Image Steganography: A Review of the Recent Advances
Ieee account.
- Change Username/Password
- Update Address
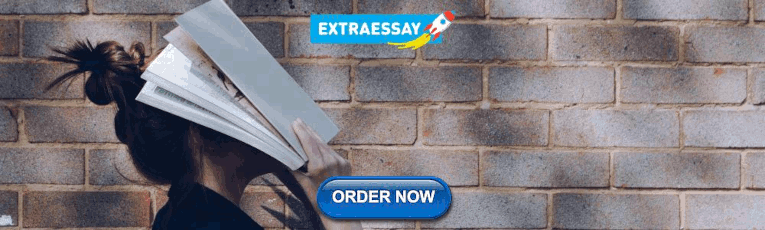
Purchase Details
- Payment Options
- Order History
- View Purchased Documents
Profile Information
- Communications Preferences
- Profession and Education
- Technical Interests
- US & Canada: +1 800 678 4333
- Worldwide: +1 732 981 0060
- Contact & Support
- About IEEE Xplore
- Accessibility
- Terms of Use
- Nondiscrimination Policy
- Privacy & Opting Out of Cookies
A not-for-profit organization, IEEE is the world's largest technical professional organization dedicated to advancing technology for the benefit of humanity. © Copyright 2024 IEEE - All rights reserved. Use of this web site signifies your agreement to the terms and conditions.
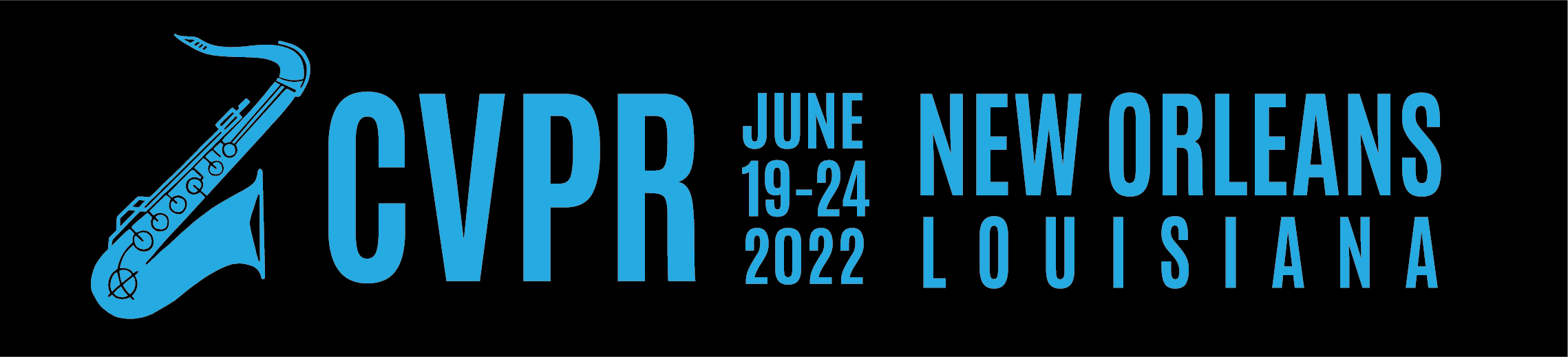
Powered by:
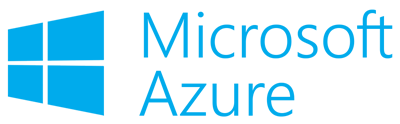
Sponsored by:
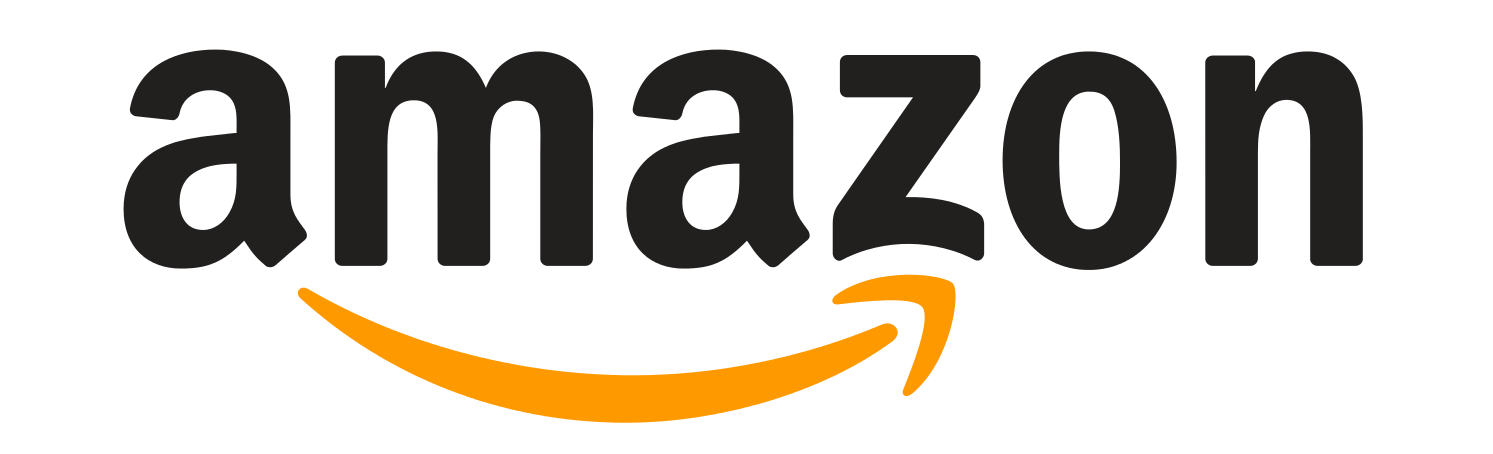
Thank you for visiting nature.com. You are using a browser version with limited support for CSS. To obtain the best experience, we recommend you use a more up to date browser (or turn off compatibility mode in Internet Explorer). In the meantime, to ensure continued support, we are displaying the site without styles and JavaScript.
- View all journals
- My Account Login
- Explore content
- About the journal
- Publish with us
- Sign up for alerts
- Open access
- Published: 30 August 2023
A Huffman code LSB based image steganography technique using multi-level encryption and achromatic component of an image
- Shahid Rahman 1 ,
- Jamal Uddin 1 ,
- Hameed Hussain 2 ,
- Aftab Ahmed 3 ,
- Ayaz Ali Khan 4 ,
- Muhammad Zakarya 3 , 5 ,
- Afzal Rahman 2 &
- Muhammad Haleem 6
Scientific Reports volume 13 , Article number: 14183 ( 2023 ) Cite this article
1972 Accesses
1 Citations
Metrics details
- Data processing
- Image processing
In the recent couple of years, due to the accelerated popularity of the internet, various organizations such as government offices, military, private companies, etc. use different transferring methods for exchanging their information. The Internet has various benefits and some demerits, but the primary bad mark is security of information transmission over an unreliable network, and widely uses of images. So, Steganography is the state of the art of implanting a message in the cover objects, that nobody can suspect or identify it. Therefore, in the field of cover steganography, it is very critical to track down a mechanism for concealing data by utilizing different blends of compression strategies. Amplifying the payload limit, and robustness, and working on the visual quality are the vital factors of this research to make a reliable mechanism. Different cover steganography research strategies have been recommended, and each adores its benefits and impediments but there is a need to foster some better cover steganography implements to accomplish dependability between the essential model of cover steganography. To handle these issues, in this paper we proposed a method in view of Huffman code, Least Significant Bits (LSB) based cover steganography utilizing Multi-Level Encryption (MLE) and colorless part (HC-LSBIS-MLE-AC) of the picture. It also used different substitution and flicking concepts, MLE, Magic matrix, and achromatic concepts for proving the proficiency, and significance of the method. The algorithm was also statistically investigated based on some Statistical Assessment Metrics (SAM) such as Mean Square Error (MSE), Peak Signal Noise Ratio (PSNR), Normalized Cross Correlation (NCC), Structural Similarity Index Metric (SSIM), etc. and different perspectives. The observational outcomes show the likelihood of the proposed algorithm and the capacity to give unwavering quality between security, payload, perception, computation, and temper protection.
Similar content being viewed by others
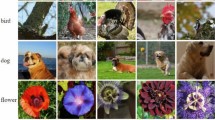
Super-resolution deep neural network (SRDNN) based multi-image steganography for highly secured lossless image transmission
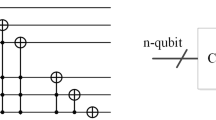
Traceable Quantum Steganography Scheme Based on Pixel Value Differencing
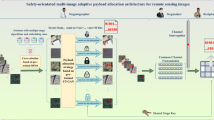
Security-oriented steganographic payload allocation for multi-remote sensing images
Introduction.
For data transmission, the Internet has become an excellent system, due to its accelerated popularity, inexpensiveness, and efficiency of it 1 . Therefore, for sharing information in digital form over the Internet, one file image is widely used and becomes very easy to send over the Internet 2 . In addition, it is very easy to copy or modify the transmitted data over the internet by unauthorized persons or attackers. There are various identified tools available to make the exploitation of secret information, security, privacy, etc. being transmitted and also to make the possibility of different vulnerabilities, attacks, and hateful threats such are scaling, cropping, tempering, spoofing, phishing, eavesdropping, privilege escalation, clickjacking, social engineering, bot, backdoor, viruses, botnet, malware and many more 3 , 4 .To deal with secure correspondence over the Internet, various techniques are proposed in the recent couple of years but each has related pros and cons. Thus, to satisfy the requirement for secure correspondence, it is important to make superior ways of making a safe framework to satisfy the requirement for transmission over the web between users. However, the ancient method used for secure communication in order to provide a safe way of transferring data over the internet is Encryption 5 , 6 , 7 . It converts normal text means plain text to cipher text using some keys. So, encryption is a way of converting plain text into cipher text within any cover media used for encryption, that there is no clue of the existing data and no one can suspect the encrypted information. Furthermore, the recipient might involve a mystery key for encryption and just the key supervisors can unscramble the mystery message utilizing the key which is to be given by the source. Using the encryption concepts there are some multimedia information mediums used such are image, text, audio, video, network, etc. 8 .
Therefore, the main idea of encryption is the notion of steganography which hides secret information within the cover objects without knowing the existence of the information within it. Steganography is the part of data concealing that encodes the mystery message that nobody can identify it. Image steganography shows an essential part of protected communication in this digital world because one file type that is rapidly used is the image 9 . Furthermore, image steganography uses an image as a cover object and inserts the secret information using different reported methods such as spatial domain, frequency domains, etc. The extensive surveys of image steganography are presented in various works as described later in “ Summary of the related works ” 10 , 11 , 12 , 13 , 14 . Every existing method has its own advantages and disadvantages in terms of payload, security, perception, temper protection, and computation which are the basic criteria of steganography, as well as, the basic needs for any steganographic methods as shown in Fig. 1 6 .
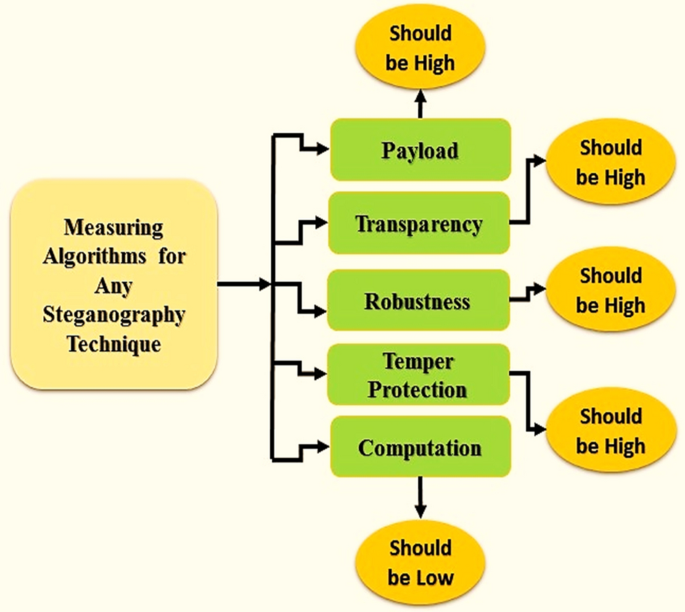
The need for image steganography techniques.
Though, we have proposed an improved and novel technique based on Multi-Level Encryption (MLE), an achromatic component of an image, and Huffman LSB. The proposed method also used some transposition, and magic matrix concepts to expand the significance and inspiration of the proposed technique. For embedding the secret message, the proposed algorithm used the Huffman coding priority concept and made the Huffman encrypted sequences, and then embedded them into the cover media. After making the Huffman encrypted sequences, different encrypted operations applied to it made the method outperform others. This is because the proposed algorithm used an embedded procedure of the cover data within the image in a manner that if anyone suspects the stego image or extracts the message, then they will not extract the encoded message version. Only the holders of the Huffman table or tree can regain the secret message correctly because the communication is between the two parties. Therefore, the proposed research work has a novel and improved contribution in terms of security, transparency, payload, and temper protection in order to make it significant. Some main objectives and contributions of the said method are given as follows.
A proposed technique that uses the Huffman coder priority for encrypting the secret message and the result is the form of Huffman encrypted sequences to improve the reliability between basic criteria such as temper protection and security.
Achromatic components of an image Hue Saturation Intensity (HSI) variety model are utilized rather than the RGB pictures variety model to decrease the handling time and increment the security.
For making deciphering the mystery message testing, and thought-provoking, the proposed method divided the I channel or plane (I-Plane) into four equal blocks and shuffled the blocks using Magic Matrix, and the method also used MLE for giving the tough time to attackers and to increase the security.
The proposed strategy was fundamentally dissected in the opinion of various key view-points (i.e. different sizes of images with different sizes of text, different images with the same text size, and different format images) to work on the proficiency and viability of the scheme.
In sum-up, the basic criterion of cover steganography is presented in Fig. 1 , which elaborates the payload maximum amount of furtive data to be implanted within the cover object. Transparency shows the quality of the image. Furthermore, robustness spectacles the retreat of the stego image, that the stego object is undamaged after embedding even if attacked. shows the opposition against various assaults of the cover object. While computation explains the time intricacy of the implanting system into the cover object 15 . The rest of the paper is organized into various sub-sections as follows. Section 2 elaborates on the abstract details of the related reported research works. Section 3 explains the proposed methodology and algorithms. Sections 4 describes experimental parameters and obtained results. Finally, Sect. 5 concludes this work along with directions for future work.
Summary of the related works
For secure communication between sender and receiver in this digital world over the internet, cover steganography plays a vital role and flourishing research area. For digital steganography, many research works have been proposed over the last decades such as LSB, pixel value differencing, randomization, cyclic, etc. and each has their related pros and cons. Notably, for inserting the mystery message inside the cover media the most well-known and habitually utilized strategy is the LSB technique 5 . The wide use of this technique is due to its simplicity and straightforwardness. Therefore, this section elaborates on the basic concepts of RGB and HSI color model, Least Significant Bit (LSB), and critical analysis of some methods presented in the literature 16 . However, how about we make sense of RGB and HSI variety models; one of the main parts of any item is its tone.
The utilization of variety in picture handling is persuaded by standard two aspects.
From a scene, that often streamlines object identification and abstraction, a color is a powerful descriptor.
Contrasted with about just too many shades of gray the people can discern thousands of color shades and intensities.
The second is predominantly significant in physical image analysis (i.e. when performed by people). The purpose of a color model or color system is to facilitate the specification of colors in some standard, and generally accepted way when working in image processing because it is the main part of it. Each color tone is addressed by a solitary point because color mode is a description of a coordinate system and subspace within that system. In practice most commonly used color models; Red, Green, Blue (RGB) model used for monitors and a broad class of color video cameras because it is the hardware-oriented models in terms of digital image processing. For printing the second color model used is Cyan, Magenta, Yellow and Black (CMYK), Cyan, Magenta, Yellow (CMY). To correspond closely with the way humans, describe and interpret color, the third color model Hue, Saturation, Intensity (HSI) is used. So, the main focus of this study is the HSI model. In HSI color Model, changing from one model to the other is an open process while creating colors in the RGB and CMY models 17 . For hardware implementations, this ideally suited this color system. The RGB framework coordinates pleasantly with the way that the natural eye is firmly viewpoint to red, green, and blue primaries. Yet, the RGB, CMY, and other comparable variety models are not appropriate for depicting colors in wording that are pragmatic for human translation.
For instance, one doesn't allude to the shade of color by giving the level of every one of the primaries creating its tone. Besides, we don't consider a variety of pictures as being made out of three essential pictures that join to frame that solitary picture 18 . So, we describe by its hue, saturation, and brightness, when humans view a color object. Hue is a color aspect that defines a clean color (i.e. Yellow, red, and orange), while saturation gives a measure of the degree to which a pure color is diluted by a white light. Whereas intensity is a particular descriptor that is basically difficult to gauge. It is one of the key aspects in describing the color sensation, and it also exemplifies the colorless thought of intensity. This quantity absolutely is measurable and simply interpretable because it is the utmost convenient descriptor of monochromatic images. In a color image, the HSI model decouples the intensity component from the color-carrying information (hue and saturation). Based on color descriptions HSI color model is an ideal tool for developing image processing algorithms because they are naturally intuitive to humans. For color generation, we can say that RGB is an ideal tool, but for color description, it is significantly more restricted. Hereafter, from the RGB image, we should be capable to excerpt intensity 19 , 20 . So, the HSI color model plays a vital role in secret information camouflage because the Intensity plane (I Plane) does not grieve the other planes, unique RGB diverts in which all planes are unequivocally co-related with one another. Besides, handling an image in the HSI model is somewhat more economical based on LSB-based techniques, etc. due to its unique properties. So, LSB is the process of embedding the secret message into cover image pixels of LSBs either randomly or sequentially shown in Fig. 2 3 , 9 , 21 . The given figure explores the concept of LSB by taking the cover image converting it into its corresponding American Standard Code Information Interchange (ASCII) values then converting it into binary values 22 . After reading the image pixels’ values and their binary forms it converts the secret message values to binary form 23 . Furthermore, it is the procedure of implanting secret data bit K replaced with cover image pixels K LSB. Equation ( 1 ) represents the embedding process.
