Have a language expert improve your writing
Run a free plagiarism check in 10 minutes, generate accurate citations for free.
- Knowledge Base
Methodology
- How to Write a Literature Review | Guide, Examples, & Templates
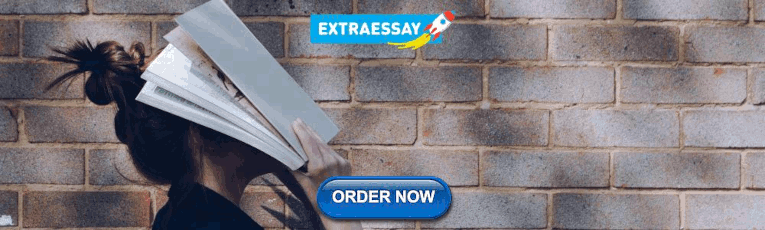
How to Write a Literature Review | Guide, Examples, & Templates
Published on January 2, 2023 by Shona McCombes . Revised on September 11, 2023.
What is a literature review? A literature review is a survey of scholarly sources on a specific topic. It provides an overview of current knowledge, allowing you to identify relevant theories, methods, and gaps in the existing research that you can later apply to your paper, thesis, or dissertation topic .
There are five key steps to writing a literature review:
- Search for relevant literature
- Evaluate sources
- Identify themes, debates, and gaps
- Outline the structure
- Write your literature review
A good literature review doesn’t just summarize sources—it analyzes, synthesizes , and critically evaluates to give a clear picture of the state of knowledge on the subject.
Instantly correct all language mistakes in your text
Upload your document to correct all your mistakes in minutes
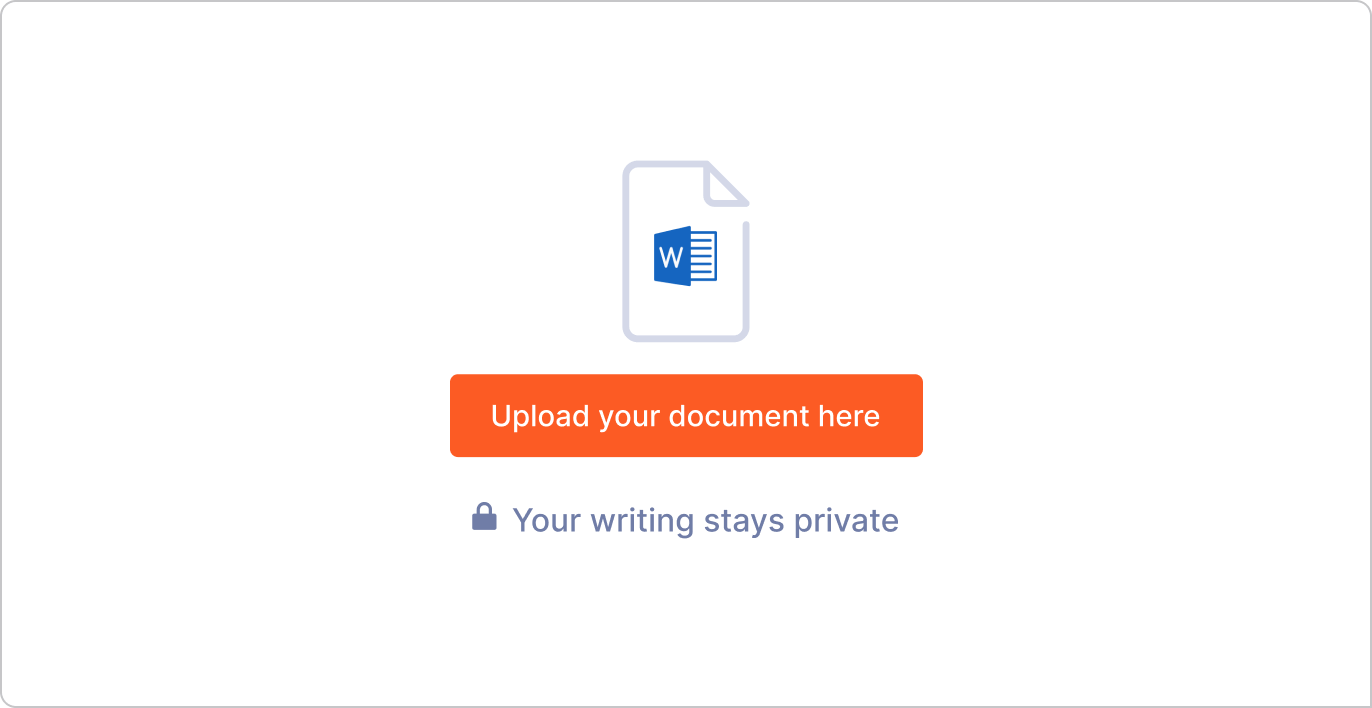
Table of contents
What is the purpose of a literature review, examples of literature reviews, step 1 – search for relevant literature, step 2 – evaluate and select sources, step 3 – identify themes, debates, and gaps, step 4 – outline your literature review’s structure, step 5 – write your literature review, free lecture slides, other interesting articles, frequently asked questions, introduction.
- Quick Run-through
- Step 1 & 2
When you write a thesis , dissertation , or research paper , you will likely have to conduct a literature review to situate your research within existing knowledge. The literature review gives you a chance to:
- Demonstrate your familiarity with the topic and its scholarly context
- Develop a theoretical framework and methodology for your research
- Position your work in relation to other researchers and theorists
- Show how your research addresses a gap or contributes to a debate
- Evaluate the current state of research and demonstrate your knowledge of the scholarly debates around your topic.
Writing literature reviews is a particularly important skill if you want to apply for graduate school or pursue a career in research. We’ve written a step-by-step guide that you can follow below.
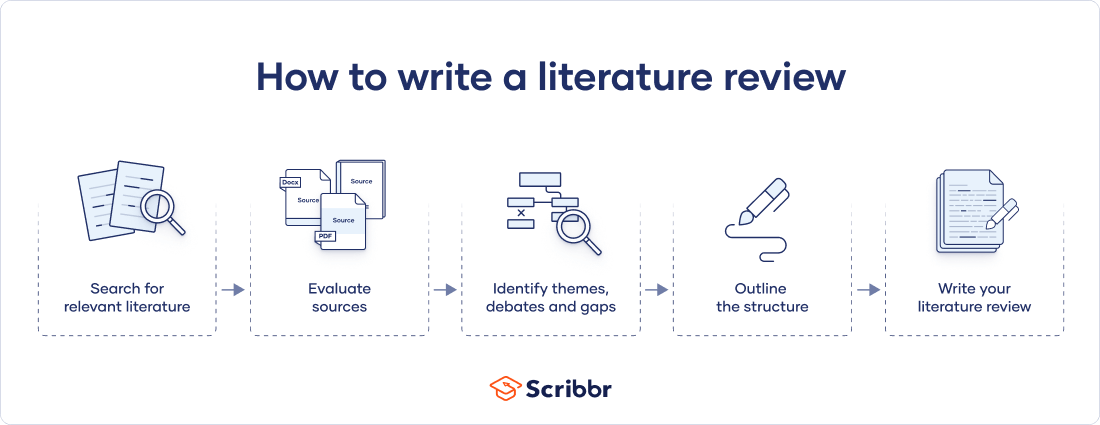
Here's why students love Scribbr's proofreading services
Discover proofreading & editing
Writing literature reviews can be quite challenging! A good starting point could be to look at some examples, depending on what kind of literature review you’d like to write.
- Example literature review #1: “Why Do People Migrate? A Review of the Theoretical Literature” ( Theoretical literature review about the development of economic migration theory from the 1950s to today.)
- Example literature review #2: “Literature review as a research methodology: An overview and guidelines” ( Methodological literature review about interdisciplinary knowledge acquisition and production.)
- Example literature review #3: “The Use of Technology in English Language Learning: A Literature Review” ( Thematic literature review about the effects of technology on language acquisition.)
- Example literature review #4: “Learners’ Listening Comprehension Difficulties in English Language Learning: A Literature Review” ( Chronological literature review about how the concept of listening skills has changed over time.)
You can also check out our templates with literature review examples and sample outlines at the links below.
Download Word doc Download Google doc
Before you begin searching for literature, you need a clearly defined topic .
If you are writing the literature review section of a dissertation or research paper, you will search for literature related to your research problem and questions .
Make a list of keywords
Start by creating a list of keywords related to your research question. Include each of the key concepts or variables you’re interested in, and list any synonyms and related terms. You can add to this list as you discover new keywords in the process of your literature search.
- Social media, Facebook, Instagram, Twitter, Snapchat, TikTok
- Body image, self-perception, self-esteem, mental health
- Generation Z, teenagers, adolescents, youth
Search for relevant sources
Use your keywords to begin searching for sources. Some useful databases to search for journals and articles include:
- Your university’s library catalogue
- Google Scholar
- Project Muse (humanities and social sciences)
- Medline (life sciences and biomedicine)
- EconLit (economics)
- Inspec (physics, engineering and computer science)
You can also use boolean operators to help narrow down your search.
Make sure to read the abstract to find out whether an article is relevant to your question. When you find a useful book or article, you can check the bibliography to find other relevant sources.
You likely won’t be able to read absolutely everything that has been written on your topic, so it will be necessary to evaluate which sources are most relevant to your research question.
For each publication, ask yourself:
- What question or problem is the author addressing?
- What are the key concepts and how are they defined?
- What are the key theories, models, and methods?
- Does the research use established frameworks or take an innovative approach?
- What are the results and conclusions of the study?
- How does the publication relate to other literature in the field? Does it confirm, add to, or challenge established knowledge?
- What are the strengths and weaknesses of the research?
Make sure the sources you use are credible , and make sure you read any landmark studies and major theories in your field of research.
You can use our template to summarize and evaluate sources you’re thinking about using. Click on either button below to download.
Take notes and cite your sources
As you read, you should also begin the writing process. Take notes that you can later incorporate into the text of your literature review.
It is important to keep track of your sources with citations to avoid plagiarism . It can be helpful to make an annotated bibliography , where you compile full citation information and write a paragraph of summary and analysis for each source. This helps you remember what you read and saves time later in the process.
The only proofreading tool specialized in correcting academic writing - try for free!
The academic proofreading tool has been trained on 1000s of academic texts and by native English editors. Making it the most accurate and reliable proofreading tool for students.
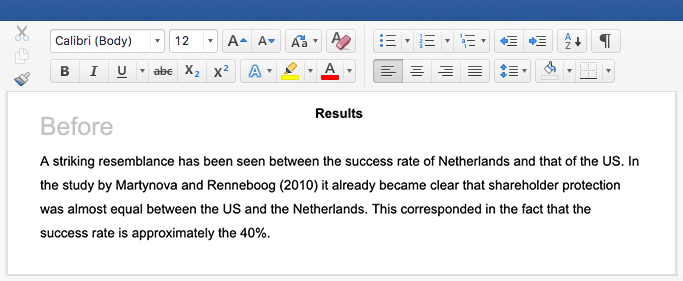
Try for free
To begin organizing your literature review’s argument and structure, be sure you understand the connections and relationships between the sources you’ve read. Based on your reading and notes, you can look for:
- Trends and patterns (in theory, method or results): do certain approaches become more or less popular over time?
- Themes: what questions or concepts recur across the literature?
- Debates, conflicts and contradictions: where do sources disagree?
- Pivotal publications: are there any influential theories or studies that changed the direction of the field?
- Gaps: what is missing from the literature? Are there weaknesses that need to be addressed?
This step will help you work out the structure of your literature review and (if applicable) show how your own research will contribute to existing knowledge.
- Most research has focused on young women.
- There is an increasing interest in the visual aspects of social media.
- But there is still a lack of robust research on highly visual platforms like Instagram and Snapchat—this is a gap that you could address in your own research.
There are various approaches to organizing the body of a literature review. Depending on the length of your literature review, you can combine several of these strategies (for example, your overall structure might be thematic, but each theme is discussed chronologically).
Chronological
The simplest approach is to trace the development of the topic over time. However, if you choose this strategy, be careful to avoid simply listing and summarizing sources in order.
Try to analyze patterns, turning points and key debates that have shaped the direction of the field. Give your interpretation of how and why certain developments occurred.
If you have found some recurring central themes, you can organize your literature review into subsections that address different aspects of the topic.
For example, if you are reviewing literature about inequalities in migrant health outcomes, key themes might include healthcare policy, language barriers, cultural attitudes, legal status, and economic access.
Methodological
If you draw your sources from different disciplines or fields that use a variety of research methods , you might want to compare the results and conclusions that emerge from different approaches. For example:
- Look at what results have emerged in qualitative versus quantitative research
- Discuss how the topic has been approached by empirical versus theoretical scholarship
- Divide the literature into sociological, historical, and cultural sources
Theoretical
A literature review is often the foundation for a theoretical framework . You can use it to discuss various theories, models, and definitions of key concepts.
You might argue for the relevance of a specific theoretical approach, or combine various theoretical concepts to create a framework for your research.
Like any other academic text , your literature review should have an introduction , a main body, and a conclusion . What you include in each depends on the objective of your literature review.
The introduction should clearly establish the focus and purpose of the literature review.
Depending on the length of your literature review, you might want to divide the body into subsections. You can use a subheading for each theme, time period, or methodological approach.
As you write, you can follow these tips:
- Summarize and synthesize: give an overview of the main points of each source and combine them into a coherent whole
- Analyze and interpret: don’t just paraphrase other researchers — add your own interpretations where possible, discussing the significance of findings in relation to the literature as a whole
- Critically evaluate: mention the strengths and weaknesses of your sources
- Write in well-structured paragraphs: use transition words and topic sentences to draw connections, comparisons and contrasts
In the conclusion, you should summarize the key findings you have taken from the literature and emphasize their significance.
When you’ve finished writing and revising your literature review, don’t forget to proofread thoroughly before submitting. Not a language expert? Check out Scribbr’s professional proofreading services !
This article has been adapted into lecture slides that you can use to teach your students about writing a literature review.
Scribbr slides are free to use, customize, and distribute for educational purposes.
Open Google Slides Download PowerPoint
If you want to know more about the research process , methodology , research bias , or statistics , make sure to check out some of our other articles with explanations and examples.
- Sampling methods
- Simple random sampling
- Stratified sampling
- Cluster sampling
- Likert scales
- Reproducibility
Statistics
- Null hypothesis
- Statistical power
- Probability distribution
- Effect size
- Poisson distribution
Research bias
- Optimism bias
- Cognitive bias
- Implicit bias
- Hawthorne effect
- Anchoring bias
- Explicit bias
A literature review is a survey of scholarly sources (such as books, journal articles, and theses) related to a specific topic or research question .
It is often written as part of a thesis, dissertation , or research paper , in order to situate your work in relation to existing knowledge.
There are several reasons to conduct a literature review at the beginning of a research project:
- To familiarize yourself with the current state of knowledge on your topic
- To ensure that you’re not just repeating what others have already done
- To identify gaps in knowledge and unresolved problems that your research can address
- To develop your theoretical framework and methodology
- To provide an overview of the key findings and debates on the topic
Writing the literature review shows your reader how your work relates to existing research and what new insights it will contribute.
The literature review usually comes near the beginning of your thesis or dissertation . After the introduction , it grounds your research in a scholarly field and leads directly to your theoretical framework or methodology .
A literature review is a survey of credible sources on a topic, often used in dissertations , theses, and research papers . Literature reviews give an overview of knowledge on a subject, helping you identify relevant theories and methods, as well as gaps in existing research. Literature reviews are set up similarly to other academic texts , with an introduction , a main body, and a conclusion .
An annotated bibliography is a list of source references that has a short description (called an annotation ) for each of the sources. It is often assigned as part of the research process for a paper .
Cite this Scribbr article
If you want to cite this source, you can copy and paste the citation or click the “Cite this Scribbr article” button to automatically add the citation to our free Citation Generator.
McCombes, S. (2023, September 11). How to Write a Literature Review | Guide, Examples, & Templates. Scribbr. Retrieved April 9, 2024, from https://www.scribbr.com/dissertation/literature-review/
Is this article helpful?
Shona McCombes
Other students also liked, what is a theoretical framework | guide to organizing, what is a research methodology | steps & tips, how to write a research proposal | examples & templates, unlimited academic ai-proofreading.
✔ Document error-free in 5minutes ✔ Unlimited document corrections ✔ Specialized in correcting academic texts
Get science-backed answers as you write with Paperpal's Research feature
What is a Literature Review? How to Write It (with Examples)
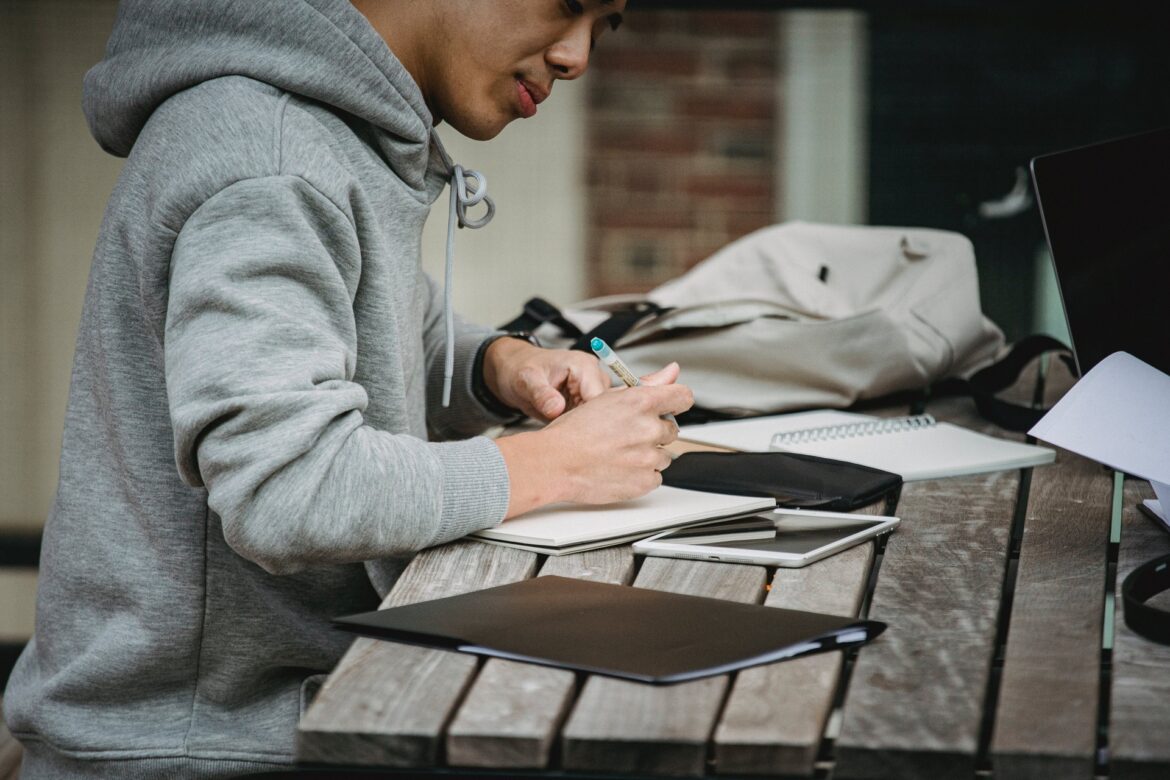
A literature review is a critical analysis and synthesis of existing research on a particular topic. It provides an overview of the current state of knowledge, identifies gaps, and highlights key findings in the literature. 1 The purpose of a literature review is to situate your own research within the context of existing scholarship, demonstrating your understanding of the topic and showing how your work contributes to the ongoing conversation in the field. Learning how to write a literature review is a critical tool for successful research. Your ability to summarize and synthesize prior research pertaining to a certain topic demonstrates your grasp on the topic of study, and assists in the learning process.
Table of Contents
- What is the purpose of literature review?
- a. Habitat Loss and Species Extinction:
- b. Range Shifts and Phenological Changes:
- c. Ocean Acidification and Coral Reefs:
- d. Adaptive Strategies and Conservation Efforts:
- How to write a good literature review
- Choose a Topic and Define the Research Question:
- Decide on the Scope of Your Review:
- Select Databases for Searches:
- Conduct Searches and Keep Track:
- Review the Literature:
- Organize and Write Your Literature Review:
- Frequently asked questions
What is a literature review?
A well-conducted literature review demonstrates the researcher’s familiarity with the existing literature, establishes the context for their own research, and contributes to scholarly conversations on the topic. One of the purposes of a literature review is also to help researchers avoid duplicating previous work and ensure that their research is informed by and builds upon the existing body of knowledge.
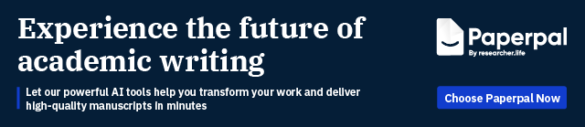
What is the purpose of literature review?
A literature review serves several important purposes within academic and research contexts. Here are some key objectives and functions of a literature review: 2
- Contextualizing the Research Problem: The literature review provides a background and context for the research problem under investigation. It helps to situate the study within the existing body of knowledge.
- Identifying Gaps in Knowledge: By identifying gaps, contradictions, or areas requiring further research, the researcher can shape the research question and justify the significance of the study. This is crucial for ensuring that the new research contributes something novel to the field.
- Understanding Theoretical and Conceptual Frameworks: Literature reviews help researchers gain an understanding of the theoretical and conceptual frameworks used in previous studies. This aids in the development of a theoretical framework for the current research.
- Providing Methodological Insights: Another purpose of literature reviews is that it allows researchers to learn about the methodologies employed in previous studies. This can help in choosing appropriate research methods for the current study and avoiding pitfalls that others may have encountered.
- Establishing Credibility: A well-conducted literature review demonstrates the researcher’s familiarity with existing scholarship, establishing their credibility and expertise in the field. It also helps in building a solid foundation for the new research.
- Informing Hypotheses or Research Questions: The literature review guides the formulation of hypotheses or research questions by highlighting relevant findings and areas of uncertainty in existing literature.
Literature review example
Let’s delve deeper with a literature review example: Let’s say your literature review is about the impact of climate change on biodiversity. You might format your literature review into sections such as the effects of climate change on habitat loss and species extinction, phenological changes, and marine biodiversity. Each section would then summarize and analyze relevant studies in those areas, highlighting key findings and identifying gaps in the research. The review would conclude by emphasizing the need for further research on specific aspects of the relationship between climate change and biodiversity. The following literature review template provides a glimpse into the recommended literature review structure and content, demonstrating how research findings are organized around specific themes within a broader topic.
Literature Review on Climate Change Impacts on Biodiversity:
Climate change is a global phenomenon with far-reaching consequences, including significant impacts on biodiversity. This literature review synthesizes key findings from various studies:
a. Habitat Loss and Species Extinction:
Climate change-induced alterations in temperature and precipitation patterns contribute to habitat loss, affecting numerous species (Thomas et al., 2004). The review discusses how these changes increase the risk of extinction, particularly for species with specific habitat requirements.
b. Range Shifts and Phenological Changes:
Observations of range shifts and changes in the timing of biological events (phenology) are documented in response to changing climatic conditions (Parmesan & Yohe, 2003). These shifts affect ecosystems and may lead to mismatches between species and their resources.
c. Ocean Acidification and Coral Reefs:
The review explores the impact of climate change on marine biodiversity, emphasizing ocean acidification’s threat to coral reefs (Hoegh-Guldberg et al., 2007). Changes in pH levels negatively affect coral calcification, disrupting the delicate balance of marine ecosystems.
d. Adaptive Strategies and Conservation Efforts:
Recognizing the urgency of the situation, the literature review discusses various adaptive strategies adopted by species and conservation efforts aimed at mitigating the impacts of climate change on biodiversity (Hannah et al., 2007). It emphasizes the importance of interdisciplinary approaches for effective conservation planning.
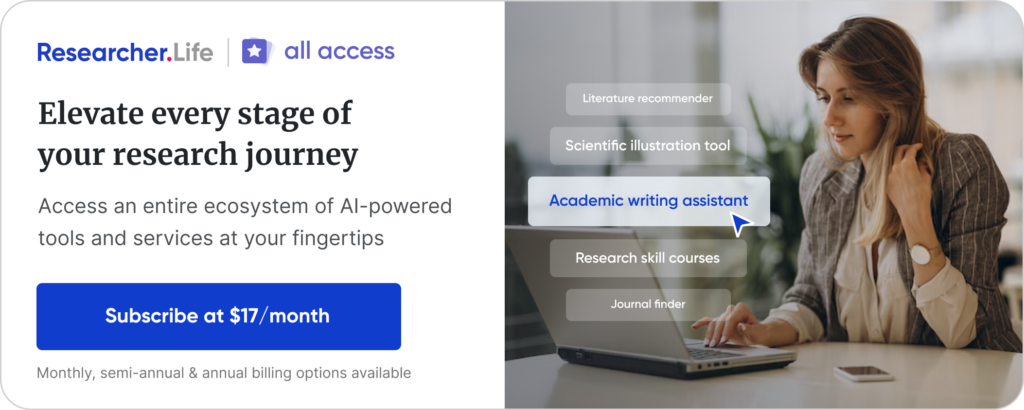
How to write a good literature review
Writing a literature review involves summarizing and synthesizing existing research on a particular topic. A good literature review format should include the following elements.
Introduction: The introduction sets the stage for your literature review, providing context and introducing the main focus of your review.
- Opening Statement: Begin with a general statement about the broader topic and its significance in the field.
- Scope and Purpose: Clearly define the scope of your literature review. Explain the specific research question or objective you aim to address.
- Organizational Framework: Briefly outline the structure of your literature review, indicating how you will categorize and discuss the existing research.
- Significance of the Study: Highlight why your literature review is important and how it contributes to the understanding of the chosen topic.
- Thesis Statement: Conclude the introduction with a concise thesis statement that outlines the main argument or perspective you will develop in the body of the literature review.
Body: The body of the literature review is where you provide a comprehensive analysis of existing literature, grouping studies based on themes, methodologies, or other relevant criteria.
- Organize by Theme or Concept: Group studies that share common themes, concepts, or methodologies. Discuss each theme or concept in detail, summarizing key findings and identifying gaps or areas of disagreement.
- Critical Analysis: Evaluate the strengths and weaknesses of each study. Discuss the methodologies used, the quality of evidence, and the overall contribution of each work to the understanding of the topic.
- Synthesis of Findings: Synthesize the information from different studies to highlight trends, patterns, or areas of consensus in the literature.
- Identification of Gaps: Discuss any gaps or limitations in the existing research and explain how your review contributes to filling these gaps.
- Transition between Sections: Provide smooth transitions between different themes or concepts to maintain the flow of your literature review.
Conclusion: The conclusion of your literature review should summarize the main findings, highlight the contributions of the review, and suggest avenues for future research.
- Summary of Key Findings: Recap the main findings from the literature and restate how they contribute to your research question or objective.
- Contributions to the Field: Discuss the overall contribution of your literature review to the existing knowledge in the field.
- Implications and Applications: Explore the practical implications of the findings and suggest how they might impact future research or practice.
- Recommendations for Future Research: Identify areas that require further investigation and propose potential directions for future research in the field.
- Final Thoughts: Conclude with a final reflection on the importance of your literature review and its relevance to the broader academic community.
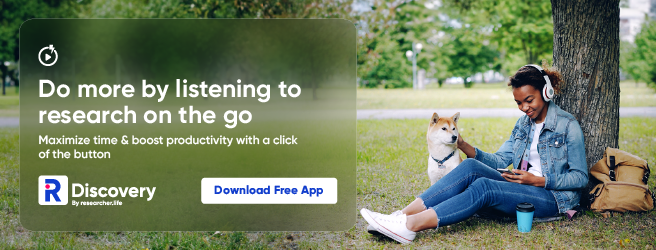
Conducting a literature review
Conducting a literature review is an essential step in research that involves reviewing and analyzing existing literature on a specific topic. It’s important to know how to do a literature review effectively, so here are the steps to follow: 1
Choose a Topic and Define the Research Question:
- Select a topic that is relevant to your field of study.
- Clearly define your research question or objective. Determine what specific aspect of the topic do you want to explore?
Decide on the Scope of Your Review:
- Determine the timeframe for your literature review. Are you focusing on recent developments, or do you want a historical overview?
- Consider the geographical scope. Is your review global, or are you focusing on a specific region?
- Define the inclusion and exclusion criteria. What types of sources will you include? Are there specific types of studies or publications you will exclude?
Select Databases for Searches:
- Identify relevant databases for your field. Examples include PubMed, IEEE Xplore, Scopus, Web of Science, and Google Scholar.
- Consider searching in library catalogs, institutional repositories, and specialized databases related to your topic.
Conduct Searches and Keep Track:
- Develop a systematic search strategy using keywords, Boolean operators (AND, OR, NOT), and other search techniques.
- Record and document your search strategy for transparency and replicability.
- Keep track of the articles, including publication details, abstracts, and links. Use citation management tools like EndNote, Zotero, or Mendeley to organize your references.
Review the Literature:
- Evaluate the relevance and quality of each source. Consider the methodology, sample size, and results of studies.
- Organize the literature by themes or key concepts. Identify patterns, trends, and gaps in the existing research.
- Summarize key findings and arguments from each source. Compare and contrast different perspectives.
- Identify areas where there is a consensus in the literature and where there are conflicting opinions.
- Provide critical analysis and synthesis of the literature. What are the strengths and weaknesses of existing research?
Organize and Write Your Literature Review:
- Literature review outline should be based on themes, chronological order, or methodological approaches.
- Write a clear and coherent narrative that synthesizes the information gathered.
- Use proper citations for each source and ensure consistency in your citation style (APA, MLA, Chicago, etc.).
- Conclude your literature review by summarizing key findings, identifying gaps, and suggesting areas for future research.
The literature review sample and detailed advice on writing and conducting a review will help you produce a well-structured report. But remember that a literature review is an ongoing process, and it may be necessary to revisit and update it as your research progresses.
Frequently asked questions
A literature review is a critical and comprehensive analysis of existing literature (published and unpublished works) on a specific topic or research question and provides a synthesis of the current state of knowledge in a particular field. A well-conducted literature review is crucial for researchers to build upon existing knowledge, avoid duplication of efforts, and contribute to the advancement of their field. It also helps researchers situate their work within a broader context and facilitates the development of a sound theoretical and conceptual framework for their studies.
Literature review is a crucial component of research writing, providing a solid background for a research paper’s investigation. The aim is to keep professionals up to date by providing an understanding of ongoing developments within a specific field, including research methods, and experimental techniques used in that field, and present that knowledge in the form of a written report. Also, the depth and breadth of the literature review emphasizes the credibility of the scholar in his or her field.
Before writing a literature review, it’s essential to undertake several preparatory steps to ensure that your review is well-researched, organized, and focused. This includes choosing a topic of general interest to you and doing exploratory research on that topic, writing an annotated bibliography, and noting major points, especially those that relate to the position you have taken on the topic.
Literature reviews and academic research papers are essential components of scholarly work but serve different purposes within the academic realm. 3 A literature review aims to provide a foundation for understanding the current state of research on a particular topic, identify gaps or controversies, and lay the groundwork for future research. Therefore, it draws heavily from existing academic sources, including books, journal articles, and other scholarly publications. In contrast, an academic research paper aims to present new knowledge, contribute to the academic discourse, and advance the understanding of a specific research question. Therefore, it involves a mix of existing literature (in the introduction and literature review sections) and original data or findings obtained through research methods.
Literature reviews are essential components of academic and research papers, and various strategies can be employed to conduct them effectively. If you want to know how to write a literature review for a research paper, here are four common approaches that are often used by researchers. Chronological Review: This strategy involves organizing the literature based on the chronological order of publication. It helps to trace the development of a topic over time, showing how ideas, theories, and research have evolved. Thematic Review: Thematic reviews focus on identifying and analyzing themes or topics that cut across different studies. Instead of organizing the literature chronologically, it is grouped by key themes or concepts, allowing for a comprehensive exploration of various aspects of the topic. Methodological Review: This strategy involves organizing the literature based on the research methods employed in different studies. It helps to highlight the strengths and weaknesses of various methodologies and allows the reader to evaluate the reliability and validity of the research findings. Theoretical Review: A theoretical review examines the literature based on the theoretical frameworks used in different studies. This approach helps to identify the key theories that have been applied to the topic and assess their contributions to the understanding of the subject. It’s important to note that these strategies are not mutually exclusive, and a literature review may combine elements of more than one approach. The choice of strategy depends on the research question, the nature of the literature available, and the goals of the review. Additionally, other strategies, such as integrative reviews or systematic reviews, may be employed depending on the specific requirements of the research.
