Have a language expert improve your writing
Run a free plagiarism check in 10 minutes, generate accurate citations for free.
- Knowledge Base
Methodology
- Random Assignment in Experiments | Introduction & Examples
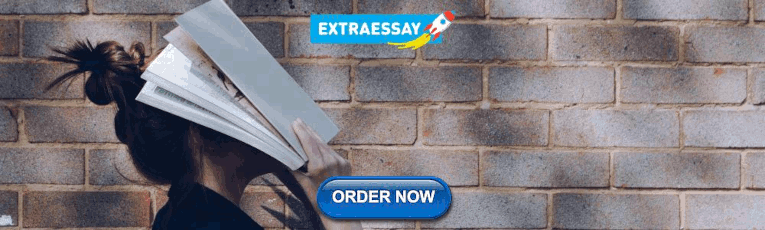
Random Assignment in Experiments | Introduction & Examples
Published on March 8, 2021 by Pritha Bhandari . Revised on June 22, 2023.
In experimental research, random assignment is a way of placing participants from your sample into different treatment groups using randomization.
With simple random assignment, every member of the sample has a known or equal chance of being placed in a control group or an experimental group. Studies that use simple random assignment are also called completely randomized designs .
Random assignment is a key part of experimental design . It helps you ensure that all groups are comparable at the start of a study: any differences between them are due to random factors, not research biases like sampling bias or selection bias .
Table of contents
Why does random assignment matter, random sampling vs random assignment, how do you use random assignment, when is random assignment not used, other interesting articles, frequently asked questions about random assignment.
Random assignment is an important part of control in experimental research, because it helps strengthen the internal validity of an experiment and avoid biases.
In experiments, researchers manipulate an independent variable to assess its effect on a dependent variable, while controlling for other variables. To do so, they often use different levels of an independent variable for different groups of participants.
This is called a between-groups or independent measures design.
You use three groups of participants that are each given a different level of the independent variable:
- a control group that’s given a placebo (no dosage, to control for a placebo effect ),
- an experimental group that’s given a low dosage,
- a second experimental group that’s given a high dosage.
Random assignment to helps you make sure that the treatment groups don’t differ in systematic ways at the start of the experiment, as this can seriously affect (and even invalidate) your work.
If you don’t use random assignment, you may not be able to rule out alternative explanations for your results.
- participants recruited from cafes are placed in the control group ,
- participants recruited from local community centers are placed in the low dosage experimental group,
- participants recruited from gyms are placed in the high dosage group.
With this type of assignment, it’s hard to tell whether the participant characteristics are the same across all groups at the start of the study. Gym-users may tend to engage in more healthy behaviors than people who frequent cafes or community centers, and this would introduce a healthy user bias in your study.
Although random assignment helps even out baseline differences between groups, it doesn’t always make them completely equivalent. There may still be extraneous variables that differ between groups, and there will always be some group differences that arise from chance.
Most of the time, the random variation between groups is low, and, therefore, it’s acceptable for further analysis. This is especially true when you have a large sample. In general, you should always use random assignment in experiments when it is ethically possible and makes sense for your study topic.
Here's why students love Scribbr's proofreading services
Discover proofreading & editing
Random sampling and random assignment are both important concepts in research, but it’s important to understand the difference between them.
Random sampling (also called probability sampling or random selection) is a way of selecting members of a population to be included in your study. In contrast, random assignment is a way of sorting the sample participants into control and experimental groups.
While random sampling is used in many types of studies, random assignment is only used in between-subjects experimental designs.
Some studies use both random sampling and random assignment, while others use only one or the other.
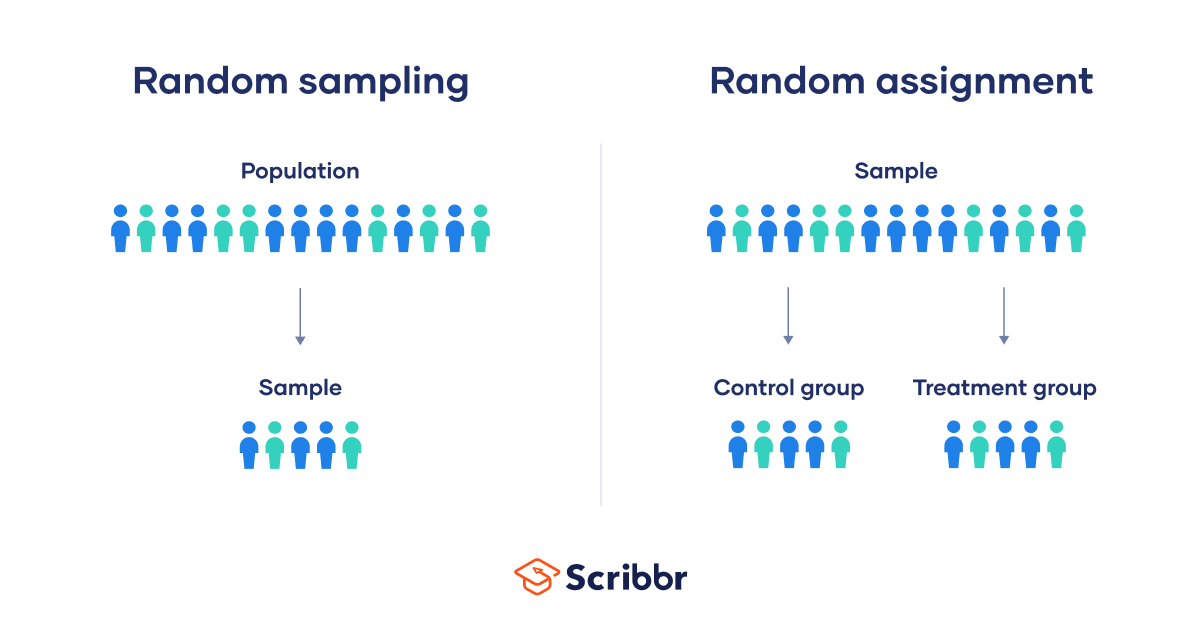
Random sampling enhances the external validity or generalizability of your results, because it helps ensure that your sample is unbiased and representative of the whole population. This allows you to make stronger statistical inferences .
You use a simple random sample to collect data. Because you have access to the whole population (all employees), you can assign all 8000 employees a number and use a random number generator to select 300 employees. These 300 employees are your full sample.
Random assignment enhances the internal validity of the study, because it ensures that there are no systematic differences between the participants in each group. This helps you conclude that the outcomes can be attributed to the independent variable .
- a control group that receives no intervention.
- an experimental group that has a remote team-building intervention every week for a month.
You use random assignment to place participants into the control or experimental group. To do so, you take your list of participants and assign each participant a number. Again, you use a random number generator to place each participant in one of the two groups.
To use simple random assignment, you start by giving every member of the sample a unique number. Then, you can use computer programs or manual methods to randomly assign each participant to a group.
- Random number generator: Use a computer program to generate random numbers from the list for each group.
- Lottery method: Place all numbers individually in a hat or a bucket, and draw numbers at random for each group.
- Flip a coin: When you only have two groups, for each number on the list, flip a coin to decide if they’ll be in the control or the experimental group.
- Use a dice: When you have three groups, for each number on the list, roll a dice to decide which of the groups they will be in. For example, assume that rolling 1 or 2 lands them in a control group; 3 or 4 in an experimental group; and 5 or 6 in a second control or experimental group.
This type of random assignment is the most powerful method of placing participants in conditions, because each individual has an equal chance of being placed in any one of your treatment groups.
Random assignment in block designs
In more complicated experimental designs, random assignment is only used after participants are grouped into blocks based on some characteristic (e.g., test score or demographic variable). These groupings mean that you need a larger sample to achieve high statistical power .
For example, a randomized block design involves placing participants into blocks based on a shared characteristic (e.g., college students versus graduates), and then using random assignment within each block to assign participants to every treatment condition. This helps you assess whether the characteristic affects the outcomes of your treatment.
In an experimental matched design , you use blocking and then match up individual participants from each block based on specific characteristics. Within each matched pair or group, you randomly assign each participant to one of the conditions in the experiment and compare their outcomes.
Sometimes, it’s not relevant or ethical to use simple random assignment, so groups are assigned in a different way.
When comparing different groups
Sometimes, differences between participants are the main focus of a study, for example, when comparing men and women or people with and without health conditions. Participants are not randomly assigned to different groups, but instead assigned based on their characteristics.
In this type of study, the characteristic of interest (e.g., gender) is an independent variable, and the groups differ based on the different levels (e.g., men, women, etc.). All participants are tested the same way, and then their group-level outcomes are compared.
When it’s not ethically permissible
When studying unhealthy or dangerous behaviors, it’s not possible to use random assignment. For example, if you’re studying heavy drinkers and social drinkers, it’s unethical to randomly assign participants to one of the two groups and ask them to drink large amounts of alcohol for your experiment.
When you can’t assign participants to groups, you can also conduct a quasi-experimental study . In a quasi-experiment, you study the outcomes of pre-existing groups who receive treatments that you may not have any control over (e.g., heavy drinkers and social drinkers). These groups aren’t randomly assigned, but may be considered comparable when some other variables (e.g., age or socioeconomic status) are controlled for.
Prevent plagiarism. Run a free check.
If you want to know more about statistics , methodology , or research bias , make sure to check out some of our other articles with explanations and examples.
- Student’s t -distribution
- Normal distribution
- Null and Alternative Hypotheses
- Chi square tests
- Confidence interval
- Quartiles & Quantiles
- Cluster sampling
- Stratified sampling
- Data cleansing
- Reproducibility vs Replicability
- Peer review
- Prospective cohort study
Research bias
- Implicit bias
- Cognitive bias
- Placebo effect
- Hawthorne effect
- Hindsight bias
- Affect heuristic
- Social desirability bias
In experimental research, random assignment is a way of placing participants from your sample into different groups using randomization. With this method, every member of the sample has a known or equal chance of being placed in a control group or an experimental group.
Random selection, or random sampling , is a way of selecting members of a population for your study’s sample.
In contrast, random assignment is a way of sorting the sample into control and experimental groups.
Random sampling enhances the external validity or generalizability of your results, while random assignment improves the internal validity of your study.
Random assignment is used in experiments with a between-groups or independent measures design. In this research design, there’s usually a control group and one or more experimental groups. Random assignment helps ensure that the groups are comparable.
In general, you should always use random assignment in this type of experimental design when it is ethically possible and makes sense for your study topic.
To implement random assignment , assign a unique number to every member of your study’s sample .
Then, you can use a random number generator or a lottery method to randomly assign each number to a control or experimental group. You can also do so manually, by flipping a coin or rolling a dice to randomly assign participants to groups.
Cite this Scribbr article
If you want to cite this source, you can copy and paste the citation or click the “Cite this Scribbr article” button to automatically add the citation to our free Citation Generator.
Bhandari, P. (2023, June 22). Random Assignment in Experiments | Introduction & Examples. Scribbr. Retrieved April 15, 2024, from https://www.scribbr.com/methodology/random-assignment/
Is this article helpful?
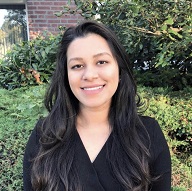
Pritha Bhandari
Other students also liked, guide to experimental design | overview, steps, & examples, confounding variables | definition, examples & controls, control groups and treatment groups | uses & examples, "i thought ai proofreading was useless but..".
I've been using Scribbr for years now and I know it's a service that won't disappoint. It does a good job spotting mistakes”
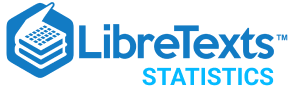
- school Campus Bookshelves
- menu_book Bookshelves
- perm_media Learning Objects
- login Login
- how_to_reg Request Instructor Account
- hub Instructor Commons
- Download Page (PDF)
- Download Full Book (PDF)
- Periodic Table
- Physics Constants
- Scientific Calculator
- Reference & Cite
- Tools expand_more
- Readability
selected template will load here
This action is not available.
