DigitalCommons@UNMC
Home > Eppley Institute > Theses & Dissertations
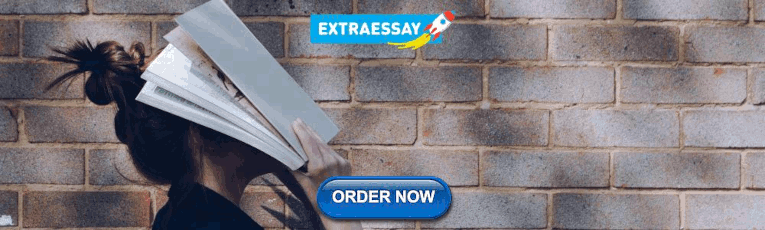
Theses & Dissertations: Cancer Research
Theses/dissertations from 2024 2024.
Novel Spirocyclic Dimer (SpiD3) Displays Potent Preclinical Effects in Hematological Malignancies , Alexandria Eiken
Chemotherapy-Induced Modulation of Tumor Antigen Presentation , Alaina C. Larson
Dying Right: Supporting Anti-Cancer Therapy Through Immunogenic Cell Death , Elizabeth Schmitz
Therapeutic Effects of BET Protein Inhibition in B-cell Malignancies and Beyond , Audrey L. Smith
Targeting KSR1 to inhibit stemness and therapy resistance , Heidi M. Vieira
Identifying the Molecular Determinants of Lung Metastatic Adaptation in Prostate Cancer , Grace M. Waldron
Identification of Mitotic Phosphatases and Cyclin K as Novel Molecular Targets in Pancreatic Cancer , Yi Xiao
Theses/Dissertations from 2023 2023
Development of Combination Therapy Strategies to Treat Cancer Using Dihydroorotate Dehydrogenase Inhibitors , Nicholas Mullen
Overcoming Resistance Mechanisms to CDK4/6 Inhibitor Treatment Using CDK6-Selective PROTAC , Sarah Truong
Theses/Dissertations from 2022 2022
Omics Analysis in Cancer and Development , Emalie J. Clement
Investigating the Role of Splenic Macrophages in Pancreatic Cancer , Daisy V. Gonzalez
Polymeric Chloroquine in Metastatic Pancreatic Cancer Therapy , Rubayat Islam Khan
Evaluating Targets and Therapeutics for the Treatment of Pancreatic Cancer , Shelby M. Knoche
Characterization of 1,1-Diarylethylene FOXM1 Inhibitors Against High-Grade Serous Ovarian Carcinoma Cells , Cassie Liu
Novel Mechanisms of Protein Kinase C α Regulation and Function , Xinyue Li
SOX2 Dosage Governs Tumor Cell Identity and Proliferation , Ethan P. Metz
Post-Transcriptional Control of the Epithelial-to-Mesenchymal Transition (EMT) in Ras-Driven Colorectal Cancers , Chaitra Rao
Use of Machine Learning Algorithms and Highly Multiplexed Immunohistochemistry to Perform In-Depth Characterization of Primary Pancreatic Tumors and Metastatic Sites , Krysten Vance
Characterization of Metastatic Cutaneous Squamous Cell Carcinoma in the Immunosuppressed Patient , Megan E. Wackel
Visceral adipose tissue remodeling in pancreatic ductal adenocarcinoma cachexia: the role of activin A signaling , Pauline Xu
Phos-Tag-Based Screens Identify Novel Therapeutic Targets in Ovarian Cancer and Pancreatic Cancer , Renya Zeng
Theses/Dissertations from 2021 2021
Functional Characterization of Cancer-Associated DNA Polymerase ε Variants , Stephanie R. Barbari
Pancreatic Cancer: Novel Therapy, Research Tools, and Educational Outreach , Ayrianne J. Crawford
Apixaban to Prevent Thrombosis in Adult Patients Treated With Asparaginase , Krishna Gundabolu
Molecular Investigation into the Biologic and Prognostic Elements of Peripheral T-cell Lymphoma with Regulators of Tumor Microenvironment Signaling Explored in Model Systems , Tyler Herek
Utilizing Proteolysis-Targeting Chimeras to Target the Transcriptional Cyclin-Dependent Kinases 9 and 12 , Hannah King
Insights into Cutaneous Squamous Cell Carcinoma Pathogenesis and Metastasis Using a Bedside-to-Bench Approach , Marissa Lobl
Development of a MUC16-Targeted Near-Infrared Antibody Probe for Fluorescence-Guided Surgery of Pancreatic Cancer , Madeline T. Olson
FGFR4 glycosylation and processing in cholangiocarcinoma promote cancer signaling , Andrew J. Phillips
Theses/Dissertations from 2020 2020
Cooperativity of CCNE1 and FOXM1 in High-Grade Serous Ovarian Cancer , Lucy Elge
Characterizing the critical role of metabolic and redox homeostasis in colorectal cancer , Danielle Frodyma
Genomic and Transcriptomic Alterations in Metabolic Regulators and Implications for Anti-tumoral Immune Response , Ryan J. King
Dimers of Isatin Derived Spirocyclic NF-κB Inhibitor Exhibit Potent Anticancer Activity by Inducing UPR Mediated Apoptosis , Smit Kour
From Development to Therapy: A Panoramic Approach to Further Our Understanding of Cancer , Brittany Poelaert
The Cellular Origin and Molecular Drivers of Claudin-Low Mammary Cancer , Patrick D. Raedler
Mitochondrial Metabolism as a Therapeutic Target for Pancreatic Cancer , Simon Shin
Development of Fluorescent Hyaluronic Acid Nanoparticles for Intraoperative Tumor Detection , Nicholas E. Wojtynek
Theses/Dissertations from 2019 2019
The role of E3 ubiquitin ligase FBXO9 in normal and malignant hematopoiesis , R. Willow Hynes-Smith
BRCA1 & CTDP1 BRCT Domainomics in the DNA Damage Response , Kimiko L. Krieger
Targeted Inhibition of Histone Deacetyltransferases for Pancreatic Cancer Therapy , Richard Laschanzky
Human Leukocyte Antigen (HLA) Class I Molecule Components and Amyloid Precursor-Like Protein 2 (APLP2): Roles in Pancreatic Cancer Cell Migration , Bailee Sliker
Theses/Dissertations from 2018 2018
FOXM1 Expression and Contribution to Genomic Instability and Chemoresistance in High-Grade Serous Ovarian Cancer , Carter J. Barger
Overcoming TCF4-Driven BCR Signaling in Diffuse Large B-Cell Lymphoma , Keenan Hartert
Functional Role of Protein Kinase C Alpha in Endometrial Carcinogenesis , Alice Hsu
Functional Signature Ontology-Based Identification and Validation of Novel Therapeutic Targets and Natural Products for the Treatment of Cancer , Beth Neilsen
Elucidating the Roles of Lunatic Fringe in Pancreatic Ductal Adenocarcinoma , Prathamesh Patil
Theses/Dissertations from 2017 2017
Metabolic Reprogramming of Pancreatic Ductal Adenocarcinoma Cells in Response to Chronic Low pH Stress , Jaime Abrego
Understanding the Relationship between TGF-Beta and IGF-1R Signaling in Colorectal Cancer , Katie L. Bailey
The Role of EHD2 in Triple-Negative Breast Cancer Tumorigenesis and Progression , Timothy A. Bielecki
Perturbing anti-apoptotic proteins to develop novel cancer therapies , Jacob Contreras
Role of Ezrin in Colorectal Cancer Cell Survival Regulation , Premila Leiphrakpam
Evaluation of Aminopyrazole Analogs as Cyclin-Dependent Kinase Inhibitors for Colorectal Cancer Therapy , Caroline Robb
Identifying the Role of Janus Kinase 1 in Mammary Gland Development and Breast Cancer , Barbara Swenson
DNMT3A Haploinsufficiency Provokes Hematologic Malignancy of B-Lymphoid, T-Lymphoid, and Myeloid Lineage in Mice , Garland Michael Upchurch
Theses/Dissertations from 2016 2016
EHD1 As a Positive Regulator of Macrophage Colony-Stimulating Factor-1 Receptor , Luke R. Cypher
Inflammation- and Cancer-Associated Neurolymphatic Remodeling and Cachexia in Pancreatic Ductal Adenocarcinoma , Darci M. Fink
Role of CBL-family Ubiquitin Ligases as Critical Negative Regulators of T Cell Activation and Functions , Benjamin Goetz
Exploration into the Functional Impact of MUC1 on the Formation and Regulation of Transcriptional Complexes Containing AP-1 and p53 , Ryan L. Hanson
DNA Polymerase Zeta-Dependent Mutagenesis: Molecular Specificity, Extent of Error-Prone Synthesis, and the Role of dNTP Pools , Olga V. Kochenova
Defining the Role of Phosphorylation and Dephosphorylation in the Regulation of Gap Junction Proteins , Hanjun Li
Molecular Mechanisms Regulating MYC and PGC1β Expression in Colon Cancer , Jamie L. McCall
Pancreatic Cancer Invasion of the Lymphatic Vasculature and Contributions of the Tumor Microenvironment: Roles for E-selectin and CXCR4 , Maria M. Steele
Altered Levels of SOX2, and Its Associated Protein Musashi2, Disrupt Critical Cell Functions in Cancer and Embryonic Stem Cells , Erin L. Wuebben
Theses/Dissertations from 2015 2015
Characterization and target identification of non-toxic IKKβ inhibitors for anticancer therapy , Elizabeth Blowers
Effectors of Ras and KSR1 dependent colon tumorigenesis , Binita Das
Characterization of cancer-associated DNA polymerase delta variants , Tony M. Mertz
A Role for EHD Family Endocytic Regulators in Endothelial Biology , Alexandra E. J. Moffitt
Biochemical pathways regulating mammary epithelial cell homeostasis and differentiation , Chandrani Mukhopadhyay
EPACs: epigenetic regulators that affect cell survival in cancer. , Catherine Murari
Role of the C-terminus of the Catalytic Subunit of Translesion Synthesis Polymerase ζ (Zeta) in UV-induced Mutagensis , Hollie M. Siebler
LGR5 Activates TGFbeta Signaling and Suppresses Metastasis in Colon Cancer , Xiaolin Zhou
LGR5 Activates TGFβ Signaling and Suppresses Metastasis in Colon Cancer , Xiaolin Zhou
Theses/Dissertations from 2014 2014
Genetic dissection of the role of CBL-family ubiquitin ligases and their associated adapters in epidermal growth factor receptor endocytosis , Gulzar Ahmad
Strategies for the identification of chemical probes to study signaling pathways , Jamie Leigh Arnst
Defining the mechanism of signaling through the C-terminus of MUC1 , Roger B. Brown
Targeting telomerase in human pancreatic cancer cells , Katrina Burchett
The identification of KSR1-like molecules in ras-addicted colorectal cancer cells , Drew Gehring
Mechanisms of regulation of AID APOBEC deaminases activity and protection of the genome from promiscuous deamination , Artem Georgievich Lada
Characterization of the DNA-biding properties of human telomeric proteins , Amanda Lakamp-Hawley
Studies on MUC1, p120-catenin, Kaiso: coordinate role of mucins, cell adhesion molecules and cell cycle players in pancreatic cancer , Xiang Liu
Epac interaction with the TGFbeta PKA pathway to regulate cell survival in colon cancer , Meghan Lynn Mendick
Theses/Dissertations from 2013 2013
Deconvolution of the phosphorylation patterns of replication protein A by the DNA damage response to breaks , Kerry D. Brader
Modeling malignant breast cancer occurrence and survival in black and white women , Michael Gleason
The role of dna methyltransferases in myc-induced lymphomagenesis , Ryan A. Hlady
Design and development of inhibitors of CBL (TKB)-protein interactions , Eric A. Kumar
Pancreatic cancer-associated miRNAs : expression, regulation and function , Ashley M. Mohr
Mechanistic studies of mitochondrial outer membrane permeabilization (MOMP) , Xiaming Pang
Novel roles for JAK2/STAT5 signaling in mammary gland development, cancer, and immune dysregulation , Jeffrey Wayne Schmidt
Optimization of therapeutics against lethal pancreatic cancer , Joshua J. Souchek
Theses/Dissertations from 2012 2012
Immune-based novel diagnostic mechanisms for pancreatic cancer , Michael J. Baine
Sox2 associated proteins are essential for cell fate , Jesse Lee Cox
KSR2 regulates cellular proliferation, transformation, and metabolism , Mario R. Fernandez
Discovery of a novel signaling cross-talk between TPX2 and the aurora kinases during mitosis , Jyoti Iyer
Regulation of metabolism by KSR proteins , Paula Jean Klutho
The role of ERK 1/2 signaling in the dna damage-induced G2 , Ryan Kolb
Regulation of the Bcl-2 family network during apoptosis induced by different stimuli , Hernando Lopez
Studies on the role of cullin3 in mitosis , Saili Moghe
Characteristics of amyloid precursor-like protein 2 (APLP2) in pancreatic cancer and Ewing's sarcoma , Haley Louise Capek Peters
Structural and biophysical analysis of a human inosine triphosphate pyrophosphatase polymorphism , Peter David Simone
Functions and regulation of Ron receptor tyrosine kinase in human pancreatic cancer and its therapeutic applications , Yi Zou
- Eppley Institute Website
- McGoogan Library
Advanced Search
- Notify me via email or RSS
- Collections
- Disciplines
Author Corner
Home | About | FAQ | My Account | Accessibility Statement
Privacy Copyright
IEEE Account
- Change Username/Password
- Update Address
Purchase Details
- Payment Options
- Order History
- View Purchased Documents
Profile Information
- Communications Preferences
- Profession and Education
- Technical Interests
- US & Canada: +1 800 678 4333
- Worldwide: +1 732 981 0060
- Contact & Support
- About IEEE Xplore
- Accessibility
- Terms of Use
- Nondiscrimination Policy
- Privacy & Opting Out of Cookies
A not-for-profit organization, IEEE is the world's largest technical professional organization dedicated to advancing technology for the benefit of humanity. © Copyright 2024 IEEE - All rights reserved. Use of this web site signifies your agreement to the terms and conditions.
Information
- Author Services
Initiatives
You are accessing a machine-readable page. In order to be human-readable, please install an RSS reader.
All articles published by MDPI are made immediately available worldwide under an open access license. No special permission is required to reuse all or part of the article published by MDPI, including figures and tables. For articles published under an open access Creative Common CC BY license, any part of the article may be reused without permission provided that the original article is clearly cited. For more information, please refer to https://www.mdpi.com/openaccess .
Feature papers represent the most advanced research with significant potential for high impact in the field. A Feature Paper should be a substantial original Article that involves several techniques or approaches, provides an outlook for future research directions and describes possible research applications.
Feature papers are submitted upon individual invitation or recommendation by the scientific editors and must receive positive feedback from the reviewers.
Editor’s Choice articles are based on recommendations by the scientific editors of MDPI journals from around the world. Editors select a small number of articles recently published in the journal that they believe will be particularly interesting to readers, or important in the respective research area. The aim is to provide a snapshot of some of the most exciting work published in the various research areas of the journal.
Original Submission Date Received: .
- Active Journals
- Find a Journal
- Proceedings Series
- For Authors
- For Reviewers
- For Editors
- For Librarians
- For Publishers
- For Societies
- For Conference Organizers
- Open Access Policy
- Institutional Open Access Program
- Special Issues Guidelines
- Editorial Process
- Research and Publication Ethics
- Article Processing Charges
- Testimonials
- Preprints.org
- SciProfiles
- Encyclopedia
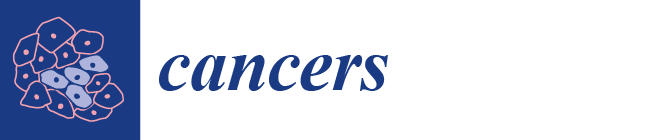
Article Menu
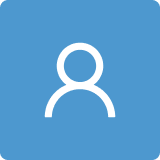
- Subscribe SciFeed
- Recommended Articles
- PubMed/Medline
- Google Scholar
- on Google Scholar
- Table of Contents
Find support for a specific problem in the support section of our website.
Please let us know what you think of our products and services.
Visit our dedicated information section to learn more about MDPI.
JSmol Viewer
Blood-based mrna tests as emerging diagnostic tools for personalised medicine in breast cancer.
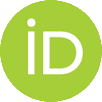
Simple Summary
1. introduction, 2. molecular diagnostic tests and companion diagnostic devices for breast cancer approved by the us food and drug administration (fda), 3. cancer diagnostics based on mrna can offer prognostically useful information beyond dna variation, 4. tissue-based mrna expression assays for breast cancer, 4.1. prosigna breast cancer prognostic gene signature assay (formerly called the pam50 test), 4.2. mammaprint test (also called the 70-gene signature), 4.3. oncotype dx breast recurrence score test, 4.4. breast cancer index, bci, 4.5. endopredict breast cancer prognostic test, 4.6. genesearch breast lymph node (bln) test kit.
Assay Trade Name (Manufacturer) | Number of Genes; Sample Type | Assay Indicated For | Description | Methodology/ Platform | FDA Numbers; References |
---|---|---|---|---|---|
Prosigna Breast Cancer Prognostic Gene Signature Assay (Veracyte, Inc.) | 50 genes; BC tissue (formalin-fixed paraffin embedded—FFPE) | HR+, LN-negative or 1–3 positive nodes, stage I or II cancers | Classification of intrinsic BC subtypes; prognostic; recurrence risk assessment; guides adjuvant endocrine and chemotherapy in postmenopausal patients | nCounter Dx Analysis System (mRNA hybridization to DNA probes) | FDA (K130010); [ , ] |
MammaPrint (Agendia, Inc.) | 70 genes; BC tissue (FFPE or fresh) | HR+ or HR−, LN-negative or 1–3 positive nodes, stage I or II cancers, ≤5.0 cm | Prognostic; recurrence risk assessment; guides adjuvant endocrine and chemotherapy in patients >50 or postmenopausal patients | Microarray-based assay; also available as a targeted RNA next-generation sequencing assay | FDA (K101454, K081092, K080252, K070675); [ , , ] |
Oncotype DX Breast Recurrence Score Test (Genomic Health) | 21 genes (16 cancer-related and 5 reference genes); BC tissue (FFPE) | HR+/HER2−, LN-negative or 1–3 positive nodes, stage I, II or IIIa cancers, ≤5.0 cm | Prognosticates distant recurrence; predicts chemotherapy benefit/guides adjuvant endocrine and chemotherapy in postmenopausal or premenopausal patients | qRT-PCR-based assay | [ , , , , ] |
Breast Cancer Index—BCI (Biotheranostic, Inc.) | 7 genes; BC tissue (FFPE) | HR+, LN-negative or 1–3 positive nodes, stage I–III cancers, invasive BC cases without evidence of recurrence | Prognosticates risk of distant recurrence; predicts likelihood of benefit from extended (>5 years) endocrine therapy; guides adjuvant endocrine and chemotherapy in patients >50 or postmenopausal patients | qRT-PCR-based assay | [ , ] |
EndoPredict (Myriad Genetics) | 12 genes (8 BC-related and 4 reference genes); BC tissue (FFPE) | HR+/HER2−, LN-negative or 1–3 positive nodes, tumour size T1–T3, grade 1–3 | Predicts distant recurrence at 10 years (and up to 15 years); guides adjuvant endocrine and chemotherapy in patients >50 or postmenopausal patients; identifies premenopausal patients who do not need chemotherapy | qRT-PCR-based assay | [ , , ] |
GeneSearch Breast Lymph Node (BLN) Test Kit (Veridex, LLC.) | 3 genes (2 metastasis-related and 1 reference); lymph node(s) removed during surgery | Patients with invasive BC, scheduled for sentinel lymph node dissection | BC metastasis detection | qRT-PCR-based assay | FDA: P060017 S001–S004, [ ] |
5. The Advantages of Using Peripheral Blood (i.e., a Blood-Based Liquid Biopsy) for Cancer Diagnostics
6. research focusing on bc-specific transcriptional profiles in peripheral blood has established their diagnostic and prognostic value, 6.1. identification of blood predictor genes that can distinguish bc patients from other cancer patients and healthy subjects, 6.2. identification of expression signatures that can distinguish between breast cancer and benign breast disease, 6.3. identification of blood expression signatures that can distinguish between lymph-node positive and negative bc, 6.4. blood gene expression alterations years before bc diagnosis, 6.5. peripheral blood transcriptomics may open the way to novel immune bc subtyping, 7. commercially available blood-based mrna tests for breast cancer, 7.1. hereditary breast cancer predisposition, 7.2. breast cancer screening, 8. current status and future perspectives, 8.1. the rna methods used for breast cancer and their expanding diagnostic potential, 8.2. the capability of supplemental blood mrna analyses to improve the diagnostic outcomes of cancer dna testing, 8.3. the potential of blood rna analyses to enable early cancer detection, 8.4. the mrna-based applications in research and under development for personalised bc management, 8.5. the issue of centralised testing and restricted availability of mrna diagnostics for bc, 8.6. the centralisation of testing and the associated individualised standardisation and validation of rna-based diagnostic assays, 8.7. the technical challenges associated with mrna diagnostic testing, 9. conclusions, supplementary materials, author contributions, conflicts of interest.
- Dalmartello, M.; La Vecchia, C.; Bertuccio, P.; Boffetta, P.; Levi, F.; Negri, E.; Malvezzi, M. European cancer mortality predictions for the year 2022 with focus on ovarian cancer. Ann Oncol. 2022 , 33 , 330–339. [ Google Scholar ] [ CrossRef ] [ PubMed ]
- International Agency for Research on Cancer, WHO. Cancer Today. 2022. Available online: https://gco.iarc.fr/today/home. (accessed on 19 October 2022).
- Szymiczek, A.; Lone, A.; Akbari, M.R. Molecular intrinsic versus clinical subtyping in breast cancer: A comprehensive review. Clin. Genet. 2021 , 99 , 613–637. [ Google Scholar ] [ PubMed ]
- Čelešnik, H.; Potočnik, U. Peripheral Blood Transcriptome in Breast Cancer Patients as a Source of Less Invasive Immune Biomarkers for Personalized Medicine, and Implications for Triple Negative Breast Cancer. Cancers 2022 , 14 , 591. [ Google Scholar ]
- McAndrew, N.P.; Finn, R.S. Clinical Review on the Management of Hormone Receptor-Positive Metastatic Breast Cancer. JCO Oncol. Pract. 2022 , 18 , 319–327. [ Google Scholar ] [ CrossRef ] [ PubMed ]
- Ferrario, C.; Christofides, A.; Joy, A.A.; Laing, K.; Gelmon, K.; Brezden-Masley, C. Novel Therapies for the Treatment of HER2-Positive Advanced Breast Cancer: A Canadian Perspective. Curr. Oncol. 2022 , 29 , 2720–2734. [ Google Scholar ] [ CrossRef ]
- Bianchini, G.; De Angelis, C.; Licata, L.; Gianni, L. Treatment landscape of triple-negative breast cancer—expanded options, evolving needs. Nat. Rev. Clin. Oncol. 2022 , 19 , 91–113. [ Google Scholar ] [ CrossRef ]
- Čelešnik, H.S.; Potočnik, U. Immunotherapy in Breast Cancer. In: Encyclopedia. Available online: https://encyclopedia.pub/entry/21561 (accessed on 27 November 2022).
- Skok, K.; Gradišnik, L.; Čelešnik, H.; Milojević, M.; Potočnik, U.; Jezernik, G.; Gorenjak, M.; Sobočan, M.; Takač, I.; Kavalar, R.; et al. MFUM-BrTNBC-1, a Newly Established Patient-Derived Triple-Negative Breast Cancer Cell Line: Molecular Characterisation, Genetic Stability, and Comprehensive Comparison with Commercial Breast Cancer Cell Lines. Cells 2021 , 11 , 117. [ Google Scholar ] [ CrossRef ]
- Skok, K.; Gradišnik, L.; Čelešnik, H.; Potočnik, U.; Kavalar, R.; Takač, I.; Maver, U. Isolation and characterization of the first Slovenian human triple-negative breast cancer cell line. Breast J. 2020 , 26 , 328–330. [ Google Scholar ]
- O’Meara, T.A.; Tolaney, S.M. Tumor mutational burden as a predictor of immunotherapy response in breast cancer. Oncotarget. 2021 , 12 , 394–400. [ Google Scholar ]
- Chen, X.; Li, J.; Gray, W.H.; Lehmann, B.D.; Bauer, J.A.; Shyr, Y.; Pietenpol, J.A. TNBCtype: A Subtyping Tool for Triple-Negative Breast Cancer. Cancer Inform. 2012 , 11 , 147–156. [ Google Scholar ]
- Hartung, C.; Porsch, M.; Stückrath, K.; Kaufhold, S.; Staege, M.S.; Hanf, V.; Lantzsch, T.; Uleer, C.; Peschel, S.; John, J.; et al. Identifying High-Risk. Triple-Negative Breast Cancer Patients by Molecular Subtyping. Breast Care 2021 , 16 , 637–647. [ Google Scholar ] [ PubMed ]
- Cancer Genome Atlas Network. Comprehensive molecular portraits of human breast tumours. Nature 2012 , 490 , 61–70. [ Google Scholar ] [ CrossRef ] [ PubMed ]
- Prat, A.; Adamo, B.; Cheang, M.C.; Anders, C.K.; Carey, L.A.; Perou, C.M. Molecular characterization of basal-like and non-basal-like triple-negative breast cancer. Oncologist 2013 , 18 , 123–133. [ Google Scholar ] [ PubMed ]
- U.S. Food & Drug Administration, FDA. 2022. Available online: www.fda.gov (accessed on 20 October 2022).
- Ravkin, H.D.; Givton, O.; Geffen, D.B.; Rubin, E. Direct comparison shows that mRNA-based diagnostics incorporate information which cannot be learned directly from genomic mutations. BMC Bioinform. 2020 , 21 , 196. [ Google Scholar ] [ CrossRef ]
- Karam, R.; Conner, B.; LaDuca, H.; McGoldrick, K.; Krempely, K.; Richardson, M.E.; Zimmermann, H.; Gutierrez, S.; Reineke, P.; Hoang, L.; et al. Assessment of Diagnostic Outcomes of RNA Genetic Testing for Hereditary Cancer. JAMA Netw. Open 2019 , 2 , e1913900. [ Google Scholar ]
- Chen, S.; Parmigiani, G. Meta-analysis of BRCA1 and BRCA2 penetrance. J. Clin. Oncol. 2007 , 25 , 1329–1333. [ Google Scholar ]
- Vietri, M.T.; Caliendo, G.; Casamassimi, A.; Cioffi, M.; De Paola, M.L.; Napoli, C.; Molinari, A.M. A novel PALB2 truncating mutation in an Italian family with male breast cancer. Oncol. Rep. 2015 , 33 , 1243–1247. [ Google Scholar ] [ CrossRef ]
- Piccolo, S.R.; Andrulis, I.L.; Cohen, A.L.; Conner, T.; Moos, P.J.; Spira, A.E.; Buys, S.S.; Johnson, W.E.; Bild, A.H. Gene-expression patterns in peripheral blood classify familial breast cancer susceptibility. BMC Med. Genomics. 2015 , 8 , 72. [ Google Scholar ] [ CrossRef ]
- Schaafsma, E.; Zhang, B.; Schaafsma, M.; Tong, C.Y.; Zhang, L.; Cheng, C. Impact of Oncotype DX testing on ER+ breast cancer treatment and survival in the first decade of use. Breast Cancer Res. 2021 , 23 , 74. [ Google Scholar ]
- Martín, M.; González-Rivera, M.; Morales, S.; de la Haba-Rodriguez, J.; González-Cortijo, L.; Manso, L.; Albanell, J.; González-Martín, A.; González, S.; Arcusa, A.; et al. Prospective study of the impact of the Prosigna assay on adjuvant clinical decision-making in unselected patients with estrogen receptor positive, human epidermal growth factor receptor negative, node negative early-stage breast cancer. Curr. Med. Res. Opin. 2015 , 31 , 1129–1137. [ Google Scholar ]
- Dowsett, M.; Sestak, I.; Lopez-Knowles, E.; Sidhu, K.; Dunbier, A.K.; Cowens, J.W.; Ferree, S.; Storhoff, J.; Schaper, C.; Cuzick, J. Comparison of PAM50 risk of recurrence score with oncotype DX and IHC4 for predicting risk of distant recurrence after endocrine therapy. J. Clin. Oncol. 2013 , 31 , 2783–2790. [ Google Scholar ] [ PubMed ]
- Gnant, M.; Filipits, M.; Greil, R.; Stoeger, H.; Rudas, M.; Bago-Horvath, Z.; Mlineritsch, B.; Kwasny, W.; Knauer, M.; Singer, C.; et al. Predicting distant recurrence in receptor-positive breast cancer patients with limited clinicopathological risk: Using the PAM50 Risk of Recurrence score in 1478 postmenopausal patients of the ABCSG-8 trial treated with adjuvant endocrine therapy alone. Ann. Oncol. 2014 , 25 , 339–345. [ Google Scholar ] [ CrossRef ] [ PubMed ]
- Veracyte. Prosigna Breast Cancer Assay. 2022. Available online: https://www.prosigna.com/ (accessed on 26 October 2022).
