
Want to create or adapt books like this? Learn more about how Pressbooks supports open publishing practices.
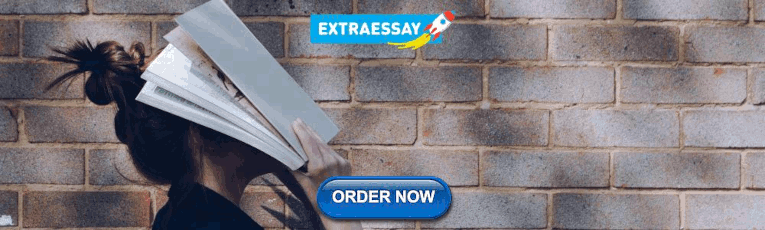
5.1 Understanding Psychological Measurement
Learning objectives.
- Define measurement and give several examples of measurement in psychology.
- Explain what a psychological construct is and give several examples.
- Distinguish conceptual from operational definitions, give examples of each, and create simple operational definitions.
- Distinguish the four levels of measurement, give examples of each, and explain why this distinction is important.
What Is Measurement?
Measurement is the assignment of scores to individuals so that the scores represent some characteristic of the individuals. This very general definition is consistent with the kinds of measurement that everyone is familiar with—for example, weighing oneself by stepping onto a bathroom scale, or checking the internal temperature of a roasting turkey by inserting a meat thermometer. It is also consistent with measurement throughout the sciences. In physics, for example, one might measure the potential energy of an object in Earth’s gravitational field by finding its mass and height (which of course requires measuring those variables) and then multiplying them together along with the gravitational acceleration of Earth (9.8 m/s 2 ). The result of this procedure is a score that represents the object’s potential energy.
Of course this general definition of measurement is consistent with measurement in psychology too. (Psychological measurement is often referred to as psychometrics .) Imagine, for example, that a cognitive psychologist wants to measure a person’s working memory capacity—his or her ability to hold in mind and think about several pieces of information all at the same time. To do this, she might use a backward digit span task, where she reads a list of two digits to the person and asks him or her to repeat them in reverse order. She then repeats this several times, increasing the length of the list by one digit each time, until the person makes an error. The length of the longest list for which the person responds correctly is the score and represents his or her working memory capacity. Or imagine a clinical psychologist who is interested in how depressed a person is. He administers the Beck Depression Inventory, which is a 21-item self-report questionnaire in which the person rates the extent to which he or she has felt sad, lost energy, and experienced other symptoms of depression over the past 2 weeks. The sum of these 21 ratings is the score and represents his or her current level of depression.
The important point here is that measurement does not require any particular instruments or procedures. It does not require placing individuals or objects on bathroom scales, holding rulers up to them, or inserting thermometers into them. What it does require is some systematic procedure for assigning scores to individuals or objects so that those scores represent the characteristic of interest.
Psychological Constructs
Many variables studied by psychologists are straightforward and simple to measure. These include sex, age, height, weight, and birth order. You can almost always tell whether someone is male or female just by looking. You can ask people how old they are and be reasonably sure that they know and will tell you. Although people might not know or want to tell you how much they weigh, you can have them step onto a bathroom scale. Other variables studied by psychologists—perhaps the majority—are not so straightforward or simple to measure. We cannot accurately assess people’s level of intelligence by looking at them, and we certainly cannot put their self-esteem on a bathroom scale. These kinds of variables are called constructs (pronounced CON-structs ) and include personality traits (e.g., extroversion), emotional states (e.g., fear), attitudes (e.g., toward taxes), and abilities (e.g., athleticism).
Psychological constructs cannot be observed directly. One reason is that they often represent tendencies to think, feel, or act in certain ways. For example, to say that a particular college student is highly extroverted (see Note 5.6 “The Big Five” ) does not necessarily mean that she is behaving in an extroverted way right now. In fact, she might be sitting quietly by herself, reading a book. Instead, it means that she has a general tendency to behave in extroverted ways (talking, laughing, etc.) across a variety of situations. Another reason psychological constructs cannot be observed directly is that they often involve internal processes. Fear, for example, involves the activation of certain central and peripheral nervous system structures, along with certain kinds of thoughts, feelings, and behaviors—none of which is necessarily obvious to an outside observer. Notice also that neither extroversion nor fear “reduces to” any particular thought, feeling, act, or physiological structure or process. Instead, each is a kind of summary of a complex set of behaviors and internal processes.
The Big Five
The Big Five is a set of five broad dimensions that capture much of the variation in human personality. Each of the Big Five can even be defined in terms of six more specific constructs called “facets” (Costa & McCrae, 1992).
The conceptual definition of a psychological construct describes the behaviors and internal processes that make up that construct, along with how it relates to other variables. For example, a conceptual definition of neuroticism (another one of the Big Five) would be that it is people’s tendency to experience negative emotions such as anxiety, anger, and sadness across a variety of situations. This definition might also include that it has a strong genetic component, remains fairly stable over time, and is positively correlated with the tendency to experience pain and other physical symptoms.
Students sometimes wonder why, when researchers want to understand a construct like self-esteem or neuroticism, they do not simply look it up in the dictionary. One reason is that many scientific constructs do not have counterparts in everyday language (e.g., working memory capacity). More important, researchers are in the business of developing definitions that are more detailed and precise—and that more accurately describe the way the world is—than the informal definitions in the dictionary. As we will see, they do this by proposing conceptual definitions, testing them empirically, and revising them as necessary. Sometimes they throw them out altogether. This is why the research literature often includes different conceptual definitions of the same construct. In some cases, an older conceptual definition has been replaced by a newer one that works better. In others, researchers are still in the process of deciding which of various conceptual definitions is the best.
Operational Definitions
An operational definition is a definition of a variable in terms of precisely how it is to be measured. These measures generally fall into one of three broad categories. Self-report measures are those in which participants report on their own thoughts, feelings, and actions, as with the Rosenberg Self-Esteem Scale. Behavioral measures are those in which some other aspect of participants’ behavior is observed and recorded. This is an extremely broad category that includes the observation of people’s behavior both in highly structured laboratory tasks and in more natural settings. A good example of the former would be measuring working memory capacity using the backward digit span task. A good example of the latter is a famous operational definition of physical aggression from researcher Albert Bandura and his colleagues (Bandura, Ross, & Ross, 1961). They let each of several children play for 20 minutes in a room that contained a clown-shaped punching bag called a Bobo doll. They filmed each child and counted the number of acts of physical aggression he or she committed. These included hitting the doll with a mallet, punching it, and kicking it. Their operational definition, then, was the number of these specifically defined acts that the child committed in the 20-minute period. Finally, physiological measures are those that involve recording any of a wide variety of physiological processes, including heart rate and blood pressure, galvanic skin response, hormone levels, and electrical activity and blood flow in the brain.

In addition to self-report and behavioral measures, researchers in psychology use physiological measures. An electroencephalograph (EEG) records electrical activity from the brain.
Wikimedia Commons – public domain.
For any given variable or construct, there will be multiple operational definitions. Stress is a good example. A rough conceptual definition is that stress is an adaptive response to a perceived danger or threat that involves physiological, cognitive, affective, and behavioral components. But researchers have operationally defined it in several ways. The Social Readjustment Rating Scale is a self-report questionnaire on which people identify stressful events that they have experienced in the past year and assigns points for each one depending on its severity. For example, a man who has been divorced (73 points), changed jobs (36 points), and had a change in sleeping habits (16 points) in the past year would have a total score of 125. The Daily Hassles and Uplifts Scale is similar but focuses on everyday stressors like misplacing things and being concerned about one’s weight. The Perceived Stress Scale is another self-report measure that focuses on people’s feelings of stress (e.g., “How often have you felt nervous and stressed?”). Researchers have also operationally defined stress in terms of several physiological variables including blood pressure and levels of the stress hormone cortisol.
When psychologists use multiple operational definitions of the same construct—either within a study or across studies—they are using converging operations . The idea is that the various operational definitions are “converging” on the same construct. When scores based on several different operational definitions are closely related to each other and produce similar patterns of results, this constitutes good evidence that the construct is being measured effectively and that it is useful. The various measures of stress, for example, are all correlated with each other and have all been shown to be correlated with other variables such as immune system functioning (also measured in a variety of ways) (Segerstrom & Miller, 2004). This is what allows researchers eventually to draw useful general conclusions, such as “stress is negatively correlated with immune system functioning,” as opposed to more specific and less useful ones, such as “people’s scores on the Perceived Stress Scale are negatively correlated with their white blood counts.”
Levels of Measurement
The psychologist S. S. Stevens suggested that scores can be assigned to individuals so that they communicate more or less quantitative information about the variable of interest (Stevens, 1946). For example, the officials at a 100-m race could simply rank order the runners as they crossed the finish line (first, second, etc.), or they could time each runner to the nearest tenth of a second using a stopwatch (11.5 s, 12.1 s, etc.). In either case, they would be measuring the runners’ times by systematically assigning scores to represent those times. But while the rank ordering procedure communicates the fact that the second-place runner took longer to finish than the first-place finisher, the stopwatch procedure also communicates how much longer the second-place finisher took. Stevens actually suggested four different levels of measurement (which he called “scales of measurement”) that correspond to four different levels of quantitative information that can be communicated by a set of scores.
The nominal level of measurement is used for categorical variables and involves assigning scores that are category labels. Category labels communicate whether any two individuals are the same or different in terms of the variable being measured. For example, if you look at your research participants as they enter the room, decide whether each one is male or female, and type this information into a spreadsheet, you are engaged in nominal-level measurement. Or if you ask your participants to indicate which of several ethnicities they identify themselves with, you are again engaged in nominal-level measurement.
The remaining three levels of measurement are used for quantitative variables. The ordinal level of measurement involves assigning scores so that they represent the rank order of the individuals. Ranks communicate not only whether any two individuals are the same or different in terms of the variable being measured but also whether one individual is higher or lower on that variable. The interval level of measurement involves assigning scores so that they represent the precise magnitude of the difference between individuals, but a score of zero does not actually represent the complete absence of the characteristic. A classic example is the measurement of heat using the Celsius or Fahrenheit scale. The difference between temperatures of 20°C and 25°C is precisely 5°, but a temperature of 0°C does not mean that there is a complete absence of heat. In psychology, the intelligence quotient (IQ) is often considered to be measured at the interval level. Finally, the ratio level of measurement involves assigning scores in such a way that there is a true zero point that represents the complete absence of the quantity. Height measured in meters and weight measured in kilograms are good examples. So are counts of discrete objects or events such as the number of siblings one has or the number of questions a student answers correctly on an exam.
Stevens’s levels of measurement are important for at least two reasons. First, they emphasize the generality of the concept of measurement. Although people do not normally think of categorizing or ranking individuals as measurement, in fact they are as long as they are done so that they represent some characteristic of the individuals. Second, the levels of measurement can serve as a rough guide to the statistical procedures that can be used with the data and the conclusions that can be drawn from them. With nominal-level measurement, for example, the only available measure of central tendency is the mode. Also, ratio-level measurement is the only level that allows meaningful statements about ratios of scores. One cannot say that someone with an IQ of 140 is twice as intelligent as someone with an IQ of 70 because IQ is measured at the interval level, but one can say that someone with six siblings has twice as many as someone with three because number of siblings is measured at the ratio level.
Key Takeaways
- Measurement is the assignment of scores to individuals so that the scores represent some characteristic of the individuals. Psychological measurement can be achieved in a wide variety of ways, including self-report, behavioral, and physiological measures.
- Psychological constructs such as intelligence, self-esteem, and depression are variables that are not directly observable because they represent behavioral tendencies or complex patterns of behavior and internal processes. An important goal of scientific research is to conceptually define psychological constructs in ways that accurately describe them.
- For any conceptual definition of a construct, there will be many different operational definitions or ways of measuring it. The use of multiple operational definitions, or converging operations, is a common strategy in psychological research.
- Variables can be measured at four different levels—nominal, ordinal, interval, and ratio—that communicate increasing amounts of quantitative information. The level of measurement affects the kinds of statistics you can use and conclusions you can draw from your data.
- Practice: Complete the Rosenberg Self-Esteem Scale and compute your overall score.
- Practice: Think of three operational definitions for sexual jealousy, decisiveness, and social anxiety. Consider the possibility of self-report, behavioral, and physiological measures. Be as precise as you can.
Practice: For each of the following variables, decide which level of measurement is being used.
- A college instructor measures the time it takes his students to finish an exam by looking through the stack of exams at the end. He assigns the one on the bottom a score of 1, the one on top of that a 2, and so on.
- A researcher accesses her participants’ medical records and counts the number of times they have seen a doctor in the past year.
- Participants in a research study are asked whether they are right-handed or left-handed.
Bandura, A., Ross, D., & Ross, S. A. (1961). Transmission of aggression through imitation of aggressive models. Journal of Abnormal and Social Psychology, 63 , 575–582.
Costa, P. T., Jr., & McCrae, R. R. (1992). Normal personality assessment in clinical practice: The NEO Personality Inventory. Psychological Assessment, 4 , 5–13.
Segerstrom, S. E., & Miller, G. E. (2004). Psychological stress and the human immune system: A meta-analytic study of 30 years of inquiry. Psychological Bulletin, 130 , 601–630.
Research Methods in Psychology Copyright © 2016 by University of Minnesota is licensed under a Creative Commons Attribution-NonCommercial-ShareAlike 4.0 International License , except where otherwise noted.
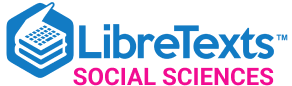
- school Campus Bookshelves
- menu_book Bookshelves
- perm_media Learning Objects
- login Login
- how_to_reg Request Instructor Account
- hub Instructor Commons
Margin Size
- Download Page (PDF)
- Download Full Book (PDF)
- Periodic Table
- Physics Constants
- Scientific Calculator
- Reference & Cite
- Tools expand_more
- Readability
selected template will load here
This action is not available.
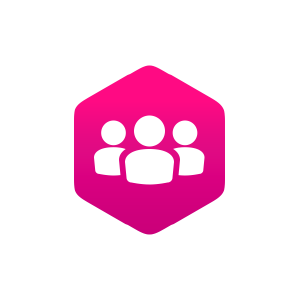
11.2: Operational definitions
- Last updated
- Save as PDF
- Page ID 135144
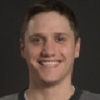
- Matthew DeCarlo, Cory Cummings, & Kate Agnelli
- Open Social Work Education
\( \newcommand{\vecs}[1]{\overset { \scriptstyle \rightharpoonup} {\mathbf{#1}} } \)
\( \newcommand{\vecd}[1]{\overset{-\!-\!\rightharpoonup}{\vphantom{a}\smash {#1}}} \)
\( \newcommand{\id}{\mathrm{id}}\) \( \newcommand{\Span}{\mathrm{span}}\)
( \newcommand{\kernel}{\mathrm{null}\,}\) \( \newcommand{\range}{\mathrm{range}\,}\)
\( \newcommand{\RealPart}{\mathrm{Re}}\) \( \newcommand{\ImaginaryPart}{\mathrm{Im}}\)
\( \newcommand{\Argument}{\mathrm{Arg}}\) \( \newcommand{\norm}[1]{\| #1 \|}\)
\( \newcommand{\inner}[2]{\langle #1, #2 \rangle}\)
\( \newcommand{\Span}{\mathrm{span}}\)
\( \newcommand{\id}{\mathrm{id}}\)
\( \newcommand{\kernel}{\mathrm{null}\,}\)
\( \newcommand{\range}{\mathrm{range}\,}\)
\( \newcommand{\RealPart}{\mathrm{Re}}\)
\( \newcommand{\ImaginaryPart}{\mathrm{Im}}\)
\( \newcommand{\Argument}{\mathrm{Arg}}\)
\( \newcommand{\norm}[1]{\| #1 \|}\)
\( \newcommand{\Span}{\mathrm{span}}\) \( \newcommand{\AA}{\unicode[.8,0]{x212B}}\)
\( \newcommand{\vectorA}[1]{\vec{#1}} % arrow\)
\( \newcommand{\vectorAt}[1]{\vec{\text{#1}}} % arrow\)
\( \newcommand{\vectorB}[1]{\overset { \scriptstyle \rightharpoonup} {\mathbf{#1}} } \)
\( \newcommand{\vectorC}[1]{\textbf{#1}} \)
\( \newcommand{\vectorD}[1]{\overrightarrow{#1}} \)
\( \newcommand{\vectorDt}[1]{\overrightarrow{\text{#1}}} \)
\( \newcommand{\vectE}[1]{\overset{-\!-\!\rightharpoonup}{\vphantom{a}\smash{\mathbf {#1}}}} \)
Learning Objectives
Learners will be able to…
- Define and give an example of indicators and attributes for a variable
- Apply the three components of an operational definition to a variable
- Distinguish between levels of measurement for a variable and how those differences relate to measurement
- Describe the purpose of composite measures like scales and indices
Conceptual definitions are like dictionary definitions. They tell you what a concept means by defining it using other concepts. In this section we will move from the abstract realm (theory) to the real world (measurement). Operationalization is the process by which researchers spell out precisely how a concept will be measured in their study. It involves identifying the specific research procedures we will use to gather data about our concepts. If conceptually defining your terms means looking at theory, how do you operationally define your terms? By looking for indicators of when your variable is present or not, more or less intense, and so forth. Operationalization is probably the most challenging part of quantitative research, but once it’s done, the design and implementation of your study will be straightforward.