The literature review format can vary depending on the specific publication guidelines. However, there are some common elements and structures that are often followed. Here is a general guideline for the format of a literature review: Introduction: Provide an overview of the topic. Define the scope and purpose of the literature review. State the research question or objective. Body: Organize the literature by themes, concepts, or chronology. Critically analyze and evaluate each source. Discuss the strengths and weaknesses of the studies. Highlight any methodological limitations or biases. Identify patterns, connections, or contradictions in the existing research. Conclusion: Summarize the key points discussed in the literature review. Highlight the research gap. Address the research question or objective stated in the introduction. Highlight the contributions of the review and suggest directions for future research.
Both annotated bibliographies and literature reviews involve the examination of scholarly sources. While annotated bibliographies focus on individual sources with brief annotations, literature reviews provide a more in-depth, integrated, and comprehensive analysis of existing literature on a specific topic. The key differences are as follows:
References
- Denney, A. S., & Tewksbury, R. (2013). How to write a literature review. Journal of criminal justice education , 24 (2), 218-234.
- Pan, M. L. (2016). Preparing literature reviews: Qualitative and quantitative approaches . Taylor & Francis.
- Cantero, C. (2019). How to write a literature review. San José State University Writing Center .
Paperpal is an AI writing assistant that help academics write better, faster with real-time suggestions for in-depth language and grammar correction. Trained on millions of research manuscripts enhanced by professional academic editors, Paperpal delivers human precision at machine speed.
Try it for free or upgrade to Paperpal Prime , which unlocks unlimited access to premium features like academic translation, paraphrasing, contextual synonyms, consistency checks and more. It’s like always having a professional academic editor by your side! Go beyond limitations and experience the future of academic writing. Get Paperpal Prime now at just US$19 a month!
Related Reads:
- Empirical Research: A Comprehensive Guide for Academics
- How to Write a Scientific Paper in 10 Steps
- Life Sciences Papers: 9 Tips for Authors Writing in Biological Sciences
- What is an Argumentative Essay? How to Write It (With Examples)
6 Tips for Post-Doc Researchers to Take Their Career to the Next Level
Self-plagiarism in research: what it is and how to avoid it, you may also like, what is hedging in academic writing , how to use ai to enhance your college..., ai + human expertise – a paradigm shift..., how to use paperpal to generate emails &..., ai in education: it’s time to change the..., is it ethical to use ai-generated abstracts without..., do plagiarism checkers detect ai content, word choice problems: how to use the right..., how to avoid plagiarism when using generative ai..., what are journal guidelines on using generative ai....
Have a language expert improve your writing
Run a free plagiarism check in 10 minutes, automatically generate references for free.
- Knowledge Base
- Dissertation
- What is a Literature Review? | Guide, Template, & Examples
What is a Literature Review? | Guide, Template, & Examples
Published on 22 February 2022 by Shona McCombes . Revised on 7 June 2022.
What is a literature review? A literature review is a survey of scholarly sources on a specific topic. It provides an overview of current knowledge, allowing you to identify relevant theories, methods, and gaps in the existing research.
There are five key steps to writing a literature review:
- Search for relevant literature
- Evaluate sources
- Identify themes, debates and gaps
- Outline the structure
- Write your literature review
A good literature review doesn’t just summarise sources – it analyses, synthesises, and critically evaluates to give a clear picture of the state of knowledge on the subject.
Instantly correct all language mistakes in your text
Be assured that you'll submit flawless writing. Upload your document to correct all your mistakes.
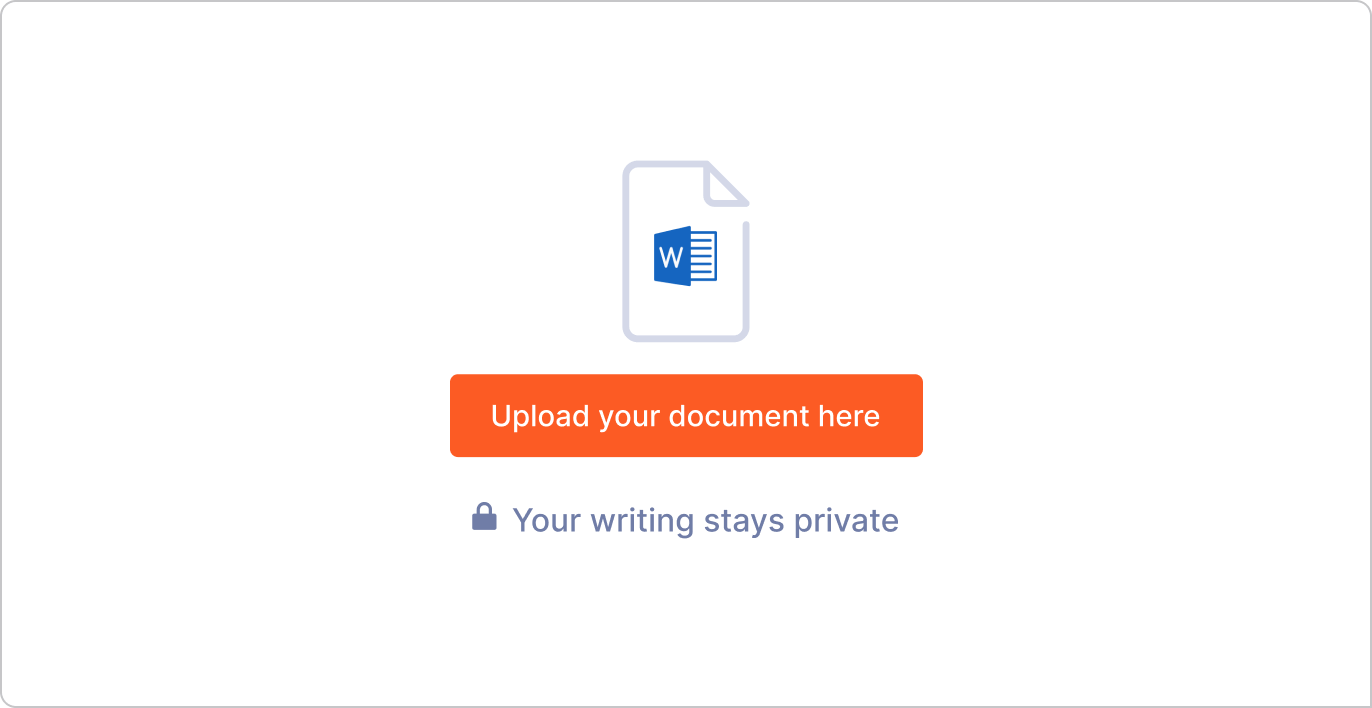
Table of contents
Why write a literature review, examples of literature reviews, step 1: search for relevant literature, step 2: evaluate and select sources, step 3: identify themes, debates and gaps, step 4: outline your literature review’s structure, step 5: write your literature review, frequently asked questions about literature reviews, introduction.
- Quick Run-through
- Step 1 & 2
When you write a dissertation or thesis, you will have to conduct a literature review to situate your research within existing knowledge. The literature review gives you a chance to:
- Demonstrate your familiarity with the topic and scholarly context
- Develop a theoretical framework and methodology for your research
- Position yourself in relation to other researchers and theorists
- Show how your dissertation addresses a gap or contributes to a debate
You might also have to write a literature review as a stand-alone assignment. In this case, the purpose is to evaluate the current state of research and demonstrate your knowledge of scholarly debates around a topic.
The content will look slightly different in each case, but the process of conducting a literature review follows the same steps. We’ve written a step-by-step guide that you can follow below.
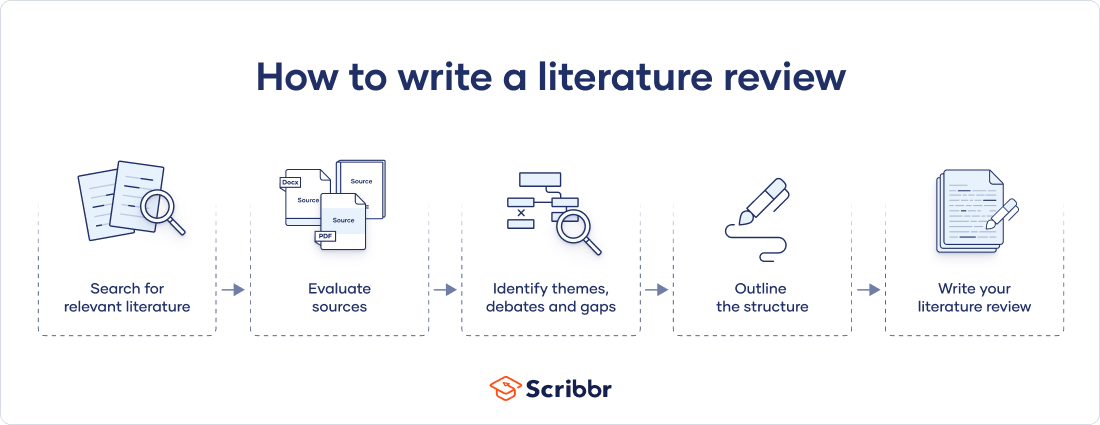
The only proofreading tool specialized in correcting academic writing
The academic proofreading tool has been trained on 1000s of academic texts and by native English editors. Making it the most accurate and reliable proofreading tool for students.
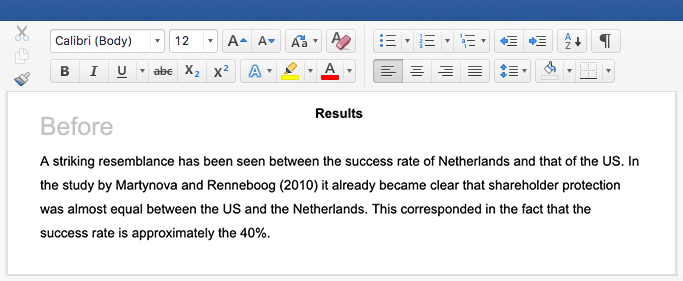
Correct my document today
Writing literature reviews can be quite challenging! A good starting point could be to look at some examples, depending on what kind of literature review you’d like to write.
- Example literature review #1: “Why Do People Migrate? A Review of the Theoretical Literature” ( Theoretical literature review about the development of economic migration theory from the 1950s to today.)
- Example literature review #2: “Literature review as a research methodology: An overview and guidelines” ( Methodological literature review about interdisciplinary knowledge acquisition and production.)
- Example literature review #3: “The Use of Technology in English Language Learning: A Literature Review” ( Thematic literature review about the effects of technology on language acquisition.)
- Example literature review #4: “Learners’ Listening Comprehension Difficulties in English Language Learning: A Literature Review” ( Chronological literature review about how the concept of listening skills has changed over time.)
You can also check out our templates with literature review examples and sample outlines at the links below.
Download Word doc Download Google doc
Before you begin searching for literature, you need a clearly defined topic .
If you are writing the literature review section of a dissertation or research paper, you will search for literature related to your research objectives and questions .
If you are writing a literature review as a stand-alone assignment, you will have to choose a focus and develop a central question to direct your search. Unlike a dissertation research question, this question has to be answerable without collecting original data. You should be able to answer it based only on a review of existing publications.
Make a list of keywords
Start by creating a list of keywords related to your research topic. Include each of the key concepts or variables you’re interested in, and list any synonyms and related terms. You can add to this list if you discover new keywords in the process of your literature search.
- Social media, Facebook, Instagram, Twitter, Snapchat, TikTok
- Body image, self-perception, self-esteem, mental health
- Generation Z, teenagers, adolescents, youth
Search for relevant sources
Use your keywords to begin searching for sources. Some databases to search for journals and articles include:
- Your university’s library catalogue
- Google Scholar
- Project Muse (humanities and social sciences)
- Medline (life sciences and biomedicine)
- EconLit (economics)
- Inspec (physics, engineering and computer science)
You can use boolean operators to help narrow down your search:
Read the abstract to find out whether an article is relevant to your question. When you find a useful book or article, you can check the bibliography to find other relevant sources.
To identify the most important publications on your topic, take note of recurring citations. If the same authors, books or articles keep appearing in your reading, make sure to seek them out.
You probably won’t be able to read absolutely everything that has been written on the topic – you’ll have to evaluate which sources are most relevant to your questions.
For each publication, ask yourself:
- What question or problem is the author addressing?
- What are the key concepts and how are they defined?
- What are the key theories, models and methods? Does the research use established frameworks or take an innovative approach?
- What are the results and conclusions of the study?
- How does the publication relate to other literature in the field? Does it confirm, add to, or challenge established knowledge?
- How does the publication contribute to your understanding of the topic? What are its key insights and arguments?
- What are the strengths and weaknesses of the research?
Make sure the sources you use are credible, and make sure you read any landmark studies and major theories in your field of research.
You can find out how many times an article has been cited on Google Scholar – a high citation count means the article has been influential in the field, and should certainly be included in your literature review.
The scope of your review will depend on your topic and discipline: in the sciences you usually only review recent literature, but in the humanities you might take a long historical perspective (for example, to trace how a concept has changed in meaning over time).
Remember that you can use our template to summarise and evaluate sources you’re thinking about using!
Take notes and cite your sources
As you read, you should also begin the writing process. Take notes that you can later incorporate into the text of your literature review.
It’s important to keep track of your sources with references to avoid plagiarism . It can be helpful to make an annotated bibliography, where you compile full reference information and write a paragraph of summary and analysis for each source. This helps you remember what you read and saves time later in the process.
You can use our free APA Reference Generator for quick, correct, consistent citations.
To begin organising your literature review’s argument and structure, you need to understand the connections and relationships between the sources you’ve read. Based on your reading and notes, you can look for:
- Trends and patterns (in theory, method or results): do certain approaches become more or less popular over time?
- Themes: what questions or concepts recur across the literature?
- Debates, conflicts and contradictions: where do sources disagree?
- Pivotal publications: are there any influential theories or studies that changed the direction of the field?
- Gaps: what is missing from the literature? Are there weaknesses that need to be addressed?
This step will help you work out the structure of your literature review and (if applicable) show how your own research will contribute to existing knowledge.
- Most research has focused on young women.
- There is an increasing interest in the visual aspects of social media.
- But there is still a lack of robust research on highly-visual platforms like Instagram and Snapchat – this is a gap that you could address in your own research.
There are various approaches to organising the body of a literature review. You should have a rough idea of your strategy before you start writing.
Depending on the length of your literature review, you can combine several of these strategies (for example, your overall structure might be thematic, but each theme is discussed chronologically).
Chronological
The simplest approach is to trace the development of the topic over time. However, if you choose this strategy, be careful to avoid simply listing and summarising sources in order.
Try to analyse patterns, turning points and key debates that have shaped the direction of the field. Give your interpretation of how and why certain developments occurred.
If you have found some recurring central themes, you can organise your literature review into subsections that address different aspects of the topic.
For example, if you are reviewing literature about inequalities in migrant health outcomes, key themes might include healthcare policy, language barriers, cultural attitudes, legal status, and economic access.
Methodological
If you draw your sources from different disciplines or fields that use a variety of research methods , you might want to compare the results and conclusions that emerge from different approaches. For example:
- Look at what results have emerged in qualitative versus quantitative research
- Discuss how the topic has been approached by empirical versus theoretical scholarship
- Divide the literature into sociological, historical, and cultural sources
Theoretical
A literature review is often the foundation for a theoretical framework . You can use it to discuss various theories, models, and definitions of key concepts.
You might argue for the relevance of a specific theoretical approach, or combine various theoretical concepts to create a framework for your research.
Like any other academic text, your literature review should have an introduction , a main body, and a conclusion . What you include in each depends on the objective of your literature review.
The introduction should clearly establish the focus and purpose of the literature review.
If you are writing the literature review as part of your dissertation or thesis, reiterate your central problem or research question and give a brief summary of the scholarly context. You can emphasise the timeliness of the topic (“many recent studies have focused on the problem of x”) or highlight a gap in the literature (“while there has been much research on x, few researchers have taken y into consideration”).
Depending on the length of your literature review, you might want to divide the body into subsections. You can use a subheading for each theme, time period, or methodological approach.
As you write, make sure to follow these tips:
- Summarise and synthesise: give an overview of the main points of each source and combine them into a coherent whole.
- Analyse and interpret: don’t just paraphrase other researchers – add your own interpretations, discussing the significance of findings in relation to the literature as a whole.
- Critically evaluate: mention the strengths and weaknesses of your sources.
- Write in well-structured paragraphs: use transitions and topic sentences to draw connections, comparisons and contrasts.
In the conclusion, you should summarise the key findings you have taken from the literature and emphasise their significance.
If the literature review is part of your dissertation or thesis, reiterate how your research addresses gaps and contributes new knowledge, or discuss how you have drawn on existing theories and methods to build a framework for your research. This can lead directly into your methodology section.
A literature review is a survey of scholarly sources (such as books, journal articles, and theses) related to a specific topic or research question .
It is often written as part of a dissertation , thesis, research paper , or proposal .
There are several reasons to conduct a literature review at the beginning of a research project:
- To familiarise yourself with the current state of knowledge on your topic
- To ensure that you’re not just repeating what others have already done
- To identify gaps in knowledge and unresolved problems that your research can address
- To develop your theoretical framework and methodology
- To provide an overview of the key findings and debates on the topic
Writing the literature review shows your reader how your work relates to existing research and what new insights it will contribute.
The literature review usually comes near the beginning of your dissertation . After the introduction , it grounds your research in a scholarly field and leads directly to your theoretical framework or methodology .
Cite this Scribbr article
If you want to cite this source, you can copy and paste the citation or click the ‘Cite this Scribbr article’ button to automatically add the citation to our free Reference Generator.
McCombes, S. (2022, June 07). What is a Literature Review? | Guide, Template, & Examples. Scribbr. Retrieved 9 April 2024, from https://www.scribbr.co.uk/thesis-dissertation/literature-review/
Is this article helpful?
Shona McCombes
Other students also liked, how to write a dissertation proposal | a step-by-step guide, what is a theoretical framework | a step-by-step guide, what is a research methodology | steps & tips.
- Resources Home 🏠
- Try SciSpace Copilot
- Search research papers
- Add Copilot Extension
- Try AI Detector
- Try Paraphraser
- Try Citation Generator
- April Papers
- June Papers
- July Papers

How To Write A Literature Review - A Complete Guide

Table of Contents
A literature review is much more than just another section in your research paper. It forms the very foundation of your research. It is a formal piece of writing where you analyze the existing theoretical framework, principles, and assumptions and use that as a base to shape your approach to the research question.
Curating and drafting a solid literature review section not only lends more credibility to your research paper but also makes your research tighter and better focused. But, writing literature reviews is a difficult task. It requires extensive reading, plus you have to consider market trends and technological and political changes, which tend to change in the blink of an eye.
Now streamline your literature review process with the help of SciSpace Copilot. With this AI research assistant, you can efficiently synthesize and analyze a vast amount of information, identify key themes and trends, and uncover gaps in the existing research. Get real-time explanations, summaries, and answers to your questions for the paper you're reviewing, making navigating and understanding the complex literature landscape easier.
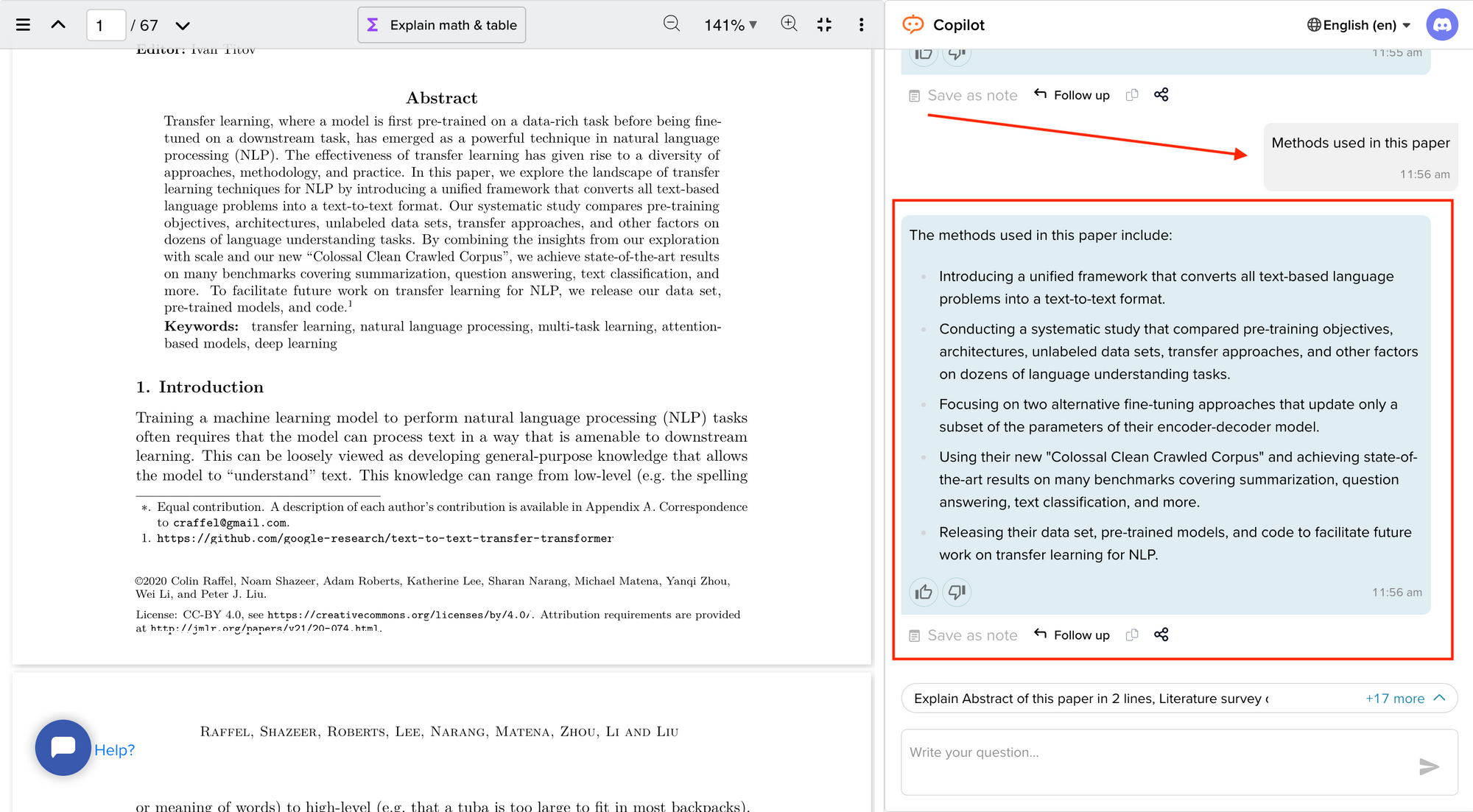
In this comprehensive guide, we will explore everything from the definition of a literature review, its appropriate length, various types of literature reviews, and how to write one.
What is a literature review?
A literature review is a collation of survey, research, critical evaluation, and assessment of the existing literature in a preferred domain.
Eminent researcher and academic Arlene Fink, in her book Conducting Research Literature Reviews , defines it as the following:
“A literature review surveys books, scholarly articles, and any other sources relevant to a particular issue, area of research, or theory, and by so doing, provides a description, summary, and critical evaluation of these works in relation to the research problem being investigated.
Literature reviews are designed to provide an overview of sources you have explored while researching a particular topic, and to demonstrate to your readers how your research fits within a larger field of study.”
Simply put, a literature review can be defined as a critical discussion of relevant pre-existing research around your research question and carving out a definitive place for your study in the existing body of knowledge. Literature reviews can be presented in multiple ways: a section of an article, the whole research paper itself, or a chapter of your thesis.
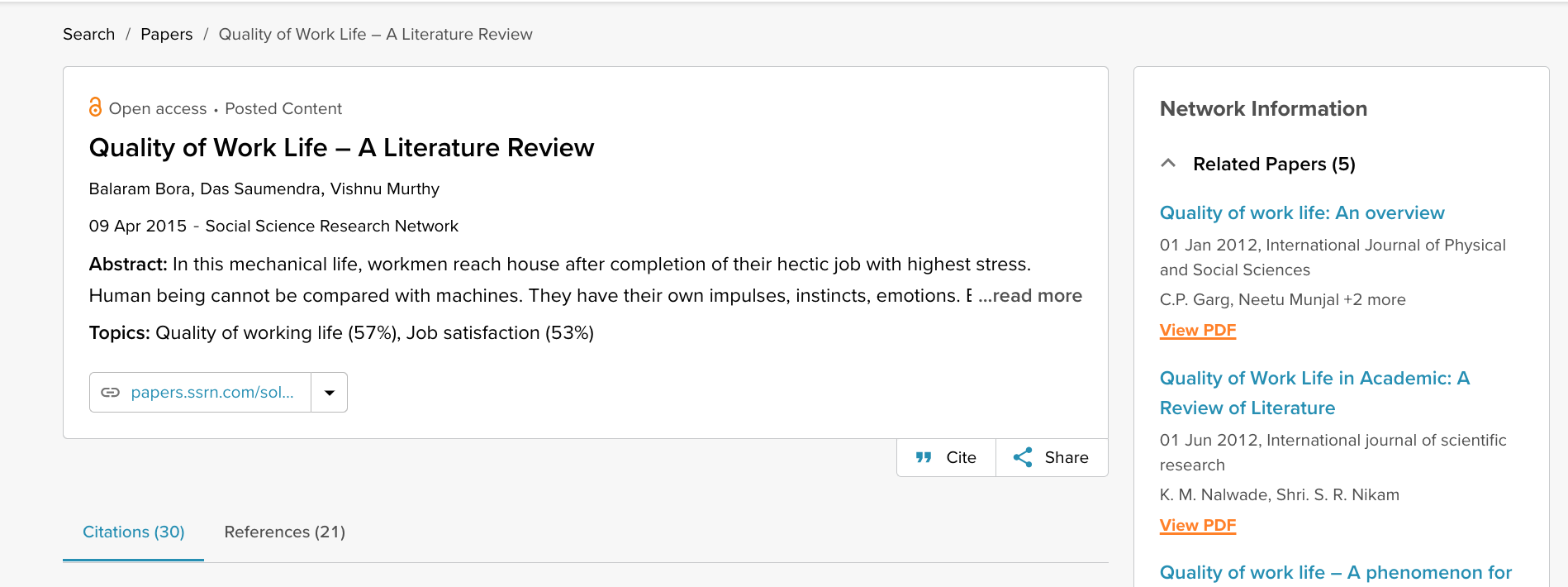
A literature review does function as a summary of sources, but it also allows you to analyze further, interpret, and examine the stated theories, methods, viewpoints, and, of course, the gaps in the existing content.
As an author, you can discuss and interpret the research question and its various aspects and debate your adopted methods to support the claim.
What is the purpose of a literature review?
A literature review is meant to help your readers understand the relevance of your research question and where it fits within the existing body of knowledge. As a researcher, you should use it to set the context, build your argument, and establish the need for your study.
What is the importance of a literature review?
The literature review is a critical part of research papers because it helps you:
- Gain an in-depth understanding of your research question and the surrounding area
- Convey that you have a thorough understanding of your research area and are up-to-date with the latest changes and advancements
- Establish how your research is connected or builds on the existing body of knowledge and how it could contribute to further research
- Elaborate on the validity and suitability of your theoretical framework and research methodology
- Identify and highlight gaps and shortcomings in the existing body of knowledge and how things need to change
- Convey to readers how your study is different or how it contributes to the research area
How long should a literature review be?
Ideally, the literature review should take up 15%-40% of the total length of your manuscript. So, if you have a 10,000-word research paper, the minimum word count could be 1500.
Your literature review format depends heavily on the kind of manuscript you are writing — an entire chapter in case of doctoral theses, a part of the introductory section in a research article, to a full-fledged review article that examines the previously published research on a topic.
Another determining factor is the type of research you are doing. The literature review section tends to be longer for secondary research projects than primary research projects.
What are the different types of literature reviews?
All literature reviews are not the same. There are a variety of possible approaches that you can take. It all depends on the type of research you are pursuing.
Here are the different types of literature reviews:
Argumentative review
It is called an argumentative review when you carefully present literature that only supports or counters a specific argument or premise to establish a viewpoint.
Integrative review
It is a type of literature review focused on building a comprehensive understanding of a topic by combining available theoretical frameworks and empirical evidence.
Methodological review
This approach delves into the ''how'' and the ''what" of the research question — you cannot look at the outcome in isolation; you should also review the methodology used.
Systematic review
This form consists of an overview of existing evidence pertinent to a clearly formulated research question, which uses pre-specified and standardized methods to identify and critically appraise relevant research and collect, report, and analyze data from the studies included in the review.
Meta-analysis review
Meta-analysis uses statistical methods to summarize the results of independent studies. By combining information from all relevant studies, meta-analysis can provide more precise estimates of the effects than those derived from the individual studies included within a review.
Historical review
Historical literature reviews focus on examining research throughout a period, often starting with the first time an issue, concept, theory, or phenomenon emerged in the literature, then tracing its evolution within the scholarship of a discipline. The purpose is to place research in a historical context to show familiarity with state-of-the-art developments and identify future research's likely directions.
Theoretical Review
This form aims to examine the corpus of theory accumulated regarding an issue, concept, theory, and phenomenon. The theoretical literature review helps to establish what theories exist, the relationships between them, the degree the existing approaches have been investigated, and to develop new hypotheses to be tested.
Scoping Review
The Scoping Review is often used at the beginning of an article, dissertation, or research proposal. It is conducted before the research to highlight gaps in the existing body of knowledge and explains why the project should be greenlit.
State-of-the-Art Review
The State-of-the-Art review is conducted periodically, focusing on the most recent research. It describes what is currently known, understood, or agreed upon regarding the research topic and highlights where there are still disagreements.
Can you use the first person in a literature review?
When writing literature reviews, you should avoid the usage of first-person pronouns. It means that instead of "I argue that" or "we argue that," the appropriate expression would be "this research paper argues that."
Do you need an abstract for a literature review?
Ideally, yes. It is always good to have a condensed summary that is self-contained and independent of the rest of your review. As for how to draft one, you can follow the same fundamental idea when preparing an abstract for a literature review. It should also include:
- The research topic and your motivation behind selecting it
- A one-sentence thesis statement
- An explanation of the kinds of literature featured in the review
- Summary of what you've learned
- Conclusions you drew from the literature you reviewed
- Potential implications and future scope for research
Here's an example of the abstract of a literature review
Is a literature review written in the past tense?
Yes, the literature review should ideally be written in the past tense. You should not use the present or future tense when writing one. The exceptions are when you have statements describing events that happened earlier than the literature you are reviewing or events that are currently occurring; then, you can use the past perfect or present perfect tenses.
How many sources for a literature review?
There are multiple approaches to deciding how many sources to include in a literature review section. The first approach would be to look level you are at as a researcher. For instance, a doctoral thesis might need 60+ sources. In contrast, you might only need to refer to 5-15 sources at the undergraduate level.
The second approach is based on the kind of literature review you are doing — whether it is merely a chapter of your paper or if it is a self-contained paper in itself. When it is just a chapter, sources should equal the total number of pages in your article's body. In the second scenario, you need at least three times as many sources as there are pages in your work.
Quick tips on how to write a literature review
To know how to write a literature review, you must clearly understand its impact and role in establishing your work as substantive research material.
You need to follow the below-mentioned steps, to write a literature review:
- Outline the purpose behind the literature review
- Search relevant literature
- Examine and assess the relevant resources
- Discover connections by drawing deep insights from the resources
- Structure planning to write a good literature review
1. Outline and identify the purpose of a literature review
As a first step on how to write a literature review, you must know what the research question or topic is and what shape you want your literature review to take. Ensure you understand the research topic inside out, or else seek clarifications. You must be able to the answer below questions before you start:
- How many sources do I need to include?
- What kind of sources should I analyze?
- How much should I critically evaluate each source?
- Should I summarize, synthesize or offer a critique of the sources?
- Do I need to include any background information or definitions?
Additionally, you should know that the narrower your research topic is, the swifter it will be for you to restrict the number of sources to be analyzed.
