- Search Menu
- Browse content in A - General Economics and Teaching
- Browse content in A1 - General Economics
- A11 - Role of Economics; Role of Economists; Market for Economists
- A13 - Relation of Economics to Social Values
- A14 - Sociology of Economics
- Browse content in C - Mathematical and Quantitative Methods
- Browse content in C0 - General
- C02 - Mathematical Methods
- Browse content in C1 - Econometric and Statistical Methods and Methodology: General
- C10 - General
- C11 - Bayesian Analysis: General
- C12 - Hypothesis Testing: General
- C13 - Estimation: General
- C14 - Semiparametric and Nonparametric Methods: General
- C15 - Statistical Simulation Methods: General
- C18 - Methodological Issues: General
- Browse content in C2 - Single Equation Models; Single Variables
- C21 - Cross-Sectional Models; Spatial Models; Treatment Effect Models; Quantile Regressions
- C22 - Time-Series Models; Dynamic Quantile Regressions; Dynamic Treatment Effect Models; Diffusion Processes
- C23 - Panel Data Models; Spatio-temporal Models
- Browse content in C3 - Multiple or Simultaneous Equation Models; Multiple Variables
- C32 - Time-Series Models; Dynamic Quantile Regressions; Dynamic Treatment Effect Models; Diffusion Processes; State Space Models
- C38 - Classification Methods; Cluster Analysis; Principal Components; Factor Models
- Browse content in C4 - Econometric and Statistical Methods: Special Topics
- C45 - Neural Networks and Related Topics
- Browse content in C5 - Econometric Modeling
- C50 - General
- C51 - Model Construction and Estimation
- C52 - Model Evaluation, Validation, and Selection
- C53 - Forecasting and Prediction Methods; Simulation Methods
- C55 - Large Data Sets: Modeling and Analysis
- C58 - Financial Econometrics
- Browse content in C6 - Mathematical Methods; Programming Models; Mathematical and Simulation Modeling
- C61 - Optimization Techniques; Programming Models; Dynamic Analysis
- C62 - Existence and Stability Conditions of Equilibrium
- C65 - Miscellaneous Mathematical Tools
- Browse content in C7 - Game Theory and Bargaining Theory
- C70 - General
- C72 - Noncooperative Games
- C73 - Stochastic and Dynamic Games; Evolutionary Games; Repeated Games
- C78 - Bargaining Theory; Matching Theory
- Browse content in C8 - Data Collection and Data Estimation Methodology; Computer Programs
- C81 - Methodology for Collecting, Estimating, and Organizing Microeconomic Data; Data Access
- Browse content in C9 - Design of Experiments
- C91 - Laboratory, Individual Behavior
- C92 - Laboratory, Group Behavior
- C93 - Field Experiments
- Browse content in D - Microeconomics
- Browse content in D0 - General
- D03 - Behavioral Microeconomics: Underlying Principles
- Browse content in D1 - Household Behavior and Family Economics
- D10 - General
- D11 - Consumer Economics: Theory
- D12 - Consumer Economics: Empirical Analysis
- D14 - Household Saving; Personal Finance
- D15 - Intertemporal Household Choice: Life Cycle Models and Saving
- D18 - Consumer Protection
- Browse content in D2 - Production and Organizations
- D20 - General
- D21 - Firm Behavior: Theory
- D22 - Firm Behavior: Empirical Analysis
- D23 - Organizational Behavior; Transaction Costs; Property Rights
- D24 - Production; Cost; Capital; Capital, Total Factor, and Multifactor Productivity; Capacity
- D25 - Intertemporal Firm Choice: Investment, Capacity, and Financing
- Browse content in D3 - Distribution
- D30 - General
- D31 - Personal Income, Wealth, and Their Distributions
- Browse content in D4 - Market Structure, Pricing, and Design
- D40 - General
- D43 - Oligopoly and Other Forms of Market Imperfection
- D44 - Auctions
- D47 - Market Design
- D49 - Other
- Browse content in D5 - General Equilibrium and Disequilibrium
- D50 - General
- D51 - Exchange and Production Economies
- D52 - Incomplete Markets
- D53 - Financial Markets
- Browse content in D6 - Welfare Economics
- D60 - General
- D61 - Allocative Efficiency; Cost-Benefit Analysis
- D62 - Externalities
- Browse content in D7 - Analysis of Collective Decision-Making
- D70 - General
- D71 - Social Choice; Clubs; Committees; Associations
- D72 - Political Processes: Rent-seeking, Lobbying, Elections, Legislatures, and Voting Behavior
- D73 - Bureaucracy; Administrative Processes in Public Organizations; Corruption
- D74 - Conflict; Conflict Resolution; Alliances; Revolutions
- D78 - Positive Analysis of Policy Formulation and Implementation
- Browse content in D8 - Information, Knowledge, and Uncertainty
- D80 - General
- D81 - Criteria for Decision-Making under Risk and Uncertainty
- D82 - Asymmetric and Private Information; Mechanism Design
- D83 - Search; Learning; Information and Knowledge; Communication; Belief; Unawareness
- D84 - Expectations; Speculations
- D85 - Network Formation and Analysis: Theory
- D86 - Economics of Contract: Theory
- D87 - Neuroeconomics
- Browse content in D9 - Micro-Based Behavioral Economics
- D90 - General
- D91 - Role and Effects of Psychological, Emotional, Social, and Cognitive Factors on Decision Making
- D92 - Intertemporal Firm Choice, Investment, Capacity, and Financing
- Browse content in E - Macroeconomics and Monetary Economics
- Browse content in E0 - General
- E00 - General
- E03 - Behavioral Macroeconomics
- Browse content in E1 - General Aggregative Models
- E17 - Forecasting and Simulation: Models and Applications
- Browse content in E2 - Consumption, Saving, Production, Investment, Labor Markets, and Informal Economy
- E20 - General
- E21 - Consumption; Saving; Wealth
- E22 - Investment; Capital; Intangible Capital; Capacity
- E23 - Production
- E24 - Employment; Unemployment; Wages; Intergenerational Income Distribution; Aggregate Human Capital; Aggregate Labor Productivity
- Browse content in E3 - Prices, Business Fluctuations, and Cycles
- E30 - General
- E31 - Price Level; Inflation; Deflation
- E32 - Business Fluctuations; Cycles
- E37 - Forecasting and Simulation: Models and Applications
- Browse content in E4 - Money and Interest Rates
- E40 - General
- E41 - Demand for Money
- E42 - Monetary Systems; Standards; Regimes; Government and the Monetary System; Payment Systems
- E43 - Interest Rates: Determination, Term Structure, and Effects
- E44 - Financial Markets and the Macroeconomy
- E47 - Forecasting and Simulation: Models and Applications
- Browse content in E5 - Monetary Policy, Central Banking, and the Supply of Money and Credit
- E50 - General
- E51 - Money Supply; Credit; Money Multipliers
- E52 - Monetary Policy
- E58 - Central Banks and Their Policies
- Browse content in E6 - Macroeconomic Policy, Macroeconomic Aspects of Public Finance, and General Outlook
- E60 - General
- E61 - Policy Objectives; Policy Designs and Consistency; Policy Coordination
- E62 - Fiscal Policy
- E63 - Comparative or Joint Analysis of Fiscal and Monetary Policy; Stabilization; Treasury Policy
- E64 - Incomes Policy; Price Policy
- E65 - Studies of Particular Policy Episodes
- E66 - General Outlook and Conditions
- Browse content in E7 - Macro-Based Behavioral Economics
- E71 - Role and Effects of Psychological, Emotional, Social, and Cognitive Factors on the Macro Economy
- Browse content in F - International Economics
- Browse content in F0 - General
- F02 - International Economic Order and Integration
- Browse content in F1 - Trade
- F14 - Empirical Studies of Trade
- Browse content in F2 - International Factor Movements and International Business
- F21 - International Investment; Long-Term Capital Movements
- F22 - International Migration
- F23 - Multinational Firms; International Business
- Browse content in F3 - International Finance
- F30 - General
- F31 - Foreign Exchange
- F32 - Current Account Adjustment; Short-Term Capital Movements
- F33 - International Monetary Arrangements and Institutions
- F34 - International Lending and Debt Problems
- F36 - Financial Aspects of Economic Integration
- F37 - International Finance Forecasting and Simulation: Models and Applications
- F38 - International Financial Policy: Financial Transactions Tax; Capital Controls
- Browse content in F4 - Macroeconomic Aspects of International Trade and Finance
- F40 - General
- F41 - Open Economy Macroeconomics
- F42 - International Policy Coordination and Transmission
- F43 - Economic Growth of Open Economies
- F44 - International Business Cycles
- F47 - Forecasting and Simulation: Models and Applications
- Browse content in F5 - International Relations, National Security, and International Political Economy
- F51 - International Conflicts; Negotiations; Sanctions
- Browse content in F6 - Economic Impacts of Globalization
- F63 - Economic Development
- F65 - Finance
- Browse content in G - Financial Economics
- Browse content in G0 - General
- G00 - General
- G01 - Financial Crises
- G02 - Behavioral Finance: Underlying Principles
- Browse content in G1 - General Financial Markets
- G10 - General
- G11 - Portfolio Choice; Investment Decisions
- G12 - Asset Pricing; Trading volume; Bond Interest Rates
- G13 - Contingent Pricing; Futures Pricing
- G14 - Information and Market Efficiency; Event Studies; Insider Trading
- G15 - International Financial Markets
- G17 - Financial Forecasting and Simulation
- G18 - Government Policy and Regulation
- G19 - Other
- Browse content in G2 - Financial Institutions and Services
- G20 - General
- G21 - Banks; Depository Institutions; Micro Finance Institutions; Mortgages
- G22 - Insurance; Insurance Companies; Actuarial Studies
- G23 - Non-bank Financial Institutions; Financial Instruments; Institutional Investors
- G24 - Investment Banking; Venture Capital; Brokerage; Ratings and Ratings Agencies
- G28 - Government Policy and Regulation
- G29 - Other
- Browse content in G3 - Corporate Finance and Governance
- G30 - General
- G31 - Capital Budgeting; Fixed Investment and Inventory Studies; Capacity
- G32 - Financing Policy; Financial Risk and Risk Management; Capital and Ownership Structure; Value of Firms; Goodwill
- G33 - Bankruptcy; Liquidation
- G34 - Mergers; Acquisitions; Restructuring; Corporate Governance
- G35 - Payout Policy
- G38 - Government Policy and Regulation
- G39 - Other
- Browse content in G4 - Behavioral Finance
- G40 - General
- G41 - Role and Effects of Psychological, Emotional, Social, and Cognitive Factors on Decision Making in Financial Markets
- Browse content in G5 - Household Finance
- G50 - General
- G51 - Household Saving, Borrowing, Debt, and Wealth
- G52 - Insurance
- G53 - Financial Literacy
- Browse content in H - Public Economics
- H0 - General
- Browse content in H1 - Structure and Scope of Government
- H11 - Structure, Scope, and Performance of Government
- H19 - Other
- Browse content in H2 - Taxation, Subsidies, and Revenue
- H22 - Incidence
- H24 - Personal Income and Other Nonbusiness Taxes and Subsidies; includes inheritance and gift taxes
- H25 - Business Taxes and Subsidies
- H26 - Tax Evasion and Avoidance
- Browse content in H3 - Fiscal Policies and Behavior of Economic Agents
- H31 - Household
- Browse content in H4 - Publicly Provided Goods
- H40 - General
- H41 - Public Goods
- Browse content in H5 - National Government Expenditures and Related Policies
- H50 - General
- H52 - Government Expenditures and Education
- H53 - Government Expenditures and Welfare Programs
- H54 - Infrastructures; Other Public Investment and Capital Stock
- H55 - Social Security and Public Pensions
- H56 - National Security and War
- H57 - Procurement
- Browse content in H6 - National Budget, Deficit, and Debt
- H63 - Debt; Debt Management; Sovereign Debt
- Browse content in H7 - State and Local Government; Intergovernmental Relations
- H70 - General
- H72 - State and Local Budget and Expenditures
- H74 - State and Local Borrowing
- H75 - State and Local Government: Health; Education; Welfare; Public Pensions
- Browse content in H8 - Miscellaneous Issues
- H81 - Governmental Loans; Loan Guarantees; Credits; Grants; Bailouts
- Browse content in I - Health, Education, and Welfare
- Browse content in I1 - Health
- I11 - Analysis of Health Care Markets
- I12 - Health Behavior
- I13 - Health Insurance, Public and Private
- I14 - Health and Inequality
- I18 - Government Policy; Regulation; Public Health
- Browse content in I2 - Education and Research Institutions
- I22 - Educational Finance; Financial Aid
- I23 - Higher Education; Research Institutions
- I28 - Government Policy
- Browse content in I3 - Welfare, Well-Being, and Poverty
- I30 - General
- I38 - Government Policy; Provision and Effects of Welfare Programs
- Browse content in J - Labor and Demographic Economics
- Browse content in J0 - General
- J00 - General
- Browse content in J1 - Demographic Economics
- J11 - Demographic Trends, Macroeconomic Effects, and Forecasts
- J12 - Marriage; Marital Dissolution; Family Structure; Domestic Abuse
- J13 - Fertility; Family Planning; Child Care; Children; Youth
- J15 - Economics of Minorities, Races, Indigenous Peoples, and Immigrants; Non-labor Discrimination
- J16 - Economics of Gender; Non-labor Discrimination
- J18 - Public Policy
- Browse content in J2 - Demand and Supply of Labor
- J20 - General
- J21 - Labor Force and Employment, Size, and Structure
- J22 - Time Allocation and Labor Supply
- J23 - Labor Demand
- J24 - Human Capital; Skills; Occupational Choice; Labor Productivity
- J26 - Retirement; Retirement Policies
- J28 - Safety; Job Satisfaction; Related Public Policy
- Browse content in J3 - Wages, Compensation, and Labor Costs
- J30 - General
- J31 - Wage Level and Structure; Wage Differentials
- J32 - Nonwage Labor Costs and Benefits; Retirement Plans; Private Pensions
- J33 - Compensation Packages; Payment Methods
- J38 - Public Policy
- Browse content in J4 - Particular Labor Markets
- J41 - Labor Contracts
- J44 - Professional Labor Markets; Occupational Licensing
- J45 - Public Sector Labor Markets
- J46 - Informal Labor Markets
- J49 - Other
- Browse content in J5 - Labor-Management Relations, Trade Unions, and Collective Bargaining
- J51 - Trade Unions: Objectives, Structure, and Effects
- J52 - Dispute Resolution: Strikes, Arbitration, and Mediation; Collective Bargaining
- Browse content in J6 - Mobility, Unemployment, Vacancies, and Immigrant Workers
- J61 - Geographic Labor Mobility; Immigrant Workers
- J62 - Job, Occupational, and Intergenerational Mobility
- J63 - Turnover; Vacancies; Layoffs
- J64 - Unemployment: Models, Duration, Incidence, and Job Search
- J65 - Unemployment Insurance; Severance Pay; Plant Closings
- J68 - Public Policy
- Browse content in J7 - Labor Discrimination
- J71 - Discrimination
- Browse content in J8 - Labor Standards: National and International
- J88 - Public Policy
- Browse content in K - Law and Economics
- Browse content in K1 - Basic Areas of Law
- K12 - Contract Law
- Browse content in K2 - Regulation and Business Law
- K22 - Business and Securities Law
- K23 - Regulated Industries and Administrative Law
- Browse content in K3 - Other Substantive Areas of Law
- K31 - Labor Law
- K32 - Environmental, Health, and Safety Law
- K34 - Tax Law
- K35 - Personal Bankruptcy Law
- Browse content in K4 - Legal Procedure, the Legal System, and Illegal Behavior
- K42 - Illegal Behavior and the Enforcement of Law
- Browse content in L - Industrial Organization
- Browse content in L1 - Market Structure, Firm Strategy, and Market Performance
- L10 - General
- L11 - Production, Pricing, and Market Structure; Size Distribution of Firms
- L13 - Oligopoly and Other Imperfect Markets
- L14 - Transactional Relationships; Contracts and Reputation; Networks
- L15 - Information and Product Quality; Standardization and Compatibility
- Browse content in L2 - Firm Objectives, Organization, and Behavior
- L21 - Business Objectives of the Firm
- L22 - Firm Organization and Market Structure
- L23 - Organization of Production
- L24 - Contracting Out; Joint Ventures; Technology Licensing
- L25 - Firm Performance: Size, Diversification, and Scope
- L26 - Entrepreneurship
- L29 - Other
- Browse content in L3 - Nonprofit Organizations and Public Enterprise
- L33 - Comparison of Public and Private Enterprises and Nonprofit Institutions; Privatization; Contracting Out
- Browse content in L4 - Antitrust Issues and Policies
- L43 - Legal Monopolies and Regulation or Deregulation
- L44 - Antitrust Policy and Public Enterprises, Nonprofit Institutions, and Professional Organizations
- Browse content in L5 - Regulation and Industrial Policy
- L51 - Economics of Regulation
- Browse content in L6 - Industry Studies: Manufacturing
- L66 - Food; Beverages; Cosmetics; Tobacco; Wine and Spirits
- Browse content in L8 - Industry Studies: Services
- L81 - Retail and Wholesale Trade; e-Commerce
- L85 - Real Estate Services
- L86 - Information and Internet Services; Computer Software
- Browse content in L9 - Industry Studies: Transportation and Utilities
- L92 - Railroads and Other Surface Transportation
- L94 - Electric Utilities
- Browse content in M - Business Administration and Business Economics; Marketing; Accounting; Personnel Economics
- Browse content in M0 - General
- M00 - General
- Browse content in M1 - Business Administration
- M12 - Personnel Management; Executives; Executive Compensation
- M13 - New Firms; Startups
- M14 - Corporate Culture; Social Responsibility
- M16 - International Business Administration
- Browse content in M2 - Business Economics
- M20 - General
- M21 - Business Economics
- Browse content in M3 - Marketing and Advertising
- M30 - General
- M31 - Marketing
- M37 - Advertising
- Browse content in M4 - Accounting and Auditing
- M40 - General
- M41 - Accounting
- M42 - Auditing
- M48 - Government Policy and Regulation
- Browse content in M5 - Personnel Economics
- M51 - Firm Employment Decisions; Promotions
- M52 - Compensation and Compensation Methods and Their Effects
- M54 - Labor Management
- Browse content in N - Economic History
- Browse content in N1 - Macroeconomics and Monetary Economics; Industrial Structure; Growth; Fluctuations
- N10 - General, International, or Comparative
- N12 - U.S.; Canada: 1913-
- Browse content in N2 - Financial Markets and Institutions
- N20 - General, International, or Comparative
- N21 - U.S.; Canada: Pre-1913
- N22 - U.S.; Canada: 1913-
- N23 - Europe: Pre-1913
- N24 - Europe: 1913-
- N25 - Asia including Middle East
- N27 - Africa; Oceania
- Browse content in N3 - Labor and Consumers, Demography, Education, Health, Welfare, Income, Wealth, Religion, and Philanthropy
- N32 - U.S.; Canada: 1913-
- Browse content in N4 - Government, War, Law, International Relations, and Regulation
- N43 - Europe: Pre-1913
- Browse content in N7 - Transport, Trade, Energy, Technology, and Other Services
- N71 - U.S.; Canada: Pre-1913
- Browse content in N8 - Micro-Business History
- N80 - General, International, or Comparative
- N82 - U.S.; Canada: 1913-
- Browse content in O - Economic Development, Innovation, Technological Change, and Growth
- Browse content in O1 - Economic Development
- O11 - Macroeconomic Analyses of Economic Development
- O12 - Microeconomic Analyses of Economic Development
- O13 - Agriculture; Natural Resources; Energy; Environment; Other Primary Products
- O16 - Financial Markets; Saving and Capital Investment; Corporate Finance and Governance
- O17 - Formal and Informal Sectors; Shadow Economy; Institutional Arrangements
- Browse content in O2 - Development Planning and Policy
- O23 - Fiscal and Monetary Policy in Development
- Browse content in O3 - Innovation; Research and Development; Technological Change; Intellectual Property Rights
- O30 - General
- O31 - Innovation and Invention: Processes and Incentives
- O32 - Management of Technological Innovation and R&D
- O33 - Technological Change: Choices and Consequences; Diffusion Processes
- O34 - Intellectual Property and Intellectual Capital
- O35 - Social Innovation
- O38 - Government Policy
- Browse content in O4 - Economic Growth and Aggregate Productivity
- O40 - General
- O43 - Institutions and Growth
- Browse content in O5 - Economywide Country Studies
- O53 - Asia including Middle East
- Browse content in P - Economic Systems
- Browse content in P1 - Capitalist Systems
- P16 - Political Economy
- P18 - Energy: Environment
- Browse content in P2 - Socialist Systems and Transitional Economies
- P26 - Political Economy; Property Rights
- Browse content in P3 - Socialist Institutions and Their Transitions
- P31 - Socialist Enterprises and Their Transitions
- P34 - Financial Economics
- P39 - Other
- Browse content in P4 - Other Economic Systems
- P43 - Public Economics; Financial Economics
- P48 - Political Economy; Legal Institutions; Property Rights; Natural Resources; Energy; Environment; Regional Studies
- Browse content in Q - Agricultural and Natural Resource Economics; Environmental and Ecological Economics
- Browse content in Q0 - General
- Q02 - Commodity Markets
- Browse content in Q3 - Nonrenewable Resources and Conservation
- Q31 - Demand and Supply; Prices
- Q32 - Exhaustible Resources and Economic Development
- Browse content in Q4 - Energy
- Q40 - General
- Q41 - Demand and Supply; Prices
- Q42 - Alternative Energy Sources
- Q43 - Energy and the Macroeconomy
- Browse content in Q5 - Environmental Economics
- Q50 - General
- Q51 - Valuation of Environmental Effects
- Q53 - Air Pollution; Water Pollution; Noise; Hazardous Waste; Solid Waste; Recycling
- Q54 - Climate; Natural Disasters; Global Warming
- Q56 - Environment and Development; Environment and Trade; Sustainability; Environmental Accounts and Accounting; Environmental Equity; Population Growth
- Browse content in R - Urban, Rural, Regional, Real Estate, and Transportation Economics
- Browse content in R0 - General
- R00 - General
- Browse content in R1 - General Regional Economics
- R10 - General
- R11 - Regional Economic Activity: Growth, Development, Environmental Issues, and Changes
- R12 - Size and Spatial Distributions of Regional Economic Activity
- Browse content in R2 - Household Analysis
- R20 - General
- R21 - Housing Demand
- R23 - Regional Migration; Regional Labor Markets; Population; Neighborhood Characteristics
- Browse content in R3 - Real Estate Markets, Spatial Production Analysis, and Firm Location
- R30 - General
- R31 - Housing Supply and Markets
- R32 - Other Spatial Production and Pricing Analysis
- R33 - Nonagricultural and Nonresidential Real Estate Markets
- R38 - Government Policy
- Browse content in R4 - Transportation Economics
- R41 - Transportation: Demand, Supply, and Congestion; Travel Time; Safety and Accidents; Transportation Noise
- Browse content in R5 - Regional Government Analysis
- R51 - Finance in Urban and Rural Economies
- Browse content in Z - Other Special Topics
- Browse content in Z1 - Cultural Economics; Economic Sociology; Economic Anthropology
- Z11 - Economics of the Arts and Literature
- Z13 - Economic Sociology; Economic Anthropology; Social and Economic Stratification
- Advance articles
- Editor's Choice
- Author Guidelines
- Submission Site
- Open Access
- About The Review of Financial Studies
- Editorial Board
- Advertising and Corporate Services
- Journals Career Network
- Self-Archiving Policy
- Dispatch Dates
- Terms and Conditions
- Journals on Oxford Academic
- Books on Oxford Academic
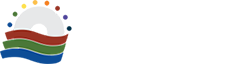
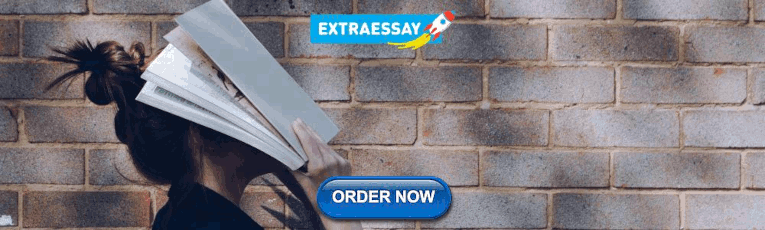
Article Contents
1. the “big data” revolution, 2. what is big data in finance research, 3. what is included in this special issue, 4. where does big data research go from here, acknowledgement, big data in finance.
- Article contents
- Figures & tables
- Supplementary Data
Itay Goldstein, Chester S Spatt, Mao Ye, Big Data in Finance, The Review of Financial Studies , Volume 34, Issue 7, July 2021, Pages 3213–3225, https://doi.org/10.1093/rfs/hhab038
- Permissions Icon Permissions
Big data is revolutionizing the finance industry and has the potential to significantly shape future research in finance. This special issue contains papers following the 2019 NBER-RFS Conference on Big Data. In this introduction to the special issue, we define the “big data” phenomenon as a combination of three features: large size, high dimension, and complex structure. Using the papers in the special issue, we discuss how new research builds on these features to push the frontier on fundamental questions across areas in finance—including corporate finance, market microstructure, and asset pricing. Finally, we offer some thoughts for future research directions.
The digital age has created mountains of data that continue to grow exponentially. The International Data Corporation estimates that the world generates more data every two days than all of humanity generated from the dawn of time to the year 2003. This “big data” revolution is reshaping the financial industry. As the Wall Street Journal wrote, “Today, the ultimate Wall Street status symbol is a trading floor comprising Carnegie Mellon Ph.D.s, not Wharton M.B.A.s.” 1 This industry transition has already started to affect the way we teach students. Along with the drop in the number of Master of Business Administration (MBA) programs, as well as the decline in applications and enrollment in MBA programs, 2 we see a surge of new programs such as Master of Business Analytics (also MBA).
The impact of big data on academic research in finance is also starting to reveal itself, but with it many questions emerge. The classical definition of big data as encompassing three V’s (volume, velocity, and variety) has a strong relation to engineering and computer science, but it does not fully reflect the opportunities and challenges that big data poses to research and practice in finance. What does big data in finance actually mean? How can financial economists benefit from the big data revolution? Does big data open new research topics for financial economists or allow us to answer traditional questions in novel and more revealing ways? Is this really a revolution for finance research or just a continuation of a gradual change? After all, large datasets always have been a feature of research in finance.
In October 2018, the National Science Foundation (NSF) provided a joint grant to the National Bureau of Economic Research (NBER) and the National Center for Supercomputing Application (NCSA) at the University of Illinois at Urbana–Champaign that aimed to explore answers to these questions. Part of the grant is dedicated to education and outreach and support a series of NBER conferences to explore the future of big data research in finance. The summer conferences, organized by Toni Whited and Mao Ye, focus on tutorial sessions on big data techniques and presentations of early ideas on big data. The winter conferences, organized by Itay Goldstein, Chester Spatt, and Mao Ye, focus on completed papers using big data and related methodologies.