- Lænkholm, A.V.; Jensen, M.B.; Eriksen, J.O.; Rasmussen, B.B.; Knoop, A.S.; Buckingham, W.; Ferree, S.; Schaper, C.; Nielsen, T.O.; Haffner, T.; et al. PAM50 Risk of Recurrence Score Predicts 10-Year Distant Recurrence in a Comprehensive Danish Cohort of Postmenopausal Women Allocated to 5 Years of Endocrine Therapy for Hormone Receptor-Positive Early Breast Cancer. J. Clin. Oncol. 2018 , 36 , 735–740. [ Google Scholar ] [ CrossRef ]
- Soliman, H.; Shah, V.; Srkalovic, G.; Mahtani, R.; Levine, E.; Mavromatis, B.; Srinivasiah, J.; Kassar, M.; Gabordi, R.; Qamar, R.; et al. MammaPrint guides treatment decisions in breast Cancer: Results of the IMPACt trial. BMC Cancer 2020 , 20 , 81. [ Google Scholar ]
- van de Vijver, M.J.; He, Y.D.; van’t Veer, L.J.; Dai, H.; Hart, A.A.; Voskuil, D.W.; Schreiber, G.J.; Peterse, J.L.; Roberts, C.; Marton, M.J.; et al. A gene-expression signature as a predictor of survival in breast cancer. N. Engl. J. Med. 2002 , 347 , 1999–2009. [ Google Scholar ] [ CrossRef ] [ PubMed ]
- Cardoso, F.; van’t Veer, L.J.; Bogaerts, J.; Slaets, L.; Viale, G.; Delaloge, S.; Pierga, J.Y.; Brain, E.; Causeret, S.; DeLorenzi, M.; et al. 70-Gene Signature as an Aid to Treatment Decisions in Early-Stage Breast Cancer. N. Engl. J. Med. 2016 , 375 , 717–729. [ Google Scholar ] [ CrossRef ]
- Andre, F.; Ismaila, N.; Allison, K.H.; Barlow, W.E.; Collyar, D.E.; Damodaran, S.; Henry, N.L.; Jhaveri, K.; Kalinsky, K.; Kuderer, N.M.; et al. Biomarkers for Adjuvant Endocrine and Chemotherapy in Early-Stage Breast Cancer: ASCO Guideline Update. J. Clin. Oncol. 2022 , 40 , 1816–1837. [ Google Scholar ] [ CrossRef ]
- Beumer, I.; Witteveen, A.; Delahaye, L.; Wehkamp, D.; Snel, M.; Dreezen, C.; Zheng, J.; Floore, A.; Brink, G.; Chan, B.; et al. Equivalence of MammaPrint array types in clinical trials and diagnostics. Breast Cancer Res. Treat. 2016 , 156 , 279–287. [ Google Scholar ]
- Krijgsman, O.; Roepman, P.; Zwart, W.; Carroll, J.S.; Tian, S.; de Snoo, F.A.; Bender, R.A.; Bernards, R.; Glas, A.M. A diagnostic gene profile for molecular subtyping of breast cancer associated with treatment response. Breast Cancer Res. Treat. 2012 , 133 , 37–47. [ Google Scholar ]
- Syed, Y.Y. Oncotype DX Breast Recurrence Score ® : A Review of its Use in Early-Stage Breast Cancer. Mol. Diagn Ther. 2020 , 24 , 621–632. [ Google Scholar ]
- Kalinsky, K.; Barlow, W.E.; Gralow, J.R.; Meric-Bernstam, F.; Albain, K.S.; Hayes, D.F.; Lin, N.U.; Perez, E.A.; Goldstein, L.J.; Chia, S.K.L.; et al. 21-Gene Assay to Inform Chemotherapy Benefit in Node-Positive Breast Cancer. N. Engl. J. Med. 2021 , 385 , 2336–2347. [ Google Scholar ] [ PubMed ]
- Genomic Health. What is the Oncotype DX ® Test, and What Makes it Unique? 2022. Available online: https://www.oncotypeiq.com/en-CA/breast-cancer/healthcare-professionals/oncotype-dx-breast-recurrence-score/about-the-test# (accessed on 7 November 2022).
- Trosman, J.R.; Van Bebber, S.L.; Phillips, K.A. Coverage policy development for personalized medicine: Private payer perspectives on developing policy for the 21-gene assay. J. Oncol. Pract. 2010 , 6 , 238–242. [ Google Scholar ] [ CrossRef ] [ PubMed ] [ Green Version ]
- Sparano, J.A.; Gray, R.J.; Makower, D.F.; Pritchard, K.I.; Albain, K.S.; Hayes, D.F.; Geyer, C.E., Jr.; Dees, E.C.; Perez, E.A.; Olson, J.A., Jr.; et al. Prospective Validation of a 21-Gene Expression Assay in Breast Cancer. N. Engl. J. Med. 2015 , 373 , 2005–2014. [ Google Scholar ] [ CrossRef ]
- Sparano, J.A.; Gray, R.J.; Makower, D.F.; Pritchard, K.I.; Albain, K.S.; Hayes, D.F.; Geyer, C.E., Jr.; Dees, E.C.; Goetz, M.P.; Olson, J.A., Jr.; et al. Adjuvant Chemotherapy Guided by a 21-Gene Expression Assay in Breast Cancer. N. Engl. J. Med. 2018 , 379 , 111–121. [ Google Scholar ]
- Sparano, J.A.; Gray, R.J.; Ravdin, P.M.; Makower, D.F.; Pritchard, K.I.; Albain, K.S.; Hayes, D.F.; Geyer, C.E., Jr.; Dees, E.C.; Goetz, M.P.; et al. Clinical and Genomic Risk to Guide the Use of Adjuvant Therapy for Breast Cancer. N. Engl. J. Med. 2019 , 380 , 2395–2405. [ Google Scholar ]
- Sparano, J.A.; Gray, R.J.; Makower, D.F.; Albain, K.S.; Saphner, T.J.; Badve, S.S.; Wagner, L.I.; Kaklamani, V.G.; Keane, M.M.; Gomez, H.L.; et al. Clinical Outcomes in Early Breast Cancer with a High 21-Gene Recurrence Score of 26 to 100 Assigned to Adjuvant Chemotherapy Plus Endocrine Therapy: A Secondary Analysis of the TAILORx Randomized Clinical Trial. JAMA Oncol. 2020 , 6 , 367–374. [ Google Scholar ] [ PubMed ]
- Sestak, I.; Zhang, Y.; Sgroi, D.; Schnabel, C.A.; Cuzick, J.M.; Dowsett, M. Residual risk assessment with the Breast Cancer Index (BCI) for prediction of late distant recurrence (DR) in patients from the TransATAC study. J. Clin. Oncol. 2018 , 36 (Suppl. S15), 529. [ Google Scholar ]
- Habel, L.A.; Sakoda, L.C.; Achacoso, N.; Ma, X.J.; Erlander, M.G.; Sgroi, D.C.; Fehrenbacher, L.; Greenberg, D.; Quesenberry, C.P., Jr. HOXB13:IL17BR and molecular grade index and risk of breast cancer death among patients with lymph node-negative invasive disease. Breast Cancer Res. 2013 , 15 , R24. [ Google Scholar ]
- Sgroi, D.C.; Sestak, I.; Cuzick, J.; Zhang, Y.; Schnabel, C.A.; Schroeder, B.; Erlander, M.G.; Dunbier, A.; Sidhu, K.; Lopez-Knowles, E.; et al. Prediction of late distant recurrence in patients with oestrogen-receptor-positive breast cancer: A prospective comparison of the breast-cancer index (BCI) assay, 21-gene recurrence score, and IHC4 in the TransATAC study population. Lancet Oncol. 2013 , 14 , 1067–1076. [ Google Scholar ] [ CrossRef ]
- Müller, B.M.; Keil, E.; Lehmann, A.; Winzer, K.J.; Richter-Ehrenstein, C.; Prinzler, J.; Bangemann, N.; Reles, A.; Stadie, S.; Schoenegg, W.; et al. The EndoPredict Gene-Expression Assay in Clinical Practice—Performance and Impact on Clinical Decisions. PLoS ONE 2013 , 8 , e68252. [ Google Scholar ]
- Myriad Genetics. EndoPredict: One Test—Three Clinical Answers for Breast Cancer Patients. 2022. Available online: https://endopredict.eu/ (accessed on 27 November 2022).
- Filipits, M.; Rudas, M.; Jakesz, R.; Dubsky, P.; Fitzal, F.; Singer, C.F.; Dietze, O.; Greil, R.; Jelen, A.; Sevelda, P.; et al. A new molecular predictor of distant recurrence in ER-positive, HER2-negative breast cancer adds independent information to conventional clinical risk factors. Clin. Cancer Res. 2011 , 17 , 6012–6020. [ Google Scholar ] [ CrossRef ] [ PubMed ]
- Sestak, I.; Martín, M.; Dubsky, P.; Kronenwett, R.; Rojo, F.; Cuzick, J.; Filipits, M.; Ruiz, A.; Gradishar, W.; Soliman, H.; et al. Prediction of chemotherapy benefit by EndoPredict in patients with breast cancer who received adjuvant endocrine therapy plus chemotherapy or endocrine therapy alone. Breast Cancer Res. Treat. 2019 , 176 , 377–386. [ Google Scholar ] [ PubMed ]
- Filipits, M.; Dubsky, P.; Rudas, M.; Greil, R.; Balic, M.; Bago-Horvath, Z.; Singer, C.F.; Hlauschek, D.; Brown, K.; Bernhisel, R.; et al. Prediction of Distant Recurrence Using EndoPredict Among Women with ER+, HER2- Node-Positive and Node-Negative Breast Cancer Treated with Endocrine Therapy Only. Clin. Cancer Res. 2019 , 25 , 3865–3872. [ Google Scholar ] [ PubMed ]
- Buus, R.; Sestak, I.; Kronenwett, R.; Denkert, C.; Dubsky, P.; Krappmann, K.; Scheer, M.; Petry, C.; Cuzick, J.; Dowsett, M. Comparison of EndoPredict and EPclin With Oncotype DX Recurrence Score for Prediction of Risk of Distant Recurrence After Endocrine Therapy. J. Natl. Cancer Inst. 2016 , 108 , djw149. [ Google Scholar ] [ PubMed ]
- Constantinidou, A.; Marcou, Y.; Simmons, T.; Bernhisel, R.; Hughes, E.; Meek, S.; Kakouri, E.I.; Georgiou, G.; Zouvani, I.; Savvidou, G.; et al. Clinical validation of EndoPredict in premenopausal women with estrogen receptor-positive (ER+), human epidermal growth factor receptor 2-negative (HER2-) primary breast cancer. J. Clin. Oncol. 2021 , 39 (Suppl. S15), 537. [ Google Scholar ] [ CrossRef ]
- Veridex. GeneSearch Breast Lymph Node (BLN) Test Kit, FDA Report/PMA P060017. 2022. Available online: https://fda.report/PMA/P060017/6/P060017B.pdf (accessed on 7 November 2022).
- Janssen Diagnostics. GeneSearch™ Breast Lymph Node (BLN) Assay Post Approval Study. ClinicalTrials.gov Identifier: NCT00595296; 2016. Available online: https://www.clinicaltrials.gov/ct2/show/record/NCT00595296?view=record (accessed on 2 November 2022).
- U.S. Food & Drug Administration. Premarket Approval, PMA Number P060017, Genesearch Breast Lymph Node (Bln) Assay. 2022. Available online: https://www.accessdata.fda.gov/scripts/cdrh/cfdocs/cfPMA/pma.cfm?start_search=1&PMANumber=P060017 (accessed on 2 November 2022).
- Cutress, R.; Agrawal, A.; Etherington, A.; Gabriel, F.G.; Jeffrey, M.; Lai, L.; Wise, M.; Cree, I.; Yiangou, C. Intra-operative assessment of axillary sentinel lymph nodes (SLN) using an RT-PCR based assay for Mammaglobin (MG) and Cytokeratin 19 (CK19). EJSO 2008 , 34 , 1159–1160. [ Google Scholar ]
- Mansel, R.E.; Goyal, A.; Douglas-Jones, A.; Woods, V.; Goyal, S.; Monypenny, I.; Sweetland, H.; Newcombe, R.G.; Jasani, B. Detection of breast cancer metastasis in sentinel lymph nodes using intra-operative real time GeneSearch BLN Assay in the operating room: Results of the Cardiff study. Breast Cancer Res. Treat. 2009 , 115 , 595–600. [ Google Scholar ] [ CrossRef ]
- Agendia. The Molecular Profile to Define and Defeat Her Unique Cancer. 2022. Available online: https://agendia.com/mammaprint/ (accessed on 27 November 2022).
- Mittempergher, L.; Delahaye, L.J.M.J.; Witteveen, A.T.; Spangler, J.B.; Hassenmahomed, F.; Mee, S.; Mahmoudi, S.; Chen, J.; Bao, S.; Snel, M.H.J.; et al. MammaPrint and BluePrint Molecular Diagnostics Using Targeted RNA Next-Generation Sequencing Technology. J. Mol. Diagn. 2019 , 21 , 808–823. [ Google Scholar ]
- Bou Zerdan, M.; Ibrahim, M.; Nakib, C.E.; Hajjar, R.; Assi, H.I. Genomic Assays in Node Positive Breast Cancer Patients: A Review. Front. Oncol. 2021 , 10 , 609100. [ Google Scholar ]
- Biotheranostics, I. Breast Cancer Index. 2022. Available online: www.breastcancerindex.com (accessed on 27 November 2022).
- Myriad Genetic Laboratories. Myriad EndoPredict Technical Specifications. 2021. Available online: https://myriad-library.s3.amazonaws.com/technical-specifications/EndoPredict-Technical-Specifications.pdf (accessed on 2 November 2022).
- Sun, L.; Legood, R.; Sadique, Z.; Dos-Santos-Silva, I.; Yang, L. Cost-effectiveness of risk-based breast cancer screening programme, China. Bull. World Health Organ. 2018 , 96 , 568–577. [ Google Scholar ] [ CrossRef ]
- Nøst, T.H.; Holden, M.; Dønnem, T.; Bøvelstad, H.; Rylander, C.; Lund, E.; Sandanger, T.M. Transcriptomic signals in blood prior to lung cancer focusing on time to diagnosis and metastasis. Sci. Rep. 2021 , 11 , 7406. [ Google Scholar ] [ CrossRef ] [ PubMed ]
- Holsbø, E.; Olsen, K.S. Metastatic Breast Cancer and Pre-Diagnostic Blood Gene Expression Profiles-The Norwegian Women and Cancer (NOWAC) Post-Genome Cohort. Front. Oncol. 2020 , 10 , 575461. [ Google Scholar ] [ PubMed ]
- Holden, M.; Holden, L.; Olsen, K.S.; Lund, E. Local in Time Statistics for detecting weak gene expression signals in blood—Illustrated for prediction of metastases in breast cancer in the NOWAC Post-genome Cohort. Adv. Genom. Genet. 2017 , 7 , 11–28. [ Google Scholar ]
- Chen, S.; Liu, M.; Liang, B.; Ge, S.; Peng, J.; Huang, H.; Xu, Y.; Tang, X.; Deng, L. Identification of human peripheral blood monocyte gene markers for early screening of solid tumors. PLoS ONE 2020 , 15 , e0230905. [ Google Scholar ]
- Han, M.; Liew, C.T.; Zhang, H.W.; Chao, S.; Zheng, R.; Yip, K.T.; Song, Z.Y.; Li, H.M.; Geng, X.P.; Zhu, L.X.; et al. Novel blood-based, five-gene biomarker set for the detection of colorectal cancer. Clin. Cancer Res. 2008 , 14 , 455–460. [ Google Scholar ] [ CrossRef ]
- Siegel, R.L.; Miller, K.D.; Fuchs, H.E.; Jemal, A. Cancer Statistics, 2021. CA Cancer J. Clin. 2021 , 71 , 7–33. [ Google Scholar ] [ CrossRef ]
- Thigpen, D.; Kappler, A.; Brem, R. The Role of Ultrasound in Screening Dense Breasts-A Review of the Literature and Practical Solutions for Implementation. Diagnostics 2018 , 8 , 20. [ Google Scholar ]
- Weedon-Fekjaer, H.; Lindqvist, B.H.; Vatten, L.J.; Aalen, O.O.; Tretli, S. Breast cancer tumor growth estimated through mammography screening data. Breast Cancer Res. 2008 , 10 , R41. [ Google Scholar ]
- DeSantis, C.E.; Ma, J.; Gaudet, M.M.; Newman, L.A.; Miller, K.D.; Goding Sauer, A.; Jemal, A.; Siegel, R.L. Breast cancer statistics, 2019. CA Cancer J. Clin. 2019 , 69 , 438–451. [ Google Scholar ]
- de Fraipont, F.; Gazzeri, S.; Cho, W.C.; Eymin, B. Circular RNAs and RNA Splice Variants as Biomarkers for Prognosis and Therapeutic Response in the Liquid Biopsies of Lung Cancer Patients. Front. Genet. 2019 , 10 , 390. [ Google Scholar ]
- Markou, A.; Tzanikou, E.; Lianidou, E. The potential of liquid biopsy in the management of cancer patients. Semin. Cancer Biol. 2022 , 84 , 69–79. [ Google Scholar ] [ CrossRef ] [ PubMed ]
- Duffy, M.J. Chapter 13—Circulating cancer biomarkers: Current status and future prospects. In Clinical Aspects and Laboratory Determination, Cancer Biomarkers ; Ramanathan, L.V., Fleisher, M., Duffy, M.J., Eds.; Elsevier: Amsterdam, The Netherlands, 2022; pp. 409–443. [ Google Scholar ]
- Adashek, J.J.; Janku, F.; Kurzrock, R. Signed in Blood: Circulating Tumor DNA in Cancer Diagnosis, Treatment and Screening. Cancers 2021 , 13 , 3600. [ Google Scholar ] [ PubMed ]
- Li, J.; Guan, X.; Fan, Z.; Ching, L.M.; Li, Y.; Wang, X.; Cao, W.M.; Liu, D.X. Non-Invasive Biomarkers for Early Detection of Breast Cancer. Cancers 2020 , 12 , 2767. [ Google Scholar ] [ PubMed ]
- Jurj, A.; Zanoaga, O.; Braicu, C.; Lazar, V.; Tomuleasa, C.; Irimie, A.; Berindan-Neagoe, I. A Comprehensive Picture of Extracellular Vesicles and Their Contents. Molecular Transfer to Cancer Cells. Cancers 2020 , 12 , 298. [ Google Scholar ]
- Liyanage, U.K.; Moore, T.T.; Joo, H.G.; Tanaka, Y.; Herrmann, V.; Doherty, G.; Drebin, J.A.; Strasberg, S.M.; Eberlein, T.J.; Goedegebuure, P.S.; et al. Prevalence of regulatory T cells is increased in peripheral blood and tumor microenvironment of patients with pancreas or breast adenocarcinoma. J. Immunol. 2002 , 169 , 2756–2761. [ Google Scholar ]
- Liew, C.C.; Ma, J.; Tang, H.C.; Zheng, R.; Dempsey, A.A. The peripheral blood transcriptome dynamically reflects system wide biology: A potential diagnostic tool. J. Lab. Clin. Med. 2006 , 147 , 126–132. [ Google Scholar ]
- Twine, N.C.; Stover, J.A.; Marshall, B.; Dukart, G.; Hidalgo, M.; Stadler, W.; Logan, T.; Dutcher, J.; Hudes, G.; Dorner, A.J.; et al. Disease-associated expression profiles in peripheral blood mononuclear cells from patients with advanced renal cell carcinoma. Cancer Res. 2003 , 63 , 6069–6075. [ Google Scholar ]
- Stoiber, D.; Assinger, A. Platelet-Leukocyte Interplay in Cancer Development and Progression. Cells 2020 , 9 , 855. [ Google Scholar ] [ CrossRef ]
- Ward, M.P.; EKane, L.; ANorris, L.; Mohamed, B.M.; Kelly, T.; Bates, M.; Clarke, A.; Brady, N.; Martin, C.M.; Brooks, R.D.; et al. Platelets, immune cells and the coagulation cascade; friend or foe of the circulating tumour cell? Mol. Cancer 2021 , 20 , 59. [ Google Scholar ]
- Sharma, P.; Sahni, N.S.; Tibshirani, R.; Skaane, P.; Urdal, P.; Berghagen, H.; Jensen, M.; Kristiansen, L.; Moen, C.; Sharma, P.; et al. Early detection of breast cancer based on gene-expression patterns in peripheral blood cells. Breast Cancer Res. 2005 , 7 , R634–R644. [ Google Scholar ]
- Aarøe, J.; Lindahl, T.; Dumeaux, V.; Saebø, S.; Tobin, D.; Hagen, N.; Skaane, P.; Lönneborg, A.; Sharma, P.; Børresen-Dale, A.L. Gene expression profiling of peripheral blood cells for early detection of breast cancer. Breast Cancer Res. 2010 , 12 , R7. [ Google Scholar ]
- Čelešnik, H.S. Triple-Negative Breast Cancer and other Breast Cancer. In: Encyclopedia. Available online: https://encyclopedia.pub/entry/21678 (accessed on 27 November 2022).
- Dumeaux, V.; Ursini-Siegel, J.; Flatberg, A.; Fjosne, H.E.; Frantzen, J.O.; Holmen, M.M.; Rodegerdts, E.; Schlichting, E.; Lund, E. Peripheral blood cells inform on the presence of breast cancer: A population-based case-control study. Int. J. Cancer. 2015 , 136 , 656–667. [ Google Scholar ] [ PubMed ]
- Suzuki, E.; Sugimoto, M.; Kawaguchi, K.; Pu, F.; Uozumi, R.; Yamaguchi, A.; Nishie, M.; Tsuda, M.; Kotake, T.; Morita, S. Gene expression profile of peripheral blood mononuclear cells may contribute to the identification and immunological classification of breast cancer patients. Breast Cancer 2019 , 26 , 282–289. [ Google Scholar ] [ PubMed ]
- Zhang, F.; Deng, Y.; Drabier, R. Multiple biomarker panels for early detection of breast cancer in peripheral blood. Biomed. Res. Int. 2013 , 2013 , 781618. [ Google Scholar ] [ PubMed ]
- Hou, H.; Lyu, Y.; Jiang, J.; Wang, M.; Zhang, R.; Liew, C.C.; Wang, B.; Cheng, C. Peripheral blood transcriptome identifies high-risk benign and malignant breast lesions. PLoS ONE 2020 , 15 , e0233713. [ Google Scholar ]
- Srivastava, S.; Hanash, S. Pan-Cancer Early Detection: Hype or Hope? Cancer Cell 2020 , 38 , 23–24. [ Google Scholar ]
- Qi, F.; Gao, F.; Cai, Y.; Han, X.; Qi, Y.; Ni, J.; Sun, J.; Huang, S.; Chen, S.; Wu, C.; et al. Complex Age- and Cancer-Related Changes in Human Blood Transcriptome-Implications for Pan-Cancer Diagnostics. Front. Genet. 2021 , 12 , 746879. [ Google Scholar ]
- Varkey, J.; Nicolaides, T. Tumor-Educated Platelets: A Review of Current and Potential Applications in Solid Tumors. Cureus 2021 , 13 , e19189. [ Google Scholar ] [ CrossRef ]
- Best, M.G.; Sol, N.; Kooi, I.; Tannous, J.; Westerman, B.A.; Rustenburg, F.; Schellen, P.; Verschueren, H.; Post, E.; Koster, J.; et al. RNA-Seq of Tumor-Educated Platelets Enables Blood-Based Pan-Cancer, Multiclass, and Molecular Pathway Cancer Diagnostics. Cancer Cell 2015 , 28 , 666–676. [ Google Scholar ] [ CrossRef ]
- Yang, B.; Xu, Q.; Wu, F.; Liu, F.; Ye, X.; Liu, G.; Shao, Z.; Meng, X.; Mougin, B.; Wu, J. Using peripheral blood mRNA signature to distinguish between breast cancer and benign breast disease in non-conclusive mammography patients. Cancer Biol. Ther. 2010 , 10 , 1235–1239. [ Google Scholar ]
- Zuckerman, N.S.; Yu, H.; Simons, D.L.; Bhattacharya, N.; Carcamo-Cavazos, V.; Yan, N.; Dirbas, F.M.; Johnson, D.L.; Schwartz, E.J.; Lee, P.P. Altered local and systemic immune profiles underlie lymph node metastasis in breast cancer patients. Int. J. Cancer. 2013 , 132 , 2537–2547. [ Google Scholar ] [ CrossRef ] [ PubMed ]
- Lund, E.; Holden, L.; Bøvelstad, H.; Plancade, S.; Mode, N.; Günther, C.C.; Nuel, G.; Thalabard, J.C.; Holden, M. A new statistical method for curve group analysis of longitudinal gene expression data illustrated for breast cancer in the NOWAC postgenome cohort as a proof of principle. BMC Med. Res. Methodol. 2016 , 16 , 28. [ Google Scholar ] [ CrossRef ]
- Lund, E.; Dumeaux, V.; Braaten, T.; Hjartåker, A.; Engeset, D.; Skeie, G.; Kumle, M. Cohort profile: The Norwegian Women and Cancer Study--NOWAC--Kvinner og kreft. Int. J. Epidemiol. 2008 , 37 , 36–41. [ Google Scholar ] [ PubMed ]
- Čelešnik, H.S. Breast Cancer Biomarkers from Peripheral Blood Cells. In: Encyclopedia. 2022. Available online: https://encyclopedia.pub/entry/21624 (accessed on 8 November 2022).
