Hungarian Method
The Hungarian method is a computational optimization technique that addresses the assignment problem in polynomial time and foreshadows following primal-dual alternatives. In 1955, Harold Kuhn used the term “Hungarian method” to honour two Hungarian mathematicians, Dénes Kőnig and Jenő Egerváry. Let’s go through the steps of the Hungarian method with the help of a solved example.
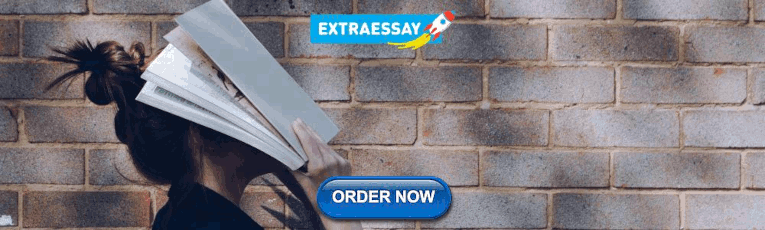
Hungarian Method to Solve Assignment Problems
The Hungarian method is a simple way to solve assignment problems. Let us first discuss the assignment problems before moving on to learning the Hungarian method.
What is an Assignment Problem?
A transportation problem is a type of assignment problem. The goal is to allocate an equal amount of resources to the same number of activities. As a result, the overall cost of allocation is minimised or the total profit is maximised.
Because available resources such as workers, machines, and other resources have varying degrees of efficiency for executing different activities, and hence the cost, profit, or loss of conducting such activities varies.
Assume we have ‘n’ jobs to do on ‘m’ machines (i.e., one job to one machine). Our goal is to assign jobs to machines for the least amount of money possible (or maximum profit). Based on the notion that each machine can accomplish each task, but at variable levels of efficiency.
Hungarian Method Steps
Check to see if the number of rows and columns are equal; if they are, the assignment problem is considered to be balanced. Then go to step 1. If it is not balanced, it should be balanced before the algorithm is applied.
Step 1 – In the given cost matrix, subtract the least cost element of each row from all the entries in that row. Make sure that each row has at least one zero.
Step 2 – In the resultant cost matrix produced in step 1, subtract the least cost element in each column from all the components in that column, ensuring that each column contains at least one zero.
Step 3 – Assign zeros
- Analyse the rows one by one until you find a row with precisely one unmarked zero. Encircle this lonely unmarked zero and assign it a task. All other zeros in the column of this circular zero should be crossed out because they will not be used in any future assignments. Continue in this manner until you’ve gone through all of the rows.
- Examine the columns one by one until you find one with precisely one unmarked zero. Encircle this single unmarked zero and cross any other zero in its row to make an assignment to it. Continue until you’ve gone through all of the columns.
Step 4 – Perform the Optimal Test
- The present assignment is optimal if each row and column has exactly one encircled zero.
- The present assignment is not optimal if at least one row or column is missing an assignment (i.e., if at least one row or column is missing one encircled zero). Continue to step 5. Subtract the least cost element from all the entries in each column of the final cost matrix created in step 1 and ensure that each column has at least one zero.
Step 5 – Draw the least number of straight lines to cover all of the zeros as follows:
(a) Highlight the rows that aren’t assigned.
(b) Label the columns with zeros in marked rows (if they haven’t already been marked).
(c) Highlight the rows that have assignments in indicated columns (if they haven’t previously been marked).
(d) Continue with (b) and (c) until no further marking is needed.
(f) Simply draw the lines through all rows and columns that are not marked. If the number of these lines equals the order of the matrix, then the solution is optimal; otherwise, it is not.
Step 6 – Find the lowest cost factor that is not covered by the straight lines. Subtract this least-cost component from all the uncovered elements and add it to all the elements that are at the intersection of these straight lines, but leave the rest of the elements alone.
Step 7 – Continue with steps 1 – 6 until you’ve found the highest suitable assignment.
Hungarian Method Example
Use the Hungarian method to solve the given assignment problem stated in the table. The entries in the matrix represent each man’s processing time in hours.
\(\begin{array}{l}\begin{bmatrix} & I & II & III & IV & V \\1 & 20 & 15 & 18 & 20 & 25 \\2 & 18 & 20 & 12 & 14 & 15 \\3 & 21 & 23 & 25 & 27 & 25 \\4 & 17 & 18 & 21 & 23 & 20 \\5 & 18 & 18 & 16 & 19 & 20 \\\end{bmatrix}\end{array} \)
With 5 jobs and 5 men, the stated problem is balanced.
\(\begin{array}{l}A = \begin{bmatrix}20 & 15 & 18 & 20 & 25 \\18 & 20 & 12 & 14 & 15 \\21 & 23 & 25 & 27 & 25 \\17 & 18 & 21 & 23 & 20 \\18 & 18 & 16 & 19 & 20 \\\end{bmatrix}\end{array} \)
Subtract the lowest cost element in each row from all of the elements in the given cost matrix’s row. Make sure that each row has at least one zero.
\(\begin{array}{l}A = \begin{bmatrix}5 & 0 & 3 & 5 & 10 \\6 & 8 & 0 & 2 & 3 \\0 & 2 & 4 & 6 & 4 \\0 & 1 & 4 & 6 & 3 \\2 & 2 & 0 & 3 & 4 \\\end{bmatrix}\end{array} \)
Subtract the least cost element in each Column from all of the components in the given cost matrix’s Column. Check to see if each column has at least one zero.
\(\begin{array}{l}A = \begin{bmatrix}5 & 0 & 3 & 3 & 7 \\6 & 8 & 0 & 0 & 0 \\0 & 2 & 4 & 4 & 1 \\0 & 1 & 4 & 4 & 0 \\2 & 2 & 0 & 1 & 1 \\\end{bmatrix}\end{array} \)
When the zeros are assigned, we get the following:

The present assignment is optimal because each row and column contain precisely one encircled zero.
Where 1 to II, 2 to IV, 3 to I, 4 to V, and 5 to III are the best assignments.
Hence, z = 15 + 14 + 21 + 20 + 16 = 86 hours is the optimal time.
Practice Question on Hungarian Method
Use the Hungarian method to solve the following assignment problem shown in table. The matrix entries represent the time it takes for each job to be processed by each machine in hours.
\(\begin{array}{l}\begin{bmatrix}J/M & I & II & III & IV & V \\1 & 9 & 22 & 58 & 11 & 19 \\2 & 43 & 78 & 72 & 50 & 63 \\3 & 41 & 28 & 91 & 37 & 45 \\4 & 74 & 42 & 27 & 49 & 39 \\5 & 36 & 11 & 57 & 22 & 25 \\\end{bmatrix}\end{array} \)
Stay tuned to BYJU’S – The Learning App and download the app to explore all Maths-related topics.
Frequently Asked Questions on Hungarian Method
What is hungarian method.
The Hungarian method is defined as a combinatorial optimization technique that solves the assignment problems in polynomial time and foreshadowed subsequent primal–dual approaches.
What are the steps involved in Hungarian method?
The following is a quick overview of the Hungarian method: Step 1: Subtract the row minima. Step 2: Subtract the column minimums. Step 3: Use a limited number of lines to cover all zeros. Step 4: Add some more zeros to the equation.
What is the purpose of the Hungarian method?
When workers are assigned to certain activities based on cost, the Hungarian method is beneficial for identifying minimum costs.
Leave a Comment Cancel reply
Your Mobile number and Email id will not be published. Required fields are marked *
Request OTP on Voice Call
Post My Comment

- Share Share
Register with BYJU'S & Download Free PDFs
Register with byju's & watch live videos.

Quadratic assignment problem
Author: Thomas Kueny, Eric Miller, Natasha Rice, Joseph Szczerba, David Wittmann (SysEn 5800 Fall 2020)
- 1 Introduction
- 2.1 Koopmans-Beckman Mathematical Formulation
- 2.2.1 Parameters
- 2.3.1 Optimization Problem
- 2.4 Computational Complexity
- 2.5 Algorithmic Discussions
- 2.6 Branch and Bound Procedures
- 2.7 Linearizations
- 3.1 QAP with 3 Facilities
- 4.1 Inter-plant Transportation Problem
- 4.2 The Backboard Wiring Problem
- 4.3 Hospital Layout
- 4.4 Exam Scheduling System
- 5 Conclusion
- 6 References
Introduction
The Quadratic Assignment Problem (QAP), discovered by Koopmans and Beckmann in 1957 [1] , is a mathematical optimization module created to describe the location of invisible economic activities. An NP-Complete problem, this model can be applied to many other optimization problems outside of the field of economics. It has been used to optimize backboards, inter-plant transportation, hospital transportation, exam scheduling, along with many other applications not described within this page.
Theory, Methodology, and/or Algorithmic Discussions
Koopmans-beckman mathematical formulation.
Economists Koopmans and Beckman began their investigation of the QAP to ascertain the optimal method of locating important economic resources in a given area. The Koopmans-Beckman formulation of the QAP aims to achieve the objective of assigning facilities to locations in order to minimize the overall cost. Below is the Koopmans-Beckman formulation of the QAP as described by neos-guide.org.
Quadratic Assignment Problem Formulation
Inner Product
Note: The true objective cost function only requires summing entries above the diagonal in the matrix comprised of elements
Since this matrix is symmetric with zeroes on the diagonal, dividing by 2 removes the double count of each element to give the correct cost value. See the Numerical Example section for an example of this note.
Optimization Problem
With all of this information, the QAP can be summarized as:
Computational Complexity
QAP belongs to the classification of problems known as NP-complete, thus being a computationally complex problem. QAP’s NP-completeness was proven by Sahni and Gonzalez in 1976, who states that of all combinatorial optimization problems, QAP is the “hardest of the hard”. [2]
Algorithmic Discussions
While an algorithm that can solve QAP in polynomial time is unlikely to exist, there are three primary methods for acquiring the optimal solution to a QAP problem:
- Dynamic Program
- Cutting Plane
Branch and Bound Procedures
The third method has been proven to be the most effective in solving QAP, although when n > 15, QAP begins to become virtually unsolvable.
The Branch and Bound method was first proposed by Ailsa Land and Alison Doig in 1960 and is the most commonly used tool for solving NP-hard optimization problems.