2. Search relevant literature
Dig deeper into search engines to discover what has already been published around your chosen topic. Make sure you thoroughly go through appropriate reference sources like books, reports, journal articles, government docs, and web-based resources.
You must prepare a list of keywords and their different variations. You can start your search from any library’s catalog, provided you are an active member of that institution. The exact keywords can be extended to widen your research over other databases and academic search engines like:
- Google Scholar
- Microsoft Academic
- Science.gov
Besides, it is not advisable to go through every resource word by word. Alternatively, what you can do is you can start by reading the abstract and then decide whether that source is relevant to your research or not.
Additionally, you must spend surplus time assessing the quality and relevance of resources. It would help if you tried preparing a list of citations to ensure that there lies no repetition of authors, publications, or articles in the literature review.
3. Examine and assess the sources
It is nearly impossible for you to go through every detail in the research article. So rather than trying to fetch every detail, you have to analyze and decide which research sources resemble closest and appear relevant to your chosen domain.
While analyzing the sources, you should look to find out answers to questions like:
- What question or problem has the author been describing and debating?
- What is the definition of critical aspects?
- How well the theories, approach, and methodology have been explained?
- Whether the research theory used some conventional or new innovative approach?
- How relevant are the key findings of the work?
- In what ways does it relate to other sources on the same topic?
- What challenges does this research paper pose to the existing theory
- What are the possible contributions or benefits it adds to the subject domain?
Be always mindful that you refer only to credible and authentic resources. It would be best if you always take references from different publications to validate your theory.
Always keep track of important information or data you can present in your literature review right from the beginning. It will help steer your path from any threats of plagiarism and also make it easier to curate an annotated bibliography or reference section.
4. Discover connections
At this stage, you must start deciding on the argument and structure of your literature review. To accomplish this, you must discover and identify the relations and connections between various resources while drafting your abstract.
A few aspects that you should be aware of while writing a literature review include:
- Rise to prominence: Theories and methods that have gained reputation and supporters over time.
- Constant scrutiny: Concepts or theories that repeatedly went under examination.
- Contradictions and conflicts: Theories, both the supporting and the contradictory ones, for the research topic.
- Knowledge gaps: What exactly does it fail to address, and how to bridge them with further research?
- Influential resources: Significant research projects available that have been upheld as milestones or perhaps, something that can modify the current trends
Once you join the dots between various past research works, it will be easier for you to draw a conclusion and identify your contribution to the existing knowledge base.
5. Structure planning to write a good literature review
There exist different ways towards planning and executing the structure of a literature review. The format of a literature review varies and depends upon the length of the research.
Like any other research paper, the literature review format must contain three sections: introduction, body, and conclusion. The goals and objectives of the research question determine what goes inside these three sections.
Nevertheless, a good literature review can be structured according to the chronological, thematic, methodological, or theoretical framework approach.
Literature review samples
1. Standalone
2. As a section of a research paper
How SciSpace Discover makes literature review a breeze?
SciSpace Discover is a one-stop solution to do an effective literature search and get barrier-free access to scientific knowledge. It is an excellent repository where you can find millions of only peer-reviewed articles and full-text PDF files. Here’s more on how you can use it:
Find the right information
Find what you want quickly and easily with comprehensive search filters that let you narrow down papers according to PDF availability, year of publishing, document type, and affiliated institution. Moreover, you can sort the results based on the publishing date, citation count, and relevance.
Assess credibility of papers quickly
When doing the literature review, it is critical to establish the quality of your sources. They form the foundation of your research. SciSpace Discover helps you assess the quality of a source by providing an overview of its references, citations, and performance metrics.
Get the complete picture in no time
SciSpace Discover’s personalized suggestion engine helps you stay on course and get the complete picture of the topic from one place. Every time you visit an article page, it provides you links to related papers. Besides that, it helps you understand what’s trending, who are the top authors, and who are the leading publishers on a topic.
Make referring sources super easy
To ensure you don't lose track of your sources, you must start noting down your references when doing the literature review. SciSpace Discover makes this step effortless. Click the 'cite' button on an article page, and you will receive preloaded citation text in multiple styles — all you've to do is copy-paste it into your manuscript.
Final tips on how to write a literature review
A massive chunk of time and effort is required to write a good literature review. But, if you go about it systematically, you'll be able to save a ton of time and build a solid foundation for your research.
We hope this guide has helped you answer several key questions you have about writing literature reviews.
Would you like to explore SciSpace Discover and kick off your literature search right away? You can get started here .
Frequently Asked Questions (FAQs)
1. how to start a literature review.
• What questions do you want to answer?
• What sources do you need to answer these questions?
• What information do these sources contain?
• How can you use this information to answer your questions?
2. What to include in a literature review?
• A brief background of the problem or issue
• What has previously been done to address the problem or issue
• A description of what you will do in your project
• How this study will contribute to research on the subject
3. Why literature review is important?
The literature review is an important part of any research project because it allows the writer to look at previous studies on a topic and determine existing gaps in the literature, as well as what has already been done. It will also help them to choose the most appropriate method for their own study.
4. How to cite a literature review in APA format?
To cite a literature review in APA style, you need to provide the author's name, the title of the article, and the year of publication. For example: Patel, A. B., & Stokes, G. S. (2012). The relationship between personality and intelligence: A meta-analysis of longitudinal research. Personality and Individual Differences, 53(1), 16-21
5. What are the components of a literature review?
• A brief introduction to the topic, including its background and context. The introduction should also include a rationale for why the study is being conducted and what it will accomplish.
• A description of the methodologies used in the study. This can include information about data collection methods, sample size, and statistical analyses.
• A presentation of the findings in an organized format that helps readers follow along with the author's conclusions.
6. What are common errors in writing literature review?
• Not spending enough time to critically evaluate the relevance of resources, observations and conclusions.
• Totally relying on secondary data while ignoring primary data.
• Letting your personal bias seep into your interpretation of existing literature.
• No detailed explanation of the procedure to discover and identify an appropriate literature review.
7. What are the 5 C's of writing literature review?
• Cite - the sources you utilized and referenced in your research.
• Compare - existing arguments, hypotheses, methodologies, and conclusions found in the knowledge base.
• Contrast - the arguments, topics, methodologies, approaches, and disputes that may be found in the literature.
• Critique - the literature and describe the ideas and opinions you find more convincing and why.
• Connect - the various studies you reviewed in your research.
8. How many sources should a literature review have?
When it is just a chapter, sources should equal the total number of pages in your article's body. if it is a self-contained paper in itself, you need at least three times as many sources as there are pages in your work.
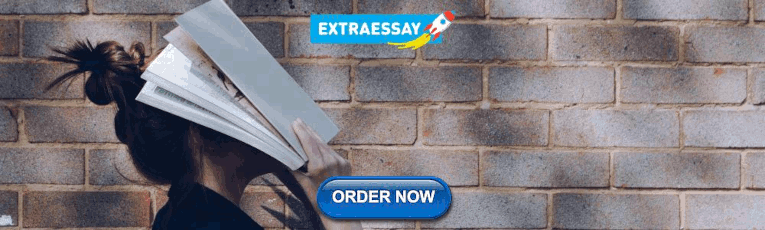
9. Can literature review have diagrams?
• To represent an abstract idea or concept
• To explain the steps of a process or procedure
• To help readers understand the relationships between different concepts
10. How old should sources be in a literature review?
Sources for a literature review should be as current as possible or not older than ten years. The only exception to this rule is if you are reviewing a historical topic and need to use older sources.
11. What are the types of literature review?
• Argumentative review
• Integrative review
• Methodological review
• Systematic review
• Meta-analysis review
• Historical review
• Theoretical review
• Scoping review
• State-of-the-Art review
12. Is a literature review mandatory?
Yes. Literature review is a mandatory part of any research project. It is a critical step in the process that allows you to establish the scope of your research, and provide a background for the rest of your work.
But before you go,
- Six Online Tools for Easy Literature Review
- Evaluating literature review: systematic vs. scoping reviews
- Systematic Approaches to a Successful Literature Review
- Writing Integrative Literature Reviews: Guidelines and Examples
You might also like

Consensus GPT vs. SciSpace GPT: Choose the Best GPT for Research
Literature Review and Theoretical Framework: Understanding the Differences

Types of Essays in Academic Writing - Quick Guide (2024)
Purdue Online Writing Lab Purdue OWL® College of Liberal Arts
Writing a Literature Review
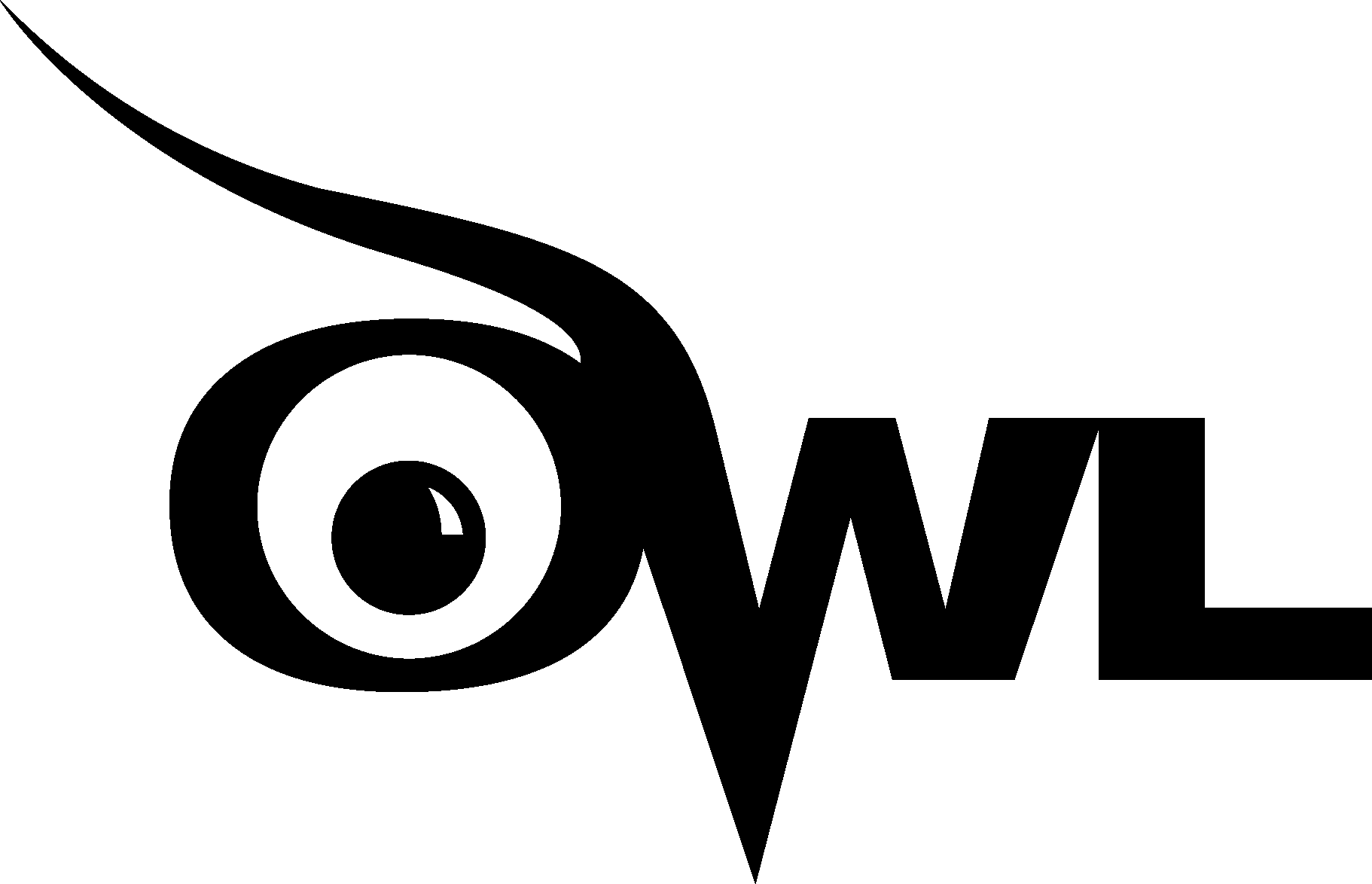
Welcome to the Purdue OWL
This page is brought to you by the OWL at Purdue University. When printing this page, you must include the entire legal notice.
Copyright ©1995-2018 by The Writing Lab & The OWL at Purdue and Purdue University. All rights reserved. This material may not be published, reproduced, broadcast, rewritten, or redistributed without permission. Use of this site constitutes acceptance of our terms and conditions of fair use.
A literature review is a document or section of a document that collects key sources on a topic and discusses those sources in conversation with each other (also called synthesis ). The lit review is an important genre in many disciplines, not just literature (i.e., the study of works of literature such as novels and plays). When we say “literature review” or refer to “the literature,” we are talking about the research ( scholarship ) in a given field. You will often see the terms “the research,” “the scholarship,” and “the literature” used mostly interchangeably.
Where, when, and why would I write a lit review?
There are a number of different situations where you might write a literature review, each with slightly different expectations; different disciplines, too, have field-specific expectations for what a literature review is and does. For instance, in the humanities, authors might include more overt argumentation and interpretation of source material in their literature reviews, whereas in the sciences, authors are more likely to report study designs and results in their literature reviews; these differences reflect these disciplines’ purposes and conventions in scholarship. You should always look at examples from your own discipline and talk to professors or mentors in your field to be sure you understand your discipline’s conventions, for literature reviews as well as for any other genre.
A literature review can be a part of a research paper or scholarly article, usually falling after the introduction and before the research methods sections. In these cases, the lit review just needs to cover scholarship that is important to the issue you are writing about; sometimes it will also cover key sources that informed your research methodology.
Lit reviews can also be standalone pieces, either as assignments in a class or as publications. In a class, a lit review may be assigned to help students familiarize themselves with a topic and with scholarship in their field, get an idea of the other researchers working on the topic they’re interested in, find gaps in existing research in order to propose new projects, and/or develop a theoretical framework and methodology for later research. As a publication, a lit review usually is meant to help make other scholars’ lives easier by collecting and summarizing, synthesizing, and analyzing existing research on a topic. This can be especially helpful for students or scholars getting into a new research area, or for directing an entire community of scholars toward questions that have not yet been answered.
What are the parts of a lit review?
Most lit reviews use a basic introduction-body-conclusion structure; if your lit review is part of a larger paper, the introduction and conclusion pieces may be just a few sentences while you focus most of your attention on the body. If your lit review is a standalone piece, the introduction and conclusion take up more space and give you a place to discuss your goals, research methods, and conclusions separately from where you discuss the literature itself.
Introduction:
- An introductory paragraph that explains what your working topic and thesis is
- A forecast of key topics or texts that will appear in the review
- Potentially, a description of how you found sources and how you analyzed them for inclusion and discussion in the review (more often found in published, standalone literature reviews than in lit review sections in an article or research paper)
- Summarize and synthesize: Give an overview of the main points of each source and combine them into a coherent whole
- Analyze and interpret: Don’t just paraphrase other researchers – add your own interpretations where possible, discussing the significance of findings in relation to the literature as a whole
- Critically Evaluate: Mention the strengths and weaknesses of your sources
- Write in well-structured paragraphs: Use transition words and topic sentence to draw connections, comparisons, and contrasts.
Conclusion:
- Summarize the key findings you have taken from the literature and emphasize their significance
- Connect it back to your primary research question
How should I organize my lit review?
Lit reviews can take many different organizational patterns depending on what you are trying to accomplish with the review. Here are some examples:
- Chronological : The simplest approach is to trace the development of the topic over time, which helps familiarize the audience with the topic (for instance if you are introducing something that is not commonly known in your field). If you choose this strategy, be careful to avoid simply listing and summarizing sources in order. Try to analyze the patterns, turning points, and key debates that have shaped the direction of the field. Give your interpretation of how and why certain developments occurred (as mentioned previously, this may not be appropriate in your discipline — check with a teacher or mentor if you’re unsure).
- Thematic : If you have found some recurring central themes that you will continue working with throughout your piece, you can organize your literature review into subsections that address different aspects of the topic. For example, if you are reviewing literature about women and religion, key themes can include the role of women in churches and the religious attitude towards women.
- Qualitative versus quantitative research
- Empirical versus theoretical scholarship
- Divide the research by sociological, historical, or cultural sources
- Theoretical : In many humanities articles, the literature review is the foundation for the theoretical framework. You can use it to discuss various theories, models, and definitions of key concepts. You can argue for the relevance of a specific theoretical approach or combine various theorical concepts to create a framework for your research.
What are some strategies or tips I can use while writing my lit review?
Any lit review is only as good as the research it discusses; make sure your sources are well-chosen and your research is thorough. Don’t be afraid to do more research if you discover a new thread as you’re writing. More info on the research process is available in our "Conducting Research" resources .
As you’re doing your research, create an annotated bibliography ( see our page on the this type of document ). Much of the information used in an annotated bibliography can be used also in a literature review, so you’ll be not only partially drafting your lit review as you research, but also developing your sense of the larger conversation going on among scholars, professionals, and any other stakeholders in your topic.
Usually you will need to synthesize research rather than just summarizing it. This means drawing connections between sources to create a picture of the scholarly conversation on a topic over time. Many student writers struggle to synthesize because they feel they don’t have anything to add to the scholars they are citing; here are some strategies to help you:
- It often helps to remember that the point of these kinds of syntheses is to show your readers how you understand your research, to help them read the rest of your paper.
- Writing teachers often say synthesis is like hosting a dinner party: imagine all your sources are together in a room, discussing your topic. What are they saying to each other?
- Look at the in-text citations in each paragraph. Are you citing just one source for each paragraph? This usually indicates summary only. When you have multiple sources cited in a paragraph, you are more likely to be synthesizing them (not always, but often
- Read more about synthesis here.
The most interesting literature reviews are often written as arguments (again, as mentioned at the beginning of the page, this is discipline-specific and doesn’t work for all situations). Often, the literature review is where you can establish your research as filling a particular gap or as relevant in a particular way. You have some chance to do this in your introduction in an article, but the literature review section gives a more extended opportunity to establish the conversation in the way you would like your readers to see it. You can choose the intellectual lineage you would like to be part of and whose definitions matter most to your thinking (mostly humanities-specific, but this goes for sciences as well). In addressing these points, you argue for your place in the conversation, which tends to make the lit review more compelling than a simple reporting of other sources.

How To Structure Your Literature Review
3 options to help structure your chapter.
By: Amy Rommelspacher (PhD) | Reviewer: Dr Eunice Rautenbach | November 2020 (Updated May 2023)
Writing the literature review chapter can seem pretty daunting when you’re piecing together your dissertation or thesis. As we’ve discussed before , a good literature review needs to achieve a few very important objectives – it should:
- Demonstrate your knowledge of the research topic
- Identify the gaps in the literature and show how your research links to these
- Provide the foundation for your conceptual framework (if you have one)
- Inform your own methodology and research design
To achieve this, your literature review needs a well-thought-out structure . Get the structure of your literature review chapter wrong and you’ll struggle to achieve these objectives. Don’t worry though – in this post, we’ll look at how to structure your literature review for maximum impact (and marks!).
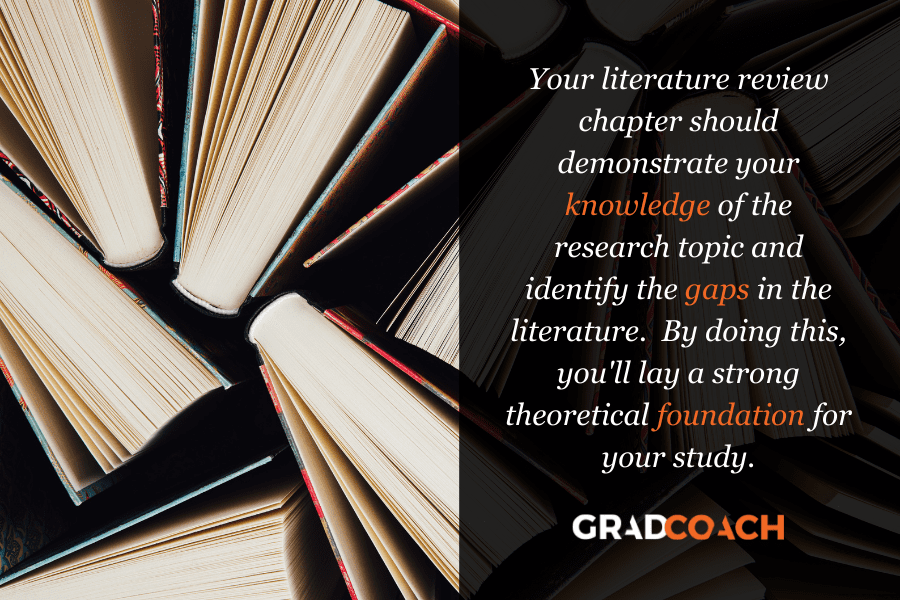
But wait – is this the right time?
Deciding on the structure of your literature review should come towards the end of the literature review process – after you have collected and digested the literature, but before you start writing the chapter.
In other words, you need to first develop a rich understanding of the literature before you even attempt to map out a structure. There’s no use trying to develop a structure before you’ve fully wrapped your head around the existing research.
Equally importantly, you need to have a structure in place before you start writing , or your literature review will most likely end up a rambling, disjointed mess.
Importantly, don’t feel that once you’ve defined a structure you can’t iterate on it. It’s perfectly natural to adjust as you engage in the writing process. As we’ve discussed before , writing is a way of developing your thinking, so it’s quite common for your thinking to change – and therefore, for your chapter structure to change – as you write.
Need a helping hand?
Like any other chapter in your thesis or dissertation, your literature review needs to have a clear, logical structure. At a minimum, it should have three essential components – an introduction , a body and a conclusion .
Let’s take a closer look at each of these.
1: The Introduction Section
Just like any good introduction, the introduction section of your literature review should introduce the purpose and layout (organisation) of the chapter. In other words, your introduction needs to give the reader a taste of what’s to come, and how you’re going to lay that out. Essentially, you should provide the reader with a high-level roadmap of your chapter to give them a taste of the journey that lies ahead.
Here’s an example of the layout visualised in a literature review introduction:
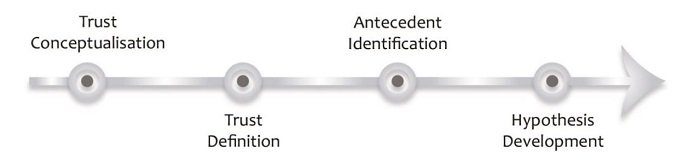
Your introduction should also outline your topic (including any tricky terminology or jargon) and provide an explanation of the scope of your literature review – in other words, what you will and won’t be covering (the delimitations ). This helps ringfence your review and achieve a clear focus . The clearer and narrower your focus, the deeper you can dive into the topic (which is typically where the magic lies).
Depending on the nature of your project, you could also present your stance or point of view at this stage. In other words, after grappling with the literature you’ll have an opinion about what the trends and concerns are in the field as well as what’s lacking. The introduction section can then present these ideas so that it is clear to examiners that you’re aware of how your research connects with existing knowledge .
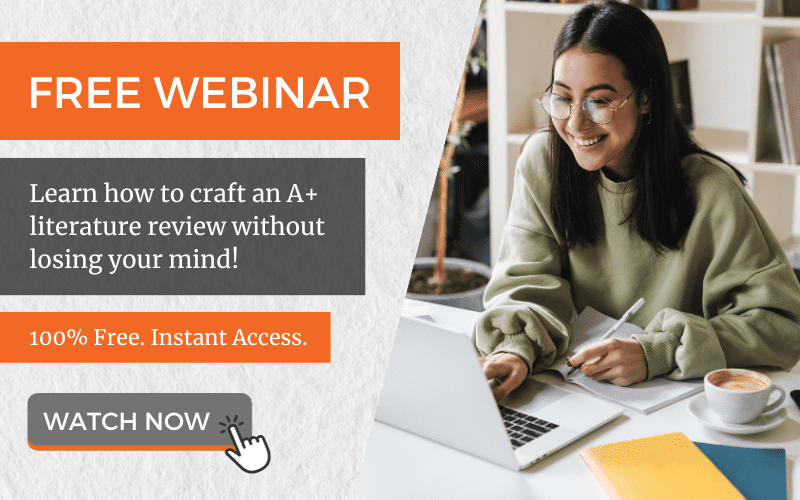
2: The Body Section
The body of your literature review is the centre of your work. This is where you’ll present, analyse, evaluate and synthesise the existing research. In other words, this is where you’re going to earn (or lose) the most marks. Therefore, it’s important to carefully think about how you will organise your discussion to present it in a clear way.
The body of your literature review should do just as the description of this chapter suggests. It should “review” the literature – in other words, identify, analyse, and synthesise it. So, when thinking about structuring your literature review, you need to think about which structural approach will provide the best “review” for your specific type of research and objectives (we’ll get to this shortly).
There are (broadly speaking) three options for organising your literature review.
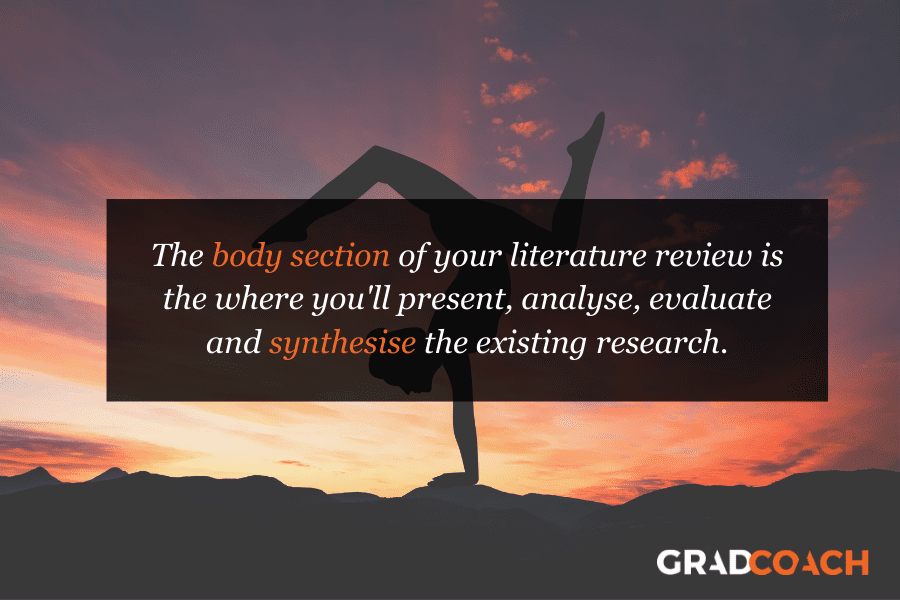
Option 1: Chronological (according to date)
Organising the literature chronologically is one of the simplest ways to structure your literature review. You start with what was published first and work your way through the literature until you reach the work published most recently. Pretty straightforward.
The benefit of this option is that it makes it easy to discuss the developments and debates in the field as they emerged over time. Organising your literature chronologically also allows you to highlight how specific articles or pieces of work might have changed the course of the field – in other words, which research has had the most impact . Therefore, this approach is very useful when your research is aimed at understanding how the topic has unfolded over time and is often used by scholars in the field of history. That said, this approach can be utilised by anyone that wants to explore change over time .
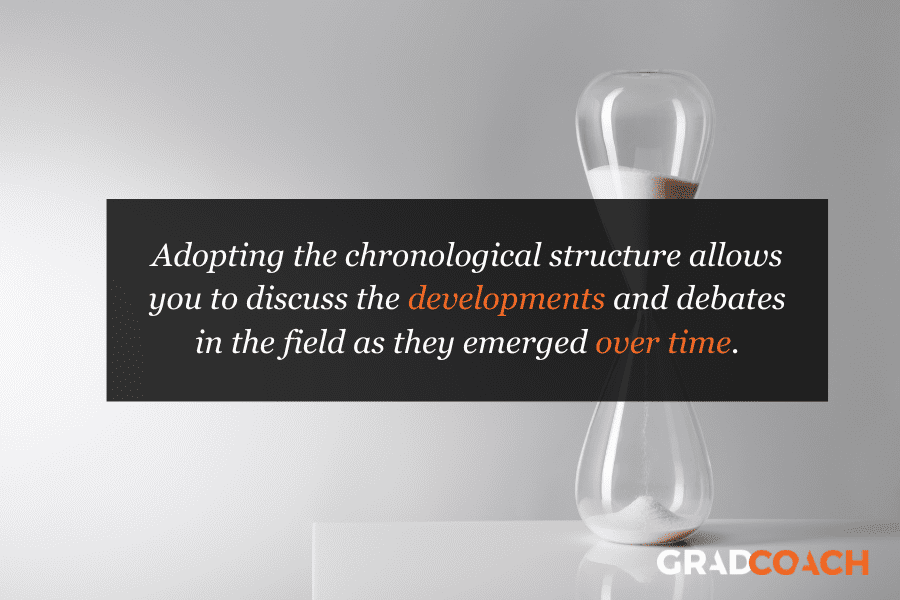
For example , if a student of politics is investigating how the understanding of democracy has evolved over time, they could use the chronological approach to provide a narrative that demonstrates how this understanding has changed through the ages.
Here are some questions you can ask yourself to help you structure your literature review chronologically.
- What is the earliest literature published relating to this topic?
- How has the field changed over time? Why?
- What are the most recent discoveries/theories?
In some ways, chronology plays a part whichever way you decide to structure your literature review, because you will always, to a certain extent, be analysing how the literature has developed. However, with the chronological approach, the emphasis is very firmly on how the discussion has evolved over time , as opposed to how all the literature links together (which we’ll discuss next ).
Option 2: Thematic (grouped by theme)
The thematic approach to structuring a literature review means organising your literature by theme or category – for example, by independent variables (i.e. factors that have an impact on a specific outcome).
As you’ve been collecting and synthesising literature , you’ll likely have started seeing some themes or patterns emerging. You can then use these themes or patterns as a structure for your body discussion. The thematic approach is the most common approach and is useful for structuring literature reviews in most fields.
For example, if you were researching which factors contributed towards people trusting an organisation, you might find themes such as consumers’ perceptions of an organisation’s competence, benevolence and integrity. Structuring your literature review thematically would mean structuring your literature review’s body section to discuss each of these themes, one section at a time.
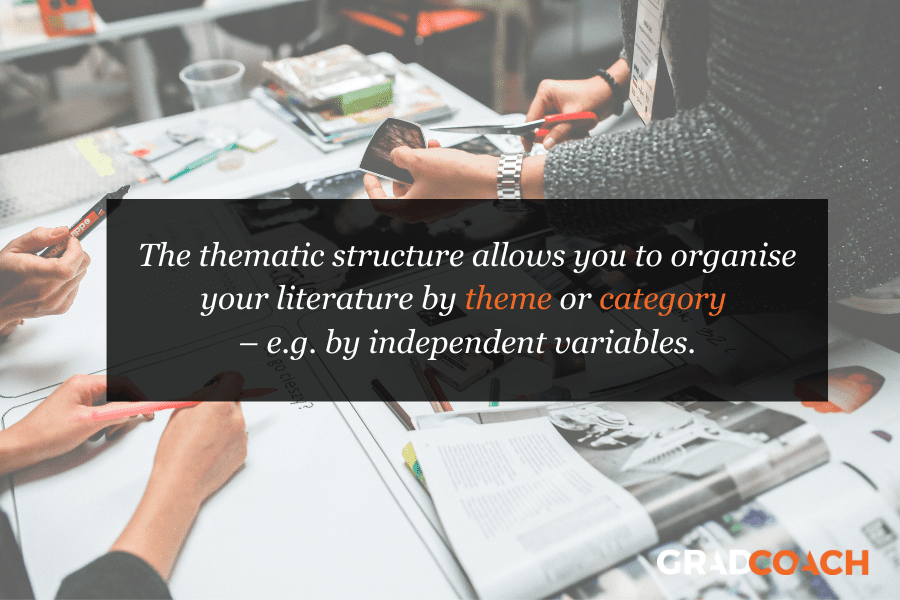
Here are some questions to ask yourself when structuring your literature review by themes:
- Are there any patterns that have come to light in the literature?
- What are the central themes and categories used by the researchers?
- Do I have enough evidence of these themes?
PS – you can see an example of a thematically structured literature review in our literature review sample walkthrough video here.
