- Search Menu
- Advance articles
- Author Guidelines
- Open Access
- Submission Site
- Why Publish with HWJ?
- About History Workshop Journal
- Editorial Board
- History Workshop Online
- Advertising and Corporate Services
- Self-Archiving Policy
- Dispatch Dates
- Terms and Conditions
- Publishers' Books for Review
- Journals on Oxford Academic
- Books on Oxford Academic
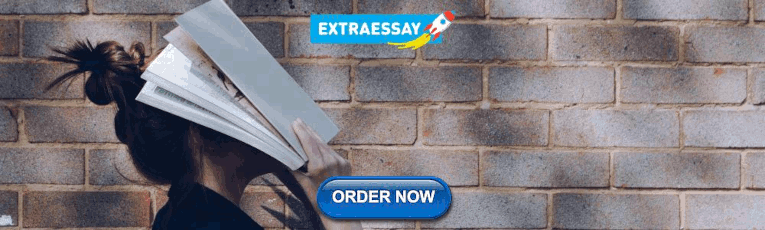
Article Contents
- FOOTBALL NARRATIVES AND THE ISSUE OF SOURCES
- AN UNDERUSED SOURCE: THE MATCH REPORT
- EXCERPTS FROM MATCH REPORTS
- THE MAKING OF MODERN ASSOCIATION FOOTBALL: THE MOTLEY ORIGINS
- MODERN FOOTBALL AND THE FOLK GAMES
- MAKING MODERN FOOTBALL: THE ROLE OF CUPS AND CLYDESIDE
- CONCLUSIONS
- APPENDIX: GRAPH AND METHODOLOGICAL NOTES
- Notes and References
- < Previous
The Origins of Football: History, Ideology and the Making of ‘The People’s Game’
Gavin Kitching is Emeritus Professor of Politics at the University of New South Wales, Sydney, Australia, and Visiting Research Fellow at the International Centre for Sports History and Culture, De Montfort University, Leicester. His current research on the origins of football is the first stage of an attempt to write a social history of his native North-East of England through the lens of football.
- Article contents
- Figures & tables
- Supplementary Data
Gavin Kitching, The Origins of Football: History, Ideology and the Making of ‘The People’s Game’, History Workshop Journal , Volume 79, Issue 1, Spring 2015, Pages 127–153, https://doi.org/10.1093/hwj/dbu023
- Permissions Icon Permissions
Recent scholarship on the origins of association football has been marked by a highly ideological debate on its ‘class’ nature. The traditional story – of a game created by ‘gentlemen’ but taken up, and ultimately dominated, by ‘ruffians’ – has been challenged by a revisionist account which presents football as an ancient ‘people’s’ or ‘plebeian’ game, briefly hijacked by upper-middle class men in the mid-Victorian period, before returning to its ‘popular’ roots from the 1880s onwards. This article suggests that, as currently conducted, the debate is both conceptually confused and bedevilled by paucity of sources. The conceptual problems derive partly from an endemic vagueness in the historical use of the term ‘football’, and partly from a persistent tendency to conflate football play with rules of play. The paucity of sources is well-known in the study of football as a medieval and early modern folk pastime. But it is also an issue in studying early forms of club football. This article uses a hitherto underused source – the match reports of the earliest amateur football clubs in Britain – as part of an attempt to address the conceptual confusion and also to present a genuinely new account of the impact of traditional ‘folk’ football on the modern game. It is suggested that the impact was both real and very short-lived.
Email alerts
Citing articles via.
- Contact History Workshop
- Recommend to your Library
Affiliations
- Online ISSN 1477-4569
- Print ISSN 1363-3554
- Copyright © 2024 Trustees of the History Workshop Journal
- About Oxford Academic
- Publish journals with us
- University press partners
- What we publish
- New features
- Open access
- Institutional account management
- Rights and permissions
- Get help with access
- Accessibility
- Advertising
- Media enquiries
- Oxford University Press
- Oxford Languages
- University of Oxford
Oxford University Press is a department of the University of Oxford. It furthers the University's objective of excellence in research, scholarship, and education by publishing worldwide
- Copyright © 2024 Oxford University Press
- Cookie settings
- Cookie policy
- Privacy policy
- Legal notice
This Feature Is Available To Subscribers Only
Sign In or Create an Account
This PDF is available to Subscribers Only
For full access to this pdf, sign in to an existing account, or purchase an annual subscription.
Click through the PLOS taxonomy to find articles in your field.
For more information about PLOS Subject Areas, click here .
Loading metrics
Open Access
Peer-reviewed
Research Article
The Anatomy of American Football: Evidence from 7 Years of NFL Game Data
* E-mail: [email protected]
Affiliation School of Information Sciences, University of Pittsburgh, Pittsburgh, PA, United States of America

Affiliation Department of Computer Science and Engineering, University of California Riverside, Riverside, CA, United States of America
- Konstantinos Pelechrinis,
- Evangelos Papalexakis
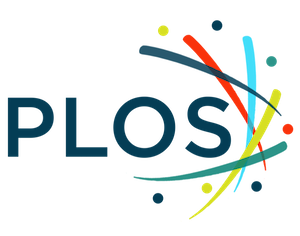
- Published: December 22, 2016
- https://doi.org/10.1371/journal.pone.0168716
- Reader Comments
How much does a fumble affect the probability of winning an American football game? How balanced should your offense be in order to increase the probability of winning by 10%? These are questions for which the coaching staff of National Football League teams have a clear qualitative answer. Turnovers are costly; turn the ball over several times and you will certainly lose. Nevertheless, what does “several” mean? How “certain” is certainly? In this study, we collected play-by-play data from the past 7 NFL seasons, i.e., 2009–2015, and we build a descriptive model for the probability of winning a game. Despite the fact that our model incorporates simple box score statistics, such as total offensive yards, number of turnovers etc., its overall cross-validation accuracy is 84%. Furthermore, we combine this descriptive model with a statistical bootstrap module to build FPM (short for Football Prediction Matchup) for predicting future match-ups. The contribution of FPM is pertinent to its simplicity and transparency, which however does not sacrifice the system’s performance. In particular, our evaluations indicate that our prediction engine performs on par with the current state-of-the-art systems (e.g., ESPN’s FPI and Microsoft’s Cortana). The latter are typically proprietary but based on their components described publicly they are significantly more complicated than FPM . Moreover, their proprietary nature does not allow for a head-to-head comparison in terms of the core elements of the systems but it should be evident that the features incorporated in FPM are able to capture a large percentage of the observed variance in NFL games.