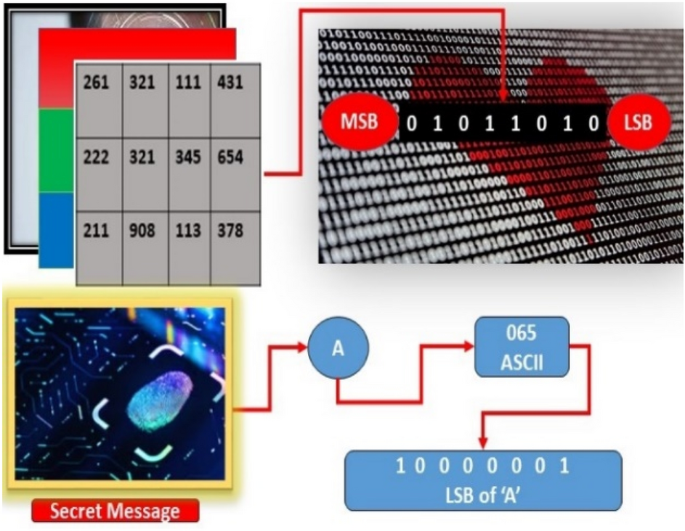
The basic concept of least significant bit (LSB).
Where SIM (i, j) presents the stego image i, where j represents row and column, CI is the cover image, and SM secret message. Different LSB-based methods are proposed recently but they still have issues to improve this research area. The human eye is very naked and has the properties to detect or suspect little change in any smooth area of the images 24 . So, encrypting the secret message with the image either randomly or sequentially, and not all pixel values are used for embedding so the change may be happening on only the embedding area of the image. Therefore, various image steganographic reported works are adapted to embed a suitable amount of message in appropriate cover objects 25 .
Lee et al. recommended a high implanting message-based image steganography where they embedded 12 KB of cover information inside the cover image and the technique focused only on the payload 26 . Zang and Wang et al. introduced proficient steganographic inserting by taking advantage of the alteration course. The author focused on embedding more messages and also try to get security 27 .
Khan et al. offered a cyclic image-based steganography method using randomization. In this paper, authors struggled to get consistency among the basic criteria and embedded 4 to 8 KB secret messages. Karim suggested a new image steganography technique in light of LSB using a secret key and achieving the security, payload but broke down the other criteria 28 . Muhammad and Sajjad proposed and magic LSB method using multi-level encryption and HSI image. It is a better technique in image steganography but has some limitations to different attacks such as scaling, noising, cropping, etc. 29 .
Rustad et al. developed a novel image steganography for improving the perceptual transparency of the image. It is an inverted LSB method using an adaptive pattern. This research achieved a high-quality stego image but the payload limit is too low 30 .
Cheng and Huang proposed a novel method of reversible encrypted image-based steganography using interpolation image and histogram shifting. In this study, the author used double scrambles operation on the pixel of the image by changing the positions of the pixels. The experimental results achieved a high embedding capacity and security 31 .
Shwe Sin et al. presents an LSB and Huffman code-based steganography. The investigational outcomes presented that the algorithm got high embedding capacity and better security 32 .
Tsai et al. presented another LSB-based image steganography algorithm in light of MSB prediction and the Huffman base method. The main objectives of the paper are high payload and secrecy and to increase the inserting pace of the mystery message inside the cover picture 33 .
M. Shahu et al. presented a novel technique, LBP-based reversible information stowing away effectively accomplishes better HC, SI quality, and strength to different assaults. Be that as it may, besides these benefits, the proposed method can be improved concerning HC. Since the proposed work utilizes the LBP-based strategy, subsequently, it considers the surface and smooth pictures as unclear while embedding the EBs. Regardless, the pixel power of surface pictures makes it more sensible to introduce more EBs when appeared differently in relation to smooth pictures. Thusly, the proposed work can be contacted to achieve higher HC in surface pictures by brushing LBP with the PVDS-based technique 34 .
Dhivya et al. proposed a proficient variable bit information embedding method founded on combined chaotic system (CCS) and integer wavelet transform (IWT). With the plan to boost the security level, CCS is created by joining chaotic maps of two 1D. The developed combined tent-logistic (CTL), combined sine-logistic (CSL) and combined sine-tent (CST) maps with improved chaotic behavior are utilized to generate the key sequences. From CTL, CSL and CS, these chaotic key sequences are then quantized to embed the secret bits in high-high, high-low and low–high sub-bands of the IWT transformed cover image, respectively. The fundamental benefit of this plan is that the number of bits to be implanted in every single sub-band coefficient is profoundly chaotic and very delicate to the underlying seeds 35 .
Ramapriya et al. proposed a clinical picture steganography technique by taking advantage of Double Tree-Complex Wavelet Change based change and picture encryption system. Then a better SSOA enhancement calculation is locked in to distinguish smooth edge blocks. Subsequently, the determination of pixels for inserting is worked with. Installing the Restricted information into the cover picture is then done utilizing a twofold network XOR encoding. After the inserting system, the stego picture is created. Subsequently, the proposed strategy shows the best outcomes with high pay load limit, security, and picture quality than the current techniques. Testing was performed on PSNR, MSE, IF, and SSIM measurements to confirm the presentation of the proposed techniques 36 .
Sahu et al. proposed a better method for two delicate watermarking plans to perform altering recognition and limitation in a picture. The proposed plans were outwardly disabled and the watermark pieces were created using the turbulent system-based determined map at both the source and getting end. Further, the chief contrives saw a constraint of ± 1 contrast between the host and watermarked pixels. As such, the idea of the watermarked picture was far superior to that of various plans. Finally, surprising results were achieved in regard to the adjusted area and limitation limit of the proposed plans. Later on, the proposed work can be connected with self-recovery of the modified pieces, and growing both spatial and change spaces to extra overhaul the force. There are different reported works presented in the literature based on image steganography, and each method has its related advantages and disadvantages depending on its embedding procedures and selection of the cover object 36 . Moreover, every examination work has attempted to cover the rudiments standards of picture steganography such are payload, strength, discernment, temper insurance, and computation. Some examination of existing techniques is introduced in Table 1 .
In sum-up, Table 1 illustrated the analysis of the diverse existing methods using the basic criterion of steganography. It also expounded the techniques used, pros and cons, main focus, embedding procedure, and limitations of each method. Though, after a detailed analysis of the existing methods many points come to mind, but some point is very vital; one is selecting an appropriate cover object and the second is the reliability between the essential criterion of steganography. Because some tried for making a reliable method but get one or two parameters but broke down the other criteria and also the reliability between the criterion which is an essential part of steganography. However, to tackle these vital needs, the proposed algorithm is designed in a manner that shows which dimension of the image is better for which size of secret message and also to make a reliable method. However, the detailed whole process of the proposed algorithm is presented in the next section.
The proposed algorithms
This section presents the whole process of the proposed algorithm, embedding and extraction process, Magic matrix, MLEA, Huffman encryption, and extraction process shown in Fig. 3 . The mathematical notations which are used throughout the paper are presented in Table 2 .
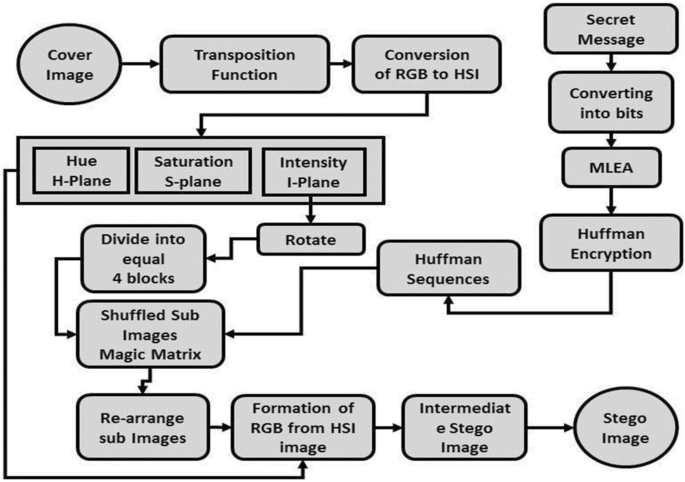
The process of encrypted algorithm used in the proposed method.
The step-by-step procedures of embedding, the extraction process, MLEA, Magic Matrix (MgMtx), and Huffman code algorithms are presented given in Algorithms 2, 3, 4, and 5 respectively. The proposed algorithm used two stages in which first we encrypt the mystery message using Huffman code priority. For mapping the one secret word to one code word the optimal codes are Huffman code mapping. Since Huffman designates a parallel worth or code to each brightness of the cover picture. For applying the Huffman code, first, we convert the 2D image with size C × R to 1D array bits’ stream with length of LHuff fewer than or equal to C × R (LHuff ≤ C × R). The given bit’s stream is used for building the Huffman table or sequences HFCS because extracting the original message bits’ stream is totally dependent on the same Huffman building table bits’ stream. To lessen the size of the cover picture performs lossless pressure of the Huffman inserting is utilized. After the size of that image is reduced because Huffman encrypts shrinkages the image size. In the context of embedding, Huffman encrypted bit stream cannot reveal or show the message bits of the cover image or anything about it. Huffman is one type of verification because the secret message bit stream is only extracted using the same Huffman table or sequences which are used for embedding. If a little bit of error occurs the Huffman table or sequences can’t extract and is unable to recover the secret message. The proposed method used MLEA, Magic Matrix, and Huffman code-based encryption to prove the efficiency, effectiveness, and dominancy of the proposed. Let’s first elaborate on numerous important concepts which are used in the proposed algorithm.
The Multi-Level Encryption (MLE) is used for the proposed algorithm having different encryption operations to improve the security and give hardness to the attackers 49 , 53 . It is used on secret data before embedding to the cover object or image for increasing security using some XOR operation to make the message unrecoverable for any assailants. While Magic matrix is used to make a matrix having no repeated numbers and the summation of that matrix either each row, each column, and both diagonals are remaining the same. It can be used for applying different operations on secret data or cover images due to its properties in terms of improving security and also giving the hard-hitting time to attackers shown in Figs. 4 , 5 , 6 , 7 and 8 sequentially 47 , 48 , 54 . Let’s try to elaborate with the help of the example given below.
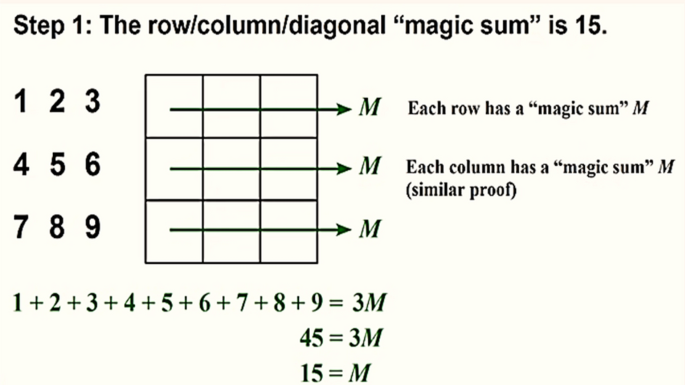
An example of the magic matrix (Steps: 1 & 2).
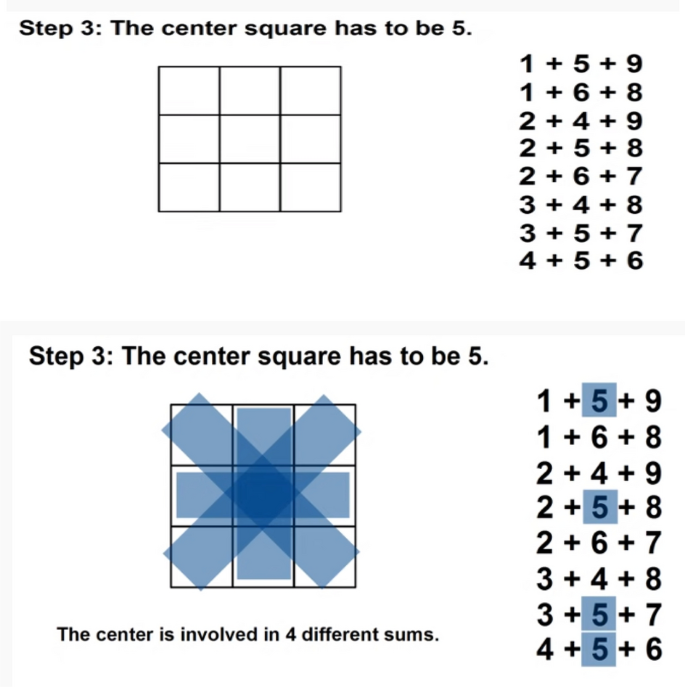
An example of the magic matrix example (Step: 3).
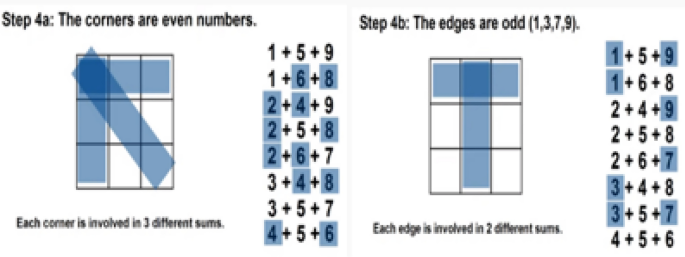
An example of the magic matrix example (Steps: 4a & 4b).
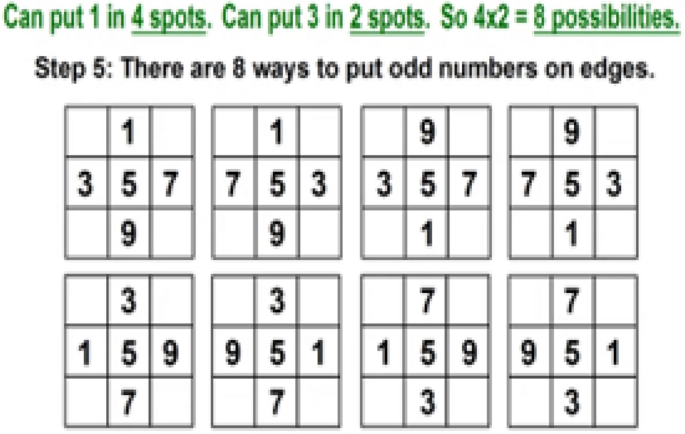
An example of the magic matrix example (Step: 5).
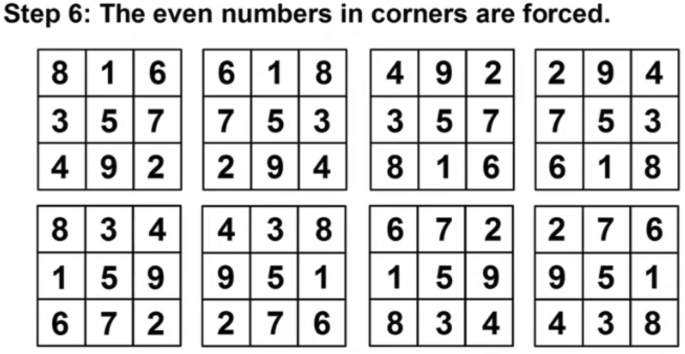
An example of the magic matrix example (Step: 6).
However, the Magic matrix is a special type of MATLAB function used for applying different shuffling, rotation, or reflecting properties. The above example in Figs. 4 , 5 , 6 , 7 and 8 respectively shows us the detailed explanation of the matrix by taking the magic matrix 3 by 3 and the required magic sum is 15. Now perform different calculations on the matrix to make the matrix magic. Either trying from the center point, starting left–right corners, or even–odd numbers to make the matrix magic. So take the value in terms of 3 by 3 and make the summation diagonally, each row and column, and check the properties of the magic matric, if found correct then the matrix is said to be magic shown in Figs. 5 , and 6 . The above example from steps 1–6 elaborates on the step by steps method that how to making the matrix magic. You can see in the above Fig. 6 , taking the square center to be 5 but the center involved 4 different sums, and if we take even numbers from the corners then each corner involved 3 different sums shown in step 4. And if we take the edges to be odd numbers then each sums involved 2 different sums. Now try to with some other numbers; suppose can we put 1 in 4 spots, or put 3 in 2 spots, so if we 2 multiply 4 then 8 possibilities occur. So there are 8 possibilities to put odd numbers on edges because we tried different ways but the result is not near to the magic matrix properties with odd numbers the different sums are low so we take the odd edges shown in step 5. Finally, the even numbers are placed at corners, and the odd numbers are placed on edges.
