Yale Economic Growth Center
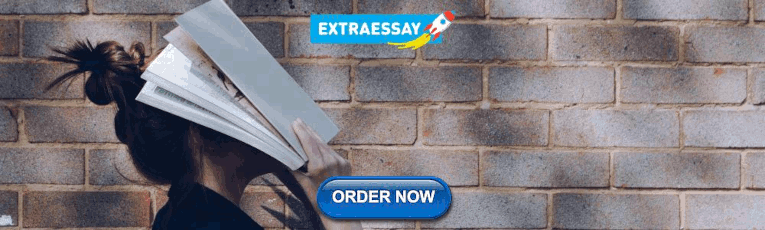
Agte et al.: "Gender Gaps and Economic Growth: Why Haven't Women Won Globally (Yet)?"
EGC Discussion Paper No. 1105, May, 2024, by members of the Gender and Growth Gaps project team: Patrick Agte, Orazio Attanasio, Pinelopi K. Goldberg, Aishwarya Lakshmi Ratan, Rohini Pande, Michael Peters, Charity Troyer Moore, and Fabrizio Zilibotti.
Does economic growth close labor market-linked gender gaps that disadvantage women? Conversely, do gender inequalities in the labor market impede growth? To inform these questions, we conduct two analyses. First, we estimate regressions using data on gender gaps in a range of labor market outcomes from 153 countries spanning two decades (1998-2018). Second, we conduct a systematic review of the recent economics literature on gender gaps in labor markets, examining 16 journals over 21 years. Our empirical analysis demonstrates that growth is not a panacea. While economic gender gaps have narrowed and growth is associated with gender gap closures specifically in incidence of paid work, the relationship between growth and labor market gaps is otherwise mixed, and results vary by specification. This result reflects, in part, the gendered nature of structural transformation, in which growth leads men to transition from agriculture to industry and services while many women exit the labor force. Disparities in hours worked and wages persist despite growth, and heterogeneity in trends and levels between regions highlight the importance of local institutions. To better understand whether gender inequalities impeded growth, we explore a nascent literature that shows that reducing gender gaps in labor markets increases aggregate productivity. Our broader review highlights how traditional explanations for gender differences do not adequately explain existing gaps and how policy responses need to be sensitive to the changing nature of economic growth. We conclude by posing open questions for future research.
P. Agte, O. Attanasio, P. Goldberg, A. Lakshmi Ratan, R. Pande, M. Peters, C. Troyer Moore, and F. Zilibotti. "Gender Gaps and Economic Growth: Why Haven't Women Won Globally (Yet)?" EGC Discussion Paper No. 1105.
View Publication
- EGC Discussion Papers on EliScholar
Report | Wages, Incomes, and Wealth
“Women’s work” and the gender pay gap : How discrimination, societal norms, and other forces affect women’s occupational choices—and their pay
Report • By Jessica Schieder and Elise Gould • July 20, 2016
Download PDF
Press release
Share this page:
What this report finds: Women are paid 79 cents for every dollar paid to men—despite the fact that over the last several decades millions more women have joined the workforce and made huge gains in their educational attainment. Too often it is assumed that this pay gap is not evidence of discrimination, but is instead a statistical artifact of failing to adjust for factors that could drive earnings differences between men and women. However, these factors—particularly occupational differences between women and men—are themselves often affected by gender bias. For example, by the time a woman earns her first dollar, her occupational choice is the culmination of years of education, guidance by mentors, expectations set by those who raised her, hiring practices of firms, and widespread norms and expectations about work–family balance held by employers, co-workers, and society. In other words, even though women disproportionately enter lower-paid, female-dominated occupations, this decision is shaped by discrimination, societal norms, and other forces beyond women’s control.
Why it matters, and how to fix it: The gender wage gap is real—and hurts women across the board by suppressing their earnings and making it harder to balance work and family. Serious attempts to understand the gender wage gap should not include shifting the blame to women for not earning more. Rather, these attempts should examine where our economy provides unequal opportunities for women at every point of their education, training, and career choices.
Introduction and key findings
Women are paid 79 cents for every dollar paid to men (Hegewisch and DuMonthier 2016). This is despite the fact that over the last several decades millions more women have joined the workforce and made huge gains in their educational attainment.
Critics of this widely cited statistic claim it is not solid evidence of economic discrimination against women because it is unadjusted for characteristics other than gender that can affect earnings, such as years of education, work experience, and location. Many of these skeptics contend that the gender wage gap is driven not by discrimination, but instead by voluntary choices made by men and women—particularly the choice of occupation in which they work. And occupational differences certainly do matter—occupation and industry account for about half of the overall gender wage gap (Blau and Kahn 2016).
To isolate the impact of overt gender discrimination—such as a woman being paid less than her male coworker for doing the exact same job—it is typical to adjust for such characteristics. But these adjusted statistics can radically understate the potential for gender discrimination to suppress women’s earnings. This is because gender discrimination does not occur only in employers’ pay-setting practices. It can happen at every stage leading to women’s labor market outcomes.
Take one key example: occupation of employment. While controlling for occupation does indeed reduce the measured gender wage gap, the sorting of genders into different occupations can itself be driven (at least in part) by discrimination. By the time a woman earns her first dollar, her occupational choice is the culmination of years of education, guidance by mentors, expectations set by those who raised her, hiring practices of firms, and widespread norms and expectations about work–family balance held by employers, co-workers, and society. In other words, even though women disproportionately enter lower-paid, female-dominated occupations, this decision is shaped by discrimination, societal norms, and other forces beyond women’s control.
This paper explains why gender occupational sorting is itself part of the discrimination women face, examines how this sorting is shaped by societal and economic forces, and explains that gender pay gaps are present even within occupations.
Key points include:
- Gender pay gaps within occupations persist, even after accounting for years of experience, hours worked, and education.
- Decisions women make about their occupation and career do not happen in a vacuum—they are also shaped by society.
- The long hours required by the highest-paid occupations can make it difficult for women to succeed, since women tend to shoulder the majority of family caretaking duties.
- Many professions dominated by women are low paid, and professions that have become female-dominated have become lower paid.
This report examines wages on an hourly basis. Technically, this is an adjusted gender wage gap measure. As opposed to weekly or annual earnings, hourly earnings ignore the fact that men work more hours on average throughout a week or year. Thus, the hourly gender wage gap is a bit smaller than the 79 percent figure cited earlier. This minor adjustment allows for a comparison of women’s and men’s wages without assuming that women, who still shoulder a disproportionate amount of responsibilities at home, would be able or willing to work as many hours as their male counterparts. Examining the hourly gender wage gap allows for a more thorough conversation about how many factors create the wage gap women experience when they cash their paychecks.
Within-occupation gender wage gaps are large—and persist after controlling for education and other factors
Those keen on downplaying the gender wage gap often claim women voluntarily choose lower pay by disproportionately going into stereotypically female professions or by seeking out lower-paid positions. But even when men and women work in the same occupation—whether as hairdressers, cosmetologists, nurses, teachers, computer engineers, mechanical engineers, or construction workers—men make more, on average, than women (CPS microdata 2011–2015).
As a thought experiment, imagine if women’s occupational distribution mirrored men’s. For example, if 2 percent of men are carpenters, suppose 2 percent of women become carpenters. What would this do to the wage gap? After controlling for differences in education and preferences for full-time work, Goldin (2014) finds that 32 percent of the gender pay gap would be closed.
However, leaving women in their current occupations and just closing the gaps between women and their male counterparts within occupations (e.g., if male and female civil engineers made the same per hour) would close 68 percent of the gap. This means examining why waiters and waitresses, for example, with the same education and work experience do not make the same amount per hour. To quote Goldin:
Another way to measure the effect of occupation is to ask what would happen to the aggregate gender gap if one equalized earnings by gender within each occupation or, instead, evened their proportions for each occupation. The answer is that equalizing earnings within each occupation matters far more than equalizing the proportions by each occupation. (Goldin 2014)
This phenomenon is not limited to low-skilled occupations, and women cannot educate themselves out of the gender wage gap (at least in terms of broad formal credentials). Indeed, women’s educational attainment outpaces men’s; 37.0 percent of women have a college or advanced degree, as compared with 32.5 percent of men (CPS ORG 2015). Furthermore, women earn less per hour at every education level, on average. As shown in Figure A , men with a college degree make more per hour than women with an advanced degree. Likewise, men with a high school degree make more per hour than women who attended college but did not graduate. Even straight out of college, women make $4 less per hour than men—a gap that has grown since 2000 (Kroeger, Cooke, and Gould 2016).
Women earn less than men at every education level : Average hourly wages, by gender and education, 2015
The data below can be saved or copied directly into Excel.
The data underlying the figure.
Source : EPI analysis of Current Population Survey Outgoing Rotation Group microdata
Copy the code below to embed this chart on your website.
Steering women to certain educational and professional career paths—as well as outright discrimination—can lead to different occupational outcomes
The gender pay gap is driven at least in part by the cumulative impact of many instances over the course of women’s lives when they are treated differently than their male peers. Girls can be steered toward gender-normative careers from a very early age. At a time when parental influence is key, parents are often more likely to expect their sons, rather than their daughters, to work in science, technology, engineering, or mathematics (STEM) fields, even when their daughters perform at the same level in mathematics (OECD 2015).
Expectations can become a self-fulfilling prophecy. A 2005 study found third-grade girls rated their math competency scores much lower than boys’, even when these girls’ performance did not lag behind that of their male counterparts (Herbert and Stipek 2005). Similarly, in states where people were more likely to say that “women [are] better suited for home” and “math is for boys,” girls were more likely to have lower math scores and higher reading scores (Pope and Sydnor 2010). While this only establishes a correlation, there is no reason to believe gender aptitude in reading and math would otherwise be related to geography. Parental expectations can impact performance by influencing their children’s self-confidence because self-confidence is associated with higher test scores (OECD 2015).
By the time young women graduate from high school and enter college, they already evaluate their career opportunities differently than young men do. Figure B shows college freshmen’s intended majors by gender. While women have increasingly gone into medical school and continue to dominate the nursing field, women are significantly less likely to arrive at college interested in engineering, computer science, or physics, as compared with their male counterparts.
Women arrive at college less interested in STEM fields as compared with their male counterparts : Intent of first-year college students to major in select STEM fields, by gender, 2014
Source: EPI adaptation of Corbett and Hill (2015) analysis of Eagan et al. (2014)
These decisions to allow doors to lucrative job opportunities to close do not take place in a vacuum. Many factors might make it difficult for a young woman to see herself working in computer science or a similarly remunerative field. A particularly depressing example is the well-publicized evidence of sexism in the tech industry (Hewlett et al. 2008). Unfortunately, tech isn’t the only STEM field with this problem.
Young women may be discouraged from certain career paths because of industry culture. Even for women who go against the grain and pursue STEM careers, if employers in the industry foster an environment hostile to women’s participation, the share of women in these occupations will be limited. One 2008 study found that “52 percent of highly qualified females working for SET [science, technology, and engineering] companies quit their jobs, driven out by hostile work environments and extreme job pressures” (Hewlett et al. 2008). Extreme job pressures are defined as working more than 100 hours per week, needing to be available 24/7, working with or managing colleagues in multiple time zones, and feeling pressure to put in extensive face time (Hewlett et al. 2008). As compared with men, more than twice as many women engage in housework on a daily basis, and women spend twice as much time caring for other household members (BLS 2015). Because of these cultural norms, women are less likely to be able to handle these extreme work pressures. In addition, 63 percent of women in SET workplaces experience sexual harassment (Hewlett et al. 2008). To make matters worse, 51 percent abandon their SET training when they quit their job. All of these factors play a role in steering women away from highly paid occupations, particularly in STEM fields.
The long hours required for some of the highest-paid occupations are incompatible with historically gendered family responsibilities
Those seeking to downplay the gender wage gap often suggest that women who work hard enough and reach the apex of their field will see the full fruits of their labor. In reality, however, the gender wage gap is wider for those with higher earnings. Women in the top 95th percentile of the wage distribution experience a much larger gender pay gap than lower-paid women.
Again, this large gender pay gap between the highest earners is partially driven by gender bias. Harvard economist Claudia Goldin (2014) posits that high-wage firms have adopted pay-setting practices that disproportionately reward individuals who work very long and very particular hours. This means that even if men and women are equally productive per hour, individuals—disproportionately men—who are more likely to work excessive hours and be available at particular off-hours are paid more highly (Hersch and Stratton 2002; Goldin 2014; Landers, Rebitzer, and Taylor 1996).
It is clear why this disadvantages women. Social norms and expectations exert pressure on women to bear a disproportionate share of domestic work—particularly caring for children and elderly parents. This can make it particularly difficult for them (relative to their male peers) to be available at the drop of a hat on a Sunday evening after working a 60-hour week. To the extent that availability to work long and particular hours makes the difference between getting a promotion or seeing one’s career stagnate, women are disadvantaged.
And this disadvantage is reinforced in a vicious circle. Imagine a household where both members of a male–female couple have similarly demanding jobs. One partner’s career is likely to be prioritized if a grandparent is hospitalized or a child’s babysitter is sick. If the past history of employer pay-setting practices that disadvantage women has led to an already-existing gender wage gap for this couple, it can be seen as “rational” for this couple to prioritize the male’s career. This perpetuates the expectation that it always makes sense for women to shoulder the majority of domestic work, and further exacerbates the gender wage gap.
Female-dominated professions pay less, but it’s a chicken-and-egg phenomenon
Many women do go into low-paying female-dominated industries. Home health aides, for example, are much more likely to be women. But research suggests that women are making a logical choice, given existing constraints . This is because they will likely not see a significant pay boost if they try to buck convention and enter male-dominated occupations. Exceptions certainly exist, particularly in the civil service or in unionized workplaces (Anderson, Hegewisch, and Hayes 2015). However, if women in female-dominated occupations were to go into male-dominated occupations, they would often have similar or lower expected wages as compared with their female counterparts in female-dominated occupations (Pitts 2002). Thus, many women going into female-dominated occupations are actually situating themselves to earn higher wages. These choices thereby maximize their wages (Pitts 2002). This holds true for all categories of women except for the most educated, who are more likely to earn more in a male profession than a female profession. There is also evidence that if it becomes more lucrative for women to move into male-dominated professions, women will do exactly this (Pitts 2002). In short, occupational choice is heavily influenced by existing constraints based on gender and pay-setting across occupations.
To make matters worse, when women increasingly enter a field, the average pay in that field tends to decline, relative to other fields. Levanon, England, and Allison (2009) found that when more women entered an industry, the relative pay of that industry 10 years later was lower. Specifically, they found evidence of devaluation—meaning the proportion of women in an occupation impacts the pay for that industry because work done by women is devalued.
Computer programming is an example of a field that has shifted from being a very mixed profession, often associated with secretarial work in the past, to being a lucrative, male-dominated profession (Miller 2016; Oldenziel 1999). While computer programming has evolved into a more technically demanding occupation in recent decades, there is no skills-based reason why the field needed to become such a male-dominated profession. When men flooded the field, pay went up. In contrast, when women became park rangers, pay in that field went down (Miller 2016).
Further compounding this problem is that many professions where pay is set too low by market forces, but which clearly provide enormous social benefits when done well, are female-dominated. Key examples range from home health workers who care for seniors, to teachers and child care workers who educate today’s children. If closing gender pay differences can help boost pay and professionalism in these key sectors, it would be a huge win for the economy and society.
The gender wage gap is real—and hurts women across the board. Too often it is assumed that this gap is not evidence of discrimination, but is instead a statistical artifact of failing to adjust for factors that could drive earnings differences between men and women. However, these factors—particularly occupational differences between women and men—are themselves affected by gender bias. Serious attempts to understand the gender wage gap should not include shifting the blame to women for not earning more. Rather, these attempts should examine where our economy provides unequal opportunities for women at every point of their education, training, and career choices.
— This paper was made possible by a grant from the Peter G. Peterson Foundation. The statements made and views expressed are solely the responsibility of the authors.
— The authors wish to thank Josh Bivens, Barbara Gault, and Heidi Hartman for their helpful comments.
About the authors
Jessica Schieder joined EPI in 2015. As a research assistant, she supports the research of EPI’s economists on topics such as the labor market, wage trends, executive compensation, and inequality. Prior to joining EPI, Jessica worked at the Center for Effective Government (formerly OMB Watch) as a revenue and spending policies analyst, where she examined how budget and tax policy decisions impact working families. She holds a bachelor’s degree in international political economy from Georgetown University.
Elise Gould , senior economist, joined EPI in 2003. Her research areas include wages, poverty, economic mobility, and health care. She is a co-author of The State of Working America, 12th Edition . In the past, she has authored a chapter on health in The State of Working America 2008/09; co-authored a book on health insurance coverage in retirement; published in venues such as The Chronicle of Higher Education , Challenge Magazine , and Tax Notes; and written for academic journals including Health Economics , Health Affairs, Journal of Aging and Social Policy, Risk Management & Insurance Review, Environmental Health Perspectives , and International Journal of Health Services . She holds a master’s in public affairs from the University of Texas at Austin and a Ph.D. in economics from the University of Wisconsin at Madison.
Anderson, Julie, Ariane Hegewisch, and Jeff Hayes 2015. The Union Advantage for Women . Institute for Women’s Policy Research.
Blau, Francine D., and Lawrence M. Kahn 2016. The Gender Wage Gap: Extent, Trends, and Explanations . National Bureau of Economic Research, Working Paper No. 21913.
Bureau of Labor Statistics (BLS). 2015. American Time Use Survey public data series. U.S. Census Bureau.
Corbett, Christianne, and Catherine Hill. 2015. Solving the Equation: The Variables for Women’s Success in Engineering and Computing . American Association of University Women (AAUW).
Current Population Survey Outgoing Rotation Group microdata (CPS ORG). 2011–2015. Survey conducted by the Bureau of the Census for the Bureau of Labor Statistics [ machine-readable microdata file ]. U.S. Census Bureau.
Goldin, Claudia. 2014. “ A Grand Gender Convergence: Its Last Chapter .” American Economic Review, vol. 104, no. 4, 1091–1119.
Hegewisch, Ariane, and Asha DuMonthier. 2016. The Gender Wage Gap: 2015; Earnings Differences by Race and Ethnicity . Institute for Women’s Policy Research.
Herbert, Jennifer, and Deborah Stipek. 2005. “The Emergence of Gender Difference in Children’s Perceptions of Their Academic Competence.” Journal of Applied Developmental Psychology , vol. 26, no. 3, 276–295.
Hersch, Joni, and Leslie S. Stratton. 2002. “ Housework and Wages .” The Journal of Human Resources , vol. 37, no. 1, 217–229.
Hewlett, Sylvia Ann, Carolyn Buck Luce, Lisa J. Servon, Laura Sherbin, Peggy Shiller, Eytan Sosnovich, and Karen Sumberg. 2008. The Athena Factor: Reversing the Brain Drain in Science, Engineering, and Technology . Harvard Business Review.
Kroeger, Teresa, Tanyell Cooke, and Elise Gould. 2016. The Class of 2016: The Labor Market Is Still Far from Ideal for Young Graduates . Economic Policy Institute.
Landers, Renee M., James B. Rebitzer, and Lowell J. Taylor. 1996. “ Rat Race Redux: Adverse Selection in the Determination of Work Hours in Law Firms .” American Economic Review , vol. 86, no. 3, 329–348.
Levanon, Asaf, Paula England, and Paul Allison. 2009. “Occupational Feminization and Pay: Assessing Causal Dynamics Using 1950-2000 U.S. Census Data.” Social Forces, vol. 88, no. 2, 865–892.
Miller, Claire Cain. 2016. “As Women Take Over a Male-Dominated Field, the Pay Drops.” New York Times , March 18.
Oldenziel, Ruth. 1999. Making Technology Masculine: Men, Women, and Modern Machines in America, 1870-1945 . Amsterdam: Amsterdam University Press.
Organisation for Economic Co-operation and Development (OECD). 2015. The ABC of Gender Equality in Education: Aptitude, Behavior, Confidence .
Pitts, Melissa M. 2002. Why Choose Women’s Work If It Pays Less? A Structural Model of Occupational Choice. Federal Reserve Bank of Atlanta, Working Paper 2002-30.
Pope, Devin G., and Justin R. Sydnor. 2010. “ Geographic Variation in the Gender Differences in Test Scores .” Journal of Economic Perspectives , vol. 24, no. 2, 95–108.
See related work on Wages, Incomes, and Wealth | Women
See more work by Jessica Schieder and Elise Gould
The Gender Wage Gap: Skills, Sorting, and Returns
Humphries, John Eric, Juanna Schrøter Joensen, and Gregory F. Veramendi. 2024. "The Gender Wage Gap: Skills, Sorting, and Returns." AEA Papers and Proceedings, 114: 259-64.

There is a large gender wage gap among college graduates. This gender gap could be partially driven by differences in college major and prior skills. We use Swedish register data to study how much of the gender gap can be explained by differences in majors, skills, and skill prices. College majors explain 60 percent of the gender wage gap, but large gaps remain within majors. We find that within-major wage gaps are driven by neither differences in multidimensional skills nor returns to these skills. In fact, women are positively selected in terms of college preparation and skills in almost every major.
Numbers, Facts and Trends Shaping Your World
Read our research on:
Full Topic List
Regions & Countries
- Publications
- Our Methods
- Short Reads
- Tools & Resources
Read Our Research On:
Gender pay gap in U.S. hasn’t changed much in two decades
The gender gap in pay has remained relatively stable in the United States over the past 20 years or so. In 2022, women earned an average of 82% of what men earned, according to a new Pew Research Center analysis of median hourly earnings of both full- and part-time workers. These results are similar to where the pay gap stood in 2002, when women earned 80% as much as men.
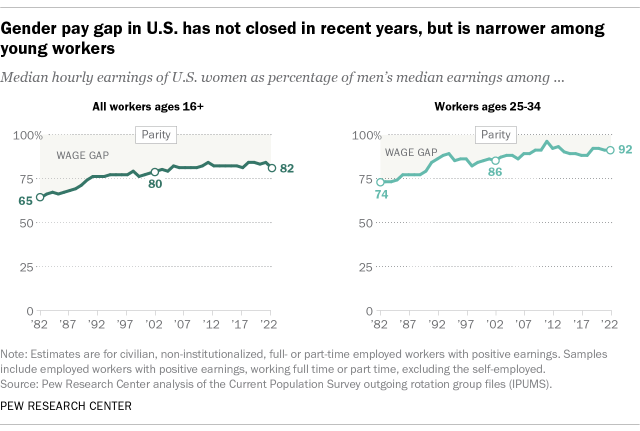
As has long been the case, the wage gap is smaller for workers ages 25 to 34 than for all workers 16 and older. In 2022, women ages 25 to 34 earned an average of 92 cents for every dollar earned by a man in the same age group – an 8-cent gap. By comparison, the gender pay gap among workers of all ages that year was 18 cents.
While the gender pay gap has not changed much in the last two decades, it has narrowed considerably when looking at the longer term, both among all workers ages 16 and older and among those ages 25 to 34. The estimated 18-cent gender pay gap among all workers in 2022 was down from 35 cents in 1982. And the 8-cent gap among workers ages 25 to 34 in 2022 was down from a 26-cent gap four decades earlier.
The gender pay gap measures the difference in median hourly earnings between men and women who work full or part time in the United States. Pew Research Center’s estimate of the pay gap is based on an analysis of Current Population Survey (CPS) monthly outgoing rotation group files ( IPUMS ) from January 1982 to December 2022, combined to create annual files. To understand how we calculate the gender pay gap, read our 2013 post, “How Pew Research Center measured the gender pay gap.”
The COVID-19 outbreak affected data collection efforts by the U.S. government in its surveys, especially in 2020 and 2021, limiting in-person data collection and affecting response rates. It is possible that some measures of economic outcomes and how they vary across demographic groups are affected by these changes in data collection.
In addition to findings about the gender wage gap, this analysis includes information from a Pew Research Center survey about the perceived reasons for the pay gap, as well as the pressures and career goals of U.S. men and women. The survey was conducted among 5,098 adults and includes a subset of questions asked only for 2,048 adults who are employed part time or full time, from Oct. 10-16, 2022. Everyone who took part is a member of the Center’s American Trends Panel (ATP), an online survey panel that is recruited through national, random sampling of residential addresses. This way nearly all U.S. adults have a chance of selection. The survey is weighted to be representative of the U.S. adult population by gender, race, ethnicity, partisan affiliation, education and other categories. Read more about the ATP’s methodology .
Here are the questions used in this analysis, along with responses, and its methodology .
The U.S. Census Bureau has also analyzed the gender pay gap, though its analysis looks only at full-time workers (as opposed to full- and part-time workers). In 2021, full-time, year-round working women earned 84% of what their male counterparts earned, on average, according to the Census Bureau’s most recent analysis.
Much of the gender pay gap has been explained by measurable factors such as educational attainment, occupational segregation and work experience. The narrowing of the gap over the long term is attributable in large part to gains women have made in each of these dimensions.
Related: The Enduring Grip of the Gender Pay Gap
Even though women have increased their presence in higher-paying jobs traditionally dominated by men, such as professional and managerial positions, women as a whole continue to be overrepresented in lower-paying occupations relative to their share of the workforce. This may contribute to gender differences in pay.
Other factors that are difficult to measure, including gender discrimination, may also contribute to the ongoing wage discrepancy.
Perceived reasons for the gender wage gap
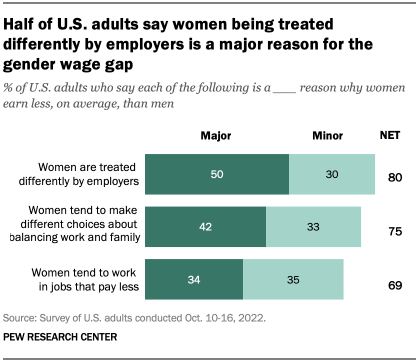
When asked about the factors that may play a role in the gender wage gap, half of U.S. adults point to women being treated differently by employers as a major reason, according to a Pew Research Center survey conducted in October 2022. Smaller shares point to women making different choices about how to balance work and family (42%) and working in jobs that pay less (34%).
There are some notable differences between men and women in views of what’s behind the gender wage gap. Women are much more likely than men (61% vs. 37%) to say a major reason for the gap is that employers treat women differently. And while 45% of women say a major factor is that women make different choices about how to balance work and family, men are slightly less likely to hold that view (40% say this).
Parents with children younger than 18 in the household are more likely than those who don’t have young kids at home (48% vs. 40%) to say a major reason for the pay gap is the choices that women make about how to balance family and work. On this question, differences by parental status are evident among both men and women.
Views about reasons for the gender wage gap also differ by party. About two-thirds of Democrats and Democratic-leaning independents (68%) say a major factor behind wage differences is that employers treat women differently, but far fewer Republicans and Republican leaners (30%) say the same. Conversely, Republicans are more likely than Democrats to say women’s choices about how to balance family and work (50% vs. 36%) and their tendency to work in jobs that pay less (39% vs. 30%) are major reasons why women earn less than men.
Democratic and Republican women are more likely than their male counterparts in the same party to say a major reason for the gender wage gap is that employers treat women differently. About three-quarters of Democratic women (76%) say this, compared with 59% of Democratic men. And while 43% of Republican women say unequal treatment by employers is a major reason for the gender wage gap, just 18% of GOP men share that view.
Pressures facing working women and men
Family caregiving responsibilities bring different pressures for working women and men, and research has shown that being a mother can reduce women’s earnings , while fatherhood can increase men’s earnings .
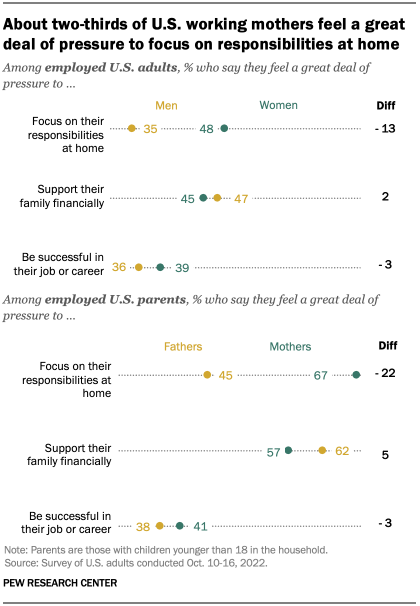
Employed women and men are about equally likely to say they feel a great deal of pressure to support their family financially and to be successful in their jobs and careers, according to the Center’s October survey. But women, and particularly working mothers, are more likely than men to say they feel a great deal of pressure to focus on responsibilities at home.
About half of employed women (48%) report feeling a great deal of pressure to focus on their responsibilities at home, compared with 35% of employed men. Among working mothers with children younger than 18 in the household, two-thirds (67%) say the same, compared with 45% of working dads.
When it comes to supporting their family financially, similar shares of working moms and dads (57% vs. 62%) report they feel a great deal of pressure, but this is driven mainly by the large share of unmarried working mothers who say they feel a great deal of pressure in this regard (77%). Among those who are married, working dads are far more likely than working moms (60% vs. 43%) to say they feel a great deal of pressure to support their family financially. (There were not enough unmarried working fathers in the sample to analyze separately.)
About four-in-ten working parents say they feel a great deal of pressure to be successful at their job or career. These findings don’t differ by gender.
Gender differences in job roles, aspirations
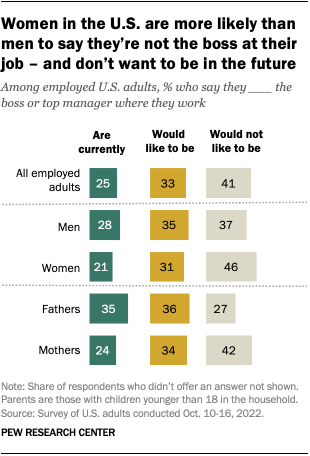
Overall, a quarter of employed U.S. adults say they are currently the boss or one of the top managers where they work, according to the Center’s survey. Another 33% say they are not currently the boss but would like to be in the future, while 41% are not and do not aspire to be the boss or one of the top managers.
Men are more likely than women to be a boss or a top manager where they work (28% vs. 21%). This is especially the case among employed fathers, 35% of whom say they are the boss or one of the top managers where they work. (The varying attitudes between fathers and men without children at least partly reflect differences in marital status and educational attainment between the two groups.)
In addition to being less likely than men to say they are currently the boss or a top manager at work, women are also more likely to say they wouldn’t want to be in this type of position in the future. More than four-in-ten employed women (46%) say this, compared with 37% of men. Similar shares of men (35%) and women (31%) say they are not currently the boss but would like to be one day. These patterns are similar among parents.
Note: This is an update of a post originally published on March 22, 2019. Anna Brown and former Pew Research Center writer/editor Amanda Barroso contributed to an earlier version of this analysis. Here are the questions used in this analysis, along with responses, and its methodology .
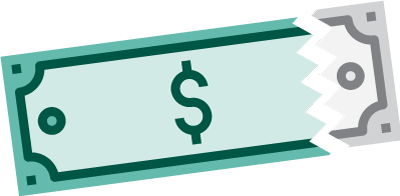
What is the gender wage gap in your metropolitan area? Find out with our pay gap calculator
- Gender & Work
- Gender Equality & Discrimination
- Gender Pay Gap
- Gender Roles
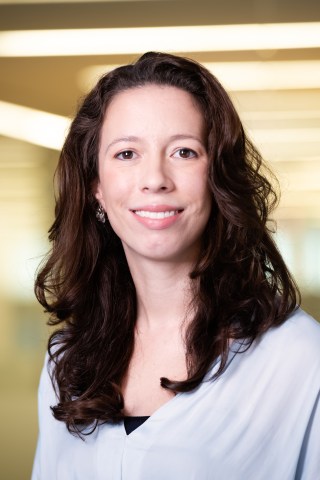
Carolina Aragão is a research associate focusing on social and demographic trends at Pew Research Center .
Half of Latinas Say Hispanic Women’s Situation Has Improved in the Past Decade and Expect More Gains
A majority of latinas feel pressure to support their families or to succeed at work, women have gained ground in the nation’s highest-paying occupations, but still lag behind men, diversity, equity and inclusion in the workplace, the enduring grip of the gender pay gap, most popular.
1615 L St. NW, Suite 800 Washington, DC 20036 USA (+1) 202-419-4300 | Main (+1) 202-857-8562 | Fax (+1) 202-419-4372 | Media Inquiries
Research Topics
- Email Newsletters
ABOUT PEW RESEARCH CENTER Pew Research Center is a nonpartisan fact tank that informs the public about the issues, attitudes and trends shaping the world. It conducts public opinion polling, demographic research, media content analysis and other empirical social science research. Pew Research Center does not take policy positions. It is a subsidiary of The Pew Charitable Trusts .
Copyright 2024 Pew Research Center

An official website of the United States government
The .gov means it’s official. Federal government websites often end in .gov or .mil. Before sharing sensitive information, make sure you’re on a federal government site.
The site is secure. The https:// ensures that you are connecting to the official website and that any information you provide is encrypted and transmitted securely.
- Publications
- Account settings
Preview improvements coming to the PMC website in October 2024. Learn More or Try it out now .
- Advanced Search
- Journal List
- Front Sociol

The Gender Pay Gap: Income Inequality Over Life Course – A Multilevel Analysis
Lisa toczek.
1 Department of Medical Sociology, Institute of the History, Philosophy and Ethics of Medicine, Faculty of Medicine, University of Ulm, Ulm, Germany
2 Department of Social Medicine, Care and Public Health Research Institute (CAPHRI), Faculty of Health, Medicine and Life Sciences, Maastricht University, Maastricht, Netherlands
Richard Peter
Maria Bohdalova , Comenius University in Bratislava, Slovakia
Associated Data
The datasets presented in this article are not readily available because the study data contain social security information. Due to legal regulations in Germany, it is not permitted to share data with social security information. Requests to access the datasets should be directed to [email protected] .
The gender pay gap has been observed for decades, and still exists. Due to a life course perspective, gender differences in income are analyzed over a period of 24 years. Therefore, this study aims to investigate income trajectories and the differences regarding men and women. Moreover, the study examines how human capital determinants, occupational positions and factors that accumulate disadvantages over time contribute to the explanation of the GPG in Germany. Therefore, this study aims to contribute to a better understanding of the GPG over the life course. The data are based on the German cohort study lidA (living at work), which links survey data individually with employment register data. Based on social security data, the income of men and women over time are analyzed using a multilevel analysis. The results show that the GPG exists in Germany over the life course: men have a higher daily average income per year than women. In addition, the income developments of men rise more sharply than those of women over time. Moreover, even after controlling for factors potentially explaining the GPG like education, work experience, occupational status or unemployment episodes the GPG persists. Concluding, further research is required that covers additional factors like individual behavior or information about the labor market structure for a better understanding of the GPG.
1 Introduction
In the European Union (EU) in 2019, women’s average gross hourly earnings were 14.1% below the earnings of men ( Eurostat, 2021a ). The gender pay gap (GPG) has existed for decades and still remains to date. According to Eurostat GPG statistics, the key priorities of gender policies are to reduce the wage differences between men and women at both the EU and national levels ( Eurostat, 2021a ). Nevertheless, the careers of men and women differ considerably in the labor market, with women being paid less than men ( Arulampalam et al., 2005 ; Radl, 2013 ; Boll et al., 2017 ). A report from the European Parliament in 2015 about gender equality assessed Germany’s performance in that field as mediocre. The federal government in Germany has already improved laws that focus on gender equality ( Botsch, 2015 ). Regarding Germany, in 2019 the earning difference between men and women were found to be 19.2% ( Eurostat, 2021a ). The reasons behind gender income inequality are complex and have multidimensional explanations.
1.1 Determinants of the GPG
The early 1990s represented a turning point for the participation of women in the labor market ( Botsch, 2015 ). In previous years, women’s participation rate in the workforce has strongly increased, from 51.9% in the year 1980 (West Germany) to 74.9% in 2019 ( OECD, 2021 ). This upward trend represents the increase of women working at older ages ( Sackmann, 2018 ). However, the gender income inequality remains. Different explaining factors of the GPG were found in previous research: patterns of employment, access to education and interruptions in the careers of men and women.