Citation: Pelechrinis K, Papalexakis E (2016) The Anatomy of American Football: Evidence from 7 Years of NFL Game Data. PLoS ONE 11(12): e0168716. https://doi.org/10.1371/journal.pone.0168716
Editor: Kimmo Eriksson, Mälardalen University, SWEDEN
Received: July 23, 2016; Accepted: November 23, 2016; Published: December 22, 2016
Copyright: © 2016 Pelechrinis, Papalexakis. This is an open access article distributed under the terms of the Creative Commons Attribution License , which permits unrestricted use, distribution, and reproduction in any medium, provided the original author and source are credited.
Data Availability: All relevant data are available within the manuscript and deposited in Github: https://github.com/kpelechrinis/footballonomics .
Funding: The author(s) received no specific funding for this work.
Competing interests: The authors have declared that no competing interests exist.
1 Introduction
While American football is viewed mainly as a physical game—and it surely is—at the same time it is probably one of the most strategic sports games, a fact that makes it appealing even to an international crowd [ 1 ]. This has led to people analyzing the game with the use of data analytics methods and game theory. For instance, after the controversial last play call of Super Bowl XLIX the Economist [ 2 ] argued by utilizing appropriate data and game theory that this play was rational and not that bad after all.
The ability to analyze and collect large volumes of data has put forward a quantification-based approach in modeling and analyzing the success in various sports during the last few years. For example, pertinent to American football, Clark et al. [ 3 ] analyzed the factors that affect the success of a field goal kick and contrary to popular belief they did not identify any situational factor (e.g., regular vs post season, home vs away etc.) as being significant. In another direction Pfitzner et al. [ 4 ] and Warner [ 5 ] studied models and systems for determining a successful betting strategy for NFL games, while the authors in [ 6 ] show that the much-discussed off-field misconduct of NFL players does not affect a team’s performance. Furthermore, the spatial information collected from the RFID sensors on NFL players has been used to evaluate quarterbacks’ decision making ability [ 7 ], while efforts to assess the impact of individual offensive linemen on passing have been presented by Alamar and Weinstein-Gould [ 8 ]. Similarly, Correia et al. [ 9 ] analyzed the passing behavior of rugby players—the most similar sport to that of American football. They found that the time required to close the gap between the first attacker and the defense explained 64% of the variance found in pass duration and this can further yield information about future pass possibilities. Nevertheless, despite the availability of play data for American football and the proliferation of the sports analytics literature as well as the literature surrounding the NFL, there are only few—publicly open—studies that have focused on predicting a game’s outcome. Furthermore, some of the existing models make strong theoretical assumptions that are hard to verify (e.g., the team strength factors obeying to a first-order autoregressive process [ 10 ]). Close with our work, Cohea and Payton developed a logistic regression model to understand the factors affecting an NFL game outcome [ 11 ]. The benefit of our model as compared to the one presented by Cohea and Payton [ 11 ] is that the number of exploratory variables we are using is much smaller, making it easy for a fan to follow. Most importantly though we combine our model with statistical bootstrap in order to facilitate future game predictions (something that the model presented in [ 11 ] is not able to perform). Of course, predictive models for NFL games have been developed by major sports networks. For example ESPN has developed the Football Power Index, which is used to make probabilistic predictions for upcoming matchups [ 12 ]. Software companies have also developed their own models (e.g., Cortana from Microsoft [ 13 ]). Nevertheless, these models are proprietary and are not open to the public.
In this study we are first interested in providing a simple model that is able to quantify the impact of various factors on the probability of wining a game of American football. How much does a turnover affect a team’s probability of winning? Can you really win a game after having turned the ball over 5 times? While coaches and players know the qualitative answer to similar questions, the goal of our work is to provide a quantitative answer. For this purpose we use play-by-play data for the last seven seasons of the National Football League (i.e., between 2009 and 2015) and we extract specific team statistics for both the winning and losing teams. We then use the Bradley-Terry regression model [ 14 , 15 ] to quantify the effect and statistical significance of each of these factors on the probability of wining a game of American football. This model is a descriptive one, i.e., it quantifies the impact of several factors on the success of an NFL team. Similar descriptive models can be useful to the coaching staff since they provide an exact quantification of the importance of each aspect of the game. They can also be helpful for the fans—especially the novice ones—for better understanding of the game. Evaluating the obtained model through cross validation provides an accuracy of 84% in predicting the winning team of a matchup.
The above descriptive model is able to provide accurate predictions when the features are known, i.e., when the performance of the two competing teams of a matchup is known. This can be helpful in post analysis of games by comparing the actual outcome of the game with the expected probability of winning the game for each team given their performance. For instance, one can identify “unexpected” wins from teams that underperformed . However, even more challenging, and one of the most intriguing tasks for professional sports analysts, is predicting the winners of the upcoming NFL matchups, which is the second objective of our work. This task can not be completed simply by the regression model that quantifies the impact of various factors on the probability of winning a game. As we will elaborate on in following sections the majority of the features in the developed model includes performance statistics (e.g., total offensive yards, number of interceptions etc.). Hence, the winner prediction problem involves also predicting the features—i.e., the performance of each team—themselves.