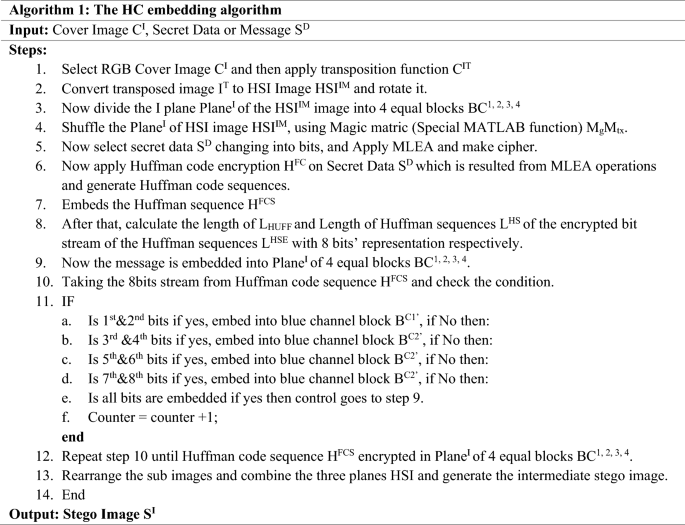
Results and discussion
The section demonstrates the investigational results based on different perspectives, and some statistical assessment matrices to demonstrate the effectiveness and enactment of the planned work. For analysis, we used some standard images namely, Lena, Mandrill, Girl, etc. for the analysis of the method shown in Fig. 9 . It is also critically analyzed in relation to safety and enactment analysis based on three distinct perspectives shown in Fig. 10 .
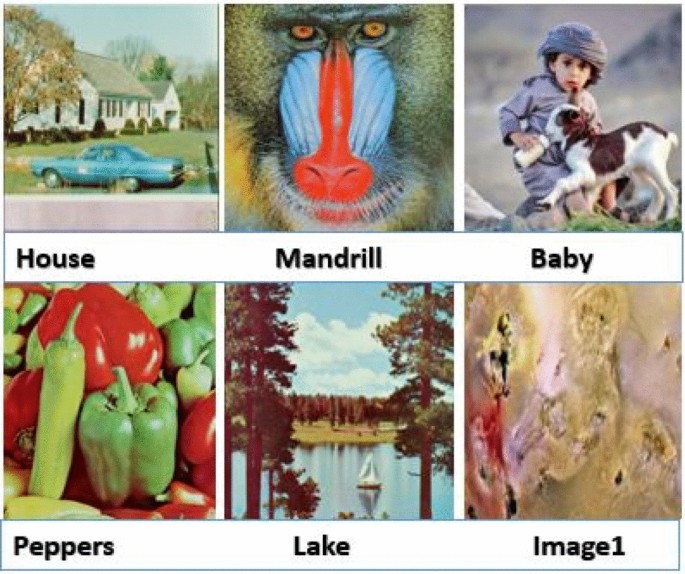
Datasets of standard images 70 , 71 .
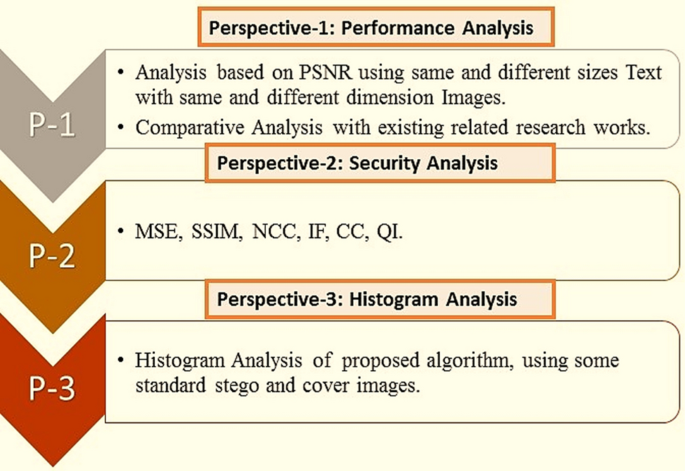
Experimental evaluation perspectives for the proposed algorithm.
Before going to the performance and security analysis of the proposed algorithm, we first explain the Quality assessment metrics used for critical analysis of the proposed algorithm shown in Table 3 55 , 56 , 57 .
Perspective 1
The performance analysis using P1 is given as follows. Using P1 the planned work is evaluated on different images of distinct dimensions with 14 kb’s secret message. Other hand, it is also analyzed based on different sizes of secret messages embedded into similar dimension images. So, the results demonstrate the connotation of the technique in view of PSNR values displayed in Table 4 . While Table 5 shows the performance of the proposed method compared with relevant existing methods using PSNR values which 6.22% outer performed.
Perspective 2
Security Analysis of the Proposed algorithm based on P2 is given. Table 6 elaborate the significance, resistance against to different attacks, quality, and performance of the propose method using different quality assessment parameters.
Results analysis
In this part, we described some enactment of the proposed method. Figure 11 a and d address the cover picture through the outcome tests. Figure 11 b, and e are stego pictures that are completely impalpable. Figure 11 c and f are decoded pictures that are vague to cover pictures. We preference the normal selection of cover pictures ''peppers'' and ''lake'' with the size of 256 × 256 as experimental tests.
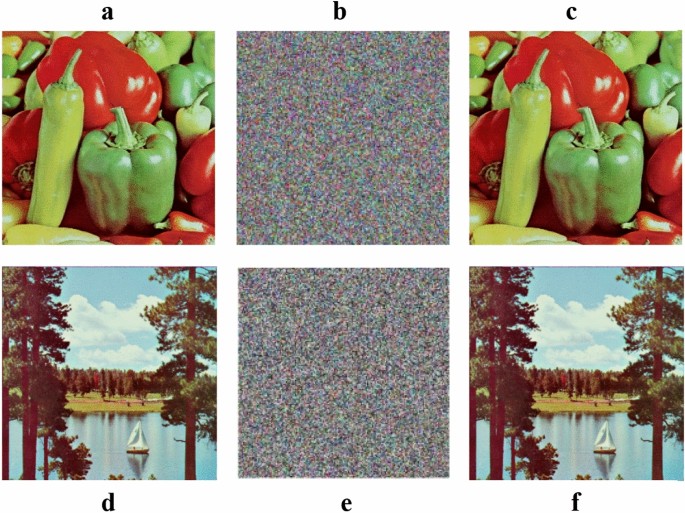
Experimental results of cover images ( a , d ), cipher images ( b , e ) and decrypted images ( c , f ).
To analyze whether the proposed encryption method can oppose differential assaults; two significant assessment factors for differential assaults investigation are used which is Unified Average Changing Intensity (UACI), and Number of Pixels Changing Rate (NPCR) 58 , 59 . The value of UACI and NPCR of “Lake” and “Pepper” are presented in Tables 7 and 8 below. We can see that UACI is close the hypothetical value of 34.5742% and NPCR is near the hypothetical value of 99.7183%, and that implies that our plan can oppose difference assaults. In the meantime, our calculation is better than the literature 60 , 61 .
Now to check the proposed algorithm on differential attack two attacks are; Noise Attack (NA), NA is used to check either the algorithm can resist against some noise attacks are not, because a good embedding algorithm should be able to resist against NA. We analyzed some standard images using noise attacks with the value of 0.01, 0.1 and 0.5 of salt and pepper noise. It can be seen in Fig. 12 by adding 0.1 salt and pepper noise are still detectible. Therefore, our algorithm has good toughness and can proficiently oppose commotion assaults.
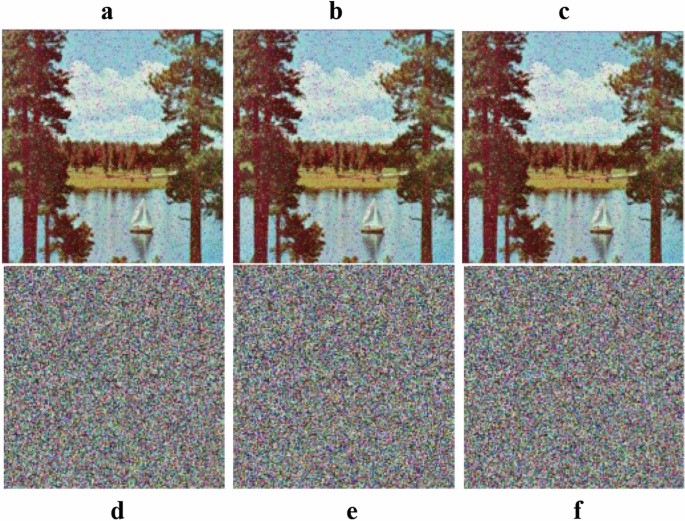
Noise attack (NA) analysis: ( a ) is decrypted image of ( d , b ) decrypted image of ( e , c ) decrypted image of ( f , d ) adding 0.01 salt and pepper noise, ( e ) adding 0.01 salt and pepper noise, ( f ) adding 0.01 salt and pepper noise.
While Cropping Attacks (CA), An ideal cryptosystem ought to be against information CA by transmission and capacity 62 , 63 . To assess its power in opposing trimming assaults, leaves behind 64 × 64, 64 × 128, 128 × 128, and 128 × 256 are obliterated from the embedded image ''Lake '' as displayed in Fig. 13 e–h. The extracted image is displayed in Fig. 13 a–d, and they can still be perceived. It demonstrates that our algorithm can oppose information editing assaults.
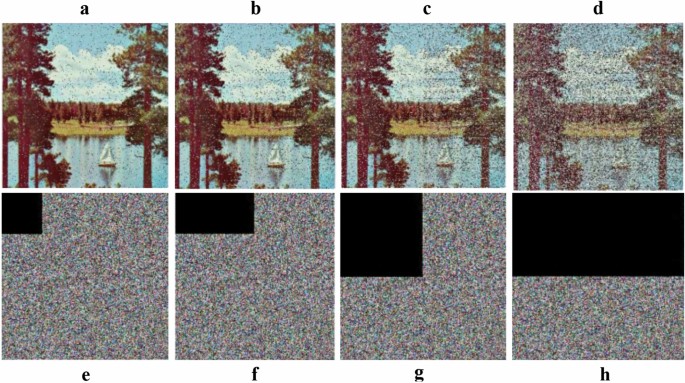
Cropping Attack (CA) analysis ( a ) decrypted image of ( e , b ) decrypted image of ( f , c ) decrypted image of ( g , d ) decrypted image of ( h , e ) 1/16 CA, ( f ) 1/8 CA, ( g ) 1/4 CA, ( h ) 1/2 CA.
Perspective 3
Histogram Analysis of the proposed algorithm based on P3 is given. Histogram Examination shows the real contrast between both stego and cover images. Due to its properties, a little difference between images can be founded, because it shows the extracted occurrences of the pixels of the image. Figure 14 shows the analysis of the proposed algorithm using three standard images namely, Lena, Mandrill, and House as both stego and cover image histograms.
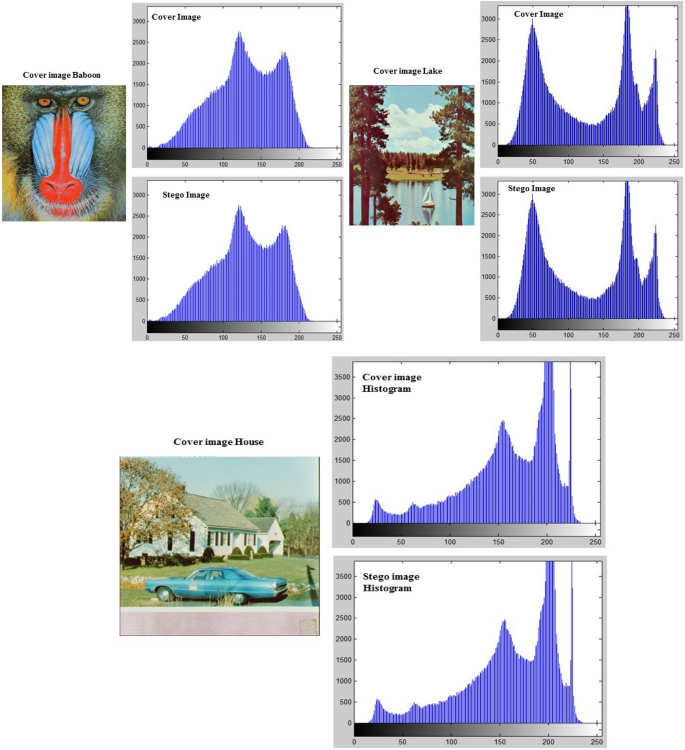
Histogram analysis of the proposed algorithm based on three standard cover and stego images.
However, the empirical results of the proposed algorithm grounded on diverse viewpoints using assessment metrics prove the improvement, efficiency, and effectiveness of the method. Our method improves the payload, high-level security, temper protection, and better visual quality of the image. Suppose attackers or any naked eye suspects the technique used in the proposed algorithm which is LSB and some improved technique of steganalysis. Then attacker can’t extract the actual contents of the secret message because the proposed method used Huffman code 64 , 65 , MLE 66 , Magic matrix 67 , 68 , and HSI (achromatic components of an image) 69 . If an attacker extracts the message up to some limits then the contents are useless, because, for extraction of the full message text, attackers need to use Huffman code, MLE, etc. for getting the actual message.
Conclusions and future research directions
In this study we proposed a novel method using Huffman code, HSI color model, MLEA, Magic matrix, and LSB substitution. Embedding the secret message, the I-plane of the HSI variety model is utilized as the cover image rather than the RGB model, for expanding the safety and reducing extra computational upstairs or processing time. The empirical outcomes of the proposed technique secure a normal of PSNR 79.29 dB over 165 standard images and also proves the control, and efficiency of the proposed method compared with some stated correlated works. The uses of the way of embedding the secret message in cover object is to makes this algorithm dreadful and abstruse and also unclear and foolish the steganalysis process. Our proposed method also used Huffman code which makes it more robust than existing methods. However, we infer that it is capable of shaping the stego picture practically identical and also has the fitness to give adeptness, and effectiveness and justify the encouraging demands of the current system and user to generate better quality stego images. So our method is easy to program, simple, and a better combination in terms of transparency and robustness. The results from different perspectives show the achievability and outperforming of our method to others. The main demerit of the proposed method is the amount of embedding the secret message not more than 20 KBs. Because for reliability, we analyzed the algorithm from different perspectives to achieve the basic criterion of steganography up to some acceptable limits. But still needs some reasonable improvements by functioning on magic matrix and MLEA extension to make the technique more dependable and also implementation of the method into the transform domain 70 , 71 . We are also now involved in an outcome further such ways as unsupervised learning (ML), related concepts of Deep Learning to grab some boundaries, and statistical and image processing assaults to produce excellent and dependable free stego images.
Data availability
The datasets produced and/or analyzed during the current study are openly accessible in the Kaggle repository, and SIPI, and can be gotten to at [ https://www.kaggle.com/datasets/mnavaidd/image-segmentation-dataset ]. Further, different pictures utilized inside the exploratory work are freely accessible on the web. All the codes used for this method will be provided for research purposes if requested by researchers.
Nabi, S. T., Kumar, M., Singh, P., Aggarwal, N. & Kumar, K. A comprehensive survey of image and video forgery techniques: Variants, challenges, and future directions. Multimed. Syst. 28 (3), 939–992. https://doi.org/10.1007/s00530-021-00873-8 (2022).
Article Google Scholar
LakshmiSirisha, B. & ChandraMohan, B. Review on spatial domain image steganography techniques. J. Discret. Math. Sci. Cryptogr. 24 (6), 1873–1883. https://doi.org/10.1080/09720529.2021.1962025 (2021).
Dhawan, S. & Gupta, R. Analysis of various data security techniques of steganography: a survey. Inf. Secur. J. 30 (2), 63–87 (2021).
Google Scholar
Bansal, K., Agrawal, A., & Bansal, N. (2020). A survey on steganography using least significant bit (lsb) embedding approach. in 2020 4th International Conference on Trends in Electronics and Informatics (ICOEI) (48184) , 64–69. https://doi.org/10.1080/19393555.2020.1801911.IEEE .
Sahu, A. K. & Swain, G. A review on LSB substitution and PVD based image steganography techniques. Indon. J. Electr. Eng. Comput. Sci. 2 (3), 712–719 (2016).
Hussain, M., Wahab, A. W. A., Idris, Y. I. B., Ho, A. T. & Jung, K. H. Image steganography in spatial domain: A survey. Signal Process. Image Commun. 65 , 46–66. https://doi.org/10.1016/j.image.2018.03.012 (2018).
Prajapati, H. A. & Chitaliya, N. G. Secured and robust dual image steganography: A survey. Int. J. Innov. Res. Comput. Commun. Eng. 3 (1), 30–37 (2015).
Subhedar, M. S. & Mankar, V. H. Current status and key issues in image steganography: A survey. Comput. Sci. Rev. 13 , 95–113. https://doi.org/10.1016/j.cosrev.2014.09.001 (2014).
Article MATH Google Scholar
Vaidya, K., Kargathara, A., & Kumbharana, C. K. Classification of Image Steganography in Substitution Technique. in Rising Threats in Expert Applications and Solutions , 253–261. (Springer, 2021).
