Problem Solving vs. Planning : A simple planning agent is very similar to problem-solving agents in that it constructs plans that achieve its goals, and then executes them. The limitations of the problem-solving approach motivates the design of planning systems.
To solve a planning problem using a state-space search approach we would let the:
- initial state = initial situation
- goal-test predicate = goal state description
- successor function computed from the set of operators
- once a goal is found, solution plan is the sequence of operators in the path from the start node to the goal node
In searches, operators are used simply to generate successor states and we can not look "inside" an operator to see how it’s defined. The goal-test predicate also is used as a "black box" to test if a state is a goal or not. The search cannot use properties of how a goal is defined in order to reason about finding path to that goal. Hence this approach is all algorithm and representation weak.
Planning is considered different from problem solving because of the difference in the way they represent states, goals, actions, and the differences in the way they construct action sequences.
Remember the search-based problem solver had four basic elements:
- Representations of actions: programs that develop successor state descriptions which represent actions.
- Representation of state: every state description is complete. This is because a complete description of the initial state is given, and actions are represented by a program that creates complete state descriptions.
- Representation of goals: a problem solving agent has only information about it's goal, which is in terms of a goal test and the heuristic function.
- Representation of plans: in problem solving, the solution is a sequence of actions.
In a simple problem: "Get a quart of milk and a bunch of bananas and a variable speed cordless drill" for a problem solving exercise we need to specify: Initial State: the agent is at home without any objects that he is wanting.
Operator Set: everything the agent can do.
Heuristic function: the # of things that have not yet been acquired.
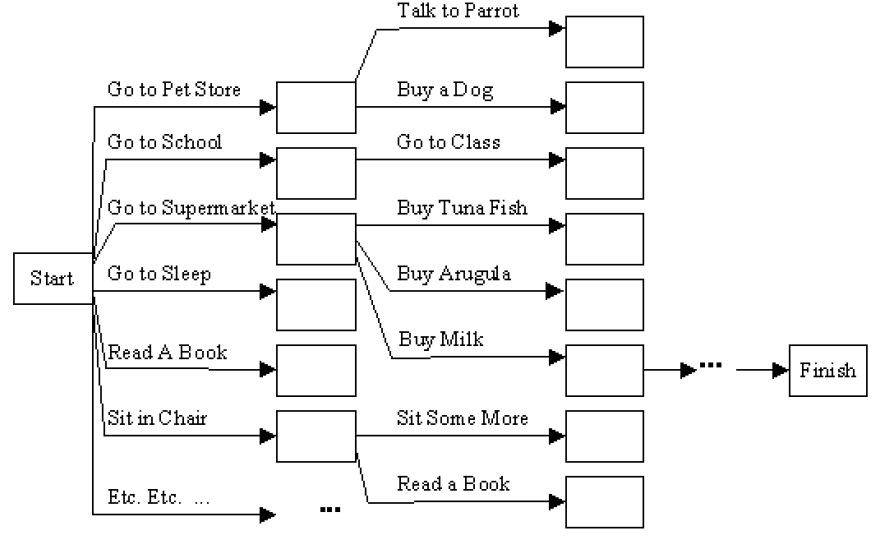
Problems with Problem solving agent:
- It is evident from the above figure that the actual branching factor would be in the thousands or millions. The heuristic evaluation function can only choose states to determine which one is closer to the goal. It cannot eliminate actions from consideration. The agent makes guesses by considering actions and the evaluation function ranks those guesses. The agent picks the best guess, but then has no idea what to try next and therefore starts guessing again.
- It considers sequences of actions beginning from the initial state. The agent is forced to decide what to do in the initial state first, where possible choices are to go to any of the next places. Until the agent decides how to acquire the objects, it can't decide where to go.
Planning emphasizes what is in operator and goal representations. There are three key ideas behind planning:
- to "open up" the representations of state, goals, and operators so that a reasoner can more intelligently select actions when they are needed
- the planner is free to add actions to the plan wherever they are needed, rather than in an incremental sequence starting at the initial state.
- most parts of the world are independent of most other parts which makes it feasible to take a conjunctive goal and solve it with a divide-and-conquer strategy.
- Brain Development
- Childhood & Adolescence
- Diet & Lifestyle
- Emotions, Stress & Anxiety
- Learning & Memory
- Thinking & Awareness
- Alzheimer's & Dementia
- Childhood Disorders
- Immune System Disorders
- Mental Health
- Neurodegenerative Disorders
- Infectious Disease
- Neurological Disorders A-Z
- Body Systems
- Cells & Circuits
- Genes & Molecules
- The Arts & the Brain
- Law, Economics & Ethics
- Neuroscience in the News
- Supporting Research
- Tech & the Brain
- Animals in Research
- BRAIN Initiative
- Meet the Researcher
- Neuro-technologies
- Tools & Techniques
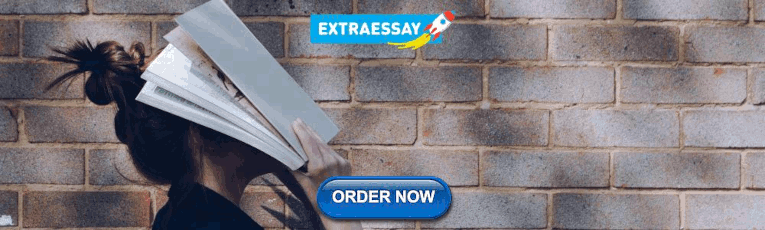
Core Concepts
- For Educators
- Ask an Expert
- The Brain Facts Book

Reasoning, Planning, and Problem-Solving
- Published 15 Aug 2019
- Author Hannah Zuckerman
- Source BrainFacts/SfN
Checking the weather forecast then planning what to wear to work doesn’t seem like a serious challenge as you age. That’s because your brain has prepared you to solve everything from the problems of your daily routine to deep conundrums.
Your brain incorporates all available information from your senses, memories, and emotions to help you reason and understand your environment. All these things support your judgement, guiding you to respond effectively to events, make decisions, and predict future outcomes — like seeing there’s a 70% chance of showers and taking an umbrella as you head out the door.
Click on the targets in the image to explore how your brain reasons, plans, and solves problems.
About the Author
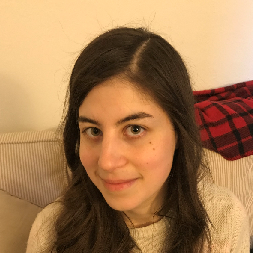
Hannah Zuckerman
Hannah is the former Production and Editorial Associate for BrainFacts.org . She graduated from Bryn Mawr College in 2014 with a degree in history. She previously worked at Princeton University Press and the art magazine Sculpture .
CONTENT PROVIDED BY
BrainFacts/SfN
Also In Learning & Memory
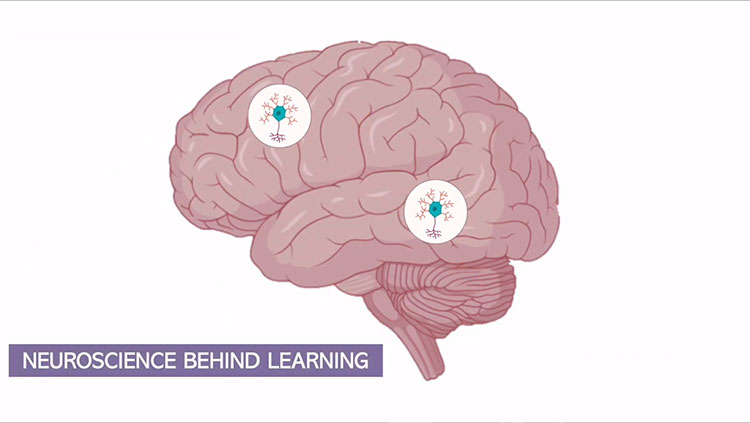
Popular articles on BrainFacts.org
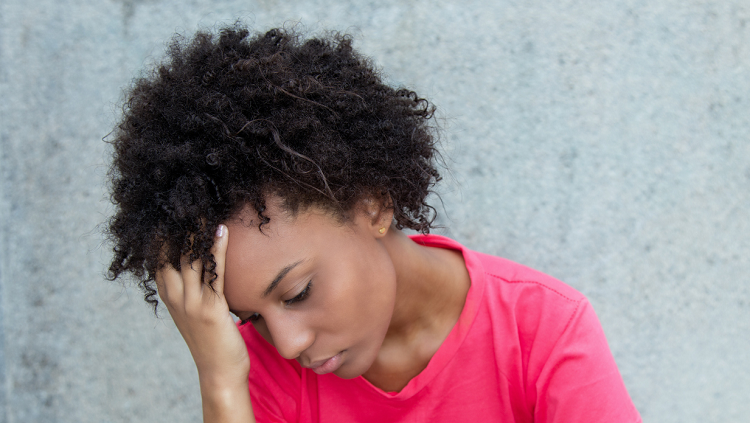
A beginner's guide to the brain and nervous system.
BrainFacts Book
Download a copy of the newest edition of the book, Brain Facts: A Primer on the Brain and Nervous System.
Brain Awareness Week
A worldwide celebration of the brain that brings together scientists, families, schools, and communities during the third week in March.
Join the Campaign
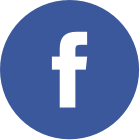
SUPPORTING PARTNERS
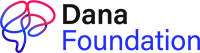
- Privacy Policy
- Accessibility Policy
- Terms and Conditions
- Manage Cookies
Some pages on this website provide links that require Adobe Reader to view.
Learn Creative Problem Solving Techniques to Stimulate Innovation in Your Organization
By Kate Eby | October 20, 2017 (updated August 27, 2021)
- Share on Facebook
- Share on LinkedIn
Link copied
In today’s competitive business landscape, organizations need processes in place to make strong, well-informed, and innovative decisions. Problem solving - in particular creative problem solving (CPS) - is a key skill in learning how to accurately identify problems and their causes, generate potential solutions, and evaluate all the possibilities to arrive at a strong corrective course of action. Every team in any organization, regardless of department or industry, needs to be effective, creative, and quick when solving problems.
In this article, we’ll discuss traditional and creative problem solving, and define the steps, best practices, and common barriers associated. After that, we’ll provide helpful methods and tools to identify the cause(s) of problematic situations, so you can get to the root of the issue and start to generate solutions. Then, we offer nearly 20 creative problem solving techniques to implement at your organization, or even in your personal life. Along the way, experts weigh in on the importance of problem solving, and offer tips and tricks.
What Is Problem Solving and Decision Making?
Problem solving is the process of working through every aspect of an issue or challenge to reach a solution. Decision making is choosing one of multiple proposed solutions — therefore, this process also includes defining and evaluating all potential options. Decision making is often one step of the problem solving process, but the two concepts are distinct.
Collective problem solving is problem solving that includes many different parties and bridges the knowledge of different groups. Collective problem solving is common in business problem solving because workplace decisions typically affect more than one person.
Problem solving, especially in business, is a complicated science. Not only are business conflicts multifaceted, but they often involve different personalities, levels of authority, and group dynamics. In recent years, however, there has been a rise in psychology-driven problem solving techniques, especially for the workplace. In fact, the psychology of how people solve problems is now studied formally in academic disciplines such as psychology and cognitive science.
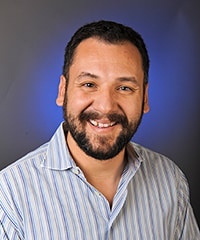
Joe Carella is the Assistant Dean for Executive Education at the University of Arizona . Joe has over 20 years of experience in helping executives and corporations in managing change and developing successful business strategies. His doctoral research and executive education engagements have seen him focus on corporate strategy, decision making and business performance with a variety of corporate clients including Hershey’s, Chevron, Fender Musical Instruments Corporation, Intel, DP World, Essilor, BBVA Compass Bank.
He explains some of the basic psychology behind problem solving: “When our brain is engaged in the process of solving problems, it is engaged in a series of steps where it processes and organizes the information it receives while developing new knowledge it uses in future steps. Creativity is embedded in this process by incorporating diverse inputs and/or new ways of organizing the information received.”
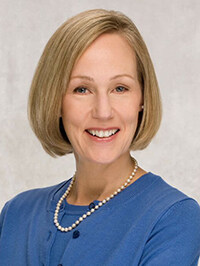
Laura MacLeod is a Professor of Social Group Work at City University of New York, and the creator of From The Inside Out Project® , a program that coaches managers in team leadership for a variety of workplaces. She has a background in social work and over two decades of experience as a union worker, and currently leads talks on conflict resolution, problem solving, and listening skills at conferences across the country.
MacLeod thinks of problem solving as an integral practice of successful organizations. “Problem solving is a collaborative process — all voices are heard and connected, and resolution is reached by the group,” she says. “Problems and conflicts occur in all groups and teams in the workplace, but if leaders involve everyone in working through, they will foster cohesion, engagement, and buy in. Everybody wins.”
10 tips that will make you more productive.
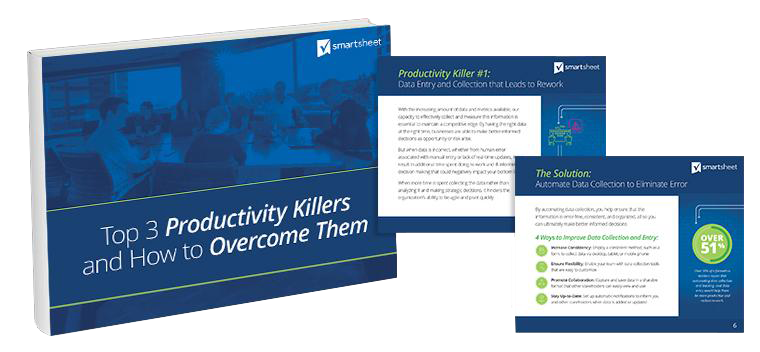
Uncover the top three factors that are killing your productivity and 10 tips to help you overcome them.
Download the free e-book to overcome my productivity killers
Project Management Guide
Your one-stop shop for everything project management
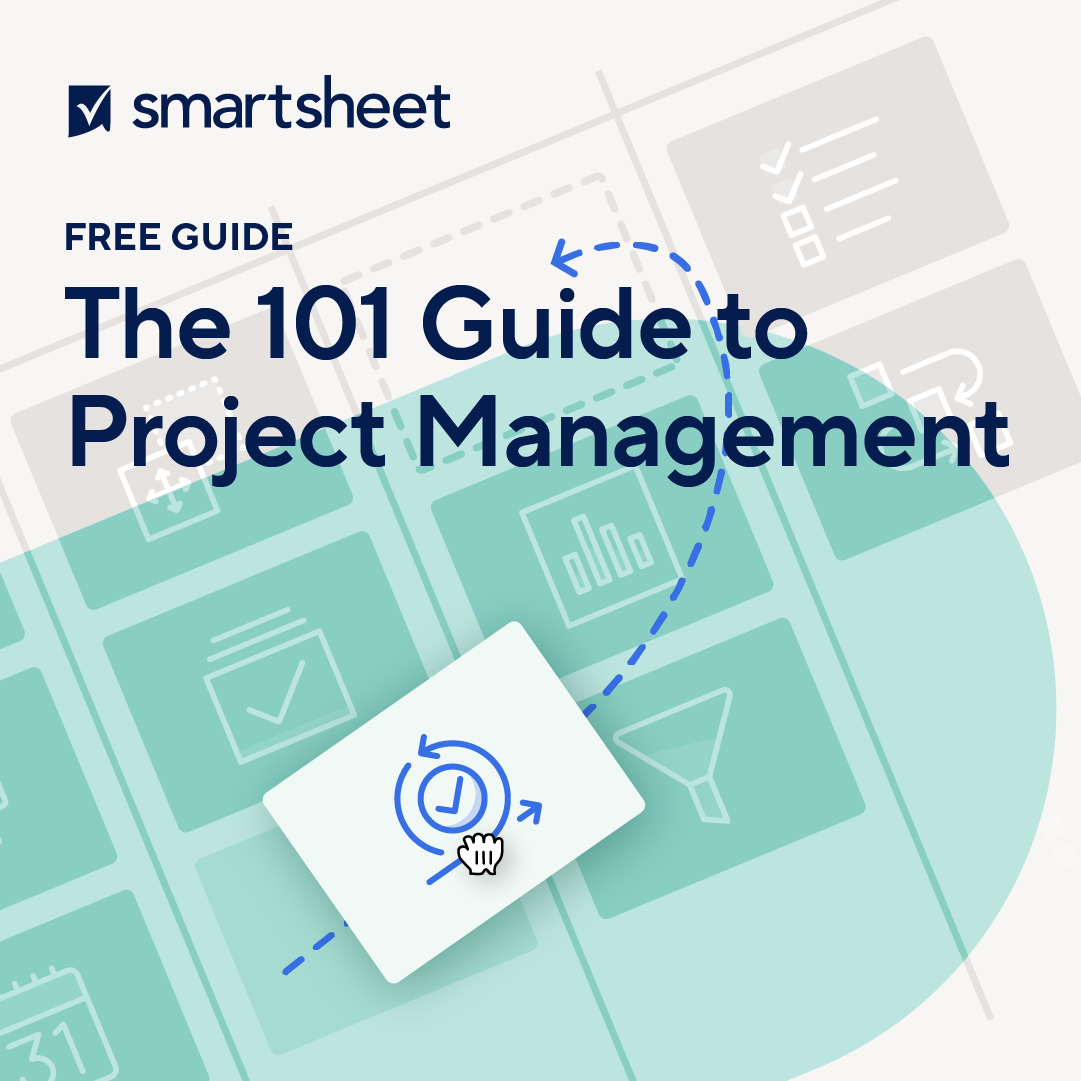
Ready to get more out of your project management efforts? Visit our comprehensive project management guide for tips, best practices, and free resources to manage your work more effectively.
View the guide
What Is the First Step in Solving a Problem?
Although problem solving techniques vary procedurally, experts agree that the first step in solving a problem is defining the problem. Without a clear articulation of the problem at stake, it is impossible to analyze all the key factors and actors, generate possible solutions, and then evaluate them to pick the best option.
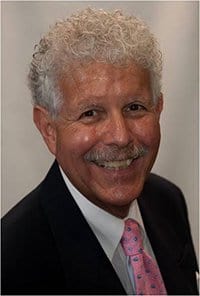
Dr. Elliott Jaffa is a behavioral and management psychologist with over 25 years of problem solving training and management experience. “Start with defining the problem you want to solve,” he says, “And then define where you want to be, what you want to come away with.” He emphasizes these are the first steps in creating an actionable, clear solution.
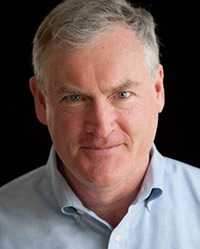
Bryan Mattimore is Co-Founder of Growth Engine, an 18-year old innovation agency based in Norwalk, CT. Bryan has facilitated over 1,000 ideation sessions and managed over 200 successful innovation projects leading to over $3 billion in new sales. His newest book is 21 Days to a Big Idea . When asked about the first critical component to successful problem solving, Mattimore says, “Defining the challenge correctly, or ‘solving the right problem’ … The three creative techniques we use to help our clients ‘identify the right problem to be solved’ are questioning assumptions, 20 questions, and problem redefinition. A good example of this was a new product challenge from a client to help them ‘invent a new iron. We got them to redefine the challenge as first: a) inventing new anti-wrinkle devices, and then b) inventing new garment care devices.”
What Are Problem Solving Skills?
To understand the necessary skills in problem solving, you should first understand the types of thinking often associated with strong decision making. Most problem solving techniques look for a balance between the following binaries:
- Convergent vs. Divergent Thinking: Convergent thinking is bringing together disparate information or ideas to determine a single best answer or solution. This thinking style values logic, speed, and accuracy, and leaves no chance for ambiguity. Divergent thinking is focused on generating new ideas to identify and evaluate multiple possible solutions, often uniting ideas in unexpected combinations. Divergent thinking is characterized by creativity, complexity, curiosity, flexibility, originality, and risk-taking.
- Pragmatics vs. Semantics: Pragmatics refer to the logic of the problem at hand, and semantics is how you interpret the problem to solve it. Both are important to yield the best possible solution.
- Mathematical vs. Personal Problem Solving: Mathematical problem solving involves logic (usually leading to a single correct answer), and is useful for problems that involve numbers or require an objective, clear-cut solution. However, many workplace problems also require personal problem solving, which includes interpersonal, collaborative, and emotional intuition and skills.
The following basic methods are fundamental problem solving concepts. Implement them to help balance the above thinking models.
- Reproductive Thinking: Reproductive thinking uses past experience to solve a problem. However, be careful not to rely too heavily on past solutions, and to evaluate current problems individually, with their own factors and parameters.
- Idea Generation: The process of generating many possible courses of action to identify a solution. This is most commonly a team exercise because putting everyone’s ideas on the table will yield the greatest number of potential solutions.
However, many of the most critical problem solving skills are “soft” skills: personal and interpersonal understanding, intuitiveness, and strong listening.
Mattimore expands on this idea: “The seven key skills to be an effective creative problem solver that I detail in my book Idea Stormers: How to Lead and Inspire Creative Breakthroughs are: 1) curiosity 2) openness 3) a willingness to embrace ambiguity 4) the ability to identify and transfer principles across categories and disciplines 5) the desire to search for integrity in ideas, 6) the ability to trust and exercise “knowingness” and 7) the ability to envision new worlds (think Dr. Seuss, Star Wars, Hunger Games, Harry Potter, etc.).”
“As an individual contributor to problem solving it is important to exercise our curiosity, questioning, and visioning abilities,” advises Carella. “As a facilitator it is essential to allow for diverse ideas to emerge, be able to synthesize and ‘translate’ other people’s thinking, and build an extensive network of available resources.”
MacLeod says the following interpersonal skills are necessary to effectively facilitate group problem solving: “The abilities to invite participation (hear all voices, encourage silent members), not take sides, manage dynamics between the monopolizer, the scapegoat, and the bully, and deal with conflict (not avoiding it or shutting down).”
Furthermore, Jaffa explains that the skills of a strong problem solver aren’t measurable. The best way to become a creative problem solver, he says, is to do regular creative exercises that keep you sharp and force you to think outside the box. Carella echoes this sentiment: “Neuroscience tells us that creativity comes from creating novel neural paths. Allow a few minutes each day to exercise your brain with novel techniques and brain ‘tricks’ – read something new, drive to work via a different route, count backwards, smell a new fragrance, etc.”
What Is Creative Problem Solving? History, Evolution, and Core Principles
Creative problem solving (CPS) is a method of problem solving in which you approach a problem or challenge in an imaginative, innovative way. The goal of CPS is to come up with innovative solutions, make a decision, and take action quickly. Sidney Parnes and Alex Osborn are credited with developing the creative problem solving process in the 1950s. The concept was further studied and developed at SUNY Buffalo State and the Creative Education Foundation.
The core principles of CPS include the following:
- Balance divergent and convergent thinking
- Ask problems as questions
- Defer or suspend judgement
- Focus on “Yes, and…” rather than “No, but…”
According to Carella, “Creative problem solving is the mental process used for generating innovative and imaginative ideas as a solution to a problem or a challenge. Creative problem solving techniques can be pursued by individuals or groups.”
When asked to define CPS, Jaffa explains that it is, by nature, difficult to create boundaries for. “Creative problem solving is not cut and dry,” he says, “If you ask 100 different people the definition of creative problem solving, you’ll get 100 different responses - it’s a non-entity.”
Business presents a unique need for creative problem solving. Especially in today’s competitive landscape, organizations need to iterate quickly, innovate with intention, and constantly be at the cutting-edge of creativity and new ideas to succeed. Developing CPS skills among your workforce not only enables you to make faster, stronger in-the-moment decisions, but also inspires a culture of collaborative work and knowledge sharing. When people work together to generate multiple novel ideas and evaluate solutions, they are also more likely to arrive at an effective decision, which will improve business processes and reduce waste over time. In fact, CPS is so important that some companies now list creative problem solving skills as a job criteria.
MacLeod reiterates the vitality of creative problem solving in the workplace. “Problem solving is crucial for all groups and teams,” she says. “Leaders need to know how to guide the process, hear all voices and involve all members - it’s not easy.”
“This mental process [of CPS] is especially helpful in work environments where individuals and teams continuously struggle with new problems and challenges posed by their continuously changing environment,” adds Carella.
