
An official website of the United States government
The .gov means it’s official. Federal government websites often end in .gov or .mil. Before sharing sensitive information, make sure you’re on a federal government site.
The site is secure. The https:// ensures that you are connecting to the official website and that any information you provide is encrypted and transmitted securely.
- Publications
- Account settings
Preview improvements coming to the PMC website in October 2024. Learn More or Try it out now .
- Advanced Search
- Journal List
- Can J Hosp Pharm
- v.67(1); Jan-Feb 2014

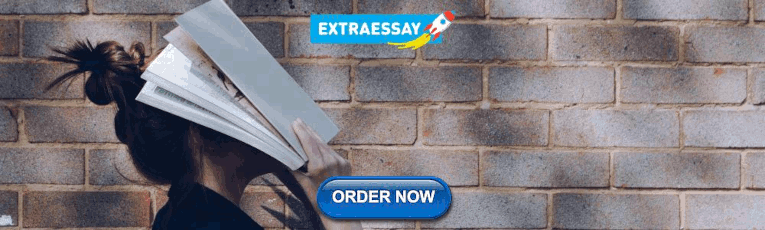
Research: Articulating Questions, Generating Hypotheses, and Choosing Study Designs
Introduction.
Articulating a clear and concise research question is fundamental to conducting a robust and useful research study. Although “getting stuck into” the data collection is the exciting part of research, this preparation stage is crucial. Clear and concise research questions are needed for a number of reasons. Initially, they are needed to enable you to search the literature effectively. They will allow you to write clear aims and generate hypotheses. They will also ensure that you can select the most appropriate research design for your study.
This paper begins by describing the process of articulating clear and concise research questions, assuming that you have minimal experience. It then describes how to choose research questions that should be answered and how to generate study aims and hypotheses from your questions. Finally, it describes briefly how your question will help you to decide on the research design and methods best suited to answering it.
TURNING CURIOSITY INTO QUESTIONS
A research question has been described as “the uncertainty that the investigator wants to resolve by performing her study” 1 or “a logical statement that progresses from what is known or believed to be true to that which is unknown and requires validation”. 2 Developing your question usually starts with having some general ideas about the areas within which you want to do your research. These might flow from your clinical work, for example. You might be interested in finding ways to improve the pharmaceutical care of patients on your wards. Alternatively, you might be interested in identifying the best antihypertensive agent for a particular subgroup of patients. Lipowski 2 described in detail how work as a practising pharmacist can be used to great advantage to generate interesting research questions and hence useful research studies. Ideas could come from questioning received wisdom within your clinical area or the rationale behind quick fixes or workarounds, or from wanting to improve the quality, safety, or efficiency of working practice.
Alternatively, your ideas could come from searching the literature to answer a query from a colleague. Perhaps you could not find a published answer to the question you were asked, and so you want to conduct some research yourself. However, just searching the literature to generate questions is not to be recommended for novices—the volume of material can feel totally overwhelming.
Use a research notebook, where you regularly write ideas for research questions as you think of them during your clinical practice or after reading other research papers. It has been said that the best way to have a great idea is to have lots of ideas and then choose the best. The same would apply to research questions!
When you first identify your area of research interest, it is likely to be either too narrow or too broad. Narrow questions (such as “How is drug X prescribed for patients with condition Y in my hospital?”) are usually of limited interest to anyone other than the researcher. Broad questions (such as “How can pharmacists provide better patient care?”) must be broken down into smaller, more manageable questions. If you are interested in how pharmacists can provide better care, for example, you might start to narrow that topic down to how pharmacists can provide better care for one condition (such as affective disorders) for a particular subgroup of patients (such as teenagers). Then you could focus it even further by considering a specific disorder (depression) and a particular type of service that pharmacists could provide (improving patient adherence). At this stage, you could write your research question as, for example, “What role, if any, can pharmacists play in improving adherence to fluoxetine used for depression in teenagers?”
TYPES OF RESEARCH QUESTIONS
Being able to consider the type of research question that you have generated is particularly useful when deciding what research methods to use. There are 3 broad categories of question: descriptive, relational, and causal.
Descriptive
One of the most basic types of question is designed to ask systematically whether a phenomenon exists. For example, we could ask “Do pharmacists ‘care’ when they deliver pharmaceutical care?” This research would initially define the key terms (i.e., describing what “pharmaceutical care” and “care” are), and then the study would set out to look for the existence of care at the same time as pharmaceutical care was being delivered.
When you know that a phenomenon exists, you can then ask description and/or classification questions. The answers to these types of questions involve describing the characteristics of the phenomenon or creating typologies of variable subtypes. In the study above, for example, you could investigate the characteristics of the “care” that pharmacists provide. Classifications usually use mutually exclusive categories, so that various subtypes of the variable will have an unambiguous category to which they can be assigned. For example, a question could be asked as to “what is a pharmacist intervention” and a definition and classification system developed for use in further research.
When seeking further detail about your phenomenon, you might ask questions about its composition. These questions necessitate deconstructing a phenomenon (such as a behaviour) into its component parts. Within hospital pharmacy practice, you might be interested in asking questions about the composition of a new behavioural intervention to improve patient adherence, for example, “What is the detailed process that the pharmacist implicitly follows during delivery of this new intervention?”
After you have described your phenomena, you may then be interested in asking questions about the relationships between several phenomena. If you work on a renal ward, for example, you may be interested in looking at the relationship between hemoglobin levels and renal function, so your question would look something like this: “Are hemoglobin levels related to level of renal function?” Alternatively, you may have a categorical variable such as grade of doctor and be interested in the differences between them with regard to prescribing errors, so your research question would be “Do junior doctors make more prescribing errors than senior doctors?” Relational questions could also be asked within qualitative research, where a detailed understanding of the nature of the relationship between, for example, the gender and career aspirations of clinical pharmacists could be sought.
Once you have described your phenomena and have identified a relationship between them, you could ask about the causes of that relationship. You may be interested to know whether an intervention or some other activity has caused a change in your variable, and your research question would be about causality. For example, you may be interested in asking, “Does captopril treatment reduce blood pressure?” Generally, however, if you ask a causality question about a medication or any other health care intervention, it ought to be rephrased as a causality–comparative question. Without comparing what happens in the presence of an intervention with what happens in the absence of the intervention, it is impossible to attribute causality to the intervention. Although a causality question would usually be answered using a comparative research design, asking a causality–comparative question makes the research design much more explicit. So the above question could be rephrased as, “Is captopril better than placebo at reducing blood pressure?”
The acronym PICO has been used to describe the components of well-crafted causality–comparative research questions. 3 The letters in this acronym stand for Population, Intervention, Comparison, and Outcome. They remind the researcher that the research question should specify the type of participant to be recruited, the type of exposure involved, the type of control group with which participants are to be compared, and the type of outcome to be measured. Using the PICO approach, the above research question could be written as “Does captopril [ intervention ] decrease rates of cardiovascular events [ outcome ] in patients with essential hypertension [ population ] compared with patients receiving no treatment [ comparison ]?”
DECIDING WHETHER TO ANSWER A RESEARCH QUESTION
Just because a question can be asked does not mean that it needs to be answered. Not all research questions deserve to have time spent on them. One useful set of criteria is to ask whether your research question is feasible, interesting, novel, ethical, and relevant. 1 The need for research to be ethical will be covered in a later paper in the series, so is not discussed here. The literature review is crucial to finding out whether the research question fulfils the remaining 4 criteria.
Conducting a comprehensive literature review will allow you to find out what is already known about the subject and any gaps that need further exploration. You may find that your research question has already been answered. However, that does not mean that you should abandon the question altogether. It may be necessary to confirm those findings using an alternative method or to translate them to another setting. If your research question has no novelty, however, and is not interesting or relevant to your peers or potential funders, you are probably better finding an alternative.
The literature will also help you learn about the research designs and methods that have been used previously and hence to decide whether your potential study is feasible. As a novice researcher, it is particularly important to ask if your planned study is feasible for you to conduct. Do you or your collaborators have the necessary technical expertise? Do you have the other resources that will be needed? If you are just starting out with research, it is likely that you will have a limited budget, in terms of both time and money. Therefore, even if the question is novel, interesting, and relevant, it may not be one that is feasible for you to answer.
GENERATING AIMS AND HYPOTHESES
All research studies should have at least one research question, and they should also have at least one aim. As a rule of thumb, a small research study should not have more than 2 aims as an absolute maximum. The aim of the study is a broad statement of intention and aspiration; it is the overall goal that you intend to achieve. The wording of this broad statement of intent is derived from the research question. If it is a descriptive research question, the aim will be, for example, “to investigate” or “to explore”. If it is a relational research question, then the aim should state the phenomena being correlated, such as “to ascertain the impact of gender on career aspirations”. If it is a causal research question, then the aim should include the direction of the relationship being tested, such as “to investigate whether captopril decreases rates of cardiovascular events in patients with essential hypertension, relative to patients receiving no treatment”.
The hypothesis is a tentative prediction of the nature and direction of relationships between sets of data, phrased as a declarative statement. Therefore, hypotheses are really only required for studies that address relational or causal research questions. For the study above, the hypothesis being tested would be “Captopril decreases rates of cardiovascular events in patients with essential hypertension, relative to patients receiving no treatment”. Studies that seek to answer descriptive research questions do not test hypotheses, but they can be used for hypothesis generation. Those hypotheses would then be tested in subsequent studies.
CHOOSING THE STUDY DESIGN
The research question is paramount in deciding what research design and methods you are going to use. There are no inherently bad research designs. The rightness or wrongness of the decision about the research design is based simply on whether it is suitable for answering the research question that you have posed.
It is possible to select completely the wrong research design to answer a specific question. For example, you may want to answer one of the research questions outlined above: “Do pharmacists ‘care’ when they deliver pharmaceutical care?” Although a randomized controlled study is considered by many as a “gold standard” research design, such a study would just not be capable of generating data to answer the question posed. Similarly, if your question was, “Is captopril better than placebo at reducing blood pressure?”, conducting a series of in-depth qualitative interviews would be equally incapable of generating the necessary data. However, if these designs are swapped around, we have 2 combinations (pharmaceutical care investigated using interviews; captopril investigated using a randomized controlled study) that are more likely to produce robust answers to the questions.
The language of the research question can be helpful in deciding what research design and methods to use. Subsequent papers in this series will cover these topics in detail. For example, if the question starts with “how many” or “how often”, it is probably a descriptive question to assess the prevalence or incidence of a phenomenon. An epidemiological research design would be appropriate, perhaps using a postal survey or structured interviews to collect the data. If the question starts with “why” or “how”, then it is a descriptive question to gain an in-depth understanding of a phenomenon. A qualitative research design, using in-depth interviews or focus groups, would collect the data needed. Finally, the term “what is the impact of” suggests a causal question, which would require comparison of data collected with and without the intervention (i.e., a before–after or randomized controlled study).
CONCLUSIONS
This paper has briefly outlined how to articulate research questions, formulate your aims, and choose your research methods. It is crucial to realize that articulating a good research question involves considerable iteration through the stages described above. It is very common that the first research question generated bears little resemblance to the final question used in the study. The language is changed several times, for example, because the first question turned out not to be feasible and the second question was a descriptive question when what was really wanted was a causality question. The books listed in the “Further Reading” section provide greater detail on the material described here, as well as a wealth of other information to ensure that your first foray into conducting research is successful.
This article is the second in the CJHP Research Primer Series, an initiative of the CJHP Editorial Board and the CSHP Research Committee. The planned 2-year series is intended to appeal to relatively inexperienced researchers, with the goal of building research capacity among practising pharmacists. The articles, presenting simple but rigorous guidance to encourage and support novice researchers, are being solicited from authors with appropriate expertise.
Previous article in this series:
Bond CM. The research jigsaw: how to get started. Can J Hosp Pharm . 2014;67(1):28–30.
Competing interests: Mary Tully has received personal fees from the UK Renal Pharmacy Group to present a conference workshop on writing research questions and nonfinancial support (in the form of travel and accommodation) from the Dubai International Pharmaceuticals and Technologies Conference and Exhibition (DUPHAT) to present a workshop on conducting pharmacy practice research.
Further Reading
- Cresswell J. Research design: qualitative, quantitative and mixed methods approaches. London (UK): Sage; 2009. [ Google Scholar ]
- Haynes RB, Sackett DL, Guyatt GH, Tugwell P. Clinical epidemiology: how to do clinical practice research. 3rd ed. Philadelphia (PA): Lippincott, Williams & Wilkins; 2006. [ Google Scholar ]
- Kumar R. Research methodology: a step-by-step guide for beginners. 3rd ed. London (UK): Sage; 2010. [ Google Scholar ]
- Smith FJ. Conducting your pharmacy practice research project. London (UK): Pharmaceutical Press; 2005. [ Google Scholar ]
Society Homepage About Public Health Policy Contact
Data-driven hypothesis generation in clinical research: what we learned from a human subject study, article sidebar.
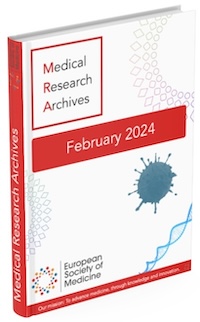
Submit your own article
Register as an author to reserve your spot in the next issue of the Medical Research Archives.
Join the Society
The European Society of Medicine is more than a professional association. We are a community. Our members work in countries across the globe, yet are united by a common goal: to promote health and health equity, around the world.
Join Europe’s leading medical society and discover the many advantages of membership, including free article publication.
Main Article Content
Hypothesis generation is an early and critical step in any hypothesis-driven clinical research project. Because it is not yet a well-understood cognitive process, the need to improve the process goes unrecognized. Without an impactful hypothesis, the significance of any research project can be questionable, regardless of the rigor or diligence applied in other steps of the study, e.g., study design, data collection, and result analysis. In this perspective article, the authors provide a literature review on the following topics first: scientific thinking, reasoning, medical reasoning, literature-based discovery, and a field study to explore scientific thinking and discovery. Over the years, scientific thinking has shown excellent progress in cognitive science and its applied areas: education, medicine, and biomedical research. However, a review of the literature reveals the lack of original studies on hypothesis generation in clinical research. The authors then summarize their first human participant study exploring data-driven hypothesis generation by clinical researchers in a simulated setting. The results indicate that a secondary data analytical tool, VIADS—a visual interactive analytic tool for filtering, summarizing, and visualizing large health data sets coded with hierarchical terminologies, can shorten the time participants need, on average, to generate a hypothesis and also requires fewer cognitive events to generate each hypothesis. As a counterpoint, this exploration also indicates that the quality ratings of the hypotheses thus generated carry significantly lower ratings for feasibility when applying VIADS. Despite its small scale, the study confirmed the feasibility of conducting a human participant study directly to explore the hypothesis generation process in clinical research. This study provides supporting evidence to conduct a larger-scale study with a specifically designed tool to facilitate the hypothesis-generation process among inexperienced clinical researchers. A larger study could provide generalizable evidence, which in turn can potentially improve clinical research productivity and overall clinical research enterprise.
Article Details
The Medical Research Archives grants authors the right to publish and reproduce the unrevised contribution in whole or in part at any time and in any form for any scholarly non-commercial purpose with the condition that all publications of the contribution include a full citation to the journal as published by the Medical Research Archives .
Skip to main page content
- AUTHOR INFO
- Institution: Google Indexer
- Sign In via User Name/Password

Hypothesis-generating research and predictive medicine
- Leslie G. Biesecker 1
- National Human Genome Research Institute, National Institutes of Health, Bethesda, Maryland 20892, USA
Genomics has profoundly changed biology by scaling data acquisition, which has provided researchers with the opportunity to interrogate biology in novel and creative ways. No longer constrained by low-throughput assays, researchers have developed hypothesis-generating approaches to understand the molecular basis of nature—both normal and pathological. The paradigm of hypothesis-generating research does not replace or undermine hypothesis-testing modes of research; instead, it complements them and has facilitated discoveries that may not have been possible with hypothesis-testing research. The hypothesis-generating mode of research has been primarily practiced in basic science but has recently been extended to clinical-translational work as well. Just as in basic science, this approach to research can facilitate insights into human health and disease mechanisms and provide the crucially needed data set of the full spectrum of genotype–phenotype correlations. Finally, the paradigm of hypothesis-generating research is conceptually similar to the underpinning of predictive genomic medicine, which has the potential to shift medicine from a primarily population- or cohort-based activity to one that instead uses individual susceptibility, prognostic, and pharmacogenetic profiles to maximize the efficacy and minimize the iatrogenic effects of medical interventions.
The goal of this article is to describe how recent technological changes provide opportunities to undertake novel approaches to biomedical research and to practice genomic preventive medicine. Massively parallel sequencing is the primary technology that will be addressed here ( Mardis 2008 ), but the principles apply to many other technologies, such as proteomics, metabolomics, transcriptomics, etc. Readers of this journal are well aware of the precipitous fall of sequencing costs over the last several decades. The consequence of this fall is that we are no longer in a scientific and medical world where the throughput (and the costs) of testing is the key limiting factor around which these enterprises are organized. Once one is released from this limiting factor, one may ask whether these enterprises should be reorganized. Here I outline the principles of how these enterprises are organized, show how high-throughput biology can allow alternative organizations of these enterprises to be considered, and show how biology and medicine are in many ways similar. The discussion includes three categories of enterprises: basic research, clinical research, and medical practice.
- The basic science hypothesis-testing paradigm
The classical paradigm for basic biological research has been to develop a specific hypothesis that can be tested by the application of a prospectively defined experiment (see Box 1 ). I suggest that one of the major (although not the only) factors that led to the development of this paradigm is that experimental design was limited by the throughput of available assays. This low throughput mandated that the scientific question had to be focused narrowly to make the question tractable. However, the paradigm can be questioned if the scientist has the ability to assay every potential attribute of a given type (e.g., all genes). If the hypothesis is only needed to select the assay, one does not need a hypothesis to apply a technology that assays all attributes. In the case of sequencing, the radical increase in throughput can release scientists from the constraint of the specific hypothesis because it has allowed them to interrogate essentially all genotypes in a genome in a single assay. This capability facilitates fundamental biological discoveries that were impossible or impractical with a hypothesis-testing mode of scientific inquiry. Examples of this approach are well demonstrated by several discoveries that followed the sequencing of a number of genomes. An example was the discovery that the human gene count was just over 20,000 ( International Human Genome Sequencing Consortium 2004 ), much lower than prior estimates. This result, although it was much debated and anticipated, was not a hypothesis that drove the human genome project, but nonetheless was surprising and led to insights into the nuances of gene regulation and transcriptional isoforms to explain the complexity of the human organism. The availability of whole genome sequence data from multiple species facilitated analyses of conservation. While it was expected that protein-coding regions, and to a lesser extent promoters and 5′- and 3′-untranslated regions of genes, would exhibit recognizable sequence conservation, it was unexpected that an even larger fraction of the genomes outside of genes are highly conserved ( Mouse Genome Sequencing Consortium 2002 ). This surprising and unanticipated discovery has spawned a novel field of scientific inquiry to determine the functional roles of these elements, which are undoubtedly important in physiology and pathophysiology. These discoveries demonstrate the power of hypothesis-generating basic research to illuminate important biological principles.
- In this window
- In a new window
Basic science hypothesis-testing and hypothesis-generating paradigms
- Clinical and translational research
The approach to clinical research grew out of the basic science paradigm as described above. The first few steps of selecting a scientific problem and developing a hypothesis are similar, with the additional step ( Box 2 ) of rigorously defining a phenotype and then carefully selecting research participants with and without that trait. As in the basic science paradigm, the hypothesis is tested by the application of a specific assay to the cases and controls. Again, this paradigm has been incredibly fruitful and should not be abandoned, but the hypothesis-generating approach can be used here as well. In this approach, a cohort of participants is consented, basic information is gathered on their health, and then a high-throughput assay, such as genome or exome sequencing, is applied to all of the participants. Again, because the assay tests all such attributes, the research design does not necessitate a priori selections of phenotypes and genes to be interrogated. Then, the researcher can examine the sequence data set for patterns and perturbations, form hypotheses about how such perturbations might affect the phenotype of the participants, and test that hypothesis with a clinical research evaluation. This approach has been used with data from genome-wide copy number assessments (array CGH and SNP arrays), but sequencing takes it to a higher level of interrogation and provides innumerable variants with which to work.
Clinical research paradigms
An example of this type of sequence-based hypothesis-generating clinical research started with a collaborative project in which we showed that mutations in the gene ACSF3 caused the biochemical phenotype of combined malonic and methylmalonic acidemia ( Sloan et al. 2011 ). At that time, the disorder was believed to be a classic pediatric, autosomal-recessive severe metabolic disorder with decompensation and sometimes death. We then queried the ClinSeq cohort ( Biesecker et al. 2009 ) to assess the carrier frequency, to estimate the population frequency of this rare disorder. Because ClinSeq is a cohort of adults with a range of atherosclerosis severity, we reasoned that this would serve as a control population for an unbiased estimate of ACSF3 heterozygote mutant alleles. Surprisingly, we identified a ClinSeq participant who was homozygous for one of the mutations identified in the children with the typical phenotype. Indeed, one potential interpretation of the data would be that the variant is, in fact, benign and was erroneously concluded to be pathogenic, based on finding it in a child with the typical phenotype. It has been shown that this error is common, with up to 20% of variants listed in databases as pathogenic actually being benign ( Bell et al. 2011 ). Further clinical research on this participant led to the surprising result that she had severely abnormal blood and urine levels of malonic and methylmalonic acid ( Sloan et al. 2011 ). This novel approach to translational research was a powerful confirmation that the mutation was indeed pathogenic, but there was another, even more important conclusion. We had conceptualized the disease completely incorrectly. Instead of being only a severe, pediatric metabolic disorder, it was instead a disorder with a wide phenotypic spectrum in which one component of the disease is a metabolic perturbation and another component is a susceptibility to severe decompensation and strokes. This research indeed raises many questions about the natural history of the disorder, whether the pediatric decompensation phenotype is attributable to modifiers, what the appropriate management of such an adult would be, etc.
Irrespective of these limitations, the understanding of the disease has markedly advanced, and the key to understanding the broader spectrum of this disease was the hypothesis-generating approach enabled by the massively parallel sequence data and the ability to phenotype patients iteratively from ClinSeq. The iterative phenotyping was essential because we could not have anticipated when the patients were originally ascertained that we would need to assay malonic and methylmalonic acid. Nor did we recognize prospectively that we should be evaluating apparently healthy patients in their seventh decade for this phenotype. Indeed, it is impossible to evaluate patients for all potential phenotypes prospectively, and it is essential to minimize ascertainment bias for patient recruitment in order to allow the discovery of the full spectrum of phenotypes associated with genomic variations. This latter issue has become a critical challenge for implementing predictive medicine, as described below.
- Predictive genomic medicine in practice
The principles of scientific inquiry are parallel to the processes of clinical diagnosis ( Box 3 ). In the classic, hypothesis-testing paradigm, clinicians gather background information including chief complaint, 2 medical and family history, and physical examination, and use these data to formulate the differential diagnosis, which is a set of potential medical diagnoses that could explain the patient's signs and symptoms. Then, the clinician selects, among the myriad of tests (imaging, biochemical, genetic, physiologic, etc.), a few tests, the results of which should distinguish among (or possibly exclude entirely) the disorders on the differential diagnosis. Like the scientist, the physician must act as a test selector, because each of the tests is low throughput, time consuming, and expensive.
Clinical practice paradigms—hypothesis testing and hypothesis generating
As in the basic and translational research discussion above, the question could be raised as to whether the differential diagnostic paradigm is necessary for genetic disorders. Indeed, the availability of clinical genome and exome sequencing heralds an era when the test could be ordered relatively early in the diagnostic process, with the clinician serving in a more interpretative role, rather than as a test selector ( Hennekam and Biesecker 2012 ). This approach has already been adopted for copy number variation, because whole genome array CGH- or SNP-based approaches have mostly displaced more specific single-gene or single-locus assays and standard chromosome analyses ( Miller et al. 2010 ). But the paradigm can be taken beyond hypothesis-generating clinical diagnosis into predictive medicine. One can now begin to envision how whole genome approaches could be used to assess risks prospectively for susceptibility to late-onset disorders or occult or subclinical disorders. The heritable cancer susceptibility syndromes are a good example of this. The current clinical approach is to order a specific gene test if a patient presents with a personal history of an atypical or early-onset form of a specific cancer syndrome, or has a compelling family history of the disease. As in the prior examples, this is because individual cancer gene testing is expensive and low throughput. One can ask the question whether this is the ideal approach or if we could be screening for these disorders from genome or exome data. Again, we applied sequencing analysis for these genes to the ClinSeq cohort because they were not ascertained for that phenotype. In a published study of 572 exomes ( Johnston et al. 2012 ), updated here to include 850 exomes, we have identified 10 patients with seven distinct cancer susceptibility syndrome mutations. These were mostly familial breast and ovarian cancer ( BRCA1 and BRCA2 ), with one patient each with paraganglioma and pheochromocytoma ( SDHC ) and one with Lynch syndrome ( MSH6 ). What is remarkable about these diagnoses is that only about half of them had a convincing personal or family history of the disease, and thus most would have not been offered testing using the current, hypothesis-testing clinical paradigm. These data suggest that screening for these disorders using genome or exome sequencing could markedly improve our ability to identify such families before they develop or die from these diseases—the ideal of predictive genomic medicine.
Despite these optimistic scenarios and examples, it remains true that our ability to perform true predictive medicine is limited. These limitations include technical factors such as incomplete sequence coverage, imperfect sequence quality, inadequate knowledge regarding the penetrance and expressivity of most variants, uncertain medical approaches and utility of pursuing variants from genomic sequencing, and the poor preparation of most clinicians for addressing genomic concerns in the clinic ( Biesecker 2013 ). Recognizing all of these limitations, it is clear that we are not prepared to launch broad-scale implementation of predictive genomic medicine, nor should all research be structured using the hypothesis-generating approach.
Hypothesis-testing approaches to science and medicine have served us well and should continue. However, the advent of massively parallel sequencing and other high-throughput technologies provides opportunities to undertake hypothesis-generating approaches to science and medicine, which in turn provide unprecedented opportunities for discovery in the research realm. This can allow the discovery of results that were not anticipated or intended by the research design, yet provide critical insights into biology and pathophysiology. Similarly, hypothesis-generating clinical research has the potential to provide these same insights and, in addition, has the potential to provide us with data that will illuminate the full spectrum of genotype–phenotype correlations, eliminating the biases that have limited this understanding in the past. Finally, applying these principles to clinical medicine can provide new pathways to diagnosis and provide the theoretical basis for predictive medicine that can detect disease susceptibility and allow health to be maintained, instead of solely focusing on the treatment of evident disease.
↵ 1 Corresponding author
E-mail lesb{at}mail.nih.gov
Article is online at http://www.genome.org/cgi/doi/10.1101/gr.157826.113 .
↵ 2 The chief complaint is a brief description of the problem that led the patient to the clinician, such as “I have a cough and fever.”
- © 2013, Published by Cold Spring Harbor Laboratory Press
This article is distributed exclusively by Cold Spring Harbor Laboratory Press for the first six months after the full-issue publication date (see http://genome.cshlp.org/site/misc/terms.xhtml ). After six months, it is available under a Creative Commons License (Attribution-NonCommercial 3.0 Unported), as described at http://creativecommons.org/licenses/by-nc/3.0/ .
- Dinwiddie DL ,
- Miller NA ,
- Hateley SL ,
- Ganusova EE ,
- Langley RJ ,
- Schilkey FD
- Biesecker LG
- Biesecker LG ,
- Mullikin JC ,
- Cherukuri PF ,
- Blakesley RW ,
- Bouffard GG ,
- Chines PS ,
- Hennekam RC ,
- ↵ International Human Genome Sequencing Consortium . 2004 . Finishing the euchromatic sequence of the human genome . Nature 431 : 931 – 945 . CrossRef Medline Google Scholar
- Johnston JJ ,
- Rubinstein WS ,
- Miller DT ,
- Aradhya S ,
- Brothman AR ,
- Carter NP ,
- Church DM ,
- Crolla JA ,
- Eichler EE ,
- ↵ Mouse Genome Sequencing Consortium. 2002 . Initial sequencing and comparative analysis of the mouse genome . Nature 420 : 520 – 562 . CrossRef Medline Google Scholar
- Chandler RJ ,
- Carrillo-Carrasco N ,
- Chandrasekaran SD ,
- O'Brien K ,