Predicting the upcoming performance of a team can be based on its past performance. A factor that makes this task particularly hard for American football is the small number of games during a season, which translates to high uncertainty. Using a central tendency metric—e.g., mean—is not able to fully capture the variability of the performance. To tackle this problem we propose to use statistical bootstrap. In brief, resampling with replacement the features from the past games of a team will allow us to simulate the matchup between the teams several times and obtain a set of winning probabilities that will allow us to predict the final winner of the game. Our approach, FPM , is shown to exhibit an accuracy of approximately 64% over the past 7 seasons, which is comparable to that of the state-of-the-art systems such as Microsoft’s Cortana and ESPN’s FPI. However, given FPM ’s simplicity it should be treated as a baseline estimation. Simply put the output probability of our model can be thought of as an anchor value for the win probability. Further adjustments can be made using information about the specific matchup (i.e., roster, weather forecast etc.), hence, making it possible to significantly outperform existing proprietary systems. We further discuss this point in detail later in this work.
Our work complements the existing literature by contributing a descriptive and easily interpretable model for American football games. We further provide a prediction engine for upcoming matchups based on statistical bootstrap and the developed Bradley-Terry regression model. We would like to emphasize here that our regression model is rather simple and easy to implement. This, in fact, is one of our main contribution, since we demonstrate that such a simple and transparent approach is able to perform on par with state-of-the-art commercial tools for which due to their proprietary nature we have no telling of how complex they are. We view this as a first step towards exploring how we can maintain a simple and interpretable model that at the same time bears high predictive quality. In the rest of the study we present the data and methods that we used (see Section 2 ). We then present our regression model as well as FPM (see Section 3 ). We finally conclude and discuss the implications of our study (see Section 4 ).
2 Materials and Methods
In this section we will present the dataset we used to perform our analysis as well as the different methodological pieces of our analysis.
NFL Dataset: In order to perform our analysis we utilize a dataset collected from NFL’s Game Center for all the games (regular and post season) between the seasons 2009 and 2015. We access the data using the Python nflgame API [ 16 ]. The dataset includes detailed play-by-play information for every game that took place during these seasons. In total, we collected information for 1,792 regular season games and 77 play-off games. Given the small sample for the play-off games and in order to have an equal contribution in our dataset from all the teams we focus our analysis on the regular season games, even though play-off games are by themselves of interest in many perspectives.
Statistical Bootstrap: In order to perform a game outcome prediction, we first need to forecast the performance of each of the contesting teams. However, we only have a (small) set of historic performance data for each team. Furthermore given that the performance of a team is not stable , using a measure of central tendency (e.g., sample mean) does not accurately capture the variability in the data. To overcome this problem we will rely on statistical bootstrap [ 17 ]. Statistical bootstrap is a robust method for estimating the unknown distribution of a population’s statistic when a sample of the population is known. The basic idea of the bootstrapping method is that in the absence of any other information about the population, the observed sample contains all the available information about the underlying distribution. Hence resampling with replacement is the best guide to what can be expected from the population distribution had the latter been available. By generating a large number of such resamples allows us to get a very accurate estimate of the required distribution. Furthermore, for data with dependencies (temporal or otherwise), appropriate block resampling retains any dependencies between data points [ 18 ]. We will utilize bootstrap in the design of FPM .
3.1 Descriptive Model
In this part of our study we will present our descriptive generalized linear model. In particular, we build a Bradley-Terry model to understand the factors that impact the probability of a team winning an American football game. This model will be later used in our future matchup prediction engine, FPM , as we describe in Section 3.2.
Let us denote with W ij the binary random variable that represents the event of home team i winning the game against visiting team j . W ij = 1 if the home team wins the game and 0 otherwise. As aforementioned our model for W ij will provide us with the probability of the home team winning the game given the set of input features, i.e., y = Pr( W ij = 1| z ). The input of this model is vector z that includes features that can potentially impact the probability of a team winning.
The features we use as the input for our model include:
Total offensive yards differential: This feature captures the difference between the home and visiting teams’ total yards (rushing and passing) produced by their offense in the game.
Penalty yards differential: This features captures the differential between the home and visiting teams’ total penalty yards in the game.
Turnovers differential: This feature captures the differential between the total turnovers produced by the teams (i.e., how many times the quarterback was intercepted, fumbles recovered by the opposing team and turns on downs).
Possession time differential: This feature captures the differential of the ball possession time between the home and visiting team.
This ratio captures the offense’s balance between rushing and passing. A perfectly balanced offense will have r = 0.5. We would like to emphasize here that r refers to the actual yardage produced and not to the passing/rushing attempts. The feature included in the model represents the differential between r home and r visiting .
Power ranking differential: This is the current difference in rankings between the home and the visiting teams. A positive differential means that the home team is stronger , i.e., ranks higher, than its opponent. For the power ranking we utilize SportsNetRank [ 19 ], which uses a directed network that represents win-lose relationships between teams. SportsNetRank captures indirectly the schedule strength of a team and it has been shown to provide a better ranking for teams as compared to the simple win-loss percentage.
- PPT PowerPoint slide
- PNG larger image
- TIFF original image
https://doi.org/10.1371/journal.pone.0168716.t001
Based on the Kolmogorov-Smirnov test the features’ ECDFs for the winning and losing teams are statistically different (at the significance level α = 0.01). The probability mass function for the home team advantage is also presented.
https://doi.org/10.1371/journal.pone.0168716.g001
Our basic data analysis above indicates that the distribution of the statistics considered is significantly different for the winning and losing teams. However, we are interested in understanding which of them are good explanatory variables of the probability of winning a game. To further delve into the details, we use our data to train the Bradley-Terry regression model and we obtain the results presented in Table 2 . Note here that, as it might be evident from the aforementioned discussion, we do not explicitly incorporate a feature for distinguishing between the home and the visiting team. Nevertheless, the response variable is the probability of the home team winning, while the features capture the differential of the respective statistics between the home and road team (i.e., the difference is ordered). Therefore, the intercept essentially captures the home team advantage—or lack thereof depending on the sign and significance of the coefficient. In fact, setting all of the explanatory variables equal to zero provides us a response equal to Pr( W ij | 0 ) = 0.555, which is equal to the home team advantage as discussed above. Furthermore, all of the coefficients—except the one for the possession time differential—are statistically significant. However, the impact of the various factors as captured by the magnitude of the coefficients range from weak to strong. For example, the number of total yards produced by the offense seem to have the weakest correlation with the probability of winning a game (i.e., empty yards). On the contrary committing turnovers quickly deteriorates the probability of winning the game and the same is true for an unbalanced offense. Finally, in S1 Text we present a standardized version of our model.