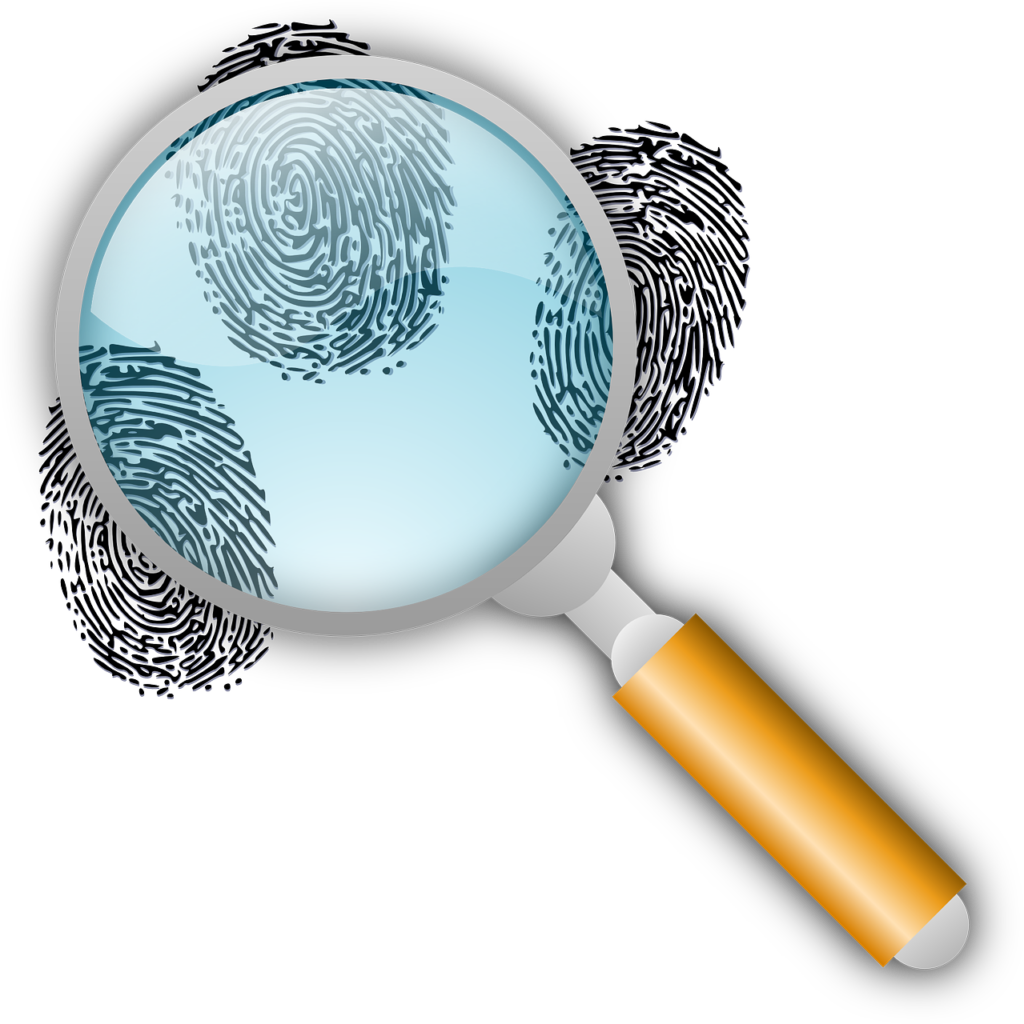
Operationalization works by identifying specific indicators that will be taken to represent the ideas we are interested in studying. If we are interested in studying masculinity, then the indicators for that concept might include some of the social roles prescribed to men in society such as breadwinning or fatherhood. Being a breadwinner or a father might therefore be considered indicators of a person’s masculinity. The extent to which a man fulfills either, or both, of these roles might be understood as clues (or indicators) about the extent to which he is viewed as masculine.
Let’s look at another example of indicators. Each day, Gallup researchers poll 1,000 randomly selected Americans to ask them about their well-being. To measure well-being, Gallup asks these people to respond to questions covering six broad areas: physical health, emotional health, work environment, life evaluation, healthy behaviors, and access to basic necessities. Gallup uses these six factors as indicators of the concept that they are really interested in, which is well-being .
Identifying indicators can be even simpler than the examples described thus far. Political party affiliation is another relatively easy concept for which to identify indicators. If you asked a person what party they voted for in the last national election (or gained access to their voting records), you would get a good indication of their party affiliation. Of course, some voters split tickets between multiple parties when they vote and others swing from party to party each election, so our indicator is not perfect. Indeed, if our study were about political identity as a key concept, operationalizing it solely in terms of who they voted for in the previous election leaves out a lot of information about identity that is relevant to that concept. Nevertheless, it’s a pretty good indicator of political party affiliation.
Choosing indicators is not an arbitrary process. As described earlier, utilizing prior theoretical and empirical work in your area of interest is a great way to identify indicators in a scholarly manner. And you conceptual definitions will point you in the direction of relevant indicators. Empirical work will give you some very specific examples of how the important concepts in an area have been measured in the past and what sorts of indicators have been used. Often, it makes sense to use the same indicators as previous researchers; however, you may find that some previous measures have potential weaknesses that your own study will improve upon.
All of the examples in this chapter have dealt with questions you might ask a research participant on a survey or in a quantitative interview. If you plan to collect data from other sources, such as through direct observation or the analysis of available records, think practically about what the design of your study might look like and how you can collect data on various indicators feasibly. If your study asks about whether the participant regularly changes the oil in their car, you will likely not observe them directly doing so. Instead, you will likely need to rely on a survey question that asks them the frequency with which they change their oil or ask to see their car maintenance records.
- What indicators are commonly used to measure the variables in your research question?
- How can you feasibly collect data on these indicators?
- Are you planning to collect your own data using a questionnaire or interview? Or are you planning to analyze available data like client files or raw data shared from another researcher’s project?
Remember, you need raw data . You research project cannot rely solely on the results reported by other researchers or the arguments you read in the literature. A literature review is only the first part of a research project, and your review of the literature should inform the indicators you end up choosing when you measure the variables in your research question.
Unlike conceptual definitions which contain other concepts, operational definition consists of the following components: (1) the variable being measured and its attributes, (2) the measure you will use, (3) how you plan to interpret the data collected from that measure to draw conclusions about the variable you are measuring.
Step 1: Specifying variables and attributes
The first component, the variable, should be the easiest part. At this point in quantitative research, you should have a research question that has at least one independent and at least one dependent variable. Remember that variables must be able to vary. For example, the United States is not a variable. Country of residence is a variable, as is patriotism. Similarly, if your sample only includes men, gender is a constant in your study, not a variable. A constant is a characteristic that does not change in your study.
When social scientists measure concepts, they sometimes use the language of variables and attributes. A variableno post refers to a quality or quantity that varies across people or situations. Attributes are the characteristics that make up a variable. For example, the variable hair color would contain attributes like blonde, brown, black, red, gray, etc. A variable’s attributes determine its level of measurement. There are four possible levels of measurement: nominal, ordinal, interval, and ratio. The first two levels of measurement are categorical , meaning their attributes are categories rather than numbers. The latter two levels of measurement are continuous , meaning their attributes are numbers.
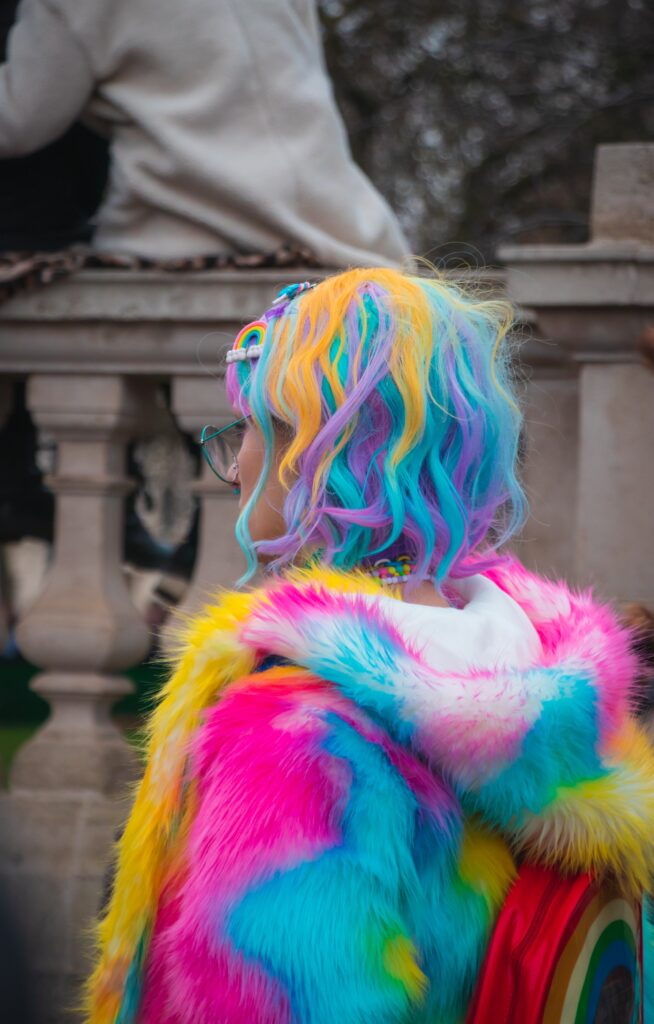
I exist to frustrate researchers’ categorizations.
Levels of measurement
Hair color is an example of a nominal level of measurement. Nominal measures are categorical, and those categories cannot be mathematically ranked. As a brown-haired person (with some gray), I can’t say for sure that brown-haired people are better than blonde-haired people. As with all nominal levels of measurement, there is no ranking order between hair colors; they are simply different. That is what constitutes a nominal level of gender and race are also measured at the nominal level.
What attributes are contained in the variable hair color ? While blonde, brown, black, and red are common colors, some people may not fit into these categories if we only list these attributes. My wife, who currently has purple hair, wouldn’t fit anywhere. This means that our attributes were not exhaustive. Exhaustiveness means that all possible attributes are listed. We may have to list a lot of colors before we can meet the criteria of exhaustiveness. Clearly, there is a point at which exhaustiveness has been reasonably met. If a person insists that their hair color is light burnt sienna , it is not your responsibility to list that as an option. Rather, that person would reasonably be described as brown-haired. Perhaps listing a category for other color would suffice to make our list of colors exhaustive.
What about a person who has multiple hair colors at the same time, such as red and black? They would fall into multiple attributes. This violates the rule of mutual exclusivity , in which a person cannot fall into two different attributes. Instead of listing all of the possible combinations of colors, perhaps you might include a multi-color attribute to describe people with more than one hair color.
Making sure researchers provide mutually exclusive and exhaustive is about making sure all people are represented in the data record. For many years, the attributes for gender were only male or female. Now, our understanding of gender has evolved to encompass more attributes that better reflect the diversity in the world. Children of parents from different races were often classified as one race or another, even if they identified with both cultures. The option for bi-racial or multi-racial on a survey not only more accurately reflects the racial diversity in the real world but validates and acknowledges people who identify in that manner. If we did not measure race in this way, we would leave empty the data record for people who identify as biracial or multiracial, impairing our search for truth.
Unlike nominal-level measures, attributes at the ordinal level can be rank ordered. For example, someone’s degree of satisfaction in their romantic relationship can be ordered by rank. That is, you could say you are not at all satisfied, a little satisfied, moderately satisfied, or highly satisfied. Note that even though these have a rank order to them (not at all satisfied is certainly worse than highly satisfied), we cannot calculate a mathematical distance between those attributes. We can simply say that one attribute of an ordinal-level variable is more or less than another attribute.
This can get a little confusing when using rating scales . If you have ever taken a customer satisfaction survey or completed a course evaluation for school, you are familiar with rating scales. “On a scale of 1-5, with 1 being the lowest and 5 being the highest, how likely are you to recommend our company to other people?” That surely sounds familiar. Rating scales use numbers, but only as a shorthand, to indicate what attribute (highly likely, somewhat likely, etc.) the person feels describes them best. You wouldn’t say you are “2” likely to recommend the company, but you would say you are not very likely to recommend the company. Ordinal-level attributes must also be exhaustive and mutually exclusive, as with nominal-level variables.
At the interval level, attributes must also be exhaustive and mutually exclusive and there is equal distance between attributes. Interval measures are also continuous, meaning their attributes are numbers, rather than categories. IQ scores are interval level, as are temperatures in Fahrenheit and Celsius. Their defining characteristic is that we can say how much more or less one attribute differs from another. We cannot, however, say with certainty what the ratio of one attribute is in comparison to another. For example, it would not make sense to say that a person with an IQ score of 140 has twice the IQ of a person with a score of 70. However, the difference between IQ scores of 80 and 100 is the same as the difference between IQ scores of 120 and 140.
While we cannot say that someone with an IQ of 140 is twice as intelligent as someone with an IQ of 70 because IQ is measured at the interval level, we can say that someone with six siblings has twice as many as someone with three because number of siblings is measured at the ratio level. Finally, at the ratio level, attributes are mutually exclusive and exhaustive, attributes can be rank ordered, the distance between attributes is equal, and attributes have a true zero point. Thus, with these variables, we can say what the ratio of one attribute is in comparison to another. Examples of ratio-level variables include age and years of education. We know that a person who is 12 years old is twice as old as someone who is 6 years old. Height measured in meters and weight measured in kilograms are good examples. So are counts of discrete objects or events such as the number of siblings one has or the number of questions a student answers correctly on an exam. The differences between each level of measurement are visualized in Table 11.1.
Table 11.1 Criteria for Different Levels of Measurement
Levels of measurement=levels of specificity
We have spent time learning how to determine our data’s level of measurement. Now what? How could we use this information to help us as we measure concepts and develop measurement tools? First, the types of statistical tests that we are able to use are dependent on our data’s level of measurement. With nominal-level measurement, for example, the only available measure of central tendency is the mode. With ordinal-level measurement, the median or mode can be used as indicators of central tendency. Interval and ratio-level measurement are typically considered the most desirable because they permit for any indicators of central tendency to be computed (i.e., mean, median, or mode). Also, ratio-level measurement is the only level that allows meaningful statements about ratios of scores. The higher the level of measurement, the more complex statistical tests we are able to conduct. This knowledge may help us decide what kind of data we need to gather, and how.
That said, we have to balance this knowledge with the understanding that sometimes, collecting data at a higher level of measurement could negatively impact our studies. For instance, sometimes providing answers in ranges may make prospective participants feel more comfortable responding to sensitive items. Imagine that you were interested in collecting information on topics such as income, number of sexual partners, number of times someone used illicit drugs, etc. You would have to think about the sensitivity of these items and determine if it would make more sense to collect some data at a lower level of measurement (e.g., asking if they are sexually active or not (nominal) versus their total number of sexual partners (ratio).
Finally, sometimes when analyzing data, researchers find a need to change a data’s level of measurement. For example, a few years ago, a student was interested in studying the relationship between mental health and life satisfaction. This student used a variety of measures. One item asked about the number of mental health symptoms, reported as the actual number. When analyzing data, my student examined the mental health symptom variable and noticed that she had two groups, those with none or one symptoms and those with many symptoms. Instead of using the ratio level data (actual number of mental health symptoms), she collapsed her cases into two categories, few and many. She decided to use this variable in her analyses. It is important to note that you can move a higher level of data to a lower level of data; however, you are unable to move a lower level to a higher level.
- Check that the variables in your research question can vary…and that they are not constants or one of many potential attributes of a variable.
- Think about the attributes your variables have. Are they categorical or continuous? What level of measurement seems most appropriate?