This special issue of the Review of Financial Studies (RFS) on big data in finance includes four papers from the first NBER-RFS Winter Conference on Big Data held on March 8, 2019, and two other papers that are closely related to this theme. The RFS has the tradition of encouraging scholars to pursue risky projects that have the potential to push the frontiers of research in finance. The NBER-RFS Conferences on Big Data and this special issue reflect the RFS’s efforts to encourage the use of big data in finance studies and provide a natural complement to the RFS FinTech initiative that was featured in the May 2019 special issue (see Goldstein, Jiang, and Karolyi 2019 for an introduction).
In this introduction, we try to define what “big data” encompasses in the context of finance research. We then review the six papers included in the special issue, discussing how they are related to each other and to the general theme. Finally, we provide some thoughts for future research directions.
It is fairly clear that a definition of big data in finance research should be different from ones that are used in engineering and statistics. Researchers in these disciplines focus on providing facilities and tools to capture, curate, manage, and process data. Financial economists, on the other hand, focus on applying these tools to address interesting economic questions. While it is risky to give a broad-based definition at this stage, we think it is important to try. The definition may be imprecise or incomplete, but it will provide a starting point for future iterations and corrections.
We thus propose three properties that together can potentially define big data in finance research: large size, high dimension, and complex structure. This definition combines the characteristics of the data with possible new research questions that cannot be addressed using “small data.” We used this definition in our call for papers for the 2019 NBER-RFS Winter Conference. “Big data” papers can feature different combinations of these three properties. We now elaborate on what each of these properties captures.
Large size: As the term “big data” suggests, it would be impossible to avoid a reference to size. This feature means that data are large in an absolute or relative sense. A natural example for absolute size is transaction-level market microstructure data. 3 In a relative sense, big data is defined relative to the best existing “small data.” Many datasets are small simply because they are a subset of a larger dataset. By subsampling or aggregating observations into categories or taking snapshots of activities in time series, large datasets are made smaller. Using the underlying larger dataset is important if it overcomes the sample selection bias in the small dataset, or if it captures important economic activities not depicted in the small dataset.
High dimension: “Big data” is not just about size. The second feature means that the data have many variables relative to the sample size. Machine learning, which is often thought of as a hallmark of big data research, is a common solution to the dimension challenge, and it is increasingly used in finance research. Machine learning techniques become economically meaningful if they satisfy, but are not limited to, the following criteria: (i) the actual economic problem involves lots of variables; (ii) the impact of the variables is highly nonlinear or involves interaction terms among the variables (high dimensionality of function class); and (iii) prediction is more important economically than statistical inference. The most natural research questions occur when the decision-makers are machines, such as algorithmic traders or robo-advisors.
Complex structure: Finally, another important feature is that data are not in the traditional row-column format. Unstructured data include text, pictures, videos, audio, and voice. Unstructured data create value if they can measure economic activities that cannot be captured using structured data. Unstructured data are often high-dimensional by nature. The first step to analyze the data is usually to extract features from the unstructured data, often with help from deep learning and computer science. For example, researchers may extract semantic information from text using natural language processing (NLP), identify tone information from voice and audio using speech recognition, and recognize geographic or facial information from images and videos using computer vision (CV).
Overall, as these features reveal, big data is not only about the size of the data, but also about other characteristics. Developments in all three categories—increased availability and capability of handling large datasets, developments in methodologies to deal with high dimensionality, and the emergence of complex datasets with new methods for processing them—have led to the increased prominence of big data in finance research.
Each of the six papers in this special issue fits into one or more of these three categories. Anand et al. (2021) analyze the agency conflicts between brokers and their customers using a particularly large dataset established by the Financial Industry Regulatory Authority (FINRA) called the Order Audit Trail System (OATS). The dataset is big also in the relative sense because the OATS data include publicly unavailable information on broker identity and do not suffer from attrition and sample selection bias from self-reported data. Easley et al. (2021) also analyze large market microstructure data and, due to high dimensionality, apply machine-learning techniques to evaluate the effectiveness of traditional market microstructure measures after machines started dominating trading. The dataset in Giglio, Liao, and Xiu (2021) is distinctive not for its size, but for its high dimensionality. They also use machine-learning techniques to develop a new framework to deal with data snooping, a major concern in empirical asset pricing. Unlike the study by Giglio, Liao, and Xiu (2021) , where high dimensionality comes from a large number of hypothesis tests that may lead to false positives in multiple testing, the high dimensionality in the paper by Erel et al. (2021) comes from the interaction terms and nonlinearity. Erel et al. (2021) show that machines can dominate humans in choosing directors, perhaps because machines suffer less from biases or agency conflicts. Papers by Benamar, Foucault, and Vega (2021) and Li et al. (2021) both use unstructured data. Benamar, Foucault, and Vega (2021) measure information demand and uncertainty using clickstream data provided by a vendor that transforms unstructured data into structured data. Li et al. (2021) transform unstructured data themselves and develop a measure of corporate culture from textual data based on earnings calls.
These six papers cover topics in asset pricing, corporate finance, and market microstructure, demonstrating the broad scope of big data techniques in finance research. We now turn to describe these papers in more detail, their relation to one another, and to the broader theme.
In the first paper in the special issue, Erel et al. (2021) show that machine learning can outperform the actual selection of new board members, currently done by humans. They demonstrate that directors who algorithms predict will perform poorly indeed do, compared to a realistic pool of candidates in out-of-sample tests. 4 Relative to algorithm-selected directors, management-selected directors who later receive predictably low shareholder approval are more likely to be male, have larger networks, and sit on more boards. One possibility is that firms that nominate predictably unpopular directors tend to be subject to homophily, while the algorithm selects a more diverse board. The authors also find that firms that nominate predictably poor directors suffer from worse corporate governance structures, which suggests that agency conflicts could be a driver for the distortion in selecting directors.
The analysis in this paper is among the first applications of machine-learning methods in corporate finance, demonstrating the broad appeal of these methods across areas of finance. The authors demonstrate the usefulness of these methods by showing that traditional OLS results are unable to adequately predict director performance. They attribute these findings to nonlinearity and interactions among variables being key in predicting future performance. These results raise interesting questions for future research, trying to understand why the interaction among variables and/or the nonlinearity in the effects of different variables are so important.
Machine learning in a corporate finance context is a key characteristic of the second paper in the special issue, written by Li et al. (2021) . The authors try to quantify the notion of corporate culture and understand its implications across firms. Corporate culture is important because it is perceived to be a key factor behind many business successes and failures ( Graham et al. 2018 ), and it is thought to be able to solve problems that cannot be regulated properly ex ante ( Guiso, Sapienza, and Zingales 2015 ). Data challenges have always made studying corporate culture a formidable task. Despite the boom in empirical studies since the mid-1980s, 5 variables of economic interest may not be measured perfectly with structured data. Indeed, in the interview evidence by Graham et al. (2018) , corporate executives suggest 11 sources of data to measure corporate culture, most of which are unstructured data.
Li et al. (2021) make progress by using NLP models to extract key features of corporate culture from earnings call transcripts, which is one source of data suggested by corporate executives. They use a semi-supervised machine-learning approach with word embedding for textual analysis instead of the traditional “bag of words” approach ( Loughran and McDonald 2011 ). The “bag of words” approach is good at predicting the tone of a document by counting positive or negative words, but it is hard to capture important semantic information in an earnings call. The authors provide a method to decompose corporate culture onto a five-dimensional space of innovation, integrity, quality, respect, and teamwork, which are the five most-often mentioned values by the S&P 500 firms (see Guiso, Sapienza, and Zingales 2015 ). Guiso, Sapienza, and Zingales (2015) find that the culture-performance link is more significant during periods of distress, and that corporate culture is shaped by major corporate events, such as mergers and acquisitions. They show that firms scoring high on the cultural values of innovation and respect are more likely to be acquirers, and firms closer in cultural value are more likely to merge.
Another area where machine-learning methods have much unexploited potential is market microstructure. The third paper in the special issue, by Easley et al. (2021) , explores an application for analyzing whether machine-based trading affects the efficacy of market microstructure measures that were developed before machines dominated trading volume. Specifically, Easley et al. (2021) examine whether six extant market microstructure measures—the Roll measure, the Roll impact, 6 volatility (VIX), Kyle’s |$\lambda$| , the Amihud measure, and the volume-synchronized probability of informed trading (VPIN)—can still predict the future values of price and liquidity.
The authors find that the answer is still positive after the rise of high-frequency and machine-based trading. The functional form to make such predictions, however, depends on the application. For example, for making predictions within the same asset, a simple logistic regression performs almost as well as complex machine-learning techniques. One explanation is that there is already a deep understanding of the market structure for a single asset. For making predictions across assets, however, machine learning strictly dominates simple logistic regression. 7 Although the rise of high-frequency and machine-based trading has made cross-asset trading more the norm, few market microstructure theories show how these cross-asset effects should, or even could, occur. Easley et al. (2021) provide strong evidence that the interactions among assets can predict market outcomes and that machine learning helps address challenges from high dimensions in cross-asset market microstructure.
Thinking about big data in the context of market microstructure research more broadly, it is often noted that large datasets were the norm in this literature for a long time. Yet, the fourth article in the special issue, by Anand et al. (2021) , pushes the boundary in this sense, analyzing a particularly large dataset to identify agency conflicts between institutional traders and their brokers. To find such agency conflicts, it is very instructive to know the brokers’ identities, which are missing in the publicly available TAQ data. Self-reported data would suffer from attrition or sample selection bias issues. Anand et al. (2021) use OATS data to surmount these two challenges, as it is comprehensive regulatory data from FINRA.
The authors find that brokers, who route more orders to affiliated alternative trading systems (ATSs), offer lower execution quality (lower fill rates and higher implementation shortfall costs) for their customers. Therefore, these brokers take the private benefit by increasing the market share and fee revenues of their own ATSs, but do not necessarily satisfy their fiduciary responsibilities to achieve the best execution for their customers. As Anand et al. (2021) use a large and comprehensive dataset, a subsample of the dataset can still generate enough statistical power, which allows the authors to establish causality using a unique controlled experiment that overlaps with their sample period: the SEC Tick Size Pilot (TSP). Based on a triple-difference analysis, the authors find that execution quality improves for TSP-treated stocks for orders handled by brokers who prefer affiliated ATSs since the TSP imposes constraints on brokers to route orders to ATS venues.
The fifth paper in the special issue, written by Benamar, Foucault, and Vega (2021) , also analyzes a large dataset in the context of trading in financial markets. Another important feature of this paper is the processing of unstructured data. Here, unlike in Li et al. (2021) , who process such data themselves, Benamar, Foucault, and Vega rely on commercial data vendors that preprocessed the raw and unstructured data into structured data. This is part of the trend in the era of big data: along with the boom of data availability, the data vending industry has grown as well. J. P. Morgan’s Big Data and AI Strategies report provides a 78-page summary of available data vendors. 8 Benamar, Foucault, and Vega (2021) measure information demand with webpage clickstream statistics from Bitly, a URL-shortening service provider. 9 They use this to understand the role of uncertainty in financial market trading, a topic that has long occupied academics studying financial markets.
Benamar, Foucault, and Vega (2021) show that information demand is a good proxy for uncertainty because, based on their theory, an exogenous increase in an asset’s uncertainty motivates investors to search for more information on it. The search for information, however, cannot fully neutralize the increase in uncertainty. Thus, a stronger information demand about future interest rates ahead of macroeconomic and monetary policy announcements (MMPAs) implies that U.S. Treasury yields exhibit both higher uncertainty and stronger sensitivity to MMPAs. They find that a one-standard-deviation increase in the number of Bitly clicks on the news related to nonfarm payroll (NFP) in the two hours preceding NFP announcements raises the sensitivity of U.S. Treasury note yields by 4 to 6 basis points (bps), depending on maturity. The increase is economically significant because the unconditional sensitivity of U.S. Treasury note yields to NFP announcements varies between 3. 5 bps and 7 bps (depending on maturity) during their sample period. They also find that such predictability mostly comes from clicks within two hours before the announcement, which highlights the usefulness of high-frequency data for measuring information demand and uncertainty.
Finally, closing the special issue is the paper by Giglio, Liao, and Xiu (2021) . This paper belongs to the asset pricing literature, in which machine-learning methods have already been explored in some depth. A recent special issue of the Review of Financial Studies featured some of this research in the context of new methods for the cross-section of returns (see Karolyi and Van Nieuwerburgh 2020 for an introduction). Giglio, Liao, and Xiu (2021) show how machine learning can be applied by proposing a new framework to rigorously perform multiple hypothesis testing in linear asset pricing models, with a focus on addressing data snooping.
The dimension challenge in Giglio, Liao, and Xiu (2021) comes from multiple testing—that is, when trying to identify which factors in the “factor zoo” add explanatory power for the cross-section of returns or to identify which funds among thousands of funds can produce positive alpha. If the number of tests is high due to a large number of factors or funds, a potentially large fraction of the tests will be positive purely by chance and lead to a high false discovery rate. Giglio, Liao, and Xiu (2021) solve data snooping and false positives using a combination of matrix completion, wild bootstrap, screening, and false discovery control. Matrix completion, a machine-learning technique, helps them to interpolate missing data and latent factors. The latent factors constructed from machine learning correct correlation among alpha test statistics. Bootstrap and screening improve the robustness of multiple testing in a finite and skewed sample. The authors illustrate their framework using a hedge fund dataset, but their toolbox can be applied in other asset pricing research as well.
The six papers in this special issue can provide a starting point for discussing big data in finance. As a burgeoning field, big data and machine learning raise many new questions. We discuss several promising lines of research. We believe the list will continue to grow and be refined over time.
4.1 Machine learning and learning machines
To date, most research using machine learning, including papers in this special issue, use machine learning to understand human behavior. One promising area of machine learning in finance is when the decision-makers are machines. For example, most existing machine-learning research in asset pricing uses monthly return data from CRSP or quarterly holding data from 13F filings. Yet traders who apply machine learning techniques often operate at a horizon that is much less than a month. Hedge funds such as Renaissance, Two Sigma Investments, D. E. Shaw Group, PDT Partners, and TGS Management Company make thousands of trades and manage tens of billions of dollars in investor assets. 10 These firms, which are faster than most traditional funds but slower than high-frequency traders, are largely outside the radar of the academic finance literature. One exception is Chinco, Clark-Joseph, and Ye (2019) , who find that machine learning aims to predict news at the minute-by-minute horizon. A promising new line of research is to bridge the gap between studies that focus on the monthly horizon or above and the studies on high-frequency traders, which focus on horizons below a second. In this underexplored territory, applying machine learning is not only natural but also necessary. Just as insights into human behavior from the psychology literature spawned the field of behavioral finance, so can insights into algorithmic behavior (or the psychology of machines) spawn an analogous blossoming of research in algorithmic behavioral finance.
4.2 Feedback effects of the big data revolution
Once machines become decision-makers, will corporations change their behavior? The widespread application of machine learning in the investment community and the feedback effects between the secondary market and corporate decisions ( Bond, Edmans, and Goldstein 2012 ) imply that firms should respond to the big data revolution. While no papers in this special issue examine feedback effects, we saw related studies at the 2020 NBER-RFS Winter Conference on Big Data. Cao et al. (2020) find that firms adjust their 10-Ks and 10-Qs to cater to machine readers. The next step following their research is perhaps to examine whether firms react to the big data revolution when making real decisions. For example, as investors increasingly become machines, will firms increasingly pursue shorter-term projects? Does the advent of “big data” reduce managers’ incentives to learn from market prices because firms now have more information sources, or does it increase incentives because prices aggregate more information from the “big data” collected by investors?
4.3 Heterogeneous impact of the big data revolution
Although big data provides more information for sophisticated players such as institutional investors and firms, the impact of big data may not always be positive. Chawla et al. (2019) show that social media, which allows enthusiasm for the market to spread much more widely than it would have otherwise ( Shiller (2015) ), can push price away from fundamentals. In Chawla et al. (2019) , the price pressures led by retail traders quickly revert, probably because sophisticated arbitragers rapidly jump in and trade against retail behavioral bias. We witnessed a much more significant impact of social media during the GameStop episode in January 2021. Retail traders coordinated using social media, resulting in the hedge fund Melvin Capital losing 53%. 11 The interaction between retail and sophisticated investors leads to extreme market volatility. The impact of big data on different types of agents and its aggregated effect on society will be an interesting new direction to explore.
4.4 More complex data
Big data in finance starts from analyzing large-size data such as trades and quotes. More recent development allows researchers to use natural language processing (NLP) to extract information from unstructured data such as text ( Gentzkow, Kelly, and Taddy 2019 ). A promising research line is to analyze data of more complex structures, such as audio, video, and images if these more complex data provide additional insights. For example, Li et al. (2021) use the transcripts of earnings call as input for their analysis in this special issue. The earnings call transcripts are small data when we compare them with the audio file that generates the transcripts. Mayew and Venkatachalam (2012) show that managerial vocal cues contain information about a firm’s fundamentals, incremental to information conveyed by linguistic content. As the NBER-RFS Big Data Conference evolves, we see submissions using more complex datasets, such as satellite images ( Gerken and Painter 2020 ). More complex datasets create value for finance researchers if they measure economic activities that cannot be captured using simpler data.
4.5 Regulations
As machines start to be major players in many areas such as trading ( Angel, Harris, and Spatt 2015 ), it will be interesting to examine whether existing regulations, which are designed mostly for humans, need to be adapted to an environment with machines. O’Hara, Yao, and Ye (2014) provide one example for such need. Regulators used to consider trades of less than 100 shares to come from retail traders, and would exempt these odd lots from the reporting requirement. Yet informed traders later became major sources of odd lots by using algorithms to slice and dice their orders to less than 100 shares to escape the reporting requirement. While much of our financial regulatory system focuses on actual realized transactions, assessing problematic aspects of the underlying algorithms is arguably more fundamental and cuts to the heart of such issues as the possibility of front running by market markers, whether brokers have satisfied their best execution responsibilities, and whether insiders are exploiting informational advantages. Spatt (2020) discusses how regulations designed years ago need to be adapted to modern reality. The traditional focus of regulators has not emphasized biases in specific algorithms.
The other promising line of research on big data will be on privacy regulations and the fairness of algorithms and data (e. g., Kearns and Roth 2020 ). The question becomes extremely important because algorithms and data increasingly became a major resource for the economy, particularly for finance. Back in 2017, the Economist published a story titled “The World’s Most Valuable Resource Is No Longer Oil, but Data,” which called for new regulations for the data economy. 12 Who owns the data, what is the price of the data, and what is the impact of unfair access to data? Easley, O’Hara, and Yang (2016) provide a theoretical analysis of the issue. It would be interesting to explore this topic empirically.
The papers in this special issue are predominantly empirical, but theoretical work is also important for big data in finance. Although high-dimensional data are often defined as when the number of variables is larger than the number of observations ( Martin and Nagel 2019 ), the dataset frequently used in finance research is typically large enough to cover the number of variables. The success of machine learning often comes from high-order interaction terms between variables ( Mullainathan and Spiess 2017 ). Indeed, the success of machine learning for the papers in this issue also comes from nonlinear terms and interactions between variables. Such high-order interactions are a natural place to develop new theoretical models to explain why one economic variable’s impact depends on its interaction with another variable. The nonlinearity also motivates theory models to explain why a variable’s impact depends largely on its value. Machine learning is one way to describe the world, and we also need theory to explain the world.
Theory may become more important in the era of machine learning and artificial intelligence for one simple reason. Human judgment can be inconsistent, whereas machines tend to make consistent decisions based on their model. Li and Ye (2020) find that their theory model can generate quantitatively accurate predictions for market liquidity in cross-section and after corporate events such as stock splits, probably because liquidity providers are now algorithms, and these algorithms probably make decisions using similar models to the theoretical models in Li and Ye (2020) .
4.7 Interdisciplinary collaborations
Future work on big data in finance may involve more scholars from other fields. We believe such collaborations will expand the tools and scope of research in finance and economics and help researchers overcome big data challenges.
Researchers can overcome the large-size challenge by collaborating with supercomputing centers. The NSF’s Extreme Science and Engineering Discovery Environment Project (XSEDE) provides computing resources and staff support to manage and store large datasets free of charge. NBER has posted videotaped lectures for researchers in economics and finance on the application process for such free resources on the webpage for the 2018 Summer Conference on Big Data. 13
Researchers can overcome the high-dimension challenge and the complex-structure challenge by collaborating with scholars from the fields of math, statistics, and computer science. The recent development in deep-learning models like natural language processing (NLP), speech recognition, and computer vision (CV) helps researchers parse textual, verbal, and visual data. Researchers can also choose to work with data vendors. J. P. Morgan’s Big Data and AI Strategies report provides a list of vendors for alternative data, such as satellite photos, sentiment measures, and credit card usages.
The NSF lists big data as one of its 10 big ideas and provides funding to support innovative, interdisciplinary research in data science. We hope this special issue is only a starting point, and that we will see more research at the intersection of big data, finance, and public policy for many years.
This introduction is written for a special issue of the Review of Financial Studies focused on big data in finance. The authors thank Ken French, Harrison Hong, Wei Jiang, Andrew Karolyi, and Jim Poterba for comments. We thank Jim Poterba and Carl Beck for help with the NBER Workshops on Big Data. Ye acknowledges support from National Science Foundation grant 1838183 and the Extreme Science and Engineering Discovery Environment (XSEDE).
1 G. Rogow, “Meet the New Kings of Wall Street,” Wall Street Journal , May 21, 2017, https://www.wsj.com/articles/the-quants-meet-the-new-kings-of-wall-street-1495389163 .
2 C. Cutter, “Elite MBA Programs Report Steep Drop in Applications,” Wall Street Journal , October 15, 2019, https://www.wsj.com/articles/elite-m-b-a-programs-report-steep-drop-in-applications-11571130001 .
3 One day of current option trading data alone is roughly two terabytes. In the 2019 NBER-RFS Summer Conference on Big Data supported by the same NSF grant, the chief economist of the U.S. Securities and Exchange Commission (SEC), S. P. Kothari, pointed out that one of the biggest data collection efforts in finance is the Consolidated Audit Trial (CAT), which provides a single, comprehensive database enabling regulators to track more efficiently and thoroughly all trading activity in equities and options throughout the U.S. markets. https://www.sec.gov/news/speech/policy-challenges-research-opportunities-era-big-data .
4 The task of measuring the performance of an individual director is challenging because directors generally act collectively on the board. The authors’ main measure of director performance is the level of shareholder support in annual director reelections, because Hart and Zingales (2017) emphasize that directors’ fiduciary duty is to represent the interests of the firm’s shareholders.
5 See Einav and Levin (2014) .
6 Roll impact is the Roll measure divided by the dollar value traded over a certain period.
7 The cross-asset effects in their paper mean using market microstructure measures in one asset, such as equity futures, to predict price and liquidity dynamics of another asset, such as fixed-income futures.
8 Kolanovic and Krishnamachari (2017) .
9 A shortened URL is a compressed link to certain webpages. For example, https://academic.oup.com/rfs/advance-articles can be shortened to https://bit.ly/3mS7yDv .
10 G. Zuckerman and B. Hope, “The Quants Run Wall Street Now,” Wall Street Journal , May 21, 2017, https://www.wsj.com/articles/the-quants-run-wall-street-now-1495389108 .
11 J. Chung, “Melvin Capital Lost 53% in January, Hurt by GameStop and Other Bets, ” Wall Street Journal, January 31, 2021, https://www.wsj.com/articles/melvin-capital-lost-53-in-january-hurt-by-gamestop-and-other-bets-11612103117 .
12 “The World’s Most Valuable Resource Is No Longer Oil, but Data,” Economist , May 6, 2017, https://www.economist.com/leaders/2017/05/06/the-worlds-most-valuable-resource-is-no-longer-oil-but-data .
13 http://www2.nber.org/si2018_video/bigdatafinancialecon/ .
Anand, A. , Samadi M. , Sokobin J. , and Venkataraman K. . 2021 . Institutional order handling and broker-affiliated trading venues . Review of Financial Studies 34:3364–402.
Google Scholar
Angel, J. J. , Harris L. E. , and Spatt C. S. . 2015 . Equity trading in the 21st century: An update . Quarterly Journal of Finance 5 : 1 – 39 .
Benamar, H. , Foucault T. , and Vega C. . 2021 . Demand for information, uncertainty, and the response of US Treasury securities to news . Review of Financial Studies 34:3403–55.
Bond, P. , Edmans A. , and Goldstein I. . 2012 . The real effects of financial markets . Annual Review of Financial Economics 4 : 339 – 60 .
Cao, S. , Jiang W. , Yang B. , and Zhang A. L. . 2020 . How to talk when a machine is listening: Corporate disclosure in the age of AI . NBER Working Paper 27950 .
Google Preview
Chawla, N. , Da Z. , Xu J. , and Ye M. . 2019 . Information diffusion on social media: Does it affect trading, return, and liquidity? Working Paper .
Chinco, A. , Clark-Joseph A. D. , and Ye M. . 2019 . Sparse signals in the cross-section of returns . Journal of Finance 74 : 449 – 92 .
Easley, D. , Lopez de Prado M. , O’Hara M. , and Zhang Z. . 2021 . Microstructure in the machine age . Review of Financial Studies 34:3316–63.
Easley, D. , O’Hara M. , and Yang L. . 2016 . Differential access to price information in financial markets . Journal of Financial and Quantitative Analysis 51 : 1071 – 1110 .
Einav, L. , and Levin J. . 2014 . Economics in the age of big data . Science 346 ( 6210 ): 715 .
Erel, I. , Stern L. , Tan C. , and Weisbach M. S. . 2021 . Selecting directors using machine learning . Review of Financial Studies 34:3226–64.
Gentzkow, M. , Kelly B. , and Taddy M. . 2019 . Text as data . Journal of Economic Literature 57 : 535 – 74 .
Gerken, W. C. , and Painter M. . 2020 . The value of differing points of view: Evidence from Financial Analysts’ Geographic Diversity . Working Paper .
Giglio, S. , Liao Y. , and Xiu D. . 2021 . Thousands of alpha tests . Review of Financial Studies 34:3456–96.
Goldstein, I. , Jiang W. , and Karolyi G. A. . 2019 . To FinTech and beyond . Review of Financial Studies 32 : 1647 – 61 .
Graham, J. R. , Grennan J. , Harvey C. R. , and Rajgopal S. . 2018 . Corporate culture: The interview evidence . Working Paper .
Guiso, L. , Sapienza P. , and Zingales L. . 2015 . The value of corporate culture . Journal of Financial Economics 117 : 60 – 76 .
Hart, O. , and Zingales L. . 2017 . Companies should maximize shareholder welfare not market value . Journal of Law, Finance, and Accounting 2 : 247 – 74 .
Karolyi, G. A. , and Van Nieuwerburgh S. . 2020 . New methods for the cross-section of returns . Review of Financial Studies 33 : 1879 – 90 .
Kearns, M. , and Roth A. . 2020 . The ethical algorithm: The science of socially aware algorithm design . Oxford : Oxford University Press .
Kolanovic, M. , and Krishnamachari R. . 2017 . Big data and AI strategies: Machine learning and alternative data approach to investing. J. P. Morgan. Available at https://www.cfasociety.org/cleveland/Lists/Events
Li, K. , Mai F. , Shen R. , and Yan X. . 2021 . Measuring corporate culture using machine learning . Review of Financial Studies 34:3265–315.
