory parameters in preterm infants. J. Neonatal-Perinat. Med. 6 , 243–249 (2013).
Mitchinson, A. R. et al. Acute postoperative pain management using massage as an adjuvant therapy: a randomized trial. Arch. Surg. 142 , 1158–1167 (2007).
Modrcin-Talbott, M. A., Harrison, L. L., Groer, M. W. & Younger, M. S. The biobehavioral effects of gentle human touch on preterm infants. Nurs. Sci. Q. 16 , 60–67 (2003).
Mok, E. & Pang Woo, C. The effects of slow-stroke back massage on anxiety and shoulder pain in elderly stroke patients. Complement. Ther. Nurs. Midwifery 10 , 209–216 (2004).
Mokaberian, M., Noripour, S., Sheikh, M. & Mills, P. J. Examining the effectiveness of body massage on physical status of premature neonates and their mothers’ psychological status. Early Child Dev. Care 192 , 2311–2325 (2021).
Mori, H. et al. Effect of massage on blood flow and muscle fatigue following isometric lumbar exercise. Med. Sci. Monit. Int. Med. J. Exp. Clin. Res. 10 , CR173–CR178 (2004).
Moyer-Mileur, L. J., Haley, S., Slater, H., Beachy, J. & Smith, S. L. Massage improves growth quality by decreasing body fat deposition in male preterm infants. J. Pediatr. 162 , 490–495 (2013).
Moyle, W. et al. Foot massage and physiological stress in people with dementia: a randomized controlled trial. J. Altern. Complement. Med. 20 , 305–311 (2014).
Muntsant, A., Shrivastava, K., Recasens, M. & Giménez-Llort, L. Severe perinatal hypoxic-ischemic brain injury induces long-term sensorimotor deficits, anxiety-like behaviors and cognitive impairment in a sex-, age- and task-selective manner in C57BL/6 mice but can be modulated by neonatal handling. Front. Behav. Neurosci. 13 , 7 (2019).
Negahban, H., Rezaie, S. & Goharpey, S. Massage therapy and exercise therapy in patients with multiple sclerosis: a randomized controlled pilot study. Clin. Rehabil. 27 , 1126–1136 (2013).
Nelson, D., Heitman, R. & Jennings, C. Effects of tactile stimulation on premature infant weight gain. J. Obstet. Gynecol. Neonatal Nurs. 15 , 262–267 (1986).
Griffin, J. W. Calculating statistical power for meta-analysis using metapower. Quant. Meth. Psychol . 17 , 24–39 (2021).
Nunes, G. S. et al. Massage therapy decreases pain and perceived fatigue after long-distance Ironman triathlon: a randomised trial. J. Physiother. 62 , 83–87 (2016).
Ohgi, S. et al. Comparison of kangaroo care and standard care: behavioral organization, development, and temperament in healthy, low-birth-weight infants through 1 year. J. Perinatol. 22 , 374–379 (2002).
O′Higgins, M., St. James Roberts, I. & Glover, V. Postnatal depression and mother and infant outcomes after infant massage. J. Affect. Disord. 109 , 189–192 (2008).
Okan, F., Ozdil, A., Bulbul, A., Yapici, Z. & Nuhoglu, A. Analgesic effects of skin-to-skin contact and breastfeeding in procedural pain in healthy term neonates. Ann. Trop. Paediatr. 30 , 119–128 (2010).
Oliveira, D. S., Hachul, H., Goto, V., Tufik, S. & Bittencourt, L. R. A. Effect of therapeutic massage on insomnia and climacteric symptoms in postmenopausal women. Climacteric 15 , 21–29 (2012).
Olsson, E., Ahlsén, G. & Eriksson, M. Skin-to-skin contact reduces near-infrared spectroscopy pain responses in premature infants during blood sampling. Acta Paediatr. 105 , 376–380 (2016).
Pauk, J., Kuhn, C. M., Field, T. M. & Schanberg, S. M. Positive effects of tactile versus kinesthetic or vestibular stimulation on neuroendocrine and ODC activity in maternally-deprived rat pups. Life Sci. 39 , 2081–2087 (1986).
Pinazo, D., Arahuete, L. & Correas, N. Hugging as a buffer against distal fear of death. Calid. Vida Salud 13 , 11–20 (2020).