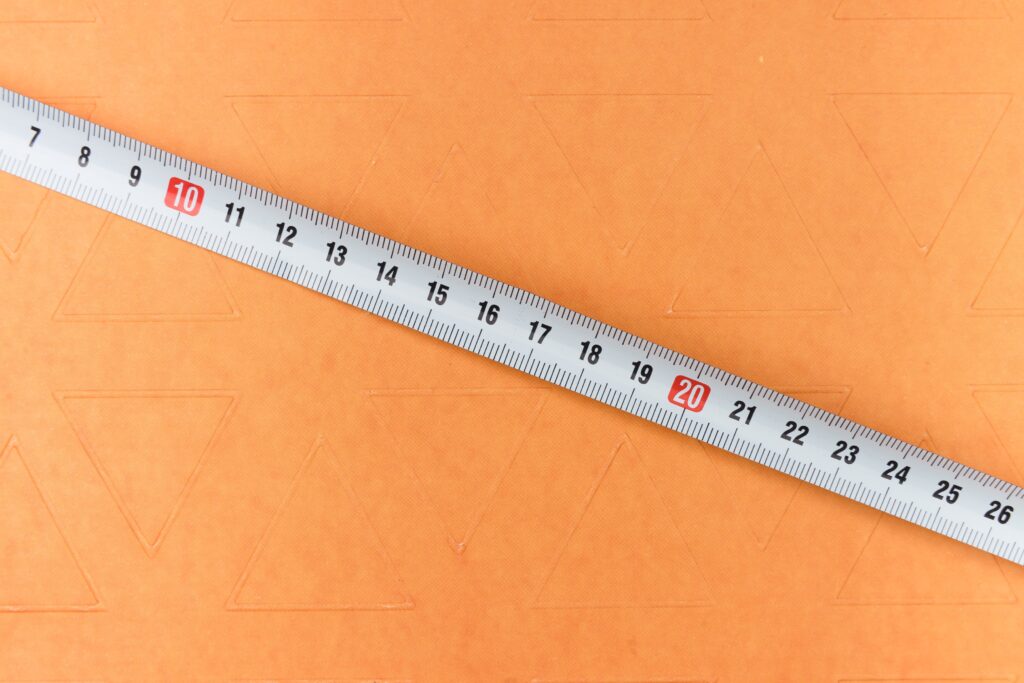
Step 2: Specifying measures for each variable
Let’s pick a social work research question and walk through the process of operationalizing variables to see how specific we need to get. I’m going to hypothesize that residents of a psychiatric unit who are more depressed are less likely to be satisfied with care. Remember, this would be a inverse relationship—as depression increases, satisfaction decreases. In this question, depression is my independent variable (the cause) and satisfaction with care is my dependent variable (the effect). Now we have identified our variables, their attributes, and levels of measurement, we move onto the second component: the measure itself.
So, how would you measure my key variables: depression and satisfaction? What indicators would you look for? Some students might say that depression could be measured by observing a participant’s body language. They may also say that a depressed person will often express feelings of sadness or hopelessness. In addition, a satisfied person might be happy around service providers and often express gratitude. While these factors may indicate that the variables are present, they lack coherence. Unfortunately, what this “measure” is actually saying is that “I know depression and satisfaction when I see them.” While you are likely a decent judge of depression and satisfaction, you need to provide more information in a research study for how you plan to measure your variables. Your judgment is subjective, based on your own idiosyncratic experiences with depression and satisfaction. They couldn’t be replicated by another researcher. They also can’t be done consistently for a large group of people. Operationalization requires that you come up with a specific and rigorous measure for seeing who is depressed or satisfied.
Finding a good measure for your variable depends on the kind of variable it is. Variables that are directly observable don’t come up very often in my students’ classroom projects, but they might include things like taking someone’s blood pressure, marking attendance or participation in a group, and so forth. To measure an indirectly observable variable like age, you would probably put a question on a survey that asked, “How old are you?” Measuring a variable like income might require some more thought, though. Are you interested in this person’s individual income or the income of their family unit? This might matter if your participant does not work or is dependent on other family members for income. Do you count income from social welfare programs? Are you interested in their income per month or per year? Even though indirect observables are relatively easy to measure, the measures you use must be clear in what they are asking, and operationalization is all about figuring out the specifics of what you want to know. For more complicated constructs, you will need compound measures (that use multiple indicators to measure a single variable).
How you plan to collect your data also influences how you will measure your variables. For social work researchers using secondary data like client records as a data source, you are limited by what information is in the data sources you can access. If your organization uses a given measurement for a mental health outcome, that is the one you will use in your study. Similarly, if you plan to study how long a client was housed after an intervention using client visit records, you are limited by how their caseworker recorded their housing status in the chart. One of the benefits of collecting your own data is being able to select the measures you feel best exemplify your understanding of the topic.
Measuring unidimensional concepts
The previous section mentioned two important considerations: how complicated the variable is and how you plan to collect your data. With these in hand, we can use the level of measurement to further specify how you will measure your variables and consider specialized rating scales developed by social science researchers.
Measurement at each level
Nominal measures assess categorical variables. These measures are used for variables or indicators that have mutually exclusive attributes, but that cannot be rank-ordered. Nominal measures ask about the variable and provide names or labels for different attribute values like social work, counseling, and nursing for the variable profession. Nominal measures are relatively straightforward.
Ordinal measures often use a rating scale. It is an ordered set of responses that participants must choose from. Figure 11.1 shows several examples. The number of response options on a typical rating scale is usualy five or seven, though it can range from three to 11. Five-point scales are best for unipolar scales where only one construct is tested, such as frequency (Never, Rarely, Sometimes, Often, Always). Seven-point scales are best for bipolar scales where there is a dichotomous spectrum, such as liking (Like very much, Like somewhat, Like slightly, Neither like nor dislike, Dislike slightly, Dislike somewhat, Dislike very much). For bipolar questions, it is useful to offer an earlier question that branches them into an area of the scale; if asking about liking ice cream, first ask “Do you generally like or dislike ice cream?” Once the respondent chooses like or dislike, refine it by offering them relevant choices from the seven-point scale. Branching improves both reliability and validity (Krosnick & Berent, 1993). [9] Although you often see scales with numerical labels, it is best to only present verbal labels to the respondents but convert them to numerical values in the analyses. Avoid partial labels or length or overly specific labels. In some cases, the verbal labels can be supplemented with (or even replaced by) meaningful graphics. The last rating scale shown in Figure 11.1 is a visual-analog scale, on which participants make a mark somewhere along the horizontal line to indicate the magnitude of their response.
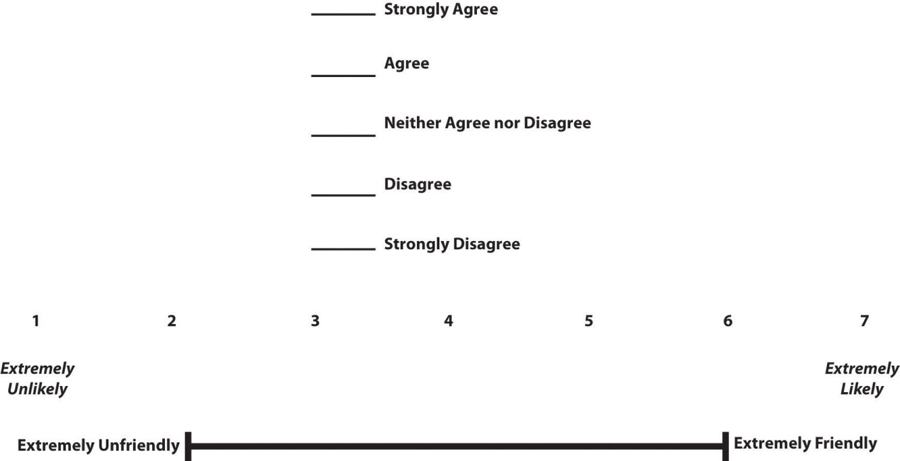
Figure 11.1 Example rating scales for closed-ended questionnaire items
Interval measures are those where the values measured are not only rank-ordered, but are also equidistant from adjacent attributes. For example, the temperature scale (in Fahrenheit or Celsius), where the difference between 30 and 40 degree Fahrenheit is the same as that between 80 and 90 degree Fahrenheit. Likewise, if you have a scale that asks respondents’ annual income using the following attributes (ranges): $0 to 10,000, $10,000 to 20,000, $20,000 to 30,000, and so forth, this is also an interval measure, because the mid-point of each range (i.e., $5,000, $15,000, $25,000, etc.) are equidistant from each other. The intelligence quotient (IQ) scale is also an interval measure, because the measure is designed such that the difference between IQ scores 100 and 110 is supposed to be the same as between 110 and 120 (although we do not really know whether that is truly the case). Interval measures allow us to examine “how much more” is one attribute when compared to another, which is not possible with nominal or ordinal measures. You may find researchers who “pretend” (incorrectly) that ordinal rating scales are actually interval measures so that we can use different statistical techniques for analyzing them. As we will discuss in the latter part of the chapter, this is a mistake because there is no way to know whether the difference between a 3 and a 4 on a rating scale is the same as the difference between a 2 and a 3. Those numbers are just placeholders for categories.
Ratio measures are those that have all the qualities of nominal, ordinal, and interval scales, and in addition, also have a “true zero” point (where the value zero implies lack or non-availability of the underlying construct). Think about how to measure the number of people working in human resources at a social work agency. It could be one, several, or none (if the company contracts out for those services). Measuring interval and ratio data is relatively easy, as people either select or input a number for their answer. If you ask a person how many eggs they purchased last week, they can simply tell you they purchased `a dozen eggs at the store, two at breakfast on Wednesday, or none at all.
Commonly used rating scales in questionnaires
The level of measurement will give you the basic information you need, but social scientists have developed specialized instruments for use in questionnaires, a common tool used in quantitative research. As we mentioned before, if you plan to source your data from client files or previously published results
Although Likert scale is a term colloquially used to refer to almost any rating scale (e.g., a 0-to-10 life satisfaction scale), it has a much more precise meaning. In the 1930s, researcher Rensis Likert (pronounced LICK-ert) created a new approach for measuring people’s attitudes (Likert, 1932). [10] It involves presenting people with several statements—including both favorable and unfavorable statements—about some person, group, or idea. Respondents then express their agreement or disagreement with each statement on a 5-point scale: Strongly Agree, Agree, Neither Agree nor Disagree, Disagree, Strongly Disagree . Numbers are assigned to each response and then summed across all items to produce a score representing the attitude toward the person, group, or idea. For items that are phrased in an opposite direction (e.g., negatively worded statements instead of positively worded statements), reverse coding is used so that the numerical scoring of statements also runs in the opposite direction. The entire set of items came to be called a Likert scale, as indicated in Table 11.2 below.
Unless you are measuring people’s attitude toward something by assessing their level of agreement with several statements about it, it is best to avoid calling it a Likert scale. You are probably just using a rating scale. Likert scales allow for more granularity (more finely tuned response) than yes/no items, including whether respondents are neutral to the statement. Below is an example of how we might use a Likert scale to assess your attitudes about research as you work your way through this textbook.
Table 11.2 Likert scale
Semantic differential scales are composite (multi-item) scales in which respondents are asked to indicate their opinions or feelings toward a single statement using different pairs of adjectives framed as polar opposites. Whereas in the above Likert scale, the participant is asked how much they agree or disagree with a statement, in a semantic differential scale the participant is asked to indicate how they feel about a specific item. This makes the semantic differential scale an excellent technique for measuring people’s attitudes or feelings toward objects, events, or behaviors. Table 11.3 is an example of a semantic differential scale that was created to assess participants’ feelings about this textbook.
Table 11.3. A semantic differential scale for measuring attitudes towards a textbook
Notice that on a Likert scale, each item is different but the choices for the scale are the same (e.g., strongly agree, agree, etc.). However, for a semantic differential scale, the thing that you are reviewing, in this case, beliefs about research content, remains the same. It is the choices that change. Semantic differential is believed to be an excellent technique for measuring people’s attitude or feelings toward objects, events, or behaviors.
This composite scale was designed by Louis Guttman and uses a series of items arranged in increasing order of intensity (least intense to most intense) of the concept. This type of scale allows us to understand the intensity of beliefs or feelings. Each item in the above Guttman scale has a weight (this is not indicated on the tool) which varies with the intensity of that item, and the weighted combination of each response is used as an aggregate measure of an observation.
Notice how the items move from lower intensity to higher intensity. A researcher reviews the yes answers and creates a score for each participant.
For more complicated measures, researchers use scales and indices (sometimes called indexes) to measure their variables because they assess multiple indicators to develop a composite (or total) score. Composite scores provide a much greater understanding of concepts than a single item could. Although we won’t delve too deeply into the process of scale development, we will cover some important topics for you to understand how scales and indices developed by other researchers can be used in your project.
Although they exhibit differences (which will later be discussed) the two have in common various factors.
- Both are ordinal measures of variables.
- Both can order the units of analysis in terms of specific variables.
- Both are composite measures .
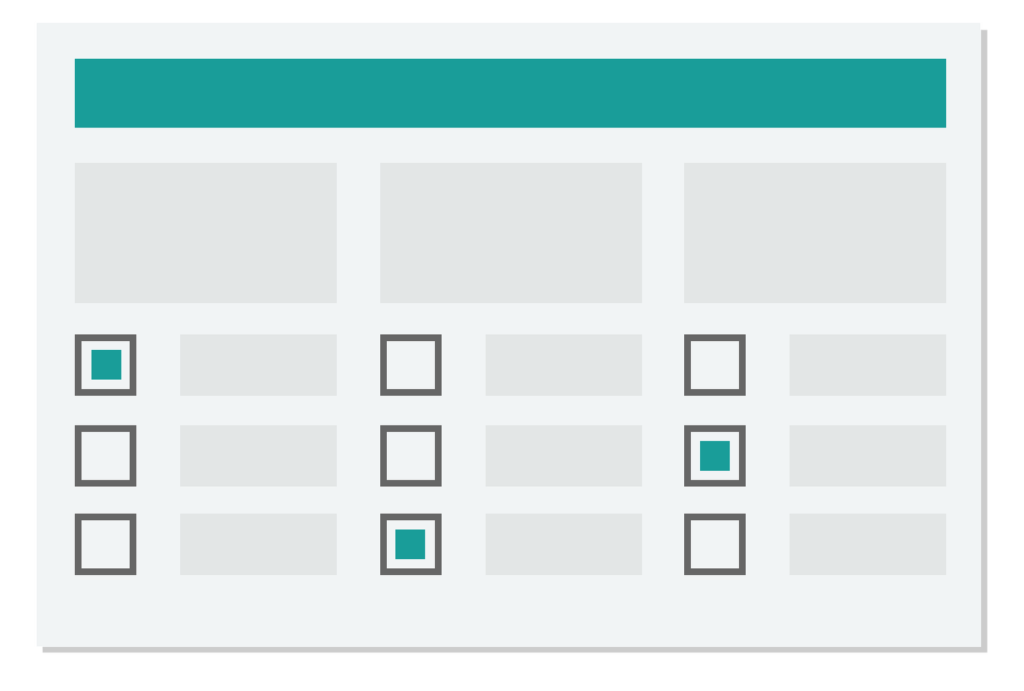
The previous section discussed how to measure respondents’ responses to predesigned items or indicators belonging to an underlying construct. But how do we create the indicators themselves? The process of creating the indicators is called scaling. More formally, scaling is a branch of measurement that involves the construction of measures by associating qualitative judgments about unobservable constructs with quantitative, measurable metric units. Stevens (1946)\(^{11}\) said, “Scaling is the assignment of objects to numbers according to a rule.” This process of measuring abstract concepts in concrete terms remains one of the most difficult tasks in empirical social science research.
The outcome of a scaling process is a scale , which is an empirical structure for measuring items or indicators of a given construct. Understand that multidimensional “scales”, as discussed in this section, are a little different from “rating scales” discussed in the previous section. A rating scale is used to capture the respondents’ reactions to a given item on a questionnaire. For example, an ordinally scaled item captures a value between “strongly disagree” to “strongly agree.” Attaching a rating scale to a statement or instrument is not scaling. Rather, scaling is the formal process of developing scale items, before rating scales can be attached to those items.
If creating your own scale sounds painful, don’t worry! For most multidimensional variables, you would likely be duplicating work that has already been done by other researchers. Specifically, this is a branch of science called psychometrics. You do not need to create a scale for depression because scales such as the Patient Health Questionnaire (PHQ-9), the Center for Epidemiologic Studies Depression Scale (CES-D), and Beck’s Depression Inventory (BDI) have been developed and refined over dozens of years to measure variables like depression. Similarly, scales such as the Patient Satisfaction Questionnaire (PSQ-18) have been developed to measure satisfaction with medical care. As we will discuss in the next section, these scales have been shown to be reliable and valid. While you could create a new scale to measure depression or satisfaction, a study with rigor would pilot test and refine that new scale over time to make sure it measures the concept accurately and consistently. This high level of rigor is often unachievable in student research projects because of the cost and time involved in pilot testing and validating, so using existing scales is recommended.
Unfortunately, there is no good one-stop=shop for psychometric scales. The Mental Measurements Yearbook provides a searchable database of measures for social science variables, though it woefully incomplete and often does not contain the full documentation for scales in its database. You can access it from a university library’s list of databases. If you can’t find anything in there, your next stop should be the methods section of the articles in your literature review. The methods section of each article will detail how the researchers measured their variables, and often the results section is instructive for understanding more about measures. In a quantitative study, researchers may have used a scale to measure key variables and will provide a brief description of that scale, its names, and maybe a few example questions. If you need more information, look at the results section and tables discussing the scale to get a better idea of how the measure works. Looking beyond the articles in your literature review, searching Google Scholar using queries like “depression scale” or “satisfaction scale” should also provide some relevant results. For example, searching for documentation for the Rosenberg Self-Esteem Scale (which we will discuss in the next section), I found this report from researchers investigating acceptance and commitment therapy which details this scale and many others used to assess mental health outcomes. If you find the name of the scale somewhere but cannot find the documentation (all questions and answers plus how to interpret the scale), a general web search with the name of the scale and “.pdf” may bring you to what you need. Or, to get professional help with finding information, always ask a librarian!