Aslam, M. A. et al . Image Steganography using Least Significant Bit (LSB)-A Systematic Literature Review. in 2022 2nd International Conference on Computing and Information Technology (ICCIT) , 32–38. https://doi.org/10.1109/ICCIT52419.2022.9711628 .
Suresh, K. S., & Kamalakannan, T. Image Steganography Based on LSB Using Various Scanning Methods in Spatial Domain .
Alatiyyat, B. F., & Narmatha, C. Survey on image steganography techniques. in 2022 2nd International Conference on Computing and Information Technology (ICCIT) , 57–64. (IEEE, 2022).
Hameed, R. S., Abd Rahim, B. H. A., Taher, M. M. & Mokri, S. S. A literature review of various steganography methods. J. Theor. Appl. Inf. Technol. 100 (5), 1–10 (2022).
Kaur, S., Singh, S., Kaur, M. & Lee, H. N. A systematic review of computational image steganography approaches. Arch. Comput. Methods Eng. 1 , 1–23 (2022).
ADS Google Scholar
Tanya Bindu, R. & Kavitha, T. A survey on various crypto-steganography techniques for real-time images. In Intelligent Cyber Physical Systems and Internet of Things: ICoICI 2022 365–373 (Springer, 2023).
Chapter Google Scholar
Sharda, S. & Budhiraja, S. Image steganography: A review. Int. J. Emerg. Technol. Adv. Eng. (IJETAE) 3 (1), 707–710 (2013).
Upendra Raju, K. & Amutha Prabha, N. Dual images in reversible data hiding with adaptive color space variation using wavelet transforms. Int. J. Intell. Unmanned Syst. 11 (1), 96–108 (2023).
Inan, Y. Quality metrics of LSB image steganography technique for color space HSI. in 11th International Conference on Theory and Application of Soft Computing, Computing with Words and Perceptions and Artificial Intelligence-ICSCCW-2021 , 67–74. (Springer, 2022).
Hassan, F. S. & Gutub, A. Improving data hiding within colour images using hue component of HSV colour space. CAAI Trans. Intell. Technol. 7 (1), 56–68 (2022).
Kumar, A., Rani, R. & Singh, S. A survey of recent advances in image steganography. Secur. Privacy 6 , e281 (2023).
Tang, L., Wu, D., Wang, H., Chen, M. & Xie, J. An adaptive fuzzy inference approach for color image steganography. Soft. Comput. 25 (16), 10987–11004 (2021).
Elshoush, H. T., Mahmoud, M. M. & Altigani, A. A new high capacity and secure image realization steganography based on ASCII code matching. Multimed. Tools Appl. 81 (4), 5191–5237 (2022).
Hemeida, F., Alexan, W. & Mamdouh, S. A comparative study of audio steganography schemes. Int. J. Comput. Dig. Syst. 10 , 555–562 (2021).
Setiadi, D. R. I. M. PSNR vs SSIM: Imperceptibility quality assessment for image steganography. Multimed. Tools Appl. 80 (6), 8423–8444 (2021).
Zhang, Y. J. Image engineering. in Handbook of Image Engineering , 55–83. (Springer, 2021).
Lee, Y. K. & Chen, L. H. High capacity image steganographic model. IEE Proc. Vis. Image Signal Process. 147 (3), 288–294 (2000).
Zhang, X. & Wang, S. Efficient steganographic embedding by exploiting modification direction. IEEE Commun. Lett. 10 (11), 781–783 (2006).
Muhammad, K., Ahmad, J., Rehman, N. U., Jan, Z. & Qureshi, R. J. A secure cyclic steganographic technique for color images using randomization. Arxiv 19 (3), 57–64 (2015).
Muhammad, K. et al. A secure method for color image steganography using gray-level modification and multi-level encryption. KSII Trans. Internet Inf. Syst. 9 (5), 1938–1962 (2015).
Rustad, S., Setiadi, D. R. I. M., Syukur, A. & Andono, P. N. Inverted LSB image steganography using adaptive pattern to improve imperceptibility. J. King Saud Univ. Comput. Inf. Sci. 34 (6), 3559–3568 (2021).
Ye, H., Su, K., Cheng, X. & Huang, S. Research on reversible image steganography of encrypted image based on image interpolation and difference histogram shift. IET Image Proc. 16 (7), 1959–1972 (2022).
Than, S. S. M. Secure data transmission in video format based on LSB and Huffman coding. Int. J. Image Graph. Signal Process. 12 (1), 10–17 (2020).
Tsai, Y. Y., Liu, H. L., Kuo, P. L. & Chan, C. S. Extending multi-MSB prediction and huffman coding for reversible data hiding in encrypted HDR images. IEEE Access 10 , 49347–49358 (2022).
Sahu, M., Padhy, N., Gantayat, S. S. & Sahu, A. K. Local binary pattern-based reversible data hiding. CAAI Trans. Intell. Technol. 7 (4), 695–709 (2022).
Dhivya, R., Padmapriya, V., Sundararaman, R., Rayappan, J. B. B. & Amirtharajan, R. Chaos assisted variable bit steganography in transform domain. Electron. Lett. 54 (23), 1332–1334 (2018).
Article ADS Google Scholar
Ramapriya, B., & Kalpana, Y. A competent medical image steganography using improved optimization algorithm with Huffman encoding techniques. in 2023 7th International Conference on Computing Methodologies and Communication (ICCMC) , 1065–1073. (IEEE, 2023).
Sahu, A. K., Hassaballah, M., Rao, R. S. & Suresh, G. Logistic-map based fragile image watermarking scheme for tamper detection and localization. Multimed. Tools Appl. 1 , 1–32 (2022).
Bhavani, Y., Kamakshi, P., Kavya Sri, E., & Sindhu Sai, Y. A survey on image steganography techniques using least significant bit. in Intelligent Data Communication Technologies and Internet of Things , 281–290. (Springer, 2022).
Sun, S. A novel edge based image steganography with 2k correction and Huffman encoding. Inf. Process. Lett. 116 (2), 93–99 (2016).
Article MathSciNet MATH Google Scholar
Das, R. & Tuithung, T. (2012). A novel steganography method for image based on Huffman Encoding. in 2012 3rd National Conference on Emerging Trends and Applications in Computer Science , 14–18. (IEEE, 2012).
Nag, A., Singh, J. P., Biswas, S., Sarkar, D. & Sarkar, P. P. A Huffman code based image steganography technique. in Applied Algorithms: First International Conference, ICAA 2014, Kolkata, India, January 13–15, 2014. Proceedings 1 , 257–265. (Springer, 2014).
Yi, X., Yang, K., Zhao, X., Wang, Y. & Yu, H. AHCM: Adaptive Huffman code mapping for audio steganography based on psychoacoustic model. IEEE Trans. Inf. Forensics Secur. 14 (8), 2217–2231 (2019).
Sari, C. A., Ardiansyah, G. & Rachmawanto, E. H. An improved security and message capacity using AES and Huffman coding on image steganography. TELKOMNIKA (Telecommun. Comput. Electron. Control) 17 (5), 2400–2409 (2019).
Sharma, N. & Batra, U. An enhanced Huffman-PSO based image optimization algorithm for image steganography. Genet. Program Evolvable Mach. 22 , 189–205 (2021).
Taha, M. S., Mahdi, M. H., Khalid, H. N., Aman, A. H. M. & Attarbashi, Z. S. A steganography embedding method based on P single/P double and Huffman coding. in 2021 3rd International Cyber Resilience Conference (CRC) , 1–6. (IEEE, 2021).
Abed, M. K., Kareem, M. M., Ibrahim, R. K., Hashim, M. M., Kurnaz, S., & Ali, A. H. Secure medical image steganography method based on pixels variance value and eight neighbors. in 2021 International Conference on Advanced Computer Applications (ACA) , 199–205. (IEEE, 2021).
Tayyeh, H. K. & Al-Jumaili, A. S. A. A combination of least significant bit and deflate compression for image steganography. Int. J. Electr. Comput. Eng. 12 (1), 358–364 (2022).
Abbas, N. A. F., Abdulredha, N., Ibrahim, R. K. & Ali, A. H. Security and imperceptibility improving of image steganography using pixel allocation and random function techniques. Int. J. Electr. Comput. Eng. 12 (1), 2088–8708 (2022).
Almazaydeh, W. E. I. A. Image steganography to hide unlimited secret text size. Int. J. Comput. Sci. Netw. Secur. 22 (4), 73–82 (2022).
MathSciNet Google Scholar
Taha, M. S., Rahem, M. S. M., Hashim, M. M. & Khalid, H. N. High payload image steganography scheme with minimum distortion based on distinction grade value method. Multimed. Tools Appl. 81 (18), 25913–25946 (2022).
Gao, G., Zhang, L., Lin, Y., Tong, S. & Yuan, C. High-performance reversible data hiding in encrypted images with adaptive Huffman code. Dig. Signal Process. 133 , 103870 (2023).
Alkhliwi, S. Huffman encoding with white tailed eagle algorithm-based image steganography technique. Eng. Technol. Appl. Sci. Res. 13 (2), 10453–10459 (2023).
Kadhem, E. L. & Baawi, S. S. A secure and high capacity image steganography approach using Huffman coding and RSA encryption. J. Al-Qadisiyah Comput. Sci. Math. 15 (2), 35 (2023).
Zairi, M., Boujiha, T. & Ouelli, A. Secure fragile watermarking based on Huffman encoding and optimal embedding strategy. Indon. J. Electr. Eng. Comput. Sci. 29 (2), 1132–1139 (2023).
Yang, Y., He, H., Chen, F., Yuan, Y. & Mao, N. Reversible data hiding in encrypted images based on time-varying Huffman coding table. IEEE Trans. Multimed. 1 , 1–12 (2023).
CAS Google Scholar
Muazu, A. A., Maiwada, U. D., Garba, A. R. I., Qabasiyu, M. G., & Danyaro, K. U. Secure data hiding and extraction using RSA Algorithm. in Advancements in Interdisciplinary Research: First International Conference, AIR 2022, Prayagraj, India, May 6–7, 2022, Revised Selected Papers , 14–28. (Springer, 2023).
Mahdi, M. H. et al. Improvement of image steganography scheme based on LSB value with two control random parameters and multi-level encryption. in IOP Conference Series: Materials Science and Engineering , vol. 518, No. 5, 052002. (IOP Publishing, 2019).
Seyedzadeh, S. M., Norouzi, B., Mosavi, M. R. & Mirzakuchaki, S. A novel color image encryption algorithm based on spatial permutation and quantum chaotic map. Nonlinear Dyn. 81 (1–2), 511–529 (2015).
Di, X., Li, J., Qi, H., Cong, L. & Yang, H. A semi-symmetric image encryption scheme based on the function projective synchronization of two hyperchaotic systems. PLoS ONE 12 (9), e0184586 (2017).
Article PubMed PubMed Central Google Scholar
Praveenkumar, P., Amirtharajan, R., Thenmozhi, K. & Rayappan, J. B. B. ‘Triple chaotic image scrambling on RGB: A random image encryption approach’. Secur. Commun. Netw. 8 (18), 3335–3345 (2015).
Chai, X.-L., Gan, Z.-H., Lu, Y., Zhang, M.-H. & Chen, Y.-R. A novel color image encryption algorithm based on genetic recombination and the four-dimensional memristive hyperchaotic system. Chin. Phys. B 25 (10), 100503 (2016).
Li, X., Zhou, C. & Xu, N. A secure and efficient image encryption algorithm based on DNA coding and spatiotemporal chaos. IJ Netw. Secur. 20 (1), 110–120 (2018).
Norouzi, B. & Mirzakuchaki, S. A fast color image encryption algorithm based on hyper-chaotic systems. Nonlinear Dyn. 78 (2), 995–1015 (2014).
Wang, X., Chang, C. C., Lin, C. C. & Chang, C. C. On the multi-level embedding of crypto-image reversible data hiding. J. Vis. Commun. Image Represent. 87 , 103556 (2022).
Yousif, S. F., Abboud, A. J. & Radhi, H. Y. Robust image encryption with scanning technology, the El-Gamal algorithm and chaos theory. IEEE Access 8 , 155184–155209 (2020).
Yousif, S. F., Abboud, A. J. & Alhumaima, R. S. A new image encryption based on bit replacing, chaos and DNA coding techniques. Multimed. Tools Appl. 81 (19), 27453–27493 (2022).
https://en.wikipedia.org/wiki/Magic_square
https://www.mathworks.com/help/matlab/ref/magic.html
Alabaichi, A., Al-Dabbas, M. A. A. A. K. & Salih, A. Image steganography using least significant bit and secret map techniques. Int. J. Electr. Comput. Eng. 10 (1), 2088–8708 (2020).
https://sipi.usc.edu/database/ .
https://www.imageprocessingplace.com/ .
Download references
Acknowledgements
This research is supported, in parts, by the University of Peshawar and, in parts, by the Abdul Wali Khan University Mardan (AWKUM), Pakistan.
Author information
Authors and affiliations.
Qurtuba University of Science and Information Technology, Peshawar, Pakistan
Shahid Rahman & Jamal Uddin
University of Buner, Khyber Pakhtunkhwa, Pakistan
Hameed Hussain & Afzal Rahman
Abdul Wali Khan University, Mardan, Pakistan
Aftab Ahmed & Muhammad Zakarya
University of Lakki Marwat, Khyber Pakhtunkhwa, Pakistan
Ayaz Ali Khan
Sohar University, Sohar, Sultanate of Oman
Muhammad Zakarya
Kardan University, Kabul, Afghanistan
Muhammad Haleem
You can also search for this author in PubMed Google Scholar
Contributions
Shahid Rahman:- Research, Methodology, Writing - Original Draft; Jamal Uddin:- Conceptualization, Methodology, Software, Writing - Review & Editing; Hameed Hussain:- Conceptualization, Visualization, Validation, Investigation; Aftab Ahmed:- Visualization, Validation, Proofreading; Ayaz Ali Khan:- Writing - Review & Editing, Revisions; Muhammad Zakarya:- Visualization, Data Curation, Proofreading; Afzal Rahman:- Visualization, Writing - Review & Editing; Muhammad Haleem:- Writing - Revised Draft, Data Curation;
Corresponding author
Correspondence to Muhammad Haleem .
Ethics declarations
Competing interests.
The authors declare no competing interests.
Additional information
Publisher's note.
Springer Nature remains neutral with regard to jurisdictional claims in published maps and institutional affiliations.
Rights and permissions
Open Access This article is licensed under a Creative Commons Attribution 4.0 International License, which permits use, sharing, adaptation, distribution and reproduction in any medium or format, as long as you give appropriate credit to the original author(s) and the source, provide a link to the Creative Commons licence, and indicate if changes were made. The images or other third party material in this article are included in the article's Creative Commons licence, unless indicated otherwise in a credit line to the material. If material is not included in the article's Creative Commons licence and your intended use is not permitted by statutory regulation or exceeds the permitted use, you will need to obtain permission directly from the copyright holder. To view a copy of this licence, visit http://creativecommons.org/licenses/by/4.0/ .
Reprints and permissions
About this article
Cite this article.
Rahman, S., Uddin, J., Hussain, H. et al. A Huffman code LSB based image steganography technique using multi-level encryption and achromatic component of an image. Sci Rep 13 , 14183 (2023). https://doi.org/10.1038/s41598-023-41303-1
Download citation
Received : 12 February 2023
Accepted : 24 August 2023
Published : 30 August 2023
DOI : https://doi.org/10.1038/s41598-023-41303-1
Share this article
Anyone you share the following link with will be able to read this content:
Sorry, a shareable link is not currently available for this article.
Provided by the Springer Nature SharedIt content-sharing initiative
This article is cited by
Four enhanced algorithms for full size image hiding in chest x-ray images.
- Apichat Heednacram
- Yossawee Keaomanee
Multimedia Tools and Applications (2024)
By submitting a comment you agree to abide by our Terms and Community Guidelines . If you find something abusive or that does not comply with our terms or guidelines please flag it as inappropriate.
Quick links
- Explore articles by subject
- Guide to authors
- Editorial policies
Sign up for the Nature Briefing: AI and Robotics newsletter — what matters in AI and robotics research, free to your inbox weekly.

- Open access
- Published: 31 October 2023
Robust steganography in practical communication: a comparative study
- Tong Qiao ORCID: orcid.org/0000-0003-4912-2132 1 ,
- Shengwang Xu 1 ,
- Shuai Wang 1 ,
- Xiaoshuai Wu 2 ,
- Ning Zheng 1 ,
- Ming Xu 1 &
- Binmin Pan 1
EURASIP Journal on Image and Video Processing volume 2023 , Article number: 15 ( 2023 ) Cite this article
933 Accesses
Metrics details
To realize the act of covert communication in a public channel, steganography is proposed. In the current study, modern adaptive steganography plays a dominant role due to its high undetectability. However, the effectiveness of modern adaptive steganography is challenged when being applied in practical communication, such as over social network. Several robust steganographic methods have been proposed, while the comparative study between them is still unknown. Thus, we propose a framework to generalize the current typical steganographic methods resisting against compression attack, and meanwhile empirically analyze advantages and disadvantages of them based on four baseline indicators, referring to as capacity, imperceptibility, undetectability, and robustness. More importantly, the robustness performance of the methods is compared in the real application, such as on Facebook, Twitter, and WeChat, which has not been comprehensively addressed in this community. In particular, the methods modifying sign of DCT coefficients perform more superiority on the social media application.