Problem Solving Best Practices
By nature, creative problem solving does not have a clear-cut set of do’s and don’ts. Rather, creating a culture of strong creative problem solvers requires flexibility, adaptation, and interpersonal skills. However, there are a several best practices that you should incorporate:
- Use a Systematic Approach: Regardless of the technique you use, choose a systematic method that satisfies your workplace conditions and constraints (time, resources, budget, etc.). Although you want to preserve creativity and openness to new ideas, maintaining a structured approach to the process will help you stay organized and focused.
- View Problems as Opportunities: Rather than focusing on the negatives or giving up when you encounter barriers, treat problems as opportunities to enact positive change on the situation. In fact, some experts even recommend defining problems as opportunities, to remain proactive and positive.
- Change Perspective: Remember that there are multiple ways to solve any problem. If you feel stuck, changing perspective can help generate fresh ideas. A perspective change might entail seeking advice of a mentor or expert, understanding the context of a situation, or taking a break and returning to the problem later. “A sterile or familiar environment can stifle new thinking and new perspectives,” says Carella. “Make sure you get out to draw inspiration from spaces and people out of your usual reach.”
- Break Down Silos: To invite the greatest possible number of perspectives to any problem, encourage teams to work cross-departmentally. This not only combines diverse expertise, but also creates a more trusting and collaborative environment, which is essential to effective CPS. According to Carella, “Big challenges are always best tackled by a group of people rather than left to a single individual. Make sure you create a space where the team can concentrate and convene.”
- Employ Strong Leadership or a Facilitator: Some companies choose to hire an external facilitator that teaches problem solving techniques, best practices, and practicums to stimulate creative problem solving. But, internal managers and staff can also oversee these activities. Regardless of whether the facilitator is internal or external, choose a strong leader who will value others’ ideas and make space for creative solutions. Mattimore has specific advice regarding the role of a facilitator: “When facilitating, get the group to name a promising idea (it will crystalize the idea and make it more memorable), and facilitate deeper rather than broader. Push for not only ideas, but how an idea might specifically work, some of its possible benefits, who and when would be interested in an idea, etc. This fleshing-out process with a group will generate fewer ideas, but at the end of the day will yield more useful concepts that might be profitably pursued.” Additionally, Carella says that “Executives and managers don’t necessarily have to be creative problem solvers, but need to make sure that their teams are equipped with the right tools and resources to make this happen. Also they need to be able to foster an environment where failing fast is accepted and celebrated.”
- Evaluate Your Current Processes: This practice can help you unlock bottlenecks, and also identify gaps in your data and information management, both of which are common roots of business problems.
MacLeod offers the following additional advice, “Always get the facts. Don’t jump too quickly to a solution – working through [problems] takes time and patience.”
Mattimore also stresses that how you introduce creative problem solving is important. “Do not start by introducing a new company-wide innovation process,” he says. “Instead, encourage smaller teams to pursue specific creative projects, and then build a process from the ground up by emulating these smaller teams’ successful approaches. We say: ‘You don’t innovate by changing the culture, you change the culture by innovating.’”
Barriers to Effective Problem Solving
Learning how to effectively solve problems is difficult and takes time and continual adaptation. There are several common barriers to successful CPS, including:
- Confirmation Bias: The tendency to only search for or interpret information that confirms a person’s existing ideas. People misinterpret or disregard data that doesn’t align with their beliefs.
- Mental Set: People’s inclination to solve problems using the same tactics they have used to solve problems in the past. While this can sometimes be a useful strategy (see Analogical Thinking in a later section), it often limits inventiveness and creativity.
- Functional Fixedness: This is another form of narrow thinking, where people become “stuck” thinking in a certain way and are unable to be flexible or change perspective.
- Unnecessary Constraints: When people are overwhelmed with a problem, they can invent and impose additional limits on solution avenues. To avoid doing this, maintain a structured, level-headed approach to evaluating causes, effects, and potential solutions.
- Groupthink: Be wary of the tendency for group members to agree with each other — this might be out of conflict avoidance, path of least resistance, or fear of speaking up. While this agreeableness might make meetings run smoothly, it can actually stunt creativity and idea generation, therefore limiting the success of your chosen solution.
- Irrelevant Information: The tendency to pile on multiple problems and factors that may not even be related to the challenge at hand. This can cloud the team’s ability to find direct, targeted solutions.
- Paradigm Blindness: This is found in people who are unwilling to adapt or change their worldview, outlook on a particular problem, or typical way of processing information. This can erode the effectiveness of problem solving techniques because they are not aware of the narrowness of their thinking, and therefore cannot think or act outside of their comfort zone.
According to Jaffa, the primary barrier of effective problem solving is rigidity. “The most common things people say are, ‘We’ve never done it before,’ or ‘We’ve always done it this way.’” While these feelings are natural, Jaffa explains that this rigid thinking actually precludes teams from identifying creative, inventive solutions that result in the greatest benefit.
“The biggest barrier to creative problem solving is a lack of awareness – and commitment to – training employees in state-of-the-art creative problem-solving techniques,” Mattimore explains. “We teach our clients how to use ideation techniques (as many as two-dozen different creative thinking techniques) to help them generate more and better ideas. Ideation techniques use specific and customized stimuli, or ‘thought triggers’ to inspire new thinking and new ideas.”
MacLeod adds that ineffective or rushed leadership is another common culprit. “We're always in a rush to fix quickly,” she says. “Sometimes leaders just solve problems themselves, making unilateral decisions to save time. But the investment is well worth it — leaders will have less on their plates if they can teach and eventually trust the team to resolve. Teams feel empowered and engagement and investment increases.”
Strategies for Problem Cause Identification
As discussed, most experts agree that the first and most crucial step in problem solving is defining the problem. Once you’ve done this, however, it may not be appropriate to move straight to the solution phase. Rather, it is often helpful to identify the cause(s) of the problem: This will better inform your solution planning and execution, and help ensure that you don’t fall victim to the same challenges in the future.
Below are some of the most common strategies for identifying the cause of a problem:
- Root Cause Analysis: This method helps identify the most critical cause of a problem. A factor is considered a root cause if removing it prevents the problem from recurring. Performing a root cause analysis is a 12 step process that includes: define the problem, gather data on the factors contributing to the problem, group the factors based on shared characteristics, and create a cause-and-effect timeline to determine the root cause. After that, you identify and evaluate corrective actions to eliminate the root cause.
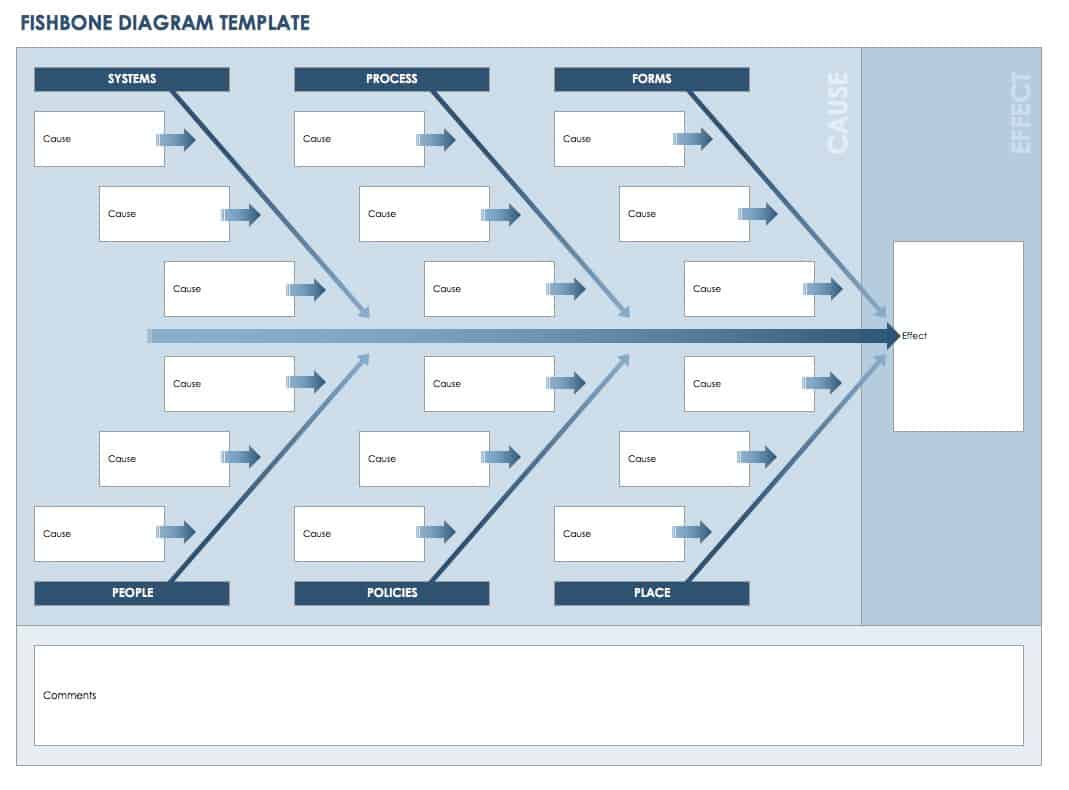
Download Fishbone Diagram Template - Excel
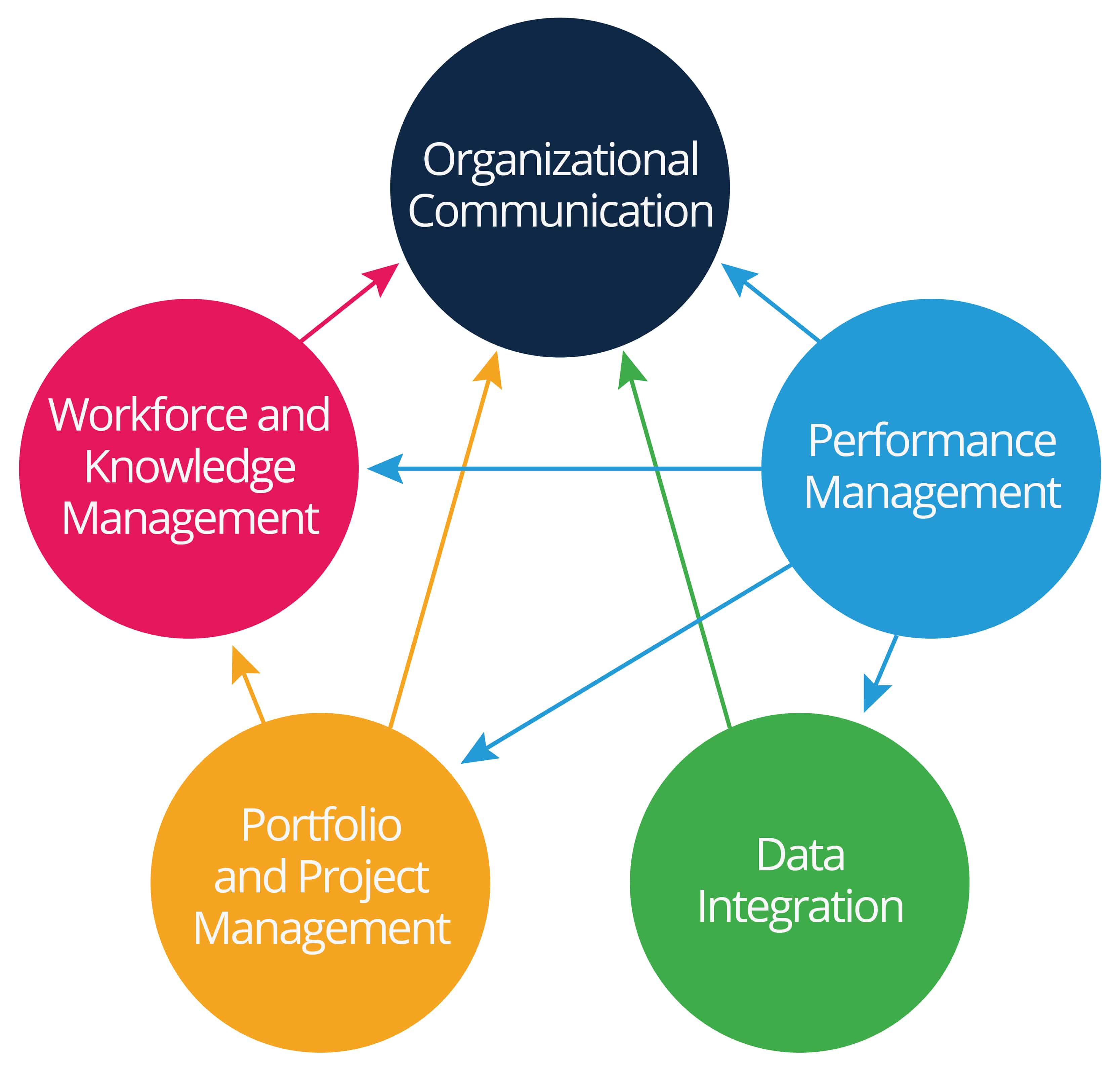
Download 5 Whys Template Excel | Word | PDF
Problem Solving Techniques and Strategies
In this section, we’ll explain several traditional and creative problem solving methods that you can use to identify challenges, create actionable goals, and resolve problems as they arise. Although there is often procedural and objective crossover among techniques, they are grouped by theme so you can identify which method works best for your organization.
Divergent Creative Problem Solving Techniques
Brainstorming: One of the most common methods of divergent thinking, brainstorming works best in an open group setting where everyone is encouraged to share their creative ideas. The goal is to generate as many ideas as possible – you analyze, critique, and evaluate the ideas only after the brainstorming session is complete. To learn more specific brainstorming techniques, read this article .
Mind Mapping: This is a visual thinking tool where you graphically depict concepts and their relation to one another. You can use mind mapping to structure the information you have, analyze and synthesize it, and generate solutions and new ideas from there. The goal of a mind map is to simplify complicated problems so you can more clearly identify solutions.
Appreciative Inquiry (AI): The basic assumption of AI is that “an organization is a mystery to be embraced.” Using this principle, AI takes a positive, inquisitive approach to identifying the problem, analyzing the causes, and presenting possible solutions. The five principles of AI emphasize dialogue, deliberate language and outlook, and social bonding.
Lateral Thinking: This is an indirect problem solving approach centered on the momentum of idea generation. As opposed to critical thinking, where people value ideas based on their truth and the absence of errors, lateral thinking values the “movement value” of new ideas: This means that you reward team members for producing a large volume of new ideas rapidly. With this approach, you’ll generate many new ideas before approving or rejecting any.
Problem Solving Techniques to Change Perspective
Constructive Controversy: This is a structured approach to group decision making to preserve critical thinking and disagreement while maintaining order. After defining the problem and presenting multiple courses of action, the group divides into small advocacy teams who research, analyze, and refute a particular option. Once each advocacy team has presented its best-case scenario, the group has a discussion (advocacy teams still defend their presented idea). Arguing and playing devil’s advocate is encouraged to reach an understanding of the pros and cons of each option. Next, advocacy teams abandon their cause and evaluate the options openly until they reach a consensus. All team members formally commit to the decision, regardless of whether they advocated for it at the beginning. You can learn more about the goals and steps in constructive controversy here .
Carella is a fan of this approach. “Create constructive controversy by having two teams argue the pros and cons of a certain idea,” he says. “It forces unconscious biases to surface and gives space for new ideas to formulate.”
Abstraction: In this method, you apply the problem to a fictional model of the current situation. Mapping an issue to an abstract situation can shed extraneous or irrelevant factors, and reveal places where you are overlooking obvious solutions or becoming bogged down by circumstances.
Analogical Thinking: Also called analogical reasoning , this method relies on an analogy: using information from one problem to solve another problem (these separate problems are called domains). It can be difficult for teams to create analogies among unrelated problems, but it is a strong technique to help you identify repeated issues, zoom out and change perspective, and prevent the problems from occurring in the future. .
CATWOE: This framework ensures that you evaluate the perspectives of those whom your decision will impact. The factors and questions to consider include (which combine to make the acronym CATWOE):
- Customers: Who is on the receiving end of your decisions? What problem do they currently have, and how will they react to your proposed solution?
- Actors: Who is acting to bring your solution to fruition? How will they respond and be affected by your decision?
- Transformation Process: What processes will you employ to transform your current situation and meet your goals? What are the inputs and outputs?
- World View: What is the larger context of your proposed solution? What is the larger, big-picture problem you are addressing?
- Owner: Who actually owns the process? How might they influence your proposed solution (positively or negatively), and how can you influence them to help you?
- Environmental Constraints: What are the limits (environmental, resource- and budget-wise, ethical, legal, etc.) on your ideas? How will you revise or work around these constraints?
Complex Problem Solving
Soft Systems Methodology (SSM): For extremely complex problems, SSM can help you identify how factors interact, and determine the best course of action. SSM was borne out of organizational process modeling and general systems theory, which hold that everything is part of a greater, interconnected system: This idea works well for “hard” problems (where logic and a single correct answer are prioritized), and less so for “soft” problems (i.e., human problems where factors such as personality, emotions, and hierarchy come into play). Therefore, SSM defines a seven step process for problem solving:
- Begin with the problem or problematic situation
- Express the problem or situation and build a rich picture of the themes of the problem
- Identify the root causes of the problem (most commonly with CATWOE)
- Build conceptual models of human activity surrounding the problem or situation
- Compare models with real-world happenings
- Identify changes to the situation that are both feasible and desirable
- Take action to implement changes and improve the problematic situation
SSM can be used for any complex soft problem, and is also a useful tool in change management .
Failure Mode and Effects Analysis (FMEA): This method helps teams anticipate potential problems and take steps to mitigate them. Use FMEA when you are designing (redesigning) a complex function, process, product, or service. First, identify the failure modes, which are the possible ways that a project could fail. Then, perform an effects analysis to understand the consequences of each of the potential downfalls. This exercise is useful for internalizing the severity of each potential failure and its effects so you can make adjustments or safeties in your plan.
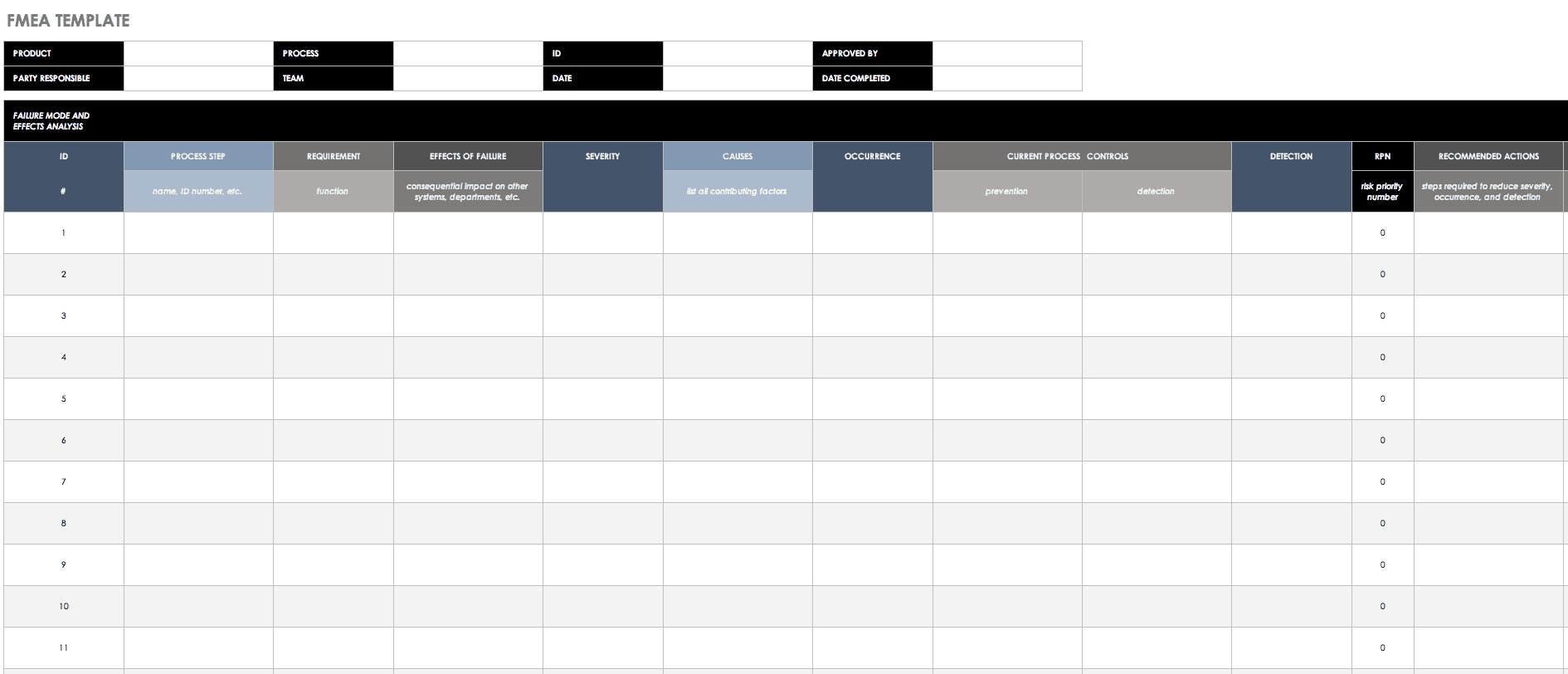
Download FMEA Template
Problem Solving Based on Data or Logic (Heuristic Methods)
TRIZ: A Russian-developed problem solving technique that values logic, analysis, and forecasting over intuition or soft reasoning. TRIZ (translated to “theory of inventive problem solving” or TIPS in English) is a systematic approach to defining and identifying an inventive solution to difficult problems. The method offers several strategies for arriving at an inventive solution, including a contradictions matrix to assess trade-offs among solutions, a Su-Field analysis which uses formulas to describe a system by its structure, and ARIZ (algorithm of inventive problem solving) which uses algorithms to find inventive solutions.
Inductive Reasoning: A logical method that uses evidence to conclude that a certain answer is probable (this is opposed to deductive reasoning, where the answer is assumed to be true). Inductive reasoning uses a limited number of observations to make useful, logical conclusions (for example, the Scientific Method is an extreme example of inductive reasoning). However, this method doesn’t always map well to human problems in the workplace — in these instances, managers should employ intuitive inductive reasoning , which allows for more automatic, implicit conclusions so that work can progress. This, of course, retains the principle that these intuitive conclusions are not necessarily the one and only correct answer.
Process-Oriented Problem Solving Methods
Plan Do Check Act (PDCA): This is an iterative management technique used to ensure continual improvement of products or processes. First, teams plan (establish objectives to meet desired end results), then do (implement the plan, new processes, or produce the output), then check (compare expected with actual results), and finally act (define how the organization will act in the future, based on the performance and knowledge gained in the previous three steps).
Means-End Analysis (MEA): The MEA strategy is to reduce the difference between the current (problematic) state and the goal state. To do so, teams compile information on the multiple factors that contribute to the disparity between the current and goal states. Then they try to change or eliminate the factors one by one, beginning with the factor responsible for the greatest difference in current and goal state. By systematically tackling the multiple factors that cause disparity between the problem and desired outcome, teams can better focus energy and control each step of the process.
Hurson’s Productive Thinking Model: This technique was developed by Tim Hurson, and is detailed in his 2007 book Think Better: An Innovator’s Guide to Productive Thinking . The model outlines six steps that are meant to give structure while maintaining creativity and critical thinking: 1) Ask “What is going on?” 2) Ask “What is success?” 3) Ask “What is the question?” 4) Generate answers 5) Forge the solution 6) Align resources.
Control Influence Accept (CIA): The basic premise of CIA is that how you respond to problems determines how successful you will be in overcoming them. Therefore, this model is both a problem solving technique and stress-management tool that ensures you aren’t responding to problems in a reactive and unproductive way. The steps in CIA include:
- Control: Identify the aspects of the problem that are within your control.
- Influence: Identify the aspects of the problem that you cannot control, but that you can influence.
- Accept: Identify the aspects of the problem that you can neither control nor influence, and react based on this composite information.
GROW Model: This is a straightforward problem solving method for goal setting that clearly defines your goals and current situation, and then asks you to define the potential solutions and be realistic about your chosen course of action. The steps break down as follows:
- Goal: What do you want?
- Reality: Where are you now?
- Options: What could you do?
- Will: What will you do?