Significance codes: ***: p < .001, **: p < .01, *: p < .05.
https://doi.org/10.1371/journal.pone.0168716.t002
While the direction of the effects for these variables are potentially intuitive for the coaching staff of NFL teams, the benefit of our quantifying approach is that it assigns specific magnitude to the importance of each factor. Clearly the conclusions drawn from the regression cannot and should not be treated as causal. Nevertheless, they provide a good understanding on what is correlated with winning games. For example, if a team wins the turnover battle by 1 it can expect to obtain an approximately 20% gain in the winning probability (all else being constant), while a 10-yard differential in the penalty yardage is correlated with just a 5% difference in the winning probability. Hence, while almost all of the factors considered are statistically significant, some of them appear to be much more important as captured by the corresponding coefficients and potential parts of the game a team could work on. Again, this descriptive model does not provide a cause-effect relationship between the covariates considered and the probability of winning .
Before turning to the FPM predictive engine we would like to further emphasize and reflect on how one should interpret and use these results. For example, one could be tempted to focus on the feature with the coefficient that exhibits the maximum absolute magnitude, that is, the differential of ratio r , and conclude that calling only run plays will increase the probability of winning, since the negative differential with the opposing team will be maximized. However, this is clearly not true as every person with basic familiarity with American football knows. At the same time the regression model is not contradicting itself. What happens is that the model developed—similar to any data driven model—is valid only for the range of values that the input variables cover. Outside of this range, the generalized linear trend might still hold or not. For example, Fig 2 depicts the distribution of ratio r for the winning and losing teams. As we can see our data cover approximately the range r ∈ [0.3, 0.98] and the trend should only be considered valid within this range (and potentially within a small ϵ outside of this range). It is interesting also to observe that the mass of the distribution for the winning teams is concentrated around r ≈ 0.64, while it is larger for the losing teams ( r ≈ 0.8). We also present at the same figure a table with the range that our features cover for both winning and losing teams. Furthermore, to reiterate, the regression model captures merely correlations (rather than cause-effect relations). Given that some of the statistics involved in the features are also correlated themselves (see Fig 3 ) and/or are result of situational football, makes it even harder to identify real causes. For instance, there appears to be a small but statistically significant negative correlation between ratio r and possession time. Furthermore, a typical tactic followed by teams leading in a game towards the end of the fourth quarter is to run the clock out by calling running plays. This can lead to a problem of reverse causality; a reduced ratio r for the leading team as compared to the counterfactual r expected had the team continued its original game-plan, which can artificially deflate the actual contribution of r differential on the probability of winning. Similarly, teams that are trailing in the score towards the end of the game will typically call plays involving long passes in order to cover more yardage faster. However, these plays are also more risky and will lead to turnovers more often, therefore, inflating the turnover differential feature. Nevertheless, this is always a problem when a field experiment cannot be designed and only observational data are available. While we cannot claim causal links between the covariates and the output variable, in what follows we present evidence that can eliminate the presence of reverse causality for the scenarios described above.
Our model is trained within the range of input variable/statistics values on the left table. The figure on the right presents the probability density function for r for the winning and losing instances respectively.
https://doi.org/10.1371/journal.pone.0168716.g002
Correlations between the different variables considered for obtaining the features for FPM . Insignificant correlations are crossed out.
https://doi.org/10.1371/journal.pone.0168716.g003
Reverse Causality: In what follows we examine the potential for reverse causality. To fast forward to our results, we do not find strong evidence for it. To reiterate, one of the problems with any model based on observational data is the direction of the effects captured by the model. For example, in our case teams that are ahead in the score towards the end of the game follow a “conservative” play call, that is, running the football more in order to minimize the probability of a turnover and more importantly use up valuable time on the clock. Hence, this can lead to a decreasing ratio r . Therefore, the negative coefficient for the r differential in our regression model might be capturing reverse causality/causation. Winning teams artificially decrease r due to conservative play calling at the end of the game. Similarly, teams that are behind in score towards the end of the game follow a more “risky” game plan and hence, this might lead to more turnovers (as compared to the other way around).
One possible way to explore whether this is the case is to examine how the values of these two statistics change over the course of the game. We begin with ratio r . If the reverse causation hypothesis were true, then the ratio r for the winning team of a game would have to reduce over the course of the game. In order to examine this hypothesis, we compute the ratio r at the end of each quarter for both the winning and losing teams. Fig 4 presents the results. As we can see during the first quarter there is a large variability for the value of r as one might have expected mainly due to the small number of drives. However, after the first quarter it seems that the value of r is stabilized. There is a slight decrease (increase) for the winning (losing) team during the fourth quarter but this change is not statistically significant. Therefore, we can more confidently reject the existence of reverse causality for ratio r .
Ratio r is stable after the first quarter for both winning (left figure) and losing (right figure) teams, allowing us to reject the reverse causation hypothesis for r .
https://doi.org/10.1371/journal.pone.0168716.g004
We now focus our attention on the turnovers and the potential reverse causation with respect to this feature. In order to examine this hypothesis, we obtain from our data the time within the game (at the minute granularity) that turnovers were committed by the winning and losing teams. We then compare the paired difference for the turnover differential until the end of the third quarter for each game. Our results show that the winning teams commit fewer turnovers than their losing opponents by the end of the third quarter ( p -value < 0.01), further supporting that avoiding turnovers will ultimately lead to a win. Of course, as we can see from Fig 5 , there is a spike of turnovers towards the end of each half (and smaller spikes towards the end of each quarter). These spikes can be potentially explained from the urgency to score since either the drive will stop if the half ends or the game will be over respectively. However, regardless of the exact reasons for these spikes, the main point is that by committing turnovers, either early in the game (e.g., during the first three quarters) or late, the chances of winning the game are significantly reduced.
Turnovers spike towards the end of each quarter, with the highest density appearing during the two-minute warning.
https://doi.org/10.1371/journal.pone.0168716.g005
In conclusion, our model provides quantifiable and actionable insights but they need to be carefully interpreted when designing play actions based on it.
