Representation Words
Words related to representation.
Below is a massive list of representation words - that is, words related to representation. The top 4 are: image , model , diversity and inclusion . You can get the definition(s) of a word in the list below by tapping the question-mark icon next to it. The words at the top of the list are the ones most associated with representation, and as you go down the relatedness becomes more slight. By default, the words are sorted by relevance/relatedness, but you can also get the most common representation terms by using the menu below, and there's also the option to sort the words alphabetically so you can get representation words starting with a particular letter. You can also filter the word list so it only shows words that are also related to another word of your choosing. So for example, you could enter "image" and click "filter", and it'd give you words that are related to representation and image.
You can highlight the terms by the frequency with which they occur in the written English language using the menu below. The frequency data is extracted from the English Wikipedia corpus, and updated regularly. If you just care about the words' direct semantic similarity to representation, then there's probably no need for this.
There are already a bunch of websites on the net that help you find synonyms for various words, but only a handful that help you find related , or even loosely associated words. So although you might see some synonyms of representation in the list below, many of the words below will have other relationships with representation - you could see a word with the exact opposite meaning in the word list, for example. So it's the sort of list that would be useful for helping you build a representation vocabulary list, or just a general representation word list for whatever purpose, but it's not necessarily going to be useful if you're looking for words that mean the same thing as representation (though it still might be handy for that).
If you're looking for names related to representation (e.g. business names, or pet names), this page might help you come up with ideas. The results below obviously aren't all going to be applicable for the actual name of your pet/blog/startup/etc., but hopefully they get your mind working and help you see the links between various concepts. If your pet/blog/etc. has something to do with representation, then it's obviously a good idea to use concepts or words to do with representation.
If you don't find what you're looking for in the list below, or if there's some sort of bug and it's not displaying representation related words, please send me feedback using this page. Thanks for using the site - I hope it is useful to you! 🐖

- representative
- participation
- proportional representation
- recognition
- illustration
- typification
- adumbration
- interpretation
- instantiation
- pictorial representation
- jurisdiction
- representation
- unrepresented
- underrepresented
- mental representation
- manifestation
- internal representation
- representatives
- representational
- equitable distribution
- disenfranchised
- proportionally
- participatory democracy
- inclusivity
- marginalized
- ethnic minorities
- affiliations
- distinction
- constituencies
- description
- understanding
- unrepresentative
- geographic diversity
- proportionate
- constitutionally
- treated unfairly
- decisionmaking
- discriminated against
- representations
- presentation
- proportional
- performance
- normalization
- advertising
- representing
- represented
- histrionics
- incarnation
- appreciation
- personification
- appropriation
- dramatization
- dramatisation
- empowerment
- explanation
- cooperation
- cosmography
- objectification
- affiliation
- consultation
- convergence
- intersection
- phantasmagoria
- abstractionism
- psychosexuality
- schematization
- diagramming
- schematisation
- visualization
- theatrical performance
- iconography
- corresponding
- geographical
- furthermore
- delineative
- constituted
- determining
- portraiture
- consideration
- independent
- corresponds
- fundamental
- constitutional
- appropriate
- orientation
- characteristics
- prototypical
- resemblance
- hyperrealism
- delineation
- apportionment
- legal representation
- cutaway model
- free agency
- mental image
- concrete representation
- public presentation
- station of the cross
- cutaway drawing
- perceptual experience
- mental object
- picturesque
- cognitive content
- portraitist
- videography
- photomontage
- decision-making
- shadowgraph
- approximation
- photographer
- illustrator
- miniaturist
- backgrounds
- commissions
- nonrepresentational
- printmaking
- discrimination
- transpressionism
- disenfranchisement
- reapportionment
- remonstrate
- photography
- gerrymandering
- photographic
- consultative
- astrophotography
- articulation
- homogeneity
- misrepresent
- accountability
- pseudophotograph
- cartography
- victimization
- transparency
- marginalization
- inclusiveness
- photomicrograph
- composition
- photoengraving
- autoradiograph
- visual information source
- emancipation
- wordshaping
- photomechanical
- telephotograph
- enfranchisement
- disfranchisement
- definiteness
- disproportion
- particularity
- elaboration
- involvement
- distinctness
- pictorialism
- stereomonoscope
- telephotography
- instantiate
- unrepresentable
- scenography
- abstractionist
- artsploitation
- underexpose
- magnetic resonance image
- positron emission tomography
- districting
- answerability
- reputability
- deconcentration
- objectiveness
- bicameralism
- corroboration
- consociational
- fashion model
- photo realism
- technical draw
- screen capture
- mathematical model
- conecept design
- block diagram
- station of cross
- scale model
- pictorial convention
- hang on wall
- graphic art
- logic diagram
- paint on canvas
- gallery open
- partiya karkeran kurdistan
- pro bono publico
That's about all the representation related words we've got! I hope this list of representation terms was useful to you in some way or another. The words down here at the bottom of the list will be in some way associated with representation, but perhaps tenuously (if you've currenly got it sorted by relevance, that is). If you have any feedback for the site, please share it here , but please note this is only a hobby project, so I may not be able to make regular updates to the site. Have a nice day! 🐭

- Cambridge Dictionary +Plus
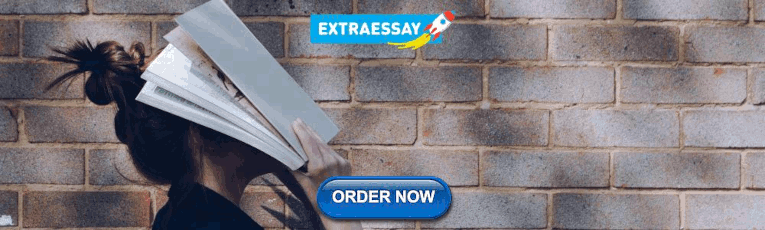
Synonyms and antonyms of represent in English
Word of the Day
Your browser doesn't support HTML5 audio
a long trip or holiday taken by car

Apples and oranges (Talking about differences, Part 2)
Learn more with +Plus
- Recent and Recommended {{#preferredDictionaries}} {{name}} {{/preferredDictionaries}}
- Definitions Clear explanations of natural written and spoken English English Learner’s Dictionary Essential British English Essential American English
- Grammar and thesaurus Usage explanations of natural written and spoken English Grammar Thesaurus
- Pronunciation British and American pronunciations with audio English Pronunciation
- English–Chinese (Simplified) Chinese (Simplified)–English
- English–Chinese (Traditional) Chinese (Traditional)–English
- English–Dutch Dutch–English
- English–French French–English
- English–German German–English
- English–Indonesian Indonesian–English
- English–Italian Italian–English
- English–Japanese Japanese–English
- English–Norwegian Norwegian–English
- English–Polish Polish–English
- English–Portuguese Portuguese–English
- English–Spanish Spanish–English
- English–Swedish Swedish–English
- Dictionary +Plus Word Lists
To add ${headword} to a word list please sign up or log in.
Add ${headword} to one of your lists below, or create a new one.
{{message}}
Something went wrong.
There was a problem sending your report.
- TheFreeDictionary
- Word / Article
- Starts with
- Free toolbar & extensions
- Word of the Day
- Free content
- representation
Synonyms for representation
- body of representatives
- illustration
- resemblance
- description
- delineation
- explanation
- remonstrance
- expostulation
the act or process of describing in lifelike imagery
A presentation to the mind in the form of an idea or image.
- internal representation
- mental representation
Related Words
- convergence
- intersection
- cognitive content
- mental object
- instantiation
- mental image
- interpretation
- phantasmagoria
- psychosexuality
- perceptual experience
- abstractionism
- concrete representation
a creation that is a visual or tangible rendering of someone or something
- adumbration
- cosmography
- cutaway drawing
- cutaway model
- presentation
- objectification
- Station of the Cross
the act of representing
- cooperation
- proportional representation
the state of serving as an official and authorized delegate or agent
- free agency
- legal representation
a body of legislators that serve in behalf of some constituency
A factual statement made by one party in order to induce another party to enter into a contract, a performance of a play.
- histrionics
- theatrical performance
- performance
- public presentation
a statement of facts and reasons made in appealing or protesting
The right of being represented by delegates who have a voice in some legislative body, an activity that stands as an equivalent of something or results in an equivalent.
- dramatisation
- dramatization
- diagramming
- schematisation
- schematization
- pictorial representation
- typification
- reporting weight
- repositioning
- repossession
- reprehensibility
- reprehensible
- reprehensibly
- reprehension
- reprehensively
- representable
- representational
- representational process
- representative
- representative sample
- representative sampling
- represented
- repressor gene
- reproachful
- reproachfully
- represent to
- represent to (someone or something)
- Represent to Witness
- represent us as
- represent us in
- represent us to
- represent you as
- represent you in
- represent you to
- represent yourself as
- represent yourself in
- represent yourself to
- Represent/Represented
- representability
- Representable functor
- representablely
- Representaciones Riquelme y Cerramientos Murcia
- Representamen
- Representance
- Representant
- Representante Especial del Secretario General
- Representatieve Vakorganisaties
- Representation (arts)
- Representation (disambiguation)
- Representation (psychology)
- Representation Agreement Resource Centre
- Representation and Maintenance of Process Knowledge
- Representation and Transportation Allowances
- Representation and Welfare Unit
- representation condition
- Représentation des Institutions Françaises de Sécurité Sociale
- Representation Fund Custodian
- Representation Language Language
- Représentation Militaire Française
- Representation of People's Act
- Representation of persons; a fiction of the law
- Representation of Stimuli as Neural Activity Project
- Representation of the People Act
- Representation of the People Acts
- Representation of the People Order
- Representation oligonucleotide microarray analysis
- Representation Oligonucleotide Microarray Analysis (ROMA)
- Representation Quality
- Representation theory
- Facebook Share
Related Words and Phrases
Bottom_desktop desktop:[300x250].
- More from M-W
- To save this word, you'll need to log in. Log In
representation
Definition of representation
Examples of representation in a sentence.
These examples are programmatically compiled from various online sources to illustrate current usage of the word 'representation.' Any opinions expressed in the examples do not represent those of Merriam-Webster or its editors. Send us feedback about these examples.
Word History
15th century, in the meaning defined at sense 1
Phrases Containing representation
- proportional representation
- self - representation
Dictionary Entries Near representation
representant
representationalism
Cite this Entry
“Representation.” Merriam-Webster.com Dictionary , Merriam-Webster, https://www.merriam-webster.com/dictionary/representation. Accessed 24 May. 2024.
Kids Definition
Kids definition of representation, legal definition, legal definition of representation, more from merriam-webster on representation.
Thesaurus: All synonyms and antonyms for representation
Nglish: Translation of representation for Spanish Speakers
Britannica English: Translation of representation for Arabic Speakers
Britannica.com: Encyclopedia article about representation
Subscribe to America's largest dictionary and get thousands more definitions and advanced search—ad free!
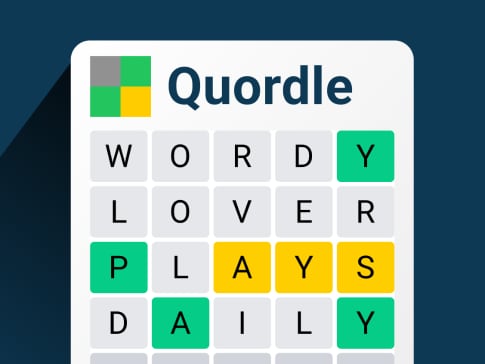
Can you solve 4 words at once?
Word of the day.
See Definitions and Examples »
Get Word of the Day daily email!
Popular in Grammar & Usage
More commonly misspelled words, your vs. you're: how to use them correctly, every letter is silent, sometimes: a-z list of examples, more commonly mispronounced words, how to use em dashes (—), en dashes (–) , and hyphens (-), popular in wordplay, the words of the week - may 24, flower etymologies for your spring garden, birds say the darndest things, a great big list of bread words, 10 scrabble words without any vowels, games & quizzes.
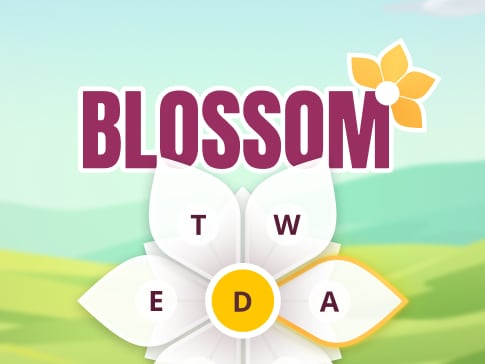
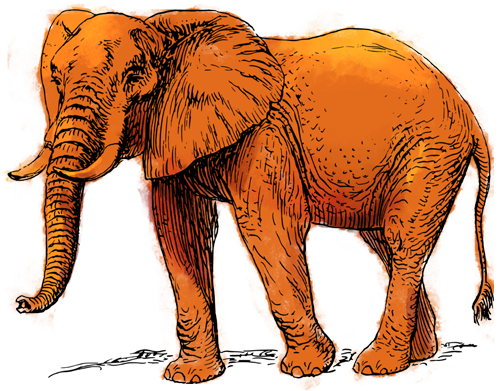
Related Words
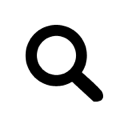
This tool helps you find words that are related to a specific word or phrase. Also check out ReverseDictionary.org and DescribingWords.io . Here are some words that are associated with representation : . You can get the definitions of these representation related words by clicking on them. Also check out describing words for representation and find more words related to representation using ReverseDictionary.org
Click words for definitions
Our algorithm is scanning multiple databases for related words. Please be patient! :)
Words Related to representation
Below is a list of words related to representation . You can click words for definitions. Sorry if there's a few unusual suggestions! The algorithm isn't perfect, but it does a pretty good job for common-ish words. Here's the list of words that are related to representation :
- illustration
- typification
- adumbration
- instantiation
- pictorial representation
- interpretation
- internal representation
- mental representation
- proportional representation
- distinction
- representative
- recognition
- participation
- jurisdiction
- constituencies
- histrionics
- dramatisation
- dramatization
- performance
- cooperation
- cosmography
- presentation
- objectification
- convergence
- intersection
- phantasmagoria
- abstractionism
- psychosexuality
- diagramming
- schematization
- schematisation
- visualization
Popular Searches
As you've probably noticed, words related to " representation " are listed above. Hopefully the generated list of term related words above suit your needs.
P.S. There are some problems that I'm aware of, but can't currently fix (because they are out of the scope of this project). The main one is that individual words can have many different senses (meanings), so when you search for a word like mean , the engine doesn't know which definition you're referring to ("bullies are mean " vs. "what do you mean ?", etc.), so consider that your search query for words like term may be a bit ambiguous to the engine in that sense, and the related terms that are returned may reflect this. You might also be wondering: What type of word is ~term~ ?
Also check out representation words on relatedwords.io for another source of associations.
Related Words runs on several different algorithms which compete to get their results higher in the list. One such algorithm uses word embedding to convert words into many dimensional vectors which represent their meanings. The vectors of the words in your query are compared to a huge database of of pre-computed vectors to find similar words. Another algorithm crawls through Concept Net to find words which have some meaningful relationship with your query. These algorithms, and several more, are what allows Related Words to give you... related words - rather than just direct synonyms.