What's this?
This Article
- doi: 10.1101/gr.157826.113 Genome Res. 2013. 23: 1051-1053 © 2013, Published by Cold Spring Harbor Laboratory Press
- Abstract Free
- » Full Text Free
- Full Text (PDF) Free
Article Category
- Alert me when this article is cited
- Alert me if a correction is posted
- Similar articles in this journal
- Similar articles in Web of Science
- Article Metrics
- Similar articles in PubMed
- Download to citation manager
- Permissions
Citing Articles
- Load citing article information
- Citing articles via Web of Science
- Citing articles via Google Scholar
Google Scholar
- Articles by Biesecker, L. G.
- Search for related content
PubMed/NCBI
- PubMed citation
Preprint Server
Navigate this article, current issue.
- April 2024, 34 (4)
From the Cover
- Eucalyptus genome evolution
- Epitranscriptomics and immunity review
- Human breast cancer ESR1 heterogeneity
- Ribosome decision graphs
- Exploratory network mediator analysis
- Alert me to new issues of Genome Research
- Advance Online Articles
- Submit a Manuscript
- GR in the News
- Editorial Board
- E-mail Alerts & RSS Feeds
- Recommend to Your Library
- Job Opportunities

- Author Info
Copyright © 2024 by Cold Spring Harbor Laboratory Press
- Print ISSN: 1088-9051
- Online ISSN: 1549-5469
2.4 Developing a Hypothesis
Learning objectives.
- Distinguish between a theory and a hypothesis.
- Discover how theories are used to generate hypotheses and how the results of studies can be used to further inform theories.
- Understand the characteristics of a good hypothesis.
Theories and Hypotheses
Before describing how to develop a hypothesis it is imporant to distinguish betwee a theory and a hypothesis. A theory is a coherent explanation or interpretation of one or more phenomena. Although theories can take a variety of forms, one thing they have in common is that they go beyond the phenomena they explain by including variables, structures, processes, functions, or organizing principles that have not been observed directly. Consider, for example, Zajonc’s theory of social facilitation and social inhibition. He proposed that being watched by others while performing a task creates a general state of physiological arousal, which increases the likelihood of the dominant (most likely) response. So for highly practiced tasks, being watched increases the tendency to make correct responses, but for relatively unpracticed tasks, being watched increases the tendency to make incorrect responses. Notice that this theory—which has come to be called drive theory—provides an explanation of both social facilitation and social inhibition that goes beyond the phenomena themselves by including concepts such as “arousal” and “dominant response,” along with processes such as the effect of arousal on the dominant response.
Outside of science, referring to an idea as a theory often implies that it is untested—perhaps no more than a wild guess. In science, however, the term theory has no such implication. A theory is simply an explanation or interpretation of a set of phenomena. It can be untested, but it can also be extensively tested, well supported, and accepted as an accurate description of the world by the scientific community. The theory of evolution by natural selection, for example, is a theory because it is an explanation of the diversity of life on earth—not because it is untested or unsupported by scientific research. On the contrary, the evidence for this theory is overwhelmingly positive and nearly all scientists accept its basic assumptions as accurate. Similarly, the “germ theory” of disease is a theory because it is an explanation of the origin of various diseases, not because there is any doubt that many diseases are caused by microorganisms that infect the body.
A hypothesis , on the other hand, is a specific prediction about a new phenomenon that should be observed if a particular theory is accurate. It is an explanation that relies on just a few key concepts. Hypotheses are often specific predictions about what will happen in a particular study. They are developed by considering existing evidence and using reasoning to infer what will happen in the specific context of interest. Hypotheses are often but not always derived from theories. So a hypothesis is often a prediction based on a theory but some hypotheses are a-theoretical and only after a set of observations have been made, is a theory developed. This is because theories are broad in nature and they explain larger bodies of data. So if our research question is really original then we may need to collect some data and make some observation before we can develop a broader theory.
Theories and hypotheses always have this if-then relationship. “ If drive theory is correct, then cockroaches should run through a straight runway faster, and a branching runway more slowly, when other cockroaches are present.” Although hypotheses are usually expressed as statements, they can always be rephrased as questions. “Do cockroaches run through a straight runway faster when other cockroaches are present?” Thus deriving hypotheses from theories is an excellent way of generating interesting research questions.
But how do researchers derive hypotheses from theories? One way is to generate a research question using the techniques discussed in this chapter and then ask whether any theory implies an answer to that question. For example, you might wonder whether expressive writing about positive experiences improves health as much as expressive writing about traumatic experiences. Although this question is an interesting one on its own, you might then ask whether the habituation theory—the idea that expressive writing causes people to habituate to negative thoughts and feelings—implies an answer. In this case, it seems clear that if the habituation theory is correct, then expressive writing about positive experiences should not be effective because it would not cause people to habituate to negative thoughts and feelings. A second way to derive hypotheses from theories is to focus on some component of the theory that has not yet been directly observed. For example, a researcher could focus on the process of habituation—perhaps hypothesizing that people should show fewer signs of emotional distress with each new writing session.
Among the very best hypotheses are those that distinguish between competing theories. For example, Norbert Schwarz and his colleagues considered two theories of how people make judgments about themselves, such as how assertive they are (Schwarz et al., 1991) [1] . Both theories held that such judgments are based on relevant examples that people bring to mind. However, one theory was that people base their judgments on the number of examples they bring to mind and the other was that people base their judgments on how easily they bring those examples to mind. To test these theories, the researchers asked people to recall either six times when they were assertive (which is easy for most people) or 12 times (which is difficult for most people). Then they asked them to judge their own assertiveness. Note that the number-of-examples theory implies that people who recalled 12 examples should judge themselves to be more assertive because they recalled more examples, but the ease-of-examples theory implies that participants who recalled six examples should judge themselves as more assertive because recalling the examples was easier. Thus the two theories made opposite predictions so that only one of the predictions could be confirmed. The surprising result was that participants who recalled fewer examples judged themselves to be more assertive—providing particularly convincing evidence in favor of the ease-of-retrieval theory over the number-of-examples theory.
Theory Testing
The primary way that scientific researchers use theories is sometimes called the hypothetico-deductive method (although this term is much more likely to be used by philosophers of science than by scientists themselves). A researcher begins with a set of phenomena and either constructs a theory to explain or interpret them or chooses an existing theory to work with. He or she then makes a prediction about some new phenomenon that should be observed if the theory is correct. Again, this prediction is called a hypothesis. The researcher then conducts an empirical study to test the hypothesis. Finally, he or she reevaluates the theory in light of the new results and revises it if necessary. This process is usually conceptualized as a cycle because the researcher can then derive a new hypothesis from the revised theory, conduct a new empirical study to test the hypothesis, and so on. As Figure 2.2 shows, this approach meshes nicely with the model of scientific research in psychology presented earlier in the textbook—creating a more detailed model of “theoretically motivated” or “theory-driven” research.

Figure 2.2 Hypothetico-Deductive Method Combined With the General Model of Scientific Research in Psychology Together they form a model of theoretically motivated research.
As an example, let us consider Zajonc’s research on social facilitation and inhibition. He started with a somewhat contradictory pattern of results from the research literature. He then constructed his drive theory, according to which being watched by others while performing a task causes physiological arousal, which increases an organism’s tendency to make the dominant response. This theory predicts social facilitation for well-learned tasks and social inhibition for poorly learned tasks. He now had a theory that organized previous results in a meaningful way—but he still needed to test it. He hypothesized that if his theory was correct, he should observe that the presence of others improves performance in a simple laboratory task but inhibits performance in a difficult version of the very same laboratory task. To test this hypothesis, one of the studies he conducted used cockroaches as subjects (Zajonc, Heingartner, & Herman, 1969) [2] . The cockroaches ran either down a straight runway (an easy task for a cockroach) or through a cross-shaped maze (a difficult task for a cockroach) to escape into a dark chamber when a light was shined on them. They did this either while alone or in the presence of other cockroaches in clear plastic “audience boxes.” Zajonc found that cockroaches in the straight runway reached their goal more quickly in the presence of other cockroaches, but cockroaches in the cross-shaped maze reached their goal more slowly when they were in the presence of other cockroaches. Thus he confirmed his hypothesis and provided support for his drive theory. (Zajonc also showed that drive theory existed in humans (Zajonc & Sales, 1966) [3] in many other studies afterward).
Incorporating Theory into Your Research
When you write your research report or plan your presentation, be aware that there are two basic ways that researchers usually include theory. The first is to raise a research question, answer that question by conducting a new study, and then offer one or more theories (usually more) to explain or interpret the results. This format works well for applied research questions and for research questions that existing theories do not address. The second way is to describe one or more existing theories, derive a hypothesis from one of those theories, test the hypothesis in a new study, and finally reevaluate the theory. This format works well when there is an existing theory that addresses the research question—especially if the resulting hypothesis is surprising or conflicts with a hypothesis derived from a different theory.
To use theories in your research will not only give you guidance in coming up with experiment ideas and possible projects, but it lends legitimacy to your work. Psychologists have been interested in a variety of human behaviors and have developed many theories along the way. Using established theories will help you break new ground as a researcher, not limit you from developing your own ideas.
Characteristics of a Good Hypothesis
There are three general characteristics of a good hypothesis. First, a good hypothesis must be testable and falsifiable . We must be able to test the hypothesis using the methods of science and if you’ll recall Popper’s falsifiability criterion, it must be possible to gather evidence that will disconfirm the hypothesis if it is indeed false. Second, a good hypothesis must be logical. As described above, hypotheses are more than just a random guess. Hypotheses should be informed by previous theories or observations and logical reasoning. Typically, we begin with a broad and general theory and use deductive reasoning to generate a more specific hypothesis to test based on that theory. Occasionally, however, when there is no theory to inform our hypothesis, we use inductive reasoning which involves using specific observations or research findings to form a more general hypothesis. Finally, the hypothesis should be positive. That is, the hypothesis should make a positive statement about the existence of a relationship or effect, rather than a statement that a relationship or effect does not exist. As scientists, we don’t set out to show that relationships do not exist or that effects do not occur so our hypotheses should not be worded in a way to suggest that an effect or relationship does not exist. The nature of science is to assume that something does not exist and then seek to find evidence to prove this wrong, to show that really it does exist. That may seem backward to you but that is the nature of the scientific method. The underlying reason for this is beyond the scope of this chapter but it has to do with statistical theory.
Key Takeaways
- A theory is broad in nature and explains larger bodies of data. A hypothesis is more specific and makes a prediction about the outcome of a particular study.
- Working with theories is not “icing on the cake.” It is a basic ingredient of psychological research.
- Like other scientists, psychologists use the hypothetico-deductive method. They construct theories to explain or interpret phenomena (or work with existing theories), derive hypotheses from their theories, test the hypotheses, and then reevaluate the theories in light of the new results.
- Practice: Find a recent empirical research report in a professional journal. Read the introduction and highlight in different colors descriptions of theories and hypotheses.
- Schwarz, N., Bless, H., Strack, F., Klumpp, G., Rittenauer-Schatka, H., & Simons, A. (1991). Ease of retrieval as information: Another look at the availability heuristic. Journal of Personality and Social Psychology, 61 , 195–202. ↵
- Zajonc, R. B., Heingartner, A., & Herman, E. M. (1969). Social enhancement and impairment of performance in the cockroach. Journal of Personality and Social Psychology, 13 , 83–92. ↵
- Zajonc, R.B. & Sales, S.M. (1966). Social facilitation of dominant and subordinate responses. Journal of Experimental Social Psychology, 2 , 160-168. ↵