A branch-and-bound algorithm consists of a systematic enumeration of candidate solutions by means of state space search: the set of candidate solutions is thought of as forming a rooted tree with the full set at the root. The algorithm explores branches of this tree, which represent subsets of the solution set. Before one lists all of the candidate solutions of a branch, the branch is checked against upper and lower estimated bounds on the optimal solution, and the branch is eliminated if it cannot produce a better solution than the best one found so far by the algorithm.
Linearizations
The first attempts to solve the QAP eliminated the quadratic term in the objective function of
in order to transform the problem into a (mixed) 0-1 linear program. The objective function is usually linearized by introducing new variables and new linear (and binary) constraints. Then existing methods for (mixed) linear integer programming (MILP) can be applied. The very large number of new variables and constraints, however, usually poses an obstacle for efficiently solving the resulting linear integer programs. MILP formulations provide LP relaxations of the problem which can be used to compute lower bounds.
Numerical Example
Qap with 3 facilities.
Applications
Inter-plant transportation problem.
The QAP was first introduced by Koopmans and Beckmann to address how economic decisions could be made to optimize the transportation costs of goods between both manufacturing plants and locations. [1] Factoring in the location of each of the manufacturing plants as well as the volume of goods between locations to maximize revenue is what distinguishes this from other linear programming assignment problems like the Knapsack Problem.
The Backboard Wiring Problem
As the QAP is focused on minimizing the cost of traveling from one location to another, it is an ideal approach to determining the placement of components in many modern electronics. Leon Steinberg proposed a QAP solution to optimize the layout of elements on a blackboard by minimizing the total amount of wiring required. [4]
When defining the problem Steinberg states that we have a set of n elements
as well as a set of r points
In his paper he derives the below formula:
In his paper Steinberg a backboard with a 9 by 4 array, allowing for 36 potential positions for the 34 components that needed to be placed on the backboard. For the calculation, he selected a random initial placement of s1 and chose a random family of 25 unconnected sets.
The initial placement of components is shown below:
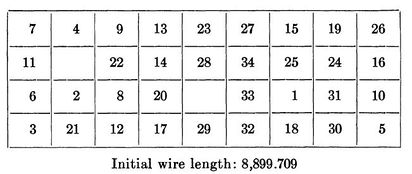
After the initial placement of elements, it took an additional 35 iterations to get us to our final optimized backboard layout. Leading to a total of 59 iterations and a final wire length of 4,969.440.
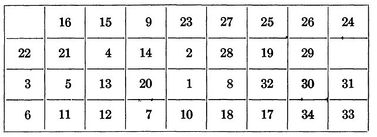
Hospital Layout
Building new hospitals was a common event in 1977 when Alealid N Elshafei wrote his paper on "Hospital Layouts as a Quadratic Assignment Problem". [5] With the high initial cost to construct the hospital and to staff it, it is important to ensure that it is operating as efficiently as possible. Elshafei's paper was commissioned to create an optimization formula to locate clinics within a building in such a way that minimizes the total distance that a patient travels within the hospital throughout the year. When doing a study of a major hospital in Cairo he determined that the Outpatient ward was acting as a bottleneck in the hospital and focused his efforts on optimizing the 17 departments there.
Elshafei identified the following QAP to determine where clinics should be placed:
For the Cairo hospital with 17 clinics, and one receiving and recording room bringing us to a total of 18 facilities. By running the above optimization Elshafei was able to get the total distance per year down to 11,281,887 from a distance of 13,973,298 based on the original hospital layout.
Exam Scheduling System
The scheduling system uses matrices for Exams, Time Slots, and Rooms with the goal of reducing the rate of schedule conflicts. To accomplish this goal, the “examination with the highest cross faculty student is been prioritized in the schedule after which the examination with the highest number of cross-program is considered and finally with the highest number of repeating student, at each stage group with the highest number of student are prioritized.” [6]
- ↑ 1.0 1.1 1.2 Koopmans, T., & Beckmann, M. (1957). Assignment Problems and the Location of Economic Activities. Econometrica, 25(1), 53-76. doi:10.2307/1907742
- ↑ 2.0 2.1 Quadratic Assignment Problem. (2020). Retrieved December 14, 2020, from https://neos-guide.org/content/quadratic-assignment-problem
- ↑ 3.0 3.1 3.2 Burkard, R. E., Çela, E., Pardalos, P. M., & Pitsoulis, L. S. (2013). The Quadratic Assignment Problem. https://www.opt.math.tugraz.at/~cela/papers/qap_bericht.pdf .
- ↑ 4.0 4.1 Leon Steinberg. The Backboard Wiring Problem: A Placement Algorithm. SIAM Review . 1961;3(1):37.
- ↑ 5.0 5.1 Alwalid N. Elshafei. Hospital Layout as a Quadratic Assignment Problem. Operational Research Quarterly (1970-1977) . 1977;28(1):167. doi:10.2307/300878
- ↑ 6.0 6.1 Muktar, D., & Ahmad, Z.M. (2014). Examination Scheduling System Based On Quadratic Assignment.
Navigation menu

How to Solve the Assignment Problem: A Complete Guide
Table of Contents
Assignment problem is a special type of linear programming problem that deals with assigning a number of resources to an equal number of tasks in the most efficient way. The goal is to minimize the total cost of assignments while ensuring that each task is assigned to only one resource and each resource is assigned to only one task. In this blog, we will discuss the solution of the assignment problem using the Hungarian method, which is a popular algorithm for solving the problem.
Understanding the Assignment Problem
Before we dive into the solution, it is important to understand the problem itself. In the assignment problem, we have a matrix of costs, where each row represents a resource and each column represents a task. The objective is to assign each resource to a task in such a way that the total cost of assignments is minimized. However, there are certain constraints that need to be satisfied – each resource can be assigned to only one task and each task can be assigned to only one resource.
Solving the Assignment Problem
There are various methods for solving the assignment problem, including the Hungarian method, the brute force method, and the auction algorithm. Here, we will focus on the steps involved in solving the assignment problem using the Hungarian method, which is the most commonly used and efficient method.
Step 1: Set up the cost matrix
The first step in solving the assignment problem is to set up the cost matrix, which represents the cost of assigning a task to an agent. The matrix should be square and have the same number of rows and columns as the number of tasks and agents, respectively.
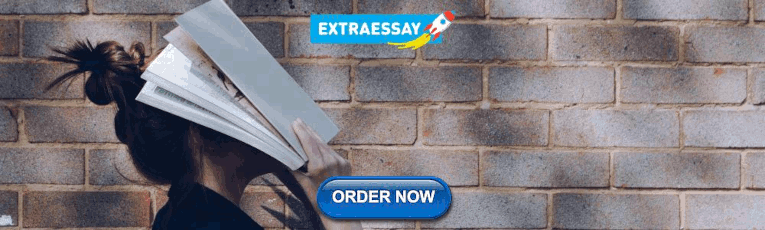
Step 2: Subtract the smallest element from each row and column
To simplify the calculations, we need to reduce the size of the cost matrix by subtracting the smallest element from each row and column. This step is called matrix reduction.
Step 3: Cover all zeros with the minimum number of lines
The next step is to cover all zeros in the matrix with the minimum number of horizontal and vertical lines. This step is called matrix covering.
Step 4: Test for optimality and adjust the matrix
To test for optimality, we need to calculate the minimum number of lines required to cover all zeros in the matrix. If the number of lines equals the number of rows or columns, the solution is optimal. If not, we need to adjust the matrix and repeat steps 3 and 4 until we get an optimal solution.
Step 5: Assign the tasks to the agents
The final step is to assign the tasks to the agents based on the optimal solution obtained in step 4. This will give us the most cost-effective or profit-maximizing assignment.
Solution of the Assignment Problem using the Hungarian Method
The Hungarian method is an algorithm that uses a step-by-step approach to find the optimal assignment. The algorithm consists of the following steps:
- Subtract the smallest entry in each row from all the entries of the row.
- Subtract the smallest entry in each column from all the entries of the column.
- Draw the minimum number of lines to cover all zeros in the matrix. If the number of lines drawn is equal to the number of rows, we have an optimal solution. If not, go to step 4.
- Determine the smallest entry not covered by any line. Subtract it from all uncovered entries and add it to all entries covered by two lines. Go to step 3.
The above steps are repeated until an optimal solution is obtained. The optimal solution will have all zeros covered by the minimum number of lines. The assignments can be made by selecting the rows and columns with a single zero in the final matrix.
Applications of the Assignment Problem
The assignment problem has various applications in different fields, including computer science, economics, logistics, and management. In this section, we will provide some examples of how the assignment problem is used in real-life situations.
Applications in Computer Science
The assignment problem can be used in computer science to allocate resources to different tasks, such as allocating memory to processes or assigning threads to processors.
Applications in Economics
The assignment problem can be used in economics to allocate resources to different agents, such as allocating workers to jobs or assigning projects to contractors.
Applications in Logistics
The assignment problem can be used in logistics to allocate resources to different activities, such as allocating vehicles to routes or assigning warehouses to customers.
Applications in Management
The assignment problem can be used in management to allocate resources to different projects, such as allocating employees to tasks or assigning budgets to departments.
Let’s consider the following scenario: a manager needs to assign three employees to three different tasks. Each employee has different skills, and each task requires specific skills. The manager wants to minimize the total time it takes to complete all the tasks. The skills and the time required for each task are given in the table below:
The assignment problem is to determine which employee should be assigned to which task to minimize the total time required. To solve this problem, we can use the Hungarian method, which we discussed in the previous blog.
Using the Hungarian method, we first subtract the smallest entry in each row from all the entries of the row:
Next, we subtract the smallest entry in each column from all the entries of the column:
We draw the minimum number of lines to cover all the zeros in the matrix, which in this case is three:
Since the number of lines is equal to the number of rows, we have an optimal solution. The assignments can be made by selecting the rows and columns with a single zero in the final matrix. In this case, the optimal assignments are:
- Emp 1 to Task 3
- Emp 2 to Task 2
- Emp 3 to Task 1
This assignment results in a total time of 9 units.
I hope this example helps you better understand the assignment problem and how to solve it using the Hungarian method.
Solving the assignment problem may seem daunting, but with the right approach, it can be a straightforward process. By following the steps outlined in this guide, you can confidently tackle any assignment problem that comes your way.