Li, S. , and Ye M. . 2020 . The share price that maximizes liquidity: A tale of two discretenesses . Working Paper .
Loughran, T. , and McDonald B. . 2011 . When is a liability not a liability? Textual analysis, dictionaries, and 10-Ks . Journal of Finance 66 : 35 – 65 .
Martin, I. , and Nagel S. . 2019 . Market efficiency in the age of big data . NBER Working Paper 26586 .
Mayew, W. J. , and Venkatachalam M. . 2012 . The power of voice: Managerial affective states and future firm performance . Journal of Finance 67 : 1 – 43 .
Mullainathan, S. , and Spiess J. . 2017 . Machine learning: An applied econometric approach . Journal of Economic Perspectives 31 : 87 – 106 .
O’Hara, M. , Yao C. , and Ye M. . 2014 . What’s not there: Odd lots and market data . Journal of Finance 69 : 2199 – 236 .
Shiller, R. J. 2015 . Irrational exuberance . Princeton, NJ : Princeton University Press .
Spatt, C. S. 2020 . Is equity market exchange structure anti-competitive? Working Paper .
Email alerts
Citing articles via.
- Recommend to your Library
Affiliations
- Online ISSN 1465-7368
- Print ISSN 0893-9454
- Copyright © 2024 Society for Financial Studies
- About Oxford Academic
- Publish journals with us
- University press partners
- What we publish
- New features
- Open access
- Institutional account management
- Rights and permissions
- Get help with access
- Accessibility
- Advertising
- Media enquiries
- Oxford University Press
- Oxford Languages
- University of Oxford
Oxford University Press is a department of the University of Oxford. It furthers the University's objective of excellence in research, scholarship, and education by publishing worldwide
- Copyright © 2024 Oxford University Press
- Cookie settings
- Cookie policy
- Privacy policy
- Legal notice
This Feature Is Available To Subscribers Only
Sign In or Create an Account
This PDF is available to Subscribers Only
For full access to this pdf, sign in to an existing account, or purchase an annual subscription.
- Open access
- Published: 12 March 2020
Current landscape and influence of big data on finance
- Md. Morshadul Hasan ORCID: orcid.org/0000-0001-9857-9265 1 ,
- József Popp ORCID: orcid.org/0000-0003-0848-4591 2 &
- Judit Oláh ORCID: orcid.org/0000-0003-2247-1711 2
Journal of Big Data volume 7 , Article number: 21 ( 2020 ) Cite this article
58k Accesses
92 Citations
32 Altmetric
Metrics details
Big data is one of the most recent business and technical issues in the age of technology. Hundreds of millions of events occur every day. The financial field is deeply involved in the calculation of big data events. As a result, hundreds of millions of financial transactions occur in the financial world each day. Therefore, financial practitioners and analysts consider it an emerging issue of the data management and analytics of different financial products and services. Also, big data has significant impacts on financial products and services. Therefore, identifying the financial issues where big data has a significant influence is also an important issue to explore with the influences. Based on these concepts, the objective of this paper was to show the current landscape of finance dealing with big data, and also to show how big data influences different financial sectors, more specifically, its impact on financial markets, financial institutions, and the relationship with internet finance, financial management, internet credit service companies, fraud detection, risk analysis, financial application management, and so on. The connection between big data and financial-related components will be revealed in an exploratory literature review of secondary data sources. Since big data in the financial field is an extremely new concept, future research directions will be pointed out at the end of this study.
Introduction
In the age of technological innovation, various types of data are available with the advance of information technologies, and data is seen as one of the most valuable commodities in managing automation systems [ 13 , 68 ]. In this sense, financial markets and technological evolution have become related to every human activity in the past few decades. Big data technology has become an integral part of the financial services industry and will continue to drive future innovation [ 12 ]. Financial innovations are also considered the fastest emerging issues in financial services. More specifically, they cover a variety of financial businesses such as online peer-to-peer lending, crowd-funding platforms, SME finance, wealth management and asset management platforms, trading management, crypto-currency, money/remittance transfer, mobile payments platforms, and so on. All of these services create thousands of pieces of data every day. Therefore, managing this data is also considered the most important factor in these services. Any damage to the data can cause serious problems for that specific financial industry. Nowadays, financial analysts use external and alternative data to make better investment decisions. In addition, financial industries use big data through different predictive analyses and monitor various spending patterns to develop large decision-making models. In this way, the industries can decide which financial products to offer [ 29 , 48 ]. Millions of data are transmitted among financial companies. That is why big data is receiving more attention in the financial services arena, where information affects important success and production factors. It has been playing increasingly important roles in consolidating our understanding of financial markets [ 71 ]. In any case, the financial industry is using trillions of pieces of data constantly in everyday decisions [ 22 ]. It plays an important role in changing the financial services sector, particularly in trade and investment, tax reform, fraud detection and investigation, risk analysis, and automation [ 37 ]. In addition, it has changed the financial industry by overcoming different challenges and gaining valuable insights to improve customer satisfaction and the overall banking experience [ 45 ]. Razin [ 65 ] pointed out that big data is also changing finance in five ways: creating transparency, analyzing risk, algorithmic trading, leveraging consumer data and transforming culture. Also, big data has a significant influence in economic analysis and economic modeling [ 16 , 21 ].
In this study, the views of different researchers, academics, and others related to big data and finance activities have been collected and analysed. This study not only attempts to test the existing theory but also to gain an in-depth understanding of the research from the qualitative data. However, research on big data in financial services is not as extensive as other financial areas. Few studies have precisely addressed big data in different financial research contexts. Though some studies have done these for some particular topics, the extensive views of big data in financial services haven’t done before with proper explanation of the influence and opportunity of big data on finance. Therefore, the need to identify the finance areas where big data has a significant influence is addressed. Also, the research related to big data and financial issues is extremely new. Therefore, this study presents the emerging issues of finance where big data has a significant influence, which has never been published yet by other researchers. That is why this research explores the influence of big data on financial services and this is the novelty of this study.
This paper seeks to explore the current landscape of big data in financial services. Particularly this study highlights the influence of big data on internet banking, financial markets, and financial service management. This study also presents a framework, which will facilitate the way how big data influence on finance. Some other services relating to finance are also highlighted here to specify the extended area of big data in financial services. These are the contribution of this study in the existing literatures.
This result of the study contribute to the existing literature which will help readers and researchers who are working on this topic and all target readers will obtain an integrated concept of big data in finance from this study. Furthermore, this research is also important for researchers who are working on this topic. The issue of big data has been explored here from different financing perspectives to provide a clear understanding for readers. Therefore, this study aims to outline the current state of big data technology in financial services. More importantly, an attempt has been made to focus on big data finance activities by concentrating on its impact on the finance sector from different dimensions.
Literature review
The concept of big data in finance has taken from the previous literatures, where some studies have been published by some good academic journals. At present, most of the areas of business are linked to big data. It has significant influence on various perspectives of business such as business process management, human resources management, R&D management [ 8 , 63 ], business analytics [ 19 , 26 , 42 , 59 , 63 ], B2B business process, marketing, and sales [ 30 , 39 , 53 , 58 ], industrial manufacturing process [ 7 , 15 , 40 ], enterprise’s operational performance measurement [ 20 , 69 , 81 ], policy making [ 2 ], supply chain management, decision, and performance [ 4 , 38 , 64 ], and so other business arenas.
Particularly, Rabhi et al. [ 63 ] mentioned big data as a significant factor of business process management& HR process to support the decision making. This study also talked about three sophisticated types of analytics techniques such as descriptive analytics, predictive analytics, and prescriptive analytics in order to improve the traditional data analytics process. Duan and Xiong [ 19 ], Grover and Kar [ 26 ], Ji et al. [ 42 ], and Pappas et al. [ 59 ] also explored the significance of big data in business analytics. Big data helps to solve business problems and data management through system infrastructure, which includes any technique to capture, store, transfer, and process data. Duan and Xiong [ 19 ] found that top-performing organizations use analytics as opposed to intuition almost five times more than do the lower performers. Business analytics and business strategy must be closely linked together to gain better analytics-driven insights. Grover and Kar [ 26 ] mentioned about firms, like Apple, Facebook, Google, Amazon, and eBay, that regularly use digitized transaction data such as storing the transaction time, purchase quantities, product prices, and customer credentials on regular basis to estimate the condition of their market for improving their business operations [ 61 , 76 ]. Holland et al. [ 39 ] showed the theoretical and empirical contributions of big data in business. This study inferred that B2B relationships from consumer search patterns, which used to evaluate and measure the online performance of competitors in the US airline market. Moreover, big data also help to foster B2B sales with customer data analytics. The use of customer’s big datasets significantly improve sales growth (monetary performance outcomes), and enhances the customer relationship performance (non-monetary performance outcomes) [ 30 ]. It also relates to market innovation with diversified opportunities.
Big data and its analytics and applications work as indicators of organizations’ ability to innovate to respond to market opportunities [ 78 ]. Also, big data impact on industrial manufacturing process to gain competitive advantages. After analyzing a case study of two company, Belhadi et al. [ 7 ] stated ‘NAPC aims for a qualitative leap with digital and big - data analytics to enable industrial teams to develop or even duplicate models of turnkey factories in Africa’. This study also identified an Overall framework of BDA capabilities in manufacturing process , and mentioned some values of Big Data Analytics for manufacturing process, such as enhancing transparency, improving performance, supporting decision-making and increasing knowledge. Also, Cui et al. [ 15 ] mentioned four most frequently big data applications (Monitoring, prediction, ICT framework, and data analytics) used in manufacturing. These are essential to realize the smart manufacturing process. Shamim et al. [ 69 ] argued that employee ambidexterity is important because employees’ big data management capabilities and ambidexterity are crucial for EMMNEs to manage the demands of global users. Also big data appeared as a frontier of the opportunity in improving firm performance. Yadegaridehkordi et al. [ 81 ] hypothesized that big data adoption has positive effect on firm performance. That study also mentioned that the policy makers, governments, and businesses can take well-informed decisions in adopting big data. According to Hofmann [ 38 ], velocity, variety, and volume significantly influence on supply chain management. For example, at first, velocity offers the biggest opportunity to intensification the efficiency of the processes in the supply chain. Next to this, variety supports different types of data volume in the supply chains is mostly new. After that, the volume is also a bigger interest for the multistage supply chains than to two-staged supply chains. Raman et al. [ 64 ] provided a new model, Supply Chain Operations Reference (SCOR), by incorporating SCM with big data. This model exposes the adoption of big data technology adds significant value as well as creates financial gain for the industry. This model is apt for the evaluation of the financial performance of supply chains. Also it works as a practical decision support means for examining competing decision alternatives along the chain as well as environmental assessment. Lamba and Singh [ 50 ] focused on decision making aspect of supply chain process and mentioned that data-driven decision-making is gaining noteworthy importance in managing logistics activities, process improvement, cost optimization, and better inventory management. Sahal et al. [ 67 ] and Xu and Duan [ 80 ] showed the relation of cyber physical systems and stream processing platform for Industry 4.0. Big data and IoT are considering as much influential forces for the era of Industry 4.0. These are also helping to achieve the two most important goals of Industry 4.0 applications (to increase productivity while reducing production cost & to maximum uptime throughout the production chain). Belhadi et al. [ 7 ] identified manufacturing process challenges, such as quality & process control (Q&PC), energy & environment efficiency (E&EE), proactive diagnosis and maintenance (PD&M), and safety & risk analysis (S&RA). Hofmann [ 38 ] also mentioned that one of the greatest challenges in the field of big data is to find new ways for storing and processing the different types of data. In addition, Duan and Xiong [ 19 ] mentioned that big data encompass more unstructured data such as text, graph, and time-series data compared to structured data for both data storage techniques and data analytics techniques. Zhao et al. [ 86 ] identified two major challenges for integrating both internal and external data for big data analytics. These are connecting datasets across the data sources, and selecting relevant data for analysis. Huang et al. [ 40 ] raised four challenges, first, the accuracy and applicability of the small data-based PSM paradigms is one kind of challenge; second, the traditional static-oriented PSM paradigms difficult to adapt to the dynamic changes of complex production systems; third, it is urgent to carry out research that focuses on forecasting-based PSM paradigms; and fourth, the determining the causal relationship quickly, economically and effectively is difficult, which affects safety predictions and safety decision-making.
The above discussion based on different area of business. Whatever, some studies (such as [ 6 , 11 , 14 , 22 , 23 , 41 , 45 , 54 , 68 , 71 , 73 , 75 , 83 , 85 ] focused different perspectives of financial services. Still, the contribution on this area is not expanded. Based on those researches, the current trends of big data in finance have specified in finding section.
Methodology
The purpose of this study is to locate academic research focusing on the related studies of big data and finance. To accomplish this research, secondary data sources were used to collect related data [ 31 , 32 , 34 ]. To collect secondary data, the study used the electronic database Scopus, the web of science, and Google scholar [ 33 ]. The keywords of this study are big data finance, finance and big data, big data and the stock market, big data in banking, big data management, and big data and FinTech. The search mainly focused only on academic and peer-reviewed journals, but in some cases, the researcher studied some articles on the Internet which were not published in academic and peer-reviewed journals. Sometimes, information from search engines helps understand the topic. The research area of big data has already been explored but data on big data in finance is not so extensive; this is why we did not limit the search to a certain time period because a time limitation may reduce the scope of the area of this research. Here, a structured and systematic data collection process was followed. Figure 1 presents the structured and systematic data collection process of this study. Certain renowned publishers, for example, Elsevier, Springer, Taylor & Francis, Wiley, Emerald, and Sage, among others, were prioritized when collecting the data for this study [ 35 , 36 ].
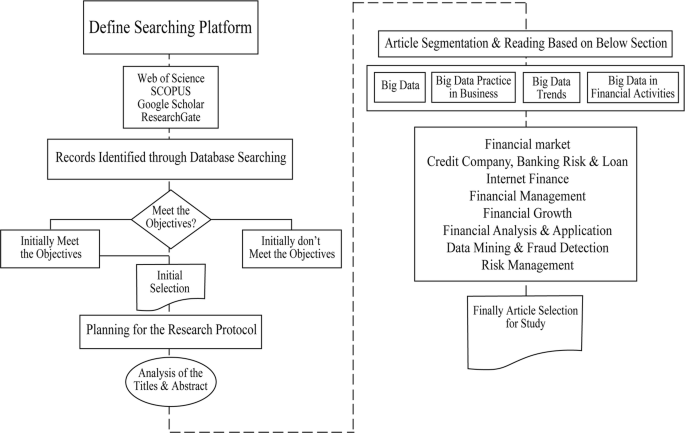
Systematic framework of the research structure. (Source: Author’s illustration)
The number of related articles collected from those databases is only 180. Following this, the collected articles were screened and a shortlist was created, featuring only 100 articles. Finally, data was used from 86 articles, of which 34 articles were directly related to ‘ Big data in Finance’ . Table 1 presents the list of those journals which will help to contribute to future research.
This literature study suggests that some major factors are related to big data and finance. In this context, it has been found that these specific factors also have a deep relationship with big data, such as financial markets, banking risk and lending, internet finance, financial management, financial growth, financial analysis and application, data mining and fraud detection, risk management, and other financial practices. Table 2 describes the focuses within the literature on the financial sector relating to big data.
Theoretical framework
After studying the literature, this study has found that big data is mostly linked to financial market, Internet finance. Credit Service Company, financial service management, financial applications and so forth. Mainly data relates with four types of financial industry such as financial market, online marketplace, lending company, and bank. These companies produce billions of data each day from their daily transaction, user account, data updating, accounts modification, and so other activities. Those companies process the billions of data and take the help to predict the preference of each consumer given his/her previous activities, and the level of credit risk for each user. Based on those data, financial institutions help in taking decisions [ 84 ]. However, different financial companies processing big data and getting help for verification and collection, credit risk prediction, and fraud detection. As the billions of data are producing from heterogeneous sources, missing data is a big concern as well as data quality and data reliability is also significant matter. Whatever, the concept of role of financial big data has taken form [ 71 ], where that study mention the sources of financial market information include the information assembled from stock market data (e.g., stock prices, stock trading volume, interest rates, and so on), social media (e.g., Facebook, twitter, newspapers, advertising, television, and so on). These data has significant roles in financial market such as predicting the market return, forecasting market volatility, valuing market position, identifying excess trading volume, analyzing the market risk, movement of the stock, option pricing, algorithmic trading, idiosyncratic volatility, and so on. Based on these discussions, a theoretical framework is illustrated in Fig. 2 .
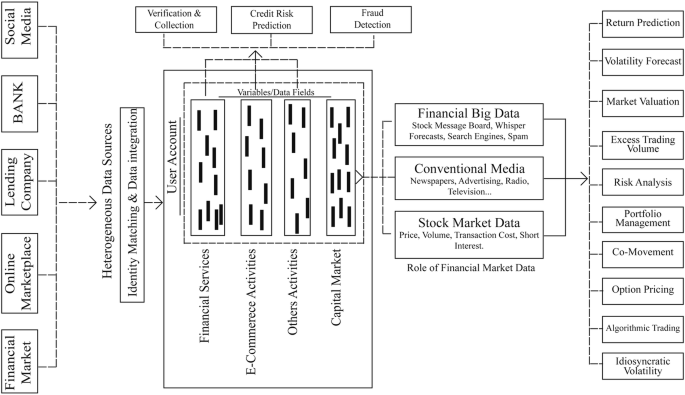
Theoretical framework of big data in financial services. Source: Author’s explanation. (This concept of this framework has been taken from Shen and Chen [ 71 ] and Zhang et al. [ 85 ])
Results and discussion
Massive data and increasingly sophisticated technologies are changing the way industries operate and compete. The financial world is also operating with these big data sets. It has not only influenced many fields of science and society, but has had an important impact on the finance industry [ 6 , 13 , 23 , 41 , 45 , 54 , 62 , 68 , 71 , 72 , 73 , 82 , 85 ]. After reviewing the literature, this study found some financial areas directly linked to big data, such as financial markets, internet credit service-companies and internet finance, financial management, analysis, and applications, credit banking risk analysis, risk management, and so forth. These areas are divided here into three groups; first, big data implications for financial markets and the financial growth of companies; second, big data implications for internet finance and value creation in internet credit-service companies; and third, big data in financial management, risk management, financial analysis, and applications. The discussion of big data in these specified financial areas is the contribution made by this study. Also, these are regarded as emerging landscape of big data in finance in this study.
Big data implications on financial markets
Financial markets always seek technological innovation for different activities, especially technological innovations that are always positively accepted, and which have a great impact on financial markets, and which have truly transforming effects on them. Shen and Chen [ 71 ] explain that the efficiency of financial markets is mostly attributed to the amount of information and its diffusion process. In this sense, social media undoubtedly plays a crucial role in financial markets. In this sense, it is considered one of the most influential forces acting on them. It generates millions of pieces of information every day in financial markets globally [ 9 ]. Big data mainly influences financial markets through return predictions, volatility forecasts, market valuations, excess trading volumes, risk analyses, portfolio management, index performance, co-movement, option pricing, idiosyncratic volatility, and algorithmic trading.
Shen and Chen [ 71 ] focus on the medium effect of big data on the financial market. This effect has two elements, effects on the efficient market hypothesis, and effects on market dynamics. The effect on the efficient market hypothesis refers to the number of times certain stock names are mentioned, the extracted sentiment from the content, and the search frequency of different keywords. Yahoo Finance is a common example of the effect on the efficient market hypothesis. On the other hand, the effect of financial big data usually relies on certain financial theories. Bollen et al. [ 9 ] emphasize that it also helps in sentiment analysis in financial markets, which represents the familiar machine learning technique with big datasets.
In another prospect, Begenau et al. [ 6 ] explore the assumption that big data strangely benefits big firms because of their extended economic activity and longer firm history. Even large firms typically produce more data compared to small firms. Big data also relates corporate finance in different ways such as attracting more financial analysis, as well as reducing equity uncertainty, cutting a firm’s cost of capital, and the costs of investors forecasting related to a financial decision. It cuts the cost of capital as investors process more data to enable large firms to grow larger. In pervasive and transformative information technology, financial markets can process more data, earnings statements, macro announcements, export market demand data, competitors’ performance metrics, and predictions of future returns. By predicting future returns, investors can reduce uncertainty about investment outcomes. In this sense Begenau et al. [ 6 ] stated that “More data processing lowers uncertainty, which reduces risk premia and the cost of capital, making investments more attractive.”.
Big data implications on internet finance and value creation at an internet credit service company
Technological advancements have caused a revolutionary transformation in financial services; especially the way banks and FinTech enterprises provide their services. Thinking about the influence of big data on the financial sector and its services, the process can be highlighted as a modern upgrade to financial access. In particular, online transactions, banking applications, and internet banking produce millions of pieces of data in a single day. Therefore, managing these millions of data is a subject to important [ 46 ]. Because managing these internet financing services has major impacts on financial markets [ 57 ]. Here, Zhang et al. [ 85 ] and Xie et al. [ 79 ] focus on data volume, service variety, information protection, and predictive correctness to show the relationship between information technologies and e-commerce and finance. Big data improves the efficiency of risk-based pricing and risk management while significantly alleviating information asymmetry problems. Also, it helps to verify and collect the data, predict credit risk status, and detect fraud [ 24 , 25 , 56 ]. Jin et al. [ 44 ], [ 47 ], Peji [ 60 ], and Hajizadeh et al. [ 28 ] identified that data mining technology plays vital roles in risk managing and fraud detection.
Big data also has a significant impact on Internet credit service companies. The first impact is to be able to assess more borrowers, even those without a good financial status. Big data also plays a vital role in credit rating bureaus. For example, the two public credit bureaus in China only have 0.3 billion individual’s ‘financial records. For other people, they at most have identity and demographic information (such as ID, name, age, marriage status, and education level), and it is not plausible to obtain reliable credit risk predictions using traditional models. This situation significantly limits financial institutions from approaching new consumers [ 85 ]. In this case, big data benefits by giving the opportunity for unlimited data access. In order to deal with credit risk effectively, financial systems take advantage of transparent information mechanisms. Big data can influence the market-based credit system of both enterprises and individuals by integrating the advantages of cloud computing and information technology. Cloud computing is another motivating factor; by using this cloud computing and big data services, mobile internet technology has opened a crystal price formation process in non-internet-based traditional financial transactions. Besides providing information to both the lenders and borrowers, it creates a positive relationship between the regulatory bodies of both banking and securities sectors. If a company has a large data set from different sources, it leads to multi-dimensional variables. However, managing these big datasets is difficult; sometimes if these datasets are not managed appropriately they may even seem a burden rather than an advantage. In this sense, the concept of data mining technology described in Hajizadeh et al. [ 28 ] to manage a huge volume of data regarding financial markets can contribute to reducing these difficulties. Managing the huge sets of data, the FinTech companies can process their information reliably, efficiently, effectively, and at a comparatively lower cost than the traditional financial institutions. They can analyze and provide services to more customers at greater depth. In addition, they can benefit from the analysis and prediction of systemic financial risks [ 82 ]. However, one critical issue is that individuals or small companies may not be able to afford to access big data directly. In this case, they can take advantage of big data through different information companies such as professional consulting companies, relevant government agencies, relevant private agencies, and so forth.
Big data in managing financial services
Big data is an emerging issue in almost all areas of business. Especially in finance, it effects with a variety of facility, such as financial management, risk management, financial analysis, and managing the data of financial applications. Big data is expressively changing the business models of financial companies and financial management. Also, it is considered a fascinating area nowadays. In this fascinating area, scientists and experts are trying to propose novel finance business models by considering big data methods, particularly, methods for risk control, financial market analysis, creating new finance sentiment indexes from social networks, and setting up information-based tools in different creative ways [ 58 ]. Sun et al. [ 73 ] mentioned the 4 V features of big data. These are volume (large data scale), variety (different data formats), velocity (real-time data streaming), and veracity (data uncertainty). These characteristics comprise different challenges for management, analytics, finance, and different applications. These challenges consist of organizing and managing the financial sector in effective and efficient ways, finding novel business models and handling traditional financial issues. The traditional financial issues are defined as high-frequency trading, credit risk, sentiments, financial analysis, financial regulation, risk management, and so on [ 73 ].
Every financial company receives billions of pieces of data every day but they do not use all of them in one moment. The data helps firms analyze their risk, which is considered the most influential factor affecting their profit maximization. Cerchiello and Giudici [ 11 ] specified systemic risk modelling as one of the most important areas of financial risk management. It mainly, emphasizes the estimation of the interrelationships between financial institutions. It also helps to control both the operational and integrated risk. Choi and Lambert [ 13 ] stated that ‘Big data are becoming more important for risk analysis’. It influences risk management by enhancing the quality of models, especially using the application and behavior scorecards. It also elaborates and interprets the risk analysis information comparatively faster than traditional systems. In addition, it also helps in detecting fraud [ 25 , 56 ] by reducing manual efforts by relating internal as well as external data in issues such as money laundering, credit card fraud, and so on. It also helps in enhancing computational efficiency, handling data storage, creating a visualization toolbox, and developing a sanity-check toolbox by enabling risk analysts to make initial data checks and develop a market-risk-specific remediation plan. Campbell-verduyn et al. [ 10 ] state “Finance is a technology of control, a point illustrated by the use of financial documents, data, models and measures in management, ownership claims, planning, accountability, and resource allocation” .
Moreover, big data techniques help to measure credit banking risk in home equity loans. Every day millions of financial operations lead to growth in companies’ databases. Managing these big databases sometimes creates problems. To resolve those problems, an automatic evaluation of credit status and risk measurements is necessary within a reasonable period of time [ 62 ]. Nowadays, bankers are facing problems in measuring the risks of credit and managing their financial databases. Big data practices are applied to manage financial databases in order to segment different risk groups. Also big data is very helpful for banks to comply with both the legal and the regulatory requirements in the credit risk and integrity risk domains [ 12 ]. A large dataset always needs to be managed with big data techniques to provide faster and unbiased estimators. Financial institutions benefit from improved and accurate credit risk evaluation. This helps to reduce the risks for financial companies in predicting a client’s loan repayment ability. In this way, more and more people get access to credit loans and at the same time banks reduce their credit risks [ 62 ].
Big data and other financial issues
One of the largest data platforms is the Internet, which is clearly playing ever-increasing roles in both the financial markets and personal finance. Information from the Internet always matters. Tumarkin and Whitelaw [ 77 ] examine the relationship between Internet message board activity and abnormal stock returns and trading volume. The study found that abnormal message activity of the stock of the Internet sector changes investors’ opinions in correlation with abnormal industry-adjusted returns, as well as causing trading volume to become abnormally high, since the Internet is the most common channel for information dissemination to investors. As a result, investors are always seeking information from the Internet and other sources. This information is mostly obtained by searching on different search engines. Drake et al. [ 18 ] found that abnormal information searches on search engines increase about two weeks prior to the earnings announcement. This study also suggests that information diffusion is not instantaneous with the release of the earnings information, but rather is spread over a period surrounding the announcement. One more significant correlation identified in this study is that information demand is positively associated with media attention and news, but negatively associated with investor distraction. Dimpfl and Jank [ 17 ] specified that search queries help predict future volatility, and their volatility will exceed the information contained in the lag volatility itself, and the volatility of the search volume will have an impact on volatility, which will last a considerable period of time. Jin et al. [ 43 ] identified that micro blogging also has a significant influence on changing the information environment, which in turn influences changes in stock market behavior.