Share This Book
- Increase Font Size
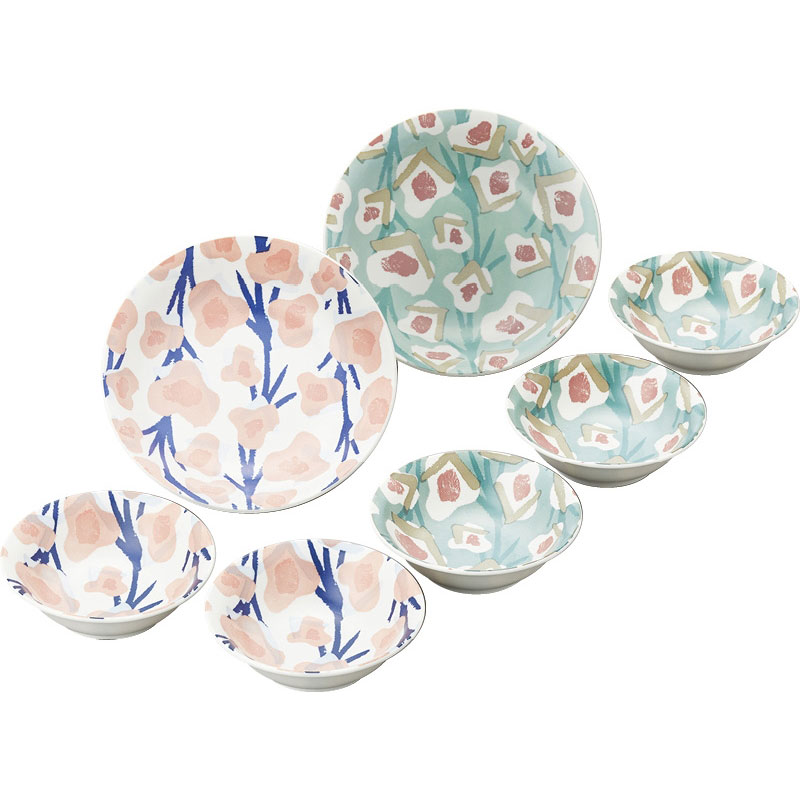
91%以上節約 ギフト プレゼント 食器・テーブルウェア
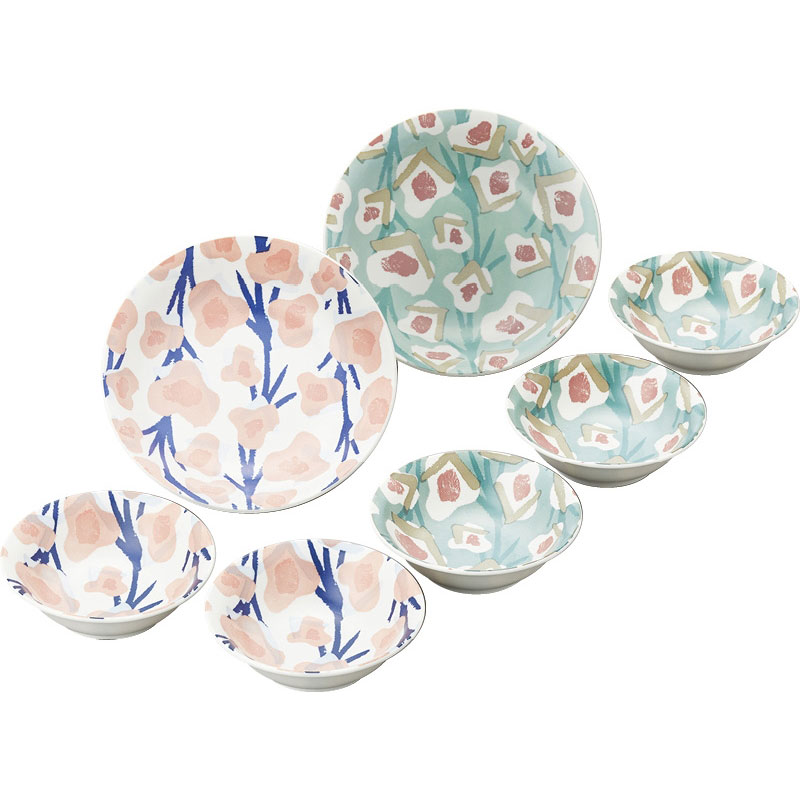
- Research article
- Open access
- Published: 03 June 2024
From concerns to benefits: a comprehensive study of ChatGPT usage in education
- Hyeon Jo ORCID: orcid.org/0000-0001-7442-4736 1
International Journal of Educational Technology in Higher Education volume 21 , Article number: 35 ( 2024 ) Cite this article
396 Accesses
5 Altmetric
Metrics details
Artificial Intelligence (AI) chatbots are increasingly becoming integral components of the digital learning ecosystem. As AI technologies continue to evolve, it is crucial to understand the factors influencing their adoption and use among students in higher education. This study is undertaken against this backdrop to explore the behavioral determinants associated with the use of the AI Chatbot, ChatGPT, among university students. The investigation delves into the role of ChatGPT’s self-learning capabilities and their influence on students’ knowledge acquisition and application, subsequently affecting the individual impact. It further elucidates the correlation of chatbot personalization with novelty value and benefits, underscoring their importance in shaping students’ behavioral intentions. Notably, individual impact is revealed to have a positive association with perceived benefits and behavioral intention. The study also brings to light potential barriers to AI chatbot adoption, identifying privacy concerns, technophobia, and guilt feelings as significant detractors from behavioral intention. However, despite these impediments, innovativeness emerges as a positive influencer, enhancing behavioral intention and actual behavior. This comprehensive exploration of the multifaceted influences on student behavior in the context of AI chatbot utilization provides a robust foundation for future research. It also offers invaluable insights for AI chatbot developers and educators, aiding them in crafting more effective strategies for AI integration in educational settings.
Introduction
In the realm of advanced language models, OpenAI’s Generative Pre-trained Transformer (GPT) has emerged as a groundbreaking tool that has begun to make significant strides in numerous disciplines (Biswas, 2023a , b ; Firat, 2023 ; Kalla, 2023 ). Education, in particular, has become a fertile ground for this innovation (Kasneci et al., 2023 ). A standout instance is ChatGPT, the latest iteration of this transformative technology, which has been rapidly adopted by a large segment of university students across the globe (Rudolph et al., 2023 ). At its core, ChatGPT is armed with the ability to produce cohesive, contextually appropriate responses predicated on preceding dialogues, providing an interactive medium akin to human conversation (King & ChatGPT, 2023 ). This interactive capacity of ChatGPT has the potential to significantly restructure the educational landscape, altering the way students absorb, interact, and engage with academic content (Fauzi et al., 2023 ). Despite the burgeoning intrigue surrounding this technology and its applications, the comprehensive examination of determinants shaping students’ behavior in the adoption and usage of ChatGPT remains a largely uncharted territory. A thorough, systematic understanding of these determinants, both facilitative and inhibitive, is essential for the more effective deployment and acceptance of such a tool in educational settings, thereby contributing to its ultimate success and efficacy. The current study seeks to shed light on this crucial aspect, aiming to provide a nuanced comprehension of the array of factors that influence university students’ behavioral intentions and patterns towards utilizing ChatGPT for their educational pursuits.
ChatGPT is replete with remarkable features courtesy of its underlying technologies: machine learning (Rospigliosi, 2023 ) and natural language processing (Maddigan & Susnjak, 2023 ). Its capabilities span across a broad spectrum, from completing texts, answering questions, to even spawning original content. In the landscape of education, these functionalities morph into a powerful apparatus that could revolutionize traditional learning modalities. Similar to a human tutor, students can converse with ChatGPT—asking questions, eliciting explanations, or diving into profound discussions about their study materials. Beyond its conversational abilities, ChatGPT’s capacity to learn from past interactions enables it to refine its responses over time, catering more accurately to the user’s distinct needs and preferences (McGee, 2023 ). This attribute of continuous learning and adaptation paves the way for a more personalized and efficient learning experience, offering custom-made academic assistance (Aljanabi, 2023 ). This intricate combination of characteristics arguably makes ChatGPT a potent tool in the educational sphere, worthy of systematic examination to optimize its potential benefits and mitigate any challenges.
ChatGPT’s unique features manifest a range of enabling factors that can significantly influence its adoption among university students. One such factor is the self-learning attribute that empowers the AI to progressively enhance its performance (Rijsdijk et al., 2007 ). This feature aligns with the ongoing learning journey of students, potentially fostering a symbiotic learning environment. Another pivotal factor is the scope for knowledge acquisition and application. As ChatGPT assists in knowledge acquisition, students can simultaneously apply their newly garnered knowledge, potentially bolstering their academic performance. Personalization of the AI forms another significant enabling factor, ensuring that the interactions are fine-tuned to each student’s distinctive needs, thereby promoting a more individual-centered learning experience. Lastly, the novelty value that ChatGPT brings to the educational realm can stimulate student engagement, making learning an exciting and enjoyable process. Unraveling the influence of these factors on students’ behavior toward ChatGPT could yield valuable insights, paving the way for the tool’s effective implementation in education.
Alongside the positive influencers, some potential detractors could hinder the adoption of ChatGPT among university students. Among these, privacy concerns stand out as paramount (Lund & Wang, 2023 ; McCallum, 2023 ). With data breaches becoming increasingly common, students might be apprehensive about sharing their academic queries and discussions with an AI tool. Technophobia is another potential inhibitor, as not all students might be comfortable interacting with an advanced AI model. Moreover, some students might experience guilt feelings while using ChatGPT, equating its assistance to a form of ‘cheating’ (Anders, 2023 ). Evaluating these potential inhibitors is crucial to forming a comprehensive understanding of the factors that shape students’ behavior towards ChatGPT. This balanced approach could help identify ways to alleviate these concerns and foster wider adoption of this promising educational tool.
Despite the growing adoption of AI tools like ChatGPT, there is a notable gap in the literature pertaining to how these tools influence behavior in an educational context. Moreover, existing studies often fail to account for both enabling and inhibiting factors. Therefore, this paper endeavors to fill this gap by offering a more balanced and comprehensive exploration. The primary objective of this study is to empirically examine the determinants—both enablers and inhibitors—that influence university students’ behavioral intentions and actual behavior towards using ChatGPT. By doing so, this study seeks to make a valuable contribution to the academic discourse surrounding the use of AI in education, while also offering practical implications for its more effective implementation in educational settings.
The rest of this paper is structured as follows: the next section reviews the relevant literature and formulates the research hypotheses. The subsequent section outlines the research methodology, followed by a presentation and discussion of the results. Finally, the paper concludes with the implications for theory and practice, and suggestions for future research.
Theoretical background and hypothesis development
This study is grounded in several theoretical frameworks that inform the understanding of AI-based language models, like ChatGPT, and their impact on individual impact, benefits, behavioral intention, and behavior.
Figure 1 displays the research mode. The model asserts that the AI’s self-learning capability influences knowledge acquisition and application, which in turn impact individual users. Personalization of the AI and the novelty value it offers are also predicted to have substantial effects on the perceived benefits, influencing the behavioral intention to use AI, culminating in actual behavior. The model also takes into account potential negative influences such as perceived risk, technophobia, and feelings of guilt on the behavioral intention to use the AI. The last two constructs in the model, behavioral intention and innovativeness, are anticipated to influence the actual behavior of the AI.
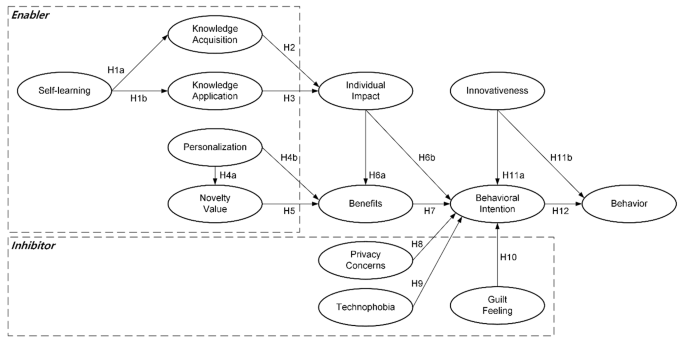
Research Model
Self-determination theory (SDT)
The SDT is indeed a critical theoretical foundation in this study. The theory, developed by Ryan and Deci ( 2000 ), posits that when tasks are intrinsically motivating and individuals perceive competence in these tasks, it leads to improved performance, persistence, and creativity. This theoretical framework supports the idea that the self-learning capability of AI, like ChatGPT, is vital in fostering intrinsic motivation among its users. Specifically, the self-learning aspect allows ChatGPT to tailor its interactions according to the individual user’s needs, thereby creating a more engaging and personally relevant learning environment. This can enhance users’ perception of their competence, resulting in more effective knowledge acquisition and application (H1).
Further, by promoting effective knowledge acquisition and application, the self-learning capability of ChatGPT can significantly impact individuals positively (H2, H3). That is, students can use the knowledge gained from interactions with ChatGPT to improve their academic performance, deepen their understanding of various subjects, and even stimulate their creativity. This aligns with the SDT’s emphasis on competence perception as a driver of enhanced performance and creativity.
Therefore, in line with the SDT, this study hypothesizes that the self-learning capability of ChatGPT, which promotes intrinsic motivation and perceived competence, can lead to improved knowledge acquisition and application, and consequently, a positive individual impact. This theoretical foundation helps to validate the first three hypotheses of the study and underscores the significance of intrinsic motivation and perceived competence in leveraging the educational potential of AI tools like ChatGPT.
- Self-learning
Self-learning is considered as an inherent capability of ChatGPT, the AI tool (Rijsdijk et al., 2007 ). This unique ability of ChatGPT allows it to conduct continuous learning, improve itself, and solve problems based on big data analysis. The concept of self-learning in AI involves the system’s ability to learn and improve from experience without being explicitly programmed (Bishop & Nasrabadi, 2006 ). Such an AI-based tool, like ChatGPT, can evolve its capabilities over time, making it a highly efficient tool for knowledge acquisition and application among students (Berland et al., 2014 ). Berland et al. ( 2014 ) pointed out that self-learning in AI can provide personalized learning experiences, thereby helping students to acquire and retain knowledge more effectively. This is due to the AI’s ability to adapt its responses based on past interactions and data, allowing for more targeted and relevant knowledge acquisition. For knowledge application, the self-learning capability of AI tools like ChatGPT can play a significant role. D’mello and Graesser ( 2013 ) argued that such AI systems could enhance learners’ ability to apply acquired knowledge, as they can simulate a variety of scenarios and provide immediate feedback. This real-time, contextual problem-solving can further strengthen knowledge application skills in students. Consequently, recognizing the potential of self-learning in AI, such as in ChatGPT, to improve both knowledge acquisition and application among university students, this study suggests the following hypotheses.
H1a. Self-learning has a positive effect on knowledge acquisition.
H1b. Self-learning has a positive effect on knowledge application.
Knowledge Acquisition
Knowledge acquisition is the process in which new information is obtained and subsequently expanded upon when additional insights are gained. A research by Nonaka ( 1994 ) emphasized the role of knowledge acquisition in enhancing organizational productivity. Another study by Alavi and Leidner ( 2001 ) suggested that knowledge acquisition can positively influence individual performance by improving the efficiency and effectiveness of task execution. Several scholars have shown the significance of knowledge acquisition on perceived usefulness in the context of learning (Al-Emran et al., 2018 ; Al-Emran & Teo, 2020 ). Given the capabilities of AI chatbots like ChatGPT in providing users with immediate, accurate, and personalized information, it’s likely that knowledge acquisition from using such a tool could lead to improved task performance or individual impact. Therefore, this study proposes the following hypothesis:
H2. Knowledge acquisition has a positive effect on individual impact.
Knowledge application
Knowledge application, as defined by Al-Emran et al. ( 2020 ), implies the facilitation of individuals to effortlessly utilize knowledge techniques via efficient storage and retrieval systems. With AI-driven chatbots like ChatGPT, this application process can be significantly enhanced. The positive influence of effective knowledge application on individual outcomes has also been corroborated by previous research (Al-Emran & Teo, 2020 ; Alavi & Leidner, 2001 ; Heisig, 2009 ). Therefore, this study suggests the following hypothesis:
H3. Knowledge application has a positive effect on individual impact.
- Personalization
The study’s conceptual framework is further informed by personalization (Maghsudi et al., 2021 ; Yu et al., 2017 ), underscoring the importance of personalized experiences in a learning environment. Literature on personalization advocates that designing experiences tailored to individual needs and preferences enhances the user’s engagement and satisfaction (Desaid, 2020 ; Jo, 2022 ). In the context of AI, personalization refers to the ability of the system, in this case, ChatGPT, to adapt its interactions based on the unique requirements and patterns of the individual user. This capacity to customize interactions could make the learning process more relevant and stimulating for the students, thereby potentially increasing the novelty value they derive from using ChatGPT (H4a).
Furthermore, the personalization theory also suggests that a personalized learning environment can lead to increased perceived benefits, as it allows students to learn at their own pace, focus on areas of interest or difficulty, and receive feedback that is specific to their learning progress (H4b). This aligns with the results of the study, which found a significant positive relationship between personalization and perceived benefits.
Personalization refers to the technology’s ability to tailor interactions based on the user’s preferences, behaviors, and individual characteristics, providing a unique and individualized user experience (Wirtz et al., 2018 ). This capacity has been found to significantly enhance users’ perception of value in their interactions with the technology (Chen et al., 2022 ). Previous research has indicated that the personalization capabilities of AI chatbots, such as ChatGPT, can enhance this novelty value, as the individualized user experience provides a unique and fresh engagement every time (Haleem et al., 2022 ; Koubaa et al., 2023 ). Research has highlighted that personalized interactions with AI chatbots can lead to increased perceived benefits, such as improved efficiency, productivity, and task performance (Makice, 2009 ). Personalization enables the technology to cater to the individual user’s needs effectively, thereby enhancing their perception of the benefits derived from using it (Wirtz et al., 2018 ). Thus, this study proposes the following hypotheses:
H4a. Personalization has a positive effect on novelty value.
H4b. Personalization has a positive effect on benefits.
Diffusion of Innovation
The diffusion of innovations theory offers a valuable lens through which to understand the adoption of new technologies (Rogers, 2010 ). The theory suggests that the diffusion and adoption of an innovation in a social system are influenced by five key factors: the innovation’s relative advantage, compatibility, complexity, trialability, and observability.
One essential aspect of the innovation, which resonates strongly with this study, is its relative advantage – the degree to which an innovation is perceived as being better than the idea it supersedes. This relative advantage often takes the form of novelty value, especially in the early stages of an innovation’s diffusion process. In this context, ChatGPT, with its self-learning capability, personalization, and interactive conversation style, can be seen as a novel approach to traditional learning methods. This novelty can make the learning process more engaging, exciting, and effective, thereby increasing the perceived benefits for students.
Rogers’ theory also highlights that these perceived benefits can significantly influence an individual’s intention to adopt the innovation. The more an individual perceives the benefits of the innovation to outweigh the costs, the more likely they are to adopt it. In the context of this study, the novelty value of using ChatGPT contributes to the perceived benefits (H5), which in turn influences the intention to use it (H7). This underscores the importance of managing the perceived novelty value of AI tools like ChatGPT in a learning environment, as it can be a crucial determinant of their adoption and use. Furthermore, Rogers’ theory stresses the importance of compatibility and simplicity of the innovation. These attributes align with the concepts of personalization and self-learning capabilities of ChatGPT, highlighting that an AI tool that aligns with users’ needs and is easy to use is more likely to be adopted.
Novelty value
Novelty value refers to the unique and new experience that users perceive when interacting with the technology (Moussawi et al., 2021 ). This sense of novelty has been associated with increased interest, engagement, and satisfaction, contributing to a more valuable and enriching user experience (Hund et al., 2021 ). In this context, the novelty value provided by AI artifacts can enhance the perceived value of using the technology (Jo, 2022 ). This relationship has been documented in prior research, showing that the novelty of technology use can lead to increased perceived benefits, possibly due to heightened user interest, engagement, and satisfaction (Huang & Benyoucef, 2013 ). Therefore, this study suggests the following hypothesis:
H5. Novelty value has a positive effect on benefits.
Technology Acceptance Model (TAM)
The TAM has been widely employed to explain user acceptance and usage of technology. TAM posits that two particular perceptions, perceived usefulness (the degree to which a person believes that using a particular system would enhance their job performance) and perceived ease of use (the degree to which a person believes that using a particular system would be free of effort), determine an individual’s attitude toward using the system, which in turn influences their behavioral intention to use it, and ultimately their actual system use.
In the context of this study, TAM offers valuable insight into how individual impact, perceived as the influence of using ChatGPT on a student’s academic performance and outcomes, can affect perceived benefits and behavioral intention (H6a, H6b). Students are more likely to use ChatGPT if they perceive it to have a positive impact on their learning outcomes. This perceived impact can enhance the perceived benefits of using ChatGPT, which can then influence their behavioral intention to use it. This aligns with Davis’s proposition that perceived usefulness positively influences behavioral intention to use a technology (Davis, 1989 ).
Moreover, the relationship between perceived benefits and behavioral intention (H7) is also consistent with TAM. According to Davis ( 1989 ), when users perceive a system as beneficial, they are more likely to form positive intentions to use it. Thus, the more students perceive the benefits of using ChatGPT, such as improved learning outcomes, personalized learning experiences, and increased engagement, the stronger their intention to use ChatGPT.
Individual impact
Individual impact refers to the perceived improvements in task performance and productivity brought about by the use of an IT such as ChatGPT (Aparicio et al., 2017 ). The perceived usefulness, which is closely related to individual impact, has a positive effect on behavioral intention to use a technology (Davis, 1989 ). In the literature, numerous studies have demonstrated that when users perceive a technology to be beneficial and impactful on their personal efficiency or productivity, they are more likely to have a positive behavioral intention towards using it (Gatzioufa & Saprikis, 2022 ; Kelly et al., 2022 ; Venkatesh et al., 2012 ). Similarly, these perceived improvements or benefits can also increase the perceived value of the technology, contributing to its overall appeal (Kim & Benbasat, 2006 ). Therefore, building upon this premise, this study proposes the following hypotheses.
H6a. Individual impact has a positive effect on benefits.
H6b. Individual impact has a positive effect on behavioral intention.
The concept of benefits is the perceived advantage or gain a user experiences from the use of the IT (Al-Fraihat et al., 2020 ). This study employed the individual impact and benefits separately. The rationale for considering individual impact and benefits as separate constructs in this research stems from the subtle differences in their underlying meanings and implications in the context of using AI chatbots like ChatGPT. While they might appear closely related, treating them separately can provide a more nuanced understanding of user experiences and perceptions. Individual impact, as defined in this research, pertains to the direct effects of using ChatGPT on the user’s performance and productivity. It encapsulates the tangible, immediate changes that occur in a user’s work efficiency and task completion speed as a result of using the AI chatbot. Therefore, individual impact serves as a measure of performance enhancement, indicating the ‘output’ side of the user’s interaction with ChatGPT. On the other hand, benefits, as gauged by the proposed survey items, are more encompassing. They extend beyond the immediate task-related effects to include the overall advantages perceived by the users. This could range from acquiring new knowledge to achieving personal or educational goals. Benefits, in essence, capture the ‘outcome’ aspect of using ChatGPT, reflecting a broader range of positive effects that contribute to user satisfaction and perceived value. By differentiating between individual impact and benefits, this research can provide a more detailed and comprehensive analysis of users’ experiences with ChatGPT.
The benefit as a determinant of behavioral intention is firmly established within the extant literature on technology acceptance and adoption. the theory of reasoned action (TRA) posits that the perception of benefits or value is directly linked to behavioral intentions (Fishbein & Ajzen, 1975 ). Similarly, the TAM proposes that perceived usefulness significantly influences behavioral intention to use a technology (Davis, 1989 ). Recent studies further confirm this relationship, which highlighted that the perceived benefit of a technology strongly predicts users’ intentions to use it (Cao et al., 2021 ; Chao, 2019 ; Yang & Wibowo, 2022 ). Given the consistent findings supporting this relationship, the following hypothesis is proposed:
H7. Benefits has a positive effect on behavioral intention.
Privacy theory and Technostress
The privacy paradox theory, formulated by Barnes ( 2006 ), provides insight into the intriguing contradiction that exists between individuals’ expressed concerns about privacy and their actual online behavior. Despite voicing concerns about their privacy, many individuals continue to disclose personal information or engage in activities that may compromise their privacy. However, in the context of AI technologies like ChatGPT, this study posits that privacy concerns may have a more pronounced impact. Particularly in an educational context, where sensitive academic information may be shared, privacy concerns can act as a deterrent, negatively influencing the behavioral intention to use the technology (H8).
On the other hand, the technostress model by Tarafdar et al. ( 2007 ) further illuminates our understanding of technology-induced stress and its impact on behavioral intention. This model proposes that individuals can experience different forms of technostress, including technophobia, which refers to the fear or anxiety experienced by some individuals when faced with new technologies. Technophobia can be particularly prevalent among individuals who lack familiarity or comfort with emerging technologies. In the context of this study, technophobia can potentially inhibit students from adopting and using AI tools like ChatGPT, thus negatively impacting their behavioral intention (H9).
Together, the privacy paradox theory and the technostress model provide a theoretical basis for understanding the potential inhibitors that could affect university students’ behavioral intention to use ChatGPT. By considering these theories, this study offers a more comprehensive perspective on the factors that can influence the adoption and use of AI technologies in an educational setting.
Privacy concerns
The concept of privacy concern refers to an individual’s anxiety or worry about the potential misuse or improper handling of personal data (Barth & de Jong, 2017 ). Privacy concern has been widely studied in the context of technology use, with a substantial body of research suggesting it is a major determinant of user behavior and intention (de Cosmo et al., 2021 ; Lutz & Tamò-Larrieux, 2021 ; Zhu et al., 2022 ). In the era of big data and pervasive digital technology, privacy concerns have increasingly become a pivotal factor shaping user behaviors (Smith et al., 2011 ). A host of empirical studies suggest that privacy concerns have a negative impact on behavioral intention. For example, Dinev and Hart ( 2006 ) observed that online privacy concerns negatively influence intention to use Internet services. Similarly, Li (2012) found that privacy concerns negatively affect users’ intention to adopt mobile apps. In the context of AI chatbots like ChatGPT, this concern could be even more pronounced, given the bot’s capacity for self-learning and data processing. Therefore, following the pattern observed in previous research, this study suggests the following hypothesis:
H8. Privacy concern has a negative effect on behavioral intention.
Technophobia
Technophobia is typically described as a personal fear or anxiety towards interacting with advanced technology, including AI systems such as ChatGPT (Ivanov & Webster, 2017 ). People suffering from technophobia often exhibit avoidance behavior when it comes to using new technology (Khasawneh, 2022 ). Several studies have empirically supported the negative relationship between technophobia and behavioral intention. For instance, Marakas et al. ( 1998 ) demonstrated that technophobia significantly reduces users’ behavioral intention to use a new IT system. Further, Selwyn (2018) found that technophobia can lead to avoidance behaviors and resistance towards adopting and using new technology, thus negatively influencing behavioral intention. Some users may fear losing control to these systems or fear making mistakes while using them, leading to a decline in their intention to use AI systems like ChatGPT. In consideration of these factors and in line with previous studies, this study posits the following hypothesis:
H9. Technophobia has a negative effect on behavioral intention.
Affect theory
The affect theory, proposed by Lazarus ( 1991 ), offers a comprehensive framework for understanding how emotions influence behavior, including technology use. According to this theory, emotional responses are elicited by the cognitive appraisal of a given situation and these emotional responses, in turn, have a significant impact on individuals’ behavior.
Applying this theory to the context of this study, it can be suggested that the use of AI technologies like ChatGPT can elicit a range of emotional responses among students. One particular emotion that is of interest in this study is guilt feeling. Guilt is typically experienced when individuals perceive that they have violated a moral standard. In the case of using AI technologies for learning, some students might perceive this as a form of ‘cheating’ or unfair advantage, thus leading to feelings of guilt.
This study hypothesizes that these guilt feelings can negatively influence students’ behavioral intention to use ChatGPT (H10). That is, students who experience guilt feelings related to using ChatGPT might be less inclined to use this tool for their learning. This underscores the importance of considering emotional factors, in addition to cognitive and behavioral factors, when exploring the determinants of technology use in an educational setting.
By integrating affect theory into the analysis, this study contributes to a more holistic understanding of the factors influencing the adoption and use of AI technologies in education. It also highlights the need for strategies that address potential guilt feelings among students to promote more positive attitudes and intentions towards using these technologies for learning.
Guilt feeling
Guilt feeling as a psychological state has been discussed extensively in consumer behavior research, often in the context of regret after certain consumption decisions (Khan et al., 2005 ; Zeelenberg & Pieters, 2007 ). Recently, guilt feeling has been associated with technology use, particularly when users perceive their technology use as excessive or inappropriate (Masood et al., 2022 ). For instance, guilt feeling could emerge among students when they feel they excessively rely on AI tools, such as ChatGPT, for completing assignments instead of their own effort and intellectual work. In line with the negative state relief model (Cialdini et al., 1973 ), individuals experiencing guilt feelings tend to take actions to alleviate these feelings. If university students feel guilty for using ChatGPT in their assignments, they might reduce their future use of this tool, thereby negatively impacting their behavioral intention to use ChatGPT. This finding is consistent with the results of a study conducted by Masood et al. ( 2022 ), which found that feelings of guilt resulting from excessive use of social networking sites predicted discontinuation. Thus, in the light of previous research suggesting a potential link between guilt feelings and behavioral intentions related to technology use, this study proposes the following hypothesis:
H10. Guilt feeling has a negative effect on behavioral intention.
- Innovativeness
Lastly, the diffusion of innovation theory (Rogers, 2010 ) lends further support to the hypotheses within the model (H12a, H12b), positing a positive influence of innovativeness on both behavioral intention and behavior. Innovativeness is defined as an individual’s predisposition to adopt new ideas, processes, or products earlier than other members of a social system (Rogers, 1983 ). It plays a critical role in technology adoption research, with prior studies highlighting the positive effects of innovativeness on both behavioral intention and actual behavior. For instance, Agarwal and Prasad ( 1998 ) found that personal innovativeness in the domain of information technology significantly influenced individuals’ intention to use a new technology. Similarly, Varma Citrin et al. ( 2000 ) discovered that consumer innovativeness positively affected both intention to use and actual use of self-service technologies. In the context of AI chatbots like ChatGPT, it is reasonable to propose that innovative university students, open to trying new technologies and applications, would exhibit stronger intentions to use ChatGPT and indeed, more likely to use it in practice. Hence, this study advances the following hypotheses:
H11a. Innovativeness has a positive effect on behavioral intention.
H11b. Innovativeness has a positive effect on behavior.
Theory of planned behavior (TPB)
The TPB, as proposed by Ajzen ( 1991 ), is a widely recognized psychological framework for understanding human behavior in various contexts, including technology adoption (Huang, 2023 ; Meng & Choi, 2019 ; Song & Jo, 2023 ). At the heart of TPB is the principle that individuals’ behavioral intentions are the most immediate and critical determinant of their actual behavior. This principle is predicated on the assumption that individuals are rational actors who make systematic use of the information available to them when deciding their course of action.
In the context of this study, the TPB supports the hypothesis that behavioral intention, influenced by various enabling and inhibiting factors, can significantly predict the actual use of ChatGPT among university students (H11). That is, students who have a stronger intention to use ChatGPT, either due to perceived benefits or other positive factors, are more likely to engage in actual usage behavior. Conversely, those with weaker intentions, perhaps due to privacy concerns or feelings of guilt, are less likely to use ChatGPT.
This hypothesis, grounded in TPB, is instrumental in linking the various enabling and inhibiting factors to the actual use of ChatGPT. It helps to consolidate the theoretical framework of the study, enabling a more holistic understanding of the behavioral dynamics at play. Moreover, it underscores the importance of cultivating positive behavioral intentions among students to encourage the actual use of AI technologies in education.
By integrating the TPB into the analysis, this study not only aligns with established psychological theories of behavior but also expands the application of these theories in the context of AI technology adoption in education.
- Behavioral intention
The relationship between behavioral intention and actual behavior is a fundamental principle in various behavior prediction models, most notably the TPB by Ajzen ( 1991 ). The TPB posits that behavioral intention is the most proximal determinant of actual behavior. The assumption is that individuals act in accordance with their intentions, given that they have sufficient control over the behavior. Empirical studies have provided substantial evidence to support this link (Gatzioufa & Saprikis, 2022 ; Sun & Jeyaraj, 2013 ). In the context of ChatGPT usage among university students, it is therefore expected that higher intentions to use ChatGPT would translate into actual usage behavior. Therefore, drawing on existing literature and theoretical models, this study suggests the following hypothesis:
H12. Behavioral intention has a positive effect on behavior.
Methodology
The instrument for this study was carefully crafted, leveraging a structured questionnaire to assess the influential factors in university students’ behavioral intentions and actual use of ChatGPT. Items in the questionnaire were adopted and adapted from the existing literature, ensuring their validity and relevance in examining the constructs of interest. Each item in the questionnaire was evaluated using a seven-point Likert scale, with 1 representing ‘strongly disagree’ and 7 denoting ‘strongly agree’. This provided a nuanced measurement of the participants’ perceptions and intentions. The survey questions were meticulously designed based on established theories and previous studies in the field, ensuring relevance to our research objectives.
Table A1 outlines a comprehensive list of constructs and their associated items used in the study, detailing how each construct is measured through specific statements. The constructs include self-learning, knowledge acquisition, knowledge application, personalization, novelty value, individual impact, benefits, privacy concern, technophobia, guilt feeling, innovativeness, behavioral intention, and behavior. Each item is attributed to sources from recent research, evidencing the rigorous grounding of these measures in existing literature. For instance, self-learning items are drawn from Chen et al. ( 2022 ), indicating the AI’s capability to enhance its functions through learning. Knowledge acquisition and application items reflect the user’s ability to gain and use knowledge via ChatGPT, sourced from Al-Emran et al. ( 2018 ) and Al-Sharafi et al. ( 2022 ), respectively. Personalization items, derived from Chen et al. ( 2022 ), measure ChatGPT’s ability to tailor responses to individual users. The novelty value construct, sourced from Hasan et al. ( 2021 ), captures the unique experience of using ChatGPT. Individual impact and benefits items, referenced from various authors, assess the practical advantages of using ChatGPT in educational settings. Privacy concern and technophobia items address potential user apprehensions, while guilt feeling items, a novel addition from Masood et al. ( 2022 ), explore emotional reactions to using AI in education. Innovativeness items, cited from Agarwal and Prasad ( 1998 ), examine user openness to new technologies. Behavioral intention and behavior constructs, finally, measure users’ future use intentions and current use patterns, demonstrating the study’s comprehensive approach to understanding AI chatbot utilization among university students.
The survey items underwent a rigorous validation process involving academic experts and a pilot study with a subset of the target population to refine their clarity and applicability. Prior to the actual distribution of the survey, a pretest was carried out with a select group of students to ensure the clarity and comprehensibility of the items. Feedback from this pretest was taken into account, and minor revisions were made to enhance the clarity of certain questions. The refined questionnaire was then disseminated to a broader group of university students, with the collected responses forming the primary data for this study. These responses were meticulously analyzed to understand the role of various factors on students’ behavioral intention and their actual usage of ChatGPT.
Questionnaire Design and Data Collection
The questionnaire was structured in two sections. The first section collected demographic information about the respondents, including their age, gender, and field of study, to capture the heterogeneity of the sample. The second section was dedicated to evaluating the constructs related to the study, each measured using multiple items.
Once the final version of the questionnaire was prepared, it was disseminated among university students. The data for this study was collected via a Google survey form. To reach a broad range of university students, the survey was disseminated through multiple channels. First, it was sent out to a network of professors at several universities. These professors then distributed the survey form to their students. During the survey phase, we explained the purpose of our study to professors across the country, asking for their assistance in distributing the survey if their students were actively using ChatGPT and were willing to participate. Additionally, some professors further shared the survey with their professional networks, expanding our reach. This approach was selected to ensure a diverse and representative sample of students from various disciplines and academic levels. Approximately 400 students were initially approached through this network. This method was chosen over others to leverage the existing academic networks, ensuring a broad yet relevant participant base for our study, reflecting diverse experiences with ChatGPT across different universities. In addition to professors, the survey was also shared within various university communities, such as student groups and clubs. This allowed the study to reach students who might not have been reached through the professors’ networks. Finally, the survey was distributed within ChatGPT community groups. This was especially important as it enabled the study to capture the perspectives of students who were already familiar with or using ChatGPT, thereby enriching the study’s insights into the factors affecting students’ behavioral intentions towards using AI tools like ChatGPT. Responses were collected over a period of several weeks, with a total of 273 responses received. The sample size of this study, 273, is considered adequate and appropriate given the number of latent variables and observation variables in the model. The literature suggests that a higher sample size is always better in SEM-based studies due to its effect on statistical power (Hair et al., 2021 ). In this case, the ratio of sample size to the number of latent variables (approximately 21:1) is within acceptable limits. Moreover, the sample size also exceeds the minimum thresholds suggested by other guidelines. For instance, Hair et al. ( 2021 ) suggested a minimum sample size of 10 times the number of items in the scale with the most items. Considering that the scale with the most items in this study contains 3 items, the sample size of 273 is well above the minimum threshold. The data, then, were analyzed.
Table 1 presents sample details. The gender split among respondents was nearly even, with 123 males (45.1%) and 150 females (54.9%), ensuring diverse gender perspectives. Age-wise, respondents ranged from under 20 to over 23 years. The largest groups were those under 20 (42.9%) and over 23 (37.4%), with 21- and 22-year-olds constituting 9.2% and 10.6%, respectively. Most respondents (89.4%) were undergraduates, with postgraduates making up 10.6%, reflecting the primary academic users of AI tools like ChatGPT. In terms of study majors, Business (44.3%) was predominant, followed by Humanities and Social Sciences (22.7%), and Science (22.0%). Arts, Sports, and Military majors each represented 5.5%. The analysis of descriptive statistics was conducted using the pivot table feature in Excel.
The theoretical framework for this study was evaluated utilizing the Partial Least Squares (PLS) approach via SmartPLS 4 software. The PLS method is a prevalent choice within the Information Systems/Information Technology domain, as substantiated by (Hair et al., 2014 ). One of the principal benefits of PLS lies in its fewer constraints concerning sample size distribution and residuals relative to covariance-based structural equation techniques such as LISREL and AMOS, as highlighted by (Hair et al., 2021 ). Our analysis employed a three-step strategy, encompassing common method bias (CMB), the measurement model, and the structural model.
Common Method Bias (CMB)
CMB refers to the spurious effect that can occur when both predictor and criterion variables are collected from the same source at the same time. It can potentially inflate or deflate the observed relationships between the constructs, leading to biased results. Thus, addressing the issue of CMB is critical to ensuring the validity of the study’s findings. In this study, we took several procedural and statistical steps to address the potential issue of CMB. Procedurally, we ensured the anonymity of the respondents and the confidentiality of their responses. We also used clear and simple language in the survey to minimize any ambiguity in the questions.
Statistically, we employed the Harman’s single factor test, which is a commonly used method to detect CMB (Podsakoff et al., 2003 ). The basic premise of this test is that if a single factor emerges from a factor analysis of all the items in the questionnaire or one general factor accounts for the majority of the covariance among the measures, then common method variance is a problem. In our case, the first of included factors explained 33.88% of the total variance, suggesting that CMB is not a substantial concern in this study. Additionally, we used the marker variable technique, which involves selecting a variable that is theoretically unrelated to other variables in the study and checking for its correlation with them. If significant correlations exist, they might be due to common method variance. Our marker variable showed negligible correlations with the study variables, further indicating that CMB is unlikely to have significantly influenced our results. In conclusion, both procedural and statistical checks suggest that CMB is not a major issue in this study, and the relationships identified between the constructs can be interpreted with confidence.
Measurement model
The measurement model in this study was assessed by examining the reliability, convergent validity, and discriminant validity of the constructs. Reliability was assessed using composite reliability (CR) and Cronbach’s alpha (CA). The values for both CR and CA should exceed the threshold of 0.7, indicating acceptable reliability. In our study, all constructs exceeded this threshold, demonstrating good reliability. Convergent Validity is the extent to which a measure correlates positively with alternate measures of the same construct. It was assessed using the average variance extracted (AVE) and the factor loadings of the indicators. All AVE values should be greater than 0.5, and all factor loadings should be significant and exceed 0.7. In our study, all constructs demonstrated satisfactory convergent validity as they met these criteria. Table 2 describes the test results of reliability and validity.
Discriminant validity refers to the extent to which a construct is truly distinct from other constructs. This was examined using the Fornell-Larcker criterion and the heterotrait-monotrait ratio (HTMT). According to the Fornell-Larcker criterion, the square root of the AVE of each construct should be greater than its highest correlation with any other construct. Table 3 shows the results of Fornell-Larcker criterion.