Although there are nearly equal numbers of men and women in the labor market, when considering women’s careers, various gender-specific barriers are occurring. The working patterns were found to have a relevant impact on the GPG in previous research. Atypical employment is increasing and this result in an expansion of the low-wage sector, which mainly affects women in Germany ( Botsch, 2015 ). Additionally, labor market integration of women has mainly been in jobs that provide few working hours and low wages ( Botsch, 2015 ). Moreover, part-time employment represents a common employment type in Germany, which is more frequent among women – as various studies have demonstrated – and explains the GPG significantly ( Boll et al., 2017 ; Ponthieux and Meurs, 2015 ; Boll and Leppin, 2015 ). In addition, the part-time employment occurs more often in occupations characterized by a high proportion of women and low wages ( Matteazzi et al., 2018 ; Boll and Leppin, 2015 ; Hasselhorn, 2020 ; Manzoni et al., 2014 ). Another employment type with few working hours and low pay is a special form of part-time work: marginal work. Marginal work is defined as earnings up to 450 Euros per month or up to 5.400 Euros annually. Also, it is also more common among women than among men ( Botsch, 2015 ; Broughton et al., 2016 ). The marginal part-time work has increased in nearly all EU countries, especially in Germany where it can be found to be above the EU average ( Broughton et al., 2016 ). Besides the working time, occupational status influences the wage differences of men and women. Female-dominated occupational sectors are characterized by lower wages compared to male-dominated ones ( Brynin and Perales, 2016 ). Additionally, in women-dominant industries, remunerations are less attractive and it often entails low-status work in sectors like retail, caregiving or education ( Boll and Leppin, 2015 ; Hasselhorn, 2020 ; Matteazzi et al., 2018 ; Brynin and Perales, 2016 ). Hence, working patterns such as the amount of working time or the occupational status are crucial determinants that contribute to explaining the GPG in Germany ( Blau and Kahn, 2017 ; Boll et al., 2017 ).
The access to education and vocational training are important factors, that influence the GPG. Both influence a first access to the labor market and are considered to be ‘door openers’ for the working life ( Manzoni et al., 2014 ). In Germany, education represents a largely stable variable over time, i.e. only few individuals increase their first educational attainment. Education influences the careers of men and women and can be seen as important an determinant of future earnings ( Boll et al., 2017 ; Bovens and Wille, 2017 ). Although women’s educational attainment caught up with those of men’s in recent years, for men, a higher qualification was still rewarded more than for women ( Botsch, 2015 ; Boll et al., 2017 ). Moreover, in previous research the impact of education on the GPG was not found to be consistent with different influences for men than for women ( Aisenbrey and Bruckner, 2008 ; Ponthieux and Meurs, 2015 ). Manzoni et al. (2014) found out, that the effect of education on career developments were dependent of their particular educational levels. In addition, regardless of the women’s educational catching-up in the last years, looking at older cohorts – born between 1950 and 1964 – women had a lower average level of education than men ( Boll et al., 2017 ).
An increasing GPG over time can also be the result of interruptions in careers, which are found more often for women than for men ( Eurostat, 2021a ; Boll and Leppin, 2015 ). Previous research of Boll and Leppin (2015) has identified explanations for the GPG in Germany by analyzing data from the German Socio-Economic Panel (SOEP) in 2011. They demonstrated that the amount of time spent in actual work was lower for women than for men. Therefore, women gain less work experience than their male counterparts ( Boll and Leppin, 2015 ). Career interruptions not only impact the accumulation of work experience but also the scope of future work. Especially in the period of family formation higher rates of part-time employment among women can be observed ( Boll et al., 2017 ; Ponthieux and Meurs, 2015 ). Moreover, work-life interruptions such as raising children or caring for family members have a major impact on the employment development and are more likely to appear for women than for men ( Ponthieux and Meurs, 2015 ). Although the employment rate of mothers has increased in recent years in Germany, it is still considerably lower than that of fathers ( Federal Statistical Office, 2021 ). Hence, taking care of children is still attributed to mothers, to the detriment of their careers ( Botsch, 2015 ). A recent study, however, found sizable wage differences between men and women who were not parents, refuting the assumption that the GPG applies only to parents ( Joshi et al., 2020 ). Other interruptions in the working lives of men and women are caused by unemployment. Azmat et al. (2006) found that in Germany, transition rates from employment to unemployment were higher for women than for men. Career interruptions have lasting negative effects on women’s wages. Therefore, it can be useful to examine unemployment when analyzing gender inequality in the labor market ( Eurostat, 2021b ).
1.2 Theoretical Background
1.2.1 human capital model.
In previous research, economic theories had been applied to explain the income differences of men and women. Two essential factors could be found: qualification and discrimination. The human capital model claims that qualifications with greater investments can be directly related to higher wages of men and women. The earnings are assumed to be based on skills and abilities that are required through education and vocational training, and work experience ( Grybaitė, 2006 ; Lips, 2013 ; Blau and Kahn, 2007 ). Educational attainment of women has caught up in recent years ( Botsch, 2015 ). However, women’s investments in qualifications were still not equally rewarded as those of men. Therefore, the expected narrowing of the GPG was not confirmed in earlier research ( Boll et al., 2017 ; Lips, 2013 ). Another determinant of the human capital model is work experience. Labor market experience contributes to a large extent to the gender inequality in earnings ( Sierminska et al., 2010 ). Hence, work experience influences the wages of men and women. On the one hand, interruptions due to family life lower especially women’s labor market experience compared to men. On the other hand, part-time employment is more frequent among women with fewer working hours and therefore less work experience. The lesser accumulation of work experience leads to lower human capital and lower earnings for women compared with men ( Blau and Kahn, 2007 ; Mincer and Polachek, 1974 ). Nonetheless, the association of work experience and income is more complex. Regarding the wages of men and women the influence of occupation itself also needs to be considered ( Lips, 2013 ). In the paper of Polachek (1981) different occupations over the careers of men and women were explained by different labor force participation over lifetime. Referring to the human capital model, it is argued that women more likely expect discontinuous employment. Therefore, women choose occupations with fewer penalties for interruptions ( Polachek, 1981 ). However, it should be questioned if working in specific occupations can be defined as a simple choice ( Lips, 2013 ). Besides, part-time employment is found to be more frequent among women, which ultimately leads to few working hours and hence low earnings ( Botsch, 2015 ; Ponthieux and Meurs, 2015 ; Boll et al., 2017 ). Though different working hours cannot be defined as a simple choice either ( Lips, 2013 ).
Earlier criticism about the human capital model discussed that the wage differences of men and women cannot only be explained by the qualification and the labor market experience ( Grybaitė, 2006 ; Lips, 2013 ). Another theoretical approach explaining the GPG refers to labor market discriminations, which effect occupations and wages ( Boll et al., 2017 ; Grybaitė, 2006 ). On the one hand, occupational sex segregation can be associated with income differences of men and women. The different occupational allocation in the labor market of men and women are defined as allocative discrimination ( Petersen and Morgan, 1995 ). In addition, occupations in female-dominated sectors are mostly characterized by low-wages compared to more male-dominated occupations ( Brynin and Perales, 2016 ). On the other hand, even with equal occupational positions and skill requirements women mostly earn less than men, this refers to the valuative discrimination ( Petersen and Morgan, 1995 ). Even within female-dominated jobs a certain discrimination exists, with men being paid more than women for the same occupation. Additionally, employment sectors with a large number of female workers are more likely to be associated with less prestige and lower earnings ( Lips, 2013 ). Achatz et al. (2005) analyzed the GPG with an employer-employee database in Germany. The authors examined the discrimination in the allocation of jobs, differences in productivity-, and firm-related characteristics. They found out that in occupational groups within companies, the wages decreased with a higher share of women in a group. Additionally, a higher proportion of women in a groups resulted in a higher wage loss for women than for men ( Achatz et al., 2005 ).
Although relevant criticism of the human capital model exists, its determinants are still found to be important in explaining the wage differences of men and women ( Boll et al., 2017 ). Nonetheless, income differences of men and women can still be found even with the same investments in human capital. The reason for this could be the occupational discrimination of women ( Brynin and Perales, 2016 ; Achatz et al., 2005 ; Lips, 2013 ). Therefore, the occupational positions can be associated as a relevant factor of the GPG.
1.2.2 Life Course Approach
Besides economic theories, there are other theoretical approaches of explaining the GPG. One of them focusses on the accumulation of disadvantages over the life course: the ‘cumulative advantage/disadvantage theory’ by Dannefer (2003) . It also involves social inequalities which can expand over time. The employment histories of men and women evolve over their working lives and during different career stages, advantages and disadvantages can accumulate. First, this life course perspective considers and underlines the dynamic approach of how factors shape each individual life course. Secondly, it can contribute to explain the different income trajectories of men and women over their working lives ( Doren and Lin, 2019 ; Dannefer, 2003 ; Härkönen et al., 2016 ; Manzoni et al., 2014 ; Barone and Schizzerotto, 2011 ).
The importance of the life course perspective was underlined by some earlier studies. They demonstrated that certain conditions in adolescence or early work-life affected future careers of men and women. Visser et al. (2016) found evidence for an accumulation of disadvantages in the labor market over working life, in particular for the lower educated. The cohort study SHARE had assessed economic and social changes over the life course in numerous European countries in several publications ( Börsch-Supan et al., 2013 ). Overall, education and vocational training, occupational positions and income illustrate parts of the social structure which in turn can demonstrate gender inequality in the labor market ( Boll and Leppin, 2015 ; Hasselhorn, 2020 ; Du Prel et al., 2019 ). Moreover, family events and labor market processes repeatedly affect one another over the life course. The work-family trajectories have consequences on employment outcomes such as earnings ( Aisenbrey and Fasang, 2017 ; Jalovaara and Fasang, 2019 ). Furthermore, the income differences of men and women are not steady but tend to be lower at the beginning of employment and increase with age ( Goldin, 2014 ; Eurostat, 2021a ). Therefore, careers should not be analyzed in a single snapshot, but with a more appropriate life course approach that takes into account factors that influences the wages of men and women over time.
1.3 Aim and Hypotheses
The aim of the present study is to examine income trajectories and to investigate the income differences of men and women over their life course. We are interested in how human capital determinants, occupational positions and the accumulation of disadvantages over time contribute to the explanation of the GPG from a life course perspective.
Focusing on older German employees, our study includes 24 years of their careers and considers possible cumulative disadvantages of women in the labor market compared to those of men. In contrast to Polachek (1981) , who analyzed the GPG as a unit over lifetime, we used a life course approach in regard to the theory of cumulative disadvantages of Dannefer (2003) . Accordingly, we analyze explaining factors of the GPG not only in a single snapshot but over the working careers of men and women. Life course data based on register data and characteristics of employment biographies with information on a daily basis are two additional important and valuable advantages of our study. Existing studies rarely have this information in the form of life course data and when they do, the data is either self-reported and retrospective including possible recall bias, or based on register data which was only collected on a yearly basis. We expect to find differences in the income of men and women over a period of time with overall higher, and more increasing earnings of men than of women.
Hypothesis 1 (H1): The differences of income trajectories throughout working life is expected to demonstrate more income over time among men than among women.
Education and vocational training, and work experience are human capital determinants. They have influence on the earnings of men and women. Although previous research estimated additional important factors contributing to the GPG, human capital capabilities continue to be relevant in explaining the wage differences of men and women ( Blau and Kahn, 2007 ; Boll et al., 2017 ). In our life course approach, we control for human capital determinants due to the information about education and vocational training, and work experience via the amount of working time (full-/part-time) for each year. We expect to find a strong influence of both determinants on the wages of men and women in Germany.
Hypothesis 2 (H2): The income differences between men and women can be explained by determinants of the human capital model.
Previous research found out that factors such as occupational status had an impact on the income differences of men and women ( Blau and Kahn, 2007 ; Boll et al., 2017 ). For a better understanding and explanation of the GPG, gender differences regarding occupational positions must be included to human capital determinants ( Boll et al., 2017 ). We assume that men and women can be found in different occupations, measured via occupational status, and these explain a substantial part of the wage differences between men and women.
Hypothesis 3 (H3): The occupational status of men and women can contribute to the explanation of the GPG.
The life-course approach acknowledges time as an important influence on the wages of men and women. Income differences of men and women can change over time and career stages, while the GPG was found to be lower at the beginning of the employment career and widened with age ( Goldin, 2014 ). Hence, the earning differences between men and women tend to be higher for older employees ( Eurostat, 2021a ; Federal Statistical Office, 2016 ). To account for the influence of age, we additionally included the age of each person in our analysis. Another factor that changes over time and contribute to explain the GPG is part-time work. In general, part-time work result in a disadvantage in pay compared to full-time employment ( Ponthieux and Meurs, 2015 ). However, explanations of the GPG due to different amount of part-time work need to include a special form of part-time work: marginal work. Marginal employment conditions are characterized by low wages and high job insecurities. Also discontinuous employment due to unemployment are characterized by job insecurities and affect the low-paid sector – therefore mainly women ( Botsch, 2015 ). Besides the human capital determinants and occupational positions as important factors explaining the GPG, the region of employment influences the wages of men and women and can also change over the career stages. Evidence from the Federal Statistical Office of Germany in 2014 noticed a divergence of the GPG trend in the formerly separated parts of Germany. The GPG among employees was wider in the Western part (24%) compared to the Eastern part of Germany, where it was found to be 9% ( Federal Statistical Office, 2016 ). Therefore, to examine income differences, the amount of less advantaged employment such as marginal work or periods of unemployment throughout the careers of men and women needs to be considered, as well as the region of employment and the age of a person.
Hypothesis 4 (H4): Factors of the living environment such as regional factors, and social disadvantage work conditions such as marginal work or unemployment, contribute to the income difference between men and women.
Our study about the GPG in Germany adds to earlier research in different ways. First, the accumulation of inequalities over the life course of men and women is known, but only few studies exist that focus on income through life course approach. We can analyze factors that influence the GPG over the careers of men and women due to the availability of social security data with daily information of each person. Besides the wages of men and women, the data additionally contains time-varying information about occupational status, working time and unemployment breaks. Therefore, we use longitudinal data of the German baby-boomers which allow us to measure changes of factors explaining the GPG over time. Second, a relevant contribution of our study is that we can consider different factors contributing to the explanation of the GPG through a life course perspective. The few studies focusing on the GPG over life course included either only determinants of the human capital model ( Joshi et al., 2020 ) or factors of occupational careers ( Moore, 2018 ). Some research included both aspects but had other disadvantages, such as Monti et al. (2020) , who could not analyze temporal evolution of the GPG with the data available. Moreover, previous research on the GPG in Germany could not trace vertical occupational segregation due to missing information of part-time workers, included only data of West Germany and used merely accumulated earnings over time ( Boll et al., 2017 ). Nonetheless, previous research demonstrated the need of analyzing the GPG via life course approach with which the accumulation of advantages and disadvantages for both, men and women, can be considered. Third, due to the usage of a multilevel framework we can examine income trajectories simultaneously at an individual and at a time-related level. Moreover, the influences of time-invariant and time-varying factors can be analyzed regarding differences in earnings of men and women. Hence, the multilevel approach examines income changes between and also within individuals. Furthermore, it acknowledges the importance of the life course perspective with including time as a factor in the model. A recent study also used growth curve modelling to explain gender inequality in the US. However, gender inequality measured through gender earnings was analyzed only across education and race without considering other variables explaining the GPG ( Doren and Lin, 2019 ). To our knowledge, there exists no research on the GPG that covers several essential determinants, hence we aim to fill those research gaps with our study.
2 Materials and Methods
The data were obtained from the cohort study lidA (living at work). The lidA sample includes two cohorts of employees (born in 1959 and in 1965) and was drawn randomly from social security data. LidA combines two major sources of information – register data of social insurance and questionnaire data derived from a survey. The survey was conducted in two waves, 2011 (t 0 ) and 2014 (t 1 ) ( Hasselhorn et al., 2014 ). The ethics commission of the University of Wuppertal approved the study.
In Germany, the social insurance system assists people in case of an emergency such as unemployment, illness, retirement, or nursing care. Employees have to make a contribution to the system depending on their income – except of civil servants or self-employed ( Federal Agency for Civic Education, 2021 ). In our analyses, we included men and women in Germany who participated in the baseline (2011) and in the follow-up (2014), were employed during both waves and subjected to social security contributions. We only included persons who agreed via written consent to the linkage of the survey data to their social security data. Thus, our sample for analysis included 3,338 individuals ( Figure 1 ).

Decision tree – inclusion and exclusion criteria in the sample for analysis.
2.2 Measurements
The social security data of the Institute for Employment Research of the German Federal Employment Agency is based on employers’ reports. The so-called “Integrated Employment Biographies” (IEB) or register data comprises information about individual employment; that is, type of employment, occupational status, episodes of unemployment and income with information about age, gender and education and vocational training. The IEB data are retrieved from employers’ yearly reports submitted to the social security authority ( Hasselhorn et al., 2014 ). The information of the register data was available on a daily basis and contained yearly information from 1993 to 2017 for each person. However, the IEB data contain missing details, especially regarding information that is not directly relevant for social security data and therefore, not of the highest priority for employers’ reports. This is particularly true for data on gender and education and vocational training. As our sample participants consented to the linkage of IEB with questionnaire data, we were able to impute the missing information on these variables with the help of the survey data. All time-varying information in the IEB is coded to the day. Our data have a multilevel structure with time of measurements (Level 1) being nested within individuals (Level 2) and defined as follows.
2.2.1 Level 1 Variables
In our analysis the variable time was based on information about the year of measurement. The starting point represents 1993 and was coded with zero. The outcome variable income was calculated from the IEB data as nominal wages in Euros (€). As time-varying variable, it can be defined as the average daily income per year of each person whose work contributes to social security and/or marginal employment. Information about the work experience due to working time was available for jobs that require social security contribution. To draw this information from the IEB data, the time-varying variable working time was computed with three different types: full- and part-time, part-time, and full-time. The data on occupational status were based on the International Standard of Classification of Occupations 2008 (ISCO-08). This time-varying variable contained information on the occupational status of each job that a person has held over the years. For the multilevel analysis, ISCO-08 was transformed from the German classification KldB 2010 (classification of occupations 2010) of the register data. ISCO-08 is structured according to the skill level and specialization of jobs, which are grouped into four hierarchical levels. Occupational status in our study was defined by the 10 major groups (level one of the classifications ISCO-08), without the group of armed forces who did not appear in our data. Therefore, the nine groups were analyzed: elementary occupations; plant and machine operators and assemblers; craft and related trades workers; skilled agricultural, forestry and fishery workers; services and sales workers; clerical support workers; technicians and associate professionals; professionals; and managers ( International Labour Office, 2012 ). Moreover, information about the number of episodes of marginal work could also be drawn from the register data. Marginal work was defined due to having at least one marginal employment per year. The time periods (episodes) of every marginal employment were counted and added up yearly. Furthermore, the duration of unemployment as time-varying variable was calculated due to information of the register data about the days of unemployment per year. In the register data unemployment is defined as being unemployed or unable to work for up to 42 days, excluding those with sickness absence benefits or disability pensions. The IEB data also provided information on the region of employment, which represents the area in which a company is located (East Germany and West Germany). This time-varying variable was available for each person over the years. A description of the Level 1 characteristics of our sample is provided in Table 2 using the last available information (2017) from the IEB data.
Characteristics of Level 1 variables a for men (n = 1,552) and women (n = 1,786).
M mean; SD standard deviation.
* p < 0.05, ** p < 0.01, *** p < 0.001.
2.2.2 Level 2 Variables
Information about the time-invariant variable education and vocational training was assessed from the survey data in 2011 (baseline). Education and vocational achievements of the sample were grouped in: low, intermediate and high education and vocational training (see Supplementary Table S1 ). The time-invariant variable gender had missing values in the register data. Therefore, we imputed the missing data using information of the survey data. The variable was coded 0 = female and 1 = male. Also based on the survey data, we included the time-invariant variable year of birth with measurements of 1959 and 1965 in the analysis. The characteristics of the Level 2 variables are displayed in Table 1 .
Characteristics of the Level 2 variables a for men (n = 1,552) and women (n = 1,786).
2.3 Statistical Analysis
The characteristics of our sample are displayed in Table 1 and Table 2 . Statistical analyses were performed using either Cramer’s V or by unpaired two sample t -test for numeric variables. Regarding the multilevel analysis, we used a so-called growth curve analysis. It demonstrates a multilevel approach for longitudinal data that model growth or decline over time. For this purpose, all daily information in the IEB were transformed into data on a yearly basis. Level 1 (year of measurements) represents the intraindividual change with time-varying variables. Interindividual changes are determined with time-invariant variables on Level 2 (individuals). Therefore, time of measurements predictors was nested within individuals. We applied a random intercept and slope model, which assumed variations in intercept and slope of individuals over time ( Singer and Willett, 2003 ; Rabe-Hesketh and Skrondal, 2012 ; Hosoya et al., 2014 ). Besides the Level 1 and Level 2 predictors, the cross-level interaction of gender*time interaction was constituted to analyze differences in income slopes of men and women over time ( Rabe-Hesketh and Skrondal, 2012 ).
Level 1 of the two-level growth model is presented below ( Eq. (1) ). y i j measures the income trajectory y for individual i at time j . True initial income for each person is represented with β 0 i . The slope of the individual change trajectory demonstrates β i j . T I M E i j stands for the measure of assessment at time j for individual i (Level 1 predictor). The residual or random error, specific to time and the individual is demonstrated by ε i j .
Eq. 2 and 3 represent the submodels of the Level 2. Eq. 2 defines the intercept γ 00 for individual i with the intercept of z i (illustrating a Level 2 predictor) and residual in the intercept v 0 i . The slope at Level 2 is represented in Eq. 3 with γ 10 and the slope error v 1 i . The effect γ 11 provides information on the extent to which the effect of the Level 1 predictor ( T I M E i j ) varies depending on the Level 2 predictor ( z i ).
To test our hypotheses, we calculated the influence of different variables with adjusting various predictors stepwise into the multilevel analysis. First, we estimated an unconditional means model which describes the outcome variation only and not its change over time (model 1). The next preliminary step was calculating the intraclass correlation coefficient (ICC) of this model 1. It identifies and partitions the two components: within- and between-person variance. The ICC estimates the proportion of total variation of the outcome y that lies between persons ( Singer and Willett, 2003 ). In the next model (model 2), we calculated an unconditional growth curve model which included time as predictor on Level 1. In model 3, the GCA was controlled for gender and time as well as the interaction of both variables. Model 4 was additionally adjusted for human capital determinant: education and vocational training, and working time. The GCA of model 5 was controlled for occupational status. The last model included year of birth, number of episodes of marginal work, duration of unemployment and region of employment (model 6 – fully adjusted model).
In Table 5 , the indices of the Akaike’s Information Criterion (AIC) were used to compare models and explore the best model fit ( Singer and Willett, 2003 ; Rabe-Hesketh and Skrondal, 2012 ). The statistical analyses were performed with IBM SPSS 25.
Goodness-of-fit statistics of the GCA.
AIC Akaike’s Information Criterion.
3.1 Descriptive
Characteristics of Level 2 variables stratified by gender are displayed in Table 1 . 1,552 men and 1,786 women were included in the analyses. It is observed that women significantly differ from men in education and vocational training. Women were less likely than men to have both low and high levels of education and vocational training.
The characteristics of Level 1 variable are represented in Table 2 . Men and women differ significantly in their occupational positions. Also, men had a higher average daily income than women. Part-time jobs are more likely among women as compared to men, who are more likely to be represented in full-time jobs. Moreover, the numbers of episodes of marginal work differ significantly between men and women.
Figure 2 displays the income trajectories over the observation period (1993–2017) among men and women. In 24 years, average daily income per year increased for both. However, men have a higher average income over their life course than women. Over time, a steeper growth of the average daily income per year can be observed for men, compared to the income development of women.

Income trajectories of men and women.
3.2 Growth Curve Analysis
Results of the multilevel analyses with average daily income per year as dependent variable concerning H1 are presented in Table 3 . The ICC of the unconditional means model (model 1) demonstrates that 74% of the total variability in income can be attributed to differences between persons and 26% to the differences within persons. Adding time as a predictor in the multilevel analysis (model 2), the variance components on Level 1 become smaller. Concluding that time accounts for 68% (from 607.34 to 197.12) of the within-person variance in average income. On Level 2, time explains 40% of the variance between persons (interindividual). However, there can be still found significant unexplained results in both levels which suggests that predictors on both levels should be further included. The GCA in model 3 was adjusted for gender (with women as reference group) and the interaction gender*time. The results show a significant effect of gender on the average income over time. The starting place (intercept) lies at 41.74€ with an incremental growth per year of 1.76€. However, regarding women as reference group, men have a higher average income. The significant interaction term also indicates different income development of men and women over time – with men having higher average income trajectory than women. As expected, no relevant change can be found in the within-person variance due to the adding of the Level 2 variable: gender. The variance on Level 2, however, become less concluding that gender accounts for 26% of the variance between persons. Overall, we can verify H1 with these results.
Growth curve models 1 to 3: Estimates of average daily income per year.
L1 = Level 1; L2 = Level 2.
Results of the GCA with average daily income per year as the dependent variable controlled by determinants of the human capital model are presented in Table 4 (model 4). In addition to the multilevel analysis of model 3, model 4 is also adjusted for: education and vocational training, and working time. The results show that the average income is found to be significantly higher for full-time workers and higher educated. There is a social gradient for income regarding education and vocational training – with decreasing levels of education, the income also reduces. People who are working full-time have a higher average income than those who work part-time or full- and part-time. The effect of gender is found to be significant with less average income of women compared to men. Moreover, the income development of men and women over time is still significantly different, with more income growth over time for men than for women. The results of the variance components demonstrate that human capital determinants are explaining 16% of the variance within person and 25% of the variance between persons. However, on both levels there can be still found significant variance and additional variables need to be considered. Our hypothesis 2 can be partially confirmed.
Growth curve models 4 to 6: Estimates of average daily income per year.
Model 5 ( Table 4 ) embeds occupational status to the analysis to find out the contribution of the occupational positions on the earning differences of men and women. Significant differences in the daily average income for each occupational group can be identified. The reference group is represented with the highest occupational group ‘manager’. In nearly all other occupations, manager had the highest average income, except of ‘technicians and associate professionals’. Moreover, the effects of occupational status on income are significant for all ISCO groups except for professionals. However, compared to education and vocational training, occupational status trends are less clear, and a social gradient cannot be identified. The estimated of the fixed effect of gender persists and stays the same, concluding that the occupational position of a person could not influence the effect of gender on income. The increase of income over time can be still found to be significant higher for men than for women. Moreover, including the Level 1 variable, occupational position cannot explain a substantial part of the within-person variance. We can identify occupational positions as significant predictor of the income, but a relevant contribution to explain the GPG cannot be observed. Therefore, we cannot approve hypothesis 3.
The results of investigating the influence of factors of the living environment are presented in Table 4 (model 6). Those, who are born earlier (1959) are found to have a higher average daily income, compared to those born in 1965. Having at least one marginal employment per year influences the average daily income negatively, as does having more unemployed days. Furthermore, average income is influenced by the region of employment, being lower in East Germany than in West Germany. The estimate of gender become a little less, but the average income and the development of income over time still substantially differs between men and women. The factors of living environment account for 10% of the variance between persons. We can only partially accept hypothesis 4.
3.3 Goodness of Fit
Table 5 displays the goodness of fit statistics for the different models of the GCA. The AIC is computed to find the best model fit. Considering the different indices of AIC, model 6 has the best fit.
4 Discussion
This study aimed to examine the income differences of men and women over their life course. We investigated how different factors can explain the GPG over time. Even after extensive control for human capital determinants, occupational factors and various factors of the living environment, the effect of gender on the average daily income persisted. Moreover, the average income development was found to be higher for men compared to women.
The accumulation of inequalities over time can be seen in the difference between men’s and women’s wages. Over the period of 24 years, our results showed that the income development of men increased more compared to women – the GPG widened with time. Due to the availability of life course data, we could consider cumulative disadvantages regarding the earnings of men and women. Moreover, the results of the variance componence also showed the importance of including time to explain the GPG ( Table 3 , model 2). Therefore, we can verify our first hypothesis. The steeper incline of income for men compared to women over time substantiates the presence of GPG in Germany. Goldin (2014) also found a small GPG when people enter the labor market and a widening gap with age. Our findings are also in line with information from the Federal Statistical Office (2016) and Eurostat (2021a) who used representative data and not use cohort specific data of the German working population.
The second hypothesis assumed that human capital determinants (education and work experience) can explain the GPG. The effects of education and vocational training on daily average income significantly differed in our results ( Table 4 , model 4). Findings of Bovens and Wille (2017) also demonstrated that the level of a person’s education determines the income level. Our results also support the previous finding, that education is most often a requirement for the achievement of a certain desired financial situation ( Du Prel et al., 2019 ). Our results also showed that the average income significantly differed considering working time. Full-time workers had higher average income, while men were more likely to work full-time compared to women. Earlier research also showed that part-time work was more frequent among women than among men ( Boll and Leppin, 2015 ; Matteazzi et al., 2018 ; Eurostat, 2021a ). After adjusting for human capital determinants, the unexplained variance was still substantial and the effect of gender remained significant. Hence, H2 can only partially be accepted.
In our third hypothesis, we assumed that the gender differences in occupational position can explain the GPG. We demonstrated that the average income differed according to the occupational status of a person. This is in line with previous findings of Blau and Kahn (2001) who assumed occupation to be an important factor of the financial status of a person. After controlling for occupational status, the effect of gender could still be found to be significant. We cannot accept H3 and therefore cannot confirm results of earlier studies ( Blau and Kahn, 2007 ; Boll et al., 2017 ). In contrast to the results of education and vocational training, we did not observe a clear social gradient of occupational status and income in our analyses. One explanation could be the classification of the occupational status. The ISCO classification is structured hierarchically on four levels. The construction is based on skill level and specialization. In our study, we used the major group structure (level one) with 10 different occupational groups. Using ISCO at level one (major groups) cannot be interpreted as a strict hierarchical order of occupations; instead, it can be considered more of a summary information on occupational status regarding skill level. Moreover, we were only able to generate the major groups of the register data and therefore cannot provide more detailed information about the occupational status. However, ISCO is applied in our study for the purpose of international comparability ( International Labour Office, 2012 ).
The accumulation of disadvantages over time could also be found in our results after controlling for factors such as unemployment or marginal employment. Having (at least one) marginal employment per year influenced the income negatively. We found that discontinuities in employment and interruptions such as unemployment also had a significant negative effect. Average income decreased when the number of days per year of unemployment increased. Furthermore, controlling for the region of employment, people in East Germany had lower daily average income compared to those in West Germany. Regarding the difference between men and women, previous findings also suggested a wider GPG in West Germany than in East Germany ( Federal Statistical Office, 2016 ). However, the GPG in West and East Germany should be compared with caution due to different societal models in the past. Moreover, different labour market characteristics and different infrastructure of childcare facilities lead to a lower GPG in East Germany than in West Germany ( Federal Ministry for Family Affairs, Senior Citizens, Women and Youth, 2020 ). The year of birth was included to eliminate cohort effects, and it was found to influence average income. Men and women born earlier (1959) had higher income than those born in 1965. The fact that they are older and have worked longer in the labor market could be an explanation. The significant effects of gender on the average income and the income trajectories remained after adjusting for these factors. Therefore, hypothesis 4 can only be partially confirmed.
4.1 Strengths and Limitations
Our study has limitations concerning the generalizability of our results due to the database. Our sample includes employees of two age groups (1959 and 1965) in Germany, who are subjected to social security. Thus, the generalizability or extension of the findings to self-employed people, civil servants and other age groups may be limited. The GPG differs considerably between the EU members. The GPG in Germany is one of the widest in the EU, with 19.2% in 2019. Netherlands and Sweden are two EU countries with similar employment rates, but still have lower GPGs with 14.6 and 11.8% ( Eurostat, 2021a ). Efforts to promote gender equality in politics in Germany are limited compared to other EU members. Women are still underrepresented, not only in the political but also in the economic area. Moreover family policy needs to further support full-time employment of women and working mothers ( Andersson et al., 2014 ; Botsch, 2015 ). Therefore, the transfer of our results to other countries should be made with caution. There are some other limitations regarding the IEB data. Information about occupational careers exist from the beginning (1975), but only for persons born in West Germany. Information about people born in East Germany was not available for the period before 1993. Hence, to counteract the systematic bias, we defined 1993 as a cut-off point, when people were either 28 or 34 years old. Additionally, we adjusted our analyses for the region of employment (East/West Germany). Furthermore, information about the marginal work and duration of unemployment were only available from 1999 onwards. Due to the composition of the IEB data, we could not include people who were unwell for long periods of time. Only persons who were unable to work for less than 42 days were included in the data. Regarding the income development of women in our study, Figure 2 shows a decrease between 1997 and 1999. Being in their thirties (32–40 years) and having to raise children at that time can be one possible explanation. Regarding family formation, in 1993 the average age of a mother at birth was 28.4 years ( Federal Statistical Office, 2020 ). At the beginning of our analysis (1993) the average age of both cohorts in the study (28 years; 34 years) is similar to the average age of a mother during that time – especially for the younger cohort. However, our data do not cover information about persons on parental leave or homemakers. Due to the lack of information in the IEB data, implications of family life contributing to a difference in pay for women cannot be included in our analysis. Furthermore, Joshi et al. (2020) could not find a GPG only for parents but also for men and women without children. Therefore, the issue of wage differences between men and women is relevant either way.
Besides these restrictions, our study exhibits several strengths. The study population is highly representative for German employees subject to social insurance contributions, born in 1959 and 1965 and is, therefore, characterized by a high external validity ( Schröder et al., 2013 ). Moreover, the IEB data itself and the nature of the data that the IEB provides, are one important strength of this study. The register data is not subject to possible recall bias. This is a relevant advantage compared to most previous studies that used self-reported data. In addition, the availability of information on a daily basis regarding many variables can be seen as another strength of the study. As a result, income trajectories could be calculated more precisely, compared to many previous studies. Furthermore, in Germany, income is used to calculate the amount of social benefit accruing to each person and therefore represents highly valid information. A further major advantage of our study is represented in our long observation period of 24 years. Only a few studies have applied the life course approach to examine the complexity of the GPG. Our life course data contain various information about employment characteristics which are relevant for the GPG and of high data quality.
Our results showed, even after controlling for relevant factors, that the GPG still persisted. There exist some explanations of the GPG regarding different behaviors of men and women in wage negotiations, which further influence different income developments ( Boll and Leppin, 2015 ). Also, structural disadvantages in the labor market can be a factor explaining the GPG. Individual behavior and labor market structures are not represented in our register data. We can only extract information that is relevant for social security contribution. Nonetheless, previous research of Blau and Kahn (2017) found a larger and more slowly decreasing GPG in the US at the top compared to other levels of the wage distribution. This ‘glass ceiling effect’ describes the reduced career opportunities of women compared to men due to frequent denial of access to leadership positions. Consequently, gender inequality can be found to be greater at the top of the wage distribution. Among European countries, previous studies have found this “glass ceiling effect” in Germany as well ( Arulampalam et al., 2005 ; Boll and Leppin, 2015 ; Huffman et al., 2017 ). However, recent results of Boll et al. (2017) could not confirm the glass ceiling effect in West Germany, thus further research is needed.
5 Conclusion
The gender pay inequalities in the German labor market from a life course perspective exist. Our results demonstrated that human capital determinants continue to be important in explaining the GPG over time. Furthermore, factors of working disadvantages such as marginal work or unemployment are important when trying to explain the income differences of men and women. For further research the availability of more work data over the life course with matching individual data would help to understand the GPG even better.
Acknowledgments
We gratefully acknowledge the support of two staff members of the University Ulm. We would like to thank Gaurav Berry for his support of the data preparation and Diego Montano for his feedback on the statistical analysis.
Data Availability Statement
Ethics statement.
The studies involving human participants were reviewed and approved by the ethics commission of the University of Wuppertal. The patients/participants provided their written informed consent to participate in this study.
Author Contributions
LT substantially contributed to the statistical analysis and interpretation of the data, and wrote the manuscript. HB discussed the results and provided critical comments on the manuscript. RP contributed to the obtaining of the funding, interpreting the data, and critically revised the manuscript for important aspects. All authors read and approved the final manuscript.
This work was supported by the German Research Foundation (DFG), grant number 393153877.
Conflict of Interest
The authors declare that the research was conducted in the absence of any commercial or financial relationships that could be construed as a potential conflict of interest.
Publisher’s Note
All claims expressed in this article are solely those of the authors and do not necessarily represent those of their affiliated organizations, or those of the publisher, the editors and the reviewers. Any product that may be evaluated in this article, or claim that may be made by its manufacturer, is not guaranteed or endorsed by the publisher.
Supplementary Material
The Supplementary Material for this article can be found online at: https://www.frontiersin.org/articles/10.3389/fsoc.2021.815376/full#supplementary-material .