OODA Loop: This acronym stands for observe, orient, decide, and act. This approach is a decision-making cycle that values agility and flexibility over raw human force. It is framed as a loop because of the understanding that any team will continually encounter problems or opponents to success and have to overcome them.
There are also many un-named creative problem solving techniques that follow a sequenced series of steps. While the exact steps vary slightly, they all follow a similar trajectory and aim to accomplish similar goals of problem, cause, and goal identification, idea generation, and active solution implementation.
MacLeod offers her own problem solving procedure, which echoes the above steps:
“1. Recognize the Problem: State what you see. Sometimes the problem is covert. 2. Identify: Get the facts — What exactly happened? What is the issue? 3. and 4. Explore and Connect: Dig deeper and encourage group members to relate their similar experiences. Now you're getting more into the feelings and background [of the situation], not just the facts. 5. Possible Solutions: Consider and brainstorm ideas for resolution. 6. Implement: Choose a solution and try it out — this could be role play and/or a discussion of how the solution would be put in place. 7. Evaluate: Revisit to see if the solution was successful or not.”
Many of these problem solving techniques can be used in concert with one another, or multiple can be appropriate for any given problem. It’s less about facilitating a perfect CPS session, and more about encouraging team members to continually think outside the box and push beyond personal boundaries that inhibit their innovative thinking. So, try out several methods, find those that resonate best with your team, and continue adopting new techniques and adapting your processes along the way.
Improve Problem Solving with Work Management in Smartsheet
Empower your people to go above and beyond with a flexible platform designed to match the needs of your team — and adapt as those needs change.
The Smartsheet platform makes it easy to plan, capture, manage, and report on work from anywhere, helping your team be more effective and get more done. Report on key metrics and get real-time visibility into work as it happens with roll-up reports, dashboards, and automated workflows built to keep your team connected and informed.
When teams have clarity into the work getting done, there’s no telling how much more they can accomplish in the same amount of time. Try Smartsheet for free, today.
Discover why over 90% of Fortune 100 companies trust Smartsheet to get work done.
How to master the seven-step problem-solving process
In this episode of the McKinsey Podcast , Simon London speaks with Charles Conn, CEO of venture-capital firm Oxford Sciences Innovation, and McKinsey senior partner Hugo Sarrazin about the complexities of different problem-solving strategies.
Podcast transcript
Simon London: Hello, and welcome to this episode of the McKinsey Podcast , with me, Simon London. What’s the number-one skill you need to succeed professionally? Salesmanship, perhaps? Or a facility with statistics? Or maybe the ability to communicate crisply and clearly? Many would argue that at the very top of the list comes problem solving: that is, the ability to think through and come up with an optimal course of action to address any complex challenge—in business, in public policy, or indeed in life.
Looked at this way, it’s no surprise that McKinsey takes problem solving very seriously, testing for it during the recruiting process and then honing it, in McKinsey consultants, through immersion in a structured seven-step method. To discuss the art of problem solving, I sat down in California with McKinsey senior partner Hugo Sarrazin and also with Charles Conn. Charles is a former McKinsey partner, entrepreneur, executive, and coauthor of the book Bulletproof Problem Solving: The One Skill That Changes Everything [John Wiley & Sons, 2018].
Charles and Hugo, welcome to the podcast. Thank you for being here.
Hugo Sarrazin: Our pleasure.
Charles Conn: It’s terrific to be here.
Simon London: Problem solving is a really interesting piece of terminology. It could mean so many different things. I have a son who’s a teenage climber. They talk about solving problems. Climbing is problem solving. Charles, when you talk about problem solving, what are you talking about?
Charles Conn: For me, problem solving is the answer to the question “What should I do?” It’s interesting when there’s uncertainty and complexity, and when it’s meaningful because there are consequences. Your son’s climbing is a perfect example. There are consequences, and it’s complicated, and there’s uncertainty—can he make that grab? I think we can apply that same frame almost at any level. You can think about questions like “What town would I like to live in?” or “Should I put solar panels on my roof?”
You might think that’s a funny thing to apply problem solving to, but in my mind it’s not fundamentally different from business problem solving, which answers the question “What should my strategy be?” Or problem solving at the policy level: “How do we combat climate change?” “Should I support the local school bond?” I think these are all part and parcel of the same type of question, “What should I do?”
I’m a big fan of structured problem solving. By following steps, we can more clearly understand what problem it is we’re solving, what are the components of the problem that we’re solving, which components are the most important ones for us to pay attention to, which analytic techniques we should apply to those, and how we can synthesize what we’ve learned back into a compelling story. That’s all it is, at its heart.
I think sometimes when people think about seven steps, they assume that there’s a rigidity to this. That’s not it at all. It’s actually to give you the scope for creativity, which often doesn’t exist when your problem solving is muddled.
Simon London: You were just talking about the seven-step process. That’s what’s written down in the book, but it’s a very McKinsey process as well. Without getting too deep into the weeds, let’s go through the steps, one by one. You were just talking about problem definition as being a particularly important thing to get right first. That’s the first step. Hugo, tell us about that.
Hugo Sarrazin: It is surprising how often people jump past this step and make a bunch of assumptions. The most powerful thing is to step back and ask the basic questions—“What are we trying to solve? What are the constraints that exist? What are the dependencies?” Let’s make those explicit and really push the thinking and defining. At McKinsey, we spend an enormous amount of time in writing that little statement, and the statement, if you’re a logic purist, is great. You debate. “Is it an ‘or’? Is it an ‘and’? What’s the action verb?” Because all these specific words help you get to the heart of what matters.
Want to subscribe to The McKinsey Podcast ?
Simon London: So this is a concise problem statement.
Hugo Sarrazin: Yeah. It’s not like “Can we grow in Japan?” That’s interesting, but it is “What, specifically, are we trying to uncover in the growth of a product in Japan? Or a segment in Japan? Or a channel in Japan?” When you spend an enormous amount of time, in the first meeting of the different stakeholders, debating this and having different people put forward what they think the problem definition is, you realize that people have completely different views of why they’re here. That, to me, is the most important step.
Charles Conn: I would agree with that. For me, the problem context is critical. When we understand “What are the forces acting upon your decision maker? How quickly is the answer needed? With what precision is the answer needed? Are there areas that are off limits or areas where we would particularly like to find our solution? Is the decision maker open to exploring other areas?” then you not only become more efficient, and move toward what we call the critical path in problem solving, but you also make it so much more likely that you’re not going to waste your time or your decision maker’s time.
How often do especially bright young people run off with half of the idea about what the problem is and start collecting data and start building models—only to discover that they’ve really gone off half-cocked.
Hugo Sarrazin: Yeah.
Charles Conn: And in the wrong direction.
Simon London: OK. So step one—and there is a real art and a structure to it—is define the problem. Step two, Charles?
Charles Conn: My favorite step is step two, which is to use logic trees to disaggregate the problem. Every problem we’re solving has some complexity and some uncertainty in it. The only way that we can really get our team working on the problem is to take the problem apart into logical pieces.
What we find, of course, is that the way to disaggregate the problem often gives you an insight into the answer to the problem quite quickly. I love to do two or three different cuts at it, each one giving a bit of a different insight into what might be going wrong. By doing sensible disaggregations, using logic trees, we can figure out which parts of the problem we should be looking at, and we can assign those different parts to team members.
Simon London: What’s a good example of a logic tree on a sort of ratable problem?
Charles Conn: Maybe the easiest one is the classic profit tree. Almost in every business that I would take a look at, I would start with a profit or return-on-assets tree. In its simplest form, you have the components of revenue, which are price and quantity, and the components of cost, which are cost and quantity. Each of those can be broken out. Cost can be broken into variable cost and fixed cost. The components of price can be broken into what your pricing scheme is. That simple tree often provides insight into what’s going on in a business or what the difference is between that business and the competitors.
If we add the leg, which is “What’s the asset base or investment element?”—so profit divided by assets—then we can ask the question “Is the business using its investments sensibly?” whether that’s in stores or in manufacturing or in transportation assets. I hope we can see just how simple this is, even though we’re describing it in words.
When I went to work with Gordon Moore at the Moore Foundation, the problem that he asked us to look at was “How can we save Pacific salmon?” Now, that sounds like an impossible question, but it was amenable to precisely the same type of disaggregation and allowed us to organize what became a 15-year effort to improve the likelihood of good outcomes for Pacific salmon.
Simon London: Now, is there a danger that your logic tree can be impossibly large? This, I think, brings us onto the third step in the process, which is that you have to prioritize.
Charles Conn: Absolutely. The third step, which we also emphasize, along with good problem definition, is rigorous prioritization—we ask the questions “How important is this lever or this branch of the tree in the overall outcome that we seek to achieve? How much can I move that lever?” Obviously, we try and focus our efforts on ones that have a big impact on the problem and the ones that we have the ability to change. With salmon, ocean conditions turned out to be a big lever, but not one that we could adjust. We focused our attention on fish habitats and fish-harvesting practices, which were big levers that we could affect.
People spend a lot of time arguing about branches that are either not important or that none of us can change. We see it in the public square. When we deal with questions at the policy level—“Should you support the death penalty?” “How do we affect climate change?” “How can we uncover the causes and address homelessness?”—it’s even more important that we’re focusing on levers that are big and movable.
Would you like to learn more about our Strategy & Corporate Finance Practice ?
Simon London: Let’s move swiftly on to step four. You’ve defined your problem, you disaggregate it, you prioritize where you want to analyze—what you want to really look at hard. Then you got to the work plan. Now, what does that mean in practice?
Hugo Sarrazin: Depending on what you’ve prioritized, there are many things you could do. It could be breaking the work among the team members so that people have a clear piece of the work to do. It could be defining the specific analyses that need to get done and executed, and being clear on time lines. There’s always a level-one answer, there’s a level-two answer, there’s a level-three answer. Without being too flippant, I can solve any problem during a good dinner with wine. It won’t have a whole lot of backing.
Simon London: Not going to have a lot of depth to it.
Hugo Sarrazin: No, but it may be useful as a starting point. If the stakes are not that high, that could be OK. If it’s really high stakes, you may need level three and have the whole model validated in three different ways. You need to find a work plan that reflects the level of precision, the time frame you have, and the stakeholders you need to bring along in the exercise.
Charles Conn: I love the way you’ve described that, because, again, some people think of problem solving as a linear thing, but of course what’s critical is that it’s iterative. As you say, you can solve the problem in one day or even one hour.
Charles Conn: We encourage our teams everywhere to do that. We call it the one-day answer or the one-hour answer. In work planning, we’re always iterating. Every time you see a 50-page work plan that stretches out to three months, you know it’s wrong. It will be outmoded very quickly by that learning process that you described. Iterative problem solving is a critical part of this. Sometimes, people think work planning sounds dull, but it isn’t. It’s how we know what’s expected of us and when we need to deliver it and how we’re progressing toward the answer. It’s also the place where we can deal with biases. Bias is a feature of every human decision-making process. If we design our team interactions intelligently, we can avoid the worst sort of biases.
Simon London: Here we’re talking about cognitive biases primarily, right? It’s not that I’m biased against you because of your accent or something. These are the cognitive biases that behavioral sciences have shown we all carry around, things like anchoring, overoptimism—these kinds of things.
Both: Yeah.
Charles Conn: Availability bias is the one that I’m always alert to. You think you’ve seen the problem before, and therefore what’s available is your previous conception of it—and we have to be most careful about that. In any human setting, we also have to be careful about biases that are based on hierarchies, sometimes called sunflower bias. I’m sure, Hugo, with your teams, you make sure that the youngest team members speak first. Not the oldest team members, because it’s easy for people to look at who’s senior and alter their own creative approaches.
Hugo Sarrazin: It’s helpful, at that moment—if someone is asserting a point of view—to ask the question “This was true in what context?” You’re trying to apply something that worked in one context to a different one. That can be deadly if the context has changed, and that’s why organizations struggle to change. You promote all these people because they did something that worked well in the past, and then there’s a disruption in the industry, and they keep doing what got them promoted even though the context has changed.
Simon London: Right. Right.
Hugo Sarrazin: So it’s the same thing in problem solving.
Charles Conn: And it’s why diversity in our teams is so important. It’s one of the best things about the world that we’re in now. We’re likely to have people from different socioeconomic, ethnic, and national backgrounds, each of whom sees problems from a slightly different perspective. It is therefore much more likely that the team will uncover a truly creative and clever approach to problem solving.
Simon London: Let’s move on to step five. You’ve done your work plan. Now you’ve actually got to do the analysis. The thing that strikes me here is that the range of tools that we have at our disposal now, of course, is just huge, particularly with advances in computation, advanced analytics. There’s so many things that you can apply here. Just talk about the analysis stage. How do you pick the right tools?
Charles Conn: For me, the most important thing is that we start with simple heuristics and explanatory statistics before we go off and use the big-gun tools. We need to understand the shape and scope of our problem before we start applying these massive and complex analytical approaches.
Simon London: Would you agree with that?
Hugo Sarrazin: I agree. I think there are so many wonderful heuristics. You need to start there before you go deep into the modeling exercise. There’s an interesting dynamic that’s happening, though. In some cases, for some types of problems, it is even better to set yourself up to maximize your learning. Your problem-solving methodology is test and learn, test and learn, test and learn, and iterate. That is a heuristic in itself, the A/B testing that is used in many parts of the world. So that’s a problem-solving methodology. It’s nothing different. It just uses technology and feedback loops in a fast way. The other one is exploratory data analysis. When you’re dealing with a large-scale problem, and there’s so much data, I can get to the heuristics that Charles was talking about through very clever visualization of data.
You test with your data. You need to set up an environment to do so, but don’t get caught up in neural-network modeling immediately. You’re testing, you’re checking—“Is the data right? Is it sound? Does it make sense?”—before you launch too far.
Simon London: You do hear these ideas—that if you have a big enough data set and enough algorithms, they’re going to find things that you just wouldn’t have spotted, find solutions that maybe you wouldn’t have thought of. Does machine learning sort of revolutionize the problem-solving process? Or are these actually just other tools in the toolbox for structured problem solving?
Charles Conn: It can be revolutionary. There are some areas in which the pattern recognition of large data sets and good algorithms can help us see things that we otherwise couldn’t see. But I do think it’s terribly important we don’t think that this particular technique is a substitute for superb problem solving, starting with good problem definition. Many people use machine learning without understanding algorithms that themselves can have biases built into them. Just as 20 years ago, when we were doing statistical analysis, we knew that we needed good model definition, we still need a good understanding of our algorithms and really good problem definition before we launch off into big data sets and unknown algorithms.
Simon London: Step six. You’ve done your analysis.
Charles Conn: I take six and seven together, and this is the place where young problem solvers often make a mistake. They’ve got their analysis, and they assume that’s the answer, and of course it isn’t the answer. The ability to synthesize the pieces that came out of the analysis and begin to weave those into a story that helps people answer the question “What should I do?” This is back to where we started. If we can’t synthesize, and we can’t tell a story, then our decision maker can’t find the answer to “What should I do?”
Simon London: But, again, these final steps are about motivating people to action, right?
Charles Conn: Yeah.
Simon London: I am slightly torn about the nomenclature of problem solving because it’s on paper, right? Until you motivate people to action, you actually haven’t solved anything.
Charles Conn: I love this question because I think decision-making theory, without a bias to action, is a waste of time. Everything in how I approach this is to help people take action that makes the world better.
Simon London: Hence, these are absolutely critical steps. If you don’t do this well, you’ve just got a bunch of analysis.
Charles Conn: We end up in exactly the same place where we started, which is people speaking across each other, past each other in the public square, rather than actually working together, shoulder to shoulder, to crack these important problems.
Simon London: In the real world, we have a lot of uncertainty—arguably, increasing uncertainty. How do good problem solvers deal with that?
Hugo Sarrazin: At every step of the process. In the problem definition, when you’re defining the context, you need to understand those sources of uncertainty and whether they’re important or not important. It becomes important in the definition of the tree.
You need to think carefully about the branches of the tree that are more certain and less certain as you define them. They don’t have equal weight just because they’ve got equal space on the page. Then, when you’re prioritizing, your prioritization approach may put more emphasis on things that have low probability but huge impact—or, vice versa, may put a lot of priority on things that are very likely and, hopefully, have a reasonable impact. You can introduce that along the way. When you come back to the synthesis, you just need to be nuanced about what you’re understanding, the likelihood.
Often, people lack humility in the way they make their recommendations: “This is the answer.” They’re very precise, and I think we would all be well-served to say, “This is a likely answer under the following sets of conditions” and then make the level of uncertainty clearer, if that is appropriate. It doesn’t mean you’re always in the gray zone; it doesn’t mean you don’t have a point of view. It just means that you can be explicit about the certainty of your answer when you make that recommendation.
Simon London: So it sounds like there is an underlying principle: “Acknowledge and embrace the uncertainty. Don’t pretend that it isn’t there. Be very clear about what the uncertainties are up front, and then build that into every step of the process.”
Hugo Sarrazin: Every step of the process.
Simon London: Yeah. We have just walked through a particular structured methodology for problem solving. But, of course, this is not the only structured methodology for problem solving. One that is also very well-known is design thinking, which comes at things very differently. So, Hugo, I know you have worked with a lot of designers. Just give us a very quick summary. Design thinking—what is it, and how does it relate?
Hugo Sarrazin: It starts with an incredible amount of empathy for the user and uses that to define the problem. It does pause and go out in the wild and spend an enormous amount of time seeing how people interact with objects, seeing the experience they’re getting, seeing the pain points or joy—and uses that to infer and define the problem.
Simon London: Problem definition, but out in the world.
Hugo Sarrazin: With an enormous amount of empathy. There’s a huge emphasis on empathy. Traditional, more classic problem solving is you define the problem based on an understanding of the situation. This one almost presupposes that we don’t know the problem until we go see it. The second thing is you need to come up with multiple scenarios or answers or ideas or concepts, and there’s a lot of divergent thinking initially. That’s slightly different, versus the prioritization, but not for long. Eventually, you need to kind of say, “OK, I’m going to converge again.” Then you go and you bring things back to the customer and get feedback and iterate. Then you rinse and repeat, rinse and repeat. There’s a lot of tactile building, along the way, of prototypes and things like that. It’s very iterative.
Simon London: So, Charles, are these complements or are these alternatives?
Charles Conn: I think they’re entirely complementary, and I think Hugo’s description is perfect. When we do problem definition well in classic problem solving, we are demonstrating the kind of empathy, at the very beginning of our problem, that design thinking asks us to approach. When we ideate—and that’s very similar to the disaggregation, prioritization, and work-planning steps—we do precisely the same thing, and often we use contrasting teams, so that we do have divergent thinking. The best teams allow divergent thinking to bump them off whatever their initial biases in problem solving are. For me, design thinking gives us a constant reminder of creativity, empathy, and the tactile nature of problem solving, but it’s absolutely complementary, not alternative.
Simon London: I think, in a world of cross-functional teams, an interesting question is do people with design-thinking backgrounds really work well together with classical problem solvers? How do you make that chemistry happen?
Hugo Sarrazin: Yeah, it is not easy when people have spent an enormous amount of time seeped in design thinking or user-centric design, whichever word you want to use. If the person who’s applying classic problem-solving methodology is very rigid and mechanical in the way they’re doing it, there could be an enormous amount of tension. If there’s not clarity in the role and not clarity in the process, I think having the two together can be, sometimes, problematic.
The second thing that happens often is that the artifacts the two methodologies try to gravitate toward can be different. Classic problem solving often gravitates toward a model; design thinking migrates toward a prototype. Rather than writing a big deck with all my supporting evidence, they’ll bring an example, a thing, and that feels different. Then you spend your time differently to achieve those two end products, so that’s another source of friction.
Now, I still think it can be an incredibly powerful thing to have the two—if there are the right people with the right mind-set, if there is a team that is explicit about the roles, if we’re clear about the kind of outcomes we are attempting to bring forward. There’s an enormous amount of collaborativeness and respect.
Simon London: But they have to respect each other’s methodology and be prepared to flex, maybe, a little bit, in how this process is going to work.
Hugo Sarrazin: Absolutely.
Simon London: The other area where, it strikes me, there could be a little bit of a different sort of friction is this whole concept of the day-one answer, which is what we were just talking about in classical problem solving. Now, you know that this is probably not going to be your final answer, but that’s how you begin to structure the problem. Whereas I would imagine your design thinkers—no, they’re going off to do their ethnographic research and get out into the field, potentially for a long time, before they come back with at least an initial hypothesis.

Want better strategies? Become a bulletproof problem solver
Hugo Sarrazin: That is a great callout, and that’s another difference. Designers typically will like to soak into the situation and avoid converging too quickly. There’s optionality and exploring different options. There’s a strong belief that keeps the solution space wide enough that you can come up with more radical ideas. If there’s a large design team or many designers on the team, and you come on Friday and say, “What’s our week-one answer?” they’re going to struggle. They’re not going to be comfortable, naturally, to give that answer. It doesn’t mean they don’t have an answer; it’s just not where they are in their thinking process.
Simon London: I think we are, sadly, out of time for today. But Charles and Hugo, thank you so much.
Charles Conn: It was a pleasure to be here, Simon.
Hugo Sarrazin: It was a pleasure. Thank you.
Simon London: And thanks, as always, to you, our listeners, for tuning into this episode of the McKinsey Podcast . If you want to learn more about problem solving, you can find the book, Bulletproof Problem Solving: The One Skill That Changes Everything , online or order it through your local bookstore. To learn more about McKinsey, you can of course find us at McKinsey.com.
Charles Conn is CEO of Oxford Sciences Innovation and an alumnus of McKinsey’s Sydney office. Hugo Sarrazin is a senior partner in the Silicon Valley office, where Simon London, a member of McKinsey Publishing, is also based.
Explore a career with us
Related articles.
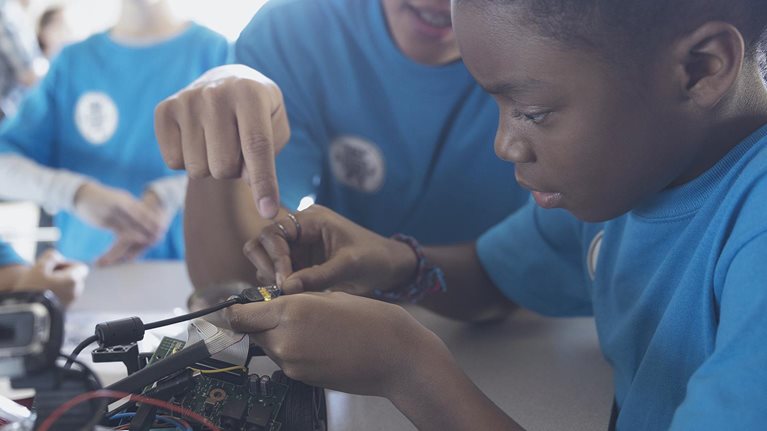
Strategy to beat the odds
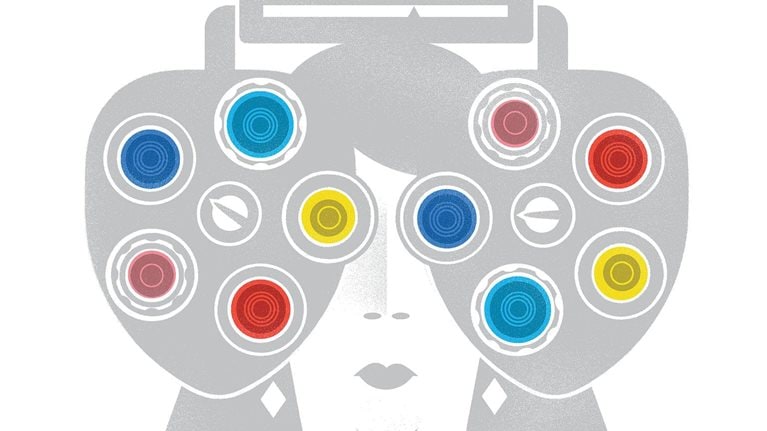
Five routes to more innovative problem solving

Want to create or adapt books like this? Learn more about how Pressbooks supports open publishing practices.
1.5 Planning, Organizing, Leading, and Controlling
Learning objectives.
- Know the dimensions of the planning-organizing-leading-controlling (P-O-L-C) framework.
- Know the general inputs into each P-O-L-C dimension.