Conclusions
Big data, machine learning, AI, and the cloud computing are fueling the finance industry toward digitalization. Large companies are embracing these technologies to implement digital transformation, bolster profit and loss, and meet consumer demand. While most companies are storing new and valuable data, the question is the implication and influence of these stored data in finance industry. In this prospect, every financial service is technologically innovative and treats data as blood circulation. Therefore, the findings of this study are reasonable to conclude that big data has revolutionized finance industry mainly with the real time stock market insights by changing trade and investments, fraud detection and prevention, and accurate risk analysis by machine learning process. These services are influencing by increasing revenue and customer satisfaction, speeding up manual processes, improving path to purchase, streamlined workflow and reliable system processing, analyze financial performance, and control growth. Despite these revolutionary service transmissions, several critical issues of big data exist in the finance world. Privacy and protection of data is one the biggest critical issue of big data services. As well as data quality of data and regulatory requirements also considered as significant issues. Even though every financial products and services are fully dependent on data and producing data in every second, still the research on big data and finance hasn’t reached its peak stage. In this perspectives, the discussion of this study reasonable to settle the future research directions. In future, varied research efforts will be important for financial data management systems to address technical challenges in order to realize the promised benefits of big data; in particular, the challenges of managing large data sets should be explored by researchers and financial analysts in order to drive transformative solutions. The common problem is that the larger the industry, the larger the database; therefore, it is important to emphasize the importance of managing large data sets for large companies compared to small firms. Managing such large data sets is expensive, and in some cases very difficult to access. In most cases, individuals or small companies do not have direct access to big data. Therefore, future research may focus on the creation of smooth access for small firms to large data sets. Also, the focus should be on exploring the impact of big data on financial products and services, and financial markets. Research is also essential into the security risks of big data in financial services. In addition, there is a need to expand the formal and integrated process of implementing big data strategies in financial institutions. In particular, the impact of big data on the stock market should continue to be explored. Finally, the emerging issues of big data in finance discussed in this study should be empirically emphasized in future research.
Availability of data and materials
Our data will be available on request.
Abbreviations
Small and medium enterprise
Research & Development
Human resource
Business to Business
Big data analytics
Supply chain management
Internet of things
Production safety management
Financial Technology
Andreasen MM, Christensen JHE, Rudebusch GD. Term structure analysis with big data: one-step estimation using bond prices. J Econom. 2019;212(1):26–46. https://doi.org/10.1016/j.jeconom.2019.04.019 .
Article MathSciNet MATH Google Scholar
Aragona B, Rosa R De. Big data in policy making. Math Popul Stud. 2018;00(00):1–7. https://doi.org/10.1080/08898480.2017.1418113 .
Article Google Scholar
Baak MA, van Hensbergen S. How big data can strengthen banking risk surveillance. Compact, 15–19. https://www.compact.nl/en/articles/how-big-data-can-strengthen-banking-risk-surveillance/ (2015).
Bag S, Wood LC, Xu L, Dhamija P, Kayikci Y. Big data analytics as an operational excellence approach to enhance sustainable supply chain performance. Resour Conserv Recycl. 2020;153:104559. https://doi.org/10.1016/j.resconrec.2019.104559 .
Barr MS, Koziara B, Flood MD, Hero A, Jagadish HV. Big data in finance: highlights from the big data in finance conference hosted at the University of Michigan October 27–28, 2016. SSRN Electron J. 2018. https://doi.org/10.2139/ssrn.3131226 .
Begenau J, Farboodi M, Veldkamp L. Big data in finance and the growth of large firms. J Monet Econ. 2018;97:71–87. https://doi.org/10.1016/j.jmoneco.2018.05.013 .
Belhadi A, Zkik K, Cherrafi A, Yusof SM, El fezazi S. Understanding big data analytics for manufacturing processes: insights from literature review and multiple case studies. Comput Ind Eng. 2019;137:106099. https://doi.org/10.1016/j.cie.2019.106099 .
Blackburn M, Alexander J, Legan JD, Klabjan D. Big data and the future of R&D management: the rise of big data and big data analytics will have significant implications for R&D and innovation management in the next decade. Res Technol Manag. 2017;60(5):43–51. https://doi.org/10.1080/08956308.2017.1348135 .
Bollen J, Mao H, Zeng X. Twitter mood predicts the stock market. J Comput Sci. 2011;2(1):1–8. https://doi.org/10.1016/j.jocs.2010.12.007 .
Campbell-verduyn M, Goguen M, Porter T. Big data and algorithmic governance: the case of financial practices. New Polit Econ. 2017;22(2):1–18. https://doi.org/10.1080/13563467.2016.1216533 .
Cerchiello P, Giudici P. Big data analysis for financial risk management. J Big Data. 2016;3(1):18. https://doi.org/10.1186/s40537-016-0053-4 .
Chen M. How the financial services industry is winning with big data. https://mapr.com/blog/how-financial-services-industry-is-winning-with-big-data/ (2018).
Choi T, Lambert JH. Advances in risk analysis with big data. Risk Anal 2017; 37(8). https://doi.org/10.1111/risa.12859 .
Corporation O. Big data in financial services and banking (Oracle Enterprise Architecture White Paper, Issue February). http://www.oracle.com/us/technologies/big-data/big-data-in-financial-services-wp-2415760.pdf (2015).
Cui Y, Kara S, Chan KC. Manufacturing big data ecosystem: a systematic literature review. Robot Comput Integr Manuf. 2020;62:101861. https://doi.org/10.1016/j.rcim.2019.101861 .
Diebold FX, Ghysels E, Mykland P, Zhang L. Big data in dynamic predictive econometric modeling. J Econ. 2019;212:1–3. https://doi.org/10.1016/j.jeconom.2019.04.017 .
Dimpfl T, Jank S. Can internet search queries help to predict stock market volatility? Eur Financ Manag. 2016;22(2):171–92. https://doi.org/10.1111/eufm.12058 .
Drake MS, Roulstone DT, Thornock JR. Investor information demand: evidence from Google Searches around earnings announcements. J Account Res. 2012;50(4):1001–40. https://doi.org/10.1111/j.1475-679X.2012.00443.x .
Duan L, Xiong Y. Big data analytics and business analytics. J Manag Anal. 2015;2(1):1–21. https://doi.org/10.1080/23270012.2015.1020891 .
Dubey R, Gunasekaran A, Childe SJ, Bryde DJ, Giannakis M, Foropon C, Roubaud D, Hazen BT. Big data analytics and artificial intelligence pathway to operational performance under the effects of entrepreneurial orientation and environmental dynamism: a study of manufacturing organisations. Int J Prod Econ. 2019. https://doi.org/10.1016/j.ijpe.2019.107599 .
Einav L, Levin J. The data revolution and economic analysis. Innov Policy Econ. 2014;14(1):1–24. https://doi.org/10.1086/674019 .
Ewen J. How big data is changing the finance industry. https://www.tamoco.com/blog/big-data-finance-industry-analytics/ (2019).
Fanning K, Grant R. Big data: implications for financial managers. J Corp Account Finance. 2013. https://doi.org/10.1002/jcaf.21872 .
Glancy FH, Yadav SB. A computational model for fi nancial reporting fraud detection. Decis Support Syst. 2011;50(3):595–601. https://doi.org/10.1016/j.dss.2010.08.010 .
Gray GL, Debreceny RS. A taxonomy to guide research on the application of data mining to fraud detection in financial statement audits. Int J Account Inform Sys. 2014. https://doi.org/10.1016/j.accinf.2014.05.006 .
Grover P, Kar AK. Big data analytics: a review on theoretical contributions and tools used in literature. Global J Flex Sys Manag. 2017;18(3):203–29. https://doi.org/10.1007/s40171-017-0159-3 .
Hagenau M, Liebmann M, Neumann D. Automated news reading: stock price prediction based on financial news using context-capturing features. Decis Support Syst. 2013;55(3):685–97. https://doi.org/10.1016/j.dss.2013.02.006 .
Hajizadeh E, Ardakani HD, Shahrabi J. Application of data mining techniques in stock markets: a survey. J Econ Int Finance. 2010;2(7):109–18.
Google Scholar
Hale G, Lopez JA. Monitoring banking system connectedness with big data. J Econ. 2019;212(1):203–20. https://doi.org/10.1016/j.jeconom.2019.04.027 .
Article MATH Google Scholar
Hallikainen H, Savimäki E, Laukkanen T. Fostering B2B sales with customer big data analytics. Ind Mark Manage. 2019. https://doi.org/10.1016/j.indmarman.2019.12.005 .
Hasan MM, Mahmud A. Risks management of ready-made garments industry in Bangladesh. Int Res J Bus Stud. 2017;10(1):1–13. https://doi.org/10.21632/irjbs.10.1.1-13 .
Hasan MM, Mahmud A, Islam MS. Deadly incidents in Bangladeshi apparel industry and illustrating the causes and effects of these incidents. J Finance Account. 2017;5(5):193–9. https://doi.org/10.11648/j.jfa.20170505.13 .
Hasan MM, Nekmahmud M, Yajuan L, Patwary MA. Green business value chain: a systematic review. Sustain Prod Consum. 2019;20:326–39. https://doi.org/10.1016/J.SPC.2019.08.003 .
Hasan MM, Parven T, Khan S, Mahmud A, Yajuan L. Trends and impacts of different barriers on Bangladeshi RMG Industry’s sustainable development. Int Res J Bus Stud. 2018;11(3):245–60. https://doi.org/10.21632/irjbs.11.3.245-260 .
Hasan MM, Yajuan L, Khan S. Promoting China’s inclusive finance through digital financial services. Global Bus Rev. 2020. https://doi.org/10.1177/0972150919895348 .
Hasan MM, Yajuan L, Mahmud A. Regional development of China’s inclusive finance through financial technology. SAGE Open. 2020. https://doi.org/10.1177/2158244019901252 .
Hill C. Where big data is taking the financial industry: trends in 2018. Big data made simple. https://bigdata-madesimple.com/where-big-data-is-taking-the-financial-industry-trends-in-2018/ (2018).
Hofmann E. Big data and supply chain decisions: the impact of volume, variety and velocity properties on the bullwhip effect. Int J Prod Res. 2017;55(17):5108–26. https://doi.org/10.1080/00207543.2015.1061222 .
Holland CP, Thornton SC, Naudé P. B2B analytics in the airline market: harnessing the power of consumer big data. Ind Mark Manage. 2019. https://doi.org/10.1016/j.indmarman.2019.11.002 .
Huang L, Wu C, Wang B. Challenges, opportunities and paradigm of applying big data to production safety management: from a theoretical perspective. J Clean Prod. 2019;231:592–9. https://doi.org/10.1016/j.jclepro.2019.05.245 .
Hussain K, Prieto E. Big data in the finance and insurance sectors. In: Cavanillas JM, Curry E, Wahlster W, editors. New horizons for a data-driven economy: a roadmap for usage and exploitation of big data in Europe. SpringerOpen: Cham; 2016. p. 2019–223. https://doi.org/10.1007/978-3-319-21569-3 .
Chapter Google Scholar
Ji W, Yin S, Wang L. A big data analytics based machining optimisation approach. J Intell Manuf. 2019;30(3):1483–95. https://doi.org/10.1007/s10845-018-1440-9 .
Jin X, Shen D, Zhang W. Has microblogging changed stock market behavior? Evidence from China. Physica A. 2016;452:151–6. https://doi.org/10.1016/j.physa.2016.02.052 .
Jin M, Wang Y, Zeng Y. Application of data mining technology in financial risk. Wireless Pers Commun. 2018. https://doi.org/10.1007/s11277-018-5402-5 .
Joshi N. How big data can transform the finance industry. BBN Times. https://www.bbntimes.com/en/technology/big-data-is-transforming-the-finance-industry .
Kh R. How big data can play an essential role in Fintech Evolutionno title. Smart Dala Collective. https://www.smartdatacollective.com/fintech-big-data-play-role-financial-evolution/ (2018).
Khadjeh Nassirtoussi A, Aghabozorgi S, Ying Wah T, Ngo DCL. Text mining for market prediction: a systematic review. Expert Syst Appl. 2014;41(16):7653–70. https://doi.org/10.1016/j.eswa.2014.06.009 .
Khan F. Big data in financial services. https://medium.com/datadriveninvestor/big-data-in-financial-services-d62fd130d1f6 (2018).
Kshetri N. Big data’s role in expanding access to financial services in China. Int J Inf Manage. 2016;36(3):297–308. https://doi.org/10.1016/j.ijinfomgt.2015.11.014 .
Lamba K, Singh SP. Big data in operations and supply chain management: current trends and future perspectives. Prod Plan Control. 2017;28(11–12):877–90. https://doi.org/10.1080/09537287.2017.1336787 .
Lien D. Business Finance and Enterprise Management in the era of big data: an introduction. North Am J Econ Finance. 2017;39:143–4. https://doi.org/10.1016/j.najef.2016.10.002 .
Liu S, Shao B, Gao Y, Hu S, Li Y, Zhou W. Game theoretic approach of a novel decision policy for customers based on big data. Electron Commer Res. 2018;18(2):225–40. https://doi.org/10.1007/s10660-017-9259-6 .
Liu Y, Soroka A, Han L, Jian J, Tang M. Cloud-based big data analytics for customer insight-driven design innovation in SMEs. Int J Inf Manage. 2019. https://doi.org/10.1016/j.ijinfomgt.2019.11.002 .
Mohamed TS. How big data does impact finance. Aksaray: Aksaray University; 2019.
Mulla J, Van Vliet B. FinQL: a query language for big data in finance. SSRN Electron J. 2015. https://doi.org/10.2139/ssrn.2685769 .
Ngai EWT, Hu Y, Wong YH, Chen Y, Sun X. The application of data mining techniques in financial fraud detection: a classification framework and an academic review of literature. Decis Support Syst. 2011;50(3):559–69. https://doi.org/10.1016/j.dss.2010.08.006 .
Niu S. Prevention and supervision of internet financial risk in the context of big data. Revista de La Facultad de Ingeniería. 2017;32(11):721–6.
Oracle. (2012) Financial services data management: big Data technology in financial services (Issue June).
Pappas IO, Mikalef P, Giannakos MN, Krogstie J, Lekakos G. Big data and business analytics ecosystems: paving the way towards digital transformation and sustainable societies. IseB. 2018;16(3):479–91. https://doi.org/10.1007/s10257-018-0377-z .
Peji M. Text mining for big data analysis in financial sector: a literature review. Sustainability. 2019. https://doi.org/10.3390/su11051277 .
Pousttchi K, Hufenbach Y. Engineering the value network of the customer interface and marketing in the data-Rich retail environment. Int J Electron Commer. 2015. https://doi.org/10.2753/JEC1086-4415180401 .
Pérez-Martín A, Pérez-Torregrosa A, Vaca M. Big Data techniques to measure credit banking risk in home equity loans. J Bus Res. 2018. https://doi.org/10.1016/j.jbusres.2018.02.008 .
Rabhi L, Falih N, Afraites A, Bouikhalene B. Big data approach and its applications in various fields: review. Proc Comput Sci. 2019;155(2018):599–605. https://doi.org/10.1016/j.procs.2019.08.084 .
Raman S, Patwa N, Niranjan I, Ranjan U, Moorthy K, Mehta A. Impact of big data on supply chain management. Int J Logist Res App. 2018;21(6):579–96. https://doi.org/10.1080/13675567.2018.1459523 .
Razin E. Big buzz about big data: 5 ways big data is changing finance. Forbes. https://www.forbes.com/sites/elyrazin/2015/12/03/big-buzz-about-big-data-5-ways-big-data-is-changing-finance/#1d055654376a (2019).
Retail banks and big data: big data as the key to better risk management. In: The Economist Intelligence Unit. https://eiuperspectives.economist.com/sites/default/files/RetailBanksandBigData.pdf (2014).
Sahal R, Breslin JG, Ali MI. Big data and stream processing platforms for Industry 4.0 requirements mapping for a predictive maintenance use case. J Manuf Sys. 2020;54:138–51. https://doi.org/10.1016/j.jmsy.2019.11.004 .
Schiff A, McCaffrey M. Redesigning digital finance for big data. SSRN Electron J. 2017. https://doi.org/10.2139/ssrn.2967122 .
Shamim S, Zeng J, Shafi Choksy U, Shariq SM. Connecting big data management capabilities with employee ambidexterity in Chinese multinational enterprises through the mediation of big data value creation at the employee level. Int Bus Rev. 2019. https://doi.org/10.1016/j.ibusrev.2019.101604 .
Shen Y (n.d.). Study on internet financial risk early warning based on big data analysis. 1919–1922.
Shen D, Chen S. Big data finance and financial markets. In: Computational social sciences (pp. 235–248). https://doi.org/10.1007/978-3-319-95465-3_12235 (2018).
Shen Y, Shen M, Chen Q. Measurement of the new economy in China: big data approach. China Econ J. 2016;9(3):304–16. https://doi.org/10.1080/17538963.2016.1211384 .
Sun Y, Shi Y, Zhang Z. Finance big data: management, analysis, and applications. Int J Electron Commer. 2019;23(1):9–11. https://doi.org/10.1080/10864415.2018.1512270 .
Sun W, Zhao Y, Sun L. Big data analytics for venture capital application: towards innovation performance improvement. Int J Inf Manage. 2018. https://doi.org/10.1016/j.ijinfomgt.2018.11.017 .
Tang Y, Xiong JJ, Luo Y, Zhang Y, Tang Y. How do the global stock markets Influence one another? Evidence from finance big data and granger causality directed network. Int J Electron Commer. 2019;23(1):85–109. https://doi.org/10.1080/10864415.2018.1512283 .
Thackeray R, Neiger BL, Hanson CL, Mckenzie JF. Enhancing promotional strategies within social marketing programs: use of Web 2.0 social media. Health Promot Pract. 2008. https://doi.org/10.1177/1524839908325335 .
Tumarkin R, Whitelaw RF. News or noise? Internet postings and stock prices. Financ Anal J. 2001;57(3):41–51. https://doi.org/10.2469/faj.v57.n3.2449 .
Wright LT, Robin R, Stone M, Aravopoulou DE. Adoption of big data technology for innovation in B2B marketing. J Business-to-Business Mark. 2019;00(00):1–13. https://doi.org/10.1080/1051712X.2019.1611082 .
Xie P, Zou C, Liu H. The fundamentals of internet finance and its policy implications in China. China Econ J. 2016;9(3):240–52. https://doi.org/10.1080/17538963.2016.1210366 .
Xu L Da, Duan L. Big data for cyber physical systems in industry 4.0: a survey. Enterp Inf Syst. 2019;13(2):148–69. https://doi.org/10.1080/17517575.2018.1442934 .
Article MathSciNet Google Scholar
Yadegaridehkordi E, Nilashi M, Shuib L, Nasir MH, Asadi M, Samad S, Awang NF. The impact of big data on firm performance in hotel industry. Electron Commer Res Appl. 2020;40:100921. https://doi.org/10.1016/j.elerap.2019.100921 .
Yang D, Chen P, Shi F, Wen C. Internet finance: its uncertain legal foundations and the role of big data in its development. Emerg Mark Finance Trade. 2017. https://doi.org/10.1080/1540496X.2016.1278528 .
Yu S, Guo S. Big data in finance. Big data concepts, theories, and application. Cham: Springer International Publishing; 2016. p. 391–412. https://doi.org/10.1007/978-3-319-27763-9 .
Yu ZH, Zhao CL, Guo SX(2017). Research on enterprise credit system under the background of big data. In: 3rd International conference on education and social development (ICESD 2017), ICESD, 903–906. https://doi.org/10.2991/wrarm-17.2017.77 .
Zhang S, Xiong W, Ni W, Li X. Value of big data to finance: observations on an internet credit Service Company in China. Financial Innov. 2015. https://doi.org/10.1186/s40854-015-0017-2 .
Zhao JL, Fan S, Hu D. Business challenges and research directions of management analytics in the big data era. J Manag Anal. 2014;1(3):169–74. https://doi.org/10.1080/23270012.2014.968643 .
Download references
Acknowledgements
All the authors are acknowledged to the reviewers who made significant comments on the review stage.
The project is funded under the program of the Minister of Science and Higher Education titled “Regional Initiative of Excellence in 2019-2022, project number 018/RID/2018/19, the amount of funding PLN 10 788 423 16”.
Author information
Authors and affiliations.
School of Finance, Nanjing Audit University, Nanjing, 211815, China
Md. Morshadul Hasan
WSB University, Cieplaka 1c, 41-300, Dabrowa Górnicza, Poland
József Popp & Judit Oláh
You can also search for this author in PubMed Google Scholar
Contributions
All the authors have the equal contribution on this paper. All authors read and approved the final manuscript.
Corresponding author
Correspondence to József Popp .
Ethics declarations
Competing interests.
There is no competing interests.
Additional information
Publisher's note.
Springer Nature remains neutral with regard to jurisdictional claims in published maps and institutional affiliations.
Rights and permissions
Open Access This article is licensed under a Creative Commons Attribution 4.0 International License, which permits use, sharing, adaptation, distribution and reproduction in any medium or format, as long as you give appropriate credit to the original author(s) and the source, provide a link to the Creative Commons licence, and indicate if changes were made. The images or other third party material in this article are included in the article's Creative Commons licence, unless indicated otherwise in a credit line to the material. If material is not included in the article's Creative Commons licence and your intended use is not permitted by statutory regulation or exceeds the permitted use, you will need to obtain permission directly from the copyright holder. To view a copy of this licence, visit http://creativecommons.org/licenses/by/4.0/ .
Reprints and permissions
About this article
Cite this article.
Hasan, M.M., Popp, J. & Oláh, J. Current landscape and influence of big data on finance. J Big Data 7 , 21 (2020). https://doi.org/10.1186/s40537-020-00291-z
Download citation
Received : 31 August 2019
Accepted : 17 February 2020
Published : 12 March 2020
DOI : https://doi.org/10.1186/s40537-020-00291-z
Share this article
Anyone you share the following link with will be able to read this content:
Sorry, a shareable link is not currently available for this article.
Provided by the Springer Nature SharedIt content-sharing initiative
- Big data finance
- Big data in financial services
- Big data in risk management
- Data management
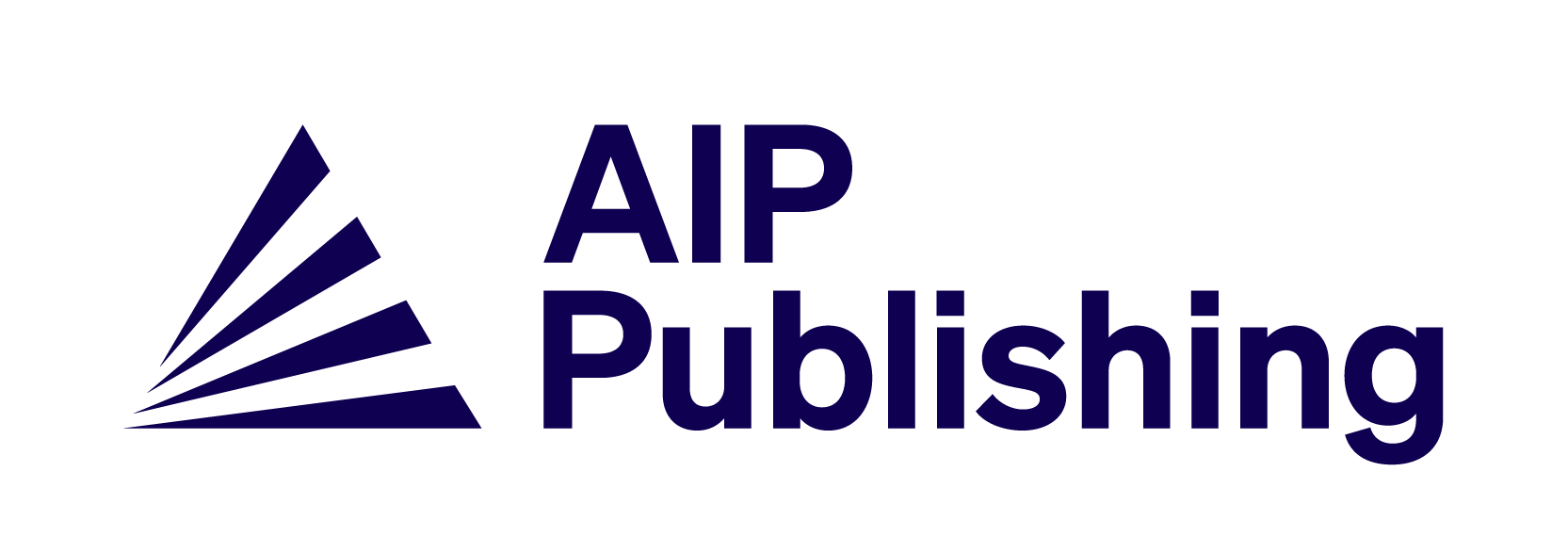
Big data in finance: A systematic literature review
- Article contents
- Figures & tables
- Supplementary Data
- Peer Review
- Reprints and Permissions
- Cite Icon Cite
- Search Site
Swati Sharma; Big data in finance: A systematic literature review. AIP Conf. Proc. 28 November 2023; 2909 (1): 030010. https://doi.org/10.1063/5.0182378
Download citation file:
- Ris (Zotero)
- Reference Manager
Big data is not a new term in finance; hence many research studies have explored different dimensions of big data in finance. In view of plethora of such studies, no single study sum-up the entire “Big Data in finance” universe. Therefore, the present study is an attempt to bring the gist of all those studies to understand the microstructure of big data in finance. The study does a systematic review of existing literature on the topic. Year-wise, Author-wise, Citation-wise, Affiliation-wise, Keywords-wise and Source-wise listing of literature are the parameter to conduct present study. Bibliometric method on Scopus database is employed. This study provides insights on trends and future scope of big data in finance. As a result, sub-sets of big data in fiancé are identified namely artificial intelligence, credit-rating, financial reporting financial crisis, stock trading, assets-pricing, portfolio optimization, banking & insurance and auditing. All these sub-set are insighted with influence of big data. The study also suggests that field like cryptocurrency, green finance, sustainability, financial accessibility is the future of big data in finance.
Sign in via your Institution
Citing articles via, publish with us - request a quote.
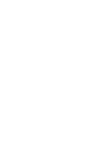
Sign up for alerts
- Online ISSN 1551-7616
- Print ISSN 0094-243X
- For Researchers
- For Librarians
- For Advertisers
- Our Publishing Partners
- Physics Today
- Conference Proceedings
- Special Topics
pubs.aip.org
- Privacy Policy
- Terms of Use
Connect with AIP Publishing
This feature is available to subscribers only.
Sign In or Create an Account
FOR EMPLOYERS
Big data in finance: benefits, use cases, & examples.