- Achatz J., Gartner H., Glück T. (2005). Bonus Oder Bias? Koelner Z.Soziol.u.Soz.Psychol 57 , 466–493. 10.1007/s11577-005-0185-6 [ CrossRef ] [ Google Scholar ]
- Aisenbrey S., Bruckner H. (2008). Occupational Aspirations and the Gender Gap in Wages . Eur. Sociological Rev. 24 , 633–649. 10.1093/esr/jcn024 [ CrossRef ] [ Google Scholar ]
- Aisenbrey S., Fasang A. (2017). The Interplay of Work and Family Trajectories over the Life Course: Germany and the United States in Comparison . Am. J. Sociol. 122 , 1448–1484. 10.1086/691128 [ CrossRef ] [ Google Scholar ]
- Andersson G., Kreyenfeld M., Mika T. (2014). Welfare State Context, Female Labour-Market Attachment and Childbearing in Germany and Denmark . J. Pop Res. 31 , 287–316. 10.1007/s12546-014-9135-3 [ CrossRef ] [ Google Scholar ]
- Arulampalam W., Booth A. L., Bryan M. L. (2005). Is There a Glass Ceiling over Europe? Exploring the Gender Pay gap across the Wages Distribution . Colchester, EX: ISER Working Paper Series, 25. [ Google Scholar ]
- Azmat G., Güell M., Manning A. (2006). Gender Gaps in Unemployment Rates in OECD Countries . J. Labor Econ. 24 , 1–37. 10.1086/497817 [ CrossRef ] [ Google Scholar ]
- Barone C., Schizzerotto A. (2011). Introduction . Eur. Societies 13 , 331–345. 10.1080/14616696.2011.568248 [ CrossRef ] [ Google Scholar ]
- Blau F. D., Kahn L. M. (2007). The Gender Pay Gap . AMP 21 , 7–23. 10.5465/amp.2007.24286161 [ CrossRef ] [ Google Scholar ]
- Blau F. D., Kahn L. M. (2017). The Gender Wage Gap: Extent, Trends, and Explanations . J. Econ. Lit. 55 , 789–865. 10.1257/jel.20160995 [ CrossRef ] [ Google Scholar ]
- Blau F., Kahn L. (2001). Understanding International Differences in the Gender Pay Gap . Ithaca, NY: NBER Working Paper 8200. Available at: https://www.nber.org/papers/w8200 (Accessed November 30, 2021). [ Google Scholar ]
- Boll C., Jahn M., Lagemann A. (2017). The Gender Lifetime Earnings gap: Exploring Gendered Pay from the Life Course Perspective . Hamburg, Germany: HWWI Research Paper, 179. [ Google Scholar ]
- Boll C., Leppin J. S. (2015). Die geschlechtsspezifische Lohnlücke in Deutschland: Umfang, Ursachen und Interpretation . Wirtschaftsdienst 95 , 249–254. 10.1007/s10273-015-1814-y [ CrossRef ] [ Google Scholar ]
- Börsch-Supan A., Brandt M., Hunkler C., Kneip T., Korbmacher J., Malter F., et al. (2013). Data Resource Profile: the Survey of Health, Ageing and Retirement in Europe (SHARE) . Int. J. Epidemiol. 42 , 992–1001. 10.1093/ije/dyt088 [ PMC free article ] [ PubMed ] [ CrossRef ] [ Google Scholar ]
- Botsch E. (2015). The Policy on Gender Equality in Germany . Available at: https://www.europarl.europa.eu/RegData/etudes/IDAN/2015/510025/IPOL_IDA(2015)510025_EN.pdf (Accessed November 25, 2020).
- Bovens M., Wille A. (2017). Education as a Cleavage . Oxford University Press. [ Google Scholar ]
- Broughton A., Green M., Rickard C., Swift S., Eichhorst W., Tobsch V., et al. (2016). Precarious Employment in Europe: Patterns, Trends and Policy Strategy . Europarl. Available at: https://www.europarl.europa.eu/RegData/etudes/STUD/2016/587285/IPOL_STU(2016)587285_EN.pdf . [ Google Scholar ]
- Brynin M., Perales F. (2016). Gender Wage Inequality: The De-gendering of the Occupational Structure . Eur. Sociol. Rev. 32 , 162–174. 10.1093/esr/jcv092 [ CrossRef ] [ Google Scholar ]
- Dannefer D. (2003). Cumulative Advantage/disadvantage and the Life Course: Cross-Fertilizing Age and Social Science Theory . J. Gerontol. B Psychol. Sci. Soc. Sci. 58 , S327–S337. 10.1093/geronb/58.6.s327 [ PubMed ] [ CrossRef ] [ Google Scholar ]
- Doren C., Lin K. Y. (2019). Diverging Trajectories or Parallel Pathways? an Intersectional and Life Course Approach to the Gender Earnings Gap by Race and Education . Socius 5 , 237802311987381–23. 10.1177/2378023119873816 [ CrossRef ] [ Google Scholar ]
- Du Prel J. B., Schrettenbrunner C., Hasselhorn H. M. (2019). Vertical and Horizontal Social Inequality and Motivation for Early Retirement . Z. Gerontol. Geriatr. 52 , 3–13. 10.1007/s00391-018-1450-4 [ PubMed ] [ CrossRef ] [ Google Scholar ]
- Eurostat (2021a). Statistics Explained: Gender Pay gap Statistics . Available at: https://ec.europa.eu/eurostat/statistics-explained/index.php?title=Gender_pay_gap_statistics (Accessed December 01, 2021).
- Eurostat (2021b). Statistics Explained: Gender Statistics . Available at: https://ec.europa.eu/eurostat/statistics-explained/index.php?title=Gender_statistics#Earnings (Accessed November 30, 2021).
- Federal Agency for Civic Education (2021). Sozialversicherung [Social Insurance] . Available at: https://www.bpb.de/nachschlagen/lexika/das-junge-politik-lexikon/321149/sozialversicherung (Accessed November 30, 2021).
- Federal Ministry for Family Affairs, Senior Citizens, Women and Youth (2020). The Road to Equal Pay for Women and Men . Available at: https://www.bmfsfj.de/bmfsfj/meta/en/publications-en/the-road-to-equal-pay-for-women-and-men-161370 (Accessed December 01, 2021).
- Federal Statistical Office (2020). Durchschnittliches Alter der Mutter bei der Geburt ihrer lebend geborenen Kinder: Deutschland, Jahre, Familienstand der Eltern [Average age of the mother at the birth of her children born alive: Germany, years, marital status of parents] . Available at: https://www-genesis.destatis.de/genesis/online (Accessed March 17, 2021).
- Federal Statistical Office (2021). Three in Four Mothers in Germany Were in Employment in 2019 . Available at: https://www.destatis.de/EN/Press/2021/03/PE21_N017_13.html (Accessed March 16, 2021).
- Federal Statistical Office (2016). Unbereinigter Verdienstunterschied nach persönlichen Merkmalen im Jahr 2014 [Gender Pay Gap by personal characteristics in 2014 (unadjusted)] . Available at: https://www.destatis.de/DE/Themen/Arbeit/Verdienste/Verdienste-Verdienstunterschiede/Tabellen/gpg-persoenlich.html (Accessed March 16, 2020).
- Goldin C. (2014). A Grand Gender Convergence: Its Last Chapter . Am. Econ. Rev. 104 , 1091–1119. 10.1257/aer.104.4.1091 [ CrossRef ] [ Google Scholar ]
- Grybaitė V. (2006). Analysis of Theoretical Approaches to Gender Pay gap . J. Business Econ. Manag. 7 , 85–91. 10.3846/16111699.2006.9636127 [ CrossRef ] [ Google Scholar ]
- Härkönen J., Manzoni A., Bihagen E. (2016). Gender Inequalities in Occupational Prestige across the Working Life: An Analysis of the Careers of West Germans and Swedes Born from the 1920s to the 1970s . Adv. Life course Res. 29 , 41–51. 10.1016/j.alcr.2016.01.001 [ CrossRef ] [ Google Scholar ]
- Hasselhorn H. M., Peter R., Rauch A., Schröder H., Swart E., Bender S., et al. (2014). Cohort Profile: the lidA Cohort Study-A German Cohort Study on Work, Age, Health and Work Participation . Int. J. Epidemiol. 43 , 1736–49. 10.1093/ije/dyu021 [ PMC free article ] [ PubMed ] [ CrossRef ] [ Google Scholar ]
- Hasselhorn H. M. (2020). “ Social Inequality in the Transition from Work to Retirement ,” in Handbook of Socioeconomic Determinants of Occupational Health . Editor Theorell T. (Cham: Springer International Publishing; ), 1–26. 10.1007/978-3-030-05031-3_32-1 [ CrossRef ] [ Google Scholar ]
- Hosoya G., Koch T., Eid M. (2014). Längsschnittdaten und Mehrebenenanalyse . Köln Z. Soziol 66 , 189–218. 10.1007/s11577-014-0262-9 [ CrossRef ] [ Google Scholar ]
- Huffman M. L., King J., Reichelt M. (2017). Equality for Whom? Organizational Policies and the Gender Gap across the German Earnings Distribution . ILR Rev. 70 , 16–41. 10.1177/0019793916673974 [ CrossRef ] [ Google Scholar ]
- International Labour Office (2012). International Standard Classification of Occupations: ISCO-08 . Geneva: International Labour Organization. [ Google Scholar ]
- Jalovaara M., Fasang A. E. (2019). Family Life Courses, Gender, and Mid-life Earnings . Eur. Sociol. Rev. 36 , 159–178. 10.1093/esr/jcz057 [ CrossRef ] [ Google Scholar ]
- Joshi H., Bryson A., Wilkinson D., Ward K. (2020). The Gender gap in Wages over the Life Course: Evidence from a British Cohort Born in 1958 . Gend. Work Organ. 28 , 397–415. 10.1111/gwao.12580 [ CrossRef ] [ Google Scholar ]
- Lips H. M. (2013). The Gender Pay Gap: Challenging the Rationalizations. Perceived Equity, Discrimination, and the Limits of Human Capital Models . Sex Roles 68 , 169–185. 10.1007/s11199-012-0165-z [ CrossRef ] [ Google Scholar ]
- Manzoni A., Harkonen J., Mayer K. U. (2014). Moving on? A Growth-Curve Analysis of Occupational Attainment and Career Progression Patterns in West Germany . Social Forces 92 , 1285–1312. 10.1093/sf/sou002 [ CrossRef ] [ Google Scholar ]
- Matteazzi E., Pailhé A., Solaz A. (2018). Part-time Employment, the Gender Wage gap and the Role of Wage-Setting Institutions: Evidence from 11 European Countries . Eur. J. Ind. Relations 24 , 221–241. 10.1177/0959680117738857 [ CrossRef ] [ Google Scholar ]
- Mincer J., Polachek S. (1974). Family Investments in Human Capital: Earnings of Women . J. Polit. Economy 82 , S76–S108. 10.1086/260293 [ CrossRef ] [ Google Scholar ]
- Monti H., Stinson M., Zehr L. (2020). How Long Do Early Career Decisions Follow Women? the Impact of Employer History on the Gender Wage Gap . J. Labor Res. 41 , 189–232. 10.1007/s12122-020-09300-9 [ CrossRef ] [ Google Scholar ]
- Moore T. S. (2018). Occupational Career Change and Gender Wage Inequality . Work and Occupations 45 , 82–121. 10.1177/0730888417742691 [ CrossRef ] [ Google Scholar ]
- OECD (2021). OECD Statistics . Available at: http://stats.oecd.org/ (Accessed March 16, 2021).
- Petersen T., Morgan L. A. (1995). Separate and Unequal: Occupation-Establishment Sex Segregation and the Gender Wage Gap . Am. J. Sociol. 101 , 329–365. 10.1086/230727 [ CrossRef ] [ Google Scholar ]
- Polachek S. W. (1981). Occupational Self-Selection: A Human Capital Approach to Sex Differences in Occupational Structure . Rev. Econ. Stat. 63 , 60. 10.2307/1924218 [ CrossRef ] [ Google Scholar ]
- Ponthieux S., Meurs D. (2015). “ Gender Inequality ,” in Handbook of Income Distribution: Volume 2 . Editors Atkinson A. B., Bourguignon F. (Burlington: Elsevier Science; ), 981–1146. 10.1016/b978-0-444-59428-0.00013-8 [ CrossRef ] [ Google Scholar ]
- Rabe-Hesketh S., Skrondal A. (2012). Multilevel and Longitudinal Modeling Using Stata . 3rd ed.. College Station, Tex: Stata Press. [ Google Scholar ]
- Radl J. (2013). Labour Market Exit and Social Stratification in Western Europe: The Effects of Social Class and Gender on the Timing of Retirement . Eur. Sociological Rev. 29 , 654–668. 10.1093/esr/jcs045 [ CrossRef ] [ Google Scholar ]
- Sackmann R. (2018). “ Demographie als Herausforderung für die Soziologie ,” in Handbuch Soziologie des Alter(n)s . Editors Schroeter K. R., Vogel C., Künemund H. (Wiesbaden: Springer VS; ), 1–23. 10.1007/978-3-658-09630-4_5-1 [ CrossRef ] [ Google Scholar ]
- Schröder H., Kersting A., Gilberg R., Steinwede J. (2013). Methodenbericht zur Haupterhebung lidA - leben in der Arbeit [Methodology Report of the main survey of lidA] . Available at: http://doku.iab.de/fdz/reporte/2013/MR_01-13.pdf (Accessed March 15, 2020).
- Sierminska E. M., Frick J. R., Grabka M. M. (2010). Examining the Gender Wealth gap . Oxford Econ. Pap. 62 , 669–690. 10.1093/oep/gpq007 [ CrossRef ] [ Google Scholar ]
- Singer J. D., Willett J. B. (2003). Applied Longitudinal Data Analysis: Modeling Change and Event Occurrence . Oxford: Oxford Univ. Press. [ Google Scholar ]
- Visser M., Gesthuizen M., Kraaykamp G., Wolbers M. H. J. (2016). Inequality Among Older Workers in the Netherlands: A Life Course and Social Stratification Perspective on Early Retirement . Eur. Sociol. Rev. 32 , 370–382. 10.1093/esr/jcw013 [ CrossRef ] [ Google Scholar ]
Does gender of firm ownership matter? Female entrepreneurs and the gender pay gap
- Original Paper
- Open access
- Published: 17 May 2024
- Volume 37 , article number 52 , ( 2024 )
Cite this article
You have full access to this open access article
- Alexander S. Kritikos ORCID: orcid.org/0000-0002-6150-8794 1 , 2 , 3 , 4 ,
- Mika Maliranta ORCID: orcid.org/0000-0003-4460-8942 5 , 6 ,
- Veera Nippala ORCID: orcid.org/0009-0007-8936-1433 5 &
- Satu Nurmi 7
1 Altmetric
We examine how the gender of business owners is related to the wages paid to female relative to male employees working in their firms. Using Finnish register data and employing firm fixed effects, we find that the gender pay gap is—starting from a gender pay gap of 11 to 12%—two to three percentage points lower for hourly wages in female-owned firms than in male-owned firms. Results are robust to how the wage is measured, as well as to various further robustness checks. More importantly, we find substantial differences between industries. While, for instance, in the manufacturing sector, the gender of the owner plays no role in the gender pay gap, in several service sector industries, like ICT or business services, no or a negligible gender pay gap can be found, but only when firms are led by female business owners. Businesses with male ownership maintain a gender pay gap of around 10% also in the latter industries. With increasing firm size, the influence of the gender of the owner, however, fades. In large firms, it seems that others—firm managers—determine wages and no differences in the pay gap are observed between male- and female-owned firms.
Similar content being viewed by others
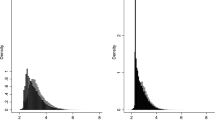
Gender pay gaps in domestic and foreign-owned firms
Gender Pay Gaps in Domestic and Foreign-Owned Firms
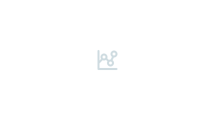
Does gender matter for job creation? Business ownership and employment growth
Avoid common mistakes on your manuscript.
1 Motivation
Faced with a still significant gender pay gap between female and male employees (Blau and Kahn 2017 ), one strand of literature argues that the gender composition of firm management may matter for the size of the “unexplained” part of the gap. To the extent that this part of the pay gap is rooted in discriminatory practices against female workers and to the extent that female superiors are more motivated than male superiors to reduce this kind of discrimination or are concerned about gender pay equality, female superiors may be able to reduce the gender pay gap. Footnote 1 Most (but not all) research in this field points to small, but significant, effects when comparing firms where male managers dominate with firms where female managers dominate (see, e.g., Abendroth et al. 2017 ; Theodoropoulos et al. 2022 ), or when the effect of a change from male to female manager is analyzed for the employees reporting to that specific manager (see, e.g., Cardoso and Winter-Ebmer 2010 ; Hensvik 2014 ). However, as female managers are usually neither major shareholders nor owners of these firms, they are not necessarily able to freely determine how wages are set. Their bounded involvement in these firms and their constrained ability to determine wages might limit their moderating influence on the gender pay gap.
Previous research focuses on management gender but does not investigate how the gender of firm ownership influences the gender pay gap. Female entrepreneurs and firm owners have different access to organizational power through their capital investment and profit-sharing than female managers, but they also have more skin in the game. As owners, they may decide about the wages of their salaried employees in a different way than managers—in small firms, for instance, there might be no interference from other managers or executives. Moreover, women who become managers in established firms may get involved in the wage-setting process where a gender pay gap already exists. By contrast, female entrepreneurs may have the ability to implement equal pay irrespective of gender from day 1. In that sense, the wage structure deserves separate attention with regard to the gender pay gap among firms run by female versus male entrepreneurs. Therefore, in this paper, focusing on entrepreneurs and firm owners, we investigate what role their gender plays in the wages paid to women relative to the wages paid to men in their respective firms.
The gender of the entrepreneur (as much as of the manager) may matter for the gender pay gap when there exists some kind of pay discrimination against female in comparison to male employees. Following the distinctions made in psychological research when investigating gender pay gaps with regard to discrimination, women employees may either suffer from explicit discrimination (which is forbidden in most European countries), when male superiors explicitly favor male over female employees, or from implicit discrimination. Implicit discrimination occurs when women confront unconscious or unintentional forms of bias (Ellemers 2018 ), where male managers, who still often comprise the majority in management, might—for instance because of their homophilous preferences—be inclined to support or promote male employees more strongly than female employees (Ertug et al. 2022 ).
Parallel research in economics also discusses discrimination, with literature basically distinguishing between two models. There is “taste-based” discrimination (Becker 1971 ; Charles and Guryan 2008 ), where managers may experience disutility if they employ workers of the opposite gender. To compensate for such disutility, employees with a different gender than their manager must agree to relatively lower wages if they want to be employed. A second approach in economics argues that gender pay gaps are the consequence of statistical discrimination. Tracing back to the model of Phelps ( 1972 ), it is claimed that employers have incomplete information in the sense that the expected future productivity of women is less predictable than men’s expected future productivity. This is because women are more likely to quit their jobs for a variety of reasons (including, for instance, motherhood or moving to another job because their partner moves; see, e.g., Cooke et al. 2009 ). Female individuals, when they are not able to signal their individual future productivity value, must accept—as a consequence of their group membership—lower wages, are denied access to jobs that involve further investments like firm-specific training, or may face search frictions (Sulis 2012 ). Recent reviews claim that the psychological approach of classifying discrimination into implicit and explicit aspects also captures these two main economic models of taste-based and statistical discrimination (Bertrand and Duflo 2017 ).
The aim of this paper is to investigate whether female entrepreneurs and firm owners are willing and able to reduce the part of the observable gender pay gap that is related to any of the described discriminatory practices. Having the ability is a necessary, but not sufficient, condition to reduce this part of the pay gap. Female entrepreneurs (as much as female managers) also need to have the willingness to do so. Theoretical research is divided on whether women superiors have the willingness to rectify the gender wage gap to the extent that it is rooted in discrimination.
On the one hand, research argues in favor of the “same gender approach,” according to which female superiors—here female entrepreneurs—have homophilous preferences that lead to common interests between them and female workers within a firm (McPherson, Smith-Lovin, and Cook 2001 ; Rudman and Goodwin 2004 ). This may unfold effects in various ways. Female entrepreneurs may support, help, or mentor female employees, for instance by promoting them more often or by paying them higher wages (Baron and Pfeffer 1994 ; Matsa and Miller 2011 ). Female entrepreneurs may also serve as role models (Ely 1994 ), creating positive spillover effects on female employees (Zimmermann 2022 ). An alternative explanation for homophilous bonds is that managers are better able, due to differences in communication styles, to assess the skills of their employees if they are of the same gender (Flabbi et al. 2019 ; Theodoropoulos et al. 2022 ). Thus, applying the same gender approach to the present analysis means that female entrepreneurs and firm owners will act as “agents of change” (Cohen and Huffman 2007 ), seeking to nullify that part of the gender pay gap that is owed to discriminatory practices in the workplace, perhaps even creating different discriminatory practices against male employees.
On the other hand, the opposing argument suggests that the principal-agent relationship between female entrepreneurs and female employees may counteract homophilous preferences, resulting in a gender pay gap that remains the same, if it is not increasing. Women may share men’s taste for discrimination with respect to women in lower positions (Deaux 1985 ). Female superiors who work in male-dominated industries need to become “one of the team.” Therefore, female superiors may feel pressure to maintain the status quo and to not ease the discrimination against female workers in such firms. This may also hold for female entrepreneurs, according to which they have to behave like “cogs in the machine” to receive acceptance from their male workers when they operate in a male-dominated industry (Kanter 1977 ). Ridgeway ( 1997 ) argues that there might exist a culturally driven persistent gender status belief according to which both female and male superiors implicitly expect superiority and greater competence of men. In a similar direction, according to the “queen bee syndrome” (Bednar and Gicheva 2014 ), individually successful women (in particular, in male-dominated environments) may feel competitive threats from other women or may hold negative stereotypical views about other women’s career commitment (Derks et al. 2011 ). Thus, applying the “cogs in the machine” approach or the “queen bee syndrome” to the present analysis means that female entrepreneurs will not seek to reduce the part of the gender pay gap that is owed to discriminatory practices in the workplace; they may even work to increase discriminatory practices against female employees.
Overall, it is not fully clear which of the two effects will dominate, thus what kind of wages female entrepreneurs and firm owners pay to their female relative to their male employees in comparison to male entrepreneurs and firm owners. Yet, it becomes obvious that approaches—like the “cogs in the machine” approach—arguing in the direction that female workers will face the same pay gap irrespective of the gender owner are mostly explained by settings where female entrepreneurs act in a male-driven environment. In such an environment, like in the manufacturing sector, there is not only a strong gender gap in entrepreneurship (Caliendo et al. 2015 ) but also the working population is mostly male. Accordingly, the wage-setting process for female entrepreneurs might be different in environments where there is more gender balance or a majority of female workers. Hence, it is interesting to conduct a heterogeneity analysis that takes these kinds of differentiations into account.
Based on these theoretical considerations, we empirically investigate in what way the gender of entrepreneurs and firm owners matters for the gender pay gap. More specifically, using Finnish register data, we analyze—for the first time, to the best of our knowledge—the size of the gender pay gap in firms started and owned solely or predominantly by female entrepreneurs in comparison to firms started and owned solely or predominantly by male entrepreneurs. We hypothesize, first, that the gender of entrepreneurs and firm owners matters in the sense that the gender pay gap will be smaller in firms that are started and owned solely or predominantly by female entrepreneurs. Secondly, given the contrasting expectations from earlier research about the influence of female managers (not owners), which may be rooted in the gender structure of industries, we explicitly take the industry and the related gender structure of employees into account. By investigating gender pay gaps for firms operating in different industries at the one-digit level, we aim to find out whether the observed gender structure of the industry matters for how female entrepreneurs and business owners pay their female employees relative to their male employees. Third, as we also have information on firm size, we are able to analyze what kind of wages are set in small versus large firms run by entrepreneurs and how the gender pay gap changes as firms become larger, controlling for the gender of the entrepreneurs. By doing so, we reveal to what extent the potential influence of the gender of the owner on gender pay gaps depends on firm size.
To investigate our research questions, we rely on unique Finnish register data provided by Statistics Finland for 2006–2015. The data links various sources delivering information on firms in the private business sector of Finland as well as wages paid in these firms (for details on data sources, see Kankaanranta and Melakari 2021 ). Using these data for our empirical setting, we analyze differences in person-level hourly or monthly wages between different firm owner groups: female-owned, male-owned, mixed or balanced ownership, and unknown or more dispersed ownership. We study the pay gap and differences in the pay gap, i.e., the difference in the estimates between different owner groups, controlling for various background factors. We further divide firms into subgroups by their industry, firm size, and relative productivity to analyze the differing pay gaps between these subgroups.
Starting from an hourly wage gap of about 11 to 12% for the hourly wages, we observe that the gender pay gap is—depending on whether we include firm fixed effects in our estimations—two to three percentage points lower for hourly wages in female-owned firms than in male-owned firms. Moreover, we find in several service sector industries no or a negligible gender pay gap, but only when firms are led by female business owners. In male-owned businesses, pay gaps are still at around 10%, also in these industries. Finally, in large firms, the influence of the gender of the owner on this pay gap disappears.
2 Previous empirical research
Previous research in this area concentrates on the question of whether the gender of managers, and other dependently employed supervisors, influences the gender wage gap. Footnote 2 These studies can be divided according to their use of different identification strategies. Some focus on the impact of female manager on the gender pay gap by analyzing how the switch from male to female managers influences the pay gap of their direct female and male subordinates. Others use a comparison by examining differences of gender wage gaps in firms where the share of female managers is high in comparison to firms with no or a low share of female managers, with earlier studies being restricted to cross-sectional analysis and few more recent studies exploiting the panel structure of the data at hand.
The analysis of Portuguese firms between 1987 and 2000 by Cardoso and Winter-Ebmer ( 2010 ) is one of the first type: they investigate to what extent the wage differentials are reduced if the management in a firm is changed from male to female. They pool all kinds of female managed firms, but restrict the analysis to firms with more than 10 employees. Their main finding is that, under such a management change, the monthly wage differential is reduced by 1.5 percentage points. Footnote 3 Hensvik ( 2014 ) finds similar results—a narrowing of the gender pay gap by 1.2 percentage points—for Swedish data when the gender of the manager changes from male to female in a firm. In her further analysis, she reveals that the gap reducing effect mostly owes to worker sorting behavior. Thus, instead of actively reducing the wage gap among the existing staff, female managers hire more highly skilled women who then receive higher wages. In contrast, Srivastava and Sherman ( 2015 ), who also analyze the influence of female managers on the wage levels of their employees after a change from male to female manager, but who restrict their investigation to one single firm in the information services (a male-dominated industry), find no support for a reduction of the gender wage gap. They even observe, in a subsample of high-performing supervisors and low-performing employees, that low performing women who switched from a male to a female supervisor had a lower salary in the following year than the same type of men who made the same switch, thus increasing the gender pay gap in this specific group. Flabbi et al. ( 2019 ), who restrict their analysis to Italian manufacturing firms, another male-dominated industry, also do not find that female managers reduce the gender pay gap and rather observe (like Srivastava and Sherman 2015 ) increases in the wage gap for less productive female workers.
Cohen and Hofman ( 2007 ) and Hirsch ( 2013 ) report observations within the second domain of comparisons. Based on cross-sectional analyses of US census data from 2000 and German data from 2008, both papers examine whether the gender wage gap is lower if the share of female management is high. Both observe that a high share of female managers is associated with a slightly lower pay gap, but they report a diverging effect in one respect: while Hirsch finds for Germany a smaller wage gap if the number of female managers is high in the second level-management, Cohen and Hofman ( 2007 ) reveal the exact opposite for the US—the gap is lower if the share of females at the top management level is high. Further research by Lucifora and Vigani ( 2022 ) of 30 European countries, for the 1995–2010 period, finds an overall gender earnings gap of 20%, with the pay gap being 5 percentage points lower if female employees work in firms with female owners.
More recent analysis exploits the panel structure of these data and includes—in contrast to the previous approaches—among others firm fixed effects. Abendroth et al. ( 2017 ) use similar German data as Hirsch ( 2013 ), but from 2012 and 2013 and they concentrate on large firms, where they investigate the within-firm variance of wages. In this subset of firms, they find a gender earnings gap of 12% for monthly incomes, which was 2.4 percentage points lower if the firm had female managers. Zimmermann ( 2022 ) and Sondergeld and Wrohlich ( 2023 ) observe similar results for different time periods between 2004 and 2018. Starting with an overall gender pay gap of about 15%, reductions in the gender pay gap range from 1.2 percentage points for the first level female management and a stronger effect for the second level female management. This confirms the earlier suggestive observations of Hirsch ( 2013 ) that the female management at the second level has a higher influence on the reduction of gender pay gap in Germany.
Hence, the reviewed empirical research on the influence of female managers on the gender pay gap shows that although firms with a larger share of female managers tend to pay wages that are associated with a lower gender wage gap, a substantial gap remains. Analyzing the influence of a change in the supervisor’s gender finds very small positive effects, no effect at all, or even negative effects for specific subgroups. It should be noted, however, that the latter two observations (of no or negative influences of female managers on the gender pay gap) are found in male-dominated industries, the manufacturing sector (Flabbi et al. 2019 ) in information services (Srivastava and Sherman 2015 ).
Yet, the access of female managers to organizational power is limited. Therefore, our approach takes a new direction. We examine how the gender of the entrepreneur or of the firm owner is related to the gender wage gap of those who are working for these entrepreneurs. There are several reasons for analyzing the wage-setting of female entrepreneurs and business owners separately from female managers. Female entrepreneurs and business owners are, through their ownership, involved in the capital investment and profit-sharing of their own firms in a different way than female managers. Therefore, as a principal, they have a different relationship with their employees than do managers and, as such, may have greater autonomy. Typically, entrepreneurs and business owners directly decide on wages, in particular when firms are small and there is no further management in between them and their employees. Footnote 4 By contrast, female managers usually enter existing firms and are confronted with existing wage inequalities that they need to correct, if they aim to address the problem. At the same time, another important difference between entrepreneurs and managers is that female (as much as male) entrepreneurs will be aware that any wage changes will affect their own earnings.
We explore in the subsequent empirical analysis the gender pay gap in firms run by female entrepreneurs, comparing it with the pay gap of firms that are run by male entrepreneurs. Thus, we aim to identify what kind of gender pay gap we observe in firms that are owned solely or predominantly by female entrepreneurs. With a comprehensive analysis of the structures of gender pay gaps with the linked owner-employer-employee data along several dimensions, we test the research questions that can be derived from the economic and psychological literatures, as presented in the introduction .
3 Data and summary statistics
3.1 data description.
For our analysis, we construct a unique data set based on Finnish register data comprising limited liability firms in the private business sector and their employees by linking various data sources maintained for research purposes by Statistics Finland, among them the Structure of Earnings Statistics (SES), the Finnish Longitudinal Employer-Employee Data (FLEED), and the Finnish Longitudinal Owner-Employer-Employee (FLOWN), as well as the Finnish Patent and Registration Office data. The data is comprehensive and rich in its content, allowing versatile wage analysis by employee gender and firm owner gender over the 2006–2015 period, as FLEED was discontinued in 2016. The data is repeated cross-section data, meaning that firms can disappear from and enter the sample, as they enter and exit the market. Similarly, employees can exit and enter the job market or change firms. The repeated nature of the data allows us to follow employees and firms over time and allows the use of firm fixed effects. Wages are observed at the end of the year, so an employee’s employment status at the end of the year defines if they contribute to the sample that year and who their employer is.
One advantage of our data is that it is a matched owner-employer-employee data set, providing us with information on employment structure within firms, i.e., the gender of all employees and their wages, which can be linked to the gender of the firm owner(s) and other firm features. Our target population is limited liability companies in the Finnish Business Register data with the size of at least one person. We include private non-agricultural business sector firms but exclude specific sectors, namely mining and quarrying; coke and refined petroleum products; electricity, gas and steam, and air conditioning supply; and water supply, sewerage, and waste management and remediation activities; as well as financial and insurance activities (according to NACE Rev. 2 the included sectors are 10-18, 20-33, 41-43, 45-47, 49-53, 55-56, 58-63, 68-82). Exclusion of these sectors is rationalized by the challenges in the measurement of productivity, which is one dimension of our analysis. Firm age is defined according to its oldest establishment in a given year to mitigate the effects of organizational changes, like mergers and acquisitions, in the firm codes. In comparisons between firm groups, we use information on productivity, dividing firms into productivity quartiles according to employment-weighted labor productivity within each 2-digit industry.
The company information is linked with information on their personnel in each year, the Structure of Earnings Statistics (SES) data by Statistics Finland, which includes detailed information on hourly and monthly wages for each wage earner, as well as part-time jobs. SES data allows us to calculate monthly wages without special payments, e.g., for overtime work, working shifts or non-standard hours, standby pay, or fringe benefits. Hourly wages can be adjusted for additional annual bonuses and allowances. The disadvantage of the SES data is its relatively low coverage of small non-organized firms with fewer than 5–10 persons. Private sector coverage of the data is about 55 to 75% of all private sector employment depending on the year and industry.
To reveal potential biases in the SES data and check the robustness of our findings, we use the FLEED (Finnish Longitudinal Employer-Employee Data) by Statistics Finland as a second data source. FLEED data include information on the gender, age, education, number of children, and other background characteristics of employees between ages 15 and 70. It includes annual income and months worked for each person, which we use to build an alternative measure for monthly wages. Although it does not allow for calculating hourly wages, the advantage of FLEED data is that it has a high coverage of firms of all sizes.
Finally, we link ownership information on the firms from the Finnish Longitudinal Owner-Employer-Employee (FLOWN) data by Statistics Finland. The Finnish Tax Authority requires firms to report their ten largest shareholders. If there are more than ten shareholders, those having at least 10% of the company stock. To improve the data coverage on person owners, we traced person owners behind enterprise-type owners in two layers. We define firms with female ownership greater than 60% of the company shares as female-owned firms, and firms with male owners greater than 60% of the shares as male-owned. Mixed firms have 40–60% of both male ownership and female ownership. The remaining firms are categorized as firms with unknown person owners. This last category is the largest in employment terms because it includes publicly listed firms and larger firms with distributed ownership.
As one extension, we identify the CEO of the firm from the Finnish Patent and Registration Office data on firm board members. We define the manager-owner of the firm as the owner with more than 50% ownership and having a CEO position at the end of the year. As a result, we can compare the results in several ways, namely owner and manager, with firm type defined according to owner gender with those on firm type defined using the manager gender or the owner-manager gender. Footnote 5
3.2 Descriptive statistics
The aim of this paper is to examine whether female entrepreneurs and business owners set wages in the relationship between female and male employees that are different from the relative wages set by male entrepreneurs and firm owners. For this analysis, we use monthly gross and hourly gross earnings, including and excluding bonuses and other special payments or allowances, separating these by gender. Tables 1 and 2 as well as Tables 3 and 4 report summary statistics of individual and firm characteristics for both the SES and the FLEED data. We have information from SES (FLEED) on nearly 470,000 (nearly 950,000) workers working in 9200 (66,600) firms in the private business sector. Among these, we observe 831 (6411) firms as female-owned, 779 (5242) firms as mixed owned, and 4196 (40,267) as male-owned. Thus, among all firms where the gender composition of the owners can be identified, 14% (12%) are run by a majority of female owners and entrepreneurs, while another 13 % (10%) of firms are owned by a mix of both genders, meaning that 73% (78%) of all firms (with an identified gender composition) have a majority of male owners, mirroring the typically higher shares of male firm owners in many industrialized economies. The other workers have jobs in 3387 (14,679) firms where the gender of the owner is unknown—typically larger firms. Employees are, on average, a little more than 40 years old, with marginally older and higher educated people working in firms with unknown owners, i.e., larger firms. Moreover, firms with unknown owners and, to a certain extent, male-owned firms employ larger shares of workers with technical occupations and have more workers higher up in the hierarchy, like professionals or technicians.
The overall share of women workers varies depending on the data source between 23% and 31% in male-owned firms and 42% and 52% in female-owned firms, but this share again varies widely across industries, as Tables 3 and 4 shows. For instance, in manufacturing, the share of female workers is between 7 and 14% in all four types of ownership structures, while between 56 and 77% are female employees in the accommodation and food services. More importantly, there are several industries in the service sector where the gender of the entrepreneur correlates with whether more males or females are employed, on average, in the business, such as wholesale and retail (G), information and communication (J), professional, scientific, and technical services (M), and administrative and support services (N), where more females work in female-owned businesses and more males work in male-owned businesses. As it turns out in Section 4, this might be one important difference for how gender pay gaps develop in male- versus female-owned businesses.
In terms of hourly earnings of female and male employees in female- and male-owned businesses (only available in the SES data), women always earn less than men on average; the differences fluctuate between three to four Euros per hour for all businesses where the gender of the owners are known, also including female-owned firms and firms with unknown person owners. Additionally, the differences in monthly earnings are similar across the three categories with known ownership, varying between 530 Euros in the FLEED data and 750 Euros in the SES data. Again, differences are higher at 800 to 900 Euros for firms with unknown ownership. It is important to highlight that, while the share of females running businesses is much lower than the share of males, the average size of these businesses is similar for the two genders as well for firms with mixed ownership. However, as Figure 1 shows, the size distributions differ for firms with different ownership structures. Female-owned firms are more commonly small than male-owned firms. Firms with less than 10 employees make up 70% of female-owned firms, but only 56% of male-owned firms. Male-owned firms are more likely than female-owned firms to have 10–50 and 50–250 employees. The largest firms with more than 250 employees are predominantly firms with unknown ownership structure.