- Balacescu, O.; Balacescu, L.; Virtic, O.; Visan, S.; Gherman, C.; Drigla, F.; Pop, L.; Bolba-Morar, G.; Lisencu, C.; Fetica, B.; et al. Blood Genome-Wide Transcriptional Profiles of HER2 Negative Breast Cancers Patients. Mediators Inflamm. 2016 , 2016 , 3239167. [ Google Scholar ] [ PubMed ]
- Foulds, G.A.; Vadakekolathu, J.; Abdel-Fatah, T.M.A.; Nagarajan, D.; Reeder, S.; Johnson, C.; Hood, S.; Moseley, P.M.; Chan, S.Y.T.; Pockley, A.; et al. Immune-Phenotyping and Transcriptomic Profiling of Peripheral Blood Mononuclear Cells from Patients with Breast Cancer: Identification of a 3 Gene Signature Which Predicts Relapse of Triple Negative Breast Cancer. Front. Immunol. 2018 , 9 , 2028. [ Google Scholar ] [ PubMed ]
- Ming, W.; Xie, H.; Hu, Z.; Chen, Y.; Zhu, Y.; Bai, Y.; Liu, H.; Sun, X.; Liu, Y.; Gu, W. Two Distinct Subtypes Revealed in Blood Transcriptome of Breast Cancer Patients with an Unsupervised Analysis. Front. Oncol. 2019 , 9 , 985. [ Google Scholar ] [ CrossRef ] [ PubMed ]
- Dumeaux, V.; Fjukstad, B.; Fjosne, H.E.; Frantzen, J.O.; Holmen, M.M.; Rodegerdts, E.; Schlichting, E.; Børresen-Dale, A.L.; Bongo, L.A.; Lund, E.; et al. Interactions between the tumor and the blood systemic response of breast cancer patients. PLoS Comput. Biol. 2017 , 13 , e1005680. [ Google Scholar ] [ CrossRef ] [ Green Version ]
- Thompson, D.; Easton, D. The genetic epidemiology of breast cancer genes. J. Mammary Gland. Biol. Neoplasia 2004 , 9 , 221–236. [ Google Scholar ] [ CrossRef ]
- Loke, S.Y.; Lee, A.S.G. The future of blood-based biomarkers for the early detection of breast cancer. Eur. J. Cancer 2018 , 92 , 54–68. [ Google Scholar ]
- Seale, K.N.; Tkaczuk, K.H.R. Circulating Biomarkers in Breast Cancer. Clin. Breast Cancer 2022 , 22 , e319–e331. [ Google Scholar ]
- Ambry Genetics. +RNAinsight, Expanded RNA Analysis for Better Variant Classification. 2021. Available online: https://www.ambrygen.com/file/material/view/1663/RNA_Flyer_FNL%20091521.pdf (accessed on 8 November 2022).
- Syantra. Syantra DX|Breast Cancer FAQs. 2022. Available online: https://ss-usa.s3.amazonaws.com/c/308494115/media/1619629a43fbddb7823212636145172/4115~collateral_FAQs_c.pdf (accessed on 8 November 2022).
- Syantra. 2022. Available online: https://www.syantra.com/ (accessed on 8 November 2022).
- StageZero. Aristotle ® . 2022. Available online: https://www.stagezerolifesciences.com/aristotle-test.html (accessed on 8 November 2022).
- Tobin, D.; Karlsson, M.; Hagen, N.; Børresen-Dale, A.; Mydland, E.; Bårdsen, K.; Jensen, M. Use of the blood based, 96-assay set for breast cancer detection. Poster. EJC Suppl. 2010 , 8 , 164. [ Google Scholar ]
- Tobin, D.; Bårdsen, K.; Kauczynska, M.; Kumar, Y.; Shroff, C.; Punia, D.; Srinivasan, V.; Børresen Dale, A.; Sharma, P.; Hollingsworth, A. Performance of a blood-based gene-expression test, BCtect, for early breast cancer detection. J. Clin. Oncol. 2009 , 27 (Suppl. S15), 11012. [ Google Scholar ]
- Mackay, J.; Szecsei, C.M. Genetic counselling for hereditary predisposition to ovarian and breast cancer. Ann. Oncol. 2010 , 21 (Suppl. S7), vii334–vii338. [ Google Scholar ] [ CrossRef ] [ PubMed ]
- Karam, R.; Krempely, K.; Richardson, M.; McGoldrick, K.; Conner, B.R.; Landrith, T.; Allen, K.; Yussuf, A.; Rana, H.; Culver, S.; et al. RNA Genetic Testing in Hereditary Cancer Improves Variant Classification and Patient Management. In In Proceedings of the Annual Clinical Genetics Meeting (ACMG), Seattle, DC, USA, 2–6 April 2019; American College of Medical Genetics and Genomics: Bethesda, MD, USA, 2019. [ Google Scholar ]
- Ambry Genetics. First Prospective Study Shows +RNAinsight™ Identifies More Patients with Increased Risk for Hereditary Cancer Than DNA-Only Testing. 2020. Available online: https://www.ambrygen.com/company/press-release/128/first-prospective-study-shows-rnainsight-identifies-more-patients-with-increased-risk-for-hereditary-cancer-than-dna-only-testing (accessed on 24 January 2023).
- Bundred, N.; Fuh, K.; Asgarian, N.; Brown, S.; Simonot, D.; Wang, X.; Shepherd, R.; Quan, M.L.; Docktor, B.J.; Maxwell, A.; et al. Abstract P2-01-02: A whole blood assay to identify breast cancer: Interim analysis of the international identify breast cancer (IDBC) study evidence supporting the Syantra DX breast cancer test. Cancer Res. 2022 , 82 (Suppl. S4), P2–01–02. [ Google Scholar ]
- Dempsey, A.A.; Tripp, J.H.; Chao, S.; Stamatiou, D.; Pilcz, T.; Ying, J.; Burakoff, R. Aristotle: A single blood test for pan-cancer screening. J. Clin. Oncol. 2020 , 38 (Suppl. S15), e15037. [ Google Scholar ] [ CrossRef ]
- DiaGenic ASA and Applied Biosystems. Diagenic Announces Launch of First Breast Cancer Gene-Expression Blood Test. Medindia . 2008. Available online: https://www.medindia.net/health-press-release/Diagenic-Announces-Launch-of-First-Breast-Cancer-Gene-Expression-Blood-Test-37529-1.htm (accessed on 27 November 2022).
- Cision, C.E. Marking of the DiaGenic’s Blood Test for Early Breast Cancer Diagnosis. 2009. Available online: https://news.cision.com/nel-asa/r/ce-marking-of-the-diagenic-s-blood-test-for-early-breast-cancer-diagnosis,c434534 (accessed on 27 November 2022).
- Elvidge, S. Gene Expression Diagnostics: A New Approach. In: Life Science Leader. 2011. Available online: https://www.lifescienceleader.com/doc/gene-expression-diagnostics-a-new-approach-0001 (accessed on 27 November 2022).
- Park, A. Freenome, Siemens Join Forces to Develop Blood Test for Breast Cancer. 2021. Available online: https://www.fiercebiotech.com/medtech/freenome-siemens-join-forces-to-develop-blood-test-for-breast-cancer (accessed on 8 November 2022).
- Philippidis, A. Blood Stake: Roche Raises Freenome Investment to $ 360M. GEN Edge 2022 , 4 , 36–43. [ Google Scholar ] [ CrossRef ]
- Stephens, K. Freenome, Siemens Healthineers Collaborate for Breast Cancer Research. 2021. Available online: https://www.proquest.com/ (accessed on 27 November 2022).
- Byron, S.A.; Van Keuren-Jensen, K.R.; Engelthaler, D.M.; Carpten, J.D.; Craig, D.W. Translating RNA sequencing into clinical diagnostics: Opportunities and challenges. Nat. Rev. Genet. 2016 , 17 , 257–271. [ Google Scholar ]
- Bernard, P.S.; Wittwer, C.T. Real-time PCR technology for cancer diagnostics. Clin. Chem. 2002 , 48 , 1178–1185. [ Google Scholar ]
- Grätz, C.; Bui, M.L.U.; Thaqi, G.; Kirchner, B.; Loewe, R.P.; Pfaffl, M.W. Obtaining Reliable RT-qPCR Results in Molecular Diagnostics-MIQE Goals and Pitfalls for Transcriptional Biomarker Discovery. Life 2022 , 12 , 386. [ Google Scholar ] [ CrossRef ]
- Narrandes, S.; Xu, W. Gene Expression Detection Assay for Cancer Clinical Use. J. Cancer 2018 , 9 , 2249–2265. [ Google Scholar ] [ CrossRef ]
- Kamps, R.; Brandão, R.D.; Bosch, B.J.; Paulussen, A.D.; Xanthoulea, S.; Blok, M.J.; Romano, A. Next-Generation Sequencing in Oncology: Genetic Diagnosis, Risk Prediction and Cancer Classification. Int. J. Mol. Sci. 2017 , 18 , 308. [ Google Scholar ] [ CrossRef ] [ PubMed ]
- Liu, X.; Powell, C.A.; Wang, X. Forward single-cell sequencing into clinical application: Understanding of cancer microenvironment at single-cell solution. Clin. Transl. Med. 2022 , 12 , e782. [ Google Scholar ] [ PubMed ]
- Fang, H.; Zeng, Y.; Zhang, L.; Chen, C.; Powell, C.A.; Wang, X. Can single cell RNA sequencing reshape the clinical biochemistry of hematology: New clusters of circulating blood cells. Clin. Transl. Med. 2021 , 11 , e671. [ Google Scholar ] [ CrossRef ]
- Kamps-Hughes, N.; Carlton, V.E.H.; Fresard, L.; Osazuwa, S.; Starks, E.; Vincent, J.J.; Albritton, S.; Nussbaum, R.L.; Nykamp, K. A Systematic Method for Detecting Abnormal mRNA Splicing and Assessing Its Clinical Impact in Individuals Undergoing Genetic Testing for Hereditary Cancer Syndromes. J. Mol. Diagn. 2022 . [ Google Scholar ] [ CrossRef ]
- Montalban, G.; Bonache, S.; Bach, V.; Gisbert-Beamud, A.; Tenés, A.; Moles-Fernández, A.; López-Fernández, A.; Carrasco, E.; Balmaña, J.; Diez, O.; et al. BRCA1 and BRCA2 whole cDNA analysis in unsolved hereditary breast/ovarian cancer patients. Cancer Genet. 2021 , 258–259 , 10–17. [ Google Scholar ]
- Murdock, D.R. Enhancing Diagnosis Through RNA Sequencing. Clin. Lab. Med. 2020 , 40 , 113–119. [ Google Scholar ] [ CrossRef ]
- Curry, P.D.K.; Broda, K.L.; Carroll, C.J. The Role of RNA-Sequencing as a New Genetic Diagnosis Tool. Curr. Genet. Med. Rep. 2021 , 9 , 13–21. [ Google Scholar ]
- Frésard, L.; Smail, C.; Ferraro, N.M.; Teran, N.A.; Li, X.; Smith, K.S.; Bonner, D.; Kernohan, K.D.; Marwaha, S.; Zappala, Z.; et al. Identification of rare-disease genes using blood transcriptome sequencing and large control cohorts. Nat. Med. 2019 , 25 , 911–919. [ Google Scholar ]
- Cummings, B.B.; Marshall, J.L.; Tukiainen, T.; Lek, M.; Donkervoort, S.; Foley, A.R.; Bolduc, V.; Waddell, L.B.; Sandaradura, S.A.; O’Grady, L.G.; et al. Improving genetic diagnosis in Mendelian disease with transcriptome sequencing. Sci. Transl. Med. 2017 , 9 , eaal5209. [ Google Scholar ]
- Landrith, T.; Li, B.; Cass, A.A.; Conner, B.R.; LaDuca, H.; McKenna, D.B.; Maxwell, K.N.; Domchek, S.; Morman, N.A.; Heinlen, C.; et al. Splicing profile by capture RNA-seq identifies pathogenic germline variants in tumor suppressor genes. NPJ Precis Oncol. 2020 , 4 , 4. [ Google Scholar ]
- Tandy-Connor, S.; Guiltinan, J.; Krempely, K.; LaDuca, H.; Reineke, P.; Gutierrez, S.; Gray, P.; Tippin Davis, B. False-positive results released by direct-to-consumer genetic tests highlight the importance of clinical confirmation testing for appropriate patient care. Genet. Med. 2018 , 20 , 1515–1521. [ Google Scholar ] [ CrossRef ] [ PubMed ]
- Rotunno, M.; Hu, N.; Su, H.; Wang, C.; Bertazzi, P.A.; Caporaso, N.; Taylor, P.R.; Landi, M.T. A blood-based gene expression signature of early-stage non-small cell lung cancer. J. Clin. Oncol. 2012 , 30 (Suppl. S30), 69. [ Google Scholar ]
- Wurdinger, T.; In ‘t Veld, S.G.J.G.; Best, M.G. Platelet RNA as Pan-Tumor Biomarker for Cancer Detection. Cancer Res. 2020 , 80 , 1371–1373. [ Google Scholar ] [ PubMed ]
- Malczewska, A.; Bodei, L.; Kidd, M.; Modlin, I.M. Blood mRNA Measurement (NETest) for Neuroendocrine Tumor Diagnosis of Image-Negative Liver Metastatic Disease. J. Clin. Endocrinol. Metab. 2019 , 104 , 867–872. [ Google Scholar ] [ PubMed ]
- Omar, H.; Lim, C.R.; Chao, S.; Lee, M.M.; Bong, C.W.; Ooi, E.J.; Yu, C.G.; Tan, S.S.; Abu Hassan, M.R.; Menon, J.; et al. Blood gene signature for early hepatocellular carcinoma detection in patients with chronic hepatitis B. J. Clin. Gastroenterol. 2015 , 49 , 150–157. [ Google Scholar ]
- Umu, S.U.; Langseth, H.; Zuber, V.; Helland, Å.; Lyle, R.; Rounge, T.B. Serum RNAs can predict lung cancer up to 10 years prior to diagnosis. Elife 2022 , 11 , e71035. [ Google Scholar ] [ CrossRef ]
- Umu, S.U.; Langseth, H.; Keller, A.; Meese, E.; Helland, Å.; Lyle, R.; Rounge, T.B. A 10-year prediagnostic follow-up study shows that serum RNA signals are highly dynamic in lung carcinogenesis. Mol. Oncol. 2020 , 14 , 235–247. [ Google Scholar ] [ CrossRef ]
- Burton, J.; Umu, S.U.; Langseth, H.; Grotmol, T.; Grimsrud, T.K.; Haugen, T.B.; Rounge, T.B. Serum RNA Profiling in the 10-Years Period Prior to Diagnosis of Testicular Germ Cell Tumor. Front Oncol. 2020 , 10 , 574977. [ Google Scholar ]
- Nielsen, T.; Wallden, B.; Schaper, C.; Ferree, S.; Liu, S.; Gao, D.; Barry, G.; Dowidar, N.; Maysuria, M.; Storhoff, J. Analytical validation of the PAM50-based Prosigna Breast Cancer Prognostic Gene Signature Assay and nCounter Analysis System using formalin-fixed paraffin-embedded breast tumor specimens. BMC Cancer 2014 , 14 , 177. [ Google Scholar ]
- Slembrouck, L.; Darrigues, L.; Laurent, C.; Mittempergher, L.; Delahaye, L.J.; Vanden Bempt, I.; Vander Borght, S.; Vliegen, L.; Sintubin, P.; Raynal, V.; et al. Decentralization of Next-Generation RNA Sequencing-Based MammaPrint ® and BluePrint ® Kit at University Hospitals Leuven and Curie Institute Paris. Transl. Oncol. 2019 , 12 , 1557–1565. [ Google Scholar ] [ CrossRef ]
- Kronenwett, R.; Bohmann, K.; Prinzler, J.; Sinn, B.V.; Haufe, F.; Roth, C.; Averdick, M.; Ropers, T.; Windbergs, C.; Brase, J.C.; et al. Decentral gene expression analysis: Analytical validation of the Endopredict genomic multianalyte breast cancer prognosis test. BMC Cancer 2012 , 12 , 456. [ Google Scholar ] [ CrossRef ] [ PubMed ]
- Wang, Y.S.; Liu, Y.H.; Tao, O.Y.; Yang, X.H.; Wu, J.; Su, F.X.; Sun, X.; Zhong, W.X.; Liao, N.; Yang, W.T. GeneSearch™ BLN Assay could replace frozen section and touch imprint cytology for intra-operative assessment of breast sentinel lymph nodes. Breast Cancer 2014 , 21 , 583–589. [ Google Scholar ] [ PubMed ]
- Matthijs, G.; Souche, E.; Alders, M.; Corveleyn, A.; Eck, S.; Feenstra, I.; Race, V.; Sistermans, E.; Sturm, M.; Weiss, M.; et al. Guidelines for diagnostic next-generation sequencing. Eur. J. Hum. Genet. 2016 , 24 , 1515. [ Google Scholar ] [ PubMed ]
- Naito, Y.; Aburatani, H.; Amano, T.; Baba, E.; Furukawa, T.; Hayashida, T.; Hiyama, E.; Ikeda, S.; Kanai, M.; Kato, M.; et al. Clinical practice guidance for next-generation sequencing in cancer diagnosis and treatment (edition 2.1). Int. J. Clin. Oncol. 2021 , 26 , 233–283. [ Google Scholar ] [ CrossRef ]
- Cardoso, F.; Piccart-Gebhart, M.; Van’t Veer, L.; Rutgers, E. TRANSBIG Consortium. The MINDACT trial: The first prospective clinical validation of a genomic tool. Mol. Oncol. 2007 , 1 , 246–251. [ Google Scholar ] [ CrossRef ]
- Viale, G.; de Snoo, F.A.; Slaets, L.; Bogaerts, J.; van’t Veer, L.; Rutgers, E.J.; Piccart-Gebhart, M.J.; Stork-Sloots, L.; Glas, A.; Russo, L.; et al. MINDACT investigators. Immunohistochemical versus molecular (BluePrint and MammaPrint) subtyping of breast carcinoma. Outcome results from the EORTC 10041/BIG 3-04 MINDACT trial. Breast Cancer Res. Treat. 2018 , 167 , 123–131. [ Google Scholar ]
- Albain, K.S.; Gray, R.J.; Makower, D.F.; Faghih, A.; Hayes, D.F.; Geyer, C.E.; Dees, E.C.; Goetz, M.P.; Olson, J.A.; Lively, T.; et al. Ethnicity, and Clinical Outcomes in Hormone Receptor-Positive, HER2-Negative, Node-Negative Breast Cancer in the Randomized TAILORx Trial. J. Natl. Cancer Inst. 2021 , 113 , 390–399. [ Google Scholar ] [ CrossRef ]
- Roy, S.; Coldren, C.; Karunamurthy, A.; Kip, N.S.; Klee, E.W.; Lincoln, S.E.; Leon, A.; Pullambhatla, M.; Temple-Smolkin, R.L.; Voelkerding, K.V.; et al. Standards and Guidelines for Validating Next-Generation Sequencing Bioinformatics Pipelines: A Joint Recommendation of the Association for Molecular Pathology and the College of American Pathologists. J. Mol. Diagn. 2018 , 20 , 4–27. [ Google Scholar ]
- Koh, E.J.; Yu, S.Y.; Kim, S.H.; Kim, S.J.; Lee, E.I.; Hwang, S.Y. Understanding Confounding Effects of Blood Handling Strategies on RNA Quality and Transcriptomic Alteration Using RNA Sequencing. BioChip J. 2021 , 15 , 187–194. [ Google Scholar ]
- Park, S.; Ahn, S.; Kim, J.Y.; Kim, J.; Han, H.J.; Hwang, D.; Park, J.; Park, H.S.; Park, S.; Kim, G.M.; et al. Blood Test for Breast Cancer Screening through the Detection of Tumor-Associated Circulating Transcripts. Int. J. Mol. Sci. 2022 , 23 , 9140. [ Google Scholar ] [ CrossRef ]
- Dubsky, P.; Van’t Veer, L.; Gnant, M.; Rudas, M.; Bago-Horvath, Z.; Greil, R.; Lujinovic, E.; Buresch, J.; Rinnerthaler, G.; Hulla, W.; et al. A clinical validation study of MammaPrint in hormone receptor-positive breast cancer from the Austrian Breast and Colorectal Cancer Study Group 8 (ABCSG-8) biomarker cohort. ESMO Open 2021 , 6 , 100006. [ Google Scholar ] [ PubMed ]
- Wylezinski, L.S.; Shaginurova, G.I.; Spurlock Iii, C.F. Longitudinal assessment and stability of long non-coding RNA gene expression profiles measured in human peripheral whole blood collected into PAXgene blood RNA tubes. BMC Res. Notes 2020 , 13 , 531. [ Google Scholar ] [ CrossRef ] [ PubMed ]
- Donohue, D.E.; Gautam, A.; Miller, S.A.; Srinivasan, S.; Abu-Amara, D.; Campbell, R.; Marmar, C.R.; Hammamieh, R.; Jett, M. Gene expression profiling of whole blood: A comparative assessment of RNA-stabilizing collection methods. PLoS ONE 2019 , 14 , e0223065. [ Google Scholar ]
- Asare, A.L.; Kolchinsky, S.A.; Gao, Z.; Wang, R.; Raddassi, K.; Bourcier, K.; Seyfert-Margolis, V. Differential gene expression profiles are dependent upon method of peripheral blood collection and RNA isolation. BMC Genom. 2008 , 9 , 474. [ Google Scholar ]
- Shen, Y.; Li, R.; Tian, F.; Chen, Z.; Lu, N.; Bai, Y.; Ge, Q.; Lu, Z. Impact of RNA integrity and blood sample storage conditions on the gene expression analysis. Onco. Targets Ther. 2018 , 11 , 3573–3581. [ Google Scholar ] [ CrossRef ] [ PubMed ]
- Ronaghi, M.; Karamohamed, S.; Pettersson, B.; Uhlén, M.; Nyrén, P. Real-time DNA sequencing using detection of pyrophosphate release. Anal. Biochem. 1996 , 242 , 84–89. [ Google Scholar ] [ PubMed ]
- Yang, Y.; Zhang, T.; Xiao, R.; Hao, X.; Zhang, H.; Qu, H.; Xie, B.; Wang, T.; Fang, X. Platform-independent approach for cancer detection from gene expression profiles of peripheral blood cells. Brief. Bioinform. 2020 , 21 , 1006–1015. [ Google Scholar ] [ CrossRef ]
Assay Trade Name (Manufacturer) | Number of Genes | Assay Indicated for | Description | Methodology/ Platform | References |
---|---|---|---|---|---|
+RNAinsight (Ambry Genetics) | Up to 91 genes (for maximum coverage) | Assessing hereditary cancer predisposition | +RNAinsight analyses functional RNA data to classify DNA variants and identify deep-intronic mutations; intended for paired RNA/DNA analyses, as a supplement to Ambry Genetics DNA-level hereditary cancer panels CancerNext, CancerNext-Expanded, CustomNext-Cancer. | RNA sequencing | [ ] |
Syantra DX Breast Cancer (Syantra Inc.) | 12-gene multi-biomarker panel | Breast cancer screening for women aged 25–80 | Enables classification of a sample as positive or negative for BC signature; demonstrated utility for early cancer screening, for women with high breast density, and for women under 50. | qRT-PCR-based assay | [ , ] |
Multi-cancer blood test Aristotle (Stage Zero Life Sciences Ltd.) | Multi-biomarker panel | Pan-cancer screening (breast, bladder, colorectum, cervix, endometrium, liver, ovary, prostate, and stomach) | Enables detection of multiple cancer molecular signatures from a single blood sample (early cancer detection). | Microarray-based assay | [ ] |
BCtect (DiaGenic ASA) | 96-assay signature | Breast cancer screening | Enables classification of a sample as positive or negative for BC signature; utility for early BC detection in both pre- and post-menopausal women, and across cancer stages and types. | qRT-PCR-based assay | [ , , ] |
The statements, opinions and data contained in all publications are solely those of the individual author(s) and contributor(s) and not of MDPI and/or the editor(s). MDPI and/or the editor(s) disclaim responsibility for any injury to people or property resulting from any ideas, methods, instructions or products referred to in the content. |
Share and Cite
Čelešnik, H.; Potočnik, U. Blood-Based mRNA Tests as Emerging Diagnostic Tools for Personalised Medicine in Breast Cancer. Cancers 2023 , 15 , 1087. https://doi.org/10.3390/cancers15041087
Čelešnik H, Potočnik U. Blood-Based mRNA Tests as Emerging Diagnostic Tools for Personalised Medicine in Breast Cancer. Cancers . 2023; 15(4):1087. https://doi.org/10.3390/cancers15041087
Čelešnik, Helena, and Uroš Potočnik. 2023. "Blood-Based mRNA Tests as Emerging Diagnostic Tools for Personalised Medicine in Breast Cancer" Cancers 15, no. 4: 1087. https://doi.org/10.3390/cancers15041087
Article Metrics
Article access statistics, supplementary material.
ZIP-Document (ZIP, 101 KiB)
Further Information
Mdpi initiatives, follow mdpi.
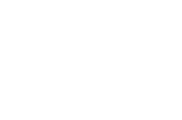
Subscribe to receive issue release notifications and newsletters from MDPI journals

An official website of the United States government
The .gov means it’s official. Federal government websites often end in .gov or .mil. Before sharing sensitive information, make sure you’re on a federal government site.
The site is secure. The https:// ensures that you are connecting to the official website and that any information you provide is encrypted and transmitted securely.
- Publications
- Account settings
Preview improvements coming to the PMC website in October 2024. Learn More or Try it out now .
- Advanced Search
- Journal List
- Front Pharmacol
Molecular Docking as a Therapeutic Approach for Targeting Cancer Stem Cell Metabolic Processes
Babak arjmand.
1 Cell Therapy and Regenerative Medicine Research Center, Endocrinology and Metabolism Molecular-Cellular Sciences Institute, Tehran University of Medical Sciences, Tehran, Iran
Shayesteh Kokabi Hamidpour
Sepideh alavi-moghadam, hanieh yavari, ainaz shahbazbadr, mostafa rezaei tavirani.
2 Proteomics Research Center, Shahid Beheshti University of Medical Sciences, Tehran, Iran
Kambiz Gilany
3 Integrative Oncology Department, Breast Cancer Research Center, Motamed Cancer Institute, ACECR, Tehran, Iran
4 Reproductive Immunology Research Center, Avicenna Research Institute, ACECR, Tehran, Iran
Bagher Larijani
5 Endocrinology and Metabolism Research Center, Endocrinology and Metabolism Clinical Sciences Institute, Tehran University of Medical Sciences, Tehran, Iran
Cancer stem cells (CSCs) are subpopulation of cells which have been demonstrated in a variety of cancer models and involved in cancer initiation, progression, and development. Indeed, CSCs which seem to form a small percentage of tumor cells, display resembling characteristics to natural stem cells such as self-renewal, survival, differentiation, proliferation, and quiescence. Moreover, they have some characteristics that eventually can demonstrate the heterogeneity of cancer cells and tumor progression. On the other hand, another aspect of CSCs that has been recognized as a central concern facing cancer patients is resistance to mainstays of cancer treatment such as chemotherapy and radiation. Owing to these details and the stated stemness capabilities, these immature progenitors of cancerous cells can constantly persist after different therapies and cause tumor regrowth or metastasis. Further, in both normal development and malignancy, cellular metabolism and stemness are intricately linked and CSCs dominant metabolic phenotype changes across tumor entities, patients, and tumor subclones. Hence, CSCs can be determined as one of the factors that correlate to the failure of common therapeutic approaches in cancer treatment. In this context, researchers are searching out new alternative or complementary therapies such as targeted methods to fight against cancer. Molecular docking is one of the computational modeling methods that has a new promise in cancer cell targeting through drug designing and discovering programs. In a simple definition, molecular docking methods are used to determine the metabolic interaction between two molecules and find the best orientation of a ligand to its molecular target with minimal free energy in the formation of a stable complex. As a comprehensive approach, this computational drug design method can be thought more cost-effective and time-saving compare to other conventional methods in cancer treatment. In addition, increasing productivity and quality in pharmaceutical research can be another advantage of this molecular modeling method. Therefore, in recent years, it can be concluded that molecular docking can be considered as one of the novel strategies at the forefront of the cancer battle via targeting cancer stem cell metabolic processes.