Option 3: Methodological
The methodological option is a way of structuring your literature review by the research methodologies used . In other words, organising your discussion based on the angle from which each piece of research was approached – for example, qualitative , quantitative or mixed methodologies.
Structuring your literature review by methodology can be useful if you are drawing research from a variety of disciplines and are critiquing different methodologies. The point of this approach is to question how existing research has been conducted, as opposed to what the conclusions and/or findings the research were.
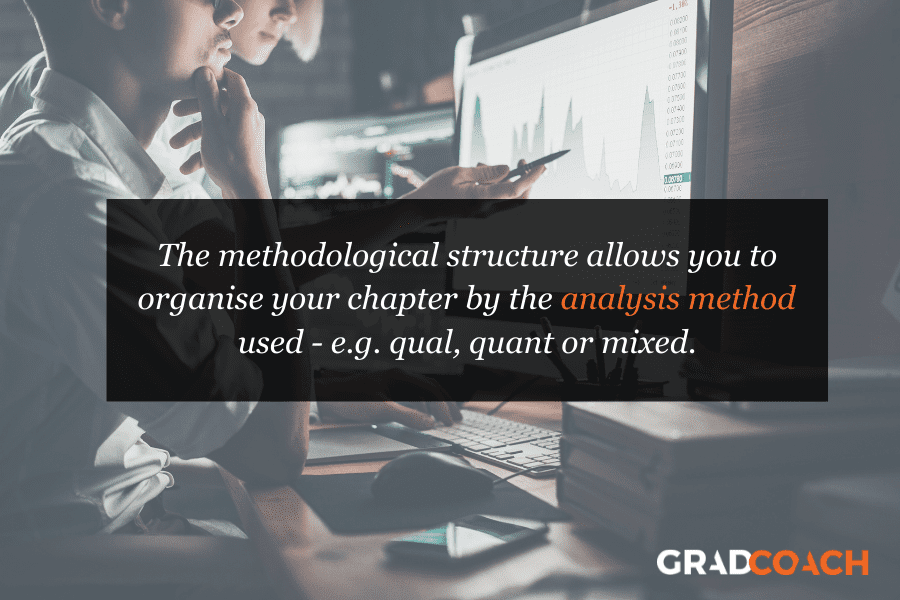
For example, a sociologist might centre their research around critiquing specific fieldwork practices. Their literature review will then be a summary of the fieldwork methodologies used by different studies.
Here are some questions you can ask yourself when structuring your literature review according to methodology:
- Which methodologies have been utilised in this field?
- Which methodology is the most popular (and why)?
- What are the strengths and weaknesses of the various methodologies?
- How can the existing methodologies inform my own methodology?
3: The Conclusion Section
Once you’ve completed the body section of your literature review using one of the structural approaches we discussed above, you’ll need to “wrap up” your literature review and pull all the pieces together to set the direction for the rest of your dissertation or thesis.
The conclusion is where you’ll present the key findings of your literature review. In this section, you should emphasise the research that is especially important to your research questions and highlight the gaps that exist in the literature. Based on this, you need to make it clear what you will add to the literature – in other words, justify your own research by showing how it will help fill one or more of the gaps you just identified.
Last but not least, if it’s your intention to develop a conceptual framework for your dissertation or thesis, the conclusion section is a good place to present this.
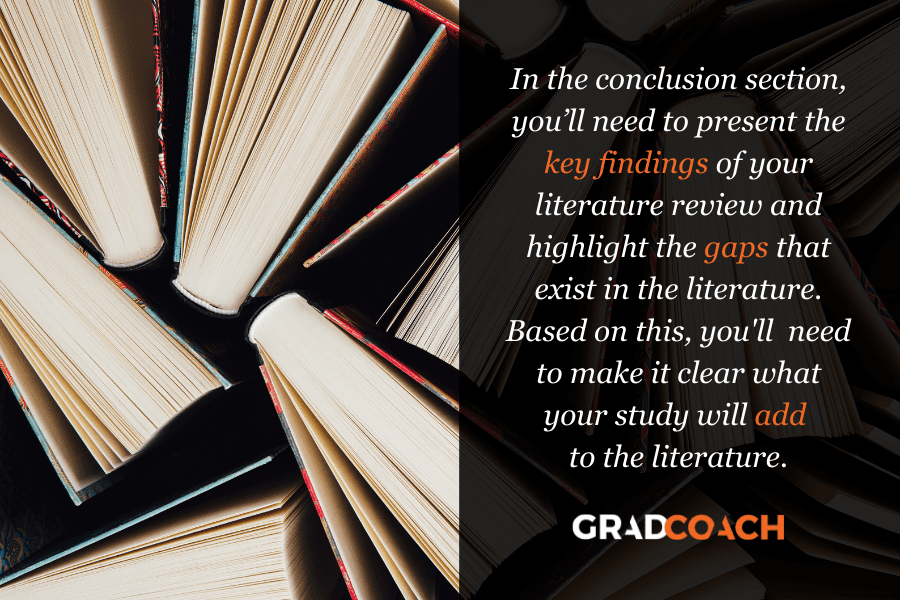
Example: Thematically Structured Review
In the video below, we unpack a literature review chapter so that you can see an example of a thematically structure review in practice.
Let’s Recap
In this article, we’ve discussed how to structure your literature review for maximum impact. Here’s a quick recap of what you need to keep in mind when deciding on your literature review structure:
- Just like other chapters, your literature review needs a clear introduction , body and conclusion .
- The introduction section should provide an overview of what you will discuss in your literature review.
- The body section of your literature review can be organised by chronology , theme or methodology . The right structural approach depends on what you’re trying to achieve with your research.
- The conclusion section should draw together the key findings of your literature review and link them to your research questions.
If you’re ready to get started, be sure to download our free literature review template to fast-track your chapter outline.
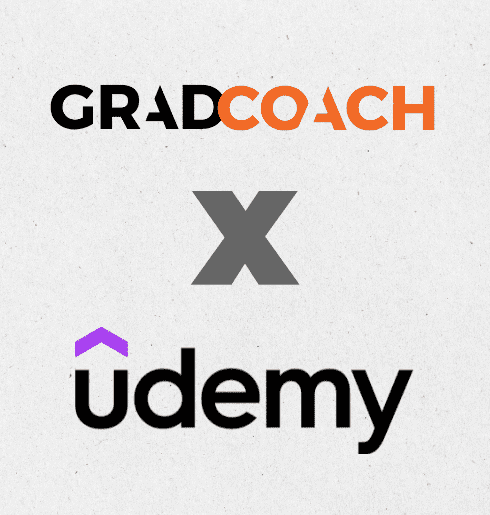
Psst… there’s more!
This post is an extract from our bestselling Udemy Course, Literature Review Bootcamp . If you want to work smart, you don't want to miss this .
You Might Also Like:
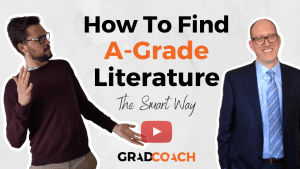
27 Comments
Great work. This is exactly what I was looking for and helps a lot together with your previous post on literature review. One last thing is missing: a link to a great literature chapter of an journal article (maybe with comments of the different sections in this review chapter). Do you know any great literature review chapters?
I agree with you Marin… A great piece
I agree with Marin. This would be quite helpful if you annotate a nicely structured literature from previously published research articles.
Awesome article for my research.
I thank you immensely for this wonderful guide
It is indeed thought and supportive work for the futurist researcher and students
Very educative and good time to get guide. Thank you
Great work, very insightful. Thank you.
Thanks for this wonderful presentation. My question is that do I put all the variables into a single conceptual framework or each hypothesis will have it own conceptual framework?
Thank you very much, very helpful
This is very educative and precise . Thank you very much for dropping this kind of write up .
Pheeww, so damn helpful, thank you for this informative piece.
I’m doing a research project topic ; stool analysis for parasitic worm (enteric) worm, how do I structure it, thanks.
comprehensive explanation. Help us by pasting the URL of some good “literature review” for better understanding.
great piece. thanks for the awesome explanation. it is really worth sharing. I have a little question, if anyone can help me out, which of the options in the body of literature can be best fit if you are writing an architectural thesis that deals with design?
I am doing a research on nanofluids how can l structure it?
Beautifully clear.nThank you!
Lucid! Thankyou!
Brilliant work, well understood, many thanks
I like how this was so clear with simple language 😊😊 thank you so much 😊 for these information 😊
Insightful. I was struggling to come up with a sensible literature review but this has been really helpful. Thank you!
You have given thought-provoking information about the review of the literature.
Thank you. It has made my own research better and to impart your work to students I teach
I learnt a lot from this teaching. It’s a great piece.
I am doing research on EFL teacher motivation for his/her job. How Can I structure it? Is there any detailed template, additional to this?
You are so cool! I do not think I’ve read through something like this before. So nice to find somebody with some genuine thoughts on this issue. Seriously.. thank you for starting this up. This site is one thing that is required on the internet, someone with a little originality!
I’m asked to do conceptual, theoretical and empirical literature, and i just don’t know how to structure it
Submit a Comment Cancel reply
Your email address will not be published. Required fields are marked *
Save my name, email, and website in this browser for the next time I comment.
- Print Friendly
Log in using your username and password
- Search More Search for this keyword Advanced search
- Latest content
- Current issue
- Write for Us
- BMJ Journals More You are viewing from: Google Indexer
You are here
- Volume 24, Issue 2
- Five tips for developing useful literature summary tables for writing review articles
- Article Text
- Article info
- Citation Tools
- Rapid Responses
- Article metrics

- http://orcid.org/0000-0003-0157-5319 Ahtisham Younas 1 , 2 ,
- http://orcid.org/0000-0002-7839-8130 Parveen Ali 3 , 4
- 1 Memorial University of Newfoundland , St John's , Newfoundland , Canada
- 2 Swat College of Nursing , Pakistan
- 3 School of Nursing and Midwifery , University of Sheffield , Sheffield , South Yorkshire , UK
- 4 Sheffield University Interpersonal Violence Research Group , Sheffield University , Sheffield , UK
- Correspondence to Ahtisham Younas, Memorial University of Newfoundland, St John's, NL A1C 5C4, Canada; ay6133{at}mun.ca
https://doi.org/10.1136/ebnurs-2021-103417
Statistics from Altmetric.com
Request permissions.
If you wish to reuse any or all of this article please use the link below which will take you to the Copyright Clearance Center’s RightsLink service. You will be able to get a quick price and instant permission to reuse the content in many different ways.
Introduction
Literature reviews offer a critical synthesis of empirical and theoretical literature to assess the strength of evidence, develop guidelines for practice and policymaking, and identify areas for future research. 1 It is often essential and usually the first task in any research endeavour, particularly in masters or doctoral level education. For effective data extraction and rigorous synthesis in reviews, the use of literature summary tables is of utmost importance. A literature summary table provides a synopsis of an included article. It succinctly presents its purpose, methods, findings and other relevant information pertinent to the review. The aim of developing these literature summary tables is to provide the reader with the information at one glance. Since there are multiple types of reviews (eg, systematic, integrative, scoping, critical and mixed methods) with distinct purposes and techniques, 2 there could be various approaches for developing literature summary tables making it a complex task specialty for the novice researchers or reviewers. Here, we offer five tips for authors of the review articles, relevant to all types of reviews, for creating useful and relevant literature summary tables. We also provide examples from our published reviews to illustrate how useful literature summary tables can be developed and what sort of information should be provided.
Tip 1: provide detailed information about frameworks and methods
- Download figure
- Open in new tab
- Download powerpoint
Tabular literature summaries from a scoping review. Source: Rasheed et al . 3
The provision of information about conceptual and theoretical frameworks and methods is useful for several reasons. First, in quantitative (reviews synthesising the results of quantitative studies) and mixed reviews (reviews synthesising the results of both qualitative and quantitative studies to address a mixed review question), it allows the readers to assess the congruence of the core findings and methods with the adapted framework and tested assumptions. In qualitative reviews (reviews synthesising results of qualitative studies), this information is beneficial for readers to recognise the underlying philosophical and paradigmatic stance of the authors of the included articles. For example, imagine the authors of an article, included in a review, used phenomenological inquiry for their research. In that case, the review authors and the readers of the review need to know what kind of (transcendental or hermeneutic) philosophical stance guided the inquiry. Review authors should, therefore, include the philosophical stance in their literature summary for the particular article. Second, information about frameworks and methods enables review authors and readers to judge the quality of the research, which allows for discerning the strengths and limitations of the article. For example, if authors of an included article intended to develop a new scale and test its psychometric properties. To achieve this aim, they used a convenience sample of 150 participants and performed exploratory (EFA) and confirmatory factor analysis (CFA) on the same sample. Such an approach would indicate a flawed methodology because EFA and CFA should not be conducted on the same sample. The review authors must include this information in their summary table. Omitting this information from a summary could lead to the inclusion of a flawed article in the review, thereby jeopardising the review’s rigour.
Tip 2: include strengths and limitations for each article
Critical appraisal of individual articles included in a review is crucial for increasing the rigour of the review. Despite using various templates for critical appraisal, authors often do not provide detailed information about each reviewed article’s strengths and limitations. Merely noting the quality score based on standardised critical appraisal templates is not adequate because the readers should be able to identify the reasons for assigning a weak or moderate rating. Many recent critical appraisal checklists (eg, Mixed Methods Appraisal Tool) discourage review authors from assigning a quality score and recommend noting the main strengths and limitations of included studies. It is also vital that methodological and conceptual limitations and strengths of the articles included in the review are provided because not all review articles include empirical research papers. Rather some review synthesises the theoretical aspects of articles. Providing information about conceptual limitations is also important for readers to judge the quality of foundations of the research. For example, if you included a mixed-methods study in the review, reporting the methodological and conceptual limitations about ‘integration’ is critical for evaluating the study’s strength. Suppose the authors only collected qualitative and quantitative data and did not state the intent and timing of integration. In that case, the strength of the study is weak. Integration only occurred at the levels of data collection. However, integration may not have occurred at the analysis, interpretation and reporting levels.
Tip 3: write conceptual contribution of each reviewed article
While reading and evaluating review papers, we have observed that many review authors only provide core results of the article included in a review and do not explain the conceptual contribution offered by the included article. We refer to conceptual contribution as a description of how the article’s key results contribute towards the development of potential codes, themes or subthemes, or emerging patterns that are reported as the review findings. For example, the authors of a review article noted that one of the research articles included in their review demonstrated the usefulness of case studies and reflective logs as strategies for fostering compassion in nursing students. The conceptual contribution of this research article could be that experiential learning is one way to teach compassion to nursing students, as supported by case studies and reflective logs. This conceptual contribution of the article should be mentioned in the literature summary table. Delineating each reviewed article’s conceptual contribution is particularly beneficial in qualitative reviews, mixed-methods reviews, and critical reviews that often focus on developing models and describing or explaining various phenomena. Figure 2 offers an example of a literature summary table. 4
Tabular literature summaries from a critical review. Source: Younas and Maddigan. 4
Tip 4: compose potential themes from each article during summary writing
While developing literature summary tables, many authors use themes or subthemes reported in the given articles as the key results of their own review. Such an approach prevents the review authors from understanding the article’s conceptual contribution, developing rigorous synthesis and drawing reasonable interpretations of results from an individual article. Ultimately, it affects the generation of novel review findings. For example, one of the articles about women’s healthcare-seeking behaviours in developing countries reported a theme ‘social-cultural determinants of health as precursors of delays’. Instead of using this theme as one of the review findings, the reviewers should read and interpret beyond the given description in an article, compare and contrast themes, findings from one article with findings and themes from another article to find similarities and differences and to understand and explain bigger picture for their readers. Therefore, while developing literature summary tables, think twice before using the predeveloped themes. Including your themes in the summary tables (see figure 1 ) demonstrates to the readers that a robust method of data extraction and synthesis has been followed.
Tip 5: create your personalised template for literature summaries
Often templates are available for data extraction and development of literature summary tables. The available templates may be in the form of a table, chart or a structured framework that extracts some essential information about every article. The commonly used information may include authors, purpose, methods, key results and quality scores. While extracting all relevant information is important, such templates should be tailored to meet the needs of the individuals’ review. For example, for a review about the effectiveness of healthcare interventions, a literature summary table must include information about the intervention, its type, content timing, duration, setting, effectiveness, negative consequences, and receivers and implementers’ experiences of its usage. Similarly, literature summary tables for articles included in a meta-synthesis must include information about the participants’ characteristics, research context and conceptual contribution of each reviewed article so as to help the reader make an informed decision about the usefulness or lack of usefulness of the individual article in the review and the whole review.
In conclusion, narrative or systematic reviews are almost always conducted as a part of any educational project (thesis or dissertation) or academic or clinical research. Literature reviews are the foundation of research on a given topic. Robust and high-quality reviews play an instrumental role in guiding research, practice and policymaking. However, the quality of reviews is also contingent on rigorous data extraction and synthesis, which require developing literature summaries. We have outlined five tips that could enhance the quality of the data extraction and synthesis process by developing useful literature summaries.
- Aromataris E ,
- Rasheed SP ,
Twitter @Ahtisham04, @parveenazamali
Funding The authors have not declared a specific grant for this research from any funding agency in the public, commercial or not-for-profit sectors.
Competing interests None declared.
Patient consent for publication Not required.
Provenance and peer review Not commissioned; externally peer reviewed.
Read the full text or download the PDF:
Thank you for visiting nature.com. You are using a browser version with limited support for CSS. To obtain the best experience, we recommend you use a more up to date browser (or turn off compatibility mode in Internet Explorer). In the meantime, to ensure continued support, we are displaying the site without styles and JavaScript.
- View all journals
- My Account Login
- Explore content
- About the journal
- Publish with us
- Sign up for alerts
- Open access
- Published: 12 April 2024
Determinants of multimodal fake review generation in China’s E-commerce platforms
- Chunnian Liu ORCID: orcid.org/0000-0002-8764-134X 1 , 2 ,
- Xutao He ORCID: orcid.org/0009-0003-3533-9573 1 , 2 &
- Lan Yi ORCID: orcid.org/0000-0003-1570-5516 1 , 2
Scientific Reports volume 14 , Article number: 8524 ( 2024 ) Cite this article
Metrics details
- Information technology
This paper develops a theoretical model of determinants influencing multimodal fake review generation using the theories of signaling, actor-network, motivation, and human–environment interaction hypothesis. Applying survey data from users of China’s three leading E-commerce platforms (Taobao, Jingdong, and Pinduoduo), we adopt structural equation modeling, machine learning technique, and Bayesian complex networks analysis to perform factor identification, path analysis, feature factor importance ranking, regime division, and network centrality analysis of full sample, male sample, and female sample to reach the following conclusions: (1) platforms’ multimodal recognition and governance capabilities exert significant negative moderating effects on merchants’ information behavior, while it shows no apparent moderating effect on users’ information behavior; users’ emotional venting, perceived value, reward mechanisms, and subjective norms positively influence multimodal fake review generation through perceptual behavior control; (2) feature factors of multimodal fake review generation can be divided into four regimes, i.e., regime 1 includes reward mechanisms and perceived social costs, indicating they are key feature factors of multimodal fake review generation; merchant perception impact is positioned in regime 2, signifying its pivotal role in multimodal fake review generation; regime 3 includes multimodal recognition and governance capabilities, supporting/disparaging merchants, and emotional venting; whereas user perception impact is positioned in regime 4, indicating its weaker influence on multimodal fake review generation; (3) both in full sample, male sample, and female sample, reward mechanisms play a crucial role in multimodal fake review generation; perceived value, hiring review control agency, multimodal recognition and governance capabilities exhibit a high degree of correlation; however, results of network centrality analysis also exhibit heterogeneity between male and female samples, i.e., male sample has different trends in closeness centrality values and betweenness centrality values than female sample. This indicates that determinants influencing multimodal fake review generation are complex and interconnected.
Similar content being viewed by others
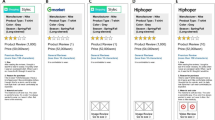
Factors that affect consumer trust in product quality: a focus on online reviews and shopping platforms
Eunsuk Sung, Won Young Chung & Daeho Lee
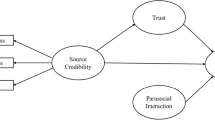
Exploring the impact of beauty vloggers’ credible attributes, parasocial interaction, and trust on consumer purchase intention in influencer marketing
Mukta Garg & Apurva Bakshi
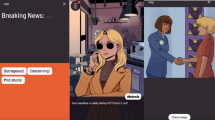
How to “inoculate” against multimodal misinformation: A conceptual replication of Roozenbeek and van der Linden (2020)
Julian Neylan, Mikey Biddlestone, … Sander van der Linden
Introduction
The rapid development of digital economy and internet has led to a growing preference for online shopping among consumers. Given virtual and uncertain nature of online shopping, consumers are increasingly relying on online reviews from early purchasers to assess product quality and merchant reputation. According to China Online Shopping Behavior Research Report (2016) published by KPMG, nearly two-thirds of consumers check others’ comments and suggestions on products when shopping online, particularly when purchasing new products 1 . Moreover, positive feedback and comments from previous consumers who have already made purchases are primary determinants influencing purchase decisions. A survey conducted by Pew Research Centre in 2016 finds that 82% of Americans would review comments before making their first online purchase 2 . Furthermore, a 2012 Nielsen survey of over 28,000 online users in 56 countries reveals that online consumer reviews are the second most trusted source of brand information, following recommendations from friends and family 3 . China is a prominent player in field of e-commerce. Taobao, Jingdong, and Pinduoduo are widely recognized as leading E-commerce platforms operating in China. Given increasing impact of online reviews on consumers’ buying decisions, shopping platforms like Taobao, Jingdong, and Pinduoduo have recognized significance of consumer reviews. Consequently, they have resorted to manipulating online reputation by adopting tactics such as posting fake reviews, thus enhancing shop rankings and brand reputation 4 . This tactic involves improving quality and quantity of reviews in an attempt to influence purchasing decisions of potential consumers, thereby resulting in a proliferation of fake reviews in e-commerce market 5 . Additionally, a study conducted by ReviewMeta finds that 16% of hotel reviews on Yelp’s website are identified as fake 6 . According to Fakespot, a fake review detection site, actual percentage of fake reviews on Amazon is estimated to be around 30% 7 . Therefore, a large number of fake reviews on e-commerce platforms may seriously affect people’s daily shopping experience.
After reviewing relative literature, we find that existing research on online fake reviews including identification of review content 8 , 9 , 10 , motivations behind generating fake reviews 11 , 12 , and impacts of fake reviews 13 , 14 , 15 . However, Some scholars note that current research on online fake reviews remains inadequate 16 , 17 , 18 . Significant gaps persist within current literature: (1) considerable attention is devoted to characterizing and detecting fake reviews, but research on underlying causal mechanisms driving fake review generation remains limited. Existing studies acknowledge influence of merchant agents, user agents, and platform agents 19 , 20 , but how these agents affect intrinsic mechanisms of fake review generation remains unclear; (2) prior research lacks quantitative assessments of relative importance of determinants in generating fake reviews, and fails to identify key determinants influencing fake review generation, there is also less research dividing determinants influencing fake review generation into distinct regimes. Although studies suggest that users’ purchasing preferences and merchants’ marketing strategies are determinants influencing fake review generation 21 , 22 , analysis of individual importance of each determinant is absent; (3) lack of research quantitatively analyzing intrinsic interrelationships among determinants of fake review generation, and oversight of interactions between these determinants. Furthermore, different influences of gender on fake review generation remain overlooked. Prior research does not explore intrinsic correlations between different motivations (determinants) of each agent, as well as heterogeneity of male and female samples within an online review context, despite evident presence of interactions among these determinants 23 , 24 and different influences of gender on human behavior 25 , 26 , 27 .
In summary, it is necessary to further explore underlying causal mechanisms behind online multimodal fake review generation. Therefore, primary research question in this study is: how to identify causal mechanisms behind online multimodal fake review generation? This primary question encompasses three key dimensions: (1) how to find determinants that influence online multimodal fake review generation from points of platform agent, user agent, and merchant agent? (2) what are key determinants that influence online multimodal fake review generation? (3) what are inherent interconnections among determinants that influence online multimodal fake review generation and is there heterogeneity of male and female samples?
To address these questions, this study aims to explore causal mechanisms behind online multimodal fake review generation, especially: (1) to identify determinants that affect online multimodal fake review generation when platform agent, user agent, and merchant agent coexist; (2) to find key feature factor of multimodal fake review generation; (3) to explore inherent correlations among determinants that influence online multimodal fake review generation in full sample, and analyze heterogeneity of male and female samples.
In addition, our research demonstrates originality through three aspects: (1) originality lies in research question. Existing research explores determinants of fake reviewgeneration 28 , 29 , yet quantification of relative importance of these determinants and inherent interrelations among these determinants remains unclear. Therefore, this study focuses on finding causal mechanisms of online multimodal fake review generation. We explore identification of determinants influencing fake review generation and relative importance of each determinant. Moreover, we analyze inherent correlations between these determinants, as well as heterogeneity of male and female samples; (2) originality extends to empirical method adopted. Most of existing literature uses singular methods to conduct relative research, such as structural equation modeling 30 . This study utilizes three leading e-commerce platforms in China, namely Taobao, Jingdong, and Pinduoduo, as primary data sources. Necessary empirical data is acquired through establishment and dissemination of rigorously designed scientific questionnaires. Moreover, we investigate determinants influencing online multimodal fake review generation. By utilizing structural equation modeling, we quantify influence of these determinants on online multimodal fake review generation. Additionally, we employ machine learning technique to assess relative importance of these determinants. Furthermore, we employ Bayesian complex networks analysis to explore inherent correlations among these determinants, as well as heterogeneity of male and female samples; (3) originality lies in theoretical framework constructed. Most of existing literature employs a singular theoretical framework to analyze influence of individual interactions involving platform agents, merchant agents, and user agents on fake review generation 31 , neglecting a multifaceted theoretical approach that accounts for influence of mass interactions among different agents on fake review generation. Consequently, our study establishes a theoretical framework for online multimodal fake signal generation by integrating theories of signaling, actor-network, motivation, and human–environment interaction hypothesis. We construct a mechanistic model of online multimodal fake review generation including platform agent, user agent, and merchant agent. In addition, through employing structural equation modeling, machine learning technique, and Bayesian complex networks analysis, our findings highlight significant influence of reward mechanisms and perceived social costs. Furthermore, intrinsic correlations of these determinants, and heterogeneity of male and female samples are observed.
Compared to existing literature, this study presents an innovative approach by incorporating the theories of signaling, actor-network, motivation, and human–environment interaction hypothesis as the foundational basis. We introduce platform agent to develop an original model of online multimodal fake review generation mechanism that encompasses interactions among individuals and masses, including platform agents, merchant agents, and user agents. Our research model serves as a valuable complement to existing research models on fake review generation. Theoretical contributions of this study include three aspects: (1) prior studies primarily focus on single theories such as signaling theory and motivation theory to investigate fake review generation 32 , 33 , our research expands application scenarios of these four theories. By integrating the theories of signaling, actor-network, motivation, and human–environment interaction hypothesis, we construct a model for online multimodal fake review generation. Furthermore, we analyze impacts of different agents on online multimodal fake review generation. (2) existing literature on fake review generation considers influence of interactions between merchants and users on fake review generation 34 , our study broadens the scope by incorporating information interaction between individuals and mass of platforms, users, and merchants. By introducing platform agents, we enrich application of information interaction theory in study of fake reviews, and expand research scenario of fake reviews. (3) most scholars employ structural equation modeling and other individual methods to explore fake review generation 35 , 36 , our research integrates structural equation modeling, machine learning technique, and Bayesian complex networks analysis to explore online multimodal fake review generation mechanisms. We uncover a black box of intrinsic causal mechanisms involved in online multimodal fake review generation. We identify key determinants influencing online multimodal fake review generation and find intrinsic correlations among these determinants, as well as heterogeneity of male and female samples. Such findings contribute to a deeper understanding of online multimodal fake review generation.
Literature review
A search of literature reveals that current research by scholars can be broadly classified into three categories.
Determinants of fake review generation
According to previous literature, main determinants of online fake review generation can be divided into two aspects: external factors from merchants 37 , 38 and internal factors from consumers 39 , 40 . External factors from merchants are primary driving factors of fake review generation. Fake reviews caused by external factors refer mainly to consumers making unintended comments to get rewards from merchants, as well as merchants hiring “water armies” to generate fake reviews. Nobahary et al. 41 classify motives for posting fake reviews of online products as sales, denigration, interference, and meaninglessness. Kolhar et al. 42 suggest that motives for posting fake reviews include merchant sales promotion, merchant rewards, consumer emotional outbursts, and malicious competition among merchants. In the field of consumer behavior, shukla et al. 43 highlight that positive fake reviews from competitors, along with comparative advantage, serve as significant reasons for merchants to actively generate similar fake reviews. Furthermore, newly registered merchants resort to review manipulation as an initial strategy aimed at enhancing reputation. Anderson et al. 44 note that suspected deceptive reviews are often published by real customers for non-profit purposes, such as spontaneous brand maintenance. Consumers generate fake reviews to fulfil psychological needs, such as providing help to others, enhancing social status, and communicating with others 45 . Rodríguez-Ferrándiz et al. 46 propose that customers’ motivations for generating online fake reviews can be influenced by many factors, such as rewards, self-esteem or sense of control, and emotion venting. Wang et al. 47 suggest that consumers may generate fake reviews due to utilitarian and hedonistic attitudes towards online review platforms. Furthermore, for firms, receiving positive reviews can boost profits and enhance reputation, giving firms strong reasons to manipulate reviews. Consumers seek financial compensation by generating negative reviews. George et al. 48 conduct an analysis of motives behind fake reviews, identifying two aspects: one involves generating positive reviews through enhancing online influence, and the other involves hiring “water armies” to generate negative reviews.
In the field of marketing, Bianchi et al. 49 reveal that consumers’ motivation to generate fake reviews stemmed from a desire for social benefits, financial rewards, consideration for others, and personal enhancement. Harrison-Walker et al. 50 explore impact of incentives on consumers’ electronic word-of-mouth behavior and determine that hiring reviewers is primary factor behind fake review proliferation. Additionally, Akhtar et al. 51 conclude that consumers engaged in generating fake reviews due to their need for social interactions, financial incentives, and self-worth reinforcement. Moon et al. 52 identify a sense of belonging and satisfaction derived from helping others as primary motives driving fake reviews generation. Zhang et al. 53 argue that fake reviews serve three purposes: generating publicity, offsetting poor quality with positive reviews, and disparagement. Khan et al. 54 suggest that merchants generate fake reviews to get illegal interests, encourage purchase of poor-quality products, or prevent consumers from buying high-quality products. Mohawesh et al. 55 categorize fake reviews based on motives such as promoting sales or seeking revenge against competitors at merchant level, and expressing dissatisfaction or seeking rewards at consumer level. Rasappan et al. 56 suggest that presence of fake characteristics in product reviews can be attributed to various factors, including adoption of a “positive review” strategy. Additionally, implementation of a “bad review threat” strategy drives users to remove negative reviews. Moreover, review mechanisms on Taobao such as restrictions on reviewing returned products, contribute to deviation of product reviews from reality. Su et al. 57 propose that association between fake reviews and product quality can help to distinguish underlying motivations behind fake reviews.
Fake review identification
Research on identifying fake reviews primarily focuses on fake review generator identification 58 , 59 and fake review content identification 60 , 61 . Mewada et al. 62 propose a method to identify fake reviews publishers by differentiating emotional content of their reviews from that of genuine reviewers. Xu et al. 63 develop an evidence theory model based on fusion of user behavioral evidence, achieving an 87% accuracy rate in identifying fake reviewers. Srisaila et al. 64 propose a novel framework for PU learning, aimed at detecting fake reviews, and experimental results substantiate effectiveness of proposed method in detecting fake reviews. Cheng et al. 65 propose a method that integrates lexical and syntactic features for identifying fake reviews, this method achieves satisfactory recognition results. Wang et al. 66 introduce a technique based on a topic-opposite sentiment dependency model to detect fake reviews. Abrar et al. 67 employ various machine learning algorithms to identify fake reviews. Hajek et al. 68 construct a multi-domain golden dataset encompassing reviews from hotels, restaurants, and other domains. Duma et al. 69 define and extract context-independent grammar rule features from reviews, employing SVM classifiers to detect fake reviews. Vidanagama et al. 70 employ rules to filter spam reviews based on inherent characteristics of blog platforms and employ LDA to extract topics from blogs to identify spam reviews within blogosphere.