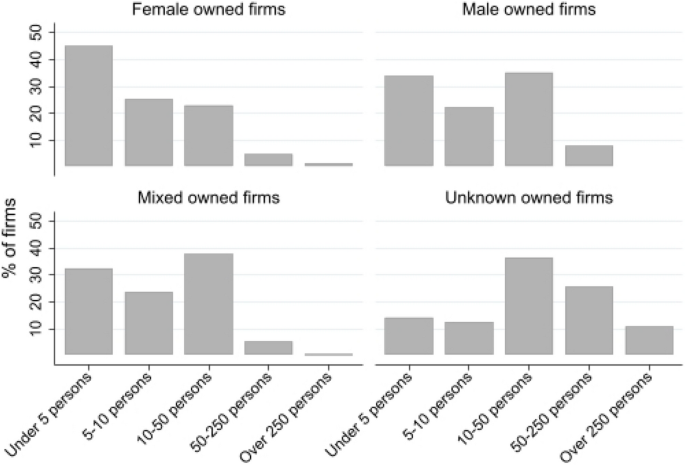
Source: Authors’ calculations based on the linked research data
Firm size distribution in 2015 (SES data).
4 Empirical analysis
4.1 empirical strategy.
To test the relationship between the entrepreneur’s gender and the gender pay gap along different dimensions, we estimate two kinds of Mincer-type (log) wage regressions at the employee level. In the first approach, we include employee gender, owner gender, their interaction, and several well-established control variables known to be relevant for explaining large parts of the gender pay gap (Blau and Kahn 2017 ). More specifically, next to gender, we control for age of the workers, the number of children below the age of 7, education level, education field, detailed occupation classes (i.e., occupation at the 3-digit level), firm size, firm age, and year dummies.
where wages are measured in natural logs and Female is an indicator variable that takes value 1 if the employee \(i\) who works in firm \(j\) is female and 0 if the employee is male. Female owner is an indicator that takes value 1 when the owner of the firm \(j\) is female and 0 otherwise. \({\alpha }_{j}\) represents firm fixed effects. The Control variables are described above.
In the second approach, we apply OLS regressions with Mincer-type (log) wage equations separately for each male- and female-owned firm. We further separate these from mixed-type firms where there is (nearly) an equal split between male and female owners and from firms with unknown owners—typically larger firms with dispersed ownership, including listed (publicly traded) companies. We define the pay gap as the coefficient of the indicator “female” in the Mincer type wage equation and study the difference in this gap between different owner groups. To further tease out various heterogeneities in the wage gap, we conduct separate regressions by dividing firms into subgroups by their industry, firm size, and relative productivity. Finally, we include robust standard errors with clustering by firms.
To assess the gender pay gaps differentiated by the gender of the entrepreneur, we present the following OLS regression for our estimation approach:
where wages are measured in natural logs and Female is an indicator variable that is 1 if the employee is female and 0 if the employee is male. The included Control variables are described above. This model is estimated separately for each firm-owner group and further for the sub-groups mentioned before. We extend this model by including firm fixed effects:
where \({\alpha }_{j}\) represents firm fixed effects that control for unobserved firm qualities that may influence the wage-setting and selection of employees to firms.
Since the model is estimated separately for female- and male-owned firms, the estimations have different samples and seemingly unrelated estimation methods are used to build a variance-covariance matrix that allows us to test for the statistical significance of the differences in the pay gap. Last, but not least, we make quantile regressions that are performed at the 10th, 25th, 50th, 75th, and 90th percentiles of the income distribution. Quantile regressions are useful for determining how the distribution of the wage gap is skewed, thus where the wage gaps are largest—at the bottom or the top incomes.
4.2 General estimation results
Table 5 provides the main results of our estimation of the gender pay gap for the full sample. In Finland, the hourly adjusted pay gap, during the observation period of 2006 to 2015, was 12.4%, when controlling for human capital, industry, and occupation beyond the basic variables, and 11.6% when also including firm fixed-effects. This gap is significantly lower in firms run by female entrepreneurs, by 3.3 percentage points in specification (1), and by 2.3 percentage points in specification (2) when we include firm fixed effects. Moreover, we observe a negative relationship between hourly wages and female owners that is offset for female employees through the positive interaction effect. This means that the reduction in the gender pay gap in female-owned firms is mostly realized through lower wage payments to male employees, while female employees realize similar wage payments in male- and female-owned firms.
In Table 6 , we present estimations separated by the gender of firm owners to further understand the heterogeneous effects between the genders—and confirm these observations for both specifications (i.e., without and with fixed effects). In firms with mixed gender ownership, pay gaps are similar to female-owned firms; in firms with unknown ownership, the pay gap is of the same size as in male-owned firms. The result of a 3 percentage points lower gender pay gap among female entrepreneurs and firm owners (when not including firm fixed effects) is generally confirmed for all kinds of variations of control variables (see panels A–D in Appendix Table A1 ). We find the same difference in the gap for additional payments (bonuses) or when occupational variables are not controlled for. In the latter case, gender pay gaps are at higher levels, while more controls lower the overall gender pay gaps (Meara et al. 2020 ), but differences between male and female business owners remain similar. This also holds for firms where the CEO and firm owner are the same person (see panel E in Appendix Table A1 ): again, the gender wage gap is 3 percentage points lower (as among female owners when compared to male owners). For monthly wages, the gender pay gap in female-owned firms is about 4 to 5 percentage points lower than in male-owned firms.
When comparing the pay gaps for firms run by male and female CEOs (groups defined by CEOs instead of the owners of the firms, see panels F–G in Appendix Table A1 ), we observe that, in firms run by a male CEO, the wage gap is, at 12.2%, very similar to male owners; under female CEOs, wage differences are only 1.6 percentage points lower when compared to male CEOs. Hence, the difference in hourly wages (i.e., the difference in the gap) between male and female employees is (in estimations without firm fixed effects) about 3 percentage points in female-owned firms when compared to male-owned firms, thus larger than the difference under female CEOs in comparison to male CEOs.
We also estimate quantile regressions to reveal the pay gaps over the wage distribution. Figure 2 presents exemplary results for 2 years, 2011 and 2015. In both years, the same patterns are observed. The gender pay gap is getting larger in both male- and female-owned firms, the higher we move up the distribution ladder. At the same time, the increase in the gap between male and female workers is getting larger at a lower rate in female-owned firms. This means that gender pay gaps are highest in the upper percentiles of the wage distribution, at 12 percentage points in female-owned and 16 in male-owned firms. Footnote 6 Thus, the difference in the gap between male and female workers is also largest, at 4 percentage points, in the upper percentiles when comparing female- with male-owned businesses, while the difference in the gap is around 1 percentage point in the lower tails, with differences being significant only in the upper five deciles of the distribution.
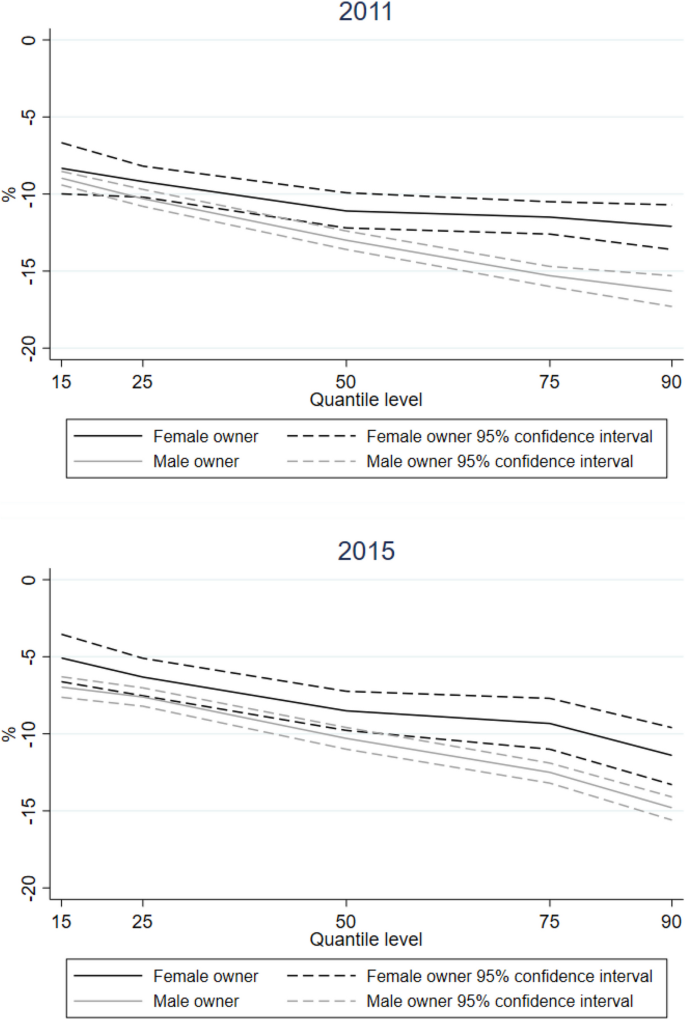
Quantile regression for gender pay gaps in 2011 and 2015. Note: The figure shows how the gender pay gap develops over the wage distribution in female- versus male-owned firms. The figure graphs the coefficients of indicator “female” in a quantile regression with hourly (SES) wage as dependent variable and as controls “female,” age, no of children <7, education (2-digit), occupation (3-digit), firm size, firm age, and industry (2-digit).
4.3 Further analyzing the gender wage gap
We continue by examining firms in various industries, with various firm sizes, and various productivity levels.
It is important to differentiate the analysis of wage gaps according to industries as there are industries with more male than female employees and vice versa. Earlier research points to potential differences: in male-dominated industries, female entrepreneurs (like female managers) may be inclined to turn against female employees (see Flabbi et al. 2019 ) to signal to male employees that they, the female entrepreneurs, are “one of the boys” (see Srivastava and Sherman 2015 , p. 1783). Our differentiation according to industries at the 1-digit level delivers several insights (Table 7 ).
Gender pay gaps in the secondary-sector industries (manufacturing and construction) and the more traditional third-sector industries (wholesale and retail) are generally larger than those in the other service-sector industries. Looking more deeply into differences, in the first three industries (with 1-digit codes C, F, and G), we observe gender pay gaps between 13.5 and 15% in male-owned businesses in the estimations without fixed effects and between 12 and 13% in the estimations with fixed effects. There are hardly lower pay gaps in female-owned business—only in construction the pay gap is significantly lower, by around 3 percentage points in female-owned firms. Much in contrast to this, in female-owned firms of service sector industries like information and communication (J), real estate (L), and administrative and support services (N), we observe no pay gaps at all, and in transportation and storage (H), and professional, scientific, and technical services (M) only a small gap of less than 5%. In these industries, gender pay gaps in male-owned businesses are still around 10%; thus, gender pay gaps are significantly lower in female-owned businesses with huge differences in (H) and, interesting enough, in information and communication (J). From the descriptive statistics, we know that, in these sectors, the share of women in the businesses greatly differ between male- and female-owned businesses. For instance, in information and communication (J), there are between 50 and 60% female employees in female-owned businesses, while in male-owned businesses, the share of male employees is above 80%. Last, but not least, the industry accommodation and food service (I) is an exception to these two “rules,” in the sense that the gender pay gap is relatively low with around 4% in the fixed effects estimation and does not differ across owner gender.
From earlier research, we know that the size of the firm may influence the size of the gender pay gap (Jones and Kaya 2023 ). Table 8 reports results for three different firm sizes (small, medium, and large firms). Two patterns can be observed. On the one hand, gender pay gaps grow larger with increasing firm size. On the other hand, the influence of female owners on reducing the gender pay gap becomes smaller the larger the firms are. While in small firms the gender pay gap in female-owned businesses is at 4% (for the estimation with fixed effects), half of the gender pay gap in male-owned businesses, in large firms the pay gaps are more or less identical at around 12%. Thus, in large firms, the influence of female owners on the gender pay gap seems to fade and it might be more in the hands of managers to decide about what kind of wages are paid in large firms.
We also examine the impact of firm size by investigating the development of gender pay gaps when firm size increases. Table 9 reports the results for male-owned and female-owned firms separately. The negative coefficient of the interaction term in both male- and female-owned firms with firm fixed effects confirms the finding of the pay gap growing larger with increasing firm size. In the model with firm fixed effects, this firm size penalty for women is larger in female-owned firms than male-owned firms, which provides additional evidence of female firm owners having less influence on the gender pay gap in larger firms.
Productivity levels
With respect to the productivity levels, we sort firms into four groups, low, medium-low, medium-high, and high productivity firms. We define productivity of the firm as value added by hour worked (Table 10 ).
The highest differences in gender pay gaps can be found by comparing hourly with monthly wages. The analysis of hourly wages shows that the generally observed pay gaps of 11 and 12% (with and without fixed effects) as well as the 2 and 3 percentage point difference between female- and male-owned businesses are confirmed for all but the low productivity firms. At the latter group, pay gaps and differences of pay gaps between male- and female-owned firms are slightly lower. Different to this, the monthly gender pay gap is getting larger between male- and female-owned businesses, the higher the productivity levels are. The difference between male- and female-owned businesses in the gender pay gap peaks at 8 percentage points for high-productivity firms. Thus, differences in the pay gaps are much stronger for monthly than for hourly wages, as productivity levels get higher. Hence, for monthly wages, gender wage gaps are sensitive to firm productivity.
4.4 Robustness check
In this section, we assess the robustness of our results with respect to several specifications. First, we note that the main results are robust to different definitions of wages (see panels H–L in Appendix Table A1 ). With different wage measures that use SES data, the difference in the gender pay gap between female- and male-owned firms ranges from around 2 percentage points to 4 percentage points. With FLEED data, the difference is slightly larger, around five percentage points.
The second robustness check concerns the financial crisis of 2008 and 2009. According to Calligaris et al. ( 2023 ), the Finnish economy suffered a long period of stagnation between 2008 and 2016, which falls in our sample period. They summarize that the financial crisis only had a limited role. Instead, the mostly exogenous downfall of mobile phone manufacturer Nokia around year 2010 had a more important role. Additionally, the financial crisis did lead to reduced global demand, which hit another important Finnish industry at the time, the forest industry. To examine the impact of these crisis industries, we perform a robustness check by excluding the electronics and forestry industries from our sample. Eliminating these industries from the sample (see panel B in Appendix Table A2 ) results in virtually the same wage gaps in male- and female-owned firms as in the whole sample, and the difference in the pay gap between male- and female-owned firms remains at around 3 percentage points.
We continue our robustness check with an analysis where we exclude firms with unknown owners from our estimation procedure (see panel C in Appendix Table A2 ). Estimation results in the sense of observed gender pay gaps and differences between male- and female-owned firms are again fully confirmed.
In the next part of the sensitivity analysis, we exclude those female and male employees from our main estimation approach (in Table 6 ) who have children younger than 7 years in their household (Appendix Table A2 panel D). Eliminating this group from the regression lowers the overall gender pay gap by 0.6 to 0.7%; however, the differences in the pay gap between male-owned and female-owned businesses remains the same. The robustness check confirms one further source of the gender pay gap; namely female workers with small children at home are more likely to be primary caregivers than equivalent male workers.
Our last robustness check addresses potential endogeneity in firm age. Our data is repeated cross-section data, allowing firms and employees to exit and enter the sample. It is possible that some of the firm and employee movements are endogenous, driven by the wage gap. As an example, a higher wage gap in the firm might increase the probability of it exiting the market or being sold off. Alternatively, it could be that the growing gap within and between male- and female-owned businesses as firms mature might be driven by a survivor bias in the sense that firms were driven out of the market because they had no gender pay gap. To investigate whether such a bias exists, our robustness check compares wages in firms that survived the whole observation period 2006–2015 with those exiting before 2015. Moreover, we differentiate between firm ages and sort them into young firms (up to 5 years old), middle aged (5 to 10 years old), and mature firms (more than 10 years old). The comparison of hourly pay gaps between firms shows nearly no difference (see Appendix Table A3 ). For instance, among middle-aged firms, the pay gap in male-owned firms was around 15% and among female-owned businesses around 7%, irrespective of whether firms closed or not. There is only one minor exception: in male-owned young firms that exited the market, the gender pay gap was slightly smaller than that in male-owned firms that survived. However, this smaller difference does not allow for the conclusion of a relevant survivor bias.
In an unreported robustness check (available upon request), we estimate a model with the share of female employees in the firm as a dependent variable. We find that female owners are associated with a significantly higher share of female employees in the firm compared to male owners. This result confirms our descriptive findings and provides evidence that the gender of the owner impacts business decisions.
4.5 Limitations and future research
Our approach is not without limitations. First, selectivity issues and the omission of further control variables may lead to some bias in our results. While our firm fixed effects approach allows controlling for selection to firms and the wages within firms, in the current analysis, we are not able to control for some issues of selection bias, as our information on wages is only available for those male and female workers who were hired. Even if female employment has risen (Bertola et al. 2007 ) such that the overall share of female and male workers in the population no longer differs in Finland (see WEF 2021 ), it is important to control for self-selection into the work force (Humlum et al. 2019 ). Second, and related to the previous point, as in many other studies, we are not able to observe wage offers that may affect the wage-setting process and the further development of wages within firms. Such information would allow for analyzing what kind of wage offers had been turned down and which ones were accepted, differentiated by gender. Similarly, we have no information on what kind of negotiation processes took place once workers were hired or when they negotiated for wage increases. This might be important as negotiation behavior may differ between male and female employees (Babcock and Laschever 2004 ). Thirdly, it would have been preferable to have more control variables, as the still existing unexplained part of the gender pay gap under female firm ownership may include effects of unmeasured productivity differentials associated with unobservable worker or job characteristics. For instance, information on formal or informal training offers, job interruption due to motherhood, and similar variables are missing, as are more recently analyzed variables such as personality traits or cognitive skills (see Blau and Kahn 2017 ). Having estimated a specification with various levels of labor productivity at the firm level, we may have captured parts of these potential effects. A last limitation is that the (FLEED) data ended in 2015. To test for the validity of the analysis for later years, we checked how the gender pay gap in Finland has developed since 2015. The raw gap has been decreasing on average by 0.5 percentage points per year: it was at 15.3% in 2021, slightly lower than that in 2015. Even though the gap has somewhat reduced, a significant gender pay gap remains which makes us confident that our analysis also holds for later years.
This research could be extended in various ways to further the understanding of the reasons for differing gender wage gaps. First, it would be interesting to examine whether gender pay gaps change—as mentioned above—when the gender of the entrepreneur changes, for instance, after a firm is sold. Future research should also try to model worker performance and productivity in all firms, for instance by applying the method of Abowd et al. ( 1999 ). This analysis may allow for explaining parts of the unexplained portion of the gender pay gap. It would be further worth conducting a decomposition analysis separately for male- and female-owned businesses to understand which parts of the gender pay gap are accounted for by differences in characteristics and which ones by unexplained components. Such an analysis would be interesting when comparing female entrepreneurs and female managers, as it would allow for revealing whether differences in gender pay gaps are observed for the same or for different variables. Lastly, it would be interesting to investigate how gender pay gaps develop across owner gender when controlling for work councils (Heinze and Wolf 2010 ).
5 Discussion and conclusions
One strand of literature on the gender pay gap discusses whether the gender of a superior influences the size of the pay gap between male and female employees. While virtually all existing research analyzing this issue is based on the comparison of wage gaps observed under dependently employed female and male managers, we focus on the gender of entrepreneurs and firm owners. We argue that not only do entrepreneurs have stronger access to organizational power through their capital investment and profit-sharing, but they are also involved in their firms in different ways than managers. At least in smaller firms, they may decide more directly about the wages of their salaried employees without interference from other managers, executives, or firm owners. Therefore, we analyze what kind of gender pay gaps are observed when comparing wage payments in firms run by female entrepreneurs and firm owners with firms run by male entrepreneurs and firm owners. Our investigation delivers several findings that are also important for the general understanding of the gender pay gap.
For our analysis, we use Finnish data covering 2006 through 2015. We observe that, starting from a gender pay gap of about 12% for hourly wages in estimations without firm fixed effects and 11% in estimations including firm fixed effects, the gender pay gap is about three percentage points lower in female-owned firms than in male-owned firms in estimations without firm fixed effects, and about two percentage points lower in estimations with firm fixed effects. Differences in the pay gap are robust when including additional compensation schemes. This finding can be interpreted in the sense that two percentage points of the so far unexplained part of the gender pay gap could be attributed to male business owners (and to firms with unknown owner structure), where male business owners may discriminate their female employees to this extent, or where (in case of unknown owner structure) there might be less sensitivity for gender pay gaps. In particular, with respect to larger firms with an unknown owner structure, one could have expected more awareness of and sensitivity towards this topic.
More importantly, looking into industries at the 1-digit level delivers highly significant differences. In the more traditional and male-dominated industries, like manufacturing or construction, the overall gender pay gaps are larger than average. Even female business owners (like female managers) seem to behave rather like “one of the boys.” In their firms where they also employ mostly male workers, gender pay gaps are not much lower. Much in contrast, in large parts of the service sector, female firm owners realize relative wages that reduce the gender pay gap partly nearly, partly fully to zero. However, it seems to be a crucial point that in these industries, female entrepreneurs also employ on average more female than male workers in their businesses. These findings have implications for past and future research: generalization of findings to other industries is not possible if the previous analysis concentrated on one industry like manufacturing (Flabbi et al. 2019 ) or on one single firm (Srivastava and Sherman 2015 ). Future research further focusing on the influence of the gender of managers on gender pay gaps should start differentiating between industries and the gender-structure of employees in these industries—to the best of our knowledge this has not been done yet.
Investigating various firm sizes reveals where the influence of the owner gender ends. While in small firms female business owners can reduce relatively low gender pay gaps by half, their influence on gender pay gaps in larger firms is rather limited. If the aim of low gender pay gaps was high on the agenda of female business owners, they would need to hire female managers in their firms who are similarly willing to address the topic of a reduction of the gender pay gap, perhaps even needing to focus on the gender structure of their employees.
Beyond the industries and firm size relationships, our analysis reveals further important differences, as the gender pay gap varies with the productivity level of the firms, as well as with the wage distribution. The highest gender pay gaps are generally found among male-owned high productivity firms, but only when looking at the monthly wages. Therefore, the comparison between male- and female-owned businesses clarifies where the differences between hourly and monthly wages originate. While in male-owned firms the monthly (but not the hourly) gender pay gap grows with productivity levels, in female-owned firms, the hourly and the monthly gender pay gap remain relatively constant across all productivity levels. It could be that, in male-owned firms, high productivity is correlated with more hours worked per month and male workers are conducting such additional work. In female-owned high-productivity firms, there seems to be no such imbalance between hours worked per month and higher productivity. These results allow for the interpretation that there are not just different wage-setting processes, but also different management practices based on gender, when comparing female- with male-owned businesses.
Moreover, our analysis further reveals that gender pay gaps are lowest among firms that have a low relative productivity level in their industry while larger gaps are found in firms with relatively high productivity levels. In that sense, our findings point toward “rents-related” wage gaps, according to which one part of the observed pay gap might be owed to wage payments by firms that can “afford” to pay premia in a discriminatory manner. Further research needs to be done in this direction.
Lastly, we observe in which parts of the wage distribution female firm owners pay wages that lower the gap when compared to male firm owners. First, we confirm for both genders that the gender pay gap is higher as wage levels increase. Moreover, in the bottom part of the wage distribution, up to the median wage, pay gaps are more or less the same for both firm owner genders. However, at the top of the wage distribution, the pay gap for female employees is 8 percentage points larger than that at the bottom of the wage distribution when females work in a male-owned business, but only four percentage points larger when females work in a female-owned firm. Thus, it is for wages above the median where female firm owners pay wages that lead to a lower gender pay gap when compared to male firm owners.
We conclude that the gender of the entrepreneur and firm owner matters for the gender pay gap and that female entrepreneurs are able and willing to reduce the gender pay gap in their firms. We also observe that the industry and the gender structure of employees in each industry matter. For manufacturing, our observations confirm the “cogs in the machine” approach, as female entrepreneurs and business owners make the same relative wage payments as male business owners; in both firm types, more male workers are employed than female workers. Contrasting to this, female firm owners seem to introduce gender neutral wages (except for the wholesale and retail industry) in industries where they hire more female than male employees. Further, it is remarkable that in all service sector industries, the gender of the entrepreneur and firm owner highly correlates with the average gender structure of the firms. Future research should investigate whether it is the industry or the gender structure of employees in the firm that drives the result of gender-neutral wages in female-owned firms.
Data availability
This study uses third party data available under license that the authors do not have permission to share. Requests for access to the data should be directed to Statistics Finland at lupa.stat.fi.
The data set used in this study contains only a binary indicator of gender. Therefore, in this study, we use both terms—women and female—when we refer to individuals labelled as women in the data. We are, however, aware that individual’s gender identity can deviate from their assigned sex at birth.
There is, of course, a huge number of studies on the gender pay gap from a more general point of view, which we do not discuss here (for an overview, see Blau and Kahn 2017 ). However, from this research, it is important to note that the largest part of the difference between the unexplained and explained gap is described by industry and occupational differences as well as by work experience (Blau and Kahn 2017 ), while it is also shown in various studies that, for most industrialized economies, the gap increases in the upper percentiles of the wage distribution; this includes Finland (Christofides et al. 2013 ). Another study investigates for the first time firm size effects on the gender pay gap (Jones and Kaya 2023 ).
Their analysis remains silent with respect to the question of how large the size of the pay gap is in female-led firms. Moreover, one must emphasize that the segregation effect in Portugal of the 1990s was still huge, leading to substantially lower wages paid to women in female-led firms than women in male-led firms.
Maliranta and Nurmi ( 2019 ) examine the (initial) characteristics of the entrepreneurs (gender, education, previous experience, the productivity performance of the firm where they previously have worked as an employee, etc.) starting their new business and, in particular, how these characteristics are related to the performance of their firm in terms of productivity, survival, and growth.
In smaller firms, the owner of the firm is also the manager of the firm without having separation between the two positions.
Albrecht et al. ( 2003 ) and Arulampalam et al. ( 2007 ) show that gender pay gaps also increase in other countries in the upper percentiles.
Abendroth A-K, Melzer S, Kalev A, Tomaskovic-Devey D (2017) Women at work: women’s access to power and the gender earnings gap. ILR Review 70:190–222. https://doi.org/10.1177/0019793916668530
Article Google Scholar
Abowd JM, Kramarz F, Margolis DN (1999) High wage workers and high wage firms. Econometrica 67:251–333. https://doi.org/10.1111/1468-0262.00020
Albrecht J, Björklund A, Vroman S (2003) Is there a glass ceiling in Sweden? J Labor Econ 21:145–177. https://doi.org/10.1086/344126
Arulampalam W, Booth AL, Bryan ML (2007) Is there a glass ceiling over Europe? Exploring the gender pay gap across the wage distribution. ILR Review 60:163–186. https://doi.org/10.1177/001979390706000201
Babcock L, Laschever S (2004) Women don’t ask: negotiation and the gender divide. Princeton University Press
Book Google Scholar
Baron JN, Pfeffer J (1994) The social psychology of organizations and inequality. Soc Psychol Q 57:190. https://doi.org/10.2307/2786876
Becker GS (1971) The economics of discrimination. University of Chicago Press, Chicago, IL
Bednar S, Gicheva D (2014) Are female supervisors more female-friendly? Amer Econ Rev 104:370–375. https://doi.org/10.1257/aer.104.5.370
Bertola G, Blau FD, Kahn LM (2007) Labor market institutions and demographic employment patterns. J Popul Econ 20(4):833–867
Bertrand M, Duflo E (2017) Field experiments on discrimination. In: Handbook of Economic Field Experiments. Elsevier, Amsterdam, vol 1, pp 309–393. https://doi.org/10.1016/bs.hefe.2016.08.004
Blau FD, Kahn LM (2017) The gender wage gap: extent, trends, and explanations. J Econ Lit 55:789–865. https://doi.org/10.1257/jel.20160995
Caliendo M, Fossen FM, Kritikos A, Wetter M (2015) The gender gap in entrepreneurship: not just a matter of personality. CESifo Econ Stud 61:202–238. https://doi.org/10.1093/cesifo/ifu023
Calligaris S, Jurvanen O, Lassi A et al (2023) The slowdown in Finnish productivity growth: causes and consequences. OECD Science, Technology and Industry Policy Papers No 139, Paris. https://doi.org/10.1787/c1fad5b3-en
Cardoso AR, Winter-Ebmer R (2010) Female-led firms and gender wage policies. ILR Review 64:143–163. https://doi.org/10.1177/001979391006400107
Charles KK, Guryan J (2008) Prejudice and wages: an empirical assessment of Becker’s The Economics of Discrimination. J Polit Econ 116:773–809. https://doi.org/10.1086/593073
Christofides LN, Polycarpou A, Vrachimis K (2013) Gender wage gaps, ‘sticky floors’ and ‘glass ceilings’ in Europe. Labour Econ 21:86–102. https://doi.org/10.1016/j.labeco.2013.01.003
Cohen PN, Huffman ML (2007) Working for the woman? Female managers and the gender wage gap. Am Sociol Rev 72:681–704. https://doi.org/10.1177/000312240707200502
Cooke TJ, Boyle P, Couch K, Feijten P (2009) A longitudinal analysis of family migration and the gender gap in earnings in the United States and Great Britain. Demography 46:147–167. https://doi.org/10.1353/dem.0.0036
Deaux K (1985) Sex and gender. Annu Rev Psychol 36:49–81. https://doi.org/10.1146/annurev.ps.36.020185.000405
Derks B, Ellemers N, Van Laar C, De Groot K (2011) Do sexist organizational cultures create the Queen Bee? British J Social Psychol 50:519–535. https://doi.org/10.1348/014466610X525280
Ellemers N (2018) Gender Stereotypes. Annu Rev Psychol 69:275–298. https://doi.org/10.1146/annurev-psych-122216-011719
Ely RJ (1994) The effects of organizational demographics and social identity on relationships among professional women. Adm Sci Q 39:203. https://doi.org/10.2307/2393234
Ertug G, Brennecke J, Kovács B, Zou T (2022) What does homophily do? A review of the consequences of homophily. Annals 16:38–69. https://doi.org/10.5465/annals.2020.0230
Flabbi L, Macis M, Moro A, Schivardi F (2019) Do female executives make a difference? The impact of female leadership on gender gaps and firm performance. Econ J 129:2390–2423. https://doi.org/10.1093/ej/uez012
Heinze A, Wolf E (2010) The intra-firm gender wage gap: a new view on wage differentials based on linked employer–employee data. J Popul Econ 23:851–879. https://doi.org/10.1007/s00148-008-0229-0
Hensvik LE (2014) Manager impartiality: worker-firm matching and the gender wage gap. ILR Review 67:395–421. https://doi.org/10.1177/001979391406700205
Hirsch B (2013) The impact of female managers on the gender pay gap: evidence from linked employer–employee data for Germany. Econ Lett 119:348–350. https://doi.org/10.1016/j.econlet.2013.03.021
Humlum MK, Nandrup AB, Smith N (2019) Closing or reproducing the gender gap? Parental transmission, social norms and education choice. J Popul Econ 32:455–500. https://doi.org/10.1007/s00148-018-0692-1
Jones M, Kaya E (2023) The UK gender pay gap: does firm size matter? Economica 90:937–952. https://doi.org/10.1111/ecca.12481
Kankaanranta P, Melakari A (2021) Creating ready-made research datasets from national administrative registers. Manuscript presented at the conference of the European Statisticians, Statistics Finland, Dec 2021. https://unece.org/sites/default/files/2021-12/SDC2021_Day1_Kankaanranta_P.pdf
Kanter RM (1977) Some effects of proportions on group life: skewed sex ratios and responses to token women. Amer J Sociol 82:965–990. https://doi.org/10.1086/226425
Lucifora C, Vigani D (2022) What if your boss is a woman? Evidence on gender discrimination at the workplace. Rev Econ Household 20:389–417. https://doi.org/10.1007/s11150-021-09562-x
Maliranta M, Nurmi S (2019) Business owners, employees, and firm performance. Small Bus Econ 52:111–129. https://doi.org/10.1007/s11187-018-0029-1
Matsa DA, Miller AR (2011) Chipping away at the glass ceiling: gender spillovers in corporate leadership. Amer Econ Rev 101:635–639. https://doi.org/10.1257/aer.101.3.635
McPherson M, Smith-Lovin L, Cook JM (2001) Birds of a feather: homophily in social networks. Annu Rev Sociol 27:415–444. https://doi.org/10.1146/annurev.soc.27.1.415
Meara K, Pastore F, Webster A (2020) The gender pay gap in the USA: a matching study. J Popul Econ 33:271–305. https://doi.org/10.1007/s00148-019-00743-8
Phelps ES (1972) The statistical theory of racism and sexism. Amer Econ Rev 62:659–661
Google Scholar
Ridgeway CL (1997) Interaction and the conservation of gender inequality: considering employment. Amer Sociol Rev 62:218. https://doi.org/10.2307/2657301
Rudman LA, Goodwin SA (2004) Gender differences in automatic in-group bias: why do women like women more than men like men? J Personal Soc Psychol 87:494–509. https://doi.org/10.1037/0022-3514.87.4.494
Sondergeld V, Wrohlich K (2023) Women in management and the gender pay gap. DIW Discussion Papers #2046, Berlin
Srivastava SB, Sherman EL (2015) Agents of change or cogs in the machine? Reexamining the influence of female managers on the gender wage gap. Amer J Sociol 120:1778–1808. https://doi.org/10.1086/681960
Sulis G (2012) Gender wage differentials in Italy: a structural estimation approach. J Popul Econ 25:53–87. https://doi.org/10.1007/s00148-010-0351-7
Theodoropoulos N, Forth J, Bryson A (2022) Are women doing it for themselves? Female managers and the gender wage gap*. Oxf Bull Econ Stat 84:1329–1355. https://doi.org/10.1111/obes.12509
World Economic Forum (2021) Global Gender Gap Report, World Economic Forum, Geneva
Zimmermann F (2022) Managing the gender wage gap—how female managers influence the gender wage gap among workers. Eur Sociol Rev 38:355–370. https://doi.org/10.1093/esr/jcab046
Download references
Acknowledgements
We would like to thank the editor-in-chief of this journal, Klaus F. Zimmermann, four anonymous referees, Jana Friedrichsen, and Katharina Wrohlich for their helpful and valuable comments. Further, we thank Statistics Finland for the access to the data and Pekka Laine for guidance with the data. We also thank the participants at the Finnish Economic Association XL Annual Meeting in Turku, at the Oxford Residence Week for Entrepreneurship, and at the Academy of International Business meeting in Warsaw for the helpful comments. Mika Maliranta and Satu Nurmi gratefully acknowledge funding from Etla–Research Institute of the Finnish Economy. Mika Maliranta and Veera Nippala gratefully acknowledge the financial support from Palkansaajasäätiö project “Innovation, productivity and economic growth.”
Open Access funding enabled and organized by Projekt DEAL.
Author information
Authors and affiliations.
German Institute for Economic Research, DIW Berlin, Berlin, Germany
Alexander S. Kritikos
University of Potsdam, Potsdam, Germany
GLO, Essen, Germany
IAB, Nuremberg, Germany
Labour Institute for Economic Research, Helsinki, Finland
Mika Maliranta & Veera Nippala
University of Jyväskylä, Jyväskylä, Finland
Mika Maliranta
Statistics Finland, Helsinki, Finland
You can also search for this author in PubMed Google Scholar
Corresponding author
Correspondence to Alexander S. Kritikos .
Ethics declarations
Competing interests.
The authors declare no competing interests.
Additional information
Responsible editor : Klaus F. Zimmermann
Publisher's Note
Springer Nature remains neutral with regard to jurisdictional claims in published maps and institutional affiliations.
Supplementary information
Below is the link to the electronic supplementary material.
Supplementary file1 (PDF 108 KB)
Rights and permissions.
Open Access This article is licensed under a Creative Commons Attribution 4.0 International License, which permits use, sharing, adaptation, distribution and reproduction in any medium or format, as long as you give appropriate credit to the original author(s) and the source, provide a link to the Creative Commons licence, and indicate if changes were made. The images or other third party material in this article are included in the article's Creative Commons licence, unless indicated otherwise in a credit line to the material. If material is not included in the article's Creative Commons licence and your intended use is not permitted by statutory regulation or exceeds the permitted use, you will need to obtain permission directly from the copyright holder. To view a copy of this licence, visit http://creativecommons.org/licenses/by/4.0/ .