A manager’s primary challenge is to solve problems creatively. While drawing from a variety of academic disciplines, and to help managers respond to the challenge of creative problem solving, principles of management have long been categorized into the four major functions of planning, organizing, leading, and controlling (the P-O-L-C framework). The four functions, summarized in the P-O-L-C figure, are actually highly integrated when carried out in the day-to-day realities of running an organization. Therefore, you should not get caught up in trying to analyze and understand a complete, clear rationale for categorizing skills and practices that compose the whole of the P-O-L-C framework.
It is important to note that this framework is not without criticism. Specifically, these criticisms stem from the observation that the P-O-L-C functions might be ideal but that they do not accurately depict the day-to-day actions of actual managers (Mintzberg, 1973; Lamond, 2004). The typical day in the life of a manager at any level can be fragmented and hectic, with the constant threat of having priorities dictated by the law of the trivial many and important few (i.e., the 80/20 rule). However, the general conclusion seems to be that the P-O-L-C functions of management still provide a very useful way of classifying the activities managers engage in as they attempt to achieve organizational goals (Lamond, 2004).
Figure 1.7 The P-O-L-C Framework
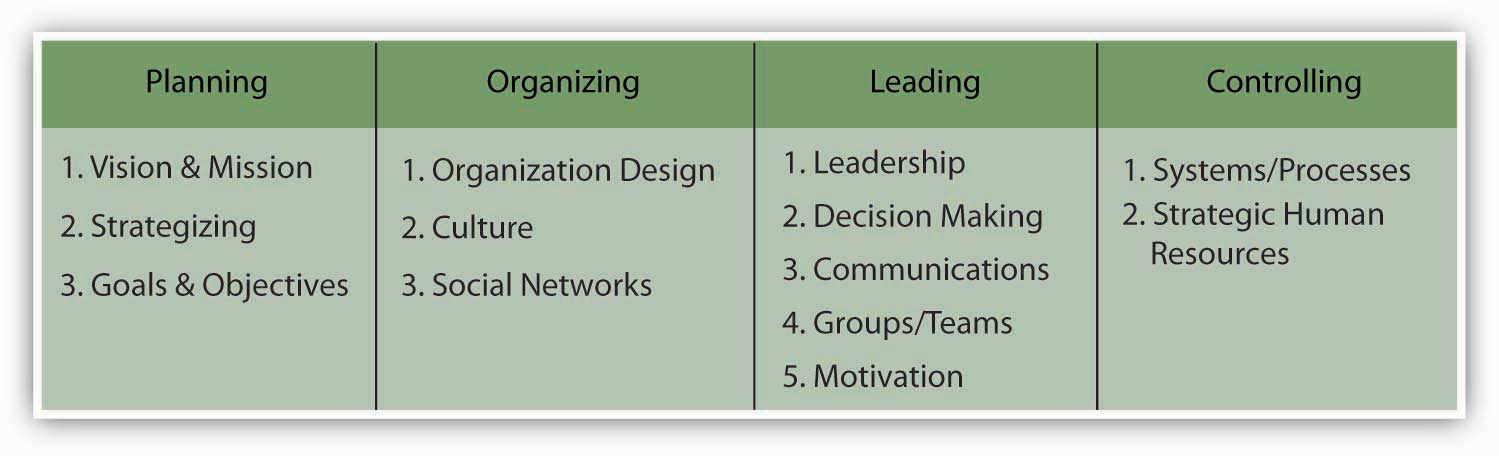
Planning is the function of management that involves setting objectives and determining a course of action for achieving those objectives. Planning requires that managers be aware of environmental conditions facing their organization and forecast future conditions. It also requires that managers be good decision makers.
Planning is a process consisting of several steps. The process begins with environmental scanning which simply means that planners must be aware of the critical contingencies facing their organization in terms of economic conditions, their competitors, and their customers. Planners must then attempt to forecast future conditions. These forecasts form the basis for planning.
Planners must establish objectives, which are statements of what needs to be achieved and when. Planners must then identify alternative courses of action for achieving objectives. After evaluating the various alternatives, planners must make decisions about the best courses of action for achieving objectives. They must then formulate necessary steps and ensure effective implementation of plans. Finally, planners must constantly evaluate the success of their plans and take corrective action when necessary.
There are many different types of plans and planning.
Strategic planning involves analyzing competitive opportunities and threats, as well as the strengths and weaknesses of the organization, and then determining how to position the organization to compete effectively in their environment. Strategic planning has a long time frame, often three years or more. Strategic planning generally includes the entire organization and includes formulation of objectives. Strategic planning is often based on the organization’s mission, which is its fundamental reason for existence. An organization’s top management most often conducts strategic planning.
Tactical planning is intermediate-range (one to three years) planning that is designed to develop relatively concrete and specific means to implement the strategic plan. Middle-level managers often engage in tactical planning.
Operational planning generally assumes the existence of organization-wide or subunit goals and objectives and specifies ways to achieve them. Operational planning is short-range (less than a year) planning that is designed to develop specific action steps that support the strategic and tactical plans.
Organizing is the function of management that involves developing an organizational structure and allocating human resources to ensure the accomplishment of objectives. The structure of the organization is the framework within which effort is coordinated. The structure is usually represented by an organization chart, which provides a graphic representation of the chain of command within an organization. Decisions made about the structure of an organization are generally referred to as organizational design decisions.
Organizing also involves the design of individual jobs within the organization. Decisions must be made about the duties and responsibilities of individual jobs, as well as the manner in which the duties should be carried out. Decisions made about the nature of jobs within the organization are generally called “job design” decisions.
Organizing at the level of the organization involves deciding how best to departmentalize, or cluster, jobs into departments to coordinate effort effectively. There are many different ways to departmentalize, including organizing by function, product, geography, or customer. Many larger organizations use multiple methods of departmentalization.
Organizing at the level of a particular job involves how best to design individual jobs to most effectively use human resources. Traditionally, job design was based on principles of division of labor and specialization, which assumed that the more narrow the job content, the more proficient the individual performing the job could become. However, experience has shown that it is possible for jobs to become too narrow and specialized. For example, how would you like to screw lids on jars one day after another, as you might have done many decades ago if you worked in company that made and sold jellies and jams? When this happens, negative outcomes result, including decreased job satisfaction and organizational commitment, increased absenteeism, and turnover.
Recently, many organizations have attempted to strike a balance between the need for worker specialization and the need for workers to have jobs that entail variety and autonomy. Many jobs are now designed based on such principles as empowerment, job enrichment and teamwork . For example, HUI Manufacturing, a custom sheet metal fabricator, has done away with traditional “departments” to focus on listening and responding to customer needs. From company-wide meetings to team huddles, HUI employees know and understand their customers and how HUI might service them best (Huimfg, 2008).
Leading involves the social and informal sources of influence that you use to inspire action taken by others. If managers are effective leaders, their subordinates will be enthusiastic about exerting effort to attain organizational objectives.
The behavioral sciences have made many contributions to understanding this function of management. Personality research and studies of job attitudes provide important information as to how managers can most effectively lead subordinates. For example, this research tells us that to become effective at leading, managers must first understand their subordinates’ personalities, values, attitudes, and emotions.
Studies of motivation and motivation theory provide important information about the ways in which workers can be energized to put forth productive effort. Studies of communication provide direction as to how managers can effectively and persuasively communicate. Studies of leadership and leadership style provide information regarding questions, such as, “What makes a manager a good leader?” and “In what situations are certain leadership styles most appropriate and effective?”
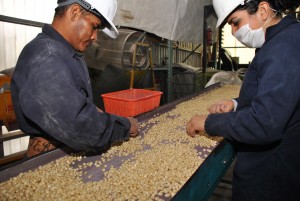
Quality control ensures that the organization delivers on its promises.
International Maize and Wheat Improvement Center – Maize seed quality control at small seed company Bidasem – CC BY-NC-SA 2.0.
Controlling
Controlling involves ensuring that performance does not deviate from standards. Controlling consists of three steps, which include (1) establishing performance standards, (2) comparing actual performance against standards, and (3) taking corrective action when necessary. Performance standards are often stated in monetary terms such as revenue, costs, or profits but may also be stated in other terms, such as units produced, number of defective products, or levels of quality or customer service.
The measurement of performance can be done in several ways, depending on the performance standards, including financial statements, sales reports, production results, customer satisfaction, and formal performance appraisals. Managers at all levels engage in the managerial function of controlling to some degree.
The managerial function of controlling should not be confused with control in the behavioral or manipulative sense. This function does not imply that managers should attempt to control or to manipulate the personalities, values, attitudes, or emotions of their subordinates. Instead, this function of management concerns the manager’s role in taking necessary actions to ensure that the work-related activities of subordinates are consistent with and contributing toward the accomplishment of organizational and departmental objectives.
Effective controlling requires the existence of plans, since planning provides the necessary performance standards or objectives. Controlling also requires a clear understanding of where responsibility for deviations from standards lies. Two traditional control techniques are budget and performance audits. An audit involves an examination and verification of records and supporting documents. A budget audit provides information about where the organization is with respect to what was planned or budgeted for, whereas a performance audit might try to determine whether the figures reported are a reflection of actual performance. Although controlling is often thought of in terms of financial criteria, managers must also control production and operations processes, procedures for delivery of services, compliance with company policies, and many other activities within the organization.
The management functions of planning, organizing, leading, and controlling are widely considered to be the best means of describing the manager’s job, as well as the best way to classify accumulated knowledge about the study of management. Although there have been tremendous changes in the environment faced by managers and the tools used by managers to perform their roles, managers still perform these essential functions.
Key Takeaway
The principles of management can be distilled down to four critical functions. These functions are planning, organizing, leading, and controlling. This P-O-L-C framework provides useful guidance into what the ideal job of a manager should look like.
- What are the management functions that comprise the P-O-L-C framework?
- Are there any criticisms of this framework?
- What function does planning serve?
- What function does organizing serve?
- What function does leading serve?
- What function does controlling serve?
Huimfg.com, http://www.huimfg.com/abouthui-yourteams.aspx (accessed October 15, 2008).
Lamond, D, “A Matter of Style: Reconciling Henri and Henry,” Management Decision 42, no. 2 (2004): 330–56.
Mintzberg, H. The Nature of Managerial Work (New York: Harper & Row, 1973); D. Lamond, “A Matter of Style: Reconciling Henri and Henry,” Management Decision 42 , no. 2 (2004): 330–56.
Principles of Management Copyright © 2015 by University of Minnesota is licensed under a Creative Commons Attribution-NonCommercial-ShareAlike 4.0 International License , except where otherwise noted.
35 problem-solving techniques and methods for solving complex problems
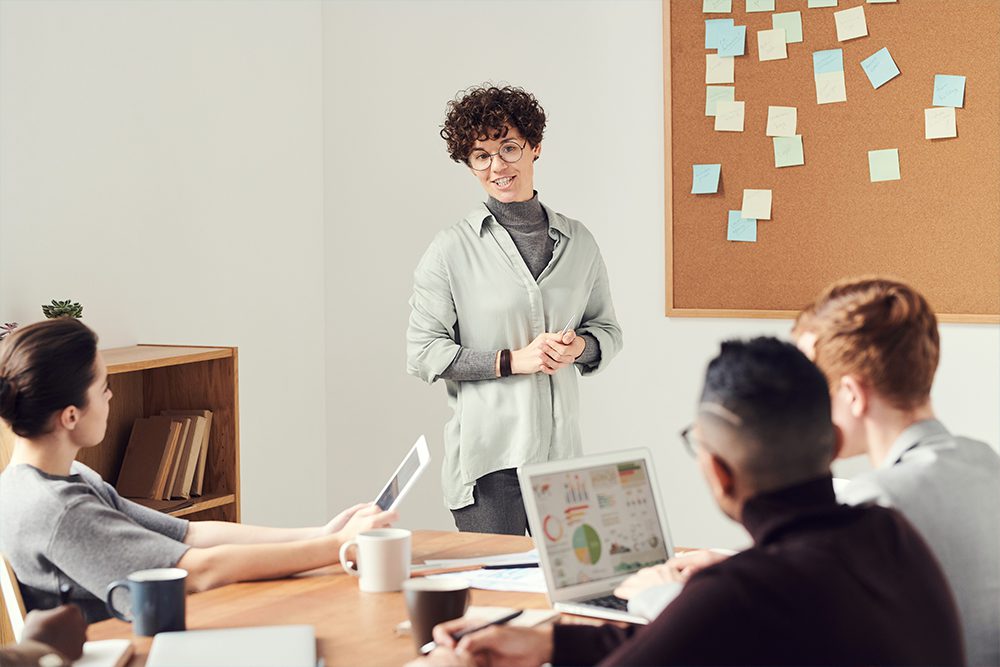
Design your next session with SessionLab
Join the 150,000+ facilitators using SessionLab.
Recommended Articles
A step-by-step guide to planning a workshop, how to create an unforgettable training session in 8 simple steps, 47 useful online tools for workshop planning and meeting facilitation.
All teams and organizations encounter challenges as they grow. There are problems that might occur for teams when it comes to miscommunication or resolving business-critical issues . You may face challenges around growth , design , user engagement, and even team culture and happiness. In short, problem-solving techniques should be part of every team’s skillset.
Problem-solving methods are primarily designed to help a group or team through a process of first identifying problems and challenges , ideating possible solutions , and then evaluating the most suitable .
Finding effective solutions to complex problems isn’t easy, but by using the right process and techniques, you can help your team be more efficient in the process.
So how do you develop strategies that are engaging, and empower your team to solve problems effectively?
In this blog post, we share a series of problem-solving tools you can use in your next workshop or team meeting. You’ll also find some tips for facilitating the process and how to enable others to solve complex problems.
Let’s get started!
How do you identify problems?
How do you identify the right solution.
- Tips for more effective problem-solving
Complete problem-solving methods
- Problem-solving techniques to identify and analyze problems
- Problem-solving techniques for developing solutions
Problem-solving warm-up activities
Closing activities for a problem-solving process.
Before you can move towards finding the right solution for a given problem, you first need to identify and define the problem you wish to solve.
Here, you want to clearly articulate what the problem is and allow your group to do the same. Remember that everyone in a group is likely to have differing perspectives and alignment is necessary in order to help the group move forward.
Identifying a problem accurately also requires that all members of a group are able to contribute their views in an open and safe manner. It can be scary for people to stand up and contribute, especially if the problems or challenges are emotive or personal in nature. Be sure to try and create a psychologically safe space for these kinds of discussions.
Remember that problem analysis and further discussion are also important. Not taking the time to fully analyze and discuss a challenge can result in the development of solutions that are not fit for purpose or do not address the underlying issue.
Successfully identifying and then analyzing a problem means facilitating a group through activities designed to help them clearly and honestly articulate their thoughts and produce usable insight.
With this data, you might then produce a problem statement that clearly describes the problem you wish to be addressed and also state the goal of any process you undertake to tackle this issue.
Finding solutions is the end goal of any process. Complex organizational challenges can only be solved with an appropriate solution but discovering them requires using the right problem-solving tool.
After you’ve explored a problem and discussed ideas, you need to help a team discuss and choose the right solution. Consensus tools and methods such as those below help a group explore possible solutions before then voting for the best. They’re a great way to tap into the collective intelligence of the group for great results!
Remember that the process is often iterative. Great problem solvers often roadtest a viable solution in a measured way to see what works too. While you might not get the right solution on your first try, the methods below help teams land on the most likely to succeed solution while also holding space for improvement.
Every effective problem solving process begins with an agenda . A well-structured workshop is one of the best methods for successfully guiding a group from exploring a problem to implementing a solution.
In SessionLab, it’s easy to go from an idea to a complete agenda . Start by dragging and dropping your core problem solving activities into place . Add timings, breaks and necessary materials before sharing your agenda with your colleagues.
The resulting agenda will be your guide to an effective and productive problem solving session that will also help you stay organized on the day!
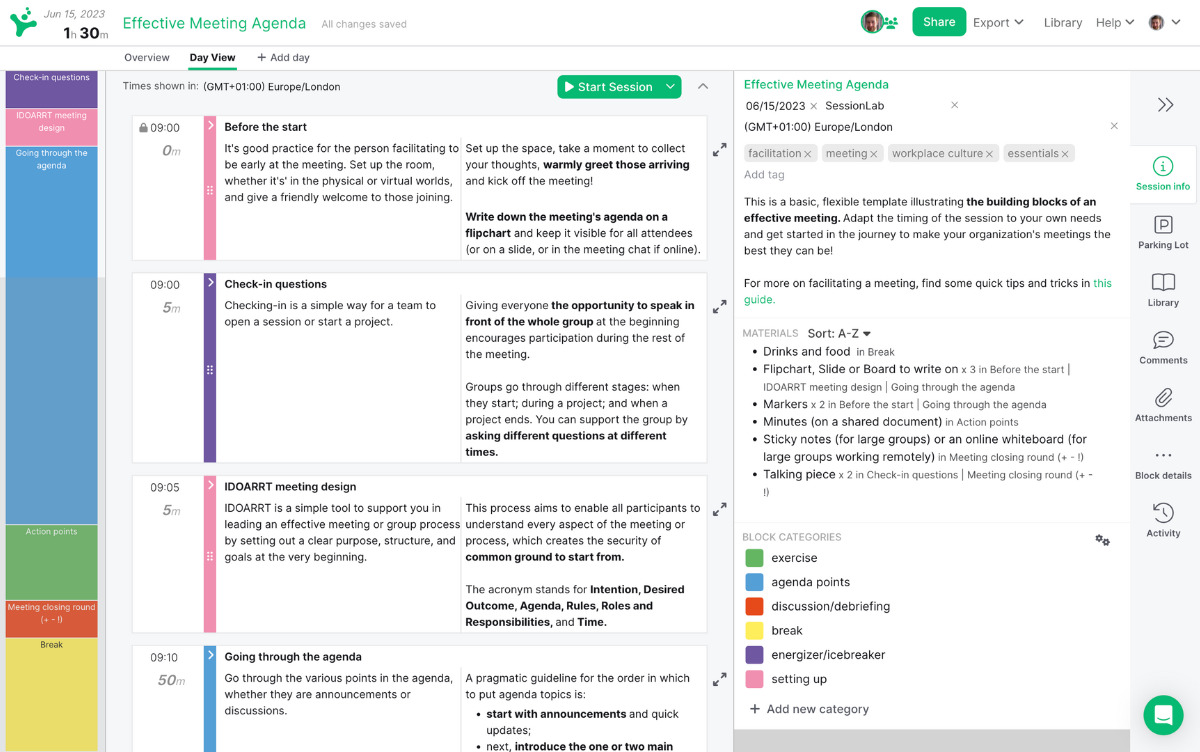
Tips for more effective problem solving
Problem-solving activities are only one part of the puzzle. While a great method can help unlock your team’s ability to solve problems, without a thoughtful approach and strong facilitation the solutions may not be fit for purpose.
Let’s take a look at some problem-solving tips you can apply to any process to help it be a success!
Clearly define the problem
Jumping straight to solutions can be tempting, though without first clearly articulating a problem, the solution might not be the right one. Many of the problem-solving activities below include sections where the problem is explored and clearly defined before moving on.
This is a vital part of the problem-solving process and taking the time to fully define an issue can save time and effort later. A clear definition helps identify irrelevant information and it also ensures that your team sets off on the right track.
Don’t jump to conclusions
It’s easy for groups to exhibit cognitive bias or have preconceived ideas about both problems and potential solutions. Be sure to back up any problem statements or potential solutions with facts, research, and adequate forethought.
The best techniques ask participants to be methodical and challenge preconceived notions. Make sure you give the group enough time and space to collect relevant information and consider the problem in a new way. By approaching the process with a clear, rational mindset, you’ll often find that better solutions are more forthcoming.
Try different approaches
Problems come in all shapes and sizes and so too should the methods you use to solve them. If you find that one approach isn’t yielding results and your team isn’t finding different solutions, try mixing it up. You’ll be surprised at how using a new creative activity can unblock your team and generate great solutions.
Don’t take it personally
Depending on the nature of your team or organizational problems, it’s easy for conversations to get heated. While it’s good for participants to be engaged in the discussions, ensure that emotions don’t run too high and that blame isn’t thrown around while finding solutions.
You’re all in it together, and even if your team or area is seeing problems, that isn’t necessarily a disparagement of you personally. Using facilitation skills to manage group dynamics is one effective method of helping conversations be more constructive.
Get the right people in the room
Your problem-solving method is often only as effective as the group using it. Getting the right people on the job and managing the number of people present is important too!
If the group is too small, you may not get enough different perspectives to effectively solve a problem. If the group is too large, you can go round and round during the ideation stages.
Creating the right group makeup is also important in ensuring you have the necessary expertise and skillset to both identify and follow up on potential solutions. Carefully consider who to include at each stage to help ensure your problem-solving method is followed and positioned for success.
Document everything
The best solutions can take refinement, iteration, and reflection to come out. Get into a habit of documenting your process in order to keep all the learnings from the session and to allow ideas to mature and develop. Many of the methods below involve the creation of documents or shared resources. Be sure to keep and share these so everyone can benefit from the work done!
Bring a facilitator
Facilitation is all about making group processes easier. With a subject as potentially emotive and important as problem-solving, having an impartial third party in the form of a facilitator can make all the difference in finding great solutions and keeping the process moving. Consider bringing a facilitator to your problem-solving session to get better results and generate meaningful solutions!
Develop your problem-solving skills
It takes time and practice to be an effective problem solver. While some roles or participants might more naturally gravitate towards problem-solving, it can take development and planning to help everyone create better solutions.
You might develop a training program, run a problem-solving workshop or simply ask your team to practice using the techniques below. Check out our post on problem-solving skills to see how you and your group can develop the right mental process and be more resilient to issues too!
Design a great agenda
Workshops are a great format for solving problems. With the right approach, you can focus a group and help them find the solutions to their own problems. But designing a process can be time-consuming and finding the right activities can be difficult.
Check out our workshop planning guide to level-up your agenda design and start running more effective workshops. Need inspiration? Check out templates designed by expert facilitators to help you kickstart your process!
In this section, we’ll look at in-depth problem-solving methods that provide a complete end-to-end process for developing effective solutions. These will help guide your team from the discovery and definition of a problem through to delivering the right solution.
If you’re looking for an all-encompassing method or problem-solving model, these processes are a great place to start. They’ll ask your team to challenge preconceived ideas and adopt a mindset for solving problems more effectively.
- Six Thinking Hats
- Lightning Decision Jam
- Problem Definition Process
- Discovery & Action Dialogue
Design Sprint 2.0
- Open Space Technology
1. Six Thinking Hats
Individual approaches to solving a problem can be very different based on what team or role an individual holds. It can be easy for existing biases or perspectives to find their way into the mix, or for internal politics to direct a conversation.
Six Thinking Hats is a classic method for identifying the problems that need to be solved and enables your team to consider them from different angles, whether that is by focusing on facts and data, creative solutions, or by considering why a particular solution might not work.
Like all problem-solving frameworks, Six Thinking Hats is effective at helping teams remove roadblocks from a conversation or discussion and come to terms with all the aspects necessary to solve complex problems.
2. Lightning Decision Jam
Featured courtesy of Jonathan Courtney of AJ&Smart Berlin, Lightning Decision Jam is one of those strategies that should be in every facilitation toolbox. Exploring problems and finding solutions is often creative in nature, though as with any creative process, there is the potential to lose focus and get lost.
Unstructured discussions might get you there in the end, but it’s much more effective to use a method that creates a clear process and team focus.
In Lightning Decision Jam, participants are invited to begin by writing challenges, concerns, or mistakes on post-its without discussing them before then being invited by the moderator to present them to the group.
From there, the team vote on which problems to solve and are guided through steps that will allow them to reframe those problems, create solutions and then decide what to execute on.
By deciding the problems that need to be solved as a team before moving on, this group process is great for ensuring the whole team is aligned and can take ownership over the next stages.
Lightning Decision Jam (LDJ) #action #decision making #problem solving #issue analysis #innovation #design #remote-friendly The problem with anything that requires creative thinking is that it’s easy to get lost—lose focus and fall into the trap of having useless, open-ended, unstructured discussions. Here’s the most effective solution I’ve found: Replace all open, unstructured discussion with a clear process. What to use this exercise for: Anything which requires a group of people to make decisions, solve problems or discuss challenges. It’s always good to frame an LDJ session with a broad topic, here are some examples: The conversion flow of our checkout Our internal design process How we organise events Keeping up with our competition Improving sales flow
3. Problem Definition Process
While problems can be complex, the problem-solving methods you use to identify and solve those problems can often be simple in design.