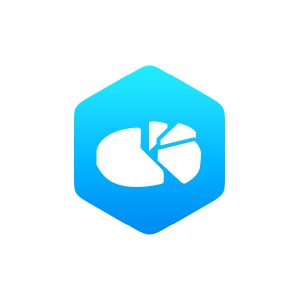
1.3: Threats to Internal Validity and Different Control Techniques
- Last updated
- Save as PDF
- Page ID 32915
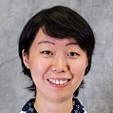
- Yang Lydia Yang
- Kansas State University
Internal validity is often the focus from a research design perspective. To understand the pros and cons of various designs and to be able to better judge specific designs, we identify specific threats to internal validity . Before we do so, it is important to note that the primary challenge to establishing internal validity in social sciences is the fact that most of the phenomena we care about have multiple causes and are often a result of some complex set of interactions. For example, X may be only a partial cause of Y or X may cause Y, but only when Z is present. Multiple causation and interactive effects make it very difficult to demonstrate causality. Turning now to more specific threats, Figure 1.3.1 below identifies common threats to internal validity.
Different Control Techniques
All of the common threats mentioned above can introduce extraneous variables into your research design, which will potentially confound your research findings. In other words, we won't be able to tell whether it is the independent variable (i.e., the treatment we give participants), or the extraneous variable, that causes the changes in the dependent variable. Controlling for extraneous variables reduces its threats on the research design and gives us a better chance to claim the independent variable causes the changes in the dependent variable, i.e., internal validity. There are different techniques we can use to control for extraneous variables.
Random assignment
Random assignment is the single most powerful control technique we can use to minimize the potential threats of the confounding variables in research design. As we have seen in Dunn and her colleagues' study earlier, participants are not allowed to self select into either conditions (spend $20 on self or spend on others). Instead, they are randomly assigned into either group by the researcher(s). By doing so, the two groups are likely to be similar on all other factors except the independent variable itself. One confounding variable mentioned earlier is whether individuals had a happy childhood to begin with. Using random assignment, those who had a happy childhood will likely end up in each condition group. Similarly, those who didn't have a happy childhood will likely end up in each condition group too. As a consequence, we can expect the two condition groups to be very similar on this confounding variable. Applying the same logic, we can use random assignment to minimize all potential confounding variables (assuming your sample size is large enough!). With that, the only difference between the two groups is the condition participants are assigned to, which is the independent variable, then we are confident to infer that the independent variable actually causes the differences in the dependent variables.
It is critical to emphasize that random assignment is the only control technique to control for both known and unknown confounding variables. With all other control techniques mentioned below, we must first know what the confounding variable is before controlling it. Random assignment does not. With the simple act of randomly assigning participants into different conditions, we take care both the confounding variables we know of and the ones we don't even know that could threat the internal validity of our studies. As the saying goes, "what you don't know will hurt you." Random assignment take cares of it.
Matching is another technique we can use to control for extraneous variables. We must first identify the extraneous variable that can potentially confound the research design. Then we want to rank order the participants on this extraneous variable or list the participants in a ascending or descending order. Participants who are similar on the extraneous variable will be placed into different treatment groups. In other words, they are "matched" on the extraneous variable. Then we can carry out the intervention/treatment as usual. If different treatment groups do show differences on the dependent variable, we would know it is not the extraneous variables because participants are "matched" or equivalent on the extraneous variable. Rather it is more likely to the independent variable (i.e., the treatments) that causes the changes in the dependent variable. Use the example above (self-spending vs. others-spending on happiness) with the same extraneous variable of whether individuals had a happy childhood to begin with. Once we identify this extraneous variable, we do need to first collect some kind of data from the participants to measure how happy their childhood was. Or sometimes, data on the extraneous variables we plan to use may be already available (for example, you want to examine the effect of different types of tutoring on students' performance in Calculus I course and you plan to match them on this extraneous variable: college entrance test scores, which is already collected by the Admissions Office). In either case, getting the data on the identified extraneous variable is a typical step we need to do before matching. So going back to whether individuals had a happy childhood to begin with. Once we have data, we'd sort it in a certain order, for example, from the highest score (meaning participants reporting the happiest childhood) to the lowest score (meaning participants reporting the least happy childhood). We will then identify/match participants with the highest levels of childhood happiness and place them into different treatment groups. Then we go down the scale and match participants with relative high levels of childhood happiness and place them into different treatment groups. We repeat on the descending order until we match participants with the lowest levels of childhood happiness and place them into different treatment groups. By now, each treatment group will have participants with a full range of levels on childhood happiness (which is a strength...thinking about the variation, the representativeness of the sample). The two treatment groups will be similar or equivalent on this extraneous variable. If the treatments, self-spending vs. other-spending, eventually shows the differences on individual happiness, then we know it's not due to how happy their childhood was. We will be more confident it is due to the independent variable.
You may be thinking, but wait we have only taken care of one extraneous variable. What about other extraneous variables? Good thinking.That's exactly correct. We mentioned a few extraneous variables but have only matched them on one. This is the main limitation of matching. You can match participants on more than one extraneous variables, but it's cumbersome, if not impossible, to match them on 10 or 20 extraneous variables. More importantly, the more variables we try to match participants on, the less likely we will have a similar match. In other words, it may be easy to find/match participants on one particular extraneous variable (similar level of childhood happiness), but it's much harder to find/match participants to be similar on 10 different extraneous variables at once.
Holding Extraneous Variable Constant
Holding extraneous variable constant control technique is self-explanatory. We will use participants at one level of extraneous variable only, in other words, holding the extraneous variable constant. Using the same example above, for example we only want to study participants with the low level of childhood happiness. We do need to go through the same steps as in Matching: identifying the extraneous variable that can potentially confound the research design and getting the data on the identified extraneous variable. Once we have the data on childhood happiness scores, we will only include participants on the lower end of childhood happiness scores, then place them into different treatment groups and carry out the study as before. If the condition groups, self-spending vs. other-spending, eventually shows the differences on individual happiness, then we know it's not due to how happy their childhood was (since we already picked those on the lower end of childhood happiness only). We will be more confident it is due to the independent variable.
Similarly to Matching, we have to do this one extraneous variable at a time. As we increase the number of extraneous variables to be held constant, the more difficult it gets. The other limitation is by holding extraneous variable constant, we are excluding a big chunk of participants, in this case, anyone who are NOT low on childhood happiness. This is a major weakness, as we reduce the variability on the spectrum of childhood happiness levels, we decreases the representativeness of the sample and generalizabiliy suffers.
Building Extraneous Variables into Design
The last control technique building extraneous variables into research design is widely used. Like the name suggests, we would identify the extraneous variable that can potentially confound the research design, and include it into the research design by treating it as an independent variable. This control technique takes care of the limitation the previous control technique, holding extraneous variable constant, has. We don't need to excluding participants based on where they stand on the extraneous variable(s). Instead we can include participants with a wide range of levels on the extraneous variable(s). You can include multiple extraneous variables into the design at once. However, the more variables you include in the design, the large the sample size it requires for statistical analyses, which may be difficult to obtain due to limitations of time, staff, cost, access, etc.
- Privacy Policy
Buy Me a Coffee

Home » Internal Validity – Threats, Examples and Guide
Internal Validity – Threats, Examples and Guide
Table of Contents

Internal Validity
Definition:
Internal validity refers to the extent to which a research study accurately establishes a cause-and-effect relationship between the independent variable (s) and the dependent variable (s) being investigated. It assesses whether the observed changes in the dependent variable(s) are actually caused by the manipulation of the independent variable(s) rather than other extraneous factors.
How to Increase Internal Validity
To enhance internal validity, researchers need to carefully design and conduct their studies. Here are some considerations for improving internal validity:
- Random Assignment: Use random assignment to allocate participants to different groups in experimental studies. Random assignment helps ensure that the groups are comparable, minimizing the influence of individual differences on the results.
- Control Group: Include a control group in experimental studies. This group should be similar to the experimental group but not exposed to the treatment or intervention being tested. The control group helps establish a baseline against which the effects of the treatment can be compared.
- Control Extraneous Variables: Identify and control for extraneous variables that could potentially influence the relationship being studied. This can be achieved through techniques like matching participants, using homogeneous samples, or statistically controlling for the variables.
- Standardized Procedures: Use standardized procedures and protocols across all participants and conditions. This helps ensure consistency in the administration of the study, reducing the potential for systematic biases.
- Counterbalancing: In studies with multiple conditions or treatment sequences, employ counterbalancing techniques. This involves systematically varying the order of conditions or treatments across participants to eliminate any potential order effects.
- Minimize Experimenter Bias: Take steps to minimize experimenter bias or expectancy effects. These biases can inadvertently influence the behavior of participants or the interpretation of results. Using blind or double-blind procedures, where the experimenter is unaware of the conditions or group assignments, can help mitigate these biases.
- Use Reliable and Valid Measures: Ensure that the measures used in the study are reliable and valid. Reliable measures yield consistent results, while valid measures accurately assess the construct being measured.
- Pilot Testing: Conduct pilot testing before the main study to refine the study design and procedures. Pilot testing helps identify potential issues, such as unclear instructions or unforeseen confounds, and allows for necessary adjustments to enhance internal validity.
- Sample Size: Increase the sample size to improve statistical power and reduce the likelihood of random variation influencing the results. Adequate sample sizes increase the generalizability and reliability of the findings.
- Researcher Bias: Researchers need to be aware of their own biases and take steps to minimize their impact on the study. This can be done through careful experimental design, blind data collection and analysis, and the use of standardized protocols.
Threats To Internal Validity
Several threats can undermine internal validity and compromise the validity of research findings. Here are some common threats to internal validity:
Events or circumstances that occur during the course of a study and affect the outcome, making it difficult to attribute the results solely to the treatment or intervention being studied.
Changes that naturally occur in participants over time, such as physical or psychological development, which can influence the results independently of the treatment or intervention.
Testing Effects
The act of being tested or measured on a particular variable in an initial assessment may influence participants’ subsequent responses. This effect can arise due to familiarity with the test or increased sensitization to the topic being studied.
Instrumentation
Changes or inconsistencies in the measurement tools or procedures used across different stages or conditions of the study. If the measurement methods are not standardized or if there are variations in the administration of tests, it can lead to measurement errors and threaten internal validity.
Selection Bias
When there are systematic differences between the characteristics of individuals selected for different groups or conditions in a study. If participants are not randomly assigned to groups or conditions, the results may be influenced by pre-existing differences rather than the treatment itself.
Attrition or Dropout
The loss of participants from a study over time can introduce bias if those who drop out differ systematically from those who remain. The characteristics of participants who drop out may affect the outcomes and compromise internal validity.
Regression to The Mean
The tendency for extreme scores on a variable to move closer to the average on subsequent measurements. If participants are selected based on extreme scores, their scores are likely to regress toward the mean in subsequent measurements, leading to erroneous conclusions about the effectiveness of a treatment.
Diffusion of Treatment
When participants in one group of a study receive knowledge or benefits from participants in another group, it can dilute the treatment effect and compromise internal validity. This can occur through communication or sharing of information among participants.
Demand Characteristics
Cues or expectations within a study that may influence participants to respond in a certain way or guess the purpose of the research. Participants may modify their behavior to align with perceived expectations, leading to biased results.
Experimenter Bias
Biases or expectations on the part of the researchers that may unintentionally influence the study’s outcomes. Researchers’ behavior, interactions, or inadvertent cues can impact participants’ responses, introducing bias and threatening internal validity.
Types of Internal Validity
There are several types of internal validity that researchers consider when designing and conducting studies. Here are some common types of internal validity:
Construct validity
Refers to the extent to which the operational definitions of the variables used in the study accurately represent the theoretical concepts they are intended to measure. It ensures that the measurements or manipulations used in the study accurately reflect the intended constructs.
Statistical Conclusion Validity
Relates to the degree to which the statistical analysis accurately reflects the relationships between variables. It involves ensuring that the appropriate statistical tests are used, the data is analyzed correctly, and the reported findings are reliable.
Internal Validity of Causal Inferences
Focuses on establishing a cause-and-effect relationship between the independent variable (treatment or intervention) and the dependent variable (outcome or response variable). It involves eliminating alternative explanations or confounding factors that could account for the observed relationship.
Temporal Precedence
Ensures that the cause (independent variable) precedes the effect (dependent variable) in time. It establishes the temporal sequence necessary for making causal claims.
Covariation
Refers to the presence of a relationship or association between the independent variable and the dependent variable. It ensures that changes in the independent variable are accompanied by corresponding changes in the dependent variable.