Reprints and permissions
About this article
Kritikos, A.S., Maliranta, M., Nippala, V. et al. Does gender of firm ownership matter? Female entrepreneurs and the gender pay gap. J Popul Econ 37 , 52 (2024). https://doi.org/10.1007/s00148-024-01030-x
Download citation
Received : 22 March 2023
Accepted : 02 May 2024
Published : 17 May 2024
DOI : https://doi.org/10.1007/s00148-024-01030-x
Share this article
Anyone you share the following link with will be able to read this content:
Sorry, a shareable link is not currently available for this article.
Provided by the Springer Nature SharedIt content-sharing initiative
- Entrepreneurship
- Gender pay gap
- Discrimination
- Linked employer-employee data
JEL classification
- Find a journal
- Publish with us
- Track your research
This website uses cookies.
By clicking the "Accept" button or continuing to browse our site, you agree to first-party and session-only cookies being stored on your device to enhance site navigation and analyze site performance and traffic. For more information on our use of cookies, please see our Privacy Policy .
- Journal of Economic Perspectives
- Winter 1998
- Understanding the "Family Gap" in Pay for Women with Children
Understanding the "Family Gap" in Pay for Women with Children
- Jane Waldfogel
- Article Information
- Comments ( 0 )
JEL Classification
- J13 Fertility; Family Planning; Child Care; Children; Youth
Cookies on GOV.UK
We use some essential cookies to make this website work.
We’d like to set additional cookies to understand how you use GOV.UK, remember your settings and improve government services.
We also use cookies set by other sites to help us deliver content from their services.
You have accepted additional cookies. You can change your cookie settings at any time.
You have rejected additional cookies. You can change your cookie settings at any time.

- Corporate information
- Employers’ Understanding of the Gender Pay Gap and Actions to tackle it - a research report 2017
- Government Equalities Office
Employers’ understanding of the gender pay gap and actions to tackle it: a research report
Published 31 January 2019
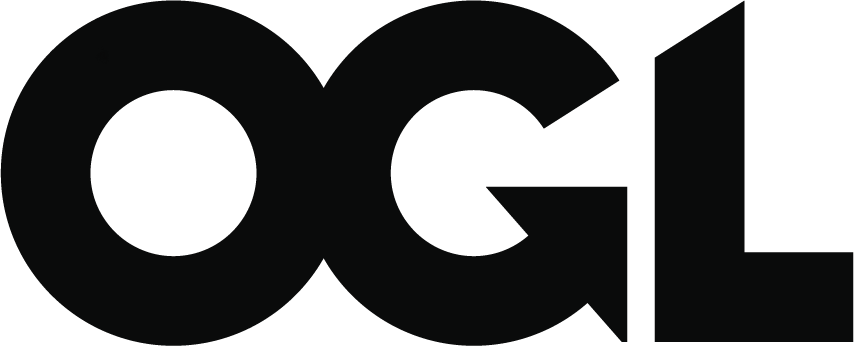
© Crown copyright 2019
This publication is licensed under the terms of the Open Government Licence v3.0 except where otherwise stated. To view this licence, visit nationalarchives.gov.uk/doc/open-government-licence/version/3 or write to the Information Policy Team, The National Archives, Kew, London TW9 4DU, or email: [email protected] .
Where we have identified any third party copyright information you will need to obtain permission from the copyright holders concerned.
This publication is available at https://www.gov.uk/government/publications/employers-understanding-of-the-gender-pay-gap-and-actions-to-tackle-it-a-research-report-2017/employers-understanding-of-the-gender-pay-gap-and-actions-to-tackle-it-a-research-report
Research report November 2017
James Murray, Paul Rieger and Hannah Gorry – OMB Research
Prepared by:
OMB Research
The Stables, Bradbourne House, East Malling, Kent ME19 6DZ
01732 220582
www.ombresearch.co.uk
This research was commissioned by the Government Equalities Office (GEO). GEO is responsible for equality strategy and legislation across government. GEO works with the Department for Education (DfE) and as such this research has been published by DfE.
The research was undertaken by OMB Research. We would like to thank all of the private, voluntary and public sector organisations that kindly took the time to participate in the quantitative survey, and those who subsequently provided additional feedback during the qualitative follow-up interviews.
1. Executive summary
1.1 introduction.
The government has recently introduced new gender pay gap (GPG) transparency regulations, [footnote 1] which are designed to encourage large employers to take informed action to close their GPG where one exists. These regulations came into force in April 2017 and affect over 9,000 GB employers across the private, voluntary and public sectors.
This research provides a baseline measure of large employers’ understanding of the GPG and the transparency regulations, and the actions they are taking to close their GPG.
The core research consisted of a telephone survey of 900 large employers (with 250 or more staff), and 30 follow-up qualitative interviews to explore the key issues in more detail. It took place between March and May 2017.
An additional phase of 22 brief qualitative interviews was conducted among employers who had stated an intention to publish their GPG results in quarter 1 2017 but did not upload their data to the portal during this time. This additional research took place in September 2017.
1.2 Understanding of the GPG
Awareness of the term ‘gender pay gap’ was extremely high (98%). Half (48%) of all respondents (typically HR directors or managers) felt they had a good understanding of what the GPG was and how it was calculated, and a further 41% believed they had a reasonable understanding but were not sure of the specifics of how it was calculated. However, the qualitative interviews found that some of those reporting a good understanding could not provide a detailed or correct explanation of the GPG when asked.
Around two-thirds (63%) of respondents (typically HR directors or managers) reported that they had a good understanding of the difference between ‘closing the GPG’ and ‘ensuring equal pay between men and women’. In most cases they were reasonably confident that this knowledge extended to the top levels of the organisation. Over half (54%) believed that their board or leadership team had a fairly good understanding of the GPG and how it differs from equal pay, and a significant minority (17%) felt they had a very good understanding.
In all of the above areas, self-reported understanding was highest among public sector organisations and those with 1,000 or more employees.
The qualitative research suggests that many employers had not engaged with the topic of GPG before the introduction of the new regulations. Therefore, levels of understanding were mixed and somewhat superficial. As mentioned above, some of those claiming a good understanding in the quantitative survey were not able to spontaneously provide a clear or correct definition of GPG. This was typically due to confusion between GPG and equal pay. To clarify, the Gender Pay Gap is a measure of the difference between the average hourly earnings of men and women. In contrast, equal pay deals with the pay differences between men and women who carry out the same work, similar work or work of equal value, and it is unlawful to pay men and women unequally based on their gender.
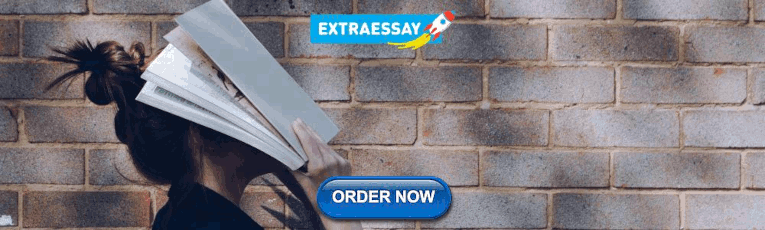
1.3 Measuring the GPG and other gender analysis
Almost one-third of organisations (31%) had measured their GPG in the previous 12 months, so prior to the new transparency regulations coming into force. The qualitative interviews suggest that many of these employers had done so as a ‘dry run’ in advance of the regulations coming into force, and incidence of ‘true’ voluntary GPG measurement (not driven by the regulations) was low. It should be noted that these employers had not always calculated their GPG in a way that was entirely consistent with the approach mandated in the new regulations.
The proportion of employers that had measured their GPG increased in line with organisation size, ranging from 23% of those with 250 to 499 employees up to 47% of those with 1,000 or more employees. It was also higher in the public sector (40%).
The majority (58%) of large employers had conducted other gender analysis in the past 12 months. The most common other analyses were calculating the proportions of male and female employees at different pay levels (41%), measuring the proportions paid bonuses (31%) and examining the differences in average bonuses paid (24%). Larger organisations with 1,000 or more employees were considerably more likely to have conducted each of these types of gender analysis, as were those that had also measured their GPG.
The results of the GPG or other gender analysis were typically communicated to senior levels of the organisation, with two-thirds (66%) sharing them with the leadership team or board and a similar proportion (62%) sharing them with senior management. This analysis has also prompted employers to take action, with 38% using it to inform or revise their HR practices and 26% developing plans or strategies to address gender issues.
However, relatively few (11%) had communicated or published the results of their gender analysis externally. This finding supports the move to mandate publication of GPG data through the new transparency regulations. It should be noted that public sector organisations were considerably more open in this respect, with 42% having communicated the results externally (compared to 11% of voluntary and just 4% of private sector employers). This may be linked to the Public Sector Equality Duty, which requires public bodies with over 150 employees to report on the diversity of their workforce, as some organisations had published gender pay gap data as part of this.
1.4 Reducing the GPG
Employer attitudes to reducing the GPG varied widely, with 24% allocating it a high priority, 37% a medium priority and 33% a low (or non) priority.
Those treating their GPG as a high priority were typically motivated by moral or ethical considerations, such as a desire to be fair, provide equal opportunities. However, one-fifth (20%) identified the new regulations as the key driver.
Employers that allocated a lower priority to reducing their GPG often did so because they believed they did not have a (large) gender pay gap. However, a significant proportion felt it did not apply because all their workers were already paid equally regardless of gender, suggesting a degree of confusion between GPG and equal pay.
The qualitative research found that although there was a wide range of attitudes towards GPG, the fact that it was often ‘new’ to employers and had not been given much consideration to date meant that a somewhat passive mindset was common. Many of the interviewed employers were waiting until they had measured and analysed their GPG data before thinking in detail about whether and how to address any gap.
Reflecting this, the majority of employers had not yet developed any plans to reduce their GPG, with 50% intending to do so but 20% having no intention to take any action. While one-fifth (21%) had developed a formalised GPG plan, only 6% had already undertaken any of the actions in this plan. The proportion that had already developed a formal plan was highest among 1,000 or more employee organisations (34%).
However, the qualitative interviews found that few of these plans had been put in place specifically to address GPG. They either related to broader gender equality strategies (not developed with GPG in mind) or referred to a range of ad hoc measures implemented for various purposes (for example, increasing staff retention, attracting more female staff).
The most common measures included in these action plans were offering or promoting flexible working (71%) and promoting parental leave policies that encouraged both men and women to share childcare (65%). Half (51%) involved trying to change the organisational culture and over one-third included voluntary internal targets (39%) and women-specific recruitment, promotion or mentoring schemes (35%).
There appeared to be some reluctance to publicise GPG reduction measures, with only one-third (33%) of those that had or intended to develop a plan indicating that they would publish this externally (for example, on their website, in their annual report).
The greatest perceived barrier to reducing the GPG was difficulty attracting women to the organisation or to certain roles, with 14% mentioning the challenge of recruiting or promoting women, 10% highlighting the male-dominated nature of their sector and 5% identifying the fact that women do (or apply for) different types of job. These issues were most prevalent in the construction, manufacturing and financial sectors.
1.5 The GPG transparency regulations
Awareness of the new GPG transparency regulations was high (88%). In terms of knowledge, over half (54%) stated that they understood what was required and how to do it, and a further fifth (20%) felt that they knew what was required but were uncertain of how to go about it. The level of understanding increased in line with organisation size.
Perhaps reflecting the fact that they still had a year before the GPG reporting deadline, half of employers had done relatively little preparation by the time of the survey (30% had reviewed the requirements but nothing more, 7% had not thought about them at all, and 11% were unaware of them). The remainder believed they were already in a position to meet the regulatory requirements (17%) or had developed a plan for how they would do so (32%).
The larger the employer, the more likely they were to have prepared for the regulations. 60% of those with 1,000 or more employees reported that they were already able to meet them or had drawn up a plan, compared to just 42% of those with 250 to 499 employees.
Readiness was also greater in the public sector, with 62% of these organisations able to meet the regulations or with a plan in place to do so.
Generally, those employers interviewed in the qualitative stage regarded complying with the regulations as a high priority, usually because it was a legal requirement and they were keen to avoid penalties. However, although important, they did not see it as an urgent matter (due to the reporting timescales). As such, they tended not to have firm timetables in place for the compliance process and these tasks were often fitted in around other commitments.
If employers needed assistance in complying with the new GPG transparency regulations, most stated they would contact Acas (30%), a legal professional or advisor (30%), an external consultant (23%) or GEO (17%). Over half (59%) had already read the GEO and Acas guidance on GPG reporting.
The most widely mentioned types of support required were guidance on how to report their GPG (19%) and on how to measure their GPG (18%). However, one-third of employers (32%) did not feel they would need any external assistance, and the qualitative research suggests that most employers found the process of collating data and making calculations to be straightforward. It should be noted that employers were yet to tackle the reporting and interpretation of their GPG data, which was anticipated to be the most challenging aspect.
The majority (57%) of employers had not yet decided on a publication date. Many of the qualitative interviewees indicated they were delaying publishing their gender pay data until others had done so, in order to see how other organisations presented and explained the data.
The quantitative survey was conducted in March and April 2017, and at this point a significant proportion of employers intended to publish their GPG data much earlier than the April 2018 deadline, with 18% planning to do so in the first 2 quarters of the 2017 to 2018 financial year. However, it should be noted that the proportion of employers who actually went on to report their results in this time period was significantly lower than implied by the survey results. The initial qualitative research found that employers’ publication plans were not set in stone, which may explain the lower than expected rate of early reporting. Most interviewees described general ambitions, rather than set dates, and there was evidence of these being pushed back in response to other priorities.
Further qualitative interviews among employers that had intended to publish in the first quarter of the 2017 to 2018 financial year confirmed that other issues or tasks had often taken priority over early compliance with the GPG regulations. In addition, these interviews found that some employers had found the process more involved than expected, others had delayed their activity due to circumstantial factors such as restructuring of the organisation or delays in receiving data from third party payroll systems providers, and a few had published their GPG results on their own websites but not on the government portal.
Just 1 in 5 employers intended to publish any additional information alongside the mandatory reporting, and in most cases this was a narrative commentary on the results (15%). Private sector organisations were least inclined to go beyond the basic requirements, with 83% either not planning to produce additional information or unsure as to whether they would do so.
A minority of organisations intended to adopt a comprehensive and active approach to communicating their GPG results (16% for employees and 11% for external stakeholders). However, most either indicated that this would depend on the results or were unsure as to their communication strategy.
2. Introduction
This report provides the findings from a study commissioned by the Government Equalities Office (GEO) and carried out by OMB Research. The research provides evidence on large employers’ understanding of the gender pay gap (GPG), the action they are taking to close it, and their response to the new GPG transparency regulations.
The research used both quantitative and qualitative methodologies and was conducted between March and May 2017. [footnote 2]
2.1 Background
The government has committed to eliminate the gender pay gap within a generation. The GPG is an overall measure which reflects differences in median [footnote 3] hourly earnings and labour market participation by gender. Currently the overall gender pay gap for all employees (18.1%) is the lowest since records began. [footnote 4]
Employers are well placed to tackle many of the issues that drive the GPG. In 2011, the government launched the Think, Act, Report initiative, a set of principles and suggestions on how to improve gender equality in the workplace. While over 300 businesses signed up to Think, Act, Report only a small proportion of these voluntarily published gender pay gap information.
New regulations introducing mandatory gender pay gap reporting for large employers should encourage employers to take informed action to close their GPG where there is one. These regulations came into force in April 2017 and require private and voluntary sector organisations with 250 or more employees to publish GPG statistics every year. The same requirements have been introduced for public sector organisations in England (and non-devolved authorities operating across Great Britain) by amending the Specific Duties regulations made under Section 153 of the Equality Act 2010.
The Government Equalities Office (GEO) commissioned OMB Research to develop a robust research programme to provide a baseline measure of large employers’ understanding of the GPG and the transparency regulations, and understand the actions they are taking to close their GPG.
The primary aims of the research were:
- to provide insight on employers’ understanding of the GPG, including current levels of awareness of the GPG, understanding of the transparency regulations, ability to interpret GPG statistics and understanding of the factors that influence their GPG
- to understand how employers are planning to comply with the regulations, including when they plan to publish their statistics and what support employers think they need to be compliant
- to gather detail on the actions employers are planning or taking to address their GPG and employers’ experiences of taking action
- to understand the perceived barriers to taking action
The core elements of this research took place between March and May 2017, so coincided with the introduction of the new GPG transparency regulations. We acknowledge that there was therefore heightened attention on GPG at the time of the survey, but do not expect this to have had a significant bearing on the results.
2.2 Methodology
The research consisted of a quantitative survey of large employers, supplemented by qualitative depth interviews with a selection of those interviewed in the main survey.
Quantitative survey
Telephone interviews were conducted with 900 large employers between 10 March and 28 April 2017, and covered private, voluntary and public sector organisations with 250 or more employees. Interviews lasted an average of 22 minutes and were conducted with HR directors or managers or other senior staff able to talk about their organisation’s strategy in relation to gender pay differences. The survey communications positioned the research as focusing on gender in the workplace but did not specifically reference GPG.
The sample was provided by the Office for National Statistics and was sourced from the Inter-Departmental Business Register (IDBR), which has comprehensive coverage of large employers. All employers in Northern Ireland, and public sector organisations in Scotland and Wales, were excluded from the sample as they are not subject to the GPG transparency regulations.
Quotas were set on broad sector, size band and Standard Industrial Classification (SIC) code to ensure good coverage of the large employer population. While these quotas were largely representative of the target population, the voluntary and public sectors were over-sampled to allow robust sub-analysis. For example, voluntary sector organisations account for 13% of the large employer universe but made up 27% of the interviews conducted. This resulted in statistical confidence intervals of:
- plus or minus 4.7% for the private sector
- plus or minus 5.6% for the voluntary sector
- plus or minus 5.5% for the public sector [footnote 5]
Table 1 sets out the profile of all large GB employers subject to the GPG transparency regulations, and the profile of the achieved interviews.
Table 1: Universe and achieved interviews by sector and size
Overall, 91% of the interviews were conducted with organisations based in England, 6% in Scotland and 3% in Wales. This exactly replicates the geographical distribution of the larger employer universe.
The final survey data was then weighted back to the true profile of large GB employers, with the weights applied based on a combination of size (employee numbers) and sector.
Qualitative depth interviews
In addition to the main survey, a total of 30 qualitative follow-up interviews were completed during May 2017. These depth interviews were conducted by telephone and lasted an average of 30 minutes.
The sample consisted of respondents to the initial quantitative survey who had given consent to be contacted for further GEO research on the gender pay gap. Interlocking quotas were set on level of engagement with GPG (derived from the survey data [footnote 6] ) and size of business. The quotas were intentionally skewed towards employers that were less engaged with GPG, in order to better understand why they did not consider it to be a priority.
The achieved interview profile is set out in the following table.
Table 2: Qualitative interviews by GPG engagement and size
Further quotas were set on broad sector. Overall, 19 of the qualitative interviews were conducted with the private sector, 6 with the voluntary sector and 5 with the public sector. As far as possible within the constraints of the above quotas, a representative spread was also achieved by SIC and region (including coverage of the devolved administrations).
Following analysis of the main research findings, further qualitative interviews were then conducted among employers who had indicated an intention to publish their GPG results in quarter 1 of the 2016 to 2017 financial year, but who had not yet done so via the GPG reporting portal. The purpose of this additional stage was to understand the reasons for not publishing within the intended timelines. We conducted 22 qualitative interviews lasting 5 to 10 minutes among a cross section of these employers. Results from this additional stage have been referenced at various points in this report, and full details of the methodology, sample and key findings can be found in Annex B.
2.3 Analysis and reporting conventions
This report contains findings from both the quantitative survey and the qualitative follow- up interviews. Where results are based on the qualitative data, this is clearly identified.
Quantitative reporting
Throughout this report, references to ‘all employers’ and the ‘total’ column in the charts and tables refer only to the employer population sampled for the survey – GB private, public and voluntary sector organisations with 250 or more employees.
Unless explicitly noted, all quantitative findings are based on weighted data. Unweighted bases (the number of responses from which the findings are derived) are displayed on tables and charts as appropriate to give an indication of the robustness of results.
The quantitative data presented in this report is from a sample of large employers rather than the total population. This means the results are subject to sampling error.
Differences between sub-groups are commented on only if they are statistically significant at the 95% confidence level (unless otherwise stated). This means that there is at least a 95% probability that any reported differences are real and not a consequence of sampling error. [footnote 7]
When interpreting the data presented in this report, please note that results may not sum to 100% due to rounding or due to employers being able to select more than one answer to a question.
Qualitative reporting
It should be noted that the qualitative phase of the research was based on interviews with a small sample of employers. Although the weight of opinion has sometimes been provided for clarity and transparency, these findings should be treated as indicative and cannot necessarily be extrapolated to the wider population.
Direct quotations have been provided as illustrative examples. However, in some cases these have been abbreviated or paraphrased for the sake of brevity and comprehension (without altering the original sense of the quote).
3. Understanding of the GPG
This chapter explores employers’ awareness and understanding of the gender pay gap. More specifically, it covers:
- awareness of the term “gender pay gap”
- understanding of what the GPG refers to and how it is calculated
- understanding of the difference between closing the GPG and ensuring equal pay between men and women
3.1 Awareness and understanding of the GPG
Overall, almost half (48%) of respondents felt they had a good understanding of what the GPG is and how it is calculated. Only 2% had never heard of the term “gender pay gap”. However, the qualitative interviews suggested that not all of those who claimed good knowledge of GPG actually had a full or correct understanding of this, as discussed later in this chapter.
Figure 1: Self-reported understanding of the GPG
Base: All respondents (Base, Don’t know). Total (900, 0%), Private (406, 0%), Voluntary (243, 0%), Public (251, 0%), 250 to 499 (412, 0%), 500 to 999 (214, 0%), 1,000 or more (274, 0%)
Public sector organisations and those with 1,000 or more employees were most likely to report that they had a good understanding of the GPG (58% and 60% respectively).
However, there were no differences based on the proportion of women on the senior management team, or in the organisation’s workforce as a whole.
Those claiming a good understanding of what the GPG is and how it is calculated were also more likely to have read the GEO and Acas guidance on the subject (76% versus 42% of those with less comprehensive understanding).
Qualitative insight
Evidence from the qualitative interviews provides further detail about levels of understanding of the GPG, how it is calculated and how to interpret it. While some of those claiming a good understanding of what the GPG is and how it is calculated could provide a detailed and accurate description, others were not able to correctly describe this. Their unprompted descriptions were sometimes vague, and sometimes incorrect (mostly due to a confusion between GPG and equal pay).
“It’s measuring the average pay of people doing the same job, making sure it is the same for men and women.” (250 to 499 employees, private sector)
Many explained that they had been unaware of the definition of the GPG before the new regulations were announced, and their understanding was primarily gained from either reading GEO and Acas guidance on the topic or from attending seminars or training courses run by law firms, recruitment agencies or professional bodies. Those reporting a good prior knowledge had often previously measured their GPG and other related gender statistics. Most were public sector organisations.
“Yes, it is the mean and median pay of men and women in the workforce. It is similar to what we have measured before, but the calculations have changed slightly with the new regulations.” (500 to 999 employees, public sector)
Those reporting limited or no understanding of the GPG and how it is calculated generally explained that they had not yet taken the time to fully engage with the new regulations and associated guidance. They were likely to confuse GPG with equal pay, or in some cases simply state that they were not sure what the GPG was beyond its connection to men and women’s pay.
“Well, that’s a difficult one. I am not sure exactly what it means. We haven’t really given it the time to find out yet.” (1,000 or more employees, voluntary sector)
The qualitative interviews also suggested a lack of understanding about how to interpret GPG data. Some respondents explained that they were unsure exactly what the different measures signified about their workforces and organisations. Others were particularly concerned about how best to present the data to protect their reputations as fair employers.
3.2 Understanding of difference between GPG and equal pay
Almost two-thirds (63%) of respondents believed they had a good understanding of the difference between ‘closing the gender pay gap’ and ‘ensuring equal pay between men and women’. A further 30% knew there was a difference but were not sure of the detail, and just 6% had either not heard of the GPG or did not know it differed from equal pay.
Figure 2: Understanding of the difference between ‘closing the GPG’ and ‘ensuring equal pay’
Base: All respondents (Base, Don’t know). Total (900, 1%). Private (406, 2%), Voluntary (243, 0%), Public (251, 0%). 250 to 499 (412, 2%), 500 to 999 (214, 1%), 1,000 or more (274, 1%)
The proportion with a good understanding was highest among public sector organisations (76%). It also increased in line with size, ranging from 57% of those with 250 to 499 employees up to 71% of those with 1,000 or more employees.
Three-quarters (74%) of those that had read the GEO and Acas guidance on the GPG had a good understanding of how this differed from equal pay (compared to 46% of those not reading the guidance).
It should be considered that this data refers to respondents’ own perceptions of their understanding, and other evidence from this research indicates that this may not always be wholly accurate. A significant proportion did not see closing their GPG as a priority because they already paid equally regardless of gender (see Chapter 5.1), suggesting a degree of conflation between the concepts of GPG and equal pay. This is consistent with the qualitative findings, as discussed below.
During the qualitative interviews, when asked to provide a definition of GPG, most respondents included reference to equality of pay between men and women working in the same roles. The difference between GPG and equal pay was not always clearly described, and many respondents felt that the 2 concepts overlapped or explained that they were confused about exactly how they differ.
“It is the difference in pay between men and women, but I am not sure exactly. I think there is a real blurring between this and equal pay.” (1,000 or more employees, public sector)
However, a number of respondents were more informed and explicitly mentioned that GPG was not the same as equal pay.
“Gender Pay Gap is the difference in average pay between men and women, but not just those doing the same job…it is not the same as equal pay.” (250 to 499 employees, voluntary sector)
Some respondents expressed concern that their staff and prospective candidates would not understand the meaning of GPG or how it differs from equal pay. They were worried that if their organisation reported a significant GPG this could be interpreted as them paying men and women different amounts for doing the same job.
“It is definitely a confusing measurement. I am sure that most people would not know the difference between this and equal pay. I am worried about what the staff might think when the numbers are published, whether they will think it means we don’t pay men and women equally.” (1,000 or more employees, private sector)
Some added that without more comprehensive and impactful communication from government on this topic, there was potential for businesses to be viewed negatively by their customers and the public at large.
3.3 Leadership team’s understanding and engagement
When asked specifically about their leadership team or board, most (54%) felt that this group had a ‘fairly’ good understanding of the gender pay gap and the difference between this and equal pay. Only a minority (17%) described their leadership team as having a ‘very’ good understanding of this issue.
Table 3: Leadership team’s perceived understanding of GPG and the difference from equal pay
Base: All respondents
Public sector organisations and those with 1,000 or more employees had the most confidence in their leadership team’s understanding of this issue, with more than three-quarters (79% and 77% respectively) judging this to be ‘very’ or ‘fairly’ good.
Qualitative exploration suggested that consideration of GPG as a specific issue had not been commonplace in the past. As such, senior management did not always have a clear understanding of the purpose of measuring GPG or the benefit to them or their employees. Engagement in the topic was therefore often said to be limited to, or focused on, how to comply with the regulations and the impact that publishing data would have.
In cases where the leadership team was said to have a strong understanding of the difference between GPG and equal pay, GPG had often been part of a broader strategy to measure and hit targets on diversity and equality. Respondents in these organisations also more commonly described senior leaders as the driving force behind both compliance with regulations and tackling GPG more generally.
“The board are all very aware of this issue, as with gender issues in general. They have implemented a range of policies and strategies in recent years.” (1,000 or more employees, private sector)
“Equality and diversity are really at the heart of everything we do here. The senior team are keen to make sure we measure this type of data and consider the diversity implications of all new policies and procedures.” (500 to 999 employees, public sector)
Some respondents explained that understanding of GPG was inconsistent within their leadership team. They noted that those in the leadership team with an HR or finance focus were more likely to be engaged in, and knowledgeable about, GPG.
HR managers sometimes reported that they had introduced GPG and the new regulations to their leadership team, who would otherwise have been unaware of it. However, a number felt that this information had probably been forgotten by most or all senior managers, and was not likely to be mentioned again until the GPG figures had been shared with them.
“I prepared a report on the dry run data a few months ago, so they know it is coming. But I don’t think they are giving it much thought at the moment.” (250 to 499 employees, private sector)
In some cases, respondents were unsure what senior manager understood about GPG. Some assumed that board members would have taken time to learn about the issue, while others were more sceptical. These more sceptical respondents explained that the leadership team were either disengaged with topics relating to gender equality in general, or were confident that they did not have a GPG and therefore were simply not concerned about it.
“This is a family business, run in a particular way. The board have lots of other things on their mind. They won’t have given this any thought yet.” (250 to 499 employees, private sector)
The qualitative interviews provide some evidence that a lack of understanding at board level can directly result in resistance to engagement with GPG and the new regulations. Some senior managers were concerned that their GPG might give the impression that their organisation did not pay men and women equally for doing the same work. They were not sure exactly what would be measured and how, leading to some ‘fear of the unknown’.
“I think some of the senior team were quite concerned about this when they first heard about the regulations. They were worried about being seen as paying unequal wages to men and women. I don’t think they fully understood it.” (500 to 999 employees, private sector)
4. Measuring the GPG and other gender analysis
This chapter looks at the extent to which employers had previously conducted analysis to identify differences between men and women’s pay in their organisation. Specifically, it covers:
- whether employers have calculated their GPG
- whether the GPG was measured in a similar way to that required by the new transparency regulations
- other types of gender analysis carried out
- how the GPG or other gender analysis is communicated and used
4.1 Measuring the GPG
Almost one-third (31%) of organisations had measured their GPG in the last 12 months, although only half (52%) of these respondents knew what their GPG was at the last measurement.
The mean GPG among organisations that had measured it in the last 12 months (n=159) was 14%, compared to the national average of 18%. However, it may be that those voluntarily measuring their GPG prior to the regulations coming into force were more engaged with the topic and therefore not representative of the wider population of large employers. This is supported by the qualitative findings, as discussed later in this chapter.
Figure 3: Employers measuring their GPG in last 12 months
Base: All respondents (Base, Don’t know if measured GPG). Total (900, 7%). Private (406, 7%), Voluntary (243, 8%), Public (251, 3%). 250 to 499 (412, 7%), 500 to 999 (214, 6%), 1,000 or more (274, 7%)
Organisations with 1,000 or more employees were most likely to have measured their GPG (47%). Smaller organisations (250 to 499 employees) were least likely to have done so (23%), but reported the highest mean GPG (18%).
Public sector organisations were also more likely to have measured their GPG, and their mean GPG was higher (17%, compared to 14% for private and 12% for voluntary).
Again, it should be considered that those that had already measured this may not be reflective of all large employers, and may have lower than average GPGs.
There are a number of reasons why the public sector may be more engaged with the GPG issue. Firstly, public bodies with over 150 employees were already reporting on the diversity of their workforce under the Public Sector Equality Duty, and some of the interviewed employers may have undertaken gender pay gap analysis as part of this.
This was supported by the qualitative interviews, which found a particularly formalised and strategic approach to monitoring equality and inclusivity among public sector organisations. Secondly, early compliance with the regulations is arguably more important to the public sector from a reputational perspective.
Respondents who had calculated their GPG were asked a series of questions to determine whether they had done so in a way that was broadly consistent with the approach required under the new government legislation on gender pay transparency.
Four-fifths (80%) had calculated the median pay gap, two-thirds (69%) had presented the difference as a percentage of male average pay and a similar proportion (66%) had calculated it based on hourly pay (rather than annual salary).
Figure 4: Consistency in GPG reporting
80% calculated the median pay gap.
4% just calculated the median gap, and 75% calculated both the median and the mean.
8% just mean.
12% don’t know.
69% presented difference as percentage of male average pay.
22% don’t know.
66% calculated on hourly pay.
52% calculated just on hourly pay, and 14% calculated on both hourly and annual pay.
26% annual pay.
8% don’t know.
Base: All that have measured GPG (Base). Total (291)
As this demonstrates, some organisations calculated their GPG in a way that is not entirely consistent with the mandated approach. However, when the mean GPG was analysed solely based on those using an approach that matches that set out in the regulations, it remained at 14%.
Among the qualitative sample, most of those interviewees who had measured their GPG in the previous 12 months had done so as a ‘dry run’ in advance of the regulations coming into force. This suggests that the incidence of ‘true’ voluntary GPG measurement (not driven by the regulations) may be low.
“We did a dip back in December, really just to see if there was anything that we needed to worry about…no, we would not have done that were it not for the regulations.” (500 to 999 employees, private sector)
The small minority of organisations that had measured their GPG for non-regulatory reasons were more focussed on gender and equality issues, and also reported that they were already taking action on GPG.
4.2 Conducting other gender analysis
Respondents were also asked whether their organisation had done any other gender analysis in the last 12 months.
Table 4: Other gender analysis undertaken in last 12 months
Over half (58%) of large employers reported that they had had conducted other gender analysis in the previous 12 months. Two-fifths (41%) had analysed the proportions of male and female employees at different pay levels, one-third (31%) had measured the proportions paid bonuses, and one-quarter (24%) had calculated the difference in the average bonuses paid.
One-third (31%) of organisations had also conducted some ‘other’ type of analysis looking at differences between male and female employees. When asked to provide details, the most widely mentioned analyses were assessing the gender balance at different levels of seniority (8% of all organisations), detailed salary bands by gender (5%), the gender balance of new employees (5%) and promotion rates by gender (4%).
Larger organisations with 1,000 or more employees were considerably more likely to have conducted each of the types of gender analysis detailed in Table 4.
Public sector organisations were most likely to have measured the proportions of males and females at different pay levels and undertaken ‘other’ gender analysis. However, private sector organisations were comparatively more likely to have assessed the proportions paid bonuses and the average value of these bonuses. This was particularly true of private sector firms with 1,000 or more employees (54% had analysed the proportion paid bonuses and 46% had calculated the difference in the value of these bonuses by gender).
Those organisations that had measured their GPG in the past 12 months were significantly more likely to have also conducted other types of gender analysis (87% versus 45% of those that had not measured their GPG).
4.3 Communicating and using GPG and other gender analysis
When asked about how they had used any of their GPG or other gender analysis, the most commonly reported actions were sharing results with the leadership team or board (66%) or senior management (62%). A further 38% indicated that it had informed their HR policies or practices and 26% had developed a plan or strategy to address the issues identified.
However, relatively few employers (11%) had communicated or published the results of their gender analysis externally.
Table 5: How employers have communicated and used their GPG or gender analysis
Base: All that have measured GPG or done other gender analysis
Smaller employers and those in the private sector were least likely to have shared the results of any gender analysis with internal audiences (for example, leadership team, senior management, the wider workforce) or to have amended their HR practices as a result.
Public sector organisations were considerably more transparent with their gender analysis, with 42% communicating the results externally and 43% sharing them with their wider workforce. In contrast, only 4% of private sector employers had published their results.
5. Reducing the GPG
This chapter looks at the extent to which employers are seeking to reduce their gender pay gap, and the approaches they are adopting to do so. Specifically, it covers:
- the degree to which reducing the GPG is a priority, and the reasons behind this
- the extent to which employers have developed (and acted on) plans to reduce their GPG
- the specific actions or measures developed to reduce their GPG
- the extent to which the success of these measures is evaluated
- the main challenges or barriers to reducing the GPG
5.1 Priority given to reducing the GPG
There was a broad spectrum of employer attitudes when it came to the perceived importance of reducing their GPG (or ensuring they continued to have no GPG in the long term). Overall, one-quarter (24%) considered this to be a high priority, one-third (37%) a medium priority, one-fifth (21%) a low priority, and 13% judged it not to be a priority at all.
Figure 5: Priority given to reducing the GPG
Base: All respondents (Base, Don’t know). Total (900, 5%). Private (406, 5%), Voluntary (243, 6%), Public (251, 4%). 250 to 499 (412, 5%), 500 to 999 (214, 4%), 1,000 or more (274, 6%)
Public sector organisations and those with 1,000 or more employees were comparatively more likely to allocate a high or medium priority to reducing their GPG (73% and 72% respectively).
Employers with greater female representation at senior management level were less inclined to treat the GPG as a high priority (13% of those where over three-quarters of the management team were female, compared to 26% of those where less than a quarter of the management team were female). This was largely because they did not believe they had a pay gap. This is consistent with the fact that, among those that had measured it, the average GPG was lower among those with a higher proportion of female senior management.
The figure below details organisations’ reasons for the priority given to reducing their GPG. This was captured via an open question, with responses coded into common themes. All reasons mentioned by 5% or more of respondents in each group have been shown.