3.2 FPM Prediction Engine
We now turn our attention on how we can use the above model to predict the outcome of a future game. In a realistic setting, in order to be able to apply this regression model we will need to provide as an input the team statistics/features. This is by itself a separate prediction problem, namely, a team performance prediction problem. Hence, we begin by evaluating the prediction performance of the Bradley-Terry regression model itself using traditional machine learning evaluation methods. In particular, we evaluate the prediction accuracy of our model through cross validation. In this way we do not need to predict the value of the features but we explore the accuracy of the pure regression model. Using 10-fold cross validation we obtain an accuracy of 84.03% ± 0.35% . To reiterate this performance is conditional to the input features being known. From the inputs required for our model only two are known before the matchup, namely, the home team (which will allow us to formulate the response variable and the rest of the features appropriately) and the SportsNetRank differential. Thus, how can we predict the rest of the features, since in a realistic setting we will not know the performance of each team beforehand? Simply put, our FPM prediction engine will need to first estimate the two teams statistics/features (i.e., total yards, penalty yards, etc.) and then use the Bradley-Terry regression model to predict the winning team.
The proposed prediction engine consists of 3 modules; a bootstrap module, a regression module and a statistical test module.
https://doi.org/10.1371/journal.pone.0168716.g006
Delving more into the evaluation of our predictive engine we present the accuracy for each season in Table 3 . We also provide the accuracy of a baseline system, where the winner of a game is predicted to be the team with the better running win-loss percentage through the current week. If two teams have the same win-loss percentage the home team is chosen as the winner since there is a slight winning bias for the home team as we have seen earlier. Note here that the baseline is very similar to the way that the league ranks the teams and decides on who will qualify for the playoffs (excluding our tie-breaker process and the league’s rules with respect to the divisions). As we can see our predictive engine improves over the baseline by approximately 9%.
FPM outperforms the baseline prediction based on win-loss standings every season in our dataset. The overall accuracy of our system is 63.4%.
https://doi.org/10.1371/journal.pone.0168716.t003
One of the reasons we utilize bootstrap in our prediction system is to better capture the variability of the teams’ performances. As one might expect this variability is better revealed as the season progresses. During a stretch of few games it is highly probable to have a team over/under-perform [ 22 ]. Hence, the bootstrap module during the beginning of the season might not perform as accurately as during the end of the season. In order to examine this we calculate the accuracy of our prediction system focusing on games that took place during specific weeks in every season. Fig 7 presents our results, where we see that there is an increasing trend as the season progresses.
During the last part of the season the bootstrap engine can exploit the variability of a team’s performance better, hence, providing better prediction accuracy. The linear trend slope is 0.01 (p-value<0.05, R 2 = 0.41).
https://doi.org/10.1371/journal.pone.0168716.g007
Finally, we examine the accuracy of FPM ’s predicted probabilities. In order to evaluate this we would ideally want to have the game played several times. If the favorite team were given a 75% probability of winning, then if the game was played 100 times we would expect the favorite to win 75 of them. However, we cannot have the game play out more than once and hence in order to evaluate the accuracy of the probabilities we will use all the games in our dataset. In particular, if the predicted probabilities were accurate, when considering all the games where the favorite was predicted to win with a probability of x %, then the favorite should have won in x % of these games. Given the continuous nature of the probabilities we quantize them into groups that cover a 5% probability range (with only exception being the range (90%, 100%], since there are very few games in the corresponding sub-groups). Fig 8 presents on the y-axis the fraction of games where the predicted favorite team won, while the x-axis corresponds to the predicted probability of win for the favorite. As we can see the data points—when considering their 95% confidence intervals—fall on the y = x axis, which translates to an accurate probability inference. The corresponding linear regression provides a slope with a 95% confidence interval of [0.76, 1.16] ( R 2 = 0.94), which essentially means that we cannot reject the null hypothesis that our data fall on the line y = x where the slope is equal to 1.
The win probability provided by our model is in alignment with the fraction of the games won by the favorite for the corresponding win probability.
https://doi.org/10.1371/journal.pone.0168716.g008
4 Discussion and Conclusions
Finally, the models themselves can be helpful to many different involved entities associated with the sport. For example, it can facilitate better understanding of the game by novice fans. The impact and importance of ratio r will allow the newcoming fans to appreciate the running game. Similarly, agents and players can use knowledge obtained by similar models for negotiating purposes. It is well-known that running backs are among the least paid players in an NFL roster for a number of reasons (e.g., high risk of serious injuries etc.). Nevertheless, they are extremely important for the success of a team as our model indicates. Moreover, our descriptive regression model can be used by media personnel for a post-game analysis. For instance, “surprising” wins can be identified, while critical parts of the game that led to the final results can also be pinpointed.
Supporting Information
S1 text. standardized fpm ..
https://doi.org/10.1371/journal.pone.0168716.s001
Author Contributions
- Conceptualization: KP.
- Data curation: KP.
- Formal analysis: KP EP.
- Investigation: KP.
- Methodology: KP EP.
- Project administration: KP EP.
- Resources: KP EP.
- Software: KP.
- Supervision: KP.
- Validation: KP.
- Visualization: KP.
- Writing – original draft: KP EP.
- 1. Lamb C, Hair J, McDaniel C (2012) Essentials of Marketing. ISBN-13: 978-0538478342.
- 2. Economist T (2015). Game theory in american football: Defending the indefensible. http://www.economist.com/blogs/gametheory/2015/02/game-theory-american-football . Accessed: 2016-01-12.
- 3. Clark T, Johnson A, Stimpson A (2013) Going for three: Predicting the likelihood of field goal success with logistic regression. In: The 7th Annual MIT Sloan Sports Analytics Conference.
- View Article
- Google Scholar
- 5. Warner J (2010) Predicting margin of victory in nfl games: Machine learning vs. the las vegas line. Technical Report.
- 7. Hochstedler J (2016) Finding the open receiver: A quantitative geospatial analysis of quarterback decision-making. In: MIT Sloan Sports Analytics Conference.