When it comes to identifying fake reviews through review content, Zhou et al. 71 design an online deception recognition system that incorporates different deception linguistic cues and features. Zhai et al. 72 generate psycholinguistic features of online merchandise reviews and combined them with textual features, using a support vector machine classifier for automatic classification of deceptive online reviews. Deshai et al. 73 propose an expert identification method for processing negative word-of-mouth online. Martinez-Torres et al. 74 use a portrait alignment compatibility model to identify fake hotel reviews by identifying clustering anomalies in reviews. Qu et al. 75 propose a Bayesian identification model to detect fake reviews. Bathla et al. 76 empirically modelled behavior of fake reviews by considering users’ rating behavior. By identifying origins of fake reviews to detect fake reviews. Fang et al. 77 conduct research involving 1,470 reviews from Amazon website. By employing a single- index selection method and a five-index integration selection method to identify spam reviews. Kaliyar et al. 78 focus on defining both intrinsic features of reviews and correlation features among reviewers based on review behavior. By employing an improved clustering algorithm to group review data and subsequently calculating deviation degree of each cluster from overall review dataset. Additionally, by utilizing clustering method, fake reviews can be identified. Ben Jabeur et al. 79 reveal that fake reviews exhibit remarkable consistency in structure, suggesting a template-driven generation process. Building upon this work, Plotkina et al. 80 introduce emotional features into analysis of reviews and employ a polygraph model to detect fake reviews. Le et al. 81 expand on prior research and develop a sophisticated multidimensional time series model for detecting fake reviews, further advancing field of review authenticity assessment.
Impact of fake reviews
Fake reviews of online products can significantly impact consumers’ willingness to purchase products. Song et al. 82 find that maliciously negative reviews have significant effects on consumers’ perceived trust and purchase intention. Duan et al. 83 demonstrate that online merchandise reviews have limited persuasive effects on movie consumers, but significant cognitive effects. Berger et al. 84 investigate effects of negative reviews on product sales, noting that these effects vary between well-known and unknown brands. Costa et al. 85 highlight potential impact of fake reviews on shaping consumer beliefs and attitudes during early and mainstream stages of product adoption. Hakami et al. 86 emphasize detrimental effects of fake reviews on review credibility, helpfulness, and overall value. Tufail et al. 87 point out that fake reviews can lead to feelings of dissatisfaction, deception, and distrust among consumers, influencing negative word-of-mouth and repeat purchase intentions. Paul et al. 88 conclude that manipulated online reviews have less influence on consumer purchase decisions and firm revenues when compared to authentic reviews. Furthermore, Ansari et al. 89 note that fake reviews may temporarily boost a firm’s visibility, increasing average ratings and review numbers. Wu et al. 90 argue that engagement in fake reviews beyond critical threshold could lead to decreased product sales and financial gains. Barbado et al. 91 highlight adverse effects of fake reviews on platforms, impacting consumer evaluations and trust. Wang et al. 92 demonstrate that fake reviews exhibit high emotional polarity and readability, suggesting that consumers can be influenced by writing style of reviews, thereby influencing decision-making. Di et al. 93 show that fake reviews heighten consumer uncertainty, diminish trust, and impact decision-making processes. Yuan et al. 94 find that consumers are less affected by fake positive reviews. Koukova et al. 95 conduct a study revealing that fake reviews increase buyer time costs and diminish trust in both reviews and respective stores. Additionally, in a scenario simulation experiment, Shen et al. 96 discover that presence of bad reviews exerts a notable influence on consumers’ perceived trust and purchase intention. Petratos et al. 97 demonstrate that online product reviews possess limited persuasive effects on movie consumers, and online product reviews do significantly impact consumers’ cognitive evaluation. Mardumyan et al. 98 conduct a setup scenario experiment to investigate effects of negative reviews on product sales and reveal that impact of negative reviews vary depending on brand’s level of recognition, as well as specific product being evaluated.
As can be seen from above, current research on fake reviews is still limited, with more focus on determinants, identification, and impact of fake reviews, while little attention is paid to inherent causal mechanisms of fake review generation. In particular, there is a lack of: (1) attention to determinants influencing online fake review generation in coexistence of platforms, merchants, and users; (2) quantitative analysis of relative importance of determinants affecting online fake review generation; (3) quantitative research on intrinsic relationships between determinants affecting online fake review generation, as well as impact of different gender groups on online fake review generation. Therefore, by referring to scholars’ research on fake reviews, based on the theories of signaling, actor-network, motivation, and human–environment interaction hypothesis, we develop an online multimodal fake review generation model, our model encompasses platforms, users, and merchants. Additionally, we design questionnaires that corresponded to variables in model to collect data. Questionnaires are distributed electronically to users of China’s three leading E-commerce platforms (Taobao, Jingdong, and Pinduoduo). Utilizing structural equation modeling, we investigate determinants influencing online multimodal fake review generation and their impact pathways. Furthermore, we employ machine learning technique to quantify importance of feature factors and divide them into distinct regimes. Moreover, by using Bayesian complex networks analysis, we examine intrinsic correlations of these feature factors in full sample and analyze heterogeneity of male and female samples.
Theoretical framework
We build our theoretical framework on information science theories including the theories of signaling, actor-network, motivation, and human–environment interaction hypothesis.
Signaling theory comprises three crucial components: sender, signal, and receiver 99 . Scholars have utilized signaling theory to investigate impact of online reviews as product signals on consumers’ purchasing choices. For instance, Wang et al. 100 examine influence of review potency and quantity on consumers’ purchasing decisions. Another study conducted by Chen et al. 101 explores effects of review potency and quantity on product sales. Furthermore, Liao et al. 102 investigate impact of review potency on video game sales. Additionally, some research divides signals into product and seller signals 103 , while other research further subdivides signals into individuals, products, and organizations 104 . Based on signal agents emitting them, some scholars adopt a broad classification scheme differentiating between internal and external signals 105 , 106 . Moreover, based on signaling theory, some scholars employ agent simulation 107 and computational experiments 108 to simulate impact of e-commerce platform signals on dynamics of consumer and merchant review behavior. Therefore, this paper aims to explore impact of merchant agent behavioral signals, user agent behavioral signals, and platform agent signal governance on online multimodal fake review generation by utilizing signaling theory.
Actor-network theory (ANT) is not only a theoretical framework but also a research methodology, and ANT focuses on heterogeneous networks 109 . ANT emphasizes importance of heterogeneous networks in understanding social phenomena. When applying this theory, major work is to explain actor interactions within heterogeneous networks in specific contexts 110 . Versatility of actor-network theory allows for its application to nearly any context, offering a unique perspective and methodology to study constantly evolving and changing information activities. ANT is a social science research method that employs a network system model to elucidate behavioral relationships among actors. In understanding phenomenon of fake review generation, ANT offers a suitable framework and valuable perspective. Therefore, this paper examines influence of signal governance by platform actors, as well as signal-generating behaviors of users and merchants, on online multimodal fake review generation from a comprehensive perspective.
As an internal driving force, motivation plays a significant role in shaping individual behavioral intentions. Self-determination theory (SDT) posits that both extrinsic and intrinsic motivations can influence individual behavior 111 . External determinants or demands, such as goal orientation, value perception, and material rewards may trigger extrinsic motivation 112 . Intrinsic motivation stems from internal needs, it includes three fundamental psychological needs: autonomy, competence, and relatedness 113 . Although two motivations are different, they interact with each other in specific contexts. Garnefeld et al. 114 conduct a study to examine influence of incentives on consumers’ electronic word-of-mouth (eWOM) communication behavior. They find that hiring reviewers is primary motivator for encouraging eWOM. Wu 115 investigates determinants that motivate consumers to provide additional reviews on online platforms and proposes a new framework to explain motivation behind consumers’ additional review behavior. Therefore, this paper highlights significance of users’ and merchants’ motivation signals in generating multimodal fake reviews.
Interaction theory 116 posits that individual behavior is determined by interaction between information environment stimulus and individual intrinsic traits. An individual’s behavior is outcome of a continuous interplay between an individual’s intrinsic traits and information environment stimuli they encounter. There exists a profound intrinsic association between individual and information environment stimuli 117 . Interplay between intrinsic traits of platforms, users, merchants and online environments determines online multimodal fake review generation. Consequently, this paper examines impact of information interactions between behavioral signals of user and merchant agents, as well as signal governance of platform agents on online multimodal fake review generation.
Therefore, based on the theories of signaling, actor-network, motivation and human–environment interaction, Fig. 1 presents our conceptual framework of multi-agent fake signal generation.
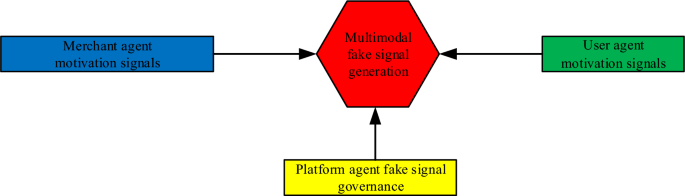
Conceptual framework of multi-agent fake signal generation. Note : red represents multimodal fake signal generation, blue represents merchant agent motivation signals, green represents user agent motivation signals, and yellow represents platform agent fake signal governance.
Based on conceptual framework, in this study, we discuss effects of emotional venting, perceived value, reward mechanisms, subjective norms, perceptual behavior control, hiring review control agency, supporting/disparaging merchants, recommending/disparaging products, perceived social costs, perceived psychological benefits, user perception impact, merchant perception impact, multimodal recognition and governance capabilities on multimodal fake review generation.
Emotional venting, perceived value, reward mechanisms, subjective norms, and perceptual behavior control
Perceptual behavior control refers to an individual’s perception of ease or difficulty in adopting a behavior 118 . Personal traits such as resources, cognition, and expertise can influence this perception. Emotions experienced in a given situation may influence an individual’s behavior 119 . Perceived value has a positive influence on behavioral control 120 . Material rewards can stimulate users to modify their previous negative reviews and generate fake reviews 121 . Additionally, subjective norms can affect perceptual behavior control and fake review generation 122 . Based on these reports, we hypothesize that: emotional venting significantly and positively affects perceptual behavior control (Hypothesis 1a); perceived value significantly and positively influences perceptual behavior control (Hypothesis 1b); reward mechanisms significantly and positively influence perceptual behavior control (Hypothesis 1c); subjective norms significantly and positively influence perceptual behavior control (Hypothesis 1d).
The mediating role of perceptual behavior control
Non-volitional determinants, such as necessary opportunities and resources, can influence information review behavior of individuals. Users’ perceptual behavior control plays a significant role in shaping their interaction with an online review. Increased support for a specific behavior enhances self-confidence in individuals, thereby leading to a subsequent increase in perceptual behavior control 123 . Similarly, reviews’ information will draw attention of individuals toward a product, thus impacting their perceptual behavior control 124 . Consequently, this research posits that users’ perceptual behavior control could potentially contribute to online multimodal fake review generation. Based on these reports, we hypothesize that: perceptual behavior control significantly and positively influences multimodal fake review generation (Hypothesis 2); perceptual behavior control mediates relationship between emotional venting and multimodal fake review generation (Hypothesis 3a); perceptual behavior control mediates relationship between perceived value and multimodal fake review generation (Hypothesis 3b); perceptual behavior control mediates relationship between reward mechanisms and multimodal fake review generation (Hypothesis 3c); perceptual behavior control mediates relationship between subjective norms and multimodal fake review generation (Hypothesis 3d).
Hiring review control agency, supporting/disparaging merchants, recommending/disparaging products, and multimodal fake review generation
Manipulation of reviews by merchants through hiring review control agencies is a widely observed and growing phenomenon. Such reviews often exhibit an excessive bias toward product promotion or denigration 125 . Merchants hire these review control agencies for two main purposes: first, to enhance visibility of their products and services; and second, to denigrate and undermine their competitors. Hence, primary purpose of hiring a review control agency is to achieve profitability. Hiring “water armies” is an extremely effective tactic for boosting commodity sales volume 126 . Additionally, for their vested interests, some merchants hire a review control agency to generate an online review. Based on these reports, we hypothesize that: hiring review control agency significantly and positively affects supporting/disparaging merchants (Hypothesis 4a); hiring review control agency significantly and positively affects recommending/disparaging products (Hypothesis 4b); hiring review control agency significantly and positively affects multimodal fake review generation (Hypothesis 4c).
The mediating role of supporting/disparaging merchants
With the rapid development of internet, competition among merchants has intensified. In context of heterogeneous multi-agent information interaction, facilitating merchants’ online reviews can effectively enhance their competitiveness. However, some merchants manipulate online reviews to misrepresent actual quality of their products 127 . When users find that a merchant fails to meet certain requirements, they will leave negative reviews of product. Moreover, Users can provide positive reviews to support merchants they approve of, thus generating multimodal fake review 128 . Users’ support or disparagement of merchants can significantly influence multimodal fake review generation 129 . Based on these reports, we hypothesize that: supporting/disparaging merchants significantly and positively affects multimodal fake review generation (Hypothesis 5); supporting/disparaging merchants mediates relationship between hiring review control agency and multimodal fake review generation (Hypothesis 6).
The mediating role of recommending/disparaging products
To express their contentment/discontentment with shopping experiences and products, users often generate excessively positive/negative online reviews. Primary motivation for improper review is to share their specific encounter with product and service, thereby providing subsequent users with valuable insights into purchasing process 130 . Online reputation has a facilitating effect on review behavior 131 . Contextual determinants significantly impact users’ inclination to generate online reviews. One of reasons behind users’ reviewing is to support merchants’ products. Based on these reports, we hypothesize that: recommending/disparaging products significantly and positively affects multimodal fake review generation (Hypothesis 7); recommending/disparaging products mediates relationship between hiring review control agency and multimodal fake review generation (Hypothesis 8).
Moderating effects of perceived social costs and perceived psychological benefits
Social exchange theory posits that individuals’ decision to exchange resources with others depends on their evaluation of perceived benefits and costs 132 . When merchants endorse or criticize merchants, recommend or disparage products, or hire review control agencies to generate multimodal fake reviews, they not only incur operational costs 133 but also bear psychological and social costs. Users’ perceived psychological benefits also play a role in shaping their information-related behaviors. When merchants get psychological benefits, they are more likely to generate multimodal fake reviews by hiring a review control agency. Based on these reports, we hypothesize that: perceived social costs negatively moderate relationship between supporting/disparaging merchants and multimodal fake review generation (Hypothesis 9a); perceived social costs negatively moderate relationship between recommending/disparaging products and multimodal fake review generation (Hypothesis 9b); perceived psychological benefits positively moderate relationship between supporting/disparaging merchants and multimodal fake review generation (Hypothesis 10a); perceived psychological benefits positively moderate relationship between recommending/disparaging products and multimodal fake review generation (Hypothesis 10b).
The moderating role of multimodal recognition and governance capabilities
Online multimodal fake review pertains to a diverse array of reviews, aligning with general notion of multimodal data. Purpose of multimodal identification is to discern instances of fake reviews. Multimodal review, in its various forms, holds greater utility value compared to unmoral online review 134 . Platform governance entails precise identification of multimodal reviews, followed by addressing hidden instances of multimodal fake reviews, ultimately ensuring an online ecosystem. Based on these reports, we hypothesize that: multimodal recognition and governance capabilities negatively moderate relationship between supporting/disparaging merchants and multimodal fake review generation (Hypothesis 11a); multimodal recognition and governance capabilities negatively moderate relationship between recommending/disparaging products and multimodal fake review generation (Hypothesis 11b); multimodal recognition and governance capabilities negatively moderate relationship between perceptual behavior control and multimodal fake review generation (Hypothesis 11c).
User perception impact, merchant perception impact, and multimodal fake review generation
Analysis of shocks perceived in online multimodal reviews includes two perspectives: intensity and emotion. A higher perceived intensity of online multimodal review views corresponds to a greater number of existing reviews. An increase in number of existing online reviews encourages users to make purchases 135 . Furthermore, sentiment expressed in online book reviews has a significant influence on book sales 136 . Therefore, perceived impact of existing online multimodal reviews can influence fake review generation. Based on these reports, we hypothesize that: user perception impact significantly and positively affects multimodal fake review generation (Hypothesis 12a); merchant perception impact significantly and positively affects multimodal fake review generation (Hypothesis 12b).
Therefore, based on conceptual framework of multi-agent fake signal generation and hypotheses, Fig. 2 presents our research model.
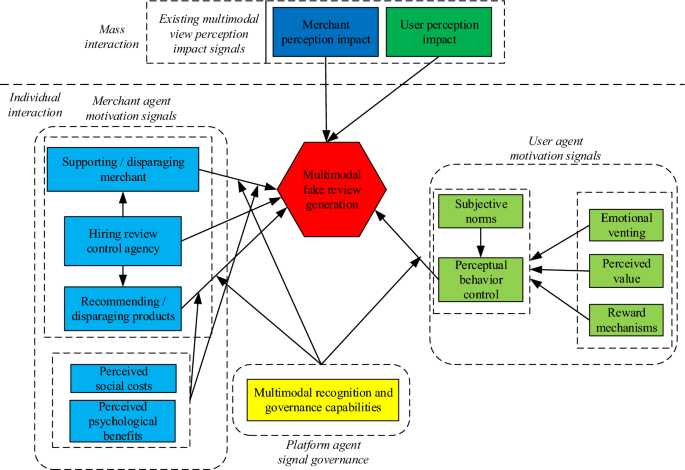
Research model. Note : red represents multimodal fake review generation, blue represents merchant-level feature factors, green represents user-level feature factors, and yellow represents platform-level feature factors.
Furthermore, upon review of relevant literature, we find that some scholars employ machine learning technique based on signaling theory to investigate impacts of electronic word of mouth as product signal on users’ purchase decisions 137 , 138 . Other researchers combine motivation theory with structural equation modeling to explore underlying motivations behind users’ additional review behavior in online reviews 139 , 140 , 141 . In addition, some scholars employ actor-network theory and complex networks analysis to examine influence of merchants and other agents on online review generation within online review environment 142 , 143 . Moreover, other scholars utilize information interaction theory and structural equation modeling to explore impacts of online reviews on user loyalty 144 , 145 , 146 . However, exploration of intrinsic mechanisms driving online fake review generation remains unclear, particularly in terms of integrating the theories of signaling, actor-network, motivation, and human–environment interaction hypothesis. Furthermore, there are few studies applying structural equation modeling, machine learning technique, and Bayesian complex networks analysis to explore intrinsic mechanisms of online fake review generation. Consequently, this study aims to address these gaps by integrating the theories of signaling, actor-network, motivation, and human–environment interaction hypothesis. Through incorporation of three methodologies, namely structural equation modeling, machine learning technique, and Bayesian complex networks analysis, this research seeks to find intrinsic mechanisms of online multimodal fake review generation, identify key determinants, and explore inherent correlations between these determinants as well as heterogeneity of male and female samples. This study can contribute to existing literature at both theoretical and methodological levels.
Study design
As three leading E-commerce platforms in China, Taobao, Jingdong, and Pinduoduo contain a considerable volume of fake reviews, it is essential to quantitatively analyze causal mechanisms behind fake review generation on these platforms. This analysis holds significant theoretical and practical implications for effectively preventing and managing fake reviews across all e-commerce platforms.
Based on the theories of signaling, actor-network, motivation, and human–environment interaction hypothesis, we develop a theoretical model for online multimodal fake review generation mechanisms. Our model encompasses platforms, users, and merchants. To gather data for our model, we design a questionnaire that consists of two parts. The first part collects basic information from participants, consisting of 9 items. The second part measures variables in model, with a total of 42 original items designed for explanatory and interpreted variables. We distribute electronic questionnaires to users of China’s three leading E-commerce platforms (Taobao, Jingdong, and Pinduoduo). We choose users from these platforms as survey respondents because they have extensive online shopping experience and frequently engage in online shopping reviews. Thus, samples from these platforms are highly representative of studying issue of fake reviews and can provide credible experimental results. Specifically, user samples from these three platforms offer strong data support for drawing trustworthy experimental conclusions. We obtain 1500 valid samples in total (500 valid samples from Taobao, Jingdong, and Pinduoduo platforms, respectively).
The following section compares the approaches used most in previous related research with the techniques and ideas of this research. Table 1 presents comparative analysis of research methodologies employed in relevant research.
According to Table 1 , most of similar literature employs methodologies such as structural equation modeling, machine learning technique, and complex network analysis to investigate related issues. Consequently, this study aims to address limitations of existing research by innovatively integrating three methods: first, employing structural equation modeling to explore intrinsic causal mechanisms behind online multimodal fake review generation, thereby uncovering black box of causal process of online multimodal fake review generation; second, employing machine learning to quantitatively analyze importance of determinants that influence online multimodal fake review generation, thus identifying key determinants of online multimodal fake review generation; finally, employing Bayesian complex networks analysis to delve into inherent correlations between these determinants and heterogeneity of male and female samples.
In summary, using survey data from users of China’s three leading E-commerce platforms (Taobao, Jingdong, and Pinduoduo), first, we employ structural equation modeling to examine mechanisms of online multimodal fake review generation, including measurement model analysis, structural model analysis, moderating effects analysis, and mediating effects analysis. Second, building on result of structural equation modeling, we use machine learning technique to further analyze key feature factors of online multimodal fake review generation and divide them into distinct regimes. Finally, based on results of machine learning technique, we investigate intrinsic correlations of these feature factors through Bayesian complex networks analysis, including network centrality analysis of full sample, male sample and female sample.
Therefore, Fig. 3 presents proposed research flow of our work.
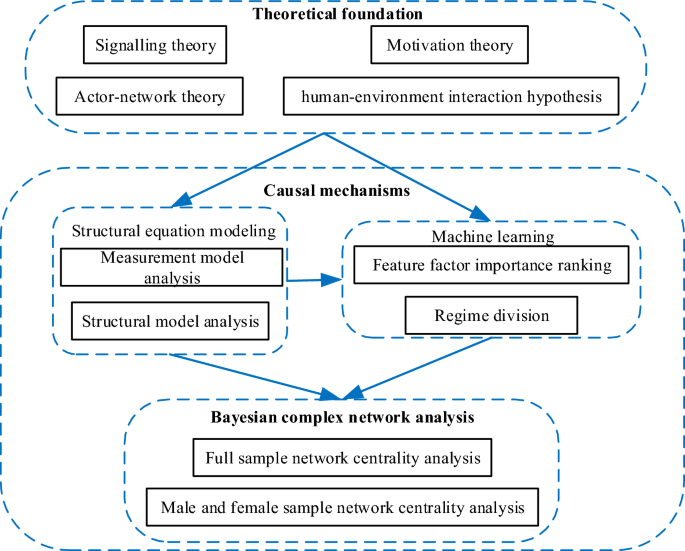
Proposed research flow of our work.
Participants
We obtain survey data from users of China’s three leading E-commerce platforms (Taobao, Jingdong, and Pinduoduo) from 15 March 2023 to 25 June 2023. Users of these three platforms have extensive online shopping experience and they frequently participate in online shopping reviews. Therefore, a sample of users from these platforms provides strong data support to draw credible experimental conclusions. Before distributing a large number of questionnaires, we conduct a pre-survey to correct terminology difficulties, improve question clarity, and enhance question differentiation. Moreover, we exclude questions that are irrelevant 167 . After eliminating any duplicate responses, we obtain a total of 1500 valid questionnaires. Sample contains 500 questionnaires from Taobao, 500 questionnaires from Jingdong, and 500 questionnaires from Pinduoduo, respectively. Table 2 presents descriptive statistics for demographic variables.
Ethical approval
All procedures performed in studies involving human participants are in accordance with the ethical standards of the institutional and/or national research committee and with the 1964 Helsinki declaration and its later amendments or comparable ethical standards.
Informed consent
Informed consent is obtained from all subjects involved in the study. All materials and methods are performed in accordance with the instructions and regulations and this research has been approved by a committee at Nanchang University, China.
To ensure scientificity and robustness of our questionnaire, final questionnaire consists of two sections. Section (i) is about personal and professional characteristics of respondents, and section (ii) is about measuring variables of theoretical framework to measure emotional venting (3 items), perceived value (3 items), reward mechanisms (2 items). subjective norms (3 items), perceptual behavior control (3 items), supporting/disparaging merchants (3 items), recommending/disparaging products (3 items), hiring review control agency (2 items), perceived social costs (2 items), perceived psychological benefits (2 items), multimodal recognition and governance capabilities (2 items), user perception impact (2 items), merchant perception impact (2 items), and multimodal fake review generation (3 items). A seven-point Likert scale (1-completely disagree to 7-completely agree) to measure items from survey respondents’ perspectives. Table 3 presents final survey items and relevant references.
Data analysis
Structural equation modeling (SEM) technique is used to test our research model. SEM is a multivariate statistical analysis method used to analyze relationships between constructs with multiple items. Two basic statistical methods are used for testing SEM: covariance-based SEM and variance-based partial least square (PLS). Covariance-based modeling is suitable for model validation and comparison, while PLS is used for complex structural models with a large number of constructs 179 , hence use of covariance-based SEM in our study. Two-step approach of Liu et al. 180 is used to carry out SEM. The first step is to perform a confirmatory factor analysis (CFA) to obtain a satisfactory measurement model, and the second is to develop a structural model and test it. Additionally, based on results of SEM, according to the study of Wang et al. 181 , we employ GBM (Gradient Boosting Regression) package in R to analyze importance of feature factors that influence online multimodal fake review generation. Furthermore, according to the study of Williams et al. 182 , we utilize BGGM (Bayesian Gaussian Graphical Models) package in R to examine inherent correlations among feature factors in full sample and analyze heterogeneity of male and female samples.
Structural equation modeling analysis
In this section, this paper employs structural equation techniques to identify factors that influence online multimodal fake review generation and analyze their impact pathways. Analysis includes reliability examinations, model fit indexes calculating, and hypotheses testing.
First, reliability examinations. In this paper, we refer to scholars Saadati et al. 183 and conduct validation determinants analysis to analyze reliability and validity of measurement model. As a result of analysis, observed variables with standardized determinants loadings below 0.670 are excluded from our study. Validation determinants analysis shows that standard error (S.E.) of each observed variable under unstandardized estimation of our model is greater than 0, indicating absence of any covariance problem among observed variables. Determinants loadings of each observational variable are found to be significant ( p < 0.001, |t|> 1.96). Standardized loading estimates of each observational indicator range from 0.668 to 0.942, all above 0.6. Conformal combination reliability CR ranges from 0.769 to 0.876, all above 0.7. Average coefficient of variation extract AVEs is also above 0.5, suggesting good convergent validity for model constructed in this paper. Moreover, square root of AVE for each observed variable exceeds correlation coefficient between variables, indicating good discriminant validity for our model. Thus, our model proposed in this paper is an acceptable model.
Second, model fit indexes calculating. We compute fit indexes of structural and measurement models, and Table 4 presents calculation results.
According to Table 4 , values of model fit indexes, including chi-square /df, comparative fit index, goodness of fit Index, adjusted goodness-of-fit index, root mean square error of approximation, tucker lewis index, and normed fit index, are greater than suggested values for both measurement and structural models. Therefore, based on study of Nan et al. 184 , 185 , Kar et al. 186 , and Shahzad et al. 187 , values of indicators are considered acceptable.
Finally, hypotheses testing. We test model paths using a significance level of p = 0.05. Upon examining path coefficient estimates, hypothesized path 4c is not significant and we remove it. After modificating our model, we retest constructed model’s impact paths, Table 5 presents final test results.
According to Table 5 , hypotheses 1a–1d, hypothesis 2, hypothesis 4a, hypothesis 4b, hypothesis 5, hypothesis 7, hypothesis 12a, and hypothesis 12b are supported. First, emotional venting, perceived value, reward mechanisms, and subjective norms exhibit significant positive indirect effects on multimodal fake review generation. Additionally, perceptual behavior control demonstrates direct and significant positive effects on multimodal fake review generation. Second, hiring review control agency directly and significantly influences both supporting/disparaging merchants, as well as recommending/disparaging products. Moreover, both supporting/disparaging merchants and recommending/disparaging products directly and significantly contribute to multimodal fake review generation. Finally, both user perception impact and merchant perception impact directly and significantly influence multimodal fake review generation.
Furthermore, according to the study of Huifeng et al. 188 , we adopt hierarchical moderated regression analysis to evaluate moderating effect of platforms’ multimodal recognition and governance capabilities. Table 6 presents test results.
According to Table 6 , hypotheses 9b and 11a are supported. First, perceived social costs exert a significant weakening influence on positive correlation between recommending/disparaging products and multimodal fake review generation. Second, platforms’ multimodal recognition and governance capabilities significantly inhibit positive relationship between supporting/disparaging merchants and multimodal fake review generation. Finally, perceived psychological benefits exert insignificant moderating effects on merchants’ multimodal fake review generation. Figure 4 presents final results of model paths and moderating effects tests.
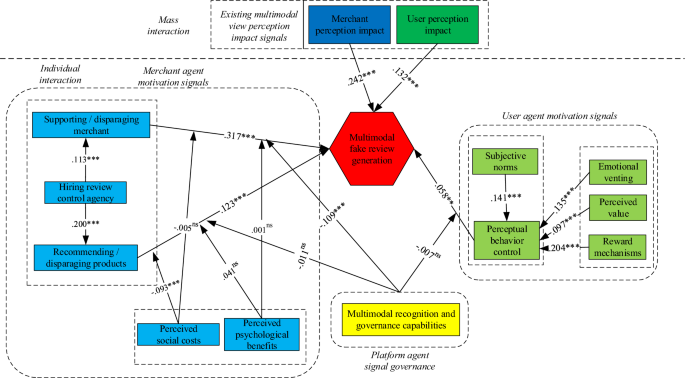
Final results of model paths and moderating effects testing. Note : * P < 0.05, ** P < 0.01, *** P < 0.001, ns indicates not significant, Red represents multimodal fake review generation, blue represents merchant-level feature factors, green represents user-level feature factors, and yellow represents platform-level feature factors.
In addition, this study employs Bootstrap method to examine mediating role of perceptual behavior control, supporting/disparaging merchants and recommending/disparaging products. More specifically, according to the study of Kim et al. 189 , mediating effects are assessed based on Bootstrap method, so this paper selects a sample size of 5000 to test mediation effects at a 95% confidence interval. Table 7 presents details analysis results.
According to Table 7 , both mediating effects of hiring review control agency on multimodal fake review generation do not include 0 ([0.0440, 0.1191], [0.0446, 0.0972]). Therefore, supporting/disparaging merchants and recommending/disparaging products serve as mediating variables between hiring review control agency and multimodal fake review generation. However, two direct effects of hiring review control agency on multimodal fake review generation do not exist (intervals of [− 0.0749, 0.0687] and [− 0.0701, 0.0895] include 0). Consequently, supporting/disparaging merchants and recommending/disparaging products fully mediate relationship between intermediary of hiring review control agency and multimodal fake review generation. Moreover, mediating effect between emotional venting and multimodal fake review generation does not include 0 ([0.0046, 0.0298]). Therefore, perceptual behavior control serves as a mediating variable between emotional venting and multimodal fake review generation. However, a direct effect of emotional venting on multimodal fake review generation exists (interval [0.2824, 0.3691] does not include 0). As a result, perceptual behavior control partially mediates relationship between emotional venting and multimodal fake review generation. Similarly, perceptual behavior control partially mediates relationship between perceived value and multimodal fake review generation, as well as between subjective norms and multimodal fake review generation. Additionally, mediating effect between reward mechanisms and multimodal fake review generation does not include 0 ([0.0456, 0.1056]). Hence, perceptual behavior control serves as a mediating variable between reward mechanisms and multimodal fake review generation. However, a direct effect of reward mechanisms on multimodal fake review generation does not exist (interval [− 0.0340, 0.1327] includes 0). Consequently, perceptual behavior control fully mediates relationship between reward mechanisms and multimodal fake review generation.
Machine learning prediction
In previous section, we use structural equation modeling to analyze online multimodal fake review generation mechanism, but we are unable to quantify weights of factors affecting online multimodal fake review generation, and we do not discover key feature factors affecting online multimodal fake review generation. Therefore, according to the study of Wang et al. 181 , this study further quantify weights of factors affecting online multimodal fake review generation and divide them into distinct regimes using GBM (Gradient Boosting Regression) package in R, to discover key feature factors affecting online multimodal fake review generation, and to further open up black box of online multimodal fake review generation mechanism.