Figure 6: Reasons for priority given to reducing the GPG (unprompted)
High priority:
- Right thing to do or want to be fair or non-discriminatory – 34%
- Important for us to provide equal pay or opportunities – 21%
- Legal requirement or regulation – 20%
- Important to address our GPG or already working to reduce (or maintain) it – 14%
- Company reputation (for example, image, attracting staff) – 10%
- Want to know reasons or extent of gap or address any issues – 8%
- Have a GPG or gender imbalance in workforce (or certain areas) – 8%
Medium priority:
- Our GPG is small or not a big issue – 14%
- Don’t have a GPG or mainly female workforce – 13%
- Haven’t calculated GPG yet or not sure if it’s an issue – 13%
- Have a GPG or gender imbalance in workforce (or certain areas) – 10%
- Other more important priorities – 9%
- Important to address our GPG or already working to reduce (or maintain) it – 9%
- Right thing to do or want to be fair or non-discriminatory – 9%
- Legal requirement or regulation – 8%
- All workers are paid equally regardless of gender – 7%
- Nothing or little we can do (for example, nature of sector, few female applicants) – 6%
Low priority:
- Don’t have a GPG or mainly female workforce – 24%
- Other more important priorities – 19%
- Nothing or little we can do (for example, nature of sector, few female applicants) – 16%
- Our GPG is small or not a big issue – 15%
- All workers are paid equally regardless of gender – 14%
- Have a set pay scale or structure – 9%
- We employ or pay based on ability not gender or other factors – 8%
- Haven’t calculated GPG yet or not sure if it’s an issue – 5%
Not a priority at all:
- Don’t have a GPG or mainly female workforce – 40%
- All workers are paid equally regardless of gender – 23%
- We employ or pay based on ability not gender or other factors – 14%
- Our GPG is small or not a big issue – 9%
- Have a set pay scale or structure – 8%
Base: All respondents. High (226), Medium (352), Low (176), Not at all (100). Top mentions (5% or more)
Among those allocating a high priority to reducing their GPG, this decision was primarily driven by moral or ethical considerations, such as a desire to be fair, provide equal opportunities. However, one-fifth of this group (20%) also highlighted the new regulations as a motivating factor.
Very few employers explicitly mentioned profit-related motives for addressing their GPG, suggesting that employers do not typically see a direct link between GPG and business performance. However, some of the more common motivations can be bridges to improved business performance (for example, company reputation).
The perceived lack, or small scale nature, of their GPG was the most significant reason given by those treating it as a medium, low or non priority. However, there also appears to be some confusion between equal pay and the GPG, with 23% of the non-priority and 14% of the low priority group indicating that this was because all their workers were already paid equally regardless of gender.
A significant proportion of employers also identified barriers to reducing their GPG as a reason for affording it a comparatively low priority (for example, there was little they could do due to the broader gender imbalance in their sector, a lack of female applicants, the fact that they had to follow a set pay scale).
The qualitative interviews further explored the level of priority afforded to reducing GPG, the reasons for this and who within the organisation was driving this.
In the vast majority of cases, respondents explained that the priority allocated to this issue was ultimately driven by the senior leadership team. HR staff were often said to be the driving force behind compliance with the regulations (see Chapter 6), and in some cases a push to improve opportunities for female staff. However, decisions to change policies and approaches on remuneration, recruitment, working conditions or contracts typically required approval from board members (or equivalent).
“I am not sure what the board will do. We will present the information to them, but it is up to them whether they take action.” (250 to 499 employees, private sector)
In a minority of cases, organisations (mainly public sector) reported that the strategic priority placed on closing GPG had been assessed along with a raft of other equality and diversity issues. They explained that these priorities were set on an annual basis, based on the perceived severity of the issue. They noted that going forward, the new GPG measurements required under the regulations would form an important source of evidence for these reviews.
The priority that employers placed on closing their GPG (or ensuring one did not develop) depended on a number of factors. These are illustrated in Figure 7 below.
Figure 7: Factors impacting priority placed on closing GPG
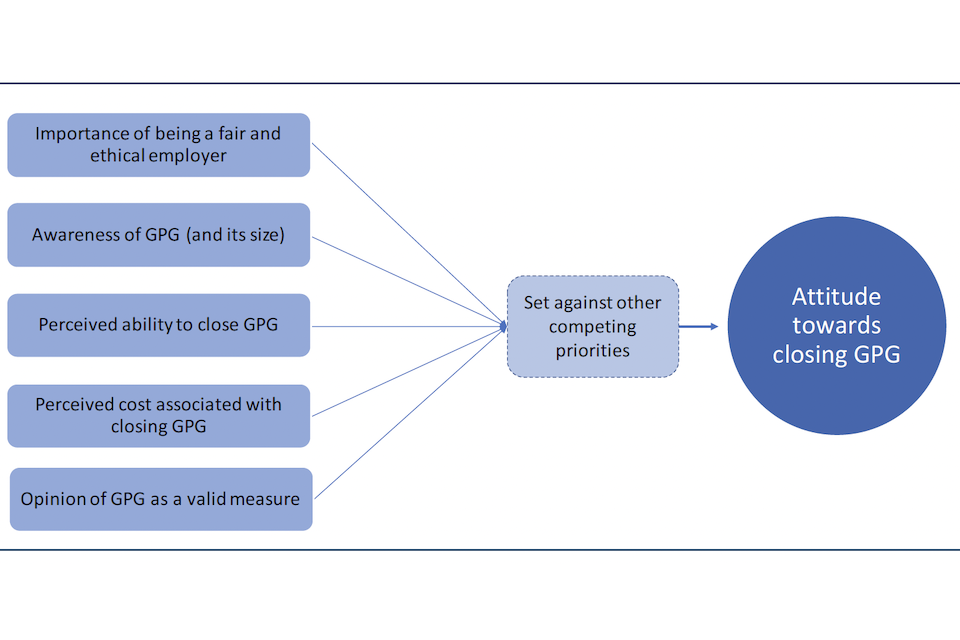
These factors are defined as follows.
Importance of being a fair and ethical employer
The degree to which organisations place importance on looking after their employees and giving them opportunities regardless of their gender or any other defining characteristic.
Awareness of GPG (and its size)
Whether an employer knows (or thinks) it has a GPG, its size, and what is causing it. This will determine its perceived negative impact on staff, reputation, recruitment and sales.
Perceived ability to close GPG
Whether an organisation believes there is anything (more) that they can do to reduce their GPG or avoid one opening up.
Perceived cost associated with closing GPG
What an employer believes the likely costs to them will be of closing their GPG (direct financial costs as well as time and resources associated with taking steps).
Opinion of GPG as a valid measure
Whether employers consider GPG as a ‘valid’ indication of how fair and ethical they are, and therefore whether they feel that closing their GPG is necessary or appropriate.
When considering these factors, it is also important to note that employers usually described multiple reasons for their current GPG priority. Furthermore, their views were often complex and multifaceted. For example, while an organisation might have a strong desire to be a fair employer and see the value in having a low (or no) GPG, it might also assume that it has no significant problem in this area, hence reducing the priority given to the issue.
In addition, the priority afforded to closing their GPG was always set against the relative importance of other issues, challenges and ambitions that the organisation faced. In this context, no employers in the qualitative sample described closing GPG as one of their top priorities overall.
5.2 Attitudes towards reducing the GPG
The qualitative research identified 4 broad groups in terms of employers’ attitudes to reducing their GPG, as summarised below.
Figure 8: Range of attitudes towards closing GPG
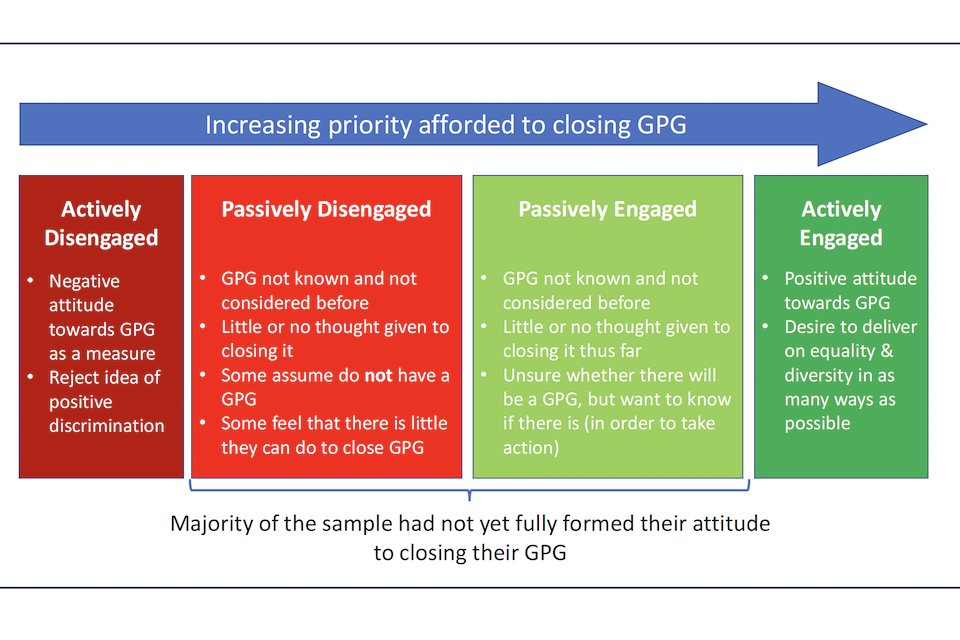
Actively engaged
A proactive attitude to addressing GPG was described in a minority of cases. These employers described a strong overriding desire to ‘do the right thing’ and be as fair and open as possible. This ambition was driven by the culture of the organisation and, in the case of some charities and public sector bodies, by the strategic priorities set out by senior leaders or trustees.
In some of these more proactive organisations (typically public sector or large employers), monitoring GPG as an indication of equality performance was considered important regardless of whether they had identified a large gap. Some explained that while they had no GPG, they considered it a priority to maintain this scenario (along with other related equality and diversity measures).
In a minority of cases, larger employers were highly motivated to deal with what they felt was an unacceptably high GPG within both their organisation and their sector as a whole. They described motivated senior leaders who were keen to deliver a shift in culture both internally and across the wider population. Others explained that they were keen to address some of the root causes of their GPG (higher rate of female staff turnover) for commercial reasons. For example, some in professional service sectors noted the high cost of training staff and the fact that this investment is ‘lost’ when these staff leave.
Passively engaged
As outlined previously, most employers had not considered GPG as a specific topic before the announcement of the new regulations, and most had not yet calculated and analysed their GPG. As such, many were unsure of the degree to which closing it would be a priority in the future, and described their attitude as one of ‘wait and see’.
These employers broadly accepted that GPG could be another useful measure of their performance in terms of being a fair and ethical employer. As such, they anticipated that were any GPG to exist (which many of this group felt was possible), it would be a priority to deal with it.
They generally recognised the potential benefits associated with reducing their GPG. In some cases, employers described these benefits in ‘altruistic’ terms, with a focus on delivering what is ethically the right thing to do. In others, the motivation to consider closing their GPG related to the potential commercial benefits associated with doing so. They explained the importance of being seen as an employer of choice, enabling them to attract and retain high calibre staff.
A minority anticipated that their (potential) customers were likely to become increasingly interested in knowing their GPG figures. Therefore, the potential to win business could be impacted if their GPG was high compared with the sector. They anticipated that this could have an impact on the priority placed on closing their GPG in the future.
Passively disengaged
Some employers described GPG as an issue that they had not considered before, but thought that they would (probably) not need to address it in the future, beyond meeting their regulatory reporting requirements.
In some cases, employers assumed that they had no GPG to deal with. A small minority had already measured their GPG, but others based this opinion on their high percentage of female employees (including at a senior level) or their rigid pay structure. Some also reported a considerable focus on equality and diversity at a general level, believing that this meant they did not have a GPG to be concerned about. It is important to note that a number of these employers displayed a limited understanding of GPG and how it differed from equal pay.
Other ‘passively disengaged’ employers described a lower GPG priority due to an assumption that they would not be able to close it. While they agreed that tackling GPG was a valid ambition, they argued that a lack of trained or interested female candidates meant that they were unable to address the issue. They often felt that they were already doing all they could to attract more female staff. As such they saw little point in prioritising a goal (reducing GPG) that they were not able to achieve. This attitude was most notable in the manufacturing and engineering sectors.
A lack of engagement with closing the GPG was attributed in some cases to a simple lack of interest in the topic among senior leaders. Some respondents in HR positions felt that their senior managers would simply not take much notice of the GPG data. They explained that their leadership teams were focused on delivering core commercial objectives, with issues relating to equality and diversity a relatively low organisational priority. Some reported that their senior leaders were unlikely to place any importance or urgency on closing their GPG unless they noticed an impact on sales or enquiries.
“If we start seeing that clients are asking about GPG it will become more of a priority.” (250 to 499 employees, private sector)
Actively disengaged
In a minority of cases, employers described a definitive and deliberate lack of engagement with closing their GPG. Some were not convinced about the validity of seeking to reduce their GPG ‘at all costs’. They felt that the measure failed to take into consideration broader factors such as the availability of women candidates in the sector or other valid reasons for differentials in average pay. They explained that their priority in relation to their (expected) GPG would be to explain rather than reduce it.
“We do not want to just reduce our GPG to get the number down. There can be legitimate reasons for a GPG.” (1,000 or more employees, private sector)
5.3 Approach to reducing the GPG
One-fifth (21%) of employers had developed a formalised action plan for reducing their GPG, but only one-third of this group (6% of all employers) had already implemented any of the specified actions. Half (50%) of all organisations intended to take action but had not yet developed any concrete plans, and one-fifth (20%) did not intend to do anything.
Figure 9: Employers’ current approach to reducing their GPG
Base: All respondents (Base, Don’t know). Total (900, 9%). Private (406, 10%), Voluntary (243, 9%), Public (251, 8%). 250 to 499 (412, 10%), 500 to 999 (214, 10%), 1,000 or more (274, 7%)
While still in the minority, large organisations (1,000 or more employees) were most likely to have already developed an action plan (34%). In contrast, one-quarter (25%) of those with 250 to 499 staff had no plans to take any action to reduce their GPG.
As might be expected, there was a correlation between the priority allocated to reducing their GPG and the degree of action taken to achieve this. Two-fifths (39%) of those treating it as a high priority had developed a formalised plan, compared to just 7% of those for whom it was a low or non priority. However, even among the high priority group, only 14% had already undertaken some or all of the actions in their plan.
Employers that had already measured their GPG or undertaken other gender analysis in the previous year were significantly more likely to have put together a formalised plan for reducing their GPG (27% versus 12% of those that had not done any gender analysis).
Those organisations that had or intended to take action to reduce their GPG were asked whether they would publish an action plan for this. As seen in the table below, half (48%) intended to publish this internally (to staff within their organisation) and one-third (33%) intended to make it available to external audiences. While only 16% reported that they definitely would not publish their action plan, a further 29% were unsure as to whether this would happen.
Table 6: Proportion of employers that will publish a GPG action plan
Base: All that have, plan or intend to take action to reduce their GPG
Although not shown in the table above, one-quarter (23%) of those asked only intended to publish their plan internally (23%), with 7% only publishing externally and 25% doing both. Public sector organisations displayed the most willingness to publish their GPG action plan externally (51%), followed by voluntary sector organisations (37%).
As detailed previously, 21% of employers had developed a formalised plan or strategy to reduce their GPG. In most cases these plans included measures to offer or promote flexible working (71%) and to promote parental leave policies that encouraged both men and women to share childcare (65%).
Figure 10: Measures included in GPG action plan
Base: All taken or planning action (Base, Don’t know). Total (204, 10%)
The minority of organisations (6%, n=59) that had developed a plan and already undertaken at least some of the specified actions were asked about how and when the impact of these would be evaluated. Most of this group (57%) planned to evaluate the impact as part of a formalised process rather than on an ad hoc basis, and 38% had already assessed the current impact of these actions.
When asked to assess the success of the actions implemented to date, the majority judged them to have been either very successful (23%) or fairly successful (38%). Most of the remainder were unsure or felt it was too early to say.
The qualitative sample was structured to cover employers that had or planned to take action to close their GPG and those that did not plan to do so (based on their responses to the quantitative survey). However, the qualitative interviews revealed some confusion and overlap between these 2 groups, often linked to limited knowledge and engagement with GPG.
Those taking or planning action
Qualitative exploration suggested that the level of formalised planning to take action on closing GPG may be even lower than the survey data suggests. Some interviewed employers in this group described plans which had been put in place specifically to address GPG within the organisation. However, others explained that the plans to which they had referred related to much broader gender equality strategies that had not been developed with GPG in mind.
Other employers interviewed in the qualitative stage explained that the action they were referring to (which may help close their GPG) was not part of any formalised plan as such, but rather a series of ad hoc measures. These had been implemented to address a number of issues, including attracting more female staff to the workplace and increasing staff retention in general, as well as promoting and increasing fairness and equality overall.
“We don’t have a specific plan for GPG, but we do a lot to make sure we have a balanced workforce.” (500 to 999 employees, private sector)
In some cases, the plans referred to in the quantitative survey were in fact not related to reducing their GPG per se. Some of these plans were related to complying with the GPG regulations (analysing and reporting the data) rather than reducing their GPG. Others were related to action aimed at eliminating unequal pay practices.
“We went through the process of moving the pay scales to single status a few years ago.” (250 to 499 employees, public sector)
Those not planning to take action
Although this group indicated in the quantitative survey that they were not planning any action to reduce their GPG, further discussion revealed that many had a number of measures in place which could potentially have an impact on GPG, but these were not designed with that purpose in mind. The most commonly mentioned of these measures included offering enhanced maternity or paternity leave and flexible working. However, the impact of these measures on their GPG had not yet been considered or measured.
“We have a good approach to flexible working, but that’s because we want to retain staff overall. We hadn’t thought about the impact on GPG before.” (250 to 499 employees, private sector)
After further discussion and consideration during the qualitative interviews, some agreed that these measures were likely to have had some impact on their GPG (although they had not yet measured this). Others were doubtful that these measures would have made any difference, suggesting that other factors (for example, lack of female candidates, slow turnover of senior staff) were likely to override any impact on their GPG.
Some employers explained that they did not expect to have a GPG and therefore had no plans to take action, but if they completed their calculations and discovered a (bigger than expected) GPG, they would reconsider.
“We have no plans to take action at the moment, but we will definitely approach this in the spirit it is intended and look at what is needed when we know more.” (500 to 999 employees, private sector)
Actions to reduce GPG
Employers described specific activities in relation to reducing their GPG. These closely mirrored those reported in the quantitative survey. They included the following.
Flexible working
Nearly all businesses in the qualitative sample offered flexible working of some kind. Doing so was often part of a general drive to retain staff or to attract and retain female employees. While only rarely described as a measure designed specifically to reduce GPG, a number of employers attributed their perceived low (or zero) GPG at least in part to these practices.
Offering enhanced maternity or paternity leave
Many employers in the qualitative sample offered enhanced maternity and paternity leave (with those who only offered statutory often explaining that this was due to a lack of available funding). They understood that such policies encourage women and men to share childcare responsibilities, and therefore enable women to return to work (sooner). However, shared parental leave was often described as being unpopular among employees and too complicated for them to take advantage of.
Making cultural changes
In a small number of cases, employers in the private sector were taking steps to encourage different attitudes and behaviour among mid-level management in relation to recruitment, professional development and negotiation of wages. They were providing information and guidance, as well as raising the topic in meetings and reviews. They wanted managers to think more about the equality and diversity implications of their staffing decisions. Some were keen to challenge what they considered to be unconscious biases towards hiring other men rather than women or considering men for promotion more readily. Some suggested that they would be able to use the GPG data as evidence to managers of the need for such a change.
Voluntary internal targets
A small minority of employers had put in place targets on the number of women employed in senior positions. The ratio of men to women targeted varied, depending on the starting point and sector (for example, a legal firm aiming for 30% of partners to be women, a voluntary sector care provider aiming for an equal split of men and women in senior positions).
Women-specific recruitment, promotion or mentoring schemes
Some employers had implemented equality or gender initiatives aimed at increasing opportunities for women to join, stay and progress.
A number had mandated that at least one woman should be invited for interview (if possible), while others insisted that there must be female representation on all interview panels. Some large employers also mentioned providing back to work interviews after maternity leave for their female staff.
Others had put in place mentoring programs aimed at encouraging junior female staff to aim for career progression through advice and guidance from women in senior positions in the organisation.
Reaching out to local education institutions was also mentioned. These employers were working with schools to raise awareness of their professions among female students. They felt that this long-term approach was important to combat one of the primary factors driving GPG.
“We try to have 50% women on all candidate shortlists…we insist on a 50/50 split in senior positions.” (500 to 999 employees, voluntary sector)
Measuring impact
The qualitative interviews pointed to a relatively informal approach to measuring the impact of actions to reduce GPG. Respondents were not able to describe any formal assessment processes linking GPG to specific actions or initiatives. They often explained that closing a GPG would take a considerable period of time, and that it would be difficult to attribute any success to specific measures.
However, where formalised plans were in place to encourage women back to work or to encourage more female applicants (both external and internal), employers were monitoring the direct impact on these particular objectives (rather than on GPG).
Furthermore, some explained that now that GPG measurement was mandatory, this would form part of the formalised assessment of these measures in the future.
5.4 Barriers to reducing the GPG
Respondents were asked to give details of the main challenge to reducing their organisation’s GPG, via an open questioning approach. The table below shows all reasons mentioned by 3% or more of respondents.
Table 7: Main challenge to reducing employers’ GPG (unprompted)
A wide array of different challenges were identified by the surveyed organisations. However, the biggest issue related to difficulties in finding or attracting women to the organisation or to certain roles, with 14% mentioning the challenge of recruiting or promoting women, 10% highlighting the male-dominated nature of the sector and 5% identifying the fact that women do (or apply for) different types of jobs. This barrier was particularly prevalent in the construction sector, with 51% of private sector firms operating in this area giving one of these 3 reasons.
Almost one-quarter of employers (22%) do not envisage any barriers, typically because they do not believe they have a significant GPG. Reflecting the fact that many organisations had not previously examined their GPG, a further 24% were unsure as to whether they would face barriers or felt it was too early to know.
Half (51%) of those organisations that did not intend to take any action to reduce their GPG, and a similar proportion (54%) of those viewing it as a non-priority, did not envisage any barriers. This again illustrates that much of the inertia around the GPG is linked to a perception (correct or otherwise) among these employers that they do not have an issue.
The qualitative interviews confirmed that many employers did not anticipate barriers because they believed that they had no GPG. In addition, respondents explained that until they knew the size and root cause of their GPG, they could not anticipate how to go about closing it or any barriers to doing so. However, a number described specific barriers to taking (more) action to close any GPG, or to the success of any such actions.
Barriers to taking action
Many of these barriers reflected the overall priority placed on closing GPG (as described previously). The relative lack of importance of GPG compared with other issues and concerns about compromising the quality of new recruits through attempting to shift the balance of gender in the workforce were both mentioned.
In addition, some employers described more practical barriers to taking action. The most commonly mentioned was a lack of available funds. Some employers explained that they could not afford to offer enhanced maternity or paternity leave. Others thought that in order to close their GPG they would need to increase the pay of some (female) members of staff. Some noted that introducing initiatives and action plans costs time and money, and that this would not be approved by senior managers unless a clear commercial benefit could be demonstrated. Lastly, a small number of employers were unable to offer flexible working arrangements because it would not be operationally viable within their current business model.
Employers were not always sure exactly what action could be taken beyond making changes to the wages or bonuses of some members of their workforce. This reflects the fact that some employers view GPG through a narrow lens, focussing on financial solutions rather than broader measures to encourage recruitment, retention and career progression among women.
While none of the employers interviewed had sought external support about how to close their GPG, a number felt that this would be useful, suggesting that lack of access to support could potentially be a barrier. Some wanted examples of how other employers had closed their GPG without directly adjusting the salaries or bonuses of staff. Others felt that the government or unions should publish guidance on how to close a GPG (along with the requirements for complying with the regulations).
Barriers to success
During the qualitative interviews, some employers expanded on their perception that a lack of suitable female applicants was a key barrier to closing their GPG. Most attributed this to the sector in which they operated being traditionally male-dominated. They felt that this issue was largely outside of their control as individual employers, although some were attempting to help address it through engagement with local schools and colleges.
“It is really difficult as a big part of the business is a very male dominated area. This is a problem that is bigger than us, it is society.” (500 to 999 employees, private sector)
Some organisations reported a general lack of available candidates (of any gender) in their sector. They explained that this meant they were unable to aim for a particular profile of candidate and simply focused on capability to fulfil the role.
Some employers offered flexible working arrangements but reported low uptake among female employees with children. They explained that most preferred to leave the organisation or take long breaks from work after their children were born. They felt that cultural norms and traditional gender roles were still adhered to strongly. As such, these deep-rooted behaviours were considered difficult to overcome.
A number of employers said that differences in attitude between men and women in the workplace also constituted a barrier to closing their GPG. Some (typically smaller) employers thought that women were less likely than men to negotiate on their starting salaries, pay rises and bonuses.
“Women tend not to negotiate in the same way as men, it is in their nature I think. That is not something you can change.” (250 to 499 employees, private sector)
Others noted that although their HR team had attempted to raise awareness of the need to encourage more women into senior roles, this was not always adhered to by men in senior positions. Again, they felt that this was the result of the ingrained culture that existed in their organisations, sometimes exacerbated by low staff turnover.
6. The GPG transparency regulations
This chapter explores employers’ understanding of the new gender pay gap transparency regulations and how they plan to comply with them. More specifically, it covers:
- awareness and knowledge of the regulations
- the extent to which employers have prepared for them
- the type of support and assistance that would benefit employers in complying
- employers’ plans for when they will publish the required gender pay data, what they will publish and how they will communicate the results
Please note that the quantitative survey was conducted between 10 March and 27 April 2017. The new transparency regulations for the private and voluntary sectors came into force in April 2017 (with these organisations required to publish their statistics by 4 April 2018) and the regulations for the public sector came into force in March 2017 (with these organisations required to publish their data by 30 March 2018).
6.1 Awareness and understanding of the regulations
Overall, 88% of all respondents were aware of the new regulations. In terms of knowledge, over half (54%) felt that they understood what was required and how to do it, and a further fifth (20%) believed they knew what was required but were less certain of how to go about it.
Figure 11: Understanding of the new GPG transparency regulations
Base: All respondents (Base, Don’t know). Total (900, 1%). Private (406, 1%), Voluntary (243, 1%), Public (251, 1%). 250 to 499 (412, 2%), 500 to 999 (214, 1%), 1,000 or more (274, 1%)
There were no significant differences in awareness levels by either sector or size. However, private sector organisations were less likely to have ‘full’ knowledge of the regulations (52% knew what was required and how to do it, compared to 58% of voluntary sector and 61% of public sector organisations). ‘Full’ knowledge also increased with size, ranging from 47% of those with 250 to 499 employees up to 65% of those with 1,000 or more employees.
Exposure to the GEO and Acas guidance on GPG was strongly associated with self-reported knowledge of the regulations. 71% of those that had read the guidance said that they understood what was required and how to do it, compared to just 27% of those who had not read it.
It was also the case that the greater the priority allocated to reducing their GPG, the better the reported understanding of the regulatory requirements. Two-thirds (64%) of those treating it as a high priority had ‘full’ knowledge of the requirements, compared to only one-third (36%) of those that did not view it as a priority at all.
6.2 Preparation for the regulations
Half of all organisations felt they were reasonably prepared for the regulations, in the sense that they either believed they were already able to meet the requirements (17%) or had developed a plan for how they would do this (32%). However, almost one-third (30%) had done no more than review the requirements, and a further 17% had not thought about the regulations or were unaware of them (prior to the survey).
Figure 12: Preparation for the GPG transparency regulations
Base: All respondents (Base, Don’t know). Total (900, 3%). Private (406, 3%), Voluntary (243, 4%), Public (251, 2%). 250 to 499 (412, 3%), 500 to 999 (214, 3%), 1,000 or more (274, 4%)
The larger the employer, the more likely they were to be fully prepared for the GPG transparency regulations. 60% of those with 1,000 or more employees believed they were already able to meet them or had drawn up a plan, compared to just 42% of those with 250 to 499 employees.
Readiness was also greater in the public sector, with 62% of these organisations stating that they were able to meet the regulations or having a plan in place to do so.
6.3 Attitudes to complying with regulations
The qualitative interviews explored how employers were approaching compliance with the regulations. We asked respondents about how much of a priority compliance was and their progress towards publishing their results.
Level of priority given to the regulations
All employers interviewed in the qualitative stage were planning to comply with the regulations. In addition, the vast majority explained that they considered it an important priority to do so. However, this was generally because they placed importance on being compliant with all regulations, rather than due to a high priority towards GPG specifically.
Furthermore, among the qualitative sample, the motivation to comply commonly came from the HR team rather than senior leadership. In many cases, senior managers were not aware of GPG or the regulations before their HR teams had informed them of this. The majority of interviewed employers also agreed that had the regulations not been introduced, they would not measure their GPG. Therefore, in many cases, the underlying motivation to comply was the avoidance of any potential sanctions or penalties associated with not doing so.
While many employers agreed that compliance with the regulations was being driven by HR and a need to meet their obligations, they often went on to explain that their senior leaders were interested (or had become interested) in the process and its outcomes.
They reported genuine interest in understanding the GPG data and what it means for the business. In some cases, this was driven by a realisation that they might need to take action to address a GPG. This suggests that the regulations have encouraged decision makers in some organisations to consider the issue of GPG more closely.
In other cases, senior leaders were primarily interested in how best to present the data to avoid casting their organisation in a negative light. In these cases, the motivation was to remain compliant while minimising any detrimental impact on the organisation.
Other employers reported that the drive to comply with the regulations originated with the leadership team. Most of these organisations had measured their GPG in the past (or conducted other gender analysis). These employers welcomed the GPG regulations as another source of data for their overall equality and diversity agenda.
The compliance process
In the qualitative interviews, employers described their progress towards complying with the regulations, what they had done or planned to do and when. Figure 13 illustrates the main stages as described by the majority of employers.
Figure 13: Preparation for the GPG transparency regulations
Pre-stage – reviewing, researching and learning:
- Reading requirements
- Attending seminars
- Reading ACAS and GEO guidelines
- Speaking to legal and HR providers
(Dry run between pre-stage and stage 1)
Stage 1 – collating data:
- Extracted from payroll system
- HR or payroll team in house
- Or external payroll provider
Stage 2 – making calculations:
- HR or payroll team
- Sometimes management level
- Produces final key measures
Stage 3 – reviewing results and writing commentary:
- Senior HR role
- Shared and debated with senior manager
(Legal advice sought between stage 3 and stage 4)
Stage 4 – published:
- Final version
At the time the qualitative stage was conducted, most of the interviewed employers had completed stage 1 (collating data), with a minority having completed stage 2 (making calculations). However, a small number were yet to take any action beyond reading guidance or attending seminars.
It is important to note that while they thought complying with the regulations was important, interviewed employers did not consider it to be an urgent priority due to the time available to comply. Other initiatives were often being given priority over GPG (including complying with other HR-related guidelines and regulations).
Therefore, while most employers had a ‘rough’ timetable, very few had planned activities in detail. Indeed, some described an approach which involved fitting the work in around other activities, as and when time allowed. The main exceptions to this somewhat fluid approach were some public sector bodies who were committed to publishing as part of their summer reporting cycles.
“We don’t have a set plan, but I am confident that in the next few weeks we will have a figure…I do not know when we will publish though.” (1,000 or more employees, voluntary sector)
A minority of employers had outsourced all or part of the compliance process. Some had handed the process to consultants or external HR experts to provide complete assurance that they were fully compliant. Others were using their external payroll providers to collate the necessary data, before conducting the calculations in house.
A small number of employers had conducted a ‘dry run’ of the data collection and calculations. They had typically completed these in late 2016 or early 2017. Employers conducted these in order to make sure that they were able to access the necessary information, to ‘practice’ making the calculations, and to see whether they were likely to have a notable GPG or not. Those who had conducted the dry run were all confident that they were able to repeat the process without any real difficulty.
Overall, employers who had started it described the process of collating data and making calculations as straightforward. Most had sought some external support or guidance in order to be completely clear on what was required, but generally agreed that the process itself was not particularly arduous or confusing. Those who were yet to start, but had already read guidance or attended seminars, were also generally confident about their ability to complete these stages.
The main (potential) difficulties reported were as follows:
- knowing exactly which payments to include or exclude, particularly in relation to contracted employees and overtime payments
- tracking down and pulling together disparate sources of data (for example, bonus payments for senior staff, contractor payments) which may not be part of the payroll
Some employers reported that their payroll software provider had updated their system to facilitate compliance with the regulations. Others explained that their providers were due to do this, and these employers were waiting until this update had occurred before proceeding with the compliance process.
The greatest challenge anticipated by employers was knowing how to interpret and present the results, and specifically what type of commentary to provide to explain them. It was for this purpose that some employers were planning to share their results (and in some cases their draft commentary) with external law firms or consultants. They wanted to ensure that they would not be opening themselves up for accusations of misrepresentation.
6.4 External support in complying with the regulations
If employers needed assistance in complying with the new GPG transparency regulations, the individual organisations they would most likely contact were Acas (30%) and the Government Equalities Office (17%). However, the survey was positioned as being on behalf of GEO, which may have put it more top of mind.
Approaching one-third (30%) would contact a legal professional or advisor and one-quarter (23%) would approach external consultants.
Table 8: Where employers would seek support with the regulations (unprompted)
The pattern was broadly similar by sector, although public sector organisations were comparatively more inclined to approach other government departments or trade associations or industry bodies. In contrast, private and voluntary sector organisations were more likely to contact legal advisors or external consultants.
Employers were also asked about the type of support that would help them, as shown in Table 9. One-fifth of employers (19%) would benefit from advice on how to report their GPG and a similar proportion (18%) from advice on how to measure it. There was also some demand for software to calculate their GPG (9%), case studies (9%) and benchmarks (7%).
However, one-third of employers (32%) did not feel they would need any external support and a further fifth (20%) were unsure as to what they might need.
Table 9: Types of support that would help employers (unprompted)
It should be noted that in many cases the types of support requirements mentioned by survey respondents were already being provided by the government (for example, the joint GEO and Acas guidance on gender pay gap reporting).
The guidance on GPG reporting produced by GEO and Acas had successfully reached the majority (59%) of employers subject to the new regulations.
Figure 14: Proportion of employers reading the GEO and Acas guidance
Base: All respondents (Base, Don’t know). Total (900, 1%). Private (406, 1%), Voluntary (243, 0%), Public (251, 1%). 250 to 499 (412, 1%), 500 to 999 (214, 0%), 1,000 or more (274, 2%)
The qualitative interviews explored the external support that employers had used so far in the process of complying with the regulations. Most of the interviewed employers had accessed some form of information or guidance at the outset of the compliance process. Many felt confident in the requirements, and simply wanted to reassure themselves that they fully understood exactly what they needed to measure and publish. However, a minority were confused about what they were required to measure and wanted a more detailed and expansive explanation than they had read on government websites.
“I wasn’t really looking to find out anything specific, I just wanted to make sure that I knew what I had to do. It was good to have someone spell it out for me.” (500 to 999 employees, private sector)
Most of those who had read the Acas or GEO guidance felt that it was clear and comprehensive. However, a minority would have liked more detailed information and illustrative examples of exactly how to present their results.
Seminars were also described as useful sources of guidance and advice. Employers had attended seminars held by law firms, recruitment consultants and HR specialists. They welcomed the opportunity to hear an expert view on how to comply and the chance to network with other HR professionals.
Only a minority of employers in the qualitative sample felt that they had support needs that had not been met. These needs related to how to report their gender pay data. In particular, employers wanted to know the acceptable and expected way to present the information and the commentary around it.
“I really want to see an example of published information, so I can understand how it should be set out.” (250 to 499 employees, voluntary sector)
6.5 Publication and communication of results
Large employers will be required to publish their gender pay data by 4 April 2018 (or 30 March in the case of public sector organisations). Perhaps reflecting the fact that the survey was conducted around one year before this deadline, over half (57%) of employers had not yet decided on their publication date.
Among those that had an expected publication date, one-third (34%) intended to do this in Quarter 4 (equivalent to 15% of all employers). However, a significant proportion intended to publish much earlier than the deadline: 8% of all employers said they planned to do so in Quarter 1. Please note that, as discussed later in this chapter, the qualitative interviews suggested that the publication dates given in the quantitative survey tended to be ambitions rather than firm plans, and there were several reasons why they might be delayed. There was also evidence that some employers did not know they were required to publish their results on the government portal as well as on their own websites.
Figure 15: Planned publication date for GPG results
Out of the 43% of all employers who had decided when to publish:
- 19% intended to publish in quarter 1 (April to June 2017)
- 23% intended to publish in quarter 2 (July to September 2017)
- 24% intended to publish in quarter 3 (October to December 2017)
- 34% intended to publish in quarter 4 (January to March 2018)
Base: All respondents (Base, Don’t intend to publish). Total (900, 0%). Private (406, 0%), Voluntary (243, 0%), Public (251, 0%). 250 to 499 (412, 0%), 500 to 999 (214, 1%), 1,000 or more (274, 0%).