- PubMed/NCBI
- 12. ESPN (2016). A guide to nfl fpi. http://www.espn.com/blog/statsinfo/post/_/id/123048/a-guide-to-nfl-fpi . Accessed: 2016-10-30.
- 13. Bing M (2016). Looking ahead with bing. http://www.bing.com/explore/predicts . Accessed: 2016-10-30.
- 15. Agresti A (2007) An introduction to categorical data analysis. Wiley series in probability and statistics. Hoboken (N.J.): Wiley-Interscience. https://doi.org/10.1002/0470114754
- 16. (2012). Nfl game center api. https://github.com/BurntSushi/nflgame . Accessed: 2016-01-12.
- 17. Efron B, Tibishirani R (1993) An Introduction to the Bootstrap. Chapman and Hall/CRC. https://doi.org/10.1007/978-1-4899-4541-9
- 19. Pelechrinis K, Papalexakis E, Faloutsos C (2016) Sportsnetrank: Network-based sports team ranking. In: ACM SIGKDD Workshop on Large Scale Sports Analytics.
- 20. Tower N (2016). Cortana predictions. https://www.firstscribe.com/blog/bing-predicts-looks-average-in-nfl-week-17-wildcard-weekend-preview/ . Accessed: 2016-02-12.
- 21. Nerd FF (2015). Nfl picks accuracy leaderboard. http://www.fantasyfootballnerd.com/nfl-picks/accuracy/ . Accessed: 2016-01-12.
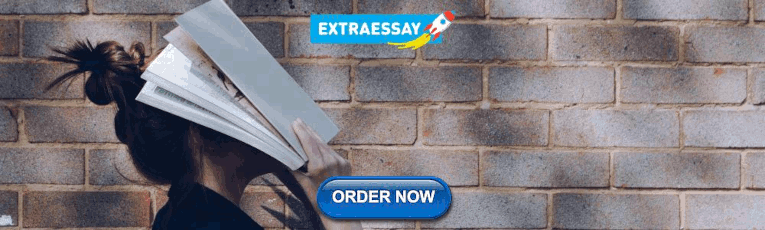
The Origins of the NFL and the Super Bowl
- First Online: 17 November 2020
Cite this chapter
- Yvan J. Kelly 6 ,
- David Berri 7 &
- Victor A. Matheson 8
Part of the book series: Palgrave Pivots in Sports Economics ((PAPISE))
478 Accesses
1 Altmetric
Professional football grew from the popularity of the collegiate game. The NFL began as a small, regional league and grew to become the preeminent sport in the United States. As it grew in size and financial stability, the NFL faced several challenges from rival leagues. In 1966, the NFL agreed to slowly merge with the AFL and to establish a World Championship game. That championship game, now known as the Super Bowl, is the most watched televised event in the country. The game has grown to become a massive event and very big business.
This is a preview of subscription content, log in via an institution to check access.
Access this chapter
- Available as EPUB and PDF
- Read on any device
- Instant download
- Own it forever
- Durable hardcover edition
- Dispatched in 3 to 5 business days
- Free shipping worldwide - see info
Tax calculation will be finalised at checkout
Purchases are for personal use only
Institutional subscriptions
In college football, while the election process was eventually moved to after the bowl games, the practice of voting on a national champion stayed in place until the formation of the Bowl Coalition in 1992.
It remains a shocking surprise that the team with the league’s most racist owner ended up with the what was, until 2020, the league’s most racist mascot.
Indeed, one sign of just how significant the game is in the United States is the fact that it almost single-handedly keeps the Roman numeral system alive in modern America.
Such is the status of the game that even the commercials can become national phenomena. The famous “1984” Apple ad, directed by Oscar nominated director Ridley Scott, aired just once during Super Bowl XVIII, but is widely credited as ushering in an age of elaborate Super Bowl commercials and has been the subject of numerous homages over the years ranging from the popular television show Futurama to the video game Fortnite (Hiltzik 2017 ). The tagline “Where’s the Beef” from a Wendy’s commercial from the same year became such a popular national catchphrase (or overused cliché depending on your particular point of view) that it made it into the Democratic presidential primary debate between Vice President Walter Mondale and Senator Gary Hart later that year.
Braunwart, B., & Carroll, B. (1984). Blondy Wallace and the Biggest Football Scandal Ever. Pro Football Researchers Association, 5, 1–16.
Google Scholar
Breech, J. (2020, January 23). Super Bowl 2020: Chiefs and 49ers Will Get 35 Percent of Tickets, Here’s How the Rest Are Distributed. CBS Sports. Retrieved from https://www.cbssports.com/nfl/news/super-bowl-2020-chiefs-and-49ers-will-get-35-percent-of-tickets-heres-how-the-rest-are-distributed/ .
Crepeau, R. C. (2014). NFL Football: A History of America’s New National Pastime . Urbana: University of Illinois Press.
Felser, L. (2008). The Birth of the New NFL: How the 1966 NFL/AFL Merger Transformed Pro Football . Guilford, CT: The Lyons Press.
Hartmann, W. R. & Klapper, D. (2017). Super Bowl Ads. Marketing Science, 37 (1), 78–96.
Hiltzik, M. (2017, January 25). A Reminder That Apple’s ‘1984’ Ad Is the Only Great Super Bowl Commercial Ever—And It’s Now 33 Years Old. Los Angeles Times . Retrieved from https://www.latimes.com/business/hiltzik/la-fi-hiltzik-1984-super-bowl-20170125-story.html .
Kerschbaumer, K. (2020, January 28). Super Bowl LIV: Inside the Numbers. Sports Video Group. Retrieved from https://www.sportsvideo.org/2020/01/28/super-bowl-liv-inside-the-numbers/ .
Leeds, M., Von Allmen, P., & Matheson, V. (2018). The Economics of Sports (6th ed.). New York, NY: Routledge.
Book Google Scholar
MacCambridge, M. (2005). America’s Game: The Epic Story of How Pro Football Captured a Nation . New York, NY: Anchor Books.