By taking the time to truly identify and define a problem before asking the group to reframe the challenge as an opportunity, this method is a great way to enable change.
Begin by identifying a focus question and exploring the ways in which it manifests before splitting into five teams who will each consider the problem using a different method: escape, reversal, exaggeration, distortion or wishful. Teams develop a problem objective and create ideas in line with their method before then feeding them back to the group.
This method is great for enabling in-depth discussions while also creating space for finding creative solutions too!
Problem Definition #problem solving #idea generation #creativity #online #remote-friendly A problem solving technique to define a problem, challenge or opportunity and to generate ideas.
4. The 5 Whys
Sometimes, a group needs to go further with their strategies and analyze the root cause at the heart of organizational issues. An RCA or root cause analysis is the process of identifying what is at the heart of business problems or recurring challenges.
The 5 Whys is a simple and effective method of helping a group go find the root cause of any problem or challenge and conduct analysis that will deliver results.
By beginning with the creation of a problem statement and going through five stages to refine it, The 5 Whys provides everything you need to truly discover the cause of an issue.
The 5 Whys #hyperisland #innovation This simple and powerful method is useful for getting to the core of a problem or challenge. As the title suggests, the group defines a problems, then asks the question “why” five times, often using the resulting explanation as a starting point for creative problem solving.
5. World Cafe
World Cafe is a simple but powerful facilitation technique to help bigger groups to focus their energy and attention on solving complex problems.
World Cafe enables this approach by creating a relaxed atmosphere where participants are able to self-organize and explore topics relevant and important to them which are themed around a central problem-solving purpose. Create the right atmosphere by modeling your space after a cafe and after guiding the group through the method, let them take the lead!
Making problem-solving a part of your organization’s culture in the long term can be a difficult undertaking. More approachable formats like World Cafe can be especially effective in bringing people unfamiliar with workshops into the fold.
World Cafe #hyperisland #innovation #issue analysis World Café is a simple yet powerful method, originated by Juanita Brown, for enabling meaningful conversations driven completely by participants and the topics that are relevant and important to them. Facilitators create a cafe-style space and provide simple guidelines. Participants then self-organize and explore a set of relevant topics or questions for conversation.
6. Discovery & Action Dialogue (DAD)
One of the best approaches is to create a safe space for a group to share and discover practices and behaviors that can help them find their own solutions.
With DAD, you can help a group choose which problems they wish to solve and which approaches they will take to do so. It’s great at helping remove resistance to change and can help get buy-in at every level too!
This process of enabling frontline ownership is great in ensuring follow-through and is one of the methods you will want in your toolbox as a facilitator.
Discovery & Action Dialogue (DAD) #idea generation #liberating structures #action #issue analysis #remote-friendly DADs make it easy for a group or community to discover practices and behaviors that enable some individuals (without access to special resources and facing the same constraints) to find better solutions than their peers to common problems. These are called positive deviant (PD) behaviors and practices. DADs make it possible for people in the group, unit, or community to discover by themselves these PD practices. DADs also create favorable conditions for stimulating participants’ creativity in spaces where they can feel safe to invent new and more effective practices. Resistance to change evaporates as participants are unleashed to choose freely which practices they will adopt or try and which problems they will tackle. DADs make it possible to achieve frontline ownership of solutions.
7. Design Sprint 2.0
Want to see how a team can solve big problems and move forward with prototyping and testing solutions in a few days? The Design Sprint 2.0 template from Jake Knapp, author of Sprint, is a complete agenda for a with proven results.
Developing the right agenda can involve difficult but necessary planning. Ensuring all the correct steps are followed can also be stressful or time-consuming depending on your level of experience.
Use this complete 4-day workshop template if you are finding there is no obvious solution to your challenge and want to focus your team around a specific problem that might require a shortcut to launching a minimum viable product or waiting for the organization-wide implementation of a solution.
8. Open space technology
Open space technology- developed by Harrison Owen – creates a space where large groups are invited to take ownership of their problem solving and lead individual sessions. Open space technology is a great format when you have a great deal of expertise and insight in the room and want to allow for different takes and approaches on a particular theme or problem you need to be solved.
Start by bringing your participants together to align around a central theme and focus their efforts. Explain the ground rules to help guide the problem-solving process and then invite members to identify any issue connecting to the central theme that they are interested in and are prepared to take responsibility for.
Once participants have decided on their approach to the core theme, they write their issue on a piece of paper, announce it to the group, pick a session time and place, and post the paper on the wall. As the wall fills up with sessions, the group is then invited to join the sessions that interest them the most and which they can contribute to, then you’re ready to begin!
Everyone joins the problem-solving group they’ve signed up to, record the discussion and if appropriate, findings can then be shared with the rest of the group afterward.
Open Space Technology #action plan #idea generation #problem solving #issue analysis #large group #online #remote-friendly Open Space is a methodology for large groups to create their agenda discerning important topics for discussion, suitable for conferences, community gatherings and whole system facilitation
Techniques to identify and analyze problems
Using a problem-solving method to help a team identify and analyze a problem can be a quick and effective addition to any workshop or meeting.
While further actions are always necessary, you can generate momentum and alignment easily, and these activities are a great place to get started.
We’ve put together this list of techniques to help you and your team with problem identification, analysis, and discussion that sets the foundation for developing effective solutions.
Let’s take a look!
- The Creativity Dice
- Fishbone Analysis
- Problem Tree
- SWOT Analysis
- Agreement-Certainty Matrix
- The Journalistic Six
- LEGO Challenge
- What, So What, Now What?
- Journalists
Individual and group perspectives are incredibly important, but what happens if people are set in their minds and need a change of perspective in order to approach a problem more effectively?
Flip It is a method we love because it is both simple to understand and run, and allows groups to understand how their perspectives and biases are formed.
Participants in Flip It are first invited to consider concerns, issues, or problems from a perspective of fear and write them on a flip chart. Then, the group is asked to consider those same issues from a perspective of hope and flip their understanding.
No problem and solution is free from existing bias and by changing perspectives with Flip It, you can then develop a problem solving model quickly and effectively.
Flip It! #gamestorming #problem solving #action Often, a change in a problem or situation comes simply from a change in our perspectives. Flip It! is a quick game designed to show players that perspectives are made, not born.
10. The Creativity Dice
One of the most useful problem solving skills you can teach your team is of approaching challenges with creativity, flexibility, and openness. Games like The Creativity Dice allow teams to overcome the potential hurdle of too much linear thinking and approach the process with a sense of fun and speed.
In The Creativity Dice, participants are organized around a topic and roll a dice to determine what they will work on for a period of 3 minutes at a time. They might roll a 3 and work on investigating factual information on the chosen topic. They might roll a 1 and work on identifying the specific goals, standards, or criteria for the session.
Encouraging rapid work and iteration while asking participants to be flexible are great skills to cultivate. Having a stage for idea incubation in this game is also important. Moments of pause can help ensure the ideas that are put forward are the most suitable.
The Creativity Dice #creativity #problem solving #thiagi #issue analysis Too much linear thinking is hazardous to creative problem solving. To be creative, you should approach the problem (or the opportunity) from different points of view. You should leave a thought hanging in mid-air and move to another. This skipping around prevents premature closure and lets your brain incubate one line of thought while you consciously pursue another.
11. Fishbone Analysis
Organizational or team challenges are rarely simple, and it’s important to remember that one problem can be an indication of something that goes deeper and may require further consideration to be solved.
Fishbone Analysis helps groups to dig deeper and understand the origins of a problem. It’s a great example of a root cause analysis method that is simple for everyone on a team to get their head around.
Participants in this activity are asked to annotate a diagram of a fish, first adding the problem or issue to be worked on at the head of a fish before then brainstorming the root causes of the problem and adding them as bones on the fish.
Using abstractions such as a diagram of a fish can really help a team break out of their regular thinking and develop a creative approach.
Fishbone Analysis #problem solving ##root cause analysis #decision making #online facilitation A process to help identify and understand the origins of problems, issues or observations.
12. Problem Tree
Encouraging visual thinking can be an essential part of many strategies. By simply reframing and clarifying problems, a group can move towards developing a problem solving model that works for them.
In Problem Tree, groups are asked to first brainstorm a list of problems – these can be design problems, team problems or larger business problems – and then organize them into a hierarchy. The hierarchy could be from most important to least important or abstract to practical, though the key thing with problem solving games that involve this aspect is that your group has some way of managing and sorting all the issues that are raised.
Once you have a list of problems that need to be solved and have organized them accordingly, you’re then well-positioned for the next problem solving steps.
Problem tree #define intentions #create #design #issue analysis A problem tree is a tool to clarify the hierarchy of problems addressed by the team within a design project; it represents high level problems or related sublevel problems.
13. SWOT Analysis
Chances are you’ve heard of the SWOT Analysis before. This problem-solving method focuses on identifying strengths, weaknesses, opportunities, and threats is a tried and tested method for both individuals and teams.
Start by creating a desired end state or outcome and bare this in mind – any process solving model is made more effective by knowing what you are moving towards. Create a quadrant made up of the four categories of a SWOT analysis and ask participants to generate ideas based on each of those quadrants.
Once you have those ideas assembled in their quadrants, cluster them together based on their affinity with other ideas. These clusters are then used to facilitate group conversations and move things forward.
SWOT analysis #gamestorming #problem solving #action #meeting facilitation The SWOT Analysis is a long-standing technique of looking at what we have, with respect to the desired end state, as well as what we could improve on. It gives us an opportunity to gauge approaching opportunities and dangers, and assess the seriousness of the conditions that affect our future. When we understand those conditions, we can influence what comes next.
14. Agreement-Certainty Matrix
Not every problem-solving approach is right for every challenge, and deciding on the right method for the challenge at hand is a key part of being an effective team.
The Agreement Certainty matrix helps teams align on the nature of the challenges facing them. By sorting problems from simple to chaotic, your team can understand what methods are suitable for each problem and what they can do to ensure effective results.
If you are already using Liberating Structures techniques as part of your problem-solving strategy, the Agreement-Certainty Matrix can be an invaluable addition to your process. We’ve found it particularly if you are having issues with recurring problems in your organization and want to go deeper in understanding the root cause.
Agreement-Certainty Matrix #issue analysis #liberating structures #problem solving You can help individuals or groups avoid the frequent mistake of trying to solve a problem with methods that are not adapted to the nature of their challenge. The combination of two questions makes it possible to easily sort challenges into four categories: simple, complicated, complex , and chaotic . A problem is simple when it can be solved reliably with practices that are easy to duplicate. It is complicated when experts are required to devise a sophisticated solution that will yield the desired results predictably. A problem is complex when there are several valid ways to proceed but outcomes are not predictable in detail. Chaotic is when the context is too turbulent to identify a path forward. A loose analogy may be used to describe these differences: simple is like following a recipe, complicated like sending a rocket to the moon, complex like raising a child, and chaotic is like the game “Pin the Tail on the Donkey.” The Liberating Structures Matching Matrix in Chapter 5 can be used as the first step to clarify the nature of a challenge and avoid the mismatches between problems and solutions that are frequently at the root of chronic, recurring problems.
Organizing and charting a team’s progress can be important in ensuring its success. SQUID (Sequential Question and Insight Diagram) is a great model that allows a team to effectively switch between giving questions and answers and develop the skills they need to stay on track throughout the process.
Begin with two different colored sticky notes – one for questions and one for answers – and with your central topic (the head of the squid) on the board. Ask the group to first come up with a series of questions connected to their best guess of how to approach the topic. Ask the group to come up with answers to those questions, fix them to the board and connect them with a line. After some discussion, go back to question mode by responding to the generated answers or other points on the board.
It’s rewarding to see a diagram grow throughout the exercise, and a completed SQUID can provide a visual resource for future effort and as an example for other teams.
SQUID #gamestorming #project planning #issue analysis #problem solving When exploring an information space, it’s important for a group to know where they are at any given time. By using SQUID, a group charts out the territory as they go and can navigate accordingly. SQUID stands for Sequential Question and Insight Diagram.
16. Speed Boat
To continue with our nautical theme, Speed Boat is a short and sweet activity that can help a team quickly identify what employees, clients or service users might have a problem with and analyze what might be standing in the way of achieving a solution.
Methods that allow for a group to make observations, have insights and obtain those eureka moments quickly are invaluable when trying to solve complex problems.
In Speed Boat, the approach is to first consider what anchors and challenges might be holding an organization (or boat) back. Bonus points if you are able to identify any sharks in the water and develop ideas that can also deal with competitors!
Speed Boat #gamestorming #problem solving #action Speedboat is a short and sweet way to identify what your employees or clients don’t like about your product/service or what’s standing in the way of a desired goal.
17. The Journalistic Six
Some of the most effective ways of solving problems is by encouraging teams to be more inclusive and diverse in their thinking.
Based on the six key questions journalism students are taught to answer in articles and news stories, The Journalistic Six helps create teams to see the whole picture. By using who, what, when, where, why, and how to facilitate the conversation and encourage creative thinking, your team can make sure that the problem identification and problem analysis stages of the are covered exhaustively and thoughtfully. Reporter’s notebook and dictaphone optional.
The Journalistic Six – Who What When Where Why How #idea generation #issue analysis #problem solving #online #creative thinking #remote-friendly A questioning method for generating, explaining, investigating ideas.
18. LEGO Challenge
Now for an activity that is a little out of the (toy) box. LEGO Serious Play is a facilitation methodology that can be used to improve creative thinking and problem-solving skills.
The LEGO Challenge includes giving each member of the team an assignment that is hidden from the rest of the group while they create a structure without speaking.
What the LEGO challenge brings to the table is a fun working example of working with stakeholders who might not be on the same page to solve problems. Also, it’s LEGO! Who doesn’t love LEGO!
LEGO Challenge #hyperisland #team A team-building activity in which groups must work together to build a structure out of LEGO, but each individual has a secret “assignment” which makes the collaborative process more challenging. It emphasizes group communication, leadership dynamics, conflict, cooperation, patience and problem solving strategy.
19. What, So What, Now What?
If not carefully managed, the problem identification and problem analysis stages of the problem-solving process can actually create more problems and misunderstandings.
The What, So What, Now What? problem-solving activity is designed to help collect insights and move forward while also eliminating the possibility of disagreement when it comes to identifying, clarifying, and analyzing organizational or work problems.
Facilitation is all about bringing groups together so that might work on a shared goal and the best problem-solving strategies ensure that teams are aligned in purpose, if not initially in opinion or insight.
Throughout the three steps of this game, you give everyone on a team to reflect on a problem by asking what happened, why it is important, and what actions should then be taken.
This can be a great activity for bringing our individual perceptions about a problem or challenge and contextualizing it in a larger group setting. This is one of the most important problem-solving skills you can bring to your organization.
W³ – What, So What, Now What? #issue analysis #innovation #liberating structures You can help groups reflect on a shared experience in a way that builds understanding and spurs coordinated action while avoiding unproductive conflict. It is possible for every voice to be heard while simultaneously sifting for insights and shaping new direction. Progressing in stages makes this practical—from collecting facts about What Happened to making sense of these facts with So What and finally to what actions logically follow with Now What . The shared progression eliminates most of the misunderstandings that otherwise fuel disagreements about what to do. Voila!
20. Journalists
Problem analysis can be one of the most important and decisive stages of all problem-solving tools. Sometimes, a team can become bogged down in the details and are unable to move forward.
Journalists is an activity that can avoid a group from getting stuck in the problem identification or problem analysis stages of the process.
In Journalists, the group is invited to draft the front page of a fictional newspaper and figure out what stories deserve to be on the cover and what headlines those stories will have. By reframing how your problems and challenges are approached, you can help a team move productively through the process and be better prepared for the steps to follow.
Journalists #vision #big picture #issue analysis #remote-friendly This is an exercise to use when the group gets stuck in details and struggles to see the big picture. Also good for defining a vision.
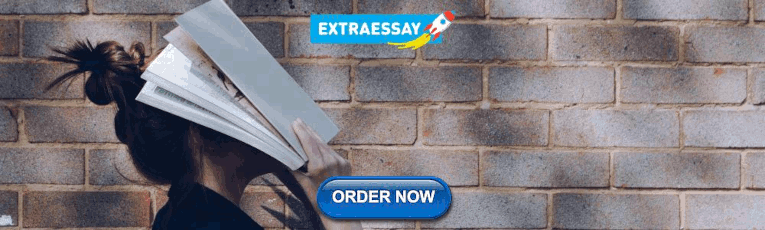
Problem-solving techniques for developing solutions
The success of any problem-solving process can be measured by the solutions it produces. After you’ve defined the issue, explored existing ideas, and ideated, it’s time to narrow down to the correct solution.
Use these problem-solving techniques when you want to help your team find consensus, compare possible solutions, and move towards taking action on a particular problem.
- Improved Solutions
- Four-Step Sketch
- 15% Solutions
- How-Now-Wow matrix
- Impact Effort Matrix
21. Mindspin
Brainstorming is part of the bread and butter of the problem-solving process and all problem-solving strategies benefit from getting ideas out and challenging a team to generate solutions quickly.
With Mindspin, participants are encouraged not only to generate ideas but to do so under time constraints and by slamming down cards and passing them on. By doing multiple rounds, your team can begin with a free generation of possible solutions before moving on to developing those solutions and encouraging further ideation.
This is one of our favorite problem-solving activities and can be great for keeping the energy up throughout the workshop. Remember the importance of helping people become engaged in the process – energizing problem-solving techniques like Mindspin can help ensure your team stays engaged and happy, even when the problems they’re coming together to solve are complex.
MindSpin #teampedia #idea generation #problem solving #action A fast and loud method to enhance brainstorming within a team. Since this activity has more than round ideas that are repetitive can be ruled out leaving more creative and innovative answers to the challenge.
22. Improved Solutions
After a team has successfully identified a problem and come up with a few solutions, it can be tempting to call the work of the problem-solving process complete. That said, the first solution is not necessarily the best, and by including a further review and reflection activity into your problem-solving model, you can ensure your group reaches the best possible result.
One of a number of problem-solving games from Thiagi Group, Improved Solutions helps you go the extra mile and develop suggested solutions with close consideration and peer review. By supporting the discussion of several problems at once and by shifting team roles throughout, this problem-solving technique is a dynamic way of finding the best solution.
Improved Solutions #creativity #thiagi #problem solving #action #team You can improve any solution by objectively reviewing its strengths and weaknesses and making suitable adjustments. In this creativity framegame, you improve the solutions to several problems. To maintain objective detachment, you deal with a different problem during each of six rounds and assume different roles (problem owner, consultant, basher, booster, enhancer, and evaluator) during each round. At the conclusion of the activity, each player ends up with two solutions to her problem.
23. Four Step Sketch
Creative thinking and visual ideation does not need to be confined to the opening stages of your problem-solving strategies. Exercises that include sketching and prototyping on paper can be effective at the solution finding and development stage of the process, and can be great for keeping a team engaged.
By going from simple notes to a crazy 8s round that involves rapidly sketching 8 variations on their ideas before then producing a final solution sketch, the group is able to iterate quickly and visually. Problem-solving techniques like Four-Step Sketch are great if you have a group of different thinkers and want to change things up from a more textual or discussion-based approach.
Four-Step Sketch #design sprint #innovation #idea generation #remote-friendly The four-step sketch is an exercise that helps people to create well-formed concepts through a structured process that includes: Review key information Start design work on paper, Consider multiple variations , Create a detailed solution . This exercise is preceded by a set of other activities allowing the group to clarify the challenge they want to solve. See how the Four Step Sketch exercise fits into a Design Sprint
24. 15% Solutions
Some problems are simpler than others and with the right problem-solving activities, you can empower people to take immediate actions that can help create organizational change.
Part of the liberating structures toolkit, 15% solutions is a problem-solving technique that focuses on finding and implementing solutions quickly. A process of iterating and making small changes quickly can help generate momentum and an appetite for solving complex problems.
Problem-solving strategies can live and die on whether people are onboard. Getting some quick wins is a great way of getting people behind the process.
It can be extremely empowering for a team to realize that problem-solving techniques can be deployed quickly and easily and delineate between things they can positively impact and those things they cannot change.
15% Solutions #action #liberating structures #remote-friendly You can reveal the actions, however small, that everyone can do immediately. At a minimum, these will create momentum, and that may make a BIG difference. 15% Solutions show that there is no reason to wait around, feel powerless, or fearful. They help people pick it up a level. They get individuals and the group to focus on what is within their discretion instead of what they cannot change. With a very simple question, you can flip the conversation to what can be done and find solutions to big problems that are often distributed widely in places not known in advance. Shifting a few grains of sand may trigger a landslide and change the whole landscape.
25. How-Now-Wow Matrix
The problem-solving process is often creative, as complex problems usually require a change of thinking and creative response in order to find the best solutions. While it’s common for the first stages to encourage creative thinking, groups can often gravitate to familiar solutions when it comes to the end of the process.
When selecting solutions, you don’t want to lose your creative energy! The How-Now-Wow Matrix from Gamestorming is a great problem-solving activity that enables a group to stay creative and think out of the box when it comes to selecting the right solution for a given problem.
Problem-solving techniques that encourage creative thinking and the ideation and selection of new solutions can be the most effective in organisational change. Give the How-Now-Wow Matrix a go, and not just for how pleasant it is to say out loud.
How-Now-Wow Matrix #gamestorming #idea generation #remote-friendly When people want to develop new ideas, they most often think out of the box in the brainstorming or divergent phase. However, when it comes to convergence, people often end up picking ideas that are most familiar to them. This is called a ‘creative paradox’ or a ‘creadox’. The How-Now-Wow matrix is an idea selection tool that breaks the creadox by forcing people to weigh each idea on 2 parameters.
26. Impact and Effort Matrix
All problem-solving techniques hope to not only find solutions to a given problem or challenge but to find the best solution. When it comes to finding a solution, groups are invited to put on their decision-making hats and really think about how a proposed idea would work in practice.
The Impact and Effort Matrix is one of the problem-solving techniques that fall into this camp, empowering participants to first generate ideas and then categorize them into a 2×2 matrix based on impact and effort.
Activities that invite critical thinking while remaining simple are invaluable. Use the Impact and Effort Matrix to move from ideation and towards evaluating potential solutions before then committing to them.
Impact and Effort Matrix #gamestorming #decision making #action #remote-friendly In this decision-making exercise, possible actions are mapped based on two factors: effort required to implement and potential impact. Categorizing ideas along these lines is a useful technique in decision making, as it obliges contributors to balance and evaluate suggested actions before committing to them.
27. Dotmocracy
If you’ve followed each of the problem-solving steps with your group successfully, you should move towards the end of your process with heaps of possible solutions developed with a specific problem in mind. But how do you help a group go from ideation to putting a solution into action?
Dotmocracy – or Dot Voting -is a tried and tested method of helping a team in the problem-solving process make decisions and put actions in place with a degree of oversight and consensus.
One of the problem-solving techniques that should be in every facilitator’s toolbox, Dot Voting is fast and effective and can help identify the most popular and best solutions and help bring a group to a decision effectively.
Dotmocracy #action #decision making #group prioritization #hyperisland #remote-friendly Dotmocracy is a simple method for group prioritization or decision-making. It is not an activity on its own, but a method to use in processes where prioritization or decision-making is the aim. The method supports a group to quickly see which options are most popular or relevant. The options or ideas are written on post-its and stuck up on a wall for the whole group to see. Each person votes for the options they think are the strongest, and that information is used to inform a decision.
All facilitators know that warm-ups and icebreakers are useful for any workshop or group process. Problem-solving workshops are no different.
Use these problem-solving techniques to warm up a group and prepare them for the rest of the process. Activating your group by tapping into some of the top problem-solving skills can be one of the best ways to see great outcomes from your session.
- Check-in/Check-out
- Doodling Together
- Show and Tell
- Constellations
- Draw a Tree
28. Check-in / Check-out
Solid processes are planned from beginning to end, and the best facilitators know that setting the tone and establishing a safe, open environment can be integral to a successful problem-solving process.
Check-in / Check-out is a great way to begin and/or bookend a problem-solving workshop. Checking in to a session emphasizes that everyone will be seen, heard, and expected to contribute.