Pope, M. H. et al. A prospective randomized three-week trial of spinal manipulation, transcutaneous muscle stimulation, massage and corset in the treatment of subacute low back pain. Spine 19 , 2571–2577 (1994).
Preyde, M. Effectiveness of massage therapy for subacute low-back pain: a randomized controlled trial. Can. Med. Assoc. J. 162 , 1815–1820 (2000).
Ramanathan, K., Paul, V. K., Deorari, A. K., Taneja, U. & George, G. Kangaroo mother care in very low birth weight infants. Indian J. Pediatr. 68 , 1019–1023 (2001).
Reddan, M. C., Young, H., Falkner, J., López-Solà, M. & Wager, T. D. Touch and social support influence interpersonal synchrony and pain. Soc. Cogn. Affect. Neurosci. 15 , 1064–1075 (2020).
Rodríguez-Mansilla, J. et al. The effects of ear acupressure, massage therapy and no therapy on symptoms of dementia: a randomized controlled trial. Clin. Rehabil. 29 , 683–693 (2015).
Rose, S. A., Schmidt, K., Riese, M. L. & Bridger, W. H. Effects of prematurity and early intervention on responsivity to tactual stimuli: a comparison of preterm and full-term infants. Child Dev. 51 , 416–425 (1980).
Scafidi, F. A. et al. Massage stimulates growth in preterm infants: a replication. Infant Behav. Dev. 13 , 167–188 (1990).
Scafidi, F. A. et al. Effects of tactile/kinesthetic stimulation on the clinical course and sleep/wake behavior of preterm neonates. Infant Behav. Dev. 9 , 91–105 (1986).
Scafidi, F. & Field, T. Massage therapy improves behavior in neonates born to HIV-positive mothers. J. Pediatr. Psychol. 21 , 889–897 (1996).
Scarr-Salapatek, S. & Williams, M. L. A stimulation program for low birth weight infants. Am. J. Public Health 62 , 662–667 (1972).
Serrano, B., Baños, R. M. & Botella, C. Virtual reality and stimulation of touch and smell for inducing relaxation: a randomized controlled trial. Comput. Hum. Behav. 55 , 1–8 (2016).
Seyyedrasooli, A., Valizadeh, L., Hosseini, M. B., Asgari Jafarabadi, M. & Mohammadzad, M. Effect of vimala massage on physiological jaundice in infants: a randomized controlled trial. J. Caring Sci. 3 , 165–173 (2014).
Sharpe, P. A., Williams, H. G., Granner, M. L. & Hussey, J. R. A randomised study of the effects of massage therapy compared to guided relaxation on well-being and stress perception among older adults. Complement. Therap. Med. 15 , 157–163 (2007).
Sherman, K. J., Cherkin, D. C., Hawkes, R. J., Miglioretti, D. L. & Deyo, R. A. Randomized trial of therapeutic massage for chronic neck pain. Clin. J. Pain. 25 , 233–238 (2009).
Shiloh, S., Sorek, G. & Terkel, J. Reduction of state-anxiety by petting animals in a controlled laboratory experiment. Anxiety, Stress Coping 16 , 387–395 (2003).
Shor-Posner, G. et al. Impact of a massage therapy clinical trial on immune status in young Dominican children infected with HIV-1. J. Altern. Complement. Med. 12 , 511–516 (2006).
Simpson, E. A. et al. Social touch alters newborn monkey behavior. Infant Behav. Dev. 57 , 101368 (2019).
Smith, S. L., Haley, S., Slater, H. & Moyer-Mileur, L. J. Heart rate variability during caregiving and sleep after massage therapy in preterm infants. Early Hum. Dev. 89 , 525–529 (2013).
Smith, S. L. et al. The effect of massage on heart rate variability in preterm infants. J. Perinatol. 33 , 59–64 (2013).
Solkoff, N. & Matuszak, D. Tactile stimulation and behavioral development among low-birthweight infants. Child Psychiatry Hum. Dev. 6 , 3337 (1975).
Srivastava, S., Gupta, A., Bhatnagar, A. & Dutta, S. Effect of very early skin to skin contact on success at breastfeeding and preventing early hypothermia in neonates. Indian J. Public Health 58 , 22–26 (2014).
Stringer, J., Swindell, R. & Dennis, M. Massage in patients undergoing intensive chemotherapy reduces serum cortisol and prolactin: massage in oncology patients reduces serum cortisol. Psycho-Oncol. 17 , 1024–1031 (2008).