As well as finding words related to other words, you can enter phrases and it should give you related words and phrases, so long as the phrase/sentence you entered isn't too long. You will probably get some weird results every now and then - that's just the nature of the engine in its current state.
Special thanks to the contributors of the open-source code that was used to bring you this list of representation themed words: @Planeshifter , @HubSpot , Concept Net , WordNet , and @mongodb .
There is still lots of work to be done to get this to give consistently good results, but I think it's at the stage where it could be useful to people, which is why I released it.
Please note that Related Words uses third party scripts (such as Google Analytics and advertisements) which use cookies. To learn more, see the privacy policy .
Recent Queries

Thank you for visiting nature.com. You are using a browser version with limited support for CSS. To obtain the best experience, we recommend you use a more up to date browser (or turn off compatibility mode in Internet Explorer). In the meantime, to ensure continued support, we are displaying the site without styles and JavaScript.
- View all journals
- My Account Login
- Explore content
- About the journal
- Publish with us
- Sign up for alerts
- Open access
- Published: 13 May 2024
Representation of internal speech by single neurons in human supramarginal gyrus
- Sarah K. Wandelt ORCID: orcid.org/0000-0001-9551-8491 1 , 2 ,
- David A. Bjånes 1 , 2 , 3 ,
- Kelsie Pejsa 1 , 2 ,
- Brian Lee 1 , 4 , 5 ,
- Charles Liu ORCID: orcid.org/0000-0001-6423-8577 1 , 3 , 4 , 5 &
- Richard A. Andersen 1 , 2
Nature Human Behaviour ( 2024 ) Cite this article
8151 Accesses
1 Citations
300 Altmetric
Metrics details
- Brain–machine interface
- Neural decoding
Speech brain–machine interfaces (BMIs) translate brain signals into words or audio outputs, enabling communication for people having lost their speech abilities due to diseases or injury. While important advances in vocalized, attempted and mimed speech decoding have been achieved, results for internal speech decoding are sparse and have yet to achieve high functionality. Notably, it is still unclear from which brain areas internal speech can be decoded. Here two participants with tetraplegia with implanted microelectrode arrays located in the supramarginal gyrus (SMG) and primary somatosensory cortex (S1) performed internal and vocalized speech of six words and two pseudowords. In both participants, we found significant neural representation of internal and vocalized speech, at the single neuron and population level in the SMG. From recorded population activity in the SMG, the internally spoken and vocalized words were significantly decodable. In an offline analysis, we achieved average decoding accuracies of 55% and 24% for each participant, respectively (chance level 12.5%), and during an online internal speech BMI task, we averaged 79% and 23% accuracy, respectively. Evidence of shared neural representations between internal speech, word reading and vocalized speech processes was found in participant 1. SMG represented words as well as pseudowords, providing evidence for phonetic encoding. Furthermore, our decoder achieved high classification with multiple internal speech strategies (auditory imagination/visual imagination). Activity in S1 was modulated by vocalized but not internal speech in both participants, suggesting no articulator movements of the vocal tract occurred during internal speech production. This work represents a proof-of-concept for a high-performance internal speech BMI.
Similar content being viewed by others
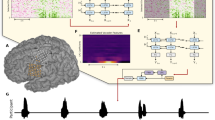
Online speech synthesis using a chronically implanted brain–computer interface in an individual with ALS
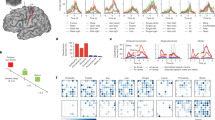
A high-performance speech neuroprosthesis
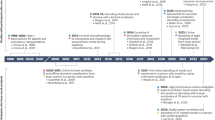
The speech neuroprosthesis
Speech is one of the most basic forms of human communication, a natural and intuitive way for humans to express their thoughts and desires. Neurological diseases like amyotrophic lateral sclerosis (ALS) and brain lesions can lead to the loss of this ability. In the most severe cases, patients who experience full-body paralysis might be left without any means of communication. Patients with ALS self-report loss of speech as their most serious concern 1 . Brain–machine interfaces (BMIs) are devices offering a promising technological path to bypass neurological impairment by recording neural activity directly from the cortex. Cognitive BMIs have demonstrated potential to restore independence to participants with tetraplegia by reading out movement intent directly from the brain 2 , 3 , 4 , 5 . Similarly, reading out internal (also reported as inner, imagined or covert) speech signals could allow the restoration of communication to people who have lost it.
Decoding speech signals directly from the brain presents its own unique challenges. While non-invasive recording methods such as functional magnetic resonance imaging (fMRI), electroencephalography (EEG) or magnetoencephalography 6 are important tools to locate speech and internal speech production, they lack the necessary temporal and spatial resolution, adequate signal-to-noise ratio or portability for building an online speech BMI 7 , 8 , 9 . For example, state-of-the-art EEG-based imagined speech decoding performances in 2022 ranged from approximately 60% to 80% binary classification 10 . Intracortical electrophysiological recordings have higher signal-to-noise ratios and excellent temporal resolution 11 and are a more suitable choice for an internal speech decoding device.
Invasive speech decoding has predominantly been attempted with electrocorticography (ECoG) 9 or stereo-electroencephalographic depth arrays 12 , as they allow sampling neural activity from different parts of the brain simultaneously. Impressive results in vocalized and attempted speech decoding and reconstruction have been achieved using these techniques 13 , 14 , 15 , 16 , 17 , 18 . However, vocalized speech has also been decoded from localized regions of the cortex. In 2009, the use of a neurotrophic electrode 19 demonstrated real-time speech synthesis from the motor cortex. More recently, speech neuroprosthetics were built from small-scale microelectrode arrays located in the motor cortex 20 , 21 , premotor cortex 22 and supramarginal gyrus (SMG) 23 , demonstrating that vocalized speech BMIs can be built using neural signals from localized regions of cortex.
While important advances in vocalized speech 16 , attempted speech 18 and mimed speech 17 , 22 , 24 , 25 , 26 decoding have been made, highly accurate internal speech decoding has not been achieved. Lack of behavioural output, lower signal-to-noise ratio and differences in cortical activations compared with vocalized speech are speculated to contribute to lower classification accuracies of internal speech 7 , 8 , 13 , 27 , 28 . In ref. 29 , patients implanted with ECoG grids over frontal, parietal and temporal regions silently read or vocalized written words from a screen. They significantly decoded vowels (37.5%) and consonants (36.3%) from internal speech (chance level 25%). Ikeda et al. 30 decoded three internally spoken vowels using ECoG arrays using frequencies in the beta band, with up to 55.6% accuracy from the Broca area (chance level 33%). Using the same recording technology, ref. 31 investigated the decoding of six words during internal speech. The authors demonstrated an average pair-wise classification accuracy of 58%, reaching 88% for the highest pair (chance level 50%). These studies were so-called open-loop experiments, in which the data were analysed offline after acquisition. A recent paper demonstrated real-time (closed-loop) speech decoding using stereotactic depth electrodes 32 . The results were encouraging as internal speech could be detected; however, the reconstructed audio was not discernable and required audible speech to train the decoding model.
While, to our knowledge, internal speech has not previously been decoded from SMG, evidence for internal speech representation in the SMG exists. A review of 100 fMRI studies 33 not only described SMG activity during speech production but also suggested its involvement in subvocal speech 34 , 35 . Similarly, an ECoG study identified high-frequency SMG modulation during vocalized and internal speech 36 . Additionally, fMRI studies have demonstrated SMG involvement in phonologic processing, for instance, during tasks while participants reported whether two words rhyme 37 . Performing such tasks requires the participant to internally ‘hear’ the word, indicating potential internal speech representation 38 . Furthermore, a study performed in people suffering from aphasia found that lesions in the SMG and its adjacent white matter affected inner speech rhyming tasks 39 . Recently, ref. 16 showed that electrode grids over SMG contributed to vocalized speech decoding. Finally, vocalized grasps and colour words were decodable from SMG from one of the same participants involved in this work 23 . These studies provide evidence for the possibility of an internal speech decoder from neural activity in the SMG.
The relationship between inner speech and vocalized speech is still debated. The general consensus posits similarities between internal and vocalized speech processes 36 , but the degree of overlap is not well understood 8 , 35 , 40 , 41 , 42 . Characterizing similarities between vocalized and internal speech could provide evidence that results found with vocalized speech could translate to internal speech. However, such a relationship may not be guaranteed. For instance, some brain areas involved in vocalized speech might be poor candidates for internal speech decoding.
In this Article, two participants with tetraplegia performed internal and vocalized speech of eight words while neurophysiological responses were captured from two implant sites. To investigate neural semantic and phonetic representation, the words were composed of six lexical words and two pseudowords (words that mimic real words without semantic meaning). We examined representations of various language processes at the single-neuron level using recording microelectrode arrays from the SMG located in the posterior parietal cortex (PPC) and the arm and/or hand regions of the primary somatosensory cortex (S1). S1 served as a control for movement, due to emerging evidence of its activation beyond defined regions of interest 43 , 44 . Words were presented with an auditory or a written cue and were produced internally as well as orally. We hypothesized that SMG and S1 activity would modulate during vocalized speech and that SMG activity would modulate during internal speech. Shared representation between internal speech, vocalized speech, auditory comprehension and word reading processes was investigated.
Task design
We characterized neural representations of four different language processes within a population of SMG and S1 neurons: auditory comprehension, word reading, internal speech and vocalized speech production. In this manuscript, internal speech refers to engaging a prompted word internally (‘inner monologue’), without correlated motor output, while vocalized speech refers to audibly vocalizing a prompted word. Participants were implanted in the SMG and S1 on the basis of grasp localization fMRI tasks (Fig. 1 ).
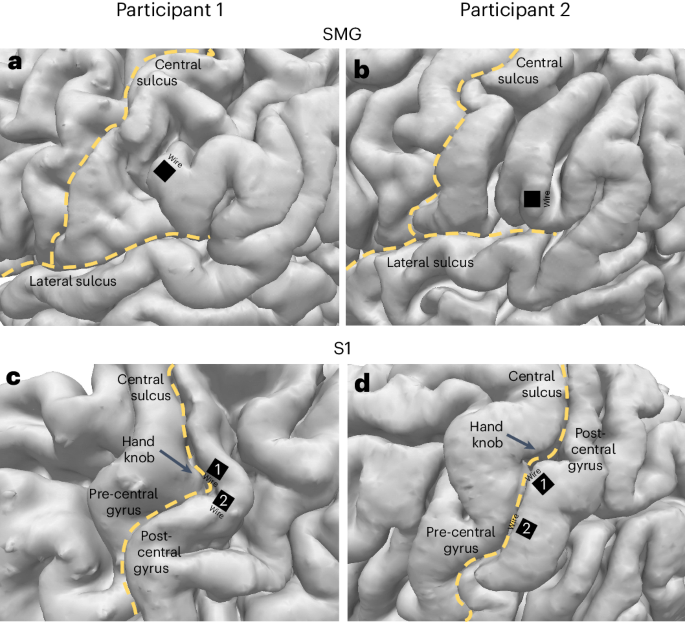
a , b , SMG implant locations in participant 1 (1 × 96 multielectrode array) ( a ) and participant 2 (1 × 64 multielectrode array) ( b ). c , d , S1 implant locations in participant 1 (2 × 96 multielectrode arrays) ( c ) and participant 2 (2 × 64 multielectrode arrays) ( d ).
The task contained six phases: an inter-trial interval (ITI), a cue phase (cue), a first delay (D1), an internal speech phase (internal), a second delay (D2) and a vocalized speech phase (speech). Words were cued with either an auditory or a written version of the word (Fig. 2a ). Six of the words were informed by ref. 31 (battlefield, cowboy, python, spoon, swimming and telephone). Two pseudowords (nifzig and bindip) were added to explore phonetic representation in the SMG. The first participant completed ten session days, composed of both the auditory and the written cue tasks. The second participant completed nine sessions, focusing only on the written cue task. The participants were instructed to internally say the cued word during the internal speech phase and to vocalize the same word during the speech phase.
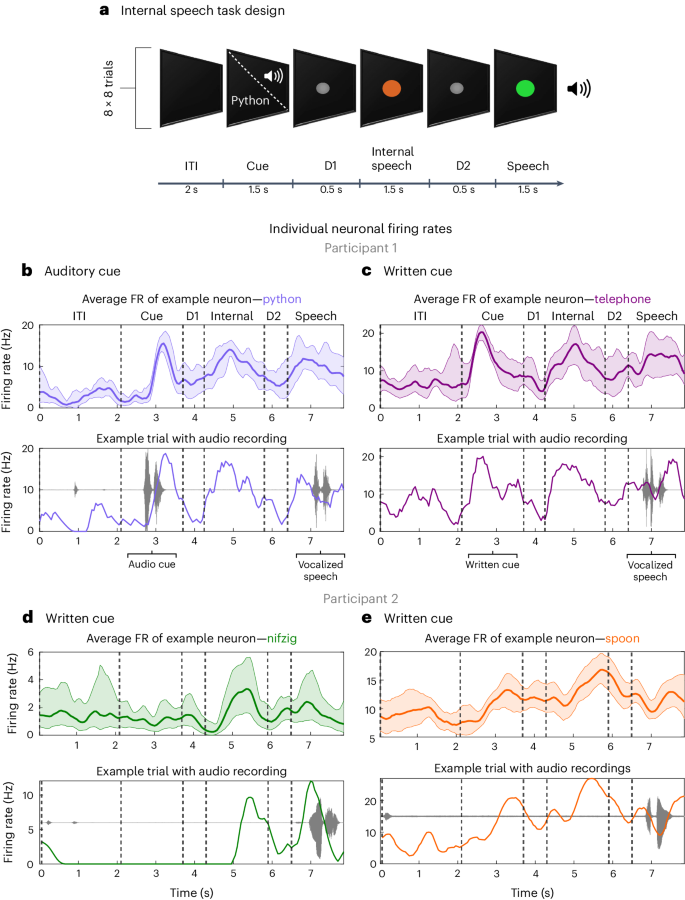
a , Written words and sounds were used to cue six words and two pseudowords in a participant with tetraplegia. The ‘audio cue’ task was composed of an ITI, a cue phase during which the sound of one of the words was emitted from a speaker (between 842 and 1,130 ms), a first delay (D1), an internal speech phase, a second delay (D2) and a vocalized speech phase. The ‘written cue’ task was identical to the ‘audio cue’ task, except that written words appeared on the screen for 1.5 s. Eight repetitions of eight words were performed per session day and per task for the first participant. For the second participant, 16 repetitions of eight words were performed for the written cue task. b – e , Example smoothed firing rates of neurons tuned to four words in the SMG for participant 1 (auditory cue, python ( b ), and written cue, telephone ( c )) and participant 2 (written cue, nifzig ( d ), and written cue, spoon ( e )). Top: the average firing rate over 8 or 16 trials (solid line, mean; shaded area, 95% bootstrapped confidence interval). Bottom: one example trial with associated audio amplitude (grey). Vertically dashed lines indicate the beginning of each phase. Single neurons modulate firing rate during internal speech in the SMG.