Unfortunately, these approaches do not guarantee that you will be able to view the scale itself or get information on how it is interpreted. Many scales cost money to use and may require training to properly administer. You may also find scales that are related to your variable but would need to be slightly modified to match your study’s needs. You could adapt a scale to fit your study, however changing even small parts of a scale can influence its accuracy and consistency. While it is perfectly acceptable in student projects to adapt a scale without testing it first (time may not allow you to do so), pilot testing is always recommended for adapted scales, and researchers seeking to draw valid conclusions and publish their results must take this additional step.
An index is a composite score derived from aggregating measures of multiple concepts (called components) using a set of rules and formulas. It is different from a scale. Scales also aggregate measures; however, these measures examine different dimensions or the same dimension of a single construct. A well-known example of an index is the consumer price index (CPI), which is computed every month by the Bureau of Labor Statistics of the U.S. Department of Labor. The CPI is a measure of how much consumers have to pay for goods and services (in general) and is divided into eight major categories (food and beverages, housing, apparel, transportation, healthcare, recreation, education and communication, and “other goods and services”), which are further subdivided into more than 200 smaller items. Each month, government employees call all over the country to get the current prices of more than 80,000 items. Using a complicated weighting scheme that takes into account the location and probability of purchase for each item, analysts then combine these prices into an overall index score using a series of formulas and rules.
Another example of an index is the Duncan Socioeconomic Index (SEI). This index is used to quantify a person’s socioeconomic status (SES) and is a combination of three concepts: income, education, and occupation. Income is measured in dollars, education in years or degrees achieved, and occupation is classified into categories or levels by status. These very different measures are combined to create an overall SES index score. However, SES index measurement has generated a lot of controversy and disagreement among researchers.
The process of creating an index is similar to that of a scale. First, conceptualize (define) the index and its constituent components. Though this appears simple, there may be a lot of disagreement on what components (concepts/constructs) should be included or excluded from an index. For instance, in the SES index, isn’t income correlated with education and occupation? And if so, should we include one component only or all three components? Reviewing the literature, using theories, and/or interviewing experts or key stakeholders may help resolve this issue. Second, operationalize and measure each component. For instance, how will you categorize occupations, particularly since some occupations may have changed with time (e.g., there were no Web developers before the Internet)? As we will see in step three below, researchers must create a rule or formula for calculating the index score. Again, this process may involve a lot of subjectivity, so validating the index score using existing or new data is important.
Scale and index development at often taught in their own course in doctoral education, so it is unreasonable for you to expect to develop a consistently accurate measure within the span of a week or two. Using available indices and scales is recommended for this reason.
Differences between scales and indices
Though indices and scales yield a single numerical score or value representing a concept of interest, they are different in many ways. First, indices often comprise components that are very different from each other (e.g., income, education, and occupation in the SES index) and are measured in different ways. Conversely, scales typically involve a set of similar items that use the same rating scale (such as a five-point Likert scale about customer satisfaction).
Second, indices often combine objectively measurable values such as prices or income, while scales are designed to assess subjective or judgmental constructs such as attitude, prejudice, or self-esteem. Some argue that the sophistication of the scaling methodology makes scales different from indexes, while others suggest that indexing methodology can be equally sophisticated. Nevertheless, indexes and scales are both essential tools in social science research.
Scales and indices seem like clean, convenient ways to measure different phenomena in social science, but just like with a lot of research, we have to be mindful of the assumptions and biases underneath. What if a scale or an index was developed using only White women as research participants? Is it going to be useful for other groups? It very well might be, but when using a scale or index on a group for whom it hasn’t been tested, it will be very important to evaluate the validity and reliability of the instrument, which we address in the rest of the chapter.
Finally, it’s important to note that while scales and indices are often made up of nominal or ordinal variables, when we analyze them into composite scores, we will treat them as interval/ratio variables.
- Look back to your work from the previous section, are your variables unidimensional or multidimensional?
- Describe the specific measures you will use (actual questions and response options you will use with participants) for each variable in your research question.
- If you are using a measure developed by another researcher but do not have all of the questions, response options, and instructions needed to implement it, put it on your to-do list to get them.
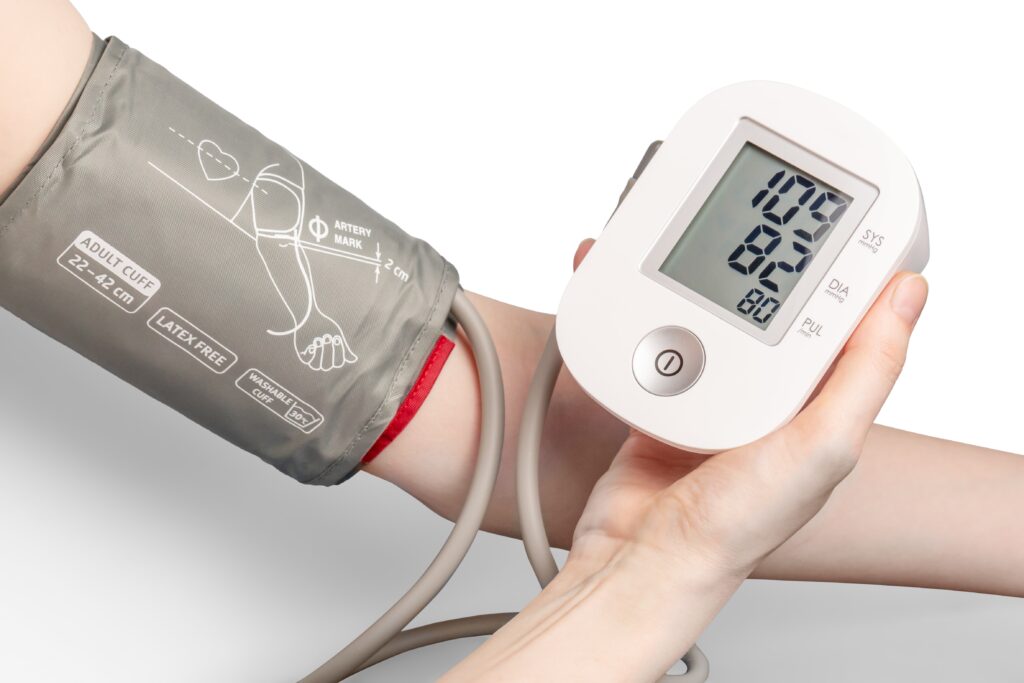
If we were operationalizing blood pressure, the cuff and reader would be the measure…but how do we interpret what is high, low, and normal blood pressure?
Step 3: How you will interpret your measures
The final stage of operationalization involves setting the rules for how the measure works and how the researcher should interpret the results. Sometimes, interpreting a measure can be incredibly easy. If you ask someone their age, you’ll probably interpret the results by noting the raw number (e.g., 22) someone provides and that it is lower or higher than other people’s ages. However, you could also recode that person into age categories (e.g., under 25, 20-29-years-old, generation Z, etc.). Even scales may be simple to interpret. If there is a scale of problem behaviors, one might simply add up the number of behaviors checked off–with a range from 1-5 indicating low risk of delinquent behavior, 6-10 indicating the student is moderate risk, etc. How you choose to interpret your measures should be guided by how they were designed, how you conceptualize your variables, the data sources you used, and your plan for analyzing your data statistically. Whatever measure you use, you need a set of rules for how to take any valid answer a respondent provides to your measure and interpret it in terms of the variable being measured.
For more complicated measures like scales, refer to the information provided by the author for how to interpret the scale. If you can’t find enough information from the scale’s creator, look at how the results of that scale are reported in the results section of research articles. For example, Beck’s Depression Inventory (BDI-II) uses 21 statements to measure depression and respondents rate their level of agreement on a scale of 0-3. The results for each question are added up, and the respondent is put into one of three categories: low levels of depression (1-16), moderate levels of depression (17-30), or severe levels of depression (31 and over).
One common mistake I see often is that students will introduce another variable into their operational definition. This is incorrect. Your operational definition should mention only one variable—the variable being defined. While your study will certainly draw conclusions about the relationships between variables, that’s not what operationalization is. Operationalization specifies what instrument you will use to measure your variable and how you plan to interpret the data collected using that measure.
Operationalization is probably the trickiest component of basic research methods, so please don’t get frustrated if it takes a few drafts and a lot of feedback to get to a workable definition. At the time of this writing, I am in the process of operationalizing the concept of “attitudes towards research methods.” Originally, I thought that I could gauge students’ attitudes toward research methods by looking at their end-of-semester course evaluations. As I became aware of the potential methodological issues with student course evaluations, I opted to use focus groups of students to measure their common beliefs about research. You may recall some of these opinions from Chapter 1 , such as the common beliefs that research is boring, useless, and too difficult. After the focus group, I created a scale based on the opinions I gathered, and I plan to pilot test it with another group of students. After the pilot test, I expect that I will have to revise the scale again before I can implement the measure in a real social work research project. At the time I’m writing this, I’m still not completely done operationalizing this concept.
Key Takeaways
- Operationalization involves spelling out precisely how a concept will be measured.
- Operational definitions must include the variable, the measure, and how you plan to interpret the measure.
- There are four different levels of measurement: nominal, ordinal, interval, and ratio (in increasing order of specificity).
- Scales and indices are common ways to collect information and involve using multiple indicators in measurement.
- A key difference between a scale and an index is that a scale contains multiple indicators for one concept, whereas an indicator examines multiple concepts (components).
- Using scales developed and refined by other researchers can improve the rigor of a quantitative study.
Use the research question that you developed in the previous chapters and find a related scale or index that researchers have used. If you have trouble finding the exact phenomenon you want to study, get as close as you can.
- What is the level of measurement for each item on each tool? Take a second and think about why the tool’s creator decided to include these levels of measurement. Identify any levels of measurement you would change and why.
- If these tools don’t exist for what you are interested in studying, why do you think that is?
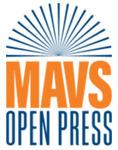
Want to create or adapt books like this? Learn more about how Pressbooks supports open publishing practices.
10.3 Operational definitions
Learning objectives.
Learners will be able to…
- Define and give an example of indicators and attributes for a variable
- Apply the three components of an operational definition to a variable
- Distinguish between levels of measurement for a variable and how those differences relate to measurement
- Describe the purpose of composite measures like scales and indices
Conceptual definitions are like dictionary definitions. They tell you what a concept means by defining it using other concepts. Operationalization occurs after conceptualization and is the process by which researchers spell out precisely how a concept will be measured in their study. It involves identifying the specific research procedures we will use to gather data about our concepts. It entails identifying indicators that can identify when your variable is present or not, the magnitude of the variable, and so forth.
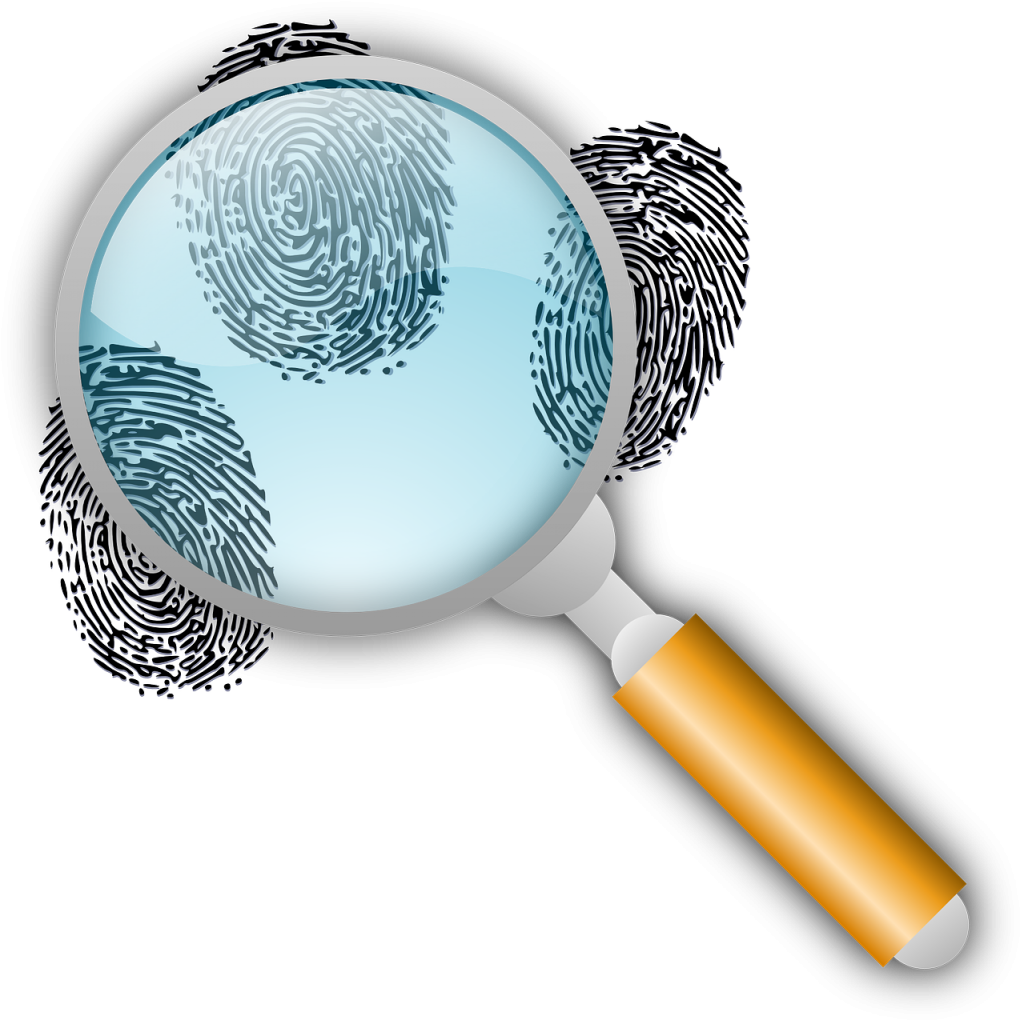
Operationalization works by identifying specific indicators that will be taken to represent the ideas we are interested in studying. Let’s look at an example. Each day, Gallup researchers poll 1,000 randomly selected Americans to ask them about their well-being. To measure well-being, Gallup asks these people to respond to questions covering six broad areas: physical health, emotional health, work environment, life evaluation, healthy behaviors, and access to basic necessities. Gallup uses these six factors as indicators of the concept that they are really interested in, which is well-being .
Identifying indicators can be even simpler than this example. Political party affiliation is another relatively easy concept for which to identify indicators. If you asked a person what party they voted for in the last national election (or gained access to their voting records), you would get a good indication of their party affiliation. Of course, some voters split tickets between multiple parties when they vote and others swing from party to party each election, so our indicator is not perfect. Indeed, if our study were about political identity as a key concept, operationalizing it solely in terms of who they voted for in the previous election leaves out a lot of information about identity that is relevant to that concept. Nevertheless, it’s a pretty good indicator of political party affiliation.
Choosing indicators is not an arbitrary process. Your conceptual definitions point you in the direction of relevant indicators and then you can identify appropriate indicators in a scholarly manner using theory and empirical evidence. Specifically, empirical work will give you some examples of how the important concepts in an area have been measured in the past and what sorts of indicators have been used. Often, it makes sense to use the same indicators as previous researchers; however, you may find that some previous measures have potential weaknesses that your own study may improve upon.
So far in this section, all of the examples of indicators deal with questions you might ask a research participant on a questionnaire for survey research. If you plan to collect data from other sources, such as through direct observation or the analysis of available records, think practically about what the design of your study might look like and how you can collect data on various indicators feasibly. If your study asks about whether participants regularly change the oil in their car, you will likely not observe them directly doing so. Instead, you would rely on a survey question that asks them the frequency with which they change their oil or ask to see their car maintenance records.
TRACK 1 (IF YOU ARE CREATING A RESEARCH PROPOSAL FOR THIS CLASS):
What indicators are commonly used to measure the variables in your research question?
- How can you feasibly collect data on these indicators?
- Are you planning to collect your own data using a questionnaire or interview? Or are you planning to analyze available data like client files or raw data shared from another researcher’s project?
Remember, you need raw data . Your research project cannot rely solely on the results reported by other researchers or the arguments you read in the literature. A literature review is only the first part of a research project, and your review of the literature should inform the indicators you end up choosing when you measure the variables in your research question.
TRACK 2 (IF YOU AREN’T CREATING A RESEARCH PROPOSAL FOR THIS CLASS):
You are interested in studying older adults’ social-emotional well-being. Specifically, you would like to research the impact on levels of older adult loneliness of an intervention that pairs older adults living in assisted living communities with university student volunteers for a weekly conversation.
- How could you feasibly collect data on these indicators?
- Would you collect your own data using a questionnaire or interview? Or would you analyze available data like client files or raw data shared from another researcher’s project?
Steps in the Operationalization Process
Unlike conceptual definitions which contain other concepts, operational definition consists of the following components: (1) the variable being measured and its attributes, (2) the measure you will use, and (3) how you plan to interpret the data collected from that measure to draw conclusions about the variable you are measuring.
Step 1 of Operationalization: Specify variables and attributes
The first component, the variable, should be the easiest part. At this point in quantitative research, you should have a research question with identifiable variables. When social scientists measure concepts, they often use the language of variables and attributes . A variable refers to a quality or quantity that varies across people or situations. Attributes are the characteristics that make up a variable. For example, the variable hair color could contain attributes such as blonde, brown, black, red, gray, etc.