How useful was this post?
Click on a star to rate it!
Average rating 0 / 5. Vote count: 0
No votes so far! Be the first to rate this post.
We are sorry that this post was not useful for you! 😔
Let us improve this post!
Tell us how we can improve this post?
Operations Research
1 Operations Research-An Overview
- History of O.R.
- Approach, Techniques and Tools
- Phases and Processes of O.R. Study
- Typical Applications of O.R
- Limitations of Operations Research
- Models in Operations Research
- O.R. in real world
2 Linear Programming: Formulation and Graphical Method
- General formulation of Linear Programming Problem
- Optimisation Models
- Basics of Graphic Method
- Important steps to draw graph
- Multiple, Unbounded Solution and Infeasible Problems
- Solving Linear Programming Graphically Using Computer
- Application of Linear Programming in Business and Industry
3 Linear Programming-Simplex Method
- Principle of Simplex Method
- Computational aspect of Simplex Method
- Simplex Method with several Decision Variables
- Two Phase and M-method
- Multiple Solution, Unbounded Solution and Infeasible Problem
- Sensitivity Analysis
- Dual Linear Programming Problem
4 Transportation Problem
- Basic Feasible Solution of a Transportation Problem
- Modified Distribution Method
- Stepping Stone Method
- Unbalanced Transportation Problem
- Degenerate Transportation Problem
- Transhipment Problem
- Maximisation in a Transportation Problem
5 Assignment Problem
- Solution of the Assignment Problem
- Unbalanced Assignment Problem
- Problem with some Infeasible Assignments
- Maximisation in an Assignment Problem
- Crew Assignment Problem
6 Application of Excel Solver to Solve LPP
- Building Excel model for solving LP: An Illustrative Example
7 Goal Programming
- Concepts of goal programming
- Goal programming model formulation
- Graphical method of goal programming
- The simplex method of goal programming
- Using Excel Solver to Solve Goal Programming Models
- Application areas of goal programming
8 Integer Programming
- Some Integer Programming Formulation Techniques
- Binary Representation of General Integer Variables
- Unimodularity
- Cutting Plane Method
- Branch and Bound Method
- Solver Solution
9 Dynamic Programming
- Dynamic Programming Methodology: An Example
- Definitions and Notations
- Dynamic Programming Applications
10 Non-Linear Programming
- Solution of a Non-linear Programming Problem
- Convex and Concave Functions
- Kuhn-Tucker Conditions for Constrained Optimisation
- Quadratic Programming
- Separable Programming
- NLP Models with Solver
11 Introduction to game theory and its Applications
- Important terms in Game Theory
- Saddle points
- Mixed strategies: Games without saddle points
- 2 x n games
- Exploiting an opponent’s mistakes
12 Monte Carlo Simulation
- Reasons for using simulation
- Monte Carlo simulation
- Limitations of simulation
- Steps in the simulation process
- Some practical applications of simulation
- Two typical examples of hand-computed simulation
- Computer simulation
13 Queueing Models
- Characteristics of a queueing model
- Notations and Symbols
- Statistical methods in queueing
- The M/M/I System
- The M/M/C System
- The M/Ek/I System
- Decision problems in queueing
- Data Structures
- Linked List
- Binary Tree
- Binary Search Tree
- Segment Tree
- Disjoint Set Union
- Fenwick Tree
- Red-Black Tree
- Advanced Data Structures
Hungarian Algorithm for Assignment Problem | Set 2 (Implementation)
- Hungarian Algorithm for Assignment Problem | Set 1 (Introduction)
- Implementation of Exhaustive Search Algorithm for Set Packing
- Greedy Approximate Algorithm for Set Cover Problem
- Introduction to Exact Cover Problem and Algorithm X
- Job Assignment Problem using Branch And Bound
- Prim's Algorithm (Simple Implementation for Adjacency Matrix Representation)
- Introduction to Disjoint Set (Union-Find Algorithm)
- Channel Assignment Problem
- Java Program for Counting sets of 1s and 0s in a binary matrix
- Top 20 Greedy Algorithms Interview Questions
- C++ Program for Counting sets of 1s and 0s in a binary matrix
- C# Program for Dijkstra's shortest path algorithm | Greedy Algo-7
- Java Program for Dijkstra's shortest path algorithm | Greedy Algo-7
- C / C++ Program for Dijkstra's shortest path algorithm | Greedy Algo-7
- Self assignment check in assignment operator
- Python Program for Dijkstra's shortest path algorithm | Greedy Algo-7
- Algorithms | Dynamic Programming | Question 7
- Assignment Operators in C
- Assignment Operators in Programming
- Merge Sort - Data Structure and Algorithms Tutorials
- Must Do Coding Questions for Companies like Amazon, Microsoft, Adobe, ...
- QuickSort - Data Structure and Algorithm Tutorials
- Bubble Sort - Data Structure and Algorithm Tutorials
- Tree Traversal Techniques - Data Structure and Algorithm Tutorials
- Binary Search - Data Structure and Algorithm Tutorials
- Insertion Sort - Data Structure and Algorithm Tutorials
- Selection Sort – Data Structure and Algorithm Tutorials
- Understanding the basics of Linked List
- Breadth First Search or BFS for a Graph
Given a 2D array , arr of size N*N where arr[i][j] denotes the cost to complete the j th job by the i th worker. Any worker can be assigned to perform any job. The task is to assign the jobs such that exactly one worker can perform exactly one job in such a way that the total cost of the assignment is minimized.
Input: arr[][] = {{3, 5}, {10, 1}} Output: 4 Explanation: The optimal assignment is to assign job 1 to the 1st worker, job 2 to the 2nd worker. Hence, the optimal cost is 3 + 1 = 4. Input: arr[][] = {{2500, 4000, 3500}, {4000, 6000, 3500}, {2000, 4000, 2500}} Output: 4 Explanation: The optimal assignment is to assign job 2 to the 1st worker, job 3 to the 2nd worker and job 1 to the 3rd worker. Hence, the optimal cost is 4000 + 3500 + 2000 = 9500.
Different approaches to solve this problem are discussed in this article .
Approach: The idea is to use the Hungarian Algorithm to solve this problem. The algorithm is as follows:
- For each row of the matrix, find the smallest element and subtract it from every element in its row.
- Repeat the step 1 for all columns.
- Cover all zeros in the matrix using the minimum number of horizontal and vertical lines.
- Test for Optimality : If the minimum number of covering lines is N , an optimal assignment is possible. Else if lines are lesser than N , an optimal assignment is not found and must proceed to step 5.
- Determine the smallest entry not covered by any line. Subtract this entry from each uncovered row, and then add it to each covered column. Return to step 3.
Consider an example to understand the approach:
Let the 2D array be: 2500 4000 3500 4000 6000 3500 2000 4000 2500 Step 1: Subtract minimum of every row. 2500, 3500 and 2000 are subtracted from rows 1, 2 and 3 respectively. 0 1500 1000 500 2500 0 0 2000 500 Step 2: Subtract minimum of every column. 0, 1500 and 0 are subtracted from columns 1, 2 and 3 respectively. 0 0 1000 500 1000 0 0 500 500 Step 3: Cover all zeroes with minimum number of horizontal and vertical lines. Step 4: Since we need 3 lines to cover all zeroes, the optimal assignment is found. 2500 4000 3500 4000 6000 3500 2000 4000 2500 So the optimal cost is 4000 + 3500 + 2000 = 9500
For implementing the above algorithm, the idea is to use the max_cost_assignment() function defined in the dlib library . This function is an implementation of the Hungarian algorithm (also known as the Kuhn-Munkres algorithm) which runs in O(N 3 ) time. It solves the optimal assignment problem.
Below is the implementation of the above approach:
Time Complexity: O(N 3 ) Auxiliary Space: O(N 2 )
Please Login to comment...
Similar reads.
- Mathematical
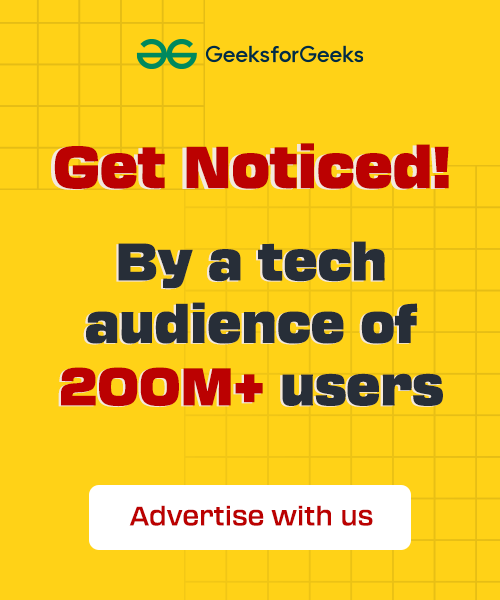
Improve your Coding Skills with Practice
What kind of Experience do you want to share?
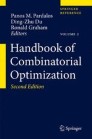
Handbook of Combinatorial Optimization pp 2741–2814 Cite as
Quadratic Assignment Problems
- Rainer E. burkard 4
- Reference work entry
- First Online: 01 January 2013
8131 Accesses
33 Citations
This chapter introduces quadratic assignment problems (QAP) as models for finding an optimal assignment among two sets of interrelated objects. References to many applications of QAPs, like in location theory, are given. Moreover, several classical combinatorial optimization problems are identified as special cases of the QAP, like the traveling salesman problem, graph partitioning, max clique problem, minimum-weight feedback arc set problem, (FASP) and packing problems in graphs. These subproblems show that the QAP is \(\mathcal{N}\mathcal{P}\) -hard.Several different formulations of the QAP are presented in detail as being basic for different solution approaches. Special emphasis is laid to the different possibilities for describing QAPs as (mixed-)integer linear programs. Moreover, QAP polyhedra are discussed.Crucial for exact solution approaches are lower bounding procedures. The paradigm of admissible transformations is introduced for describing several variants of Gilmore–Lawler type bounds. Moreover, bounds based on eigenvalues and bounds found by semidefinite programming are discussed in detail. Among exact solution methods, special emphasis is laid on branch-and-bound approaches. With respect to heuristics, not only simple construction heuristics and improvement heuristics are described, but also several metaheuristics like simulated annealing, tabu search, greedy randomized search, genetic algorithms, and ant systems are discussed. Links to available computer programs are delivered.As QAPs are \(\mathcal{N}\mathcal{P}\) -hard problems, there is a special interest in polynomially solvable special cases. A comprehensive survey of results in this direction is given. Moreover, QAPs show an interesting asymptotic behavior, as the ratio of best and worst solution value tends to one in probability. This phenomenon is thoroughly discussed and implications of this strange behavior are shown.Finally, related problems are shortly treated. So, the quadratic bottleneck assignment problem is introduced and lower bounds, efficiently solvable special cases, as well as asymptotic results are reported. Moreover, cubic assignment problems, biquadratic assignment problems, and the quadratic semi-assignment problem are surveyed. An extensive bibliography provides the most important links to the literature in this field.