If you are running a series of meetings, setting a consistent pattern of checking in and checking out can really help your team get into a groove. We recommend this opening-closing activity for small to medium-sized groups though it can work with large groups if they’re disciplined!
Check-in / Check-out #team #opening #closing #hyperisland #remote-friendly Either checking-in or checking-out is a simple way for a team to open or close a process, symbolically and in a collaborative way. Checking-in/out invites each member in a group to be present, seen and heard, and to express a reflection or a feeling. Checking-in emphasizes presence, focus and group commitment; checking-out emphasizes reflection and symbolic closure.
29. Doodling Together
Thinking creatively and not being afraid to make suggestions are important problem-solving skills for any group or team, and warming up by encouraging these behaviors is a great way to start.
Doodling Together is one of our favorite creative ice breaker games – it’s quick, effective, and fun and can make all following problem-solving steps easier by encouraging a group to collaborate visually. By passing cards and adding additional items as they go, the workshop group gets into a groove of co-creation and idea development that is crucial to finding solutions to problems.
Doodling Together #collaboration #creativity #teamwork #fun #team #visual methods #energiser #icebreaker #remote-friendly Create wild, weird and often funny postcards together & establish a group’s creative confidence.
30. Show and Tell
You might remember some version of Show and Tell from being a kid in school and it’s a great problem-solving activity to kick off a session.
Asking participants to prepare a little something before a workshop by bringing an object for show and tell can help them warm up before the session has even begun! Games that include a physical object can also help encourage early engagement before moving onto more big-picture thinking.
By asking your participants to tell stories about why they chose to bring a particular item to the group, you can help teams see things from new perspectives and see both differences and similarities in the way they approach a topic. Great groundwork for approaching a problem-solving process as a team!
Show and Tell #gamestorming #action #opening #meeting facilitation Show and Tell taps into the power of metaphors to reveal players’ underlying assumptions and associations around a topic The aim of the game is to get a deeper understanding of stakeholders’ perspectives on anything—a new project, an organizational restructuring, a shift in the company’s vision or team dynamic.
31. Constellations
Who doesn’t love stars? Constellations is a great warm-up activity for any workshop as it gets people up off their feet, energized, and ready to engage in new ways with established topics. It’s also great for showing existing beliefs, biases, and patterns that can come into play as part of your session.
Using warm-up games that help build trust and connection while also allowing for non-verbal responses can be great for easing people into the problem-solving process and encouraging engagement from everyone in the group. Constellations is great in large spaces that allow for movement and is definitely a practical exercise to allow the group to see patterns that are otherwise invisible.
Constellations #trust #connection #opening #coaching #patterns #system Individuals express their response to a statement or idea by standing closer or further from a central object. Used with teams to reveal system, hidden patterns, perspectives.
32. Draw a Tree
Problem-solving games that help raise group awareness through a central, unifying metaphor can be effective ways to warm-up a group in any problem-solving model.
Draw a Tree is a simple warm-up activity you can use in any group and which can provide a quick jolt of energy. Start by asking your participants to draw a tree in just 45 seconds – they can choose whether it will be abstract or realistic.
Once the timer is up, ask the group how many people included the roots of the tree and use this as a means to discuss how we can ignore important parts of any system simply because they are not visible.
All problem-solving strategies are made more effective by thinking of problems critically and by exposing things that may not normally come to light. Warm-up games like Draw a Tree are great in that they quickly demonstrate some key problem-solving skills in an accessible and effective way.
Draw a Tree #thiagi #opening #perspectives #remote-friendly With this game you can raise awarness about being more mindful, and aware of the environment we live in.
Each step of the problem-solving workshop benefits from an intelligent deployment of activities, games, and techniques. Bringing your session to an effective close helps ensure that solutions are followed through on and that you also celebrate what has been achieved.
Here are some problem-solving activities you can use to effectively close a workshop or meeting and ensure the great work you’ve done can continue afterward.
- One Breath Feedback
- Who What When Matrix
- Response Cards
How do I conclude a problem-solving process?
All good things must come to an end. With the bulk of the work done, it can be tempting to conclude your workshop swiftly and without a moment to debrief and align. This can be problematic in that it doesn’t allow your team to fully process the results or reflect on the process.
At the end of an effective session, your team will have gone through a process that, while productive, can be exhausting. It’s important to give your group a moment to take a breath, ensure that they are clear on future actions, and provide short feedback before leaving the space.
The primary purpose of any problem-solving method is to generate solutions and then implement them. Be sure to take the opportunity to ensure everyone is aligned and ready to effectively implement the solutions you produced in the workshop.
Remember that every process can be improved and by giving a short moment to collect feedback in the session, you can further refine your problem-solving methods and see further success in the future too.
33. One Breath Feedback
Maintaining attention and focus during the closing stages of a problem-solving workshop can be tricky and so being concise when giving feedback can be important. It’s easy to incur “death by feedback” should some team members go on for too long sharing their perspectives in a quick feedback round.
One Breath Feedback is a great closing activity for workshops. You give everyone an opportunity to provide feedback on what they’ve done but only in the space of a single breath. This keeps feedback short and to the point and means that everyone is encouraged to provide the most important piece of feedback to them.
One breath feedback #closing #feedback #action This is a feedback round in just one breath that excels in maintaining attention: each participants is able to speak during just one breath … for most people that’s around 20 to 25 seconds … unless of course you’ve been a deep sea diver in which case you’ll be able to do it for longer.
34. Who What When Matrix
Matrices feature as part of many effective problem-solving strategies and with good reason. They are easily recognizable, simple to use, and generate results.
The Who What When Matrix is a great tool to use when closing your problem-solving session by attributing a who, what and when to the actions and solutions you have decided upon. The resulting matrix is a simple, easy-to-follow way of ensuring your team can move forward.
Great solutions can’t be enacted without action and ownership. Your problem-solving process should include a stage for allocating tasks to individuals or teams and creating a realistic timeframe for those solutions to be implemented or checked out. Use this method to keep the solution implementation process clear and simple for all involved.
Who/What/When Matrix #gamestorming #action #project planning With Who/What/When matrix, you can connect people with clear actions they have defined and have committed to.
35. Response cards
Group discussion can comprise the bulk of most problem-solving activities and by the end of the process, you might find that your team is talked out!
Providing a means for your team to give feedback with short written notes can ensure everyone is head and can contribute without the need to stand up and talk. Depending on the needs of the group, giving an alternative can help ensure everyone can contribute to your problem-solving model in the way that makes the most sense for them.
Response Cards is a great way to close a workshop if you are looking for a gentle warm-down and want to get some swift discussion around some of the feedback that is raised.
Response Cards #debriefing #closing #structured sharing #questions and answers #thiagi #action It can be hard to involve everyone during a closing of a session. Some might stay in the background or get unheard because of louder participants. However, with the use of Response Cards, everyone will be involved in providing feedback or clarify questions at the end of a session.
Save time and effort discovering the right solutions
A structured problem solving process is a surefire way of solving tough problems, discovering creative solutions and driving organizational change. But how can you design for successful outcomes?
With SessionLab, it’s easy to design engaging workshops that deliver results. Drag, drop and reorder blocks to build your agenda. When you make changes or update your agenda, your session timing adjusts automatically , saving you time on manual adjustments.
Collaborating with stakeholders or clients? Share your agenda with a single click and collaborate in real-time. No more sending documents back and forth over email.
Explore how to use SessionLab to design effective problem solving workshops or watch this five minute video to see the planner in action!
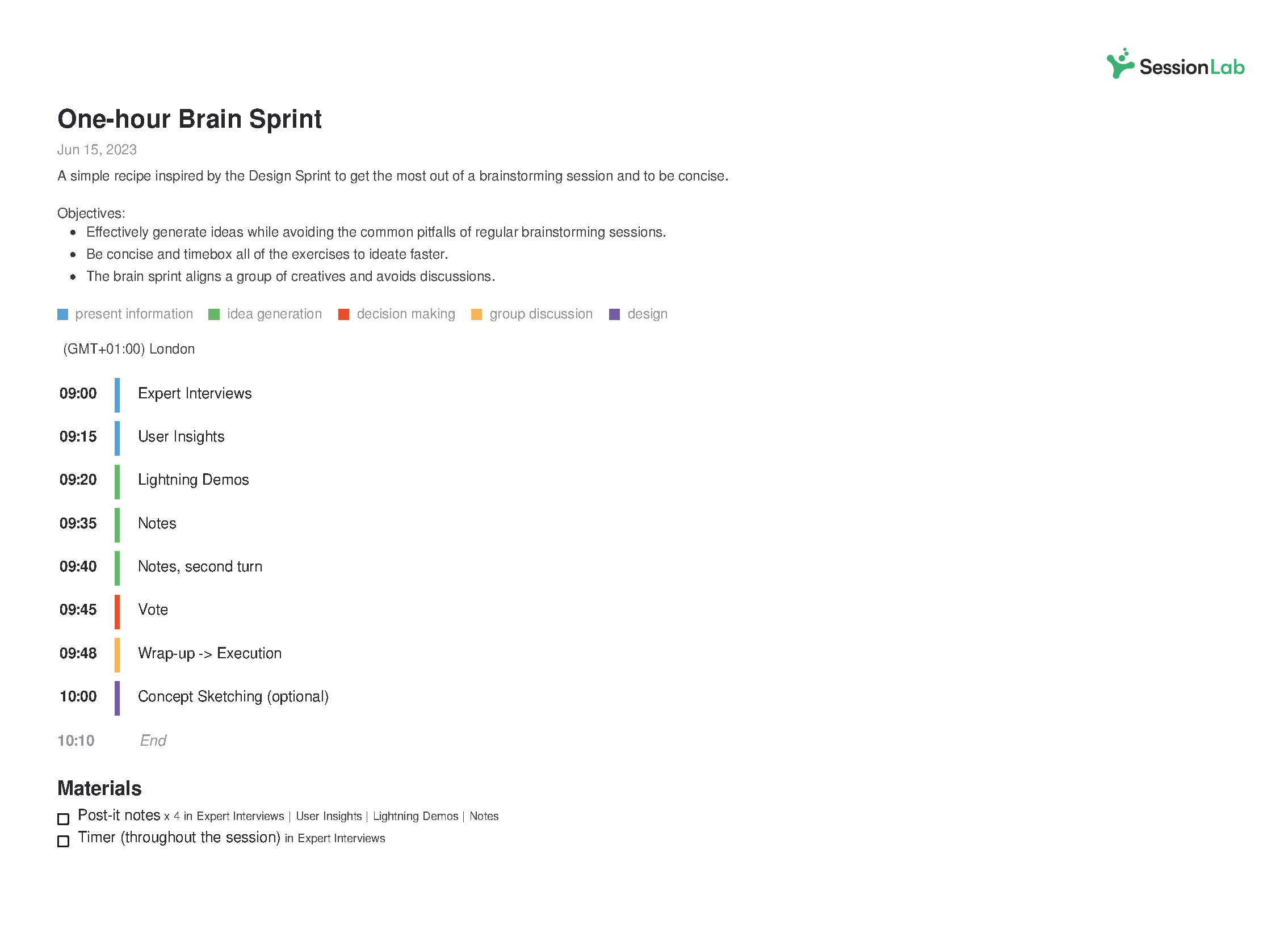
Over to you
The problem-solving process can often be as complicated and multifaceted as the problems they are set-up to solve. With the right problem-solving techniques and a mix of creative exercises designed to guide discussion and generate purposeful ideas, we hope we’ve given you the tools to find the best solutions as simply and easily as possible.
Is there a problem-solving technique that you are missing here? Do you have a favorite activity or method you use when facilitating? Let us know in the comments below, we’d love to hear from you!
thank you very much for these excellent techniques
Certainly wonderful article, very detailed. Shared!
Your list of techniques for problem solving can be helpfully extended by adding TRIZ to the list of techniques. TRIZ has 40 problem solving techniques derived from methods inventros and patent holders used to get new patents. About 10-12 are general approaches. many organization sponsor classes in TRIZ that are used to solve business problems or general organiztational problems. You can take a look at TRIZ and dwonload a free internet booklet to see if you feel it shound be included per your selection process.
Leave a Comment Cancel reply
Your email address will not be published. Required fields are marked *
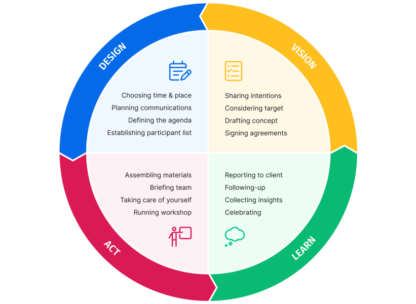
Going from a mere idea to a workshop that delivers results for your clients can feel like a daunting task. In this piece, we will shine a light on all the work behind the scenes and help you learn how to plan a workshop from start to finish. On a good day, facilitation can feel like effortless magic, but that is mostly the result of backstage work, foresight, and a lot of careful planning. Read on to learn a step-by-step approach to breaking the process of planning a workshop into small, manageable chunks. The flow starts with the first meeting with a client to define the purposes of a workshop.…
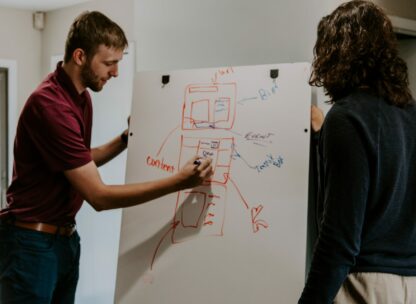
How does learning work? A clever 9-year-old once told me: “I know I am learning something new when I am surprised.” The science of adult learning tells us that, in order to learn new skills (which, unsurprisingly, is harder for adults to do than kids) grown-ups need to first get into a specific headspace. In a business, this approach is often employed in a training session where employees learn new skills or work on professional development. But how do you ensure your training is effective? In this guide, we'll explore how to create an effective training session plan and run engaging training sessions. As team leader, project manager, or consultant,…
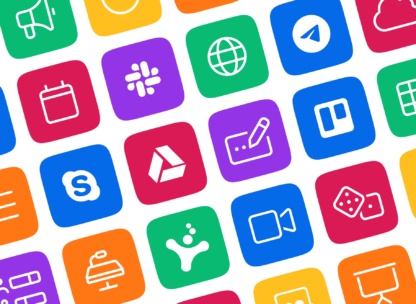
Effective online tools are a necessity for smooth and engaging virtual workshops and meetings. But how do you choose the right ones? Do you sometimes feel that the good old pen and paper or MS Office toolkit and email leaves you struggling to stay on top of managing and delivering your workshop? Fortunately, there are plenty of online tools to make your life easier when you need to facilitate a meeting and lead workshops. In this post, we’ll share our favorite online tools you can use to make your job as a facilitator easier. In fact, there are plenty of free online workshop tools and meeting facilitation software you can…
Design your next workshop with SessionLab
Join the 150,000 facilitators using SessionLab
Sign up for free
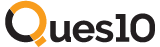
Welcome back.
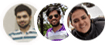
Continue with email
Mumbai University > Computer Engineering > Sem 7 > Artificial Intelligence
Marks: 5 Marks
Year: Dec 2015, May 2016
More From Forbes
The problem behind the problem: the human factor.

- Share to Facebook
- Share to Twitter
- Share to Linkedin
With Daniel Lee, President & Partner of Carpedia International
When workarounds become permanent solutions, your business begins to look like a certain children's song.
Missteps are common in the world of business. We can all remember these very public examples:
- A product or service is launched or discontinued without sufficient demand forecasting (“New Coke” in the 1980s)
- A technology or trend is underestimated (Kodak's digital photography hesitation in the 1990s)
- Expansion into a new market is executed too hastily (Target’s rapid rollout of more than 100 Canadian stores in the early 2010s)
- Labor is cut without fully assessing the impact on customer satisfaction (The US Post Office in the 2000s, 2010s and 2020s)
- Large-scale change programs are rolled out without adequate training or prototyping (General Electric's ambitious digital transformation in the late 2010s).
Beyond these high-profile stories, nearly every business has its own list of lessons learned. (Our firm has several – you can find them here .)
Northern Lights Could Show Up Yet Again Tonight Here s An Updated Aurora Borealis Forecast
Ios 17.5—apple issues update now warning to all iphone users, michael cohen testifies ex trump fixer confirms he called trump cheeto dusted cartoon villain in fiery cross examination, there’s a hole in the bucket.
“There’s a hole in the bucket.” This is a children’s song that has been around in various forms for more than 200 years.
It tells a simple story of Henry, whose bucket leaks. Liza tells him to fix it. But to do that, he needs straw. To cut the straw, he needs a knife. To use the knife, he needs to sharpen it. But the sharpening stone must be damp, so he needs water. But to fetch water, he needs the bucket, and the bucket has a hole in it.
The moral of the story: Problems are often more complicated than they first appear to be.
So, if we learn this as children, why do we often forget it as business leaders?
Three reasons stand out.
First, business moves fast. The bottom line doesn’t always wait for a complete solution. Second, the closer we are to a problem, the harder it may be for us to see the way out. This “proximity paradox” occurs when workarounds stick around, and stop being seen as workarounds. The third, and arguably the most impactful, reason is that most problems involve people, and people are complicated.
In consulting, it’s our job to find and help fix problems, layer by layer. On paper, solutions might seem relatively simple. Apply management principles, develop performance measurement tools, streamline processes, target the point of constraint, reduce inefficiency, and maximize productivity. These are logical stages of process improvement and organizational alignment.
But if logical equaled simple, all organizations would operate at peak performance. The fact that most achieve roughly 60 to 70% of their maximum productivity for any given process tells us there’s a hole in the bucket. That operational improvement must account for the human factor.
The Human Factor
Years ago, our firm engaged in a process improvement project for an automotive parts manufacturer. One of our consultants observed that the plant had created its own time zone. All the clocks in the plant were set ten minutes ahead of the actual time. No one knew why except a single long-term employee, who eventually recalled that the clocks were changed many years before so that shift workers could finish early to catch a bus that came on the hour.
The bus had not run on the hour for several years, but the practice had continued. This caused a problem at the injection molding machines because in the ten-minute gap, the plastic congealed, leaving the next shift to spend their first fifteen minutes purging the machines.
Management was advised of the molding machine problem and decided to end the early departure practice and implement a “hot change” at the end of the shift so that the machines could keep running.
Three months later, nothing had changed. Not even the clocks.
What seemed like a simple change had a multitude of implications throughout the plant and directly impacted the activities of employees, production schedulers, and frontline managers. Changing the process affected how crews were scheduled and appeared to ask people to work more than they had previously. The planning standards and staging areas needed to be modified to reflect new input and output expectations, and managers now had to be physically on the floor at shift change to make sure the transition ran smoothly. Coordinating this among different shifts and different departments was more complicated than it looked on paper.
We see this scenario play out repeatedly. Few process changes are simple. They often have implications for other parts of the organization, and sometimes those implications are far deeper than leaders realize until the changes are made. And sometimes, the logical solution doesn’t address the underlying problem.
At a hotel resort property, there was a mismatch between when guests dined, and when the stewarding staff were available to clean dishes. A design was configured to stagger shift starts to better align staffing with the demand patterns of the restaurants. But, unique to this location, the stewarding employees lived in a neighboring community and relied on a single bus for transportation. It ran only twice per day, and the bus schedule did not align with the new shifts. This situation highlighted the often-complex balance between cost containment and labor management.
The Not-So-Quick Fix
Complex problems require respect, careful consideration, flexibility, and hands-on solutions. When tackling the layers added or impacted by the human factor, there are no shortcuts to effectiveness. Transformation of any kind within an organization takes time and effort. The following guiding principles offer a good place to start.
1. Create a Results Strategy and an Implementation Plan
The Results Strategy is a working road map and a problem-solving tool that guides the allocation of time and resources. It articulates, in practical terms , how results will be achieved and how the impact will be realized in key indicators and financial statements. It identifies potential issues and complications that need to be considered. The strategy also sets expectations regarding activities, focus, timing, and participation.
Understanding the depths of the changes being implemented is critical to success. The objective is not to make a few method changes and claim victory. Sustainable solutions often require transitioning to a new way of managing the organization.
2. Provide Managers with a Supportive Environment
Managers are the lynchpin to improvement. How they schedule work, communicate expectations, follow up on performance, and problem-solve issues with their staff are vital elements to a successful change initiative. Leaders must make sure their front-line managers are prepared sufficiently in advance of a change program and have the appropriate tools they need. One key area to focus on is the alignment between strategic objectives, day-to-day performance planning, and the measurement systems designed to highlight where there are disconnects.
3. Recognize That Bumps Are Part of the Road
Change affects people differently, and adjustments will not always be smooth. Many people experience what has been termed an “Emotional Cycle of Change,” navigating five distinct stages before successfully adapting. Recognizing this cycle helps people normalize the range of emotions they may experience and can help an organization respond empathetically, provide necessary support, maintain resilience, and move forward effectively.
Leaders need to anticipate challenges and resistance and make sure managers understand why these reactions happen and how to manage them.
4. View the Path to High Performance as Never-Ending
High performance is not an end goal but an ongoing journey. Leaders must nurture a culture of continuous improvement where each level of achievement paves the way to the next.
In addressing the human factor, the not-so-quick fixes teach a valuable lesson in business problem-solving. They underscore the need for an analytical and empathetic approach to operational dilemmas, ensuring that solutions foster not just immediate benefits but sustainable progress—an approach where “fixing the hole in the bucket” considers the bucket’s purpose, design, and the person carrying the water.
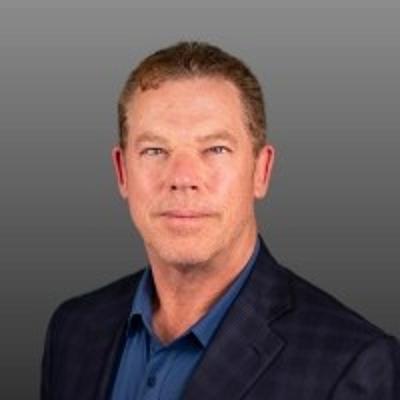
- Editorial Standards
- Reprints & Permissions
Goolee puzzles: brain boost!
Goolee sdn bhd, designed for ipad.
- Offers In-App Purchases
Screenshots
Description.
Relax and exercise your brain with challenging goolee puzzles. Unlock Your Inner Genius with Goolee: Elevate Your Problem-Solving Skills to New Heights! Are you ready to take your thinking to the next level? Introducing Goolee – the ultimate challenge for your mind. With Goolee's self-paced puzzles and games, you'll hone essential skills like goal-setting, analytical thinking, and decision-making. But here's the twist: Goolee isn't about beating others – it's about overcoming your own limits. Set your own goals, pace yourself, and watch as your problem-solving prowess reaches new heights. Remember, there's no cheating or shortcuts here – just pure, family-friendly fun. So dive in, challenge yourself, and let Goolee become your partner in high-order thinking. With each level conquered, you'll find yourself one step closer to mastering the art of problem-solving. And with our scoreboard tracking your progress, you'll always know where you stand. Are you ready to outsmart the toughest puzzles? Join Goolee today and unleash your inner genius!
Version 1.3.2
-In App Purchase -New Challenge
App Privacy
The developer, Goolee Sdn Bhd , indicated that the app’s privacy practices may include handling of data as described below. For more information, see the developer’s privacy policy .
Data Used to Track You
The following data may be used to track you across apps and websites owned by other companies:
- Financial Info
- Contact Info
- Identifiers
Data Linked to You
The following data may be collected and linked to your identity:
Privacy practices may vary, for example, based on the features you use or your age. Learn More
Information
- 100 DIAMONDS $0.99
- 1000 DIAMONDS $9.99
- 250 DIAMONDS $1.99
- 2500 COINS $1.99
- 5000 COINS $2.99
- 10 000 COINS $9.99
- 1000 COINS $0.99
- 500 DIAMONDS $2.99
- Developer Website
- App Support
- Privacy Policy
You Might Also Like
MonsterDisguise
Idle Rush Hour
Marble Shooter - Zumba Classic
Snakes & Ladders King
Go Game - 2 Players
Checkers 10x10
New discrete-time zeroing neural network for solving time-dependent linear equation with boundary constraint
- Open access
- Published: 13 May 2024
- Volume 57 , article number 140 , ( 2024 )
Cite this article
You have full access to this open access article
- Naimeng Cang 1 ,
- Feng Qiu 1 ,
- Shan Xue 1 , 2 ,
- Zehua Jia 1 ,
- Dongsheng Guo 1 ,
- Zhijun Zhang 3 &
- Weibing Li 4
Recently, continuous- and discrete-time models of a zeroing neural network (ZNN) have been developed to provide online solutions for the time-dependent linear equation (TDLE) with boundary constraint. This paper presents a novel approach to address the bound-constrained TDLE (BCTDLE) problem by proposing a new discrete-time ZNN (DTZNN) model. The proposed DTZNN model is designed using the Taylor difference formula to discretize the previous continuous-time ZNNN (CTZNN) model. Theoretical analysis indicates the computational property of the proposed DTZNN model, and numerical results further demonstrate its validity. The applicability of the proposed DTZNN model is finally confirmed via its application to the motion planning of a PUMA560 robotic arm.