Table 4 displays the HTMT matrix. Notably, knowledge acquisition and knowledge application showed a 0.954 HTMT value, above the 0.85 norm. Despite exceeding the threshold, these values suggest a moderate overlap. Although related, the constructs are distinct: knowledge acquisition involves generating new knowledge, while knowledge application focuses on immediate knowledge integration. Given their different roles in AI-assisted learning, and supported by literature allowing higher HTMT values within the same network, both constructs are retained in our study. Future research should further investigate their differentiation.
Structural model
A Structural Equation Modeling (SEM) analysis was carried out to scrutinize the hypothesized interconnections among the constructs using Partial Least Squares (PLS). For hypothesis testing and path coefficient determination, this study employed a bootstrapping procedure, setting the subsample size at 5000. Overall, the structural model describes approximately 38.0% of the variability in behavior. Figure 2 ; Table 5 details of the significance test results of SEM.
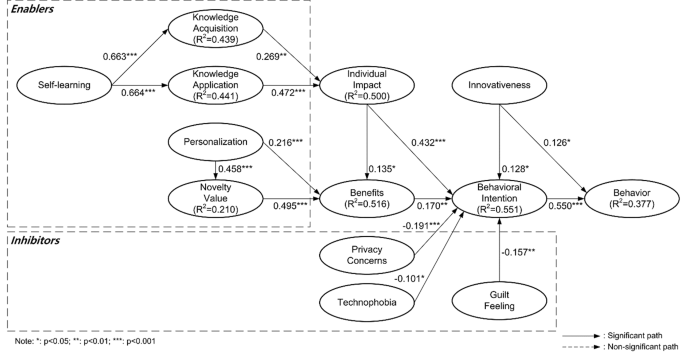
The Structural Model with Path Coefficients
The results of this study confirmed a positive correlation between self-learning of ChatGPT and knowledge acquisition and application, consistent with prior research on AI-driven learning tools (H1a, H1b) (Jarrahi et al., 2023 ). The results mean that as students engage with ChatGPT, they acquire new knowledge, which is then processed and incorporated into their existing knowledge base. Also, interacting with ChatGPT not only helps students gain new knowledge but also aids them in applying this knowledge in various scenarios, consequently resulting in a higher individual impact. The ability of AI to leverage vast data and improve problem-solving provides a fertile ground for enhanced learning. Yet, the novelty of this finding in the context of AI like ChatGPT calls for more studies to solidify this understanding.
Additionally, the results revealed that knowledge acquisition and application significantly influenced individual impact (H2, H3), mirroring the findings of Arpaci et al., (2020), Al-Emran et al. ( 2018 ), Al-Emran and Teo ( 2020 ), and Alavi and Leidner ( 2001 ). The findings suggest that as learners more actively gain knowledge from ChatGPT, they experience improvements in their productivity, task completion speed, and overall performance. Further, as learners effectively utilize the knowledge acquired from ChatGPT, they can better achieve their goals and enhance their work capabilities. This reinforces the importance of AI tools in achieving task completion and boosting productivity. Nevertheless, the variance in individual impact explained by these two factors indicates that other elements may also be at play.
Personalization was found to significantly correlate with novelty value and benefits (H4a, H4b), supporting Kapoor et al.‘s assertion of personalized experiences driving perceived value (Kapoor & Vij, 2018 ). As well the results are keeping with the observations in previous works (Haleem et al., 2022 ; Koubaa et al., 2023 ). The analysis suggests that the tailored experience delivered by ChatGPT is perceived as novel by the users. As users find these tailored experiences new and intriguing, the novelty value of the AI tool is enhanced. Similarly, as the AI tool continuously learns and adapts to individual users’ needs, it helps learners achieve their learning objectives more effectively and efficiently. This aligns with the perception of increased benefits, as users recognize the tool’s contribution to their learning outcomes. However, the degree of this impact varies, suggesting that personalization’s impact may be contingent on other factors that need exploration.
The positive effect of novelty value on perceived benefits (H5) found in this study aligns with diffusion theory (Rogers, 2010 ) and past study on novelty of AI (Jo, 2022 ). The unique and different learning experience that ChatGPT offers might stimulate users’ curiosity and interest in learning. It could make the learning process more enjoyable, keeping users engaged and motivated, which, in turn, enhances their learning outcomes and the perceived benefits from using the chatbot. Yet, the relative strength of this relationship indicates that novelty may not be the only driver of perceived benefits.
Regarding the relationship between individual impact and benefits, and behavioral intention (H6a, H6b), our findings are in line with the TAM (Davis, 1989 ), further solidifying its relevance in the AI context. These findings imply that when users perceive a higher individual impact from using ChatGPT (i.e., improved efficiency, productivity, or task accomplishment), they also perceive more benefits and express stronger intentions to continue using it. Moreover, the immediate positive effects of using ChatGPT, such as accomplishing tasks more quickly or increasing productivity, directly contribute to users’ willingness to continue using it. Yet, the relative effect sizes suggest that while individual impact affects both outcomes, its influence is stronger on behavioral intention, a facet worthy of further examination.
In line with prior studies (de Cosmo et al., 2021 ; Lutz & Tamò-Larrieux, 2021 ; Zhu et al., 2022 ), privacy concern was negatively associated with behavioral intention (H8). The results coincide with the privacy calculus theory (Dienlin & Metzger, 2016 ; Dinev & Hart, 2006 ), which suggests that when privacy concerns outweigh the perceived benefits, it can deter future usage intention. In congruent with previous findings (Marakas et al., 1998 ; Selwyn, 2013 ), this study found technophobia negatively impacting behavioral intention (H9), adding a novel dimension to our understanding of AI acceptance. This result highlights the need for easy-to-use, user-friendly interfaces and perhaps educational programs to mitigate fear and promote user confidence when engaging with AI chatbots. Similarly, the guilt feeling affecting behavioral intention (H10) provides a new angle for exploration in AI usage context.
Lastly, the results confirmed the strong effect of behavioral intention on actual behavior (H11), as suggested by (Ajzen, 1991 ). Also, innovativeness positively affected behavioral intention and behavior (H12a, H12b), adding to the growing literature on innovation adoption (Rogers, 2010 ). In the context of using AI chatbots, such as ChatGPT, students who are more innovative (who have higher levels of innovativeness) demonstrated a stronger intention to use and an actual higher usage of the chatbot. This could be interpreted to mean that these students are more inclined to explore and make use of new technologies in their learning processes, which in turn influences their behavior in a positive manner. Furthermore, the positive correlation between innovativeness and behavioral intention may also be linked to the tendency of innovative individuals to perceive less risk in trying out new technologies. This lack of perceived risk, coupled with their natural proclivity towards novelty, may increase their intention to utilize AI chatbots. However, the precise mechanisms through which innovativeness operates in the AI context warrant further study.
Theoretical contributions
The current study, in its quest to uncover the relationships between AI chatbot variables and their effects on university students, has made several valuable theoretical contributions to the existing body of literature on AI and education. Prior to this research, much of the literature focused on the use of AI chatbots in commercial and customer service settings, leaving the educational context relatively unexplored (Ashfaq et al., 2020 ; Chung et al., 2020 ; Pillai & Sivathanu, 2020 ). This study has filled this gap by focusing specifically on university students and their interaction with AI chatbots, thereby providing new insights and extending the knowledge boundary of the AI field to encompass the educational sector.
Additionally, this study’s emphasis on the self-learning capabilities of ChatGPT as a significant determinant of knowledge acquisition and application among students is a significant contribution to the existing body of literature. Previous research primarily centered on the chatbot’s features and functionalities (Haleem et al., 2022 ; Hocutt et al., 2022 ), leaving the learning capabilities of these AI systems underexplored. By focusing on the self-learning feature of ChatGPT, this study has expanded the discourse on AI capabilities and their impact on knowledge dissemination in the educational context.
This research significantly contributes to the understanding of innovativeness’s impact on behavioral intention and behavior in AI chatbot usage. While prior studies focused mainly on business contexts (BARIŞ, 2020 ; Heo & Lee, 2018 ; Selamat & Windasari, 2021 ), applying these insights to AI chatbots is relatively new, marking this study as a pioneer. Innovativeness as a determinant in this realm is novel for several reasons. First, it shifts focus from technology attributes to user characteristics, highlighting individual differences in technology adoption often overshadowed by technocentric views. Second, our study provides empirical evidence that innovativeness positively influences both behavioral intention and behavior, challenging previous assertions that limited its influence to early adoption stages (Agarwal & Prasad, 1998 ). By applying innovativeness in an AI chatbot context, with its unique interactional dynamics, the research broadens the theoretical construct of innovativeness, emphasizing the relevance of individual traits in emerging technology usage. Furthermore, this study’s integration of innovativeness into the research model encourages future research to consider other personal traits affecting technology usage, promoting a more comprehensive, user-centric approach to technology adoption. Hence, exploring innovativeness in AI chatbot usage offers significant theoretical insights and opens avenues for future research in technology usage behaviors.
This research makes a notable theoretical contribution by exploring the negative effects of privacy concern, technophobia, and guilt feeling on behavioral intention towards AI chatbot use, areas often overlooked in favor of positive influences (Ashfaq et al. 2020 ; Huang, 2021 ; Nguyen et al., 2021 ; Rafiq et al. 2022 ). Privacy concern, consistent with prior studies (Dinev & Hart, 2006 ), is critically examined in AI chatbots, where data privacy is paramount due to the potential for extensive personal data sharing. This study extends understanding by focusing on AI chatbots, a context where privacy concerns have heightened relevance. Technophobia’s impact, previously explored in technology adoption (Khasawneh, 2022 ; Kotze et al., 2016 ; Koul & Eydgahi, 2020 ), is uniquely applied to AI chatbots, shedding light on how fear and anxiety towards advanced technology can affect usage intentions. This perspective enriches existing knowledge by situating technophobia within the realm of cutting-edge AI technologies. Guilt feeling, a relatively unexplored concept in technology use, especially in AI chatbots, is also investigated. This study reveals how guilt, potentially stemming from AI reliance for learning or work, can deter usage intention, thus addressing a significant gap in technology adoption research.
This research notably contributes to theory by examining the effects of innovativeness on behavioral intention and behavior in AI chatbot usage, a relatively unexplored area, especially outside of business contexts (BARIŞ, 2020 ; Heo & Lee, 2018 ; Selamat & Windasari, 2021 ). This study stands out as one of the first to apply these concepts to AI chatbots, marking a novel approach in several ways. Firstly, it shifts the focus from technology attributes to user characteristics, emphasizing individual differences in technology adoption—a perspective often overshadowed by a technocentric focus. This approach diverges from traditional technology acceptance models, spotlighting the user’s innovativeness. Secondly, by demonstrating empirically that innovativeness positively affects both behavioral intention and behavior, this research underscores the dynamic role of this personal trait in driving technology usage. Furthermore, the study broadens the theoretical construct of innovativeness by applying it to the unique interactional dynamics of AI chatbots, thereby highlighting the relevance of individual traits in using emerging technologies. Lastly, integrating innovativeness into the research model sets a precedent for future studies to include other personal traits or user characteristics that may influence technology usage behavior. This approach promises a more holistic, user-centric understanding of technology adoption and use. Overall, exploring the impact of innovativeness in AI chatbot usage provides valuable theoretical insights and opens avenues for future research, enhancing our understanding of technology adoption in this rapidly evolving field.
The research significantly enhances theoretical understanding by substantiating the relationships between constructs like knowledge acquisition and application, individual impact, and benefits in the AI chatbot context. Previously, educational and organizational literature recognized the importance of knowledge acquisition and application for performance (Al-Emran et al., 2018 ; Al-Emran & Teo, 2020 ; Bhatt, 2001 ; Grant, 1996 ; Heisig, 2009 ). This study extends these principles to AI chatbot usage, underscoring their critical role in effective technology utilization. The study’s innovation lies in demonstrating how these cognitive processes interact with the individual impact construct. This interaction offers a comprehensive view of a user’s learning journey within the AI chatbot environment. Furthermore, the research goes beyond by illustrating that user-perceived benefits are influenced by both AI chatbot personalization and individual impact, the latter being a synergistic result of knowledge acquisition and application. This interplay provides a nuanced view of the factors contributing to perceived benefits, deepening our theoretical grasp of what renders AI chatbot usage beneficial. By presenting a complex, interconnected model of AI chatbot usage, this research contributes to a more thorough understanding of the dynamics involved. It encourages a multi-dimensional approach in examining AI chatbots’ adoption and usage factors. The findings also prompt further scholarly inquiry into how these relationships vary with context, AI chatbot type, or user characteristics. Thus, this study lays a foundation for future research, aiming to enrich the comprehension of AI chatbot usage dynamics.
Lastly, this study’s comprehensive research model, encompassing multiple constructs and their interrelationships, serves as a robust theoretical framework for future research in AI chatbot usage in educational settings. The findings of this study, highlighting the significance of various constructs and their relationships, provide a roadmap for scholars, guiding future research to better understand the dynamics of AI chatbot usage and its effects on students’ learning experiences. Furthermore, our research model takes a holistic approach by combining both positive and negative predictors of behavioral intention. This approach can offer a more comprehensive understanding of the intricate factors influencing AI chatbot use among university students.
Managerial implications
The findings of this study have numerous practical implications, particularly for educators, students, and developers of AI chatbot technologies.
Firstly, the results underscore the potential benefits of employing AI chatbots, like ChatGPT, as supplementary learning tools in educational settings. Given the significant positive effect of ChatGPT’s self-learning capabilities on knowledge acquisition and application among students, educators could consider integrating such AI chatbots into their teaching methods (Essel et al., 2022 ; Mohd Rahim et al., 2022 ; Vázquez-Cano et al., 2021 ). The integration of AI chatbots could take various forms, such as using chatbots to provide additional learning resources, facilitate interactive learning exercises, or offer personalized tutoring. Given the increasingly widespread use of online and blended learning methods in education (Crawford et al., 2020 ), the potential of AI chatbots to enhance students’ learning experiences should not be overlooked.
Secondly, this study’s findings may serve as a guide for students in maximizing their learning outcomes while using AI chatbots. Understanding that the self-learning capabilities of chatbots significantly enhance knowledge acquisition and application, students could be more inclined to utilize these tools for learning (Al-Sharafi et al., 2022 ). Furthermore, recognizing that personalization contributes positively to novelty value and benefits might motivate students to engage more deeply with personalized learning experiences offered by AI chatbots.
Thirdly, the present study’s results offer crucial insights into key features that users find valuable in an AI chatbot within an educational context. The demonstrated significance of self-learning capabilities, personalization, and novelty value indicates distinct areas that developers can focus on to amplify user experience and educational outcomes. Understanding the paramount importance of self-learning capabilities can shape the development strategies of AI chatbots. For instance, developers can focus on improving the ability of AI chatbots like ChatGPT to learn from user interactions, thereby enhancing the quality of responses over time. It would require advanced machine learning algorithms to grasp the context of user inquiries accurately, ensuring a consistent learning experience for the users. As noted by (Komiak & Benbasat, 2006 ), the AI’s ability to learn and adapt based on user input and data over time can lead to improved user satisfaction and ultimately, enhanced learning outcomes. Personalization is another pivotal feature that developers need to consider. This could involve customizing chatbot responses according to the user’s level of understanding, learning pace, and areas of interest. Personalization also extends to recognizing users’ specific learning goals and offering resources or guidance to help them achieve these goals. This feature can increase the value that users derive from their interactions with the chatbot (Jo, 2022 ). Lastly, the novelty value of AI chatbots in educational contexts cannot be underestimated. Developers can capitalize on this by introducing innovative features and interaction modes that keep users engaged and make learning exciting. Such features could include gamified learning activities, integration with other learning resources, and more interactive and responsive dialogue systems. It is well established in literature that perceived novelty can positively impact users’ attitudes and behaviors towards a new technology (Hasan et al., 2021 ; Jo, 2022 ).
Fourthly, the study’s findings related to the negative impact of privacy concerns, technophobia, and guilt feelings on behavioral intentions provide crucial insights for AI chatbot developers and educational institutions. Privacy concerns form a significant barrier to the acceptance of AI technologies in various fields, including education (Belen Saglam et al., 2021 ; Ischen et al., 2020 ; Manikonda et al., 2018 ). The concerns typically arise from the vast amounts of personal data AI systems gather, analyze, and store (Kokolakis, 2017 ). In response, developers can strengthen the privacy features of AI chatbots, clearly communicate their data handling practices to users, and ensure compliance with stringent data protection regulations. For instance, incorporating robust encryption methods, anonymization techniques, and allowing users to control the data they share can allay privacy concerns. Educational institutions can also provide guidance on safe and responsible use of AI technologies to further assuage these concerns. Technophobia, or the fear of technology, can also hinder the acceptance and use of AI chatbots. To address this, developers can design user-friendly interfaces, provide extensive user support, and gradually introduce advanced features to avoid overwhelming users. Moreover, educational institutions can play a vital role in mitigating technophobia by offering appropriate training and support to help students become comfortable with using these technologies. It has been suggested that familiarity and understanding significantly reduce technophobia (Khasawneh, 2018a ; Xi et al., 2022 ). Guilt feeling, as revealed in this study, is another factor that negatively influences behavioral intention. This can occur when users feel guilty about relying heavily on AI chatbots for learning or assignments. To address this, educational institutions should foster an environment that encourages balanced use of AI tools. This could involve providing guidelines on ethical AI use, setting boundaries on AI tool usage in assignments, and integrating AI tools as supplemental rather than primary learning resources.
Finally, the significant impact of perceived benefits and individual impact on behavioral intentions underscores the importance of demonstrating the tangible benefits of AI chatbot use in education to users. Making these benefits clear to users could encourage greater adoption and more effective use of AI chatbots in educational settings.
Limitation and Future Research
While this study makes several important contributions to our understanding of AI chatbots’ use in an educational context, it also opens up avenues for further investigation. The study’s primary limitation is its focus on university students, which may limit the generalizability of the findings. Future studies could explore different demographics, such as younger students, adult learners, or professionals engaging in continuous education, to provide a broader understanding of AI chatbot utilization in diverse learning contexts. The scope of the research could also be extended to include various types of AI chatbot technologies. As AI continues to advance, chatbots are becoming more diverse in their capabilities and functionalities. Hence, research considering different kinds of AI chatbots, their specific features, and their effects on learning outcomes could provide further insight. Moreover, this study primarily focuses on individual impacts and user perceptions. Future research could also look into institutional perspectives and the macro-level impacts of AI chatbot adoption in education. For instance, studies could investigate how the implementation of AI chatbots affects teaching strategies, curriculum development, and institutional resource allocation. Lastly, it would be insightful to conduct longitudinal studies to understand the long-term effects of AI chatbot usage on student performance and attitudes towards AI technologies. This approach could reveal trends and impacts that may not be immediately apparent in cross-sectional studies.
Data availability
The data used in this study are available from the corresponding author upon reasonable request.
Agarwal, R., & Prasad, J. (1998). A conceptual and operational definition of personal innovativeness in the domain of Information Technology. Information Systems Research , 9 (2), 204–215.
Article Google Scholar
Ajzen, I. (1991). The theory of Planned Behavior. Organizational Behavior and Human Decision Processes , 50 (2), 179–211. https://doi.org/10.1016/0749-5978(91)90020-T .
Al-Emran, M., & Teo, T. (2020). Do Knowledge Acquisition and Knowledge sharing really affect E-Learning adoption? An empirical study. Education and Information Technologies , 25 (3), 1983–1998.
Al-Emran, M., Mezhuyev, V., & Kamaludin, A. (2018). Students’ perceptions towards the integration of knowledge management processes in M-Learning systems: A preliminary study. International Journal of Engineering Education , 34 (2), 371–380.
Google Scholar
Al-Emran, M., Arpaci, I., & Salloum, S. A. (2020). An empirical examination of continuous intention to Use M-Learning: An Integrated Model. Education and Information Technologies , 25 (4), 2899–2918. https://doi.org/10.1007/s10639-019-10094-2 .
Al-Fraihat, D., Joy, M., & Sinclair, J. (2020). Evaluating E-Learning systems Success: An empirical study. Computers in Human Behavior , 102 , 67–86.
Al-Sharafi, M. A., Al-Emran, M., Iranmanesh, M., Al-Qaysi, N., Iahad, N. A., & Arpaci, I. (2022). Understanding the impact of Knowledge Management factors on the sustainable use of Ai-Based chatbots for Educational purposes using a Hybrid Sem-Ann Approach. Interactive Learning Environments , 1–20.
Alavi, M., & Leidner, D. E. (2001). Knowledge Management and Knowledge Management systems: Conceptual foundations and Research Issues. MIS Quarterly , 107–136.
Aljanabi, M. (2023). Chatgpt: Future directions and open possibilities. Mesopotamian Journal of Cybersecurity , 2023 , 16–17.
Anders, B. A. (2023). Is using Chatgpt Cheating, Plagiarism, both, neither, or Forward thinking? Patterns , 4 (3).
Aparicio, M., Bacao, F., & Oliveira, T. (2017). Grit in the path to E-Learning success. Computers in Human Behavior , 66 , 388–399.
Ashfaq, M., Yun, J., Yu, S., & Loureiro, S. M. C. (2020). I, Chatbot: Modeling the determinants of users’ satisfaction and continuance intention of Ai-Powered Service agents. Telematics and Informatics , 54 , 101473.
BARIŞ, A. (2020). A New Business Marketing Tool: Chatbot. GSI Journals Serie B: Advancements in Business and Economics , 3 (1), 31–46.
Barnes, S. B. (2006). A Privacy Paradox: Social Networking in the United States. first monday .
Barth, S., & de Jong, M. D. T. (2017). The privacy Paradox – investigating discrepancies between expressed privacy concerns and actual online behavior – a systematic literature review. Telematics and Informatics , 34 (7), 1038–1058. https://doi.org/10.1016/j.tele.2017.04.013 .
Belen Saglam, R., Nurse, J. R., & Hodges, D. (2021). Privacy Concerns in Chatbot Interactions: When to Trust and When to Worry. HCI International 2021-Posters: 23rd HCI International Conference, HCII 2021, Virtual Event, July 24–29, 2021, Proceedings, Part II 23.
Berland, M., Baker, R. S., & Blikstein, P. (2014). Educational Data Mining and Learning analytics: Applications to Constructionist Research. Technology Knowledge and Learning , 19 , 205–220.
Bhatt, G. D. (2001). Knowledge Management in Organizations: Examining the Interaction between technologies, techniques, and people. Journal of Knowledge Management .
Bishop, C. M., & Nasrabadi, N. M. (2006). Pattern Recognition and Machine Learning (Vol. 4). Springer.
Biswas, S. S. (2023a). Potential use of Chat Gpt in global warming. Annals of Biomedical Engineering , 51 (6), 1126–1127.
Biswas, S. S. (2023b). Role of Chat Gpt in Public Health. Annals of Biomedical Engineering , 1–2.
Brill, T. M., Munoz, L., & Miller, R. J. (2019). Siri, Alexa, and other Digital assistants: A study of customer satisfaction with Artificial Intelligence Applications. Journal of Marketing Management , 35 (15–16), 1401–1436.
Cao, G., Duan, Y., Edwards, J. S., & Dwivedi, Y. K. (2021). Understanding managers’ attitudes and behavioral intentions towards using Artificial Intelligence for Organizational decision-making. Technovation , 106 , 102312.
Chao, C. M. (2019). Factors determining the behavioral intention to Use Mobile Learning: An application and extension of the Utaut Model. Frontiers in Psychology , 10 , 1652.
Chen, Q., Gong, Y., Lu, Y., & Tang, J. (2022). Classifying and measuring the Service Quality of Ai Chatbot in Frontline Service. Journal of Business Research , 145 , 552–568.
Chung, M., Ko, E., Joung, H., & Kim, S. J. (2020). Chatbot E-Service and customer satisfaction regarding luxury brands. Journal of Business Research , 117 , 587–595.
Cialdini, R. B., Darby, B. L., & Vincent, J. E. (1973). Transgression and altruism: A case for Hedonism. Journal of Experimental Social Psychology , 9 (6), 502–516.
Crawford, J., Butler-Henderson, K., Rudolph, J., Malkawi, B., Glowatz, M., Burton, R., Magni, P., & Lam, S. (2020). Covid-19: 20 countries’ higher education Intra-period Digital pedagogy responses. Journal of Applied Learning & Teaching , 3 (1), 1–20.
D’mello, S., & Graesser, A. (2013). Autotutor and Affective Autotutor: Learning by talking with cognitively and emotionally Intelligent computers that talk back. ACM Transactions on Interactive Intelligent Systems (TiiS) , 2 (4), 1–39.
Davis, F. D. (1989). Perceived usefulness, perceived ease of Use, and user Acceptance of Information Technology. MIS Quarterly , 13 (3), 319–340.
de Cosmo, L. M., Piper, L., & Di Vittorio, A. (2021). The role of attitude toward chatbots and privacy concern on the relationship between attitude toward Mobile Advertising and behavioral intent to Use Chatbots. Italian Journal of Marketing , 2021 (1), 83–102. https://doi.org/10.1007/s43039-021-00020-1 .
Desaid, D. (2020). A study of personalization effect . on Users Satisfaction with E Commerce Websites.
Dienlin, T., & Metzger, M. J. (2016). An extended privacy Calculus model for Snss: Analyzing Self-Disclosure and Self-Withdrawal in a Representative U.S. Sample. Journal of Computer-Mediated Communication , 21 (5), 368–383. https://doi.org/10.1111/jcc4.12163 .
Dinev, T., & Hart, P. (2006). An extended privacy Calculus model for E-Commerce transactions. Information Systems Research , 17 (1), 61–80.
Essel, H. B., Vlachopoulos, D., Tachie-Menson, A., Johnson, E. E., & Baah, P. K. (2022). The impact of a virtual teaching Assistant (Chatbot) on students’ learning in Ghanaian higher education. International Journal of Educational Technology in Higher Education , 19 (1), 1–19.
Fauzi, F., Tuhuteru, L., Sampe, F., Ausat, A. M. A., & Hatta, H. R. (2023). Analysing the role of Chatgpt in improving Student Productivity in Higher Education. Journal on Education , 5 (4), 14886–14891.
Firat, M. (2023). How Chat Gpt Can Transform Autodidactic Experiences and Open Education. Department of Distance Education, Open Education Faculty, Anadolu Unive .
Fishbein, M., & Ajzen, I. (1975). Belief, attitude, intention, and Behavior: An introduction to theory and research . Addison-Wesley.
Gatzioufa, P., & Saprikis, V. (2022). A Literature Review on Users’ Behavioral Intention toward Chatbots’ Adoption. Applied Computing and Informatics , ahead-of-print (ahead-of-print). https://doi.org/10.1108/ACI-01-2022-0021 .
Grant, R. M. (1996). Toward a knowledge-based theory of the firm. Strategic Management Journal , 17 (S2), 109–122.
Hair, J. F., Sarstedt, M., Hopkins, L., & Kuppelwieser, V. G. (2014). Partial least squares structural equation modeling (Pls-Sem): An Emerging Tool in Business Research. European Business Review , 26 (2), 106–121.
Hair, J. F., Hult, G. T. M., Ringle, C. M., & Sarstedt, M. (2021). A primer on partial least squares structural equation modeling (Pls-Sem) . Sage.
Haleem, A., Javaid, M., & Singh, R. P. (2022). An era of Chatgpt as a significant futuristic support Tool: A study on features, abilities, and challenges. BenchCouncil Transactions on Benchmarks Standards and Evaluations , 2 (4), 100089. https://doi.org/10.1016/j.tbench.2023.100089 .
Hasan, R., Shams, R., & Rahman, M. (2021). Consumer Trust and Perceived Risk for Voice-controlled Artificial Intelligence: The case of Siri. Journal of Business Research , 131 , 591–597.
Heisig, P. (2009). Harmonisation of Knowledge management–comparing 160 km frameworks around the Globe. Journal of Knowledge Management , 13 (4), 4–31.
Heo, M., & Lee, K. J. (2018). Chatbot as a New Business Communication Tool: The case of Naver Talktalk. Business Communication Research and Practice , 1 (1), 41–45.
Hocutt, D. L., Ranade, N., & Verhulsdonck, G. (2022). Localizing content: The roles of Technical & Professional communicators and Machine Learning in Personalized Chatbot responses. Technical Communication , 69 (4), 114.
Huang, Y. C. (2023). Integrated concepts of the Utaut and Tpb in virtual reality behavioral intention. Journal of Retailing and Consumer Services , 70 , 103127.
Huang, Z., & Benyoucef, M. (2013). From E-Commerce to Social Commerce: A close look at design features. Electronic Commerce Research and Applications , 12 (4), 246–259.
Huang, C. Y., Yang, M. C., & Huang, C. Y. (2021). An empirical study on factors influencing consumer adoption intention of an Ai-Powered Chatbot for Health and Weight Management. International Journal of Performability Engineering , 17 (5), 422–432.
Hund, A., Wagner, H. T., Beimborn, D., & Weitzel, T. (2021). Digital Innovation: Review and Novel Perspective. The Journal of Strategic Information Systems , 30 (4), 101695. https://doi.org/10.1016/j.jsis.2021.101695 .
Ischen, C., Araujo, T., Voorveld, H., van Noort, G., & Smit, E. (2020). Privacy Concerns in Chatbot Interactions. Chatbot Research and Design: Third International Workshop, CONVERSATIONS 2019, Amsterdam, The Netherlands, November 19–20, 2019, Revised Selected Papers 3.
Ivanov, S. H., & Webster, C. (2017). Adoption of Robots, Artificial Intelligence and Service Automation by Travel, Tourism and Hospitality Companies–a Cost-Benefit Analysis. Artificial Intelligence and Service Automation by Travel, Tourism and Hospitality Companies–A Cost-Benefit Analysis .
Jarrahi, M. H., Askay, D., Eshraghi, A., & Smith, P. (2023). Artificial Intelligence and Knowledge Management: A Partnership between Human and Ai. Business Horizons , 66 (1), 87–99. https://doi.org/10.1016/j.bushor.2022.03.002 .
Jo, H. (2022). Continuance intention to Use Artificial Intelligence Personal Assistant: Type, gender, and use experience. Heliyon , 8 (9), e10662. https://doi.org/10.1016/j.heliyon.2022.e10662 .
Kalla, D., & Smith, N. (2023). Study and Analysis of Chat Gpt and its impact on different fields of Study. International Journal of Innovative Science and Research Technology , 8 (3).
Kapoor, A. P., & Vij, M. (2018). Technology at the dinner table: Ordering Food Online through Mobile apps. Journal of Retailing and Consumer Services , 43 , 342–351.
Kasneci, E., Sessler, K., Küchemann, S., Bannert, M., Dementieva, D., Fischer, F., Gasser, U., Groh, G., Günnemann, S., Hüllermeier, E., Krusche, S., Kutyniok, G., Michaeli, T., Nerdel, C., Pfeffer, J., Poquet, O., Sailer, M., Schmidt, A., Seidel, T., & Kasneci, G. (2023). Chatgpt for Good? On opportunities and challenges of large Language models for Education. Learning and Individual Differences , 103 , 102274. https://doi.org/10.1016/j.lindif.2023.102274 .
Kelly, S., Kaye, S. A., & Oviedo-Trespalacios, O. (2022). A Multi-Industry Analysis of the Future Use of Ai Chatbots. Human Behavior and Emerging Technologies , 2022 .
Khan, U., Dhar, R., & Wertenbroch, K. (2005). A behavioral decision theory perspective on hedonic and utilitarian choice. Inside consumption (pp. 166–187). Routledge.
Khasawneh, O. Y. (2018a). Technophobia without boarders: The influence of Technophobia and Emotional Intelligence on Technology Acceptance and the moderating influence of Organizational Climate. Computers in Human Behavior , 88 , 210–218. https://doi.org/10.1016/j.chb.2018.07.007 .
Khasawneh, O. Y. (2018b). Technophobia: Examining its hidden factors and defining it. Technology in Society , 54 , 93–100.
Khasawneh, O. Y. (2022). Technophobia: How students’ Technophobia impacts their Technology Acceptance in an online class. International Journal of Human–Computer Interaction , 1–10. https://doi.org/10.1080/10447318.2022.2085398 .
Kim, D., & Benbasat, I. (2006). The effects of Trust-assuring arguments on Consumer Trust in Internet stores: Application of toulmin’s model of Argumentation. Information Systems Research , 17 (3), 286–300.
King, M. R., & ChatGPT. (2023). A conversation on Artificial Intelligence, Chatbots, and Plagiarism in Higher Education. Cellular and Molecular Bioengineering , 16 (1), 1–2.
Kokolakis, S. (2017). Privacy attitudes and privacy behaviour: A review of current research on the privacy Paradox Phenomenon. Computers & Security , 64 , 122–134.
Komiak, S. Y., & Benbasat, I. (2006). The effects of personalization and familiarity on Trust and Adoption of Recommendation agents. MIS Quarterly , 941–960.
Kotze, T. G., Anderson, O., & Summerfield, K. (2016). Technophobia: Gender differences in the adoption of high-technology Consumer products. South African Journal of Business Management , 47 (1), 21–28.
Koubaa, A., Boulila, W., Ghouti, L., Alzahem, A., & Latif, S. (2023). Exploring Chatgpt Capabilities and Limitations: A Critical Review of the Nlp Game Changer . https://doi.org/10.20944/preprints202303.0438.v1 .
Koul, S., & Eydgahi, A. (2020). The Impact of Social Influence, Technophobia, and Perceived Safety on Autonomous Vehicle Technology Adoption. Periodica Polytechnica Transportation Engineering , 48 (2), 133–142.
Lazarus, R. S. (1991). Emotion and adaptation . Oxford University Press.
Lund, B. D., & Wang, T. (2023). Chatting about Chatgpt: How may ai and gpt impact academia and libraries? Library Hi Tech News.
Lutz, C., & Tamò-Larrieux, A. (2021). Do Privacy Concerns About Social Robots Affect Use Intentions? Evidence from an Experimental Vignette Study [Original Research]. Frontiers in Robotics and AI , 8 . https://doi.org/10.3389/frobt.2021.627958 .
Maddigan, P., & Susnjak, T. (2023). Chat2vis: Generating Data Visualisations Via Natural Language Using Chatgpt, Codex and Gpt-3 Large Language Models. Ieee Access .
Maghsudi, S., Lan, A., Xu, J., & van Der Schaar, M. (2021). Personalized education in the Artificial Intelligence Era: What to expect Next. IEEE Signal Processing Magazine , 38 (3), 37–50.
Makice, K. (2009). Twitter Api: Up and running: Learn how to build applications with the Twitter Api . O’Reilly Media, Inc.
Manikonda, L., Deotale, A., & Kambhampati, S. (2018). What’s up with Privacy? User Preferences and Privacy Concerns in Intelligent Personal Assistants. Proceedings of the 2018 AAAI/ACM Conference on AI, Ethics, and Society.
Marakas, G. M., Yi, M. Y., & Johnson, R. D. (1998). The Multilevel and Multifaceted Character of Computer Self-Efficacy: Toward clarification of the Construct and an Integrative Framework for Research. Information Systems Research , 9 (2), 126–163.
Masood, A., Luqman, A., Feng, Y., & Shahzad, F. (2022). Untangling the adverse effect of Sns stressors on Academic Performance and its impact on students’ social media discontinuation intention: The moderating role of guilt. SAGE Open , 12 (1), 21582440221079905. https://doi.org/10.1177/21582440221079905 .
McCallum, S. (2023). Chatgpt Banned in Italy over Privacy Concerns . BBC. Retrieved May 1 from https://www.bbc.com/news/technology-65139406 .
McGee, R. W. (2023). Is Chat Gpt Biased against Conservatives? An Empirical Study. An Empirical Study (February 15, 2023) .
McLean, G., & Osei-Frimpong, K. (2019). Hey Alexa… examine the variables influencing the Use of Artificial Intelligent in-home Voice assistants. Computers in Human Behavior , 99 , 28–37.
Meng, B., & Choi, K. (2019). Tourists’ intention to use location-based services (lbs). International Journal of Contemporary Hospitality Management , 31 (8), 3097–3115. https://doi.org/10.1108/IJCHM-09-2018-0734 .
Mohd Rahim, N. I., Iahad, A., Yusof, N., A. F., & Al-Sharafi, A., M (2022). Ai-Based Chatbots Adoption Model for higher-education institutions: A hybrid pls-sem-neural Network Modelling Approach. Sustainability , 14 (19), 12726.
Moussawi, S., Koufaris, M., & Benbunan-Fich, R. (2021). How perceptions of intelligence and anthropomorphism affect adoption of Personal Intelligent agents. Electronic Markets , 31 (2), 343–364. https://doi.org/10.1007/s12525-020-00411-w .
Nguyen, D. M., Chiu, Y. T. H., & Le, H. D. (2021). Determinants of Continuance Intention Towards Banks’ Chatbot Services in Vietnam: A Necessity for Sustainable Development. Sustainability , 13 (14), 7625. https://www.mdpi.com/2071-1050/13/14/7625 .
Nonaka, I. (1994). A dynamic theory of Organizational Knowledge Creation. Organization Science , 5 (1), 14–37.
Pillai, R., & Sivathanu, B. (2020). Adoption of Ai-Based chatbots for hospitality and tourism. International Journal of Contemporary Hospitality Management , 32 (10), 3199–3226. https://doi.org/10.1108/IJCHM-04-2020-0259 .
Podsakoff, P. M., MacKenzie, M., Scott, B., Lee, J. Y., & Podsakoff, N. P. (2003). Common method biases in behavioral research: A critical review of the literature and recommended remedies. Journal of Applied Psychology , 885 (879). 10.1037.
Rafiq, F., Dogra, N., Adil, M., & Wu, J. Z. (2022). Examining Consumer’s intention to adopt Ai-Chatbots in Tourism using partial least squares structural equation modeling method. Mathematics , 10 (13), 2190.
Rijsdijk, S. A., Hultink, E. J., & Diamantopoulos, A. (2007). Product Intelligence: Its conceptualization, measurement and impact on consumer satisfaction. Journal of the Academy of Marketing Science , 35 , 340–356.
Rogers, E. (1983). Diffusion of Innovations, 3rd Editio Ed. In: New York.
Rogers, E. M. (2010). Diffusion of innovations . Simon and Schuster.
Rospigliosi, P. (2023). Artificial Intelligence in Teaching and Learning: What questions should we ask of Chatgpt? In (Vol. 31, pp. 1–3). Taylor & Francis.
Rudolph, J., Tan, S., & Tan, S. (2023). Chatgpt: Bullshit Spewer or the end of traditional assessments in higher education? Journal of Applied Learning and Teaching , 6 (1).
Ryan, R. M., & Deci, E. L. (2000). Self-determination theory and the facilitation of intrinsic motivation, Social Development, and well-being. American Psychologist , 55 (1), 68.
Selamat, M. A., & Windasari, N. A. (2021). Chatbot for smes: Integrating customer and business owner perspectives. Technology in Society , 66 , 101685.
Selwyn, N. (2013). Discourses of Digital Disruption in Education: A Critical Analysis. In Fifth International Roundtable on Discourse Analysis, City University Hong Kong, May 23–25, 2013 (pp. 1–28).
Smith, H. J., Dinev, T., & Xu, H. (2011). Information Privacy Research: An Interdisciplinary Review. MIS Quarterly , 989–1015.
Song, H. G., & Jo, H. (2023). Understanding the Continuance Intention of Omnichannel: Combining Tam and Tpb. Sustainability , 15 (4), 3039.
Sun, Y., & Jeyaraj, A. (2013). Information Technology Adoption and Continuance: A longitudinal study of individuals’ behavioral intentions. Information & Management , 50 (7), 457–465.
Tarafdar, M., Tu, Q., Ragu-Nathan, B. S., & Ragu-Nathan, T. (2007). The impact of technostress on role stress and Productivity. Journal of Management Information Systems , 24 (1), 301–328.
Urbach, N., Smolnik, S., & Riempp, G. (2010). An empirical investigation of employee Portal Success. The Journal of Strategic Information Systems , 19 (3), 184–206. https://doi.org/10.1016/j.jsis.2010.06.002 .
Varma Citrin, A., Sprott, D. E., Silverman, S. N., & Stem, D. E. (2000). Adoption of internet shopping: The role of consumer innovativeness. Industrial Management & Data Systems , 100 (7), 294–300. https://doi.org/10.1108/02635570010304806 .
Vázquez-Cano, E., Mengual-Andrés, S., & López-Meneses, E. (2021). Chatbot to Improve Learning Punctuation in Spanish and to Enhance Open and Flexible Learning environments. International Journal of Educational Technology in Higher Education , 18 (1), 33. https://doi.org/10.1186/s41239-021-00269-8 .
Venkatesh, V., Thong, J. Y. L., & Xu, X. (2012). Consumer Acceptance and Use of Information Technology: Extending the Unified Theory of Acceptance and Use of Technology. MIS Quarterly , 36 (1), 157–178. https://doi.org/10.2307/41410412 .
Wirtz, J., Patterson, P. G., Kunz, W. H., Gruber, T., Lu, V. N., Paluch, S., & Martins, A. (2018). Brave New World: Service Robots in the Frontline. Journal of Service Management , 29 (5), 907–931. https://doi.org/10.1108/JOSM-04-2018-0119 .
Xi, W., Zhang, X., & Ayalon, L. (2022). When less intergenerational closeness helps: The influence of intergenerational physical proximity and technology attributes on Technophobia among older adults. Computers in Human Behavior , 131 , 107234. https://doi.org/10.1016/j.chb.2022.107234 .
Yang, R., & Wibowo, S. (2022). User Trust in Artificial Intelligence: A comprehensive conceptual Framework. Electronic Markets , 32 (4), 2053–2077. https://doi.org/10.1007/s12525-022-00592-6 .
Yu, H., Miao, C., Leung, C., & White, T. J. (2017). Towards Ai-Powered personalization in Mooc Learning. Npj Science of Learning , 2 (1), 15.
Zeelenberg, M., & Pieters, R. (2007). A theory of Regret Regulation 1.0. Journal of Consumer Psychology , 17 (1), 3–18.
Zhu, X., Cao, Q., & Liu, C. (2022). Mechanism of Platform Interaction on Social Media Users&Rsquo Intention to Disclose Privacy: A Case Study of Tiktok App. Information , 13 (10), 461. https://www.mdpi.com/2078-2489/13/10/461 .
Download references
Acknowledgements
The author received no financial support for the research, authorship, and/or publication of this article.
Author information
Authors and affiliations.
Headquarters, HJ Institute of Technology and Management, Jungdong-ro 71 39, Bucheon-si, Gyeonggi-do, 14721, Republic of Korea
You can also search for this author in PubMed Google Scholar
Corresponding author
Correspondence to Hyeon Jo .
Ethics declarations
Declaration of generative ai and ai-assisted technologies in the writing process.
During the preparation of this work the author(s) used ChatGPT in order to improve language and readability. After using this tool/service, the author(s) reviewed and edited the content as needed and take(s) full responsibility for the content of the publication.
Additional information
Publisher’s note.
Springer Nature remains neutral with regard to jurisdictional claims in published maps and institutional affiliations.
Rights and permissions
Open Access This article is licensed under a Creative Commons Attribution 4.0 International License, which permits use, sharing, adaptation, distribution and reproduction in any medium or format, as long as you give appropriate credit to the original author(s) and the source, provide a link to the Creative Commons licence, and indicate if changes were made. The images or other third party material in this article are included in the article’s Creative Commons licence, unless indicated otherwise in a credit line to the material. If material is not included in the article’s Creative Commons licence and your intended use is not permitted by statutory regulation or exceeds the permitted use, you will need to obtain permission directly from the copyright holder. To view a copy of this licence, visit http://creativecommons.org/licenses/by/4.0/ .
Reprints and permissions
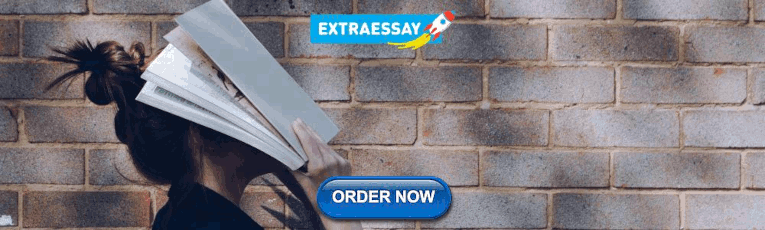
About this article
Cite this article.
Jo, H. From concerns to benefits: a comprehensive study of ChatGPT usage in education. Int J Educ Technol High Educ 21 , 35 (2024). https://doi.org/10.1186/s41239-024-00471-4
Download citation
Received : 05 February 2024
Accepted : 25 April 2024
Published : 03 June 2024
DOI : https://doi.org/10.1186/s41239-024-00471-4
Share this article
Anyone you share the following link with will be able to read this content:
Sorry, a shareable link is not currently available for this article.
Provided by the Springer Nature SharedIt content-sharing initiative
- Artificial Intelligence
Why the Pandemic Probably Started in a Lab, in 5 Key Points