1 Introduction
Steganography, one type of information hiding, is a science and art of transmitting secret bits in a covert channel [ 1 , 2 ]. Different from watermarking technique for protecting copyrights [ 3 ], steganography conceals the existence of covert communication by embedding message into media as cover. Conventional media, including text [ 4 ], image [ 5 ], audio [ 6 ], video [ 7 ], are widely used for steganography, among which images are the most popular.
Image steganography algorithms are generally divided into two categories: non-adaptive steganography and adaptive steganography [ 8 , 9 , 10 , 11 , 12 , 13 , 14 , 15 ]. Non-adaptive steganographic algorithms such as Least Significant Bit replacement (LSBr) and Least Significant Bit matching (LSBm) steganography, only randomly embed secret message by modifying pixels/coefficients of the image, where selection of embedding position is nearly independent of image content. On the contrary, adaptive steganographic algorithms with minimizing a distortion function can achieve high undetectability. Typically, spatial-domain algorithms such as HUGO [ 16 ], WOW [ 17 ], HILL [ 18 ], and S-UNIWARD [ 19 ] select the appropriate embedding region according to the texture complexity of the image; similarly, frequency-domain algorithms embed secret message by adaptively modifying DCT coefficients including UED [ 20 ], UERD [ 21 ], J-UNIWARD [ 19 ], and SI-UNIWARD [ 19 ]. In an alternative manner, the performance of adaptive strategy has been increasingly improved by minimizing non-additive distortion in steganography [ 22 , 23 , 24 ].
However, it hardly holds true that adaptive steganography can extract secret information entirely and correctly when stego image suffers attack from a dirty channel [ 25 ], such as compression, cropping, scaling, even adding noise. The dirty channel denotes a public channel where the transmitted stego image might be attacked by the server provider. The goal of steganography is to achieve covert communication between sender and receiver, and the secret message can be completely reconstructed even in the dirty channel. In this context, the design of robust steganographic algorithm has attracted more attention in the community of image steganography.
Some algorithms have already made breakthroughs. Zhang et al. first proposed a series of robust steganographic methods [ 26 , 27 , 28 ]. The core idea behind those algorithms is that the mapping relationship among features from cover source, which basically remains stable, should be mainly addressed. For instance, the magnitude and position relationship of DCT coefficients among inter or intra blocks are utilized. That is because the relationship basically remains its characteristic before and after compression. In the following, we simply generalize the methods [ 26 , 27 , 28 ]) in the early stage:
DCRAS [ 26 ]: First, the DCT coefficients are divided into \(8 \times 8\) blocks. Next, through comparing the magnitude of target DCT coefficient and the mean of its neighbouring ones among the same sub-band, the relationship is mapped to a set of cover elements \(\{0, 1\}\) . Specifically, if the target DCT is larger than the mean of its neighbouring ones, cover element with 1 is extracted; otherwise, cover element with 0 is acquired. Finally, the secret bits encoded by RS are embedded into cover elements to generate the stego elements, which help us to acquire robust stego image.
FRAS [ 27 ]: First, the robust regions are located by adopting Harris–Laplacian features [ 29 ]. Next, on the basis of DCRAS, the cover elements can be obtained, which further strikes the balance between undetectability and robustness. Thus, the performance of FRAS is superior to DCRAS.
DMAS [ 28 ]: Relying on JPEG compression quantization table, with the help of dither modulation, the cover elements can be successfully extracted. Through taking full advantage of compression characteristics, the constructed embedding domain is more stable than its predecessors, leading to that the modification magnitude of DCT coefficients is smaller. Thus, both robust and undetectable performance is remarkably improved.
In the early stage, people pay more attention on the robust domain. While in the current stage, for instance, Tao et al. [ 30 ] proposed a novel robust steganographic algorithm via intermediate images based on coefficient adjustment. Based on the transmission channel matching, the authors of [ 31 ] repeatedly compressed the image to reduce the impact caused by JPEG compression of the channel, that directly improves the robustness of the transmitted stego image. In addition, Zhu et al. proposed a robust steganography method by modifying sign of DCT coefficients [ 25 ]. Recently, robust steganographic algorithms have been further developed based on generalized dither modulation and expanded embedding domain [ 32 ], and based on the strategy of adaptive dither adjustment [ 33 ]. Encouragingly, the aforementioned algorithms perform very well when resisting attacks by JPEG compression while ensuring a high accuracy of correctly extracting secret information.
It should be addressed that in this paper, we are not prepared to list all the state of the arts while mainly revisit the typical methods in the current stage. That is because the methods in the current stages (lack of comprehensive comparison) improve the performance of the methods in the early stage. Then, the rest of this paper is organized as follows. In Sect. 2 , we first propose to formulate the problems of current robust steganographic algorithms; we mainly overview three typical algorithms, which is followed by experimental results in Sect. 3 . Finally, the concluding remarks are drawn in Sect. 4 .
2 Typical robust steganography
2.1 framework and metric.
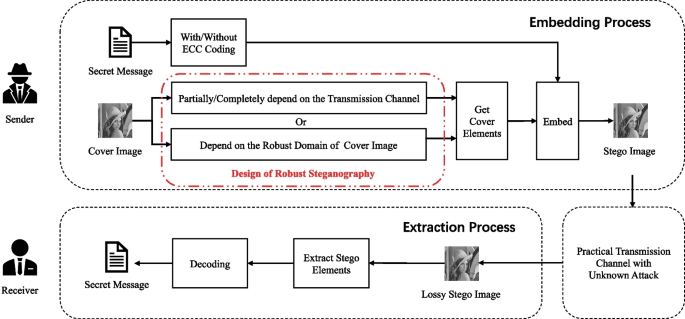
Framework for covert communication in a dirty channel; this framework has been adopted by most current robust steganography
In the current study, the simulated channels in the laboratory are often used to attack stego images instead of real transmission channels. Various channels might offer different attacks, among which JPEG compression is widely-adopted. In this context, we propose to address some limitations of current arts.
First, some steganographic methods heavily depend on the quality factor (QF) of JPEG compression from the transmission channel, where the property of transmission channel is required in advance. Therefore, if the transmission channel becomes unknown, the robustness of steganography cannot be guaranteed, even resulting in complete failure of secret information extraction.
Second, many robust steganographic methods only consider a single attack, which is unreal in practical communication. In particular, one method can resist well against JPEG compression while fail to deal with the case of re-scaling. In addition, robust steganography relying on the correlation feature between neighboring pixels (or DCT coefficients) probably fails when the relationship is destroyed by multiple attacks from the transmission channel.
To our knowledge, to complete the task of robust steganography, most of current studies are prone to sacrifice the embedding capacity for improving the security of covert communication. For clarity, it is proposed to generalize a framework of robust steganography as Fig. 1 reports. For a sender, she/he would like to embed the secret message into a cover image, in which cover elements are selected for embedding generally relying on the design of robust steganography, leading to a stego image; for a receiver, the secret message can be successfully extracted by carrying out the extraction process even in a dirty channel, in which lossy compression unavoidably happens. Moreover, for instance, we also provide a use case of robust steganography in Fig. 2 , by iteratively simulating procedure of JPEG compression, the robust DCT coefficients from the 64 subbands, which always remain unchanged before and after compression, are selected.
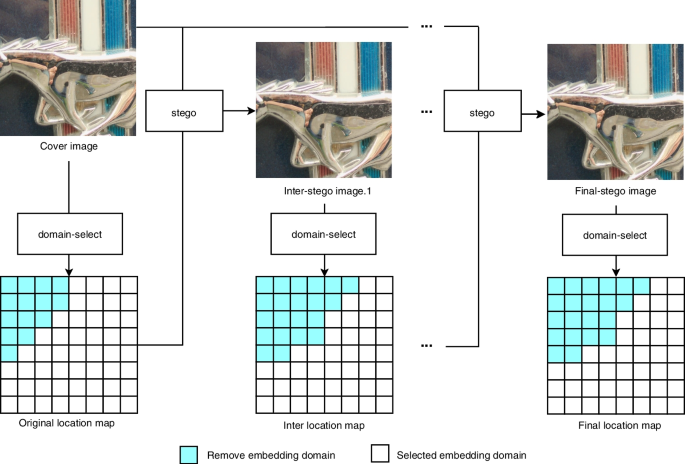
Use case of robust steganography
Besides, four indicators are used to measure the overall performance of different algorithms:
Capacity: the stego image carries the maximum number of the secret bits.
Imperceptibility: the stego image is indistinguishable from the cover image in human visual perception.
Undetectability: the stego image can bypass the detection from modern advanced steganalysis.
Robustness: the stego image capable of successfully transmitting secret bits, is immune to known or unknown post-processing attacks.
Without loss of generality, the technique of steganography needs to move from the laboratory to the real world for a wide range of applications. Then the fashionable social network replaces the simulated transmission channel for cover communication, such as Facebook, Twitter, and WeChat, in which various unknown attacks are adopted to threaten stego images. To address those challenges, the study of robust steganography should mainly focus on the robustness of stego image itself (active scheme), rather than relying on the transmission channel (passive scheme). That is because the vulnerability of transmission channel based algorithm is possibly exposed when social network ceaselessly adjusts its corresponding attack strategy such as QFs of JPEG compression (see Subsection 3.6 of Sect. 3 ). Next, we generally overview three typical robust steganographic methods, and provide the comprehensive comparison in the numerical experiments.
In general, two mainstream categories of robust steganography are proposed. The first one mainly relies on the transmission channel, see [ 30 , 31 ] for instance; the second one mainly relies on the robust domain, see [ 25 ] for instance. In this section, we mainly introduce the main ideas of three typical robust steganographic algorithms [ 25 , 30 , 31 ], and highlight the advantages and limitations. The representative steganographic methods we chose are chronologically proposed to deal with the problem of robustness of covert communication, dependent of different strategies.
2.2 Transmission channel matching
The main idea of [ 31 ] is to adapt the image to the transmission channel, that is, to repeatedly compress the image with the same QF to reduce extraction error, leading to the improved robustness of the stego image. It is worth noting that before transmission, a “cover image” has to be optimized.
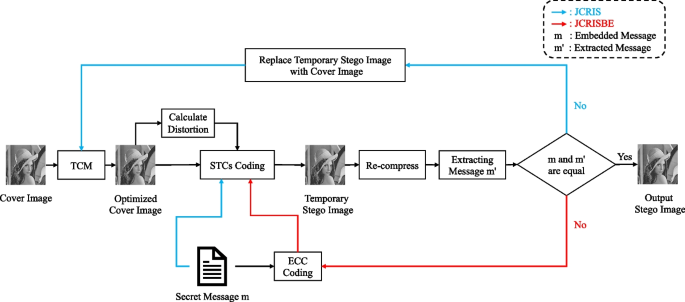
Scheme of transmission channel matching [ 31 ]
As Fig. 3 illustrates, the principal of TCM (Transmission Channel Matching) is actually that the strategy of multiple JPEG compression helps us reduce/eliminate errors caused by quantization and rounding, that is straightforward formulated as
where \({\textbf{D}}\) denotes the original DCT coefficients from a JPEG image, \({\textbf{Q}}\) the quantization step, and \({\text {F}_\text {IDCT}}(\cdot )\) represents the inverse DCT operation. After rounding, we can obtain the de-compressed image \({\textbf{S}}\) in the spatial domain. Then we generate the new DCT matrix \(\textbf{D}^{(1)}\) by transforming \(\textbf{S}\) to DCT coefficients:
where \({\text {F}_\text {DCT}}(\cdot )\) denotes DCT operation. Then, let us define \({{\text {N}_\text {diff}}} ({\textbf{D}}, {\textbf{D}}^{(1)})\) as the number of different DCT coefficients between \({\textbf{D}}\) and \(\textbf{D}^{(1)}\) . Since that the effect on DCT coefficients from JPEG compression attack is mainly caused by rounding and quantization operation, the number \({{\text {N}_\text {diff}}}\) can be further decreased (to zero for optimal solution) by multiply compressing an image.
Furthermore, let us extend our description of TCM by defining DCT coefficients as \({\textbf{D}}\) from a cover image \({\textbf{X}}\) ; meanwhile the QF pre-set by the dirty channel, denoted as \(\textbf{Q}_{\text{channel}}\) , is prior-known (easily acquired by up-loading and down-loading the same image). Before applying TCM operation, the size of the image should be calibrated, matching to the channel. First, it is proposed to obtain \({\textbf{D}}^{(1)}\) by \(\textbf{Q}_{\textrm{channel}}\) . If \({\text {N}_\text {diff}}({\textbf{D}}, {\textbf{D}}^{(1)}) = 0\) , the cover image \({\textbf{X}}\) is used for embedding; on the contrary, \({\textbf{X}}^{\prime }\) re-constructed by \({\textbf{D}}^{(1)}\) is re-compressed to obtain \({\textbf{D}}^{(2)}\) . Next, in the case of \({\text {N}^{\prime }_\text {diff}}({\textbf{D}}^{(1)}, {\textbf{D}}^{(2)}) = 0\) , \({\textbf{X}}^{\prime }\) is used for embedding; otherwise, we calculate the ratio \(\frac{{\text {N}_\text {diff}}}{\text {N}^{\prime }_\text {diff}}\) . When the ratio is greater than 0.98, the original cover image \({\textbf{X}}\) is used for embedding. If the above conditions cannot be met, in virtue of Eqs. ( 1 ) and ( 2 ), we re-do the aforementioned procedure using the twice-compressed image until the optimal cover image is acquired.
During the embedding process, two benchmark adaptive steganographic algorithms for JPEG image are adopted, namely J-UNIWARD [ 19 ] and UED [ 20 ]. More importantly, to further improve the robustness, the mechanism of ECC (Error Correction Coding) is established, leading to two specific schemes. In particular, JCRIS (JPEG Compression ResIstant Solution) embeds the secret message without ECC into the optimized cover image. If the secret message of the stego image cannot be correctly extracted, the stego image needs to be re-compressed again as TCM operation until the secret message are correctly extracted. Different from JCRIS, prior to embedding, JCRISBE (JPEG Compression ResIstant Solution with Bch codE) first needs to encode the secret message.
JCRIS has the higher embedding capacity while the advantages of JCRISBE are the stronger robustness and higher security. Moreover, the robustness of stego image is improved by adopting both two algorithms. Experimental results show that the correct rate of the secret message extraction can reach more than 99%. It should be noted that The TCM-based robust steganography works very well in the dirty channel with stable property. However, when the dirty channel arises the compression attack by using randomly-selected QF, the method probably fails.
2.3 Modifying coefficients of cover image
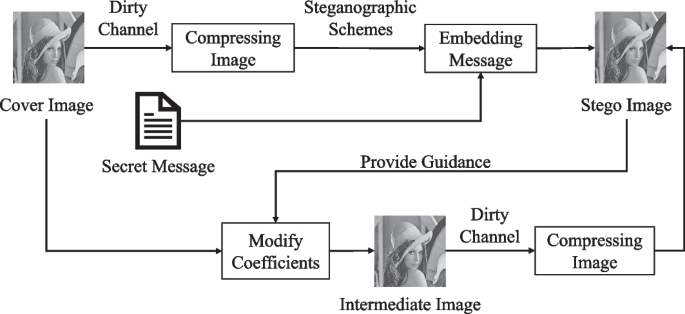
Scheme of modifying coefficients of cover image [ 30 ]
Tao et al. [ 30 ] proposed a robust steganographic scheme in another manner. The main idea behind the method focuses on Modifying Coefficients of Cover Image (MCCI) in virtue of the DCT coefficients of the stego image. Figure 4 illustrates the general framework of MCCI. In fact, MCCI successfully simulates the procedure of compression attack in a dirty channel as that of generating stego image. In this well-designed framework, the stego image generated by compression is completely the same as the stego images directly generated by using a modern adaptive embedding algorithm, such as J-UNIWARD [ 19 ] or UERD [ 21 ].
Given that a cover image is used for embedding, let us compress the cover image with a prior previously known QF in a dirty channel. Next, we apply some adaptive steganographic scheme to embed a secret message into the compressed cover image for generating stego one. Meanwhile, the DCT coefficients of the original cover image are adjusted according to the stego image for obtaining an intermediate image. Finally, the intermediate image are transmitted in the dirty channel, leading to a new stego image. This method ensures that the stego image generated by compressing the intermediate image is completely identical to the one generated by directly embedding at the compressed cover image. Moreover, the secret message can be correctly extracted at the receiver side. It is worth noting that the key step of MCCI is to generate the correct immediate image.
Given DCT coefficient matrix \({\textbf{I}}\) from an intermediate image, coefficient matrix \({\textbf{D}}\) from a cover image \({\textbf{X}}\) and \({\textbf{D}}^\prime\) from its corresponding stego one \({\textbf{Y}}\) , we are trying to find an \(\varvec{\alpha }\) to make the following equation hold:
where \(\varvec{\alpha }\) denotes a dithering map whose elements are integers. \(\varvec{\epsilon }\) denotes a set of integers, quantization step \({\textbf{Q}}_{{\text {cover}}}\) from cover image and \({\textbf{Q}}_{{\text {channel}}}\) from the dirty channel. In such tricky manner, we can first acquire an intermediate image based on \(\textbf{I}\) with the equivalent \({\textbf{Q}}_{{\text {cover}}}\) , and then generate the stego image \({\textbf{Y}}\) via transmitting the intermediate image in the dirty channel.