Graphical Abstract

Introduction
Cancer is considered as one of the worldwide life-threatening and the leading causes of human mortality ( Vineis and Wild, 2014 ; Organization, 2020 ). According to the latest data released by the International Agency for Research on Cancer (IARC) on 14 December 2020, the annual incidence of cancer in 2020 reached 19.3 million cases and 10 million deaths. Furthermore, evidence based on the World Health Organization (WHO) suggests that there would be 29.5 million new cancer diagnoses and 16.4 million cancer deaths per year by 2040 ( Shah et al., 2019 ; Sung et al., 2021 ). Accordingly, given the rapid development of oncology researches and the advancement of novel biotechnology approaches, determining different aspects of cancer progression can pave the way for improved cancer prognosis and treatment alternatives ( Goyal et al., 2006 ; Charmsaz et al., 2018 ; Pucci et al., 2019 ). Herein, one of the challenges in the field of cancer treatment is the heterogeneity of tumor cells, which may lead to anti-cancer drug resistance or cancer treatment failure. Therefore, a full understanding of tumor heterogeneity can provide a clear picture of cancer progression and lead to the discovery of new cancer therapy options by researchers ( y Cajal et al., 2020 ). Tumor heterogeneity is a condition in which tumor cells differ in various biological aspects such as function, differentiation, tumorigenesis, and sensitivity to anti-cancer therapies ( Prager et al., 2019 ). In addition, depending on the type of heterogeneity, heterogeneous groups of tumor cells can have the same or distinct genomic content ( Prager et al., 2019 ). In addition, heterogeneous populations of tumor cells can have the same or different genome content depending on the type of heterogeneity ( Bedard et al., 2013 ). Hereupon, tumor heterogeneity can be divided into three types: 1) intertumor heterogeneity which is related to the variation of tumor cells among different patients, 2) intersite heterogeneity which is referred to the variation of cells among distinct tumors within a patient such as tumors in the primary site and tumors in the metastatic site, and 3) intratumor heterogeneity which is linked with heterogeneous populations of cells in a single tumor ( Piraino et al., 2019 ). Oncology studies were shown that the cancer stem cells (CSCs) model is one of the models responsible for the generation of heterogeneous populations of cells, especially intratumor heterogeneity type ( Prasetyanti and Medema, 2017 ; Turnquist et al. ). Moreover, it can be caused by different factors such as genetics, epigenetics, and various micro-environmental features ( Wang et al., 2015 ). Indeed, CSCs are a subgroup of cancerous tumor cells that display stemness abilities in the same manner as normal stem cells. For instance, they can self-renew to form the same daughter cells and give rise to differentiated multiple lineages of cells which form tumors. Additionally, the quiescence state is one of the distinguishing characteristics of cancer and normal stem cells, and it can play a role in therapeutic resistance and cancer progression ( Hung et al., 2019 ; Lee et al., 2020 ). Furthermore, CSCs can make the treatment process more challenging because of their resistance to therapeutic approaches such as chemo and radiation therapies. The mentioned therapeutic resistance can be due to a variety of factors and mechanisms, including tumor environment, epigenetic effects, multidrug resistance proteins (MRPs) expression, various signaling pathways, effective mechanisms in DNA damage resistance, and the epithelial-to-mesenchymal transition (EMT) process ( Phi et al., 2018 ). On the other hand, the function of metabolic pathways and processes are crucial in the growth, proliferation and survival of CSCs. In this respect, many investigations at the cellular and molecular level were indicated that unique forms of metabolic processes such as oxidative phosphorylation (OXPHOS), carbohydrate, and lipid metabolisms are observed in CSCs ( Chae and Kim, 2018 ; Yadav et al., 2020 ). Therefore, the science of metabolomics, as well as the understanding of alterations associated with metabolic processes, could be useful in recognizing CSC behaviors and developing specific therapeutic methods for various types of cancers ( Gilany et al., 2018 ; Rahim et al., 2018 ; Arjmand, 2019a , 2019b ; Goodarzi et al., 2019 ; Larijani et al., 2019 ; Tayanloo-Beik et al., 2020 ), as well as the understanding of alterations associated with metabolic processes, could be useful in recognizing CSC behaviors and developing specific therapeutic methods for various types of cancers ( Cuyàs et al., 2017 ). Additionally, scientists have been pushed to employ targeted approaches for treating cancer due to the problems in CSCs resistance to therapeutic methods. Molecular docking is one of the targeted approaches that play an important role in drug discovery and pharmaceutical researches. This computer-assisted drug design method is based on mathematical algorithms in which the effective biological binding-conformation between the drug and the target molecule can be evaluated. Indeed, the mentioned drug designing is based on the molecular structure that makes it possible to model and predict the molecular interactions as well as evaluate the biochemical processes ( Meng et al., 2011 ; Phillips et al., 2018 ). Hereupon, in the present study, the cellular and molecular characteristics, signaling pathways, metabolic processes, and drug resistance of CSCs have been reviewed. We have also focused our discussion on molecular docking as a novel therapeutic approach in CSCs targeting.
The Biology of Cancer Stem Cells
CSCs are a subset of cancer cells or tumor-initiating cells (TICs) that serve as stem cells and contribute to the original tumor’s phenotypic variety ( Lobo et al., 2007 ). They are found in variable amounts in different tumors. Furthermore, evaluating cell surface markers is the main strategy for detecting CSCs. Normal stem cells and CSCs have many similar characteristics ( Jin et al., 2017 ; Khatami et al., 2019 ) such as 1) Self-Renewal ( Lobo et al., 2007 ) 2) Differentiation capacity ( Mohr et al., 2015 ) 3) Tumorigenesis ( Zhu and Fan, 2018 ) 4) Capacity of developing resistance to drugs/cytotoxic substances and radiation ( Schöning et al., 2017 ). Despite their similarities, there are some distinctions between cancer and somatic stem cells. The first is the origin of these two types of stem cells: natural somatic stem cells arise during embryonic development and separate from each other. They differentiate and produce a variety of mature cells, while CSCs are differentiated from normal adult stem cells or by multiple mutations in a single cell. The second is the ability to regenerate itself: somatic stem cells regenerate more regular than CSCs, although both types of cells can regenerate themselves. Finally, the organogenesis ability of these two cells is studied: both cells have the ability to organogenesis, but CSCs produce abnormal tissue, whereas somatic stem cells’ organogenesis produces normal tissue ( Gjorevski et al., 2014 ).
Cancer Stem Cells Isolation Markers
Since, CSCs are a small part of a big heterogeneous cell population of human cancer, isolation and division of such small human cancer cells can be a significant step in a delicate study of various aspects of cancer. Herein, identifying CSCs markers is a key factor ( Tang et al., 2007 ). Most of the CSCs markers originate from human embryonic stem cells (hESCs) or adult stem cells ( Jin et al., 2017 ; Najafi et al., 2019 ). The expression of CSCs isolation markers varies depending on a number of factors, including cell lines, tumor histotypes, isolation methods, and survey CSCs markers in vivo or in vitro investigations ( Tirino et al., 2013 ). On the one hand, CSCs markers have a beneficial therapeutic effect on several types of cancers by targeting CSCs in order to eliminate tumor recurrences ( Jin et al., 2017 ; Najafi et al., 2019 ). Moreover, the majority of surface markers can be harmed by interactions between enzymes and tumor tissues, and this destruction could be regarded a disadvantage ( Abbaszadegan et al., 2017 ). Some various CSCs markers with their unique characteristics were reviewed in Table 1 .
Most frequently applied markers for cancer stem cells isolation.
CSCs Marker | Marker type | Expression location | Function | Cancer Type | References |
---|---|---|---|---|---|
CD44 | Surface marker | Leukocytes, Endothelial cells, Hepatocytes, Mesenchymal cells | Activation of tyrosine kinase receptors by binding to extracellular matrix, Cell migration, Distinction, Increasing the speed of tumor cells entrance into blood vessels in metastasis | Breast, prostate, lung | ; |
CD133 | Surface marker | Embryonic epithelial stem cell, Hematopoietic stem cells | Organizer of the plasma membrane topology, Conservation of plasma membrane`s lipid structure, Development of head and neck squamous cell carcinoma | Breast, prostate, lung, head, neck | ; ; |
CD117 | Surface marker | Mesenchymal adult stem cells, Cardiac adult stem cell, Ovary | Stem cell factor`s receptor, Drug target molecules | Ovarian | |
CD90 | Surface marker | Between normal hematopoietic stem cells and leukemic CSCs | Identifying leukemic CSCs from hematopoietic stem cell subpopulation | Leukemia | ; |
CD24 | Surface marker | Pancreatic carcinoma | Identifying CSCs in pancreas cancer | Pancreas | ; |
ALDH1 | Intracellular marker | Normal stem cells, Malignant stem cells, Progenitor cells | Regulator of stem cells propagation and distinction | Breast | ; |
P63 | Basal cell marker | Basal regenerative cells of many epithelial tissues, Prostate, Urothelial | Prostate progression, Diagnostic factor of prostate cancer | Prostate | ; |
ALDH1: Aldehyde dehydrogenase isoform 1; CSCs, Cancer stem cells.
Cancer Stem Cells Signaling Pathways
In general, signaling pathways can help to precisely regulate the biological function of both CSCs and regular stem cells. Numerous signaling pathways such as Wnt, Notch, Hh, nuclear factor-κB (NF-κB), Janus kinase/signal transducers and activators of transcription (JAK-STAT), phosphoinositide 3-kinase/AKT/mammalian target of rapamycin (PI3K/AKT/mTOR), transforming growth factor (TGF)/SMAD, and peroxisome proliferator-activated receptor (PPAR) are among the intracellular factors that make a major contribution in regulating stem cell functions. Therefore, excessive or abnormal activity and even suppression of mentioned signal transduction pathways can convert the normal stem cells into cancerous. These pathways are regulated and controlled by the function of factors such as diverse proteins, microRNAs, long noncoding RNAs, and endogenous or exogenous factors, just as they change the self-renewal, survival, proliferation, differentiation, and usually tumorigenesis of CSCs ( Table2 ). Signaling pathways interact with one another in a large and complicated network known as “crosstalk,” which is a crucial fact. Subsequently, crosstalk between signaling pathways can influence the regulation of several phenotypic features and drug resistance in CSCs ( Matsui, 2016 ; Yang L et al., 2020 ). Hereupon, a deep understanding of the signaling processes underlying CSCs can pave the way for small molecules and pharmacological inhibitors to target them ( Du et al., 2019 ).
CSCs signaling pathways characteristics.
Signaling Pathway | Examples of Ligands | Receptors/Co-receptors | Function in CSCs | Type of Cancer | References | |
---|---|---|---|---|---|---|
Wnt | WNT1, WNT2 | Members of the Frizzled, LRP5and LRP6 | Self-renewal | Ductal breast carcinomas | ; ; | |
WNT2B, WNT3 | ROR1 and ROR2 | Tumorigenesis | Colorectal | |||
WNT3A, WNT4 | PTK7 | Dedifferentiation | Papillary thyroid | |||
WNT5A, WNT5B | RYK | Apoptosis regulation | Esophageal | |||
WNT6, WNT7A | MUSK | Metastasis | Colorectal | |||
WNT7B, WNT8A, WNT8B, WNT9A, WNT9B, WNT10A, WNT10B, WNT11, WNT16 | Proteoglycan families | — | — | |||
Notch | Delta-like proteins (DLL1, DLL3, DLL4) | Notch1 | Proliferation | Glioblastoma | ; | |
Jagged proteins (JAG1 and JAG2) | Notch2 | Cell survival | Leukemia | |||
— | Notch3 | Self-renewal | Ovarian | |||
— | Notch4 | Differentiation | Colon | |||
— | — | Migration | Gastric | |||
— | — | Metastasis | Breast | |||
— | — | Apoptosis inhibition | Pancreatic | |||
— | — | Cell fate specification | Prostate | |||
— | — | Asymmetric division | Skin | |||
— | — | — | Non small-cell lung | |||
— | — | — | Liver | |||
Hh | Shh | Ptch1, and to a lesser extent, Ptch2, Cdon | Self-renewal | CML | ; | |
Ihh | Boc | Tumor growth | AML | |||
Dhh | Gas1 | Differentiate into transient amplifying cells | ALL | |||
— | — | Metastasis | Glioma, Multiple Myeloma | |||
— | — | — | Metastatic Melanoma | |||
— | — | — | Breast | |||
— | — | — | Gastric | |||
— | — | — | Colon | |||
— | — | — | Pancreatic | |||
— | — | — | Prostate | |||
— | — | — | Small Cell and | |||
— | — | — | Non-Small Cell | |||
— | — | — | Lung Cancer | |||
NF-κB | Lipopolysaccharide | TLRs | Inflammation | Gastrointestinal | ||
IL-1β | TNFR | Stress responses | Genitourinary | |||
TNF-α | IL-1R | Cell survival | Gynecological | |||
bacterial cell components | CD40 | Proliferation | Head | |||
— | BAFFR | Tumorigenesis | Neck | |||
— | LTβR | Some key angiogenesis factors and adhesion molecules expression, Self-renewal | Breast | |||
— | — | Metastasis | Multiple myeloma | |||
— | — | Apoptosis regulation | Blood cancer | |||
JAK-STAT | ILE, PDGF-C, OSM, CXCL12, HGF, TGF-β, EGF, Gastrin, IGF, Mk, BDNF, NT-3, gp130 | ILFR, PDGFR, OSMR, CXCR7, c-MET, TGFR, EGFR, GRPR, IGF1R, Notch-1/2, TrkB, TrkC, IL-6/IL-6Rα | Tumorigenesis, Metastasis, Chemoresistance, EMT transition, Proliferation, Inflammation, Survival | Prostate, Breast, Gastric, Lung | ||
PI3K/AKT/mTOR | Insulin and epithelial growth factor | ErbB-1; HER1, HER2 (c-ErbB-2), HER3 (c-ErbB-3), and HER4 (c-ErbB-4) CXCR4, IGF-1R | Cell proliferation, Angiogenesis, Metabolism, Differentiation, Survival, Self-renewal, Tumorigenesis | Ovarian, Cervical, Breast, Glioblastoma, Gastric, Pancreatic, Colorectal, Prostate, Hepatocellular | ; ; | |
TGF/SMAD | TGF-β1, 2 and 3 | TGFβR1, TGFβR2 | Cell proliferation, Epithelial-mesenchymal transition, Differentiation, Angiogenesis, Inflammation | Liver, Breast, Gastric, Skin, Glioblastoma, Leukemia Colorectal | ; | |
PPAR | Lipid-derived substrates | PPAR-α, PPAR-δ, PPAR-γ | Proliferation, Maintenance of sphere-formation ability, Expression of CSC Markers | Colorectal, Ovarian, Glioblastoma, Breast | ; |
ALL: acute lymphocytic leukemia; AML, acute myeloid leukemia; BAFFR, B cell-activating factor receptor; BDNF, Brain-derived neurotrophic factor; Boc, Brother of Cdon; CAM, cell adhesion molecule; CDON, CAM-related downregulated by oncogenes; CML, chronic myeloid leukaemia; c-Met, Mesenchymal-epithelial transition factor; CXCL, C-X-C motif chemokine ligand; CXCR, C-X-C chemokine receptor; Dhh, Desert hedgehog; DLL, Delta-like proteins; EGF, epidermal growth factor; EGFR, epidermal growth factor receptor; EMT, Epithelial-to-mesenchymal transition; ErbB-1, Erythroblastic leukemia viral oncogene homolog 1; GAS1, Growth Arrest Specific 1; Gp130, Glycoprotein 130; GRPR, Gastrin-releasing peptide receptor HER, human epidermal growth factor receptor; HGF, hepatocyte growth factor; IGF, Insulin-like growth factor; IGF1R, Insulin-like growth factor receptor 1; Ihh, Indian hedgehog; IL-1β, Interleukin 1 beta; IL-1R, Interleukin-1 receptor; IL-6, Interleukin 6; IL-6Rα, Interleukin 6 receptor alpha; ILFR, leukemia inhibitory factor receptor; JAG, jagged protein; JAK-STAT, Janus kinase/signal transducer and activator of transcription; LRP, Low-density lipoprotein receptor-related protein; LTβR, lymphotoxin beta receptor; MK, Heparin-binding growth factor Midkine; MUSK, muscle associated receptor tyrosine kinase; NF-κB, Nuclear factor kappa-light-chain-enhancer of activated B cells; NT-3, Neurotrophin-3; OSM, Oncostatin M; OSMR, Oncostatin M receptor; PDGF-C, Platelet-derived growth factor C; PDGF-R, Platelet-derived growth factor receptors; PI3K/AKT/mTOR, Phosphoinositide 3-kinase/AKT/mammalian target of rapamycin; PPAR, Peroxisome proliferator-activated receptor; Ptch, Patched; PTK7, Protein tyrosine kinase 7; ROR, Receptor tyrosine kinase-like orphan receptor; RYK, receptor tyr kinase; SHH, sonic hedgehog; TGF-β, transforming growth factor beta; TGFβR, transforming growth factor beta receptor; TLRs, Toll-like receptors; TNF-α, tumor necrosis factor alpha; TNFR, tumor necrosis factor receptor; TrkB, Tropomyosin receptor kinase B; TrkC, Tropomyosin receptor kinase C.
Cancer Stem Cells Metabolic Processes
Metabolic reprogramming is one of critically important characteristics of CSCs compared to other cancer and non-cancer cells, which plays a pivotal role in demonstration of cell functions such as proliferation, fate determination and the cancer progression. In this process, the cellular energy metabolism used by CSCs, such as different types of hydrophobic natural compounds and organic substances metabolisms, adenosine triphosphate (ATP) production pathways, etc., differs from that of other cells. In other words, the presence of more metabolites and high-energy compounds in CSCs suggests that they have a different metabolic profile compared to the other. Furthermore, studies demonstrate that oncogenic mutations, tumor suppressants, and, particularly tumor microenvironment features, can all have a major impact on the many components engaged in such metabolic processes. Nevertheless, mentioned metabolic processes and the components involved can be considered as therapeutic targets for cancer treatment ( Mukha and Dubrovska, 2020 ; Peixoto and Lima, 2018 ; Zhu et al., 2020 ).
CSCs such as normal cells are used glucose through glycolysis process to gain energy and survive. The methods for glucose metabolism in a CSC include OXPHOS and glycolytic phosphorylation, which are selected based on the presence of oxygen. Moreover, they play an important role in differentiation, self-renewal and homeostasis. Generally, high glucose levels increase the number of CSCs, while low glucose levels lower the quantity of CSCs ( Falahzadeh et al., 2019 ). CSCs are adaptable cells that can cope with a wide range of situations, including low oxygen levels, insufficient blood vessel development, hyperoxidation, and hypoxia ( Luo and Wicha, 2015 ). If the CSCs are in a state of hypoxia (lack of oxygen), the proper metabolic pathway is chosen. Herein, they enter the glycolytic pathway, which eventually leads to the formation of lactate ( Yi et al., 2018 ). According to Warburg, CSCs require more energy than other normal cells due to their high growth and proliferation. Although the glycolytic process provides less energy, CSCs prefer it since it is shorter ( Dando et al., 2015 ). Lactate produced by the glycolytic pathway has the ability to influence CSC function and is involved in processes including metastasis, angiogenesis, and differentiation ( Tamada et al., 2012 ). If the cell is in a state of hyperoxia (low oxygen), it enters the oxidative pathway, where pyruvate created from glucose enters the mitochondria. Then proceeds via the Krebs cycle and OXPHOS pathway to make energy. According to researches, the first alteration in CSC metabolism is a shift from aerobic to anaerobic sugar metabolism, in which oncogenes such as Akt 1 and C-Myc can regulate the glycolytic pathway by acting on the Warburg effect ( Dando et al., 2015 ).
Metabolisms Related to Mitochondria
Almost every cell activity relies on the hydrolysis of energy-rich compounds such as ATP. Hereupon, the continuous production and replenishment of such energetic compounds are prioritized by the cells ( Dunn and Grider, 2020 ). Mitochondria are one of the major organelles of cells in which contributes significantly in energy transduction by producing energy-carrying molecules. The mitochondria play a key role by activating the OXPHOS, tricarboxylic acid cycle (TCA), and fatty acid oxidation (FAO) in the cell. Additionally, biosynthetic precursors production, innate immune activation, modulation of the reactive oxygen species (ROS), control of calcium homeostasis, and trigger to apoptotic process are also some of the major activities of mitochondria within a cell ( Zong et al., 2016 ). Owing to the mitochondria biosynthetic and bioenergetics activities, compelling evidence suggests that it also have a crucial impact on CSCs function ( De Francesco et al., 2018 ). The difference in the amount of energy required for cancer stem cells compare to other cells can lead to differences in the quantity of mitochondrial function in them. Studies show that mitochondrial function can be affected by the type of tumor heterogeneity. Evidence also points that epigenetic and micro environmental features are among the factors that can result in altered mitochondrial function in CSCs ( García-Heredia and Carnero, 2020 ). Investigations at the cellular and molecular level imply that changes leading to the production of cancer stem cells can increase the mitochondrial mass ( Shin and Cheong, 2019 ) and membrane potential which are a reflection of electrical and biochemical alterations in CSCs mitochondria ( Zhang et al., 2015 ). Furthermore, changes in mitochondrial DNA (mtDNA) can also affect the expression of some nuclear genes during the retrograde signaling that ultimately lead to inducing EMT process and producing CSCs ( Guha et al., 2014 ). Reciprocally, many mitochondrial proteins are encoded by nuclear DNA (nDNA). Accordingly, mutations or changes in nDNA may eventually lead to altered mitochondrial activity in CSCs ( Guerra et al., 2017 ). In addition to the interaction between mitochondria and the nucleus, disruption of some signaling pathways can affect the role of mitochondria in tumorigenesis. For instance, one of the major functions of PI3K/AKT/mTOR pathway is the regulation of pre-apoptotic proteins such as B-cell lymphoma 2 Associated X, Apoptosis Regulator (BAX) in relation to mitochondria which ultimately leads to apoptosis through this organelle. However, overexpression of apoptosis inhibitor genes in CSCs causes abnormal activation of the mentioned signaling pathway, which can lead to cancer cell proliferation, survival, and drug resistance of cancer cells ( Frasson et al., 2015 ; Liu et al., 2020 ). Whereas the study of mitochondrial role in relation to other parts of cell on a large scale can be challenging, it should be narrow down the study to the major functions of mitochondria. Therefore, to promote research in the assessment of CSCs, such part particularly focuses on tricarboxylic acid cycle (TCA) and electron transport-linked phosphorylation process, synthesis and degradation of lipids, reactive oxygen species (ROS) generation system, and alternative metabolic pathways such as amino acid metabolism in CSCs.
Tricarboxylic Acid Cycle (TCA) and Electron Transport-Linked Phosphorylation Process
Unlike normal cells, CSCs require metabolic adaptation in order to supply fuel and materials for tumorigenesis purposes. Both TCA and OXPHOS which occur alternately following aerobic glycolysis, play an important role in the development of CSCs features. For instance, a reduction in the amount of TCA enzymes can be seen in some CSCs. Additionally, the TCA cycle is associated with different processes such as FAO, glutamine metabolism, and so on. Hence, the TCA cycle can play a key role in the development of stemness capabilities in CSCs under the influence of other metabolites ( Yadav et al., 2020 ). Along with TCA, OXPHOS has an important role in tumorigenesis. As already mentioned, glycolysis is the preferred energy production process compared to the OXPHOS in many CSCs. Although mitochondrial-related bioenergetics processes can produce higher rates of energy-rich compounds, the glycolysis pathway can provide the factors needed for the growth and proliferation of CSCs more timely and rapidly. However, CSCs in some types of cancers such as leukemia, ovarian, glioblastoma, breast, lung, and pancreatic ductal adenocarcinoma (PDAC) do not comply with this rule and prefer the OXPHOS pathway rather than glycolysis ( Peixoto and Lima, 2018 ; Snyder et al., 2018 ). OXPHOS-dependent CSCs can acquire their needed energy from the uptake and chemical changes on some metabolites such as pyruvate, lactate, ketone bodies, and some amino acids. However, extracellular uptake is not the only way to get the nutrients needed by OXPHOS-dependent CSCs functions ( Gentric et al., 2017 ; Jagust et al., 2019a ). They can also supply the required nutrients through metabolic symbiosis with glycolysis to perform their bioenergetics and biosynthetic processes. Interestingly, the restriction of nutrient levels in the surrounding microenvironment of OXPHOS-dependent CSCs has not a huge impact on cell functions. Because in specific tumor microenvironments, they can counteract this limitation with their selective advantages. Therefore, this strategy can make a significant contribution to CSCs survival ( Krstic et al., 2017 ; Zhu et al., 2020 ). Since mitochondrial-related processes have important effects on the energy and materials supplying of CSCs to grow and develop tumors, targeting different components of these processes can be an efficient approach in the treatment of various types of cancers ( Jagust et al., 2019b ).
Synthesis and Degradation of Lipids
Lipid, as one of the cell membrane`s basic constitutive elements, is necessary for different cell activities, such as signaling conduction, energy production, etc. Sterols, monoglycerides, diacylglycerides, triglycerides, phospholipids, and glycolipids are different components of lipid structure. additionally, most of the lipids originated from fatty acids ( Snaebjornsson et al., 2020 ; Visweswaran et al., 2020 ). Furthermore, lipid droplets (LDs) act as a lipid storage and in comparison with normal cells, cancer cells have more LDs. Regarding the metabolism of lipid, CSCs have been affected by this kind of metabolism through different strategies such as CSCs maintaining, complying energy desire of CSCs ( Visweswaran et al., 2020 ), increasing CSCs numbers ( Mancini et al., 2018 ), and protecting CSCs from chemotherapeutic agents-induced peroxidation ( Begicevic et al., 2019 ). Moreover, NANOG, sterol regulatory element-binding transcription factor 1(SREBP1), MYC, stearoyl-CoA desaturase (SCD), fatty acid synthase (FASN), ACVL3, CD36, carnitine palmitoyltransferase 1 (CPT1A), and carnitine palmitoyltransferase 1B (CPT1B) are some main modulators for this metabolism. In this respect, there are some alterations in lipid metabolism which lead to different outcomes, such as the effectiveness on the capability of self-renewal, invasion, metastasis, and drug resistance ( Giacomini et al., 2020 ). On the other hand, CSC biomass production, stimulation of the Wnt/-catenin, and Hippo/YAP signaling pathways are some of the other effects that have been linked to CSC activity and cancer progression ( Chae and Kim, 2018 ; Yi et al., 2018 ; Jagust et al., 2019b ). In this context, the altered lipid metabolism can also have some therapeutic effects in the field of CSCs by the CSCs blockage and lessen CSCs chemoresistance ultimately, lipid metabolism contains different signaling pathways that conserve undifferentiating status and the survival of CSCs. Some of these signaling pathways are Notch signaling, Hippo cascades, Hedgehog (Hh) signaling, and Wnt signaling ( Giacomini et al., 2020 ).
Reactive Oxygen Species Generation System
In addition to energy production processes, other pathways can play vital roles in multiple aspects of the generation and maintenance of the CSCs function. ROS production is one of these pathways which contribute to cancer recurrence, CSCs metastasis, and resistance to conventional therapies. Generation of ROS can be a consequence of electron transferring through mitochondrial membrane. In addition, enzymes in some other organelles and even immune reactions can play a role in the production of these oxygen species. Studies have also shown that chemotherapy and radiotherapy can eventually lead to increased ROS within cells ( Liou and Storz, 2010 ; Zhou et al., 2014 ). In general, the antioxidant system acts as a defense barrier against increasing ROS. Maintaining a balance between the amount of antioxidants and ROS can play an important role in cell stability and homeostasis. If this balance is upset and the ROS level increases, cellular stress and eventually cell death occurs ( Poljsak et al., 2013 ; Kurutas, 2016 ). In contrast, in the case of CSCs, the expression of antioxidants is much higher than in ROS production and keeps the ROS levels low ( Shi et al., 2012 ). Hence, it can promote self-renewal, survival, and resistance to anti-cancer treatments. According to the stated argumentation, ROS can be an appropriate objective for discovering targeted therapies to fight against cancer. For instance, using approaches to increase ROS levels or disruption of antioxidant systems within CSCs can lead to cell aging and apoptosis. Therefore, an effective step can be taken to treat various types of cancer by extensively and accurately examining of ROS modulation in CSCs ( Zhou et al., 2014 ; Ding et al., 2015 ).