By Alina Chan
Dr. Chan is a molecular biologist at the Broad Institute of M.I.T. and Harvard, and a co-author of “Viral: The Search for the Origin of Covid-19.”
This article has been updated to reflect news developments.
On Monday, Dr. Anthony Fauci returned to the halls of Congress and testified before the House subcommittee investigating the Covid-19 pandemic. He was questioned about several topics related to the government’s handling of Covid-19, including how the National Institute of Allergy and Infectious Diseases, which he directed until retiring in 2022, supported risky virus work at a Chinese institute whose research may have caused the pandemic.
For more than four years, reflexive partisan politics have derailed the search for the truth about a catastrophe that has touched us all. It has been estimated that at least 25 million people around the world have died because of Covid-19, with over a million of those deaths in the United States.
Although how the pandemic started has been hotly debated, a growing volume of evidence — gleaned from public records released under the Freedom of Information Act, digital sleuthing through online databases, scientific papers analyzing the virus and its spread, and leaks from within the U.S. government — suggests that the pandemic most likely occurred because a virus escaped from a research lab in Wuhan, China. If so, it would be the most costly accident in the history of science.
Here’s what we now know:
1 The SARS-like virus that caused the pandemic emerged in Wuhan, the city where the world’s foremost research lab for SARS-like viruses is located.
- At the Wuhan Institute of Virology, a team of scientists had been hunting for SARS-like viruses for over a decade, led by Shi Zhengli.
- Their research showed that the viruses most similar to SARS‑CoV‑2, the virus that caused the pandemic, circulate in bats that live r oughly 1,000 miles away from Wuhan. Scientists from Dr. Shi’s team traveled repeatedly to Yunnan province to collect these viruses and had expanded their search to Southeast Asia. Bats in other parts of China have not been found to carry viruses that are as closely related to SARS-CoV-2.