For each of the four language processes, we observed selective modulation of individual neurons’ firing rates (Fig. 2b–e ). In general, the firing rates of neurons increased during the active phases (cue, internal and speech) and decreased during the rest phases (ITI, D1 and D2). A variety of activation patterns were present in the neural population. Example neurons were selected to demonstrate increases in firing rates during internal speech, cue and vocalized speech. Both the auditory (Fig. 2b ) and the written cue (Fig. 2c–e ) evoked highly modulated firing rates of individual neurons during internal speech.
These stereotypical activation patterns were evident at the single-trial level (Fig. 2b–e , bottom). When the auditory recording was overlaid with firing rates from a single trial, a heterogeneous neural response was observed (Supplementary Fig. 1a ), with some SMG neurons preceding or lagging peak auditory levels during vocalized speech. In contrast, neural activity from primary sensory cortex (S1) only modulated during vocalized speech and produced similar firing patterns regardless of the vocalized word (Supplementary Fig. 1b ).
Population activity represented selective tuning for individual words
Population analysis in the SMG mirrored single-neuron patterns of activation, showing increases in tuning during the active task phases (Fig. 3a,d ). Tuning of a neuron to a word was determined by fitting a linear regression model to the firing rate in 50-ms time bins ( Methods ). Distinctions between participant 1 and participant 2 were observed. Specifically, participant 1 exhibited strong tuning, whereas the number of tuned units was notably lower in participant 2. Based on these findings, we exclusively ran the written cue task with participant number 2. In participant 1, representation of the auditory cue was lower compared with the written cue (Fig. 3b , cue). However, this difference was not observed for other task phases. In both participants, the tuned population activity in S1 increased during vocalized speech but not during the cue and internal speech phases (Supplementary Fig. 3a,b ).
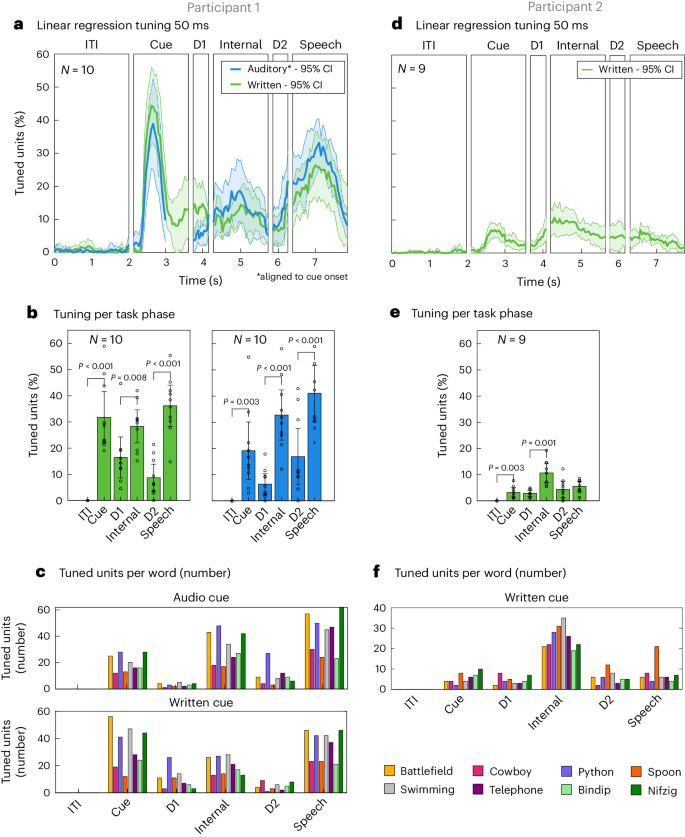
a , The average percentage of tuned neurons to words in 50-ms time bins in the SMG over the trial duration for ‘auditory cue’ (blue) and ‘written cue’ (green) tasks for participant 1 (solid line, mean over ten sessions; shaded area, 95% confidence interval of the mean). During the cue phase of auditory trials, neural data were aligned to audio onset, which occurred within 200–650 ms following initiation of the cue phase. b , The average percentage of tuned neurons computed on firing rates per task phase, with 95% confidence interval over ten sessions. Tuning during action phases (cue, internal and speech) following rest phases (ITI, D1 and D2) was significantly higher (paired two-tailed t -test, d.f. 9, P ITI_CueWritten < 0.001, Cohen’s d = 2.31; P ITI_CueAuditory = 0.003, Cohen’s d = 1.25; P D1_InternalWritten = 0.008, Cohen’s d = 1.08; P D1_InternalAuditory < 0.001, Cohen’s d = 1.71; P D2_SpeechWritten < 0.001, Cohen’s d = 2.34; P D2_SpeechAuditory < 0.001, Cohen’s d = 3.23). c , The number of neurons tuned to each individual word in each phase for the ‘auditory cue’ and ‘written cue’ tasks. d , The average percentage of tuned neurons to words in 50-ms time bins in the SMG over the trial duration for ‘written cue’ (green) tasks for participant 2 (solid line, mean over nine sessions; shaded area, 95% confidence interval of the mean). Due to a reduced number of tuned units, only the ‘written cue’ task variation was performed. e , The average percentage of tuned neurons computed on firing rates per task phase, with 95% confidence interval over nine sessions. Tuning during cue and internal phases following rest phases ITI and D1 was significantly higher (paired two-tailed t -test, d.f. 8, P ITI_CueWritten = 0.003, Cohen’s d = 1.38; P D1_Internal = 0.001, Cohen’s d = 1.67). f , The number of neurons tuned to each individual word in each phase for the ‘written cue’ task.
Source data
To quantitatively compare activity between phases, we assessed the differential response patterns for individual words by examining the variations in average firing rate across different task phases (Fig. 3b,e ). In both participants, tuning during the cue and internal speech phases was significantly higher compared with their preceding rest phases ITI and D1 (paired t -test between phases. Participant 1: d.f. 9, P ITI_CueWritten < 0.001, Cohen’s d = 2.31; P ITI_CueAuditory = 0.003, Cohen’s d = 1.25; P D1_InternalWritten = 0.008, Cohen’s d = 1.08; P D1_InternalAuditory < 0.001, Cohen’s d = 1.71. Participant 2: d.f. 8, P ITI_CueWritten = 0.003, Cohen’s d = 1.38; P D1_Internal = 0.001, Cohen’s d = 1.67). For participant 1, we also observed significantly higher tuning to vocalized speech than to tuning in D2 (d.f. 9, P D2_SpeechWritten < 0.001, Cohen’s d = 2.34; P D2_SpeechAuditory < 0.001, Cohen’s d = 3.23). Representation for all words was observed in each phase, including pseudowords (bindip and nifzig) (Fig. 3c,f ). To identify neurons with selective activity for unique words, we performed a Kruskal–Wallis test (Supplementary Fig. 3c,d ). The results mirrored findings of the regression analysis in both participants, albeit weaker in participant 2. These findings suggest that, while neural activity during active phases differed from activity during the ITI phase, neural responses of only a few neurons varied across different words for participant 2.
The neural population in the SMG simultaneously represented several distinct aspects of language processing: temporal changes, input modality (auditory, written for participant 1) and unique words from our vocabulary list. We used demixed principal component analysis (dPCA) to decompose and analyse contributions of each individual component: timing, cue modality and word. In Fig. 4 , demixed principal components (PCs) explaining the highest amount of variance were plotted by projecting data onto their respective dPCA decoder axis.
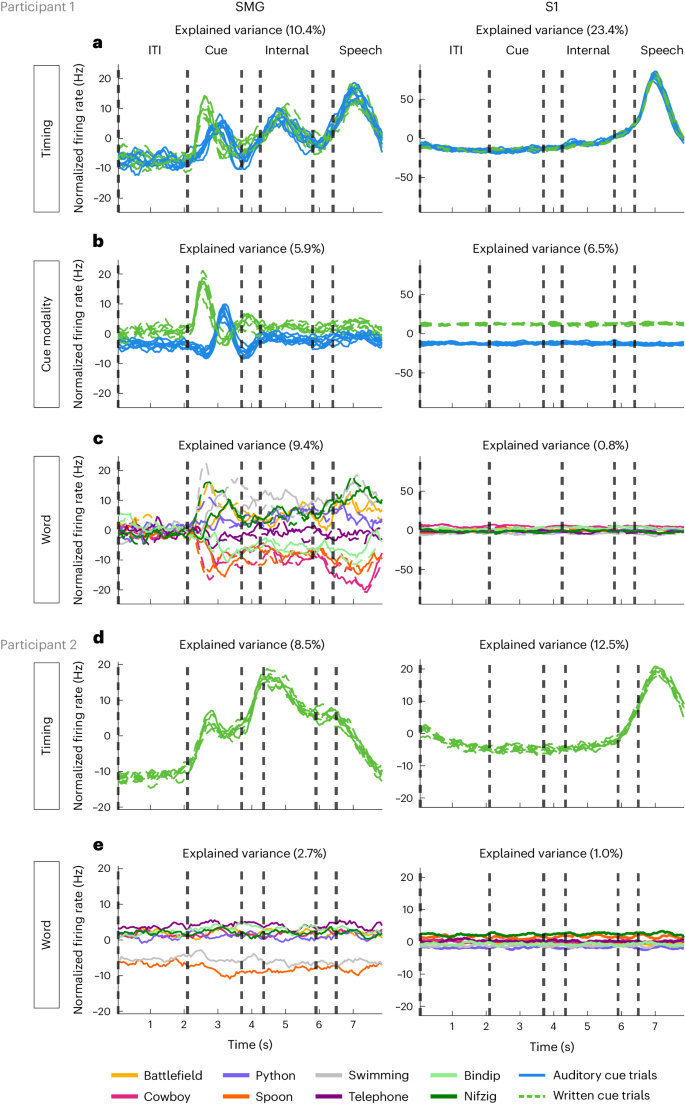
a – e , dPCA was performed to investigate variance within three marginalizations: ‘timing’, ‘cue modality’ and ‘word’ for participant 1 ( a – c ) and ‘timing’ and ‘word’ for participant 2 ( d and e ). Demixed PCs explaining the highest variance within each marginalization were plotted over time, by projecting the data onto their respective dPCA decoder axis. In a , the ‘timing’ marginalization demonstrates SMG modulation during cue, internal speech and vocalized speech, while S1 only represents vocalized speech. The solid blue lines (8) represent the auditory cue trials, and dashed green lines (8) represent written cue trials. In b , the ‘cue modality’ marginalization suggests that internal and vocalized speech representation in the SMG are not affected by the cue modality. The solid blue lines (8) represent the auditory cue trials, and dashed green lines (8) represent written cue trials. In c , the ‘word’ marginalization shows high variability for different words in the SMG, but near zero for S1. The colours (8) represent individual words. For each colour, solid lines represent auditory trials and dashed lines represent written cue trials. d is the same as a , but for participant 2. The dashed green lines (8) represent written cue trials. e is the same as c , but for participant 2. The colours (8) represent individual words during written cue trials. The variance for different words in the SMG (left) was higher than in S1 (right), but lower in comparison with SMG in participant 1 ( c ).
For participant 1, the ‘timing’ component revealed that temporal dynamics in the SMG peaked during all active phases (Fig. 4a ). In contrast, temporal S1 modulation peaked only during vocalized speech production, indicating a lack of synchronized lip and face movement of the participant during the other task phases. While ‘cue modality’ components were separable during the cue phase (Fig. 4b ), they overlapped during subsequent phases. Thus, internal and vocalized speech representation may not be influenced by the cue modality. Pseudowords had similar separability to lexical words (Fig. 4c ). The explained variance between words was high in the SMG and was close to zero in S1. In participant 2, temporal dynamics of the task were preserved (‘timing’ component). However, variance to words was reduced, suggesting lower neuronal ability to represent individual words in participant 2. In S1, the results mirrored findings from S1 in participant 1 (Fig. 4d,e , right).
Internal speech is decodable in the SMG
Separable neural representations of both internal and vocalized speech processes implicate SMG as a rich source of neural activity for real-time speech BMI devices. The decodability of words correlated with the percentage of tuned neurons (Fig. 3a–f ) as well as the explained dPCA variance (Fig. 4c,e ) observed in the participants. In participant 1, all words in our vocabulary list were highly decodable, averaging 55% offline decoding and 79% (16–20 training trials) online decoding from neurons during internal speech (Fig. 5a,b ). Words spoken during the vocalized phase were also highly discriminable, averaging 74% offline (Fig. 5a ). In participant 2, offline internal speech decoding averaged 24% (Supplementary Fig. 4b ) and online decoding averaged 23% (Fig. 5a ), with preferential representation of words ‘spoon’ and ‘swimming’.
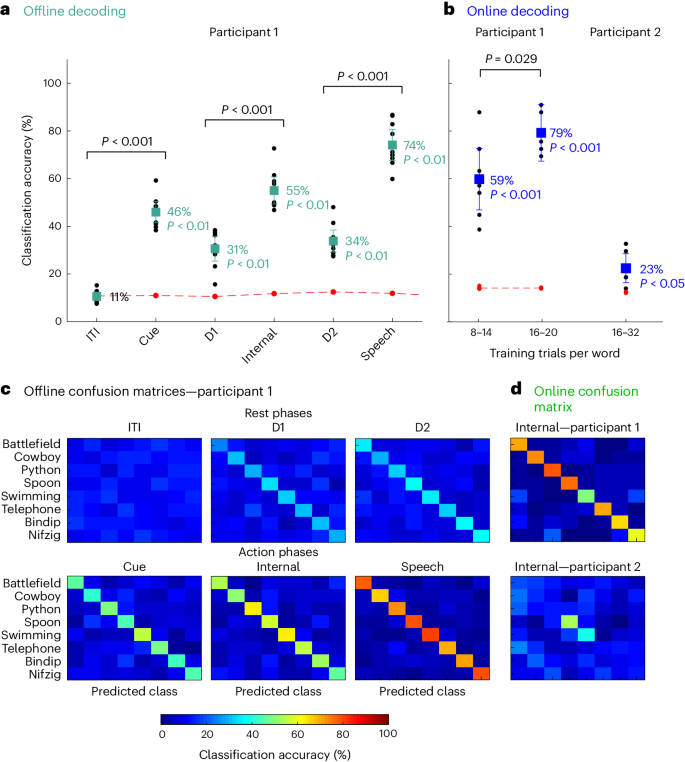
a , Offline decoding accuracies: ‘audio cue’ and ‘written cue’ task data were combined for each individual session day, and leave-one-out CV was performed (black dots). PCA was performed on the training data, an LDA model was constructed, and classification accuracies were plotted with 95% confidence intervals, over the session means. The significance of classification accuracies were evaluated by comparing results with a shuffled distribution (averaged shuffle results over 100 repetitions indicated by red dots; P < 0.01 indicates that the average mean is >99.5th percentile of shuffle distribution, n = 10). In participant 1, classification accuracies during action phases (cue, internal and speech) following rest phases (ITI, D1 and D2) were significantly higher (paired two-tailed t -test: n = 10, d.f. 9, for all P < 0.001, Cohen’s d = 6.81, 2.29 and 5.75). b , Online decoding accuracies: classification accuracies for internal speech were evaluated in a closed-loop internal speech BMI application on three different session days for both participants. In participant 1, decoding accuracies were significantly above chance (averaged shuffle results over 1,000 repetitions indicated by red dots; P < 0.001 indicates that the average mean is >99.95th percentile of shuffle distribution) and improved when 16–20 trials per words were used to train the model (two-sample two-tailed t -test, n (8–14) = 8, d.f. 11, n (16–20) = 5, P = 0.029), averaging 79% classification accuracy. In participant 2, online decoding accuracies were significant (averaged shuffle results over 1,000 repetitions indicated by red dots; P < 0.05 indicates that average mean is >97.5th percentile of shuffle distribution, n = 7) and averaged 23%. c , An offline confusion matrix for participant 1: confusion matrices for each of the different task phases were computed on the tested data and averaged over all session days. d , An online confusion matrix: a confusion matrix was computed combining all online runs, leading to a total of 304 trials (38 trials per word) for participant 1 and 448 online trials for participant 2. Participant 1 displayed comparable online decoding accuracies for all words, while participant 2 had preferential decoding for the words ‘swimming’ and ‘spoon’.