Elimination of Confounding Variables
Involves controlling for and minimizing the influence of extraneous variables that could affect the relationship between the independent and dependent variables. It helps isolate the true effect of the independent variable on the dependent variable.
Selection bias Control
Ensures that the process of assigning participants to different groups or conditions (randomization) is unbiased. Random assignment helps create equivalent groups, reducing the influence of participant characteristics on the dependent variable.
Controlling for Testing Effects
Involves minimizing the impact of repeated testing or measurement on participants’ responses. Counterbalancing, using control groups, or employing appropriate time intervals between assessments can help control for testing effects.
Controlling for Experimenter Effects
Aims to minimize the influence of the experimenter on participants’ responses. Blinding, using standardized protocols, or automating data collection processes can reduce the potential for experimenter bias.
Replication
Conducting the study multiple times with different samples or settings to verify the consistency and generalizability of the findings. Replication enhances internal validity by ensuring that the observed effects are not due to chance or specific characteristics of the study sample.
Internal Validity Examples
Here are some real-time examples that illustrate internal validity:
Drug Trial: A pharmaceutical company conducts a clinical trial to test the effectiveness of a new medication for treating a specific disease. The study uses a randomized controlled design, where participants are randomly assigned to receive either the medication or a placebo. The internal validity is high because the random assignment helps ensure that any observed differences between the groups can be attributed to the medication rather than other factors.
Education Intervention: A researcher investigates the impact of a new teaching method on student performance in mathematics. The researcher selects two comparable groups of students from the same school and randomly assigns one group to receive the new teaching method while the other group continues with the traditional method. By controlling for factors such as the school environment and student characteristics, the study enhances internal validity by isolating the effects of the teaching method.
Psychological Experiment: A psychologist conducts an experiment to examine the relationship between sleep deprivation and cognitive performance. Participants are randomly assigned to either a sleep-deprived group or a control group. The internal validity is strengthened by manipulating the independent variable (amount of sleep) and controlling for other variables that could influence cognitive performance, such as age, gender, and prior sleep habits.
Quasi-Experimental Study: A researcher investigates the impact of a new traffic law on accident rates in a specific city. Since random assignment is not feasible, the researcher selects two similar neighborhoods: one where the law is implemented and another where it is not. By comparing accident rates before and after the law’s implementation in both areas, the study attempts to establish a causal relationship while acknowledging potential confounding variables, such as driver behavior or road conditions.
Workplace Training Program: An organization introduces a new training program aimed at improving employee productivity. To assess the effectiveness of the program, the company implements a pre-post design where performance metrics are measured before and after the training. By tracking changes in productivity within the same group of employees, the study attempts to attribute any improvements to the training program while controlling for individual differences.
Applications of Internal Validity
Internal validity is a crucial concept in research design and is applicable across various fields of study. Here are some applications of internal validity:
Experimental Research
Internal validity is particularly important in experimental research, where researchers manipulate independent variables to determine their effects on dependent variables. By ensuring strong internal validity, researchers can confidently attribute any observed changes in the dependent variable to the manipulation of the independent variable, establishing a cause-and-effect relationship.
Quasi-experimental Research
Quasi-experimental studies aim to establish causal relationships but lack random assignment to groups. Internal validity becomes crucial in such designs to minimize alternative explanations for the observed effects. Careful selection and control of potential confounding variables help strengthen internal validity in quasi-experimental research.
Observational Studies
While observational studies may not involve experimental manipulation, internal validity is still relevant. Researchers need to identify and control for confounding variables to establish a relationship between variables of interest and rule out alternative explanations for observed associations.
Program Evaluation
Internal validity is essential in evaluating the effectiveness of interventions, programs, or policies. By designing rigorous evaluation studies with strong internal validity, researchers can determine whether the observed outcomes can be attributed to the specific intervention or program being evaluated.
Clinical Trials
Internal validity is critical in clinical trials to determine the effectiveness of new treatments or therapies. Well-designed randomized controlled trials (RCTs) with strong internal validity can provide reliable evidence on the efficacy of interventions and guide clinical decision-making.
Longitudinal Studies
Longitudinal studies track participants over an extended period to examine changes and establish causal relationships. Maintaining internal validity throughout the study helps ensure that observed changes in the dependent variable(s) are indeed caused by the independent variable(s) under investigation and not other factors.
Psychology and Social Sciences
Internal validity is pertinent in psychological and social science research. Researchers aim to understand human behavior and social phenomena, and establishing strong internal validity allows them to draw accurate conclusions about the causal relationships between variables.
Advantages of Internal Validity
Internal validity is essential in research for several reasons. Here are some of the advantages of having high internal validity in a study:
- Causal Inference: Internal validity allows researchers to make valid causal inferences. When a study has high internal validity, it establishes a cause-and-effect relationship between the independent variable (treatment or intervention) and the dependent variable (outcome). This provides confidence that changes in the dependent variable are genuinely due to the manipulation of the independent variable.
- Elimination of Confounding Factors: High internal validity helps eliminate or control confounding factors that could influence the relationship being studied. By systematically accounting for potential confounds, researchers can attribute the observed effects to the intended independent variable rather than extraneous variables.
- Accuracy of Measurements: Internal validity ensures accurate and reliable measurements. Researchers employ rigorous methods to measure variables, reducing measurement errors and increasing the validity and precision of the data collected.
- Replicability and Generalizability: Studies with high internal validity are more likely to yield consistent results when replicated by other researchers. This is important for the advancement of scientific knowledge, as replication strengthens the validity of findings and allows for the generalizability of results across different populations and settings.
- Intervention Effectiveness: High internal validity helps determine the effectiveness of interventions or treatments. By controlling for confounding factors and utilizing robust research designs, researchers can accurately assess whether an intervention produces the desired outcomes or effects.
- Enhanced Decision-making: Studies with high internal validity provide a solid basis for decision-making. Policymakers, practitioners, and professionals can rely on research with high internal validity to make informed decisions about the implementation of interventions or treatments in real-world settings.
- Validity of Theory Development: Internal validity contributes to the development and refinement of theories. By establishing strong cause-and-effect relationships, researchers can build and test theories, enhancing our understanding of underlying mechanisms and contributing to theoretical advancements.
- Scientific Credibility: Research with high internal validity enhances the overall credibility of the scientific field. Studies that prioritize internal validity uphold the rigorous standards of scientific inquiry and contribute to the accumulation of reliable knowledge.
Limitations of Internal Validity
While internal validity is crucial for research, it is important to recognize its limitations. Here are some limitations or considerations associated with internal validity:
- Artificial Experimental Settings: Research studies with high internal validity often take place in controlled laboratory settings. While this allows for rigorous control over variables, it may limit the generalizability of the findings to real-world settings. The controlled environment may not fully capture the complexity and variability of natural settings, potentially affecting the external validity of the study.
- Demand Characteristics and Experimenter Effects: Participants in a study may behave differently due to demand characteristics or their awareness of being in a research setting. They might alter their behavior to align with their perceptions of the expected or desired responses, which can introduce bias and compromise internal validity. Similarly, experimenter effects, such as unintentional cues or biases conveyed by the researcher, can influence participant responses and affect internal validity.
- Selection Bias: The process of selecting participants for a study may introduce biases and limit the generalizability of the findings. For example, if participants are not randomly selected or if they self-select into the study, the sample may not represent the larger population, impacting both internal and external validity.
- Reactive or Interactive Effects: Participants’ awareness of being observed or their exposure to the experimental manipulation may elicit reactive or interactive effects. These effects can influence their behavior, leading to artificial responses that may not be representative of their natural behavior in real-world situations.
- Limited Sample Characteristics: The characteristics of the sample used in a study can affect internal validity. If the sample is not diverse or representative of the population of interest, it can limit the generalizability of the findings. Additionally, small sample sizes may reduce statistical power and increase the likelihood of chance findings.
- Time-related Factors: Internal validity can be influenced by factors related to the timing of the study. For example, the immediate effects observed in a short-term study may not reflect the long-term effects of an intervention. Additionally, history or maturation effects occurring during the course of the study may confound the relationship being studied.
- Exclusion of Complex Variables: To establish internal validity, researchers often simplify the research design by focusing on a limited number of variables. While this allows for controlled experimentation, it may neglect the complex interactions and multiple factors that exist in real-world situations. This limitation can impact the ecological validity and external validity of the findings.
- Publication Bias: Publication bias occurs when studies with significant or positive results are more likely to be published, while studies with null or negative results remain unpublished or overlooked. This bias can distort the body of evidence and compromise the overall internal validity of the research field.
Alo see Validity
About the author
Muhammad Hassan
Researcher, Academic Writer, Web developer
You may also like

Validity – Types, Examples and Guide

Alternate Forms Reliability – Methods, Examples...

Construct Validity – Types, Threats and Examples

Reliability Vs Validity

Internal Consistency Reliability – Methods...

Split-Half Reliability – Methods, Examples and...
Statistical Thinking: A Simulation Approach to Modeling Uncertainty
Internal validity evidence and random assignment.
Medical researchers may be interested in showing that a drug helps improve people’s health (the cause of improvement is the drug), while educational researchers may be interested in showing a curricular innovation improves students’ learning (the curricular innovation causes improved learning). To attribute a causal relationship, there are three criteria a researcher needs to establish:
- Temporal Precedence: The cause needs to happen BEFORE the effect.
- Covariation of the Cause and Effect: There needs to be a correlational relationship between the cause and effect.
- No Plausible Alternative Explanations: ALL other possible explanations for the effect need to be ruled out.
Because of this third criteria, attributing a cause-and-effect relationship is very difficult. (You can read more about each of these criteria at the Web Center for Social Research Methods .)
Experimental studies have their strength in meeting this third criteria. To rule out ALL other possible explanations for the effect, the control group and the treatment group need to be “identical” with respect to every possible characteristic (aside from the treatment) that could explain differences. This way the only characteristic that will be different is that the treatment group gets the treatment and the control group doesn’t. If there are differences in the outcome, then it must be attributable to the treatment, because the other possible explanations are ruled out.
So, the key is to make the control and treatment groups “identical” when you are forming them. One thing that makes this task (slightly) easier is that they don’t have to be exactly identical, only probabilistically equivalent . This means, for example, that if you were matching groups on age that you don’t need the two groups to have identical age distributions; they would only need to have roughly the same AVERAGE age. Here roughly means “the average ages should be the same within what we expect because of sampling error.”
Now we just need to create the groups so that they have, on average, the same characteristics … for EVERY POSSIBLE CHARCTERISTIC that could explain differences in the outcome. Zoinks! 13
It turns out that creating probabilistically equivalent groups is a really difficult problem. One method that works pretty well for doing this is to randomly assign participants to the groups. This works best when you have large sample sizes, but even with small sample sizes random assignment has the advantage of at least removing the systematic bias between the two groups (any differences are due to chance and will probably even out between the groups). As Wikipedia’s page on random assignment points out,
Random assignment of participants helps to ensure that any differences between and within the groups are not systematic at the outset of the experiment. Thus, any differences between groups recorded at the end of the experiment can be more confidently attributed to the experimental procedures or treatment. … Random assignment does not guarantee that the groups are matched or equivalent. The groups may still differ on some preexisting attribute due to chance. The use of random assignment cannot eliminate this possibility, but it greatly reduces it.
Internal validity is the degree to which cause-and-effect inferences are accurate and meaningful. Causal attribution is the goal for many researchers. Thus, by using random assignment we have a pretty high degree of evidence for internal validity; we have a much higher belief in causal inferences. Much like evidence used in a court of law, it is useful to think about validity evidence on a continuum. We will visualize this continuum as a barometer. For example, a barometer visualizing the internal validity evidence for a study that employed random assignment in the design might be:

The degree of internal validity evidence is high (in the upper-third). How high depends on other factors such as sample size.
To learn more about random assignment, you can read the following:
- The research report, Random Assignment Evaluation Studies: A Guide for Out-of-School Time Program Practitioners
According to Wiktionary the earliest usage of the work “zoinks” was by Norville “Shaggy” Rogers on the show Scooby-Doo. ↩︎
Statistical Thinking: A Simulation Approach to Modeling Uncertainty (UM STAT 216 edition)
3.7 internal validity evidence and random assignment.