Avoid common mistakes on your manuscript.
1 Introduction
Linear and nonlinear equations are fundamental mathematical tools that play essential roles in several industrial applications, such as robot path planning, image recognition, and signal processing (Zhao 2013 ; Tsiligianni et al. 2015 ; Zhang and Jin 2017 ; Li et al. 2019 ). Extensive work has been conducted on solving linear and nonlinear equations, typically using numerical algorithms (Sharma 2005 ; Neta et al. 2014 ; Abdelmalek 1977 ; Donoho et al. 2012 ; Zeng et al. 2014 ; Spedicato et al. 2000 ; Morigi and Sgallari 2001 ) and neurodynamic methods (Kumar 2022a , b ; Kumar 2023 ; Cichocki et al. 1992 ; Xia et al. 1999 ; Liang and Tso 2002 ). Among the numerical algorithms, direct methods and iterative methods are mainly used. In Abdelmalek ( 1977 ), the minimum \(L_{\infty }\) solution of the linear equation was first proposed. In Donoho et al. ( 2012 ), the sparse approximate solution of the linear equation was obtained by using the stagewise orthogonal matching pursuit algorithm. Many iterative algorithms have also been developed to bring solutions to the linear equation, such as adaptive iterative thresholding algorithms (Zeng et al. 2014 ), abstraction algorithms (Spedicato et al. 2000 ), and regularizing Lanczos iterative algorithms (Morigi and Sgallari 2001 ). However, these numerical algorithms may encounter challenges such as the accumulation of numerical errors, slow convergence rates, and low stability (Zhang et al. 2013 ).
In recent years, neural network models have garnered widespread attention for addressing modeling and control problems in both linear and nonlinear dynamic systems (Zhang et al. 2018 ; Li et al. 2019 ; Xiao et al. 2020 ; Liao et al. 2024 ; Kumar 2022a , b ; Kumar 2023 ). Particularly, the introduction of novel neural network models such as the Lyapunov-stability-based context-layered recurrent pi-sigma neural network (CLRPSNN) (Kumar 2022a ), the memory recurrent Elman neural network (MRENN) (Kumar 2022b ), and the higher-order recurrent neural network (HORNN) (Kumar 2023 ) have provided new approaches for solving the identification and control problems of nonlinear dynamic systems. The CLRPSNN model effectively addresses the issue of nonlinear system identification by introducing an additional layer of context nodes, demonstrating significant performance advantages in simulation results. However, stability has always been a crucial consideration in the design of neural network models. The MRENN and HORNN models innovate in stability design, ensuring stability and convergence of the models by combining Lyapunov stability criteria and recursive learning rate schemes. This emphasis on stability makes these models more reliable and practical when dealing with nonlinear dynamic systems.
Similar to numerical algorithms, neurodynamic methods also significantly improve the efficiency of solving the linear equation. Cichocki et al. ( 1992 ) proposed different recurrent neural networks (RNNs) to address the linear equation with an inequality constraint. Xia et al. ( 1999 ) designed an RNN model that can converge faster and provide more accurate solutions to the linear equation with an inequality constraint. The discrete-time form of such an RNN model was further deduced by Liang and Tso ( 2002 ). It is worth noting that these studies are based on the assumption of time-invariant when solving linear equations. However, many systems in practical engineering applications are always time-varying. Therefore, directly applying these methods to solve time-varying linear equations may yield poor results. Moreover, the mathematical domain of the variables involved in a linear equation must be set during the solution process (Zhang et al. 2018 ; Park et al. 1998 ). For example, it is assumed that many robotic arms can lead to task failure or even damage if the physical constraints of the joints are exceeded. Thus, appropriate methods must be investigated to ensure that joint angle, velocity, and/or acceleration are within the proper mathematical intervals (Guo et al. 2018 ; Zhang et al. 2013 ). In other words, solving time-varying underdetermined linear equations with boundary constraint is of importance in practical engineering applications.
In many industrial applications, there is a high demand for the real-time performance of linear equation, making them time-dependent. Existing studies are less concerned with methods for solving time-dependent linear equation (TDLE) with boundary constraint. To solve a given time-dependent problem, a representative RNN was presented and refined by Zhang and Guo ( 2015 ); Zhang and Yi ( 2011 ); Zhang et al. ( 2012 , 2015 ). The main idea of this model was to define the error control equation and then derive the RNN (depicted in an ordinary equation) so that the computational error can converge globally and eventually become 0, hence the name zeroing neural network (ZNN). Focusing on solving the bound-constrained TDLE (BCTDLE), Xu et al. ( 2019a ) developed the continuous-time ZNN (CTZNN) model, which they successfully employed to solve time-varying linear equations and inequality systems (Xu et al. 2019b ). Sun and Liu ( 2020 ) designed a novel noise-resistant CTZNN model for solving time-varying Lyapunov equations. However, directly implementing the CTZNN model into hardware in practical engineering applications is challenging.
Therefore, for the purpose of possible hardware implementation, researchers discretize the CTZNN model using difference equations. The traditional numerical differentiation algorithm for discretizing continuous systems is typically implemented using the Euler difference formula. Specifically, in Guo and Zhang ( 2012 ), a one-step iteration description of the DTZNN model is proposed for dynamic matrix inversion. The simulation results show that this model has an error mode of \(O({{\varepsilon }^{2}})\) , that is, when the sampling interval \(\varepsilon\) decreases by a factor of 10, the steady-state calculation error (SSCE) decreases by a factor of 100. Unlike the traditional Euler difference formula, in Guo et al. ( 2017 ), a new Taylor-type difference rule is constructed. For the approximation of first-order derivatives, this rule has been proven to have a smaller SSCE than the Euler difference rule and possesses an error mode of \(O({{\varepsilon }^{3}})\) , that is, when the sampling interval \(\varepsilon\) decreases by a factor of 10, the SSCE decreases by a factor of 1000. Using the new Taylor difference rule, researchers have successively developed Taylor difference formulas with the error modes \(O({{\varepsilon }^{4}})\) and \(O({{\varepsilon }^{5}})\) . Specifically, Huang et al. ( 2022 ) developed a Taylor difference formula with an error mode of \(O({{\varepsilon }^{4}})\) to solve time-variant underdetermined nonlinear systems under bound constraint. Different from the proposed DTZNN model in this paper, their model focuses on solving nonlinear systems and may not necessarily be applicable to linear equations, whereas DTZNN model proposed in this paper exhibits superior accuracy. Cai et al. ( 2021 ) also discretized the CTZNN model based on Taylor series expansion. However, their model mainly focuses on solving a time-varying system of linear equation and inequality, and does not consider boundary constraint. A recent study (Ma and Guo 2021 ) showed that the DTZNN model can effectively solve the BCTDLE with an SSCE of the order \(O(\varepsilon ^{4})\) , where \(\varepsilon\) is the sampling distance.
Following but differing from the previous work (Ma and Guo 2021 ), this paper establishes a new DTZNN model that can effectively solve the TDLE with boundary constraint. The validity of such a model is supported by both theoretical and numerical results. Considering the importance of robotic arms in industrial applications (Xu et al. 2019a ; Ma and Guo 2021 ; Zhang et al. 2019 ), this paper further applies the proposed DTZNN model to the PUMA560 robotic arm to demonstrate the applicability of the model. The primary contributions of this paper can be summarized as follows:
The new DTZNN model, which has not been previously reported, is studied to solve the TDLE with boundary constraint. Notably, the proposed model is clearly different from the previous DTZNN model (Ma and Guo 2021 ), and achieves better performance on computing and solving the BCTDLE.
Theoretical analysis denotes the computational characteristic of the proposed DTZNN model, and numerical results verify its validity. More importantly, these results show that the DTZNN calculation error at steady state is of order \(O(\varepsilon ^{5})\) . This is the first time that a computing model with \(O(\varepsilon ^{5})\) mode is presented to solve the BCTDLE.
The proposed DTZNN model is utilized for robotic arms by solving the linear kinematic equation that involves joint physical constraint. Simulation results using the PUMA560 robotic arm with different examples demonstrate the effectiveness and practicality of the proposed DTZNN model.
The upcoming sections of this paper are structured as follows. Section 2 presents the problem statement and the ZNN models for the TDLE with boundary constraint. Section 3 describes the proposed DTZNN composition and theoretical analysis. Section 4 provides numerical validation experiments with the proposed DTZNN model. Section 5 shows the applicability of the DTZNN model to robotic arms with joint constraints. Section 6 concludes this paper.
2 Preliminary
This section outlines the problem to address the TDLE with boundary constraint. To probe the matter further, the continuous- and discrete-time models of ZNN are shown as basis.
2.1 Problem statement
The TDLE with boundary constraint considered in this paper is formulated as follows (Lu et al. 2019 ; Xu et al. 2019a ):
where matrix \(G(t)\in R^{m\times n}\) (with \(m<n\) ) is time-dependent and full-rank, vector \(h(t)\in R^{m}\) is time-dependent and smooth, vector \(x(t)\in R^n\) is unknown and must be obtained by solving ( 1 ), and \(x^\pm\) is the bounds of x ( t ). This paper aims to find a viable x ( t ) that satisfies both the linear equation and the boundary constraint outlined in ( 1 ).
As shown in Xu et al. ( 2019b ), solving the BCTDLE ( 1 ) can be transformed into finding the solution of the system as follows:
with \(U=[-I;I]\in R^{2n\times n}\) , \(\vartheta =[-x^{-};x^{+}]\in R^{2n}\) , and \(V(t)=\text {diag}\{y_{1}(t),\cdots ,y_{2n}(t)\}\in R^{2n\times 2n}\) . \(I\in R^{n\times n}\) is the identity matrix, and y ( t ) is an unknown vector that must still be determined when solving ( 2 ). The matrix–vector form of ( 2 ) is then expressed as follows:
with \(Q(t)\in R^{(m+2n)\times (3n)}\) , \(w\left( t\right) \in R^{(3n)}\) , and \(r(t)\in R^{(m+2n)}\) being
In this way, solving the BCTDLE ( 1 ) is equivalent to solving the matrix–vector equation presented in ( 3 ) for \(t\geqslant 0\) .
2.2 CTZNN model
In this subsection, to solve the BCTDLE ( 1 ), following the ZNN design principle (Xu et al. 2019b ), the error equation \(e(t)\in R^{(m+2n)}\) is defined by
Then, a decay exponent formula is introduced to achieve the convergence of e ( t ) to 0 (Xu et al. 2019b ), and the resultant CTZNN model is derived and formulated as follows (Lu et al. 2019 ):
where vectors \(\dot{w}(t)\) and \(\dot{r}(t)\) denote the time derivatives of \(w\left( t\right)\) and \(r\left( t\right)\) , respectively, and matrices \(P(t)\in R^{(m+2n)\times (3n)}\) and \(M(t)\in R^{(m+2n)\times (3n)}\) are
with \(P^+(t)\in R^{(3n)\times (m+2n)}\) being the right pseudoinverse matrix of P ( t ). The following theoretical conclusion regarding the CTZNN model ( 5 ) is given and proved in Xu et al. ( 2019b ).
When considering a solvable BCTDLE ( 1 ), the CTZNN model ( 5 ) can produce an exact time-dependent solution of ( 1 ).
2.3 DTZNN model
The study of discrete-time models of ZNN is of practical interest because of hardware implementation and development of numerical algorithms (Guo et al. 2017 ; Mathews and Fink 2004 ). The widely-used method for deriving a discrete-time model is by using the Euler difference formula (Mathews and Fink 2004 ), which is given by
where \({w}_k={w}(t_k=k\varepsilon )\) , \(\varepsilon =t_{k+1}-t_k\) represents the sampling interval, \(k=0,1,2,\ldots\) is the number of iterations, and \(O(\varepsilon )\) is the truncation error.
Evidently, the discretization of the CTZNN model ( 5 ) via Euler difference formula ( 6 ) yields the following expression:
where \(P_k^+=P^+(t_k=k\varepsilon )\) , \(Q_k=Q(t_k=k\varepsilon )\) , \(M_k=M(t_k=k\varepsilon )\) , \({\dot{r}}_k={\dot{r}}(t_k=k\varepsilon )\) , \(r_k=r(t_k=k\varepsilon )\) , and \(\mu =\lambda \varepsilon >0\) is the step size.
By eliminating \(O(\varepsilon ^2)\) from ( 7 ), the following DTZNN model to solve the BCTDLE ( 1 ) is derived below:
In relation to the DTZNN model ( 7 ), it is a variation law with an \(O(\varepsilon ^2)\) error form. That is, when the sampling interval \(\varepsilon\) is reduced by a factor of 10, the stationary error is reduced by a factor of 100. Such a DTZNN model may not satisfy the high-precision requirement in practice. Thus, a new DTZNN model with better performance on computing and solving the BCTDLE ( 1 ) is proposed in this paper.
3 New DTZNN model
In this section, on the basis of the Taylor difference formula (Cai et al. 2021 ), the new DTZNN model is developed to solve the BCTDLE ( 1 ). Theoretical analysis is also provided to denote the computational characteristic of the proposed DTZNN model.
3.1 Model formulation
The approximation of a first order derivative via Taylor series expansion has been the subject of numerous studies (Zhang et al. 2019 ). In the previous study (Cai et al. 2021 ), the Taylor difference formula that has higher accuracy and lower steady-state error than the conventional Euler difference formula ( 6 ) has been constructed.
The following Taylor difference formula (Cai et al. 2021 ) is shown to approximate the first order derivative:
with \(k=6,7,8,\cdots\) and \(f(\cdot )\) being the objective function.
To discretize the CTZNN model ( 5 ) using the above Taylor difference formula ( 9 ), the following vector form of ( 9 ) is presented for the approximation of \(\dot{w}_{k}\) :
By applying the Taylor difference formula ( 10 ) to the discretization of the CTZNN model ( 5 ), the discrete-time expression is given by
By eliminating \(O(\varepsilon ^5)\) from ( 11 ), the DTZNN model proposed in this paper to solve the BCTDLE ( 1 ) is expressed as follows:
Regarding the proposed DTZNN model ( 12 ), it requires seven initial states (i.e., \(w_0\) , \(w_1\) , \(w_2\) , \(w_3\) , \(w_4\) , \(w_5\) and \(w_6\) ) to activate the iterative computation. Thus, given an initial value \(w_0\) , the rest are found by the DTZNN model ( 8 ) and are computed as follows:
The procedure of the proposed DTZNN model ( 12 ) to solve the BCTDLE problem ( 1 ) is as follows:
Initialization: Given the time duration T , sampling gap \(\varepsilon\) , step size \(\mu\) , constraint boundary \(\left[ -{{x}^{-}},{{x}^{+}} \right]\) . Initialize \({{t}_{0}}\) , \({{w}_{0}}\) , \({{G}_{0}}\) , \({{\dot{G}}_{0}}\) , \({{h}_{0}}\) , and \({{\dot{h}}_{0}}\) . Receive \(P_{0}^{+}\) , \({{r}_{0}}\) , \({{\dot{r}}_{0}}\) , \({{Q}_{0}}\) and \({{M}_{0}}\) . Compute \({{\left\| {{e}_{0}} \right\| }_{2}}={{\left\| {{Q}_{0}}{{w}_{0}}-{{r}_{0}} \right\| }_{2}}\) .
First Loop (with \(k = 0, 1, 2, 3, 4, 5\) ): Compute \({{w}_{k+1}}\) through ( 8 ). Receive \(P_{_{k+1}}^{+}\) , \({{r}_{_{k+1}}}\) , \({{\dot{r}}_{_{k+1}}}\) , \({{Q}_{_{k+1}}}\) and \({{M}_{_{k+1}}}\) . Compute \({{\left\| {{e}_{_{k+1}}} \right\| }_{2}}={{\left\| {{Q}_{_{k+1}}}{{w}_{_{k+1}}}-{{r}_{_{k+1}}} \right\| }_{2}}\) .
Second Loop (with \(k = 6, \ldots {}\) , int \((T) / \varepsilon )\) ): Compute \({{w}_{k+1}}\) through ( 12 ). Receive \(P_{_{k+1}}^{+}\) , \({{r}_{_{k+1}}}\) , \({{\dot{r}}_{_{k+1}}}\) , \({{Q}_{_{k+1}}}\) and \({{M}_{_{k+1}}}\) . Compute \({{\left\| {{e}_{_{k+1}}} \right\| }_{2}}={{\left\| {{Q}_{_{k+1}}}{{w}_{_{k+1}}}-{{r}_{_{k+1}}} \right\| }_{2}}\) .
Output: Save \({{w}_{k}}\) and \({{\left\| {{e}_{_{k}}} \right\| }_{2}}={{\left\| {{Q}_{_{k}}}{{w}_{_{k}}}-{{r}_{_{k}}} \right\| }_{2}}\) , and plot figures.
An important criterion for measuring the performance of numerical algorithms is computational complexity. For ( 12 ), the overall computational complexity is \(O((m+2n)\times (3n))\) (with \(m<n\in R\) ). Thus, the proposed DTZNN model ( 12 ) has low computational complexity, i.e., \(O(n^2)\) .
3.2 Theoretical analysis
In this subsection, the computational characteristic of the proposed DTZNN model ( 12 ) is analyzed and proved theoretically.
The proposed DTZNN model ( 12 ) is characterized by zero stability and consistency, and thus has convergence property.
By analyzing the characteristic polynomial, it has been determined that the proposed DTZNN model in Equation ( 12 ) exhibits zero stability (Guo et al. 2018 ). Then, from the derivation of ( 12 ), its truncation error is \(O({\varepsilon }^5)\) , reflecting its consistency. Referring to the findings of Griffiths and Higham ( 2010 ), the zero stability and consistency are essential for the convergence of the proposed DTZNN model ( 12 ). With that, the proof is now fully established. \(\square\)
The proposed DTZNN model ( 12 ) can produce a precise time-dependent solution of the solvable BCTDLE ( 1 ).
By following Lemma 3 , the solution \(w_k\) computed by the proposed DTZNN model ( 12 ) can converge to a theoretical solution \(w_k^*=w^*(t_k=k\varepsilon )\) of ( 3 ). Mathematically, \(w_k\rightarrow w_k^*\) if k is large enough (Huang et al. 2022 ). By virtue of \(Q_kw_k-r_k=0\) and the given definitions of \(Q_k\) and \(r_k\) , the following equation can be deduced:
\(\square\)
Knowing that \(V_{k} y_{k} \ge 0, U=[-I; I] \in R^{2 n \times n}, \text{ and } \vartheta =\left[ -x^{-}; x^{+}\right] \in R^{n \times n}\) , ( 13 ) is reformulated as stated below:
( 14 ) indicates that, when k is large enough, the DTZNN model ( 12 ) has \(x_k\rightarrow x_k^*(t_k=k\varepsilon )\) for the BCTDLE ( 1 ) to hold. This statement further shows that the proposed DTZNN model ( 12 ) can offer a precise time-dependent solution of the solvable BCTDLE ( 1 ). With that, the proof is now fully established. \(\square\)
Considering the BCTDLE ( 1 ) is solved by the proposed DTZNN model ( 12 ), the SSCE varies in the mode of \(O({\varepsilon }^5)\) .
Based on the error function ( 4 ), the SSCE of ( 12 ) is given by
According to Lemmas 3 and 4 , \(w_k=w_k^*+O({\varepsilon }^{5})\) with a large enough k . Then, the following result on the SSCE of ( 12 ) is obtained:
\(\square\) which means that the DTZNN calculation error at steady state is of order \(O(\varepsilon ^{5})\) . Therefore, considering the BCTDLE ( 1 ) is solved by the proposed DTZNN model ( 12 ), the SSCE varies in the mode of \(O({\varepsilon }^5)\) . With that, the proof is now fully established. \(\square\)
To summarize, Lemmas 3 – 5 provide theoretical guarantees that the proposed DTZNN model ( 12 ) can effectively solve the BCTDLE ( 1 ).
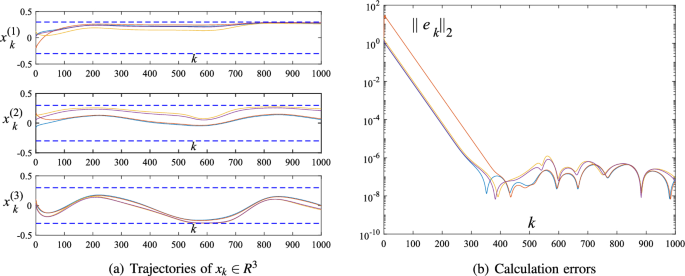
Numerical results for the previous DTZNN model ( 16 ) with \(\varepsilon =0.01\) and \(\mu =0.1\) to solve the BCTDLE ( 1 )
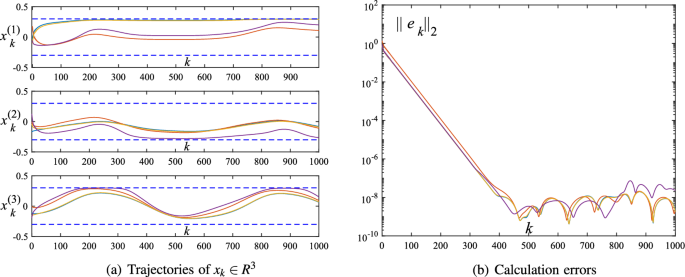
Numerical results for the proposed DTZNN model ( 12 ) with \(\varepsilon =0.01\) and \(\mu =0.1\) to solve the BCTDLE ( 1 )
4 Numerical verification and comparison
This section showcases numerical simulations to verify the superiority and effectiveness of the proposed DTZNN model ( 12 ) in solving the BCTDLE ( 1 ). Notably, the recent research (Guo et al. 2017 ) indicates that the DTZNN model via Taylor difference formula can outperform the DTZNN model ( 8 ) via Euler difference formula. Thus, this section only offers the comparative numerical results of using the proposed DTZNN model ( 12 ) and the previous DTZNN model in Guo et al. ( 2017 ). For convenience, the previous DTZNN model in Guo et al. ( 2017 ) is expressed as follows:
These simulations are also performed via MATLAB R2021b on a digital computer equipped with an AMD Ryzen 7 5800 H processor with Radeon Graphics @3.20 GHz, 32 GB of memory, and Windows 10 OS.
4.1 Model comparison
In this subsection, numerical simulation comparative experiments are conducted using the following example to demonstrate the effectiveness and superiority of the proposed DTZNN model ( 12 ) in solving BCTDLE ( 1 ). In the ensuing numerical experiments, the DTZNN models ( 12 ) and ( 16 ) are used to solve the BCTDLE ( 1 ) with the following coefficients:
The relevant numerical results are provided in Figs. 1 , 2 , 3 , 4 and Table 1 .
