No internet connection.
All search filters on the page have been cleared., your search has been saved..
- Sign in to my profile My Profile
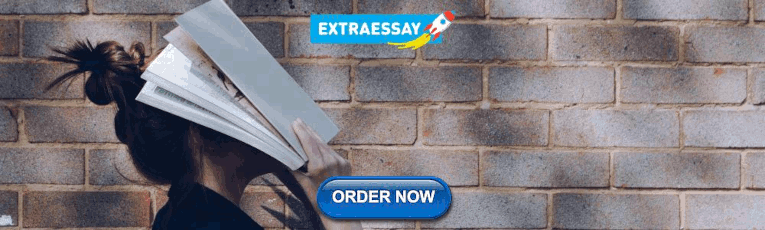
100 Questions (and Answers) About Action Research
- By: Luke Duesbery & Todd Twyman
- Publisher: SAGE Publications, Inc.
- Series: SAGE 100 Questions and Answers
- Publication year: 2020
- Online pub date: February 08, 2021
- Discipline: Business and Management
- Methods: Action research , Research questions , Research design
- DOI: https:// doi. org/10.4135/9781544305455
- Keywords: decision making , health sciences , journals , social justice , social media , students , teaching Show all Show less
- Print ISBN: 9781544305431
- Online ISBN: 9781071849408
- Buy the book icon link
Subject index
100 Questions (and Answers) About Action Research identifies and answers the essential questions on the process of systematically approaching your practice from an inquiry-oriented perspective, with a focus on improving that practice. This unique text offers progressive instructors an alternative to the research status quo and serves as a reference for readers to improve their practice as advocates for those they serve. The Question and Answer format makes this an ideal supplementary text for traditional research methods courses, and also a helpful guide for practitioners in education, social work, criminal justice, health, business, and other applied disciplines.
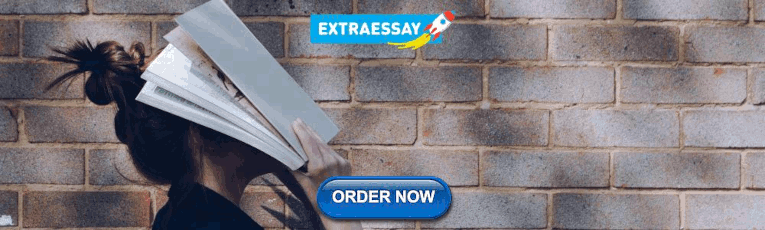
Front Matter
- Acknowledgments
- About the Authors
- What Is This Book About?
- What Is Action Research, and Where Did It Come From?
- What Are the Key Features of Action Research I Should Remember?
- How Is Action Research Like and Unlike Other Research?
- How Do You Choose Your Action Research Style?
- Why Should I Bother With Action Research?
- How Is Action Research Cyclical?
- How Much Time Does an Action Research Project Really Take?
- What Is an Action Research Disposition?
- Why Is Knowing Yourself Important While Doing Action Research?
- Do I Need to Know About Statistics for Action Research?
- What’s the Difference Between Theory and Conceptual Frameworks in Action Research?
- How Does Action Research Fit in Education?
- How Does Action Research Fit in the Health Sciences?
- How Does Action Research Fit in the Behavioral and Social Sciences?
- How Does Action Research Fit in Business, Management, and Industry?
- How Can a Gender Theory Approach Impact Action Research?
- How Does Critical Race Theory Impact Action Research?
- What Is the Difference Between Exploratory and Confirmatory Action Research?
- What Are the Weaknesses of Action Research?
- How Can Action Research Be a Form of Social Justice?
- Why Is Ethics Important in Educational Research and Action Research in Particular?
- Do I Need to Get Some Kind of Approval for My Action Research?
- What Is Informed Consent, and Why Is It Important?
- Who Are the Stakeholders in Action Research, and Why Are They Important?
- Why Is It Important to Strive for Confidentiality and Anonymity?
- Should I Treat Children in Action Research Differently Than Adults?
- What Does a Sample Consent Letter Look Like?
- What’s a Literature Review, and Do I Need One?
- What Are the Steps in Doing an Efficient Literature Review or Synthesis?
- Should I Read Action Research Articles?
- How Do I Evaluate the Articles I Find?
- How Do I Plan for My Action Research Project Before I Start?
- How Do I Narrow Down My Topic?
- How Do I Make Sure My Research Is Rigorous?
- What Do Good Action Research Questions Look Like?
- What Is the Difference Between Qualitative and Quantitative Approaches to Action Research Design?
- What Are Common Qualitative Research Designs in Action Research?
- What Are Common Quantitative Research Designs in Action Research?