NFL History. (2020). Super Bowl Winners . ESPN. Retrieved from http://www.espn.com/nfl/superbowl/history/winners .
Pro Football Hall of Fame. (2020). Birth of Pro Football. Pro Football Hall of Fame. Retrieved from https://www.profootballhof.com/football-history/birth-of-pro-football/ .
Remember the AFL. (2020). Minority Players and the American Football League . Retrieved from http://www.remembertheafl.com/MinorityPlayers.htm .
Roos, D., & Klosowski, T. (2010, October 13). How to Get Super Bowl Tickets. HowStuffWorks . Retrieved from https://entertainment.howstuffworks.com/how-to-get-super-bowl-tickets.htm .
Ross, C. (1999). Outside the Lines: African Americans and the Integration of the National Football League . New York: New York University Press.
Shallow, L. (2019, February 1). How Does the NFL Pick Super Bowl Cities? CNN. Retrieved from https://www.cnn.com/2019/02/01/us/how-nfl-picks-super-bowl-cities/index.html .
Steinberg, B. (2019, March 13). CBS, NBC to Swap Super Bowl Broadcasts. Variety. Retrieved from https://variety.com/2019/tv/news/cbs-nbc-swap-super-bowl-1203162667/ .
Stewart, M. (2002). The Super Bowl . New York, NY: Franklin Press.
Surdam, D. G. (2013). Run to Glory and Profits: The Economic Rise of the NFL During the 1950s . Lincoln: University of Nebraska Press.
Veblen, T. (2009). Theory of the Leisure Class . Oxford, UK: Oxford University Press.
Download references
Author information
Authors and affiliations.
Flagler College, St. Augustine, FL, USA
Yvan J. Kelly
Department of Economics and Finance, Southern Utah University, Cedar City, UT, USA
David Berri
Department of Economics and Accounting, College of the Holy Cross, Worcester, MA, USA
Victor A. Matheson
You can also search for this author in PubMed Google Scholar
Corresponding author
Correspondence to Yvan J. Kelly .
Rights and permissions
Reprints and permissions
Copyright information
© 2020 The Author(s), under exclusive license to Springer Nature Switzerland AG
About this chapter
Kelly, Y.J., Berri, D., Matheson, V.A. (2020). The Origins of the NFL and the Super Bowl. In: The Economics of the Super Bowl. Palgrave Pivots in Sports Economics. Palgrave Macmillan, Cham. https://doi.org/10.1007/978-3-030-46370-0_2
Download citation
DOI : https://doi.org/10.1007/978-3-030-46370-0_2
Published : 17 November 2020
Publisher Name : Palgrave Macmillan, Cham
Print ISBN : 978-3-030-46369-4
Online ISBN : 978-3-030-46370-0
eBook Packages : Economics and Finance Economics and Finance (R0)
Share this chapter
Anyone you share the following link with will be able to read this content:
Sorry, a shareable link is not currently available for this article.
Provided by the Springer Nature SharedIt content-sharing initiative
- Publish with us
Policies and ethics
- Find a journal
- Track your research
Academia.edu no longer supports Internet Explorer.
To browse Academia.edu and the wider internet faster and more securely, please take a few seconds to upgrade your browser .
- We're Hiring!
- Help Center
History of Football
- Most Cited Papers
- Most Downloaded Papers
- Newest Papers
- Save to Library
- Last »
- Sociology of Football Follow Following
- Football Culture Follow Following
- Anthropology of Sport Follow Following
- Futebol Follow Following
- Football (soccer) Follow Following
- Antropologia Social Follow Following
- Sports History Follow Following
- Physcial Education Follow Following
- Petrologi Follow Following
- Economics of Football (soccer) Follow Following
Enter the email address you signed up with and we'll email you a reset link.
- Academia.edu Publishing
- We're Hiring!
- Help Center
- Find new research papers in:
- Health Sciences
- Earth Sciences
- Cognitive Science
- Mathematics
- Computer Science
- Academia ©2024
Evolution of soccer as a research topic
Affiliation.
- 1 James R. Urbaniak, MD Sports Sciences Institute Duke Health, Durham, North Carolina. Electronic address: [email protected].
- PMID: 32599029
- DOI: 10.1016/j.pcad.2020.06.011
Soccer has not only the largest number of worldwide participants, it is also the most studied sport, with nearly 14,000 citations listed on Pubmed and nearly 60% more articles than the next most studied sport. Research about soccer was limited until the late 1970s when exponential growth began; approximately 98% of all soccer-related research publications have occurred since 1980. This vast repository of soccer research shows trends in various major (e.g., 'sex' or 'age group' or 'performance' or 'injury') and specialty (e.g., agility, deceleration, elbow-head impact injuries, behavior) topics. Examining trends of the various topics provides insights into which subjects have come in and out of favor as well as what topics or demographics have been neglected and worthy of inquiry. A further examination can be used by students to learn the most productive researchers, which programs have a strong history of inquiry, and what journals have demonstrated a commitment to publishing research on soccer.
Keywords: Association football; Pubmed; Research history.
Copyright © 2020 Elsevier Inc. All rights reserved.
Publication types
- Age Factors
- Biomedical Research / trends*
- Cardiorespiratory Fitness
- Health Status
- Middle Aged
- Periodicals as Topic / trends*
- Sex Factors
- Soccer / trends*
- Time Factors
- Young Adult
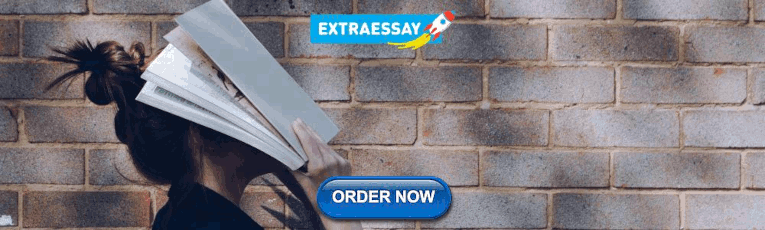
COMMENTS
The most significant aim of the research was to explore football fans' perceptions of increasing DVA rates following football games. Although research has acknowledged the increased popularity of ...