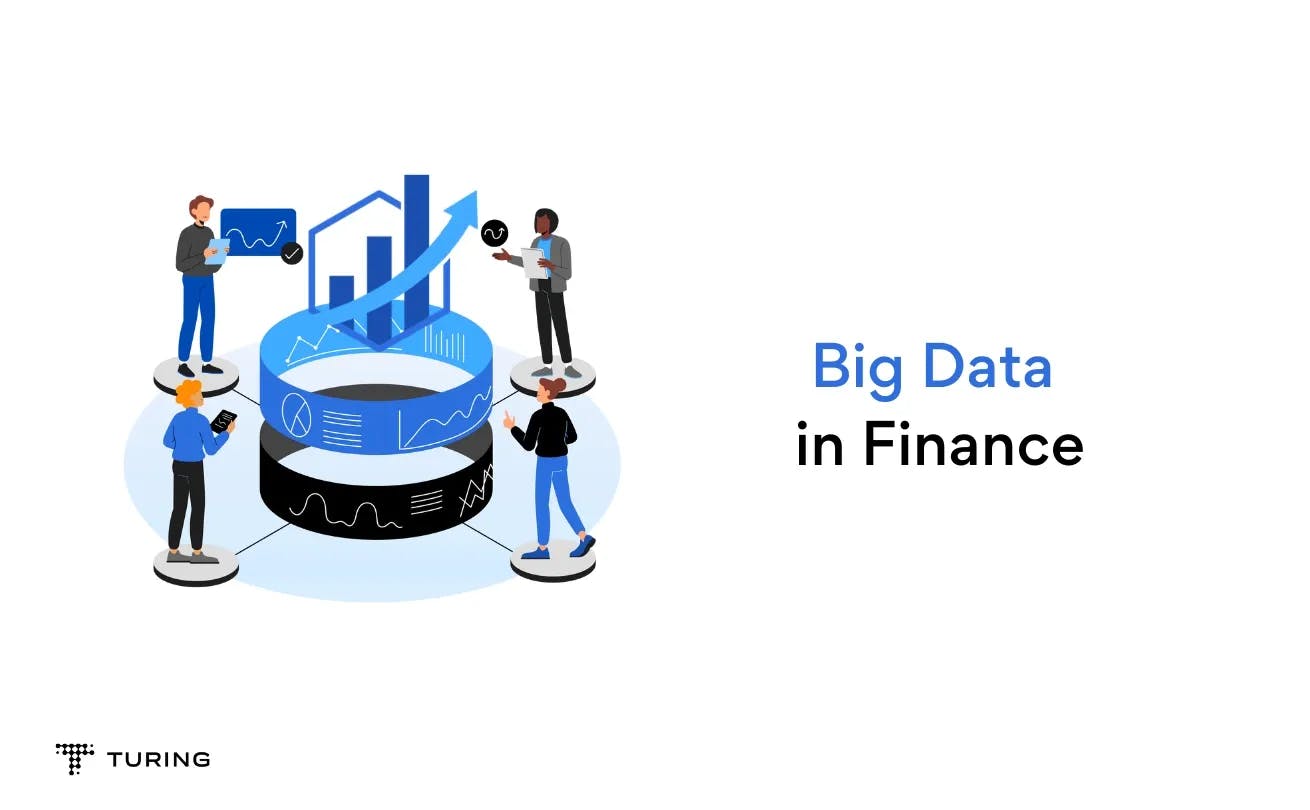
Subhasish Dutta
Subhasish is a science graduate but a passionate writer, and wordsmith who writes website content, blogs, articles, and social media content on technologies, equity market, traveling, and other domains. He has worked with Affnosys and FTI Technologies as a content writer.
Frequently Asked Questions
Big data plays a critical role in the banking sector by helping them make data-driven decisions, improve operational efficiency, manage risk more efficiently, and enhance customer experiences. Banks can also use the large dataset to assess loan applicants' creditworthiness, analyze market trends, and detect fraud.
Yes, big data plays a critical role in the FinTech industry. FinTech companies leverage big data technology to analyze customer behavior, develop innovative and personalized products and services, and improve their operations.
Big data empowers accounting and finance professionals with the necessary tools and insights to thrive in a data-driven world. Be it risk management, cost reduction, or automating routine financial tasks, big data in finance allows financial analysts to gain deeper insights into a company's financial performance and make informed decisions.
Big data has a significant impact on finance and the growth of large companies by helping them analyze large volumes of data to gain valuable insights into customer behavior, market trends, and risk factors and identify areas of improvement. This can help in reducing costs, improving revenues and profits, enhancing customer experiences, and overall business growth.
The "V's" of big data in finance are the fundamentals of big data in finance. The 4 main V's are:
Volume: Financial institutions generate massive volumes of data daily, including transaction records, customer information, market data, and more. Managing and processing this large data volume is a fundamental challenge.
Velocity: Data is constantly generated in the financial industry since it operates in real time. For example, customer transactions, high-frequency trading, algorithmic trading, and news feeds generate data at a rapid pace.
Variety: The finance industry generates data from multiple resources and the data comes in different formats. The data can be structured (coming from databases) or unstructured (coming from social media, and news articles).
Veracity: Veracity relates to the accuracy and reliability of data. Inaccurate or incomplete data can lead to inaccurate analysis and wrong decisions making.
Big data analytics has significantly transformed the financial sector in several ways including improved risk assessment, fraud detection, personalized services, regulatory compliance, market insights, new product development, and optimizing operational efficiencies.
Hire remote developers
Tell us the skills you need and we'll find the best developer for you in days, not weeks.
Influence of Big Data on Financial Accounting
- Research Note
- Published: 05 May 2018
- Volume 24 , pages 205–206, ( 2018 )
Cite this article
- Josef Horák ORCID: orcid.org/0000-0002-6672-850X 1 &
- Jiřina Bokšová 1
2058 Accesses
2 Citations
Explore all metrics
This is a preview of subscription content, log in via an institution to check access.
Access this article
Price includes VAT (Russian Federation)
Instant access to the full article PDF.
Rent this article via DeepDyve
Institutional subscriptions
Author information
Authors and affiliations.
Škoda Auto University, Na Karmeli 1457, 293 01, Mladá Boleslav, Czech Republic
Josef Horák & Jiřina Bokšová
You can also search for this author in PubMed Google Scholar
Corresponding author
Correspondence to Josef Horák .
Rights and permissions
Reprints and permissions
About this article
Horák, J., Bokšová, J. Influence of Big Data on Financial Accounting. Int Adv Econ Res 24 , 205–206 (2018). https://doi.org/10.1007/s11294-018-9681-0
Download citation
Published : 05 May 2018
Issue Date : May 2018
DOI : https://doi.org/10.1007/s11294-018-9681-0
Share this article
Anyone you share the following link with will be able to read this content:
Sorry, a shareable link is not currently available for this article.
Provided by the Springer Nature SharedIt content-sharing initiative
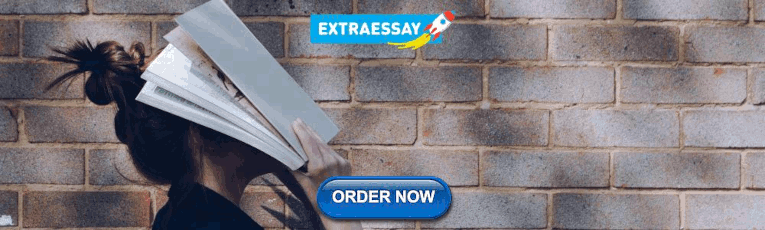
JEL Classification
- Find a journal
- Publish with us
- Track your research
To read this content please select one of the options below:
Please note you do not have access to teaching notes, big data analytics and financial reporting quality: qualitative evidence from canada.
Journal of Financial Reporting and Accounting
ISSN : 1985-2517
Article publication date: 8 July 2022
Issue publication date: 21 March 2023
Big Data analytics (BDA) and its implications for the accounting profession continue to be a key issue that requires more research and evaluation. As a result, the purpose of this study is to evaluate the impact of BDA on financial reporting quality, as well as to assess the accounting challenges associated with Big Data. It provides qualitative evidence from Canada.
Design/methodology/approach
This study used a qualitative approach to ascertain the thoughts and perceptions of auditors, financial analysts and accountants at Canadian audit and accounting firms in BDA and its impact on financial reporting quality, using semi-structured interviews. To obtain their consent to participate in the interview, 127 auditors, financial analysts and accountants from Canadian audit and accounting firms were initially approached. The final number of respondents was 41, representing a response rate of 32%.
The authors’ findings underscored the relevance of Big Data and BDA in affecting financial report quality and revealed that BDA had a significant effect on improving financial reporting quality. Big Data improves accounting reporting and expert judgment by providing professional. In summary, participants agreed that when analytical methods in Big Data are implemented effectively, businesses may possibly achieve a variety of benefits, including customized goods, simplified processes, improved risk assessment process and, finally, increased risk management.
Practical implications
The authors’ findings indicate that BDA may help predict investment returns and risks, estimate future investment opportunities, forecast revenues, detect fraud and susceptibility early and identify economic growth opportunities. As a result, auditors, financial analysts, accountants, investors and other strategic decision-makers should be aware of these findings to make informed choices.
Originality/value
Big Data has become the norm in recent years; accountants and other decision-makers have struggled to analyze massive amounts of data. This limits their capacity to profit from such data even more. Therefore, this study is motivated by the lack of research on Big Data’s influence on financial report quality.
- Big Data analytics
- Accounting reporting quality
- Qualitative evidence
- Canadian evidence
Saleh, I. , Marei, Y. , Ayoush, M. and Abu Afifa, M.M. (2023), "Big Data analytics and financial reporting quality: qualitative evidence from Canada", Journal of Financial Reporting and Accounting , Vol. 21 No. 1, pp. 83-104. https://doi.org/10.1108/JFRA-12-2021-0489
Emerald Publishing Limited
Copyright © 2022, Emerald Publishing Limited
Related articles
We’re listening — tell us what you think, something didn’t work….
Report bugs here
All feedback is valuable
Please share your general feedback
Join us on our journey
Platform update page.
Visit emeraldpublishing.com/platformupdate to discover the latest news and updates
Questions & More Information
Answers to the most commonly asked questions here
- Architecture and Design
- Asian and Pacific Studies
- Business and Economics
- Classical and Ancient Near Eastern Studies
- Computer Sciences
- Cultural Studies
- Engineering
- General Interest
- Geosciences
- Industrial Chemistry
- Islamic and Middle Eastern Studies
- Jewish Studies
- Library and Information Science, Book Studies
- Life Sciences
- Linguistics and Semiotics
- Literary Studies
- Materials Sciences
- Mathematics
- Social Sciences
- Sports and Recreation
- Theology and Religion
- Publish your article
- The role of authors
- Promoting your article
- Abstracting & indexing
- Publishing Ethics
- Why publish with De Gruyter
- How to publish with De Gruyter
- Our book series
- Our subject areas
- Your digital product at De Gruyter
- Contribute to our reference works
- Product information
- Tools & resources
- Product Information
- Promotional Materials
- Orders and Inquiries
- FAQ for Library Suppliers and Book Sellers
- Repository Policy
- Free access policy
- Open Access agreements
- Database portals
- For Authors
- Customer service
- People + Culture
- Journal Management
- How to join us
- Working at De Gruyter
- Mission & Vision
- De Gruyter Foundation
- De Gruyter Ebound
- Our Responsibility
- Partner publishers

Your purchase has been completed. Your documents are now available to view.
Review on Financial Innovations in Big Data Era
The rise of Big Data brings the financial innovation opportunities as well as challenges. This paper reviews different fields of big-data-based financial innovations as well as the scientific discoveries and theoretical breakthroughs of risk analysis with respect to these financial innovations. Based on the current research status, several key problems are put forward and their relative solutions are discussed. The three mean aspects are listed as the pricing and risk measuring for data-driven financial innovation products or services; the changes that data-driven financial innovation would bring to finance industry, which involve operation, resources allocation and ecosystem; and questions and solutions of systemic risk management based on Big Data analytics. Finally, predictions towards the hotspots frontier and developing trends for further data-driven financial innovation are proposed.
1 Introduction
With the advantage of Big Data era, data has become another crucial driving force for financial market to innovate following the existing elements: Cost, regulation and technique. The Interment and social media are now available to record the spread of information, online trading data, personal identity and behaviours, which can hardly be obtained before. Inherent logic and cross-correlation could be inferred among different dimensions of data through analysis and integration of “massive multidimensional data”, which inconvenient for financial institutions to realize and understand the personal characteristics and behaviors of the participants in financial market, thus booming a series of financial products and services innovation.
Along with a lot of opportunities, big data driving force also brings the finance industry some difficulties and challenges. Big Data Prospect Report from McKinsey Global Institute see real innovative potential in finance industry among all, but also notices its difficulties to form a data driven innovation ideas, due to the research of pricing and risk management of “data-driven” financial innovations is still in its preliminary stage, and the relevant theories need to be further refined and justified. At present, the research of financial innovation, asset pricing and risk management based on financial big data is just unfolding, and now look at the overall situation of financial system instead of merely the stock market in previous studies. It is far from being a unified framework, despite some important scientific discoveries and theoretical breakthroughs. Edelman [ 1 ] and Varian [ 2 ] published Using Internet data for economic research and Big Data: New tricks for econometrics in Journal of Economic Perspectives , establishing the formation of research paradigm in this field. Certain important scientific discoveries and theoretical breakthroughs are found about financial innovation and risk analysis theory based on big data, and can be divided into three directions — financial innovation of online P2P lending, the behavior evolution of securities market participants, systemic risk in the network perspective and its impact to financial institutions.
This paper first reviews important scientific discoveries and theoretical breakthroughs of financial innovation and risk analysis theory based on Big Data in above three directions, then extracts key problems worth exploring at the production, industry and system level based on the research status and development trends at domestic and abroad; meanwhile, discusses the methodology and significance of solving the problems above. Finally, taking important achievement and practical into account to predict possible hotspots frontier and developing trends of this field in the future.
2 Study on the Financial Innovations of Online P2P Lending Platform
Online P2P lending platform is an important financial innovation driven by big data, which replaces the traditional form of lending channel from banks and other financial institutions and bridges the demand-side and the supply-side of money through information technology. Since it emerged, this financial innovation has drawn continuous attention of academia. According to the research perspective, the relevant achievements can be divided into four categories as follows:
2.1 Study on the Decision Preference of Investors of Online P2P Lending Platform
Previous studies about online P2P lending platform were meanly focus on the decision-making strategies of investors. Paravisini, et al. [ 3 ] estimated investors’ risk preference by studying their portfolio choices in online P2P lending platform; Zhang [ 4 ] studied from perspectives of informational social impact and herding behavior to explain how individual investors’ participation of online financial community influenced their credit risk preference in online P2P lending marketplaces under different financial situations. The research concluded that online financial participants preferred to higher risk than non-participants in period without the threat of financial crisis, while, they were more risk averse than non-participants during financial crisis period. Li, et al. [ 5 ] studied the heterogeneous decision-making behavior of borrowers in online P2P lending platform. Krumme, et al. [ 6 ] studied the dynamic behaviors of online P2P lending. Yum, et al. [ 7 ] examined how individual investors form their decisions among the group information in the online P2P lending. Ceyhan, et al. [ 8 ] studied the dynamics of bidding behavior in the online P2P loan auction. They observed herding behavior and then built a model to explain this phenomenon. Krumme, et al. [ 6 ] and Li, et al. [ 9 ] studied the group behaviors of online P2P lending markets participants with the effects of social interactions and multidimensional friendship networks. Herzenstein, et al. [ 10 ] studied herding behavior in P2P loan auctions. Their paper indicated every 1% increase in the number of bids would boost the likelihood of follow-up bid by 15%, unless the target amount has been achieved, herding diminished (a 1% increase in bids increases the likelihood of an additional bid by only 5%). They also found a positive association between herding in the loan auction and its subsequent performance concluded that herding strategy in P2P loan auctions benefits bidders. Lee and Lee [ 11 ] , Luo and Lin [ 12 ] discovered similar fact. The largest online P2P lending platform in South Korea is Popfunding.com, which enables lenders to vote on the trustworthiness of borrowers who make loan requests. Yum, et al. [ 7 ] explored how the collective wisdom works for lending decisions by studied the case of Popfunding.com. They showed that the voting mechanism in Popfunding.com is a more efficient information conduit to improve efficiency when the information censorship was defective. However, facing sufficient verifiable information, lenders turned to rely on their own reasoning and ignore the collective opinion of the market. Li, et al. [ 13 ] studied the factors that affect project success rate of borrowing in Chinese P2P micro credit lending.
2.2 Study on Determination of the Interest Rate of the Online P2P Lending Plat Form
Since Peer-to-peer (P2P) lending is an innovative financial intermediary, it does matter to determine its interest rates. Chen et al. [ 14 ] applied the mathematical modeling approach to find that neither the complete information environment nor the incomplete information environment, the auction model proposed by Prosper can offer borrowers the lowest interest rate. For Prosper, who charges fees according to the volume of lending, this transaction method brings the largest volume of lending. In the study of the whole transaction recognition, Redmond, et al. [ 15 ] tried to using the data of Prosper’s customers who made transactions of both borrowing and lending to distinguish whether their behavior indicated arbitrage or money-laundry. Based on individual cash flows network model, they showed that there existed some customers who try to arbitrage by the spread, however, their behaviors did not always indicate significantly positive profits due to the possibility of defaults. Burtch, et al. [ 16 ] carried out similar research. They established capital flows of loans among nations by collecting data from Kiva.org, and their findings suggested that the cultural differences and regional differences would affect the chance of successful lending. However, when taking the collateral regime of the third party into account, the influences of these differences on successful borrowing and lending would decline. Lin, et al. [ 17 ] analyzed the data between 2007 and 2008 of the Prosper with social network function to study the effects of the circle of friends in the online P2P lending market with asymmetric information. Their findings indicated that, usually, borrowers with creditworthy friends were more likely to raise money, and with a lower interest rate. When tracing back this phenomenon’s reason, they found that these kinds of borrowers had lower default rate. Researches about how borrowers’ characteristics would influence their borrowing behavior also focusing on their credit grade, debt-to-income ratio, FICO score, revolving line utilization (see Emekter, et al. [ 18 ] ), unaudited personal financial disclosure (see Michels [ 19 ] ), gender (see Barasinska and Schafer [ 20 ] ), appearance (see Ravina [ 21 ] ) and the description of the borrowing reasons (see Larrimore, et al. [ 22 ] and Herzenstein, et al. [ 23 ] ). In general, in the online P2P lending market with asymmetric information, both the borrowers’ individual financial properties (credit grade, previous borrowing behaviors, financial situation etc). And unquantifiable functions such as the description of the borrowing reasons and other properties of the borrowers would affect the successful rate of loan and interest rate.
2.3 Study on Product Pricing and Return of the Online P2P Lending Platform
Based on the analysis of the online P2P lending behavior, scholars had done further studies on the aspects of the product pricing and the return. For instance, Lin, et al. [ 17 ] studied the friendship in the online P2P lending pattern. Herzenstein, et al. [ 23 ] investigated the unverified information’s influence on long-term debts’ performance. Luo and Lin [ 12 ] applied decision tree model to study the herding behavior in the online P2P lending, their findings showed that the investors’ gains would significantly decline due to the herding behavior. Michels [ 19 ] used the online P2P lending data to investigate the unverifiable disclosures’ effects on the behavior of borrowing and lending, the results showed that the additional unverifiable disclosure was associated with a 1.27% reduction in interest rate and an 8%increase in bidding activity. Ashta and Assadi [ 24 ] did the research on the perspective of social networking tools to detect whether efficient information dissemination could lower the trading cost. Meanwhile, some scholars shed more light on the auction in online P2P lending communities from the perspective of traditional economic theories. For example, the research on the bidding process of online auctions in P2P lending communities (see Herzenstein, et al. [ 25 ] ), and the research on the bilateral market pricing strategy in online P2P lending market (see Qiu, et al. [ 26 ] ).
2.4 Study on Credit Risk of the Online P2P Lending Platform
Although there are numerous advantages of online P2Plending mode, its risks are also obvious, including financial fraud, identity theft, and money-laundry, which would also appear in the traditional lending and these risks would be boosted by the Internet. Berger and Gleisner [ 27 ] studied on the data based on more than 14000 loans and found that online financial intermediation significantly improved the credit conditions of the borrowers. Iyer, et al. [ 28 ] , Iyer, et al. [ 29 ] investigated how the lenders judge the credit grade of the borrowers in online P2P loans. Wang and Lin [ 30 ] did research on the dynamic credit risk management of online P2P loans, and they pointed out that ordinary personal credit rating method such as FICO score cannot adapt to the dynamic credit risk management elastically. Emekter, et al. [ 18 ] also investigated the individual credit risk in online P2P lending. Moreover, Freedman and Jin [ 31 ] studied the asymmetric information in online P2P loans, they discussed in this pattern, how the social network decrease the risks brought by the asymmetric information; Lin [ 32 ] took this aspect into consideration as well. Weiss, et al. [ 33 ] did empirical research on how online P2P lending mode reduces the risk of adverse selection from the aspects of market level. Puro, et al. [ 34 ] and Zhao, et al. [ 35 ] investigated how to recommend appropriate products to the customers in online P2P lending market from the perspective of individual portfolio risk management, including suggestions on initial interest rate and loan amount. Luo, et al. [ 36 ] built a model to help investors to evaluate the potential benefits and risks of investment correctly in online P2P lending, thus helping them with the optimal investment decision. Riggins and Weber [ 37 ] considered the recognition bias in the online P2P lending and proposed an analytic model.
Existing research of online P2P platform mainly focused on single platform. The research on: Cross-platform credit risk analysis and industry risk contagion; profound analysis for the financial fraud, bankruptcy in China P2P industry; relationship between the market participants’ online information searching behavior and the financing capacity of the P2P platform, requires further research focus.
3 Study on Behavior Evolution in Social Media of Stock Market Participants
In the era of big data, information creation, interaction and transformation becomes different in social media as before. All kinds of information obtained from social media provided possibility to research for relationship between information and investor behaviors. This section classified the existing research into three catalogs according to behavior evolution in social media of stock market participants.
3.1 Study on Information Release Behavior of Participant in Social Media
When it comes to the behavior of releasing information in the social media, the academia has two distinct points of view: Noise and information. The debates can be dated back to Batsell [ 38 ] , Bennett [ 39 ] , Goldstein [ 40 ] , Harmon [ 41 ] , Maremount [ 42 ] and Medill [ 43 ] over “implicating content between investing facts and fiction which comes from electronic message boards” in Seattle Times, Dow Jones News Service, Dallas Morning News, New York Times, Wall Street Journal and Chicago Daily Herald .
Wysocki [ 44 ] started academic study in this field, using a sample of over 3,000 stocks listed on Yahoo! electronic message boards, the study analyzed relationship between the number of posts and changes of stock price movement. The study showed that cumulative posting volume is highest for firms with extreme past returns and accounting performance on average, high market capitalization, high price-earnings and market-to-book ratios, high volatility and trading volume, high analyst following and low institutional holdings. And overnight message-posting volume was founded to predict changes in the next day stock trading volume and returns; indirectly proving posting behavior on electronic message boards was related to underlying firm characteristics, not noise. The tricky thing is, Tumarkin and Whitelaw [ 45 ] captured nearly 200,000 messages from Raging Bull during April 1999 to February 2000 and found a statistically insignificant relationship between posting behaviors on electronic message boards and excess returns, which also proved from another side that market was efficient, and contents on electronic message boards were noise. Afterwards, the conclusion of researches did by Browen, et al. [ 46 ] , Clarkson, et al. [ 47 ] and Dewally [ 48 ] on electronic message boards also supported implicating contents as finance information. With the development of social media, economists gradually focused on information content in social media other than electronic message boards, such as spam(Bohme and Holz [ 49 ] , Hanke and Hauser [ 50 ] ), blog (Hu, et al. [ 51 ] and Saxton [ 52 ] ), searching engine (Mondria, et al. [ 53 ] , Zhang, et al. [ 54 ] ). For instance, Hank and Hauser regarded unsolicited e-mails as spam. They investigated the effects of stock spam e-mails on excess returns, turnover, and intra-day price range. The research proved that private information in spam could influence stock price; Hu, et al. [ 51 ] investigated the relationship of the blog visibility of SP 500 firms in Blog pulse and its capital market valuation. They found a positive association between a firm’s blog visibility and its capital market valuation. Moreover, the visibility of firm’s blog would influence its stock trading. Zhang, et al. [ 54 ] obtained information content indicator of individual stocks in Baidu search engine with search-engine-based data mining algorithm, and found that this indicator could explain abnormal returns in stock. The empirical test proved that information content in search engines was not noise.
3.2 Study on Building Proxy Based on Information Content from Participant
This section of study regards content of information released in the social media from stock market participant as the premise, further research on text content classification, key words extraction, indicators of the whole market based on data mining algorithm and nature language processing tools had been done to study the relationship between these indicators and asset pricing.
Antweiler and Frank [ 55 ] obtained data that contained 1.5 million messages of 45 listed companies, which were ranked in the Dow Jones Internet index (Dow Jones Internet Index) and the Dow Jones industrial average (the Dow Jones Industrial Average) on Yahoo! Finance and Raging Bull. They built a Bullishness indicator by using the Naive Bayes text Classification methods, and found that this indicator could predict the volatility of market, which was “statistically significant but small economically correlated (statistically significant but economically small)” between the indicator and returns. Das and Chen [ 56 ] got posts on Yahoo! Finance message boards of 24 high-tech companies which were ranked in the Morgan Stanley High-Tech Index (MSH), using voting scheme method to extract indicator of investor sentiment, and discovered the significant correlation between the indicator and trading volume as well as stock volatility. Zhang, et al. [ 57 ] used a variety of text classifier models to build the Sentiment Indexes of individual investors from posts on message board of Thelion! Wall Street Pit, and proved this sentiment index to be a significant directional indicator of “same-day positive but next-day negative”.
Meanwhile, Bollen, et al. [ 58 ] extracted the indicator characterizing public sentiment from Twitter by using software Opinion Finder and GPOMS, which considered to be significantly improving the prediction direction of Dow Jones industrial average (DJIA). Similar studies of constructing such indicator based on text content in social media also carried out by Felton and Kim [ 59 ] , Gu, et al. [ 60 ] , De Choudhury, et al. [ 61 ] etc.
3.3 Study on Financial Theory of Structuring Proxy Variables Based on Big Data
Based on the research mentioned before, scholars are not satisfied with discovering interesting phenomenon from information extracted from the social media, but rather prefer to construct new proxy variables to verify existing finance theories or reasonable scientific hypothesis.