Public sector organisations were significantly more inclined to publish their GPG data early, with 30% intending to do this by in the first 2 quarters of the 2017 to 2018 financial year (compared to 15% for the private sector and 20% for the voluntary sector).
Those employers that had calculated their GPG at some point in the previous 12 months (prior to the regulations coming into force) were also more likely to publish early, with 24% planning to do this by the end of Quarter 2.
The qualitative interviews indicated that employers’ plans for when they publish their gender pay data were generally not set in stone. Most described general ambitions, rather than set dates. Some described how these ambitions (for example to publish as soon as possible) had not been met because other priorities had taken precedence.
“I have had the data from the payroll team for a while, but I haven’t got around to sitting down and analysing it yet…But there is still plenty of time.” (250 to 499 employees, private sector)
In other cases, employers explained that although they planned to publish at a particular time, they were not yet fully aware of how complex or difficult the process of collating and interpreting the data would be. Therefore, they noted that their intended reporting date could potentially slip, particularly if they needed to seek support or advice from a third party or from their parent company on the matter.
It is also important to note that many employers said they were delaying publishing their gender pay data until other employers (in their sector) had done so. They wanted to see how other employers presented the data and the explanations they provided for any GPG they reported.
“We certainly don’t want to be the first to publish. It is a question of not putting your head above the parapet.” (500 to 999 employees, private sector)
Subsequent qualitative follow-up interviews were conducted with employers who had intended to publish their GPG results in quarter 1 of the 2016 to 2017 financial year, but who had not yet done so via the GPG reporting portal. [footnote 8] This identified 4 reasons for this delay in publication:
The process of collating data and or or running calculations was more involved than expected, meaning that the process had been slowed.
Significant unexpected circumstantial factors had either made the process more complex, delayed receipt of relevant data, pushed early compliance down the list of priorities for the organisation, or led to a deliberate delay in publication.
Early compliance had become less of a priority since their participation in the original survey because other issues had taken precedence and the issue of GPG had become less ‘top-of-mind’.
Results had already been published on the employers’ websites, but they were not aware that they were required to publish on the government portal.
The quantitative survey also captured data on whether employers planned to publish any additional information alongside the mandatory reporting requirements. One-fifth (20%) intended to do so, with this most likely to be an accompanying narrative commentary (15%). Reflecting the fact that the vast majority of employers had not yet run the final analysis, at the time of the survey one-third (31%) were unsure whether they would publish any additional information.
Table 10: Whether plan to publish any additional information
Private sector organisations were more likely to just provide the analysis required by the regulations but not publish any further information, with 83% either not planning to produce additional information or unsure as to whether they would do so.
The likelihood of publishing additional information increased among large organisations with 1,000 or more employees (27%), with almost one-quarter of this group planning to produce a narrative commentary (22%).
The qualitative interviews showed that those employers who intended to publish additional information fell into 2 groups.
The first group knew or were very confident that they would not have a GPG. They therefore wanted to produce a positive narrative to accompany the data. This intention was more common among public sector organisations, some of whom described a strong desire to promote their equality and inclusivity agenda to the public.
The second believed that they had a (large) GPG and were unlikely to be able to close it. These employers wanted to provide context and a rationale explaining the data, and in some cases provide an explanation of how GPG is calculated and how it differs from equal pay.
In addition, some of those not currently planning to publish additional information were open to the possibility of doing so, depending on the results of their GPG calculations. Most were waiting to see whether they felt it necessary to explain their results or not.
In the quantitative survey, employers were asked about their plans for how they would communicate their GPG results, both to current or potential employees and to external stakeholders such as clients, suppliers and investors. Communication strategies were similar for both groups, and in many cases the approach adopted will depend on the results of the GPG analysis.
Only a minority intended to adopt an active engagement strategy (16% for employees and 11% for stakeholders), but a further fifth would at least draw these audiences’ attention to the results (20% and 21% respectively).
Figure 16: Planned approach to communicating GPG results
Base: All respondents (900)
Public (49%) and voluntary (43%) sector organisations were more likely than private sector (33%) employers to communicate their results to staff (adopt an active engagement strategy or draw their attention to the results).
Most employers in the qualitative sample were waiting to see their results before deciding how widely to communicate them. Overall, interviewed employers were more likely to be planning a wide and comprehensive strategy if they felt they had good news to share.
However, most had not considered exactly how they would go about this. Some (usually smaller private sector employers) described a general policy of protecting information about themselves, and avoiding data entering the public domain as much as possible. They therefore planned only the bare minimum in terms of publishing and distributing their gender pay data.
Employers across the qualitative sample were planning to share their results with staff by either publishing then on their intranets or via internal memos or newsletters. Some were also considering holding staff meetings to explain the results in full. They were concerned that staff may misinterpret them without a clear explanation.
Some public sector employers were planning to promote their results among their staff and the public as part of a wider strategy of openness. Others were considering how to use their GPG data internally to support women in their workforce and help them progress their careers (for example, by covering it at seminars and other events).
7. Employer profile and practices
This chapter reports on the characteristics and practices of large employers (250 or more employees), with a particular focus on areas that are relevant to the gender pay gap.
These questions were primarily included in the survey to provide context to the key GPG findings. When interpreting the results in this chapter, it should be considered that the data was self-reported and respondents were not asked to prepare in advance. As such, the figures on workforce gender balance and take-up of parental leave are based on estimates provided during the interview and are unlikely to be fully accurate. For some of the areas covered in this chapter (for example, gender profile of workforce) there is likely to be more accurate and robust data available in the public domain.
Specifically, this chapter covers:
- the gender balance of the workforce and senior management team
- the types of flexible working practices offered
- whether maternity, paternity and shared parental leave is paid at the statutory rate or an enhanced rate
- the proportions of new mothers returning to work after maternity leave, new fathers taking full paternity leave and new parents taking shared parental leave
7.1 Gender profile of workforce and senior management team
The survey data suggests that females are slightly under-represented in large organisations, with 56% of employers reporting that women made up no more than 50% of their total workforce. Just 8% were female dominated (76% to 100% of employees are female), whereas 21% were male dominated (0% to 25% female).
Table 11: Proportion of employees that are women (respondent estimates)
However, the above pattern was driven by the private sector, which accounts for 75% of all GB organisations with 250 or more employees. When looking at the voluntary and public sectors in isolation, women actually made up the majority of the workforce sectors (80% and 74% respectively reported that over half of their employees were female).
The larger the organisation, the more equal the gender balance of the workforce. Women accounted for over half of the workforce in 48% of 1,000 or more employee organisations, 41% of 500 to 999 employee organisations and 37% of 250 to 499 employee organisations.
The gender imbalance was significantly more pronounced when it came to the senior management teams of large employers. Just 18% reported that the majority of their senior management were women, and 43% indicated that women accounted for no more than a quarter of all senior positions.
Table 12: Proportion of senior management team that are women (respondent estimates)
Again, the overall results were driven by the private sector, with half (52%) of these companies reporting that women made up no more than 25% of their senior management team (and 5% with no female representation at a senior level).
However, although women accounted for the majority of all voluntary and public sector employees, they were less well represented at senior levels in these organisations. Only 42% of voluntary sector and 34% of public sector organisations reported that more than half of their senior management team were women.
There was relatively little difference by employer size in this respect, although those with less than 1,000 employees were more likely to indicate that 0% to 25% of their senior management were female (46% compared to 33% of 1,000 or more employee organisations).
7.2 Flexible working practices offered to employees
The vast majority (99%) of large employers offered some form of flexible working arrangements to their staff. The most common was part time work (97%), but three-quarters (74%) allowed home working and two-thirds (64%) offered job sharing opportunities. The majority (70%) also adopted other flexible working practices, such as compressed hours, flexitime or annualised hours.
However, it should be considered that this data relates to the flexible working arrangements offered to any of their employees, and it does not necessarily follow that these opportunities are universally available – it may depend on job role or level.
Table 13: Flexible working arrangements offered to any employees
There was no difference by sector or size when it came to the proportion offering any flexible working arrangements. However, voluntary and public sector organisations and those with 1,000 or more staff tended to provide a greater range of different flexible working options.
7.3 Maternity, paternity and shared parental leave
Most employers paid maternity, paternity and shared parental leave at the statutory rate. Maternity leave was comparatively more likely to be offered at an enhanced rate, with 43% of employers doing this in some or all cases (compared to 34% for paternity and 25% for shared parental leave). [footnote 9] The proportion of employers paying leave at an enhanced rate was highest in the public sector and among those with 1,000 or more employees.
Figure 17: Pay rates for maternity, paternity and shared parental leave
Out of all employers:
- 55% paid maternity leave at the standard rate, 33% at the enhanced rate, and 11% a combination of these
- 64% paid paternity leave at the standard rate, 27% at the enhanced rate, and 7% a combination of these
- 69% paid shared parental leave at the standard rate, 19% at the enhanced rate, and 6% a combination of these
Base: All respondents (Base, Don’t know). Total (900, 2% to 6%). Private (406, 2% to 6%), Voluntary (243, 2% to 6%), Public (251, 3% to 10%). 250 to 499 employees (412, 3% to 8%), 500 to 999 employees (214, 0% to 5%), 1,000 or more employees (274, 2% to 5%).
Employers estimated that the majority of women (82%) returned to work after their maternity leave, and that two-thirds of fathers took their full paternity leave entitlement. They estimated that 3% of new parents took shared parental leave, but it should be noted that this was only an estimate and not all employees were necessarily eligible for the policy. [footnote 10]
Figure 18: Return to work after maternity leave, and uptake of paternity and shared parental leave (respondent estimates)
82% of those who took maternity leave returned to work
63% of new fathers took full paternity leave
3% of new parents took shared parental leave
Base: all respondents (900). Mean proportions.
There are some issues to consider which may have affected respondents’ ability to provide accurate responses to the above questions. Firstly, employers will not necessarily be aware which of their male staff were new fathers if they did not request paternity leave, and hence would not be able to accurately estimate take-up rates.
Secondly, eligibility for shared parental leave depends on the employment status of both parents, so the employer will not always be aware which new parents were eligible.
The table below provides a more detailed breakdown of the proportion of women that employers estimated had returned to work after maternity leave in the last year. There was little difference in this respect by sector or size.
Table 14: Proportion returning to work after maternity leave (in last year)
As detailed below, uptake of paternity leave was greatest in the public sector, with an average of 77% of new fathers taking their full 2-week allocation.
Table 15: Proportion of new fathers that took full 2-week paternity leave (in last year)
The proportion of new parents taking shared parental leave was extremely low across the board, irrespective of size or sector. This strongly suggests that the initial childcare burden is primarily falling on women, which has wider implications for the GPG as women are still taking longer career breaks than men.
Table 16: Proportion of new parents that took shared parental leave (in last year)
In three-quarters (74%) of 250 to 499 employee organisations and two-thirds (66%) of private sector companies, no new parents took shared parental leave.
8. Annex A. Full survey results
This annex provides the results to each individual question in the quantitative survey. Results have been shown at the total level, by sector and by employer size.
8.1 S1b – Please can I take a note of your job title?
8.2 s2 – which of the following best describes your organisation, 8.3 s3a and s3b – how many employees does your organisation currently employ in great britain, 8.4 s4a and s4b – what percentage of these employees are women, 8.5 s5a and s5b – now thinking just about those working in senior management positions, what percentage of these are women, 8.6 s6 – thinking about your organisation’s employment practices, do you offer any of the following flexible working arrangements to any of your employees, 8.7 s14a – do you currently pay the following to employees at the statutory rate or at an enhanced rate – maternity leave, 8.8 s14b – do you currently pay the following to employees at the statutory rate or at an enhanced rate – paternity leave, 8.9 s14c – do you currently pay the following to employees at the statutory rate or at an enhanced rate – shared parental leave, 8.10 s13 – in the last year, approximately what percentage of those taking maternity leave returned to work, 8.11 s10 – in the last year, approximately what percentage of new fathers that you employ have taken their full 2-week paternity leave, 8.12 s12 – in the last year, what percentage of new parents that you employ have taken shared parental leave, 8.13 a1 – before today, had you heard of the term “gender pay gap”, 8.14 a2 – which of the following statements best describes your understanding of the gender pay gap, 8.15 a3 – which of the following statements best describes your understanding of the difference between ‘closing the gender pay gap’ and ‘ensuring equal pay between men and women’, 8.16 a4 – thinking about your organisation’s leadership team or board, how much understanding do you think they have of the gender pay gap and the difference between this and equal pay, 8.17 b1 – can i just check, in the last 12 months, has your organisation calculated its gender pay gap, 8.18 b2 – what was your organisation’s gender pay gap when you last measured it.
Base: All organisations that had measured their GPG
8.19 B3a – Did you calculate mean pay gap, median pay gap or both?
8.20 b3b – did you present this difference as a percentage of male average pay, 8.21 b3c – did you calculate this based on hourly pay or annual salary, 8.22 b4a – i’m now going to read out some other types of analysis you may have done in this area. for each one please could you tell me whether your organisation has done this in the last 12 months. so firstly, have you analysed the proportions of male and female employees that were paid bonuses, 8.23 b4b – have you calculated the difference in the average bonuses paid to male and female employees, 8.24 b4c – have you analysed the proportions of male and female employees at different levels of the organisation’s pay hierarchy, 8.25 b5a – and have you done any other analysis looking at differences between male and female employees in your organisation in the last 12 months, 8.26 b5b – what other gender analysis of this type have you done, 8.27 b6 – thinking about how you have used any of this gender pay gap or other gender analysis, have you done any of the following.
Base: All that have measured their GPG or done other gender analysis
8.28 C1 – How much of a priority to your organisation is reducing your Gender Pay Gap?
8.29 c2 – why is reducing the gender pay gap a high priority for your organisation.
Base: All where reducing GPG is a high priority
8.30 C2 – Why is reducing the Gender Pay Gap a medium priority for your organisation?
Base: All where reducing GPG is a medium priority
8.31 C2 – Why is reducing the Gender Pay Gap a low priority for your organisation?
Base: All where reducing GPG is a low priority
8.32 C2 – Why is reducing the Gender Pay Gap not a priority at all for your organisation?
Base: All where reducing GPG is not a priority at all
8.33 C3 – Which of the following best describes your organisation’s current approach to reducing your Gender Pay Gap?
8.34 c4 – have you or do you intend to publish an action plan for how you will try and reduce your gender pay gap, 8.35 c5 – still thinking about the formalised plan or strategy that you have developed to reduce your gender pay gap, which of the following specific actions or measures does this include, 8.36 c7 – will the impact of these actions be evaluated as part of a formalised process or on a more ad hoc basis.
Base: All that have taken action to reduce their GPG
8.37 C8 – And have you already evaluated the current impact of these actions?
8.38 c9 – how successful do you think these actions have been to date, 8.39 c10 – in your view, what is the main challenge to reducing your organisation’s gpg, 8.40 d1 – which of the following statements best describes how well informed you consider yourself to be about these new regulations, 8.41 d2 – to what extent has your organisation prepared for these regulations, 8.42 d3 – if you needed support in complying with the new regulations, where would you look to for advice, 8.43 d10 – what, if any, external support or assistance would help you comply with the gender pay gap regulations, 8.44 d4 – have you read the guidance on gender pay gap reporting that has been produced by the government equalities office and acas, 8.45 d5 – the regulations require relevant employers to publish their gender pay data within 12 months of the 6 april 2017 (or 31 march for the public sector). when does your organisation intend to publish its results, 8.46 d6 – do you plan to externally publish any additional information beyond that required by the regulations, 8.47 d7 – what else do you plan to publish, 8.48 d8 – which of the following best describes your current or expected approach to communicating your gender pay gap results to current employees and potential recruits , 8.49 d9 – and how will you communicate the results to clients, suppliers, investors and other external stakeholders, 8.50 sic classification (idbr data) - private sector only.
Base: All private sector organisations
8.51 Government Office Region (IDBR data)
9. annex b. summary report of qualitative follow-up research on gpg reporting, 9.1 b1. background and objectives.
In Spring 2017, OMB conducted research for GEO to further understand the attitudes of large employers towards compliance with the gender pay gap (GPG) transparency regulations. This consisted of a quantitative survey of 900 employers and in-depth qualitative interviews with 30 of those respondents.
As part of this research, employers were asked when they intended to publish their GPG data. Within the quantitative sample, 81 respondents said that they intended to publish their GPG results in quarter 1 of the financial year 2016 to 2017. If this is extrapolated to the whole population, we would expect that around 8% of all large employers would publish in quarter 1, which is higher than the number who actually published their results on the official GPG portal in quarter 1.
GEO therefore commissioned further research among those employers that expected to publish in quarter 1 to understand why this did not happen. This summary outlines the main findings from the follow-up research, conducted by OMB Research in September 2017.
9.2 B2. Methodology
A total of 22 qualitative telephone interviews were conducted with employers who had indicated an intention to publish their GPG results in quarter 1 of the 2016 to 2017 financial year, but who had not yet published via the GPG reporting portal. The sample consisted of participants from the main quantitative survey who had agreed to be contacted for further research. We conducted a brief interview (5 to 10 minutes in duration) to explore the progress they had made towards compliance, their overall perceptions of the process and what had delayed their progress. Where relevant, we also explored the impact of previous experience of measuring GPG on employers’ experiences of complying with the new reporting regulations (for example, had it made the process easier or more difficult, and why?).
We interviewed a spread of employers by size, sector and whether they had previously measured their GPG. The achieved interview profile closely matched the profile of all those employers reporting an intention to publish their GPG results in quarter 1 of the 2016 to 2017 financial year. The breakdown of the 22 interviews was as follows:
- size: 10 employers with 250 to 499 employees, 4 employers with 500 to 999 employees, and 8 employers with 1,000 or more employees
- sector: 10 public sector, 7 private sector, 5 voluntary sector
- previously measured GPG: 12 yes, 10 no
We also achieved a geographical spread of employers across the sample.
9.3 B3. Summary of key findings
Progress towards compliance.
Employers were at different stages on their compliance journeys. The vast majority had started gathering the data required for calculations and over half the sample had completed their calculations and were either in the process of writing supporting statements or had already done so. Of those who had already completed their calculations and supporting statements, 3 had already published their results on their own websites and the others were awaiting approval from their boards or other organisations within their group.
A number of employers were in the process of either checking what data to include, collating the data from their payroll and other systems or running the calculations. Only a small minority were yet to engage with the compliance process at all.
Experiences and perceptions of the data collation, calculation and interpretation process varied across the sample, and the impact of this on their progress also varied (explored in the next section). In some cases, the experience had been more arduous than expected, while others reported a relatively straightforward process. However, most explained that the process required diversion of resources which were also required elsewhere, meaning that GPG data collation and calculation were sometimes paused in order to allow staff to work on other tasks.
“It was quite tricky gathering the data. It was probably because it was the first time we had done it, and didn’t know what to expect. There are plenty of other things that we needed to do too, so we didn’t have as much time on this in the end.” (250 to 499 employees, private sector)
When considering reasons for not complying as early as initially intended, it is important to note that employers weigh up the amount of work involved in completing the compliance process against the negative impact of not doing so. Employers consistently explained that the relatively long-term deadline for compliance meant that they were less inclined to make additional effort to do so in the short-term, particularly when set against other, more time-pressured priorities.
“It has just ended up on the back burner. We know we don’t have to report on it until next year, so it has taken a back seat at the moment.” (1,000 or more employees, private sector)
Furthermore, some employers explained that as they approached the GPG deadline date, the urgency and priority given to compliance would increase.
Reasons for delayed compliance
We explored the degree to which employers felt they had ‘slipped’ in terms of their original publication intentions, and what they felt were the reasons for this. Four broad groups of employers emerged across the qualitative sample, summarised below in order of their prevalence:
Reason 1. A more involved process: A number of employers (across a range of sizes and sectors) explained that while they had the best intentions to publish early, and were initially optimistic that they would be able to do so, the process of collating data or running calculations was more arduous than they had expected. Most of these employers reported challenges at the early stages of data collation. Some explained that it had taken longer to identify and clarify exactly what figures to include in the calculations (for example, bonuses and other benefits).
“Once we started to look at it in detail, it took longer to understand exactly what type of data was required to do the calculations.” (250 to 499 employees, public sector)
Others reported a complex or difficult process of transferring and checking certain types of data. This was either the result of particular ways in which salaries and bonuses had been recorded in the past, or due to the complexity of the business (for example, multiple subsidiaries or departments with different systems in place).
“I had to extract some of the data by hand, which took ages.” (1,000 or more employees, private sector)
A minority were delayed by senior management seeking further clarification about the meaning of the results before agreeing to publish. These employers explained that the initial priority to publish early had been driven by the HR function, and that this was not fully shared by senior management, who were not as engaged in the topic and had a limited understanding of GPG (and how it differs from equal pay).
Reason 2. Circumstantial barriers: A second group of employers described more specific, often external, barriers or issues. These had either made the process of compliance considerably more complex, pushed compliance down the list of priorities for the organisation as a whole and for the HR function in particular, or led to a deliberate delay in publication.
In some cases, organisations had merged or acquired other operations, making the process of compliance more complex, and meaning that other tasks associated with the new working arrangements were deemed more important. In other cases, employers had been instructed to wait before publishing so that all organisations in a larger group could publish simultaneously. Lastly, some employers explained that their compliance process had been delayed by their payroll IT provider. They had not yet been provided with the data reports required to make calculations.
“Two colleges have merged, meaning that we have 2 sets of data to deal with now. We have pulled the stats together now, but there was a delay.” (500 to 999 employees, public sector)
“We didn’t have a firm plan of when to publish, but our best intentions were to do so quickly and get it out of the way…[but] we had a hic-up with the payroll provider not being able to run the report.” (500 to 999 employees, public sector)
Reason 3. Level of engagement reduced versus other priorities: In a minority of cases, employers did not describe any specific reasons for a delay in compliance. Rather, they explained that doing so had simply become less of a priority in the intervening weeks and months, with other more pressing issues taking precedence. They also explained that, at the time of the quantitative survey, the new regulations were more ‘top-of-mind’ due to PR coverage and communications about the topic. This had contributed to a degree of optimism and good intentions around compliance, which had subsequently diminished. Some expected further communication and publicity about the regulations as the deadline for publication becomes closer, and suggested that this would likely encourage them to push ahead with the compliance process with more urgency.
“We haven’t really got started, it’s just a lack of time. At the time the regulations came in there was lots of awareness and we wanted to get it out of the way, but we just haven’t had the time.” (500 to 999 employees, private sector)
Reason 4. Misinterpretation of ‘publishing’: Three employers in our sample (all from the public or voluntary sectors) said that they had already published their GPG results. When probed in more detail, all explained that by ‘publish’ they were referring to publishing on their own websites and not on the government portal. In one case, the employer was aware of the need to publish on the government site, but was not planning to do so until the deadline of the end of March 2018. However, the other employers were not aware that they were required to do this, and assumed that by publishing on their own website, they had already complied.
“We put this on our website as part of our Pay Policy Statement in April. It was straightforward really. I didn’t realise that we had to report it too.” (250 to 499 employees, public sector)
“Yes, we published online quite a while ago. We haven’t registered it yet as we do not have to do this until next year.” (250 to 499 employees, voluntary sector)
Understanding of publication requirements
Once the lack of understanding around the meaning of ‘publishing’ GPG results described above was first discovered, we explored perceptions and understanding of their publishing and reporting obligations in all subsequent interviews (covering a total of 20 of the 22 employers). The majority of employers in the sample were fully aware of their obligations to publish their GPG data on the government portal. However, 5 (including the 2 previously mentioned) employers assumed that they only had to publish on their own websites and a further 2 were unsure, but said that they would have checked again before the deadline.
All the employers in this qualitative sample who did not realise that they had to publish on the government portal were either voluntary or public sector organisations. Some local authorities had assumed that because they were publishing their data as part of other public-facing information about pay in general, no further action would be required. One voluntary sector employer thought that only public sector organisations were required to publish their data with central government.
“We will have to make it public, that is the requirement, so we will make sure it is on our internet site.” (1,000 or more employees, public sector)
“I didn’t realise that we would need to report it to the government too. I thought that was public sector only.” (1,000 or more employees, voluntary sector)
Impact of previous GPG measurement
Just over half the sample had previously measured their GPG in some way, with the majority of these having done so as a ‘dry run’ after being notified that they would be required to publish their GPG results under the new regulations. In most cases, the primary motivation for completing the dry run was to identify whether or not they had a significant GPG early on, in order to allow time to address this before publishing the official data. In many cases their focus was not on testing the exact approach to calculating the data, but rather on gaining a ‘rough idea’ of the results. Consequently, while the dry run had some positive impact in terms of preparing staff for what they would need to do to comply, in many cases it had not prepared them fully for what was required in terms of identifying and collating the precise data or interpreting the results.
“We did a rough dry run which made it a bit easier…at least we were not starting from scratch. But it didn’t prepare us for the challenges of getting all the information from our database.” (250 to 499 employees, voluntary sector)
In a minority of cases, public sector employers explained that they had already been collecting GPG data as part of their on-going policies on equality. Some explained that this had meant that they were very confident about collating the data for the new regulations, and had therefore completed this quite quickly. In some cases, these employers had already published on their own websites, with delays in ‘official’ publication related to a need for senior managers to sign-off on the portal submission.
In some other cases, employers felt that their past experience of calculating GPG had little impact on their ability to make the necessary calculations under the new regulations. They explained that their previous GPG measurements involved simpler calculations, meaning that they still needed to work out the best way to approach the new requirements. However, while it did not help them, they did not feel that their previous ‘habits’ (in terms of making calculations) had impeded their ability to carry out the new calculations either.
“We have done it before as part of our equality survey. It didn’t really make a difference either way. The only real difference was calculating the quartiles, but that was not a big problem to add.” (250 to 499 employees, public sector)
© OMB Research Ltd Reference: DFE-RR747 ISBN: 978-1-78105-830-5
The views expressed in this report are the authors’ and do not necessarily reflect those of the Department for Education.
‘The Equality Act 2010 (Gender Pay Gap Information) Regulations 2017’ for the private and voluntary sectors and ‘The Equality Act 2010 (Specific Duties and Public Authorities) Regulations 2017’ for the public sector. ↩
A stage of additional qualitative telephone interviews was conducted in September 2017. ↩
The median is used for the headline GPG figure, although the mean is also used in some cases. ↩
This is based on the Office for National Statistics analysis of median earnings for all employees (full and part time): The gender pay gap - what is it and what affects it? ↩
Calculated at the 95% level of confidence, and showing the ‘worst case’ scenario of 50% of the sample answering in the same way. ↩
Employers were allocated to an engagement band based on their responses to the following 2 questions in the quantitative survey: Which of the following best describes your organisation’s current approach to reducing your Gender Pay Gap? How much of a priority to your organisation is reducing your Gender Pay Gap? ↩
Strictly speaking, calculations of statistical significance apply only to samples that have been selected using a probability sampling design. However, in practice it is reasonable to assume that these calculations provide a good indication of significant differences for quota sampling (as used for this research). ↩
More detailed findings from this additional qualitative phase can be found in Annex B. ↩
In this context, ‘combination’ referred to cases where employers provided statutory rates to some staff and enhanced rates to others (for example, based on seniority or length of employment). ↩
Eligibility for Shared Parental Leave is contingent on employment history, and both parents must qualify. ↩
Is this page useful?
- Yes this page is useful
- No this page is not useful
Help us improve GOV.UK
Don’t include personal or financial information like your National Insurance number or credit card details.
To help us improve GOV.UK, we’d like to know more about your visit today. Please fill in this survey (opens in a new tab) .

Connors Newsletter
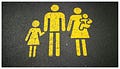
New Gender Pay Gap Study from Connors
Read the newest study from connors institute researchers which will be published in the journal of working-class studies in june 2024.
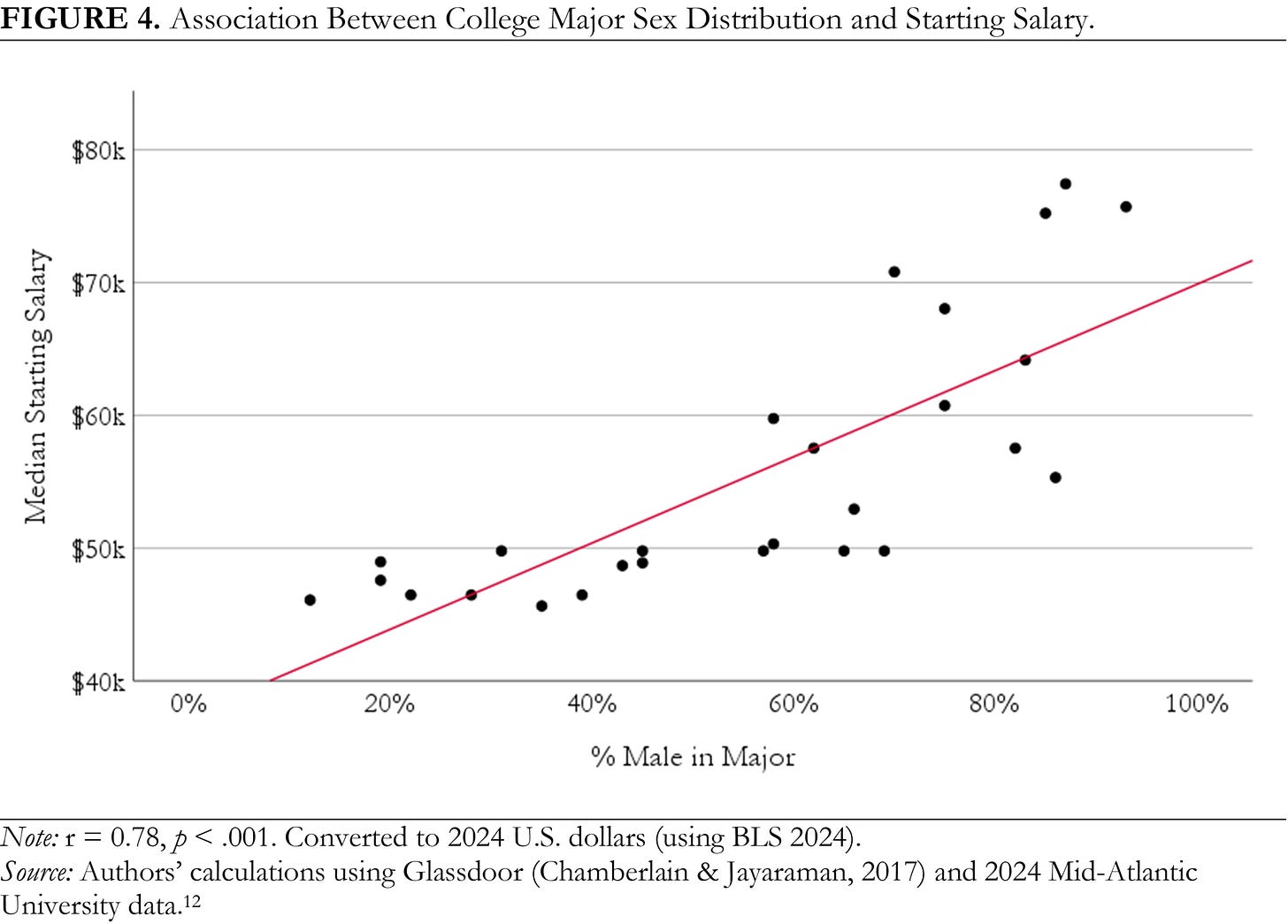
Connors Institute researchers—Director Lawrence Eppard and research fellows Annie Linker, Madison Laughman, and Katie Bonomo—have released a new report on the gender pay gap in the United States.
It will be published in the Journal of Working-Class Studies in June 2024—but you can read all about it now below!
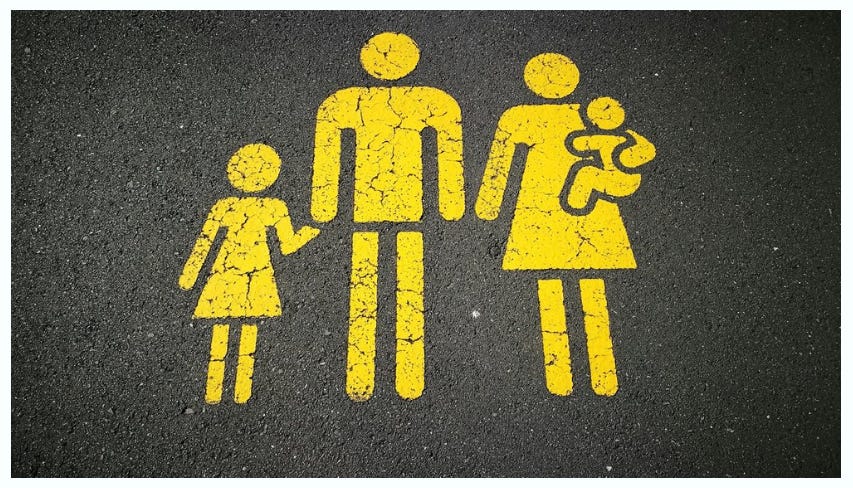
“This may be confusing for many Americans, as the public and political discourse around this issue can often obscure what is actually being measured”
The most recent data published by the U.S. Census Bureau (Guzman & Kollar, 2023, p. 8) show that the median earnings of American men who worked full-time, year-round was $62,350 in 2022, while it was $52,360 for their female counterparts. This translates into a gender pay ratio of 84% and gender pay gap of 16%.
In Figure 1 (above) and Table 1 (below) you can see how this gap has changed over time . In the first year that the Census Bureau provides data (1960), for instance, the gender pay ratio was 61% and the gender pay gap was 39%. These numbers have improved significantly over time as American women entered the paid labor force in much larger numbers (see Figure 2 below).
It is important to note that traditional measures of the gender pay gap reflect the ratio of female to male earnings for a specific group of people: full-time workers across all U.S. industries without regard for myriad differences between these workers. Thus, these measures “do not reflect a direct comparison of women and men doing identical work” (Bleiweis, 2020).
This may be confusing for many Americans, as the public and political discourse around this issue can often obscure [5] what is actually being measured.
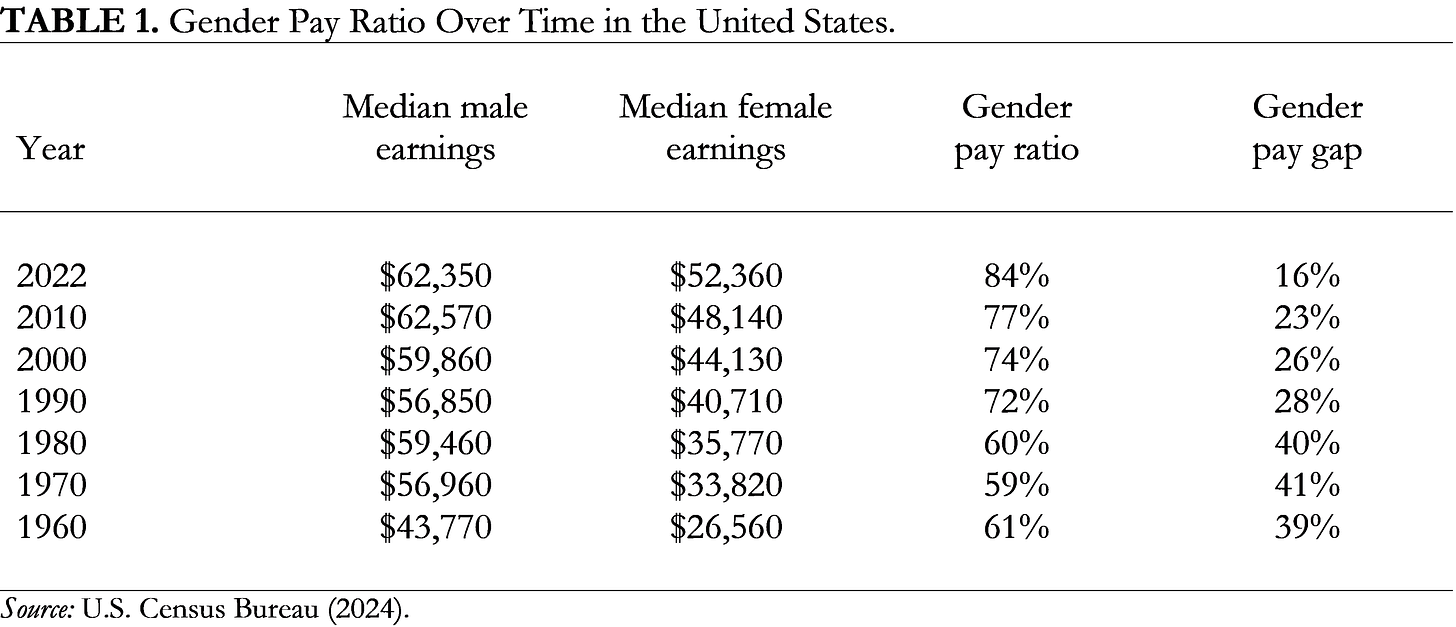
During former President Barack Obama’s re-election campaign in 2012, for instance, his team made this claim in an advertisement : “The son of a single mom, proud father of two daughters, President Obama knows that women being paid 77 cents on the dollar for doing the same work as men isn't just unfair, it hurts families” (Jacobson, 2012). As PolitiFact’s Louis Jacobson explained at the time :
“The Obama campaign took a legitimate statistic and described it in a way that makes it sound much more dramatic than it actually is. The 77-cent figure is real, but it does not factor in occupations held, hours worked or length of tenure. Describing that statistic as referring to the pay for women ‘doing the same work as men’ earns it a rating of Mostly False” (2012).