In participant 1, trial data from both types of cue (auditory and written) were concatenated for offline analysis, since SMG activity was only differentiable between the types of cue during the cue phase (Figs. 3a and 4b ). This resulted in 16 trials per condition. Features were selected via principal component analysis (PCA) on the training dataset, and PCs that explained 95% of the variance were kept. A linear discriminant analysis (LDA) model was evaluated with leave-one-out cross-validation (CV). Significance was computed by comparing results with a null distribution ( Methods ).
Significant word decoding was observed during all phases, except during the ITI (Fig. 5a , n = 10, mean decoding value above 99.5th percentile of shuffle distribution is P < 0.01, per phase, Cohen’s d = 0.64, 6.17, 3.04, 6.59, 3.93 and 8.26, confidence interval of the mean ± 1.73, 4.46, 5.21, 5.67, 4.63 and 6.49). Decoding accuracies were significantly higher in the cue, internal speech and speech condition, compared with rest phases ITI, D1 and D2 (Fig. 5a , paired t -test, n = 10, d.f. 9, for all P < 0.001, Cohen’s d = 6.81, 2.29 and 5.75). Significant cue phase decoding suggested that modality-independent linguistic representations were present early within the task 45 . Internal speech decoding averaged 55% offline, with the highest session at 72% and a chance level of ~12.5% (Fig. 5a , red line). Vocalized speech averaged even higher, at 74%. All words were highly decodable (Fig. 5c ). As suggested from our dPCA results, individual words were not significantly decodable from neural activity in S1 (Supplementary Fig. 4a ), indicating generalized activity for vocalized speech in the S1 arm region (Fig. 4c ).
For participant 2, SMG significant word decoding was observed during the cue, internal and vocalized speech phases (Supplementary Fig. 4b , n = 9, mean decoding value above 97.5th/99.5th percentile of shuffle distribution is P < 0.05/ P < 0.01, per phase Cohen’s d = 0.35, 1.15, 1.09, 1.44, 0.99 and 1.49, confidence interval of the mean ± 3.09, 5.02, 6.91, 8.14, 5.45 and 4.15). Decoding accuracies were significantly higher in the cue and internal speech condition, compared with rest phases ITI and D1 (Supplementary Fig. 4b , paired t -test, n = 9, d.f. 8, P ITI_Cue = 0.013, Cohen’s d = 1.07, P D1_Internal = 0.01, Cohen’s d = 1.11). S1 decoding mirrored results in participant 1, suggesting that no synchronized face movements occurred during the cue phase or internal speech phase (Supplementary Fig. 4c ).
High-accuracy online speech decoder
We developed an online, closed-loop internal speech BMI using an eight-word vocabulary (Fig. 5b ). On three separate session days, training datasets were generated using the written cue task, with eight repetitions of each word for each participant. An LDA model was trained on the internal speech data of the training set, corresponding to only 1.5 s of neural data per repetition for each class. The trained decoder predicted internal speech during the online task. During the online task, the vocalized speech phase was replaced with a feedback phase. The decoded word was shown in green if correctly decoded, and in red if wrongly decoded (Supplementary Video 1 ). The classifier was retrained after each run of the online task, adding the newly recorded data. Several online runs were performed on each session day, corresponding to different datapoints on Fig. 5b . When using between 8 and 14 repetitions per words to train the decoding model, an average of 59% classification accuracy was obtained for participant 1. Accuracies were significantly higher (two-sample two-tailed t -test, n (8–14) = 8, n (16–20) = 5, d.f. 11, P = 0.029) the more data were added to train the model, obtaining an average of 79% classification accuracy with 16–20 repetitions per word. The highest single run accuracy was 91%. All words were well represented, illustrated by a confusion matrix of 304 trials (Fig. 5d ). In participant 2, decoding was statistically significant, but lower compared with participant 1. The lower number of tuned units (Fig. 3a–f ) and reduced explained variance between words (Fig. 4e , left) could account for these findings. Additionally, preferential representation of words ‘spoon’ and ‘swimming’ was observed.
Shared representations between internal speech, written words and vocalized speech
Different language processes are engaged during the task: auditory comprehension or visual word recognition during the cue phase, and internal speech and vocalized speech production during the speech phases. It has been widely assumed that each of these processes is part of a highly distributed network, involving multiple cortical areas 46 . In this work, we observed significant representation of different language processes in a common cortical region, SMG, in our participants. To explore the relationships between each of these processes, for participant 1 we used cross-phase classification to identify the distinct and common neural codes separately in the auditory and written cue datasets. By training our classifier on the representation found in one phase (for example, the cue phase) and testing the classifier on another phase (for example, internal speech), we quantified generalizability of our models across neural activity of different language processes (Fig. 6 ). The generalizability of a model to different task phases was evaluated through paired t -tests. No significant difference between classification accuracies indicates good generalization of the model, while significantly lower classification accuracies suggest poor generalization of the model.
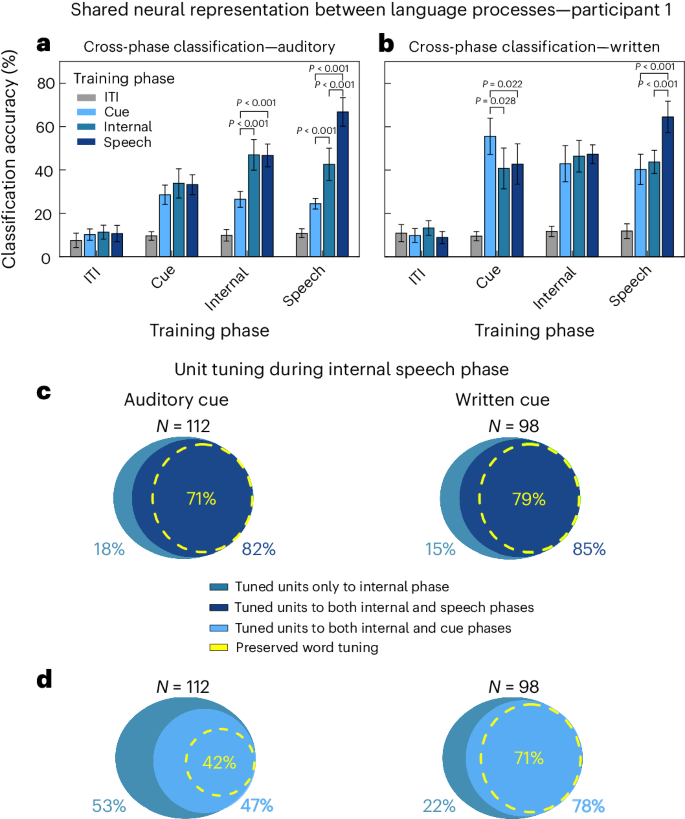
a , Evaluating the overlap of shared information between different task phases in the ‘auditory cue’ task. For each of the ten session days, cross-phase classification was performed. It consisted in training a model on a subset of data from one phase (for example, cue) and applying it on a subset of data from ITI, cue, internal and speech phases. This analysis was performed separately for each task phase. PCA was performed on the training data, an LDA model was constructed and classification accuracies were plotted with a 95% confidence interval over session means. Significant differences in performance between phases were evaluated between the ten sessions (paired two-tailed t -test, FDR corrected, d.f. 9, P < 0.001 for all, Cohen’s d ≥ 1.89). For easier visibility, significant differences between ITI and other phases were not plotted. b , Same as a for the ‘written cue’ task (paired two-tailed t -test, FDR corrected, d.f. 9, P Cue_Internal = 0.028, Cohen’s d > 0.86; P Cue_Speech = 0.022, Cohen’s d = 0.95; all others P < 0.001 and Cohen’s d ≥ 1.65). c , The percentage of neurons tuned during the internal speech phase that are also tuned during the vocalized speech phase. Neurons tuned during the internal speech phase were computed as in Fig. 3b separately for each session day. From these, the percentage of neurons that were also tuned during vocalized speech was calculated. More than 80% of neurons during internal speech were also tuned during vocalized speech (82% in the ‘auditory cue’ task, 85% in the ‘written cue’ task). In total, 71% of ‘auditory cue’ and 79% ‘written cue’ neurons also preserved tuning to at least one identical word during internal speech and vocalized speech phases. d , The percentage of neurons tuned during the internal speech phase that were also tuned during the cue phase. Right: 78% of neurons tuned during internal speech were also tuned during the written cue phase. Left: a smaller 47% of neurons tuned during the internal speech phase were also tuned during the auditory cue phase. In total, 71% of neurons preserved tuning between the written cue phase and the internal speech phase, while 42% of neurons preserved tuning between the auditory cue and the internal speech phase.
The strongest shared neural representations were found between visual word recognition, internal speech and vocalized speech (Fig. 6b ). A model trained on internal speech was highly generalizable to both vocalized speech and written cued words, evidence for a possible shared neural code (Fig. 6b , internal). In contrast, the model’s performance was significantly lower when tested on data recorded in the auditory cue phase (Fig. 6a , training phase internal: paired t -test, d.f. 9, P Cue_Internal < 0.001, Cohen’s d = 2.16; P Cue_Speech < 0.001, Cohen’s d = 3.34). These differences could stem from the inherent challenges in comparing visual and auditory language stimuli, which differ in processing time: instantaneous for text versus several hundred milliseconds for auditory stimuli.
We evaluated the capability of a classification model, initially trained to distinguish words during vocalized speech, in its ability to generalize to internal and cue phases (Fig. 6a,b , training phase speech). The model demonstrated similar levels of generalization during internal speech and in response to written cues, as indicated by the lack of significance in decoding accuracy between the internal and written cue phase (Fig. 6b , training phase speech, cue–internal). However, the model generalized significantly better to internal speech than to representations observed during the auditory cue phase (Fig. 6a , training phase speech, d.f. 9, P Cue_Internal < 0.001, Cohen’s d = 2.85).
Neuronal representation of words at the single-neuron level was highly consistent between internal speech, vocalized speech and written cue phases. A high percentage of neurons were not only active during the same task phases but also preserved identical tuning to at least one word (Fig. 6c,d ). In total, 82–85% of neurons active during internal speech were also active during vocalized speech. In 71–79% of neurons, tuning was preserved between the internal speech and vocalized speech phases (Fig. 6c ). During the cue phase, 78% of neurons active during internal speech were also active during the written cue (Fig. 6d , right). However, a lower percentage of neurons (47%) were active during the auditory cue phase (Fig. 6d , left). Similarly, 71% of neurons preserved tuning between the written cue phase and the internal speech phase, while 42% of neurons preserved tuning between the auditory cue phase and the internal speech phase.
Together with the cross-phase analysis, these results suggest strong shared neural representations between internal speech, vocalized speech and the written cue, both at the single-neuron and at the population level.
Robust decoding of multiple internal speech strategies within the SMG
Strong shared neural representations in participant 1 between written, inner and vocalized speech suggest that all three partly represent the same cognitive process or all cognitive processes share common neural features. While internal and vocalized speech have been shown to share common neural features 36 , similarities between internal speech and the written cue could have occurred through several different cognitive processes. For instance, the participant’s observation of the written cue could have activated silent reading. This process has been self-reported as activating internal speech, which can involve ‘hearing’ a voice, thus having an auditory component 42 , 47 . However, the participant could also have mentally pictured an image of the written word while performing internal speech, involving visual imagination in addition to language processes. Both hypotheses could explain the high amount of shared neural representation between the written cue and the internal speech phases (Fig. 6b ).
We therefore compared two possible internal sensory strategies in participant 1: a ‘sound imagination’ strategy in which the participant imagined hearing the word, and a ‘visual imagination’ strategy in which the participant visualized the word’s image (Supplementary Fig. 5a ). Each strategy was cued by the modalities we had previously tested (auditory and written words) (Table 1 ). To assess the similarity of these internal speech processes to other task phases, we conducted a cross-phase decoding analysis (as performed in Fig. 6 ). We hypothesized that, if the high cross-decoding results between internal and written cue phases primarily stemmed from the participant engaging in visual word imagination, we would observe lower decoding accuracies during the auditory imagination phase.
Both strategies demonstrated high representation of the four-word dataset (Supplementary Fig. 5b , highest 94%, chance level 25%). These results suggest our speech BMI decoder is robust to multiple types of internal speech strategy.
The participant described the ‘sound imagination’ strategy as being easier and more similar to the internal speech condition of the first experiment. The participant’s self-reported strategy suggests that no visual imagination was performed during internal speech. Correspondingly, similarities between written cue and internal speech phases may stem from internal speech activation during the silent reading of the cue.
In this work, we demonstrated a decoder for internal and vocalized speech, using single-neuron activity from the SMG. Two chronically implanted, speech-abled participants with tetraplegia were able to use an online, closed-loop internal speech BMI to achieve on average 79% and 23% classification accuracy with 16–32 training trials for an eight-word vocabulary. Furthermore, high decoding was achievable with only 24 s of training data per word, corresponding to 16 trials each with 1.5 s of data. Firing rates recorded from S1 showed generalized activation only during vocalized speech activity, but individual words were not classifiable. In the SMG, shared neural representations between internal speech, the written cue and vocalized speech suggest the occurrence of common processes. Robust control could be achieved using visual and auditory internal speech strategies. Representation of pseudowords provided evidence for a phonetic word encoding component in the SMG.
Single neurons in the SMG encode internal speech
We demonstrated internal speech decoding of six different words and two pseudowords in the SMG. Single neurons increased their firing rates during internal speech (Fig. 2 , S1 and S2), which was also reflected at the population level (Fig. 3a,b,d,e ). Each word was represented in the neuronal population (Fig. 3c,f ). Classification accuracy and tuning during the internal speech phase were significantly higher than during the previous delay phase (Figs. 3b,e and 5a , and Supplementary Figs. 3c,d and 4b ). This evidence suggests that we did not simply decode sustained activity from the cue phase but activity generated by the participant performing internal speech. We obtained significant offline and online internal speech decoding results in two participants (Fig. 5a and Supplementary Fig. 4b ). These findings provide strong evidence for internal speech processing at the single-neuron level in the SMG.
Neurons in S1 are modulated by vocalized but not internal speech
Neural activity recorded from S1 served as a control for synchronized face and lip movements during internal speech. While vocalized speech robustly activated sensory neurons, no increase of baseline activity was observed during the internal speech phase or the auditory and written cue phases in both participants (Fig. 4 , S1). These results underline no synchronized movement inflated our decoding accuracy of internal speech (Supplementary Fig. 4a,c ).