Levels of measurement
A variable’s attributes determine its level of measurement. There are four possible levels of measurement: nominal, ordinal, interval, and ratio. The first two levels of measurement are categorical , meaning their attributes are categories rather than numbers. The latter two levels of measurement are continuous , meaning their attributes are numbers within a range.
Nominal level of measurement
Hair color is an example of a nominal level of measurement. At the nominal level of measurement , attributes are categorical, and those categories cannot be mathematically ranked. In all nominal levels of measurement, there is no ranking order; the attributes are simply different. Gender and race are two additional variables measured at the nominal level. A variable that has only two possible attributes is called binary or dichotomous . If you are measuring whether an individual has received a specific service, this is a dichotomous variable, as the only two options are received or not received.
What attributes are contained in the variable hair color ? Brown, black, blonde, and red are common colors, but if we only list these attributes, many people may not fit into those categories. This means that our attributes were not exhaustive. Exhaustiveness means that every participant can find a choice for their attribute in the response options. It is up to the researcher to include the most comprehensive attribute choices relevant to their research questions. We may have to list a lot of colors before we can meet the criteria of exhaustiveness. Clearly, there is a point at which exhaustiveness has been reasonably met. If a person insists that their hair color is light burnt sienna , it is not your responsibility to list that as an option. Rather, that person would reasonably be described as brown-haired. Perhaps listing a category for other color would suffice to make our list of colors exhaustive.
What about a person who has multiple hair colors at the same time, such as red and black? They would fall into multiple attributes. This violates the rule of mutual exclusivity , in which a person cannot fall into two different attributes. Instead of listing all of the possible combinations of colors, perhaps you might include a multi-color attribute to describe people with more than one hair color.
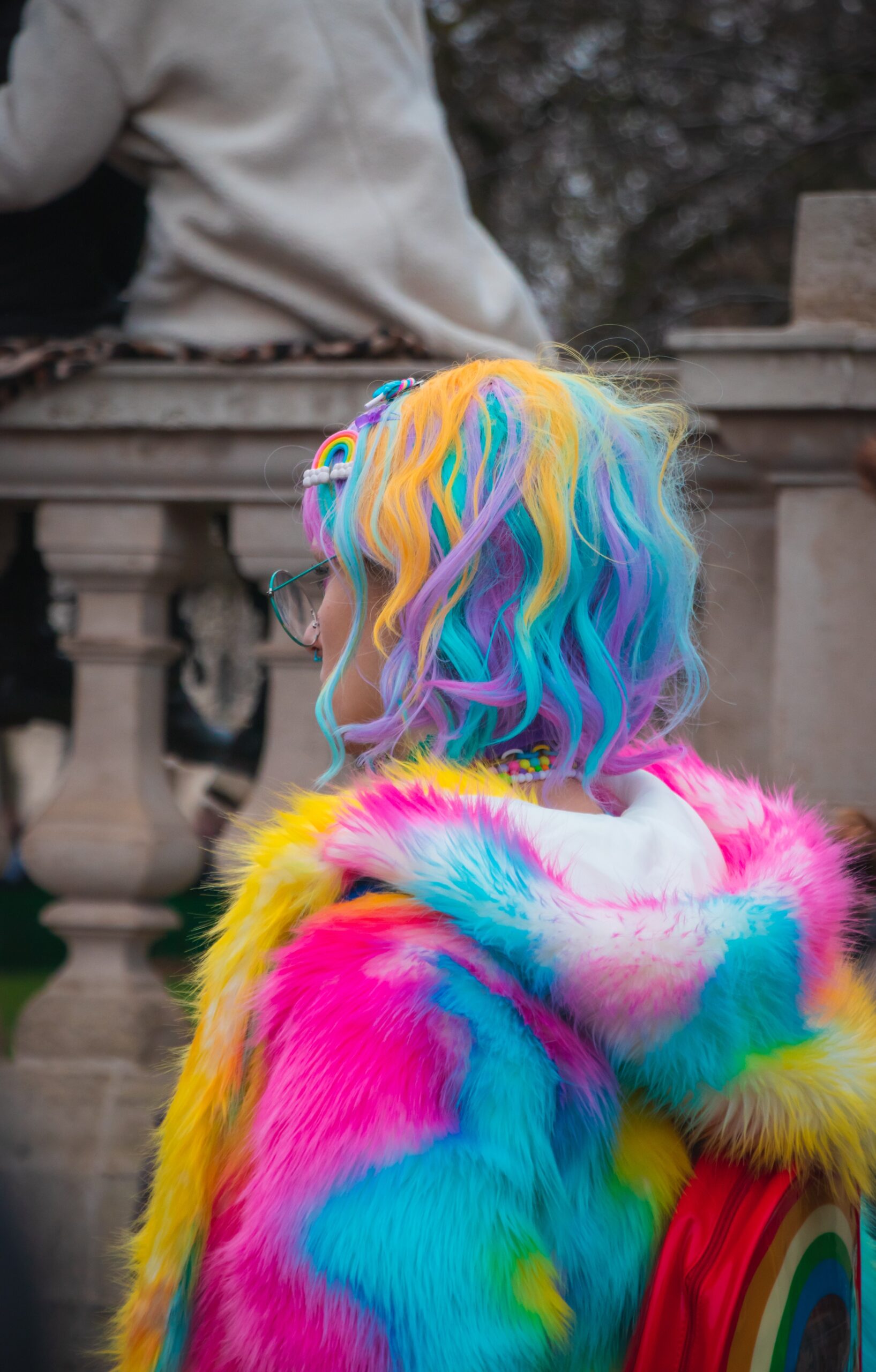
Making sure researchers provide mutually exclusive and exhaustive attribute options is about making sure all people are represented in the data record. For many years, the attributes for gender were only male or female. Now, our understanding of gender has evolved to encompass more attributes that better reflect the diversity in the world. Children of parents from different races were often classified as one race or another, even if they identified with both. The option for bi-racial or multi-racial on a survey not only more accurately reflects the racial diversity in the real world but also validates and acknowledges people who identify in that manner. If we did not measure race in this way, we would leave empty the data record for people who identify as biracial or multiracial, impairing our search for truth.
Ordinal level of measurement
Unlike nominal-level measures, attributes at the ordinal level of measurement can be rank-ordered. For example, someone’s degree of satisfaction in their romantic relationship can be ordered by magnitude of satisfaction. That is, you could say you are not at all satisfied, a little satisfied, moderately satisfied, or highly satisfied. Even though these have a rank order to them (not at all satisfied is certainly worse than highly satisfied), we cannot calculate a mathematical distance between those attributes. We can simply say that one attribute of an ordinal-level variable is more or less than another attribute. A variable that is commonly measured at the ordinal level of measurement in social work is education (e.g., less than high school education, high school education or equivalent, some college, associate’s degree, college degree, graduate degree or higher). Just as with nominal level of measurement, ordinal-level attributes should also be exhaustive and mutually exclusive.
Rating scales for ordinal-level measurement
The fact that we cannot specify exactly how far apart the responses for different individuals in ordinal level of measurement can become clear when using rating scales . If you have ever taken a customer satisfaction survey or completed a course evaluation for school, you are familiar with rating scales such as, “On a scale of 1-5, with 1 being the lowest and 5 being the highest, how likely are you to recommend our company to other people?” Rating scales use numbers, but only as a shorthand, to indicate what attribute (highly likely, somewhat likely, etc.) the person feels describes them best. You wouldn’t say you are “2” likely to recommend the company, but you would say you are “not very likely” to recommend the company. In rating scales the difference between 2 = “ not very likely” and 3 = “ somewhat likely” is not quantifiable as a difference of 1. Likewise, we couldn’t say that it is the same as the difference between 3 = “ somewhat likely ” and 4 = “ very likely .”
Rating scales can be unipolar rating scales where only one dimension is tested, such as frequency (e.g., Never, Rarely, Sometimes, Often, Always) or strength of satisfaction (e.g., Not at all, Somewhat, Very). The attributes on a unipolar rating scale are different magnitudes of the same concept.
There are also bipolar rating scales where there is a dichotomous spectrum, such as liking or disliking (Like very much, Like somewhat, Like slightly, Neither like nor dislike, Dislike slightly, Dislike somewhat, Dislike very much). The attributes on the ends of a bipolar scale are opposites of one another. Figure 10.1 shows several examples of bipolar rating scales.
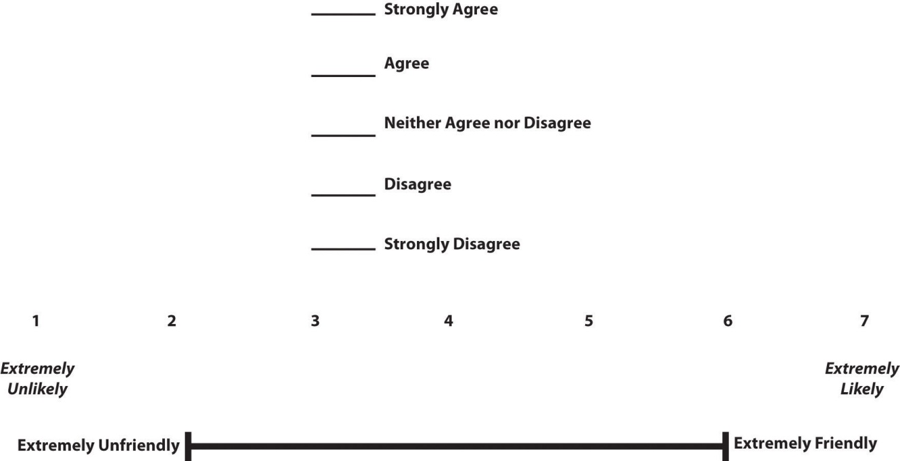
Interval level of measurement
Interval measures are continuous, meaning the meaning and interpretation of their attributes are numbers, rather than categories. Temperatures in Fahrenheit and Celsius are interval level, as are IQ scores and credit scores. Just like variables measured at the ordinal level, the attributes for variables measured at the interval level should be mutually exclusive and exhaustive, and are rank-ordered. In addition, they also have an equal distance between the attribute values.
The interval level of measurement allows us to examine “how much more” is one attribute when compared to another, which is not possible with nominal or ordinal measures. In other words, the unit of measurement allows us to compare the distance between attributes. The value of one unit of measurement (e.g., one degree Celsius, one IQ point) is always the same regardless of where in the range of values you look. The difference of 10 degrees between a temperature of 50 and 60 degrees Fahrenheit is the same as the difference between 60 and 70 degrees Fahrenheit.
We cannot, however, say with certainty what the ratio of one attribute is in comparison to another. For example, it would not make sense to say that a person with an IQ score of 140 has twice the IQ of a person with a score of 70. However, the difference between IQ scores of 80 and 100 is the same as the difference between IQ scores of 120 and 140.
You may find research in which ordinal-level variables are treated as if they are interval measures for analysis. This can be a problem because as we’ve noted, there is no way to know whether the difference between a 3 and a 4 on a rating scale is the same as the difference between a 2 and a 3. Those numbers are just placeholders for categories.
Ratio level of measurement
The final level of measurement is the ratio level of measurement . Variables measured at the ratio level of measurement are continuous variables, just like with interval scale. They, too, have equal intervals between each point. However, the ratio level of measurement has a true zero, which means that a value of zero on a ratio scale means that the variable you’re measuring is absent. For example, if you have no siblings, the a value of 0 indicates this (unlike a temperature of 0 which does not mean there is no temperature). What is the advantage of having a “true zero?” It allows you to calculate ratios. For example, if you have a three siblings, you can say that this is half the number of siblings as a person with six.
At the ratio level, the attribute values are mutually exclusive and exhaustive, can be rank-ordered, the distance between attributes is equal, and attributes have a true zero point. Thus, with these variables, we can say what the ratio of one attribute is in comparison to another. Examples of ratio-level variables include age and years of education. We know that a person who is 12 years old is twice as old as someone who is 6 years old. Height measured in meters and weight measured in kilograms are good examples. So are counts of discrete objects or events such as the number of siblings one has or the number of questions a student answers correctly on an exam. Measuring interval and ratio data is relatively easy, as people either select or input a number for their answer. If you ask a person how many eggs they purchased last week, they can simply tell you they purchased `a dozen eggs at the store, two at breakfast on Wednesday, or none at all.
The differences between each level of measurement are visualized in Table 10.2.
Levels of measurement=levels of specificity
We have spent time learning how to determine a variable’s level of measurement. Now what? How could we use this information to help us as we measure concepts and develop measurement tools? First, the types of statistical tests that we are able to use depend on level of measurement. With nominal-level measurement, for example, the only available measure of central tendency is the mode. With ordinal-level measurement, the median or mode can be used. Interval- and ratio-level measurement are typically considered the most desirable because they permit any indicators of central tendency to be computed (i.e., mean, median, or mode). Also, ratio-level measurement is the only level that allows meaningful statements about ratios of scores. The higher the level of measurement, the more options we have for the statistical tests we are able to conduct. This knowledge may help us decide what kind of data we need to gather, and how.
That said, we have to balance this knowledge with the understanding that sometimes, collecting data at a higher level of measurement could negatively impact our studies. For instance, sometimes providing answers in ranges may make prospective participants feel more comfortable responding to sensitive items. Imagine that you were interested in collecting information on topics such as income, number of sexual partners, number of times someone used illicit drugs, etc. You would have to think about the sensitivity of these items and determine if it would make more sense to collect some data at a lower level of measurement (e.g., nominal: asking if they are sexually active or not) versus a higher level such as ratio (e.g., their total number of sexual partners).
Finally, sometimes when analyzing data, researchers find a need to change a variable’s level of measurement. For example, a few years ago, a student was interested in studying the association between mental health and life satisfaction. This student used a variety of measures. One item asked about the number of mental health symptoms, reported as the actual number. When analyzing data, the student examined the mental health symptom variable and noticed that she had two groups, those with none or one symptoms and those with many symptoms. Instead of using the ratio level data (actual number of mental health symptoms), she collapsed her cases into two categories, few and many. She decided to use this variable in her analyses. It is important to note that you can move a higher level of data to a lower level of data; however, you are unable to move a lower level to a higher level.
- Check that the variables in your research question can vary…and that they are not constants or one of many potential attributes of a variable.
- Think about the attributes your variables have. Are they categorical or continuous? What level of measurement seems most appropriate?
Step 2 of Operationalization: Specify measures for each variable
Let’s pick a social work research question and walk through the process of operationalizing variables to see how specific we need to get. Suppose we hypothesize that residents of a psychiatric unit who are more depressed are less likely to be satisfied with care. Remember, this would be an inverse relationship—as levels of depression increase, satisfaction decreases. In this hypothesis, level of depression is the independent (or predictor) variable and satisfaction with care is the dependent (or outcome) variable.
How would you measure these key variables? What indicators would you look for? Some might say that levels of depression could be measured by observing a participant’s body language. They may also say that a depressed person will often express feelings of sadness or hopelessness. In addition, a satisfied person might be happy around service providers and often express gratitude. While these factors may indicate that the variables are present, they lack coherence. Unfortunately, what this “measure” is actually saying is that “I know depression and satisfaction when I see them.” In a research study, you need more precision for how you plan to measure your variables. Individual judgments are subjective, based on idiosyncratic experiences with depression and satisfaction. They couldn’t be replicated by another researcher. They also can’t be done consistently for a large group of people. Operationalization requires that you come up with a specific and rigorous measure for seeing who is depressed or satisfied.
Finding a good measure for your variable depends on the kind of variable it is. Variables that are directly observable might include things like taking someone’s blood pressure, marking attendance or participation in a group, and so forth. To measure an indirectly observable variable like age, you would probably put a question on a survey that asked, “How old are you?” Measuring a variable like income might first require some more conceptualization, though. Are you interested in this person’s individual income or the income of their family unit? This might matter if your participant does not work or is dependent on other family members for income. Do you count income from social welfare programs? Are you interested in their income per month or per year? Even though indirect observables are relatively easy to measure, the measures you use must be clear in what they are asking, and operationalization is all about figuring out the specifics about how to measure what you want to know. For more complicated variables such as constructs, you will need compound measures that use multiple indicators to measure a single variable.
How you plan to collect your data also influences how you will measure your variables. For social work researchers using secondary data like client records as a data source, you are limited by what information is in the data sources you can access. If a partnering organization uses a given measurement for a mental health outcome, that is the one you will use in your study. Similarly, if you plan to study how long a client was housed after an intervention using client visit records, you are limited by how their caseworker recorded their housing status in the chart. One of the benefits of collecting your own data is being able to select the measures you feel best exemplify your understanding of the topic.
Composite measures
Depending on your research design, your measure may be something you put on a survey or pre/post-test that you give to your participants. For a variable like age or income, one well-worded item may suffice. Unfortunately, most variables in the social world are not so simple. Depression and satisfaction are multidimensional concepts. Relying on a indicator that is a single item on a questionnaire like a question that asks “Yes or no, are you depressed?” does not encompass the complexity of constructs.