Amino Acid Metabolism as an Alternative Metabolic Pathway
CSCs are flexible cells that rely on alternative fuels such as the amino acid glutamine to maximize their growth and proliferation under different environmental conditions ( De Francesco et al., 2018 ). In glucose deficiency, the growth and survival of CSCs are highly dependent on glutamine, which enters the cell through its specific vectors during the path of glutaminolysis and is converted to glutamate by the enzyme mitochondrial glutaminase, thus entering the Krebs cycle ( Deshmukh et al., 2016 ). Glutamine, as a source of nitrogen, plays an important role in mediating metabolites, which eventually synthesize various substances, including protein, lipids, and nucleotide acids ( Deshmukh et al., 2016 ). CSCs of various tumors, including the pancreas, pancreas, ovaries, and lungs, are glutamine-dependent ( Deshmukh et al., 2016 ). The pentose phosphate (PPP) pathway is also used as an alternative pathway for fuel generation in CSCs. PPP is performed in two forms: reversible (non-oxidative) and irreversible (oxidative) ( Giacomini et al., 2020 ), which is an alternative pathway for glucose metabolism during the irreversible pathway of PPP. In this pathway, glucose 6-phosphate (G6P) is converted to ribose 5-phosphate in several steps with the production of nicotinamide adenine dinucleotide phosphate (NADPH), and finally essential nucleotides are synthesized by forming ribose groups ( Riganti et al., 2012 ; Polat et al., 2021 ). However, in reversible PPP, ribose 5-phosphate is converted to glyceride aldehyde 3 phosphate in a series of reversible reactions and is eventually used for glycolysis ( Polat et al., 2021 ). Ketone bodies (acetone, acetate, 3-hydroxybutyrate) are among the high-energy fuels used by CSCs to grow and propagate metastases ( Jagust et al., 2019b ). When there is not enough glucose in CSCs, ketone bodies are released into the blood and converted directly to Acetyl-CoA by the two enzymes OXCT1 and ACAT1. Then acetyl-CoA enters the citric acid (CAC) cycle and produces more ATP in the cell ( Ozsvari et al., 2017 ). In addition to glutamine, lysine is another amino acid that CSCs use to make fuel, as well as TICs, which contain many enzymes; They perform the process of lysine catabolism ( Jagust et al., 2019b ). As a result of the lysine pathway, glutamate is synthesized and cysteine uptake is increased in CSCs ( Peixoto and Lima, 2018 ).
The Chemoresistance of Cancer Stem Cells
Chemoresistance is defined as a pivotal factor of defeated chemotherapy treatment in various cancers. This factor relapses affected agents of chemotherapy such as cell death and tumor bulk`s size decrement. In this respect, CSCs considerably execute the role of referred relapsing and also it has the capability of showing resistance against chemotherapeutics by its insensitivity ( Abdullah and Chow, 2013 ; Zhao, 2016 ). Chemoresistance of CSCs leads to a high risk of metastases, less survival speed ( Nunes et al., 2018 ), and the permanence of CSCs ( Chuthapisith et al., 2010 ). Furthermore, a comparison between normal cancer cells and CSCs revealed that, CSCs intrinsically have a higher amount of chemotherapy resistance than normal cancer cells ( Thomas et al., 2014 ). Many factors are involved in CSCs resistance occurrence, which some of them are as detailed below:
- • Tumor microenvironment (TME): One of the factors involved in the regulation of stemness characteristics and chemoresistance of CSCs, is the autocrine and paracrine interactions of CSCs with the components of their surrounding environment, which is referred to as the TME. In recent years, the key role of TME and its components including extracellular matrix, immune cells, endothelial cells, cancer-associated adipocytes (CAAs), and cancer-associated fibroblasts (CAFs) in the onset, metastasis, recurrence, and drug resistance of cancer have been investigated. The results of these studies show that targeting the TME can be an effective approach in the treatment of cancer ( Gaggianesi et al., 2021 ).
- • Epigenetics : Another major factor in the chemoresistance of CSCs is the role of mechanisms followed epigenetic alterations. Studies reveal that epigenetic processes such as DNA methylation, nucleosome remodeling, histone modification, and non-coding RNAs changes are generally associated with the development of normal stem cell characteristics. However, disruption in the normal function of epigenetic factors lead to the development of tumorigenic properties in CSCs ( Toh et al., 2017 ).
- • Epithelial Mesenchymal Transition (EMT) : EMT is a biological phenomenon during processes such as embryonic development, wound healing, and tissue regeneration. However, in the case of cancer, EMT can suppress epithelial features and convert the cell into the mesenchymal state through signaling pathways such as Wnt, Notch, and Hedgehog, which can lead to the development of tumor features ( Singh and Settleman, 2010 ).
- • Multidrug resistance (MDR) : High levels of MDR is another main factor involved in the chemoresistance of CSCs that occurs after applying long-term or high-dose treatment for cancer patients. Generally, two mechanisms can be considered for the effect of MDR on CSCs: 1) Preventing the drug from reaching an effective concentration: studies imply that the function of efflux pumps such as P-glycoprotein (P-gp) encoded by ABCB1, transporters, and enzymes such as cytochrome P450 and glutathione S-transferase play significant roles in mediating drug resistance. 2) Drug detoxification: based on studies, it has been realized that avoiding apoptosis and activating DNA repair mechanisms are of fundamental importance to induce continuous growth and proliferation of CSCs. ( Cho and Kim, 2020 ).
- • The quiescent state : Quiescence or dormancy is a survival strategy for CSCs. In the quiescent state, cell division stops for a while, and cells live with minimal metabolic activity, but still retain the ability to reactivate the cell cycle ( Chen et al., 2021 ). In this state, both intrinsic (e.g., p53 signaling, reactive oxygen species, hypoxia inducible factor-1a, nuclear factor of activated T cells c1, and negative regulators of mTOR) and non-intrinsic factors (e.g., Tie2/angiopoietin-1, TGF-b and bone morphogenic proteins, thrombopoietin, N-cadherin and integrins, osteopontin, and Wnt/b-catenin signaling) are involved ( Li and Bhatia, 2011 ). According to studies, TME and epigenetic mechanisms have a major contribution to the maintenance of the quiescentstate of CSCs as well as evade immune surveillance and destruction, and tumor relapse. Therefore, the presence and persistence of the quiescence or dormancy state in CSCs can lead to the survival of CSCs and cell resistance to treatments such as chemotherapy ( Chen et al., 2021 ).
- • Self-renewal : Self-renewal is one of the noted hallmarks of CSCs that results from a malfunction of self-renewal pathways (SRPs). Studies indicate that Hh, Wnt, Notch, and B-cell-specific Moloney murine leukemia virus integration site 1 (BMI1) pathways have a crucial role in inducing the self-renewal in CSCs. In recent years, the targeting of SRPs has attracted attention as an efficient therapeutic approach in cancer treatment to reduce cancer recurrence and chemotherapy resistance possibility ( Borah et al., 2015 ).
It should also be noted that according to metabolic studies, cancer cells that have undergone chemoresistance are metabolically altered and adapted. For example, processes such as fatty acid oxidation, glutaminolysis activation, glycolysis activation, lactate production, adaptive mitochondrial reprogramming, ornithine decarboxylase, and polyamine production, and PPP and NADPH production can be observed in chemoresistance cancer cells ( Chen et al., 2020 ).
Molecular Docking Study; A Therapeutic Approach for Anti-Cancer Drug Designing
Drug Discovery is considered as a multi-process platform in which a specific chemical compound with desired biological activity on the drug target can be selected and eventually enter the drug development as a candidate drug. In this platform, both chemical compounds and biological targets are evaluated from different aspects by using various approaches. Since both drug discovery and development are time-consuming and cost-intensive programs, they pose many challenges for researchers in drug designing and discovering for various diseases such as different types of cancer. Therefore, the use of new technologies can pave the way discovering new drugs with high therapeutic potential and take a big step towards disease treatment. Compound screening assays are one of mentioned methods that can help with hit identification, validation, lead generation, and optimization processes, as well as evaluating the compounds’ effects on the therapeutic target. With the advancement of technology and the integration of computational science with biological and pharmaceutical studies, approaches such as virtual screening are widely applied in drug designing and discovering programs ( Reddy et al., 2007 ; Hughes et al., 2011 ; Mohs and Greig, 2017 ; Cui et al., 2020 ). In this context, virtual screening aims to evaluate and filter a limited number of suitable chemical compounds from large libraries of small molecules by using mathematical calculations. Structure-based virtual screening (SBVS) is one of the virtual screening methods which attempts to model and analyze the efficient biological binding-conformation between a ligand and a target molecule by using the molecular docking technique ( Liao et al., 2013 ). Molecular docking is one of the cutting-edge computational drug designing technologies in which the most effective and stable state form of the ligand-receptor complex can be predicted ( Morris and Lim-Wilby, 2008 ). The determination of the three-dimensional structure of the target and ligand molecules is at the top of the entire process priority list. Therefore, some techniques such as x-ray crystallography, nuclear magnetic resonance (NMR) spectroscopy, cryo-electron microscopy (cryo-EM), and homology modeling are not only useful in determining molecular structure, but also as complementary tools in drug development ( Allen and Stokes, 2013 ; Kershaw et al., 2013 ; Sturlese et al., 2015 ; Lohning et al., 2017 ). Molecular docking includes searching algorithms and scoring function as two fundamental aspects of docking programs. Searching algorithms can be defined as a process that can lead to exploring the predominant and effective matching docking modes of ligand to the molecular target among the myriad configurations. Because a large number of binding modes are actually found between a ligand and a biological target molecule, searching algorithms not only can consider the optimum possible orientations of the ligand with the target but also can be an economical and time-saving solution in the docking process ( Meng et al., 2011 ; Salmaso and Moro, 2018 ). Molecular dynamics, distance geometry methods, point complementary methods, fragment-based methods, Mote Carlo methods, genetic algorithms, systematic searches, and incremental construction, are some of the examples of search algorithms that can be used in modeling and evaluating the binding form of a ligand molecule to the objective receptor .
After the algorithm searching, it is time for the scoring function to step into the docking arena to find the good pose between the ligand and the target molecule. Scoring function refers to a process in which putative docking modes are ranked by evaluating their binding affinity and lowest binding energy to achieve top-ranked poses between a ligand and a target molecule. Force field function, Empirical scoring functions, knowledge based scoring functions, knowledge-based potentials, machine learning based scoring functions, comparative assessment of scoring functions, physics-based methods, and descriptor-based scoring functions are some of the examples of scoring function classifications in molecular docking ( Madhavilatha and Babu, 2019 ; Sethi et al., 2019 ).
Regarding scoring function, the study of Wang et al. (2003) is one of the best examples of meticulous studies of popular scoring functions in molecular docking. In this study, the authors compared 11 popular scoring functions, including four scoring functions of the LigFit module in Cerius2 (LigScore, PLP, PMF, and LUDI), four scoring functions of the CScore module in SYBYL (FScore, G-Score, D-Score, and ChemScore), the scoring function of the AutoDock program, and two stand-alone scoring functions (DrugScore and X-Score) by performing them on 100 protein−ligand complexes to scrutinize their performance and sift the most effective and efficient methods among them. In this regard, after generating a set of docked conformations for each ligand by Autodac software, each 11 scoring function was tested and implemented on the maintained set and significant results were obtained. In this study, the authors used root-mean-square deviation ≤2.0 Å as a criterion for examining those scoring functions. Based on the analysis of the mentioned criterion, it was concluded that six scoring functions, including PLP, F-Score, LigScore, DrugScore, LUDI, and X-Score achieved high success rates (about 66–76%). In addition, the study implied that the combination of some of those scoring functions and generating the consensus scoring scheme can also increase the success rate (about 80%) ( Figures 1 , ,2). 2 ). In addition to success rates, the authors examined the correlation between 100 complexes’ binding scores and experimentally determined protein-ligand binding affinities. As a result of this experiment, X-Score, PLP, DrugScore, and G-Score could represent correlation coefficients of more than 0.50, which demonstrate superiority over other scoring functions. Since the best scoring function should perform excellently in both docking and scoring, the three scoring functions, including X-Score, DrugScore, and PLP, can be considered the top scoring functions in molecular docking, according to the study by Wang et al. ( Wang et al., 2003 ).

Success rates of double combinations of the six relatively successful scoring functions in consensus scoring. All numbers are in percent ( Wang et al., 2003 ).

Success rates of triple combinations of the six relatively successful scoring functions in consensus scoring. All numbers are in percent (R. Wang et al., 2003 ).
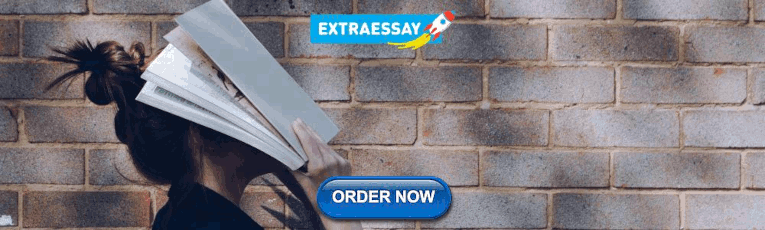
Targeting Cancer Stem Cells Metabolic Process by Molecular Docking
Molecular docking is a substantial method for estimating the interaction between macromolecules such as protein and small molecules such as ligand. On the other hand, molecular docking is also capable of analyzing the molecular kinds of behavioral variability for those molecules which are located at the binding site of a targeted protein. Also, molecular docking is a computational approach and some docking programs are required to carry out its many functions. Some of the most considerable docking programs are Gold, Fred, and Flex. Moreover, they are useful in the prevision of protein and ligand`s binding conjunction ( Kumar et al., 2013 ; Pagadala et al., 2017 ). In this respect, molecular docking is applied in different CSCs-related pathways including metabolic pathways and signaling pathways. Shedding light on metabolic pathways, the activity modification which can be applied by cancer cells, result in the production of metabolic precursors which leads to cancer cells anabolic and energetic requirement fulfilling. Furthermore, different metabolic pathways take a part in tumor progression and malignant tumor alterations. Accordingly, metabolic reprogramming is considered as one of the cancer insignias ( Jagust et al., 2019b ).
Herein, there will be a few examples in the context of some molecular docking usages in metabolic pathways such as 1) mitoketoscins application in targeting metabolic tumor promoters (OXCT1 and ACAT1) in both ketone re-utilization and mitochondrial function ( Ozsvari et al., 2017 ). 2) Survivin protein interaction with andrographolide, which can lead to having an influence on human breast CSCs apoptosis ( Wanandi et al., 2020 ). 3) S-phase kinase-associated protein-2 (Skp2) inhibition process by compound #25 which can result in CSCs survival suppression ( Chan et al., 2013 ).
The other molecular docking-affected pathway in CSCs is the signaling pathway. This pathway is useful for targeted CSCs therapies expansion ( Koury et al., 2017 ), embryonic evolvement, maintaining CSCs, etc. ( Karamboulas and Ailles, 2013 ). Some molecular inhibitor agents of Wnt, Notch, Hh, and some other signaling pathways are implying as one of the important effects of molecular docking process on signaling pathways ( Yang Y et al., 2020 ).
Modulating some target proteins is a striking aspect of molecular docking which has done by natural products. Natural products are able to be considered on the ground of multi-targeting drugs. As such, alkaloids are one of the natural products that have the strength to act as an anticancer lead molecule in the molecular docking process of CSCs. In this regard, Jaitak et al. provided an in-depth analysis of multitargeting drugs as an effective strategy to fight against CSCs and prevent disease recurrence. In this study, the authors examined the effect of some alkaloids that have anticancer potentiality by focusing on the Hh pathway in cancer stem cells. After selection and preparation of target ligands and proteins, Grid parameter selection and validation, implementation of glide docking module of Schrö6; dinger Maestro 9.6 suite, and determination of ADME profile for the studied alkanoid ligands, significant results were discovered. For instance, according to the findings of this study, emetine, and cortistatin, were able to target CSCs maintenance feature by binding to sonic Hh, smoothened (Smo) and, gli protein. Therefore, these two drugs can be applied as multi-targeting drugs in a combination with cancer chemotherapy compounds. Moreover, solamargine alkaloid could also have a good effect on gli protein and sonic hedgehog due to its pharmacophores. Furthermore, both solasonine and tylophorine modulated the Hh pathway and exert anticancer effects on CSCs by affecting only gli proteins. However, unlike other drugs, solamargine and solasonine need to improve the properties of ADME features ( Jaitak, 2016 ).
In addition to alkaloids, the targeting of overexpressed CD44 surface marker in triple-negative breast cancer (TNBC) tissues can have an anticancer effect on CSCs. Regarding targeting CD44 surface markers, Yang et al. determined the positive role of drug carriers including Gambogic acid (GA)-loaded, zirconium-89 (89Zr)-labeled, chitosan (CS)-decorated multifunctional liposomes (MLPs) on TNBC CSCs by designing two in vitro and in vivo experiments. In this study, researchers examined 3D mammospheres and TNBC tissues of 32 women who were diagnosed with TNBC and found that the CD44 surface marker was overexpressed in the disease. Therefore, in this study, 89Zr@CS-MLPs were constructed and predicted how the drug carriers interact with CD44 surface markers in TNBC by applying molecular docking and dynamics simulations methods. The results obtained from the in vitro (examination on tumor cell lines) and in vivo (examination on mice) experiments were implied that 89Zr@CS-MLPs has a great potentiality for TNBC-targeted therapy as a drug carrier. Additionally, since Zr has a long half-life, it can also be used as an ideal radiolabel for positron emission tomography (PET) imaging of cancer. Moreover, 89Zr@CS-GA-MLPs have a high ability to target CSCs in vivo ( Yang R et al., 2020 ).
In 2021, Hongwiangchan et al. demonstrated that hydroquinone 5-O-cinnamoyl ester of renieramycin M (CIN-RM) can be recognized as a fundamental approach in targeting lung CSCs which has been confirmed by molecular docking computational analysis. The effect of CIN-RM is based on the reduction of CSCs markers and upstream inhibition of the AKT pathway. As a result of inhibition of Akt, the expression level of transcription factors involved in self-renewal, such as c-Myc, Nanog, Oct4, and Sox2, are decreased. It is, therefore, CSCs are suppressed and tumor growth can be inhibited. To a lesser extent, CIN-RM also induces its inhibitory effect on the mTOR pathway, but the inhibitory effect of CIN-RM on the protein kinase C (PKC) signal pathway was not significant. Another important result obtained in this study is that CIN-RM even has an effect on inactivating the AKT pathway related to c-Myc regulation of lung non-stem cancer cells, which can be a promising therapeutic approach in cancer treatment ( Figure 3 ) ( Hongwiangchan et al., 2021 ).

Mechanism of action of drugs analyzed by molecular docking on the metabolic processes of CSCs. The ligands and targets have been investigated by molecular docking. Nine effective drugs, including compound #25, andrographolide, mitoketoscins, emetine, cortistatin, solamargine, solasonine, tylophorine, and CIN-RM are known to affect the biological processes and signaling pathways of CSCs. 1) Compound #25 prevents the assembly of the Skp2-SCF complex by binding to Skp2. Hence, it inhibits two pathways including non-proteolytic K63-linked ubiquitination of Akt and ubiquitination and degradation of p27, which ultimately inhibit the development of tumor features. 2) Andrographolide increases intrinsic apoptosis in CSCs (especially in breast cancer) by inhibiting survivin, caspase-9, and caspase-3.3) Mitoketoscins stop the recycling of ketone bodies into Acetyl-CoA by inhibiting two proteins, including OXCT1 and ACAT1. Hence ATP production is stopped and oxidative mitochondrial metabolism in CSCs is inhibited. 4) Emetine and 5) Cortistatin, can target CSCs by binding to sonic Hh, Smo and, gli protein. 6) Solamargine can affect sonic hedgehog and gli proteins by its pharmacophores. 7) Solasonine and 8) Tylophorine modulate the Hh pathway by affecting gli proteins. 9) CIN-RM can lead to upstream inhibition of the Akt pathway and reduction of CSCs markers, which decrease the expression level of transcription factors involved in self-renewal, such as c-Myc, Nanog, Oct4, and Sox2. CIN-RM can inhibit mTOR pathway. Abbreviations: ATP, Adenosine triphosphate; CIN-RM, Hydroquinone 5-O-cinnamoyl ester of renieramycin M; CSCs, Cancer stem cells; Hh, Hedgehog; mTOR, Mammalian target of rapamycin; Smo, smoothened ( Chan et al., 2013 ; Hongwiangchan et al., 2021 ; Jaitak, 2016 ; Liu et al., 2014 ; Madhunapantula et al., 2011 ; Ozsvari et al., 2017 ; Wanandi et al., 2020 ).
Conclusion and Future Perspective
A comprehensive analyzing of CSCs, including signaling pathways and metabolic activities, as well as recognizing their distinctions with normal cells, is an essential technique in cancer targeted treatment ( Bjerkvig et al., 2005 ; Shyh-Chang and Ng, 2017 ). Major obstacles to molecular binding as a targeted therapeutic technique include receptor flexibility, ligand flexibility, and drug resistance, all of which have contributed to cancer therapy failure ( Meng et al., 2011 ). Computational methods or computer tools, which are a form of artificial intelligence (AI), have recently proven to be a useful approach in a variety of domains, including structure prediction, molecular bond modeling, and junction prediction ( Menke et al., 2021 ). The mentioned methods are divided into two groups: classical and machine learning (ML), of which ML is widely used in molecular binding. Computational methods play a key role in molecular binding for drug design and discovery, and results can be analyzed cheaper and often faster than other conventional methods ( Torres et al., 2019 ). Eventually, with the advancement of science and the identification of various computational methods, computing software and hardware are still being updated, and researchers are looking for the most accurate way to target CSCs for cancer treatment ( Phillips et al., 2018 ).
Author Contributions
All authors contributed to the study conception and design. SK Hamidpour, HY, and AS wrote the first draft. MT and KG helped to study and gather information. SA-M extensively edited the manuscript. BL participated in a critical review. BA helped supervise the project and gave final approval of the version to be published.
Conflict of Interest
The authors declare that the research was conducted in the absence of any commercial or financial relationships that could be construed as a potential conflict of interest.
Publisher’s Note
All claims expressed in this article are solely those of the authors and do not necessarily represent those of their affiliated organizations, or those of the publisher, the editors, and the reviewers. Any product that may be evaluated in this article, or claim that may be made by its manufacturer, is not guaranteed or endorsed by the publisher.