As one example of how these groups of workers may differ, women made up slightly less than half (47%) of the overall labor force in one 2018 analysis —but were a strong majority (78%) of workers in low-paying occupations (AAUW, 2024). This can be starkly illustrated by the percentage of females in low-paying jobs like childcare (93% female), housekeeping (88%), and cashiering (73%) in this analysis, versus the proportion in high-paying work like certain jobs in engineering (11-12% female) and computer-related work (19-26%) (AAUW, 2024).
As economist Astrid Kunze notes in her review of the gender pay gap literature in developed countries, “Women are systematically working in relatively low-paid occupations and men in more highly-paid occupations; this may reflect genuine job barriers or differences in preferences by gender for different kinds of jobs” (2017, p. 24).
Explaining the Gap
Some of the most influential work that attempts to help us better understand the complexities of the gender pay gap in the U.S. and the reasons behind it comes from the field of economics, particularly scholars like Harvard University’s Claudia Goldin , Princeton University’s Henrik Klevin , and Cornell University’s Francine Blau and Lawrence Kahn .
Their analyses and those of other leading scholars point to two factors in particular as the most important drivers of the gender pay gap in the U.S. today: sex differences in childcare responsibilities (often referred to as the “motherhood penalty” or “child penalty” ) as well as sex differences in occupation [6] (often referred to as “occupational segregation” ).
The Motherhood/Child Penalty
Economist Claudia Goldin, who won the Nobel Prize in 2023 [7] for her work on the gender pay gap, argues that sex differences in childcare responsibilities are the primary driver of the gap:
“Historically, much of the gender gap in earnings could be explained by differences in education and occupational choices. However, Goldin has shown that the bulk of this earnings difference is now between men and women in the same occupation, and that it largely arises with the birth of the first child” (Nobel, 2023).
In one notable study that Goldin and her colleagues conducted, they compared male and female MBA students who had graduated from the same elite program. Despite nearly identical earnings when they first graduated and entered the labor force, a pay gap emerged over time after the birth of children:
“Differential changes by sex in labor market activity in the period surrounding a first birth play a key role in this process. The presence of children is associated with less accumulated job experience, more career interruptions, shorter work hours, and substantial earnings declines for female but not for male MBAs” (Bertrand et. al., 2010, p. 252).
As Goldin recently explained: “It is really the child, the family responsibilities, that lead to these large differences [in wages]” (LaBlanc, 2023).
Henrik Klevin and his colleagues have documented the motherhood penalty across countries (see Figure 3 below) (Klevin et. al., 2023). Their work is incredibly valuable—it not only shows how children impact female wages virtually anywhere you look around the world, but that this impact varies significantly from country to country. There is much we can learn from these differences when attempting to achieve greater pay parity. Klevin and his colleagues have even made these data available to the public online—you can check it out yourself via their Child Penalty Atlas .
A recent analysis from Payscale (2024), a compensation software and data company based in Seattle, WA, comes to similar conclusions to those of Goldin and Klevin:
“Most interestingly, when we control gender pay gap analysis for job characteristics, we observe pay parity. Earnings of women without children keep pace with earnings of men without children. This supports research that suggests that having a child or being able to have a child is the primary or true cause of gender pay disparities. . . Research shows women’s income decreases because they reduce their working hours to balance childcaring responsibilities more than men” (Payscale, 2024).
The Payscale study’s authors claim to explain even more of the pay gap than many previous studies. In their 2024 report they asserted that, when they controlled for a variety of variables to ensure a comparison of similar workers (people with equivalent jobs and qualifications), the gender pay ratio all but disappeared—from 83% when uncontrolled to 99% when controlled. This would leave only 1% of the gap unexplained by measurable factors. For comparison, one of the most well-cited analyses of the gender pay gap (Blau & Kahn, 2017) left 38% unexplained.
The Payscale report does not lay out their research methods in a traditional manner , making it very difficult to discern the full list of controls that they used. Based on our reading of their report it seems that, at minimum, the following variables were likely included as controls: occupation, industry, job tenure, hours worked, experience, education, age, race, parent status, remote work status, and location (Payscale, 2024).
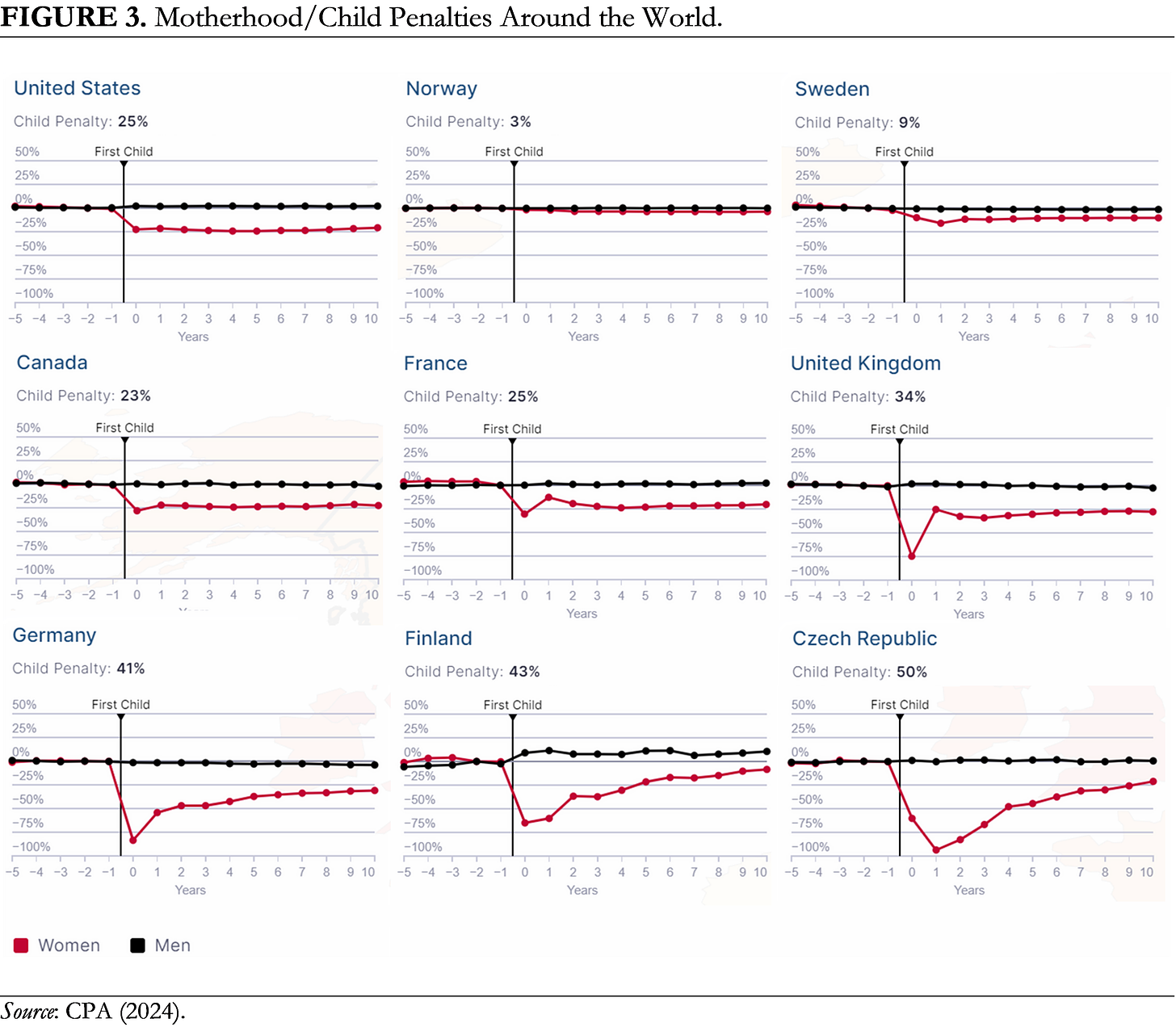
According to the authors of the Payscale report, the most powerful factor in their analysis was sex differences in childcare: “The ‘motherhood penalty’ explains the gender pay gap” (Payscale, 2024).
Given the difficulty in assessing Payscale’s methodology based on the limited information they provide online, their findings cannot be given nearly the same weight as those from leading peer-reviewed studies.
Occupational Segregation
The presence of a child is clearly impactful on women’s wages in the U.S. Another crucial factor to consider is occupational segregation.
In their well-cited 2017 analysis , economists Francine Blau and Lawrence Kahn found three variables that stood out as particularly influential, explaining most of the pay gap in their study: occupational segregation, sex differences in industry, and sex differences in labor force experience, with occupational segregation playing the largest role (Blau & Kahn, 2017, p. 799). [8] Blau explains their findings :
“[W]e have considerable occupational segregation by gender. . . Men and women also tend to work in different industries. . . [T]ogether, occupation and industry explain about half of the gender wage gap. Among professional workers, women are more likely to be in relatively lower-paying jobs, such as elementary school teachers, whereas men would be more likely to be in higher-paying jobs, like lawyers or doctors. Women also tend to be more concentrated in lower-paying service occupations, like childcare workers. Gender differences in college major are really important and are related to the occupational differences. We've had equalization in terms of gender opportunities in education to the point that women are now exceeding men. But despite some convergence there are still sizable differences in college major and these are very closely tied to labor market outcomes. In STEM [science, technology, engineering, and mathematics] fields, for instance, women are particularly underrepresented” (Eppard & Blau, 2020, p. 32).
Andrew Chamberlain and Jyotsna Jayaraman (2017) came to a similar conclusion in their research, focusing specifically on the different types of majors that male and female college students end up choosing:
“[T]he biggest cause of today’s gender pay gap is that men and women sort into different jobs—men into higher-paying positions and women into traditionally lower-paying jobs. . . During college, men and women gravitate toward different majors. . . This puts men and women on different career tracks—with different pay—after college. . . Many college majors that lead to high-paying roles in tech and engineering are male dominated, while majors that lead to lower-paying roles in social sciences and liberal arts tend to be female-dominated, placing men in higher-paying career pathways, on average. . . Nine of the 10 highest paying majors we examined are male-dominated. . . Choice of college major can have a dramatic impact on jobs and pay later on. Our results suggest that gender imbalances among college majors are an important and often overlooked driver of the gender pay gap” (p. 2).
Why Such Inequalities?
Taken together, the weight of the evidence suggests that the two biggest reasons for unequal pay between male and female workers in the U.S. are the motherhood penalty [9] and occupational segregation. So why do these sex differences in childcare responsibilities and occupations exist? There have been a variety of reasons put forth.
Some of the reasons for occupational segregation may stem from differences in the characteristics of men and women themselves. On average, for instance, men and women tend to prefer different professions —this shows up quite starkly in college , where there are large differences in the types of majors that women and men tend to choose (Chamberlain & Jayaraman, 2017). These preferences could be the result of innate differences between men and women, differential societal pressures and expectations by sex, or some combination.
There are observed group differences in psychological traits between the sexes which may play a role here, such as differences in competitiveness, risk aversion, importance placed on family, agreeableness, and importance placed on work/money, among others (Blau & Kahn, 2017, p. 838). These differences may influence the types of occupations that women and men end up choosing, the promotions they seek, the number of hours they end up working, the work/family balance they desire, their perceived job performance, etc. [10]
We cannot rule out the presence of discrimination. A recent meta-analysis (Schaerer et. al., 2023) of 85 experimental employment audit studies conducted from 1976 to 2020 involving over 360,000 job applications found that, over the last decade at least, women do not seem to be discriminated against when it comes to job callbacks:
“The present meta-analysis finds that discrimination against female applicants for jobs historically held by men has declined significantly and is no longer observable in the last decade. In contrast, bias against male applicants for female-typed jobs has remained robust and stable over the years. These results thus demonstrate both welcome declines in and the stubborn persistence of different forms of gender discrimination. Contrary to the beliefs of laypeople and academics revealed in our forecasting survey, after years of widespread gender bias in so many aspects of professional life, at least some societies have clearly moved closer to equal treatment when it comes to applying for many jobs” (Schaerer et. al., 2023).
This is of course a sign of great progress toward gender equality. But these results are for callbacks alone and do not mean that there might not be unequal treatment after the callback—such as in the actual decision to hire, as well as later decisions about pay, workplace treatment, promotions, firing, etc.
Take the Payscale study discussed earlier as an example. Even though the controlled gender pay gap in their analysis was only 1%, there were specific occupations where it was much more pronounced, including drivers (17%) and some jobs in religious organizations (13%). And while the overall controlled gender pay gap was 1% for the lowest job levels, it grew to 6% at the highest job levels for women of all races and 11% for Hispanic women (Payscale, 2024). So while it is plausible that discrimination is not the primary factor driving the overall gender pay gap in the U.S. today, gender norms still may be strong enough in some industries and workplaces so that they continue to contribute to gender inequality.
Why do we have such differences in childcare by sex? As we have discussed, mothers are more likely to either drop out of the labor force for a period of time when they have a child, scale back their hours, and/or deprioritize their careers in some other manner much more than working fathers:
“Parenthood leads some women to put their careers on hold, whether by choice or necessity. . . Fathers, however, are more likely to hold a job or be looking for one than men who don’t have children at home. . . Among those who do have a job, fathers also work a bit more each week, on average, than men who do not have children at home” (Kochhar, 2023).
Astrid Kunze similarly notes that, unlike many fathers, when mothers have a child, “they decide whether and when to return to work, and, if they return to work, whether to work full-time or part-time” (2017, p. 13).
This has a substantial impact on the money women are able to earn as well as their career advancement. It also may impact not only how much a mother works but the types of jobs she is willing/able to take on.
As is likely the case for occupational segregation, it is plausible that some combination of both individual preferences on the part of women themselves as well as societal pressures and expectations contribute to sex differences in childcare responsibilities.
In the spring of 2024, we undertook an exploratory study into the gender pay gap in the U.S., hoping to learn more about the processes responsible for occupational segregation. We were particularly interested in sex differences in college major selection and whether they are moderated by social class.
Our two hypotheses were that (a) female students would be overrepresented in lower-paying majors and (b) working-class females, while still overrepresented in these majors, would be more likely to choose higher-paying majors compared with non-working-class females, given their backgrounds and the greater salience of economic security for them.
The first hypothesis follows from previous research which suggests the presence of such differential decision-making by sex.
The second hypothesis assumes that, while working-class women will be influenced in their college major choice by their sex, they will also be influenced by their social class in ways that non-working-class women are not. We posit that economic security will be a more salient concern for them when compared with non-working-class women, thus moderating the impact of sex and leading to less unequal representation in higher-paying majors.
In order to explore these hypotheses, we first secured detailed enrollment data from a regional university in the Mid-Atlantic region of the United States (henceforth referred to by the pseudonym “Mid-Atlantic University” or “MAU”). [11] These data allowed us not only to calculate the percentage of women and men in each major at this university, but to examine whether these percentages were different for first-generation (our proxy for working-class) female students compared with non-first-generation ones.
After completing this analysis, we then distributed a Qualtrics survey to a convenience sample of students at the university, receiving 38 responses. This survey asked a number of open-ended questions about how these students arrived at their college major decision. We then analyzed their responses for any patterns that might help further illuminate our quantitative findings.
The scatterplot in Figure 4 (below) displays the association between sex and college major selection in our analysis of enrollment data. The x-axis (horizontal axis) is the percentage of men in each major, while the y-axis (vertical axis) displays the median starting salaries in the first five years that these majors are in the labor force. As you can see, there is a strong correlation (r = 0.78, p < .001) between sex and starting salary. As you move left-to-right on the horizontal axis, the percentage of men in each major increases, corresponding with a significant increase in starting salaries on the vertical axis by the time you get to the most male-dominated majors.
Table 2 (below) displays our analysis of the possible moderation by social class of the relationship between sex and college major selection. We calculated a cross-tabulation of the average starting salaries for the highest-paying, middle-paying, and lowest-paying college major tiers. There was more than a $20k difference between the highest-paying ($67,709) and lowest-paying ($47,242) tiers, with middle-paying majors yielding a $51,664 average starting salary.
In this same table we analyzed the percentages of first-generation and non-first-generation women who chose majors in these three tiers. We found that there was little difference between the two groups. Nearly identical percentages of both first-generation (10.6%) and non-first-generation (10.2%) women choose the highest-paying majors, while similarly high percentages of first-generation (65.7%) and non-first-generation (63.4%) women chose the lowest-paying majors.
To deepen our analysis and further illuminate these quantitative findings, we surveyed a convenience sample of undergraduate students from MAU to better understand their choice of college major, receiving 38 responses.
There were three overwhelmingly dominant themes (see Table 3 below) in the female MAU undergraduates’ survey responses explaining why they chose their college majors:
Having a personal interest in a particular area of study.
Desiring to pick a major that will lead to a job that she knows she will like.
Desiring a major that matches her abilities and that she knows she will succeed in.
Another slightly less frequent but still popular theme was the desire to improve society and/or help people (see Table 3 below).
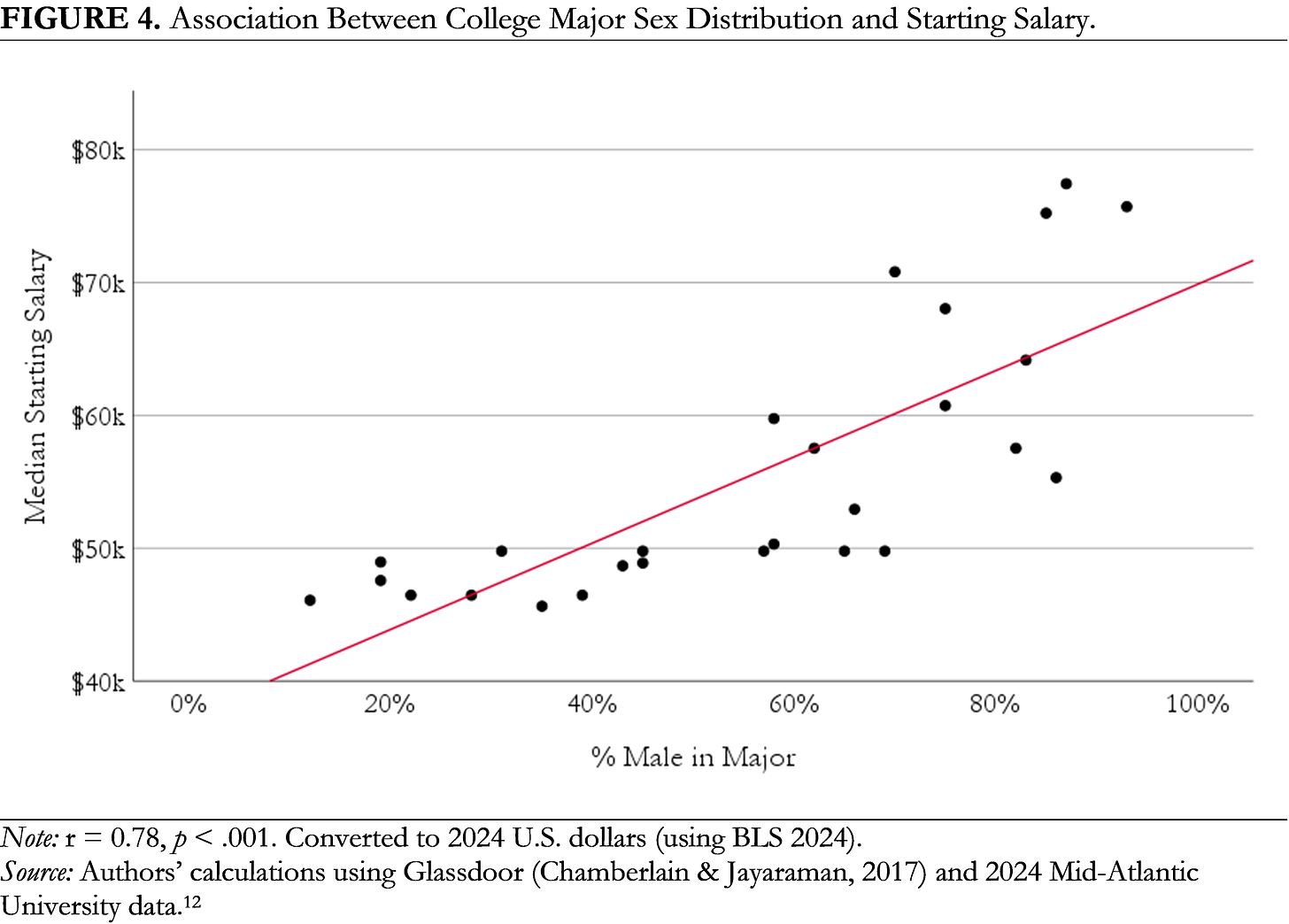
Although researchers have made plausible arguments about the contributions of several factors, the motherhood penalty and occupational segregation are widely cited as two of the most important causes of the gender pay gap in the U.S. today.
In this article we discussed an exploratory study we conducted in spring 2024 into one particular stage in the process of occupational segregation: the choice of college major.
We hypothesized that (a) female students would be overrepresented in lower-paying majors, and (b) working-class females, while still overrepresented in these majors, would be more likely to choose higher-paying majors, given their backgrounds and the greater salience of economic security for them compared with non-working-class females.
Using enrollment data from a university in the Mid-Atlantic region of the U.S., our first hypothesis was supported: females were overrepresented, to a significant degree, in majors with the lowest starting salaries. The strong correlation between sex and college major selection aligned with previous research in this area.
Our second hypothesis was not supported: the distribution of working-class females across salary tiers was virtually identical to that of non-working-class females.
In the fall of 2024 we plan to begin expanding our study into a full empirical investigation, surveying far more students, conducting focus groups , adding questions about students’ future plans regarding work/family balance, adding statistical complexity to our analysis of enrollment data, possibly including more college campuses, and more.
Author Bios
Lawrence M. Eppard is a sociology faculty member and Director of the Connors Institute for Nonpartisan Research & Civic Engagement at Shippensburg University. He also hosts Connors’s Utterly Moderate Podcast ( ConnorsInstitute.org ).
Annie Linker is an undergraduate research fellow at the Connors Institute.
Madison Laughman is a graduate research fellow at the Connors Institute.
Katie Bonomo is an undergraduate research fellow at the Connors Institute.
Bibliography
American Association of University Women (AAUW). (2024). Fast Facts: Occupational Segregation. Retrieved May 17, 2024, from https://www.aauw.org/resources/article/occupational-segregation/ .
Bertrand, Marianne, Claudia Goldin, & Lawrence F. Katz. (2010). Dynamics of the Gender Gap for Young Professionals in the Financial and Corporate Sectors. American Economic Journal: Applied Economics, 2 (July), 228-255.
Blau, Francine D., & Lawrence M. Kahn. (2017). The Gender Wage Gap: Extent, Trends, and Explanations. Journal of Economic Literature , 55 (3), 789-865.
Bleiweis, Robin. (2020). Quick Facts About the Gender Wage Gap. Center for American Progress. Retrieved May 17, 2024, from https://www.americanprogress.org/article/quick-facts-gender-wage-gap/ .
Chamberlain, Andrew, & Jyotsna Jayaraman. (2017). The Pipeline Problem: How College Majors Contribute to the Gender Pay Gap. Glassdoor. Retrieved Feb. 27, 2024, from https://research.glassdoor.com/site-us/wp-content/uploads/sites/2/2017/04/FULL-STUDY-PDF-Gender-Pay-Gap2FCollege-Major.pdf .
Child Penalty Atlas (CPA). (2024). Retrieved May 24, 2024, from https://childpenaltyatlas.org/
Eppard, Lawrence M., & Francine Blau. (2020). Minding the Gap: Explaining Gender Differences in Wages in the United States. Sociation, 19 (1), 31-35.
Federal Research Bank of St. Louis. (2024). Labor Force Participation Rate – Women. Retrieved May 22, 2024, from https://fred.stlouisfed.org/series/LNS11300002 .
Guzman, Gloria, & Melissa Kollar. (2023). Income in the United States: 2022. United States Census Bureau. Retrieved May 24, 2024, from https://www.census.gov/content/dam/Census/library/publications/2023/demo/p60-279.pdf .
Jacobson, Louis. (2012). Barack Obama Ad Says Women Are Paid “77 Cents on the Dollar for Doing the Same Work as Men.” PolitiFact. Retrieved May 21, 2024, from https://www.politifact.com/factchecks/2012/jun/21/barack-obama/barack-obama-ad-says-women-are-paid-77-cents-dolla/ .
Klevin, Henrik, Camille Landais, & Gabriel Leite-Mariante. (2023). The Child Penalty Atlas. NBER. Retrieved May 24, 2024, from https://www.nber.org/papers/w31649 .
Kochhar, Rakesh. (2023). The Enduring Grip of the Gender Pay Gap. Pew Research Center. Retrieved May 17, 2024, from https://www.pewresearch.org/social-trends/2023/03/01/the-enduring-grip-of-the-gender-pay-gap/ .
Kunze, Astrid. (2017). The Gender Wage Gap in Developed Countries. IZA Institute of Labor Economics. Retrieved May 21, 2024, from https://docs.iza.org/dp10826.pdf .
The Nobel Foundation (Nobel). 2023. Press Release. Retrieved May 21, 2024, from https://www.nobelprize.org/prizes/economic-sciences/2023/press-release .
Payscale. (2024). Gender Pay Gap Report. Retrieved May 16, 2024, from https://www.payscale.com/research-and-insights/gender-pay-gap/#module-14 .
Schaerer, Michael, Christilene du Plessis, My Hoang Bao Nguyen, Robbie C.M. van Aert, Leo Tiokhin, Daniël Lakens, Elena Giulia Clemente, Thomas Pfeiffer, Anna Dreber, Magnus Johannesson, Cory J. Clark, Gender Audits Forecasting Collaboration, and Eric Luis Uhlmann. (2023). On the Trajectory of Discrimination: A Meta-Analysis and Forecasting Survey Capturing 44 Years of Field Experiments on Gender and Hiring Decisions. Organizational Behavior and Human Decision Processes, 179 (November), 1-26.
Unsiloed Podcast with Greg LaBlanc (LaBlanc). (2023). Understanding the Gender Wage Gap featuring Claudia Goldin. YouTube. Retrieved May 21, 2024, from YouTube.
U.S. Bureau of Labor Statistics. (2024). CPI Inflation Calculator. Retrieved May 24, 2024, from https://data.bls.gov/cgi-bin/cpicalc.pl .
U.S. Census Bureau (2024). Equal Pay Day: March 12, 2024. Retrieved May 24, 2024, from https://www.census.gov/newsroom/stories/equal-pay-day.html .
[1] Director of the Connors Institute for Nonpartisan Research & Civic Engagement and associate professor of sociology at Shippensburg University. Connors’s website: ConnorsInstitute.org . Connors’s email: [email protected] .
[2] Connors Institute research fellow and Shippensburg University undergraduate student.
[3] Connors Institute research fellow and Shippensburg University graduate student.
[4] Connors Institute research fellow and Shippensburg University undergraduate student.
[5] As it does with so many important and complicated societal issues .
[6] “So-called women’s jobs, which are jobs that have historically had majority-female workforces, such as home health aides and child care workers, tend to offer lower pay and fewer benefits than so-called men’s jobs, which are jobs that have had predominantly male workforces, including jobs in trades such as building and construction” (Bleiweis, 2020).
[7] More precisely she won the “2023 Sveriges Riksbank Prize in Economic Sciences in Memory of Alfred Nobel.”
[8] “[G]ender differences in occupations and industries are quantitatively the most important measurable factors explaining the gender wage gap (in an accounting sense). . . [G]ender differences in location in the labor market, a factor long highlighted in research on the gender wage gap, remain exceedingly relevant” (Blau & Kahn, 2017, p. 854).
[9] Astrid Kunze explains that, “Traditionally, most men work full time and continuously throughout their lives. For women, the employment picture is much more varied” (2017, p. 13). And the authors of the Payscale report find that, “The most likely explanation for the widening gender pay gap by age is some women becoming mothers and leaving the workforce, incurring the aforementioned motherhood penalty” (2024).
[10] It should be noted that all the independent variables we discuss—from occupational segregation to industry differences to psychological traits and beyond—may impact the gender pay gap directly and/or each other as well. Competitiveness may impact occupational segregation in several ways, for instance—which industries you consider pursuing/avoiding, which promotions you seek/earn, your job tenure, your work/family balance, etc.
[11] No citation or bibliography entry included for MAU enrollment data in order to protect anonymity. This is an ethical consideration and IRB requirement .
[12] No citation or bibliography entry included for MAU enrollment data in order to protect anonymity. This is an ethical consideration and IRB requirement .
Ready for more?
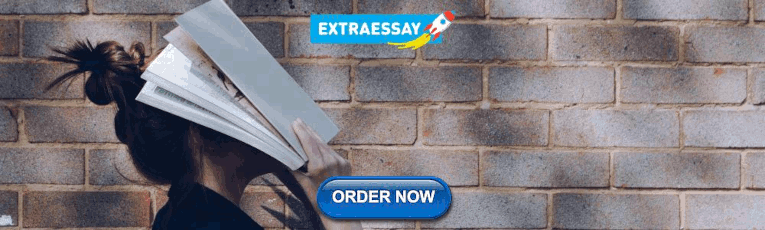
IMAGES
COMMENTS
trends in the US gender wage gap and on their sources (in a descriptive sense). Accounting for the sources of the level and changes in the gender pay gap will provide guidance for understanding recent research studying gender and the labor market. Figure 1 shows the long-run trends in the gender pay gap over the 1955-2014 period based on two
The study uses meta-analysis as a research tool to estimate gender pay gap from 263 prior studies that estimate the gender pay gap on the workforce. The study concludes that raw gender pay differential has steadily declined across the globe but the pay gap is still persistent.
The gender wage gap refers to the differences between the wages earned by women and men in comparable jobs that generate equal values (OECD 2021). At first glance it seems like a clear and uncontroversial definition; however, applying this definition to data is less straight forward. ... Published articles and research papers on the impact of ...
Introduction. The gender pay gap, the disparity in earnings between male and female workers, has been the focus of empirical research in the US for decades, as well as legislative and executive action under the Obama administration [1, 2].Trends dating back to the 1960s show a long period in which women's earnings were approximately 60% of their male counterparts, followed by increases in ...
A good share of the increase in the gender pay gap takes place when women are between the ages of 35 and 44. In 2022, women ages 25 to 34 earned about 92% as much as men of the same ages, but women ages 35 to 44 and 45 to 54 earned 83% as much. The ratio dropped to 79% among those ages 55 to 64.
the US gender wage gap and their sources (in a descriptive sense). Accounting for the sources of the level and changes in the gen-der pay gap will provide guidance for under-standing recent research studying gender and the labor market. Figure 1 shows the long-run trends in the gender pay gap over the 1955-2014 period
By 2010, conventional human capital variables taken together explained little of the gender wage gap, while gender differences in occupation and industry continued to be important. Moreover, the gender pay gap declined much more slowly at the top of the wage distribution than at the middle or bottom and by 2010 was noticeably higher at the top.
Research has shown that the gender pay gap increases substantially during this time and is relatively stable thereafter (Manning and Swaffield 2008). These early-career dynamics are the subject of their own literature (discussed ... 2 Our paper estimates conditional average gender gaps across various levels of pre -labor market human capital (i.e.
The pay gap has declined throughout the distribution since 2006, but it has declined more for the most educated women. Current economics-of-gender research focuses heavily on the top end; equal emphasis should be placed on mechanisms driving gender inequality for non-college-educated workers.
The findings suggest that the gender pay gap has a significant impact on women's economic empowerment, limiting their financial independence and autonomy. The study also highlights the need for ...
To inform these questions, we conduct two analyses. First, we estimate regressions using data on gender gaps in a range of labor market outcomes from 153 countries spanning two decades (1998-2018). Second, we conduct a systematic review of the recent economics literature on gender gaps in labor markets, examining 16 journals over 21 years.
Find out with our pay gap calculator. In 2019 women in the United States earned 82% of what men earned, according to a Pew Research Center analysis of median annual earnings of full-time, year-round workers. The gender wage gap varies by age and metropolitan area, and in most places, has narrowed since 2000. See how women's wages compare with ...
To understand how gender pay gaps change with employees' firm tenure, I build on Correll and Benard (2006) and distinguish between information- and status-based theories of pay disparities. Information-based approaches, such as statistical discrimination, emphasize that managers are uncertain of applicants' future productivity (e.g., Akerlof, 1970; Bidwell, 2011; Halaby, 1988; Jovanovic ...
The gender wage gap and education. In the general workforce, women's annual earnings were 82.3% of men's in 2020, 4 and this gender gap in pay has remained relatively stable in the United States over the past 15 years. 5 For women of color, the gap is even wider; Hispanic women earned just 57 cents and Black women earned 64 cents for every $1 earned by White, non-Hispanic men in 2020. 6 The ...
Studies of the gender wage gap using data from the late 1980s through the early 2000s consistently identify an unconditional gender wage gap at research universities of about 20%. The unexplained portion of the gap is in the range of 4% to 6% of salaries, or 20% to 30% of the unconditional gap.
This brief2 compiles recent research on the impact of equal pay laws and policies on the gender wage gap. It presents studies under five topic areas: (1) salary history bans; (2) pay transparency policies; (3) gender and salary negotiations; (4) gender bias in performance management and performance-related pay; and (5) occupational segregation ...
While the focus of the research is on the gender pay gap, the paper has presented more broadly interesting empirical insights into the perception of discrimination at work by female and male employees. The traditional approach for explaining the gender pay gap has mainly focused on the weaknesses of women compared to men. This approach, however ...
overall gender wage gap over the distribution of wages. The 2M research team found that the raw gender wage gap was 17.4 percent at the mean and about 20 percent at the median. The raw wage gap increases between the 50th thand 75 percentile and remains greatest at the higher percentiles. In
In reality, however, the gender wage gap is wider for those with higher earnings. Women in the top 95th percentile of the wage distribution experience a much larger gender pay gap than lower-paid women. Again, this large gender pay gap between the highest earners is partially driven by gender bias.
There is a large gender wage gap among college graduates. This gender gap could be partially driven by differences in college major and prior skills. We use Swedish register data to study how much of the gender gap can be explained by differences in majors, skills, and skill prices. College majors explain 60 percent of the gender wage gap, but ...
The gender gap in pay has remained relatively stable in the United States over the past 20 years or so. In 2022, women earned an average of 82% of what men earned, according to a new Pew Research Center analysis of median hourly earnings of both full- and part-time workers. These results are similar to where the pay gap stood in 2002, when ...
The gender pay gap has been observed for decades, and still exists. Due to a life course perspective, gender differences in income are analyzed over a period of 24 years. Therefore, this study aims to investigate income trajectories and the differences regarding men and women. ... Hamburg, Germany: HWWI Research Paper, 179. [Google Scholar ...
We examine how the gender of business owners is related to the wages paid to female relative to male employees working in their firms. Using Finnish register data and employing firm fixed effects, we find that the gender pay gap is—starting from a gender pay gap of 11 to 12%—two to three percentage points lower for hourly wages in female-owned firms than in male-owned firms. Results are ...
Table 4.4: The gender pay gap comparing married to unmarried people (£ per hour) Table 4.5: The gender pay gap by marital status and age (£ per hour) Table 4.6: The gender pay gap by presence of dependent children in household Table 4.7: Housework and paid work hours (UKHLS; 45,533 observations) Table 4.8: The pay gap by educational level
As the gender gap in pay between women and men has been narrowing, the 'family gap' in pay between mothers and nonmothers has been widening. One reason may be the institutional structure in the United States, which has emphasized equal pay and opportunity policies but not family policies, in contrast to other countries that have implemented both.
The gender pay gap or gender wage gap is the average difference between the ... A paper published in 2019 by the International Monetary Fund predicted that women are significantly more likely to be displaced by automation than male workers. ... A 2010 research review by the majority staff of the United States Congress Joint Economic Committee ...
The government has recently introduced new gender pay gap (GPG) transparency regulations, which are designed to encourage large employers to take informed action to close their GPG where one ...
The most recent data published by the U.S. Census Bureau (Guzman & Kollar, 2023, p. 8) show that the median earnings of American men who worked full-time, year-round was $62,350 in 2022, while it was $52,360 for their female counterparts. This translates into a gender pay ratio of 84% and gender pay gap of 16%. In Figure 1 (above) and Table 1 (below) you can see how this gap has changed over time.