First, sample division. According to the study of Moussa et al. 190 , Training set includes 960 sample data, validation set includes 240 sample data, and test set includes 300 sample data.
Second, model prediction performance evaluation. According to the study of Spee et al. 191 , Accuracy of model is evaluated using Eq. ( 1 ), Eq. ( 1 ) calculates ratio of correct predictions to all predictions. Correct predictions include true positive (TP) and true negative (TN) classes, while predictions themselves consist of true positives (TP), false positives (FP), true negatives (TN), and false negatives (FN). As mentioned in Eq. ( 2 ), precision measures proportion of true positive predictions (TP) relative to all positive predictions, including both true positives (TP) and false positives (FP). On the other hand, recall quantifies percentage of true positive predictions (TP) out of all positive instances in testing dataset, considering both true positives (TP) and false negatives (FN) as shown in Eq. ( 3 ). F1-value, as demonstrated in Eq. ( 4 ), is harmonic mean of precision and recall. It is calculated by multiplying precision and recall by two and then dividing result by their sum. Table 8 presents model evaluation metrics, Fig. 5 a,b present Receiver Operating Characteristic (ROC) curves and Andrews curves. True Positive Rate (TPR) on vertical coordinate of ROC curve represents proportion of actual positive instances among all positive instances in predicted positive class. Similarly, False Positive Rate (FPR) on horizontal coordinate represents proportion of actual negative instances among all negative instances in predicted positive class. In this context, “1” denotes a positive class, while “0” denotes a negative class. True Positive Rate (TPR) is evaluated using Eq. ( 5 ), and False Positive Rate (FPR) is evaluated using Eq. ( 6 ). Additionally, AUC value represents area under ROC curve. A higher AUC value indicates better predictive performance of model in terms of classification. Figure 5 c,d present training set accuracy, and changes in prediction error as regression tree increases. “0” denotes absence of multimodal fake review generation, and “1” denotes presence of multimodal fake review generation.
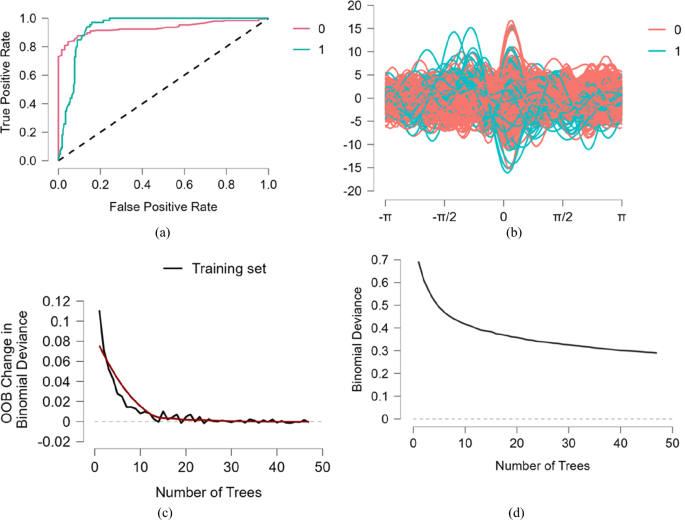
ROC curve, Andrews curves, model training set accuracy, and prediction error. Note : ( a ) is ROC curves, ( b ) is Andrews curves, ( c ) is model training set accuracy, and ( d ) is model prediction error.
According to Table 8 , recall, F1 score, and AUC achieve values greater than 0.85. According to Fig. 5 a,b, “0” and “1” curves reside within realm of meaningful sensitivity, they deviate from reference line, and model’s classification performance is also apparent. According to Fig. 5 c,d, as number of regression trees increases, model’s loss function continues to decrease, indicating that training accuracy is improving, and prediction error is gradually reducing. Therefore, all evaluation metrics demonstrate a good predictive performance of our model.
Third, feature factor importance ranking and regime division. Table 9 and Fig. 6 a present results of relative importance ranking analysis for feature factors. Table 10 presents results of regime division. Moreover, we get four conclusions: (1) reward mechanisms are key feature factor of online multimodal fake review generation. This implies that material incentives serve as driving force behind users’ generation of such deceptive content. Merchants’ reward information holds a significant influence over online multimodal fake review generation, particularly in context of user interactions with complex information. From a policy perspective, this discovery holds substantial practical value. Future governmental policies should focus on regulating incentivization strategies like cashback offers for positive reviews. Such measures can deter unscrupulous merchants from inducing users to generate multimodal fake reviews, ultimately fostering a healthier internet ecosystem; (2) perceived social costs significantly influence online multimodal fake review generation, whereas perceived psychological benefits exhibit no such impact. This finding further validates outcomes of previous structural equation modeling analysis: perceived psychological benefits do not exert a significant moderating effect on merchants’ multimodal fake review generation. This suggests that merchants are influenced by social costs, and display risk-averse behavior 192 , thereby exhibiting risk-averse tendencies; (3) user-level motivational determinants exert a greater impact compared to merchant-level motivational determinants. Importance ratio of all feature factors about user agents is found to be 50.317%, whereas that of merchant agents is 40.13%. This discrepancy may be attributed to fact that users generate fake reviews at almost no cost, Conversely, merchants that generate fake reviews have to bear social cost of pressure; (4) we can divide feature factors into four regimes. The first regime includes reward mechanisms and perceived social costs. The second regime includes subjective norms, recommending/disparaging products, hiring review control agency, and merchant perception impact. The third regime includes supporting/disparaging merchants, emotional venting, multimodal recognition and governance capabilities, and perceptual behavior control. The fourth regime includes perceived psychological benefits, user perception impact, and perceived value.
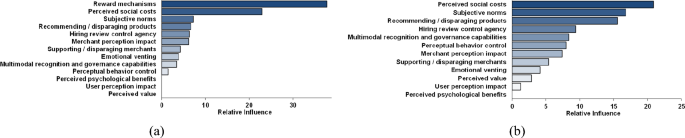
Feature factors ranking & Robustness test result.
Finally, robustness test. To ensure robustness of our empirical findings, according to the study of Jain et al. 193 , we remove the most significant feature factor, namely “reward mechanisms”, to examine remaining feature factors’ importance ranking. Figure 6 b presents test result.
Upon comparing Fig. 6 a,b, in general, robustness test result aligns with previous benchmark findings. This is because top five feature factors remain unchanged, and following elimination of pivotal “reward mechanisms”, perceived social costs are still the second most important determinant of online multimodal fake review generation. Moreover, final three feature factors remain unchanged. Hence, feature factors’ importance ranking is robust.
Bayesian complex networks analysis
In previous section, we employ structural equation modeling to establish a causal relationship between feature factors influencing online multimodal fake review generation. Additionally, we utilize machine learning technique to explore importance of each feature factor. However, we are unable to find inherent associations between these feature factors. Therefore, to develop a comprehensive understanding of inherent relationships between variables that influence online multimodal fake review generation, according to the study of Williams et al. 182 , we investigate significance of these variables and their associations, as well as heterogeneity of male and female samples through BGGM (Bayesian Gaussian Graphical Models) package in R, to conduct Bayesian complex networks analysis, including network centrality analysis of full sample, male sample, and female sample. From perspective of probability theory 194 , a Bayesian complex network represents joint distribution of a set of random variables, according to chain rule and conditional independence, joint distribution of a series of random variables \(X = \{ X_{1} , \ldots ,X_{n} \}\) can be written as Eq. ( 7 ). Variables’ connections are defined as network links. We employ R language with BGGM (Bayesian Gaussian Graphical Models) package to conduct complex networks analysis. This package allows for fitting of Bayesian Gaussian Graphical Models, facilitating hypothesis testing, estimation, and validation. Additionally, this package enables comparisons between Gaussian graphical models and prediction of individual nodes 195 .
Note: \(\pi (X_{i} )\) is collection of parent of \(X_{i} ,\pi (X_{i} ) \subseteq \{ X_{1} , \ldots ,X_{i - 1} \}\) , given value of \(\pi (X_{i} )\) ; \(X_{i}\) is conditionally independent of other variables 196 in \(\{ X_{1} , \ldots ,X_{i - 1} \}\) .
Network centrality analysis of full sample, male sample and female sample. Network centrality value primarily signifies position and role of each node in network. Our focus revolves around four key aspects: closeness centrality, betweenness centrality, strength centrality, and expected influence centrality 197 . Figure 7 a,b present network centrality analysis of full sample, male sample and female sample of online multimodal fake review generation.
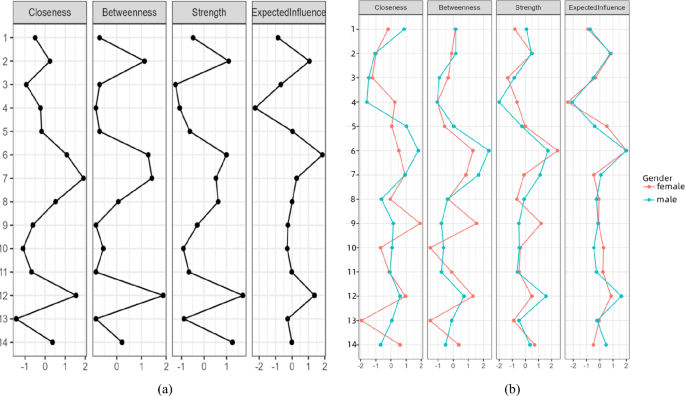
Network centrality analysis of full sample, male sample and female sample of online multimodal fake review generation. Note : sample size N = 1500; in ( a ), 1—multimodal fake review generation; 2—merchant perception impact; 3—user perception impact; 4—multimodal recognition and governance capabilities; 5—perceived psychological benefits; 6—perceived social costs; 7—hiring review control agency; 8—supporting/disparaging merchants; 9—recommending/disparaging products; 10—perceptual behavior control; 11—subjective norms; 12—reward mechanisms; 13—perceived value; 14—emotional venting; in ( b ), 1—multimodal fake review generation; 2—merchant perception impact; 3—user perception impact; 4—multimodal recognition and governance capabilities; 5—perceived psychological benefits; 6—perceived social costs; 7—hiring review control agency; 8—supporting/disparaging merchants; 9—recommending/disparaging products; 10—perceptual behavior control; 11—subjective norms; 12—reward mechanisms; 13—perceived value; 14—emotional venting.
Closeness centrality is a measure that quantifies average shortest path length between a node and all other nodes in a given network 198 . This measure indicates level of influence that a node has in generating multimodal fake reviews. A higher value signifies a greater degree of centrality and a closer proximity to other nodes. Based on the study of Elmezain et al. 199 , closeness centrality value is evaluated using Eq. ( 8 ). According to Fig. 7 a, in full sample, hiring review control agency, reward mechanisms, and perceived social costs exhibit higher values of centrality. This suggests that they have stronger connections with other nodes and occupy a more intermediate position in network. Consequently, fake review prevention efforts should prioritize these determinants. According to Fig. 7 b, in both male and female sample, closeness centrality values of reward mechanisms, perceived social costs, and hiring review control agency are all higher, aligning with findings of analysis conducted on full sample. However, male sample exhibits a higher closeness centrality value for perceived social costs compared to female sample. This indicates that men tend to be more rational in considering costs involved when generating online multimodal fake reviews.
Note: \(d(u,v)\) is the shortest path length from node \(u\) to node \(v\) .
Betweenness centrality measures effectiveness of a node in acting as a bridge on the shortest path between two other nodes 200 . Nodes with higher betweenness centrality values have greater control in network. Based on the study of Liu et al. 201 , betweenness centrality value is evaluated using Eq. ( 9 ). According to Fig. 7 a,b, in both full sample, male sample, and female sample, all nodes in online multimodal fake review generation exhibit non-zero betweenness centrality. This indicates that every node in network acts as a “bridge” connecting and influencing other nodes.
Note: \(v\) is node that we want to compute betweenness centrality, \(s\) and \(t\) are two other distinct nodes in network, \(\sigma_{st}\) is total number of shortest paths from node s to node t, and \(\sigma_{st} (v)\) is number of paths passing through node v from node s to node t in the shortest path.
Strength centrality is a natural generalization of node degree in a powerless network. Sum of weights of edges that are directly associated with a given node determines strength centrality 202 . A higher value of strength centrality indicates a stronger direct connection of node with other nodes. Based on the study of Abbasi et al. 203 , strength centrality value is evaluated using Eq. ( 10 ). Figure 7 a demonstrates that reward mechanisms exhibit the largest value of strength centrality, suggesting that they have the greatest node strength in network and the most direct connections with other nodes. User perception impact demonstrates the smallest value of strength centrality, indicating that it has the most indirect connections to other nodes. This observation further reinforces significance of reward mechanisms as a key node in network. Moreover, intervening in reward mechanisms can have a substantial impact on effectiveness of fake review governance. Additionally, according to Fig. 7 b, strength centrality values of perceived social costs are higher in both male and female sample, indicating that perceived social costs play a significant role in online multimodal fake review generation.
Note: \(N(v)\) denotes set of nodes directly connected to node v, i.e., neighbors of node v, and \(w_{uv}\) denotes weights of edges between node u and node v.
Expected influence centrality is used to describe magnitude of a node’s influence on network 204 . A larger value of expected influence signifies a greater influence on entire social relationship network. Based on the study of Schmidt et al. 205 , expected influence centrality value is evaluated using Eq. ( 11 ). According to Fig. 7 a, in full sample, variables with the highest positive expected impact on online multimodal fake review generation are reward mechanisms and perceived social costs. This suggests that even small fluctuations in reward mechanisms and perceived social costs can lead to significant changes in online multimodal fake review generation. These two factors are the most critical in influencing online multimodal fake review generation. Additionally, variable with the largest negative expected impact is platform’s multimodal recognition and governance capabilities. This indicates that platform’s multimodal recognition and governance capabilities strongly inhibits multimodal fake review generation. Furthermore, findings remain consistent in male and female sample, where reward mechanisms and perceived social costs also have the highest positive expected impact on online multimodal fake review generation. Similarly, negative expected impact of multimodal recognition and governance capabilities is also the highest, emphasizing importance of enhancing platform’s capacity to govern fake reviews.
Note: \(N(v)\) is set of nodes directly connected to node v, \(w_{uv}\) is weight of edge between node u and node v, and \(C(u)\) is some measure of centrality of node u, such as degree centrality, closeness centrality, or betweenness centrality.
A comprehensive comparison of centrality indicators suggests that, in full sample, closeness centrality and betweenness centrality exhibit similar trends, while strength centrality and expected influence centrality show roughly similar trends. Reward mechanisms indicators have relatively high values, indicating that this node plays a crucial role in online multimodal fake review generation. This may be attributed to users, driven by material rewards from merchants, being inclined to generate online multimodal fake reviews for benefits. Additionally, results of network centrality analysis exhibite heterogeneity between male and female samples, i.e., male sample has different trends in closeness centrality values and betweenness centrality values than female sample. Furthermore, when comparing results with importance ranking of feature factors obtained through machine learning technique, reward mechanisms are key feature factor of online multimodal fake review generation. This finding further validates robustness of our results.
In present study targeting China’s E-commerce platforms, determinants of online multimodal fake review generation are studied. Results show that determinants influencing online multimodal fake review generation are complex and interconnected. This study expands outcomes in previous research to some extent, especially concerning causal mechanisms of online multimodal fake review generation.
First, we find that platforms’ multimodal recognition and governance capabilities have a significant moderating effect on merchants’ fake review generation, but not on that of users. Results highlight influence of platforms’ signal governance on both merchant and user-generated fake review signals 206 . Empirical findings are consistent with previous research, providing support for proposed hypothesis 207 , 208 , 209 . We attribute this finding to our consideration of heterogeneous multi-agent complex signal interactions 210 . This suggests that platforms’ multimodal recognition and governance capabilities to regulate fake reviews can greatly impact merchants while having minimal influence on users. Studies indicate that platforms can effectively regulate merchants’ tendencies to induce online reviews, thereby limiting impact of merchant-generated reviews 211 , 212 , 213 . Consequently, adoption of specific review-generating regulatory strategies represents good option for platforms, elucidating stringent measures implemented by certain e-commerce platforms against merchant-generated reviews, such as Taobao’s limitation of rewarding positive reviews and offering cashback promotions 214 , 215 , 216 . Specifically, the stronger platforms’ multimodal recognition and governance capabilities, the greater limiting effect on merchants’ online multimodal fake review generation. However, impact of user-generated online multimodal fake reviews remains unaffected by platforms’ multimodal recognition and governance capabilities 217 , 218 . This result may be attributed to fact that users do not bear any costs for generating fake reviews, whereas merchants bear some social costs for generating fake reviews 219 . Additionally, Binder et al. 220 investigate into influence of platform regulation on volume of online reviews generated by merchants and consumers, highlighting greater impact on merchants. Li et al. 221 demonstrate that platforms’ signals for governing fake reviews significantly impact merchants’ online fake review generation but have no effect on consumers. Handan‐Nader et al. 222 observe that platforms’ review governing signals exert stronger influence on merchants than on consumers in context of fake review generation. Dai et al. 223 conclude that consumers are minimally impacted by platforms’ regulatory strategy and regulatory strength when generating product reviews.
Second, by introducing perceptual behavior control and quantifying its importance, this study demonstrates positive mediating role of perceptual behavior control in users’ multimodal fake review generation. This indicates that perceptual behavior control is an important determinant influencing users’ online multimodal fake review generation 224 , 225 , 226 . Our empirical evidence reveals that perceptual behavior control exerts both direct and indirect positive influence on users’ multimodal fake review generation. This indicates that users are external determinants in online multimodal fake review generation 227 , 228 , 229 . Specifically, within context of e-commerce platforms and driven by factors like emotional venting, perceived value, reward mechanisms, or subjective norms, users generate fake reviews through perceptual behavior control 230 , 231 , 232 . These findings not only complement but also extend outcomes found in partial mediation models proposed by Román et al. 233 , Petrescu et al. 234 , and Shahraki-Mohammadi et al. 235 . Furthermore, these findings are consistent with previous research, for example, Palese et al. 236 demonstrate significant influence of consumers’ emotional attitudes and subjective norms on perceptual behavior control, which mediates interplay between emotional attitudes, subjective norms, and online review behaviors. Niechwiej-Szwedo et al. 237 investigate into interrelation among positive Internet Word-of-Mouth (IWOM), perceptual behavior control in green consumption, and green consumption intention, revealing that positive IWOM significantly impacts both perceptual behavior control in green consumption and green consumption intentions, with the latter fully mediated by the former. In addition, Laszlo et al. 238 explore determinants of users’ online knowledge payment behavior, highlighting partial mediation role of user-perceived behavior control in translating normative beliefs into intentions to pay for online knowledge services. Knijnenburg et al. 239 reveal significant influence of consumers’ subjective norms and perceived behavior control on purchase intentions, with the latter serving as complete mediator between subjective norms and consumers’ purchase intentions.
Third, results show that reward mechanisms and perceived social costs emerge as the two most critical feature factors, reward mechanisms have the greatest impact on online multimodal fake review generation. Specifically, individuals are motivated to generate online product fake reviews to get various rewards, such as cash, points, or gift vouchers from merchants 240 , 241 . Outcomes of this investigation are consistent with frequently observed occurrences of positive reviews being exchanged for incentives. Moreover, these findings are consistent with results in existing literature, with many studies indicating significant positive impact of reward mechanisms on generating fake reviews 242 , 243 . While previous research points out that rewarding users is a key reason for disinformation generation 244 , they do not quantify degree of influence exerted by reward mechanisms. We find that reward mechanisms are primary determinants influencing users’ motivation to generate online multimodal fake reviews and quantify importance of reward mechanisms. This further enriches previous study 245 , 246 . Moreover, we highlight importance of perceived social costs, ranking it second in importance. This finding aligns with application of perceived cost theory to domain of online disinformation research 247 . Merchants perceive significant social pressure when interacting with other heterogeneous agents, thus driving them to be risk-averse 248 . Moreover, some researchers provide elucidation on this matter. For example, Chang et al. 249 identify that perceived social costs significantly shape consumers’ inclination to share content within their social network on digital platforms. In addition, we find that merchants’ perceived psychological benefits do not affect online multimodal fake review generation, further substantiating previous structural equation modeling analyses. For such issue, Guan et al. 250 reveal that online merchants who generate multimodal fake reviews demonstrate higher degree of risk aversion, as they carefully weigh costs associated with generating fake reviews. Importance of user-level motivational determinants is greater than that of merchant-level motivational determinants, likely due to the minimal cost users incur, as opposed to costs borne by merchants 251 . Furthermore, according to previous research 252 , 253 , we divide feature factors into distinct regimes based on their importance. Specifically, regime 1 encompasses reward mechanisms and perceived social costs. Regime 2 includes subjective norms, supporting/disparaging products, hiring review control agency, and merchant perception impact. Regime 3 includes supporting/disparaging merchants, perceptual behavior control, emotional venting, multimodal recognition and governance capabilities. Regime 4 encompasses perceived psychological benefits, user perception impact, and perceived value. This reasonable division of determinants is good for accurately identifying key determinants, thereby governing fake review generation. These findings significantly broaden existing research conducted by Shih et al. 28 and Triberti et al. 29 regarding determinants impacting fake review generation.
Finally, based on the study of Yang et al. 254 and Kudo et al. 255 , this study analyzes intrinsic associations of determinants that affect online multimodal fake review generation in full sample and analyzes heterogeneity of male and female samples. Both in full sample, male sample, and female sample, reward mechanisms have the most significant influence on online multimodal fake review generation. This result indicates high possibility for both male and female consumers to generate online fake reviews to get material rewards from merchants. The finding is consistent not only with empirical observations but also with prior research in relative fields. For example, Deng et al. 256 observe a similar phenomenon in their investigation of impact of leader’s reward neglect on employees’ propensity for silence. Additionally, perceived value, hiring review control agency, multimodal recognition and governance capabilities are strongly correlated, indicating a close interconnection between them. This implies that platforms’ multimodal recognition and governance capabilities are closely related to merchants’ online fake review generation behavior, a phenomenon explained by Sheng et al. 207 and Ma et al. 208 . However, results of network centrality analysis also exhibite heterogeneity between male and female samples, i.e., male sample has different trends in closeness centrality values and betweenness centrality values than female sample. Effective interventions targeting these variables can yield significant results in terms of multimodal disinformation governance. This indicates that gender may play an important role in online fake review generation, leading to observable disparities in engagement of fake review generation between male and female consumers. Existing literature supports this opinion, for example, Fjendbo et al. 257 explore impact of incentive performance pay on teachers’ motivation, and reveal that male teachers demonstrate greater emphasis on significance of performance-based compensation, and male teachers’ motivation appears to be more responsive to incentive performance pay in comparison to female teachers. Guenther et al. 258 highlight dissimilarities of males and females responding to incentives when engaging in risky behaviors.
Conclusions and future research
The majority of extant literature focuses on examining determinants, identification, and impact of fake review generation, but lacks insight into underlying causal mechanisms behind online fake review generation. Furthermore, prevalent scholarly works tend to rely on singular theoretical frameworks in investigating fake review generation, constructing research models that overlook influence of individual and mass interactions within the context of coexisting platforms, merchants, and users. Consequently, identified gaps in current research underscore imperative and significance of this study. The model we propose for fake review generation encompasses interactions among platforms, merchants, and users, offering valuable addition to current models investigating fake review generation. This research integrates the theories of signaling, actor-network, motivation, and human–environment interaction hypothesis to develop an original model elucidating mechanism of online multimodal fake review generation. The model takes into account individual and mass interactions in presence of multi-agents, including platforms, merchants, and users. Our study employs structural equation modeling to analyze online multimodal false review generation using data from China’s three leading e-commerce platforms, namely Taobao, Jingdong, and Pinduoduo. Additionally, our research investigates key determinants influencing online multimodal fake review generation through machine learning technique. By employing structural equation modeling and machine learning technique, our study uncovers causal mechanisms of online multimodal fake review generation and identifies reward mechanisms as key determinant influencing online multimodal fake review generation. Furthermore, our study reveals significant correlations among determinants contributing to online multimodal fake review generation.
This study presents several key theoretical implications. First, integration of the theories of signaling, actor-network, motivation, and human–environment interaction hypothesis serves as foundational framework, significantly broadening applicability of these established research paradigms. Second, by examining dynamics of individual and mass interactions among platforms, merchants, and users in shaping proliferation of online multimodal fake reviews, an original model is developed to elucidate generation mechanism under coalescence of these key agents. This model extends boundaries of information interaction theory and mechanisms for understanding fake review generation. Third, employing structural equation modeling, machine learning technique, and Bayesian complex networks, this study advances examination of online multimodal fake review generation, offering a fresh perspective on study of online fake reviews.
Considering bad impacts of fake reviews, our findings carry significant practical implications for merchants, online platforms, and public policy. First, merchants are advised to acknowledge that product quality, brand recognition, and authentic reviews serve as primary influences within digital marketplace. Rather than wasting resources towards fake review generation, efforts should be put towards enhancing brand integrity and fostering consumer confidence. Second, fake reviews can negatively affect both consumers and merchants, they also pose threats to online platforms. Investment in technological solutions, such as application of deep learning and other AI methods for bias management, review management, and service functionalities, is necessary. Third, online fake review necessitates attention from public policymakers. Guidelines should be implemented to curtail merchant manipulation through formulation of policies and provision of technical support, thereby ensuring sustained and healthy progression of e-commerce.
Despite these contributions, this study has several limitations, which serve to inform future research directions. First, environmental uncertainty may influence platforms’ multimodal recognition and governance capabilities, which we do not consider in this study. Hence, future researchers could explore impacts of environmental factors on online multimodal fake review generation. Second, incorporating individual differences and cross-cultural variables may uncover currently undiscussed variances. Subsequent studies could introduce moderating factors to expand the model. Finally, since this study may limit its generalizability as it is analyzed through a sample of platforms in China, more studies should be conducted in other countries and regions.
Data availability
Datasets used and/or analyzed during current study are available from corresponding author upon reasonable request.
Dai, W., Arnulf, J. K., Iao, L., Wan, P. & Dai, H. Like or want? Gender differences in attitudes toward online shopping in China. Psychol. Mark. 36 , 354–362. https://doi.org/10.1002/mar.21183 (2019).
Article Google Scholar
Nam, T. Who is dating and gaming online? Categorizing, profiling, and predicting online daters and gamers. Comput. Hum. Behav. 73 , 152–160. https://doi.org/10.1016/j.chb.2017.03.044 (2017).
Galindo-Losada, J., Ayala-Tipan, C., Santórum, M., Carrión-Toro, M. & Acosta-Vargas, P. WYDISWYG: A method to design user interfaces combining design principles and quality factors. Electronics https://doi.org/10.3390/electronics12132772 (2023).
Li, X. Could deal promotion improve merchants’ online reputations? The moderating role of prior reviews. J. Manag. Inf. Syst. 33 , 171–201. https://doi.org/10.1080/07421222.2016.1172450 (2016).
Salminen, J., Kandpal, C., Kamel, A. M., Jung, S.-G. & Jansen, B. J. Creating and detecting fake reviews of online products. J. Retail. Consum. Serv. https://doi.org/10.1016/j.jretconser.2021.102771 (2022).
Balasubramanian, A. et al. An analysis of popular online erectile dysfunction supplements. J. Sex Med. 16 , 843–852. https://doi.org/10.1016/j.jsxm.2019.03.269 (2019).
Article PubMed PubMed Central Google Scholar
He, S., Hollenbeck, B. & Proserpio, D. The market for fake reviews. Mark. Sci. 41 , 896–921. https://doi.org/10.1287/mksc.2022.1353 (2022).
Petrescu, M., Ajjan, H. & Harrison, D. L. Man vs machine—detecting deception in online reviews. J. Bus. Res. https://doi.org/10.1016/j.jbusres.2022.113346 (2023).
Singhal, R. & Kashef, R. A weighted stacking ensemble model with sampling for fake reviews detection. IEEE Trans. Comput. Soc. Syst. https://doi.org/10.1109/tcss.2023.3268548 (2024).
Athira, A. B., Kumar, S. D. M. & Chacko, A. M. A systematic survey on explainable AI applied to fake news detection. Eng. Appl. Artif. Intell. https://doi.org/10.1016/j.engappai.2023.106087 (2023).
Hlee, S., Lee, H., Koo, C. & Chung, N. Fake reviews or not: Exploring the relationship between time trend and online restaurant reviews. Telemat. Inform. https://doi.org/10.1016/j.tele.2020.101560 (2021).
Perach, R., Joyner, L., Husbands, D. & Buchanan, T. Why do people share political information and misinformation online? Developing a bottom-up descriptive framework. Soc. Media Soc. https://doi.org/10.1177/20563051231192032 (2023).
Kim, J. M., Park, K.K.-C. & Mariani, M. M. Do online review readers react differently when exposed to credible versus fake online reviews?. J. Bus. Res. https://doi.org/10.1016/j.jbusres.2022.113377 (2023).
Udry, J. & Barber, S. J. The illusory truth effect: A review of how repetition increases belief in misinformation. Curr. Opin. Psychol. https://doi.org/10.1016/j.copsyc.2023.101736 (2024).
Article PubMed Google Scholar
Toder Alon, A. & Tahar, H. Employing face reading technology to study the effects of the fake news ‘message sidedness on consumers’ emotional valence and arousal. Online Inf. Rev. 48 , 374–389. https://doi.org/10.1108/oir-01-2023-0005 (2023).
Li, L., Lee, K. Y., Lee, M. & Yang, S.-B. Unveiling the cloak of deviance: Linguistic cues for psychological processes in fake online reviews. Int. J. Hosp. Manag. https://doi.org/10.1016/j.ijhm.2020.102468 (2020).
Bodaghi, A., Schmitt, K. A., Watine, P. & Fung, B. C. M. A literature review on detecting, verifying, and mitigating online misinformation. IEEE Trans. Comput. Soc. Syst. https://doi.org/10.1109/tcss.2023.3289031 (2024).
Tajrian, M., Rahman, A., Kabir, M. A. & Islam, M. R. A review of methodologies for fake news analysis. IEEE Access 11 , 73879–73893. https://doi.org/10.1109/access.2023.3294989 (2023).
Qandos, N. et al. Multiscale cascaded domain-based approach for Arabic fake reviews detection in e-commerce platcforms. J. King Saud Univ. Comput. Inf. Sci. https://doi.org/10.1016/j.jksuci.2024.101926 (2024).
Sáez-Ortuño, L., Forgas-Coll, S., Huertas-Garcia, R. & Puertas-Prats, E. Chasing spammers: Using the Internet protocol address for detection. Psychol. Mark. https://doi.org/10.1002/mar.21985 (2024).
Luo, J., Luo, J., Nan, G. & Li, D. Fake review detection system for online E-commerce platforms: A supervised general mixed probability approach. Decis. Support Syst. https://doi.org/10.1016/j.dss.2023.114045 (2023).
Zhang, Z. et al. Spammer detection via ranking aggregation of group behavior. Expert Syst. Appl. https://doi.org/10.1016/j.eswa.2022.119454 (2023).
Li, Y., Zhang, Z., Pedersen, S., Liu, X. & Zhang, Z. The influence of relative popularity on negative fake reviews: A case study on restaurant reviews. J. Bus. Res. https://doi.org/10.1016/j.jbusres.2023.113895 (2023).
He, L., Xu, G., Jameel, S., Wang, X. & Chen, H. Graph-aware deep fusion networks for online spam review detection. IEEE Trans. Comput. Soc. Syst. 10 , 2557–2565. https://doi.org/10.1109/tcss.2022.3189813 (2023).
Li, Y., Sun, Q. & Sheng, F. Consumer inoculation and gender in combating negative product reviews. J. Retail. Consum. Serv. https://doi.org/10.1016/j.jretconser.2023.103703 (2024).
Badi, I., McCarty, D. & Kim, H. W. Risky behaviors and road safety: An exploration of age and gender influences on road accident rates. PLoS ONE https://doi.org/10.1371/journal.pone.0296663 (2024).
Pignolet, Y.-A., Schmid, S. & Seelisch, A. Gender-specific homophily on Instagram and implications on information spread. Sci. Rep. https://doi.org/10.1038/s41598-023-51117-w (2024).