- Abbaszadegan M. R., Bagheri V., Razavi M. S., Momtazi A. A., Sahebkar A., Gholamin M. (2017). Isolation, Identification, and Characterization of Cancer Stem Cells: A Review . J. Cel Physiol 232 ( 8 ), 2008–2018. 10.1002/jcp.25759 [ PubMed ] [ CrossRef ] [ Google Scholar ]
- Abdullah L. N., Chow E. K. (2013). Mechanisms of Chemoresistance in Cancer Stem Cells . Clin. Transl Med. 2 ( 1 ), 3–9. 10.1186/2001-1326-2-3 [ PMC free article ] [ PubMed ] [ CrossRef ] [ Google Scholar ]
- Ajani J. A., Song S., Hochster H. S., Steinberg I. B. (2015). Cancer Stem Cells: The Promise and the Potential. Paper Presented at the Seminars in Oncology . [ PubMed ] [ Google Scholar ]
- Allen G. S., Stokes D. L. (2013). “ Modeling, Docking, and Fitting of Atomic Structures to 3D Maps from Cryo-Electron Microscopy ,” in Electron Crystallography of Soluble and Membrane Proteins (Berlin, Germany: Springer; ), 229–241. 10.1007/978-1-62703-176-9_13 [ PMC free article ] [ PubMed ] [ CrossRef ] [ Google Scholar ]
- Arjmand B. (2019a). Genomics, Proteomics, and Metabolomics . [ Google Scholar ]
- Arjmand B. (2019b). Genomics, Proteomics, and Metabolomics: Stem Cells Monitoring in Regenerative Medicine . Berlin, Germany: Springer Nature. [ Google Scholar ]
- Bao B., Ahmad A., Azmi A. S., Ali S., Sarkar F. H. (2013). Overview of Cancer Stem Cells (CSCs) and Mechanisms of Their Regulation: Implications for Cancer Therapy . Curr. Protoc. Pharmacol. 14 ( 1 ), 14.25. 10.1002/0471141755.ph1425s61 [ PMC free article ] [ PubMed ] [ CrossRef ] [ Google Scholar ]
- Bedard P. L., Hansen A. R., Ratain M. J., Siu L. L. (2013). Tumour Heterogeneity in the Clinic . Nature 501 ( 7467 ), 355–364. 10.1038/nature12627 [ PMC free article ] [ PubMed ] [ CrossRef ] [ Google Scholar ]
- Begicevic R. R., Arfuso F., Falasca M. (2019). Bioactive Lipids in Cancer Stem Cells . World J. Stem Cell 11 ( 9 ), 693–704. 10.4252/wjsc.v11.i9.693 [ PMC free article ] [ PubMed ] [ CrossRef ] [ Google Scholar ]
- Bellomo C., Caja L., Moustakas A. (2016). Transforming Growth Factor β as Regulator of Cancer Stemness and Metastasis . Br. J. Cancer 115 ( 7 ), 761–769. 10.1038/bjc.2016.255 [ PMC free article ] [ PubMed ] [ CrossRef ] [ Google Scholar ]
- Bjerkvig R., Tysnes B. B., Aboody K. S., Najbauer J., Terzis A. J. (2005). Opinion: the Origin of the Cancer Stem Cell: Current Controversies and New Insights . Nat. Rev. Cancer 5 ( 11 ), 899–904. 10.1038/nrc1740 [ PubMed ] [ CrossRef ] [ Google Scholar ]
- Borah A., Raveendran S., Rochani A., Maekawa T., Kumar D. S. (2015). Targeting Self-Renewal Pathways in Cancer Stem Cells: Clinical Implications for Cancer Therapy . Oncogenesis 4 ( 11 ), e177. 10.1038/oncsis.2015.35 [ PMC free article ] [ PubMed ] [ CrossRef ] [ Google Scholar ]
- Chae Y. C., Kim J. H. (2018). Cancer Stem Cell Metabolism: Target for Cancer Therapy . BMB Rep. 51 ( 7 ), 319–326. 10.5483/bmbrep.2018.51.7.112 [ PMC free article ] [ PubMed ] [ CrossRef ] [ Google Scholar ]
- Chan C. H., Morrow J. K., Li C. F., Gao Y., Jin G., Moten A., et al. (2013). Pharmacological Inactivation of Skp2 SCF Ubiquitin Ligase Restricts Cancer Stem Cell Traits and Cancer Progression . Cell 154 ( 3 ), 556–568. 10.1016/j.cell.2013.06.048 [ PMC free article ] [ PubMed ] [ CrossRef ] [ Google Scholar ]
- Charmsaz S., Prencipe M., Kiely M., Pidgeon G. P., Collins D. M. (2018). Innovative Technologies Changing Cancer Treatment . Cancers (Basel) 10 ( 6 ), 208. 10.3390/cancers10060208 [ PMC free article ] [ PubMed ] [ CrossRef ] [ Google Scholar ]
- Chen K., Zhang C., Ling S., Wei R., Wang J., Xu X. (2021). The Metabolic Flexibility of Quiescent CSC: Implications for Chemotherapy Resistance . Cel Death Dis. 12 ( 9 ), 1–12. 10.1038/s41419-021-04116-6 [ PMC free article ] [ PubMed ] [ CrossRef ] [ Google Scholar ]
- Chen M., Sharma A., Lin Y., Wu Y., He Q., Gu Y., et al. (2019). Insluin and Epithelial Growth Factor (EGF) Promote Programmed Death Ligand 1(PD-L1) Production and Transport in colon Cancer Stem Cells . BMC cancer 19 ( 1 ), 153. 10.1186/s12885-019-5364-3 [ PMC free article ] [ PubMed ] [ CrossRef ] [ Google Scholar ]
- Chen X., Chen S., Yu D. (2020). Metabolic Reprogramming of Chemoresistant Cancer Cells and the Potential Significance of Metabolic Regulation in the Reversal of Cancer Chemoresistance . Metabolites 10 ( 7 ), 289. 10.3390/metabo10070289 [ PMC free article ] [ PubMed ] [ CrossRef ] [ Google Scholar ]
- Cho Y., Kim Y. K. (2020). Cancer Stem Cells as a Potential Target to Overcome Multidrug Resistance . Front. Oncol. 10 , 764. 10.3389/fonc.2020.00764 [ PMC free article ] [ PubMed ] [ CrossRef ] [ Google Scholar ]
- Chuthapisith S., Eremin J., El-Sheemey M., Eremin O. (2010). Breast Cancer Chemoresistance: Emerging Importance of Cancer Stem Cells . Surg. Oncol. 19 ( 1 ), 27–32. 10.1016/j.suronc.2009.01.004 [ PubMed ] [ CrossRef ] [ Google Scholar ]
- Cochrane C. R., Szczepny A., Watkins D. N., Cain J. E. (2015). Hedgehog Signaling in the Maintenance of Cancer Stem Cells . Cancers (Basel) 7 ( 3 ), 1554–1585. 10.3390/cancers7030851 [ PMC free article ] [ PubMed ] [ CrossRef ] [ Google Scholar ]
- Cui W., Aouidate A., Wang S., Yu Q., Li Y., Yuan S. (2020). Discovering Anti-cancer Drugs via Computational Methods . Front. Pharmacol. 11 , 733. 10.3389/fphar.2020.00733 [ PMC free article ] [ PubMed ] [ CrossRef ] [ Google Scholar ]
- Cuyàs E., Verdura S., Fernández-Arroyo S., Bosch-Barrera J., Martin-Castillo B., Joven J., et al. (2017). Metabolomic Mapping of Cancer Stem Cells for Reducing and Exploiting Tumor Heterogeneity . Oncotarget 8 ( 59 ), 99223–99236. 10.18632/oncotarget.21834 [ PMC free article ] [ PubMed ] [ CrossRef ] [ Google Scholar ]
- Dando I., Dalla Pozza E., Biondani G., Cordani M., Palmieri M., Donadelli M. (2015). The Metabolic Landscape of Cancer Stem Cells . IUBMB life 67 ( 9 ), 687–693. 10.1002/iub.1426 [ PubMed ] [ CrossRef ] [ Google Scholar ]
- De Francesco E. M., Sotgia F., Lisanti M. P. (2018). Cancer Stem Cells (CSCs): Metabolic Strategies for Their Identification and Eradication . Biochem. J. 475 ( 9 ), 1611–1634. 10.1042/BCJ20170164 [ PMC free article ] [ PubMed ] [ CrossRef ] [ Google Scholar ]
- Deshmukh A., Deshpande K., Arfuso F., Newsholme P., Dharmarajan A. (2016). Cancer Stem Cell Metabolism: a Potential Target for Cancer Therapy . Mol. Cancer 15 ( 1 ), 69–10. 10.1186/s12943-016-0555-x [ PMC free article ] [ PubMed ] [ CrossRef ] [ Google Scholar ]
- Ding S., Li C., Cheng N., Cui X., Xu X., Zhou G. (2015). Redox Regulation in Cancer Stem Cells . Oxid Med. Cel Longev 2015 , 750798. 10.1155/2015/750798 [ PMC free article ] [ PubMed ] [ CrossRef ] [ Google Scholar ]
- Dong J. J., Ying L., Shi K. Q. (2019). Expression of the Wnt Ligands Gene Family and its Relationship to Prognosis in Hepatocellular Carcinoma . Cancer Cel Int 19 ( 1 ), 34–11. 10.1186/s12935-019-0743-z [ PMC free article ] [ PubMed ] [ CrossRef ] [ Google Scholar ]
- Du F. Y., Zhou Q. F., Sun W. J., Chen G. L. (2019). Targeting Cancer Stem Cells in Drug Discovery: Current State and Future Perspectives . World J. Stem Cell 11 ( 7 ), 398–420. 10.4252/wjsc.v11.i7.398 [ PMC free article ] [ PubMed ] [ CrossRef ] [ Google Scholar ]
- Dunn J., Grider M. H. (2020). Physiology, Adenosine Triphosphate (ATP) . [ Google Scholar ]
- Falahzadeh K., Jalalvand M., Alavi-Moghadam S., Bana N., Negahdari B. (2019). “ Trying to Reveal the Mysteries of Stem Cells Using “Omics” Strategies ,” in Genomics, Proteomics, and Metabolomics (Berlin, Germany: Springer; ), 1–50. 10.1007/978-3-030-27727-7_1 [ CrossRef ] [ Google Scholar ]
- Frasson C., Rampazzo E., Accordi B., Beggio G., Pistollato F., Basso G., et al. (2015). Inhibition of PI3K Signalling Selectively Affects Medulloblastoma Cancer Stem Cells . Biomed. Research International 2015 , 973912. 10.1155/2015/973912 [ PMC free article ] [ PubMed ] [ CrossRef ] [ Google Scholar ]
- Gaggianesi M., Di Franco S., Pantina V. D., Porcelli G., D'Accardo C., Verona F., et al. (2021). Messing up the Cancer Stem Cell Chemoresistance Mechanisms Supported by Tumor Microenvironment . Front. Oncol. 2021 , 2847. 10.3389/fonc.2021.702642 [ PMC free article ] [ PubMed ] [ CrossRef ] [ Google Scholar ]
- García-Heredia J. M., Carnero A. (2020). Role of Mitochondria in Cancer Stem Cell Resistance . Cells 9 ( 7 ), 1693. [ PMC free article ] [ PubMed ] [ Google Scholar ]
- Gentric G., Mieulet V., Mechta-Grigoriou F. (2017). Heterogeneity in Cancer Metabolism: New Concepts in an Old Field . Antioxid. Redox Signal. 26 ( 9 ), 462–485. 10.1089/ars.2016.6750 [ PMC free article ] [ PubMed ] [ CrossRef ] [ Google Scholar ]
- Giacomini I., Ragazzi E., Pasut G., Montopoli M. (2020). The Pentose Phosphate Pathway and its Involvement in Cisplatin Resistance . Int. J. Mol. Sci. 21 ( 3 ), 937. 10.3390/ijms21030937 [ PMC free article ] [ PubMed ] [ CrossRef ] [ Google Scholar ]
- Gilany K., Jafarzadeh N., Mani-Varnosfaderani A., Minai-Tehrani A., Sadeghi M. R., Darbandi M., et al. (2018). Metabolic Fingerprinting of Seminal Plasma from Non-obstructive Azoospermia Patients: Positive versus Negative Sperm Retrieval . J. Reprod. Infertil 19 ( 2 ), 109–114. [ PMC free article ] [ PubMed ] [ Google Scholar ]
- Gjorevski N., Ranga A., Lutolf M. P. (2014). Bioengineering Approaches to Guide Stem Cell-Based Organogenesis . Development 141 ( 9 ), 1794–1804. 10.1242/dev.101048 [ PubMed ] [ CrossRef ] [ Google Scholar ]
- Goodarzi P., Alavi-Moghadam S., Payab M., Larijani B., Rahim F., Gilany K., et al. (2019). Metabolomics Analysis of Mesenchymal Stem Cells . Int. J. Mol. Cel Med 8 ( Suppl. 1 ), 30–40. 10.22088/IJMCM.BUMS.8.2.30 [ PMC free article ] [ PubMed ] [ CrossRef ] [ Google Scholar ]
- Gopalan V., Islam F., Lam A. K.-y. (2018). “ Surface Markers for the Identification of Cancer Stem Cells ,” in Cancer Stem Cells (Berlin, Germany: Springer; ), 17–29. 10.1007/978-1-4939-7401-6_2 [ PubMed ] [ CrossRef ] [ Google Scholar ]
- Goyal L., Hingmire S., Parikh P. M. (2006). Newer Diagnostic Methods in Oncology . Med. J. Armed Forces India 62 ( 2 ), 162–168. 10.1016/S0377-1237(06)80062-6 [ PMC free article ] [ PubMed ] [ CrossRef ] [ Google Scholar ]
- Grisanzio C., Signoretti S. (2008). p63 in Prostate Biology and Pathology . J. Cel Biochem 103 ( 5 ), 1354–1368. 10.1002/jcb.21555 [ PubMed ] [ CrossRef ] [ Google Scholar ]
- Guerra F., Arbini A. A., Moro L. (2017). Mitochondria and Cancer Chemoresistance . Biochim. Biophys. Acta Bioenerg. 1858 ( 8 ), 686–699. 10.1016/j.bbabio.2017.01.012 [ PubMed ] [ CrossRef ] [ Google Scholar ]
- Guha M., Srinivasan S., Ruthel G., Kashina A. K., Carstens R. P., Mendoza A., et al. (2014). Mitochondrial Retrograde Signaling Induces Epithelial-Mesenchymal Transition and Generates Breast Cancer Stem Cells . Oncogene 33 ( 45 ), 5238–5250. 10.1038/onc.2013.467 [ PMC free article ] [ PubMed ] [ CrossRef ] [ Google Scholar ]
- Hongwiangchan N., Sriratanasak N., Wichadakul D., Aksorn N., Chamni S., Chanvorachote P. (2021). Hydroquinone 5-O-Cinnamoyl Ester of Renieramycin M Suppresses Lung Cancer Stem Cells by Targeting Akt and Destabilizes C-Myc . Pharmaceuticals (Basel) 14 ( 11 ), 1112. 10.3390/ph14111112 [ PMC free article ] [ PubMed ] [ CrossRef ] [ Google Scholar ]
- Hughes J. P., Rees S., Kalindjian S. B., Philpott K. L. (2011). Principles of Early Drug Discovery . Br. J. Pharmacol. 162 ( 6 ), 1239–1249. 10.1111/j.1476-5381.2010.01127.x [ PMC free article ] [ PubMed ] [ CrossRef ] [ Google Scholar ]
- Hung K. F., Yang T., Kao S. Y. (2019). Cancer Stem Cell Theory: Are We Moving Past the Mist? J. Chin. Med. Assoc. 82 ( 11 ), 814–818. 10.1097/JCMA.0000000000000186 [ PubMed ] [ CrossRef ] [ Google Scholar ]
- Jagust P., de Luxán-Delgado B., Parejo-Alonso B., Sancho P. (2019b). Metabolism-based Therapeutic Strategies Targeting Cancer Stem Cells . Front. Pharmacol. 10 , 203. 10.3389/fphar.2019.00203 [ PMC free article ] [ PubMed ] [ CrossRef ] [ Google Scholar ]
- Jagust P., de Luxán-Delgado B., Parejo-Alonso B., Sancho P. (2019). Metabolism-Based Therapeutic Strategies Targeting Cancer Stem Cells . Front. Pharmacol. 10 , 203. 10.3389/fphar.2019.00203 [ PMC free article ] [ PubMed ] [ CrossRef ] [ Google Scholar ]
- Jaitak V. (2016). Molecular Docking Study of Natural Alkaloids as Multi-Targeted Hedgehog Pathway Inhibitors in Cancer Stem Cell Therapy . Comput. Biol. Chem. 62 , 145–154. [ PubMed ] [ Google Scholar ]
- Jin W. (2020). Role of JAK/STAT3 Signaling in the Regulation of Metastasis, the Transition of Cancer Stem Cells, and Chemoresistance of Cancer by Epithelial-Mesenchymal Transition . Cells 9 ( 1 ), 217. 10.3390/cells9010217 [ PMC free article ] [ PubMed ] [ CrossRef ] [ Google Scholar ]
- Jin X., Jin X., Kim H. (2017). Cancer Stem Cells and Differentiation Therapy . Tumour Biol. 39 ( 10 ), 1010428317729933. 10.1177/1010428317729933 [ PubMed ] [ CrossRef ] [ Google Scholar ]
- Karamboulas C., Ailles L. (2013). Developmental Signaling Pathways in Cancer Stem Cells of Solid Tumors . Biochim. Biophys. Acta 1830 ( 2 ), 2481–2495. 10.1016/j.bbagen.2012.11.008 [ PubMed ] [ CrossRef ] [ Google Scholar ]
- Kershaw N. M., Wright G. S., Sharma R., Antonyuk S. V., Strange R. W., Berry N. G., et al. (2013). X-ray Crystallography and Computational Docking for the Detection and Development of Protein-Ligand Interactions . Curr. Med. Chem. 20 ( 4 ), 569–575. 10.2174/0929867311320040008 [ PubMed ] [ CrossRef ] [ Google Scholar ]
- Khatami F., Tavangar S. M., Pour N. K. (2019). “ Genomics, Proteomics, and Metabolomics of Cancer Stem Cells (CSCs) ,” in Genomics, Proteomics, and Metabolomics (Berlin, Germany: Springer; ), 159–179. 10.1007/978-3-030-27727-7_9 [ CrossRef ] [ Google Scholar ]
- Klonisch T., Wiechec E., Hombach-Klonisch S., Ande S. R., Wesselborg S., Schulze-Osthoff K., et al. (2008). Cancer Stem Cell Markers in Common Cancers - Therapeutic Implications . Trends Mol. Med. 14 ( 10 ), 450–460. 10.1016/j.molmed.2008.08.003 [ PubMed ] [ CrossRef ] [ Google Scholar ]
- Koury J., Zhong L., Hao J. (2017). Targeting Signaling Pathways in Cancer Stem Cells for Cancer Treatment . Stem Cell Int. 2017 , 2925869. 10.1155/2017/2925869 [ PMC free article ] [ PubMed ] [ CrossRef ] [ Google Scholar ]
- Krstic J., Trivanovic D., Jaukovic A., Santibanez J. F., Bugarski D. (2017). Metabolic Plasticity of Stem Cells and Macrophages in Cancer . Front. Immunol. 8 , 939. 10.3389/fimmu.2017.00939 [ PMC free article ] [ PubMed ] [ CrossRef ] [ Google Scholar ]
- Kumar A., Bhanja A., Bhattacharyya J., Jaganathan B. G. (2016). Multiple Roles of CD90 in Cancer . Tumour Biol. 37 ( 9 ), 11611–11622. 10.1007/s13277-016-5112-0 [ PubMed ] [ CrossRef ] [ Google Scholar ]
- Kumar D., Shankar S., Srivastava R. K. (2013). Rottlerin-induced Autophagy Leads to the Apoptosis in Breast Cancer Stem Cells: Molecular Mechanisms . Mol. Cancer 12 ( 1 ), 171. 10.1186/1476-4598-12-171 [ PMC free article ] [ PubMed ] [ CrossRef ] [ Google Scholar ]
- Kuramoto K., Yamamoto M., Suzuki S., Togashi K., Sanomachi T., Kitanaka C., et al. (2021). Inhibition of the Lipid Droplet-Peroxisome Proliferator-Activated Receptor α Axis Suppresses Cancer Stem Cell Properties . Genes (Basel) 12 ( 1 ), 99. 10.3390/genes12010099 [ PMC free article ] [ PubMed ] [ CrossRef ] [ Google Scholar ]
- Kurutas E. B. (2016). The Importance of Antioxidants Which Play the Role in Cellular Response against Oxidative/nitrosative Stress: Current State . Nutr. J. 15 ( 1 ), 71. 10.1186/s12937-016-0186-5 [ PMC free article ] [ PubMed ] [ CrossRef ] [ Google Scholar ]
- Larijani B., Goodarzi P., Payab M., Alavi-Moghadam S., Rahim F., Bana N., et al. (2019). Metabolomics and Cell Therapy in Diabetes Mellitus . Int. J. Mol. Cel Med 8 ( Suppl. 1 ), 41–48. 10.22088/IJMCM.BUMS.8.2.41 [ PMC free article ] [ PubMed ] [ CrossRef ] [ Google Scholar ]
- Lee S. H., Reed-Newman T., Anant S., Ramasamy T. S. (2020). Regulatory Role of Quiescence in the Biological Function of Cancer Stem Cells . Stem Cel Rev Rep 16 ( 6 ), 1185–1207. 10.1007/s12015-020-10031-8 [ PubMed ] [ CrossRef ] [ Google Scholar ]
- Li L., Bhatia R. (2011). Stem Cell Quiescence . Clin. Cancer Res. 17 ( 15 ), 4936–4941. 10.1158/1078-0432.CCR-10-1499 [ PMC free article ] [ PubMed ] [ CrossRef ] [ Google Scholar ]
- Liao C., Peach M. L., Yao R., Nicklaus M. C. (2013). “ Molecular Docking and Structure-Based Virtual Screening ,” in Future Medicine . 10.4155/ebo.13.181 [ CrossRef ] [ Google Scholar ]
- Liou G. Y., Storz P. (2010). Reactive Oxygen Species in Cancer . Free Radic. Res. 44 ( 5 ), 479–496. 10.3109/10715761003667554 [ PMC free article ] [ PubMed ] [ CrossRef ] [ Google Scholar ]
- Liu R., Chen Y., Liu G., Li C., Song Y., Cao Z., et al. (2020). PI3K/AKT Pathway as a Key Link Modulates the Multidrug Resistance of Cancers . Cell Death Dis 11 ( 9 ), 797. 10.1038/s41419-020-02998-6 [ PMC free article ] [ PubMed ] [ CrossRef ] [ Google Scholar ]
- Liu S., Chen S., Zeng J. (2018). TGF-β S-ignaling: A C-omplex R-ole in T-umorigenesis (Review) . Mol. Med. Rep. 17 ( 1 ), 699–704. 10.3892/mmr.2017.7970 [ PubMed ] [ CrossRef ] [ Google Scholar ]
- Liu Y., Liu X., Chen L.-C., Du W.-Z., Cui Y.-Q., Piao X.-Y., et al. (2014). Targeting Glioma Stem Cells via the Hedgehog Signaling Pathway . Neuroimmunology and Neuroinflammation 1 , 51–59. [ Google Scholar ]
- Lobo N. A., Shimono Y., Qian D., Clarke M. F. (2007). The Biology of Cancer Stem Cells . Annu. Rev. Cel Dev. Biol. 23 , 675–699. 10.1146/annurev.cellbio.22.010305.104154 [ PubMed ] [ CrossRef ] [ Google Scholar ]
- Lohning A. E., Levonis S. M., Williams-Noonan B., Schweiker S. S. (2017). A Practical Guide to Molecular Docking and Homology Modelling for Medicinal Chemists . Curr. Top. Med. Chem. 17 ( 18 ), 2023–2040. 10.2174/1568026617666170130110827 [ PubMed ] [ CrossRef ] [ Google Scholar ]
- Luo M., Wicha M. S. (2015). Metabolic Plasticity of Cancer Stem Cells . Oncotarget 6 ( 34 ), 35141–35142. 10.18632/oncotarget.6177 [ PMC free article ] [ PubMed ] [ CrossRef ] [ Google Scholar ]
- Madhavilatha K. N., Babu G. R. M. (2019). Systematic Approach for Enrichment of Docking Outcome Using Consensus Scoring Functions . J. Phys. Conf. Ser. 1228 , 012019. 10.1088/1742-6596/1228/1/012019 [ CrossRef ] [ Google Scholar ]
- Madhunapantula S. V., Mosca P. J., Robertson G. P. (2011). The Akt Signaling Pathway: an Emerging Therapeutic Target in Malignant Melanoma . Cancer Biol. Ther. 12 ( 12 ), 1032–1049. 10.4161/cbt.12.12.18442 [ PMC free article ] [ PubMed ] [ CrossRef ] [ Google Scholar ]
- Mancini R., Noto A., Pisanu M. E., De Vitis C., Maugeri-Saccà M., Ciliberto G. (2018). Metabolic Features of Cancer Stem Cells: the Emerging Role of Lipid Metabolism . Oncogene 37 ( 18 ), 2367–2378. 10.1038/s41388-018-0141-3 [ PubMed ] [ CrossRef ] [ Google Scholar ]
- Matsui W. H. (2016). Cancer Stem Cell Signaling Pathways . Medicine (Baltimore) 95 ( Suppl. 1 ), S8. 10.1097/MD.0000000000004765 [ PMC free article ] [ PubMed ] [ CrossRef ] [ Google Scholar ]
- Meng X. Y., Zhang H. X., Mezei M., Cui M. (2011). Molecular Docking: a Powerful Approach for Structure-Based Drug Discovery . Curr. Comput. Aided Drug Des. 7 ( 2 ), 146–157. 10.2174/157340911795677602 [ PMC free article ] [ PubMed ] [ CrossRef ] [ Google Scholar ]
- Menke J., Maskri S., Koch O. (2021). Computational Ion Channel Research: from the Application of Artificial Intelligence to Molecular Dynamics Simulations . Cell Physiol. Biochem. Int. J. Exp. Cell. Physiol. Biochem. Pharmacol. 55 ( S3 ), 14–45. [ PubMed ] [ Google Scholar ]
- Miricescu D., Totan A., Stanescu-Spinu I.-I., Badoiu S. C., Stefani C., Greabu M. (2021). PI3K/AKT/mTOR Signaling Pathway in Breast Cancer: From Molecular Landscape to Clinical Aspects . Int. J. Mol. Sci. 22 ( 1 ), 173. 10.3390/ijms22179512 [ PMC free article ] [ PubMed ] [ CrossRef ] [ Google Scholar ]
- Mohr M., Zänker K. S., Dittmar T. (2015). Cancer (Stem) Cell Differentiation: An Inherent or Acquired Property? Med. Hypotheses 85 ( 6 ), 1012–1018. 10.1016/j.mehy.2015.08.017 [ PubMed ] [ CrossRef ] [ Google Scholar ]
- Mohs R. C., Greig N. H. (2017). Drug Discovery and Development: Role of Basic Biological Research . Alzheimers Dement (N Y) 3 ( 4 ), 651–657. 10.1016/j.trci.2017.10.005 [ PMC free article ] [ PubMed ] [ CrossRef ] [ Google Scholar ]
- Morris G. M., Lim-Wilby M. (2008). “ Molecular Docking ,” in Molecular Modeling of Proteins (Berlin, Germany: Springer; ), 365–382. 10.1007/978-1-59745-177-2_19 [ PubMed ] [ CrossRef ] [ Google Scholar ]
- Mukha A., Dubrovska A. (2020). Metabolic Targeting of Cancer Stem Cells . Front. Oncol. 10 , 537930. 10.3389/fonc.2020.537930 [ PMC free article ] [ PubMed ] [ CrossRef ] [ Google Scholar ]
- Najafi M., Farhood B., Mortezaee K. (2019). Cancer Stem Cells (CSCs) in Cancer Progression and Therapy . J. Cel Physiol 234 ( 6 ), 8381–8395. 10.1002/jcp.27740 [ PubMed ] [ CrossRef ] [ Google Scholar ]
- Niehrs C. (2012). The Complex World of WNT Receptor Signalling . Nat. Rev. Mol. Cel Biol 13 ( 12 ), 767–779. 10.1038/nrm3470 [ PubMed ] [ CrossRef ] [ Google Scholar ]
- Nunes T., Hamdan D., Leboeuf C., El Bouchtaoui M., Gapihan G., Nguyen T. T., et al. (2018). Targeting Cancer Stem Cells to Overcome Chemoresistance . Int. J. Mol. Sci. 19 ( 12 ), 4036. 10.3390/ijms19124036 [ PMC free article ] [ PubMed ] [ CrossRef ] [ Google Scholar ]
- Organization W. H. (2020). WHO Report on Cancer: Setting Priorities, Investing Wisely and Providing Care for All . Geneve, Switzerland: WHO. [ Google Scholar ]
- Ozsvari B., Sotgia F., Simmons K., Trowbridge R., Foster R., Lisanti M. P. (2017). Mitoketoscins: Novel Mitochondrial Inhibitors for Targeting Ketone Metabolism in Cancer Stem Cells (CSCs) . Oncotarget 8 ( 45 ), 78340–78350. 10.18632/oncotarget.21259 [ PMC free article ] [ PubMed ] [ CrossRef ] [ Google Scholar ]
- Pagadala N. S., Syed K., Tuszynski J. (2017). Software for Molecular Docking: a Review . Biophys. Rev. 9 ( 2 ), 91–102. 10.1007/s12551-016-0247-1 [ PMC free article ] [ PubMed ] [ CrossRef ] [ Google Scholar ]
- Peixoto J., Lima J. (2018). Metabolic Traits of Cancer Stem Cells . Dis. Model. Mech. 11 ( 8 ), 33464. 10.1242/dmm.033464 [ PMC free article ] [ PubMed ] [ CrossRef ] [ Google Scholar ]
- Phi L. T. H., Sari I. N., Yang Y.-G., Lee S.-H., Jun N., Kim K. S., et al. (2018). Cancer Stem Cells (CSCs) in Drug Resistance and Their Therapeutic Implications in Cancer Treatment . Stem Cell Int. 2018 , 5416923. 10.1155/2018/5416923 [ PMC free article ] [ PubMed ] [ CrossRef ] [ Google Scholar ]
- Phillips M. A., Stewart M. A., Woodling D. L., Xie Z.-R. (2018). Has Molecular Docking Ever Brought us a Medicine. Molecular Docking . London, UK: IntechOpen, 141–178. [ Google Scholar ]
- Piraino S. W., Thomas V., O’Donovan P., Furney S. J. (2019). Mutations: Driver versus Passenger . [ Google Scholar ]
- Polat I. H., Tarrado-Castellarnau M., Bharat R., Perarnau J., Benito A., Cortés R., et al. (2021). Oxidative Pentose Phosphate Pathway Enzyme 6-Phosphogluconate Dehydrogenase Plays a Key Role in Breast Cancer Metabolism . Biology (Basel) 10 ( 2 ), 85. 10.3390/biology10020085 [ PMC free article ] [ PubMed ] [ CrossRef ] [ Google Scholar ]
- Poljsak B., Šuput D., Milisav I. (2013). Achieving the Balance between ROS and Antioxidants: when to Use the Synthetic Antioxidants . Oxid Med. Cel Longev 2013 , 956792. 10.1155/2013/956792 [ PMC free article ] [ PubMed ] [ CrossRef ] [ Google Scholar ]
- Prager B. C., Xie Q., Bao S., Rich J. N. (2019). Cancer Stem Cells: the Architects of the Tumor Ecosystem . Cell Stem Cell 24 ( 1 ), 41–53. 10.1016/j.stem.2018.12.009 [ PMC free article ] [ PubMed ] [ CrossRef ] [ Google Scholar ]
- Prasetyanti P. R., Medema J. P. (2017). Intra-tumor Heterogeneity from a Cancer Stem Cell Perspective . Mol. Cancer 16 ( 1 ), 41–49. 10.1186/s12943-017-0600-4 [ PMC free article ] [ PubMed ] [ CrossRef ] [ Google Scholar ]
- Pucci C., Martinelli C., Ciofani G. (2019). Innovative Approaches for Cancer Treatment: Current Perspectives and New Challenges . Ecancermedicalscience 13 , 961. 10.3332/ecancer.2019.961 [ PMC free article ] [ PubMed ] [ CrossRef ] [ Google Scholar ]
- Rahim F., Arjmand B., Shirbandi K., Payab M., Larijani B. (2018). Stem Cell Therapy for Patients with Diabetes: a Systematic Review and Meta-Analysis of Metabolomics-Based Risks and Benefits . Stem Cel Investig 5 , 40. 10.21037/sci.2018.11.01 [ PMC free article ] [ PubMed ] [ CrossRef ] [ Google Scholar ]
- Ramón Y Cajal S., Sesé M., Capdevila C., Aasen T., De Mattos-Arruda L., Diaz-Cano S. J., et al. (2020). Clinical Implications of Intratumor Heterogeneity: Challenges and Opportunities . J. Mol. Med. (Berl) 98 ( 2 ), 161–177. 10.1007/s00109-020-01874-2 [ PMC free article ] [ PubMed ] [ CrossRef ] [ Google Scholar ]
- Reddy A. S., Pati S. P., Kumar P. P., Pradeep H. N., Sastry G. N. (2007). Virtual Screening in Drug Discovery -- a Computational Perspective . Curr. Protein Pept. Sci. 8 ( 4 ), 329–351. 10.2174/138920307781369427 [ PubMed ] [ CrossRef ] [ Google Scholar ]
- Riganti C., Gazzano E., Polimeni M., Aldieri E., Ghigo D. (2012). The Pentose Phosphate Pathway: an Antioxidant Defense and a Crossroad in Tumor Cell Fate . Free Radic. Biol. Med. 53 ( 3 ), 421–436. 10.1016/j.freeradbiomed.2012.05.006 [ PubMed ] [ CrossRef ] [ Google Scholar ]
- Salmaso V., Moro S. (2018). Bridging Molecular Docking to Molecular Dynamics in Exploring Ligand-Protein Recognition Process: An Overview . Front. Pharmacol. 9 ( 923 ), 923. 10.3389/fphar.2018.00923 [ PMC free article ] [ PubMed ] [ CrossRef ] [ Google Scholar ]