This is a preview of subscription content, log in via an institution .
Buying options
- Available as PDF
- Read on any device
- Instant download
- Own it forever
- Available as EPUB and PDF
- Durable hardcover edition
- Dispatched in 3 to 5 business days
- Free shipping worldwide - see info
Tax calculation will be finalised at checkout
Purchases are for personal use only
Recommended Reading
E.H.L. Aarts, J. Korst, Simulated Annealing and Boltzmann Machines: A Stochastic Approach to Combinatorial Optimization and Neural Computing (Wiley, Chichester, 1989)
MATH Google Scholar
E. Aarts, J.K. Lenstra (eds.), Local Search in Combinatorial Optimization (Wiley, Chichester, 1997)
W.P. Adams, M. Guignard, P.M. Hahn, W.L. Hightower, A level-2 reformulation-linearization technique bound for the quadratic assignment problem. Eur. J. Oper. Res. 180 , 983–996 (2007)
MathSciNet MATH Google Scholar
W.P. Adams, T.A. Johnson, Improved linear programming-based lower bounds for the quadratic assignment problem, in Quadratic Assignment and Related Problems , ed. by P.M. Pardalos, H. Wolkowicz. DIMACS Series on Discrete Mathematics and Theoretical Computer Science, vol. 16 (AMS, Providence, 1994), pp. 43–75
Google Scholar
W.P. Adams, H.D. Sherali, A tight linearization and an algorithm for zero-one quadratic programming problems. Manag. Sci. 32 , 1274–1290 (1986)
W.P. Adams, H.D. Sherali, Linearization strategies for a class of zero-one mixed integer programming problems. Oper. Res. 38 , 217–226 (1990)
R.K. Ahuja, K.C. Jha, J.B. Orlin, D. Sharma, Very large-scale neighborhood search for the quadratic assignment problem. INFORMS J. Comput. 19 , 646–657 (2007)
R.K. Ahuja, J.B. Orlin, A. Tivari, A greedy genetic algorithm for the quadratic assignment problem. Working paper 3826-95, Sloan School of Management, MIT, 1995
H. Albrecher, A note on the asymptotic behaviour of bottleneck problems. Oper. Res. Lett. 33 , 183–186 (2005)
H. Albrecher, R.E. Burkard, E. Çela, An asymptotical study of combinatorial optimization problems by means of statistical mechanics. J. Comput. Appl. Math. 186 , 148–162 (2006)
K.M. Anstreicher, Eigenvalue bounds versus semidefinite relaxations for the quadratic assignment problem. SIAM J. Optim. 11 , 254–265 (2000)
K.M. Anstreicher, Recent advances in the solution of quadratic assignment problems. Math. Program. 97 , 27–42 (2003)
K.M. Anstreicher, N.W. Brixius, A new bound for the quadratic assignment problem based on convex quadratic programming. Math. Program. 89 , 341–357 (2001)
K.M. Anstreicher, N.W. Brixius, J.P. Goux, J. Linderoth, Solving large quadratic assignment problems on computational grids. Math. Program. 91 , 563–588 (2002)
E.M. Arkin, R. Hassin, M. Sviridenko, Approximating the maximum quadratic assignment problem. Inform. Process. Lett. 77 , 13–16 (2001)
S. Arora, A. Frieze, H. Kaplan, A new rounding procedure for the assignment problem with applications to dense graph arrangement problems, in Proceedings of the 37-th Annual IEEE Symposium on Foundations of Computer Science (FOCS) , 1996, pp. 21–30
A.A. Assad, W. Xu, On lower bounds for a class of quadratic 0–1 programs. Oper. Res. Lett. 4 , 175–180 (1985)
E. Balas, J.B. Mazzola, Nonlinear programming: I. Linearization techniques. Math. Program. 30 , 1–21 (1984)
E. Balas, J.B. Mazzola, Nonlinear programming: II. Dominance relations and algorithms. Math. Program. 30 , 22–45 (1984)
A.I. Barvinok, Computational complexity of orbits in representations of symmetric groups. Adv. Soviet Math. 9 , 161–182 (1992)
MathSciNet Google Scholar
R. Battiti, G. Tecchiolli, The reactive tabu search. ORSA J. Comput. 6 , 126–140 (1994)
M. Bayat, M. Sedghi, Quadratic assignment problems, in Facility Location , ed. by R.Z. Farahani, M. Hekmatfar (Springer, Berlin, 2010), pp. 111–143
M.S. Bazaraa, O. Kirca, Branch and bound based heuristics for solving the quadratic assignment problem. Naval Res. Logist. Q. 30 , 287–304 (1983)
M.S. Bazaraa, H.D. Sherali, Benders’ partitioning scheme applied to a new formulation of the quadratic assignment problem. Naval Res. Logist. Q. 27 , 29–41 (1980)
M.S. Bazaraa, H.D. Sherali, On the use of exact and heuristic cutting plane methods for the quadratic assignment problem. J. Oper. Res. Soc. 33 , 991–1003 (1982)
A. Billionet, S. Elloumi, Best reduction of the quadratic semi-assignment problem. Discrete Appl. Math. 109 , 197–213 (2001)
G. Birkhoff, Tres observaciones sobre el algebra lineal. Univ. Nac. Tucumán Rev. Ser. A, 5 , 147–151 (1946)
A. Blanchard, S. Elloumi, A. Faye, M. Wicker, Un algorithme de génération de coupes pour le problème de l’affectation quadratique. INFOR 41 , 35–49 (2003)
S.H. Bokhari, A shortest tree algorithm for optimal assignments across space and time in a distributed processor system. IEEE Trans. Softw. Eng. 7 , 583–589 (1981)
B. Bollobás, Extremal Graph Theory (Academic, London, 1978)
A.A. Bolotnikov, On the best balance of the disk with masses on its periphery. Problemi Mashinostroenia 6 , 68–74 (1978) (in Russian)
E. Bonomi, J. Lutton, The asymptotic behavior of quadratic sum assignment problems: A statistical mechanics approach. Eur. J. Oper. Res. 26 , 295–300 (1986)
A. Bruengger, J. Clausen, A. Marzetta, M. Perregaard, Joining forces in solving large-scale quadratic assignment problems in parallel, in Proceedings of the 11-th IEEE International Parallel Processing Symposium (IPPS) , Geneva, Switzerland, 1997, pp. 418–427
E.S. Buffa, G.C. Armour, T.E. Vollmann, Allocating facilities with CRAFT. Harv. Bus. Rev. 42 , 136–158 (1962)
S. Burer, D. Vandebussche, Solving lift-and-project relaxations of binary integer programs. SIAM J. Optim. 16 , 726–750 (2006)
R.E. Burkard, Die Störungsmethode zur Lösung quadratischer Zuordnungsprobleme. Oper. Res. Verfahren 16 , 84–108 (1973)
R.E. Burkard, Quadratische Bottleneckprobleme. Oper. Res. Verfahren 18 , 26–41 (1974)
R.E. Burkard, Locations with spatial interactions: the quadratic assignment problem, in Discrete Location Theory , ed. by P.B. Mirchandani, R.L. Francis (Wiley, 1991)
R.E. Burkard, Admissible transformations and assignment problems. Vietnam J. Math. 35 , 373–386 (2007)
R.E. Burkard, T. Bönniger, A heuristic for quadratic boolean programs with applications to quadratic assignment problems. Eur. J. Oper. Res. 13 , 374–386 (1983)
R.E. Burkard, E. Çela, Heuristics for biquadratic assignment problems and their computational comparison. Eur. J. Oper. Res. 83 , 283–300 (1995)
R.E. Burkard, E. Çela, V.M. Demidenko, N.N. Metelski, G.J. Woeginger, Perspectives of easy and hard cases of the quadratic assignment problems. SFB Report 104, Institute of Mathematics, Technical University Graz, Austria, 1997
R.E. Burkard, E. Çela, V.M. Demidenko, N.N. Metelski, G.J. Woeginger, A unified approach to simple special cases of extremal permutation. Optimization 44 , 123–138 (1998)
R.E. Burkard, E. Çela, B. Klinz, On the biquadratic assignment problem, in Quadratic Assignment and Related Problems , ed. by P.M. Pardalos, H. Wolkowicz. DIMACS Series on Discrete Mathematics and Theoretical Computer Science, vol. 16 (AMS, Providence, 1994), pp. 117–146
R.E. Burkard, E. Çela, G. Rote, G.J. Woeginger, The quadratic assignment problem with an Anti-Monge and a Toeplitz matrix: easy and hard cases. Math. Program. B 82 , 125–158 (1998)
R.E. Burkard, M. Dell’Amico, S. Martello, Assignment Problems (SIAM, Philadelphia, 2009). ISBN 978-0-898716-63-4, revised reprint 2012, ISBN 978-1-611972-22-1
R.E. Burkard, U. Derigs, Assignment and Matching Problems: Solution Methods with Fortran Programs . Lecture Notes in Economics and Mathematical Systems, vol. 184 (Springer, Berlin, 1980)
R.E. Burkard, U. Fincke, On random quadratic bottleneck assignment problems. Math. Program. 23 , 227–232 (1982)
R.E. Burkard, U. Fincke, The asymptotic probabilistic behavior of the quadratic sum assignment problem. Z. Oper. Res. 27 , 73–81 (1983)
R.E. Burkard, U. Fincke, Probabilistic asymptotic properties of some combinatorial optimization problems. Discrete Appl. Math. 12 , 21–29 (1985)
R.E. Burkard, W. Hahn, U. Zimmermann, An algebraic approach to assignment problems. Math. Program. 12 , 318–327 (1977)
R.E. Burkard, S.E. Karisch, F. Rendl, QAPLIB—a quadratic assignment problem library. J. Global Optim. 10 , 391–403 (1997). An on-line version is available via World Wide Web at the following URL: http://www.seas.upenn.edu/qaplib/
R.E. Burkard, B. Klinz, R. Rudolf, Perspectives of Monge properties in optimization. Discrete Appl. Math. 70 , 95–161 (1996)
R.E. Burkard, J. Offermann, Entwurf von Schreibmaschinentastaturen mittels quadratischer Zuordnungsprobleme. Z. Oper. Res. 21 , B121–B132 (1977) (in German)