A previous imaging study achieved significant offline decoding of several different internal speech sentences performed by patients with mild ALS 6 . Together with our findings, these results suggest that a BMI speech decoder that does not rely on any movement may translate to communication opportunities for patients suffering from ALS and locked-in syndrome.
Different face activities are observable but not decodable in arm area of S1
The topographic representation of body parts in S1 has recently been found to be less rigid than previously thought. Generalized finger representation was found in a presumably S1 arm region of interest (ROI) 44 . Furthermore, an fMRI paper found observable face and lip activity in S1 leg and hand ROIs. However, differentiation between two lip actions was restricted to the face ROI 43 . Correspondingly, we observed generalized face and lip activity in a predominantly S1 arm region for participant 1 (see ref. 48 for implant location) and a predominantly S1 hand region for participant 2 during vocalized speech (Fig. 4a,d and Supplementary Figs. 1 and 4a,b ). Recorded neural activity contained similar representations for different spoke words (Fig. 4c,e ) and was not significantly decodable (Supplementary Fig. 4a,c ).
Shared neural representations between internal and vocalized speech
The extent to which internal and vocalized speech generalize is still debated 35 , 42 , 49 and depends on the investigated brain area 36 , 50 . In this work, we found on average stronger representation for vocalized (74%) than internal speech (Fig. 5a , 55%) in participant 1 but the opposite effect in participant 2 (Supplementary Fig. 4b , 24% internal, 21% vocalized speech). Additionally, cross-phase decoding of vocalized speech from models trained on data during internal speech resulted in comparable classification accuracies to those of internal speech (Fig. 6a,b , internal). Most neurons tuned during internal speech were also tuned to at least one of the same words during vocalized speech (71–79%; Fig. 6c ). However, some neurons were only tuned during internal speech, or to different words. These observations also applied to firing rates of individual neurons. Here, we observed neurons that had higher peak rates during the internal speech phase than the vocalized speech phase (Supplementary Fig. 1 : swimming and cowboy). Together, these results further suggest neural signatures during internal and vocalized speech are similar but distinct from one another, emphasizing the need for developing speech models from data recorded directly on internal speech production 51 .
Similar observations were made when comparing internal speech processes with visual word processes. In total, 79% of neurons were active both in the internal speech phase and the written cue phase, and 79% preserved the same tuning (Fig. 6d , written cue). Additionally, high cross-decoding between both phases was observed (Fig. 6b , internal).
Shared representation between speech and written cue presentation
Observation of a written cue may engage a variety of cognitive processes, such as visual feature recognition, semantic understanding and/or related language processes, many of which modulate similar cortical regions as speech 45 . Studies have found that silent reading can evoke internal speech; it can be modulated by a presumed author’s speaking speed, voice familiarity or regional accents 35 , 42 , 47 , 52 , 53 . During silent reading of a cued sentence with a neutral versus increased prosody (madeleine brought me versus MADELEINE brought me), one study in particular found that increased left SMG activation correlated with the intensity of the produced inner speech 54 .
Our data demonstrated high cross-phase decoding accuracies between both written cue and speech phases in our first participant (Fig. 6b ). Due to substantial shared neural representation, we hypothesize that the participant’s silent reading during the presentation of the written cue may have engaged internal speech processes. However, this same shared representation could have occurred if visual processes were activated in the internal speech phase. For instance, the participant could have performed mental visualization of the written word instead of generating an internal monologue, as the subjective perception of internal speech may vary between individuals.
Investigating internal speech strategies
In a separate experiment, participant 1 was prompted to execute different mental strategies during the internal speech phase, consisting of ‘sound imagination’ or ‘visual word imagination’ (Supplementary Fig. 5a ). We found robust decoding during the internal strategy phase, regardless of which mental strategy was performed (Supplementary Fig. 5b ). This participant reported the sound strategy was easier to execute than the visual strategy. Furthermore, this participant reported that the sound strategy was more similar to the internal speech strategy employed in prior experiments. This self-report suggests that the patient did not perform visual imagination during the internal speech task. Therefore, shared neural representation between internal and written word phases during the internal speech task may stem from silent reading of the written cue. Since multiple internal mental strategies are decodable from SMG, future patients could have flexibility with their preferred strategy. For instance, people with a strong visual imagination may prefer performing visual word imagination.
Audio contamination in decoding result
Prior studies examining neural representation of attempted or vocalized speech must potentially mitigate acoustic contamination of electrophysiological brain signals during speech production 55 . During internal speech production, no detectable audio was captured by the audio equipment or noticed by the researchers in the room. In the rare cases the participant spoke during internal speech (three trials), the trials were removed. Furthermore, if audio had contaminated the neural data during the auditory cue or vocalized speech, we would have probably observed significant decoding in all channels. However, no significant classification was detected in S1 channels during the auditory cue phase nor the vocalized speech phase (Supplementary Fig. 2b ). We therefore conclude that acoustic contamination did not artificially inflate observed classification accuracies during vocalized speech in the SMG.
Single-neuron modulation during internal speech with a second participant
We found single-neuron modulation to speech processes in a second participant (Figs. 2d,e and 3f , and Supplementary Fig. 2d ), as well as significant offline and online classification accuracies (Fig. 5a and Supplementary Fig. 4b ), confirming neural representation of language processes in the SMG. The number of neurons distinctly active for different words was lower compared with the first participant (Fig. 2e and Supplementary Fig. 3d ), limiting our ability to decode with high accuracy between words in the different task phases (Fig. 5a and Supplementary Fig. 4b ).
Previous work found that single neurons in the PPC exhibited a common neural substrate for written action verbs and observed actions 56 . Another study found that single neurons in the PPC also encoded spoken numbers 57 . These recordings were made in the superior parietal lobule whereas the SMG is in the inferior parietal lobule. Thus, it would appear that language-related activity is highly distributed across the PPC. However, the difference in strength of language representation between each participant in the SMG suggests that there is a degree of functional segregation within the SMG 37 .
Different anatomical geometries of the SMG between participants mean that precise comparisons of implanted array locations become difficult (Fig. 1 ). Implant locations for both participants were informed from pre-surgical anatomical/vasculature scans and fMRI tasks designed to evoke activity related to grasp and dexterous hand movements 48 . Furthermore, the number of electrodes of the implanted array was higher in the first participant (96) than in the second participant (64). A pre-surgical assessment of functional activity related to language and speech may be required to determine the best candidate implant locations within the SMG for online speech decoding applications.
Impact on BMI applications
In this work, an online internal speech BMI achieved significant decoding from single-neuron activity in the SMG in two participants with tetraplegia. The online decoders were trained on as few as eight repetitions of 1.5 s per word, demonstrating that meaningful classification accuracies can be obtained with only a few minutes’ worth of training data per day. This proof-of-concept suggests that the SMG may be able to represent a much larger internal vocabulary. By building models on internal speech directly, our results may translate to people who cannot vocalize speech or are completely locked in. Recently, ref. 26 demonstrated a BMI speller that decoded attempted speech of the letters of the NATO alphabet and used those to construct sentences. Scaling our vocabulary to that size could allow for an unrestricted internal speech speller.
To summarize, we demonstrate the SMG as a promising candidate to build an internal brain–machine speech device. Different internal speech strategies were decodable from the SMG, allowing patients to use the methods and languages with which they are most comfortable. We found evidence for a phonetic component during internal and vocalized speech. Adding to previous findings indicating grasp decoding in the SMG 23 , we propose the SMG as a multipurpose BMI area.
Experimental model and participant details
Two male participants with tetraplegia (33 and 39 years) were recruited for an institutional review board- and Food and Drug Administration-approved clinical trial of a BMI and gave informed consent to participate (Institutional Review Board of Rancho Los Amigos National Rehabilitation Center, Institutional Review Board of California Institute of Technology, clinical trial registration NCT01964261 ). This clinical trial evaluated BMIs in the PPC and the somatosensory cortex for grasp rehabilitation. One of the primary effectiveness objectives of the study is to evaluate the effectiveness of the neuroport in controlling virtual or physical end effectors. Signals from the PPC will allow the subjects to control the end effector with accuracy greater than chance. Participants were compensated for their participation in the study and reimbursed for any travel expenses related to participation in study activities. The authors affirm that the human research participant provided written informed consent for publication of Supplementary Video 1 . The first participant suffered a spinal cord injury at cervical level C5 1.5 years before participating in the study. The second participant suffered a C5–C6 spinal cord injury 3 years before implantation.
Method details
Data were collected from implants located in the left SMG and the left S1 (for anatomical locations, see Fig. 1 ). For description of pre-surgical planning, localization fMRI tasks, surgical techniques and methodologies, see ref. 48 . Placement of electrodes was based on fMRI tasks involving grasp and dexterous hand movements.
The first participant underwent surgery in November 2016 to implant two 96-channel platinum-tipped multi-electrode arrays (NeuroPort Array, Blackrock Microsystems) in the SMG and in the ventral premotor cortex and two 7 × 7 sputtered iridium oxide film (SIROF)-tipped microelectrode arrays with 48 channels each in the hand and arm area of S1. Data were collected between July 2021 and August 2022. The second participant underwent surgery in October 2022 and was implanted with SIROF-tipped 64-channel microelectrode arrays in S1 (two arrays), SMG, ventral premotor cortex and primary motor cortex. Data were collected in January 2023.
Data collection
Recording began 2 weeks after surgery and continued one to three times per week. Data for this work were collected between 2021 and 2023. Broadband electrical activity was recorded from the NeuroPort Arrays using Neural Signal Processors (Blackrock Microsystems). Analogue signals were amplified, bandpass filtered (0.3–7,500 Hz) and digitized at 30,000 samples s −1 . To identify putative action potentials, these broadband data were bandpass filtered (250–5,000 Hz) and thresholded at −4.5 the estimated root-mean-square voltage of the noise. For some of the analyses, waveforms captured at these threshold crossings were then spike sorted by manually assigning each observation to a putative single neuron; for others, multiunit activity was considered. For participant 1, an average of 33 sorted SMG units (between 22 and 56) and 83 sorted S1 units (between 59 and 96) were recorded per session. For participant 2, an average of 80 sorted SMG units (between 69 and 92) and 81 sorted S1 units (between 61 and 101) were recorded per session. Auditory data were recorded at 30,000 Hz simultaneously to the neural data. Background noise was reduced post-recording by using the noise reduction function of the program ‘Audible’.
Experimental tasks
We implemented different tasks to study language processes in the SMG. The tasks cued six words informed by ref. 31 (spoon, python, battlefield, cowboy, swimming and telephone) as well as two pseudowords (bindip and nifzig). The participants were situated 1 m in front of a light-emitting diode screen (1,190 mm screen diagonal), where the task was visualized. The task was implemented using the Psychophysics Toolbox 58 , 59 , 60 extension for MATLAB. Only the written cue task was used for participant 2.
Auditory cue task
Each trial consisted of six phases, referred to in this paper as ITI, cue, D1, internal, D2 and speech. The trial began with a brief ITI (2 s), followed by a 1.5-s-long cue phase. During the cue phase, a speaker emitted the sound of one of the eight words (for example, python). Word duration varied between 842 and 1,130 ms. Then, after a delay period (grey circle on screen; 0.5 s), the participant was instructed to internally say the cued word (orange circle on screen; 1.5 s). After a second delay (grey circle on screen; 0.5 s), the participant vocalized the word (green circle on screen, 1.5 s).
Written cue task
The task was identical to the auditory cue task, except words were cued in writing instead of sound. The written word appeared on the screen for 1.5 s during the cue phase. The auditory cue was played between 200 ms and 650 ms later than the written cue appeared on the screen, due to the utilization of varied sound outputs (direct computer audio versus Bluetooth speaker).
One auditory cue task and one written cue task were recorded on ten individual session days in participant 1. The written cue task was recorded on seven individual session days in participant 2.
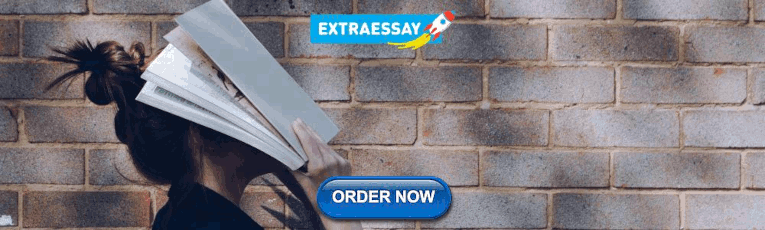
Control experiments
Three experiments were run to investigate internal strategies and phonetic versus semantic processing.
Internal strategy task
The task was designed to vary the internal strategy employed by the participant during the internal speech phase. Two internal strategies were tested: a sound imagination and a visual imagination. For the ‘sound imagination’ strategy, the participant was instructed to imagine what the sound of the word sounded like. For the ‘visual imagination’ strategy, the participant was instructed to perform mental visualization from the written word. We also tested if the cue modality (auditory or written) influenced the internal strategy. A subset of four words were used for this experiment. This led to four different variations of the task.
The internal strategy task was run on one session day with participant 1.
Online task
The ‘written cue task’ was used for the closed-loop experiments. To obtain training data for the online task, a written cue task was run. Then, a classification model was trained only on the internal speech data of the task (see ‘Classification’ section). The closed-loop task was nearly identical to the ‘written cue task’ but replaced the vocalized speech phase by a feedback phase. Feedback was provided by showing the word on the screen either in green if correctly classified or in red if wrongly classified. See Supplementary Video 1 for an example of the participant performing the online task. The online task was run on three individual session days.
Error trials
Trials in which participants accidentally spoke during the internal speech part (3 trials) or said the wrong word during the vocalized speech part (20 trials) were removed from all analysis.
Total number of recording trials
For participant 1, we collected offline datasets composed of eight trials per word across ten sessions. Trials during which participant errors occurred were excluded. In total, between 156 and 159 trials per word were included, with a total of 1,257 trials for offline analysis. On four non-consecutive session days, the auditory cue task was run first, and on six non-consecutive days, the written cue task was run first. For online analysis, datasets were recorded on three different session days, for a total of 304 trials. Participant 2 underwent a similar data collection process, with offline datasets comprising 16 trials per word using the written cue modality over nine sessions. Error trials were excluded. In total, between 142 and 144 trials per word were kept, with a total of 1,145 trials for offline analysis. For online analysis, datasets were recorded on three session days, leading to a total of 448 online trials.
Quantification and statistical analysis
Analyses were performed using MATLAB R2020b and Python, version 3.8.11.
Neural firing rates
Firing rates of sorted units were computed as the number of spikes occurring in 50-ms bins, divided by the bin width and smoothed using a Gaussian filter with kernel width of 50 ms to form an estimate of the instantaneous firing rates (spikes s −1 ).
Linear regression tuning analysis
To identify units exhibiting selective firing rate patterns (or tuning) for each of the eight words, linear regression analysis was performed in two different ways: (1) step by step in 50-ms time bins to allow assessing changes in neuronal tuning over the entire trial duration; (2) averaging the firing rate in each task phase to compare tuning between phases. The model returns a fit that estimates the firing rate of a unit on the basis of the following variables:
where FR corresponds to the firing rate of the unit, β 0 to the offset term equal to the average ITI firing rate of the unit, X is the vector indicator variable for each word w , and β w corresponds to the estimated regression coefficient for word w . W was equal to 8 (battlefield, cowboy, python, spoon, swimming, telephone, bindip and nifzig) 23 .