For more complex variables, researchers use scales and indices (sometimes called indexes) because they use multiple items to develop a composite (or total) score as a measure for a variable. As such, they are called composite measures . Composite measures provide a much greater understanding of concepts than a single item could.
It can be complex to delineate between multidimensional and unidimensional concepts. If satisfaction were a key variable in our study, we would need a theoretical framework and conceptual definition for it. Perhaps we come to view satisfaction has having two dimensions: a mental one and an emotional one. That means we would need to include indicators that measured both mental and emotional satisfaction as separate dimensions of satisfaction. However, if satisfaction is not a key variable in your theoretical framework, it may make sense to operationalize it as a unidimensional concept.
Although we won’t delve too deeply into the process of scale development, we will cover some important topics for you to understand how scales and indices developed by other researchers can be used in your project.
Need to make better sense of the following content:
Measuring abstract concepts in concrete terms remains one of the most difficult tasks in empirical social science research.
A scale , XXXXXXXXXXXX .
The scales we discuss in this section are a different from “rating scales” discussed in the previous section. A rating scale is used to capture the respondents’ reactions to a given item on a questionnaire. For example, an ordinally scaled item captures a value between “strongly disagree” to “strongly agree.” Attaching a rating scale to a statement or instrument is not scaling. Rather, scaling is the formal process of developing scale items, before rating scales can be attached to those items.
If creating your own scale sounds painful, don’t worry! For most constructs, you would likely be duplicating work that has already been done by other researchers. Specifically, this is a branch of science called psychometrics. You do not need to create a scale for depression because scales such as the Patient Health Questionnaire (PHQ-9) [1] , the Center for Epidemiologic Studies Depression Scale (CES-D) [2] , and Beck’s Depression Inventory [3] (BDI) have been developed and refined over dozens of years to measure variables like depression. Similarly, scales such as the Patient Satisfaction Questionnaire (PSQ-18) have been developed to measure satisfaction with medical care. As we will discuss in the next section, these scales have been shown to be reliable and valid. While you could create a new scale to measure depression or satisfaction, a study with rigor would pilot test and refine that new scale over time to make sure it measures the concept accurately and consistently before using it in other research. This high level of rigor is often unachievable in smaller research projects because of the cost and time involved in pilot testing and validating, so using existing scales is recommended.
Unfortunately, there is no good one-stop-shop for psychometric scales. The Mental Measurements Yearbook provides a list of measures for social science variables, though it is incomplete and may not contain the full documentation for instruments in its database. It is available as a searchable database by many university libraries.
Perhaps an even better option could be looking at the methods section of the articles in your literature review. The methods section of each article will detail how the researchers measured their variables, and often the results section is instructive for understanding more about measures. In a quantitative study, researchers may have used a scale to measure key variables and will provide a brief description of that scale, its names, and maybe a few example questions. If you need more information, look at the results section and tables discussing the scale to get a better idea of how the measure works.
Looking beyond the articles in your literature review, searching Google Scholar or other databases using queries like “depression scale” or “satisfaction scale” should also provide some relevant results. For example, searching for documentation for the Rosenberg Self-Esteem Scale, I found this report about useful measures for acceptance and commitment therapy which details measurements for mental health outcomes. If you find the name of the scale somewhere but cannot find the documentation (i.e., all items, response choices, and how to interpret the scale), a general web search with the name of the scale and “.pdf” may bring you to what you need. Or, to get professional help with finding information, ask a librarian!
Unfortunately, these approaches do not guarantee that you will be able to view the scale itself or get information on how it is interpreted. Many scales cost money to use and may require training to properly administer. You may also find scales that are related to your variable but would need to be slightly modified to match your study’s needs. You could adapt a scale to fit your study, however changing even small parts of a scale can influence its accuracy and consistency. Pilot testing is always recommended for adapted scales, and researchers seeking to draw valid conclusions and publish their results should take this additional step.
Types of scales
Likert scales.
Although Likert scale is a term colloquially used to refer to almost any rating scale (e.g., a 0-to-10 life satisfaction scale), it has a much more precise meaning. In the 1930s, researcher Rensis Likert (pronounced LICK-ert) created a new approach for measuring people’s attitudes (Likert, 1932) . [4] It involves presenting people with several statements—including both favorable and unfavorable statements—about some person, group, or idea. Respondents then express their approval or disapproval with each statement on a 5-point rating scale: Strongly Approve , Approve , Undecided , Disapprove, Strongly Disapprove . Numbers are assigned to each response a nd then summed across all items to produce a score representing the attitude toward the person, group, or idea. For items that are phrased in an opposite direction (e.g., negatively worded statements instead of positively worded statements), reverse coding is used so that the numerical scoring of statements also runs in the opposite direction. The scores for the entire set of items are totaled for a score for the attitude of interest. This type of scale came to be called a Likert scale, as indicated in Table 10.3 below. Scales that use similar logic but do not have these exact characteristics are referred to as “Likert-type scales.”
Semantic Differential Scales
Semantic differential scales are composite scales in which respondents are asked to indicate their opinions or feelings toward a single statement using different pairs of adjectives framed as polar opposites. Whereas in a Likert scale, a participant is asked how much they approve or disapprove of a statement, in a semantic differential scale the participant is asked to indicate how they about a specific item using several pairs of opposites. This makes the semantic differential scale an excellent technique for measuring people’s feelings toward objects, events, or behaviors. Table 10.4 provides an example of a semantic differential scale that was created to assess participants’ feelings about this textbook.
Guttman Scales
A specialized scale for measuring unidimensional concepts was designed by Louis Guttman. A Guttman scale (also called cumulative scale ) uses a series of items arranged in increasing order of intensity (least intense to most intense) of the concept. This type of scale allows us to understand the intensity of beliefs or feelings. Each item in the Guttman scale below has a weight (this is not indicated on the tool) which varies with the intensity of that item, and the weighted combination of each response is used as an aggregate measure of an observation.
Table XX presents an example of a Guttman Scale. Notice how the items move from lower intensity to higher intensity. A researcher reviews the yes answers and creates a score for each participant.
Example Guttman Scale Items
- I often felt the material was not engaging Yes/No
- I was often thinking about other things in class Yes/No
- I was often working on other tasks during class Yes/No
- I will work to abolish research from the curriculum Yes/No
An index is a composite score derived from aggregating measures of multiple indicators. At its most basic, an index sums up indicators. A well-known example of an index is the consumer price index (CPI), which is computed every month by the Bureau of Labor Statistics of the U.S. Department of Labor. The CPI is a measure of how much consumers have to pay for goods and services (in general) and is divided into eight major categories (food and beverages, housing, apparel, transportation, healthcare, recreation, education and communication, and “other goods and services”), which are further subdivided into more than 200 smaller items. Each month, government employees call all over the country to get the current prices of more than 80,000 items. Using a complicated weighting scheme that takes into account the location and probability of purchase for each item, analysts then combine these prices into an overall index score using a series of formulas and rules.
Another example of an index is the Duncan Socioeconomic Index (SEI). This index is used to quantify a person’s socioeconomic status (SES) and is a combination of three concepts: income, education, and occupation. Income is measured in dollars, education in years or degrees achieved, and occupation is classified into categories or levels by status. These very different measures are combined to create an overall SES index score. However, SES index measurement has generated a lot of controversy and disagreement among researchers.
The process of creating an index is similar to that of a scale. First, conceptualize the index and its constituent components. Though this appears simple, there may be a lot of disagreement on what components (concepts/constructs) should be included or excluded from an index. For instance, in the SES index, isn’t income correlated with education and occupation? And if so, should we include one component only or all three components? Reviewing the literature, using theories, and/or interviewing experts or key stakeholders may help resolve this issue. Second, operationalize and measure each component. For instance, how will you categorize occupations, particularly since some occupations may have changed with time (e.g., there were no Web developers before the Internet)? As we will see in step three below, researchers must create a rule or formula for calculating the index score. Again, this process may involve a lot of subjectivity, so validating the index score using existing or new data is important.
Differences between scales and indices
Though indices and scales yield a single numerical score or value representing a concept of interest, they are different in many ways. First, indices often comprise components that are very different from each other (e.g., income, education, and occupation in the SES index) and are measured in different ways. Conversely, scales typically involve a set of similar items that use the same rating scale (such as a five-point Likert scale about customer satisfaction).
Second, indices often combine objectively measurable values such as prices or income, while scales are designed to assess subjective or judgmental constructs such as attitude, prejudice, or self-esteem. Some argue that the sophistication of the scaling methodology makes scales different from indexes, while others suggest that indexing methodology can be equally sophisticated. Nevertheless, indexes and scales are both essential tools in social science research.
Scales and indices seem like clean, convenient ways to measure different phenomena in social science, but just like with a lot of research, we have to be mindful of the assumptions and biases underneath. What if the developers of scale or an index were influenced by unconscious biases? Or what if it was validated using only White women as research participants? Is it going to be useful for other groups? It very well might be, but when using a scale or index on a group for whom it hasn’t been tested, it will be very important to evaluate the validity and reliability of the instrument, which we address in the rest of the chapter.
Finally, it’s important to note that while scales and indices are often made up of items measured at the nominal or ordinal level, the scores on the composite measurement are continuous variables.
Looking back to your work from the previous section, are your variables unidimensional or multidimensional?
- Describe the specific measures you will use (actual questions and response options you will use with participants) for each variable in your research question.
- If you are using a measure developed by another researcher but do not have all of the questions, response options, and instructions needed to implement it, put it on your to-do list to get them.
- Describe at least one specific measure you would use (actual questions and response options you would use with participants) for the dependent variable in your research question.
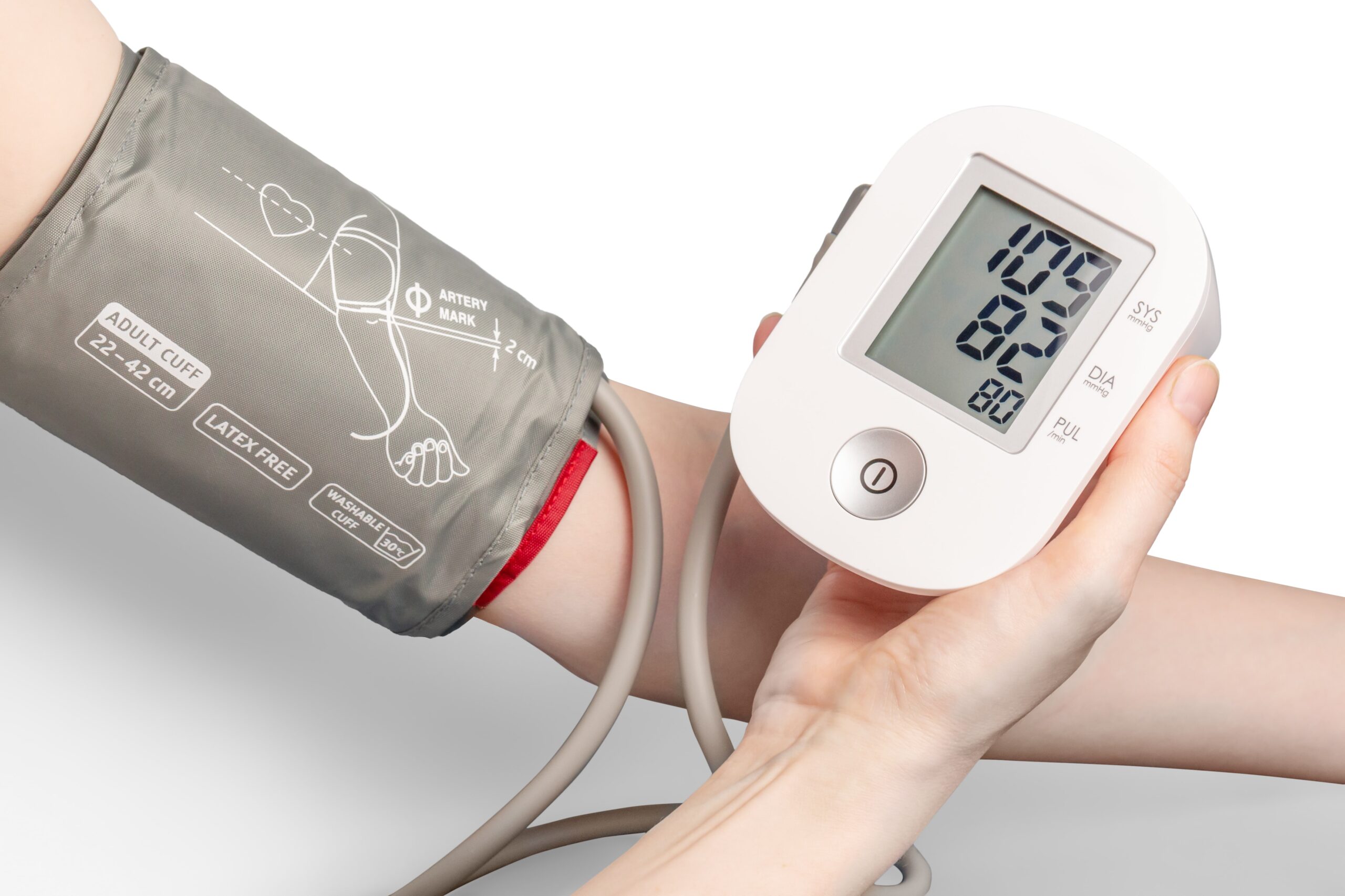
Step 3 in Operationalization: Determine how to interpret measures
The final stage of operationalization involves setting the rules for how the measure works and how the researcher should interpret the results. Sometimes, interpreting a measure can be incredibly easy. If you ask someone their age, you’ll probably interpret the results by noting the raw number (e.g., 22) someone provides and that it is lower or higher than other people’s ages. However, you could also recode that person into age categories (e.g., under 25, 20-29-years-old, generation Z, etc.). Even scales or indices may be simple to interpret. If there is an index of problem behaviors, one might simply add up the number of behaviors checked off–with a range from 1-5 indicating low risk of delinquent behavior, 6-10 indicating the student is moderate risk, etc. How you choose to interpret your measures should be guided by how they were designed, how you conceptualize your variables, the data sources you used, and your plan for analyzing your data statistically. Whatever measure you use, you need a set of rules for how to take any valid answer a respondent provides to your measure and interpret it in terms of the variable being measured.
For more complicated measures like scales, refer to the information provided by the author for how to interpret the scale. If you can’t find enough information from the scale’s creator, look at how the results of that scale are reported in the results section of research articles. For example, Beck’s Depression Inventory (BDI-II) uses 21 statements to measure depression and respondents rate their level of agreement on a scale of 0-3. The results for each question are added up, and the respondent is put into one of three categories: low levels of depression (1-16), moderate levels of depression (17-30), or severe levels of depression (31 and over) ( NEEDS CITATION) .
Operationalization is a tricky component of basic research methods, so don’t get frustrated if it takes a few drafts and a lot of feedback to get to a workable operational definition.
Key Takeaways
- Operationalization involves spelling out precisely how a concept will be measured.
- Operational definitions must include the variable, the measure, and how you plan to interpret the measure.
- There are four different levels of measurement: nominal, ordinal, interval, and ratio (in increasing order of specificity).
- Scales and indices are common ways to collect information and involve using multiple indicators in measurement.
- A key difference between a scale and an index is that a scale contains multiple indicators for one concept, whereas an indicator examines multiple concepts (components).
- Using scales developed and refined by other researchers can improve the rigor of a quantitative study.
Use the research question that you developed in the previous chapters and find a related scale or index that researchers have used. If you have trouble finding the exact phenomenon you want to study, get as close as you can.
- What is the level of measurement for each item on each tool? Take a second and think about why the tool’s creator decided to include these levels of measurement. Identify any levels of measurement you would change and why.
- If these tools don’t exist for what you are interested in studying, why do you think that is?
Using your working research question, find a related scale or index that researchers have used to measure the dependent variable. If you have trouble finding the exact phenomenon you want to study, get as close as you can.
- What is the level of measurement for each item on the tool? Take a second and think about why the tool’s creator decided to include these levels of measurement. Identify any levels of measurement you would change and why.
- Kroenke, K., Spitzer, R. L., & Williams, J. B. (2001). The PHQ-9: validity of a brief depression severity measure. Journal of general internal medicine, 16(9), 606–613. https://doi.org/10.1046/j.1525-1497.2001.016009606.x ↵
- Radloff, L. S. (1977). The CES-D scale: A self report depression scale for research in the general population. Applied Psychological Measurements, 1, 385-401. ↵
- Beck, A. T., Ward, C. H., Mendelson, M., Mock, J., & Erbaugh, J. (1961). An inventory for measuring depression. Archives of general psychiatry, 4, 561–571. https://doi.org/10.1001/archpsyc.1961.01710120031004 ↵
- Likert, R. (1932). A technique for the measurement of attitudes. Archives of Psychology, 140 , 1–55. ↵
process by which researchers spell out precisely how a concept will be measured in their study
Clues that demonstrate the presence, intensity, or other aspects of a concept in the real world
unprocessed data that researchers can analyze using quantitative and qualitative methods (e.g., responses to a survey or interview transcripts)
“a logical grouping of attributes that can be observed and measured and is expected to vary from person to person in a population” (Gillespie & Wagner, 2018, p. 9)
The characteristics that make up a variable
variables whose values are organized into mutually exclusive groups but whose numerical values cannot be used in mathematical operations.
variables whose values are mutually exclusive and can be used in mathematical operations
The lowest level of measurement; categories cannot be mathematically ranked, though they are exhaustive and mutually exclusive
Exhaustive categories are options for closed ended questions that allow for every possible response (no one should feel like they can't find the answer for them).