R.E. Burkard, F. Rendl, A thermodynamically motivated simulation procedure for combinatorial optimization problems. Eur. J. Oper. Res. 17 , 169–174 (1984)
R.E. Burkard, R. Rissner, Polynomially solvable special cases of the quadratic bottleneck assignment problem. J. Combin. Optim. 22 , 845–856 (2011)
R.E. Burkard, U. Zimmermann, Combinatorial optimization in linearly ordered semimodules: a survey, in Modern Applied Mathematics , ed. by B. Korte (North Holland, Amsterdam, 1982), pp. 392–436
P. Carraresi, F. Malucelli, A new lower bound for the quadratic assignment problem. Oper. Res. 40 (Suppl. 1), S22–S27 (1992)
P. Carraresi, F. Malucelli, A reformulation scheme and new lower bounds for the QAP, in Quadratic Assignment and Related Problems , ed. by P. Pardalos, H. Wolkowicz. DIMACS Series in Discrete Mathematics and Theoretical Computer Science, vol. 16 (AMS, Providence, RI, 1994), 147–160
E. Çela, The Quadratic Assignment Problem: Theory and Algorithms (Kluwer Academic, Dordrecht, 1998)
E. Çela, N.S. Schmuck, S. Wimer, G.J. Woeginger, The Wiener maximum quadratic assignment problem. J. Discrete Optim. 8 , 411–416 (2011)
V. Černý, Thermodynamical approach to the traveling salesman problem: an efficient simulation algorithm. J. Optim. Theory Appl. 45 , 41–51 (1985)
J. Chakrapani, J. Skorin-Kapov, Massively parallel tabu search for the quadratic assignment problem. Ann. Oper. Res. 41 , 327–342 (1993)
J. Chakrapani, J. Skorin-Kapov, A constructive method to improve lower bounds for the quadratic assignment problem, in Quadratic Assignment and Related Problems , ed. by P. Pardalos, H. Wolkowicz. DIMACS Series in Discrete Mathematics and Theoretical Computer Science, vol. 16 (AMS, Providence, 1994), pp. 161–171
D. Chhajed, T.J. Lowe, m -Median and m -center problems with mutual communication: solvable special cases. Oper. Res. 40 , S56–S66 (1992)
P. Chrétienne, A polynomial algorithm to optimally schedule tasks on a virtual distributed system under tree-like precedence constraints. Eur. J. Oper. Res. 43 , 225–230 (1989)
N. Christofides, M. Gerrard, A graph theoretic analysis of bounds for the quadratic assignment problem, in Studies on Graphs and Discrete Programming , ed. by P. Hansen (North Holland, Amsterdam, 1981), pp. 61–68
J. Clausen, S.E. Karisch, M. Perregaard, F. Rendl, On the applicability of lower bounds for solving rectilinear quadratic assignment problems in parallel. Comput. Optim. Appl. 10 , 127–147 (1998)
J. Clausen, M. Perregaard, Solving large quadratic assignment problems in parallel. Comput. Optim. Appl. 8 , 111–127 (1997)
A. Colorni, M. Dorigo, V. Maniezzo, The ant system: optimization by a colony of cooperating agents. IEEE Trans. Syst. Man Cybern. Part B 26 , 29–41 (1996)
A. Colorni, V. Maniezzo, The ant system applied to the quadratic assignment problem. IEEE T. Knowl. Data En. 11 , 769–778 (1999)
D.T. Connolly, An improved annealing scheme for the QAP. Eur. J. Oper. Res. 46 , 93–100 (1990)
K. Conrad, Das Quadratische Zuweisungsproblem und zwei seiner Spezialfälle (Mohr-Siebeck, Tübingen, 1971)
D. Cyganski, R.F. Vaz, V.G. Virball, Quadratic assignment problems with the Palubeckis’ algorithm are degenerate. IEEE Trans. Circ. Syst. I 41 , 481–484 (1994)
J.R. Daduna, S. Voß, Practical experiences in schedule synchronization, in Computer-Aided Transit Schedule , ed. by J.R. Daduna, I. Branco, J.M. Pinto Paix ao. Lecture Notes in Economics and Mathematical Systems, vol. 430 (Springer, 1995), pp. 39–55
L. Davis, Genetic Algorithms and Simulated Annealing (Pitman, London, 1987)
S.A. de Carvalho Jr., S. Rahmann, Microarray layout as a quadratic assignment problem, in Proceedings of the German Conference in Bioinformatics (GCB) , ed. by D.H. Huson, O. Kohlbacher, A. Lupus, K. Nieselt, A. Zell. Lecture Notes in Computer Science, vol. P-38 (Springer, Berlin, 2006), pp. 11–20
E. de Klerk, R. Sotirov, Exploiting group symmetry in semidefinite programming relaxations of the quadratic assignment problem. Math. Program. 122 , 225–246 (2010)
V.M. Demidenko, A. Dolgui, Efficiently solvable cases of the quadratic assignment problem with generalized monotonic and incomplete Anti-Monge matrices. Cybern. Syst. Anal. 43 , 112–125 (2007)
V.M. Demidenko, G. Finke, V.S. Gordon, Well solvable cases of the quadratic assignment problem with monotone and bimonotone matrices. J. Math. Model. Algorithms 5 , 167–187 (2006)
V.G. Deĭneko, G.J. Woeginger, A solvable case of the quadratic assignment problem. Oper. Res. Lett. 22 , 13–17 (1998)
V.G. Deĭneko, G.J. Woeginger, A study of exponential neighborhoods for the travelling salesman problem and the quadratic assignment problem. Math. Program. Ser. A 87 , 519–542 (2000)
J.W. Dickey, J.W. Hopkins, Campus building arrangement using TOPAZ. Transp. Res. 6 , 59–68 (1972)
Y. Ding, H. Wolkowicz, A low dimensional semidefinite relaxation for the quadratic assignment problem. Math. Oper. Res. 34 , 1008–1022 (2009)
A.A. Dobrynin, R. Entriger, I. Gutman, Wiener index of trees: theory and applications. Acta Appl. Math. 66 , 211–249 (2001)
M. Dorigo, Optimization, learning, and natural algorithms. Ph.D. Thesis, Dipartimento die Elettronica e Informazione, Politecnico di Milano, Milano, Italy, 1992 (in Italian)
Z. Drezner, A new genetic algorithm for the quadratic assignment problem. INFORMS J. Comput. 15 , 320–330 (2003)
Z. Drezner, Compound genetic algorithms for the quadratic assignment problem. Oper. Res. Lett. 33 , 475–480 (2005)
M.E. Dyer, A.M. Frieze, C.J.H. McDiarmid, On linear programs with random costs. Math. Program. 35 , 3–16 (1986)
C.S. Edwards, The derivation of a greedy approximator for the Koopmans-Beckmann quadratic assignment problem, in Proceedings of the 77-th Combinatorial Programming Conference (CP77) , Liverpool, 1977, pp. 55–86
C.S. Edwards, A branch and bound algorithm for the Koopmans-Beckmann quadratic assignment problem. Math. Program. Study 13 , 35–52 (1980)
A.N. Elshafei, Hospital layout as a quadratic assignment problem. Oper. Res. Q. 28 , 167–179 (1977)
R. Enkhbat, T. Javzandulam, A global search algorithm for the quadratic assignment problem. J. Mongolian Math. Soc. 4 , 16–28 (2000)
G. Erdoğan, B. Tansel, A note on a polynomial time solvable case of the quadratic assignment problem. J. Discrete Optim. 3 , 382–384 (2006)
G. Erdoğan, B. Tansel, A branch and cut algorithm for quadratic assignment problems based on linearizations. Comput. Oper. Res. 34 , 1085–1106 (2007)
A. Faye, F. Roupin, A cutting plane algorithm based upon a semidefinite relaxation for the quadratic assignment problem, in Algorithms—ESA 2005 , ed. by G.S. Brodal, S. Leonardi. Lecture Notes in Computer Science, vol. 3669 (Springer, Heidelberg, 2005), pp. 850–861
T.A. Feo, M.G.C. Resende, Greedy randomized adaptive search procedures. J. Global Optim. 6 , 109–133 (1995)
G. Finke, R.E. Burkard, F. Rendl, Quadratic assignment problems. Ann. Discrete Math. 31 , 61–82 (1987)
M. Fischetti, M. Monaci, D. Salvagnin, Three ideas for the quadratic assignment problem. Oper. Res. 60 , 954–964 (2012)
C. Fleurent, J. Ferland, Genetic hybrids for the quadratic assignment problem, in Quadratic Assignment and Related Problems , ed. by P. Pardalos, H. Wolkowicz. DIMACS Series in Discrete Mathematics and Theoretical Computer Science, vol. 16 (AMS, Providence, 1994), pp. 173–187
J.B.G. Frenk, M. van Houweninge, A.H.G. Rinnooy Kan, Asymptotic properties of the quadratic assignment problem. Math. Oper. Res. 10 , 100–116 (1985)
R.L. Freeman, D.C. Gogerty, G.W. Graves, R.B.S. Brooks, A mathematical model of supply for space operations. Oper. Res. 14 , 1–15 (1966)
A.M. Frieze, J. Yadegar, On the quadratic assignment problem. Discrete Appl. Math. 5 , 89–98 (1983)
A.M. Frieze, J. Yadegar, S. El-Horbaty, D. Parkinson, Algorithms for assignment problems on an array processor. Parallel Comput. 11 , 151–162 (1989)
L.M. Gambardella, E.D. Taillard, M. Dorigo, Ant colonies for the QAP. J. Oper. Res. Soc. 50 , 167–176 (1999)
M.R. Garey, D.S. Johnson, Computers and Intractability: A Guide to the Theory of NP-Completeness (W.H. Freeman and Company, New York, 1979)
J.W. Gavett, N.V. Plyter, The optimal assignment of facilities to locations by branch and bound. Oper. Res. 14 , 210–232 (1966)
A.M. Geoffrion, Lagrangean relaxation and its uses in integer programming. Math. Program. Study 2 , 82–114 (1974)
A.M. Geoffrion, G.W. Graves, Scheduling parallel production lines with changeover costs: practical applications of a quadratic assignment/LP approach. Oper. Res. 24 , 595–610 (1976)
P.C. Gilmore, Optimal and suboptimal algorithms for the quadratic assignment problem. SIAM J. Appl. Math. 10 , 305–313 (1962)