Shih, H.-P., Lai, K.-H. & Cheng, T. C. E. Complied by belief consistency: The cognitive-information lens of user-generated persuasion. J. Theor. Appl. Electron. Commerc. Res. 18 , 372–393. https://doi.org/10.3390/jtaer18010020 (2023).
Article ADS Google Scholar
Triberti, S., Bryanov, K. & Vziatysheva, V. Determinants of individuals’ belief in fake news: A scoping review determinants of belief in fake news. PLoS ONE https://doi.org/10.1371/journal.pone.0253717 (2021).
Bao, Z. & Zhu, Y. Understanding online reviews adoption in social network communities: An extension of the information adoption model. Inf. Technol. People https://doi.org/10.1108/itp-03-2022-0158 (2023).
Yu, W., Payton, B., Sun, M., Jia, W. & Huang, G. Toward an integrated framework for misinformation and correction sharing: A systematic review across domains. New Media Soc. 25 , 2241–2267. https://doi.org/10.1177/14614448221116569 (2022).
Hong, I. B. & Kim, M. Understanding the influence of a host’s guest perceptions on sharing intention on the airbnb platform: A signaling theory perspective. Telemat. Inform. https://doi.org/10.1016/j.tele.2023.102096 (2024).
Chen, L., Li, W., Chen, H. & Geng, S. Detection of fake reviews: Analysis of sellers’ manipulation behavior. Sustainability https://doi.org/10.3390/su11174802 (2019).
Chao, J., Zhao, C., Zhang, F. & Su, C. Network embedding-based approach for detecting collusive spamming groups on E-commerce platforms. Secur. Commun. Netw. 2022 , 1–13. https://doi.org/10.1155/2022/4354086 (2022).
Akhtar, N., Siddiqi, U. I., Islam, T. & Paul, J. Consumers’ untrust and behavioral intentions in the backdrop of hotel booking attributes. Int. J. Contemp. Hosp. Manag. 34 , 2026–2047. https://doi.org/10.1108/ijchm-07-2021-0845 (2022).
Ennaouri, M. & Zellou, A. Machine learning approaches for fake reviews detection: A systematic literature review. J. Web Eng. https://doi.org/10.13052/jwe1540-9589.2254 (2023).
Yu, C., Zuo, Y., Feng, B., An, L. & Chen, B. An individual-group-merchant relation model for identifying fake online reviews: An empirical study on a Chinese e-commerce platform. Inf. Technol. Manag. 20 , 123–138. https://doi.org/10.1007/s10799-018-0288-1 (2018).
Jin, C., Yang, L. & Hosanagar, K. To brush or not to brush: Product rankings, consumer search, and fake orders. Inf. Syst. Res. 34 , 532–552. https://doi.org/10.1287/isre.2022.1128 (2023).
Nakayama, M. & Wan, Y. Exploratory study on anchoring: Fake vote counts in consumer reviews affect judgments of information quality. J. Theor. Appl. Electron. Commerc. Res. 12 , 1–20. https://doi.org/10.4067/s0718-18762017000100002 (2017).
Hajek, P., Barushka, A. & Munk, M. Fake consumer review detection using deep neural networks integrating word embeddings and emotion mining. Neural Comput. Appl. 32 , 17259–17274. https://doi.org/10.1007/s00521-020-04757-2 (2020).
Nobahary, S., Gharaee Garakani, H., Khademzadeh, A. & Karuppiah, M. Detecting noncooperation nodes mechanisms in wireless networks: A survey. Secur. Commun. Netw. 2022 , 1–20. https://doi.org/10.1155/2022/6486816 (2022).
Kolhar, M. E-commerce review system to detect false reviews. Sci. Eng. Ethics 24 , 1577–1588. https://doi.org/10.1007/s11948-017-9959-2 (2018).
Shukla, A. D. & Goh, J. M. Fighting fake reviews: Authenticated anonymous reviews using identity verification. Bus. Horizons 67 , 71–81. https://doi.org/10.1016/j.bushor.2023.08.002 (2024).
Anderson, E. T. & Simester, D. I. Reviews without a purchase: Low ratings, loyal customers, and deception. J. Mark. Res. 51 , 249–269. https://doi.org/10.1509/jmr.13.0209 (2014).
Farhoudinia, B., Ozturkcan, S. & Kasap, N. Fake news in business and management literature: A systematic review of definitions, theories, methods and implications. Aslib J. Inf. Manag. https://doi.org/10.1108/ajim-09-2022-0418 (2023).
Rodríguez-Ferrándiz, R. An overview of the fake news phenomenon: From untruth-driven to post-truth-driven approaches. Media Commun. https://doi.org/10.17645/mac.v11i2.6315 (2023).
Wang, Y., Zamudio, C. & Jewell, R. D. The more they know: Using transparent online communication to combat fake online reviews. Bus. Horizons 66 , 753–764. https://doi.org/10.1016/j.bushor.2023.03.004 (2023).
George, J., Gerhart, N. & Torres, R. Uncovering the truth about fake news: A research model grounded in multi-disciplinary literature. J. Manag. Inf. Syst. https://doi.org/10.1080/07421222.2021.1990608 (2021).
Bianchi, F. P. & Tafuri, S. Spreading of misinformation on mass media and digital platforms regarding vaccines. A systematic scoping review on stakeholders, policymakers, and sentiments/behavior of Italian consumers. Hum. Vaccines Immunother. https://doi.org/10.1080/21645515.2023.2259398 (2023).
Harrison-Walker, L. J. & Jiang, Y. Suspicion of online product reviews as fake: Cues and consequences. J. Bus. Res. https://doi.org/10.1016/j.jbusres.2023.113780 (2023).
Akhtar, N., Ahmad, W., Siddiqi, U. I. & Akhtar, M. N. Predictors and outcomes of consumer deception in hotel reviews: The roles of reviewer type and attribution of service failure. J. Hosp. Tour. Manag. 39 , 65–75. https://doi.org/10.1016/j.jhtm.2019.03.004 (2019).
Moon, S., Kim, M.-Y. & Iacobucci, D. Content analysis of fake consumer reviews by survey-based text categorization. Int. J. Res. Mark. 38 , 343–364. https://doi.org/10.1016/j.ijresmar.2020.08.001 (2021).
Zhang, Z., Li, Y., Li, H. & Zhang, Z. Restaurants’ motivations to solicit fake reviews: A competition perspective. Int. J. Hosp. Manag. https://doi.org/10.1016/j.ijhm.2022.103337 (2022).
Khan, J. A. et al. Can end-user feedback in social media be trusted for software evolution: Exploring and analyzing fake reviews. Concurr. Comput. Pract. Exp. https://doi.org/10.1002/cpe.7990 (2023).
Mohawesh, R., Al-Hawawreh, M., Maqsood, S. & Alqudah, O. Factitious or fact? Learning textual representations for fake online review detection. Clust. Comput.-J. Netw. Softw. Tools Appl. https://doi.org/10.1007/s10586-023-04148-x (2023).
Rasappan, P., Premkumar, M., Sinha, G. & Chandrasekaran, K. Transforming sentiment analysis for e-commerce product reviews: Hybrid deep learning model with an innovative term weighting and feature selection. Inf. Process. Manag. https://doi.org/10.1016/j.ipm.2024.103654 (2024).
Su, L. & Zhu, D. H. A picture is worth a thousand words: Understanding the predictors of picture sharing in online consumer reviews. Heliyon https://doi.org/10.1016/j.heliyon.2023.e22789 (2023).
Karnyoto, A. S., Sun, C., Liu, B. & Wang, X. TB-BCG: topic-based BART counterfeit generator for fake news detection. Mathematics https://doi.org/10.3390/math10040585 (2022).
Shahzad, K., Khan, S. A., Ahmad, S. & Iqbal, A. A scoping review of the relationship of big data analytics with context-based fake news detection on digital media in data age. Sustainability https://doi.org/10.3390/su142114365 (2022).
Kumar, A., Gopal, R. D., Shankar, R. & Tan, K. H. Fraudulent review detection model focusing on emotional expressions and explicit aspects: Investigating the potential of feature engineering. Decis. Support Syst. https://doi.org/10.1016/j.dss.2021.113728 (2022).
Zhou, Y. et al. Does the review deserve more helpfulness when its title resembles the content? Locating helpful reviews by text mining. Inf. Process. Manag. https://doi.org/10.1016/j.ipm.2019.102179 (2020).
Mewada, A. & Dewang, R. K. Research on false review detection methods: A state-of-the-art review. J. King Saud Univ. Comput. Inf. Sci. 34 , 7530–7546. https://doi.org/10.1016/j.jksuci.2021.07.021 (2022).
Xu, Y. et al. Slanderous user detection with modified recurrent neural networks in recommender system. Inf. Sci. 505 , 265–281. https://doi.org/10.1016/j.ins.2019.07.081 (2019).
Article MathSciNet Google Scholar
Srisaila, A., Rajani, D., Madhavi, M. V. D. N. S., Asha Shiny, X. S. & Amarendra, K. Modelling a dense hybrid network model for fake review analysis using learning approaches. Soft Comput. 28 , 3519–3532. https://doi.org/10.1007/s00500-023-09609-4 (2024).
Cheng, L.-C., Wu, Y. T., Chao, C.-T. & Wang, J.-H. Detecting fake reviewers from the social context with a graph neural network method. Decis. Support Syst. https://doi.org/10.1016/j.dss.2023.114150 (2024).
Wang, Y., Zamudio, C. S. & Jewell, D. R. The more they know: Using transparent online communication to combat fake online reviews. Bus. Horizons https://doi.org/10.1016/j.jbusres.2023.114200 (2023).
Akhtar, N., Ahmad, W., Siddiqi, U. I. & Akhtar, M. N. Predictors and outcomes of consumer deception in hotel reviews: The roles of reviewer type and attribution of service failure. J. Hosp. Tour. Manag. https://doi.org/10.3390/electronics12153280 (2023).
Hajek, P., Hikkerova, L. & Sahut, J.-M. Fake review detection in e-Commerce platforms using aspect-based sentiment analysis. J. Bus. Res. https://doi.org/10.1016/j.jbusres.2023.114143 (2023).
Duma, R. A. et al. DHMFRD—TER: A deep hybrid model for fake review detection incorporating review texts, emotions, and ratings. Multimed. Tools Appl. 83 , 4533–4549. https://doi.org/10.1007/s11042-023-15193-4 (2023).
Vidanagama, D. U., Silva, A. T. P. & Karunananda, A. S. Ontology based sentiment analysis for fake review detection. Expert Syst. Appl. https://doi.org/10.1016/j.eswa.2022.117869 (2022).
Zhou, X. & Zafarani, R. A survey of fake news. ACM Comput. Surv. 53 , 1–40. https://doi.org/10.1145/3395046 (2020).
Zhai, Y., Song, X., Chen, Y. & Lu, W. A study of mobile medical app user satisfaction incorporating theme analysis and review sentiment tendencies. Int. J. Environ. Res. Public Health https://doi.org/10.3390/ijerph19127466 (2022).
Deshai, N. & Rao, B. B. Transparency in healthcare and e-commerce: Detecting online fake reviews using a dense neural network model with relevance mapping. Soft Comput. 27 , 9861–9875. https://doi.org/10.1007/s00500-023-08437-w (2023).
Martínez-Torres, M. R., Arenas-Marquez, F. J., Olmedilla, M. & Toral, S. L. Identifying the features of reputable users in eWOM communities by using Particle Swarm Optimization. Technol. Forecast. Soc. Chang. 133 , 220–228. https://doi.org/10.1016/j.techfore.2018.04.017 (2018).
Qu, Z. et al. Detecting fake reviews with generative adversarial networks for mobile social networks. Secur. Commun. Netw. 2022 , 1–11. https://doi.org/10.1155/2022/1164125 (2022).
Bathla, G., Singh, P., Singh, R. K., Cambria, E. & Tiwari, R. Intelligent fake reviews detection based on aspect extraction and analysis using deep learning. Neural Comput. Appl. 34 , 20213–20229. https://doi.org/10.1007/s00521-022-07531-8 (2022).
Fang, Y., Wang, H., Zhao, L., Yu, F. & Wang, C. Dynamic knowledge graph based fake-review detection. Appl. Intell. 50 , 4281–4295. https://doi.org/10.1007/s10489-020-01761-w (2020).
Kaliyar, R. K., Goswami, A., Narang, P. & Sinha, S. FNDNet—a deep convolutional neural network for fake news detection. Cognit. Syst. Res. 61 , 32–44. https://doi.org/10.1016/j.cogsys.2019.12.005 (2020).
Ben Jabeur, S., Ballouk, H., Ben Arfi, W. & Sahut, J.-M. Artificial intelligence applications in fake review detection: Bibliometric analysis and future avenues for research. J. Bus. Res. https://doi.org/10.1016/j.jbusres.2022.113631 (2023).
Plotkina, D., Munzel, A. & Pallud, J. Illusions of truth—experimental insights into human and algorithmic detections of fake online reviews. J. Bus. Res. 109 , 511–523. https://doi.org/10.1016/j.jbusres.2018.12.009 (2020).
Le, T.-K.-H., Li, Y.-Z. & Li, S.-T. Do reviewers’ words and behaviors help detect fake online reviews and spammers? Evidence from a hierarchical model. Ieee Access https://doi.org/10.1109/ACCESS.2022.3167511 (2022).
Song, Y., Wang, L., Zhang, Z. & Hikkerova, L. Do fake reviews promote consumers’ purchase intention?. J. Bus. Res. https://doi.org/10.1016/j.jbusres.2023.113971 (2023).
Duan, W., Gu, B. & Whinston, A. B. Do online reviews matter?—An empirical investigation of panel data. Decis. Support Syst. 45 , 1007–1016. https://doi.org/10.1016/j.dss.2008.04.001 (2008).
Berger, J., Sorensen, A. T. & Rasmussen, S. J. Positive effects of negative publicity: When negative reviews increase sales. Mark. Sci. 29 , 815–827. https://doi.org/10.1287/mksc.1090.0557 (2010).
Costa Filho, M., Nogueira Rafael, D., Salmonson Guimarães Barros, L. & Mesquita, E. Mind the fake reviews! Protecting consumers from deception through persuasion knowledge acquisition. J. Bus. Res. https://doi.org/10.1016/j.jbusres.2022.113538 (2023).
Ali Hakami, N. & Hosni Mahmoud, H. A. Deep learning analysis for reviews in arabic E-commerce sites to detect consumer behavior towards sustainability. Sustainability https://doi.org/10.3390/su141912860 (2022).
Tufail, H., Ashraf, M. U., Alsubhi, K. & Aljahdali, H. M. The effect of fake reviews on e-commerce during and after Covid-19 pandemic: SKL-based fake reviews detection. IEEE Access 10 , 25555–25564. https://doi.org/10.1109/access.2022.3152806 (2022).
Paul, H. & Nikolaev, A. Fake review detection on online E-commerce platforms: A systematic literature review. Data Min. Knowl. Discov. 35 , 1830–1881. https://doi.org/10.1007/s10618-021-00772-6 (2021).
Ansari, S. & Gupta, S. Customer perception of the deceptiveness of online product reviews: A speech act theory perspective. Int. J. Inf. Manag. https://doi.org/10.1016/j.ijinfomgt.2020.102286 (2021).
Wu, Y., Ngai, E. W. T., Wu, P. & Wu, C. Fake online reviews: Literature review, synthesis, and directions for future research. Decis. Support Syst. https://doi.org/10.1016/j.dss.2020.113280 (2020).
Barbado, R., Araque, O. & Iglesias, C. A. A framework for fake review detection in online consumer electronics retailers. Inf. Process. Manag. 56 , 1234–1244. https://doi.org/10.1016/j.ipm.2019.03.002 (2019).
Wang, C. et al. The effects of money on fake rating behavior in E-commerce: electrophysiological time course evidence from consumers. Front. Neurosci. https://doi.org/10.3389/fnins.2018.00156 (2018).
Di Domenico, G. & Ding, Y. Between brand attacks and broader narratives: How direct and indirect misinformation erode consumer trust. Curr. Opin. Psychol. https://doi.org/10.1016/j.copsyc.2023.101716 (2023).
Yuan, L., Jiang, H., Shen, H., Shi, L. & Cheng, N. Sustainable development of information dissemination: A review of current fake news detection research and practice. Systems https://doi.org/10.3390/systems11090458 (2023).
Koukova, N. T., Wang, R.J.-H. & Isaac, M. S. “If you loved our product”: Do conditional review requests harm retailer loyalty?. J. Retail. 99 , 85–101. https://doi.org/10.1016/j.jretai.2022.09.002 (2023).
Shen, Q., Han, S., Han, Y. & Chen, X. User review analysis of dating apps based on text mining. PLoS ONE https://doi.org/10.1371/journal.pone.0283896 (2023).
Petratos, P. N. & Faccia, A. Fake news, misinformation, disinformation and supply chain risks and disruptions: Risk management and resilience using blockchain. Ann. Oper. Res. 327 , 735–762. https://doi.org/10.1007/s10479-023-05242-4 (2023).
Mardumyan, A. & Siret, I. When review verification does more harm than good: How certified reviews determine customer–brand relationship quality. J. Bus. Res. https://doi.org/10.1016/j.jbusres.2023.113756 (2023).
Guest, D. E., Sanders, K., Rodrigues, R. & Oliveira, T. Signalling theory as a framework for analysing human resource management processes and integrating human resource attribution theories: A conceptual analysis and empirical exploration. Hum. Resour. Manag. J. 31 , 796–818. https://doi.org/10.1111/1748-8583.12326 (2020).
Wang, Y. et al. Towards easy comparison of local businesses using online reviews. Comput. Graphics Forum 37 , 63–74. https://doi.org/10.1111/cgf.13401 (2018).
Article CAS Google Scholar
Chen, Y., Sun, S., Zhao, X., Zhou, H. & Wang, F. Objective facts or misleading hype? Associations between features of E-cigarette marketing and sales on a Chinese E-commerce platform. Int. J. Environ. Res. Public Health https://doi.org/10.3390/ijerph17186711 (2020).
Liao, S.-L., Shen, Y.-C. & Chu, C.-H. The effects of sales promotion strategy, product appeal and consumer traits on reminder impulse buying behaviour. Int. J. Consum. Stud. 33 , 274–284. https://doi.org/10.1111/j.1470-6431.2009.00770.x (2009).
Meents, S. & Verhagen, T. Reducing consumer risk in electronic marketplaces: The signaling role of product and seller information. Comput. Hum. Behav. 86 , 205–217. https://doi.org/10.1016/j.chb.2018.04.047 (2018).
Brown, J. A., Rudie, J. D., Bandrowski, A., Van Horn, J. D. & Bookheimer, S. Y. The UCLA multimodal connectivity database: A web-based platform for brain connectivity matrix sharing and analysis. Front Neuroinform. 6 , 28. https://doi.org/10.3389/fninf.2012.00028 (2012).
Choi, H. S., Ko, M. S., Medlin, D. & Chen, C. The effect of intrinsic and extrinsic quality cues of digital video games on sales: An empirical investigation. Decis. Support Syst. 106 , 86–96. https://doi.org/10.1016/j.dss.2017.12.005 (2018).
Mavlanova, T., Benbunan-Fich, R. & Lang, G. The role of external and internal signals in E-commerce. Decis. Support Syst. 87 , 59–68. https://doi.org/10.1016/j.dss.2016.04.009 (2016).
Kokkodis, M., Lappas, T. & Kane, G. C. Optional purchase verification in e-commerce platforms: More representative product ratings and higher quality reviews. Prod. Oper. Manag. 31 , 2943–2961. https://doi.org/10.1111/poms.13731 (2022).
Alsubari, S. N. et al. Computational intelligence based recurrent neural network for identification deceptive review in the E-commerce domain. Comput. Intell. Neurosci. 2022 , 1–14. https://doi.org/10.1155/2022/4656846 (2022).
Rydin, Y. Using actor-network theory to understand planning practice: Exploring relationships between actants in regulating low-carbon commercial development. Plann. Theory 12 , 23–45. https://doi.org/10.1177/1473095212455494 (2012).
Ozuem, W., Ranfagni, S., Willis, M., Rovai, S. & Howell, K. Exploring customers’ responses to online service failure and recovery strategies during Covid-19 pandemic: An actor-network theory perspective. Psychol. Mark. 38 , 1440–1459. https://doi.org/10.1002/mar.21527 (2021).
Parent, N. Basic need satisfaction through social media engagement: A developmental framework for understanding adolescent social media use. Hum. Dev. 67 , 1–17. https://doi.org/10.1159/000529449 (2023).
Zaman, M. et al. Motives for posting fake reviews: Evidence from a cross-cultural comparison. J. Bus. Res. https://doi.org/10.1016/j.jbusres.2022.113359 (2023).
García-Jurado, A., Pérez-Barea, J. J. & Fernández-Navarro, F. Towards digital sustainability: Profiles of millennial reviewers, reputation scores and intrinsic motivation matter. Sustainability https://doi.org/10.3390/su13063297 (2021).
Garnefeld, I., Helm, S. & Grötschel, A.-K. May we buy your love? Psychological effects of incentives on writing likelihood and valence of online product reviews. Electron. Mark. 30 , 805–820. https://doi.org/10.1007/s12525-020-00425-4 (2020).
Wu, P. F. Motivation crowding in online product reviewing: A qualitative study of amazon reviewers. Inf. Manag. https://doi.org/10.1016/j.im.2019.04.006 (2019).
Dunkel, A., Hartmann, M. C., Hauthal, E., Burghardt, D. & Purves, R. S. From sunrise to sunset: Exploring landscape preference through global reactions to ephemeral events captured in georeferenced social media. PLoS ONE 18 , e0280423. https://doi.org/10.1371/journal.pone.0280423 (2023).
Article CAS PubMed PubMed Central Google Scholar
Compton, A., Roop, B. W., Parrell, B. & Lammert, A. C. Stimulus whitening improves the efficiency of reverse correlation. Behav. Res. Methods https://doi.org/10.3758/s13428-022-01946-w (2022).
Wang, H., Li, C., Liu, J. & Zhang, S. Research on farmers’ willingness of land transfer behavior based on food security. Sustainability https://doi.org/10.3390/su11082338 (2019).
Merrikhi, Y., Mirzaei, A., Kok, M. A., Meredith, M. A. & Lomber, S. G. Deafness induces complete crossmodal plasticity in a belt region of dorsal auditory cortex. Eur. J. Neurosci. https://doi.org/10.1111/ejn.16075 (2023).
Miguel, L., Marques, S. & Duarte, A. P. The influence of consumer ethnocentrism on purchase of domestic fruits and vegetables: Application of the extended theory of planned behaviour. Br. Food J. 124 , 599–618. https://doi.org/10.1108/bfj-11-2021-1208 (2022).
Helms, Y. B. et al. Applications and recruitment performance of web-based respondent-driven sampling: Scoping review. J. Med. Internet Res. 23 , e17564. https://doi.org/10.2196/17564 (2021).
Tajeddini, K., Mostafa Rasoolimanesh, S., Chathurika Gamage, T. & Martin, E. Exploring the visitors’ decision-making process for Airbnb and hotel accommodations using value-attitude-behavior and theory of planned behavior. Int. J. Hosp. Manag. https://doi.org/10.1016/j.ijhm.2021.102950 (2021).
Melnyk, V., Carrillat, F. A. & Melnyk, V. The influence of social norms on consumer behavior: A meta-analysis. J. Mark. 86 , 98–120. https://doi.org/10.1177/00222429211029199 (2021).
Yao, J. & Oppewal, H. Unit pricing matters more when consumers are under time pressure. Eur. J. Mark. 50 , 1094–1114. https://doi.org/10.1108/ejm-03-2015-0122 (2016).
Wokes, J. E. T., McLean, N. R. & Boyd, M. Fake news, defamation, online reviews, and their potential devastating consequences for aesthetic plastic surgeons. Aesthet Surg J 42 , NP546–NP553. https://doi.org/10.1093/asj/sjac080 (2022).
Stanton, S. J., Sinnott-Armstrong, W. & Huettel, S. A. Neuromarketing: Ethical implications of its use and potential misuse. J. Bus. Ethics 144 , 799–811. https://doi.org/10.1007/s10551-016-3059-0 (2016).
Huang, A. H., Chen, K., Yen, D. C. & Tran, T. P. A study of factors that contribute to online review helpfulness. Comput. Hum. Behav. 48 , 17–27. https://doi.org/10.1016/j.chb.2015.01.010 (2015).
Lotto, M. et al. Characterization of false or misleading fluoride content on instagram: Infodemiology study. J. Med. Internet Res. 24 , e37519. https://doi.org/10.2196/37519 (2022).
Si, H. et al. An effective identification technology for online news comment spammers in internet media. IEEE Access 7 , 37792–37806. https://doi.org/10.1109/access.2019.2900474 (2019).
An, Q., Ma, Y., Du, Q., Xiang, Z. & Fan, W. Role of user-generated photos in online hotel reviews: An analytical approach. J. Hosp. Tour. Manag. 45 , 633–640. https://doi.org/10.1016/j.jhtm.2020.11.002 (2020).
Sankar, H. et al. Intelligent sentiment analysis approach using edge computing-based deep learning technique. Softw. Pract. Exp. 50 , 645–657. https://doi.org/10.1002/spe.2687 (2020).
Lamb, Y., Cai, W. & McKenna, B. Exploring the complexity of the individualistic culture through social exchange in online reviews. Int. J. Inf. Manag. https://doi.org/10.1016/j.ijinfomgt.2020.102198 (2020).
Mariani, M. M. & Nambisan, S. Innovation analytics and digital innovation experimentation: The rise of research-driven online review platforms. Technol. Forecast. Soc. Chang. https://doi.org/10.1016/j.techfore.2021.121009 (2021).
Xiao, S., Chen, G., Zhang, C. & Li, X. Complementary or substitutive? A novel deep learning method to leverage text-image interactions for multimodal review helpfulness prediction. Expert Syst. Appl. https://doi.org/10.1016/j.eswa.2022.118138 (2022).
Naab, T. K., Ruess, H.-S. & Küchler, C. The influence of the deliberative quality of user comments on the number and quality of their reply comments. New Media Soc. https://doi.org/10.1177/14614448231172168 (2023).
Milhomem, A. C. et al. Tissue remodeling after implantation with polymethylmethacrylate: An experimental study in mice. Aesth. Plast. Surg. 47 , 1205–1216. https://doi.org/10.1007/s00266-022-03179-w (2023).
Shah, A. M., Muhammad, W., Lee, K. & Tawalbeh, L. A. Examining the determinants of patient perception of physician review helpfulness across different disease severities: A machine learning approach. Comput. Intell. Neurosci. 2022 , 1–15. https://doi.org/10.1155/2022/8623586 (2022).
Srivastava, P. R., Sharma, D. & Kaur, I. Differential effects of online signals on sales performance of local brand clothing products. J. Enterp. Inf. Manag. 35 , 1522–1547. https://doi.org/10.1108/jeim-01-2020-0039 (2021).
Kurniawan, A. C., Rachmawati, N. L., Ayu, M. M., Ong, A. K. S. & Redi, A. A. N. P. Determinants of satisfaction and continuance intention towards online food delivery service users in Indonesia post the COVID-19 pandemic. Heliyon https://doi.org/10.1016/j.heliyon.2023.e23298 (2024).
Labsomboonsiri, S., Mathews, S., Luck, E. & Mulcahy, R. Recognise me or pay me? How rewards for online restaurant reviews impact continuity: A cross-country investigation. Int. J. Hosp. Manag. https://doi.org/10.1016/j.ijhm.2022.103289 (2022).
Zhou, M. et al. Characterizing Chinese consumers’ intention to use live e-commerce shopping. Technol. Soc. https://doi.org/10.1016/j.techsoc.2021.101767 (2021).
Cui, Y., Mou, J., Cohen, J., Liu, Y. & Kurcz, K. Understanding consumer intentions toward cross-border m-commerce usage: A psychological distance and commitment-trust perspective. Electron. Commer. Res. Appl. https://doi.org/10.1016/j.elerap.2019.100920 (2020).
Wang, S. & Xu, Y. Complex network-based evolutionary game for knowledge transfer of social E-commerce platform enterprise’s operation team under strategy imitation preferences. Sustainability https://doi.org/10.3390/su142215383 (2022).
Cai, X., Cebollada, J. & Cortiñas, M. Impact of seller- and buyer-created content on product sales in the electronic commerce platform: The role of informativeness, readability, multimedia richness, and extreme valence. J. Retail. Consum. Serv. https://doi.org/10.1016/j.jretconser.2022.103141 (2023).
Jia, Q., Li, Y. & Wang, S. Design is more than looks: Research on the affordance of review components on consumer loyalty. Psychol. Res. Behav. Manag. 15 , 3347–3366. https://doi.org/10.2147/prbm.S384024 (2022).
Shiu, J. Y., Liao, S. T. & Tzeng, S.-Y. How does online streaming reform e-commerce? An empirical assessment of immersive experience and social interaction in China. Human. Soc. Sci. Commun. https://doi.org/10.1057/s41599-023-01731-w (2023).
Chatterjee, S., Chaudhuri, R., Kumar, A., Lu Wang, C. & Gupta, S. Impacts of consumer cognitive process to ascertain online fake review: A cognitive dissonance theory approach. J. Bus. Res. https://doi.org/10.1016/j.jbusres.2022.113370 (2023).
Saura, J. R., Palacios-Marqués, D. & Ribeiro-Soriano, D. Leveraging SMEs technologies adoption in the Covid-19 pandemic: A case study on Twitter-based user-generated content. J. Technol. Transf. 48 , 1696–1722. https://doi.org/10.1007/s10961-023-10023-z (2023).
Qi, X., Chang, W., Liu, A., Sun, J. & Fan, M. Exploring the influence of emotionality and expertise on online wine reviews: Does greater knowledge lead to less review?. Int. J. Contemp. Hosp. Manag. https://doi.org/10.1108/ijchm-08-2023-1302 (2024).
Lam, T., Heales, J. & Hartley, N. The role of positive online reviews in risk-based consumer behaviours: An information processing perspective. Aslib J. Inf. Manag. https://doi.org/10.1108/ajim-03-2023-0102 (2023).
Qin, C., Zeng, X., Liang, S. & Zhang, K. Do live streaming and online consumer reviews jointly affect purchase intention?. Sustainability https://doi.org/10.3390/su15086992 (2023).
Alghamdi, J., Luo, S. & Lin, Y. A comprehensive survey on machine learning approaches for fake news detection. Multimed. Tools Appl. https://doi.org/10.1007/s11042-023-17470-8 (2023).
Sharma, S. P., Singh, L. & Tiwari, R. Design of an efficient integrated feature engineering based deep learning model using CNN for customer’s review helpfulness prediction. Wirel. Pers. Commun. https://doi.org/10.1007/s11277-023-10834-1 (2024).
Liu, Y., Ding, X., Chi, M., Wu, J. & Ma, L. Assessing the helpfulness of hotel reviews for information overload: A multi-view spatial feature approach. Inf. Technol. Tour. 26 , 59–87. https://doi.org/10.1007/s40558-023-00280-x (2023).
Liu, C. J. et al. Machine learning-based e-commerce platform repurchase customer prediction model. PLoS ONE https://doi.org/10.1371/journal.pone.0243105 (2020).
Sun, X., Han, M. & Feng, J. Helpfulness of online reviews: Examining review informativeness and classification thresholds by search products and experience products. Decis. Support Syst. https://doi.org/10.1016/j.dss.2019.113099 (2019).
Saumya, S., Singh, J. P., Baabdullah, A. M., Rana, N. P. & Dwivedi, Y. K. Ranking online consumer reviews. Electron. Commer. Res. Appl. 29 , 78–89. https://doi.org/10.1016/j.elerap.2018.03.008 (2018).
Nilashi, M. et al. Analysis of customers’ satisfaction with baby products: The moderating role of brand image. J. Retail. Consum. Serv. 7 , 3. https://doi.org/10.1016/j.jretconser.2023.103334 (2023).
Hong Lee, S., Lee, H. & Hyun Kim, J. Enhancing the prediction of user satisfaction with metaverse service through machine learning. Comput. Mater. Continua 72 , 4983–4997. https://doi.org/10.32604/cmc.2022.027943 (2022).