- Schöning J. P., Monteiro M., Gu W. (2017). Drug Resistance and Cancer Stem Cells: the Shared but Distinct Roles of Hypoxia‐inducible Factors HIF 1α and HIF 2α . Clin. Exp. Pharmacol. Physiol. 44 ( 2 ), 153–161. [ PubMed ] [ Google Scholar ]
- Sethi A., Joshi K., Sasikala K., Alvala M. (2019). Molecular Docking in Modern Drug Discovery: Principles and Recent Applications . Drug Discov. Develop. New Adv 2019 , 27–39. [ Google Scholar ]
- Shah S. C., Kayamba V., Peek R. M., Jr, Heimburger D. (2019). Cancer Control in Low- and Middle-Income Countries: Is it Time to Consider Screening? J. Glob. Oncol. 5 , 1–8. 10.1200/JGO.18.00200 [ PMC free article ] [ PubMed ] [ CrossRef ] [ Google Scholar ]
- Shi X., Zhang Y., Zheng J., Pan J. (2012). Reactive Oxygen Species in Cancer Stem Cells . Antioxid. Redox Signal. 16 ( 11 ), 1215–1228. 10.1089/ars.2012.4529 [ PMC free article ] [ PubMed ] [ CrossRef ] [ Google Scholar ]
- Shin M. K., Cheong J. H. (2019). Mitochondria-centric Bioenergetic Characteristics in Cancer Stem-like Cells . Arch. Pharm. Res. 42 ( 2 ), 113–127. 10.1007/s12272-019-01127-y [ PMC free article ] [ PubMed ] [ CrossRef ] [ Google Scholar ]
- Shyh-Chang N., Ng H. H. (2017). The Metabolic Programming of Stem Cells . Genes Dev. 31 ( 4 ), 336–346. 10.1101/gad.293167.116 [ PMC free article ] [ PubMed ] [ CrossRef ] [ Google Scholar ]
- Singh A., Settleman J. (2010). EMT, Cancer Stem Cells and Drug Resistance: an Emerging axis of Evil in the War on Cancer . Oncogene 29 ( 34 ), 4741–4751. 10.1038/onc.2010.215 [ PMC free article ] [ PubMed ] [ CrossRef ] [ Google Scholar ]
- Snaebjornsson M. T., Janaki-Raman S., Schulze A. (2020). Greasing the Wheels of the Cancer Machine: the Role of Lipid Metabolism in Cancer . Cell Metab 31 ( 1 ), 62–76. 10.1016/j.cmet.2019.11.010 [ PubMed ] [ CrossRef ] [ Google Scholar ]
- Snyder V., Reed-Newman T. C., Arnold L., Thomas S. M., Anant S. (2018). Cancer Stem Cell Metabolism and Potential Therapeutic Targets . Front. Oncol. 8 , 203. 10.3389/fonc.2018.00203 [ PMC free article ] [ PubMed ] [ CrossRef ] [ Google Scholar ]
- Sturlese M., Bellanda M., Moro S. (2015). NMR-assisted Molecular Docking Methodologies . Mol. Inform. 34 ( 8 ), 513–525. 10.1002/minf.201500012 [ PubMed ] [ CrossRef ] [ Google Scholar ]
- Sung H., Ferlay J., Siegel R. L., Laversanne M., Soerjomataram I., Jemal A., et al. (2021). Global Cancer Statistics 2020: GLOBOCAN Estimates of Incidence and Mortality Worldwide for 36 Cancers in 185 Countries . CA A. Cancer J. Clin. 71 ( 3 ), 209–249. 10.3322/caac.21660 [ PubMed ] [ CrossRef ] [ Google Scholar ]
- Tamada M., Nagano O., Tateyama S., Ohmura M., Yae T., Ishimoto T., et al. (2012). Modulation of Glucose Metabolism by CD44 Contributes to Antioxidant Status and Drug Resistance in Cancer Cells . Cancer Res. 72 ( 6 ), 1438–1448. 10.1158/0008-5472.CAN-11-3024 [ PubMed ] [ CrossRef ] [ Google Scholar ]
- Tang C., Ang B. T., Pervaiz S. (2007). Cancer Stem Cell: Target for Anti-cancer Therapy . FASEB J. 21 ( 14 ), 3777–3785. 10.1096/fj.07-8560rev [ PubMed ] [ CrossRef ] [ Google Scholar ]
- Tayanloo-Beik A., Sarvari M., Payab M., Gilany K., Alavi-Moghadam S., Gholami M., et al. (2020). OMICS Insights into Cancer Histology; Metabolomics and Proteomics Approach . Clin. Biochem. 84 , 13–20. 10.1016/j.clinbiochem.2020.06.008 [ PubMed ] [ CrossRef ] [ Google Scholar ]
- Thomas M., Coyle K., Sultan M., Vaghar-Kashani A., Marcato P. (2014). Chemoresistance in Cancer Stem Cells and Strategies to Overcome Resistance . Chemotherapy 3 ( 125 ), 2. [ Google Scholar ]
- Tirino V., Desiderio V., Paino F., De Rosa A., Papaccio F., La Noce M., et al. (2013). Cancer Stem Cells in Solid Tumors: an Overview and New Approaches for Their Isolation and Characterization . FASEB J. 27 ( 1 ), 13–24. 10.1096/fj.12-218222 [ PubMed ] [ CrossRef ] [ Google Scholar ]
- Toh T. B., Lim J. J., Chow E. K. (2017). Epigenetics in Cancer Stem Cells . Mol. Cancer 16 ( 1 ), 29–20. 10.1186/s12943-017-0596-9 [ PMC free article ] [ PubMed ] [ CrossRef ] [ Google Scholar ]
- Torres P. H. M., Sodero A. C. R., Jofily P., Silva-Jr F. P., Jr (2019). Key Topics in Molecular Docking for Drug Design . Int. J. Mol. Sci. 20 ( 18 ), 4574. 10.3390/ijms20184574 [ PMC free article ] [ PubMed ] [ CrossRef ] [ Google Scholar ]
- Turnquist C., Watson R. A., Protheroe A., Verrill C., Sivakumar S. (2019). Tumor Heterogeneity: Does it Matter? Expert Rev. Anticancer Ther. 19 ( 10 ), 857–867. 10.1080/14737140.2019.1667236 [ PubMed ] [ CrossRef ] [ Google Scholar ]
- Tyagi S., Gupta P., Saini A. S., Kaushal C., Sharma S. (2011). The Peroxisome Proliferator-Activated Receptor: A Family of Nuclear Receptors Role in Various Diseases . J. Adv. Pharm. Technol. Res. 2 ( 4 ), 236–240. 10.4103/2231-4040.90879 [ PMC free article ] [ PubMed ] [ CrossRef ] [ Google Scholar ]
- Vineis P., Wild C. P. (2014). Global Cancer Patterns: Causes and Prevention . Lancet 383 ( 9916 ), 549–557. 10.1016/S0140-6736(13)62224-2 [ PubMed ] [ CrossRef ] [ Google Scholar ]
- Visweswaran M., Arfuso F., Warrier S., Dharmarajan A. (2020). Aberrant Lipid Metabolism as an Emerging Therapeutic Strategy to Target Cancer Stem Cells . Stem cells 38 ( 1 ), 6–14. 10.1002/stem.3101 [ PubMed ] [ CrossRef ] [ Google Scholar ]
- Wanandi S. I., Limanto A., Yunita E., Syahrani R. A., Louisa M., Wibowo A. E., et al. (2020). In Silico and In Vitro Studies on the Anti-cancer Activity of Andrographolide Targeting Survivin in Human Breast Cancer Stem Cells . PloS one 15 ( 11 ), e0240020. 10.1371/journal.pone.0240020 [ PMC free article ] [ PubMed ] [ CrossRef ] [ Google Scholar ]
- Wang A., Chen L., Li C., Zhu Y. (2015). Heterogeneity in Cancer Stem Cells . Cancer Lett. 357 ( 1 ), 63–68. 10.1016/j.canlet.2014.11.040 [ PubMed ] [ CrossRef ] [ Google Scholar ]
- Wang R., Lu Y., Wang S. (2003). Comparative Evaluation of 11 Scoring Functions for Molecular Docking . J. Med. Chem. 46 ( 12 ), 2287–2303. 10.1021/jm0203783 [ PubMed ] [ CrossRef ] [ Google Scholar ]
- Xia P., Xu X. Y. (2015). PI3K/Akt/mTOR Signaling Pathway in Cancer Stem Cells: from Basic Research to Clinical Application . Am. J. Cancer Res. 5 ( 5 ), 1602–1609. [ PMC free article ] [ PubMed ] [ Google Scholar ]
- Yadav U. P., Singh T., Kumar P., Sharma P., Kaur H., Sharma S., et al. (2020). Metabolic Adaptations in Cancer Stem Cells . Front. Oncol. 10 , 1010. 10.3389/fonc.2020.01010 [ PMC free article ] [ PubMed ] [ CrossRef ] [ Google Scholar ]
- Yang L., Shi P., Zhao G., Xu J., Peng W., Zhang J., et al. (2020). Targeting Cancer Stem Cell Pathways for Cancer Therapy . Signal. Transduct Target. Ther. 5 ( 1 ), 8–35. 10.1038/s41392-020-0110-5 [ PMC free article ] [ PubMed ] [ CrossRef ] [ Google Scholar ]
- Yang R., Lu M., Ming L., Chen Y., Cheng K., Zhou J., et al. (2020). 89Zr-Labeled Multifunctional Liposomes Conjugate Chitosan for PET-Trackable Triple-Negative Breast Cancer Stem Cell Targeted Therapy . Int. J. Nanomedicine 15 , 9061–9074. 10.2147/IJN.S262786 [ PMC free article ] [ PubMed ] [ CrossRef ] [ Google Scholar ]
- Yang Y., Li X., Wang T., Guo Q., Xi T., Zheng L. (2020). Emerging Agents that Target Signaling Pathways in Cancer Stem Cells . J. Hematol. Oncol. 13 , 60–18. 10.1186/s13045-020-00901-6 [ PMC free article ] [ PubMed ] [ CrossRef ] [ Google Scholar ]
- Yi M., Li J., Chen S., Cai J., Ban Y., Peng Q., et al. (2018). Emerging Role of Lipid Metabolism Alterations in Cancer Stem Cells . J. Exp. Clin. Cancer Res. 37 ( 1 ), 1–18. 10.1186/s13046-018-0784-5 [ PMC free article ] [ PubMed ] [ CrossRef ] [ Google Scholar ]
- Yu S. S., Cirillo N. (2020). The Molecular Markers of Cancer Stem Cells in Head and Neck Tumors . J. Cel Physiol 235 ( 1 ), 65–73. 10.1002/jcp.28963 [ PubMed ] [ CrossRef ] [ Google Scholar ]
- Zhang B. B., Wang D. G., Guo F. F., Xuan C. (2015). Mitochondrial Membrane Potential and Reactive Oxygen Species in Cancer Stem Cells . Fam. Cancer 14 ( 1 ), 19–23. 10.1007/s10689-014-9757-9 [ PubMed ] [ CrossRef ] [ Google Scholar ]
- Zhao J. (2016). Cancer Stem Cells and Chemoresistance: The Smartest Survives the Raid . Pharmacol. Ther. 160 , 145–158. 10.1016/j.pharmthera.2016.02.008 [ PMC free article ] [ PubMed ] [ CrossRef ] [ Google Scholar ]
- Zhou D., Shao L., Spitz D. R. (2014). Reactive Oxygen Species in normal and Tumor Stem Cells . Adv. Cancer Res. 122 , 1–67. 10.1016/B978-0-12-420117-0.00001-3 [ PMC free article ] [ PubMed ] [ CrossRef ] [ Google Scholar ]
- Zhu P., Fan Z. (2018). Cancer Stem Cells and Tumorigenesis . Biophys. Rep. 4 ( 4 ), 178–188. 10.1007/s41048-018-0062-2 [ PMC free article ] [ PubMed ] [ CrossRef ] [ Google Scholar ]
- Zhu X., Chen H. H., Gao C. Y., Zhang X. X., Jiang J. X., Zhang Y., et al. (2020). Energy Metabolism in Cancer Stem Cells . World J. Stem Cell 12 ( 6 ), 448–461. 10.4252/wjsc.v12.i6.448 [ PMC free article ] [ PubMed ] [ CrossRef ] [ Google Scholar ]
- Zong W. X., Rabinowitz J. D., White E. (2016). Mitochondria and Cancer . Mol. Cel 61 ( 5 ), 667–676. 10.1016/j.molcel.2016.02.011 [ PMC free article ] [ PubMed ] [ CrossRef ] [ Google Scholar ]

Chemical Society Reviews
Exploring pathological link between antimicrobial and amyloid peptides.

* Corresponding authors
a Department of Chemical, Biomolecular, and Corrosion Engineering, The University of Akron, Ohio 44325, USA E-mail: [email protected]
b Division of Endocrinology and Diabetes, Department of Pediatrics, School of Medicine, Stanford University, Palo Alto, CA 94304, USA
c The Wallace H. Coulter Department of Biomedical Engineering, Georgia Institute of Technology and Emory University, Atlanta, Georgia 30332, USA
d Cancer Innovation Laboratory, National Cancer Institute, Frederick, MD 21702, USA
e Computational Structural Biology Section, Frederick National Laboratory for Cancer Research, Frederick, MD 21702, USA E-mail: [email protected]
f Department of Human Molecular Genetics and Biochemistry Sackler School of Medicine, Tel Aviv University, Tel Aviv 69978, Israel
Amyloid peptides (AMYs) and antimicrobial peptides (AMPs) are considered as the two distinct families of peptides, characterized by their unique sequences, structures, biological functions, and specific pathological targets. However, accumulating evidence has revealed intriguing pathological connections between these peptide families in the context of microbial infection and neurodegenerative diseases. Some AMYs and AMPs share certain structural and functional characteristics, including the ability to self-assemble, the presence of β-sheet-rich structures, and membrane-disrupting mechanisms. These shared features enable AMYs to possess antimicrobial activity and AMPs to acquire amyloidogenic properties. Despite limited studies on AMYs–AMPs systems, the cross-seeding phenomenon between AMYs and AMPs has emerged as a crucial factor in the bidirectional communication between the pathogenesis of neurodegenerative diseases and host defense against microbial infections. In this review, we examine recent developments in the potential interplay between AMYs and AMPs, as well as their pathological implications for both infectious and neurodegenerative diseases. By discussing the current progress and challenges in this emerging field, this account aims to inspire further research and investments to enhance our understanding of the intricate molecular crosstalk between AMYs and AMPs. This knowledge holds great promise for the development of innovative therapies to combat both microbial infections and neurodegenerative disorders.
Article information

Download Citation
Permissions.

Y. Tang, Y. Zhang, D. Zhang, Y. Liu, R. Nussinov and J. Zheng, Chem. Soc. Rev. , 2024, Advance Article , DOI: 10.1039/D3CS00878A
This article is licensed under a Creative Commons Attribution 3.0 Unported Licence . You can use material from this article in other publications without requesting further permissions from the RSC, provided that the correct acknowledgement is given.
Read more about how to correctly acknowledge RSC content .
Social activity
Search articles by author.
This article has not yet been cited.
Advertisements

Method enables fast, accurate estimates of cardiovascular state to inform blood pressure management
If patients receiving intensive care or undergoing major surgery develop excessively high or low blood pressures, they could suffer severe organ dysfunction. It's not enough for their care team to know that pressure is abnormal. To choose the correct drug to treat the problem, doctors must know why blood pressure has changed. A new MIT study presents the mathematical framework needed to derive that crucial information accurately and in real time.
The mathematical approach, described in a recent study in IEEE Transactions on Biomedical Engineering , produces proportional estimates of the two critical factors underlying blood pressure changes: the heart's rate of blood output (cardiac output) and the arterial system's resistance to that blood flow (systemic vascular resistance). By applying the new method to previously collected data from animal models, the researchers show that their estimates, derived from minimally invasive measures of peripheral arterial blood pressure, accurately matched estimates using additional information from an invasive flow probe placed on the aorta. Moreover, the estimates accurately tracked the changes induced in the animals by the various drugs physicians use to correct aberrant blood pressure.
"Estimates of resistance and cardiac output from our approach provide information that can readily be used to guide hemodynamic management decisions in real time," the study authors wrote.
With further testing leading to regulatory approval, the authors said, the method would be applicable during heart surgeries, liver transplants, intensive care unit treatment and many other procedures affecting cardiovascular function or blood volume.
"Any patient who is having cardiac surgery could need this," said study senior author Emery N. Brown, Edward Hood Taplin Professor of Medical Engineering and Computational Neuroscience in The Picower Institute for Learning and Memory, the Institute for Medical Engineering and Science and the Department of Brain and Cognitive Sciences at MIT. Brown is also an anesthesiologist at Massachusetts General Hospital and a professor of anesthesiology at Harvard Medical School. "So might any patient undergoing a more normal surgery but who might have a compromised cardiovascular system such as ischemic heart disease. You can't have the blood pressure being all over the place."
The study's lead author is electrical engineering and computer science (EECS) graduate student Taylor Baum, who is co-supervised by Brown and Munther Dahleh, William A. Coolidge Professor in EECS.
Algorithmic advance
The idea that cardiac output and systemic resistance are the two key components of blood pressure comes from the two-element Windkessel model. The new study is not the first to use the model to estimate these components from blood pressure measurements, but previous attempts ran into a tradeoff between quick estimate updates and the accuracy of estimates; methods would either provide more erroneous estimates at every beat or more reliable estimates that are updated at minute time scales. Led by Baum, the MIT team overcame the tradeoff with a new approach of applying statistical and signal processing techniques such as "state-space" modeling.
"Our estimates, updated at every beat, are not just informed by the current beat; but they incorporate where things were in previous beats as well," Baum said. "It's that combination of past history and current observations that produces a more reliable estimate while still at a beat-by-beat time scale."
Notably, the resulting estimates of cardiac output and systemic resistance are "proportional," meaning that they are each inextricably linked in the math with another co-factor, rather than estimated on their own. But application of the new method to data collected in an older study from six animals showed that the proportional estimates from recordings using minimally invasive catheters provide comparable information for cardiovascular system management.
One key finding was that the proportional estimates made based on arterial blood pressure readings from catheters inserted in various locations away from the heart (e.g., the leg or the arm) mirrored estimates derived from more invasive catheters placed within the aorta. The significance of the finding is that a system using the new estimation method could in some cases rely on a minimally invasive catheter in various peripheral arteries, thereby avoiding the need for a riskier placement of a central artery catheter or a pulmonary artery catheter directly in the heart, the clinical gold standard for cardiovascular state estimation.
Another key finding was that when the animals received each of five drugs that doctors use to regulate either systemic vascular resistance or cardiac output, the proportional estimates tracked the resulting changes properly. The finding therefore suggests that the proportional estimates of each factor are accurately reflecting their physiological changes.
Toward the clinic
With these encouraging results, Baum and Brown said, the current method can be readily implemented in clinical settings to inform perioperative care teams about underlying causes of critical blood pressure changes. They are actively pursuing regulatory approval of use of this method in a clinical device.
Additionally, the researchers are pursuing more animal studies to validate an advanced blood pressure management approach that uses this method.
They have developed a closed-loop system, informed by this estimation framework, to precisely regulate blood pressure in an animal model. Upon completion of the animal studies, they will apply for regulatory clearance to test the system in humans.
In addition to Baum, Dahleh and Brown, the paper's other authors are Elie Adam, Christian Guay, Gabriel Schamberg, Mohammadreza Kazemi and Thomas Heldt.
The National Science Foundation, the National Institutes of Health, a Mathworks Fellowship, The Picower Institute for Learning and Memory and The JPB Foundation supported the study.
- Heart Disease
- Hypertension
- Today's Healthcare
- Blood Clots
- Personalized Medicine
- Stroke Prevention
- Wounds and Healing
- Laparoscopic surgery
- Robotic surgery
- Minimally invasive procedure
- Blood transfusion
- Blood pressure
- Mechanical ventilation
- Plastic surgery
Story Source:
Materials provided by Picower Institute at MIT . Note: Content may be edited for style and length.
Journal Reference :
- Taylor E. Baum, Elie Adam, Christian S. Guay, Gabriel Schamberg, Mohammadreza Kazemi, Thomas Heldt, Emery N. Brown. Dynamic Estimation of Cardiovascular State From Arterial Blood Pressure Recordings . IEEE Transactions on Biomedical Engineering , 2024; 1 DOI: 10.1109/TBME.2024.3408808
Cite This Page :
Explore More
- 'Dancing Molecules' Heal Cartilage Damage
- Robotics and How Flies Assess Winds
- Lampreys: 'Jaw-Dropping' Evolutionary Origin
- Making AI 1,000 Times More Energy Efficient
- Controlling Mosquitoes Through Genetic Breeding
- Hidden Elements from Ancient Alchemy Lab
- Drug Shows Promise in Clearing HIV from Brain
- Nitrogen Emissions Have a Net Cooling Effect
- New Zealand's Flightless Birds Retreating
- Ice Sheets Melt Fast, Regrow Slowly: Sponginess
Trending Topics
Strange & offbeat.

Local Weather
Get the daily forecast and severe weather alerts in your inbox!

- Copy article link
Cooler temperatures to come, but air quality expected to stay poor
Allison allsop.
- Jul 26, 2024
- Jul 26, 2024 Updated 5 hrs ago
Smoke fills the sky over Casper on Tuesday, July 23 due to fires in Canada, Oregon and Washington.
After blazing temperatures in the high 90s and low 100s this week, Casper residents and visitors will be thrilled to know that this weekend will only be in the high 80s.
In addition to the hot temperatures, residents were also plagued by a thick smoky haze this week from surrounding wildfires, which will continue into the weekend.
For the last week, the air quality index has been elevated between 60 and 125. When measuring air quality, measurements below 50 are considered “good” meaning it won’t have very much effect on anyone’s health. Measurements of 50-100 are considered moderate. At moderate air quality levels, individuals who are unusually sensitive to air quality.
On Tuesday, air quality in Casper reached measurements of 118 according to AirNow, a website that tracks air quality measurements and trends across the country.
People are also reading…
- World's biggest soda ash company gears up for operations in Wyoming
- Game and Fish stocks "Trojan" brook trout into Snake River tributary
- Casper's Alibi coming to an end
- Hageman calls Kamala Harris “DEI hire”
- Surprise blast of rock, water and steam in Yellowstone sends dozens running for safety
- Game and fish to remove brook trout from Bighorn Mountains stream
- Flags to be flown at half-staff at Capitol, in Goshen, Niobrara and Platte Counties
- Search begins for missing art professor in Laramie
- Buffalo lawyer disbarred for neglecting vaccine lawsuit, clients
- 63rd annual Crazy Days happening downtown this weekend
- Casper man accused of hallucinating gunfight, breaking windows while high on meth
- Casper city councilors felt 'blindsided' by airport board's decision to end Delta flight subsidy
- Trump campaign releases letter on his injury, treatment after assassination attempt
- Biden drops out of race, endorses VP Harris after disastrous debate inflamed age concerns
- 14-year-old accused of Denver murder arrested in Casper
Measurements of air quality between 101 and 150 are considered unhealthy for sensitive groups such as those with heart or lung disease, older adults, pregnant people and children.
Extended exposure to polluted air can be similar to smoking cigarettes, according to one article published by Berkeley Earth.
Eight hours of exposure in an area with an air quality reading of 120 is equal to smoking 2.3 cigarettes.
In order to reduce exposure, especially for sensitive groups, individuals should lessen the time they spend outdoors. They can also invest in a HEPA air cleaner to remove harmful pollutants from the air inside the home. Windows should also remain closed if possible to further reduce the possibility of smoke particles entering the home. Some air conditioning units may have a recirculation option that will help reduce the amount of outside air coming in.
Air quality measurements are expected to stay within the 50s to 60s this weekend.
Allison Allsop is the education and health reporter for the Casper Star-Tribune. She can be reached at 307-266-0544 or [email protected] . Her Twitter account is @allisonallsop
Education/Health Reporter
- Author twitter
- Author email
Get email notifications on {{subject}} daily!
{{description}}
Email notifications are only sent once a day, and only if there are new matching items.
Followed notifications
Please log in to use this feature, related to this story, most popular, fire danger "very high" in yellowstone national park.
Fire danger across Yellowstone is being upgraded and Stage 1 fire restrictions will kick as Wyoming’s dry season kicks into high gear, accordi…
Watch Now: Related Video
New york city turns to ai-powered scanners in push to keep guns out of the subway system, netanyahu discusses one of the hostages with trump at mar-a-lago, casper rc fly-in, sunken treasure: is champagne nestled in a 19th-century shipwreck still fit for a toast.
- Andrew Towne
- Notifications
Get up-to-the-minute news sent straight to your device.
News Alerts
Breaking news.
Thank you for visiting nature.com. You are using a browser version with limited support for CSS. To obtain the best experience, we recommend you use a more up to date browser (or turn off compatibility mode in Internet Explorer). In the meantime, to ensure continued support, we are displaying the site without styles and JavaScript.
- View all journals
- Explore content
- About the journal
- Publish with us
- Sign up for alerts
- Brief Communication
- Published: 09 January 2024
Delivery of a sebum modulator by an engineered skin microbe in mice
- Nastassia Knödlseder 1 ,
- María-José Fábrega 1 na1 ,
- Javier Santos-Moreno 1 na1 ,
- Joan Manils ORCID: orcid.org/0000-0001-8429-1295 2 , 3 ,
- Lorena Toloza 1 ,
- Maria Marín Vilar 1 nAff11 ,
- Cristina Fernández 1 ,
- Katrina Broadbent 4 ,
- Julien Maruotti 5 ,
- Hélène Lemenager 5 ,
- Carlo Carolis ORCID: orcid.org/0000-0003-4240-1139 4 ,
- Christos C. Zouboulis ORCID: orcid.org/0000-0003-1646-2608 6 ,
- Concepció Soler ORCID: orcid.org/0000-0001-6502-5012 2 , 7 ,
- Rolf Lood 8 ,
- Holger Brüggemann 9 &
- Marc Güell ORCID: orcid.org/0000-0003-4000-7912 1 , 10
Nature Biotechnology ( 2024 ) Cite this article
6178 Accesses
3 Citations
358 Altmetric
Metrics details
- Applied microbiology
- Genetic engineering
Microorganisms can be equipped with synthetic genetic programs for the production of targeted therapeutic molecules. Cutibacterium acnes is the most abundant commensal of the human skin, making it an attractive chassis to create skin-delivered therapeutics. Here, we report the engineering of this bacterium to produce and secrete the therapeutic molecule neutrophil gelatinase-associated lipocalin, in vivo, for the modulation of cutaneous sebum production.