Medical researchers may be interested in showing that a drug helps improve people’s health (the cause of improvement is the drug), while educational researchers may be interested in showing a curricular innovation improves students’ learning (the curricular innovation causes improved learning). To attribute a causal relationship, there are three criteria a researcher needs to establish:
- Temporal Precedence: The cause needs to happen BEFORE the effect.
- Covariation of the Cause and Effect: There needs to be a correlational relationship between the cause and effect.
- No Plausible Alternative Explanations: ALL other possible explanations for the effect need to be ruled out.
Because of this third criteria, attributing a cause-and-effect relationship is very difficult. (You can read more about each of these criteria at the Web Center for Social Research Methods .)
Experimental studies have their strength in meeting this third criteria. To rule out ALL other possible explanations for the effect, the control group and the treatment group need to be “identical” with respect to every possible characteristic (aside from the treatment) that could explain differences. This way the only characteristic that will be different is that the treatment group gets the treatment and the control group doesn’t. If there are differences in the outcome, then it must be attributable to the treatment, because the other possible explanations are ruled out.
So, the key is to make the control and treatment groups “identical” when you are forming them. One thing that makes this task (slightly) easier is that they don’t have to be exactly identical, only probabilistically equivalent . This means, for example, that if you were matching groups on age that you don’t need the two groups to have identical age distributions; they would only need to have roughly the same AVERAGE age. Here roughly means “the average ages should be the same within what we expect because of sampling error.”
Now we just need to create the groups so that they have, on average, the same characteristics … for EVERY POSSIBLE CHARCTERISTIC that could explain differences in the outcome. Zoinks! 17
It turns out that creating probabilistically equivalent groups is a really difficult problem. One method that works pretty well for doing this is to randomly assign participants to the groups. This works best when you have large sample sizes, but even with small sample sizes random assignment has the advantage of at least removing the systematic bias between the two groups (any differences are due to chance and will probably even out between the groups). As Wikipedia’s page on random assignment points out,
Random assignment of participants helps to ensure that any differences between and within the groups are not systematic at the outset of the experiment. Thus, any differences between groups recorded at the end of the experiment can be more confidently attributed to the experimental procedures or treatment. … Random assignment does not guarantee that the groups are matched or equivalent. The groups may still differ on some preexisting attribute due to chance. The use of random assignment cannot eliminate this possibility, but it greatly reduces it.
Internal validity is the degree to which cause-and-effect inferences are accurate and meaningful. Causal attribution is the goal for many researchers. Thus, by using random assignment we have a pretty high degree of evidence for internal validity; we have a much higher belief in causal inferences. Much like evidence used in a court of law, it is useful to think about validity evidence on a continuum. We will visualize this continuum as a barometer. For example, a barometer visualizing the internal validity evidence for a study that employed random assignment in the design might be:

The degree of internal validity evidence is high (in the upper-third). How high depends on other factors such as sample size.
To learn more about random assignment, you can read the following:
- The research report, Random Assignment Evaluation Studies: A Guide for Out-of-School Time Program Practitioners
According to Wiktionary the earliest usage of the work “zoinks” was by Norville “Shaggy” Rogers on the show Scooby-Doo. ↩︎
The conflict between random assignment and treatment preference: implications for internal validity
Affiliation.
- 1 Center for Psychiatric Rehabilitation, University of Chicago, 7230 Arbor Drive, Tinley Park, IL 60477, USA.
- PMID: 24011479
- DOI: 10.1016/S0149-7189(03)00014-4
The gold standard for most clinical and services outcome studies is random assignment to treatment condition because this kind of design diminishes many threats to internal validity. Although we agree with the power of randomized clinical trials, we argue in this paper that random assignment raises other, unanticipated threats to internal validity as a result of failing to consider treatment preference in research participant behavior. Treatment preference arises from an individual's knowledge and appraisal of treatment options. Treatment preferences impact: (1) the recruitment phase because people consider whether they want to participate in a study that involves the possibility of receiving an undesirable treatment or waiting for treatment, (2) degree of engagement in the intervention condition, and (3) attrition from the study. The benefits and limitations of research strategies that augment randomization while respecting treatment preference are reviewed including: approaches that enhance enrollment and engagement; pilot testing assumptions about randomization; and partially randomized clinical trials.
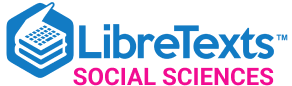
- school Campus Bookshelves
- menu_book Bookshelves
- perm_media Learning Objects
- login Login
- how_to_reg Request Instructor Account
- hub Instructor Commons
- Download Page (PDF)
- Download Full Book (PDF)
- Periodic Table
- Physics Constants
- Scientific Calculator
- Reference & Cite
- Tools expand_more
- Readability
selected template will load here
This action is not available.
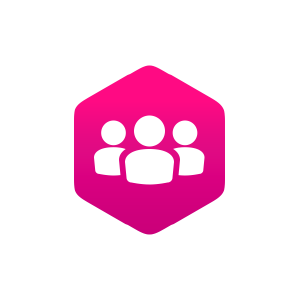
5.2: Improving Internal and External Validity
- Last updated
- Save as PDF
- Page ID 26233
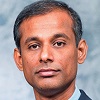
- Anol Bhattacherjee
- University of South Florida via Global Text Project
The best research designs are those that can assure high levels of internal and external validity. Such designs would guard against spurious correlations, inspire greater faith in the hypotheses testing, and ensure that the results drawn from a small sample are generalizable to the population at large. Controls are required to assure internal validity (causality) of research designs, and can be accomplished in four ways: (1) manipulation, (2) elimination, (3) inclusion, and (4) statistical control, and (5) randomization.
In manipulation , the researcher manipulates the independent variables in one or more levels (called “treatments”), and compares the effects of the treatments against a control group where subjects do not receive the treatment. Treatments may include a new drug or different dosage of drug (for treating a medical condition), a, a teaching style (for students), and so forth. This type of control is achieved in experimental or quasi-experimental designs but not in nonexperimental designs such as surveys. Note that if subjects cannot distinguish adequately between different levels of treatment manipulations, their responses across treatments may not be different, and manipulation would fail.
The elimination technique relies on eliminating extraneous variables by holding them constant across treatments, such as by restricting the study to a single gender or a single socioeconomic status. In the inclusion technique, the role of extraneous variables is considered by including them in the research design and separately estimating their effects on the dependent variable, such as via factorial designs where one factor is gender (male versus female). Such technique allows for greater generalizability but also requires substantially larger samples. In statistical control , extraneous variables are measured and used as covariates during the statistical testing process.
Finally, the randomization technique is aimed at canceling out the effects of extraneous variables through a process of random sampling, if it can be assured that these effects are of a random (non-systematic) nature. Two types of randomization are: (1) random selection , where a sample is selected randomly from a population, and (2) random assignment , where subjects selected in a non-random manner are randomly assigned to treatment groups.
Randomization also assures external validity, allowing inferences drawn from the sample to be generalized to the population from which the sample is drawn. Note that random assignment is mandatory when random selection is not possible because of resource or access constraints. However, generalizability across populations is harder to ascertain since populations may differ on multiple dimensions and you can only control for few of those dimensions.
Have a language expert improve your writing
Run a free plagiarism check in 10 minutes, automatically generate references for free.
- Knowledge Base
- Methodology
- Random Assignment in Experiments | Introduction & Examples
Random Assignment in Experiments | Introduction & Examples
Published on 6 May 2022 by Pritha Bhandari . Revised on 13 February 2023.
In experimental research, random assignment is a way of placing participants from your sample into different treatment groups using randomisation.
With simple random assignment, every member of the sample has a known or equal chance of being placed in a control group or an experimental group. Studies that use simple random assignment are also called completely randomised designs .
Random assignment is a key part of experimental design . It helps you ensure that all groups are comparable at the start of a study: any differences between them are due to random factors.
Table of contents
Why does random assignment matter, random sampling vs random assignment, how do you use random assignment, when is random assignment not used, frequently asked questions about random assignment.
Random assignment is an important part of control in experimental research, because it helps strengthen the internal validity of an experiment.
In experiments, researchers manipulate an independent variable to assess its effect on a dependent variable, while controlling for other variables. To do so, they often use different levels of an independent variable for different groups of participants.
This is called a between-groups or independent measures design.
You use three groups of participants that are each given a different level of the independent variable:
- A control group that’s given a placebo (no dosage)
- An experimental group that’s given a low dosage
- A second experimental group that’s given a high dosage
Random assignment to helps you make sure that the treatment groups don’t differ in systematic or biased ways at the start of the experiment.
If you don’t use random assignment, you may not be able to rule out alternative explanations for your results.
- Participants recruited from pubs are placed in the control group
- Participants recruited from local community centres are placed in the low-dosage experimental group
- Participants recruited from gyms are placed in the high-dosage group
With this type of assignment, it’s hard to tell whether the participant characteristics are the same across all groups at the start of the study. Gym users may tend to engage in more healthy behaviours than people who frequent pubs or community centres, and this would introduce a healthy user bias in your study.
Although random assignment helps even out baseline differences between groups, it doesn’t always make them completely equivalent. There may still be extraneous variables that differ between groups, and there will always be some group differences that arise from chance.
Most of the time, the random variation between groups is low, and, therefore, it’s acceptable for further analysis. This is especially true when you have a large sample. In general, you should always use random assignment in experiments when it is ethically possible and makes sense for your study topic.
Prevent plagiarism, run a free check.
Random sampling and random assignment are both important concepts in research, but it’s important to understand the difference between them.
Random sampling (also called probability sampling or random selection) is a way of selecting members of a population to be included in your study. In contrast, random assignment is a way of sorting the sample participants into control and experimental groups.
While random sampling is used in many types of studies, random assignment is only used in between-subjects experimental designs.
Some studies use both random sampling and random assignment, while others use only one or the other.
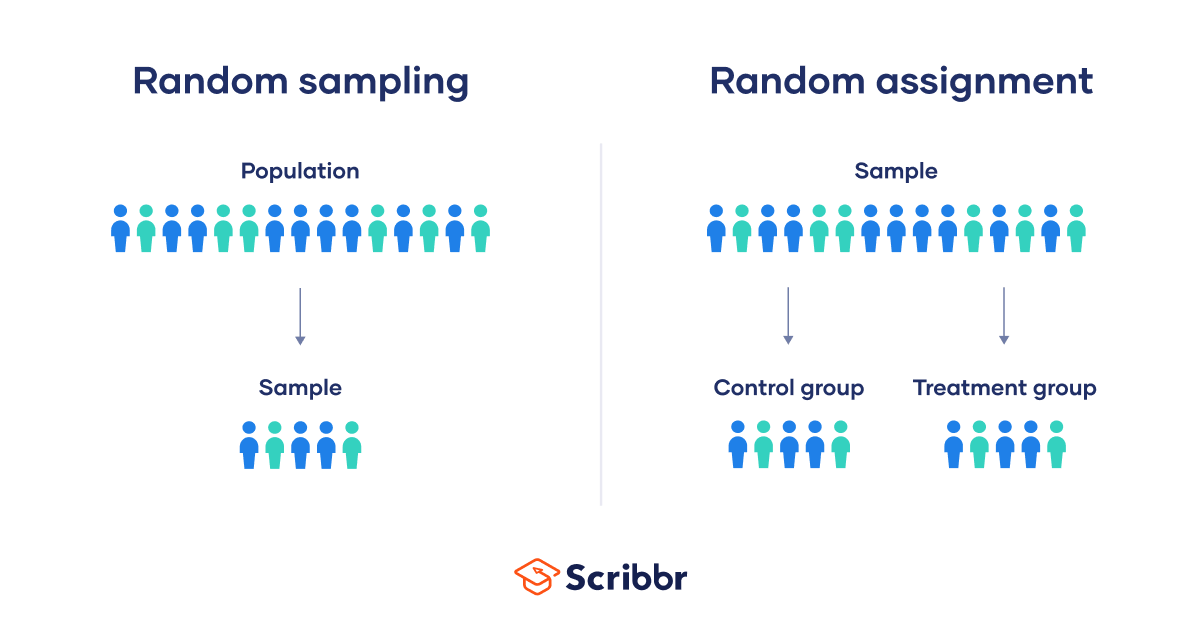
Random sampling enhances the external validity or generalisability of your results, because it helps to ensure that your sample is unbiased and representative of the whole population. This allows you to make stronger statistical inferences .