Suman Rao, P. N., Udani, R. & Nanavati, R. Kangaroo mother care for low birth weight infants: a randomized controlled trial. Indian Pediatr. 45 , 17–23 (2008).
Sumioka, H. et al. A huggable device can reduce the stress of calling an unfamiliar person on the phone for individuals with ASD. PLoS ONE 16 , e0254675 (2021).
Sumioka, H., Nakae, A., Kanai, R. & Ishiguro, H. Huggable communication medium decreases cortisol levels. Sci. Rep. 3 , 3034 (2013).
Suzuki, M. et al. Physical and psychological effects of 6-week tactile massage on elderly patients with severe dementia. Am. J. Alzheimer’s Dis. Other Dement. 25 , 680–686 (2010).
Thomson, L. J. M., Ander, E. E., Menon, U., Lanceley, A. & Chatterjee, H. J. Quantitative evidence for wellbeing benefits from a heritage-in-health intervention with hospital patients. Int. J. Art. Ther. 17 , 63–79 (2012).
Triplett, J. L. & Arneson, S. W. The use of verbal and tactile comfort to alleviate distress in young hospitalized children. Res. Nurs. Health 2 , 17–23 (1979).
Walach, H., Güthlin, C. & König, M. Efficacy of massage therapy in chronic pain: a pragmatic randomized trial. J. Altern. Complement. Med. 9 , 837–846 (2003).
Walker, S. C. et al. C‐low threshold mechanoafferent targeted dynamic touch modulates stress resilience in rats exposed to chronic mild stress. Eur. J. Neurosci. 55 , 2925–2938 (2022).
Weinrich, S. P. & Weinrich, M. C. The effect of massage on pain in cancer patients. Appl. Nurs. Res. 3 , 140–145 (1990).
Wheeden, A. et al. Massage effects on cocaine-exposed preterm neonates. Dev. Behav. Pediatr. 14 , 318–322 (1993).
White, J. L. & Labarba, R. C. The effects of tactile and kinesthetic stimulation on neonatal development in the premature infant. Dev. Psychobiol. 9 , 569–577 (1976).
Wilkie, D. J. et al. Effects of massage on pain intensity, analgesics and quality of life in patients with cancer pain: a pilot study of a randomized clinical trial conducted within hospice care delivery. Hosp. J. 15 , 31–53 (2000).
Willemse, C. J. A. M., Toet, A. & van Erp, J. B. F. Affective and behavioral responses to robot-initiated social touch: toward understanding the opportunities and limitations of physical contact in human–robot interaction. Front. ICT 4 , 12 (2017).
Willemse, C. J. A. M. & van Erp, J. B. F. Social touch in human–robot interaction: robot-initiated touches can induce positive responses without extensive prior bonding. Int. J. Soc. Robot. 11 , 285–304 (2019).
Woods, D. L., Beck, C. & Sinha, K. The effect of therapeutic touch on behavioral symptoms and cortisol in persons with dementia. Res. Complement. Med. 16 , 181–189 (2009).
Yamaguchi, M., Sekine, T. & Shetty, V. A salivary cytokine panel discriminates moods states following a touch massage intervention. Int. J. Affect. Eng. 19 , 189–198 (2020).
Yamazaki, R. et al. Intimacy in phone conversations: anxiety reduction for Danish seniors with hugvie. Front. Psychol. 7 , 537 (2016).
Yang, M.-H. et al. Comparison of the efficacy of aroma-acupressure and aromatherapy for the treatment of dementia-associated agitation. BMC Complement. Altern. Med. 15 , 93 (2015).
Yates, C. C. et al. The effects of massage therapy to induce sleep in infants born preterm. Pediatr. Phys. Ther. 26 , 405–410 (2014).
Yu, H. et al. Social touch-like tactile stimulation activates a tachykinin 1-oxytocin pathway to promote social interactions. Neuron 110 , 1051–1067 (2022).
Lakens, D. Calculating and reporting effect sizes to facilitate cumulative science: a practical primer for t -tests and ANOVAs. Front. Psychol. 4 , 863 (2013).
Page, M. J., et al. The PRISMA 2020 statement: an updated guideline for reporting systematic reviews. Syst. Rev. https://doi.org/10.1186/s13643-021-01626-4 (2021).