The closest known relatives to SARS-CoV-2 were found in southwestern China and in Laos.
Large cities
Mine in Yunnan province
Cave in Laos
South China Sea

The closest known relatives to SARS-CoV-2
were found in southwestern China and in Laos.
philippines

The closest known relatives to SARS-CoV-2 were found
in southwestern China and Laos.
Sources: Sarah Temmam et al., Nature; SimpleMaps
Note: Cities shown have a population of at least 200,000.

There are hundreds of large cities in China and Southeast Asia.

There are hundreds of large cities in China
and Southeast Asia.

The pandemic started roughly 1,000 miles away, in Wuhan, home to the world’s foremost SARS-like virus research lab.

The pandemic started roughly 1,000 miles away,
in Wuhan, home to the world’s foremost SARS-like virus research lab.

The pandemic started roughly 1,000 miles away, in Wuhan,
home to the world’s foremost SARS-like virus research lab.
- Even at hot spots where these viruses exist naturally near the cave bats of southwestern China and Southeast Asia, the scientists argued, as recently as 2019 , that bat coronavirus spillover into humans is rare .
- When the Covid-19 outbreak was detected, Dr. Shi initially wondered if the novel coronavirus had come from her laboratory , saying she had never expected such an outbreak to occur in Wuhan.
- The SARS‑CoV‑2 virus is exceptionally contagious and can jump from species to species like wildfire . Yet it left no known trace of infection at its source or anywhere along what would have been a thousand-mile journey before emerging in Wuhan.
2 The year before the outbreak, the Wuhan institute, working with U.S. partners, had proposed creating viruses with SARS‑CoV‑2’s defining feature.
- Dr. Shi’s group was fascinated by how coronaviruses jump from species to species. To find viruses, they took samples from bats and other animals , as well as from sick people living near animals carrying these viruses or associated with the wildlife trade. Much of this work was conducted in partnership with the EcoHealth Alliance, a U.S.-based scientific organization that, since 2002, has been awarded over $80 million in federal funding to research the risks of emerging infectious diseases.
- The laboratory pursued risky research that resulted in viruses becoming more infectious : Coronaviruses were grown from samples from infected animals and genetically reconstructed and recombined to create new viruses unknown in nature. These new viruses were passed through cells from bats, pigs, primates and humans and were used to infect civets and humanized mice (mice modified with human genes). In essence, this process forced these viruses to adapt to new host species, and the viruses with mutations that allowed them to thrive emerged as victors.
- By 2019, Dr. Shi’s group had published a database describing more than 22,000 collected wildlife samples. But external access was shut off in the fall of 2019, and the database was not shared with American collaborators even after the pandemic started , when such a rich virus collection would have been most useful in tracking the origin of SARS‑CoV‑2. It remains unclear whether the Wuhan institute possessed a precursor of the pandemic virus.
- In 2021, The Intercept published a leaked 2018 grant proposal for a research project named Defuse , which had been written as a collaboration between EcoHealth, the Wuhan institute and Ralph Baric at the University of North Carolina, who had been on the cutting edge of coronavirus research for years. The proposal described plans to create viruses strikingly similar to SARS‑CoV‑2.
- Coronaviruses bear their name because their surface is studded with protein spikes, like a spiky crown, which they use to enter animal cells. T he Defuse project proposed to search for and create SARS-like viruses carrying spikes with a unique feature: a furin cleavage site — the same feature that enhances SARS‑CoV‑2’s infectiousness in humans, making it capable of causing a pandemic. Defuse was never funded by the United States . However, in his testimony on Monday, Dr. Fauci explained that the Wuhan institute would not need to rely on U.S. funding to pursue research independently.

The Wuhan lab ran risky experiments to learn about how SARS-like viruses might infect humans.
1. Collect SARS-like viruses from bats and other wild animals, as well as from people exposed to them.

2. Identify high-risk viruses by screening for spike proteins that facilitate infection of human cells.

2. Identify high-risk viruses by screening for spike proteins that facilitate infection of
human cells.

In Defuse, the scientists proposed to add a furin cleavage site to the spike protein.
3. Create new coronaviruses by inserting spike proteins or other features that could make the viruses more infectious in humans.

4. Infect human cells, civets and humanized mice with the new coronaviruses, to determine how dangerous they might be.

- While it’s possible that the furin cleavage site could have evolved naturally (as seen in some distantly related coronaviruses), out of the hundreds of SARS-like viruses cataloged by scientists, SARS‑CoV‑2 is the only one known to possess a furin cleavage site in its spike. And the genetic data suggest that the virus had only recently gained the furin cleavage site before it started the pandemic.
- Ultimately, a never-before-seen SARS-like virus with a newly introduced furin cleavage site, matching the description in the Wuhan institute’s Defuse proposal, caused an outbreak in Wuhan less than two years after the proposal was drafted.
- When the Wuhan scientists published their seminal paper about Covid-19 as the pandemic roared to life in 2020, they did not mention the virus’s furin cleavage site — a feature they should have been on the lookout for, according to their own grant proposal, and a feature quickly recognized by other scientists.
- Worse still, as the pandemic raged, their American collaborators failed to publicly reveal the existence of the Defuse proposal. The president of EcoHealth, Peter Daszak, recently admitted to Congress that he doesn’t know about virus samples collected by the Wuhan institute after 2015 and never asked the lab’s scientists if they had started the work described in Defuse. In May, citing failures in EcoHealth’s monitoring of risky experiments conducted at the Wuhan lab, the Biden administration suspended all federal funding for the organization and Dr. Daszak, and initiated proceedings to bar them from receiving future grants. In his testimony on Monday, Dr. Fauci said that he supported the decision to suspend and bar EcoHealth.
- Separately, Dr. Baric described the competitive dynamic between his research group and the institute when he told Congress that the Wuhan scientists would probably not have shared their most interesting newly discovered viruses with him . Documents and email correspondence between the institute and Dr. Baric are still being withheld from the public while their release is fiercely contested in litigation.
- In the end, American partners very likely knew of only a fraction of the research done in Wuhan. According to U.S. intelligence sources, some of the institute’s virus research was classified or conducted with or on behalf of the Chinese military . In the congressional hearing on Monday, Dr. Fauci repeatedly acknowledged the lack of visibility into experiments conducted at the Wuhan institute, saying, “None of us can know everything that’s going on in China, or in Wuhan, or what have you. And that’s the reason why — I say today, and I’ve said at the T.I.,” referring to his transcribed interview with the subcommittee, “I keep an open mind as to what the origin is.”
3 The Wuhan lab pursued this type of work under low biosafety conditions that could not have contained an airborne virus as infectious as SARS‑CoV‑2.
- Labs working with live viruses generally operate at one of four biosafety levels (known in ascending order of stringency as BSL-1, 2, 3 and 4) that describe the work practices that are considered sufficiently safe depending on the characteristics of each pathogen. The Wuhan institute’s scientists worked with SARS-like viruses under inappropriately low biosafety conditions .

In the United States, virologists generally use stricter Biosafety Level 3 protocols when working with SARS-like viruses.
Biosafety cabinets prevent
viral particles from escaping.
Viral particles
Personal respirators provide
a second layer of defense against breathing in the virus.
DIRECT CONTACT
Gloves prevent skin contact.
Disposable wraparound
gowns cover much of the rest of the body.

Personal respirators provide a second layer of defense against breathing in the virus.
Disposable wraparound gowns
cover much of the rest of the body.
Note: Biosafety levels are not internationally standardized, and some countries use more permissive protocols than others.

The Wuhan lab had been regularly working with SARS-like viruses under Biosafety Level 2 conditions, which could not prevent a highly infectious virus like SARS-CoV-2 from escaping.
Some work is done in the open air, and masks are not required.
Less protective equipment provides more opportunities
for contamination.

Some work is done in the open air,
and masks are not required.
Less protective equipment provides more opportunities for contamination.
- In one experiment, Dr. Shi’s group genetically engineered an unexpectedly deadly SARS-like virus (not closely related to SARS‑CoV‑2) that exhibited a 10,000-fold increase in the quantity of virus in the lungs and brains of humanized mice . Wuhan institute scientists handled these live viruses at low biosafet y levels , including BSL-2.
- Even the much more stringent containment at BSL-3 cannot fully prevent SARS‑CoV‑2 from escaping . Two years into the pandemic, the virus infected a scientist in a BSL-3 laboratory in Taiwan, which was, at the time, a zero-Covid country. The scientist had been vaccinated and was tested only after losing the sense of smell. By then, more than 100 close contacts had been exposed. Human error is a source of exposure even at the highest biosafety levels , and the risks are much greater for scientists working with infectious pathogens at low biosafety.
- An early draft of the Defuse proposal stated that the Wuhan lab would do their virus work at BSL-2 to make it “highly cost-effective.” Dr. Baric added a note to the draft highlighting the importance of using BSL-3 to contain SARS-like viruses that could infect human cells, writing that “U.S. researchers will likely freak out.” Years later, after SARS‑CoV‑2 had killed millions, Dr. Baric wrote to Dr. Daszak : “I have no doubt that they followed state determined rules and did the work under BSL-2. Yes China has the right to set their own policy. You believe this was appropriate containment if you want but don’t expect me to believe it. Moreover, don’t insult my intelligence by trying to feed me this load of BS.”
- SARS‑CoV‑2 is a stealthy virus that transmits effectively through the air, causes a range of symptoms similar to those of other common respiratory diseases and can be spread by infected people before symptoms even appear. If the virus had escaped from a BSL-2 laboratory in 2019, the leak most likely would have gone undetected until too late.
- One alarming detail — leaked to The Wall Street Journal and confirmed by current and former U.S. government officials — is that scientists on Dr. Shi’s team fell ill with Covid-like symptoms in the fall of 2019 . One of the scientists had been named in the Defuse proposal as the person in charge of virus discovery work. The scientists denied having been sick .
4 The hypothesis that Covid-19 came from an animal at the Huanan Seafood Market in Wuhan is not supported by strong evidence.
- In December 2019, Chinese investigators assumed the outbreak had started at a centrally located market frequented by thousands of visitors daily. This bias in their search for early cases meant that cases unlinked to or located far away from the market would very likely have been missed. To make things worse, the Chinese authorities blocked the reporting of early cases not linked to the market and, claiming biosafety precautions, ordered the destruction of patient samples on January 3, 2020, making it nearly impossible to see the complete picture of the earliest Covid-19 cases. Information about dozens of early cases from November and December 2019 remains inaccessible.
- A pair of papers published in Science in 2022 made the best case for SARS‑CoV‑2 having emerged naturally from human-animal contact at the Wuhan market by focusing on a map of the early cases and asserting that the virus had jumped from animals into humans twice at the market in 2019. More recently, the two papers have been countered by other virologists and scientists who convincingly demonstrate that the available market evidence does not distinguish between a human superspreader event and a natural spillover at the market.
- Furthermore, the existing genetic and early case data show that all known Covid-19 cases probably stem from a single introduction of SARS‑CoV‑2 into people, and the outbreak at the Wuhan market probably happened after the virus had already been circulating in humans.

An analysis of SARS-CoV-2’s evolutionary tree shows how the virus evolved as it started to spread through humans.
SARS-COV-2 Viruses closest
to bat coronaviruses
more mutations

Source: Lv et al., Virus Evolution (2024) , as reproduced by Jesse Bloom

The viruses that infected people linked to the market were most likely not the earliest form of the virus that started the pandemic.

- Not a single infected animal has ever been confirmed at the market or in its supply chain. Without good evidence that the pandemic started at the Huanan Seafood Market, the fact that the virus emerged in Wuhan points squarely at its unique SARS-like virus laboratory.
5 Key evidence that would be expected if the virus had emerged from the wildlife trade is still missing.

In previous outbreaks of coronaviruses, scientists were able to demonstrate natural origin by collecting multiple pieces of evidence linking infected humans to infected animals.
Infected animals
Earliest known
cases exposed to
live animals
Antibody evidence
of animals and
animal traders having
been infected
Ancestral variants
of the virus found in
Documented trade
of host animals
between the area
where bats carry
closely related viruses
and the outbreak site

Infected animals found
Earliest known cases exposed to live animals
Antibody evidence of animals and animal
traders having been infected
Ancestral variants of the virus found in animals
Documented trade of host animals
between the area where bats carry closely
related viruses and the outbreak site

For SARS-CoV-2, these same key pieces of evidence are still missing , more than four years after the virus emerged.