Although MCCI has remarkable advantage of correct extraction of secret message, it possibly fails to resist JPEG compression attack when the dirty channel with a metabolic QF or double compression.
2.4 Modifying sign of DCT coefficients
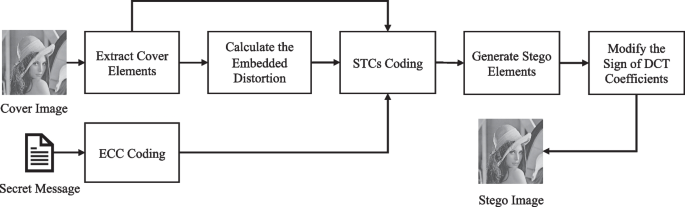
Scheme of modifying sign of DCT coefficients [ 25 ]
When the JPEG image is transmitted in a dirty channel, the sign of DCT coefficients is not easy to be changed, which can be utilized for designing a robust steganographic method. Zhu et al. [ 25 ] proposed a scheme by Modifying Sign of DCT Coefficients (MSDC) (see Fig. 5 for illustration). First of all, the suitable DCT coefficients are selected and optimized for embedding. While instead of original DCT coefficients, the cover elements are generated based on the sign of DCT coefficients, referring to as 1 (positive value) or 0 (negative value). Next, relying on the STCs, the stego elements (mapping to the sign of DCT coefficients from stego image) are acquired, which follow the same rule of generating cover element. Meanwhile, the secret message is encoded by ECC before embedding. Then, the key step of MSDC is to design the optimal cost function for STCs coding.
To design well-performed cost function, it is proposed to borrow the idea from J-UNIWARD [ 19 ] for achieving better undetectability, where the complex texture areas are usually assigned small values. Furthermore, in virtue of DCT coefficient \(d_{i,g}\) , the cost function of MSDC can be formulated as
where \(\rho ^\text{J}\) represents the cost function of J-UNIWARD. g and i respectively represent the g -th DCT block and the i -th position sorted by zig-zag in the DCT block. Here, \(\alpha\) is used to limit the maximum of the DCT coefficients \({\textbf{D}}\) for embedding; \(\beta\) represents a threshold, and \(\gamma > 1\) serves as the gain.
For clarity, the STCs is also given, which can be formulated by
where \({\textbf{c}}\) denotes the cover elements, the secret message \(\textbf{m}\) , and stego elements \(\textbf{s}\) . We select the parity-check matrix \(\textbf{H}\) as a key. Finally, we compare the cover elements with the stego elements which are used for embedding. If two values are identical, the DCT coefficient remains unchanged; otherwise, the sign of the DCT coefficient is flipped.
Obviously, MSDC has strong robustness due to that the sign nearly remains invariant after compression. Moreover, the algorithm preferentially selects non-zero DCT coefficients with small absolute values for modification. However, as the payload increases, the number of modified DCT coefficients also increases, leading to the enlarged extraction error or perceptual distortion.
3 Comparison experiment and analysis
3.1 experiment setup.
In this section, we will conduct the comprehensive comparison experiments, in which the current benchmark methods TCM (Transmission Channel Matching), MCCI (Modifying Coefficients of Cover Image), and MSDC (Modifying Sign of DCT Coefficients) are compared based on the indicators: capacity, imperceptibility, undetectability, and robustness. The baseline dataset BOSSbase ver. 1.01 [ 34 ] contains 10,000 grayscale raw images in PGM format with 512 \(\times\) 512 pixels. First of all, all images are compressed with QF 75, 85, and 95; the payload used for embedding ranges from 0.05 to 0.1 bpnzac (bit per non-zero AC DCT coefficients). Meanwhile, we use STCs [ 35 ] encoding to minimize the distortion embedding. Besides, it should be noted that for fair comparison, the embedding strategy of three methods refers to the prior-art J-UNIWARD.
3.2 Capacity performance
The capacity refers to the maximum number of secret bits embedded into the image. In the frequency domain, the capacity is measured by the number of non-zero AC coefficients for embedding. Specifically, all 10,000 images are compressed with QF = {75, 85, 95}, serving as cover source. Meanwhile, the JPEG compression attack from the simulated dirty channel uses QF 75. As QF is decreased, the number of non-zero AC coefficients reduces. It is worth noting that the comparison experiments are conducted under the same condition. Among three methods, the capacity of MCCI is the number of non-zero AC coefficients of the cover image while the capacity of the others is constrained by the corresponding algorithms (see Table 1 ).
The number of non-zero AC coefficients changes when QF changes. Accordingly, MCCI has the largest capacity, where all non-zero AC coefficients can be used as cover elements for embedding. While both TCM and MSDC have to select robust DCT coefficients as cover elements for embedding. Since that TCM needs to multiply compress the transmitted images with the same QF for reserving the invariance of DCT coefficients, the similar capacity appears among original cover images with different QFs. As a matter of fact, TCM and MSDC sacrifice to some extent capacity for guaranteeing robustness.
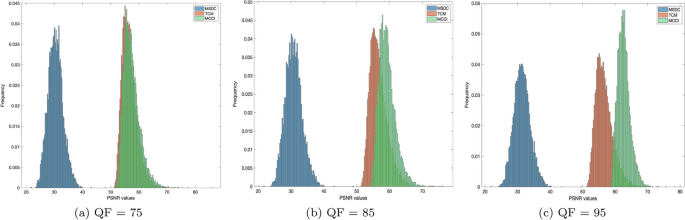
Normalized histogram comparison of PSNR values from 10,000 images
3.3 Imperceptibility performance
The stego image should remain perceptual distortion as small as possible, referring to imperceptibility. In this section, we select stego images with 0.1 payload and QF = {75, 85, 95}. To evaluate the imperceptibility of different stego images, the baseline PSNR (Peak Signal to Noise Ratio) serves as a metric. The higher the PSNR value is, the less perceptual distortion the stego image suffers.
As shown in Table 2 , the average PSNR values of 10,000 images are compared. MSDC has the lowest average PSNR value among different QFs. MCCI has the highest PSNR value, that is close to TCM. With increasing QF, the average PSNR of three methods basically has the incremental trend. In addition, Fig. 6 shows the normalized histogram of PSNR values from 10,000 images. It can be obviously observed that MSDC has the lowest PSNR values among three QFs. When QF = 75, the histograms of TMC and MCCI are nearly overlapped, meaning the very similar PSNR values. It should be noted that the overall distribution of TMC basically remains unchanged.
MSDC modifies the sign of the DCT coefficient, leading to the most changes of the image content compared to the others. Thus, the smallest PSNR values are from MSDC. If the selection of DCT coefficients is further optimized, we believe the the PSNR values of MSDC can be enhanced. Due to that TCM generates stego images by repeatedly compressing cover images with different QF, the PSNR values are very similar among three methods.
3.4 Undetectability performance
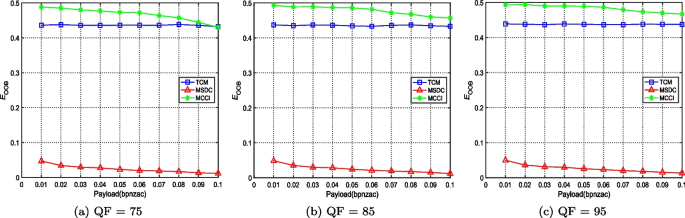
Undetectability comparison with payloads from 0.01 to 0.1
In this section, we evaluate the undetectability of three methods by using the benchmark steganalytic detector. The ensemble classifier [ 36 ], equipped with the rich model features DCTR-8000D [ 37 ], is our optimal choice. We randomly select 5,000 stego images and 5,000 cover images for training; the remaining images are used for testing. The \(P_{{\text {E}}}\) (classification error rate) is defined as
where \(P_{{\text {FA}}}\) is the false alarm rate and \(P_{{\text {MD}}}\) is the missed detection rate. In this paper, we use the ensemble’s “out-of-bag” (OOB) error \(E_{{\text {OOB}}}\) instead of \(P_{{\text {E}}}\) , since that \(E_{{\text {OOB}}}\) is an unbiased estimate of the \(P_{{\text {E}}}\) (see [ 21 ]).
As shown in Fig. 7 , if the small payloads (not larger than 0.1) are adopted for evaluation, MSDC has the lowest \(E_{{\text {OOB}}}\) while MCCI has the highest undetectability. Basically, the changes of QF cannot impact the undetectability among three methods.
Moreover, we propose to compare the undetectability of TCM and MCCI using more payloads {0.1, 0.2, 0.3, 0.4, 0.5} (see Fig. 8 for illustration). Due to the poor undetectability of MDSC, we remove the results of comparison experiments. TMC usually chose non-zero AC coefficients with strong robustness as cover elements. Therefore, the capacity is limited, leading to that as payload increases, TMC has to use the high-cost DCT coefficients. On the contrary, in the framework of MCCI, the stego image is the same as the stego one generated directly by the prior-art J-UNIWARD. Therefore, It has the optimal undetectability. Among three methods, MSDC has a relatively low undetectability, that is kind of different from the results of [ 25 ], where the CCPEV [ 38 ] and CCJRM [ 39 ] features are used. Nevertheless, MSDC changes the sign of the DCT coefficients, directly leading to the unsatisfactory undetectability.
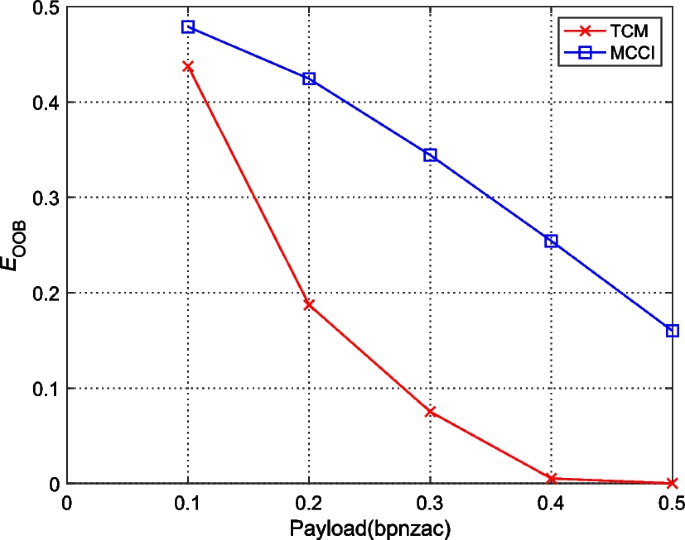
Undetectability of TCM and MCCI with payloads from 0.1 to 0.5
To enrich our experiments, it is proposed to illustrate the undetectable performance of methods [ 26 , 27 , 28 ] in the early stage. Here, it is proposed to evaluate the performance by using the ensemble detector equipped with CCPEV [ 38 ] features. As Table 3 illustrates, DCRAS performs worst while FRAS to some degree improves the performance due to that the cover elements are optimally selected in the robust domain. It can be observed that DMAS performs best based on the embedding domain constructed by dither modulation. In this case, the modification of DCT coefficients caused by embedding tends to the minimum compared to the others. Nevertheless, the methods in the early stage provide a new manner to design robust steganographic scheme, where the robust domain is proposed by referring to robust watermarking, that more or less guides the design of the following methods in the current stage.
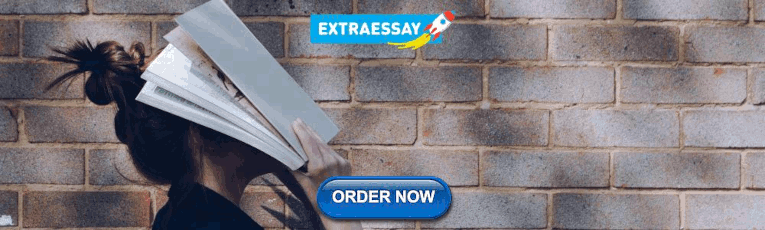
3.5 Robustness performance
In this section, we propose to adopt extraction error rate for comparing the robustness performance of three methods, which can be calculated by
where \(n_{{\text {error}}}\) denotes the number of incorrectly extracted bits, and \(n_{{\text {msg}}}\) is the total number of secret bits. The smaller \(R_{{\text {error}}}\) means the stronger robustness. Alternatively, we define the number of correct extraction, which represents the total number of images that can completely reconstruct the secret information.
As Fig. 9 reports, all secret bits can be perfectly correctly extracted in MCCI. That is because the stego image generated by MCCI is the same as the stego image directly generated by modern adaptive steganography. And the number of correct extraction in TCM is also satisfying. While MSDC cannot perform very well when QF equals 75 as the cases of QF = { 85, 95}. In addition, Fig. 10 illustrates the \(R_{{\text {error}}}\) results of three compared methods. As we expected, the performance of both MSDC and MCCI is satisfying, nearly approaching to zero error. However, the \(R_{{\text {error}}}\) of TCM is slightly larger than the others among three QFs.
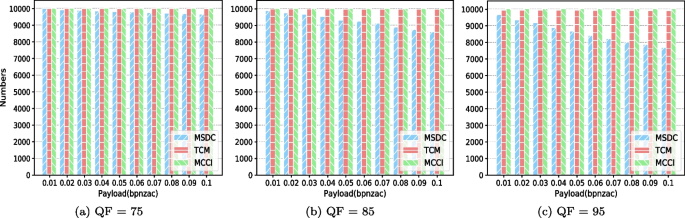
Number of images that can completely reconstruct secret bits
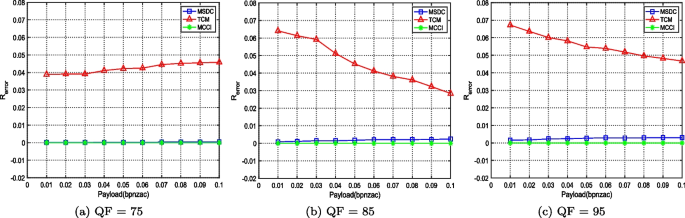
\(R_{{\text {error}}}\) performance of resisting JPEG compression
TCM is prone to multiply compress the image before transmission. It is always trying to remove images that are not suitable for embedding by setting an empirical threshold. In our experiment, strictly as the setting of [ 31 ], all the images are used for embedding, which unavoidably contains the unsatisfactory samples (unsuccessfully excluded), directly resulting in that the \(R_{{\text {error}}}\) increases. However, the number of those samples is not very large, that can explain the better performance in Fig. 9 .
3.6 Practical performance on social network platform
In this section, we will evaluate the practical performance of three baseline methods on Social Network Platform (SNP), such as Facebook, Twitter, and WeChat. Also, two baseline modern adaptive steganographic schemes, namely J-UNIWARD and UERD, are also compared. First, we randomly select 50 images in the BOSSbase, and compress them with QF 85. Then we embed secret message by payload 0.1. All the stego images are uploaded to Facebook or Twitter, and then downloaded; otherwise, the stego images are transmitted through WeChat. The robustness performance is measured by \(R_{{\text {c}}}\) and \(R_{{\text {error}}}\) (see Eq. ( 8 )). \(R_{{\text {c}}}\) denotes the ratio of the number of images in which the secret message can be completely extracted to the number of all the tested stego images.
As Table 4 reports, it can be seen that by adopting MSDC, the secret message can be completely extracted among 47 stego images, meaning that MSDC performs very well on Facebook. However, all the images of MCCI or TCM fail, where the results of them are not as good as that in the simulated channel (see Fig. 10 ). In addition, as we expected, both two non-robust steganographic schemes are invalid for covert communication in the real application.
To our knowledge, it hardly holds true that the social network platform, such as Facebook, only adopts simply once JPEG compression, where the metabolic dirty channel probably remarkably impacts the robust steganography heavily relying on the transmission channel such as MCCI and TCM. Prior to embedding, MCCI or TCM needs to learn the property of the dirty channel, such as QF. While the learned property will be invalid as the dirty channel continuously changes. On the contrary, the dependence of MSDC on the channel is minimal, which is nearly immune to the changes of the transmission channel, leading to the best performance among three methods. In fact, in our early observation (at the time both MCCI and TCM were published in 2018), Facebook indeed fixes the QF as 71. In 2019, the strategy of Facebook was changed, in which original images with QF not larger than 85 are re-compressed with QF 71; otherwise, the re-compression is proceeded with various QFs (see [ 32 ]). However, in our recent observation (till the date of paper submission), Facebook completely breaks the prior rule of compression, that continuously changes QF even though the images have the same original QF before uploading. Meanwhile, when the downloaded images from Facebook are uploaded again, the compression strategy is still unpredictable.
Moreover, we conduct the experiments on Twitter. When comparing the transmitted image via repeatedly uploading and downloading, it can be clearly observed that the image is post-processed by updating the strategy of compression. As Table 5 illustrates, TCM fails to complete the task of robust steganography. That is because the requirement (the ratio \(\frac{{\text {N}_\text {diff}}}{{\text {N}^{\prime }_\text {diff}}}>\) 0.98) of transmission channel cannot be met as multiple uploading and downloading. Besides, the modern adaptive steganographic schemes are still invalid. On the contrary, MSDC without heavily relying on the channel performs best. Compared with the results on Facebook, the \(R_{{\text {error}}}\) of MSDC decreases while the number of correct extraction to some extent is reduced. It is worth noting that the DCT coefficient (carrying secret bit) with its absolute value equal to 1, more easily changes to zero (caused by compression from Twitter) at the receiver side, that directly leads to the incorrect extraction of secret bits of MSDC.