This is a preview of subscription content, access via your institution
Access options
Access Nature and 54 other Nature Portfolio journals
Get Nature+, our best-value online-access subscription
$29.99 / 30 days
cancel any time
Subscribe to this journal
Receive 12 print issues and online access
$209.00 per year
only $17.42 per issue
Buy this article
- Purchase on Springer Link
- Instant access to full article PDF
Prices may be subject to local taxes which are calculated during checkout
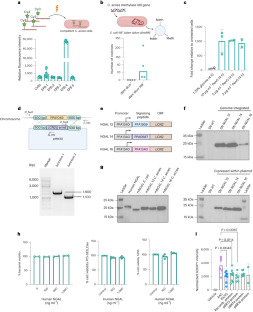
Similar content being viewed by others
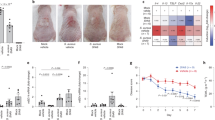
Development of a human skin commensal microbe for bacteriotherapy of atopic dermatitis and use in a phase 1 randomized clinical trial
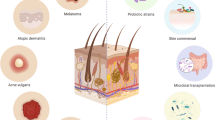
Recent advances in single-cell engineered live biotherapeutic products research for skin repair and disease treatment
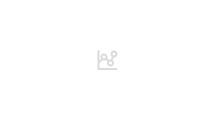
Early development of the skin microbiome: therapeutic opportunities
Data availability.
Sequencing datasets generated during this study are available from the NCBI Sequence Read Archive (SRA) with BioProject ID number PRJNA1007560 (ref. 63 ). The mass spectrometry proteomics data were deposited to the ProteomeXchange Consortium via the PRIDE 64 partner repository with the dataset identifier PXD044802 (ref. 65 ). Source data are provided with this paper.
Leventhal, D. S. et al. Immunotherapy with engineered bacteria by targeting the STING pathway for anti-tumor immunity. Nat. Commun. 11 , 2739 (2020).
Article CAS PubMed PubMed Central Google Scholar
Isabella, V. M. et al. Development of a synthetic live bacterial therapeutic for the human metabolic disease phenylketonuria. Nat. Biotechnol. 36 , 857–864 (2018).
Article CAS PubMed Google Scholar
Mao, N., Cubillos-Ruiz, A., Cameron, D. E. & Collins, J. J. Probiotic strains detect and suppress cholera in mice. Sci. Transl. Med. 10 , eaao2586 (2018).
Article PubMed PubMed Central Google Scholar
Danino, T. et al. Programmable probiotics for detection of cancer in urine. Sci. Transl. Med. 7 , 289ra84 (2015).
Hwang, I. Y. et al. Engineered probiotic Escherichia coli can eliminate and prevent Pseudomonas aeruginosa gut infection in animal models. Nat. Commun. 8 , 15028 (2017).
Kurtz, C. B. et al. An engineered Nissle improves hyperammonemia and survival in mice and shows dose-dependent exposure in healthy humans. Sci. Transl. Med. 11 , eaau7975 (2019).
Robert, S. et al. Oral delivery of glutamic acid decarboxylase (GAD)-65 and IL10 by Lactococcus lactis reverses diabetes in recent-onset NOD mice. Diabetes 63 , 2876–2887 (2014).
Ma, J. et al. Engineered probiotics. Microb. Cell Fact. 21 , 72 (2022).
Zhou, Z. et al. Engineering probiotics as living diagnostics and therapeutics for improving human health. Microb. Cell Fact. 19 , 56 (2020).
Chen, Y. E. et al. Engineered skin bacteria induce antitumor T cell responses against melanoma. Science 380 , 203–210 (2023).
Öhnstedt, E. et al. Engineered bacteria to accelerate wound healing: an adaptive, randomised, double-blind, placebo-controlled, first-in-human phase 1 trial. EClinicalMedicine 60 , 102014 (2023).
Maura, D., Elmekki, N. & Goddard, C. A. The ammonia oxidizing bacterium Nitrosomonas eutropha blocks T helper 2 cell polarization via the anti-inflammatory cytokine IL-10. Sci. Rep. 11 , 14162 (2021).
Lee, N. Y. et al. Dermal microflora restoration with ammonia-oxidizing bacteria Nitrosomonas eutropha in the treatment of keratosis pilaris: a randomized clinical trial. J. Drugs Dermatol. 17 , 285–288 (2018).
PubMed Google Scholar
Byrd, A. L., Belkaid, Y. & Segre, J. A. The human skin microbiome. Nat. Rev. Microbiol. 16 , 143–155 (2018).
Boxberger, M., Cenizo, V., Cassir, N. & La Scola, B. Challenges in exploring and manipulating the human skin microbiome. Microbiome 9 , 1–14 (2021).
Article Google Scholar
Naik, S. et al. Compartmentalized control of skin immunity by resident commensals. Science 337 , 1115–1119 (2012).
Grice, E. A. et al. Topographical and temporal diversity of the human skin microbiome. Science 324 , 1190 (2009).
Conwill, A. et al. Anatomy promotes neutral coexistence of strains in the human skin microbiome. Cell Host Microbe 30 , 171–182 (2022).
Zouboulis, C. C.Acne and sebaceous gland function. Clin. Dermatol . 22 , 360–366 (2004).
Gribbon, E. M., Cunliffe, W. J. & Holland, K. T. Interaction of Propionibacterium acnes with skin lipids in vitro. J. Gen. Microbiol. 139 , 1745–1751 (1993).
Fournière, M., Latire, T., Souak, D., Feuilloley, M. G. J. & Bedoux, G. Staphylococcus epidermidis and Cutibacterium acnes : two major sentinels of skin microbiota and the influence of cosmetics. Microorganisms 8 , 1752 (2020).
Paetzold, B. et al. Skin microbiome modulation induced by probiotic solutions. Microbiome 7 , 95 (2019).
Oh, J., Byrd, A. L., Park, M., Kong, H. H. & Segre, J. A.Temporal stability of the human skin microbiome. Cell 165 , 854–866 (2016).
Rosenthal, M., Goldberg, D., Aiello, A., Larson, E. & Foxman, B.Skin microbiota: microbial community structure and its potential association with health and disease. Infect. Genet. and Evol. 11 , 839–848 (2011).
Dréno, B. et al. Cutibacterium acnes ( Propionibacterium acnes ) and acne vulgaris: a brief look at the latest updates. J. Eur. Acad. Dermatol. Venereol. 32 , 5–14 (2018).
Article PubMed Google Scholar
Moradi Tuchayi, S. et al. Acne vulgaris. Nat. Rev. Dis. Primers 1 , 15029 (2015).
Layton, A. The use of isotretinoin in acne. Dermatoendocrinol. 1 , 162 (2009).
Nelson, A. M. et al. Temporal changes in gene expression in the skin of patients treated with isotretinoin provide insight into its mechanism of action. Dermatoendocrinol. 1 , 177 (2009).
Nelson, A. M. et al. Neutrophil gelatinase–associated lipocalin mediates 13- cis retinoic acid–induced apoptosis of human sebaceous gland cells. J. Clin. Invest. 118 , 1468 (2008).
Lumsden, K. R. et al. Isotretinoin increases skin-surface levels of neutrophil gelatinase-associated lipocalin in patients treated for severe acne. Br. J. Dermatol. 165 , 302–310 (2011).
Novickii, V. et al. Different permeabilization patterns of splenocytes and thymocytes to combination of pulsed electric and magnetic field treatments. Bioelectrochemistry 122 , 183–190 (2018).
Knödlseder, N. et al. Engineering selectivity of Cutibacterium acnes phages by epigenetic imprinting. PLoS Pathog. 18 , e1010420 (2022).
Deptula, P. et al. Complete genome sequences and methylome analyses of Cutibacterium acnes subsp. acnes s trains DSM 16379 and DSM 1897T. Microbiol. Resour. Announc. 9 , e00705–e00720 (2020).
Johnson, B. H. & Hecht, M. H.Recombinant proteins can be isolated from E. coli cells by repeated cycles of freezing and thawing. Nat. Biotechnol. 12 , 1357–1360 (1994).
Article CAS Google Scholar
Sharma, S. et al. A simple and cost-effective freeze-thaw based method for Plasmodium DNA extraction from dried blood spot. Iran. J. Parasitol. 14 , 29 (2019).
PubMed PubMed Central Google Scholar
Harju, S., Fedosyuk, H. & Peterson, K. R. Rapid isolation of yeast genomic DNA: Bust n’ Grab. BMC Biotechnol. 4 , 8 (2004).
Park, S. F. & Stewart, G. S. High-efficiency transformation of Listeria monocytogenes by electroporation of penicillin-treated cells. Gene 94 , 129–132 (1990).
Pyne, M. E., Moo-Young, M., Chung, D. A. & Chou, C. P. Development of an electrotransformation protocol for genetic manipulation of Clostridium pasteurianum . Biotechnol. Biofuels 6 , 50 (2013).
Sörensen, M. et al. Mutagenesis of Propionibacterium acnes and analysis of two CAMP factor knock-out mutants. J. Microbiol. Methods 83 , 211–216 (2010).
Nazipi, S., Stødkilde, K., Scavenius, C. & Brüggemann, H.The skin bacterium Propionibacterium acnes employs two variants of hyaluronate lyase with distinct properties. Microorganisms 5 , 57 (2017).
Allhorn, M., Arve, S., Brüggemann, H. & Lood, R. A. A novel enzyme with antioxidant capacity produced by the ubiquitous skin colonizer Propionibacterium acnes. Sci. Rep. https://doi.org/10.1038/srep36412 (2016).
Pedrolli, D. B. et al. Engineering microbial living therapeutics: the synthetic biology toolbox. Trends Biotechnol. 37 , 100–115 (2019).
Amalaradjou, M. A. R. & Bhunia, A. K. Bioengineered probiotics, a strategic approach to control enteric infections. Bioengineered 4 , 379–387 (2013).
Charbonneau, M. R., Isabella, V. M., Li, N. & Kurtz, C. B. Developing a new class of engineered live bacterial therapeutics to treat human diseases. Nat. Commun. 11 , 1738 (2020).
Norville, J.E. et al. Assembly of radically recoded E. coli genome segments. Preprint at bioRxiv https://doi.org/10.1101/070417 (2016).
Holland, C. et al. Proteomic identification of secreted proteins of Propionibacterium acnes . BMC Microbiol. 10 , 230 (2010).
Yu, Y., Champer, J. & Kim, J.Analysis of the surface, secreted, and intracellular proteome of Propionibacterium acnes. EuPA Open Proteom. 9 , 1–7 (2015).
Lumsden, K. R. The Innate Immune Protein Neutrophil Gelatinase-Associated Lipocalin is Involved in the Early Therapeutic Response to 13-cis Retinoic Acid in Acne Patients . Dissertation, Pennsylvania State University (2009).
Wagner, E. F., Schonthaler, H. B., Guinea-Viniegra, J. & Tschachler, E. Psoriasis: what we have learned from mouse models. Nat. Rev. Rheumatol. 6 , 704–714 (2010).
Zomer, H. D. & Trentin, A. G. Skin wound healing in humans and mice: challenges in translational research. J. Dermatol. Sci. 90 , 3–12 (2018).
Niehues, H. et al. 3D skin models for 3R research: the potential of 3D reconstructed skin models to study skin barrier function. Exp. Dermatol. 27 , 501–511 (2018).
Emmert, H., Rademacher, F., Gläser, R. & Harder, J. Skin microbiota analysis in human 3D skin models—‘Free your mice’. Exp. Dermatol. 29 , 1133–1139 (2020).
Jore, J. P., van Luijk, N., Luiten, R. G., van der Werf, M. J. & Pouwels, P. H. Efficient transformation system for Propionibacterium freudenreichii based on a novel vector. Appl. Environ. Microbiol. 67 , 499–503 (2001).
Lood, R. Propionibacterium acnes and its phages . Ph.D. thesis, Lund University (2011).
Kay, M. A., He, C.-Y. & Chen, Z.-Y. A robust system for production of minicircle DNA vectors. Nat. Biotechnol. 28 , 1287–1289 (2010).
Johnston, C. D. et al. Systematic evasion of the restriction-modification barrier in bacteria. Proc. Natl Acad. Sci. USA 116 , 11454–11459 (2019).
Datsenko, K. A. & Wanner, B. L. One-step inactivation of chromosomal genes in Escherichia coli K-12 using PCR products. Proc. Natl Acad. Sci. USA 97 , 6640–6645 (2000).
Koontz, L. TCA precipitation. Methods Enzymol. 541 , 3–10 (2014).
Zouboulis, C. C., Seltmann, H., Neitzel, H. & Orfanos, C. E. Establishment and characterization of an immortalized human sebaceous gland cell line (SZ95). J. Invest. Dermatol. 113 , 1011–1020 (1999).
Bolyen, E. et al. Reproducible, interactive, scalable and extensible microbiome data science using QIIME 2. Nat. Biotechnol. 37 , 852–857 (2019).
Callahan, B. J. et al. DADA2: high-resolution sample inference from Illumina amplicon data. Nat. Methods 13 , 581–583 (2016).
Pruesse, E. et al. SILVA: a comprehensive online resource for quality checked and aligned ribosomal RNA sequence data compatible with ARB. Nucleic Acids Res. 35 , 7188–7196 (2007).
Knödlseder, N. et al. Mouse skin microbiome samples before and after C. acnes application. NCBI Bioproject, PRJNA1007560. Metagenomics. https://www.ncbi.nlm.nih.gov/bioproject/?term=PRJNA1007560 (2023).
Perez-Riverol, Y. et al. The PRIDE database resources in 2022: a hub for mass spectrometry-based proteomics evidences. Nucleic Acids Res. 50 , D543–D552 (2022).
Knödlseder, N. et al. Engineered skin microbiome-assisted delivery to the pilosebaceous unit. ProteomeXchange, PXD044802. Proteomics. https://www.ebi.ac.uk/pride/archive/projects/PXD044802 (2023).
Download references
Acknowledgements
We want to thank C.D. Johnston from the Fred Hutchinson Cancer Center for kindly providing the JMC2 and JMC3 MC producer strains. We would like to thank T.F. Meyer for the opportunity to visit his lab and M. Sörensen for her technical support and time during the research stay. Furthermore, we want to thank B. Paetzold for the interesting discussions. We want to express our gratitude to E. Sabido from the UPF/CRG proteomics unit for proteomic analysis support. We thank the entire Synbio team for their support and helpful discussions. N.K. thanks A. Rahmeh, M. Pallares and M. Pol for useful discussions and input.
This work was funded by the Office of Naval Research (award N62909-18-1-2155), INNOValora (INNOV21-09-1; SynFlora) given by Universitat Pompeu Fabra and Indústria del Coneixement of the Catalan government (AGAUR; IdC 2019 PROD 00057) and SKINDEV ‘Skin microbial devices’ by European Innovation Council (101098826), all granted to M.G., as well as by research grants PID2020-114477RB-I00 to C.S. and PID2021-126249OA-I00 to J.M. from the MCIN/AEI/10.13039/501100011033. N.K. is funded by a Maria Maetzu–UPF fellowship (AEI–MM-CEX2018-000792-M D.COMAS–Programa estatal de fomento investigación científica y técnica de exceléncia, unidades de exceléncia María de Maeztu 2018) and by the Sociedad Española de Químicos Cosméticos (Beca de la SEQC para la presentación de trabajos en el 33rd IFSCC Congress; KNÖDLSEDER, NASTASSIA). M.J.F. is funded by a Juan de la Cierva Fellowship from the Spanish government (award FJC 2018-037096-I). N.K. also received an EMBO short-term fellowship (fellowship no. 8240). J.S.M. is funded by a Marie Skłodowska-Curie Individual Fellowship (European Union’s Horizon 2020 Research and Innovation Program under Marie Skłodowska-Curie grant agreement 882387), a Juan de la Cierva Incorporación Fellowship (IJC2020-043621-I/MCIN/AEI/10.13039/501100011033 and NextGenerationEU/PRTR from the European Union) and a DCEXS MM Postdoc Project (Unidad de Excelencia María de Maeztu, funded by the AEI, ref. CEX2018-000792-M).
The graphic illustrations in this study were generated using BioRender.com . Data plots were generated using GraphPad Prism 9.
Author information
Maria Marín Vilar
Present address: Vall d’Hebron Institute of Oncology, Barcelona, Spain
These authors contributed equally: María José Fábrega, Javier Santos-Moreno.
Authors and Affiliations
Department of Medicine and Life Sciences, Universitat Pompeu Fabra, Barcelona, Spain
Nastassia Knödlseder, María-José Fábrega, Javier Santos-Moreno, Lorena Toloza, Maria Marín Vilar, Cristina Fernández & Marc Güell
Immunity, Inflammation and Cancer Group, Oncobell Program, Institut d’Investigació Biomèdica de Bellvitge, Barcelona, Spain
Joan Manils & Concepció Soler
Serra Húnter Programme, Immunology Unit, Department of Pathology and Experimental Therapy, School of Medicine, Universitat de Barcelona, Barcelona, Spain
Joan Manils
Protein Technologies Facility, Center of Genomic Regulation, Barcelona, Spain
Katrina Broadbent & Carlo Carolis
Phenocell SAS, Grasse, France
Julien Maruotti & Hélène Lemenager
Hochschulklinik für Dermatologie, Venerologie und Allergologie, Immunologisches Zentrum; Städtisches Klinikum Dessau; and Medizinische Hochschule Brandenburg Theodor Fontane und Fakultät für Gesundheitswissenschaften Brandenburg, Dessau-Roßlau, Germany
Christos C. Zouboulis
Immunology Unit, Department of Pathology and Experimental Therapy, School of Medicine, Universitat de Barcelona, Barcelona, Spain
Concepció Soler
Department of Clinical Sciences, Division of Infection Medicine, Lund University, Lund, Sweden
Department of Biomedicine, Aarhus University, Aarhus, Denmark
Holger Brüggemann
ICREA, Institució Catalana de Recerca i Estudis Avançats, Barcelona, Spain
You can also search for this author in PubMed Google Scholar
Contributions
N.K. conceptualized, designed and carried out the experiments with the guidance of M.G. M.J.F., L.T. and K.B. contributed to the cell culture work. M.M., L.T. and J.S.M. contributed to the bacterial work. J.S.M. with the help of N.K. designed and created the IIIB methylase-proficient E. coli strain. N.K., J. Manils and C.F. performed the mouse experiments and processed the samples. C.C.Z. provided the SZ95 cell line and guidance. J. Maruotti and H.L. provided the Pci-SEB_Cau cell lines, conducted the initial experiments and provided guidance. H.B. analyzed the 16S datasets and contributed with experimental design and advice. R.L. provided support and guidance for the in vivo production of proteins and acquisition of samples. C.C. and K.B. purified the proteins used in this study. C.S. contributed with advice and guidance. N.K., M.G., M.J.F., J.S.M. and J. Manils drafted the initial manuscript. All authors read, edited, and approved the final manuscript.
Corresponding author
Correspondence to Marc Güell .
Ethics declarations
Competing interests.
M.G., N.K., M.J.F. and J.S.M. are inventors of a European patent application submitted by University Pompeu Fabra.
Peer review
Peer review information.
Nature Biotechnology thanks Peter Larson and the other, anonymous, reviewer(s) for their contribution to the peer review of this work.
Additional information
Publisher’s note Springer Nature remains neutral with regard to jurisdictional claims in published maps and institutional affiliations.
Supplementary information
Supplementary information.
Supplementary Fig. 1–4, Table 1 and unmodified blots
Reporting Summary
Supplementary data.
Source data for supplementary figures.
Source data
Source data figs. 1 and 2.
Statistical source data for main figures.
Source Data Fig. 1f
Unprocessed western blot.
Source Data Fig. 1g
Source data fig. 2d.
Unprocessed microscope images.
Rights and permissions
Springer Nature or its licensor (e.g. a society or other partner) holds exclusive rights to this article under a publishing agreement with the author(s) or other rightsholder(s); author self-archiving of the accepted manuscript version of this article is solely governed by the terms of such publishing agreement and applicable law.
Reprints and permissions
About this article
Cite this article.
Knödlseder, N., Fábrega, MJ., Santos-Moreno, J. et al. Delivery of a sebum modulator by an engineered skin microbe in mice. Nat Biotechnol (2024). https://doi.org/10.1038/s41587-023-02072-4
Download citation
Received : 06 March 2023
Accepted : 17 November 2023
Published : 09 January 2024
DOI : https://doi.org/10.1038/s41587-023-02072-4
Share this article
Anyone you share the following link with will be able to read this content:
Sorry, a shareable link is not currently available for this article.
Provided by the Springer Nature SharedIt content-sharing initiative
This article is cited by
Cutibacterium improves skin condition.
- Agustina Taglialegna
Nature Reviews Microbiology (2024)
Quick links
- Explore articles by subject
- Guide to authors
- Editorial policies
Sign up for the Nature Briefing: Microbiology newsletter — what matters in microbiology research, free to your inbox weekly.

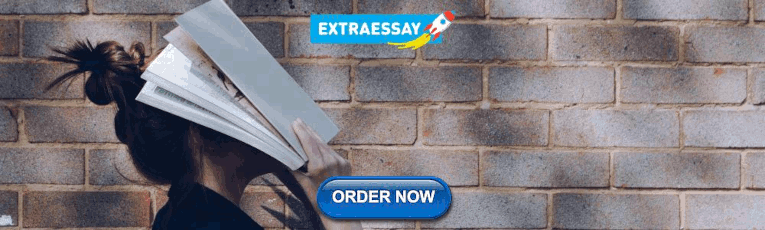
IMAGES
VIDEO
COMMENTS
Blood cancer, also referred to as haematological malignancy, is a collection of cancers that affect the blood, bone marrow, and lymphatic systems. Early and accurate blood cancer detection is essential for effective treatment and enhanced patient outcomes. Deep learning algorithms have emerged as potent instruments for medical image analysis and disease detection in recent years. This paper ...
INTRODUCTION Blood cancer represents a large group of different malignancies. This group includes cancers of the bone marrow, blood, and lymphatic system, which includes lymph nodes, lymphatic ...
This editorial integrates the views of Blood Cancer Discovery 's editors-in-chief and scientific editors to explore the current and near-future landscape of the study of hematologic malignancies—from the most intriguing new developments in clinical and basic research to the greatest upcoming challenges and how they will be confronted. This is ...
Find pioneering research in Blood Cancer Journal, an open access journal with special collections, 12.8 Impact Factor and 3 days to first decision.
Since the second half of the 20th century, our knowledge about the biology of cancer has made extraordinary progress. Today, we understand cancer at the genomic and epigenomic levels, and we have identified the cell that starts neoplastic transformation ...
Diagnosis of blood cancer using leukemia microarray gene data and machine learning approach has become an important medical research today.
Read the latest Research articles from Blood Cancer Journal
In this review, we explore at the most recent advances in nanomedicine in the treatment of blood cancer, from preclinical research to clinical trials. Additionally, this review paper highlights and explores the benefits of nanoplatforms that strongly influences, targeted therapies.
Papers focusing on lymphoma, leukaemia or myeloma patients with chemotherapy, radiotherapy or blood transfusion in periods of remission or relapse were included. However, retrospective studies with other forms of cancer and reviews of the literature were excluded.
Digital image processing (DIP) is commonly used in cancer detection, such as classifying brain tumors, detecting lung cancer cells (Al-Tarawneh, 2012 ), and detecting breast cancer in mammographic images (CharanKhan and Khurshid, 2018 ). Some research has also focused on enhancing blood microscopic images and detecting leukemia automatically.
Theses/Dissertations from 2022. Omics Analysis in Cancer and Development, Emalie J. Clement. Investigating the Role of Splenic Macrophages in Pancreatic Cancer, Daisy V. Gonzalez. Polymeric Chloroquine in Metastatic Pancreatic Cancer Therapy, Rubayat Islam Khan.
Leukemia (blood cancer) begins in the bone marrow and causes the formation of a large number of abnormal cells. The most common types of leukemia known are Acute lymphoblastic leukemia (ALL), Acute myeloid leukemia (AML), Chronic lymphocytic leukemia (CLL) and Chronic myeloid leukemia (CML). This thesis makes an effort to devise a methodology for the detection of Leukemia using image ...
The concepts of hematopoiesis and the generation of blood and immune cells from hematopoietic stem cells are some steady concepts in the field of hematology. However, the knowledge of hematopoietic cells arising from solid tumor cancer stem cells is novel. In the solid tumor microenvironment, hematopoietic cells play pivotal roles in tumor growth and progression. Recent studies have reported ...
Blood now publishes 1 to 2 Key Point summaries of research papers - specifically, Regular Articles, Brief Reports. The purpose of these short, bullet-pointed statements is to identify the most relevant outcomes of the paper and to provide a synopsis encapsulating the significance of the research and its implications for readers.
The primary focus of this thesis is on cancer cells of different invasive potential, and char-acterization of inherent properties that differ between non-invasive and invasive strains of similar origin. To understand our motivation we will outline some of the main aspects of cancer cells, especially in respect to their survival and spreading.
However, it was just my luck that the blood tests showed my blood matched perfectly with Michael's. My mother said, "Do it for your brother," and those words stuck out to me like black against white.
Hematologic malignancies are among the most common cancers, and understanding their incidence and death is crucial for targeting prevention, clinical practice improvement, and research resources ...
Pediatric Blood and Cancer recognizes the many benefits of archiving research data. Pediatric Blood and Cancer expects you to archive all the data from which your published results are derived in a public repository.
Stem cell transplant is also the best therapy for cancer but it given after the common therapies to recover the patient from blood loss and help in making the patient healthy.
When caused by cancer, blood can appear in the stool itself or on toilet paper, and the color can vary from brighter red to black. Experts suggest consulting a healthcare provider if you continue ...
Molecular diagnostic tests help clinicians understand the underlying biological mechanisms of their patients' breast cancer (BC) and facilitate clinical management. Several tissue-based mRNA tests are used routinely in clinical practice, particularly for assessing the BC recurrence risk, which can guide treatment decisions. However, blood-based mRNA assays have only recently started to ...
Additionally, scientists have been pushed to employ targeted approaches for treating cancer due to the problems in CSCs resistance to therapeutic methods. Molecular docking is one of the targeted approaches that play an important role in drug discovery and pharmaceutical researches.
Amyloid peptides (AMYs) and antimicrobial peptides (AMPs) are considered as the two distinct families of peptides, characterized by their unique sequences, structures, biological functions, and specific pathological targets.
The Stockholm3 blood test is equally effective at detecting prostate cancer in different ethnic groups, a new paper reports. The test produces significantly better results than the current PSA ...
The most important reason for the high cost of prescription drugs is the existence of monopoly 4, 5. For many new drugs, there are no other alternatives. In the case of cancer, even when there are ...
A Thesis Submitted to the W.A. Franke Honors College In Partial Fulfillment of the bachelor's degree ... addiction. Finally, Part II of this paper will explore the University of Arizona's current medical ... long-term use of opioids for chronic non-cancer pain, the relative potency of commonly used ...
A new mathematical method, validated with experimental animal data, provides a fast, reliable and minimally invasive way of determining how to treat critical blood pressure changes during surgery ...
Air temperatures are dropping slightly this weekend, but the air quality will remain poor. Poor air quality can have negative effects on certain populations.
An engineered skin microbe produces a therapeutic molecule that reduces sebum in mice.