You use a simple random sample to collect data. Because you have access to the whole population (all employees), you can assign all 8,000 employees a number and use a random number generator to select 300 employees. These 300 employees are your full sample.
Random assignment enhances the internal validity of the study, because it ensures that there are no systematic differences between the participants in each group. This helps you conclude that the outcomes can be attributed to the independent variable .
- A control group that receives no intervention
- An experimental group that has a remote team-building intervention every week for a month
You use random assignment to place participants into the control or experimental group. To do so, you take your list of participants and assign each participant a number. Again, you use a random number generator to place each participant in one of the two groups.
To use simple random assignment, you start by giving every member of the sample a unique number. Then, you can use computer programs or manual methods to randomly assign each participant to a group.
- Random number generator: Use a computer program to generate random numbers from the list for each group.
- Lottery method: Place all numbers individually into a hat or a bucket, and draw numbers at random for each group.
- Flip a coin: When you only have two groups, for each number on the list, flip a coin to decide if they’ll be in the control or the experimental group.
- Use a dice: When you have three groups, for each number on the list, roll a die to decide which of the groups they will be in. For example, assume that rolling 1 or 2 lands them in a control group; 3 or 4 in an experimental group; and 5 or 6 in a second control or experimental group.
This type of random assignment is the most powerful method of placing participants in conditions, because each individual has an equal chance of being placed in any one of your treatment groups.
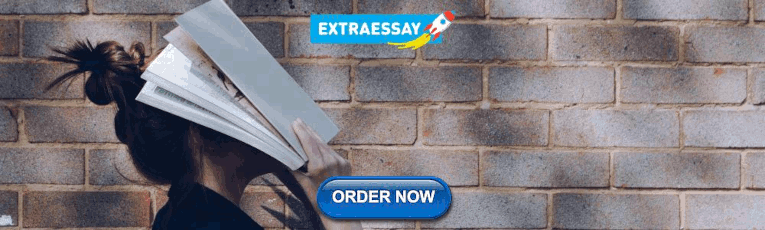
Random assignment in block designs
In more complicated experimental designs, random assignment is only used after participants are grouped into blocks based on some characteristic (e.g., test score or demographic variable). These groupings mean that you need a larger sample to achieve high statistical power .
For example, a randomised block design involves placing participants into blocks based on a shared characteristic (e.g., college students vs graduates), and then using random assignment within each block to assign participants to every treatment condition. This helps you assess whether the characteristic affects the outcomes of your treatment.
In an experimental matched design , you use blocking and then match up individual participants from each block based on specific characteristics. Within each matched pair or group, you randomly assign each participant to one of the conditions in the experiment and compare their outcomes.
Sometimes, it’s not relevant or ethical to use simple random assignment, so groups are assigned in a different way.
When comparing different groups
Sometimes, differences between participants are the main focus of a study, for example, when comparing children and adults or people with and without health conditions. Participants are not randomly assigned to different groups, but instead assigned based on their characteristics.
In this type of study, the characteristic of interest (e.g., gender) is an independent variable, and the groups differ based on the different levels (e.g., men, women). All participants are tested the same way, and then their group-level outcomes are compared.
When it’s not ethically permissible
When studying unhealthy or dangerous behaviours, it’s not possible to use random assignment. For example, if you’re studying heavy drinkers and social drinkers, it’s unethical to randomly assign participants to one of the two groups and ask them to drink large amounts of alcohol for your experiment.
When you can’t assign participants to groups, you can also conduct a quasi-experimental study . In a quasi-experiment, you study the outcomes of pre-existing groups who receive treatments that you may not have any control over (e.g., heavy drinkers and social drinkers).
These groups aren’t randomly assigned, but may be considered comparable when some other variables (e.g., age or socioeconomic status) are controlled for.
In experimental research, random assignment is a way of placing participants from your sample into different groups using randomisation. With this method, every member of the sample has a known or equal chance of being placed in a control group or an experimental group.
Random selection, or random sampling , is a way of selecting members of a population for your study’s sample.
In contrast, random assignment is a way of sorting the sample into control and experimental groups.
Random sampling enhances the external validity or generalisability of your results, while random assignment improves the internal validity of your study.
Random assignment is used in experiments with a between-groups or independent measures design. In this research design, there’s usually a control group and one or more experimental groups. Random assignment helps ensure that the groups are comparable.
In general, you should always use random assignment in this type of experimental design when it is ethically possible and makes sense for your study topic.
To implement random assignment , assign a unique number to every member of your study’s sample .
Then, you can use a random number generator or a lottery method to randomly assign each number to a control or experimental group. You can also do so manually, by flipping a coin or rolling a die to randomly assign participants to groups.
Cite this Scribbr article
If you want to cite this source, you can copy and paste the citation or click the ‘Cite this Scribbr article’ button to automatically add the citation to our free Reference Generator.
Bhandari, P. (2023, February 13). Random Assignment in Experiments | Introduction & Examples. Scribbr. Retrieved 15 April 2024, from https://www.scribbr.co.uk/research-methods/random-assignment-experiments/
Is this article helpful?
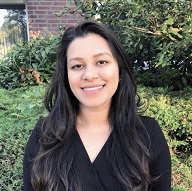
Pritha Bhandari
Other students also liked, a quick guide to experimental design | 5 steps & examples, controlled experiments | methods & examples of control, control groups and treatment groups | uses & examples.
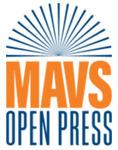
Want to create or adapt books like this? Learn more about how Pressbooks supports open publishing practices.
14.1 What is experimental design and when should you use it?
Learning objectives.
Learners will be able to…
- Describe the purpose of experimental design research
- Describe nomethetic causality and the logic of experimental design
- Identify the characteristics of a basic experiment
- Discuss the relationship between dependent and independent variables in experiments
- Identify the three major types of experimental designs
Pre-awareness check (Knowledge)
What are your thoughts on the phrase ‘experiment’ in the realm of social sciences? In an experiment, what is the independent variable?
The basics of experiments
In social work research, experimental design is used to test the effects of treatments, interventions, programs, or other conditions to which individuals, groups, organizations, or communities may be exposed to. There are a lot of experiments social work researchers can use to explore topics such as treatments for depression, impacts of school-based mental health on student outcomes, or prevention of abuse of people with disabilities. The American Psychological Association defines an experiment as:
a series of observations conducted under controlled conditions to study a relationship with the purpose of drawing causal inferences about that relationship. An experiment involves the manipulation of an independent variable , the measurement of a dependent variable , and the exposure of various participants to one or more of the conditions being studied. Random selection of participants and their random assignment to conditions also are necessary in experiments .
In experimental design, the independent variable is the intervention, treatment, or condition that is being investigated as a potential cause of change (i.e., the experimental condition ). The effect, or outcome, of the experimental condition is the dependent variable. Trying out a new restaurant, dating a new person – we often call these things “experiments.” However, a true social science experiment would include recruitment of a large enough sample, random assignment to control and experimental groups, exposing those in the experimental group to an experimental condition, and collecting observations at the end of the experiment.
Social scientists use this level of rigor and control to maximize the internal validity of their research. Internal validity is the confidence researchers have about whether the independent variable (e.g, treatment) truly produces a change in the dependent, or outcome, variable. The logic and features of experimental design are intended to help establish causality and to reduce threats to internal validity , which we will discuss in Section 14.5 .
Experiments attempt to establish a nomothetic causal relationship between two variables—the treatment and its intended outcome. We discussed the four criteria for establishing nomothetic causality in Section 4.3 :
- plausibility,
- covariation,
- temporality, and
- nonspuriousness.
Experiments should establish plausibility , having a plausible reason why their intervention would cause changes in the dependent variable. Usually, a theory framework or previous empirical evidence will indicate the plausibility of a causal relationship.
Covariation can be established for causal explanations by showing that the “cause” and the “effect” change together. In experiments, the cause is an intervention, treatment, or other experimental condition. Whether or not a research participant is exposed to the experimental condition is the independent variable. The effect in an experiment is the outcome being assessed and is the dependent variable in the study. When the independent and dependent variables covary, they can have a positive association (e.g., those exposed to the intervention have increased self-esteem) or a negative association (e.g., those exposed to the intervention have reduced anxiety).
Since researcher controls when the intervention is administered, they can be assured that changes in the independent variable (the treatment) happens before changes in the dependent variable (the outcome). In this way, experiments assure temporality .
Finally, one of the most important features of experiments is that they allow researchers to eliminate spurious variables to support the criterion of nonspuriousness . True experiments are usually conducted under strictly controlled conditions. The intervention is given in the same way to each person, with a minimal number of other variables that might cause their post-test scores to change.
The logic of experimental design
How do we know that one phenomenon causes another? The complexity of the social world in which we practice and conduct research means that causes of social problems are rarely cut and dry. Uncovering explanations for social problems is key to helping clients address them, and experimental research designs are one road to finding answers.
Just because two phenomena are related in some way doesn’t mean that one causes the other. Ice cream sales increase in the summer, and so does the rate of violent crime; does that mean that eating ice cream is going to make me violent? Obviously not, because ice cream is great. The reality of that association is far more complex—it could be that hot weather makes people more irritable and, at times, violent, while also making people want ice cream. More likely, though, there are other social factors not accounted for in the way we just described this association.
As we have discussed, experimental designs can help clear up at least some of this fog by allowing researchers to isolate the effect of interventions on dependent variables by controlling extraneous variables . In true experimental design (discussed in the next section) and quasi-experimental design, researchers accomplish this w ith a control group or comparison group and the experimental group . The experimental group is sometimes called the treatment group because people in the experimental group receive the treatment or are exposed to the experimental condition (but we will call it the experimental group in this chapter.) The control/comparison group does not receive the treatment or intervention. Instead they may receive what is known as “treatment as usual” or perhaps no treatment at all.
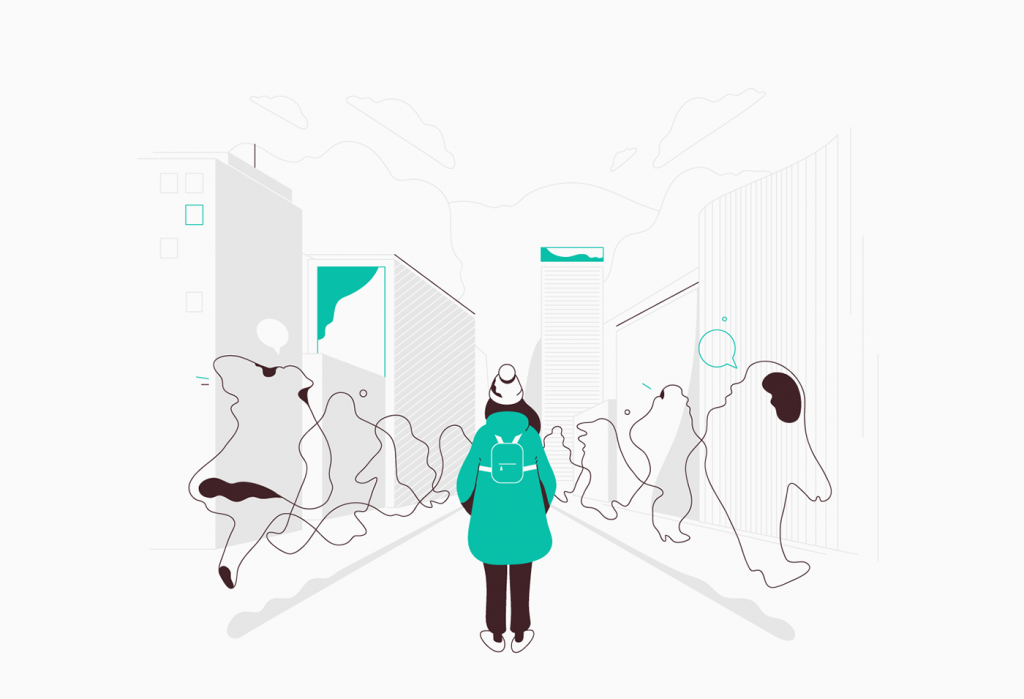
In a well-designed experiment, the control group should look almost identical to the experimental group in terms of demographics and other relevant factors. What if we want to know the effect of CBT on social anxiety, but we have learned in prior research that men tend to have a more difficult time overcoming social anxiety? We would want our control and experimental groups to have a similar portions of men, since ostensibly, both groups’ results would be affected by the men in the group. If your control group has 5 women, 6 men, and 4 non-binary people, then your experimental group should be made up of roughly the same gender balance to help control for the influence of gender on the outcome of your intervention. (In reality, the groups should be similar along other dimensions, as well, and your group will likely be much larger.) The researcher will use the same outcome measures for both groups and compare them, and assuming the experiment was designed correctly, get a pretty good answer about whether the intervention had an effect on social anxiety.