F. Glover, Improved linear integer programming formulations of nonlinear integer problems. Manag. Sci. 22 , 455–460 (1975)
F. Glover, Tabu search—Part I. ORSA J. Comput. 1 , 190–206 (1989)
F. Glover, Tabu search—Part II. ORSA J. Comput. 2 , 4–32 (1989)
F. Glover, Scatter search and path relinking, in New Ideas in Optimization , ed. by D. Corne, M. Dorigo, F. Glover, D. Dasgupta, P. Moscato, R. Poli, K.V. Price (McGraw Hill, Maidenhead, 1999), pp. 297–316
F. Glover, M. Laguna, Tabu Search . (Kluwer Academic Publ., Norwell, 1997)
M.X. Goemans, D.P. Williamson, Improved approximation algorithms for maximum cut and satisfiability problems using semidefinite programming. J. ACM 42 , 1115–1145 (1995)
D.E. Goldberg, Genetic Algorithms in Search, Optimization and Machine Learning (Addison-Wesley, Wokingham, 1989)
G.W. Graves, A.B. Whinston, An algorithm for the quadratic assignment problem. Manag. Sci. 17 , 453–471 (1970)
H. Greenberg, A quadratic assignment problem without column constraints. Naval Res. Logist. Q. 16 , 417–422 (1969)
S.W. Hadley, Continuous optimization approaches for the quadratic assignment problem. PhD thesis, University of Waterloo, Ontario, 1989
S.W. Hadley, F. Rendl, H. Wolkowicz, Bounds for the quadratic assignment problem using continuous optimization techniques, in Proceedings of the 1-st Integer Programming and Combinatorial Optimization Conference (IPCO) , University of Waterloo Press, Waterloo, 1990, pp. 237–248
S.W. Hadley, F. Rendl, H. Wolkowicz, A new lower bound via projection for the quadratic assignment problem. Math. Oper. Res. 17 , 727–739 (1992)
S.W. Hadley, F. Rendl, H. Wolkowicz, Nonsymmetric quadratic assignment problems and the Hoffman-Wielandt inequality. Linear Algebra Appl. 58 , 109–124 (1992)
P.M. Hahn, T. Grant, Lower bounds for the quadratic assignment problem based upon a dual formulation. Oper. Res. 46 , 912–922 (1998)
P.M. Hahn, T. Grant, N. Hall, Solution of the quadratic assignment problem using the Hungarian method. Eur. J. Oper. Res. 108 , 629–640 (1998)
P.M. Hahn, Y.-R. Zhu, M. Guignard, W.L. Hightower, M.J. Saltzman, A level-3 reformulation-linearization technique-based bound for the quadratic assignment problem. INFORMS J. Comput. 24 , 202–209 (2012)
G.G. Hardy, J.E. Littlewood, G. Pólya, Inequalities (Cambridge University Press, New York, 1952)
M. Hanan, J.M. Kurtzberg, A review of the placement and quadratic assignment problems. SIAM Rev. 14 , 324–342 (1972)
D.R. Heffley, Assigning runners to a relay team, in Optimal Strategies in Sports , ed. by S.P. Ladany, R.E. Machol (North-Holland, Amsterdam, 1977), pp. 169–171
J.H. Holland, Adaptation in Natural and Artificial Systems (University of Michigan Press, Ann Arbor, 1975)
L.J. Hubert, Assignment Methods in Combinatorial Data Analysis (Marcel Dekker, New York, 1987)
T. James, C. Rego, F. Glover, Multistart tabu search and diversification strategies for the quadratic assignment problem. IEEE Trans. Syst. Man Cybern. Part A Syst. Hum. 39 , 579–596 (2009)
D.S. Johnson, C.H. Papadimitriou, M. Yannakakis, How easy is local search, J. Comput. Syst. Sci. 37 , 79–100 (1988)
T.A. Johnson, New linear programming-based solution procedures for the quadratic assignment problem. Ph.D. Thesis, Clemson University, SC, 1992
M. Jünger, Polyhedral Combinatorics and the Acyclic Subdigraph Problem (Heldermann Verlag, Berlin, 1985)
M. Jünger, V. Kaibel, A basic study of the QAP polytope. Technical Report 96.215, Institut für Informatik, Universität zu Köln, Germany, 1996
M. Jünger, V. Kaibel, On the SQAP polytope. SIAM J. Optim. 11 , 444–463 (2000)
V. Kaibel, Polyhedral combinatorics of the quadratic assignment problem. Ph.D. Thesis, Universität zu Köln, Germany, 1997
S.E. Karisch, Nonlinear approaches for quadratic assignment and graph partition problems. Ph.D. Thesis, Graz University of Technology, Austria, 1995
S.E. Karisch, E. Çela, J. Clausen, T. Espersen, A dual framework for lower bounds of the quadratic assignment problem based on linearization. Computing 63 , 351–403 (1999)
S.E. Karisch, F. Rendl, Lower bounds for the quadratic assignment problem via triangle decompositions. Math. Program. 71 , 137–151 (1995)
R.M. Karp, Reducibility among combinatorial problems, in Complexity of Computer Computations , ed. by R.E. Miller, J.W. Thatcher (Plenum, New York, 1972), pp. 85–103
L. Kaufman, F. Broeckx, An algorithm for the quadratic assignment problem using Benders’ decomposition. Eur. J. Oper. Res. 2 , 204–211 (1978)
B. Kernighan, S. Lin, An efficient heuristic procedure for partitioning graphs. Bell Syst. J. 49 , 291–307 (1972)
S. Kirkpatrick, C.D. Gelatt, M.P. Vecchi, Optimization by simulated annealing. Science 220 , 671–680 (1983)
T.C. Koopmans, M.J. Beckmann, Assignment problems and the location of economic activities. Econometrica 25 , 53–76 (1957)
J. Krarup, P.M. Pruzan, Computer-aided layout design. Math. Program. Study 9 , 75–94 (1978)
A.V. Krushevski, The linear programming problem on a permutation group, in Proceedings of the Seminar on Methods of Mathematical Modeling and Theory of Electrical Circuits , vol. 3, Institute of Cybernetics of the Academy of Sciences of Ukraine, Kiev, 1964, pp. 364–371 (Russian)
P.J.M. van Laarhoven, E.H.L. Aarts, Simulated Annealing: Theory and Applications (D. Reidel Publishing Company, Dordrecht, 1988)
A.M. Land, A problem of assignment with interrelated costs. Oper. Res. Q. 14 , 185–198 (1963)
G. Laporte, H. Mercure, Balancing hydraulic turbine runners: a quadratic assignment problem. Eur. J. Oper. Res. 35 , 378–382 (1988)
E.L. Lawler, The quadratic assignment problem. Manag. Sci. 9 , 586–599 (1963)
E.L. Lawler, J.K. Lenstra, A.H.G. Rinnooy Kan, D.B. Shmoys (eds.), The Traveling Salesman Problem (Wiley, Chichester, 1985)
T. Lengauer, Combinatorial Algorithms for Integrated Circuit Layout (Wiley, Chichester, 1990)
W. Leontief, Input-Output Economics (Oxford University Press, New York, 1966)
Y. Li, P.M. Pardalos, Generating quadratic assignment test problems with known optimal permutations. Comput. Optim. Appl. 1 , 163–184 (1992)
Y. Li, P.M. Pardalos, K.G. Ramakrishnan, M.G.C. Resende, Lower bounds for the quadratic assignment problem. Ann. Oper. Res. 50 , 387–410 (1994)
Y. Li, P.M. Pardalos, M.G.C. Resende, A greedy randomized adaptive search procedure for the quadratic assignment problem, in Quadratic Assignment and Related Problems , ed. by P. Pardalos, H. Wolkowicz. DIMACS Series in Discrete Mathematics and Theoretical Computer Science, vol. 16 (AMS, Providence, 1994), pp. 237–261
M.H. Lim, Y. Yuan, S. Omatu, Extensive testing of a hybrid genetic algorithm for solving quadratic assignment problems. Comput. Optim. Appl. 23 , 47–64 (2002)
E.M. Loiola, N.M. Maia de Abreu, P.O. Boaventura-Netto, P.M. Hahn, T. Querido, A survey for the quadratic assignment problem. Eur. J. Oper. Res. 176 , 657–690 (2007)
L. Lovász, A. Schrijver, Cones of matrices and set functions and 0–1 optimization. SIAM J. Optim. 1 , 166–190 (1991)
E.J. McCormick, Human Factors Engineering (McGraw-Hill, New York, 1970)
F. Malucelli, Quadratic assignment problems: solution methods and applications. Ph.D. Thesis, Dipartimento di Informatica, Universitá di Pisa, Italy, 1993
F. Malucelli, A polynomially solvable class of quadratic semi-assignment problems. Eur. J. Oper. Res. 91 , 619–622 (1996)
F. Malucelli, D. Pretolani, Lower bounds for the quadratic semi-assignment problem. Eur. J. Oper. Res. 83 , 365–375 (1995)
T. Mautor, C. Roucairol, A new exact algorithm for the solution of quadratic assignment problems. Discrete Appl. Math. 55 , 281–293 (1994)
T. Mavridou, P.M. Pardalos, L.S. Pitsoulis, M.G.C. Resende, A GRASP for the biquadratic assignment problem. Eur. J. Oper. Res. 105 , 613–621 (1998)
N. Metropolis, A. Rosenbluth, M. Rosenbluth, A. Teller, E. Teller, Equations of state calculations by fast computing machines. J. Chem. Phys. 21 , 1087–1092 (1953)
I.Z. Milis, V.F. Magirou, A Lagrangean relaxation algorithm for sparse quadratic assignment problems. Oper. Res. Lett. 17 , 69–76 (1995)
G. Miranda, H.P.L. Luna, G.R. Mateus, R.P.M. Ferreira, A performance guarantee heuristic for electronic components placement problems including thermal effects. Comput. Oper. Res. 32 , 2937–2957 (2005)
P.B. Mirchandani, T. Obata, Locational decisions with interactions between facilities: the quadratic assignment problem a review. Working Paper Ps-79-1, Rensselaer Polytechnic Institute, Troy, New York, May 1979
L. Mirsky, The spread of a matrix. Mathematika 3 , 127–130 (1956)
A. Misevicius, An improved hybrid optimization algorithm for the quadratic assignment problem. Math. Model. Anal. 9 , 149–168 (2004)