- What Are Some Better Quantitative Action Research Designs?
- What Are Some Common Mixed-Method Action Research Designs?
- Can a Program Evaluation Also Be Action Research?
- How Do I Choose My Action Research Design?
- What Are Referents, and Which Work Best With Action Research?
- How Do I Randomly Choose My Participants?
- How Do I Not Randomly Choose My Participants?
- How Do I Create Groups?
- What Is a Case Study?
- When and How Would I Use Interviews?
- In General, What Are the Different Kinds of Interviews?
- How Do I Write Interview Questions and Interpret the Answers?
- Why Would I Use Focus Groups?
- How Do I Conduct Focus Groups?
- How Do Ethnographic Data Collection Methods Support Action Research?
- Can You Tell Me What I Need to Know About Surveys?
- How Do I Write Good Survey Questions?
- Can I Use Direct Observations in Action Research?
- What Are the Elements of Conducting Effective Observations?
- How Do Interval Observations Enrich Action Research?
- What Are Experimental Designs, and Are They Practical in Action Research?
- What Are Quasi-experimental Designs, and Where Do They Fit in?
- Can You Tell Me More About Action Research Designs That Use Groups?
- Do I Collect Data Once or a Bunch of Times?
- Are Single-Case and Single-Subject Designs Different?
- I Wrote My Question and Chose My Design. What Kind of Data Do I Need?
- How Are Descriptive and Inferential Statistics Different?
- What Is Visual Data Analysis?
- What Does It Mean to Triangulate My Data?
- What’s the Big Deal About Reliability?
- What’s the Big Deal About Validity?
- Why Do I Need to Care About Internal Validity?
- What Kind of Threats to Internal Validity Are Common in Action Research?
- What Are Blocking Variables?
- What Are Ordinal, Interval, and Categorical Data Types, and Which Works Best With Action Research?
- Does Generalizability Matter in Action Research?
- Now I Have All These Data. What Is the Best Way to Present My Results?
- How Do I Tell a Story With My Data?
- What Is the Difference Between the Mean and Median, and Why Is It Important?
- How Do Inferential Statistics Work?
- How Can I Use Inferential Statistics in My Action Research?
- What Is an Effect Size, and Who Is Cohen?
- How Do I Deal With Missing Data?
- What Are Some Good Action Research Analytic Tools I Can Use?
- Why Is Using Data Displays an Important Step?
- What Are the Elements of a Good Table?
- What Are the Elements of a Good Graph?
- How Do I Evaluate the Quality of My Action Research Project?
- Now That I Am Done, How Do I Add Value to the Field?
- What Professional Organizations Are Available to Give Me Support?
- Are Social Media Outlets Appropriate for Sharing My Action Research?
- Can I Formally Publish My Action Research Project?
- What Are the Most Helpful Action Research Journals?
- Should I Repeat (Replicate) My Action Research Project?
- Is Action Research in Any Way Political? How Do I Navigate That?
- How Can I Create an Inquiry-Oriented Disposition in My Everyday Life?
- Why Develop an Action Research Community?
- Why Would I Want or Need to Write a Report on My Action Research?
- What Are the Key Elements of an Action Research Report?
- I Really Liked Learning About Action Research. What Is Next?
- What Other Books in This Series Might Interest Me, and How Do They Fit Together?
Back Matter
- Appendix : Important Action Research Literature
Sign in to access this content
Get a 30 day free trial, more like this, sage recommends.
We found other relevant content for you on other Sage platforms.
Have you created a personal profile? Login or create a profile so that you can save clips, playlists and searches
- Sign in/register
Navigating away from this page will delete your results
Please save your results to "My Self-Assessments" in your profile before navigating away from this page.
Sign in to my profile
Please sign into your institution before accessing your profile
Sign up for a free trial and experience all Sage Learning Resources have to offer.
You must have a valid academic email address to sign up.
Get off-campus access
- View or download all content my institution has access to.
Sign up for a free trial and experience all Sage Learning Resources has to offer.
- view my profile
- view my lists
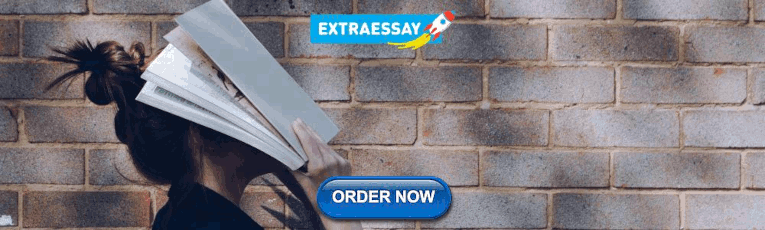