Random assignment [/pb_glossary], also called randomization, entails using a random process to decide which participants are put into the control or experimental group (which participants receive an intervention and which do not). By randomly assigning participants to a group, you can reduce the effect of extraneous variables on your research because there won’t be a systematic difference between the groups.
Do not confuse random assignment with random sampling . Random sampling is a method for selecting a sample from a population and is rarely used in psychological research. Random assignment is a method for assigning participants in a sample to the different conditions, and it is an important element of all experimental research in psychology and other related fields. Random sampling helps a great deal with external validity, or generalizability , whereas random assignment increases internal validity .
Other Features of Experiments that Help Establish Causality
To control for spuriousness (as well as meeting the three other criteria for establishing causality), experiments try to control as many aspects of the research process as possible: using control groups, having large enough sample sizes, standardizing the treatment, etc. Researchers in large experiments often employ clinicians or other research staff to help them. Researchers train their staff members exhaustively, provide pre-scripted responses to common questions, and control the physical environment of the experiment so each person who participates receives the exact same treatment. Experimental researchers also document their procedures, so that others can review them and make changes in future research if they think it will improve on the ability to control for spurious variables.
An interesting example is Bruce Alexander’s (2010) Rat Park experiments. Much of the early research conducted on addictive drugs, like heroin and cocaine, was conducted on animals other than humans, usually mice or rats. The scientific consensus up until Alexander’s experiments was that cocaine and heroin were so addictive that rats, if offered the drugs, would consume them repeatedly until they perished. Researchers claimed this behavior explained how addiction worked in humans, but Alexander was not so sure. He knew rats were social animals and the experimental procedure from previous experiments did not allow them to socialize. Instead, rats were kept isolated in small cages with only food, water, and metal walls. To Alexander, social isolation was a spurious variable, causing changes in addictive behavior not due to the drug itself. Alexander created an experiment of his own, in which rats were allowed to run freely in an interesting environment, socialize and mate with other rats, and of course, drink from a solution that contained an addictive drug. In this environment, rats did not become hopelessly addicted to drugs. In fact, they had little interest in the substance. To Alexander, the results of his experiment demonstrated that social isolation was more of a causal factor for addiction than the drug itself.
One challenge with Alexander’s findings is that subsequent researchers have had mixed success replicating his findings (e.g., Petrie, 1996; Solinas, Thiriet, El Rawas, Lardeux, & Jaber, 2009). Replication involves conducting another researcher’s experiment in the same manner and seeing if it produces the same results. If the causal relationship is real, it should occur in all (or at least most) rigorous replications of the experiment.
Replicability
[INSERT A PARAGRAPH ABOUT REPLICATION/REPRODUCTION HERE. CAN USE/REFERENCE THIS IF IT’S HELPFUL; include glossary definition as well as other general info]
To allow for easier replication, researchers should describe their experimental methods diligently. Researchers with the Open Science Collaboration (2015) [1] conducted the Reproducibility Project , which caused a significant controversy regarding the validity of psychological studies. The researchers with the project attempted to reproduce the results of 100 experiments published in major psychology journals since 2008. What they found was shocking. Although 97% of the original studies reported significant results, only 36% of the replicated studies had significant findings. The average effect size in the replication studies was half that of the original studies. The implications of the Reproducibility Project are potentially staggering, and encourage social scientists to carefully consider the validity of their reported findings and that the scientific community take steps to ensure researchers do not cherry-pick data or change their hypotheses simply to get published.
Generalizability
Let’s return to Alexander’s Rat Park study and consider the implications of his experiment for substance use professionals. The conclusions he drew from his experiments on rats were meant to be generalized to the population. If this could be done, the experiment would have a high degree of external validity , which is the degree to which conclusions generalize to larger populations and different situations. Alexander argues his conclusions about addiction and social isolation help us understand why people living in deprived, isolated environments may become addicted to drugs more often than those in more enriching environments. Similarly, earlier rat researchers argued their results showed these drugs were instantly addictive to humans, often to the point of death.
Neither study’s results will match up perfectly with real life. There are clients in social work practice who may fit into Alexander’s social isolation model, but social isolation is complex. Clients can live in environments with other sociable humans, work jobs, and have romantic relationships; does this mean they are not socially isolated? On the other hand, clients may face structural racism, poverty, trauma, and other challenges that may contribute to their social environment. Alexander’s work helps understand clients’ experiences, but the explanation is incomplete. Human existence is more complicated than the experimental conditions in Rat Park.
Effectiveness versus Efficacy
Social workers are especially attentive to how social context shapes social life. This consideration points out a potential weakness of experiments. They can be rather artificial. When an experiment demonstrates causality under ideal, controlled circumstances, it establishes the efficacy of an intervention.
How often do real-world social interactions occur in the same way that they do in a controlled experiment? Experiments that are conducted in community settings by community practitioners are less easily controlled than those conducted in a lab or with researchers who adhere strictly to research protocols delivering the intervention. When an experiment demonstrates causality in a real-world setting that is not tightly controlled, it establishes the effectiveness of the intervention.
The distinction between efficacy and effectiveness demonstrates the tension between internal and external validity. Internal validity and external validity are conceptually linked. Internal validity refers to the degree to which the intervention causes its intended outcomes, and external validity refers to how well that relationship applies to different groups and circumstances than the experiment. However, the more researchers tightly control the environment to ensure internal validity, the more they may risk external validity for generalizing their results to different populations and circumstances. Correspondingly, researchers whose settings are just like the real world will be less able to ensure internal validity, as there are many factors that could pollute the research process. This is not to suggest that experimental research findings cannot have high levels of both internal and external validity, but that experimental researchers must always be aware of this potential weakness and clearly report limitations in their research reports.
Types of Experimental Designs
Experimental design is an umbrella term for a research method that is designed to test hypotheses related to causality under controlled conditions. Table 14.1 describes the three major types of experimental design (pre-experimental, quasi-experimental, and true experimental) and presents subtypes for each. As we will see in the coming sections, some types of experimental design are better at establishing causality than others. It’s also worth considering that true experiments, which most effectively establish causality , are often difficult and expensive to implement. Although the other experimental designs aren’t perfect, they still produce useful, valid evidence and may be more feasible to carry out.
Key Takeaways
- Experimental designs are useful for establishing causality, but some types of experimental design do this better than others.
- Experiments help researchers isolate the effect of the independent variable on the dependent variable by controlling for the effect of extraneous variables .
- Experiments use a control/comparison group and an experimental group to test the effects of interventions. These groups should be as similar to each other as possible in terms of demographics and other relevant factors.
- True experiments have control groups with randomly assigned participants; quasi-experimental types of experiments have comparison groups to which participants are not randomly assigned; pre-experimental designs do not have a comparison group.
TRACK 1 (IF YOU ARE CREATING A RESEARCH PROPOSAL FOR THIS CLASS):
- Think about the research project you’ve been designing so far. How might you use a basic experiment to answer your question? If your question isn’t explanatory, try to formulate a new explanatory question and consider the usefulness of an experiment.
- Why is establishing a simple relationship between two variables not indicative of one causing the other?
TRACK 2 (IF YOU AREN’T CREATING A RESEARCH PROPOSAL FOR THIS CLASS):
Imagine you are interested in studying child welfare practice. You are interested in learning more about community-based programs aimed to prevent child maltreatment and to prevent out-of-home placement for children.
- Think about the research project stated above. How might you use a basic experiment to look more into this research topic? Try to formulate an explanatory question and consider the usefulness of an experiment.
- Open Science Collaboration. (2015). Estimating the reproducibility of psychological science. Science, 349 (6251), aac4716. Doi: 10.1126/science.aac4716 ↵
an operation or procedure carried out under controlled conditions in order to discover an unknown effect or law, to test or establish a hypothesis, or to illustrate a known law.
treatment, intervention, or experience that is being tested in an experiment (the independent variable) that is received by the experimental group and not by the control group.
Ability to say that one variable "causes" something to happen to another variable. Very important to assess when thinking about studies that examine causation such as experimental or quasi-experimental designs.
circumstances or events that may affect the outcome of an experiment, resulting in changes in the research participants that are not a result of the intervention, treatment, or experimental condition being tested
causal explanations that can be universally applied to groups, such as scientific laws or universal truths
as a criteria for causal relationship, the relationship must make logical sense and seem possible
when the values of two variables change at the same time
as a criteria for causal relationship, the cause must come before the effect
an association between two variables that is NOT caused by a third variable
variables and characteristics that have an effect on your outcome, but aren't the primary variable whose influence you're interested in testing.
the group of participants in our study who do not receive the intervention we are researching in experiments with random assignment
the group of participants in our study who do not receive the intervention we are researching in experiments without random assignment
in experimental design, the group of participants in our study who do receive the intervention we are researching
The ability to apply research findings beyond the study sample to some broader population,
This is a synonymous term for generalizability - the ability to apply the findings of a study beyond the sample to a broader population.
performance of an intervention under ideal and controlled circumstances, such as in a lab or delivered by trained researcher-interventionists
The performance of an intervention under "real-world" conditions that are not closely controlled and ideal
the idea that one event, behavior, or belief will result in the occurrence of another, subsequent event, behavior, or belief
Doctoral Research Methods in Social Work Copyright © by Mavs Open Press. All Rights Reserved.
Share This Book
Internal vs. External Validity In Psychology
Julia Simkus
Editor at Simply Psychology
BA (Hons) Psychology, Princeton University
Julia Simkus is a graduate of Princeton University with a Bachelor of Arts in Psychology. She is currently studying for a Master's Degree in Counseling for Mental Health and Wellness in September 2023. Julia's research has been published in peer reviewed journals.
Learn about our Editorial Process
Saul Mcleod, PhD
Editor-in-Chief for Simply Psychology
BSc (Hons) Psychology, MRes, PhD, University of Manchester
Saul Mcleod, PhD., is a qualified psychology teacher with over 18 years of experience in further and higher education. He has been published in peer-reviewed journals, including the Journal of Clinical Psychology.
Olivia Guy-Evans, MSc
Associate Editor for Simply Psychology
BSc (Hons) Psychology, MSc Psychology of Education
Olivia Guy-Evans is a writer and associate editor for Simply Psychology. She has previously worked in healthcare and educational sectors.
Internal validity centers on demonstrating clear casual relationships within the bounds of a specific study and external validity relates to demonstrating the applicability of findings beyond that original study situation or population.
Researchers have to weigh these considerations in designing methodologically rigorous and generalizable studies.

Internal Validity
Internal validity refers to the degree of confidence that the causal relationship being tested exists and is trustworthy.
It tests how likely it is that your treatment caused the differences in results that you observe. Internal validity is largely determined by the study’s experimental design and methods .
Studies that have a high degree of internal validity provide strong evidence of causality, so it makes it possible to eliminate alternative explanations for a finding.
Studies with low internal validity provide weak evidence of causality. The less chance there is for confounding or extraneous variables , the higher the internal validity and the more confident we can be in our findings.
In order to assume cause and effect in a research study, the cause must precede the effect in terms of time, the cause and effect must vary together, and there must be no other explanations for the relationship observed. If these three criteria are observed, you can be confident that a study is internally valid.
An example of a study with high internal validity would be if you wanted to run an experiment to see if using a particular weight-loss pill will help people lose weight.
To test this hypothesis, you would randomly assign a sample of participants to one of two groups: those who will take the weight-loss pill and those who will take a placebo pill.
You can ensure that there is no bias in how participants are assigned to the groups by blinding the research assistants , so they don’t know which participants are in which groups during the experiment. The participants are also blinded, so they do not know whether they are receiving the intervention or not.