Nilashi, M. et al. Revealing travellers’ satisfaction during COVID-19 outbreak: Moderating role of service quality. J. Retail. Consum. Serv. https://doi.org/10.1016/j.jretconser.2021.102783 (2022).
Xu, X., Fan, R., Wang, D., Wang, Y. & Wang, Y. The role of consumer reviews in e-commerce platform credit supervision: A signaling game model based on complex network. Electron. Commer. Res. Appl. https://doi.org/10.1016/j.elerap.2023.101347 (2024).
Li, R., Li, Y.-Q., Ruan, W.-Q., Zhang, S.-N. & Wang, M.-Y. Sentiment mining of online reviews of peer-to-peer accommodations: Customer emotional heterogeneity and its influencing factors. Tour. Manag. https://doi.org/10.1016/j.tourman.2022.104704 (2023).
Bu, Z., Li, H., Cao, J., Wu, Z. & Zhang, L. Game theory based emotional evolution analysis for chinese online reviews. Knowl.-Based Syst. 103 , 60–72. https://doi.org/10.1016/j.knosys.2016.03.026 (2016).
Chen, J., Song, N., Su, Y., Zhao, S. & Zhang, Y. Learning user sentiment orientation in social networks for sentiment analysis. Inf. Sci. 616 , 526–538. https://doi.org/10.1016/j.ins.2022.10.135 (2022).
Chen, K., Luo, P. & Wang, H. Investigating transitive influences on WOM: From the product network perspective. Electron. Commer. Res. 17 , 149–167. https://doi.org/10.1007/s10660-016-9241-8 (2016).
Anand, S., Rahul, Mallik, A. & Kumar, S. Integrating node centralities, similarity measures, and machine learning classifiers for link prediction. Multimed. Tools Appl. 81 , 38593–38621. https://doi.org/10.1007/s11042-022-12854-8 (2022).
Banerjee, S. & Chua, A. Y. K. Understanding online fake review production strategies. J. Bus. Res. https://doi.org/10.1016/j.jbusres.2022.113534 (2023).
Kim, D. J., Ferrin, D. L. & Rao, H. R. A trust-based consumer decision-making model in electronic commerce: The role of trust, perceived risk, and their antecedents. Decis. Support Syst. 44 , 544–564. https://doi.org/10.1016/j.dss.2007.07.001 (2008).
Sheth, J. N. & Sharma, A. The impact of the product to service shift in industrial markets and the evolution of the sales organization. Ind. Mark. Manag. 37 , 260–269. https://doi.org/10.1016/j.indmarman.2007.07.010 (2008).
Hennig-Thurau, T., Walsh, G. & Walsh, G. Electronic word-of-mouth: Motives for and consequences of reading customer articulations on the internet. Int. J. Electron. Commer. 8 , 51–74. https://doi.org/10.1080/10864415.2003.11044293 (2014).
Dixit, S., Jyoti Badgaiyan, A. & Khare, A. An integrated model for predicting consumer’s intention to write online reviews. J. Retail. Consum. Serv. 46 , 112–120. https://doi.org/10.1016/j.jretconser.2017.10.001 (2019).
Elliott, D., Grierson, L. E., Hayes, S. J. & Lyons, J. Action representations in perception, motor control and learning: Implications for medical education. Med. Educ. 45 , 119–131. https://doi.org/10.1111/j.1365-2923.2010.03851.x (2011).
Lawrence, D. S., Christoff, T. E. & Escamilla, J. H. Predicting procedural justice behavior: Examining communication and personality. Polic. Int. J. Police Strateg. Manag. 40 , 141–154. https://doi.org/10.1108/pijpsm-07-2016-0107 (2017).
Dreber, A., Fudenberg, D. & Rand, D. G. Who cooperates in repeated games: The role of altruism, inequity aversion, and demographics. J. Econ. Behav. Org. 98 , 41–55. https://doi.org/10.1016/j.jebo.2013.12.007 (2014).
Leonidou, L. C., Leonidou, C. N. & Kvasova, O. Cultural drivers and trust outcomes of consumer perceptions of organizational unethical marketing behavior. Eur. J. Mark. 47 , 525–556. https://doi.org/10.1108/03090561311297445 (2013).
Chaturvedi, I., Cambria, E., Welsch, R. E. & Herrera, F. Distinguishing between facts and opinions for sentiment analysis: Survey and challenges. Inf. Fusion 44 , 65–77. https://doi.org/10.1016/j.inffus.2017.12.006 (2018).
Park, D.-H. & Lee, J. eWOM overload and its effect on consumer behavioral intention depending on consumer involvement. Electron. Commer. Res. Appl. 7 , 386–398. https://doi.org/10.1016/j.elerap.2007.11.004 (2008).
Slyke, C., Shim, J. T., Johnson, R. & Jiang, J. Concern for information privacy and online consumer purchasing. J. Assoc. Inf. Syst. 7 , 415–444. https://doi.org/10.17705/1jais.00092 (2006).
Ru, X., Wang, S., Chen, Q. & Yan, S. Exploring the interaction effects of norms and attitudes on green travel intention: An empirical study in eastern China. J. Clean. Prod. 197 , 1317–1327. https://doi.org/10.1016/j.jclepro.2018.06.293 (2018).
Liu, S. & Rhemtulla, M. Treating random effects as observed versus latent predictors: The bias-variance tradeoff in small samples. Br. J. Math. Stat. Psychol. 75 , 158–181. https://doi.org/10.1111/bmsp.12253 (2022).
Wang, L. et al. GBRT-based estimation of terrestrial latent heat flux in the haihe river basin from satellite and reanalysis datasets. Remote Sens. https://doi.org/10.3390/rs13061054 (2021).
Williams, D. R. & Mulder, J. Bayesian hypothesis testing for Gaussian graphical models: Conditional independence and order constraints. J. Math. Psychol. https://doi.org/10.1016/j.jmp.2020.102441 (2020).
Saadati, K. et al. Evaluation of psychometric properties of the eating restriction questionnaire and food involvement inventory in Iranian adults. Sci. Rep. 13 , 13553. https://doi.org/10.1038/s41598-023-39885-x (2023).
Article ADS CAS PubMed PubMed Central Google Scholar
Nan, D., Lee, H., Kim, Y. & Kim, J. H. My video game console is so cool! A coolness theory-based model for intention to use video game consoles. Technol. Forecast. Soc. Chang. https://doi.org/10.1016/j.techfore.2021.121451 (2022).
Nan, D., Shin, E., Barnett, G. A., Cheah, S. & Kim, J. H. Will coolness factors predict user satisfaction and loyalty? Evidence from an artificial neural network–structural equation model approach. Inf. Process. Manag. https://doi.org/10.1016/j.ipm.2022.103108 (2022).
Kar, M. B., Aruna, M. & Kunar, B. M. Structural equation modelling of work related musculoskeletal disorders among dumper operators. Sci. Rep. https://doi.org/10.1038/s41598-023-40507-9 (2023).
Shahzad, M. F., Xu, S., Khan, K. I. & Hasnain, M. F. Effect of social influence, environmental awareness, and safety affordance on actual use of 5G technologies among Chinese students. Sci. Rep. https://doi.org/10.1038/s41598-023-50078-4 (2023).
Huifeng, P., Ha, H.-Y. & Lee, J.-W. Perceived risks and restaurant visit intentions in China: Do online customer reviews matter?. J. Hosp. Tour. Manag. 43 , 179–189. https://doi.org/10.1016/j.jhtm.2020.04.005 (2020).
Kim, H. J. et al. The significance of pain catastrophizing in clinical manifestations of patients with lumbar spinal stenosis: Mediation analysis with bootstrapping. Spine J. 15 , 238–246. https://doi.org/10.1016/j.spinee.2014.09.002 (2015).
Moussa, K. et al. A comparative study of predicting the availability of power line communication nodes using machine learning. Sci. Rep. 13 , 12658. https://doi.org/10.1038/s41598-023-39120-7 (2023).
Spee, B. T. M. et al. Machine learning revealed symbolism, emotionality, and imaginativeness as primary predictors of creativity evaluations of western art paintings. Sci. Rep. 13 , 12966. https://doi.org/10.1038/s41598-023-39865-1 (2023).
Bornkessel, A., Furberg, R. & Lefebvre, R. C. Social media: Opportunities for quality improvement and lessons for providers-a networked model for patient-centered care through digital engagement. Curr. Cardiol. Rep. 16 , 504. https://doi.org/10.1007/s11886-014-0504-5 (2014).
Jain, P. K., Yekun, E. A., Pamula, R. & Srivastava, G. Consumer recommendation prediction in online reviews using Cuckoo optimized machine learning models. Comput. Electr. Eng. https://doi.org/10.1016/j.compeleceng.2021.107397 (2021).
Liao, Y., Xu, B., Wang, J. & Liu, X. A new method for assessing the risk of infectious disease outbreak. Sci. Rep. 7 , 40084. https://doi.org/10.1038/srep40084 (2017).
Huysman, M. & Wulf, V. IT to support knowledge sharing in communities, towards a social capital analysis. J. Inf. Technol. 21 , 40–51. https://doi.org/10.1057/palgrave.jit.2000053 (2006).
Marvin, H. J. P. et al. A holistic approach to food safety risks: Food fraud as an example. Food Res. Int. 89 , 463–470. https://doi.org/10.1016/j.foodres.2016.08.028 (2016).
Mascaro, S. et al. Modeling COVID-19 disease processes by remote elicitation of causal Bayesian networks from medical experts. BMC Med. Res. Methodol. 23 , 76. https://doi.org/10.1186/s12874-023-01856-1 (2023).
Jin, H. et al. Parallel computation of hierarchical closeness centrality and applications. World Wide Web 22 , 3047–3064. https://doi.org/10.1007/s11280-018-0605-y (2018).
Elmezain, M., Othman, E. A. & Ibrahim, H. M. Temporal degree-degree and closeness-closeness: A new centrality metrics for social network analysis. Mathematics https://doi.org/10.3390/math9222850 (2021).
Feng, Y., Wang, H., Chang, C. & Lu, H. Intrinsic correlation with betweenness centrality and distribution of shortest paths. Mathematics https://doi.org/10.3390/math10142521 (2022).
Liu, J.-R., Guo, S.-Z., Lu, Z.-M., Yu, F.-X. & Li, H. An approximate flow betweenness centrality measure for complex network. IEICE Trans. Inf. Syst. E96.D , 727–730. https://doi.org/10.1587/transinf.E96.D.727 (2013).
Liu, A. & Porter, M. A. Spatial strength centrality and the effect of spatial embeddings on network architecture. Phys. Rev. E 101 , 062305. https://doi.org/10.1103/PhysRevE.101.062305 (2020).
Article ADS CAS PubMed Google Scholar
Abbasi, A., Altmann, J. & Hossain, L. Identifying the effects of co-authorship networks on the performance of scholars: A correlation and regression analysis of performance measures and social network analysis measures. J. Informetr. 5 , 594–607. https://doi.org/10.1016/j.joi.2011.05.007 (2011).
Ringe, N. & Wilson, S. L. Pinpointing the powerful: Covoting network centrality as a measure of political influence. Legis. Stud. Q. 41 , 739–769. https://doi.org/10.1111/lsq.12129 (2016).
Schmidt, M. N. & Morup, M. Nonparametric Bayesian modeling of complex networks: An introduction. IEEE Signal Process. Mag. 30 , 110–128. https://doi.org/10.1109/msp.2012.2235191 (2013).
Hasiloglu, M. & Kaya, O. An analysis of price, service and commission rate decisions in online sales made through E-commerce platforms. Comput. Ind. Eng. https://doi.org/10.1016/j.cie.2021.107688 (2021).
Sheng, Q. et al. Characterizing multi-domain false news and underlying user effects on Chinese Weibo. Inf. Process. Manag. https://doi.org/10.1016/j.ipm.2022.102959 (2022).
Ma, Y., Chen, G. & Wei, Q. Finding users preferences from large-scale online reviews for personalized recommendation. Electron. Commer. Res. 17 , 3–29. https://doi.org/10.1007/s10660-016-9240-9 (2016).
Melchior, C. & Oliveira, M. A systematic literature review of the motivations to share fake news on social media platforms and how to fight them. New Media Soc. 26 , 1127–1150. https://doi.org/10.1177/14614448231174224 (2023).
Zhu, L., Li, H., He, W. & Hong, C. What influences online reviews’ perceived information quality?. Electron. Libr. 38 , 273–296. https://doi.org/10.1108/el-09-2019-0208 (2020).
Long, F. & Liu, Y. Platform manipulation in online retail marketplace with sponsored advertising. Mark. Sci. 43 , 317–345. https://doi.org/10.1287/mksc.2023.1446 (2024).
Bulchand-Gidumal, J. & Melián-González, S. Fighting fake reviews with blockchain-enabled consumer-generated reviews. Curr. Issues Tour. 27 , 739–753. https://doi.org/10.1080/13683500.2023.2173054 (2023).
Wang, Q., Zhang, W., Li, J., Ma, Z. & Chen, J. Benefits or harms? The effect of online review manipulation on sales. Electron. Commer. Res. Appl. https://doi.org/10.1016/j.elerap.2022.101224 (2023).
Arora, A. & Jain, T. Data sharing between platform and seller: An analysis of contracts, privacy, and regulation. Eur. J. Oper. Res. 313 , 1105–1118. https://doi.org/10.1016/j.ejor.2023.09.035 (2024).
Zhang, M., Li, Y., Sun, L., Wang, G. A. & Du, J. The effects of comparative reviews on product sales. J. Interact. Mark. https://doi.org/10.1177/10949968231196578 (2023).
Owusu, G. M. Y. et al. Proposal of a guide for the interpretation, simplification of the regulatory process and good tax compliance, case of digital taxpayers, influencers. PLoS ONE https://doi.org/10.1371/journal.pone.0286617 (2023).
Monsalve-Obreque, P. et al. Proposal to improve the E-commerce platform development process with an exploratory case study in Chile. Appl. Sci. https://doi.org/10.3390/app13148362 (2023).
Mueller, M. L. & Farhat, K. Regulation of platform market access by the United States and China: Neo-mercantilism in digital services. Policy Internet 14 , 348–367. https://doi.org/10.1002/poi3.305 (2022).
Sharma, A. & Shafiq, M. O. A Comprehensive artificial intelligence based user intention assessment model from online reviews and social media. Appl. Artif. Intell. https://doi.org/10.1080/08839514.2021.2014193 (2022).
Binder, M., Heinrich, B., Hopf, M. & Schiller, A. Global reconstruction of language models with linguistic rules—explainable AI for online consumer reviews. Electron. Mark. 32 , 2123–2138. https://doi.org/10.1007/s12525-022-00612-5 (2022).
Li, L., Zheng, H., Chen, D. & Zhu, B. Whose reviews are most valuable for predicting the default risk of peer-to-peer lending platforms? Evidence from China. Electron. Commer. Res. https://doi.org/10.1007/s10660-022-09571-7 (2022).
Handan-Nader, C. Do fake online comments pose a threat to regulatory policymaking? Evidence from Internet regulation in the United States. Policy Internet 15 , 100–116. https://doi.org/10.1002/poi3.327 (2022).
Dai, X., Qin, K. & Wu, L. Study on effect of collaborative governance participation willingness of online food delivery platform restaurants and consumers from perspective of control theory: Based on moderating effects of perceived risks. Front. Psychol. https://doi.org/10.3389/fpsyg.2023.1149538 (2023).
Chen, S. & Chen, Z. Influencing factors of consumers’ participation in express packaging recycling: A questionnaire survey on the current situation of five provinces in China. J. Mater. Cycl. Waste Manag. 26 , 986–1000. https://doi.org/10.1007/s10163-023-01875-6 (2024).
Zhang, W., Zhu, W., Nie, J., Andrasik, F. & Blom, X. N. The effect of emotion background on pathological internet users’ comments on online news: Evidence from online text analysis. Cyberpsychol. J. Psychosoc. Res. Cyberspace https://doi.org/10.5817/cp2022-4-8 (2022).
Li, X. et al. Influencing factors and mechanisms on consumers’ behavior: A case of tea tourism in China. Int. J. Tour. Res. https://doi.org/10.1002/jtr.2637 (2024).
de Gregorio, F., Fox, A. K. & Yoon, H. J. Pseudo-reviews: Conceptualization and consumer effects of a new online phenomenon. Comput. Hum. Behav. https://doi.org/10.1016/j.chb.2020.106545 (2021).
Kim, J. M., Park, K.K.-C., Mariani, M. & Wamba, S. F. Investigating reviewers’ intentions to post fake vs. authentic reviews based on behavioral linguistic features. Technol. Forecast. Soc. Chang. https://doi.org/10.1016/j.techfore.2023.122971 (2024).
Hamed, S. K., Ab Aziz, M. J. & Yaakub, M. R. A review of fake news detection approaches: A critical analysis of relevant studies and highlighting key challenges associated with the dataset, feature representation, and data fusion. Heliyon https://doi.org/10.1016/j.heliyon.2023.e20382 (2023).
Bigné, E., Zanfardini, M. & Andreu, L. How online reviews of destination responsibility influence tourists’ evaluations: An exploratory study of mountain tourism. J. Sustain. Tour. 28 , 686–704. https://doi.org/10.1080/09669582.2019.1699565 (2019).
Liz-López, H. et al. Generation and detection of manipulated multimodal audiovisual content: Advances, trends and open challenges. Inf. Fusion https://doi.org/10.1016/j.inffus.2023.102103 (2024).
Qiu, J. & Wang, S. A deep matching model for detecting reviews mismatched with products in e-commerce. Appl. Soft Comput. https://doi.org/10.1016/j.asoc.2022.109624 (2022).
Román, S., Riquelme, I. P. & Iacobucci, D. Fake or credible? Antecedents and consequences of perceived credibility in exaggerated online reviews. J. Bus. Res. https://doi.org/10.1016/j.jbusres.2022.113466 (2023).
Petrescu, M. et al. Innocent until proven guilty: Suspicion of deception in online reviews. Eur. J. Mark. 56 , 1184–1209. https://doi.org/10.1108/ejm-10-2019-0776 (2022).
Shahraki-Mohammadi, A., Keikha, L. & Zahedi, R. Investigate the relationship between the retraction reasons and the quality of methodology in non-Cochrane retracted systematic reviews: A systematic review. Syst. Rev. https://doi.org/10.1186/s13643-023-02439-3 (2024).
Palese, B., Piccoli, G. & Lui, T.-W. Effective use of online review systems: Congruent managerial responses and firm competitive performance. Int. J. Hosp. Manag. https://doi.org/10.1016/j.ijhm.2021.102976 (2021).
Niechwiej-Szwedo, E., Colpa, L. & Wong, A. M. F. Visuomotor behaviour in amblyopia: Deficits and compensatory adaptations. Neural Plast. 2019 , 6817839. https://doi.org/10.1155/2019/6817839 (2019).
Laszlo, S. & Federmeier, K. D. Never seem to find the time: Evaluating the physiological time course of visual word recognition with regression analysis of single item ERPs. Lang. Cognit. Process 29 , 642–661. https://doi.org/10.1080/01690965.2013.866259 (2014).
Knijnenburg, B. P., Willemsen, M. C., Gantner, Z., Soncu, H. & Newell, C. Explaining the user experience of recommender systems. User Model. User-Adapt. Interact. 22 , 441–504. https://doi.org/10.1007/s11257-011-9118-4 (2012).
Zhang, Y. et al. Money matters? Effect of reward types on customers’ review behaviors. J. Res. Interact. Mark. https://doi.org/10.1108/jrim-01-2023-0027 (2023).
Ai, J., Gursoy, D., Liu, Y. & Lv, X. Effects of offering incentives for reviews on trust: Role of review quality and incentive source. Int. J. Hosp. Manag. https://doi.org/10.1016/j.ijhm.2021.103101 (2022).
Han, Y., Wu, W., Liang, Y. & Zhang, L. Peer grading eliciting truthfulness based on autograder. IEEE Trans. Learn. Technol. 16 , 353–363. https://doi.org/10.1109/tlt.2022.3216946 (2023).
Jeong, J. et al. An empirical investigation of personalized recommendation and reward effect on customer behavior: A stimulus–organism–response (SOR) model perspective. Sustainability https://doi.org/10.3390/su142215369 (2022).
Hwang, Y. & Mattila, A. S. The effect of smart shopper self-perceptions on word-of-mouth behaviors in the loyalty reward program context. J. Hosp. Tour. Res. 46 , 243–266. https://doi.org/10.1177/1096348020985212 (2021).
Bush, G. Attention-deficit/hyperactivity disorder and attention networks. Neuropsychopharmacology 35 , 278–300. https://doi.org/10.1038/npp.2009.120 (2010).
Rogelberg, S. G., King, E. B. & Alonso, A. How we can bring I-O psychology science and evidence-based practices to the public. Ind. Org. Psychol. 15 , 259–272. https://doi.org/10.1017/iop.2021.142 (2022).
Espino-Rodríguez, T. F. & Rodríguez-Díaz, M. The influence of outsourcing activities on the perception of service quality. An empirical study based on online reviews by hotel customers. J. Hosp. Tour. Technol. 12 , 689–711. https://doi.org/10.1108/jhtt-03-2020-0064 (2021).
Shen, A., Wang, P. & Ma, Y. When crowding-in and when crowding-out? The boundary conditions on the relationship between negative online reviews and online sales. Manag. Decis. Econ. 43 , 2016–2032. https://doi.org/10.1002/mde.3505 (2021).
Chang, H. H., Lu, Y.-Y. & Li, P. R. The Yale model of green message sharing and environmental consciousness on social media platforms. Online Inf. Rev. 47 , 333–355. https://doi.org/10.1108/oir-04-2021-0200 (2022).
Guan, Z., Shi, X., Ying, H., Xue, R. & Qiao, X. An empirical study on traditional offline retailer’s switching intention towards community-based group buying program: A push–pull-mooring model. Electron. Mark. https://doi.org/10.1007/s12525-024-00702-6 (2024).
Han, S. & Anderson, C. K. Customer motivation and response bias in online reviews. Cornell Hosp. Q. 61 , 142–153. https://doi.org/10.1177/1938965520902012 (2020).
Xiang, L., Jin, S., Shao, W. & Gao, M. Evaluation of crosstalk in nonlinear regime for weakly coupled multi-core fiber with random perturbations using a split-step numerical model. J. Lightwave Technol. 41 , 5729–5736. https://doi.org/10.1109/jlt.2023.3268457 (2023).
Wickramasingha, S. Geographies of dissociation: Informality, ethical codes and fragmented labour regimes in the Sri Lankan apparel industry. J. Econ. Geogr. https://doi.org/10.1093/jeg/lbad010 (2023).
Yang, Q. & Tang, Y. Big data-based human resource performance evaluation model using Bayesian network of deep learning. Appl. Artif. Intell. https://doi.org/10.1080/08839514.2023.2198897 (2023).
Kudo, H. et al. Bayesian network highlights the contributing factors for efficient arsenic phytoextraction by Pteris vittata in a contaminated field. Sci. Total Environ. 899 , 165654. https://doi.org/10.1016/j.scitotenv.2023.165654 (2023).
Deng, T., Lai, Y. & Tang, C. Does the leader’s negative feedback benefit employees’ creative process engagement? The mediating effect of impression management motivation. Leadersh. Organ. Dev. J. 44 , 103–119. https://doi.org/10.1108/lodj-12-2021-0571 (2023).
Fjendbo, T. H. Leading employees of different genders: The importance of gender for the leadership-motivation relationship. Rev. Public Pers. Adm. 41 , 651–673. https://doi.org/10.1177/0734371x20925520 (2020).
Guenther, B., Galizzi, M. M. & Sanders, J. G. Heterogeneity in risk-taking during the COVID-19 pandemic: Evidence from the UK lockdown. Front. Psychol. https://doi.org/10.3389/fpsyg.2021.643653 (2021).
Download references
Acknowledgements
This research is supported by grants from National Natural Science Foundation of China (NSFC) (72064027), National Natural Science Foundation of China (NSFC) (72364024), and Jiangxi Province Philosophy and Social Science Key Research Base (22SKJD05 & 23ZXSKJD29).
Author information
Authors and affiliations.
School of Public Policy and Administration, Nanchang University, Nanchang, 330031, China
Chunnian Liu, Xutao He & Lan Yi
Digital Literacy and Skills Enhancement Research Center, Jiangxi Province Philosophy and Social Science Key Research Base, Nanchang University, Nanchang, 330031, China
You can also search for this author in PubMed Google Scholar
Contributions
C.L. designs the study and writes the protocol. X.H. manages the data collection and writes the first draft of the manuscript. L.Y. undertakes the statistical analysis. All authors contribute to and approve the final manuscript.
Corresponding author
Correspondence to Lan Yi .
Ethics declarations
Competing interests.
The authors declare no competing interests.
Additional information
Publisher's note.
Springer Nature remains neutral with regard to jurisdictional claims in published maps and institutional affiliations.
Rights and permissions
Open Access This article is licensed under a Creative Commons Attribution 4.0 International License, which permits use, sharing, adaptation, distribution and reproduction in any medium or format, as long as you give appropriate credit to the original author(s) and the source, provide a link to the Creative Commons licence, and indicate if changes were made. The images or other third party material in this article are included in the article's Creative Commons licence, unless indicated otherwise in a credit line to the material. If material is not included in the article's Creative Commons licence and your intended use is not permitted by statutory regulation or exceeds the permitted use, you will need to obtain permission directly from the copyright holder. To view a copy of this licence, visit http://creativecommons.org/licenses/by/4.0/ .
Reprints and permissions
About this article
Cite this article.
Liu, C., He, X. & Yi, L. Determinants of multimodal fake review generation in China’s E-commerce platforms. Sci Rep 14 , 8524 (2024). https://doi.org/10.1038/s41598-024-59236-8
Download citation
Received : 12 October 2023
Accepted : 08 April 2024
Published : 12 April 2024
DOI : https://doi.org/10.1038/s41598-024-59236-8
Share this article
Anyone you share the following link with will be able to read this content:
Sorry, a shareable link is not currently available for this article.
Provided by the Springer Nature SharedIt content-sharing initiative
By submitting a comment you agree to abide by our Terms and Community Guidelines . If you find something abusive or that does not comply with our terms or guidelines please flag it as inappropriate.
Quick links
- Explore articles by subject
- Guide to authors
- Editorial policies
Sign up for the Nature Briefing newsletter — what matters in science, free to your inbox daily.

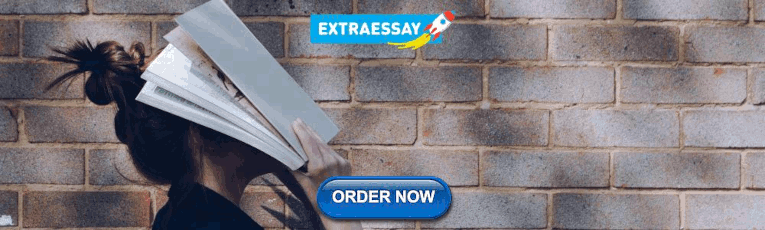
COMMENTS
A Review of the Theoretical Literature" (Theoretical literature review about the development of economic migration theory from the 1950s to today.) Example literature review #2: "Literature review as a research methodology: An overview and guidelines" (Methodological literature review about interdisciplinary knowledge acquisition and ...
The theoretical framework (or foundation of theory) The empirical research; The research gap; The closing section; We then progress to the sample literature review (from an A-grade Master's-level dissertation) to show how these concepts are applied in the literature review chapter. You can access the free resources mentioned in this video below.
The current article proposes a format for conducting a theoretical literature review, using path-goal leadership theory and the learning organization as two examples to showcase the benefits of ...
The literature review sample and detailed advice on writing and conducting a review will help you produce a well-structured report. But remember that a literature review is an ongoing process, and it may be necessary to revisit and update it as your research progresses. ... Theoretical Review: A theoretical review examines the literature based ...
2. MOTIVATE YOUR RESEARCH in addition to providing useful information about your topic, your literature review must tell a story about how your project relates to existing literature. popular literature review narratives include: ¡ plugging a gap / filling a hole within an incomplete literature ¡ building a bridge between two "siloed" literatures, putting literatures "in conversation"
A literature review is a survey of scholarly sources on a specific topic. It provides an overview of current knowledge, allowing you to identify relevant theories, methods, and gaps in the existing research. There are five key steps to writing a literature review: Search for relevant literature. Evaluate sources. Identify themes, debates and gaps.
Conducting a literature review, selecting a theoretical framework, and building a conceptual framework are some of the most difficult elements of a research study. It takes time to understand the relevant research, identify a theoretical framework that provides important insights into the study, and formulate a conceptual framework that ...
Quality research is about building onto the existing work of others, "standing on the shoulders of giants", as Newton put it.The literature review chapter of your dissertation, thesis or research project is where you synthesise this prior work and lay the theoretical foundation for your own research.. Long story short, this chapter is a pretty big deal, which is why you want to make sure ...
Theoretical Review. This form aims to examine the corpus of theory accumulated regarding an issue, concept, theory, and phenomenon. The theoretical literature review helps to establish what theories exist, the relationships between them, the degree the existing approaches have been investigated, and to develop new hypotheses to be tested.
The current article proposes a format for conducting a theoretical literature review, using path-goal leadership theory and the learning organization as two examples to showcase the benefits of using a theoretical literature review. Incorporating theoretical literature reviews to some of the AHRD's journals could help in identifying and ...
Theoretical: In many humanities articles, the literature review is the foundation for the theoretical framework. You can use it to discuss various theories, models, and definitions of key concepts. You can argue for the relevance of a specific theoretical approach or combine various theorical concepts to create a framework for your research.
A literature review may consist of simply a summary of key sources, but in the social sciences, a literature review usually has an organizational pattern and combines both summary and synthesis, often within specific conceptual categories.A summary is a recap of the important information of the source, but a synthesis is a re-organization, or a reshuffling, of that information in a way that ...
The key here is to focus first on the literature relevant to the puzzle. In this example, the tokenism literature sets up a puzzle derived from a theory and contradictory empirical evidence. Let's consider what each of these means... The literature(s) from which you develop the theoretical/empirical puzzle that drives your research question.
Sue's example illustrates that carrying out a comprehensive literature review is a required step in any research project. First, a researcher cannot conduct the study. 1. without gaining a deep understanding of the research topic and learning from the work of other scholars and researchers in the field (Creswell, 2018).
Demonstrate your knowledge of the research topic. Identify the gaps in the literature and show how your research links to these. Provide the foundation for your conceptual framework (if you have one) Inform your own methodology and research design. To achieve this, your literature review needs a well-thought-out structure.
Steps for Conducting a Lit Review; Finding "The Literature" Organizing/Writing; APA Style This link opens in a new window; Chicago: Notes Bibliography This link opens in a new window; MLA Style This link opens in a new window; Sample Literature Reviews. Sample Lit Reviews from Communication Arts; Have an exemplary literature review? Get Help!
This essay starts with a discussion of the literature review, theoretical framework, and conceptual framework as components of a manuscript. This discussion includes similarities and distinctions ...
Literature reviews offer a critical synthesis of empirical and theoretical literature to assess the strength of evidence, develop guidelines for practice and policymaking, and identify areas for future research.1 It is often essential and usually the first task in any research endeavour, particularly in masters or doctoral level education. For effective data extraction and rigorous synthesis ...
A theoretical review of related literature for example, may also look to break down geography or issues pertaining to a single country into its different parts or along rural and urban divides. Organize the Literature: Group the literature into thematic clusters based on the identified themes.
A literature review is a compilation of current knowledge on a particular topic derived from the critical evaluation of different scholarly sources such as books, articles, and publications, which is then presented in an organized manner to relate to a specific research problem being investigated. It highlights the methods, relevant theories, and gaps in existing research on a particular ...
Theoretical Framework and Literature Review We are on the cusp of a completely "new era", and changes must be made in education to ensure that all students leave school prepared to face the challenges of a redefined world. (Thornburg, 2000) 2.1 Introduction Collaborative learning through structured blending of online tutorials, and lecture
The following literature review contributes to the intention of the research framing the groundwork for future research about the role these leaders will play in the long-term sustainability of HBCUs. In doing so review of the literature on measures of GN include test identifications describing the purpose of the test, settings, and who uses them.
This paper develops a theoretical model of determinants influencing multimodal fake review generation using the theories of signaling, actor-network, motivation, and human-environment ...