In this model, β symbolizes the change of firing rate from baseline for each word. A t -statistic was calculated by dividing each β coefficient by its standard error. Tuning was based on the P value of the t -statistic for each β coefficient. A follow-up analysis was performed to adjust for false discovery rate (FDR) between the P values 61 , 62 . A unit was defined as tuned if the adjusted P value is <0.05 for at least one word. This definition allowed for tuning of a unit to zero, one or multiple words during different timepoints of the trial. Linear regression was performed for each session day individually. A 95% confidence interval of the mean was computed by performing the Student’s t -inverse cumulative distribution function over the ten sessions.
Kruskal–Wallis tuning analysis
As an alternative tuning definition, differences in firing rates between words were tested using the Kruskal–Wallis test, the non-parametric analogue to the one-way analysis of variance (ANOVA). For each neuron, the analysis was performed to evaluate the null hypothesis that data from each word come from the same distribution. A follow-up analysis was performed to adjust for FDR between the P values for each task phase 61 , 62 . A unit was defined as tuned during a phase if the adjusted P value was smaller than α = 0.05.
Classification
Using the neuronal firing rates recorded during the tasks, a classifier was used to evaluate how well the set of words could be differentiated during each phase. Classifiers were trained using averaged firing rates over each task phase, resulting in six matrices of size n , m , where n corresponds to the number of trials and m corresponds to the number of recorded units. A model for each phase was built using LDA, assuming an identical covariance matrix for each word, which resulted in best classification accuracies. Leave-one-out CV was performed to estimate decoding performance, leaving out a different trial across neurons at each loop. PCA was applied on the training data, and PCs explaining more than 95% of the variance were selected as features and applied to the single testing trial. A 95% confidence interval of the mean was computed as described above.
Cross-phase classification
To estimate shared neural representations between different task phases, we performed cross-phase classification. The process consisted in training a classification model (as described above) on one of the task phases (for example, ITI) and to test it on the ITI, cue, imagined speech and vocalized speech phases. The method was repeated for each of the ten sessions individually, and a 95% confidence interval of the mean was computed. Significant differences in classification accuracies between phases decoded with the same model were evaluated using a paired two-tailed t -test. FDR correction of the P values was performed (‘Linear regression tuning analysis’) 61 , 62 .
Classification performance significance testing
To assess the significance of classification performance, a null dataset was created by repeating classification 100 times with shuffled labels. Then, different percentile levels of this null distribution were computed and compared to the mean of the actual data. Mean classification performances higher than the 97.5th percentile were denoted with P < 0.05 and higher than 99.5th percentile were denoted with P < 0.01.
dPCA analysis
dPCA was performed on the session data to study the activity of the neuronal population in relation to the external task parameters: cue modality and word. Kobak et al. 63 introduced dPCA as a refinement of their earlier dimensionality reduction technique (of the same name) that attempts to combine the explanatory strengths of LDA and PCA. By deconstructing neuronal population activity into individual components, each component relates to a single task parameter 64 .
This text follows the methodology outlined by Kobak et al. 63 . Briefly, this involved the following steps for N neurons:
First, unlike in PCA, we focused not on the matrix, X , of the original data, but on the matrices of marginalizations, X ϕ . The marginalizations were computed as neural activity averaged over trials, k , and some task parameters in analogy to the covariance decomposition done in multivariate analysis of variance. Since our dataset has three parameters: timing, t , cue modality, \(c\) (for example, auditory or visual), and word, w (eight different words), we obtained the total activity as the sum of the average activity with the marginalizations and a final noise term
The above notation of Kobak et al. is the same as used in factorial ANOVA, that is, \({X}_{{tcwk}}\) is the matrix of firing rates for all neurons, \(< \bullet { > }_{{ab}}\) is the average over a set of parameters \(a,b,\ldots\) , \(\bar{X}= < {X}_{{tcwk}}{ > }_{{tcwk}}\) , \({\bar{X}}_{t}= < {X}_{{tcwk}}-\bar{X}{ > }_{{cwk}}\) , \({\bar{X}}_{{tc}}= < {X}_{{tcwk}}-\bar{X}-{\bar{X}}_{t}-{\bar{X}}_{c}-{\bar{X}}_{w}{ > }_{{wk}}\) and so on. Finally, \({{{\epsilon }}}_{{tcwk}}={X}_{{tcwk}}- < {X}_{{tcwk}}{ > }_{k}\) .
Participant 1 datasets were composed of N = 333 (SMG), N = 828 (S1) and k = 8. Participant 2 datasets were composed of N = 547 (SMG), N = 522 (S1) and k = 16. To create balanced datasets, error trials were replaced by the average firing rate of k − 1 trials.
Our second step reduced the number of terms by grouping them as seen by the braces in the equation above, since there is no benefit in demixing a time-independent pure task, \(a\) , term \({\bar{X}}_{a}\) from the time–task interaction terms \({\bar{X}}_{{ta}}\) since all components are expected to change with time. The above grouping reduced the parametrization down to just five marginalization terms and the noise term (reading in order): the mean firing rate, the task-independent term, the cue modality term, the word term, the cue modality–word interaction term and the trial-to-trial noise.
Finally, we gained extra flexibility by having two separate linear mappings \({F}_{\varphi }\) for encoding and \({D}_{\varphi }\) for decoding (unlike in PCA, they are not assumed to be transposes of each other). These matrices were chosen to minimize the loss function (with a quadratic penalty added to avoid overfitting):
Here, \({{\mu }}=(\lambda\Vert X\Vert)^{2}\) , where λ was optimally selected through tenfold CV in each dataset.
We refer the reader to Kobak et al. for a description of the full analytic solution.
Reporting summary
Further information on research design is available in the Nature Portfolio Reporting Summary linked to this article.
Data availability
The data supporting the findings of this study are openly available via Zenodo at https://doi.org/10.5281/zenodo.10697024 (ref. 65 ). Source data are provided with this paper.
Code availability
The custom code developed for this study is openly available via Zenodo at https://doi.org/10.5281/zenodo.10697024 (ref. 65 ).
Hecht, M. et al. Subjective experience and coping in ALS. Amyotroph. Lateral Scler. Other Mot. Neuron Disord. 3 , 225–231 (2002).
Google Scholar
Aflalo, T. et al. Decoding motor imagery from the posterior parietal cortex of a tetraplegic human. Science 348 , 906–910 (2015).
CAS PubMed PubMed Central Google Scholar
Andersen, R. A. Machines that translate wants into actions. Scientific American https://www.scientificamerican.com/article/machines-that-translate-wants-into-actions/ (2019).
Andersen, R. A., Aflalo, T. & Kellis, S. From thought to action: the brain–machine interface in posterior parietal cortex. Proc. Natl Acad. Sci. USA 116 , 26274–26279 (2019).
Andersen, R. A., Kellis, S., Klaes, C. & Aflalo, T. Toward more versatile and intuitive cortical brain machine interfaces. Curr. Biol. 24 , R885–R897 (2014).
Dash, D., Ferrari, P. & Wang, J. Decoding imagined and spoken phrases from non-invasive neural (MEG) signals. Front. Neurosci. 14 , 290 (2020).
PubMed PubMed Central Google Scholar
Luo, S., Rabbani, Q. & Crone, N. E. Brain–computer interface: applications to speech decoding and synthesis to augment communication. Neurotherapeutics https://doi.org/10.1007/s13311-022-01190-2 (2022).
Article PubMed PubMed Central Google Scholar
Martin, S., Iturrate, I., Millán, J. D. R., Knight, R. T. & Pasley, B. N. Decoding inner speech using electrocorticography: progress and challenges toward a speech prosthesis. Front. Neurosci. 12 , 422 (2018).
Rabbani, Q., Milsap, G. & Crone, N. E. The potential for a speech brain–computer interface using chronic electrocorticography. Neurotherapeutics 16 , 144–165 (2019).
Lopez-Bernal, D., Balderas, D., Ponce, P. & Molina, A. A state-of-the-art review of EEG-based imagined speech decoding. Front. Hum. Neurosci. 16 , 867281 (2022).
Nicolas-Alonso, L. F. & Gomez-Gil, J. Brain computer interfaces, a review. Sensors 12 , 1211–1279 (2012).
Herff, C., Krusienski, D. J. & Kubben, P. The potential of stereotactic-EEG for brain–computer interfaces: current progress and future directions. Front. Neurosci. 14 , 123 (2020).
Angrick, M. et al. Speech synthesis from ECoG using densely connected 3D convolutional neural networks. J. Neural Eng. https://doi.org/10.1088/1741-2552/ab0c59 (2019).
Herff, C. et al. Generating natural, intelligible speech from brain activity in motor, premotor, and inferior frontal cortices. Front. Neurosci. 13 , 1267 (2019).
Kellis, S. et al. Decoding spoken words using local field potentials recorded from the cortical surface. J. Neural Eng. 7 , 056007 (2010).
Makin, J. G., Moses, D. A. & Chang, E. F. Machine translation of cortical activity to text with an encoder–decoder framework. Nat. Neurosci. 23 , 575–582 (2020).
Metzger, S. L. et al. A high-performance neuroprosthesis for speech decoding and avatar control. Nature 620 , 1037–1046 (2023).
Moses, D. A. et al. Neuroprosthesis for decoding speech in a paralyzed person with anarthria. N. Engl. J. Med. 385 , 217–227 (2021).
Guenther, F. H. et al. A wireless brain–machine interface for real-time speech synthesis. PLoS ONE 4 , e8218 (2009).
Stavisky, S. D. et al. Neural ensemble dynamics in dorsal motor cortex during speech in people with paralysis. eLife 8 , e46015 (2019).
Wilson, G. H. et al. Decoding spoken English from intracortical electrode arrays in dorsal precentral gyrus. J. Neural Eng. 17 , 066007 (2020).
Willett, F. R. et al. A high-performance speech neuroprosthesis. Nature 620 , 1031–1036 (2023).
Wandelt, S. K. et al. Decoding grasp and speech signals from the cortical grasp circuit in a tetraplegic human. Neuron https://doi.org/10.1016/j.neuron.2022.03.009 (2022).
Anumanchipalli, G. K., Chartier, J. & Chang, E. F. Speech synthesis from neural decoding of spoken sentences. Nature 568 , 493–498 (2019).
Bocquelet, F., Hueber, T., Girin, L., Savariaux, C. & Yvert, B. Real-time control of an articulatory-based speech synthesizer for brain computer interfaces. PLoS Comput. Biol. 12 , e1005119 (2016).
Metzger, S. L. et al. Generalizable spelling using a speech neuroprosthesis in an individual with severe limb and vocal paralysis. Nat. Commun. 13 , 6510 (2022).
Meng, K. et al. Continuous synthesis of artificial speech sounds from human cortical surface recordings during silent speech production. J. Neural Eng. https://doi.org/10.1088/1741-2552/ace7f6 (2023).
Proix, T. et al. Imagined speech can be decoded from low- and cross-frequency intracranial EEG features. Nat. Commun. 13 , 48 (2022).
Pei, X., Barbour, D. L., Leuthardt, E. C. & Schalk, G. Decoding vowels and consonants in spoken and imagined words using electrocorticographic signals in humans. J. Neural Eng. 8 , 046028 (2011).
Ikeda, S. et al. Neural decoding of single vowels during covert articulation using electrocorticography. Front. Hum. Neurosci. 8 , 125 (2014).
Martin, S. et al. Word pair classification during imagined speech using direct brain recordings. Sci. Rep. 6 , 25803 (2016).
Angrick, M. et al. Real-time synthesis of imagined speech processes from minimally invasive recordings of neural activity. Commun. Biol. 4 , 1055 (2021).
Price, C. J. The anatomy of language: a review of 100 fMRI studies published in 2009. Ann. N. Y. Acad. Sci. 1191 , 62–88 (2010).
PubMed Google Scholar
Langland-Hassan, P. & Vicente, A. Inner Speech: New Voices (Oxford Univ. Press, 2018).
Perrone-Bertolotti, M., Rapin, L., Lachaux, J.-P., Baciu, M. & Lœvenbruck, H. What is that little voice inside my head? Inner speech phenomenology, its role in cognitive performance, and its relation to self-monitoring. Behav. Brain Res. 261 , 220–239 (2014).
CAS PubMed Google Scholar
Pei, X. et al. Spatiotemporal dynamics of electrocorticographic high gamma activity during overt and covert word repetition. NeuroImage 54 , 2960–2972 (2011).
Oberhuber, M. et al. Four functionally distinct regions in the left supramarginal gyrus support word processing. Cereb. Cortex 26 , 4212–4226 (2016).
Binder, J. R. Current controversies on Wernicke’s area and its role in language. Curr. Neurol. Neurosci. Rep. 17 , 58 (2017).
Geva, S. et al. The neural correlates of inner speech defined by voxel-based lesion–symptom mapping. Brain 134 , 3071–3082 (2011).
Cooney, C., Folli, R. & Coyle, D. Opportunities, pitfalls and trade-offs in designing protocols for measuring the neural correlates of speech. Neurosci. Biobehav. Rev. 140 , 104783 (2022).
Dash, D. et al. Interspeech (International Speech Communication Association, 2020).
Alderson-Day, B. & Fernyhough, C. Inner speech: development, cognitive functions, phenomenology, and neurobiology. Psychol. Bull. 141 , 931–965 (2015).
Muret, D., Root, V., Kieliba, P., Clode, D. & Makin, T. R. Beyond body maps: information content of specific body parts is distributed across the somatosensory homunculus. Cell Rep. 38 , 110523 (2022).
Rosenthal, I. A. et al. S1 represents multisensory contexts and somatotopic locations within and outside the bounds of the cortical homunculus. Cell Rep. 42 , 112312 (2023).
Leuthardt, E. et al. Temporal evolution of gamma activity in human cortex during an overt and covert word repetition task. Front. Hum. Neurosci. 6 , 99 (2012).
Indefrey, P. & Levelt, W. J. M. The spatial and temporal signatures of word production components. Cognition 92 , 101–144 (2004).
Alderson-Day, B., Bernini, M. & Fernyhough, C. Uncharted features and dynamics of reading: voices, characters, and crossing of experiences. Conscious. Cogn. 49 , 98–109 (2017).
Armenta Salas, M. et al. Proprioceptive and cutaneous sensations in humans elicited by intracortical microstimulation. eLife 7 , e32904 (2018).
Cooney, C., Folli, R. & Coyle, D. Neurolinguistics research advancing development of a direct-speech brain–computer interface. iScience 8 , 103–125 (2018).
Soroush, P. Z. et al. The nested hierarchy of overt, mouthed, and imagined speech activity evident in intracranial recordings. NeuroImage https://doi.org/10.1016/j.neuroimage.2023.119913 (2023).
Soroush, P. Z. et al. The nested hierarchy of overt, mouthed, and imagined speech activity evident in intracranial recordings. NeuroImage 269 , 119913 (2023).
Alexander, J. D. & Nygaard, L. C. Reading voices and hearing text: talker-specific auditory imagery in reading. J. Exp. Psychol. Hum. Percept. Perform. 34 , 446–459 (2008).