Gavin Kitching is Emeritus Professor of Politics at the University of New South Wales, Sydney, Australia, and Visiting Research Fellow at the International Centre for Sports History and Culture, De Montfort University, Leicester. His current research on the origins of football is the first stage of an attempt to write a social history of his native North-East of England through the lens of ...
ABSTRACT. Background:Football is evolving in many ways, including technical and physical demands as well as the scientific research underpinning and providing many recommendations to practitioners on how to optimise performance of players and by default, team performance.Evolution is a natural process and necessary to grow and develop and research into football is no different.
Abstract. Recent scholarship on the origins of association football has been marked by a highly ideological debate on its 'class' nature. The traditional story - of a game created by 'gentlemen' but taken up, and ultimately dominated, by 'ruffians' - has been challenged by a revisionist account which presents football as an ancient 'people's' or 'plebeian' game ...
Probing and learned, NFL Football tells an epic American success story peopled by larger-than-life figures and driven by ambition, money, sweat, and dizzying social and technological changes. 978--252-09653-2. History. Founded as an obscure sports body, the National Football League has grown into a multi-billion-dollar colossus and cultural ...
Academia.edu is a platform for academics to share research papers. The Origins of Football: History, Ideology and the Making of 'The People's Game ... "FEATURE: MODERN SPORT: SOCIETY AND COMPETITION The Origins of Football: History, Ideology and the Making of 'The People's Game'." History Workshop Journal 79, no. 1 (March 1, 2015): 127-53
While American football is viewed mainly as a physical game—and it surely is—at the same time it is probably one of the most strategic sports games, a fact that makes it appealing even to an international crowd [ 1 ]. This has led to people analyzing the game with the use of data analytics methods and game theory.
From its humble and financially tenuous beginnings in 1920, the National Football League has grown to become the premier spectator sport of the United States (Crepeau 2014).Likewise, its championship game, the Super Bowl, has become North America's most watched sporting event (Stewart 2002).The NFL's growth in popularity is demonstrated by the size of its weekly television viewership and ...
The purpose of this paper, therefore, is threefold: (1) to critically revisit and reread football studies as a field and the scholarship of the analogue moment; (2) to outline the need for an evolution to digital football studies, to appraise current work in the area and consolidate the existing knowledge base; and (3) to consider some ...
Islam & Rahman (2021) indicate that the first form of the game (football) for which there is scientific evidence was an exercise from a military manual dating back to the 2nd and 3rd centuries BC ...
Paper presented at the Annual conference of ASMCF, held at the Université de Paris XIII, Villetaneuse, 4-6 September 2003. It owes much to the research for Chapter 3 of my book Football in France. A Cultural History (Oxford: Berg, 2003), entitled 'Towns and cities: a socio-economic geography of French football clubs'.
about the early history of pro football. [See PFRA Annual, 1986 - Ed.] When Rooney read the paper, he realized he had a piece of research of incalculable importance. Unfortunately, by that time the man had departed. As best Rooney could recall, the visitor's name was Nelson Ross. But although Rooney tried to track down Ross, the
Football Fortunes by Frank P. Jozsa. Call Number: GV955.5.N35 J69 2010. ISBN: 9780786446414. Published/Created: 2010-02-24. This author has written a number of books on the business end of sports but this title is particular to how the business end of the NFL developed into a billion dollar industry.
The breadth of topics featured and methodologies deployed in football research and scholarship is an exciting time for the field and also prompts us to continue to reflect, understand and inform future research. ... Our call for papers asked scholars to submit papers that spoke to the management, marketing or governance of association football ...
Recent scholarship on the origins of association football has been marked by a highly ideological debate on its 'class' nature. The traditional story - of a game created by 'gentlemen' but taken up, and ultimately dominated, by 'ruffians' - has been challenged by a revisionist account which presents football as an ancient 'people's' or 'plebeian' game, briefly ...
Studies analyzing >1 sport were considered if football was included and was a primary focus of discussion in the paper. If inclusion of a study was in question, the full article was obtained and reviewed independently by 2 authors (J.R.P. and M.L.M.) to decide on inclusion or exclusion. ... particularly if orthopaedic/football research is a ...
Football, besides having the largest number of participants, is also the most extensively studied sport, with nearly 14,000 references in the PubMed database. Research on football was limited ...
There has previously been a fair amount of research in statistical modelling and forecasting in relation to football. The prediction models are generally either based on detailed statistics of actions on the pitch [ 6 - 9 ] or on a prior ranking system which estimates the relative strengths of the teams [ 10 - 12 ].
Football is one of the most popular and widely played sports in the world, involving two teams of 11 players who use their feet, head, or body to move the ball into the opponent's goal. Learn about the history, rules, and significant players of football from Britannica, the trusted source of knowledge and information.
This vast repository of soccer research shows trends in various major (e.g., 'sex' or 'age group' or 'performance' or 'injury') and specialty (e.g., agility, deceleration, elbow-head impact injuries, behavior) topics. Examining trends of the various topics provides insights into which subjects have come in and out of favor as well as what ...
Important events, moments and memories in the history of football have elicited a wide variety of writings - which range from autobiographical and biographical works to journalistic and scholarly pieces. Reminiscing memorable moments, recounting affective memories or signifying crucial events have been the hallmarks of these forms of writings.
On the occurrence of haematoma of the ear in football players 2 … soccer paper: 1889: Ruptured bowel in a match against Grimsby Town 3 … Football-related death: ... Maybe a student wants to continue their education at a program with an extensive history of sport science research about soccer. Another student might want to study under a ...
That first set of draft rules for football adopted by the FA in. 1863 allowed a free kick at goal after 'fair catch' of the ball claims it by making a mark with his heel'), throwing of the ball. mate, and running with the ball in hand after a fair catch or a catch. ball 'on first bound'.
India Football: The Rising Billion. April 2013. Conference: Soccerex Global Forum 2013. At: Manchester. Authors: Amit Mantri. Federation of Indian Chambers of Commerce and Industry. Citations (2 ...