For SARS-CoV-2, these same key pieces of evidence are still missing ,
more than four years after the virus emerged.
- Despite the intense search trained on the animal trade and people linked to the market, investigators have not reported finding any animals infected with SARS‑CoV‑2 that had not been infected by humans. Yet, infected animal sources and other connective pieces of evidence were found for the earlier SARS and MERS outbreaks as quickly as within a few days, despite the less advanced viral forensic technologies of two decades ago.
- Even though Wuhan is the home base of virus hunters with world-leading expertise in tracking novel SARS-like viruses, investigators have either failed to collect or report key evidence that would be expected if Covid-19 emerged from the wildlife trade . For example, investigators have not determined that the earliest known cases had exposure to intermediate host animals before falling ill. No antibody evidence shows that animal traders in Wuhan are regularly exposed to SARS-like viruses, as would be expected in such situations.
- With today’s technology, scientists can detect how respiratory viruses — including SARS, MERS and the flu — circulate in animals while making repeated attempts to jump across species . Thankfully, these variants usually fail to transmit well after crossing over to a new species and tend to die off after a small number of infections. In contrast, virologists and other scientists agree that SARS‑CoV‑2 required little to no adaptation to spread rapidly in humans and other animals . The virus appears to have succeeded in causing a pandemic upon its only detected jump into humans.
The pandemic could have been caused by any of hundreds of virus species, at any of tens of thousands of wildlife markets, in any of thousands of cities, and in any year. But it was a SARS-like coronavirus with a unique furin cleavage site that emerged in Wuhan, less than two years after scientists, sometimes working under inadequate biosafety conditions, proposed collecting and creating viruses of that same design.
While several natural spillover scenarios remain plausible, and we still don’t know enough about the full extent of virus research conducted at the Wuhan institute by Dr. Shi’s team and other researchers, a laboratory accident is the most parsimonious explanation of how the pandemic began.
Given what we now know, investigators should follow their strongest leads and subpoena all exchanges between the Wuhan scientists and their international partners, including unpublished research proposals, manuscripts, data and commercial orders. In particular, exchanges from 2018 and 2019 — the critical two years before the emergence of Covid-19 — are very likely to be illuminating (and require no cooperation from the Chinese government to acquire), yet they remain beyond the public’s view more than four years after the pandemic began.
Whether the pandemic started on a lab bench or in a market stall, it is undeniable that U.S. federal funding helped to build an unprecedented collection of SARS-like viruses at the Wuhan institute, as well as contributing to research that enhanced them . Advocates and funders of the institute’s research, including Dr. Fauci, should cooperate with the investigation to help identify and close the loopholes that allowed such dangerous work to occur. The world must not continue to bear the intolerable risks of research with the potential to cause pandemics .
A successful investigation of the pandemic’s root cause would have the power to break a decades-long scientific impasse on pathogen research safety, determining how governments will spend billions of dollars to prevent future pandemics. A credible investigation would also deter future acts of negligence and deceit by demonstrating that it is indeed possible to be held accountable for causing a viral pandemic. Last but not least, people of all nations need to see their leaders — and especially, their scientists — heading the charge to find out what caused this world-shaking event. Restoring public trust in science and government leadership requires it.
A thorough investigation by the U.S. government could unearth more evidence while spurring whistleblowers to find their courage and seek their moment of opportunity. It would also show the world that U.S. leaders and scientists are not afraid of what the truth behind the pandemic may be.
More on how the pandemic may have started

Where Did the Coronavirus Come From? What We Already Know Is Troubling.
Even if the coronavirus did not emerge from a lab, the groundwork for a potential disaster had been laid for years, and learning its lessons is essential to preventing others.
By Zeynep Tufekci

Why Does Bad Science on Covid’s Origin Get Hyped?
If the raccoon dog was a smoking gun, it fired blanks.
By David Wallace-Wells

A Plea for Making Virus Research Safer
A way forward for lab safety.
By Jesse Bloom
The Times is committed to publishing a diversity of letters to the editor. We’d like to hear what you think about this or any of our articles. Here are some tips . And here’s our email: [email protected] .
Follow the New York Times Opinion section on Facebook , Instagram , TikTok , WhatsApp , X and Threads .
Alina Chan ( @ayjchan ) is a molecular biologist at the Broad Institute of M.I.T. and Harvard, and a co-author of “ Viral : The Search for the Origin of Covid-19.” She was a member of the Pathogens Project , which the Bulletin of the Atomic Scientists organized to generate new thinking on responsible, high-risk pathogen research.
- Share full article
Advertisement
Help | Advanced Search
Computer Science > Computer Vision and Pattern Recognition
Title: the crystal ball hypothesis in diffusion models: anticipating object positions from initial noise.
Abstract: Diffusion models have achieved remarkable success in text-to-image generation tasks; however, the role of initial noise has been rarely explored. In this study, we identify specific regions within the initial noise image, termed trigger patches, that play a key role for object generation in the resulting images. Notably, these patches are ``universal'' and can be generalized across various positions, seeds, and prompts. To be specific, extracting these patches from one noise and injecting them into another noise leads to object generation in targeted areas. We identify these patches by analyzing the dispersion of object bounding boxes across generated images, leading to the development of a posterior analysis technique. Furthermore, we create a dataset consisting of Gaussian noises labeled with bounding boxes corresponding to the objects appearing in the generated images and train a detector that identifies these patches from the initial noise. To explain the formation of these patches, we reveal that they are outliers in Gaussian noise, and follow distinct distributions through two-sample tests. Finally, we find the misalignment between prompts and the trigger patch patterns can result in unsuccessful image generations. The study proposes a reject-sampling strategy to obtain optimal noise, aiming to improve prompt adherence and positional diversity in image generation.
Submission history
Access paper:.
- HTML (experimental)
- Other Formats

References & Citations
- Google Scholar
- Semantic Scholar
BibTeX formatted citation

Bibliographic and Citation Tools
Code, data and media associated with this article, recommenders and search tools.
- Institution
arXivLabs: experimental projects with community collaborators
arXivLabs is a framework that allows collaborators to develop and share new arXiv features directly on our website.
Both individuals and organizations that work with arXivLabs have embraced and accepted our values of openness, community, excellence, and user data privacy. arXiv is committed to these values and only works with partners that adhere to them.
Have an idea for a project that will add value for arXiv's community? Learn more about arXivLabs .
Google research shows the fast rise of AI-generated misinformation
Artificial intelligence has become a source of misinformation with lightning speed.
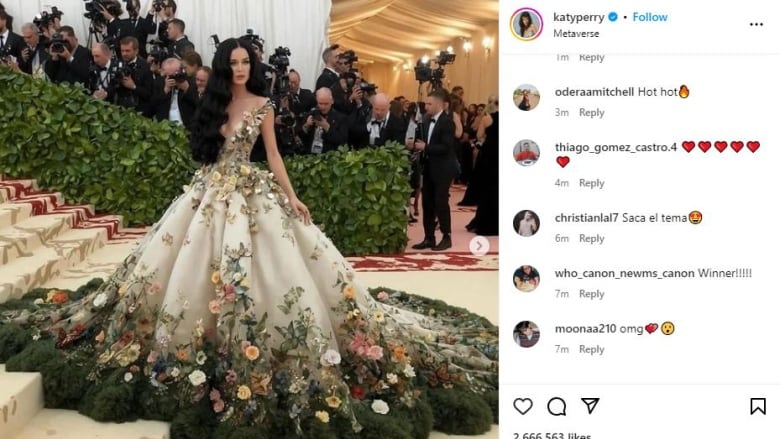
Social Sharing
From fake images of war to celebrity hoaxes, artificial intelligence technology has spawned new forms of reality-warping misinformation online. New analysis co-authored by Google researchers shows just how quickly the problem has grown.
The research, co-authored by researchers from Google, Duke University and several fact-checking and media organizations, was published in a preprint last week. The paper introduces a massive new dataset of misinformation going back to 1995 that was fact-checked by websites like Snopes.
According to the researchers, the data reveals that AI-generated images have quickly risen in prominence, becoming nearly as popular as more traditional forms of manipulation.
- Don't believe your eyes — fake photos have been a problem for a long time
- Analysis With rise of AI-generated images, distinguishing real from fake is about to get a lot harder
The work was first reported by 404 Media after being spotted by the Faked Up newsletter, and it clearly shows that "AI-generated images made up a minute proportion of content manipulations overall until early last year," the researchers wrote.
Last year saw the release of new AI image-generation tools by major players in tech, including OpenAI, Microsoft and Google itself. Now, AI-generated misinformation is "nearly as common as text and general content manipulations," the paper said.
The researchers note that the uptick in fact-checking AI images coincided with a general wave of AI hype, which may have led websites to focus on the technology. The dataset shows that fact-checking AI has slowed down in recent months, with traditional text and image manipulation seeing an increase.
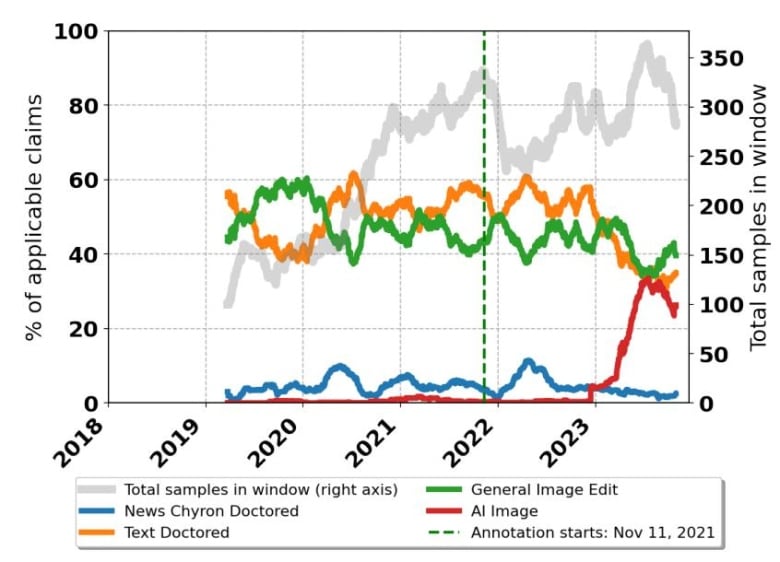
The study looked at other forms of media, too, and found that video hoaxes now make up roughly 60 per cent of all fact-checked claims that include media.
That doesn't mean AI-generated misinformation has slowed down, said Sasha Luccioni, a leading AI ethics researcher at machine learning platform Hugging Face.
"Personally, I feel like this is because there are so many [examples of AI misinformation] that it's hard to keep track!" Luccioni said in an email. "I see them regularly myself, even outside of social media, in advertising, for instance."
- Explicit fake images of Taylor Swift prove laws haven't kept pace with tech, experts say
- Fake photos, but make it fashion. Why the Met Gala pics are just the beginning of AI deception
AI has been used to generate fake images of real people, with concerning effects. For example, fake nude images of Taylor Swift circulated earlier this year. 404 Media reported that the tool used to create the images was Microsoft's AI-generation software, which it licenses from ChatGPT maker OpenAI — prompting the tech giant to close a loophole allowing the images to be generated.
The technology has also fooled people in more innocuous ways. Recent fake photos showing Katy Perry attending the Met Gala in New York — in reality, she never did — fooled observers on social media and even the star's own parents.
The rise of AI has caused headaches for social media companies and Google itself. Fake celebrity images have been featured prominently in Google image search results in the past, thanks to SEO-driven content farms. Using AI to manipulate search results is against Google's policies.
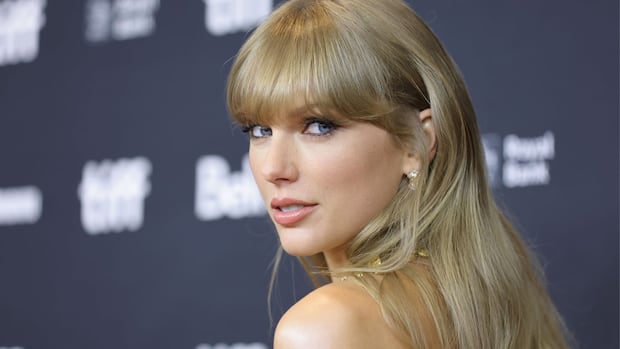
Taylor Swift deepfakes taken offline. It’s not so easy for regular people
Google spokespeople were not immediately available for comment. Previously, a spokesperson told technology news outlet Motherboard that "when we find instances where low-quality content is ranking highly, we build scalable solutions that improve the results not for just one search, but for a range of queries."
To deal with the problem of AI fakes, Google has launched such initiatives as digital waterma rking , which flags AI-generated images as fake with a mark that is invisible to the human eye. The company, along with Microsoft, Intel and Adobe, is also exploring giving creators the option to add a visible watermark to AI-generated images.
"I think if Big Tech companies collaborated on a standard of AI watermarks, that would definitely help the field as a whole at this point," Luccioni said.
ABOUT THE AUTHOR
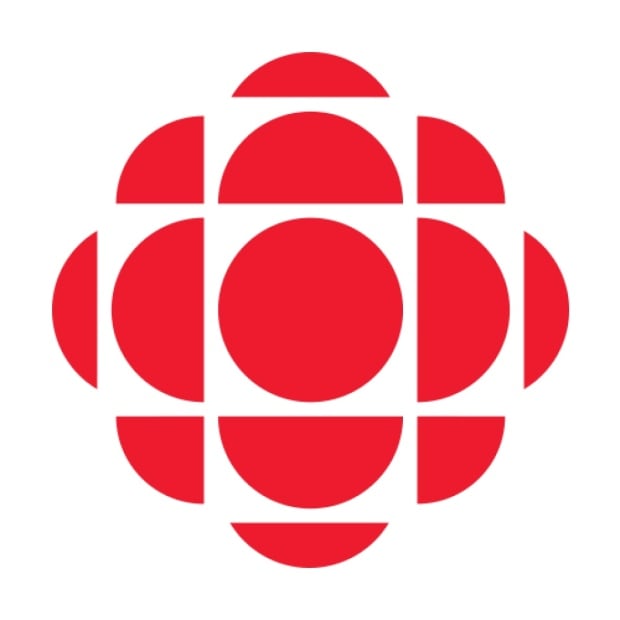
Jordan Pearson is a Toronto-based journalist and the former executive editor of Motherboard.
Related Stories
- Go Public Facebook account takeovers are targeting people you know, turning friendship into fraud
- This article is real — but AI-generated deepfakes look damn close and are scamming people
How generative AI could accelerate software product time to market
In today’s fast-paced and dynamic business landscape, software product managers (PMs) are crucial to product innovation and success, acting as the “mini CEOs” of products. In the quest to address customers’ needs, PMs are increasingly turning to generative AI (gen AI), with adoption of the technology growing exponentially in a matter of months. 1 See “ Unleashing developer productivity with generative AI ,” McKinsey, June 27, 2023.
Over the past two decades, several trends have shaped the evolution of the PM role into a mini CEO: the shift to cloud, the growing importance of data in decision making, an increased focus on customer-led design, and growing expectations for responsible stewardship regarding privacy, sustainability, and inclusion. Now, gen AI is expected to reshape the role of PMs in a much more substantive way. This technology has the potential to not only augment and expand PMs’ roles and responsibilities but also fundamentally rewire the product development life cycle (PDLC) to achieve better customer outcomes on a shorter timeline.
About the research
To understand the impact of generative AI (gen AI) on product management quality of output, experience, product time to market, and productivity, McKinsey recruited 40 product managers (PMs) with varying levels of product management experience from the United States, Canada, Europe, and Latin America to participate in a study. Data collected about each PM included years of experience working as a PM, familiarity with various technology stacks, adoption level of generative AI tools, and time typically spent on various tasks.
Before participating in the study, the PMs attended a brief training workshop to learn about the case context and familiarize themselves with the tools. Participants were then asked to play the PM role for a fictional company and to work individually through five activities at their own pace. The activities mirrored the real-life work of PMs across the three phases of the product development life cycle (PDLC)—discovery, viability, and build—and required the PMs to generate deliverables including a market research document, a press release with frequently asked questions, a product one-pager, a product requirements document, and a product backlog. Tasks were arranged in chronological order along the PDLC to emulate their real-life execution flow to the extent possible.
McKinsey divided participants into three groups and asked them to complete activities in the three stages of the PDLC using varying levels of access to gen AI tools. PMs either had access to task-specific gen AI tools (such as Copy.ai), ChatGPT only, or no gen AI tools. Each group rotated, and start and end times were recorded for all groups to measure the time spent on each task.
After the study, PMs were asked about their experiences performing the activities and how helpful they found gen AI tools to be for completing each one. McKinsey team members then reviewed deliverables to assess their quality (accuracy and completeness). Finally, a post-study survey gathered participants’ feedback on the overall experience and the likelihood that they will adopt the tools in the future.
Given the technology’s newness and rapid pace of evolution, many product leaders are unsure which tools to select, which use cases to start with, and how to help PMs make the most of gen AI opportunities while adapting ways of working. McKinsey recently conducted empirical research on PMs in Europe and the Americas to understand the potential impact of using gen AI–based tools in their roles (see sidebar, “About the research”). The results were promising. Gen AI accelerated product time to market by 5 percent, improved PM productivity by 40 percent, and even uplifted employee experience by 100 percent. This article details findings from the study to offer an early look at the quantifiable impact of gen AI on PM roles and offers insights on how product leaders can translate these findings into impact.
The impact of gen AI on product management tasks
About quantumblack, ai by mckinsey.
QuantumBlack, McKinsey’s AI arm, helps companies transform using the power of technology, technical expertise, and industry experts. With thousands of practitioners at QuantumBlack (data engineers, data scientists, product managers, designers, and software engineers) and McKinsey (industry and domain experts), we are working to solve the world’s most important AI challenges. QuantumBlack Labs is our center of technology development and client innovation, which has been driving cutting-edge advancements and developments in AI through locations across the globe.
Participants in the PM study were divided into three groups. Each group rotated through the three phases of the PDLC to individually complete the activities using varying levels of access to gen AI tools. The PMs’ experience and performance were then assessed, and several insights emerged.
Accelerated time to market
PMs who used gen AI tools—either generic tools such as ChatGPT or task-specific tools—took less time, on average, to complete activities than PMs who did not use them, resulting in an acceleration of time to market by about 5 percent across a six-month PDLC. The time savings were driven by the use of gen AI to synthesize user research and write press releases in the discovery phase; develop product one-pagers and write product requirement documents in the viability phase; and create product backlogs in the build phase. The acceleration in time to market assumes that current PDLC activities are completed more quickly and the roles and activities involved beyond PMs are rewired to support the acceleration. The adoption of gen AI tools may allow PMs to shift time and resources to more-strategic activities such as defining the product vision, creating a long-term road map, and conducting customer-facing activities.
Significant uplift in PM experience
Strikingly, 100 percent of the participants reported an improvement in their activity experience when using gen AI tools (Exhibit 1). All but one of the PMs reported the tools were helpful with the tasks and that they would be highly or somewhat likely to use these tools in their work after the study ended. And three out of four believed the quality of their deliverables was either largely improved or somewhat improved compared to what they achieved without them. PMs perceived the tools as automating their “high toil” tasks and enabling them to focus on what mattered.
Increased productivity
Gen AI tools had almost twice as much positive impact on content-heavy tasks—gathering and synthesizing information, creating and polishing content, and brainstorming—as on content-light tasks such as data gathering and visualization (Exhibit 2). In part, this is because content-heavy tasks have more potential for automation and because gen AI excels in extracting insights from unstructured data and outpacing human content generation. In other words, content-heavy tasks inherently possess greater opportunities to harness the full potential of gen AI.
General-purpose tools such as ChatGPT were more readily adopted by PMs than task-specific tools, and they resulted in about twice the productivity gains. This result is likely attributable to the fact that general tools are more familiar to PMs and thus easier to use than specialized tools. Furthermore, some specific gen AI tools are designed to address more tailored use cases and therefore require specific input and prompts that PMs are not accustomed to. In contrast, general-purpose tools allow PMs to iterate with flexibility and use the tools as partners in solving problems.
Improved quality of deliverables
Observation of the PMs and review of their deliverables suggest that on average, gen AI tools enabled them to iterate more rapidly and produce more accurate and complete output. However, the impact of gen AI generally varied based on the experience levels of the PMs using them. PMs with more years of experience maintained a high quality of output, whereas more junior PMs gained productivity but at the expense of quality.
Our hypothesis is that senior PMs, given their familiarity with the deliverables and their stronger product sense, can better review the gen AI tools’ output and ensure high accuracy and writing fluency. More junior PMs, conversely, are still learning how to produce high-quality deliverables and cannot yet effectively review gen AI outputs. Clearly, gen AI cannot replace the foundational skills needed to be a PM, but it can help PMs develop those skills.
An increase in the quality of deliverables has broader strategic implications. Better insights and synthesis of customer needs, potential impact, and solution overview can lead to a virtuous cycle of higher-quality products and better adoption. To meet these goals, some organizations are turning to gen AI tools to accelerate their journey toward product-led growth.
Subscribe to the McKinsey on Building Products podcast
Implications for product leaders.
Technology leaders can use these findings to translate higher productivity, improved experience, and quality improvements into greater customer and product impact. As with most fundamental shifts in operating model, adopting gen AI in product management requires a thoughtful, phased approach. Leaders can keep five steps in mind as they embark on this journey to minimize the risks of using gen AI in product management.
Develop a road map linked to customer outcomes
Creating a road map for gen AI adoption is essential to build momentum and conviction within the organization. Leaders can start by establishing goals for the adoption. For software engineers, the goal of adopting gen AI tools is typically to meet productivity goals. For PMs, however, goals should be linked to customer outcomes, such as better customer satisfaction scores driven by product quality.
Once leaders have established targets for customer outcomes, they should carefully assess and prioritize key use cases in the product development process based on holistic customer impact and the maturity of tools. One common pitfall is picking use cases ad hoc based on the most readily available tool, rather than strategically selecting use cases that align with the organization’s goals and have the potential to deliver the most significant customer impact.
Once use cases are identified, product leaders can take a phased approach to gen AI adoption, starting with simpler use cases, such as document writing and idea generation. Introducing use cases to a small group of PMs before rolling them out to everyone allows for easy testing and refinement of the approach, which is critical to understand where PMs need coaching or oversight to mitigate risks and ensure that quality does not decline. In parallel, product leaders can set a baseline for organization-wide PM capability as well as PDLC maturity. Our research shows that output quality is highly contingent on PM experience. Baselining helps leaders understand which PM skills may need augmentation as they begin planning to adopt at scale. To get the most out of this adoption, leaders will need to rewire the PDLC to be aligned with the maturity baseline and inform the pace and approach for rollout.
Integrate tooling into PM flow
Another foundational aspect of effective gen AI adoption is establishing the right AI tool stack for PMs—which can be challenging given how quickly the landscape is evolving. Each new tool brings a unique mix of strengths and capabilities, and leaders must consider how new tools integrate into existing PM workflows and their potential in distinct use cases as well as across categories. Taking these steps smooths the adoption process by minimizing switching between tools and enables structured and unstructured data (for example, product instrumentation data, latest details on product ideas or epics, customer feedback, and market trends) to flow seamlessly across the PM tool stack to ensure a single source of truth.
Provide PMs with gen AI coaching
As with much of the workforce, most PMs today will require a combination of upskilling and coaching to use the technology effectively to augment their daily work. Organizations should not expect people to adopt new tools organically and derive benefit from them. However, gen AI tools are only as effective as the PMs using them. Every PM has a distinct set of core skills, resulting in a wide variance in individuals’ effectiveness and outcomes when adopting gen AI tools. To ensure PMs can benefit from the use of these tools, organizations should invest in capability building to upskill PMs.
Provide risk controls
Although it may be tempting to implement gen AI tools quickly across the PDLC, measures to mitigate risks associated with ethics, privacy, intellectual property (IP), legal considerations, and interpretability 2 Gen AI tools are considered “interpretable” when PMs can readily discern how they arrived at the output they created. are crucial. PMs can be trained to identify potential gen AI–related risks (for example, privacy and IP infringement) and concrete actions they can take to mitigate them (for example, review guidelines or consult with the legal function).
As leaders update governance, they should consider three potential risks: first, data privacy is always a factor when tools can access confidential information. Proper training, strict access controls, and robust privacy safeguards are crucial. Second, companies must ensure their gen AI usage complies with all applicable (and, again, rapidly evolving) legal and regulatory changes, including changes to the European Union’s General Data Protection Regulation (GDPR). And third, organizations must set clear guidelines on the ethical use of AI for PMs, including respect for IP and proper attribution of generated ideas.
Reimagine the product development life cycle
While augmenting PM tasks provides some benefits, the ability to harness the power of gen AI in product development comes from reimagining and rewiring the PDLC. Some fundamental shifts in the PDLC could arise, including compression of the PDLC driven by gen AI–enabled PM and engineering execution; blurred boundaries between the discovery and viability phases as gen AI empowers PMs to rapidly prototype and test new customer-backed product ideas; and a shift in PMs’ responsibilities as they become more proficient in building marketing and sales collateral to accelerate commercial launch and adoption. Leaders should be aware of how the PDLC will change and prepare PMs to adapt accordingly.
In a rapidly changing business landscape, the transformative potential of gen AI for product management cannot be overstated. By embracing this cutting-edge technology, PMs can gain a decisive edge as they discover untapped opportunities and streamline product development processes. But research shows that simply adopting gen AI is not enough to realize the technology’s full potential. Product leaders should formulate a structured, phased approach anchored in priority customer outcomes to take advantage of the technology’s potential to deliver extraordinary benefits. In addition, they should consider adopting the gen AI functionality in tools they may already have. The pace of innovation in this domain is high, and delays in figuring out adoption approaches will have long-term consequences for talent and ways of working.
Chandra Gnanasambandam and Martin Harrysson are senior partners in McKinsey’s Bay Area office, where Rikki Singh is a partner and Chaitra Yarlagadda is a consultant.
The authors wish to thank Alejo Navarro Goldaraz, An Yan, Andrey Suprun, Annie Fang, Anuj Kohli, Ashay Makim, Assata Quinichett, Camilla Zanon di Valgiurata, Dev Seth, Dmitry Gafarov, Doria Du, Gustavo Torres da Silva, Lucy Xia, and Ryan Skinner for their contributions to this article.
Explore a career with us
Related articles.
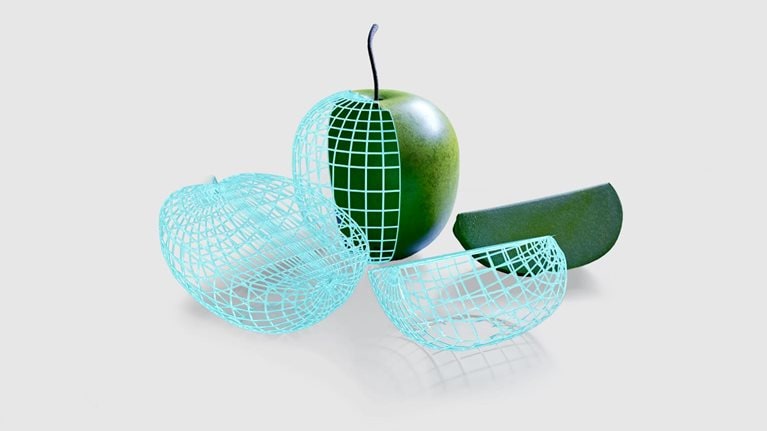
What is generative AI?