Filik, R. & Barber, E. Inner speech during silent reading reflects the reader’s regional accent. PLoS ONE 6 , e25782 (2011).
Lœvenbruck, H., Baciu, M., Segebarth, C. & Abry, C. The left inferior frontal gyrus under focus: an fMRI study of the production of deixis via syntactic extraction and prosodic focus. J. Neurolinguist. 18 , 237–258 (2005).
Roussel, P. et al. Observation and assessment of acoustic contamination of electrophysiological brain signals during speech production and sound perception. J. Neural Eng. 17 , 056028 (2020).
Aflalo, T. et al. A shared neural substrate for action verbs and observed actions in human posterior parietal cortex. Sci. Adv. 6 , eabb3984 (2020).
Rutishauser, U., Aflalo, T., Rosario, E. R., Pouratian, N. & Andersen, R. A. Single-neuron representation of memory strength and recognition confidence in left human posterior parietal cortex. Neuron 97 , 209–220.e3 (2018).
Brainard, D. H. The psychophysics toolbox. Spat. Vis. 10 , 433–436 (1997).
Pelli, D. G. The VideoToolbox software for visual psychophysics: transforming numbers into movies. Spat. Vis. 10 , 437–442 (1997).
Kleiner, M. et al. What’s new in psychtoolbox-3. Perception 36 , 1–16 (2007).
Benjamini, Y. & Hochberg, Y. Controlling the false discovery rate: a practical and powerful approach to multiple testing. J. R. Stat. Soc. B 57 , 289–300 (1995).
Benjamini, Y. & Yekutieli, D. The control of the false discovery rate in multiple testing under dependency. Ann. Stat. 29 , 1165–1188 (2001).
Kobak, D. et al. Demixed principal component analysis of neural population data. eLife 5 , e10989 (2016).
Kobak, D. dPCA. GitHub https://github.com/machenslab/dPCA (2020).
Wandelt, S. K. Data associated to manuscript “Representation of internal speech by single neurons in human supramarginal gyrus”. Zenodo https://doi.org/10.5281/zenodo.10697024 (2024).
Download references
Acknowledgements
We thank L. Bashford and I. Rosenthal for helpful discussions and data collection. We thank our study participants for their dedication to the study that made this work possible. This research was supported by the NIH National Institute of Neurological Disorders and Stroke Grant U01: U01NS098975 and U01: U01NS123127 (S.K.W., D.A.B., K.P., C.L. and R.A.A.) and by the T&C Chen Brain-Machine Interface Center (S.K.W., D.A.B. and R.A.A.). The funders had no role in study design, data collection and analysis, decision to publish or preparation of the paper.
Author information
Authors and affiliations.
Division of Biology and Biological Engineering, California Institute of Technology, Pasadena, CA, USA
Sarah K. Wandelt, David A. Bjånes, Kelsie Pejsa, Brian Lee, Charles Liu & Richard A. Andersen
T&C Chen Brain-Machine Interface Center, California Institute of Technology, Pasadena, CA, USA
Sarah K. Wandelt, David A. Bjånes, Kelsie Pejsa & Richard A. Andersen
Rancho Los Amigos National Rehabilitation Center, Downey, CA, USA
David A. Bjånes & Charles Liu
Department of Neurological Surgery, Keck School of Medicine of USC, Los Angeles, CA, USA
Brian Lee & Charles Liu
USC Neurorestoration Center, Keck School of Medicine of USC, Los Angeles, CA, USA
You can also search for this author in PubMed Google Scholar
Contributions
S.K.W., D.A.B. and R.A.A. designed the study. S.K.W. and D.A.B. developed the experimental tasks and collected the data. S.K.W. analysed the results and generated the figures. S.K.W., D.A.B. and R.A.A. interpreted the results and wrote the paper. K.P. coordinated regulatory requirements of clinical trials. C.L. and B.L. performed the surgery to implant the recording arrays.
Corresponding author
Correspondence to Sarah K. Wandelt .
Ethics declarations
Competing interests.
The authors declare no competing interests.
Peer review
Peer review information.
Nature Human Behaviour thanks Abbas Babajani-Feremi, Matthew Nelson and Blaise Yvert for their contribution to the peer review of this work. Peer reviewer reports are available.
Additional information
Publisher’s note Springer Nature remains neutral with regard to jurisdictional claims in published maps and institutional affiliations.
Supplementary information
Supplementary information.
Supplementary Figs. 1–5.
Reporting Summary
Peer review file, supplementary video 1.
The video shows the participant performing the internal speech task in real time. The participant is cued with a word on the screen. After a delay, an orange dot appears, during which the participant performs internal speech. Then, the decoded word appears on the screen, in green if it is correctly decoded and in red if it is wrongly decoded.
Supplementary Data
Source data for Fig. 3.
Source data for Fig. 4.
Source data for Fig. 5.
Source Data Fig. 3
Statistical source data.
Source Data Fig. 5
Source data fig. 6, rights and permissions.
Open Access This article is licensed under a Creative Commons Attribution 4.0 International License, which permits use, sharing, adaptation, distribution and reproduction in any medium or format, as long as you give appropriate credit to the original author(s) and the source, provide a link to the Creative Commons licence, and indicate if changes were made. The images or other third party material in this article are included in the article’s Creative Commons licence, unless indicated otherwise in a credit line to the material. If material is not included in the article’s Creative Commons licence and your intended use is not permitted by statutory regulation or exceeds the permitted use, you will need to obtain permission directly from the copyright holder. To view a copy of this licence, visit http://creativecommons.org/licenses/by/4.0/ .
Reprints and permissions
About this article
Cite this article.
Wandelt, S.K., Bjånes, D.A., Pejsa, K. et al. Representation of internal speech by single neurons in human supramarginal gyrus. Nat Hum Behav (2024). https://doi.org/10.1038/s41562-024-01867-y
Download citation
Received : 15 May 2023
Accepted : 16 March 2024
Published : 13 May 2024
DOI : https://doi.org/10.1038/s41562-024-01867-y
Share this article
Anyone you share the following link with will be able to read this content:
Sorry, a shareable link is not currently available for this article.
Provided by the Springer Nature SharedIt content-sharing initiative
This article is cited by
Brain-reading device is best yet at decoding âinternal speechâ.
- Miryam Naddaf
Nature (2024)
Quick links
- Explore articles by subject
- Guide to authors
- Editorial policies
Sign up for the Nature Briefing newsletter — what matters in science, free to your inbox daily.

Synonyms of 'representation' in British English
Additional synonyms, synonyms of 'representation' in american english.
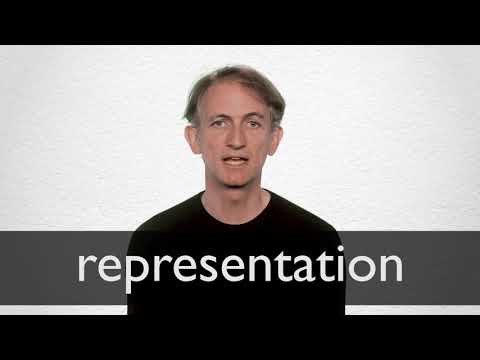
Browse alphabetically representation
- represent someone as something or someone
- represent yourself as something or someone
- representation
- representative
- All ENGLISH synonyms that begin with 'R'
Quick word challenge
Quiz Review
Score: 0 / 5

Wordle Helper

Scrabble Tools
No Taxation Without Representation: the Core of American Revolutionary Ideals and Democratic Governance
This essay about “Taxation without Representation” explores the historical significance and lasting impact of this phrase from the American Revolution. It highlights how the slogan expressed the colonists’ demands for democratic rights and their resistance to British taxation policies. The essay discusses the evolution of this concept into a broader movement for equality and representation in the newly formed United States, influencing various marginalized groups to fight for their rights in the democratic process. It concludes by reflecting on the global relevance of these principles today.
How it works
In the tapestry of history, few refrains reverberate with the same revolutionary resonance as “Taxation without Representation.” These four words encapsulate the very essence of the American Revolutionary ethos, serving as an enduring reminder of the bedrock principle upon which democratic governance stands. To delve into its import is to embark on a journey through the tumultuous yet transformative epoch of American history, where the seeds of democracy were sown amidst impassioned calls for justice and liberty.
The genesis of “Taxation without Representation” traces its roots back to the mid-18th century, a time when the American colonies found themselves increasingly at loggerheads with their British overlords.
For decades, these colonies had thrived under British dominion, but as their populations burgeoned and their economies flourished, so too did their yearning for autonomy and self-determination. However, the British Parliament, buoyed by its triumph in the Seven Years’ War, sought to exert greater control over its North American dominions, including through levying taxes sans colonial representation.
The imposition of taxes such as the Stamp Act of 1765 and the Townshend Acts of 1767 served as tinder for colonial discontent. Colonists, proud inheritors of English heritage, perceived these taxes not only as fiscal burdens but as affronts to their rights as subjects of the British Crown. They contended that, as English subjects, they were entitled to the same liberties and privileges as those residing in Britain, including representation in the legislative bodies that imposed taxes upon them.
It was amidst this maelstrom of unrest that the rallying cry of “Taxation without Representation” resounded. Reverberating in town squares, taverns, and legislative chambers across the colonies, this succinct yet potent phrase encapsulated the colonists’ defiance and their refusal to bow to unjust authority. It emerged as the mantra of a burgeoning movement, uniting colonists from diverse backgrounds and laying the groundwork for a revolution that would irrevocably alter the trajectory of history.
At its core, “Taxation without Representation” embodied more than a mere protest against unfair taxation; it epitomized a fundamental belief in the precepts of democracy and self-governance. The colonists recognized that genuine sovereignty could only be attained through the consent of the governed, and they were prepared to stake all in securing that right. In so doing, they challenged the very underpinnings of monarchical rule and laid the foundation for a novel form of governance—one predicated on the principles of popular sovereignty and democratic representation.
The American Revolution, with its trials, tribulations, and triumphs, constituted a struggle for the soul of a nation. It was a battle to emancipate from the fetters of colonial subjugation and forge a new path grounded in the ideals of liberty, equality, and justice. And at its heart lay the principle of “Taxation without Representation,” a principle that not only shaped the course of the revolution but also left an indelible imprint on the fabric of American democracy.
In the years subsequent to the revolution, the architects of the United States Constitution endeavored to enshrine the principles of “Taxation without Representation” into the very fabric of the nascent nation. The Constitution instituted a system of governance wherein power ultimately vested in the people, with representatives elected to safeguard their interests and uphold their rights. The concept of representation emerged as a cornerstone of American democracy, ensuring that all citizens possessed a voice in the decisions that impacted their lives.
However, while the American Revolution secured political autonomy for the colonies, the quest for genuine representation would persist for generations to come. Women, African Americans, Native Americans, and other marginalized groups would wage their own battles for inclusion, challenging the notion that democracy was the exclusive domain of white, property-owning men. The legacy of “Taxation without Representation” thus transcended the bounds of the revolution, serving as a clarion call for successive generations of activists endeavoring to broaden the horizons of democracy and ensure that all voices were heard.
Today, the principle of “Taxation without Representation” continues to resonate across the globe. It serves as a poignant reminder that democracy entails more than mere governance—it embodies a commitment to equity, justice, and the rule of law. It underscores the truth that genuine sovereignty resides with the people and that governments are accountable to those they serve. And it impels us to uphold these principles in the face of tyranny and injustice, just as our forebears did more than two centuries past.
In summation, “Taxation without Representation” stands as a testament to the enduring potency of democratic ideals and the fortitude of those who labor to uphold them. It serves as a reminder that the struggle for freedom and justice is perpetual, demanding perpetual vigilance and dedication from each successive generation. And it serves as a beacon of hope for all who espouse the transformative potential of ordinary citizens to effect extraordinary change.

Cite this page
No Taxation Without Representation: The Core of American Revolutionary Ideals and Democratic Governance. (2024, May 21). Retrieved from https://papersowl.com/examples/no-taxation-without-representation-the-core-of-american-revolutionary-ideals-and-democratic-governance/
"No Taxation Without Representation: The Core of American Revolutionary Ideals and Democratic Governance." PapersOwl.com , 21 May 2024, https://papersowl.com/examples/no-taxation-without-representation-the-core-of-american-revolutionary-ideals-and-democratic-governance/
PapersOwl.com. (2024). No Taxation Without Representation: The Core of American Revolutionary Ideals and Democratic Governance . [Online]. Available at: https://papersowl.com/examples/no-taxation-without-representation-the-core-of-american-revolutionary-ideals-and-democratic-governance/ [Accessed: 24 May. 2024]
"No Taxation Without Representation: The Core of American Revolutionary Ideals and Democratic Governance." PapersOwl.com, May 21, 2024. Accessed May 24, 2024. https://papersowl.com/examples/no-taxation-without-representation-the-core-of-american-revolutionary-ideals-and-democratic-governance/
"No Taxation Without Representation: The Core of American Revolutionary Ideals and Democratic Governance," PapersOwl.com , 21-May-2024. [Online]. Available: https://papersowl.com/examples/no-taxation-without-representation-the-core-of-american-revolutionary-ideals-and-democratic-governance/. [Accessed: 24-May-2024]
PapersOwl.com. (2024). No Taxation Without Representation: The Core of American Revolutionary Ideals and Democratic Governance . [Online]. Available at: https://papersowl.com/examples/no-taxation-without-representation-the-core-of-american-revolutionary-ideals-and-democratic-governance/ [Accessed: 24-May-2024]
Don't let plagiarism ruin your grade
Hire a writer to get a unique paper crafted to your needs.
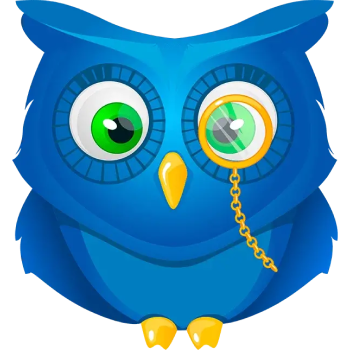
Our writers will help you fix any mistakes and get an A+!
Please check your inbox.
You can order an original essay written according to your instructions.
Trusted by over 1 million students worldwide
1. Tell Us Your Requirements
2. Pick your perfect writer
3. Get Your Paper and Pay
Hi! I'm Amy, your personal assistant!
Don't know where to start? Give me your paper requirements and I connect you to an academic expert.
short deadlines
100% Plagiarism-Free
Certified writers
- Skip to main content
- Keyboard shortcuts for audio player
The humble beginning of the word "president"

Manoush Zomorodi
Fiona Geiran
Sanaz Meshkinpour
TRH: Mark Forsyth
Part 4 of the TED Radio Hour episode The History Behind Three Words
When George Washington took power, the U.S. House and Senate debated tirelessly how to address him. Writer Mark Forsyth explains how and why the U.S. leader is called "president."
About Mark Forsyth
Mark Forsyth is the author of The Elements of Eloquence , The Etymologicon and A Short History of Drunkenness . He is the creator of The Inky Fool , a blog about words, phrases, grammar, rhetoric, and prose.
This segment of the TED Radio Hour was produced by Fiona Geiran and edited by Sanaz Meshkinpour. You can follow us on Facebook @ TEDRadioHour and email us at [email protected].