Mutually exclusive categories are options for closed ended questions that do not overlap, so people only fit into one category or another, not both.
Level of measurement that follows nominal level. Has mutually exclusive categories and a hierarchy (rank order), but we cannot calculate a mathematical distance between attributes.
An ordered set of responses that participants must choose from.
A rating scale where the magnitude of a single trait is being tested
A rating scale in which a respondent selects their alignment of choices between two opposite poles such as disagreement and agreement (e.g., strongly disagree, disagree, agree, strongly agree).
A level of measurement that is continuous, can be rank ordered, is exhaustive and mutually exclusive, and for which the distance between attributes is known to be equal. But for which there is no zero point.
The highest level of measurement. Denoted by mutually exclusive categories, a hierarchy (order), values can be added, subtracted, multiplied, and divided, and the presence of an absolute zero.
measurements of variables based on more than one one indicator
An empirical structure for measuring items or indicators of the multiple dimensions of a concept.
measuring people’s attitude toward something by assessing their level of agreement with several statements about it
Composite (multi-item) scales in which respondents are asked to indicate their opinions or feelings toward a single statement using different pairs of adjectives framed as polar opposites.
A composite scale using a series of items arranged in increasing order of intensity of the construct of interest, from least intense to most intense.
a composite score derived from aggregating measures of multiple concepts (called components) using a set of rules and formulas
Doctoral Research Methods in Social Work Copyright © by Mavs Open Press. All Rights Reserved.
Share This Book
Operational Definition
- Living reference work entry
- First Online: 04 December 2023
- Cite this living reference work entry
- Li Shouxin 2
Operational definition is the definition of variables in a psychological study using specific activities or detailed operational procedures. In the psychological experiment, the operational definition of variables makes the research less metaphysical and more concrete, so the final conclusions are verifiable. Motivation, for example, is defined in general psychology as a psychological tendency or internal urge to actuate and sustain an individual’s actions toward a certain goal, but in specific experiments that study how gorillas solve problems, the operational definition of motivation is how long gorillas starve – the longer they starve, the stronger their motivation to eat. Operational definition does not describe the characteristics and properties of the defined variables; it is to make them observable and measurable. For example, academic achievement is a student’s test scores in various different subjects; cognitive style is distinguished according to the subjects’ score in...
This is a preview of subscription content, log in via an institution to check access.
Access this chapter
Institutional subscriptions
Further Reading
Kantowitz BH, Roediger HL, Elmes DG (2015) Experimental psychology, 10th edn. Cengage Learning, Boston
Google Scholar
Zhang X-M, Hua S (2014) Experimental psychology. Beijing Normal University Publishing Group, Beijing
Download references
Author information
Authors and affiliations.
School of Psychology, Shandong Normal University, Jinan, China
You can also search for this author in PubMed Google Scholar
Corresponding author
Correspondence to Li Shouxin .
Rights and permissions
Reprints and permissions
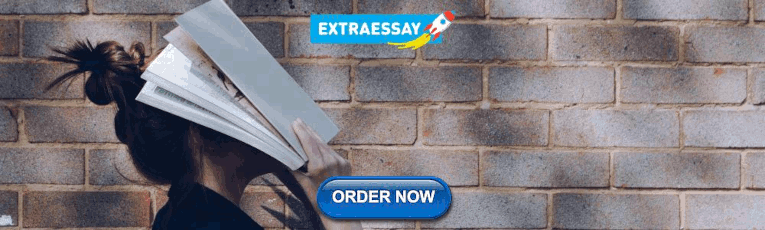
Copyright information
© 2024 Encyclopedia of China Publishing House
About this entry
Cite this entry.
Shouxin, L. (2024). Operational Definition. In: The ECPH Encyclopedia of Psychology. Springer, Singapore. https://doi.org/10.1007/978-981-99-6000-2_220-1
Download citation
DOI : https://doi.org/10.1007/978-981-99-6000-2_220-1
Received : 14 November 2023
Accepted : 15 November 2023
Published : 04 December 2023
Publisher Name : Springer, Singapore
Print ISBN : 978-981-99-6000-2
Online ISBN : 978-981-99-6000-2
eBook Packages : Springer Reference Behavioral Science and Psychology Reference Module Humanities and Social Sciences Reference Module Business, Economics and Social Sciences
- Publish with us
Policies and ethics
- Find a journal
- Track your research
Scientific Research and Methodology
2.2 conceptual and operational definitions.
Research studies usually include terms that must be carefully and precisely defined, so that others know exactly what has been done and there are no ambiguities. Two types of definitions can be given: conceptual definitions and operational definitions .
Loosely speaking, a conceptual definition explains what to measure or observe (what a word or a term means for your study), and an operational definitions defines exactly how to measure or observe it.
For example, in a study of stress in students during a university semester. A conceptual definition would describe what is meant by ‘stress.’ An operational definition would describe how the ‘stress’ would be measured.
Sometimes the definitions themselves aren’t important, provided a clear definition is given. Sometimes, commonly-accepted definitions exist, so should be used unless there is a good reason to use a different definition (for example, in criminal law, an ‘adult’ in Australia is someone aged 18 or over ).
Sometimes, a commonly-accepted definition does not exist, so the definition being used should be clearly articulated.
Example 2.2 (Operational and conceptual definitions) Players and fans have become more aware of concussions and head injuries in sport. A Conference on concussion in sport developed this conceptual definition ( McCrory et al. 2013 ) :
Concussion is a brain injury and is defined as a complex pathophysiological process affecting the brain, induced by biomechanical forces. Several common features that incorporate clinical, pathologic and biomechanical injury constructs that may be utilised in defining the nature of a concussive head injury include: Concussion may be caused either by a direct blow to the head, face, neck or elsewhere on the body with an “impulsive” force transmitted to the head. Concussion typically results in the rapid onset of short-lived impairment of neurological function that resolves spontaneously. However, in some cases, symptoms and signs may evolve over a number of minutes to hours. Concussion may result in neuropathological changes, but the acute clinical symptoms largely reflect a functional disturbance rather than a structural injury and, as such, no abnormality is seen on standard structural neuroimaging studies. Concussion results in a graded set of clinical symptoms that may or may not involve loss of consciousness. Resolution of the clinical and cognitive symptoms typically follows a sequential course. However, it is important to note that in some cases symptoms may be prolonged.
While this is all helpful… it does not explain how to identify a player with concussion during a game.
Rugby decided on this operational definition ( Raftery et al. 2016 ) :
… a concussion applies with any of the following: The presence, pitch side, of any Criteria Set 1 signs or symptoms (table 1)… [ Note : This table includes symptoms such as ‘convulsion,’ ‘clearly dazed,’ etc.]; An abnormal post game, same day assessment…; An abnormal 36–48 h assessment…; The presence of clinical suspicion by the treating doctor at any time…
Example 2.3 (Operational and conceptual definitions) Consider a study requiring water temperature to be measured.
An operational definition would explain how the temperature is measured: the thermometer type, how the thermometer was positioned, how long was it left in the water, and so on.

Example 2.4 (Operational definitions) Consider a study measuring stress in first-year university students.
Stress cannot be measured directly, but could be assessed using a survey (like the Perceived Stress Scale (PSS) ( Cohen et al. 1983 ) ).
The operational definition of stress is the score on the ten-question PSS. Other means of measuring stress are also possible (such as heart rate or blood pressure).
Meline ( 2006 ) discusses five studies about stuttering, each using a different operational definition:
- Study 1: As diagnosed by speech-language pathologist.
- Study 2: Within-word disfluences greater than 5 per 150 words.
- Study 3: Unnatural hesitation, interjections, restarted or incomplete phrases, etc.
- Study 4: More than 3 stuttered words per minute.
- Study 5: State guidelines for fluency disorders.
A study of snacking in Australia ( Fayet-Moore et al. 2017 ) used this operational definition of ‘snacking’:
…an eating occasion that occurred between meals based on time of day. — Fayet-Moore et al. ( 2017 ) (p. 3)
A study examined the possible relationship between the ‘pace of life’ and the incidence of heart disease ( Levine 1990 ) in 36 US cities. The researchers used four different operational definitions for ‘pace of life’ (remember the article was published in 1990!):
- The walking speed of randomly chosen pedestrians.
- The speed with which bank clerks gave ‘change for two $20 bills or [gave] two $20 bills for change.’
- The talking speed of postal clerks.
- The proportion of men and women wearing a wristwatch.
None of these perfectly measure ‘pace of life,’ of course. Nonetheless, the researchers found that, compared to people on the West Coast,
… people in the Northeast walk faster, make change faster, talk faster and are more likely to wear a watch… — Levine ( 1990 ) (p. 455)
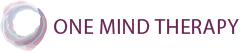
Operational Definition Psychology – Definition, Examples, and How to Write One
Elizabeth Research
Every good psychology study contains an operational definition for the variables in the research. An operational definition allows the researchers to describe in a specific way what they mean when they use a certain term. Generally, operational definitions are concrete and measurable. Defining variables in this way allows other people to see if the research has validity . Validity here refers to if the researchers are actually measuring what they intended to measure.
Definition: An operational definition is the statement of procedures the researcher is going to use in order to measure a specific variable.
We need operational definitions in psychology so that we know exactly what researchers are talking about when they refer to something. There might be different definitions of words depending on the context in which the word is used. Think about how words mean something different to people from different cultures. To avoid any confusion about definitions, in research we explain clearly what we mean when we use a certain term.
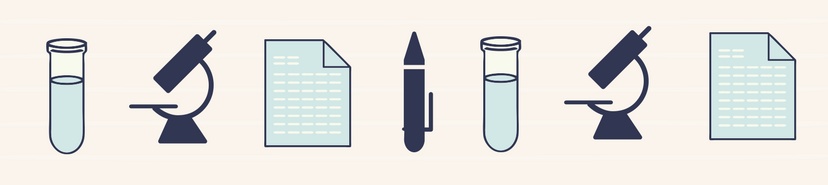
Operational Definition Examples
Example one:.
A researcher wants to measure if age is related to addiction. Perhaps their hypothesis is: the incidence of addiction will increase with age. Here we have two variables, age and addiction. In order to make the research as clear as possible, the researcher must define how they will measure these variables. Essentially, how do we measure someone’s age and how to we measure addiction?
Variable One: Age might seem straightforward. You might be wondering why we need to define age if we all know what age is. However, one researcher might decide to measure age in months in order to get someone’s precise age, while another researcher might just choose to measure age in years. In order to understand the results of the study, we will need to know how this researcher operationalized age. For the sake of this example lets say that age is defined as how old someone is in years.
Variable Two: The variable of addiction is slightly more complicated than age. In order to operationalize it the researcher has to decide exactly how they want to measure addiction. They might narrow down their definition and say that addiction is defined as going through withdrawal when the person stops using a substance. Or the researchers might decide that the definition of addiction is: if someone currently meets the DSM-5 diagnostic criteria for any substance use disorder. For the sake of this example, let’s say that the researcher chose the latter.
Final Definition: In this research study age is defined as participant’s age measured in years and the incidence of addiction is defined as whether or not the participant currently meets the DSM-5 diagnostic criteria for any substance use disorder.
Example Two
A researcher wants to measure if there is a correlation between hot weather and violent crime. Perhaps their guiding hypothesis is: as temperature increases so will violent crime. Here we have two variables, weather and violent crime. In order to make this research precise the researcher will have to operationalize the variables.
Variable One: The first variable is weather. The researcher needs to decide how to define weather. Researchers might chose to define weather as outside temperature in degrees Fahrenheit. But we need to get a little more specific because there is not one stable temperature throughout the day. So the researchers might say that weather is defined as the high recorded temperature for the day measured in degrees Fahrenheit.
Variable Two: The second variable is violent crime. Again, the researcher needs to define how violent crime is measured. Let’s say that for this study it they use the FBI’s definition of violent crime . This definition describes violent crime as “murder and nonnegligent manslaughter, forcible rape, robbery, and aggravated assault”.
However, how do we actually know how many violent crimes were committed on a given day? Researchers might include in the definition something like: the number of people arrested that day for violent crimes as recorded by the local police.
Final Definition: For this study temperature was defined as high recorded temperature for the day measured in degrees Fahrenheit. Violent crime was defined as the number of people arrested in a given day for murder, forcible rape, robbery, and aggravated assault as recorded by the local police.
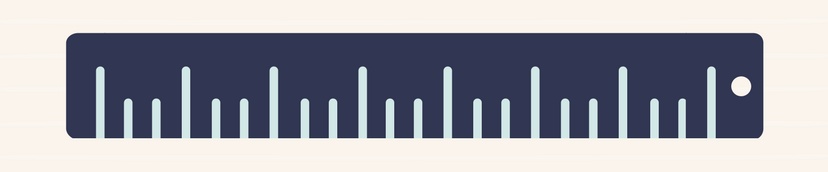
How to Write an Operational Definition
For the last example take the opportunity to see if you can write a clear operational definition for yourself. Imagine that you are creating a research study and you want to see if group therapy is helpful for treating social anxiety.
Variable One: How are you going to define group therapy? here are some things you might want to consider when creating your operational definition:
- What type of group therapy?
- Who is leading the therapy group?
- How long do people participate in the therapy group for?
- How can you “measure” group therapy?
There is no one way to write the operational definition for this variable. You could say something like group therapy was defined as a weekly cognitive behavioral therapy group led by a licensed MFT held over the course of ten weeks. Remember there are many ways to write an operational definition. You know you have written an effective one if another researcher could pick it up and create a very similar variable based on your definition.
Variable Two: The second variable you need to define is “effective treatment social anxiety”. Again, see if you can come up with an operational definition of this variable. This is a little tricky because you will need to be specific about what an effective treatment is as well as what social anxiety is. Here are some things to consider when writing your definition:
- How do you know a treatment is effective?
- How do you measure the effectiveness of treatment?
- Who provides a reliable definition of social anxiety?
- How can you measure social anxiety?
Again, there is no one right way to write this operational definition. If someone else could recreate the study using your definition it is probably an effective one. Here as one example of how you could operationalize the variable: social anxiety was defined as meeting the DSM-5 criteria for social anxiety and the effectiveness of treatment was defined as the reduction of social anxiety symptoms over the 10 week treatment period.
Final Definition: Take your definition for variable one and your definition for variable two and write them in a clear and succinct way. It is alright for your definition to be more than one sentence.
Why We Need Operational Definitions
There are a number of reasons why researchers need to have operational definitions including:
- Replicability
- Generalizability
- Dissemination
The first reason was mentioned earlier in the post when reading research others should be able to assess the validity of the research. That is, did the researchers measure what they intended to measure? If we don’t know how researchers measured something it is very hard to know if the study had validity.
The next reason it is important to have an operational definition is for the sake of replicability . Research should be designed so that if someone else wanted to replicate it they could. By replicating research and getting the same findings we validate the findings. It is impossible to recreate a study if we are unsure about how they defined or measured the variables.
Another reason we need operational definitions is so that we can understand how generalizable the findings are. In research, we want to know that the findings are true not just for a small sample of people. We hope to get findings that generalize to the whole population. If we do not have operational definitions it is hard to generalize the findings because we don’t know who they generalize to.
Finally, operational definitions are important for the dissemination of information. When a study is done it is generally published in a peer-reviewed journal and might be read by other psychologists, students, or journalists. Researchers want people to read their research and apply their findings. If the person reading the article doesn’t know what they are talking about because a variable is not clear it will be hard to them to actually apply this new knowledge.
Receive updates from my blog!
Quickonomics
Operational Research
Definition of operational research.
Operational Research, often known as Operations Research (OR) in the United States, is a discipline that applies advanced analytical methods to help make better decisions. It involves the use of mathematical models, statistics, and algorithms to solve complex problems in a wide range of areas, including logistics, business, engineering, and healthcare. The goal of operational research is to provide a scientific basis for decision-making by identifying the optimal solution to a problem or the best way to operate within given constraints.
Imagine a large logistics company that needs to optimize its delivery routes to minimize costs and maximize efficiency. The company has a fleet of delivery trucks, each with different capacities, and serves customers spread across a wide geographic area with varying demands.
Using operational research techniques, the company can develop a mathematical model representing its delivery network, including variables for truck capacities, delivery times, fuel costs, distances, and customer demand. By applying optimization algorithms to this model, the company can identify the most efficient routes and schedules for its trucks, taking into account constraints such as delivery windows and vehicle capacities.
As a result, the company can reduce fuel consumption, improve delivery times, and lower operational costs, all of which contribute to increased profitability and customer satisfaction.