Da, et al. [ 62 ] creatively applied search volume index of stock codes from Google Trends as the proxy variables of investor attention (Merton [ 63 ] ; Sims [ 64 ] ; Hirshleifer and Teoh [ 65 ] ; Grullon, et al. [ 66 ] ; Chemmanur and Yan [ 67 ] ; Chan [ 68 ] ; Fang and Peress [ 69 ] ; Barber and Odean [ 70 ] ; Seasholes and Wu [ 71 ] ). Plenty of studies showed the increasing of investor attention could forecast the rise of stock price two weeks later, and the prices would reverse within a year, which could explain the phenomenon of first day premium and long-run underperformance in IPOs. Bank, et al. [ 72 ] also adopted daily data of search volume index from Google Insights to build proxy variables of investor attention. Their study indicated the increasing of investor attention would bring short-term positive return and promote market liquidity, and the enhancement of market liquidity was attributed to the reduction of market information asymmetry by investors’ searching behavior. Vlastakis and Markellos [ 73 ] adopted Google Trends search volume index as proxy variable of information demands. Their study showed that in consideration of market return and information supply, a positive relationship between information demands and historical and implied volatility was caught, and a higher demand of information was required under the high return. Drake, et al. [ 74 ] also used Google Trends search volume index as a proxy for investor information needs, and found that the information demand from investors began to increase two weeks before the publish of earnings announcement, peaking at the exact day of announcement publishing, and could last a period of time. Thus proved market information is non-instant transmission, which verified the positive relationship between information demand and media attention. Dzielinski [ 75 ] applied Google Trends search volume index as a proxy variable of economic uncertainty, and found economic uncertainty usually associated with stock market overall yield and volatility. Yu and Zhang [ 76 ] , Zhang, et al. [ 77 ] studied the relationship between Chinese investor attention and stock market by introducing Baidu Index, which similarly discovered that investor attention would cause abnormal stock returns. Liu, et al. [ 78 ] applied Baidu Index as proxy variable of media attention and investor attention, and found the changes of investor attention due to media information dissemination were the direct cause of abnormal stock returns. In addition, Zhang, et al. [ 79 ] defined Baidu News as proxy variable of Internet information arrival (Lamoureux and Lastrapes [ 80 ] , Kalev, et al. [ 81 ] , Wagner and Marsh [ 82 ] , Fleming, et al. [ 83 ] and McMillan and Garcia [ 84 ] ), combining with SMEs board Index to study Mixture Distribution Hypothesis. This empirical study indicated Internet information arrival could better explain volatility persistence in stock market compared to other variables.
At present, researches on the behavior evolution of stock market participants in social media mainly focused on the relationship between the capital market and information contained in a single social media. The impact of cross-correlation of multiple data sources on stock market and behavior evolution of other market participants in the financial system (e.g., Banking and Insurance) still required further studies.
4 Systemic Risk and Financial Institutions: A Network Perspective
Banking industry is a critical part of the financial market, which directly linked to safety of the financial system. After the financial crisis of 2008, the academic research turned to focus on the risk contagion in the banking system, which is the improper solution to a single bank crisis may lead to the falling of the whole banking system and the whole financial market. Existing researches on risk contagion and systemic risk study mainly focused on the following field:
4.1 Theoretical Studies Based on Social Media of Information Diffusion Network in Financial Market
This kind of research mainly focuses on the equilibrium between fraud information sender and receiver, and how does this equilibrium affect asset pricing. The cheap-talk model proposed by Crawford and Sobel [ 85 ] is the most widely used framework. In this framework, it is assumed that the sender has private information that could affect the firm’s future cash flows; however, they choose different releasing strategies (release true or false information) to affect their receivers in order to maximize their own profit. On the other hand, the receivers will learn from the sender’s past behavior and try to distinguish whether the information is true or false, in order to make best use of secondhand information to maximize their profit. Existing literature mainly focused on single information source and multi information source. The multi-information sources research is based on the characteristics of information diffusion network due to multi information source in social media, to theoretically explain the influence of information source fraud behavior on asset pricing.
On the single information source perspective, Benabou and Laroque [ 86 ] applied this framework to the information diffusion process in the stock market, presenting the equilibrium of single sender and receiver in the market to analyze the welfare loss in the condition of information manipulation. This result provided good explanation to certain kinds of information manipulation behavior in the market. Several scholars extended their study with individual behavior: Crawford [ 87 ] and Chen [ 88 ] studied on information diffusion equilibrium and the change of market pricing efficiency considering receivers’ naive bias; Bommel [ 89 ] and Garcia and Sangiorgi [ 90 ] argued that senders might intentionally release rumors without solid information and built an equilibrium of senders which only spreading rumors; Liu [ 91 ] extend the model with the limitations information cost and information time horizon.
As information in the social media are cross linked with multi-sources, study on the multi information source is the extension of single one; meanwhile, fraud information releasing could simulate information manipulation in the social media. Klumpp [ 92 ] considered the equilibrium of M information sources as information sender to deliver message to N (larger than M ) receiver, and this model is supported by Becker and Milbourn [ 93 ] in studying American credit rating market. This study first introduces information diffusion into Cheap-Talk model and found the similar equilibrium in oligopoly market that is an important innovation and development. Hong, et al. [ 94 ] developed a contagion model of rumor diffusion and found out that the diffusion rate and return of assets are strongly related. Besides, Feng, et al. [ 95 ] built a rumor diffusion network based on agent-based computational model and found asset pricing and volatility are influenced by individual’s network, diffusion rate and information source characteristics.
4.2 On the Financial Crisis Contagion with Network Approach
This type of study mainly focused on the controversial problem of risk analysis and prediction on risk contagion, and tried to explain from the perspective of financial network. Profound study on financial network and structure may provide better explanations on dynamics of the financial system and further strengthen the whole system and offer effective recommendations to the authority (Schweitzer, et al. [ 96 ] ). Lux [ 97 ] argued that the network theory is critical to the study on economic system.
Allen and Gale [ 98 ] started to research on the financial structure with direct-linking method. They figured out that completeness of inter-bank direct loans is of great importance to the risk contagion. When all the banks connected with loans, the exogenous shocks would diversify and there would be no contagion of risk. However, when this kind of connection is not completed, external shocks would cluster on certain banks, and once they went bankrupt, their early clearance and corresponding damage will contagion to the other banks in the system without direct debt. Watts [ 99 ] proposed the definition of global cascade. His research showed that in the stochastic network with correlated nodes, global cascade will be dependent on the overall correlation of the network if the network is spear; on the other hand, is the network is concentrated; the global cascade will depend on the stability of each node. In the first case, global cascade probability follows the power-law distribution and hub nodes are important to global cascade; while in the second situation, global cascade probability follows bimodal distribution and the nodes above average links level can all trigger the global cascade. Besides, the second case is more robust-yet-fragile, which means that the network can survive after multiple times of external shocks before a certain one leading to systemic collapse. At last, he found that heterogeneity of each node has two sides of impacts on the stability of the system: on one hand, the increase of heterogeneity raises the probability of global cascade; on the other hand, when such heterogeneity approaching the peak will reduce the possibility of collapse.
Thurner, et al. [ 100 ] emphasized the importance and necessity of contract between banks for the purpose of risk diversification in banking system. Elsinger, et al. [ 101 ] focused on mutual credit obligation banking system and offer the single clearing mechanism in which banks repay the outstanding loans based on priority and limited liability. In the meantime, they use comparative static analysis to identify the relationship of the clearing payment vector, the cash flow and nominal liability, which means the clearing payment vector being the concave function of the cash flow and nominal liability. When the nominal liability is given, the clearing payment vector and the cash flow is positively related, the more the cash flow, the larger the vector.
For the random network perspective, Aleksiejuk and Holyst [ 102 ] applied two-dimensional directed percolation model to represent the whole financial system to analyze the situation that single bank bankruptcy result in the whole system collapse. They further explained the reason why few banks’ bankruptcies lead to the collapse of the whole banking industry in the Great depression period. May and Arinaminpathy [ 103 ] studied the influence of the direct link (debt) between financial institutions that lead to the risk contagion with random networks.
4.3 Banking Systemic Risk Measurement with Network Approach
Ever since the financial crisis in 2008, the measurement and control of systemic risk has become a hot research field. The banking system is one of the most crucial elements in the financial system, and both domestic and foreign scholars had carried out exhaustive and profound studies on the measurement and control of banking sectors. However, such studies had conducted even before the financial crisis in 2008. For example, Angelini, Maresca and Russo [ 104 ] , applied of the lending statistics among banks in Italy, building an interbank lending network and studying its impact on the systemic risk. The research found that only 4% of the banks in Italy are large enough to trigger systemic crisis. The result is significantly smaller than that in American banking system, which attribute to the relatively smaller cash flow in Italian banking system and different network structure. Elsinger, Lehar and Summer [ 105 ] used data from Australian banks and the open market data to study the two kinds of risk-spread patterns which may lead to systemic risk: Asset correlation and lending relationship. They found that the measurement that only takes into account the lending relationship significantly underestimates single bank default impact on the entire banking system; however, the asset correlation is the primary risk spread means of systemic risk. They also discovered that the probability of risk contagion by asset correlation is quite limited, but once it happens, most of banks will fail together.
Since the outbreak of the financial crisis in 2008, more scholars studied the systematic risk precaution and management. For example, Huang, Zhou and Zhu [ 106 ] proposed analyzing the systematic risks in financial system by drawing on the high frequency data in debt default insurance, and thus increased the accuracy in predicting asset correlations. Further, they added the inter-financial-institutional correlation data to construct a micro-macro model for financial system stress test. Hu, Zhao, Hua and Wong [ 107 ] , employing the commercial intelligent approach to construct the network-based banking systemic risk management model. By analyzing the real data of interbank financial relationship from The Federal Deposit Insurance Corporation, they studied the risk level of each individual bank in the banking system. They concluded that when huge impact on the market occurs, the inter-bank payment relationship is more capable to influence the survival of each individual bank than the correlation of bank holding portfolio. This conclusion may offer some clues for financial regulators to work out more effective risk-precaution mechanism. After the emergence of Internet data, Cerchiello and Giudici [ 108 ] used the finance-related tweets data and financial data to describe the systematic risks of financial market. They described the risks-network in the banking system with tweets data and Gauss model. Basing on that, the researchers made improvement in asset pricing variance and covariance matrix by combing the two data sources and Bayesian posterior approach, which offers a new perspective for systemic risk.
At present, the researches on the systematic risks from the network perspective and their influences on financial institutions raised increasing interests from financial experts. However, there is not profound theoretical interpretation concerning the spread of risks and the measurement of systematic risks. It is, therefore, possible to consider combination of big-data-based analytics and agent-based computation models to develop new principles and approaches for market supervision and systematic risks management.
5 Key Problems of Financial Innovations in Big Data Era
There are four dimensions of key problems of financial innovations in Big Data era:
5.1 Product Level
Big data analysis provides new information sources and client classification criteria for financial institutions, thus allowing them to use these information to design financial products that have never been used before (e.g., catastrophe insurance of insurance company, market sentiment based investment fund, etc.), as well as launching differentiated financial products and services according to different types of clients. At the same time, pricing and risk measuring problems towards “data-driven” financial innovative products or services arise.
5.2 Industry Level
Combining transaction (operational) data with on-line(off-line) non-structured data, applying data mining and analytics, financial service institutions (bank, insurance and security, etc.) will achieve remarkable optimization and innovation on operating cost, service efficiency, business mode and other aspects. So, how will “data-driven” financial innovation change current finance industry operation, allocation of resources and ecosystems?
5.3 Systemic Level
On the one hand, although “data-driven” financial innovation brings good solutions for risk measurement and management, it may cause unpredictable impacts on the financial system (e.g., the subprime crisis in 2007); on the other hand, bid data analytical method could become the powerful weapon of financial supervision institutions for systemic risk measurement and management. Meanwhile, the problems of supervision on the “data driven” financial innovation from the perspective of systematic risk and systematic risk management using “Big data” analytic methods arise.
5.4 Relative Solutions and Significance
To solve the key problems above, researchers should cooperate with traditional financial institutions or companies, such as banks, insurance companies, trading agencies, Internet finance companies, to combine available non-structured data with account data of these financial institutions to study product pricing principle; its impact on industry innovation modes; and effect to the systematic risk of the financial system based on data driven financial innovation.
Theoretically, traditional financial innovation theory was established on cost reducing, supervision aversion and technological updating, but the “data-driven” financial innovation theory has not yet matured. The research based on the cross fusion of financial innovation theory, risk management theory and relevant research methods of data science will provide a profound understanding of the development and evolution of “data-driven” financial innovation. Besides, the research results will also put forward a new method and perspective for the financial innovation theories of product pricing, industry mode, risk management, etc. Furthermore, it will make a fundamental contribution to the development of the “big data era” financial innovation related theory.
In practice, on the one hand, the research results using public individual data from multiple financial institutions and the Internet will provide a solid theoretical foundation for the optimization and design of product pricing, cost control, operation mode and service innovation of the financial institutions. On the other hand, the outcome from study of big data method applications in industry supervision and systematic risk measurement will provide methodological support and policy recommendations for regulatory authorities to manage the industry’s internal risk and financial systematic risk.
6 Future Research Frontiers and Development Trend
Among research on big data based financial innovation and risk management study, some area have already made series of significant theoretical breakthrough, such as on the innovation of P2P internet lending platform; securities market participant’s behavior evolution in social medias; systemic risk from network perspective and the effect exerted on financial institutions. However, as it is easier to obtain more various and cross-correlated micro-financial data in the big data time, the information cost is greatly reduced, which brings a new opportunity for finance industry. Based comprehensive consideration on the important achievements and practical requirements that already obtained, we conclude the research frontier and development trend in this field as following:
Using big data to extend the researches on securities market individual participant’s behavior and evolution to the entire financial system participant’s behavior and evolution. Even though the existing research had already applied big data to microscopic behavior study in financial market, but with the continuous development of big data, it is possible to reflect market participant’s behavior more precisely with comprehensive cross-correlated data. Especially, from the perspective of behavior evolution of big data background to integrate the cross-correlated data among banks, insurance companies, P2P platforms, and exchange insinuations to carry on microscopic analysis will be an important research direction in the future.
By extending the existing financial innovation model, financial service institutions can integrate their own transaction data and possible external data to carry on product pricing and service innovation research. In the future research with the development of information technology, it is possible to study on the cost reduction in financial service institution; the promotion of the risk measure method; understanding customers’ incomplete aspects, and regulations. Specifically, research on the innovation of resources integration of financial institutions internally, in the meantime, the new business model and service innovation among different financial institutions would also be considered.
Developing market regulation and systematic risk management method based on big data and agent-based computational models. Due to lack of account data, scholars can only focus on the false information on Internet and asset pricing without considering the cash flows and investor trading behavior. Thus, only relying on the changes of characteristics in Internet information diffusion is not enough to estimate whether information manipulation exists or not effectively. From regulation perspective, as long as the transaction data is available, the influence of misleading information to asset pricing in the security market requires further studying.
Supported by National Natural Science Foundation of China (71320107003, 71532009, 71201112), Core Projects in Tianjin Education Bureaus Social Science Program (2014ZD13)
Acknowledgements
The authors gratefully acknowledge the editor and two anonymous referees for their insightful comments and helpful suggestions that led to a marked improvement of the article.
[1] Edelman B. Using Internet data for economic research. Journal of Economic Perspectives, 2012, 26(2): 189–206. 10.1257/jep.26.2.189 Search in Google Scholar
[2] Varian H R. Big Data: New tricks for econometrics. Journal of Economic Perspectives, 2014, 28(2): 3–28. 10.1257/jep.28.2.3 Search in Google Scholar
[3] Paravisini D, Rappoport V, Ravina E. Risk aversion and wealth: Evidence from person-to-person lending portfolios. National Bureau of Economic Research Working Paper, 2013. 10.2139/ssrn.1507902 Search in Google Scholar
[4] Zhang Z. Credit risk preference in e-finance: An empirical analysis of P2P lending. Pacific Asia Conference on Information Systems Working Paper, 2014. Search in Google Scholar
[5] Li S, Qiu J, Lin Z, et al. Do borrowers make homogeneous decisions in online P2P lending market? An empirical study of PPDai in China. In Service Systems and Service Management (ICSSSM), 2011 8th International Conference on (pp. 1–6). IEEE. 10.1109/ICSSSM.2011.5959504 Search in Google Scholar
[6] Krumme K A, Herrero S. Lending behavior and community structure in an online peer-to-peer economic network. In Computational Science and Engineering, 2009. CSE’09. IEEE on International Conference, 2009, 4: 613–618. 10.1109/CSE.2009.185 Search in Google Scholar
[7] Yum H, Lee B, Chae M. From the wisdom of crowds to my own judgment in microfinance through online peer-to-peer lending platforms. Electronic Commerce Research and Applications, 2012, 11(5): 469–483. 10.1016/j.elerap.2012.05.003 Search in Google Scholar
[8] Ceyhan S, Shi X, Leskovec J. Dynamics of bidding in a P2P lending service: Effects of herding and predicting loan success. In Proceedings of the 20th international conference on World Wide Web, ACM, 2011, 547–556. 10.1145/1963405.1963483 Search in Google Scholar
[9] Li S, Lin Z X, Qiu J X, et al. How friendship networks work in online P2P lending markets. Nankai Business Review International, 2015, 6(1): 42–67. 10.1108/NBRI-01-2014-0010 Search in Google Scholar
[10] Herzenstein M, Dholakia U M, Andrews R L. Strategic herding behavior in peer-to-peer loan auctions. Journal of Interactive Marketing, 2011, 25(1): 27–36. 10.1016/j.intmar.2010.07.001 Search in Google Scholar
[11] Lee E, Lee B. Herding behavior in online P2P lending: An empirical investigation. Electronic Commerce Research and Applications, 2012, 11(5): 495–503. 10.1016/j.elerap.2012.02.001 Search in Google Scholar
[12] Luo B, Lin Z. A decision tree model for herd behavior and empirical evidence from the online P2P lending market. Information Systems and e-Business Management, 2013, 11(1): 141–160. 10.1007/s10257-011-0182-4 Search in Google Scholar
[13] Li Y, Guo Y, Zhang W. The analysis of impact factors on loan performance in Chinese P2P microfinance market. Journal of Financial Research, 2013(7): 126–138. Search in Google Scholar
[14] Chen N, Ghosh A, Lambert N S. Auctions for social lending: A theoretical analysis. Games and Economic Behavior, 2014, 86: 369–391. 10.1016/j.geb.2013.05.004 Search in Google Scholar
[15] Redmond U, Cunningham P. A temporal network analysis reveals the unprofitability of arbitrage in the prosper marketplace. Expert Systems with Applications, 2013, 40(9): 3715–3721. 10.1016/j.eswa.2012.12.077 Search in Google Scholar
[16] Burtch G, Ghose A, Wattal S. An empirical examination of the antecedents and consequences of contribution patterns in crowd-funded markets. Information Systems Research, 2013, 24(3): 499–519. 10.1287/isre.1120.0468 Search in Google Scholar
[17] Lin M, Prabhala N R, Viswanathan S. Judging borrowers by the company they keep: Friendship networks and information asymmetry in online peer-to-peer lending. Management Science, 2013, 59(1): 17–35. 10.1287/mnsc.1120.1560 Search in Google Scholar
[18] Emekter R, Tu Y, Jirasakuldech B, et al. Evaluating credit risk and loan performance in online Peer-to-Peer (P2P) lending. Applied Economics, 2015, 47(1): 54–70. 10.1080/00036846.2014.962222 Search in Google Scholar
[19] Michels J. Do unverifiable disclosures matter? Evidence from peer-to-peer lending. Accounting Review, 2012, 87(4): 1385–1413. 10.2308/accr-50159 Search in Google Scholar
[20] Barasinska N, Schafer D. Is crowd funding different? Evidence on the relation between gender and funding success from a German Peer-to-Peer lending platform. German Economic Review, 2014, 15(4): 436–452. 10.1111/geer.12052 Search in Google Scholar
[21] Ravina E. The effect of beauty and personal characteristics in credit markets. SSRN Working Paper (1107307), 2008. 10.2139/ssrn.1107307 Search in Google Scholar
[22] Larrimore L, Jiang L, Larrimore J, et al. Peer to peer lending: The relationship between language features, trustworthiness, and persuasion success. Journal of Applied Communication Research, 2011, 39(19): 19–37. 10.1080/00909882.2010.536844 Search in Google Scholar
[23] Herzenstein M, Sonenshein S, Dholakia U M. Tell me a good story and I may lend you money: The role of narratives in peer-to-peer lending decisions. Journal of Marketing Research, 2011, 48(SPL): S138–S149. 10.1509/jmkr.48.SPL.S138 Search in Google Scholar
[24] Ashta A, Assadi D. Does social lending incorporate social technologies? The use of web 2.0 technologies in online P2P lending. CEB Working Papers, 2009. Search in Google Scholar
[25] Herzenstein M, Andrews R L, Dholakia U, et al. The democratization of personal consumer loans? Determinants of success in online peer-to-peer lending communities. Boston University School of Management Research Paper, 2008. Search in Google Scholar
[26] Qiu J, Laine J, Lin Z, et al. Pricing strategies in electronic two-sided markets — Evidence from the online P2P lending marketplace. SIGBPS Workshop on Business Processes and Services (BPS’12), 2012. Search in Google Scholar
[27] Berger S C, Gleisner F. Emergence of financial intermediaries in electronic markets: The case of online P2P lending. BuR-Business Research, 2009, 2(1): 39–65. 10.1007/BF03343528 Search in Google Scholar
[28] Iyer R, Ijaz A, Erzo K, et al. Inferring asset quality: Determining borrower creditworthiness in peer-to-peer lending markets. 2010. Search in Google Scholar
[29] Iyer R, Khwaja A, Luttmer E, et al. Screening in new credit markets: Can individual lenders infer borrower creditworthiness in peer-to-peer lending? In AFA 2011 Denver Meetings Paper, 2009. 10.2139/ssrn.1570115 Search in Google Scholar
[30] Wang Y, Lin Z. The importance of objective and dynamic credit evaluation in P2P lending market. Working Paper, 2014. Search in Google Scholar
[31] Freedman S, Jin G Z. Do social networks solve information problems for peer-to-peer lending? Evidence from Prosper.com. Working Paper, 2008. 10.2139/ssrn.1936057 Search in Google Scholar
[32] Lin M. Peer-to-peer lending: An empirical study. AMCIS 2009 Doctoral Consortium, 2009: 17. Search in Google Scholar
[33] Weiss G N, Pelger K, Horsch A. Mitigating adverse selection in P2P lending: Empirical evidence from Prosper.com. Available at SSRN 1650774, 2010. 10.2139/ssrn.1650774 Search in Google Scholar
[34] Puro L, Teich J E, Wallenius H, et al. Borrower decision aid for people-to-people lending. Decision Support Systems, 2010, 49(1): 52–60. 10.1016/j.dss.2009.12.009 Search in Google Scholar
[35] Zhao H, Wu L, Liu Q, et al. Investment recommendation in P2P lending: A portfolio perspective with risk management. Working Paper, 2014. 10.1109/ICDM.2014.104 Search in Google Scholar
[36] Luo C, Xiong H, Zhou W, et al. Enhancing investment decisions in P2P lending: an investor composition perspective. In Proceedings of the 17th ACM SIGKDD International Conference on Knowledge Discovery and Data Mining, ACM, 2011, 292–300. 10.1145/2020408.2020458 Search in Google Scholar
[37] Riggins F J, Weber D M. A model of peer-to-peer (P2P) social lending in the presence of identification bias. In Proceedings of the 13th International Conference on Electronic Commerce, ACM, 2011, 23. 10.1145/2378104.2378127 Search in Google Scholar
[38] Batsell J. Gossip central — Internet message boards can leave some stocks hanging by a thread. Seattle Times, 1998. Search in Google Scholar
[39] Bennett J. Traffic on financial web pages rises when the market falls. Dow Jones News Service, 1998. Search in Google Scholar
[40] Goldstein A. Money messages: Electronic message boards are a good way to get investing facts and fiction. Dallas Morning News, 1998. Search in Google Scholar
[41] Harmon A. The market turmoil: Investors on line. New York Times, 1998. Search in Google Scholar
[42] Maremount M. Predeal trading in U.S. surgical puts spotlight on cyberinvestors. Wall Street Journal, May 28, 1998. Search in Google Scholar
[43] Medill G. Chicago firm wants to know what Yahoo! left messages. Chicago Daily Herald, 1998. Search in Google Scholar
[44] Wysocki P. Cheap talk on the web: The determinants of postings on stock message boards. University of Michigan Business School Working Paper (98025), 1998. 10.2139/ssrn.160170 Search in Google Scholar
[45] Tumarkin R, Whitelaw R F. News or noise? Internet postings and stock prices. Financial Analysts Journal, 2001, 57(3): 41–51. 10.2469/faj.v57.n3.2449 Search in Google Scholar
[46] Bowen R M, Davis A K, Rajgopal S. Determinants of revenue recognition practices for Internet firms. Contemporary Accounting Research, 2002, 19(4): 523–562. 10.1506/9728-4YG8-GC3L-FPFA Search in Google Scholar
[47] Clarkson, Joyce P D, Tutticci I. Market reaction to takeover rumour in internet discussion sites. Accounting and Finance, 2006, 46(1): 31–52. 10.1111/j.1467-629X.2006.00160.x Search in Google Scholar
[48] Dewally M. Internet investment advice: Investing with a rock of salt. Financial Analysts Journal, 2003, 59(4): 65–77. 10.2469/faj.v59.n4.2546 Search in Google Scholar
[49] Bohme R, Holz T. The effect of stock spam on financial markets. SSRN Working Paper, 2006. 10.2139/ssrn.897431 Search in Google Scholar
[50] Hanke M, Hauser F. On the effects of stock spam e-mails. Journal of Financial Markets, 2008, 11(1): 57–83. 10.1016/j.finmar.2007.10.001 Search in Google Scholar
[51] Hu N, Liu L, Tripathy A, et al. Value relevance of blog visibility. Journal of Business Research, 2011, 64(12): 1361–1368. 10.1016/j.jbusres.2010.12.025 Search in Google Scholar
[52] Saxton G. Financial blogs and information asymmetry between firm insiders and outsiders. Proceedings of American Accounting Association, Anaheim, CA, USA, 2008. Search in Google Scholar
[53] Mondria J, Wu T, Zhang Y. The determinants of international investment and attention allocation: Using internet search query data. Journal of International Economics, 2010, 82(1): 85–95. 10.1016/j.jinteco.2010.04.007 Search in Google Scholar
[54] Zhang Y, Zhang W, Jin X, et al. Does the Internet know more? Open source information and asset pricing. Systems Engineering — Theory & Practice, 2011, 31(4): 577–586. Search in Google Scholar
[55] Antweiler W, Frank M Z. Is all that talk just noise? The information content of internet stock message boards. Journal of Finance, 2004, 59(3): 1259–1294. 10.1111/j.1540-6261.2004.00662.x Search in Google Scholar
[56] Das S R, Chen M Y. Yahoo! for Amazon: Sentiment extraction from small talk on the web. Management Science, 2007, 53(9): 1375–1388. 10.1287/mnsc.1070.0704 Search in Google Scholar
[57] Zhang Y, Swanson P E, Prombutr W. Measuring effects on stock returns of sentiment indexes created from stock message boards. Journal of Financial Research, 2012, 35(1): 79–114. 10.1111/j.1475-6803.2011.01310.x Search in Google Scholar
[58] Bollen J, Mao H, Zeng X. Twitter mood predicts the stock market. Journal of Computational Science, 2011, 2(1): 1–8. 10.1016/j.jocs.2010.12.007 Search in Google Scholar