H.D. Mittelmann, J. Peng, Estimating bounds for quadratic assignment problems with Hamming and Manhattan distance matrices based on semidefinte programming. SIAM J. Optim. 20 , 3408–3426 (2010)
N. Mladenović, P. Hansen, Variable neighborhood search. Comput. Oper. Res. 24 , 1097–1100 (1997)
J. Mosevich, Balancing hydraulic turbine runners—a discrete combinatorial optimization problem. Eur. J. Oper. Res. 26 , 202–204 (1986)
K.A. Murthy, P. Pardalos, Y. Li, A local search algorithm for the quadratic assignment problem. Informatica 3 , 524–538 (1992)
H. Müller-Merbach, Optimale Reihenfolgen (Springer, Berlin, 1970), pp. 158–171
C.E. Nugent, T.E. Vollmann, J. Ruml, An experimental comparison of techniques for the assignment of facilities to locations. J. Oper. Res. 16 , 150–173 (1969)
M.W. Padberg, M.P. Rijal, Location, Scheduling, Design and Integer Programming (Kluwer Academic, Boston, 1996)
G.S. Palubeckis, A generator of test quadratic assignment problems with known optimal solution. USSR Comput. Math. Math. Phys. 28 , 97–98 (1988) [Translated from Zh. Vychisl. Mat. Fiz. 28 , 1740–1743 (1988)]
G.S. Palubeckis, The use of special graphs for obtaining lower bounds in the geometric quadratic assignment problem. Informatica 8 , 377–400 (1997)
G.S. Palubeckis, Generating hard test instances with known optimal solution for the rectilinear quadratic assignment problem. J. Global Optim. 15 , 127–156 (1999)
G.S. Palubeckis, An algorithm for construction of test cases for the quadratic assignment problem. Informatica 11 , 281–296 (2000)
C.H. Papadimitriou, D. Wolfe, The complexity of facets resolved, in Proceedings of the 25-th Annual IEEE Symposium on the Foundations of Computer Science (FOCS) , Portland, USA, 1985, pp. 74–78
P. Pardalos, J. Crouse, A parallel algorithm for the quadratic assignment problem, in Proceedings of the Supercomputing Conference 1989 , ACM, 1989, pp. 351–360
P. Pardalos, F. Rendl, H. Wolkowicz, The quadratic assignment problem: a survey and recent developments, in Quadratic Assignment and Related Problems , ed. by P. Pardalos, H. Wolkowicz. DIMACS Series in Discrete Mathematics and Theoretical Computer Science, vol. 16 (AMS, Providence, 1994), pp. 1–42
P.M. Pardalos, L.S. Pitsoulis, M.G.C. Resende, A parallel GRASP implementation for solving the quadratic assignment problem, in Parallel Algorithms for Irregular Problems: State of the Art , ed. by A. Ferreira, J.D.P. Rolim (Kluwer Academic, Norwell, 1995), pp. 115–133
P.M. Pardalos, K.G. Ramakrishnan, M.G.C. Resende, Y. Li, Implementation of a variable reduction based lower bound in a branch and bound algorithm for the quadratic assignment problem. SIAM J. Optim. 7 , 280–294 (1997)
P.M. Pardalos, J. Xue, The maximum clique problem. J. Global Optim. 4 , 301–328 (1994)
J. Peng, H.D. Mittelmann, X. Li, A new relaxation framework for quadratic assignment problems based on matrix splitting. Math. Program. Comput. 2 , 59–77 (2010)
L. Pitsoulis, P.M. Pardalos, Quadratic assignment problem, in Encyclopedia of Optimization, 2nd edn., ed. by Ch.A. Floudas, P.M. Pardalos (Springer, Berlin, 2009), pp. 3119–3149
J. Povh, F. Rendl, Copositive and semidefinite relaxations of the quadratic assignment problem. J. Discrete Optim. 6 , 231–241 (2009)
M. Queyranne, Performance ratio of heuristics for triangle inequality quadratic assignment problems. Oper. Res. Lett. 4 , 231–234 (1986)
A.S. Ramkumar, S.G. Ponnambalam, N. Jawahar, A population-based hybrid ant system for quadratic assignment formulations in facility layout design. Int. J. Adv. Manuf. Technol. 44 , 548–558 (2008)
G. Reinelt, The Linear Ordering Problem: Algorithms and Applications (Heldermann Verlag, Berlin, 1985)
F. Rendl, Ranking scalar products to improve bounds for the quadratic assignment problem. Eur. J. Oper. Res. 20 , 363–372 (1985)
F. Rendl, Quadratic assignment problems on series-parallel digraphs. Z. Oper. Res. 30 , 161–173 (1986)
F. Rendl, R. Sotirov, Bounds for the quadratic assignment problem using the bundle method. Math. Program. B 109 , 505–524 (2007)
F. Rendl, H. Wolkowicz, Applications of parametric programming and eigenvalue maximization to the quadratic assignment problem. Math. Program. 53 , 63–78 (1992)
M.G.C. Resende, K.G. Ramakrishnan, Z. Drezner, Computing lower bounds for the quadratic assignment problem with an interior point algorithm for linear programming. Oper. Res. 43 , 781–791 (1995)
W.T. Rhee, A note on asymptotic properties of the quadratic assignment problem. Oper. Res. Lett. 7 , 197–200 (1988)
W.T. Rhee, Stochastic analysis of the quadratic assignment problem. Math. Oper. Res. 16 , 223–239 (1991)
J.M. Rodríguez, F.C. MacPhee, D.J. Bonham, V.C. Bhavsar, Solving the quadratic assignment and dynamic plant layout problems using a new hybrid meta-heuristic approach. Int. J. High Perform. Comput. Netw. 4 , 286–294 (2006)
C. Roucairol, A reduction method for quadratic assignment problems. Oper. Res. Verfahren 32 , 183–187 (1979)
C. Roucairol, A parallel branch and bound algorithm for the quadratic assignment problem. Discrete Appl. Math. 18 , 221–225 (1987)
F. Roupin, Semidefinite relaxations of the quadratic assignment problem in a Lagrangian framework. Int. J. Math. Oper. Res. 1 , 144–162 (2009)
S. Sahni, T. Gonzalez, P-complete approximation problems. J. Assoc. Comput. Mach. 23 , 555–565 (1976)
A. Schäffer, M. Yannakakis, Simple local search problems that are hard to solve. SIAM J. Comput. 20 , 56–87 (1991)
J. Skorin-Kapov, Tabu search applied to the quadratic assignment problem. ORSA J. Comput. 2 , 33–45 (1990)
J. Skorin-Kapov, Extensions of tabu search adaptation to the quadratic assignment problem. Comput. Oper. Res. 21 , 855–865 (1994)
H.S. Stone, Multiprocessor scheduling with the aid of network flow algorithms. IEEE Trans. Softw. Eng. 3 , 85–93 (1977)
Y.G. Stoyan, V.Z. Sokolovskii, S.V. Yakovlev, A method for balancing discretely distributed masses under rotation. Energomashinostroenia 2 , 4–5 (1982) (in Russian)
Th. Stützle, S. Fernandes, New benchmark instances for the QAP and the experimental analysis of algorithms, in EvoCOP 2004 , ed. by J. Gottlieb, G.R. Raidl. Lecture Notes in Computer Science, vol. 3004 (Springer, Berlin, 2004), pp. 199–209
W. Szpankowski, Combinatorial optimization problems for which almost every algorithm is asymptotically optimal! Optimization 33 , 359–367 (1995)
E. Taillard, Robust tabu search for the quadratic assignment problem. Parallel Comput. 17 , 443–455 (1991)
D.M. Tate and A.E. Smith, A genetic approach to the quadratic assignment problem. Comput. Oper. Res. 22 , 73–83 (1995)
L.-Y. Tseng, S.-C. Liang, A hybrid metaheuristic for the quadratic assignment problem. Comput. Optim. Appl. 34 , 85–113 (2006)
S. Tsutsui, Parallel ant colony optimization for the quadratic assignment problems with symmetric multi processing, in ANTS 2008 , ed. by M. Dorigo et al. Lecture Notes in Computer Science, vol. 5217 (Springer, Berlin, 2008), pp. 363–370
I. Ugi, J. Bauer, J. Friedrich, J. Gasteiger, C. Jochum, W. Schubert, Neue Anwendungsgebiete für Computer in der Chemie. Angewandte Chemie 91 (1979), 99–111
S. Voß, Heuristics for nonlinear assignment problems, in Nonlinear Assignment Problems , ed. by M. Desrochers, J.M. Rousseau. Lecture Notes in Economics and Mathematical Systems, vol. 386 (Springer, Berlin, 2000), pp. 137–152
H. Wiener, Structural determination of paraffin boiling points. J. Am. Chem. Soc. 69 , 17–20 (1947)
M.R. Wilhelm, T.L. Ward, Solving quadratic assignment problems by simulated annealing. IEEE Trans. 19 , 107–119 (1987)
T. Winter, U. Zimmermann, Dispatch of trams in storage yards. Ann. Oper. Res. 96 , 287–315 (2000)
X. Wu, H.D. Mittelmann, X. Wang, J. Wang, On computation of performance bounds of optimal index assignment, in Data Compression Conference (DCC) 2010 , IEEE, pp. 189–198. doi:10.1109/DCC.2010.24
Q. Zhao, Semidefinite programming for assignment and partitioning problems. Ph.D. Thesis, University of Waterloo, Ontario, 1996
S.B. Zahir Azami, P. Duhamel, O. Rioul, Joint source-channel coding: panorama of methods, in Proceedings of the CNES Workshop on Data Compression, Toulouse (France), November 1996, pp. 1232–1254, http://www.comelec.enst.fr/~rioul/research/dvips/csccp.ps
H. Zhang, C. Beltran-Royo, L. Ma, Solving the quadratic assignment problem by means of general purpose mixed integer linear programming solvers. Ann. Oper. Res. publ. (2012), DOI 10.1007/s10479-012-1079-4, http://www.optimization-online.org
Q. Zhao, S.E. Karisch, F. Rendl, H. Wolkowicz, Semidefinite relaxations for the quadratic assignment problem. J. Combin. Optim. 2 , 71–109 (1998)
Download references
Author information
Authors and affiliations.