Why Operational Research Matters
Operational research is crucial for efficient and effective decision-making in many aspects of modern business and society. By providing a rigorous, scientific approach to problem-solving, OR enables organizations to optimize their operations, improve performance, and maximize returns on investment. Its applications can be found in a myriad of fields, including:
– **Supply Chain Management**: Optimizing production schedules, inventory levels, and distribution networks. – **Healthcare**: Allocating resources in hospitals, designing treatment protocols, and managing patient flow. – **Finance**: Risk management, portfolio optimization, and predictive modeling for investment. – **Transportation**: Routing and scheduling for airlines, railroads, and shipping companies, and traffic flow optimization in urban areas. – **Public Services**: Planning and operational efficiency improvement in services like policing, firefighting, and emergency response.
Operational research thus plays a pivotal role in making complex systems more efficient, sustainable, and responsive to the needs of society.
Frequently Asked Questions (FAQ)
What are the key methods and tools used in operational research.
Operational research uses a wide range of methods and tools, including linear programming, integer programming, stochastic models, simulation, and queueing theory. Software tools commonly used in OR include optimization solvers like CPLEX and Gurobi, simulation software such as Simul8 and AnyLogic, and statistical analysis tools like R and Python for data analysis.
Can operational research methods be applied to small businesses or startups?
Yes, operational research techniques can be scaled to benefit businesses of all sizes, including small businesses and startups. For instance, a small e-commerce company might use OR to determine the most cost-effective shipping strategies or to optimize inventory levels based on demand forecasts. The key is to identify the specific challenges and constraints of the business and then apply the appropriate OR methods to find solutions.
How does operational research differ from other decision-making approaches?
Operational research is distinguished by its systematic and quantitative approach to decision-making. Unlike heuristic or based solely on expert judgment approaches, OR uses rigorous mathematical models to analyze complex systems and predict the outcomes of different decisions under various scenarios. This scientific approach allows for more precise, objective, and optimal decision-making, especially in complex and uncertain environments.
What are the challenges in implementing operational research solutions?
One challenge in implementing OR solutions is the need for accurate and comprehensive data, as the quality of the input data significantly affects the accuracy of the model’s predictions. Additionally, developing and solving complex mathematical models requires specialized knowledge and computational resources. Finally, there can be resistance to change within organizations, so effective communication and demonstration of the value added by OR solutions are crucial for their adoption and success.
Operational research, with its ability to provide clarity and optimization in decision-making processes, remains an indispensable tool in navigating the complexities of modern operations, offering substantial benefits to those who employ its methodologies effectively.
To provide the best experiences, we and our partners use technologies like cookies to store and/or access device information. Consenting to these technologies will allow us and our partners to process personal data such as browsing behavior or unique IDs on this site and show (non-) personalized ads. Not consenting or withdrawing consent, may adversely affect certain features and functions.
Click below to consent to the above or make granular choices. Your choices will be applied to this site only. You can change your settings at any time, including withdrawing your consent, by using the toggles on the Cookie Policy, or by clicking on the manage consent button at the bottom of the screen.
Operational and Technical Definition of Terms
Quiz by alvin cipriano.
Feel free to use or edit a copy
includes Teacher and Student dashboards
Measure skills from any curriculum
Tag the questions with any skills you have. Your dashboard will track each student's mastery of each skill.
- edit the questions
- save a copy for later
- start a class game
- automatically assign follow-up activities based on students’ scores
- assign as homework
- share a link with colleagues
- print as a bubble sheet
- Q 1 / 5 Score 0 Which is not a purpose of an operational definition? 29 So others could be able to assess the validity of the research To understand how generalize the findings are in a research To be able possibly recreate a study/ experiment So others will not be able to replicate the research
Our brand new solo games combine with your quiz, on the same screen
Correct quiz answers unlock more play!
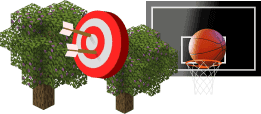
- Q 1 Which is not a purpose of an operational definition? So others could be able to assess the validity of the research To understand how generalize the findings are in a research To be able possibly recreate a study/ experiment So others will not be able to replicate the research 20 s
- Q 2 Using many jargon will make your research paper credible and appealing to readers. false true True or False 20 s
- Q 3 Why are definitions included in technical writing? To improve reader comprehension To attract more readers To make a document more formal To improve the overall appearance of a document 20 s
- Q 4 Parenthetical definitions are ___________________________ More extensive More formal In a format that allows readers to compare the word to terms they are familiar with. Synonyms or short phrases 20 s
- Q 5 What does it mean to use context clues to figure out what technical words mean? Guess what you think the word means by looking at the way that it is spelled. Use other words in the sentence and in the paragraph to help you figure out what the technical word means. Look up the word in the glossary at the back of the book where they will have a definition for you to copy. Figure out what the word means by asking your teacher or someone else to tell you what it is. 20 s
Teachers give this quiz to your class
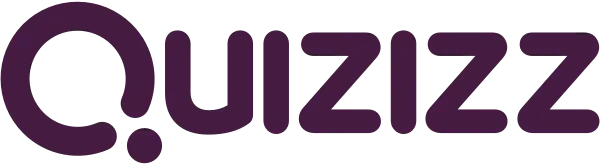
Have an account?

Technical and Operational Definitions
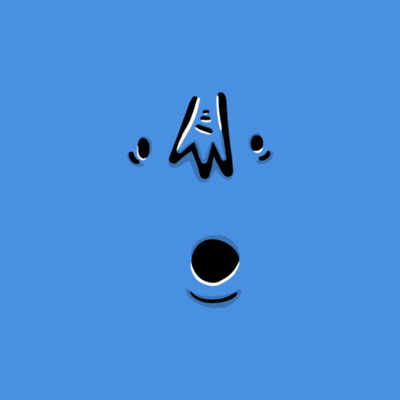
17 questions
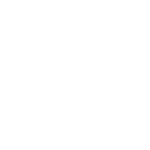
Introducing new Paper mode
No student devices needed. Know more
It is a very detailed description of term, process, or phenomenon
Technical Definition
Operational Definition
Parenthical
The term is defines based on how this term is used in the research study
Operational
The 2 types of definition can be used in research.
The definition of variable in terms of the actual producers used by the researcher to measure.
Example: Overweight - a condition when a woman reaches 150 lbs and 160 lbs for men
Expanded Definition
Example: Vein is any of the tubes forming part of the blood circulation system of the body, carrying the most cases oxygen depleted blood toward the heart.
Technical definitions are used to name a new development or a new technology in technical field.
A one or more paragraphs that attempt to explain a complex term.
Technicial Definition
Example: Happiness - a person feels having no problem whether at home or in school.
Operational Definitions
Technical Definitions
Expanded Definitions
The definition is taken from dictionary which present the underlying understanding of the term.
Adding a synonym ( a term with same meaning in parenthesis)
It presents he focal point of any research as it provides description of the issues that a research team decides to address.
Statement of the Problem
Research Objectives
Significance of the Study
These are the goals which the research aims to achieve
This presents the coverage of the study and its restrictions
Scope and Limitation
Definition of Terms
This provides information on what and how the study will contribute and who will benefit from it.
Hypotheses - These are peculations upon the outcome of a research or experiment or an educated prediction that can be used.
Explore all questions with a free account
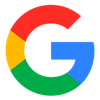
Continue with email
Continue with phone

What are operational definitions in science?
An operational definition is designed to model or represent a concept or theoretical definition, also known as a construct. Scientists should describe the operations (procedures, actions, or processes) that define the concept with enough specificity such that other investigators can replicate their research.
What is an operational definition example?
a description of something in terms of the operations (procedures, actions, or processes) by which it could be observed and measured. For example, the operational definition of anxiety could be in terms of a test score, withdrawal from a situation, or activation of the sympathetic nervous system.
Which is the best example of an operational definition?
An Operational Definition is the definition of a variable in terms of the operations or techniques used to measure or manipulate it. Examples: -“Height” as defined by the number of feet/inches a person is tall.
What is the operational definition of an experiment?
Experimental Operational Definition: Steps taken by a researcher to produce certain experimental conditions.
How do you write an operational definition?

Why is an operational definition important?
Your operational definitions describe the variables you will use as indicators and the procedures you will use to observe or measure them. You need an operational definition because you can’t measure anything without one, no matter how good your conceptual definition might be.
What is conceptual definition and operational definition?
A conceptual definition describes a thing in terms of its abstract characteristics and relationships to other conceptual entities. An operational definition describes a thing in terms of the actual physical actions it takes, almost like a recipe.
What is the operational definition of an element?
An element is a fundamental item that can’t be easily broken into smaller pieces. In chemistry and physics, an element is a substance that can’t be broken down by non-nuclear reactions.
What is the difference between operational definition and conceptual definition?
1. A conceptual definition describes what a concept means by relating it to other abstract concepts. 2. An operational definition describes a concept in terms of its observable and measurable characteristics or behaviors, by specifying how the concept can be observed in actual practice.
Which one is the operational definition?
► An operational definition is how we (the. researcher) decide to measure our the variables. in our study (variable = anything that can be measured). ◦ There are usually hundreds of ways to measure a DV (e.g. behavior).
What is not an operational definition?
Definition of nonoperational : not operational: such as. a : not functional or ready for use a nonoperational clock. b : not of or relating to the operation of a business or machine Layoffs were limited to nonoperational positions.
What is an operational definition of a variable?
Operational variables (or operationalizing definitions) refer to how you will define and measure a specific variable as it is used in your study. This enables another psychologist to replicate your research and is essential in establishing reliability (achieving consistency in the results).
What is true about the operational definition?
An operational definition, when applied to data collection, is a clear, concise detailed definition of a measure. The need for operational definitions is fundamental when collecting all types of data.
What is the operational definition of memory?
Operational definitions are used to create a clear explanation of a subjective matter, e.g. memory. Memory is an internal process not able to be observed in the physical world. Memory must be studied through recall tests, e.g. how many words a person can recall from a list they are exposed to prior to the test.
What is an operational definition quizlet?
Operational Definition. A statement of the precise meaning of a variable, procedure or concept within a study.
What are the three parts of an operational definition?
Deming, an operational definition has three elements that help you to apply it: Criteria: The standard against which to evaluate the results of the test. Test: A specific procedure for measuring a characteristic. Decision: The determination as to whether the test results show that the characteristic meets the criteria.
What is the limitation of operational definition?
An action required or prohibited by higher authority, such as a constraint or a restraint, and other restrictions that limit the commander.
How do you identify technical and operational definitions?
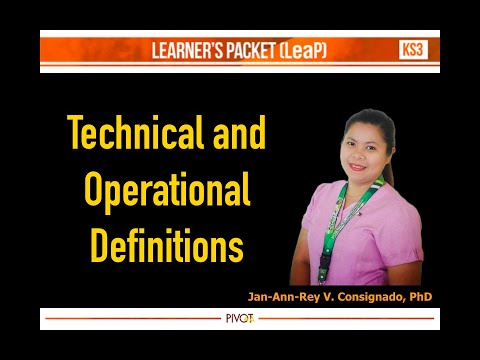
What are the types of definition?
This lesson will introduce you to the three different types of definitions: formal, informal, and extended. Formal Definitions. A formal definition.
What is an operational definition of an independent variable?
An operational definition describes exactly what the independent variable is and how it is measured. Doing this helps ensure that the experiments know exactly what they are looking at or manipulating, allowing them to measure it and determine if it is the IV that is causing changes in the DV.
Why is an operational definition important quizlet?
Operational Definitions are important because. We must be able to clearly define and measure the behaviour during the entire behaviour change process. There must be an agreement on what the target behaviour is.
What is conceptual definition and example?
The definition of conceptual is something having to do with the mind, or with mental concepts or philosophical or imaginary ideas. An example of conceptual is when you formulate an abstract philosophy to explain the world which cannot be proven or seen.
What is the difference between conceptual variable and the operational definition of a variable?
Conceptual variables are about abstract constructs; operational variables (“operational definitions”) are the concrete operations, measures, or procedures used to measure the concept in practice.
What is the operational definition of television?
1 : an electronic system of sending images and sound over a wire or through space by devices that change light and sound into electrical signals and then change these back into light and sound. 2 : a piece of equipment with a screen and speakers that reproduces images and sound.
What is the operational definition of chemical reaction?
A chemical reaction is a process that causes changes in substances, which results in new compounds that have a different chemical identity and properties than before the reaction. The process changes atoms’ electron positions, but do not change its nuclei.
Privacy Overview
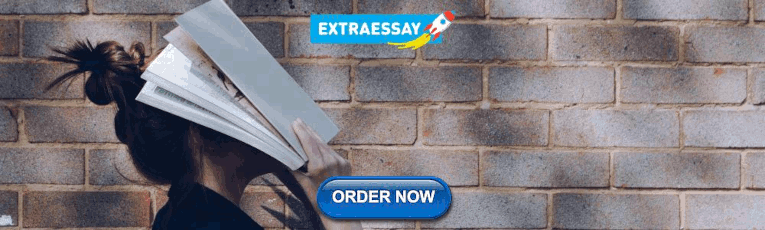
IMAGES
VIDEO
COMMENTS
operational definition a statement that maps one or more empirical measures onto one or more theoretical constructs. (ties the observable to the unobservable. we are interested in the unobservable, but all we have is data which is observable)
a. An operational definition allows others to replicate the procedure. b. An operational definition provides more context and includes many examples of the concept described. c. An operational definition is easier to translate into multiple languages than a dictionary definition. d.
Operational Definitions. An operational definition is a definition of a variable in terms of precisely how it is to be measured. These measures generally fall into one of three broad categories. Self-report measures are those in which participants report on their own thoughts, feelings, and actions, as with the Rosenberg Self-Esteem Scale. Behavioral measures are those in which some other ...
Without transparent and specific operational definitions, researchers may measure irrelevant concepts or inconsistently apply methods. Operationalization reduces subjectivity, minimizes the potential for research bias, and increases the reliability of your study. Your choice of operational definition can sometimes affect your results.
Operationalization involves spelling out precisely how a concept will be measured. Operational definitions must include the variable, the measure, and how you plan to interpret the measure. There are four different levels of measurement: nominal, ordinal, interval, and ratio (in increasing order of specificity).
Operationalization involves spelling out precisely how a concept will be measured. Operational definitions must include the variable, the measure, and how you plan to interpret the measure. There are four different levels of measurement: nominal, ordinal, interval, and ratio (in increasing order of specificity).
Operational Definition. Operational definition is the definition of variables in a psychological study using specific activities or detailed operational procedures. In the psychological experiment, the operational definition of variables makes the research less metaphysical and more concrete, so the final conclusions are verifiable.
Operational Definitions. An essential component of an operational definition is measurement. A simple and accurate definition of. measurement is the assignment of numbers to a variable in which we are interested. These numbers will provide the raw material for our statistical analysis. Measurement is so common and taken for granted that we ...
Example 2.2 (Operational and conceptual definitions) Players and fans have become more aware of concussions and head injuries in sport. A Conference on concussion in sport developed this conceptual definition (McCrory et al. 2013):. Concussion is a brain injury and is defined as a complex pathophysiological process affecting the brain, induced by biomechanical forces.
The numbers that represent observations exist on one of four scales of measurement: nominal, ordinal, interval, or ratio. The scale of measurement is important because it determines the types of mathematical operations and statistical analyses that are appropriate. On a nominal scale of measurement, numbers simply represent labels and have no ...
An operational definition allows the researchers to describe in a specific way what they mean when they use a certain term. Generally, operational definitions are concrete and measurable. Defining variables in this way allows other people to see if the research has validity. Validity here refers to if the researchers are actually measuring what ...
Defining Operationalization. Operationalization is the process of defining abstract concepts in measurable terms, so they can be observed, measured, and analyzed. This process is essential in research because it enables researchers to transform abstract concepts into observable variables with measurable qualities, allowing them to collect data ...
Finding out the indicators for the particular dimension of the concept you want to study. Step 3. Decide on operationalization issues. Based on the indicators, determine what questions to ask, what observations to make, what scores to give, and what attributes to have for the measurement.
Definition of Operational Research. Operational Research, often known as Operations Research (OR) in the United States, is a discipline that applies advanced analytical methods to help make better decisions. It involves the use of mathematical models, statistics, and algorithms to solve complex problems in a wide range of areas, including ...
Quiz your students on Operational and Technical Definition of Terms practice problems using our fun classroom quiz game Quizalize and personalize your teaching. ... So others could be able to assess the validity of the research. To understand how generalize the findings are in a research.
Technical and Operational Definitions quiz for KG students. Find other quizzes for English and more on Quizizz for free! ... The 2 types of definition can be used in research. 4. Multiple Choice. Edit. 30 seconds. 1 pt. The definition of variable in terms of the actual producers used by the researcher to measure.
An operational definition is designed to model or represent a concept or theoretical definition, also known as a construct. Scientists should describe the operations (procedures, actions, or processes) that define the concept with enough specificity such that other investigators can replicate their research. Table of Contents hide. 1.
Conceptual definition and operational definition mean the same thing Some psychological concepts cannot be operationaly defined Conceptual definition and operational definition are completely different and unrelated to each other Question 7 1 pts Dr. Ellison finds a relationship between the amount of sleep and problem solving. Specifically ...