If participants drop out of the study, their characteristics are examined to ensure there is no systematic bias regarding who left.
It is important to have a well-thought-out research procedure to mitigate the threats to internal validity.
External Validity
External validity refers to the extent to which the results of a research study can be applied or generalized to another context.
This is important because if external validity is established, the studies’ findings can be generalized to a larger population as opposed to only the relatively few subjects who participated in the study. Unlike internal validity, external validity doesn’t assess causality or rule out confounders.
There are two types of external validity: ecological validity and population validity.
- Ecological validity refers to whether a study’s findings can be generalized to other situations or settings. A high ecological validity means that there is a high degree of similarity between the experimental setting and another setting, and thus we can be confident that the results will generalize to that other setting.
- Population validity refers to how well the experimental sample represents other populations or groups. Using random sampling techniques , such as stratified sampling or cluster sampling, significantly helps increase population validity.
An example of a study with high external validity would be if you hypothesize that practicing mindfulness two times per week will improve the mental health of those diagnosed with depression.
You recruit people who have been diagnosed with depression for at least a year and are between 18–29 years old. Choosing this representative sample with a clearly defined population of interest helps ensure external validity.
You give participants a pre-test and a post-test measuring how often they experienced symptoms of depression in the past week.
During the study, all participants were given individual mindfulness training and asked to practice mindfulness daily for 15 minutes as part of their morning routine.
You can also replicate the study’s results using different methods of mindfulness or different samples of participants.
Trade-off Between Internal and External Validity
There tends to be a negative correlation between internal and external validity in experimental research. This means that experiments that have high internal validity will likely have low external validity and vice versa.
This happens because experimental conditions that produce higher degrees of internal validity (e.g., artificial labs) tend to be highly unlikely to match real-world conditions. So, the external validity is weaker because a lab environment is much different than the real world.
On the other hand, to produce higher degrees of external validity, you want experimental conditions that match a real-world setting (e.g., observational studies ).
However, this comes at the expense of internal validity because these types of studies increase the likelihood of confounding variables and alternative explanations for differences in outcomes.
A solution to this trade-off is replication! You want to conduct the research in multiple environments and settings – first in a controlled, artificial environment to establish the existence of a causal relationship and then in a “real-world” setting to analyze if the results are generalizable.
Threats to Internal Validity
Attrition refers to the loss of study participants over time. Participants might drop out or leave the study which means that the results are based solely on a biased sample of only the people who did not choose to leave.
Differential rates of attrition between treatment and control groups can skew results by affecting the relationship between your independent and dependent variables and thus affect the internal validity of a study.
Confounders
A confounding variable is an unmeasured third variable that influences, or “confounds,” the relationship between an independent and a dependent variable by suggesting the presence of a spurious correlation.
Confounders are threats to internal validity because you can’t tell whether the predicted independent variable causes the outcome or if the confounding variable causes it.
Participant Selection Bias
This is a bias that may result from the selection or assignment of study groups in such a way that proper randomization is not achieved.
If participants are not randomly assigned to groups, the sample obtained might not be representative of the population intended to be studied. For example, some members of a population might be less likely to be included than others due to motivation, willingness to take part in the study, or demographics.
Experimenter Bias
Experimenter bias occurs when an experimenter behaves in a different way with different groups in a study, impacting the results and threatening internal validity. This can be eliminated through blinding.
Social Interaction (Diffusion)
Diffusion refers to when the treatment in research spreads within or between treatment and control groups. This can happen when there is interaction or observation among the groups.
Diffusion poses a threat to internal validity because it can lead to resentful demoralization. This is when the control group is less motivated because they feel resentful over the group that they are in.
Historical Events
Historical events might influence the outcome of studies that occur over longer periods of time. For example, changes in political leadership, natural disasters, or other unanticipated events might change the conditions of the study and influence the outcomes.
Instrumentation
Instrumentation refers to any change in the dependent variable in a study that arises from changes in the measuring instrument used. This happens when different measures are used in the pre-test and post-test phases.
Maturation refers to the impact of time on a study. If the outcomes of the study vary as a natural result of time, it might not be possible to determine whether the effects seen in the study were due to the study treatment or simply due to the impact of time.
Statistical Regression
Regression to the mean refers to the fact that if one sample of a random variable is extreme, the next sampling of the same random variable is likely going to be closer to its mean.
This is a threat to internal validity as participants at extreme ends of treatment can naturally fall in a certain direction due to the passage of time rather than being a direct effect of an intervention.
Repeated Testing
Testing your research participants repeatedly with the same measures will influence your research findings because participants will become more accustomed to the testing. Due to familiarity, or awareness of the study’s purpose, many participants might achieve better results over time.
Threats to External Validity
Sample features.
If some feature(s) of the sample used were responsible for the effect, this could lead to limited generalizability of the findings.
This is a bias that may result from the selection or assignment of study groups in such a way that proper randomization is not achieved. If participants are not randomly assigned to groups, the sample obtained might not be representative of the population intended to be studied.
For example, some members of a population might be less likely to be included than others due to motivation, willingness to take part in the study, or demographics.
Situational Factors
Factors such as the setting, time of day, location, researchers’ characteristics, noise, or the number of measures might affect the generalizability of the findings.
Aptitude-Treatment Interaction → Aptitude-Treatment Interaction to the concept that some treatments are more or less effective for particular individuals depending upon their specific abilities or characteristics.
Hawthorne Effect
The Hawthorne Effect refers to the tendency for participants to change their behaviors simply because they know they are being studied.
Experimenter Effect
Experimenter bias occurs when an experimenter behaves in a different way with different groups in a study, impacting the results and threatening the external validity.
John Henry Effect
The John Henry Effect refers to the tendency for participants in a control group to actively work harder because they know they are in an experiment and want to overcome the “disadvantage” of being in the control group.
Factors that Improve Internal Validity
Blinding refers to a practice where the participants (and sometimes the researchers) are unaware of what intervention they are receiving.
This reduces the influence of extraneous factors and minimizes bias, as any differences in outcome can thus be linked to the intervention and not to the participant’s knowledge of whether they were receiving a new treatment or not.
Random Sampling
Using random sampling to obtain a sample that represents the population that you wish to study will improve internal validity.
Random Assignment
Using random assignment to assign participants to control and treatment groups ensures that there is no systematic bias among the research groups.
Strict Study Protocol
Highly controlled experiments tend to improve internal validity. Experiments that occur in lab settings tend to have higher validity as this reduces variability from sources other than the treatment.
Experimental Manipulation
Manipulating an independent variable in a study as opposed to just observing an association without conducting an intervention improves internal validity.
Factors that Improve External Validity
Replication.
Conducting a study more than once with a different sample or in a different setting to see if the results will replicate can help improve external validity.
If multiple studies have been conducted on the same topic, a meta-analysis can be used to determine if the effect of an independent variable can be replicated, thus making it more reliable.
Replication is the strongest method to counter threats to external validity by enhancing generalizability to other settings, populations, and conditions.
Field Experiments
Conducting a study outside the laboratory, in a natural, real-world setting will improve external validity (however, this will threaten the internal validity)
Probability Sampling
Using probability sampling will counter selection bias by making sure everyone in a population has an equal chance of being selected for a study sample.
Recalibration
Recalibration is the use of statistical methods to maintain accuracy, standardization, and repeatability in measurements to assure reliable results.
Reweighting groups, if a study had uneven groups for a particular characteristic (such as age), is an example of calibration.
Inclusion and Exclusion Criteria
Setting criteria as to who can be involved in the research and who cannot be involved will ensure that the population being studied is clearly defined and that the sample is representative of the population.
Psychological Realism
Psychological realism refers to the process of making sure participants perceive the experimental manipulations as real events so as to not reveal the purpose of the study and so participants don’t behave differently than they would in real life based on knowing the study’s goal.

- Bipolar Disorder
- Therapy Center
- When To See a Therapist
- Types of Therapy
- Best Online Therapy
- Best Couples Therapy
- Best Family Therapy
- Managing Stress
- Sleep and Dreaming
- Understanding Emotions
- Self-Improvement
- Healthy Relationships
- Student Resources
- Personality Types
- Guided Meditations
- Verywell Mind Insights
- 2023 Verywell Mind 25
- Mental Health in the Classroom
- Editorial Process
- Meet Our Review Board
- Crisis Support
Internal Validity vs. External Validity in Research
Both help determine how meaningful the results of the study are
Arlin Cuncic, MA, is the author of The Anxiety Workbook and founder of the website About Social Anxiety. She has a Master's degree in clinical psychology.
:max_bytes(150000):strip_icc():format(webp)/ArlinCuncic_1000-21af8749d2144aa0b0491c29319591c4.jpg)
Rachel Goldman, PhD FTOS, is a licensed psychologist, clinical assistant professor, speaker, wellness expert specializing in eating behaviors, stress management, and health behavior change.
:max_bytes(150000):strip_icc():format(webp)/Rachel-Goldman-1000-a42451caacb6423abecbe6b74e628042.jpg)
Verywell / Bailey Mariner
- Internal Validity
- External Validity
Internal validity is a measure of how well a study is conducted (its structure) and how accurately its results reflect the studied group.
External validity relates to how applicable the findings are in the real world. These two concepts help researchers gauge if the results of a research study are trustworthy and meaningful.
Conclusions are warranted
Controls extraneous variables
Eliminates alternative explanations
Focus on accuracy and strong research methods
Findings can be generalized
Outcomes apply to practical situations
Results apply to the world at large
Results can be translated into another context
What Is Internal Validity in Research?
Internal validity is the extent to which a research study establishes a trustworthy cause-and-effect relationship. This type of validity depends largely on the study's procedures and how rigorously it is performed.
Internal validity is important because once established, it makes it possible to eliminate alternative explanations for a finding. If you implement a smoking cessation program, for instance, internal validity ensures that any improvement in the subjects is due to the treatment administered and not something else.
Internal validity is not a "yes or no" concept. Instead, we consider how confident we can be with study findings based on whether the research avoids traps that may make those findings questionable. The less chance there is for "confounding," the higher the internal validity and the more confident we can be.
Confounding refers to uncontrollable variables that come into play and can confuse the outcome of a study, making us unsure of whether we can trust that we have identified the cause-and-effect relationship.
In short, you can only be confident that a study is internally valid if you can rule out alternative explanations for the findings. Three criteria are required to assume cause and effect in a research study:
- The cause preceded the effect in terms of time.
- The cause and effect vary together.
- There are no other likely explanations for the relationship observed.
Factors That Improve Internal Validity
To ensure the internal validity of a study, you want to consider aspects of the research design that will increase the likelihood that you can reject alternative hypotheses. Many factors can improve internal validity in research, including:
- Blinding : Participants—and sometimes researchers—are unaware of what intervention they are receiving (such as using a placebo on some subjects in a medication study) to avoid having this knowledge bias their perceptions and behaviors, thus impacting the study's outcome
- Experimental manipulation : Manipulating an independent variable in a study (for instance, giving smokers a cessation program) instead of just observing an association without conducting any intervention (examining the relationship between exercise and smoking behavior)
- Random selection : Choosing participants at random or in a manner in which they are representative of the population that you wish to study
- Randomization or random assignment : Randomly assigning participants to treatment and control groups, ensuring that there is no systematic bias between the research groups
- Strict study protocol : Following specific procedures during the study so as not to introduce any unintended effects; for example, doing things differently with one group of study participants than you do with another group
Internal Validity Threats
Just as there are many ways to ensure internal validity, there is also a list of potential threats that should be considered when planning a study.