Next, it is proposed to conduct our experiments on WeChat, which is one of the most popular instant-messaging App. Here, we consider two models for covert communication, that is original model and non-original model. In the original model, the image is sent with selection of original image ; In the non-original model, the image is directly sent with default selection. As we expected, in the original model, among five compared schemes, the secret bits can be perfectly extracted from all testing images (see Table 6 ). By checking the DCT coefficients and QF before and after transmission on WeChat, we cannot observe any changes; meanwhile, the capacity of image is reduced. Thus, we infer that the unknown lossless compression happens in the original model. Specifically, lossless compression is a manner of compression without losing any data, where the original data can be perfectly reconstructed from the compressed data (see [ 40 ] for details). Unfortunately, as Table 7 illustrates, in the non-original model, all the methods fail to transmit stego images on WeChat. That is because WeChat non-maliciously attacks the images using multiple post-processing manners except compression.
Recently, some new robust steganographic methods are proposed successively. We select the typical methods designed based on the proposed framework. It is worth noting that ESS (Enhanced Sign Steganography) [ 12 ] and SSR (Sign Steganography Revisited) [ 13 ] are both MSDC-based, DMMR (Dither Modulation and Modification with Re-compression) [ 41 ] is TCM-based, and SRJS (Secure Robust JPEG Steganography) [ 42 ] is MCCI-based. Besides, we add the updated classical steganography PQ-UNIWARD [ 43 ] for comparison. Since that the color images are usually transmitted on SNPs, we intend to carry out the experiments on ALASKA dataset [ 44 ], where 50 testing color images are selected for verifying the effectiveness of the compared methods. In Tables 8 and 9 , by observation, the robust steganography methods can successfully extract the secret images while the traditional steganography PQ-UNIWARD cannot complete the task of robust steganography. Besides, although SRJS performs very well in the simulated settings, it unavoidably exposes its limitation in the practical SNPs.
On SNP such as Facebook, Twitter, and WeChat, the transmission channel, that is characterized as black box , behaves in the more complicated system than the dirty channel in the simulated settings which only consider image compression attack. In virtue of our empirical analysis in this subsection, in the case that the channel is not completely acquainted, it is suggested to adopt the scheme relying on robust domain such as MSDC while carefully adopting the scheme heavily relying on the transmission channel such as MCCI and TCM.
4 Conclusion
In this paper, we mainly overview three current typical robust steganographic methods. More importantly, the performance of them is comprehensively compared relying on the baseline indicators, referring to as capacity, imperceptibility, undetectability, and robustness. Also, both strength and limitation of three methods are empirically analyzed. Although three methods perform very well, none of them can achieve the best results in all four indicators. Nevertheless, in the simulated transmission channel, MCCI is basically our optimal choice for robust steganography. That is because the stego image generated by MCCI is the most similar to the stego one by modern adaptive steganography such as J-UNIWARD. In the practical application, for instance covert communication on Facebook, it is no doubt that MSDC performs best in the aspect of robustness.
Moreover, based on the empirical results and analysis, we list the following studies for improving current algorithms:
To obtain better undetectability performance, the design of robust steganography should combine with modern adaptive scheme, together with its optimized cost function.
To further improve the robustness performance, the selection of cover elements is of importance, in which the property of DCT coefficients including their adjacent relationship can be further dug out. Also, the other known attacks, such as re-scaling and noise-adding, or more unknown attacks, should be addressed.
In an alternative manner, the robustness performance can be improved by to some extent reducing capacity. In the real scenario, we segment a set of secret message into several subsets, which are embedded into multiple images rather than using only one image.
The carrier may not only be limited to DCT coefficients or pixels. We can also investigate other ways of hiding the secret message into the image files, the property of which remains unchanged in covert communication.
In the future study, we will mainly focus on improving the robustness of the steganography method to ensure that the transmitted stego images can resist against known and unknown attacks from social network. Moreover, we address again that moving the steganography from the laboratory to the real world is of importance.
Availability of data and materials
The datasets used and analyzed in the current study are available from the corresponding author upon reasonable request.
Abbreviations
Least Significant Bit replacement
Least Significant Bit matching
Highly Undetectable steGo
Wavelet Obtained Weights
Spatial UNIversal WAvelet Relative Distortion
Uniform Embedding Distortion
Uniform Embedding Revisited Distortion
JPEG UIversal WAvelet Relative Distortion
Side Information UNIversal WAvelet Relative Distortion
Reed-solomon
DCT Coefficients Relationship based Adaptive Steganography
Feature Regions based Adaptive Steganography
Dither Modulation based Adaptive Steganography
Joint Photographic Experts Group
Quality factor
Transmission Channel Matching
Error Correction Coding
JPEG Compression ResIstant Solution
JPEG Compression ResIstant Solution with Bch codE
Modifying Coefficients of Cover Image
Modifying Sign of DCT Coefficients
Syndrome-trellis codes
Portable graymap file format
Peak Signal to Noise Ratio
Social Networking Platform
Enhanced Sign Steganography
Sign Steganography Revisited
Dither Modulation and Modification with Re-compression
Secure Robust JPEG Steganography
P.C. Mandal, I. Mukherjee, G. Paul, B. Chatterji, Digital image steganography: a literature survey. Inf. Sci. (2022). https://doi.org/10.1016/j.ins.2022.07.120
Article Google Scholar
M. Hussain, A.W.A. Wahab, Y.I.B. Idris, A.T. Ho, K.-H. Jung, Image steganography in spatial domain: a survey. Signal Process. Image Commun. 65 , 46–66 (2018)
M. Asikuzzaman, M.R. Pickering, An overview of digital video watermarking. IEEE Trans. Circuits Syst. Video Technol. 28 (9), 2131–2153 (2017)
T.-Y. Liu, W.-H. Tsai, A new steganographic method for data hiding in Microsoft word documents by a change tracking technique. IEEE Trans. Inf. Forensics Secur. 2 (1), 24–30 (2007)
B. Li, S. Tan, M. Wang, J. Huang, Investigation on cost assignment in spatial image steganography. IEEE Trans. Inf. Forensics Secur. 9 (8), 1264–1277 (2014)
Y. Huang, C. Liu, S. Tang, S. Bai, Steganography integration into a low-bit rate speech codec. IEEE Trans. Inf. Forensics Secur. 7 (6), 1865–1875 (2012)
D. Xu, R. Wang, Y.Q. Shi, Data hiding in encrypted H. 264/AVC video streams by codeword substitution. IEEE Trans. Inf. Forensics Secur. 9 (4), 596–606 (2014)
M. Hussain, A.W.A. Wahab, A.T. Ho, N. Javed, K.-H. Jung, A data hiding scheme using parity-bit pixel value differencing and improved rightmost digit replacement. Signal Process. Image Commun. 50 , 44–57 (2017)
A.A. Zakaria, M. Hussain, A.W.A. Wahab, M.Y.I. Idris, N.A. Abdullah, K.-H. Jung, High-capacity image steganography with minimum modified bits based on data mapping and LSB substitution. Appl. Sci. 8 (11), 2199 (2018)
Y. Zhang, X. Luo, Y. Guo, C. Qin, F. Liu, Multiple robustness enhancements for image adaptive steganography in lossy channels. IEEE Trans. Circuits Syst. Video Technol. 30 (8), 2750–2764 (2019)
Y. Wang, M. Tang, Z. Wang, High-capacity adaptive steganography based on LSB and hamming code. Optik 213 , 164685 (2020)
T. Qiao, S. Wang, X. Luo, Z. Zhu, Robust steganography resisting jpeg compression by improving selection of cover element. Signal Process. 183 , 108048 (2021)
X. Wu, T. Qiao, Y. Chen, M. Xu, N. Zheng, X. Luo, Sign steganography revisited with robust domain selection. Signal Process. 196 , 108522 (2022)
K. Zeng, K. Chen, W. Zhang, Y. Wang, N. Yu, Improving robust adaptive steganography via minimizing channel errors. Signal Process. 195 , 108498 (2022)
G. Xie, J. Ren, S. Marshall, H. Zhao, R. Li, A novel gradient-guided post-processing method for adaptive image steganography. Signal Process. 203 , 108813 (2023)
T. Pevnỳ, T. Filler, P. Bas, Using high-dimensional image models to perform highly undetectable steganography. In: International Workshop on Information Hiding, (Springer, 2010), pp. 161–177
V. Holub, J. Fridrich, Designing steganographic distortion using directional filters. In: 2012 IEEE International Workshop on Information Forensics and Security (WIFS), (IEEE, 2012), pp. 234–239
B. Li, M. Wang, J. Huang, X. Li, A new cost function for spatial image steganography. In: 2014 IEEE International Conference on Image Processing (ICIP), (IEEE, 2014), pp. 4206–4210
V. Holub, J. Fridrich, Digital image steganography using universal distortion. In: Proceedings of the First ACM Workshop on Information Hiding and Multimedia Security, (ACM, 2013), pp. 59–68
L. Guo, J. Ni, Y.Q. Shi, Uniform embedding for efficient jpeg steganography. IEEE Trans. Inf. Forensics Secur. 9 (5), 814–825 (2014)
L. Guo, J. Ni, W. Su, C. Tang, Y.-Q. Shi, Using statistical image model for jpeg steganography: uniform embedding revisited. IEEE Trans. Inf. Forensics Secur. 10 (12), 2669–2680 (2015)
T. Denemark, J. Fridrich, Improving steganographic security by synchronizing the selection channel. In: Proceedings of the 3rd ACM Workshop on Information Hiding and Multimedia Security, (2015), pp. 5–14
B. Li, M. Wang, X. Li, S. Tan, J. Huang, A strategy of clustering modification directions in spatial image steganography. IEEE Trans. Inf. Forensics Secur. 10 (9), 1905–1917 (2015)
W. Zhang, Z. Zhang, L. Zhang, H. Li, N. Yu, Decomposing joint distortion for adaptive steganography. IEEE Trans. Circuits Syst. Video Technol. 27 (10), 2274–2280 (2016)
Z. Zhu, N. Zheng, T. Qiao, M. Xu, Robust steganography by modifying sign of DCT coefficients. IEEE Access 7 , 168613–168628 (2019)
Y. Zhang, X. Luo, C. Yang, D. Ye, F. Liu, A jpeg-compression resistant adaptive steganography based on relative relationship between DCT coefficients. In: 2015 10th International Conference on Availability, Reliability and Security, (IEEE, 2015), pp. 461–466
Y. Zhang, X. Luo, C. Yang, F. Liu, Joint jpeg compression and detection resistant performance enhancement for adaptive steganography using feature regions selection. Multimed. Tools Appl. 76 (3), 3649–3668 (2017)
Y. Zhang, C. Qin, W. Zhang, F. Liu, X. Luo, On the fault-tolerant performance for a class of robust image steganography. Signal Process. 146 , 99–111 (2018)
J.-S. Tsai, W.-B. Huang, Y.-H. Kuo, M.-F. Horng, Joint robustness and security enhancement for feature-based image watermarking using invariant feature regions. Signal Process. 92 (6), 1431–1445 (2012)
J. Tao, S. Li, X. Zhang, Z. Wang, Towards robust image steganography. IEEE Trans. Circuits Syst. Video Technol. 29 (2), 594–600 (2018)
Z. Zhao, Q. Guan, H. Zhang, X. Zhao, Improving the robustness of adaptive steganographic algorithms based on transport channel matching. IEEE Trans. Inf. Forensics Secur. 14 (7), 1843–1856 (2018)
X. Yu, K. Chen, Y. Wang, W. Li, W. Zhang, N. Yu, Robust adaptive steganography based on generalized dither modulation and expanded embedding domain. Signal Process. 168 , 107343 (2020)
F. Li, K. Wu, C. Qin, J. Lei, Anti-compression jpeg steganography over repetitive compression networks. Signal Process. (2020). https://doi.org/10.1016/j.sigpro.2020.107454
P. Bas, T. Filler, T. Pevnỳ, break our steganographic system: the ins and outs of organizing boss. In: International Workshop on Information Hiding, (Springer, 2011), pp. 59–70
T. Filler, J. Judas, J. Fridrich, Minimizing additive distortion in steganography using syndrome-trellis codes. IEEE Trans. Inf. Forensics Secur. 6 (3), 920–935 (2011)
J. Kodovsky, J. Fridrich, V. Holub, Ensemble classifiers for steganalysis of digital media. IEEE Trans. Inf. Forensics Secur. 7 (2), 432–444 (2011)
V. Holub, J. Fridrich, Low-complexity features for jpeg steganalysis using undecimated DCT. IEEE Trans. Inf. Forensics Secur. 10 (2), 219–228 (2014)
T. Pevny, J. Fridrich, Merging markov and dct features for multi-class jpeg steganalysis. In: Security, steganography, and watermarking of multimedia contents IX, International Society for Optics and Photonicsvol. 6505, 650503 (2007).
J. Kodovskỳ, J. Fridrich, Steganalysis of jpeg images using rich models. In: Media Watermarking, Security, and Forensics 2012, International Society for Optics and Photonics vol. 8303, 83030 (2012).
K. Sayood, Introduction to data compression (2017)
Z. Yin, L. Ke, Robust adaptive steganography based on dither modulation and modification with re-compression. IEEE Trans. Signal Inf. Process. 7 , 336–345 (2021)
Google Scholar
W. Lu, J. Zhang, X. Zhao, W. Zhang, J. Huang, Secure robust jpeg steganography based on autoencoder with adaptive bch encoding. IEEE Trans. Circuits Syst. Video Technol. 31 (7), 2909–2922 (2020)
J. Butora, Y. Yousfi, J. Fridrich, How to pretrain for steganalysis. In: Proceedings of the 2021 ACM Workshop on Information Hiding and Multimedia Security, (2021), pp. 143–148
R. Cogranne, Q. Giboulot, P. Bas, The alaska steganalysis challenge: A first step towards steganalysis. In: Proceedings of the ACM Workshop on Information Hiding and Multimedia Security, (2019), pp. 125–137
Download references
Acknowledgments
Not applicable
This work was supported by Zhejiang Provincial Natural Science Foundation of China (No. LZ23F020006).
Author information
Authors and affiliations.
School of Cyberspace, Hangzhou Dianzi University, Hangzhou, China
Tong Qiao, Shengwang Xu, Shuai Wang, Ning Zheng, Ming Xu & Binmin Pan
College of Computer Science and Electronic Engineering, Hunan University, Changsha, China
Xiaoshuai Wu
Chinese Aeronautical Establishment, Beijing, China
You can also search for this author in PubMed Google Scholar
Contributions
TQ, SX, and SW designed and implemented the study and wrote the paper. XW, BL, NZ, MX and BP gave advice and revised the paper. The authors read and approved the final manuscript.
Corresponding author
Correspondence to Tong Qiao .
Ethics declarations
Competing interests.
The authors declare that they have no competing interests.
Additional information
Publisher's note.
Springer Nature remains neutral with regard to jurisdictional claims in published maps and institutional affiliations.
Rights and permissions
Open Access This article is licensed under a Creative Commons Attribution 4.0 International License, which permits use, sharing, adaptation, distribution and reproduction in any medium or format, as long as you give appropriate credit to the original author(s) and the source, provide a link to the Creative Commons licence, and indicate if changes were made. The images or other third party material in this article are included in the article's Creative Commons licence, unless indicated otherwise in a credit line to the material. If material is not included in the article's Creative Commons licence and your intended use is not permitted by statutory regulation or exceeds the permitted use, you will need to obtain permission directly from the copyright holder. To view a copy of this licence, visit http://creativecommons.org/licenses/by/4.0/ .
Reprints and permissions
About this article
Cite this article.
Qiao, T., Xu, S., Wang, S. et al. Robust steganography in practical communication: a comparative study. J Image Video Proc. 2023 , 15 (2023). https://doi.org/10.1186/s13640-023-00615-y
Download citation
Received : 14 August 2020
Accepted : 25 October 2023
Published : 31 October 2023
DOI : https://doi.org/10.1186/s13640-023-00615-y
Share this article
Anyone you share the following link with will be able to read this content:
Sorry, a shareable link is not currently available for this article.
Provided by the Springer Nature SharedIt content-sharing initiative
- Steganography
- Undetectability
- Social network
Help | Advanced Search
Computer Science > Cryptography and Security
Title: steganography of steganographic networks.
Abstract: Steganography is a technique for covert communication between two parties. With the rapid development of deep neural networks (DNN), more and more steganographic networks are proposed recently, which are shown to be promising to achieve good performance. Unlike the traditional handcrafted steganographic tools, a steganographic network is relatively large in size. It raises concerns on how to covertly transmit the steganographic network in public channels, which is a crucial stage in the pipeline of steganography in real world applications. To address such an issue, we propose a novel scheme for steganography of steganographic networks in this paper. Unlike the existing steganographic schemes which focus on the subtle modification of the cover data to accommodate the secrets. We propose to disguise a steganographic network (termed as the secret DNN model) into a stego DNN model which performs an ordinary machine learning task (termed as the stego task). During the model disguising, we select and tune a subset of filters in the secret DNN model to preserve its function on the secret task, where the remaining filters are reactivated according to a partial optimization strategy to disguise the whole secret DNN model into a stego DNN model. The secret DNN model can be recovered from the stego DNN model when needed. Various experiments have been conducted to demonstrate the advantage of our proposed method for covert communication of steganographic networks as well as general DNN models.