Wilson, D. B. Practical meta-analysis effect size calculator (Version 2023.11.27). https://campbellcollaboration.org/research-resources/effect-size-calculator.html (2023).
Viechtbauer, W. Conducting meta-analyses in R with the metafor package. J. Stat. Softw https://doi.org/10.18637/jss.v036.i03 (2010).
Scammacca, N., Roberts, G. & Stuebing, K. K. Meta-analysis with complex research designs: dealing with dependence from multiple measures and multiple group comparisons. Rev. Educ. Res. 84 , 328–364 (2014).
Pustejovsky, J. E. & Tipton, E. Meta-analysis with robust variance estimation: expanding the range of working models. Prev. Sci. Off. J. Soc. Prev. Res. 23 , 425–438 (2022).
Cook, R. D. in International Encyclopedia of Statistical Science (ed. M. Lovric) S. 301–302 (Springer, 2011).
Higgins, J. P. T., Thompson, S. & Deeks, J. Measuring inconsistency in meta-analyses. BMJ https://doi.org/10.1136/bmj.327.7414.557 (2003).
Oberauer, K. The importance of random slopes in mixed models for Bayesian hypothesis testing. Psychol. Sci. 33 , 648–665 (2022).
Nakagawa, S. et al. The orchard plot: cultivating a forest plot for use in ecology, evolution, and beyond. Res. Synth. Methods 12 , 4–12 (2021).
Download references
Acknowledgements
We thank A. Frick and E. Chris for supporting the initial literature search and coding. We also thank A. Dreisoerner, T. Field, S. Koole, C. Kuhn, M. Henricson, L. Frey Law, J. Fraser, M. Cumella Reddan, and J. Stringer, who kindly responded to our data requests and provided additional information or data with respect to single studies. J.P. was supported by the German National Academy of Sciences Leopoldina (LPDS 2021-05). H.H. was supported by the Marietta-Blau scholarship of the Austrian Agency for Education and Internationalisation (OeAD) and the Deutsche Forschungsgemeinschaft (DFG, German Research Foundation, project ID 422744262 – TRR 289). C.K. received funding from OCENW.XL21.XL21.069 and V.G. from the European Research Council (ERC) under European Union’s Horizon 2020 research and innovation programme, grant ‘HelpUS’ (758703) and from the Dutch Research Council (NWO) grant OCENW.XL21.XL21.069. The funders had no role in study design, data collection and analysis, decision to publish or preparation of the manuscript.
Open access funding provided by Ruhr-Universität Bochum.
Author information
Julian Packheiser
Present address: Social Neuroscience, Faculty of Medicine, Ruhr University Bochum, Bochum, Germany
These authors contributed equally: Julian Packheiser, Helena Hartmann.
Authors and Affiliations
Social Brain Lab, Netherlands Institute for Neuroscience, Royal Netherlands Academy of Art and Sciences, Amsterdam, the Netherlands
Julian Packheiser, Helena Hartmann, Kelly Fredriksen, Valeria Gazzola, Christian Keysers & Frédéric Michon
Center for Translational and Behavioral Neuroscience, University Hospital Essen, Essen, Germany
Helena Hartmann
Clinical Neurosciences, Department for Neurology, University Hospital Essen, Essen, Germany
You can also search for this author in PubMed Google Scholar
Contributions
J.P. contributed to conceptualization, methodology, formal analysis, investigation, data curation, writing the original draft, review and editing, visualization, supervision and project administration. HH contributed to conceptualization, methodology, formal analysis, investigation, data curation, writing the original draft, review and editing, visualization, supervision and project administration. K.F. contributed to investigation, data curation, and review and editing. C.K. and V.G. contributed to conceptualization, and review and editing. F.M. contributed to conceptualization, methodology, formal analysis, investigation, writing the original draft, and review and editing.
Corresponding author
Correspondence to Julian Packheiser .
Ethics declarations
Competing interests.
The authors declare no competing interests.
Peer review
Peer review information.
Nature Human Behaviour thanks Ville Harjunen, Rebecca Boehme and the other, anonymous, reviewer(s) for their contribution to the peer review of this work. Peer reviewer reports are available.
Additional information
Publisher’s note Springer Nature remains neutral with regard to jurisdictional claims in published maps and institutional affiliations.
Supplementary information
Supplementary information.