- Attrition : Participants dropping out or leaving a study, which means that the results are based on a biased sample of only the people who did not choose to leave (and possibly who all have something in common, such as higher motivation)
- Confounding : A situation in which changes in an outcome variable can be thought to have resulted from some type of outside variable not measured or manipulated in the study
- Diffusion : This refers to the results of one group transferring to another through the groups interacting and talking with or observing one another; this can also lead to another issue called resentful demoralization, in which a control group tries less hard because they feel resentful over the group that they are in
- Experimenter bias : An experimenter behaving in a different way with different groups in a study, which can impact the results (and is eliminated through blinding)
- Historical events : May influence the outcome of studies that occur over a period of time, such as a change in the political leader or a natural disaster that occurs, influencing how study participants feel and act
- Instrumentation : This involves "priming" participants in a study in certain ways with the measures used, causing them to react in a way that is different than they would have otherwise reacted
- Maturation : The impact of time as a variable in a study; for example, if a study takes place over a period of time in which it is possible that participants naturally change in some way (i.e., they grew older or became tired), it may be impossible to rule out whether effects seen in the study were simply due to the impact of time
- Statistical regression : The natural effect of participants at extreme ends of a measure falling in a certain direction due to the passage of time rather than being a direct effect of an intervention
- Testing : Repeatedly testing participants using the same measures influences outcomes; for example, if you give someone the same test three times, it is likely that they will do better as they learn the test or become used to the testing process, causing them to answer differently
What Is External Validity in Research?
External validity refers to how well the outcome of a research study can be expected to apply to other settings. This is important because, if external validity is established, it means that the findings can be generalizable to similar individuals or populations.
External validity affirmatively answers the question: Do the findings apply to similar people, settings, situations, and time periods?
Population validity and ecological validity are two types of external validity. Population validity refers to whether you can generalize the research outcomes to other populations or groups. Ecological validity refers to whether a study's findings can be generalized to additional situations or settings.
Another term called transferability refers to whether results transfer to situations with similar characteristics. Transferability relates to external validity and refers to a qualitative research design.
Factors That Improve External Validity
If you want to improve the external validity of your study, there are many ways to achieve this goal. Factors that can enhance external validity include:
- Field experiments : Conducting a study outside the laboratory, in a natural setting
- Inclusion and exclusion criteria : Setting criteria as to who can be involved in the research, ensuring that the population being studied is clearly defined
- Psychological realism : Making sure participants experience the events of the study as being real by telling them a "cover story," or a different story about the aim of the study so they don't behave differently than they would in real life based on knowing what to expect or knowing the study's goal
- Replication : Conducting the study again with different samples or in different settings to see if you get the same results; when many studies have been conducted on the same topic, a meta-analysis can also be used to determine if the effect of an independent variable can be replicated, therefore making it more reliable
- Reprocessing or calibration : Using statistical methods to adjust for external validity issues, such as reweighting groups if a study had uneven groups for a particular characteristic (such as age)
External Validity Threats
External validity is threatened when a study does not take into account the interaction of variables in the real world. Threats to external validity include:
- Pre- and post-test effects : When the pre- or post-test is in some way related to the effect seen in the study, such that the cause-and-effect relationship disappears without these added tests
- Sample features : When some feature of the sample used was responsible for the effect (or partially responsible), leading to limited generalizability of the findings
- Selection bias : Also considered a threat to internal validity, selection bias describes differences between groups in a study that may relate to the independent variable—like motivation or willingness to take part in the study, or specific demographics of individuals being more likely to take part in an online survey
- Situational factors : Factors such as the time of day of the study, its location, noise, researcher characteristics, and the number of measures used may affect the generalizability of findings
While rigorous research methods can ensure internal validity, external validity may be limited by these methods.
Internal Validity vs. External Validity
Internal validity and external validity are two research concepts that share a few similarities while also having several differences.
Similarities
One of the similarities between internal validity and external validity is that both factors should be considered when designing a study. This is because both have implications in terms of whether the results of a study have meaning.
Both internal validity and external validity are not "either/or" concepts. Therefore, you always need to decide to what degree a study performs in terms of each type of validity.
Each of these concepts is also typically reported in research articles published in scholarly journals . This is so that other researchers can evaluate the study and make decisions about whether the results are useful and valid.
Differences
The essential difference between internal validity and external validity is that internal validity refers to the structure of a study (and its variables) while external validity refers to the universality of the results. But there are further differences between the two as well.
For instance, internal validity focuses on showing a difference that is due to the independent variable alone. Conversely, external validity results can be translated to the world at large.
Internal validity and external validity aren't mutually exclusive. You can have a study with good internal validity but be overall irrelevant to the real world. You could also conduct a field study that is highly relevant to the real world but doesn't have trustworthy results in terms of knowing what variables caused the outcomes.
Examples of Validity
Perhaps the best way to understand internal validity and external validity is with examples.
Internal Validity Example
An example of a study with good internal validity would be if a researcher hypothesizes that using a particular mindfulness app will reduce negative mood. To test this hypothesis, the researcher randomly assigns a sample of participants to one of two groups: those who will use the app over a defined period and those who engage in a control task.
The researcher ensures that there is no systematic bias in how participants are assigned to the groups. They do this by blinding the research assistants so they don't know which groups the subjects are in during the experiment.
A strict study protocol is also used to outline the procedures of the study. Potential confounding variables are measured along with mood , such as the participants' socioeconomic status, gender, age, and other factors. If participants drop out of the study, their characteristics are examined to make sure there is no systematic bias in terms of who stays in.
External Validity Example
An example of a study with good external validity would be if, in the above example, the participants used the mindfulness app at home rather than in the laboratory. This shows that results appear in a real-world setting.
To further ensure external validity, the researcher clearly defines the population of interest and chooses a representative sample . They might also replicate the study's results using different technological devices.
A Word From Verywell
Setting up an experiment so that it has both sound internal validity and external validity involves being mindful from the start about factors that can influence each aspect of your research.
It's best to spend extra time designing a structurally sound study that has far-reaching implications rather than to quickly rush through the design phase only to discover problems later on. Only when both internal validity and external validity are high can strong conclusions be made about your results.
San Jose State University. Internal and external validity .
Michael RS. Threats to internal & external validity: Y520 strategies for educational inquiry .
Pahus L, Burgel PR, Roche N, Paillasseur JL, Chanez P. Randomized controlled trials of pharmacological treatments to prevent COPD exacerbations: applicability to real-life patients . BMC Pulm Med . 2019;19(1):127. doi:10.1186/s12890-019-0882-y
By Arlin Cuncic, MA Arlin Cuncic, MA, is the author of The Anxiety Workbook and founder of the website About Social Anxiety. She has a Master's degree in clinical psychology.
The Federal Register
The daily journal of the united states government, request access.
Due to aggressive automated scraping of FederalRegister.gov and eCFR.gov, programmatic access to these sites is limited to access to our extensive developer APIs.
If you are human user receiving this message, we can add your IP address to a set of IPs that can access FederalRegister.gov & eCFR.gov; complete the CAPTCHA (bot test) below and click "Request Access". This process will be necessary for each IP address you wish to access the site from, requests are valid for approximately one quarter (three months) after which the process may need to be repeated.
An official website of the United States government.
If you want to request a wider IP range, first request access for your current IP, and then use the "Site Feedback" button found in the lower left-hand side to make the request.
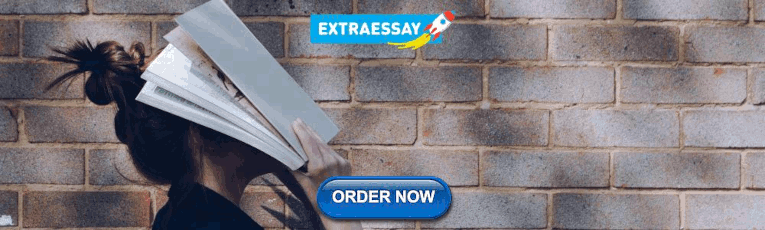
IMAGES
VIDEO
COMMENTS
Random assignment is an important part of control in experimental research, because it helps strengthen the internal validity of an experiment and avoid biases. In experiments, researchers manipulate an independent variable to assess its effect on a dependent variable, while controlling for other variables.
Random assignment. Matching; Holding Extraneous Variable Constant; Building Extraneous Variables into Design; Internal validity is often the focus from a research design perspective. To understand the pros and cons of various designs and to be able to better judge specific designs, we identify specific threats to internal validity. Before we do ...
How to Increase Internal Validity. To enhance internal validity, researchers need to carefully design and conduct their studies. Here are some considerations for improving internal validity: Random Assignment: Use random assignment to allocate participants to different groups in experimental studies. Random assignment helps ensure that the ...
Random assignment increases the likelihood that the treatment groups are the same at the onset of a study. ... This is particularly important for eliminating sources of bias and strengthening the internal validity of an experiment. Random assignment is the best method for inferring a causal relationship between a treatment and an outcome.
Internal validity is the confidence you can have that the independent variable is responsible (caused) changes in the dependent variable. Random assignment increases internal validity by reducing the risk of systematic pre-existing differences between the levels of the independent variable. Studies that use random assignment are called experiments.
Internal validity is the degree to which cause-and-effect inferences are accurate and meaningful. Causal attribution is the goal for many researchers. Thus, by using random assignment we have a pretty high degree of evidence for internal validity; we have a much higher belief in causal inferences. Much like evidence used in a court of law, it ...
However, even the most rigorous applications of random assignment and blinding do not immunize an RCT against threats to validity; the RCT design remains vulnerable to some biases that undermine confidence that the outcomes are solely attributed to the intervention under evaluation (Frieden, 2017; Horwitz et al., 2017).
The use of random assignment cannot eliminate this possibility, but it greatly reduces it. Internal validity is the degree to which cause-and-effect inferences are accurate and meaningful. Causal attribution is the goal for many researchers. Thus, by using random assignment we have a pretty high degree of evidence for internal validity; we have ...
Because random assignment helps ensure that the groups are comparable when the experiment begins, you can be more confident that the treatments caused the post-study differences. Random assignment helps increase the internal validity of your study. Comparing the Vitamin Study With and Without Random Assignment
Abstract. The gold standard for most clinical and services outcome studies is random assignment to treatment condition because this kind of design diminishes many threats to internal validity. Although we agree with the power of randomized clinical trials, we argue in this paper that random assignment raises other, unanticipated threats to ...
23) advocated for the use of random assignment to experimental conditions as a method for mitigating the threat to an experiment's internal validity: "… with satisfactory randomisation, its validity is, indeed, wholly unimpaired" (for a historical account of Fisher's advocacy for randomization, see Hall, 2007).
Internal and external validity relate to the findings of studies and experiments. Internal validity evaluates a study's experimental design and methods. You must have a valid experimental design to be able to draw sound scientific conclusions. External validity assesses the applicability or generalizability of the findings to the real world.
Controls are required to assure internal validity (causality) of research designs, and can be accomplished in four ways: (1) manipulation, (2) elimination, (3) inclusion, and (4) statistical control, and (5) randomization. In manipulation, the researcher manipulates the independent variables in one or more levels (called "treatments"), and ...
Overall validity increases as biases are reduced, and the robustness of our evidence increases as we conduct multiple studies. RCTs are generally the best we have for the type of questions they can address. Randomised controlled trials are nonetheless not always as random as thought.
Random assignment is an important part of control in experimental research, because it helps strengthen the internal validity of an experiment. In experiments, researchers manipulate an independent variable to assess its effect on a dependent variable, while controlling for other variables.
Random assignment is a method for assigning participants in a sample to the different conditions, and it is an important element of all experimental research in psychology and other related fields. Random sampling helps a great deal with external validity, or generalizability, whereas random assignment increases internal validity.
However, this comes at the expense of internal validity because these types of studies increase the likelihood of confounding variables and alternative explanations for differences in outcomes. ... Using random sampling to obtain a sample that represents the population that you wish to study will improve internal validity. Random Assignment .
The essential difference between internal validity and external validity is that internal validity refers to the structure of a study (and its variables) while external validity refers to the universality of the results. But there are further differences between the two as well. For instance, internal validity focuses on showing a difference ...
Chapter 7: Experimental Research Designs. experiment. Click the card to flip 👆. a research method which can confidently assert a causal relation between the independent and dependent variables; includes manipulation and random assignment; tend to be very high in internal validity. Click the card to flip 👆.
Which of the following statements is true of random assignment and random sampling? a) Random assignment is necessary for internal validity, whereas random assignment is necessary for external validity. b) They both are necessary for frequency claims. c) They both mean the same thing. d) Random sampling is more important than random assignment.
On September 27, 2023, USCIS updated its policy to increase the validity period to a maximum of 5 years for initial and renewal EADs for certain noncitizens who must apply for employment authorization, including applicants for asylum or withholding of removal, adjustment of status under section 245 of the INA, 8 U.S.C. 1255, and suspension of ...