[59] Felton J, Kim J. Warnings from the Enron message board. Journal of Investing, 2002, 11(3): 29–52. 10.3905/joi.2002.319512 Search in Google Scholar
[60] Gu B, Konana P, Rajagopalan B, et al. Competition among virtual communities and user valuation: The case of investing-related communities. Information Systems Research, 2007, 18(1): 68–85. 10.1287/isre.1070.0114 Search in Google Scholar
[61] De Choudhury M, Sundaram H, John A, et al. Can blog communication dynamics be correlated with stock market activity? In Proceedings of the 19 ACM Conference on Hypertext and Hypermedia, Pittsburgh, PA, USA: ACM, 2008. 10.1145/1379092.1379106 Search in Google Scholar
[62] Da Z, Engelberg J, Gao P. In search of attention. The Journal of Finance, 2011, 66(5): 1461–1499. 10.1111/j.1540-6261.2011.01679.x Search in Google Scholar
[63] Merton R C. A simple model of capital market equilibrium with incomplete information. The Journal of Finance, 1987, 42(3): 483–510. 10.1111/j.1540-6261.1987.tb04565.x Search in Google Scholar
[64] Sims C A. Implications of rational inattention. Journal of monetary Economics, 2003, 50(3): 665–690. 10.1016/S0304-3932(03)00029-1 Search in Google Scholar
[65] Hirshleifer D, Teoh S H. Limited attention, information disclosure, and financial reporting. Journal of Accounting and Economics, 2003, 36(1): 337–386. 10.1016/j.jacceco.2003.10.002 Search in Google Scholar
[66] Grullon G, Kanatas G, Weston J P. Advertising, breadth of ownership, and liquidity. Review of Financial Studies, 2004, 17(2): 439–461. 10.1093/rfs/hhg039 Search in Google Scholar
[67] Chemmanur T, Yan A. Product market advertising and new equity issues. Journal of Financial Economics, 2009, 92(1): 40–65. 10.1016/j.jfineco.2008.02.009 Search in Google Scholar
[68] Chan W S. Stock price reaction to news and no-news: Drift and reversal after headlines. Journal of Financial Economics, 2003, 70(2): 223–260. 10.1016/S0304-405X(03)00146-6 Search in Google Scholar
[69] Fang L, Peress J. Media coverage and the cross-section of stock returns. The Journal of Finance, 2009, 64(5): 2023–2052. 10.1111/j.1540-6261.2009.01493.x Search in Google Scholar
[70] Barber B M, Odean T. All that glitters: The effect of attention and news on the buying behavior of individual and institutional investors. Review of Financial Studies, 2008, 21(2): 785–818. 10.1002/9781118467411.ch7 Search in Google Scholar
[71] Seasholes M S, Wu G. Predictable behavior, profits, and attention. Journal of Empirical Finance, 2007, 14(5): 590–610. 10.1016/j.jempfin.2007.03.002 Search in Google Scholar
[72] Bank M, Larch M, Peter G. Google search volume and its influence on liquidity and returns of German stocks. Financial markets and portfolio management, 2011, 25(3): 239–264. 10.1007/s11408-011-0165-y Search in Google Scholar
[73] Vlastakis N, Markellos R N. Information demand and stock market volatility. Journal of Banking and Finance, 2012, 36(6): 1808–1821. 10.1016/j.jbankfin.2012.02.007 Search in Google Scholar
[74] Drake M S, Roulstone D T, Thornock J R. Investor information demand: Evidence from Google searches around earnings announcements. Journal of Accounting Research, 2012, 50(4): 1001–1040. 10.2139/ssrn.1669507 Search in Google Scholar
[75] Dzielinski M. Measuring economic uncertainty and its impact on the stock market. Finance Research Letters, 2012, 9(3): 167–175. 10.1016/j.frl.2011.10.003 Search in Google Scholar
[76] Yu Q, Zhang B. Limited attention and stock performance: An empirical study using Baidu index as the proxy for investor attention. Journal of Financial Research, 2012, 385: 152–165. Search in Google Scholar
[77] Zhang W, Shen D, Zhang Y, et al. Open source information, investor attention, and asset pricing. Economic Modelling, 2013, 33(0): 613–619. 10.1016/j.econmod.2013.03.018 Search in Google Scholar
[78] Liu F, Ye Q, Li Y J. Impacts of interactions between news attention and investor attention on stock returns: Empirical investigation on financial shares in China. Journal of Management Sciences in China, 2014, 17(1): 72–85. Search in Google Scholar
[79] Zhang Y, Feng L, Jin X, et al. Internet information arrival and volatility of SME PRICE INDEX. Physica A: Statistical Mechanics and Its Applications, 2014, 399: 70–74. 10.1016/j.physa.2013.12.034 Search in Google Scholar
[80] Lamoureux C G, Lastrapes W D. Heteroskedasticity in stock return data: Volume versus GARCH effects. The Journal of Finance, 1990, 45(1): 221–229. 10.1111/j.1540-6261.1990.tb05088.x Search in Google Scholar
[81] Kalev P S, Liu W M, Pham P K, et al. Public information arrival and volatility of intraday stock returns. Journal of Banking and Finance, 2004, 28(6): 1441–1467. 10.1016/S0378-4266(03)00126-2 Search in Google Scholar
[82] Wagner N, Marsh T A. Surprise volume and heteroskedasticity in equity market returns. Quantitative Finance, 2005, 5(2): 153–168. 10.1080/14697680500147978 Search in Google Scholar
[83] Fleming J, Kirby C, Ostdiek B. Stochastic volatility, trading volume, and the daily flow of information. The Journal of Business, 2006, 79(3): 1551–1590. 10.1086/500685 Search in Google Scholar
[84] McMillan D G, Garcia R Q. Does information help intra-day volatility forecasts?. Journal of Forecasting, 2013, 32(1): 1–9. 10.1002/for.1243 Search in Google Scholar
[85] Crawford V P, Sobel J. Strategic information transmission. Econometrica: Journal of the Econometric Society, 1982, 50(6): 1431–1451. 10.2307/1913390 Search in Google Scholar
[86] Benabou R, Laroque G. Using privileged information to manipulate markets: Insiders, gurus, and credibility. The Quarterly Journal of Economics, 1992, 59(3): 921–958. 10.2307/2118369 Search in Google Scholar
[87] Crawford V P. Lying for strategic advantage: Rational and boundedly rational misrepresentation of intentions. American Economic Review, 2003, 93(1): 133–149. 10.1257/000282803321455197 Search in Google Scholar
[88] Chen Y. Perturbed communication games with honest senders and naive receivers. Journal of Economic Theory, 2011, 146(2): 401–424. 10.1016/j.jet.2010.08.001 Search in Google Scholar
[89] Bommel J V. Rumors. The Journal of Finance, 2003, 58(4): 1499–1520. 10.1111/1540-6261.00575 Search in Google Scholar
[90] Garcia D, Sangiorgi F. Information sales and strategic trading. Review of Financial Studies, 2011, 24(9): 3069–3104. 10.2139/ssrn.1107330 Search in Google Scholar
[91] Liu Q. Information acquisition and reputation dynamics. The Review of Economic Studies, 2011, 78(4): 1400–1425. 10.1093/restud/rdq039 Search in Google Scholar
[92] Klumpp T. Communication in financial markets with several informed traders. Economic Theory, 2007, 33(3): 437–456. 10.1007/s00199-006-0148-9 Search in Google Scholar
[93] Becker B, Milbourn T. How did increased competition affect credit ratings?. Journal of Financial Economics, 2011, 101(3): 493–514. 10.3386/w16404 Search in Google Scholar
[94] Hong D, Hong H G, Ungureanu A. An epidemiological approach to opinion and price-volume dynamics. AFA 2012 Chicago Meetings Paper, 2011. 10.2139/ssrn.1569418 Search in Google Scholar
[95] Feng X, Zhang W, Zhang Y, et al. Information identification in different networks with heterogeneous information sources. Journal of Systems Science and Complexity, 2014, 27(1): 92–116. 10.1007/s11424-014-3297-0 Search in Google Scholar
[96] Schweitzer F, Fagiolo G, Sornette D, et al. Economic networks: The new challenges. Science, 2009, 325(5939): 422–425. 10.1126/science.1173644 Search in Google Scholar PubMed
[97] Lux T. Network theory is sorely required. Nature, 2011, 469(7330): 303–303. Search in Google Scholar
[98] Allen F, Gale D. Financial contagion. Journal of Political Economy, 2000, 108(1): 1–33. 10.1086/262109 Search in Google Scholar
[99] Watts D J. A simple model of global cascades on random networks. Proceedings of the National Academy of Sciences, 2002, 99(9): 5766–5771. 10.1515/9781400841356.497 Search in Google Scholar
[100] Thurner S, Hanel R, Pichler S. Risk trading, network topology and banking regulation. Quantitative Finance, 2003, 3(4): 306–319. 10.1088/1469-7688/3/4/307 Search in Google Scholar
[101] Elsinger H, Lehar A, Summer M. Using market information for banking system risk assessment. Available at SSRN 787929, 2005. 10.2139/ssrn.787929 Search in Google Scholar
[102] Aleksiejuk A, Holyst J A. A simple model of bank bankruptcies. Physica A: Statistical Mechanics and Its Applications, 2001, 299(1–2): 198–204. 10.1016/S0378-4371(01)00296-5 Search in Google Scholar
[103] May R M, Arinaminpathy N. Systemic risk: The dynamics of model banking systems. Journal of the Royal Society Interface, 2010, 7(46): 823–838. 10.1098/rsif.2009.0359 Search in Google Scholar
[104] Angelini P, Maresca G, Russo D. Systemic risk in the netting system. Journal of Banking and Finance, 1996, 20(5): 853–868. 10.1016/0378-4266(95)00029-1 Search in Google Scholar
[105] Elsinger H, Lehar A, Summer M. Risk assessment for banking systems. Management Science, 2006, 52(9): 1301–1314. 10.1287/mnsc.1060.0531 Search in Google Scholar
[106] Huang X, Zhou H, Zhu H. A framework for assessing the systemic risk of major financial institutions. Journal of Banking and Finance, 2009, 33(11): 2036–2049. 10.1016/j.jbankfin.2009.05.017 Search in Google Scholar
[107] Hu D, Zhao J L, Hua Z, et al. Network-based modeling and analysis of systemic risk in banking systems. MIS Quarterly, 2012, 36(4): 1269–1291. 10.2307/41703507 Search in Google Scholar
[108] Cerchiello P, Giudici P. How to measure the quality of financial tweets. Quality and Quantity, 2015, 1–19. 10.1007/s11135-015-0229-6 Search in Google Scholar
© 2016 Walter de Gruyter GmbH, Berlin/Boston
- X / Twitter
Supplementary Materials
Please login or register with De Gruyter to order this product.
Journal and Issue
Articles in the same issue.

Published: 5 April 2024 Contributors: Tim Mucci, Cole Stryker
Big data analytics refers to the systematic processing and analysis of large amounts of data and complex data sets, known as big data, to extract valuable insights. Big data analytics allows for the uncovering of trends, patterns and correlations in large amounts of raw data to help analysts make data-informed decisions. This process allows organizations to leverage the exponentially growing data generated from diverse sources, including internet-of-things (IoT) sensors, social media, financial transactions and smart devices to derive actionable intelligence through advanced analytic techniques.
In the early 2000s, advances in software and hardware capabilities made it possible for organizations to collect and handle large amounts of unstructured data. With this explosion of useful data, open-source communities developed big data frameworks to store and process this data. These frameworks are used for distributed storage and processing of large data sets across a network of computers. Along with additional tools and libraries, big data frameworks can be used for:
- Predictive modeling by incorporating artificial intelligence (AI) and statistical algorithms
- Statistical analysis for in-depth data exploration and to uncover hidden patterns
- What-if analysis to simulate different scenarios and explore potential outcomes
- Processing diverse data sets, including structured, semi-structured and unstructured data from various sources.
Four main data analysis methods – descriptive, diagnostic, predictive and prescriptive – are used to uncover insights and patterns within an organization's data. These methods facilitate a deeper understanding of market trends, customer preferences and other important business metrics.
IBM named a Leader in the 2024 Gartner® Magic Quadrant™ for Augmented Data Quality Solutions.
Structured vs unstructured data
What is data management?
The main difference between big data analytics and traditional data analytics is the type of data handled and the tools used to analyze it. Traditional analytics deals with structured data, typically stored in relational databases . This type of database helps ensure that data is well-organized and easy for a computer to understand. Traditional data analytics relies on statistical methods and tools like structured query language (SQL) for querying databases.
Big data analytics involves massive amounts of data in various formats, including structured, semi-structured and unstructured data. The complexity of this data requires more sophisticated analysis techniques. Big data analytics employs advanced techniques like machine learning and data mining to extract information from complex data sets. It often requires distributed processing systems like Hadoop to manage the sheer volume of data.
These are the four methods of data analysis at work within big data:
The "what happened" stage of data analysis. Here, the focus is on summarizing and describing past data to understand its basic characteristics.
The “why it happened” stage. By delving deep into the data, diagnostic analysis identifies the root patterns and trends observed in descriptive analytics.
The “what will happen” stage. It uses historical data, statistical modeling and machine learning to forecast trends.
Describes the “what to do” stage, which goes beyond prediction to provide recommendations for optimizing future actions based on insights derived from all previous.
The following dimensions highlight the core challenges and opportunities inherent in big data analytics.
The sheer volume of data generated today, from social media feeds, IoT devices, transaction records and more, presents a significant challenge. Traditional data storage and processing solutions are often inadequate to handle this scale efficiently. Big data technologies and cloud-based storage solutions enable organizations to store and manage these vast data sets cost-effectively, protecting valuable data from being discarded due to storage limitations.
Data is being produced at unprecedented speeds, from real-time social media updates to high-frequency stock trading records. The velocity at which data flows into organizations requires robust processing capabilities to capture, process and deliver accurate analysis in near real-time. Stream processing frameworks and in-memory data processing are designed to handle these rapid data streams and balance supply with demand.
Today's data comes in many formats, from structured to numeric data in traditional databases to unstructured text, video and images from diverse sources like social media and video surveillance. This variety demans flexible data management systems to handle and integrate disparate data types for comprehensive analysis. NoSQL databases , data lakes and schema -on-read technologies provide the necessary flexibility to accommodate the diverse nature of big data.
Data reliability and accuracy are critical, as decisions based on inaccurate or incomplete data can lead to negative outcomes. Veracity refers to the data's trustworthiness, encompassing data quality, noise and anomaly detection issues. Techniques and tools for data cleaning, validation and verification are integral to ensuring the integrity of big data, enabling organizations to make better decisions based on reliable information.
Big data analytics aims to extract actionable insights that offer tangible value. This involves turning vast data sets into meaningful information that can inform strategic decisions, uncover new opportunities and drive innovation. Advanced analytics, machine learning and AI are key to unlocking the value contained within big data, transforming raw data into strategic assets.
Data professionals, analysts, scientists and statisticians prepare and process data in a data lakehouse, which combines the performance of a data lakehouse with the flexibility of a data lake to clean data and ensure its quality. The process of turning raw data into valuable insights encompasses several key stages:
- Collect data: The first step involves gathering data, which can be a mix of structured and unstructured forms from myriad sources like cloud, mobile applications and IoT sensors. This step is where organizations adapt their data collection strategies and integrate data from varied sources into central repositories like a data lake, which can automatically assign metadata for better manageability and accessibility.
- Process data: After being collected, data must be systematically organized, extracted, transformed and then loaded into a storage system to ensure accurate analytical outcomes. Processing involves converting raw data into a format that is usable for analysis, which might involve aggregating data from different sources, converting data types or organizing data into structure formats. Given the exponential growth of available data, this stage can be challenging. Processing strategies may vary between batch processing, which handles large data volumes over extended periods and stream processing, which deals with smaller real-time data batches.
- Clean data: Regardless of size, data must be cleaned to ensure quality and relevance. Cleaning data involves formatting it correctly, removing duplicates and eliminating irrelevant entries. Clean data prevents the corruption of output and safeguard’s reliability and accuracy.
- Analyze data: Advanced analytics, such as data mining, predictive analytics, machine learning and deep learning, are employed to sift through the processed and cleaned data. These methods allow users to discover patterns, relationships and trends within the data, providing a solid foundation for informed decision-making.
Under the Analyze umbrella, there are potentially many technologies at work, including data mining, which is used to identify patterns and relationships within large data sets; predictive analytics, which forecasts future trends and opportunities; and deep learning , which mimics human learning patterns to uncover more abstract ideas.
Deep learning uses an artificial neural network with multiple layers to model complex patterns in data. Unlike traditional machine learning algorithms, deep learning learns from images, sound and text without manual help. For big data analytics, this powerful capability means the volume and complexity of data is not an issue.
Natural language processing (NLP) models allow machines to understand, interpret and generate human language. Within big data analytics, NLP extracts insights from massive unstructured text data generated across an organization and beyond.
Structured Data
Structured data refers to highly organized information that is easily searchable and typically stored in relational databases or spreadsheets. It adheres to a rigid schema, meaning each data element is clearly defined and accessible in a fixed field within a record or file. Examples of structured data include:
- Customer names and addresses in a customer relationship management (CRM) system
- Transactional data in financial records, such as sales figures and account balances
- Employee data in human resources databases, including job titles and salaries
Structured data's main advantage is its simplicity for entry, search and analysis, often using straightforward database queries like SQL. However, the rapidly expanding universe of big data means that structured data represents a relatively small portion of the total data available to organizations.
Unstructured Data
Unstructured data lacks a pre-defined data model, making it more difficult to collect, process and analyze. It comprises the majority of data generated today, and includes formats such as:
- Textual content from documents, emails and social media posts
- Multimedia content, including images, audio files and videos
- Data from IoT devices, which can include a mix of sensor data, log files and time-series data
The primary challenge with unstructured data is its complexity and lack of uniformity, requiring more sophisticated methods for indexing, searching and analyzing. NLP, machine learning and advanced analytics platforms are often employed to extract meaningful insights from unstructured data.
Semi-structured data
Semi-structured data occupies the middle ground between structured and unstructured data. While it does not reside in a relational database, it contains tags or other markers to separate semantic elements and enforce hierarchies of records and fields within the data. Examples include:
- JSON (JavaScript Object Notation) and XML (eXtensible Markup Language) files, which are commonly used for web data interchange
- Email, where the data has a standardized format (e.g., headers, subject, body) but the content within each section is unstructured
- NoSQL databases, can store and manage semi-structured data more efficiently than traditional relational databases
Semi-structured data is more flexible than structured data but easier to analyze than unstructured data, providing a balance that is particularly useful in web applications and data integration tasks.
Ensuring data quality and integrity, integrating disparate data sources, protecting data privacy and security and finding the right talent to analyze and interpret data can present challenges to organizations looking to leverage their extensive data volumes. What follows are the benefits organizations can realize once they see success with big data analytics:
Real-time intelligence
One of the standout advantages of big data analytics is the capacity to provide real-time intelligence. Organizations can analyze vast amounts of data as it is generated from myriad sources and in various formats. Real-time insight allows businesses to make quick decisions, respond to market changes instantaneously and identify and act on opportunities as they arise.
Better-informed decisions
With big data analytics, organizations can uncover previously hidden trends, patterns and correlations. A deeper understanding equips leaders and decision-makers with the information needed to strategize effectively, enhancing business decision-making in supply chain management, e-commerce, operations and overall strategic direction.
Cost savings
Big data analytics drives cost savings by identifying business process efficiencies and optimizations. Organizations can pinpoint wasteful expenditures by analyzing large datasets, streamlining operations and enhancing productivity. Moreover, predictive analytics can forecast future trends, allowing companies to allocate resources more efficiently and avoid costly missteps.
Better customer engagement
Understanding customer needs, behaviors and sentiments is crucial for successful engagement and big data analytics provides the tools to achieve this understanding. Companies gain insights into consumer preferences and tailor their marketing strategies by analyzing customer data.
Optimized risk management strategies
Big data analytics enhances an organization's ability to manage risk by providing the tools to identify, assess and address threats in real time. Predictive analytics can foresee potential dangers before they materialize, allowing companies to devise preemptive strategies.
As organizations across industries seek to leverage data to drive decision-making, improve operational efficiencies and enhance customer experiences, the demand for skilled professionals in big data analytics has surged. Here are some prominent career paths that utilize big data analytics:
Data scientist
Data scientists analyze complex digital data to assist businesses in making decisions. Using their data science training and advanced analytics technologies, including machine learning and predictive modeling, they uncover hidden insights in data.
Data analyst
Data analysts turn data into information and information into insights. They use statistical techniques to analyze and extract meaningful trends from data sets, often to inform business strategy and decisions.
Data engineer
Data engineers prepare, process and manage big data infrastructure and tools. They also develop, maintain, test and evaluate data solutions within organizations, often working with massive datasets to assist in analytics projects.
Machine learning engineer
Machine learning engineers focus on designing and implementing machine learning applications. They develop sophisticated algorithms that learn from and make predictions on data.
Business intelligence analyst
Business intelligence (BI) analysts help businesses make data-driven decisions by analyzing data to produce actionable insights. They often use BI tools to convert data into easy-to-understand reports and visualizations for business stakeholders.
Data visualization specialist
These specialists focus on the visual representation of data. They create data visualizations that help end users understand the significance of data by placing it in a visual context.
Data architect
Data architects design, create, deploy and manage an organization's data architecture. They define how data is stored, consumed, integrated and managed by different data entities and IT systems.
IBM and Cloudera have partnered to create an industry-leading, enterprise-grade big data framework distribution plus a variety of cloud services and products — all designed to achieve faster analytics at scale.
IBM Db2 Database on IBM Cloud Pak for Data combines a proven, AI-infused, enterprise-ready data management system with an integrated data and AI platform built on the security-rich, scalable Red Hat OpenShift foundation.
IBM Big Replicate is an enterprise-class data replication software platform that keeps data consistent in a distributed environment, on-premises and in the hybrid cloud, including SQL and NoSQL databases.
A data warehouse is a system that aggregates data from different sources into a single, central, consistent data store to support data analysis, data mining, artificial intelligence and machine learning.
Business intelligence gives organizations the ability to get answers they can understand. Instead of using best guesses, they can base decisions on what their business data is telling them — whether it relates to production, supply chain, customers or market trends.
Cloud computing is the on-demand access of physical or virtual servers, data storage, networking capabilities, application development tools, software, AI analytic tools and more—over the internet with pay-per-use pricing. The cloud computing model offers customers flexibility and scalability compared to traditional infrastructure.
Purpose-built data-driven architecture helps support business intelligence across the organization. IBM analytics solutions allow organizations to simplify raw data access, provide end-to-end data management and empower business users with AI-driven self-service analytics to predict outcomes.

Fintechs, Researchers Lobby CFPB to Ease Bank Data Restrictions
By Evan Weinberger

The Consumer Financial Protection Bureau’s push to allow consumers to easily share their financial data will only entrench big banks’ power unless the agency makes it simpler for fintechs, among others, to use the data for research, advocates say.
The open banking proposal , mandated by the 2010 Dodd-Frank Act and released in October, would let consumers access their bank and credit card data at no cost and move it to fintech apps such as Wealthfront or Venmo. But it also restricts the use of data to “what is reasonably necessary to provide the consumer’s requested product or service.”
Consumer ...
Learn more about Bloomberg Law or Log In to keep reading:
Learn about bloomberg law.
AI-powered legal analytics, workflow tools and premium legal & business news.
Already a subscriber?
Log in to keep reading or access research tools.
- Work & Careers
- Life & Arts
Data centres have turned Big Tech into big spenders
Try unlimited access Only 1 € for 4 weeks
Then 69 € per month. Complete digital access to quality FT journalism on any device. Cancel anytime during your trial.
- Global news & analysis
- Expert opinion
- Special features
- FirstFT newsletter
- Videos & Podcasts
- Android & iOS app
- FT Edit app
- 10 gift articles per month
Explore more offers.
Standard digital.
- FT Digital Edition
Premium Digital
Print + premium digital, ft professional, weekend print + standard digital, weekend print + premium digital.
Complete digital access to quality FT journalism with expert analysis from industry leaders. Pay a year upfront and save 20%.
- Global news & analysis
- Exclusive FT analysis
- FT App on Android & iOS
- FirstFT: the day's biggest stories
- 20+ curated newsletters
- Follow topics & set alerts with myFT
- FT Videos & Podcasts
- 20 monthly gift articles to share
- Lex: FT's flagship investment column
- 15+ Premium newsletters by leading experts
- FT Digital Edition: our digitised print edition
- Weekday Print Edition
- Videos & Podcasts
- Premium newsletters
- 10 additional gift articles per month
- FT Weekend Print delivery
- Everything in Standard Digital
- Everything in Premium Digital
FT Weekend newspaper delivered Saturday plus complete digital access.
- 10 monthly gift articles to share
- Everything in Print
- Make and share highlights
- FT Workspace
- Markets data widget
- Subscription Manager
- Workflow integrations
- Occasional readers go free
- Volume discount
Billed Quarterly at 265 €. Complete digital access plus the FT newspaper delivered Monday-Saturday.
Terms & Conditions apply
Explore our full range of subscriptions.
Why the ft.
See why over a million readers pay to read the Financial Times.
International Edition
Artificial intelligence in strategy
Can machines automate strategy development? The short answer is no. However, there are numerous aspects of strategists’ work where AI and advanced analytics tools can already bring enormous value. Yuval Atsmon is a senior partner who leads the new McKinsey Center for Strategy Innovation, which studies ways new technologies can augment the timeless principles of strategy. In this episode of the Inside the Strategy Room podcast, he explains how artificial intelligence is already transforming strategy and what’s on the horizon. This is an edited transcript of the discussion. For more conversations on the strategy issues that matter, follow the series on your preferred podcast platform .
Joanna Pachner: What does artificial intelligence mean in the context of strategy?
Yuval Atsmon: When people talk about artificial intelligence, they include everything to do with analytics, automation, and data analysis. Marvin Minsky, the pioneer of artificial intelligence research in the 1960s, talked about AI as a “suitcase word”—a term into which you can stuff whatever you want—and that still seems to be the case. We are comfortable with that because we think companies should use all the capabilities of more traditional analysis while increasing automation in strategy that can free up management or analyst time and, gradually, introducing tools that can augment human thinking.
Joanna Pachner: AI has been embraced by many business functions, but strategy seems to be largely immune to its charms. Why do you think that is?
Subscribe to the Inside the Strategy Room podcast
Yuval Atsmon: You’re right about the limited adoption. Only 7 percent of respondents to our survey about the use of AI say they use it in strategy or even financial planning, whereas in areas like marketing, supply chain, and service operations, it’s 25 or 30 percent. One reason adoption is lagging is that strategy is one of the most integrative conceptual practices. When executives think about strategy automation, many are looking too far ahead—at AI capabilities that would decide, in place of the business leader, what the right strategy is. They are missing opportunities to use AI in the building blocks of strategy that could significantly improve outcomes.
I like to use the analogy to virtual assistants. Many of us use Alexa or Siri but very few people use these tools to do more than dictate a text message or shut off the lights. We don’t feel comfortable with the technology’s ability to understand the context in more sophisticated applications. AI in strategy is similar: it’s hard for AI to know everything an executive knows, but it can help executives with certain tasks.
When executives think about strategy automation, many are looking too far ahead—at AI deciding the right strategy. They are missing opportunities to use AI in the building blocks of strategy.
Joanna Pachner: What kind of tasks can AI help strategists execute today?
Yuval Atsmon: We talk about six stages of AI development. The earliest is simple analytics, which we refer to as descriptive intelligence. Companies use dashboards for competitive analysis or to study performance in different parts of the business that are automatically updated. Some have interactive capabilities for refinement and testing.