Supplementary Figs. 1–21 and Tables 1–4.
Reporting Summary
Peer review file, supplementary table 1.
List of studies included in and excluded from the meta-analyses/review.
Supplementary Table 2
PRISMA checklist, manuscript.
Supplementary Table 3
PRISMA checklist, abstract.
Source Data Fig. 2
Effect size/error (columns ‘Hedges_g’ and ‘variance’) information for each study/cohort/effect included in the analysis. Source Data Fig. 3 Effect size/error (columns ‘Hedges_g’ and ‘variance’) together with moderator data (column ‘Outcome’) for each study/cohort/effect included in the analysis. Source Data Fig. 4 Effect size/error (columns ‘Hedges_g’ and ‘variance’) together with moderator data (columns ‘dyad_type’ and ‘skin_to_skin’) for each study/cohort/effect included in the analysis. Source Data Fig. 5 Effect size/error (columns ‘Hedges_g’ and ‘variance’) together with moderator data (column ‘touch_type’) for each study/cohort/effect included in the analysis. Source Data Fig. 6 Effect size/error (columns ‘Hedges_g’ and ‘variance’) together with moderator data (column ‘clin_sample’) for each study/cohort/effect included in the analysis. Source Data Fig. 7 Effect size/error (columns ‘Hedges_g’ and ‘variance’) together with moderator data (column ‘familiarity’) for each study/cohort/effect included in the analysis. Source Data Fig. 7 Effect size/error (columns ‘Hedges_g’ and ‘variance’) together with moderator data (columns ‘touch_duration’ and ‘sessions’) for each study/cohort/effect included in the analysis.
Rights and permissions
Open Access This article is licensed under a Creative Commons Attribution 4.0 International License, which permits use, sharing, adaptation, distribution and reproduction in any medium or format, as long as you give appropriate credit to the original author(s) and the source, provide a link to the Creative Commons licence, and indicate if changes were made. The images or other third party material in this article are included in the article’s Creative Commons licence, unless indicated otherwise in a credit line to the material. If material is not included in the article’s Creative Commons licence and your intended use is not permitted by statutory regulation or exceeds the permitted use, you will need to obtain permission directly from the copyright holder. To view a copy of this licence, visit http://creativecommons.org/licenses/by/4.0/ .
Reprints and permissions
About this article
Cite this article.
Packheiser, J., Hartmann, H., Fredriksen, K. et al. A systematic review and multivariate meta-analysis of the physical and mental health benefits of touch interventions. Nat Hum Behav (2024). https://doi.org/10.1038/s41562-024-01841-8
Download citation
Received : 16 August 2023
Accepted : 29 January 2024
Published : 08 April 2024
DOI : https://doi.org/10.1038/s41562-024-01841-8
Share this article
Anyone you share the following link with will be able to read this content:
Sorry, a shareable link is not currently available for this article.
Provided by the Springer Nature SharedIt content-sharing initiative
Quick links
- Explore articles by subject
- Guide to authors
- Editorial policies
Sign up for the Nature Briefing newsletter — what matters in science, free to your inbox daily.

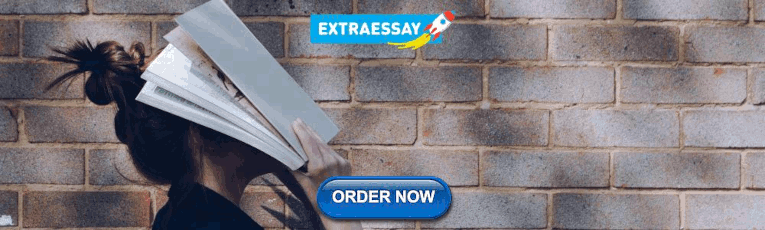
COMMENTS
This review paper outlines the effort devoted to the development and integration of robotic technology for rehabilitation. ... The use of robots in rehabilitation has since grown substantially ... et al. Rehabilitation of arm function after stroke. Literature review. Annals of Physical and Rehabilitation Medicine. 2009; 52 (3):269-293 ...
Rehabilitation is the process of treating post-stroke consequences. Impaired limbs are considered the common outcomes of stroke, which require a professional therapist to rehabilitate the impaired limbs and restore fully or partially its function. Due to the shortage in the number of therapists and other considerations, researchers have been working on developing robots that have the ability ...