Technology’s generational moment with generative AI: A CIO and CTO guide

The economic potential of generative AI: The next productivity frontier

Press Release Details
Nvidia nim revolutionizes model deployment, now available to transform world’s millions of developers into generative ai developers.
- 150+ Partners Across Every Layer of AI Ecosystem Embedding NIM Inference Microservices to Speed Enterprise AI Application Deployments From Weeks to Minutes
- NVIDIA Developer Program Members Gain Free Access to NIM for Research, Development and Testing
TAIPEI, Taiwan, June 02, 2024 (GLOBE NEWSWIRE) -- COMPUTEX -- NVIDIA today announced that the world’s 28 million developers can now download NVIDIA NIM ™ — inference microservices that provide models as optimized containers — to deploy on clouds, data centers or workstations, giving them the ability to easily build generative AI applications for copilots, chatbots and more, in minutes rather than weeks.
These new generative AI applications are becoming increasingly complex and often utilize multiple models with different capabilities for generating text, images, video, speech and more. NVIDIA NIM dramatically increases developer productivity by providing a simple, standardized way to add generative AI to their applications.
NIM also enables enterprises to maximize their infrastructure investments. For example, running Meta Llama 3-8B in a NIM produces up to 3x more generative AI tokens on accelerated infrastructure than without NIM. This lets enterprises boost efficiency and use the same amount of compute infrastructure to generate more responses.
Nearly 200 technology partners — including Cadence, Cloudera , Cohesity , DataStax , NetApp , Scale AI and Synopsys — are integrating NIM into their platforms to speed generative AI deployments for domain-specific applications, such as copilots, code assistants and digital human avatars. Hugging Face is now offering NIM — starting with Meta Llama 3 .
“Every enterprise is looking to add generative AI to its operations, but not every enterprise has a dedicated team of AI researchers,” said Jensen Huang, founder and CEO of NVIDIA. “Integrated into platforms everywhere, accessible to developers everywhere, running everywhere — NVIDIA NIM is helping the technology industry put generative AI in reach for every organization.”
Enterprises can deploy AI applications in production with NIM through the NVIDIA AI Enterprise software platform. Starting next month, members of the NVIDIA Developer Program can access NIM for free for research, development and testing on their preferred infrastructure.
40+ NIM Microservices Power Gen AI Models Across Modalities NIM containers are pre-built to speed model deployment for GPU-accelerated inference and can include NVIDIA CUDA ® software, NVIDIA Triton Inference Server ™ and NVIDIA TensorRT™-LLM software.
Over 40 NVIDIA and community models are available to experience as NIM endpoints on ai.nvidia.com , including Databricks DBRX , Google’s open model Gemma, Meta Llama 3, Microsoft Phi-3, Mistral Large, Mixtral 8x22B and Snowflake Arctic.
Developers can now access NVIDIA NIM microservices for Meta Llama 3 models from the Hugging Face AI platform. This lets developers easily access and run the Llama 3 NIM in just a few clicks using Hugging Face Inference Endpoints, powered by NVIDIA GPUs on their preferred cloud.
Enterprises can use NIM to run applications for generating text, images and video, speech and digital humans. With NVIDIA BioNeMo ™ NIM microservices for digital biology, researchers can build novel protein structures to accelerate drug discovery.
Dozens of healthcare companies are deploying NIM to power generative AI inference across a range of applications, including surgical planning, digital assistants, drug discovery and clinical trial optimization.
With new NVIDIA ACE NIM microservices , developers can easily build and operate interactive, lifelike digital humans in applications for customer service, telehealth, education, gaming and entertainment.
Hundreds of AI Ecosyst em Partners Embedding NIM Platform providers including Canonical , Red Hat , Nutanix and VMware (acquired by Broadcom) are supporting NIM on open-source KServe or enterprise solutions. AI application companies Hippocratic AI , Glean , Kinetica and Redis are also deploying NIM to power generative AI inference.
Leading AI tools and MLOps partners — including Amazon SageMaker, Microsoft Azure AI, Dataiku, DataRobot, deepset , Domino Data Lab, LangChain , Llama Index , Replicate , Run.ai, Saturn Cloud , Securiti AI and Weights & Biases — have also embedded NIM into their platforms to enable developers to build and deploy domain-specific generative AI applications with optimized inference.
Global system integrators and service delivery partners Accenture, Deloitte, Infosys, Latentview , Quantiphi , SoftServe, TCS and Wipro have created NIM competencies to help the world’s enterprises quickly develop and deploy production AI strategies.
Enterprises can run NIM-enabled applications virtually anywhere, including on NVIDIA-Certified Systems ™ from global infrastructure manufacturers Cisco, Dell Technologies , Hewlett-Packard Enterprise , Lenovo and Supermicro, as well as server manufacturers ASRock Rack , ASUS , GIGABYTE , Ingrasys , Inventec , Pegatron , QCT, Wistron and Wiwynn. NIM microservices have also been integrated into Amazon Web Services , Google Cloud , Azure and Oracle Cloud Infrastructure .
Titans of Industry Amp Up Gener ative AI With NIM Industry leaders Foxconn, Pegatron, Amdocs , Lowe’s, ServiceNow and Siemens are among the businesses using NIM for generative AI applications in manufacturing, healthcare, financial services, retail, customer service and more:
- Foxconn — the world’s largest electronics manufacturer — is using NIM in the development of domain-specific LLMs embedded into a variety of internal systems and processes in its AI factories for smart manufacturing, smart cities and smart electric vehicles.
- Pegatron — a Taiwanese electronics manufacturing company — is leveraging NIM for Project TaME, a Taiwan Mixtral of Experts model designed to advance the development of local LLMs for industries.
- Amdocs — a leading global provider of software and services to communications and media companies — is using NIM to run a customer billing LLM that significantly lowers the cost of tokens, improves accuracy by up to 30% and reduces latency by 80%, driving near real-time responses.
- Lowe’s — a FORTUNE ® 50 home improvement company — is using generative AI for a variety of use cases. For example, the retailer is leveraging NVIDIA NIM inference microservices to elevate experiences for associates and customers.
- ServiceNow — the AI platform for business transformation — announced earlier this year that it was one of the first platform providers to access NIM to enable fast, scalable and more cost-effective LLM development and deployment for its customers. NIM microservices are integrated within the Now AI multimodal model and are available to customers that have ServiceNow’s generative AI experience, Now Assist, installed.
- Siemens — a global technology company focused on industry, infrastructure, transport and healthcare — is integrating its operational technology with NIM microservices for shop floor AI workloads. It is also building an on-premises version of its Industrial Copilot for Machine Operators using NIM.
Availability Developers can experiment with NVIDIA microservices at ai.nvidia.com at no charge. Enterprises can deploy production-grade NIM microservices with NVIDIA AI Enterprise running on NVIDIA-Certified Systems and leading cloud platforms. Starting next month, members of the NVIDIA Developer Program will gain free access to NIM for research and testing.
Watch Huang’s COMPUTEX keynote to learn more about NVIDIA NIM.
About NVIDIA NVIDIA (NASDAQ: NVDA) is the world leader in accelerated computing.
For further information, contact: Anna Kiachian NVIDIA Corporation +1-650-224-9820 [email protected]
Certain statements in this press release including, but not limited to, statements as to: the benefits, impact, performance, features, and availability of NVIDIA’s products and technologies, including NVIDIA NIM, NVIDIA CUDA, NVIDIA Triton Inference Server, NVIDIA TensorRT-LLM software, NVIDIA Developer program, NVIDIA BioNeMo, NVIDIA-Certified Systems, and NVIDIA AI Enterprise; our collaborations and partnerships with third parties and the benefits and impacts thereof; third parties using or adopting our products or technologies; every enterprise looking to add generative AI to its operations; and NVIDIA NIM helping the technology industry put generative AI in reach for every organization are forward-looking statements that are subject to risks and uncertainties that could cause results to be materially different than expectations. Important factors that could cause actual results to differ materially include: global economic conditions; our reliance on third parties to manufacture, assemble, package and test our products; the impact of technological development and competition; development of new products and technologies or enhancements to our existing product and technologies; market acceptance of our products or our partners' products; design, manufacturing or software defects; changes in consumer preferences or demands; changes in industry standards and interfaces; unexpected loss of performance of our products or technologies when integrated into systems; as well as other factors detailed from time to time in the most recent reports NVIDIA files with the Securities and Exchange Commission, or SEC, including, but not limited to, its annual report on Form 10-K and quarterly reports on Form 10-Q. Copies of reports filed with the SEC are posted on the company's website and are available from NVIDIA without charge. These forward-looking statements are not guarantees of future performance and speak only as of the date hereof, and, except as required by law, NVIDIA disclaims any obligation to update these forward-looking statements to reflect future events or circumstances.
© 2024 NVIDIA Corporation. All rights reserved. NVIDIA, the NVIDIA logo, BioNeMo, CUDA, NVIDIA NIM, NVIDIA Triton Inference Server and TensorRT are trademarks and/or registered trademarks of NVIDIA Corporation in the U.S. and other countries. Other company and product names may be trademarks of the respective companies with which they are associated. Features, pricing, availability and specifications are subject to change without notice.
A photo accompanying this announcement is available at: https://www.globenewswire.com/NewsRoom/AttachmentNg/4fe99b19-66e7-4473-b9ff-f1380eae0ff8
NVIDIA NIM revolutionizes model deployment for every layer of the AI ecosystem.
Quick links.
- Email Alerts
- Request Printed Materials
- Download Library
To receive notifications via email, enter your email address and select at least one subscription below. After submitting your information, you will receive an email. You must click the link in the email to activate your subscription. You can sign up for additional subscriptions at any time.
Email Alert Sign Up Confirmation
Investor contact.
2788 San Tomas Expressway Santa Clara, CA 95051
- Contact Investor Relations
Investor Resources
- Request Information
- Stock Quote & Chart
- Historical Price Lookup
- Investment Calculator
- Fundamentals
- Analyst Coverage
- Management Team
- Board of Directors
- Governance Documents
- Committee Composition
- Contact the Board
- Corporate Sustainability
- Events & Presentations
Financial Info
- Financial Reports
- SEC Filings
- Quarterly Results
- Annual Reports and Proxies
Investors and others should note that we announce material financial information to our investors using our investor relations website, press releases, SEC filings and public conference calls and webcasts. We intend to use our @NVIDIA Twitter account, NVIDIA Facebook page, NVIDIA LinkedIn page and company blog as a means of disclosing information about our company, our services and other matters and for complying with our disclosure obligations under Regulation FD. The information we post through these social media channels may be deemed material. Accordingly, investors should monitor these accounts and the blog, in addition to following our press releases, SEC filings and public conference calls and webcasts. This list may be updated from time to time.

- Privacy Policy
- Manage My Privacy
- Do Not Sell or Share My Data
- Terms of Service
- Accessibility
- Corporate Policies
Gen Z really are the hardest to work with—even managers of their own generation say they’re difficult. Instead bosses plan to hire more of their millennial counterparts

It’s no secret that Gen Z often gets flak for, in the words of the Sister Act star Whoopi Goldberg, not “ busting their behinds ” at work quite like previous generations did.
Earlier this year, the Oscar-winning actress Jodie Foster complained that Gen Zers don’t show up to work until 10:30 a.m., and an MIT interviewer blasted the generation for always “being late.” Just last month, one CEO vented his gripe with Gen Z when a young job candidate refused to do a 90-minute task because it “ looked like a lot of work .”
But it’s not just Gen Xers and baby boomers who have taken stock of how different (or rather, difficult) the youngest generation of workers are. Now even Gen Z hiring managers are complaining about their own generation’s work style.
Resume Genius asked 625 U.S. hiring managers which generation is the most challenging to work with, and 45% pointed to Gen Z. What’s more, 50% of Gen Z hiring managers admitted that their own generation is the most difficult to manage.
Perhaps surpringly, baby boomers—who have been caught in their fair share of criticism too for not being in touch with today’s workers after buying McMansions in the suburbs on one wage and then refusing to retire —were voted as the easiest to manage.
However, being easy to work with isn’t making the oldest generation of workers any more hirable: In fact, baby boomers are the last people employers expect to hire right now.
While just 4% of the hiring managers surveyed expect to hire baby boomers in the year ahead, a third admitted they will probably end up hiring Gen Zers.
Despite being the most difficult generation to work with, Gen Z are the second most likely-to-be-hired job candidate of choice. The most popular? Their slightly more seasoned millennial peers (45%).
In comparison, only 14% of the hiring managers surveyed expect to hire Gen X workers in the year ahead.
Workers get easier to manage with age
The survey didn’t delve into why Gen Z workers are more challenging to work with than their peers, but it’s hard to ignore the fact that latest cohort of workers came of age during the pandemic.
Not only did Gen Z miss out on major milestone college experiences, from frat parties to literally tossing their graduation caps in the air, but they also didn’t get to taste the world of work through summer internships before diving into the job market.
It’s why employers are now giving graduates extra training to get them up to speed. Take the world’s Big Four consulting firms: Deloitte , PwC, KPMG , and EY are all offering incoming junior hires soft-skills training , including lessons on how to speak up in meetings.
“It’s wholly understandable that students who missed out on face-to-face activities during COVID may now be stronger in certain fields, such as working independently, and less confident in others, such as presentations to groups,” Ian Elliott, the chief people officer at PwC UK, said in sympathy with young workers.
Plus, COVID-19 isn’t entirely to blame for management’s struggle with Gen Z: As Resume Genius’s survey suggests, workers get easier to manage with age.
While 45% of those surveyed described Gen Z as the most challenging to work with, this dropped to 26% for millennials, 13% for Gen X, and 9% for baby boomers.
In the end, being “difficult” is probably just another rite of passage. After all, millennials will remember being labeled work-shy snowflakes before climbing the corporate ranks into management and putting the heat on Gen Z.
But instead of trying to wrestle with making the youngest generation of workers easier to manage, the research’s author suggests businesses bend to Gen Z.
“Gen Z has already shaken things up, but they’re not here to break things: They bring a unique blend of talent and bold ideas that can rejuvenate any workforce,” wrote Geoffrey Scott, senior hiring manager at Resume Genius.
For example, according to the research, Gen Z managers are most likely to make hiring decisions based on what candidates put down as their “hobbies and interests” on their CV, over professional experience.
“Gen Zers might have a bad rep, but they have the power to transform workplaces for the better,” Scott concludes.
Latest in Success

Reclaiming my birth name as a Black woman made me a stronger executive leader—after 20-plus years as ‘Shauna’

I survived a boardroom panic attack with a paper clip (and a tale to sell)

Meet the 5 types of Gen Z freelancers—and their secret weapon

Jodie Foster finds Gen Z ‘really annoying’ to work with—but she wishes she had their ability to say no earlier in her career

Gen X is set to be a hoagie, not sandwich generation—chipping in longer for their aging parents and children

Microsoft’s chief scientist: Step aside, prompt engineers—AI will start prompting you instead
Most popular.

He taught at MIT, worked at Morgan Stanley, and convinced Bill Ackman and Galaxy to back his $200 million crypto fund by his early 30s. His future is now in jeopardy

Whole Foods founder said Jeff Bezos is a ‘genius’ who got rid of the ‘whole paycheck’ stigma

The ‘funflation’ economy is dying as a consumer attitude of ‘hard pass’ takes over and major artists cancel concert tours

Traders who scooped up Warren Buffett’s Berkshire Hathaway shares at a massive $620,000 discount during glitch will have their deals canceled by the NYSE

Millennials’ midlife crisis looks different from their parents’ sports cars and mistresses—it’s a ‘crisis of purpose and engagement’

The Fed was too focused on the soft landing and has stuck the U.S. economy with higher prices, Stifel chief economist says
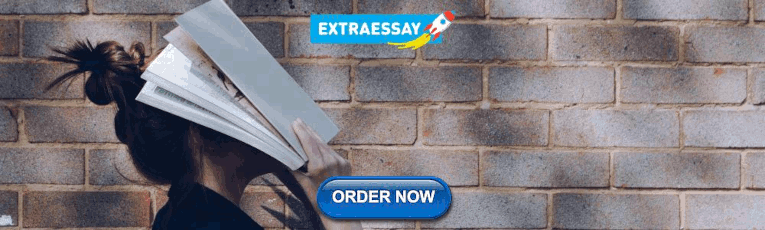
IMAGES
VIDEO
COMMENTS
For the study above, the hypothesis being tested would be "Captopril decreases rates of cardiovascular events in patients with essential hypertension, relative to patients receiving no treatment". Studies that seek to answer descriptive research questions do not test hypotheses, but they can be used for hypothesis generation.
Hypothesis generation is an early and critical step in any hypothesis-driven clinical research project. Because it is not yet a well-understood cognitive process, the need to improve the process goes unrecognized. Without an impactful hypothesis, the significance of any research project can be questionable, regardless of the rigor or diligence applied in other steps of the study, e.g., study ...
Hypothesis-generating research typically is conducted when existing theory or knowledge is insufficient to explain particular phenomena. Popular "tools" for hypothesis generation in preclinical research include gene expression microarray studies; hypotheses for clinical or epidemiological research may be generated secondary to a project's ...
Generate a hypothesis in advance through pre-analyzing a problem (i.e., generation of a prestage hypothesis ). 3. Collect data related to the prestage hypothesis by appropriate means such as experiment, observation, database search, and Web search (i.e., data collection). 4. Process and transform the collected data as needed. 5.
The hypothesis-generating mode of research has been primarily practiced in basic science but has recently been extended to clinical-translational work as well. Just as in basic science, this approach to research can facilitate insights into human health and disease mechanisms and provide the crucially needed data set of the full spectrum of ...
6. Write a null hypothesis. If your research involves statistical hypothesis testing, you will also have to write a null hypothesis. The null hypothesis is the default position that there is no association between the variables. The null hypothesis is written as H 0, while the alternative hypothesis is H 1 or H a.
Hypothesis Generation is a literature-based discovery approach that utilizes existing literature to automatically generate implicit biomedical associations and provide reasonable predictions for future research. Despite its potential, current hypothesis generation methods face challenges when applied to research on biological mechanisms.
Simple hypothesis. A simple hypothesis is a statement made to reflect the relation between exactly two variables. One independent and one dependent. Consider the example, "Smoking is a prominent cause of lung cancer." The dependent variable, lung cancer, is dependent on the independent variable, smoking. 4.
Keywords: Clinical research; Observational study; Scientific hypothesis generation; Secondary data analytic tool; Think-aloud . Introduction A hypothesis is an educated guess or statement about the relationship between 2 or more variables 2,3. Scientific hypothesis generation is a critical step in scientific research that
A research hypothesis is a statement that proposes a possible explanation for an observable phenomenon or pattern. It guides the direction of a study and predicts the outcome of the investigation. A research hypothesis is testable, i.e., it can be supported or disproven through experimentation or observation. Characteristics of a good hypothesis
One way is to generate a research question using the techniques discussed in this chapter ... The second way is to describe one or more existing theories, derive a hypothesis from one of those theories, test the hypothesis in a new study, and finally reevaluate the theory. This format works well when there is an existing theory that addresses ...
a hypothesis-generating study is just a hypothesis. A hypothe-sis cannot be generated and confirmed in the same study, and it cannot be confirmed with a new hypothesis-generating study. Confirmatory studies are essential for scientific progress. Jonas Ranstam, Statistical Editor
Step 5: Present your findings. The results of hypothesis testing will be presented in the results and discussion sections of your research paper, dissertation or thesis.. In the results section you should give a brief summary of the data and a summary of the results of your statistical test (for example, the estimated difference between group means and associated p-value).
Hypothesis generation is a process beginning with an educated guess whereas hypothesis testing is a process to conclude that the educated guess is true/false or the relationship between the variables is statistically significant or not. This latter part could be used for further research using statistical proof.
Hypothesis generation: qualitative research to identify potentials of behavioral change regarding energy consumption. The qualitative expressions are based on data that includes semi-structured interviews, observations and social experiments. The energy-saving benefits are considered without a reduction in performance.
Is hypothesis-generating qualitative or quantitative? In qualitative research, a hypothesis is used in the form of a clear statement concerning the problem to be investigated. Unlike in quantitative research, where hypotheses are only developed to be tested, qualitative research can lead to hypothesis-testing and hypothesis-generating outcomes.
The Cardiorenal and Metabolic Disease Research Center (CMDRC) at UMMC offers a Pilot Projects Program (PPP) through the Centers of Biomedical Research Excellence (COBRE). These pilot grants are intended to assist investigators in generating sufficient data to be competitive for extramural funding. A focus on obesity, cardiorenal or metabolic diseases is required.
In the context of this study, the TPB supports the hypothesis that behavioral intention, influenced by various enabling and inhibiting factors, can significantly predict the actual use of ChatGPT among university students (H11). ... Although related, the constructs are distinct: knowledge acquisition involves generating new knowledge, while ...
Dr. Chan is a molecular biologist at the Broad Institute of M.I.T. and Harvard, and a co-author of "Viral: The Search for the Origin of Covid-19." This article has been updated to reflect news ...
Diffusion models have achieved remarkable success in text-to-image generation tasks; however, the role of initial noise has been rarely explored. In this study, we identify specific regions within the initial noise image, termed trigger patches, that play a key role for object generation in the resulting images. Notably, these patches are ``universal'' and can be generalized across various ...
The research, co-authored by researchers from Google, Duke University and several fact-checking and media organizations, was published in a preprint last week. The paper introduces a massive new ...
The latest generation of AI-enabled project management tools can improve product managers' productivity, quality, and experience. ... Our hypothesis is that senior PMs, given their familiarity with the deliverables and their stronger product sense, can better review the gen AI tools' output and ensure high accuracy and writing fluency ...
150+ Partners Across Every Layer of AI Ecosystem Embedding NIM Inference Microservices to Speed Enterprise AI Application Deployments From Weeks to Minutes NVIDIA Developer Program Members Gain Free Access to NIM for Research, Development and Testing TAIPEI, Taiwan, June 02, 2024 (GLOBE NEWSWIRE) - COMPUTEX - NVIDIA today announced that the world's 28 million developers can now download ...
Resume Genius asked 625 U.S. hiring managers which generation is the most challenging to work with, and 45% pointed to Gen Z. What's more, 50% of Gen Z hiring managers admitted that their own ...