The second level is diagnostic intelligence, which is the ability to look backward at the business and understand root causes and drivers of performance. The level after that is predictive intelligence: being able to anticipate certain scenarios or options and the value of things in the future based on momentum from the past as well as signals picked in the market. Both diagnostics and prediction are areas that AI can greatly improve today. The tools can augment executives’ analysis and become areas where you develop capabilities. For example, on diagnostic intelligence, you can organize your portfolio into segments to understand granularly where performance is coming from and do it in a much more continuous way than analysts could. You can try 20 different ways in an hour versus deploying one hundred analysts to tackle the problem.
Predictive AI is both more difficult and more risky. Executives shouldn’t fully rely on predictive AI, but it provides another systematic viewpoint in the room. Because strategic decisions have significant consequences, a key consideration is to use AI transparently in the sense of understanding why it is making a certain prediction and what extrapolations it is making from which information. You can then assess if you trust the prediction or not. You can even use AI to track the evolution of the assumptions for that prediction.
Those are the levels available today. The next three levels will take time to develop. There are some early examples of AI advising actions for executives’ consideration that would be value-creating based on the analysis. From there, you go to delegating certain decision authority to AI, with constraints and supervision. Eventually, there is the point where fully autonomous AI analyzes and decides with no human interaction.
Because strategic decisions have significant consequences, you need to understand why AI is making a certain prediction and what extrapolations it’s making from which information.
Joanna Pachner: What kind of businesses or industries could gain the greatest benefits from embracing AI at its current level of sophistication?
Yuval Atsmon: Every business probably has some opportunity to use AI more than it does today. The first thing to look at is the availability of data. Do you have performance data that can be organized in a systematic way? Companies that have deep data on their portfolios down to business line, SKU, inventory, and raw ingredients have the biggest opportunities to use machines to gain granular insights that humans could not.
Companies whose strategies rely on a few big decisions with limited data would get less from AI. Likewise, those facing a lot of volatility and vulnerability to external events would benefit less than companies with controlled and systematic portfolios, although they could deploy AI to better predict those external events and identify what they can and cannot control.
Third, the velocity of decisions matters. Most companies develop strategies every three to five years, which then become annual budgets. If you think about strategy in that way, the role of AI is relatively limited other than potentially accelerating analyses that are inputs into the strategy. However, some companies regularly revisit big decisions they made based on assumptions about the world that may have since changed, affecting the projected ROI of initiatives. Such shifts would affect how you deploy talent and executive time, how you spend money and focus sales efforts, and AI can be valuable in guiding that. The value of AI is even bigger when you can make decisions close to the time of deploying resources, because AI can signal that your previous assumptions have changed from when you made your plan.
Joanna Pachner: Can you provide any examples of companies employing AI to address specific strategic challenges?
Yuval Atsmon: Some of the most innovative users of AI, not coincidentally, are AI- and digital-native companies. Some of these companies have seen massive benefits from AI and have increased its usage in other areas of the business. One mobility player adjusts its financial planning based on pricing patterns it observes in the market. Its business has relatively high flexibility to demand but less so to supply, so the company uses AI to continuously signal back when pricing dynamics are trending in a way that would affect profitability or where demand is rising. This allows the company to quickly react to create more capacity because its profitability is highly sensitive to keeping demand and supply in equilibrium.
Joanna Pachner: Given how quickly things change today, doesn’t AI seem to be more a tactical than a strategic tool, providing time-sensitive input on isolated elements of strategy?
Yuval Atsmon: It’s interesting that you make the distinction between strategic and tactical. Of course, every decision can be broken down into smaller ones, and where AI can be affordably used in strategy today is for building blocks of the strategy. It might feel tactical, but it can make a massive difference. One of the world’s leading investment firms, for example, has started to use AI to scan for certain patterns rather than scanning individual companies directly. AI looks for consumer mobile usage that suggests a company’s technology is catching on quickly, giving the firm an opportunity to invest in that company before others do. That created a significant strategic edge for them, even though the tool itself may be relatively tactical.
Joanna Pachner: McKinsey has written a lot about cognitive biases and social dynamics that can skew decision making. Can AI help with these challenges?
Yuval Atsmon: When we talk to executives about using AI in strategy development, the first reaction we get is, “Those are really big decisions; what if AI gets them wrong?” The first answer is that humans also get them wrong—a lot. [Amos] Tversky, [Daniel] Kahneman, and others have proven that some of those errors are systemic, observable, and predictable. The first thing AI can do is spot situations likely to give rise to biases. For example, imagine that AI is listening in on a strategy session where the CEO proposes something and everyone says “Aye” without debate and discussion. AI could inform the room, “We might have a sunflower bias here,” which could trigger more conversation and remind the CEO that it’s in their own interest to encourage some devil’s advocacy.
We also often see confirmation bias, where people focus their analysis on proving the wisdom of what they already want to do, as opposed to looking for a fact-based reality. Just having AI perform a default analysis that doesn’t aim to satisfy the boss is useful, and the team can then try to understand why that is different than the management hypothesis, triggering a much richer debate.
In terms of social dynamics, agency problems can create conflicts of interest. Every business unit [BU] leader thinks that their BU should get the most resources and will deliver the most value, or at least they feel they should advocate for their business. AI provides a neutral way based on systematic data to manage those debates. It’s also useful for executives with decision authority, since we all know that short-term pressures and the need to make the quarterly and annual numbers lead people to make different decisions on the 31st of December than they do on January 1st or October 1st. Like the story of Ulysses and the sirens, you can use AI to remind you that you wanted something different three months earlier. The CEO still decides; AI can just provide that extra nudge.
Joanna Pachner: It’s like you have Spock next to you, who is dispassionate and purely analytical.
Yuval Atsmon: That is not a bad analogy—for Star Trek fans anyway.
Joanna Pachner: Do you have a favorite application of AI in strategy?
Yuval Atsmon: I have worked a lot on resource allocation, and one of the challenges, which we call the hockey stick phenomenon, is that executives are always overly optimistic about what will happen. They know that resource allocation will inevitably be defined by what you believe about the future, not necessarily by past performance. AI can provide an objective prediction of performance starting from a default momentum case: based on everything that happened in the past and some indicators about the future, what is the forecast of performance if we do nothing? This is before we say, “But I will hire these people and develop this new product and improve my marketing”— things that every executive thinks will help them overdeliver relative to the past. The neutral momentum case, which AI can calculate in a cold, Spock-like manner, can change the dynamics of the resource allocation discussion. It’s a form of predictive intelligence accessible today and while it’s not meant to be definitive, it provides a basis for better decisions.
Joanna Pachner: Do you see access to technology talent as one of the obstacles to the adoption of AI in strategy, especially at large companies?
Yuval Atsmon: I would make a distinction. If you mean machine-learning and data science talent or software engineers who build the digital tools, they are definitely not easy to get. However, companies can increasingly use platforms that provide access to AI tools and require less from individual companies. Also, this domain of strategy is exciting—it’s cutting-edge, so it’s probably easier to get technology talent for that than it might be for manufacturing work.
The bigger challenge, ironically, is finding strategists or people with business expertise to contribute to the effort. You will not solve strategy problems with AI without the involvement of people who understand the customer experience and what you are trying to achieve. Those who know best, like senior executives, don’t have time to be product managers for the AI team. An even bigger constraint is that, in some cases, you are asking people to get involved in an initiative that may make their jobs less important. There could be plenty of opportunities for incorporating AI into existing jobs, but it’s something companies need to reflect on. The best approach may be to create a digital factory where a different team tests and builds AI applications, with oversight from senior stakeholders.
The big challenge is finding strategists to contribute to the AI effort. You are asking people to get involved in an initiative that may make their jobs less important.
Joanna Pachner: Do you think this worry about job security and the potential that AI will automate strategy is realistic?
Yuval Atsmon: The question of whether AI will replace human judgment and put humanity out of its job is a big one that I would leave for other experts.
The pertinent question is shorter-term automation. Because of its complexity, strategy would be one of the later domains to be affected by automation, but we are seeing it in many other domains. However, the trend for more than two hundred years has been that automation creates new jobs, although ones requiring different skills. That doesn’t take away the fear some people have of a machine exposing their mistakes or doing their job better than they do it.
Joanna Pachner: We recently published an article about strategic courage in an age of volatility that talked about three types of edge business leaders need to develop. One of them is an edge in insights. Do you think AI has a role to play in furnishing a proprietary insight edge?
Yuval Atsmon: One of the challenges most strategists face is the overwhelming complexity of the world we operate in—the number of unknowns, the information overload. At one level, it may seem that AI will provide another layer of complexity. In reality, it can be a sharp knife that cuts through some of the clutter. The question to ask is, Can AI simplify my life by giving me sharper, more timely insights more easily?
Joanna Pachner: You have been working in strategy for a long time. What sparked your interest in exploring this intersection of strategy and new technology?
Yuval Atsmon: I have always been intrigued by things at the boundaries of what seems possible. Science fiction writer Arthur C. Clarke’s second law is that to discover the limits of the possible, you have to venture a little past them into the impossible, and I find that particularly alluring in this arena.
AI in strategy is in very nascent stages but could be very consequential for companies and for the profession. For a top executive, strategic decisions are the biggest way to influence the business, other than maybe building the top team, and it is amazing how little technology is leveraged in that process today. It’s conceivable that competitive advantage will increasingly rest in having executives who know how to apply AI well. In some domains, like investment, that is already happening, and the difference in returns can be staggering. I find helping companies be part of that evolution very exciting.
Explore a career with us
Related articles.

Strategic courage in an age of volatility
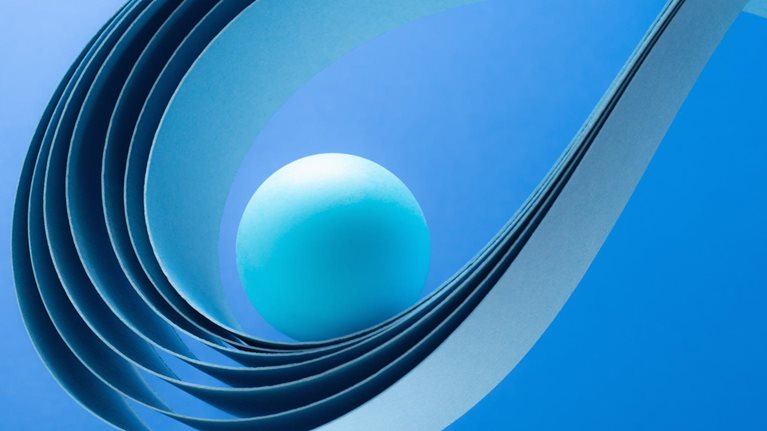
Bias Busters Collection

Using Dimensions grant data for strengthening research futures
Dimensions’ extensive grant data allows a variety of stakeholders: government and funders, academic institutions and researchers to have a holistic view of research funding dynamics across the entire research lifecycle, from inception to impact.
When Dimensions began aggregating grant data in 2013, the aim was to democratize access to different aspects of the research process, including information about research funding. At the time of writing in May 2024, the Dimensions grant data included details of 7.1million grants, totaling USD 2.6 trillion in funding.
Indicators of emerging trends and a global picture of funding
Funded grants offer a glimpse into the future of research, providing insights into the trajectory of scientific inquiry and innovation. One can view grant data as markers of emerging trends, indicating the direction in which various fields are headed. Take for example the field of next-generation sequencing, or NGS , a range of modern sequencing methods revolutionizing the pace of discovery in fields ranging from molecular biology to genomics. Dimensions data unveils the dynamics of financial support from both private and public funders and shows that grants began surfacing around 2008-2009 for research on the topic. These are the kinds of insights that could aid in predicting the trajectory of emerging technologies, enabling companies to better strategize their patent applications and market entry.
Because the Dimensions grant data is extensive and interconnected, it offers a comprehensive perspective on the global resource allocation within fields of research. For a study titled Prospective Research Trend Analysis on Zero-Energy Building (ZEB): An Artificial Intelligence Approach , authors used Dimensions “due to its ability to organize and provide considerable global R&D grant data systematically. “The research category feature offered by Dimensions.ai was used to narrow down data collection to research fields relevant to ZEB to reduce noise in the collected data,” they write.
Pointers for interventions and strategic-decision-making
In addition, by analyzing the projects slated for funding, stakeholders gain invaluable foresight, allowing for interventions where necessary and strategic decision-making. Take for instance a study published in the Lancet . Described as the “first comprehensive global analysis of cancer research funding,” covering approximately $24.5 billion of global investment, drawn from 66,388 public and philanthropic awards,” it was based on content analysis of research award data from 2016 to 2020 extracted from the Dimensions database. By analyzing funding flows from public and philanthropic awards for cancer research, researchers unearthed multiple disconnects between funding distribution and real-world needs. Other examples of publications that have used Dimensions grant data to understand trends, include Using a Modern Linked Research Database to Examine Gender Disparities in Orthopaedic Grant Funding from 2010 to 2022 and Global trends in psycho-oncology research investments 2016–2020: A content analysis .
Funded grants are crucial components of the research ecosystem, representing not just financial investment but also the collective trust in the vision and capability of researchers and the topic under investigation. Each grant awarded is the culmination of a rigorous process, where researchers present their ideas to funding bodies, seeking validation and support. Historically, agencies lacked insights into each other’s funding activities, hindering collaboration and synergy. However, with data sources like Dimensions, linking and standardizing data across disaggregated systems has become feasible. Dimensions aggregates not just grants but also publications, citations, clinical trials, patents, and policy papers. Advanced techniques such as natural language processing and machine learning facilitate the connection of research metadata, including researcher profiles, grants, and publications of various types.
For more information download the Dimensions Report: A Guide to the Dimensions Data Approach If you want to learn about how you can use Dimensions to access and analyze grant data, contact the Dimensions team .
Does cancer research funding need a reset?
Providing valuable data on funding flows to academia for market model development, how to use dimensions ‘similar documents’ search to discover funding trends.
- Election 2024
- Entertainment
- Newsletters
- Photography
- Personal Finance
- AP Investigations
- AP Buyline Personal Finance
- AP Buyline Shopping
- Press Releases
- Israel-Hamas War
- Russia-Ukraine War
- Global elections
- Asia Pacific
- Latin America
- Middle East
- Election Results
- Delegate Tracker
- AP & Elections
- Auto Racing
- 2024 Paris Olympic Games
- Movie reviews
- Book reviews
- Personal finance
- Financial Markets
- Business Highlights
- Financial wellness
- Artificial Intelligence
- Social Media
The larger the nonprofit, the more likely it is run by a white man, says new Candid diversity report
Candid CEO Ann Mei Chang poses for a photo at the nonprofit’s headquarters on Wednesday, Jan. 31, 2024, in New York. Chang, CEO since 2021, believes her organization can help the philanthropic sector work more efficiently by making more data from donors and grantees available to the public.(AP Photo/Peter K. Afriyie)
Candid CEO Ann Mei Chang poses for a photo at the nonprofits’s headquarters on Wednesday, Jan. 31, 2024, in New York. Chang, CEO since 2021, believes her organization can help the philanthropic sector work more efficiently by making more data from donors and grantees available to the public. (AP Photo/Peter K. Afriyie)
- Copy Link copied

NEW YORK (AP) — White men are most likely to lead the largest, best-funded nonprofits, while women of color tend to lead the organizations with the fewest financial resources, according to a study from the nonprofit data research organization Candid.
“ The State of Diversity in the U.S. Nonprofit Sector ” report released by Candid on Thursday is the largest demographic study of the nonprofit sector, based on diversity information provided by nearly 60,000 public charities.
According to the study, white CEOs lead 74% of organizations with more than $25 million in annual revenue, with white men heading 41% of those nonprofits, despite being only about 30% of the population. Women of color, who make up about 20% of the U.S. population, lead 14% of the organizations with more than $25 million in revenue and 28% of the smallest nonprofits — those with less than $50,000 in revenue.
The Candid report provides data for nonprofits who have complained for years that minority-led nonprofits attract fewer donations, government resources and sales, even after the racial reckoning following the murder of George Floyd and promises from funders of all sizes seeking change. Many groups argue that when the leadership of a charity comes from the community it is serving, its needs are met more effectively. According to a report from the Ms. Foundation for Women and the consulting group Strength in Numbers, less than 1% of the $67 billion that foundations donated in 2017 was earmarked specifically for minority women and girls.
“Our mission is to use data to help make the whole sector more efficient, effective and equitable,” Candid CEO Ann Mei Chang told The Associated Press. “We think that data is a force for good and can help everybody trying to do good, to do good better.”
The report’s findings are based on data gathered from the Demographics via Candid initiative, where nonprofits voluntarily report the diversity numbers of their organizations. Cathleen Clerkin, Candid’s associate vice president of research, said authors of the report compared its findings to other sector-wide data and found them to be consistent.
Because the diversity information was self-reported, Clerkin said Candid studied whether nonprofits would be more likely to share their information because they were more diverse, but found that was not the case. What was more likely to determine whether a nonprofit reported its diversity information was how much they depended on outside donations, said Clerkin, adding that Candid hopes the report will encourage more charities to provide its organization’s information.
The report found that environmental and animal welfare groups were least likely to have diverse leadership, with 88% having a white CEO. Nearly three-quarters of religious nonprofits had white CEOs, according to the report.
Portia Allen-Kyle, chief of staff and interim head of external affairs at the racial justice nonprofit Color of Change, said the report’s findings were not surprising. “The backsliding of Black leadership and other underrepresented populations is exactly what we unfortunately expect to see in an era of attacks on the tools of Black power like affirmative action, like DEI (diversity, equity and inclusion), et cetera,” she said. ”It’s a nonprofit space where disproportionately white leaders disproportionately receive resources from these white, ultrawealthy donors, while Black leaders from the most impacted communities are expected to often turn water into wine, using nothing but pennies on the dollar.”
Allen-Kyle said the fact that the report also finds that women of color are overrepresented as leaders of the smallest charities is also not a surprise. “With these small nonprofits, especially with advocacy, Black women are going to be doing this work regardless and they’re doing it on nothing and whether or not they get paid because they believe in it,” she said.
The report also found that Latinos were underrepresented as nonprofit CEOs in nearly every state.
“We have been talking about that for decades,” said Frankie Miranda, president and CEO of the Hispanic Federation, which supports Latino communities and nonprofits. “It’s the reason the Hispanic Federation was created in 1990 — to advocate for Latino-led, Latino-serving providers because we were not part of the conversation when decision-making around funding and support was happening.”
That has led to Hispanic Federation becoming one of the nation’s largest grantmakers for Latino nonprofits. However, even though its findings are not unexpected, the Candid study is still extraordinarily valuable, Miranda said.
“This study will validate our argument,” he said. “This is critically important for us to be able to say, ‘Here’s the proof.’ It’s proof for major donors that you need to do better when it comes to diversity within your organization. Your institution needs to have the cultural competency to understand the importance of investing in our organizations, the importance of getting to know these organizations. They know how to serve these communities.”
Associated Press coverage of philanthropy and nonprofits receives support through the AP’s collaboration with The Conversation US, with funding from Lilly Endowment Inc. The AP is solely responsible for this content. For all of AP’s philanthropy coverage, visit https://apnews.com/hub/philanthropy .
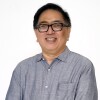
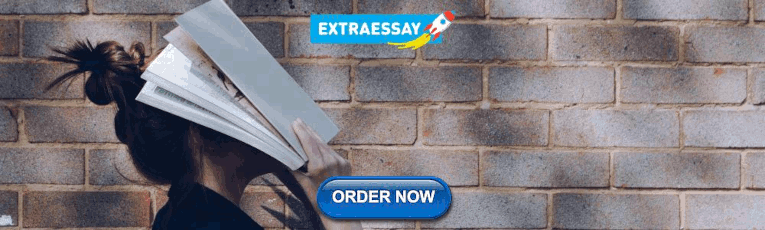
IMAGES
VIDEO
COMMENTS
3 One day of current option trading data alone is roughly two terabytes. In the 2019 NBER-RFS Summer Conference on Big Data supported by the same NSF grant, the chief economist of the U.S. Securities and Exchange Commission (SEC), S. P. Kothari, pointed out that one of the biggest data collection efforts in finance is the Consolidated Audit Trial (CAT), which provides a single, comprehensive ...
The connection between big data and financial-related components will be revealed in an exploratory literature review of secondary data sources. Since big data in the financial field is an extremely new concept, future research directions will be pointed out at the end of this study.
Big Data in Finance. Itay Goldstein, Chester S. Spatt & Mao Ye. Working Paper 28615. DOI 10.3386/w28615. Issue Date March 2021. Big data is revolutionizing the finance industry and has the potential to significantly shape future research in finance. This special issue contains articles following the 2019 NBER/ RFS conference on big data.
series of NBER conferences to explore the future of big data research in finance. The summer conferences, organized by Toni Whited and Mao Ye, focus on tutorial sessions on big data ... Review of Financial Studies (RFS) on big data in finance include s four papers from the first NBER/RFS Winter Conference on B ig Data held on March 8, 2019, and
In conjunction with big data, algorithmic trading is thus resulting in highly optimized insights for traders to maximize their portfolio returns. 2. Big data analytics in financial models. Big data analytics presents an exciting opportunity to improve predictive modeling to better estimate the rates of return and outcomes on investments. Access ...
In the financial sector, the big data movement refers to the analysis of vast amounts of data with the goal of making better informed investment decisions, improving corporate operations, and enhancing decision-making processes on both the buy and supply sides of transactions (Hasan et al., 2020).Big data analysis frequently draws on artificial intelligence (AI) models and has created a ...
The digital age has created mountains of data that continue to grow exponentially. The International Data Corporation estimates that the world generates more data every two days than all of humanity generated from the dawn of time to the year 2003. This "big data" revolution is reshaping the financial industry.
This study provides insights on trends and future scope of big data in finance. As a result, sub-sets of big data in fiancé are identified namely artificial intelligence, credit-rating, financial reporting financial crisis, stock trading, assets-pricing, portfolio optimization, banking & insurance and auditing.
By conducting co-occurrence network analysis on 52 peer-reviewed articles published from 2010 to 2020, three broad themes emerged, entailing big data implications for accounting practice, education, and research design. A further examination of the themes revealed few empirical studies on the phenomenon, as conceptual research dominates the field.
While the general use of big data has been the subject of frequent discussions, this book will take a more focused look at big data applications in the financial sector. With contributions from researchers, practitioners, and entrepreneurs involved at the forefront of big data in finance, the book discusses technological and business-inspired ...
The research question addressed in this work is: What are the major themes in existing research in big data and where are the resulting gaps in the accounting and finance literature? An analysis is presented of 47 accounting, finance and information systems journals from 2007-2016. We identify and sample the relevant literature to derive a ...
In response to the rapid growth of research on big data, ... Banks are introducing new ways to advertise their products and services to facilitate customers' improved financial decisions. Big data composes a crucial role in customer retention and investing in new features to attract more customers for banks. Recent trends show that big data ...
Finance big data (FBD) is becoming one of the most promising areas of management and governance in the financial sector. It is significantly changing business models in financial companies. Many researchers argue that Big Data is fueling the transformation of finance and business at-large in the ways that we cannot as yet assess.
The "V's" of big data in finance are the fundamentals of big data in finance. The 4 main V's are: Volume: Financial institutions generate massive volumes of data daily, including transaction records, customer information, market data, and more. Managing and processing this large data volume is a fundamental challenge.
This paper reviews research that uses big data and/or machine learning methods to provide insight relevant for equity valuation. Given the huge volume of research in this area, the review focuses on studies that either use or inform on accounting variables. ... Section 2 describes studies that use big data or ML methods to predict financial ...
1 Artificial Intelligence, Machine Learning and Big data in Financial Services 15 1.1. Introduction 15 1.2. AI systems, ML and the use of big data 16 ... Growth in AI-related research and investment in AI start-ups 19 Figure 2.1. Examples of AI applications in some financial market activities 21 Figure 2.2. AI use by hedge funds (H1 2018) 22
Financial experts and academics are increasingly interested in developing big data financial risk prevention and control capabilities based on cutting-edge technologies like big data, machine learning (ML), and neural networks (NN), as well as accelerating the implementation of intelligent risk prevention and control platforms. This research ...
1.1. Financial risks in the big data era. According to the "2020 China Internet Finance Development Report" issued by iResearch 1, investment in financial technologies was 112 billion yuan, an increase of 19.4%, whereas investment in AI in banking was 14.3 billion yuan, an increase of 28.8%.
These meetings principally focused on the identification of problems connected with big data and similarly, aimed at identifying the potential influence of big data on financial accounting. The scientific methods were primarily based on design thinking research and human-centered design, methods best suited for research using data from interviews.
Big Data has become the norm in recent years; accountants and other decision-makers have struggled to analyze massive amounts of data. This limits their capacity to profit from such data even more. Therefore, this study is motivated by the lack of research on Big Data's influence on financial report quality.
The rise of Big Data brings the financial innovation opportunities as well as challenges. This paper reviews different fields of big-data-based financial innovations as well as the scientific discoveries and theoretical breakthroughs of risk analysis with respect to these financial innovations. Based on the current research status, several key problems are put forward and their relative ...
The potential value of open financial data ranges from 1 percent to as much as 5 percent of GDP, depending on economic structure and levels of financial access. Aggregating the potential GDP impact across the 24 use cases to the economy level, we find significant value at stake overall and for all market participants.
What is big data analytics? Big data analytics refers to the systematic processing and analysis of large amounts of data and complex data sets, known as big data, to extract valuable insights. Big data analytics allows for the uncovering of trends, patterns and correlations in large amounts of raw data to help analysts make data-informed decisions.
, A long short-term memory network stock price prediction with leading indicators, Big Data 9 (5) (2021) 343 - 357. Google Scholar [24] Luo S. , Tian C. , Financial high-frequency time series forecasting based on sub-step grid search long short-term memory network , IEEE Access 8 ( 2020 ) 203183 - 203189 .
A data scientist uses data to understand and explain the phenomena around them, and help organizations make better decisions. Working as a data scientist can be intellectually challenging, analytically satisfying, and put you at the forefront of new technological advances. Data scientists have become more common and in demand, as big data ...
The Consumer Financial Protection Bureau's push to allow consumers to easily share their financial data will only entrench big banks' power unless the agency makes it simpler for fintechs, among others, to use the data for research, advocates say. The open banking proposal, mandated by the 2010 Dodd-Frank Act and released in October, would ...
At the start of the year, Meta announced a new $800mn data centre in Indiana. Alphabet is planning a $3bn project to set up a data centre campus in Indiana and expand capacity in Virginia ...
Yuval Atsmon: When people talk about artificial intelligence, they include everything to do with analytics, automation, and data analysis. Marvin Minsky, the pioneer of artificial intelligence research in the 1960s, talked about AI as a "suitcase word"—a term into which you can stuff whatever you want—and that still seems to be the case.
When Dimensions began aggregating grant data in 2013, the aim was to democratize access to different aspects of the research process, including information about research funding. At the time of writing in May 2024, the Dimensions grant data included details of 7.1million grants, totaling USD 2.6 trillion in funding. Grants.
White men are most likely to lead the largest, best-funded nonprofits, while women of color tend to lead the organizations with the fewest financial resources, according to a study from the nonprofit data research organization Candid released Thursday.