Web Resources
Related TED Bio: Mark Forsyth
Related TED Video: Does your vote count? The Electoral College explained
Related NPR Links
'Black AF History' examines American history from the perspective of Black people
Throughline: The Contradictions of Abraham Lincoln
Throughline: The 4th Amendment: Search and Seizure

Hawaiian Word of the Week: Kilo
- May 21, 2024
—Stargazer, seer, to observe, to examine.
Previous ʻōlelo Puka Hoʻomaʻemaʻe Mei Kupulau ʻApelila All ʻŌlelo of the Week
“As we journey through school, work, and home, make sure to kilo the things around you. The signs will reveal more than you expect when you, the kilo, allow yourself the time to kilo.”
—Ikaika Mendez, Hawaiian language and music graduate, Ke Kulanui o Hawaiʻi ma Mānoa (University of Hawaiʻi at Mānoa)
For more information on other elements of the definition and usage, go to the UH Hilo Wehewehe Wikiwiki .
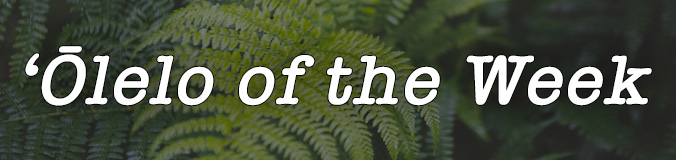
Related Posts:
- Hawaiian Word of the Week: Hoʻomaʻemaʻe
- Hawaiian Word of the Week: Puka
- Hawaiian Word of the Week: Kupulau
- previous post: ConFest 2024: Kānaka Maoli, Asian American theatre take spotlight
- next post: Image of the Week: Shama
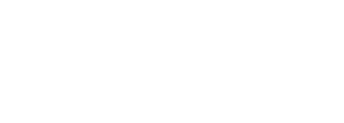
If required, information contained on this website can be made available in an alternative format upon request. Get Adobe Acrobat Reader
About Calendar COVID-19 Updates Directory Emergency Information For Media MyUH Work at UH
Gagana Samoa
Kapasen Chuuk
Kajin Majôl
ʻŌlelo Hawaiʻi
- Administrative
To revisit this article, visit My Profile, then View saved stories .
- What Is Cinema?
- Newsletters
Biden’s Critical Words for the ICC After Calls for Netanyahu Arrest Warrant
By Eric Lutz
Benjamin Netanyahu had been concerned for weeks that the International Criminal Court could seek his arrest over his role in the Israel-Hamas war—so much so, apparently, that he had asked President Joe Biden to intervene on his behalf. On Monday, as the prospect of war crime charges against the Israeli prime minister and members of his cabinet appears to be growing more likely, the president hailed the arrest warrants against Netanyahu and other top Israeli officials as “outrageous.” Biden added that the US will always stand with Israel in threats to its security: “Whatever this prosecutor might imply, there is no equivalence—none—between Israel and Hamas.”
Earlier Monday, ICC prosecutor Karim Khan told CNN’s Christiane Amanpour that the court is seeking arrest warrants for Netanyahu, Israel Defense Minister Yoav Gallant , and Hamas leader Yahya Sinwar over the terror attack on Israel and the US ally’s military response, which has left tens of thousands of Palestinians dead and provoked international criticism. Two other Hamas leaders, Mohammed Diab Ibrahim al-Masri and Ismail Haniyeh , are also facing ICC arrest warrants. “Nobody is above the law,” Khan told Amanpour.
Both Israel and Hamas decried the calls from the ICC. Israel’s president, Isaac Herzog , said the prosecutor’s announcement was “beyond outrageous.” Hamas said in a statement that it “strongly denounces” the potential charges. The warrant requests, now under consideration by an ICC panel, are a striking international rebuke against the Netanyahu regime and comes just days after Benny Gantz —Israel’s war cabinet minister— threatened to resign from his post if Netanyahu does not establish a post-war plan for Gaza by June 8. “If you put the national over personal, you will find in us partners in the struggle,” Gantz said. “But if you choose the path of fanatics and lead the entire nation into the abyss, we will be forced to quit the government.”
But Netanyahu—who is also being urged by US officials to “connect its military operations [in Gaza] to a political strategy“—has remained defiant, dismissing Gantz’s remarks as “washed-up words” and playing hardball with the US, even blocking Israeli military and intelligence officials from meeting with American counterparts at various points in the conflict.
It remains to be seen if the potential ICC arrest warrants could alter Netanyahu’s war effort, which has continued to expand into Rafah —the city Biden had warned would be his “ red line .” But war crime charges would further isolate Netanyahu on the world stage—and put even more pressure on Biden to break more significantly with him.
More Great Stories From Vanity Fair
All the Highlights From the 2024 Cannes Film Festival
Cover Star Ayo Edebiri Is Making Hollywood Her Playground
Why It Took Decades to Bring the Masterpiece One Hundred Years of Solitude to the Screen
Griffin Dunne on the Tragic Death That Reshaped His Family
Hamptonites Are Losing It Over the Congestion Pricing Program
Bibbidi Bobbidi Who Will Disney’s Next CEO Be?
Donald Trump’s Campaign Threatens Legal Action Over Apprentice Movie
The Vatican’s Secret Role in the Science of IVF
Listen to VF ’s Still Watching Podcast Hosts and the Bridgerton Cast Dish on Season 3
By Kase Wickman
By Hillary Busis
By Stephanie Krikorian
Contributing Editor
By signing up you agree to our User Agreement and Privacy Policy & Cookie Statement . This site is protected by reCAPTCHA and the Google Privacy Policy and Terms of Service apply.
- Daily Crossword
- Word Puzzle
- Word Finder
- Word of the Day
- Synonym of the Day
- Word of the Year
- Language stories
- All featured
- Gender and sexuality
- All pop culture
- Writing hub
- Grammar essentials
- Commonly confused
- All writing tips
- Pop culture
- Writing tips
Advertisement
- graphic representation
noun as in alphabet
Strong matches
- fundamentals
- hieroglyphs
Discover More
Example sentences.
This astonishingly simple yet devastatingly graphic representation of mass carnage attracts many thousands of visitors every day.
The line is a graphic representation of the vibrations of the membrane and the waves of sound in the air.
While the scheme of graphic representation is entirely different, the facts represented are the same.
Another consequence of the doctrine of valency was that it permitted the graphic representation of the molecule.
Graphic Representation; typical examples of finding a component, a resultant, or an equilibrant.
The graphic representation, therefore, of the motion of each pendulum would be a line as in fig. 4.
Related Words
Words related to graphic representation are not direct synonyms, but are associated with the word graphic representation . Browse related words to learn more about word associations.
noun as in letters of a writing system
On this page you'll find 14 synonyms, antonyms, and words related to graphic representation, such as: abcs, characters, elements, fundamentals, hieroglyphs, and ideograph.
From Roget's 21st Century Thesaurus, Third Edition Copyright © 2013 by the Philip Lief Group.
- SI SWIMSUIT
- SI SPORTSBOOK
T-Wolves Fans Heckled Draymond Green During TNT Broadcast With Harsh Two-Word Chant
Mike mcdaniel | may 23, 2024.
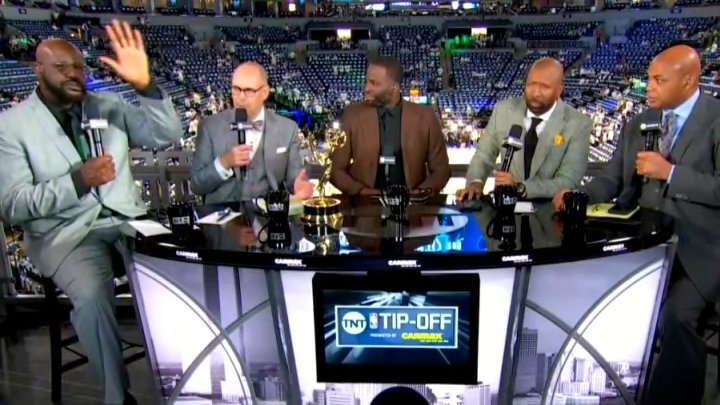
- Minnesota Timberwolves
- Golden State Warriors
There is no love lost between Golden State Warriors star Draymond Green and Minnesota Timberwolves fans.
The angst between the Timberwolves' faithful and Green stems from the Warriors forward escalating an in-game altercation between the two teams earlier this season, one that led to Green putting Timberwolves center Rudy Gobert in a headlock .
Timberwolves fans weren't fond of Green at the time, and they're clearly not fond of him now. As Green joined the set of TNT's Inside the NBA on Wednesday ahead of Game 1 between the Timberwolves and Dallas Mavericks, fans serenaded Green with a simple two-word chant:
"Draymond sucks! Draymond sucks!" the fans yelled as TNT's pre-game show went live.
Timberwolves fans chant "Draymond sucks" ahead of Game 1 of the Western Conference Finals in Minnesota. pic.twitter.com/e7nkxaqIQC — Awful Announcing (@awfulannouncing) May 23, 2024
Green is no stranger to hostility from opposing fan bases, and seemingly took it in stride with a big smile on his face.
The Mavericks took Game 1 on Wednesday, 108-105, to take a 1-0 series lead.
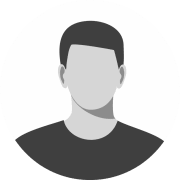
MIKE MCDANIEL
Follow MikeMcDanielSI
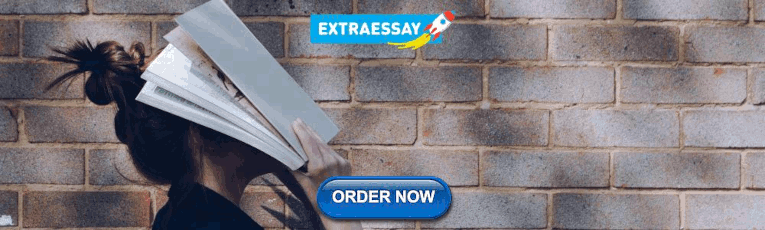
IMAGES
VIDEO
COMMENTS
Find 40 different ways to say REPRESENTATION, along with antonyms, related words, and example sentences at Thesaurus.com.
Words related to representation play a crucial role in shaping our understanding of various concepts, ideas, and experiences. Through language, we are able to express our perspectives, advocate for marginalized communities, and challenge existing power structures.
REPRESENTATION - Synonyms, related words and examples | Cambridge English Thesaurus
Synonyms for REPRESENTATION: depiction, image, portrait, drawing, picture, illustration, photograph, view, resemblance, delineation
The representation of something through art or imagery. The description or portrayal of someone or something in a particular way. A theatrical performance. (politics) The role of a representative in government. ( law) The lawyers and staff who argue on behalf of another in court. ( representations) Formal statements made to an authority.
Most related words/phrases with sentence examples define Representation meaning and usage. Thesaurus for Representation. Related terms for representation- synonyms, antonyms and sentences with representation. Lists. synonyms. antonyms. definitions. sentences. thesaurus. Parts of speech. nouns. verbs. adjectives. Synonyms
Another way to say Representation? Synonyms for Representation (other words and phrases for Representation). Synonyms for Representation. 1 852 other terms for representation- words and phrases with similar meaning. Lists. synonyms. antonyms. definitions. sentences. thesaurus. words. phrases. idioms. Parts of speech. nouns. verbs. adjectives ...
Words Related to Representation Related words are words that are directly connected to each other through their meaning, even if they are not synonyms or antonyms. This connection may be general or specific, or the words may appear frequently together. Related: show; interpretation; representational;
Synonyms for REPRESENTATION: portrayal, account, depiction, description, illustration, image, likeness, model, picture, portrait, …
Below is a massive list of representation words - that is, words related to representation. The top 4 are: image, model, diversity and inclusion. You can get the definition(s) of a word in the list below by tapping the question-mark icon next to it. The words at the top of the list are the ones most associated with representation, and as you go ...
Enter a word, phrase, description, or pattern above to find synonyms, related words, and more. CivicSearch preview: Search U.S. local government meetings . Synonyms and related words for representation from OneLook Thesaurus, a powerful English thesaurus and brainstorming tool that lets you describe what you're looking for in plain terms.
A representation acts or serves on behalf or in place of something. A lawyer provides legal representation for his client. A caricature is an exaggerated representation or likeness of a person. ... Declare your independence and master these words related to the American Revolution. Learn all about the conflict between the colonists and the ...
REPRESENT - Synonyms, related words and examples | Cambridge English Thesaurus
Find 88 different ways to say REPRESENT, along with antonyms, related words, and example sentences at Thesaurus.com.
Synonyms for REPRESENTATIONS: pictures, depictions, portraits, drawings, images, photographs, illustrations, resemblances, likenesses, views
Synonyms for representation in Free Thesaurus. Antonyms for representation. 40 synonyms for representation: body of representatives, committee, embassy, delegates ...
Another way to say Representations? Synonyms for Representations (other words and phrases for Representations).
Plural for the description or portrayal of someone or something in a particular way. (politics) Plural for the role of a representative in government. ( law) Plural for the lawyers and staff who argue on behalf of another in court. ( representations) Plural for formal statements made to an authority. Plural for a visual graph or map, especially ...
representation: [noun] one that represents: such as. an artistic likeness or image. a statement or account made to influence opinion or action. an incidental or collateral statement of fact on the faith of which a contract is entered into. a dramatic production or performance. a usually formal statement made against something or to effect a ...
Words Related to representation. Below is a list of words related to representation. You can click words for definitions. Sorry if there's a few unusual suggestions! The algorithm isn't perfect, but it does a pretty good job for common-ish words. Here's the list of words that are related to representation: image;
Representation for all words was observed in each phase, including pseudowords (bindip and nifzig) (Fig. 3c,f). To identify neurons with selective activity for unique words, we performed a Kruskal ...
Synonyms for REPRESENTATION in English: body of representatives, committee, embassy, delegates, delegation, picture, model, image, portrait, illustration, …
In the tapestry of history, few refrains reverberate with the same revolutionary resonance as "Taxation without Representation." These four words encapsulate the very essence of the American Revolutionary ethos, serving as an enduring reminder of the bedrock principle upon which democratic governance stands.
When George Washington took power, the U.S. House and Senate debated tirelessly how to address him. Writer Mark Forsyth explains how and why the U.S. leader is called "president."
In New York City there are 50 attorneys for every 1,000 residents. That ratio drops to roughly 1.7 attorneys per 1,000 residents in Chautauqua County and 5.3 in Erie County
Kilo. —Stargazer, seer, to observe, to examine. Previous ʻōlelo Puka Hoʻomaʻemaʻe Mei Kupulau ʻApelila All ʻŌlelo of the Week. "As we journey through school, work, and home, make sure to kilo the things around you. The signs will reveal more than you expect when you, the kilo, allow yourself the time to kilo.".
Biden's Critical Words for the ICC After Calls for Netanyahu Arrest Warrant. A prosecutor for the International Criminal Court on Monday said he was seeking charges related to the war in Gaza ...
Find 13 different ways to say GRAPHIC REPRESENTATION, along with antonyms, related words, and example sentences at Thesaurus.com.
As Green joined the set of TNT's Inside the NBA on Wednesday ahead of Game 1 between the Timberwolves and Dallas Mavericks, fans serenaded Green with a simple two-word chant: "Draymond sucks ...