Purpose of Review Research in assistive and rehabilitation robotics is a growing, promising, and challenging field emerged due to various social and medical needs such as aging populations, neuromuscular, and musculoskeletal disorders. Such robots can be used in various day-to-day scenarios or to support motor functionality, training, and rehabilitation. This paper reflects on the human-robot ...
This paper gives an overall review of research status in rehabilitation robot technology. In order to study the status of rehabilitation robot technology, they are divided into two categories. One of them is auxiliary and replacement robots and another is training and therapeutic robots. The fixed and mobile robots, intelligent artificial limbs and supporting tools of the auxiliary and ...
This review article presents an in-depth examination of research and development in the fields of rehabilitation, assistive technologies, and humanoid robots. It focuses on parallel robots designed for human body joints with three degrees of freedom, specifically the neck, shoulder, wrist, hip, and ankle. A systematic search was conducted across multiple databases, including Scopus, Web of ...
Robot-assisted rehabilitation is an exciting field which aims to incorporate relevant developments in robotics related to rehabilitation with the intention of defining new methodologies for intervening problems related to muscular, neuromuscular, and osseous diseases. In this study, a systematic and comprehensive literature analysis is conducted to identify the contribution of artificial ...
Lower limb rehabilitation exoskeleton robots integrate sensing, control, and other technologies and exhibit the characteristics of bionics, robotics, information and control science, medicine, and other interdisciplinary areas. In this review, the typical products and prototypes of lower limb exoskeleton rehabilitation robots are introduced and state-of-the-art techniques are analyzed and ...
A comprehensive literature review on rehabilitation robots is carried out to identify the key issues. The main design requirements and development complications are identified and the various approaches used in past robots are reviewed. It begins with a survey of existing human rehabilitation devices designed for use in human assistance and ...
In Section 3.3, the applications of rehabilitation robots for physical therapy, including the upper and lower extremities, with their leading technologies are evaluated. In addition, a number of novel robots introduced in the past decade and recent control strategies, evaluation, and safety assessments are presented.
Passive robotic rehabilitation devices, although less complex and cheaper, cannot supply energy to the affected limbs, hence are limited compared to active devices and are out of the scope of this work. Finally, current challenges in the field are also pointed out in the last section. 2. Robotic Systems for Lower-Limb Rehabilitation.
The innovations in the development of the next-generation rehabilitation robots can lead to significant benefits to human beings. In this paper, a critical literature review is conducted to identify the limitations of existing works and clarify the prosperous research directions in the development of assistive robots.
As interdisciplinary researchers with backgrounds in the social sciences and sciences, we wish to pursue the literature review through the lens of social science and interdisciplinary studies, rather than the dominant science-based field of robotics and rehabilitation. 1.2.2 Robot and Rehabilitation in Social Science Research
In this paper, a comprehensive review on developed home-based rehabilitation technologies of the last 10 years (2011-2020), categorizing them into upper and lower limb devices and considering both commercialized and state-of-the-art realms. ... Its feasibility has been evaluated through several studies using state-of-the-art home robots. The ...
Among the methods of hand function rehabilitation after stroke, robot-assisted rehabilitation is widely used, and the use of hand rehabilitation robots can provide functional training of the hand or assist the paralyzed hand with activities of daily living. However, patients with hand disorders consistently report that the needs of some users are not being met. The purpose of this review is to ...
Neuromotor rehabilitation and improvement of upper limb functions are necessary to improve the life quality of patients who have experienced injuries or have pathological outcomes. Modern approaches, such as robotic-assisted rehabilitation can help to improve rehabilitation processes and thus improve upper limb functions. Therefore, the aim of this study was to investigate the role of robots ...
The literature search identified 43 papers published on robot rehabilitation for PWD that met the criteria. These publications were primarily from five conferences and 38 journals. ... An Exploratory Literature Review of Robots and Their Interaction as Assistive Technology for Persons with Disabilities: Focus on Promoting Activity and ...
A literature review was performed following PRISMA guidelines [] on biomechanical and neuromuscular assessment in upper-limb stroke rehabilitation.The review was composed of two independent searches on (1) biomechanical robotic devices, and (2) electrophysiological digital signal processing.
This pre-registered systematic review and multilevel meta-analysis examined the effects of receiving touch for promoting mental and physical well-being, quantifying the efficacy of touch ...