Submission history
Access paper:.
- Other Formats
References & Citations
- Google Scholar
- Semantic Scholar
BibTeX formatted citation

Bibliographic and Citation Tools
Code, data and media associated with this article, recommenders and search tools.
- Institution
arXivLabs: experimental projects with community collaborators
arXivLabs is a framework that allows collaborators to develop and share new arXiv features directly on our website.
Both individuals and organizations that work with arXivLabs have embraced and accepted our values of openness, community, excellence, and user data privacy. arXiv is committed to these values and only works with partners that adhere to them.
Have an idea for a project that will add value for arXiv's community? Learn more about arXivLabs .
image steganography Recently Published Documents
Total documents.
- Latest Documents
- Most Cited Documents
- Contributed Authors
- Related Sources
- Related Keywords
Image Steganography Technique Based on Integer Wavelet Transform Using Most Significant Bit Categories
Security and imperceptibility improving of image steganography using pixel allocation and random function techniques.
Information security is one of the main aspects of processes and methodologies in the technical age of information and communication. The security of information should be a key priority in the secret exchange of information between two parties. In order to ensure the security of information, there are some strategies that are used, and they include steganography and cryptography. An effective digital image-steganographic method based on odd/even pixel allocation and random function to increase the security and imperceptibility has been improved. This lately developed outline has been verified for increasing the security and imperceptibility to determine the existent problems. Huffman coding has been used to modify secret data prior embedding stage; this modified equivalent secret data that prevent the secret data from attackers to increase the secret data capacities. The main objective of our scheme is to boost the peak-signal-to-noise-ratio (PSNR) of the stego cover and stop against any attack. The size of the secret data also increases. The results confirm good PSNR values in addition of these findings confirmed the proposed method eligibility.
A combination of least significant bit and deflate compression for image steganography
Steganography is one of the cryptography techniques where secret information can be hidden through multimedia files such as images and videos. Steganography can offer a way of exchanging secret and encrypted information in an untypical mechanism where communicating parties can only interpret the secret message. The literature has shown a great interest in the least significant bit (LSB) technique which aims at embedding the secret message bits into the most insignificant bits of the image pixels. Although LSB showed a stable performance of image steganography yet, many works should be done on the message part. This paper aims to propose a combination of LSB and Deflate compression algorithm for image steganography. The proposed Deflate algorithm utilized both LZ77 and Huffman coding. After compressing the message text, LSB has been applied to embed the text within the cover image. Using benchmark images, the proposed method demonstrated an outperformance over the state of the art. This can proof the efficacy of using Deflate as a data compression prior to the LSB embedding.
Hybrid image steganography method using Lempel Ziv Welch and genetic algorithms for hiding confidential data
Quality metrics of lsb image steganography technique for color space hsi, blockchain-based secure data sharing scheme using image steganography and encryption techniques for telemedicine applications, reference-free differential histogram-correlative detection of steganography: performance analysis.
<span lang="EN-US">Recent <span>research has demonstrated the effectiveness of utilizing neural networks for detect tampering in images. However, because accessing a database is complex, which is needed in the classification process to detect tampering, reference-free steganalysis attracted attention. In recent work, an approach for least significant bit (LSB) steganalysis has been presented based on analyzing the derivatives of the histogram correlation. In this paper, we further examine this strategy for other steganographic methods. Detecting image tampering in the spatial domain, such as image steganography. It is found that the above approach could be applied successfully to other kinds of steganography with different orders of histogram-correlation derivatives. Also, the limits of the ratio stego-image to cover are considered, where very small ratios can escape this detection method unless </span> modified.</span>
Securing the COVID Patients’ Medical Records Using Encrypted Image Steganography
Gan-based spatial image steganography with cross feedback mechanism, cnn auto-encoder network using dilated inception for image steganography, export citation format, share document.
Video Steganography Techniques: A Comprehensive Review and Performance Evaluation
- Conference paper
- First Online: 23 April 2024
- Cite this conference paper
- Hemant Kumar ORCID: orcid.org/0000-0001-5719-1889 13 ,
- Pushpa Mamoria ORCID: orcid.org/0000-0002-5748-7302 13 ,
- Shivani Kumari ORCID: orcid.org/0009-0001-7330-1619 14 &
- Manoj Kumar Misra 15
Part of the book series: Lecture Notes in Networks and Systems ((LNNS,volume 918))
Included in the following conference series:
- International Conference on Cryptology & Network Security with Machine Learning
This paper discusses various steganography techniques and their parameters. Based on these parameters, we make some comparative analysis among different steganography methods in various research domains. We take LSB, DCT, hash function, and DWT methods for comparisons. The important parameters of the mentioned techniques are PSNR, embedding capacity, and MSE. Based on these parameters, we found that LSB method is most appropriate for implementation of video steganography having negligible error. Video steganography is a mean of hiding videos from one format to another format. The primary objective of video steganography technique is to conceal an important message (information or data) inside of an ordinary video such that an unauthorized person cannot reveal the message.
This is a preview of subscription content, log in via an institution to check access.
Access this chapter
- Available as PDF
- Read on any device
- Instant download
- Own it forever
- Available as EPUB and PDF
- Compact, lightweight edition
- Free shipping worldwide - see info
Tax calculation will be finalised at checkout
Purchases are for personal use only
Institutional subscriptions
Data Availability
The data used to support the findings of this study are available from the corresponding author upon request.
Rajkumar G, Malemath V (2017) Video steganography: secure data hiding technique. Int J Comput Netw Inform Secur 9:38–45. https://doi.org/10.5815/ijcnis.2017.09.05
Article Google Scholar
Kumar H, Singh A (2016) Internet of Things: a comprehensive analysis and security implementation through elliptic curve cryptography. Int J Curr Eng Technol 6(2):498–502
Google Scholar
Subramanian N, Elharrouss O, Al-Maadeed S, Bouridane A (2021) Image steganography: a review of the recent advances. IEEE Access 9:23409–23423. https://doi.org/10.1109/ACCESS.2021.3053998
Hossain S, Mukhopadhyay S, Ray B, Ghosal SK, Sarkar R (2022) A secured image steganography method based on ballot transform and genetic algorithm. Multimed Tools Appl 81:38429–38458
Sakshi S, Verma S, Chaturvedi P, Yadav SA (2022) Least significant bit steganography for text and image hiding. In: 2022 3rd International Conference on Intelligent Engineering and Management (ICIEM), pp 415–421
Singh R, Verma S, Yadav SA, Vikram Singh S (2022) Copy-move forgery detection using SIFT and DWT detection techniques. In: 2022 3rd International Conference on Intelligent Engineering and Management (ICIEM), pp 338–343
Rathore MS, Poongodi M, Saurabh P, Lilhore UK, Bourouis S, Alhakami W, Osamor J, Hamdi M (2022) A novel trust-based security and privacy model for Internet of Vehicles using encryption and steganography. Comput Electr Eng 102:108205
Peter G, Sherine A, Teekaraman Y, Kuppusamy R, Radhakrishnan A (2022) Histogram shifting-based quick response steganography method for secure communication. Wirel Commun Mobile Comput 2022:1–11
Li J, Zhang M, Niu K, Yang X (2022) A review of motion vector-based video steganography. Secur Commun Netw 2022:2946812. https://doi.org/10.1155/2022/2946812
Hassaballah M, Hameed MA, Awad AI, Muhammad K (2021) A novel image steganography method for industrial internet of things security. IEEE Trans Industr Inf 17:7743–7751
Rustad S, Setiadi DR, Syukur A, Andono PN (2021) Inverted LSB image steganography using adaptive pattern to improve imperceptibility. J King Saud Univ Comput Inf Sci 34:3559–3568
Fateh M, Rezvani M (2021) Irani Y (2021) A new method of coding for steganography based on LSB matching revisited. Secur Commun Netw 6610678(1–6610678):15
Soomro K, Zamir AR, Shah M (2012) UCF101: a dataset of 101 human actions classes from videos in the wild. ArXiv, abs/1212.0402
https://www-nlpir.nist.gov/projects/trecvid/past.data.table.html
Kuehne H, Jhuang H, Garrote E, Poggio TA, Serre T (2011) HMDB: a large video database for human motion recognition. Int Conf Comput Vis 2011:2556–2563
Misra MK, Chaturvedi A, Tripathi SP, Shukla V (2019) A unique key sharing protocol among three users using non-commutative group for electronic health record system. J Discr Math Sci Cryptogr 22(8):1435–1451. https://doi.org/10.1080/09720529.2019.1692450
Article MathSciNet Google Scholar
Shukla V, Chaturvedi A, Misra MK (2021) On authentication schemes using polynomials over non commutative rings. Wireless Pers Commun 118(1):1–9. https://doi.org/10.1007/s11277-020-08008
Mishra MK, Shukla V, Chaturvedi A, Bhattacharya P, Tanwar S (2023) A secure authenticated key agreement protocol using polynomials. In: Proceedings of International Conference on Recent Innovations in Computing. Lecture Notes in Electrical Engineering, vol 1001, pp 1001. Springer, Singapore. https://doi.org/10.1007/978-981-19-9876-8_44
Download references
Author information
Authors and affiliations.
CSJM University, Kanpur, UP, India
Hemant Kumar & Pushpa Mamoria
Rama University, Kanpur, UP, India
Shivani Kumari
PSIT, Kanpur, UP, India
Manoj Kumar Misra
You can also search for this author in PubMed Google Scholar
Corresponding author
Correspondence to Hemant Kumar .
Editor information
Editors and affiliations.
Department of Mathematics, Pranveer Singh Institute of Technology, Kanpur, India
Atul Chaturvedi
Department of Mathematics, Indian Institute of Technology Jammu, Jammu, India
Sartaj Ul Hasan
Applied Statistics Unit, Indian Statistical Institute, Kolkata, India
Bimal Kumar Roy
Department of Mathematics, Bar-Ilan University, Ramat-Gan, Israel
Boaz Tsaban
Ethics declarations
Conflicts of interest.
The authors declare that they have no conflicts of interest.
Rights and permissions
Reprints and permissions
Copyright information
© 2024 The Author(s), under exclusive license to Springer Nature Singapore Pte Ltd.
About this paper
Cite this paper.
Kumar, H., Mamoria, P., Kumari, S., Misra, M.K. (2024). Video Steganography Techniques: A Comprehensive Review and Performance Evaluation. In: Chaturvedi, A., Hasan, S.U., Roy, B.K., Tsaban, B. (eds) Cryptology and Network Security with Machine Learning. ICCNSML 2023. Lecture Notes in Networks and Systems, vol 918. Springer, Singapore. https://doi.org/10.1007/978-981-97-0641-9_3
Download citation
DOI : https://doi.org/10.1007/978-981-97-0641-9_3
Published : 23 April 2024
Publisher Name : Springer, Singapore
Print ISBN : 978-981-97-0640-2
Online ISBN : 978-981-97-0641-9
eBook Packages : Engineering Engineering (R0)
Share this paper
Anyone you share the following link with will be able to read this content:
Sorry, a shareable link is not currently available for this article.
Provided by the Springer Nature SharedIt content-sharing initiative
- Publish with us
Policies and ethics
- Find a journal
- Track your research
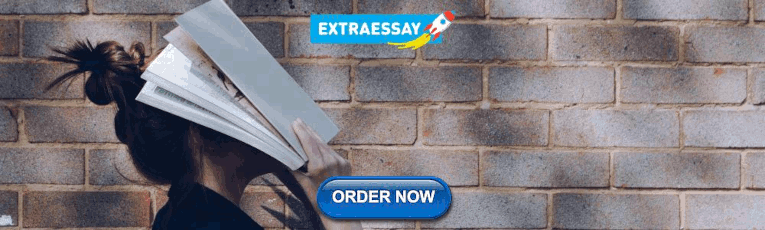
IMAGES
VIDEO
COMMENTS
Image Steganography is the process of hiding information which can be text, image or video inside a cover image. The secret information is hidden in a way that it not visible to the human eyes. Deep learning technology, which has emerged as a powerful tool in various applications including image steganography, has received increased attention recently. The main goal of this paper is to explore ...
A number of future directions are presented that can assist researchers in determining the direction of future research in image steganography. With the rapid growth of multimedia technologies, many images are communicated over public channels. ... Publication year range is selected between 2011 to 2022 from reputable databases only. Papers in ...
In this review paper, we delve into various aspects of steganography, explor ing its. techniques, detection methods, evaluation criteria, and practical applications. We analyze. steganalysis ...
At the same time, in order to improve the capacity and security of image steganography, scholars have tried to apply image segmentation to image steganography, such as Yamini, et al. [20] used real-time adaptive color image segmentation and color correlation between pixels to identify adaptive regions of the image, and used cubic interpolation for the selected regions to extract features from ...
PDF | On Jan 25, 2022, Muhammad Adnan Aslam and others published Image Steganography using Least Significant Bit (LSB) - A Systematic Literature Review | Find, read and cite all the research you ...
We reviewed several articles on steganography methods in this research paper. Steganography is an ancient and robust technique used in a variety of applications, including confidential data sharing. When used in conjunction with cryptography, steganography becomes more powerful. ... Cite this paper. Paul, T., Ghosh, S., Majumder, A. (2022). A ...
The rest of the paper is framed as follows: Section 2 explains the fundamental concepts. Section 3 specifies the general model of steganography. ... Our work bridges the research gap in applying deep steganography to address the challenges in mocap data hiding. ... 2022, Article 108205. Manjari Singh Rathore, …, Mounir Hamdi. Video ...
A review of the deep learning based image steganography techniques is presented in this paper. For completeness, the recent traditional steganography techniques are also discussed briefly. The three key parameters (security, embedding capacity, and invisibility) for measuring the quality of an image steganographic technique are described.
These CVPR 2022 papers are the Open Access versions, provided by the Computer Vision Foundation. Except for the watermark, they are identical to the accepted versions; the final published version of the proceedings is available on IEEE Xplore. ... Image steganography aims to hide secret images into a container image, where the secret is hidden ...
Image steganography is a scheme that hides secret information in a cover image without being perceived. Most of the existing steganography methods are more concerned about the visual similarity between the stego image and the cover image, and they ignore the recovery accuracy of secret information. In this paper, the steganography method based on invertible neural networks is proposed, which ...
To handle these issues, in this paper we proposed a method in view of Huffman code, Least Significant Bits (LSB) based cover steganography utilizing Multi-Level Encryption (MLE) and colorless part ...
Development of an effective and efficient data hiding technique using steganography: The research paper proposes a method that combines steganography and encryption algorithms to ensure the confidentiality and integrity of the transmitted data. The goal is to hide the message inside a cover medium to remain undetectable to attackers.
This paper aims to present a systematic review of image steganography approaches, including their basic concepts, performance parameters, and types, and a number of future directions are presented that can assist researchers in determining the direction of future research in image Steganography. With the rapid growth of multimedia technologies, many images are communicated over public channels.
Steganography, one type of information hiding, is a science and art of transmitting secret bits in a covert channel [1, 2].Different from watermarking technique for protecting copyrights [], steganography conceals the existence of covert communication by embedding message into media as cover.Conventional media, including text [], image [], audio [], video [], are widely used for steganography ...
Steganography is the art of concealing information in a cover media in such a way that the presence of the information is unknown. Digital image steganography accomplishes the potential for protected communication that is crucial in most of the applications nowadays. Steganography has several beneficial applications.
Steganography is a technique for covert communication between two parties. With the rapid development of deep neural networks (DNN), more and more steganographic networks are proposed recently, which are shown to be promising to achieve good performance. Unlike the traditional handcrafted steganographic tools, a steganographic network is relatively large in size. It raises concerns on how to ...
Explore the latest full-text research PDFs, articles, conference papers, preprints and more on IMAGE STEGANOGRAPHY. Find methods information, sources, references or conduct a literature review on ...
Steganography is the art of concealing data into any multimedia for covert communication [].Steganography can be divided into many types based on the cover object used to attain security, like text, image, audio, video, and network [26,27,28,29,30,31].Further, the steganography can be divided into two domains based on the techniques used to hide data in any multimedia, i.e., spatial domain ...
Image Pixels . Lsb Technique. Steganography is one of the cryptography techniques where secret information can be hidden through multimedia files such as images and videos. Steganography can offer a way of exchanging secret and encrypted information in an untypical mechanism where communicating parties can only interpret the secret message.
The method of steganography is used to keep data safe. Video steganography is a technique for concealing a secret message or piece of information (picture or video) within a video file. As a result, this study examines the various ways of video steganography and determines which is the most effective.
Section 2 presents the state of the art of neural style transfer and deep learning-based image steganography technologies. Section 3 presents the proposed image steganography frameworks for neural style transfer in detail. Then, Section 4 carries out the experiment comparison and analysis. Section 5 gives the conclusion and future research ...
This paper focuses on the study of steganoprahy as an anti-forensic method. The purpose of steganography techniques is to hide information from individuals unrelated to its content.
Both the FBI and BJS data show dramatic declines in U.S. violent and property crime rates since the early 1990s, when crime spiked across much of the nation. Using the FBI data, the violent crime rate fell 49% between 1993 and 2022, with large decreases in the rates of robbery (-74%), aggravated assault (-39%) and murder/nonnegligent ...