Institute of Optimization and Discrete Mathematics, Graz University of Technology, Steyrergasse 30, 8010, Graz, Austria
Rainer E. burkard
You can also search for this author in PubMed Google Scholar
Corresponding author
Correspondence to Rainer E. burkard .
Editor information
Editors and affiliations.
Department of Industrial and Systems Eng, University of Florida, Gainesville, Florida, USA
Panos M. Pardalos
Department of Computer Science, University of Texas, Dallas, Richardson, Texas, USA
Ding-Zhu Du
Dept. Comp. Sci. & Engineering, University of California, San Diego, La Jolla, California, USA
Ronald L. Graham
Rights and permissions
Reprints and permissions
Copyright information
© 2013 Springer Science+Business Media New York
About this entry
Cite this entry.
burkard, R.E. (2013). Quadratic Assignment Problems. In: Pardalos, P., Du, DZ., Graham, R. (eds) Handbook of Combinatorial Optimization. Springer, New York, NY. https://doi.org/10.1007/978-1-4419-7997-1_22
Download citation
DOI : https://doi.org/10.1007/978-1-4419-7997-1_22
Published : 26 July 2013
Publisher Name : Springer, New York, NY
Print ISBN : 978-1-4419-7996-4
Online ISBN : 978-1-4419-7997-1
eBook Packages : Mathematics and Statistics Reference Module Computer Science and Engineering
Share this entry
Anyone you share the following link with will be able to read this content:
Sorry, a shareable link is not currently available for this article.
Provided by the Springer Nature SharedIt content-sharing initiative
- Publish with us
Policies and ethics
- Find a journal
- Track your research
(Stanford users can avoid this Captcha by logging in.)
- Send to text email RefWorks EndNote printer
The Quadratic Assignment Problem : Theory and Algorithms
Available online.
- SpringerLink
More options
- Find it at other libraries via WorldCat
- Contributors
Description
Creators/contributors, contents/summary.
- Preface. List of Figures. List of Tables.
- 1. Problem Statement and Complexity Aspects.
- 2. Exact Algorithms and Lower Bounds.
- 3. Heuristics and Asymptotic Behavior.
- 4. QAPs on Specially Structured Matrices.
- 5. Two More Restricted Versions of the QAP.
- 6. QAPs Arising as Optimization Problems in Graphs.
- 7. On the Biquadratic Assignment Problem (BIQAP). References. Notation Index. Subject Index.
- (source: Nielsen Book Data)
Bibliographic information

- Stanford Home
- Maps & Directions
- Search Stanford
- Emergency Info
- Terms of Use
- Non-Discrimination
- Accessibility
© Stanford University , Stanford , California 94305 .
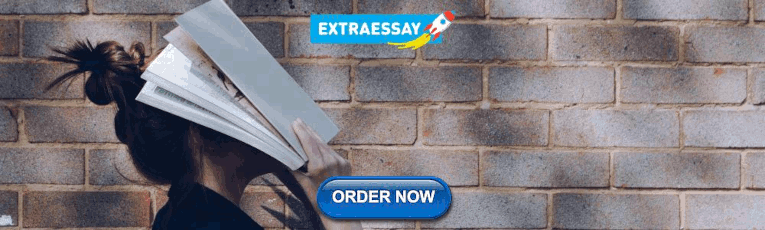
IMAGES
VIDEO
COMMENTS
The Hungarian algorithm, aka Munkres assignment algorithm, utilizes the following theorem for polynomial runtime complexity (worst case O(n 3)) and guaranteed optimality: If a number is added to or subtracted from all of the entries of any one row or column of a cost matrix, then an optimal assignment for the resulting cost matrix is also an ...
THE HUNGARIAN METHOD FOR THE ASSIGNMENT. PROBLEM'. H. W. Kuhn. Bryn Y a w College. Assuming that numerical scores are available for the perform- ance of each of n persons on each of n jobs, the "assignment problem" is the quest for an assignment of persons to jobs so that the sum of the. n scores so obtained is as large as possible.
The Hungarian method is a computational optimization technique that addresses the assignment problem in polynomial time and foreshadows following primal-dual alternatives. In 1955, Harold Kuhn used the term "Hungarian method" to honour two Hungarian mathematicians, Dénes Kőnig and Jenő Egerváry. Let's go through the steps of the Hungarian method with the help of a solved example.
The Quadratic Assignment Problem (QAP) is an optimization problem that deals with assigning a set of facilities to a set of locations, considering the pairwise distances and flows between them. The problem is to find the assignment that minimizes the total cost or distance, taking into account both the distances and the flows. The distance ...
The quadratic assignment problem (QAP) has considered one of the most significant combinatorial optimization problems due to its variant and significant applications in real life such as scheduling, production, computer manufacture, chemistry, facility location, communication, and other fields. QAP is NP-hard problem that is impossible to be solved in polynomial time when the problem size ...
This paper has always been one of my favorite "children," combining as it does elements of the duality of linear programming and combinatorial tools from graph theory. It may be of some interest to tell the story of its origin.
An assignment is called complete (or incomplete)ifitcontains k = n (or k<n, respectively) person-object pairs. We want to find a complete assignment with maximum total benefit, assuming that there exists at least one complete assignment. The dual assignment problem is given by (see e.g. [BeT89], [PaS82], [Roc84]) minimize n j=1 p j + n i=1 π ...
also considers problems related to the QAP, e.g. the biquadratic assignment problem, and discusses the relationship between the QAP and other well known combinatorial optimization problems, e.g. the traveling salesman problem, the graph partitioning problem, etc. The paper will appear in the Handbook of Combinatorial Optimization to be published
The assignment problem, also known as the maximum weighted bipartite matching problem, is a widely-studied problem applicable to many domains [2]. It can be stated as follows: given a set of workers, a set of jobs, and a set of ratings indicating how well each worker can perform each job, determine the best possible assignment of workers
the general assignment problem to a 0-1 problem. Thus, by putting the two ideas together, the Hungarian Method was born. I tested the algorithm by solving 12 by 12 problems with random 3-digit ratings by hand. I could do any such problem, with pencil and paper, in no more than 2 hours. This seemed to be much better than any other method known ...
#quadraticassignmentproblem #quadratic #assignmentproblem #qapComplete Playlist of Analysis Of Algorithms (DAA):👇👇👇👇👇👇👇👇👇👇👇👇👇 https://www.youtub...
The Quadratic Assignment Problem (QAP), discovered by Koopmans and Beckmann in 1957, is a mathematical optimization module created to describe the location of invisible economic activities. An NP-Complete problem, this model can be applied to many other optimization problems outside of the field of economics. It has been used to optimize ...
Step 1: Set up the cost matrix. The first step in solving the assignment problem is to set up the cost matrix, which represents the cost of assigning a task to an agent. The matrix should be square and have the same number of rows and columns as the number of tasks and agents, respectively.
heuristics tends to vary with certain problem characteristics [Tat1995]. 3.1 Local Search A local search starts from some initial assignment and repeatedly tries to im-prove the current assignment by local changes. If in the neighborhood of the current assignment a better assignment is found, it replaces the current assignment and the
For implementing the above algorithm, the idea is to use the max_cost_assignment() function defined in the dlib library. This function is an implementation of the Hungarian algorithm (also known as the Kuhn-Munkres algorithm) which runs in O(N 3) time. It solves the optimal assignment problem. Below is the implementation of the above approach:
The quadratic assignment problem (QAP) is considered one of the most difficult optimization problems to solve optimally. The QAP is a combinatorial optimization problem stated for the first time by Koopmans and Beckmann ().Early papers on the subject include Gilmore (), Pierce and Crowston (), Lawler (), and Love and Wong ().The problem is defined as follows.
This tutorial explains what is assignment problem in analysis of algorithm aoa or job assignment problem in design and analysis of algorithm daa in urdu and ...
in the assignment problem were either 0 or 1, then we could solve the assignment problem using the algorithm for the maximum cardinality bipartite matching problem: We construct a bipartite graph which has a node for every person iand every job j, and an edge from ito jif c ij = 0. A matching in this graph of size k
The international literature identifies the quadratic assignment problem (QAP) as the problem of finding a minimum cost allocation of facilities into locations, taking the costs as the sum of all possible distance-flow products. The main motivation for this survey is the continuous interest in QAP, shown by a number of researchers worldwide ...
Biquadratic Assignment Problem. A generalization of the QAP is the biquadratic assignment problem (BiQAP), which is essentially a quartic assignment problem with cost coefficients formed by the products of two four-dimensional arrays. More specifically, consider two n 4 × n 4 arrays F = ( f ijkl ) and D = ( d mpst ).
The QAP problem was introduced by Koopmans and Beckmann in 1957 as a mathematical model for the assignment of a set of economic activities to a set of locations, with taking into account the distance and flow between the facilities and the costs of placing a facility in a specific location.
The quadratic assignment problem (QAP) was introduced by Koopmans and Beckmann in 1957 as a mathematical model for the location of indivisible economical activities [].Consider the problem of allocating n facilities to n locations, with the cost being a function of the distance and flow between the facilities plus costs associated with placing a facility at a certain location.
The quadratic assignment problem (QAP) was introduced in 1957 by Koopmans and Beckmann to model a plant location problem. Since then the QAP has been object of numerous investigations by mathematicians, computers scientists, ope- tions researchers and practitioners. Nowadays the QAP is widely considered as a classical combinatorial optimization ...