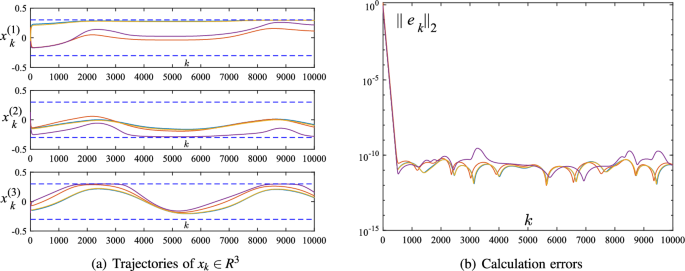
Numerical results for the previous DTZNN model ( 16 ) with \(\varepsilon =0.001\) and \(\mu =0.1\) to solve the BCTDLE ( 1 )
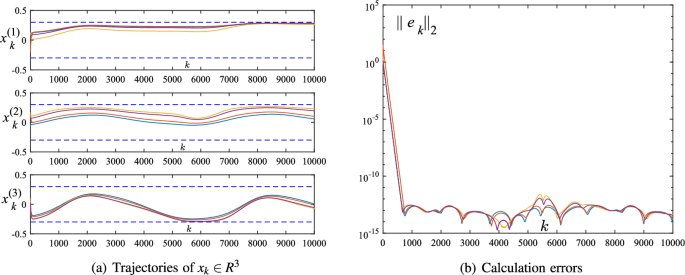
Numerical results for the proposed DTZNN model ( 12 ) with \(\varepsilon =0.001\) and \(\mu =0.1\) to solve the BCTDLE ( 1 )
Figure 1 presents the simulation results of the previous DTZNN model ( 16 ) using \(\varepsilon =0.01\) and \(\mu =0.1\) and four different initial values to solve the BCTDLE ( 1 ), where \(x_k^{(i)}(i=1,2,3)\) indicates the i th element of the feasible solution vector \(x_k\) of ( 1 ). In Fig. 1 a, the trajectories of \(x_k\) are time-dependent, and the changing values are within the constraint boundary \([x^-,x^+]\) . These results mean that the exact solution of ( 1 ) can be obtained by applying the previous DTZNN model ( 16 ). In Fig. 1 b, the calculation errors \(\Vert e_{k}\Vert _{2}\) of ( 16 ) converge, and the SSCEs are in the order of \({10}^{-6}\) . According to these results, the previous DTZNN model ( 16 ) can effectively solve the BCTDLE ( 1 ).
Figure 2 presents the simulation results of the proposed DTZNN model ( 12 ) using \(\varepsilon =0.01\) and \(\mu =0.1\) and four different initial values to solve the BCTDLE ( 1 ). In Fig. 2 a, the trajectories of \(x_k\) are time-dependent as well and numerically bounded (or say, they satisfy \(x_k\in [x^-,x^+]\) ). In Fig. 2 b, the calculation errors of ( 12 ) demonstrate convergence, with the SSCE values reaching the order of \({10}^{-8}\) . These findings provide strong evidence for the validity of ( 12 ) in solving ( 1 ). More importantly, comparing the results in Figs. 1 b and 2 b, the SSCE of the proposed model ( 12 ) is 100 times smaller than that of the previous model ( 16 ). This comparison indicates that ( 12 ) has smaller errors and higher accuracy than ( 16 ). Therefore, the proposed DTZNN model ( 12 ) offers greater advantages than the previous DTZNN model ( 16 ) in solving the TDLE with boundary constraint, i.e., ( 1 ).
By reducing the sampling interval \(\varepsilon\) by a factor of 10 and repeating the above numerical experiments, the corresponding results of using the DTZNN models ( 16 ) and ( 12 ) are shown in Figs. 3 and 4 , respectively. In such two figures, as computed by ( 16 ) or ( 12 ), the trajectories of \(x_k\) are time-dependent and satisfy \(x_k\in [x^-,x^+]\) . The related computational errors converge with the SSCE being small enough. These simulation results verify again the effectiveness of the DTZNN models ( 16 ) and ( 12 ) in solving the BCTDLE ( 1 ). In particular, comparing Figs. 3 b and 4 b, the SSCEs of ( 16 ) and ( 12 ) for solving ( 1 ) are \({10}^{-{10}}\) and \({10}^{-{13}}\) orders of magnitude, respectively. The latter is about 1000 times smaller than the former. Therefore, the proposed DTZNN model ( 12 ) can be more advantageous than the previous DTZNN model ( 16 ) in solving the BCTDLE ( 1 ).
For further investigation, the SSCEs of the DTZNN models ( 16 ) and ( 12 ) are compared and validated using different values of \(\varepsilon\) and \(\mu\) , with a fixed initial state of \(w_0=0.2\) and the same sampling time. The detailed data are provided in Table 1 , which demonstrates that the proposed model ( 12 ) is computationally better than the previous model ( 16 ). These results also verify that the calculation error variation mode of ( 12 ) is \(O(\varepsilon ^5)\) . Specifically, when \(\varepsilon =0.001\) , the SSCE can reach the order of \({10}^{-14}\) . In addition to this, the performance of the proposed DTZNN model ( 12 ) on computing and solving ( 1 ) can be improved by decreasing the sampling interval \(\varepsilon\) or increasing \(\mu\) in the appropriate range.
Overall, the simulation results presented in Figs. 1 , 2 , 3 , 4 and Table 1 confirm the superiority and efficacy of the proposed DTZNN model ( 12 ) in solving the BCTDLE ( 1 ) in comparison with the previous DTZNN model ( 16 ).
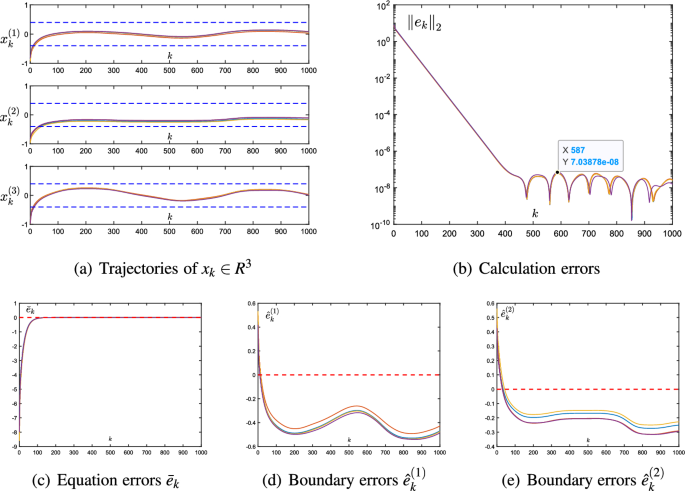
Calculation errors for the proposed DTZNN model ( 12 ) with different \(\varepsilon\) and \(\mu\) to solve the BCTDLE ( 1 )
4.2 Influence of \(\varepsilon\) and \(\mu\)
In the previous section, the effectiveness and superiority of the proposed DTZNN model ( 12 ) in solving the BCTDLE problem are demonstrated through simulation comparisons. The proposed DTZNN model ( 12 ), it has two adjustable parameters (i.e., \(\varepsilon\) and \(\mu\) ). Since different values of \(\varepsilon\) and \(\mu\) can have an effect on the solution effectiveness, this section delves into the influence of these two adjustable parameters on the proposed DTZNN model ( 12 ) using a new example. The time period for the simulation is set to \(T = 10\) s. The coefficient matrix and the constraint boundary of the BCTDLE ( 1 ) to be solved are given as follows:
To better demonstrate the effectiveness of the proposed DTZNN model ( 12 ) in solving BCTDLE ( 1 ), two additional computational errors are introduced: the equation error \({{\bar{e}}_{k}}=G(t)x(t)-h(t)\) and the constraint boundary error \({{\hat{e}}_{k}}=Ux(t)-\vartheta\) .
Figure 5 a clearly illustrates that the feasible solution of the BCTDLE ( 1 ) under the influence of the proposed DTZNN ( 12 ) model, undergoes rapid convergence from an initially out-of-boundary state and reaches the boundary constraint range. This result clearly validates the effectiveness and convergence properties of the proposed DTZNN model ( 12 ). Figure 5 b indicates that when the iteration count k is sufficiently large, the SSCE \({\left\| {{e}_{k}} \right\| }_{2}\) converges, and the maximum value is in the smaller order of magnitude of \({{10}^{-8}}\) after stabilization. This outcome suggests that the proposed DTZNN model ( 12 ) can effectively solve BCTDLE ( 1 ). From Fig. 5 c, it can be seen that with increasing iteration count k and after reaching stability, the equation error \({{\bar{e}}_{k}}\) converges to 0. The trend of the variation in \({\hat{e}}_{k}\) in Fig. 5 d and e also illustrates that under the influence of the proposed DTZNN model ( 12 ), \({x}_{k}\) transitions from initially exceeding the boundary to returning within the boundary constraint range. Therefore, in Fig. 5 a, \({x}_{k}\) is the exact solution of BCTDLE ( 1 ), confirming that the proposed DTZNN model ( 12 ) can effectively solve BCTDLE ( 1 ).
The above simulation results are obtained with the sampling interval \(\varepsilon = 0.01\) . To showcase the high precision characteristics of the proposed DTZNN model ( 12 ) and highlight the impact of the sampling interval on the solution effectiveness, the sampling interval is further reduced to \(\varepsilon = 0.001\) , while keeping other parameters constant. The relevant simulation results for solving BCTDLE ( 1 ) are presented below.
Figures 6 a, d, and e reveal that even with four different initial values exceeding the boundary constraints, \({x}_{^{k}}\) can rapidly converge within the boundary constraint range under the influence of the proposed DTZNN model ( 12 ). Comparing Fig. 5 b with Fig. 6 b, it is also noticeable that as the sampling interval \(\varepsilon\) decreases, the computational performance improves. Specifically, \((9.35241\times {{10}^{-13}})/(7.03878\times {{10}^{-8}})\approx 1.32870\times {{10}^{-5}}\) , indicating that the proposed DTZNN model ( 12 ) follows an \(O({{\varepsilon }^{5}})\) error model, that is, as the sampling interval \(\varepsilon\) is reduced by a factor of 10, the SSCE can be reduced by a factor of 100000. Therefore, it is possible to appropriately decrease the value of \(\varepsilon\) based on practical requirements to enhance computational accuracy and obtain more precise time-varying solutions.
In addition to the sampling interval \(\varepsilon\) , the proposed DTZNN model ( 12 ) has another adjustable parameter, \(\mu\) . To further explore the influnce of the two parameters on the proposed DTZNN model ( 12 ), six sets of simulation experiments are conducted. From Fig. 7 a, c, and e, it can be observed that with the sampling interval \(\varepsilon = 0.01\) , the SSCEs of the proposed DTZNN model ( 12 ) for solving BCTDLE ( 1 ) decreases as the step size \(\mu\) increases. This similar conclusion can be obtained from Fig. 7 b, d, and f. While the impact of increasing \(\mu\) on the SSCE is not as pronounced as decreasing \(\varepsilon\) , in practical situations, the sampling interval cannot be made arbitrarily small owing to some constraints. Therefore, when adjusting the sampling interval to the minimum achievable under practical conditions, increasing \(\mu\) can be employed to further reduce the SSCE and enhance precision.
By comparing the simulation results between the left and right of Fig. 7 , it can be observed that, with the step size \(\mu\) held constant, as the sampling interval \(\varepsilon\) decreases from 0.01 to 0.001, the SSCEs reduce from the order of \({10}^{-8}\) to \({10}^{-13}\) . This magnitude reduction once again confirms that the proposed DTZNN model ( 12 ) exhibits an error model of \(O({{\varepsilon }^{5}})\) . Therefore, the numerical simulation results above strongly demonstrate the effectiveness and superiority of the proposed DTZNN model ( 12 ) in solving BCTDLE ( 1 ).
It is worth noting that the main difference in this section compared to the previous one lies in the fact that the initial values exceed the boundary constraint. Therefore, the comparison of simulation results show that while keeping the sampling interval \(\varepsilon\) and the step size \(\mu\) constant, the SSCE in this section is reduced by a factor of 10 compared to the previous section. However, both sections still keep an \(O({{\varepsilon }^{5}})\) error model. Such a phenomenon occurs not only in the proposed DTZNN model ( 12 ) but also in the previous DTZNN model ( 16 ), where model accuracy is sacrificed to ensure regression to within the boundary constraint. However, the proposed DTZNN model has smaller calculation errors and demonstrates greater fault tolerance, thus making it more widely applicable in practical engineering scenarios.
5 DTZNN application to robotics
This section presents the application of the proposed DTZNN model ( 12 ) to the robotic arms with joint physical constraints to demonstrate the applicability of the model.
5.1 Motion planning of robotic arms
The motion planning of a robotic arm involves generating the joint trajectory \(\theta (t)\in R^n\) in real-time to accurately follow the desired Cartesian path \(r(t)\in R^m\) of the end-effector (Li et al. 2019 ).
In particular, taking into consideration the feedback and joint limits, the motion planning of robotic arms is achieved by efficiently solving the TDLE with boundary constraints as follows (Xu et al. 2019b ):
The above equation involves several key variables, including Jacobian matrix \(J(\theta (t))\in R^{m\times n}\) , joint velocity \(\dot{\theta }(t)\in R^n\) , time derivative of r ( t ) denoted as \(\dot{r}(t)\in R^m\) , the feedback parameter \(k>0\in R\) , and the differentiable nonlinear mapping function \(\phi (\cdot )\) . In addition, \(\theta ^\pm\) and \({\dot{\theta }}^\pm\) represent the limits of \(\theta (t)\) and \(\dot{\theta }(t)\) , respectively.
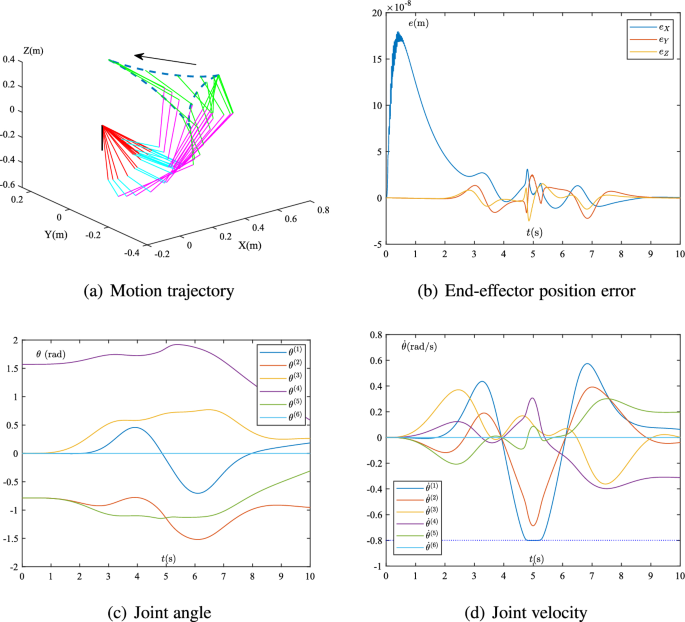
Simulation results of the motion planning scheme ( 19 ) with \(\varepsilon =0.01\) and \(\mu =0.1\) for PUMA560 robotic arm tracking the tricuspid path
It follows from (Zhang et al. 2004 ) that the boundary constraints in ( 17 ) are unified as
with \(\rho >0\in R\) . Then, the following reformulation of ( 17 ) is further obtained:
with \(\delta ^{-}=\max \{\rho (\theta ^{-}-\theta ), \dot{\theta }^{-}\}\) and \(\delta ^{+}=\min \{\rho (\theta ^{+}-\theta ), \dot{\theta }^{+}\}\) . At this point, solving ( 18 ) is the same as solving the BCTDLE ( 1 ), with the correlation coefficients as follows:
Moreover, the following augmented coefficient matrices and vectors are presented:
Therefore, the proposed DTZNN model ( 12 ) for the BCTDLE ( 1 ) can be introduced to solve ( 18 ) and ( 17 ), where \(w_k=[{\dot{\theta }}_k;y_k] \in R^{3n}\) . Notably, the Taylor difference formula ( 10 ) is also employed to calculate the joint angle \(\theta _k=\theta \left( t_k=k\varepsilon \right)\) at every instance. On the basis of ( 12 ), the following detailed formulation for realizing the motion planning of robotic arms with joint physical constraints is provided:
Hereafter, ( 19 ) is called the new motion planing scheme for physically-constrained robotic arms. With regard to such a motion planning scheme ( 19 ), seven initial values must still be determined to start the iterative computation. Similarly, given the initial joint angle \(\theta _{0}\) and \(w_{0}\) , the rest is obtained via the following iterative computation:
where \(i=0,1,\ldots ,6\) .
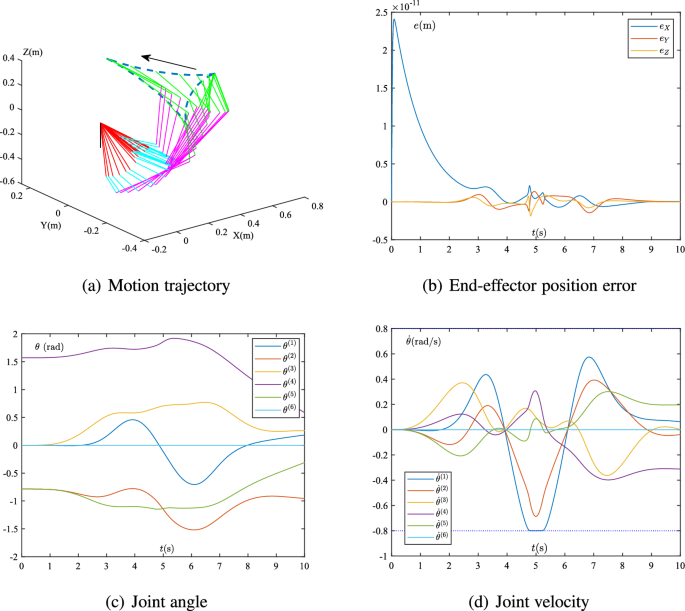
Simulation results of the motion planning scheme ( 19 ) with \(\varepsilon =0.001\) and \(\mu =0.1\) for PUMA560 robotic arm tracking the tricuspid path
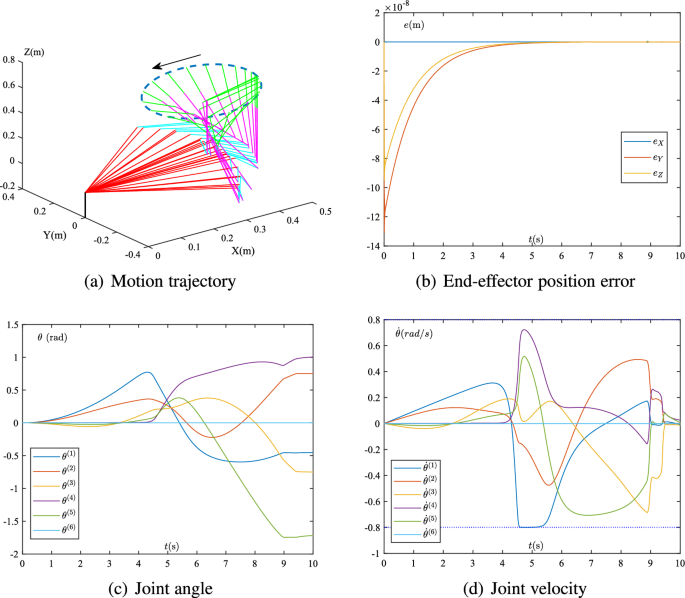
Simulation results of the motion planning scheme ( 19 ) with \(\varepsilon =0.01\) and \(\mu =0.1\) for PUMA560 robotic arm tracking the circular path
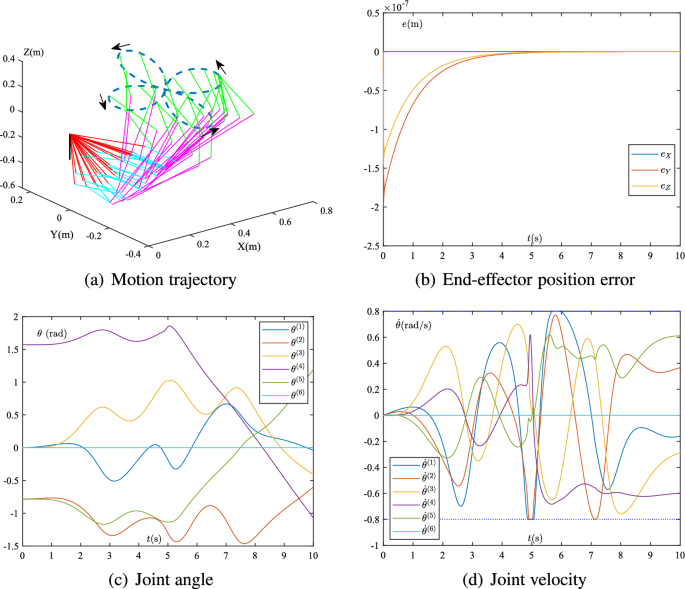
Simulation results of the motion planning scheme ( 19 ) with \(\varepsilon =0.01\) and \(\mu =0.1\) for PUMA560 robotic arm tracking the Rhodonea path
5.2 Simulation verification
To demonstrate the effectiveness of the new motion planning scheme ( 19 ), simulations are conducted using the PUMA560 robotic arm for a set desired path [i.e., the tricuspid path ( 20 ), circular path ( 21 ), and Rhodonea path ( 22 )]. In this way, the validity and practicality of the proposed DTZNN model ( 12 ) is thus confirmed.
In these simulations, the limits of the PUMA560 robotic arm are defined as
The desired end-effector position vectors for the tricuspid path, circular path, and Rhodonea path are respectively designed as
where \(\phi =2\pi {{\sin }^{2}}({\pi t}/{2T})\) , time \(t\in [0,T]\) , the parameter \(\alpha\) is constant, and r is the radius of the desired path. In addition, \(i_{x_0}\) , \(i_{y_0}\) and \(i_{z_0}\) respectively denote the X-axis, Y-axis and Z-axis components of the initial position vector of the end-effector.
To better show the simulation effect, the PUMA560 end-effector path tracking periods are all set to \(T=10s\) , and the initial joint configuration is set to \(\theta _0=[0;0;0;0;0;0]\) rad. The related simulation results are depicted in Figs. 8 , 9 , 10 , 11 , where \(t\in \left\{ 0,\varepsilon ,2\varepsilon ,\ldots ,10\right\}\) , \(e=\phi (\theta \left( t\right) )-r\left( t\right) \in R^3\) , and \({\dot{\theta }}^{(i)}\) and \(\theta ^{(i)} (i=1,2,\cdots ,6)\) represent the i th element of \(\dot{\theta }(t)\) and \(\theta (t)\) .
Figure 8 presents the results of the new motion planning scheme ( 19 ) with \(\varepsilon =0.01\) and \(\mu =0.1\) for the PUMA560 robotic arm tracking the tricuspid path. In Figs. 8 a and b, it can be observed that the PUMA560 end-effector trajectory closely follows the desired tricuspid path, and the maximum positioning error is \({6.11775\times 10}^{-7}\) m. In particular, Fig. 8 c denotes that the value of joint angle \(\theta\) calculated by ( 19 ) remains within its limits. In Fig. 8 d, the joint velocity \(\dot{\theta }\) stays within the limits even after the lower limit is reached. That is, \({\dot{\theta }}^-\le \dot{\theta }(t)\le {\dot{\theta }}^+\) and \(\theta ^-\le \theta (t)\le \theta ^+\) are satisfied. These simulation results demonstrate that the new scheme ( 19 ) can effectively implement the motion planning of the PUMA560 robotic arm in the presence of joint physical constraints and further verify the practical application of the proposed DTZNN model ( 12 ).
To further present the high accuracy characteristics of the new motion planning scheme ( 19 ), the simulation is repeated with the sampling interval \(\varepsilon\) reduced by a factor of 10 (i.e., from \(\varepsilon =0.01\) to \(\varepsilon =0.001\) ) and the rest of the condition parameters unchanged. The first two subfigures of Fig. 8 show that the PUMA560 end-effector successfully tracks the desired path again, with the maximum error is \({1.34149\times 10}^{-11}\) m. The rest of the subfigures of Figs. 8 c and d denote that joint angle \(\theta\) and joint velocity \(\dot{\theta }\) also satisfy \({\dot{\theta }}^-\le \dot{\theta }(t)\le {\dot{\theta }}^+\) and \(\theta ^-\le \theta (t)\le \theta ^+\) , respectively, thus demonstrating the effectiveness of the new motion planning scheme ( 19 ). Notably, the PUMA560 end-effector positioning error decreases by a factor of 10,000 as the sampling interval \(\varepsilon\) decreases, that is, \({(1.34149\times 10}^{-11})/{(6.11775\times 10}^{-7})\approx 2.19278\times {10}^{-5}\) . This finding underscores the importance of the sampling interval \(\varepsilon\) for the new motion planning scheme ( 19 ) and reflects an \(O(\varepsilon ^5)\) error variation pattern. Therefore, in practical applications, \(\varepsilon\) in the new motion planning scheme ( 19 ) should be set small enough to ensure the high planning accuracy required for robotic arms.
For further investigation, the tracking path of the PUMA560 end-effector is set to a circular path and a Rhodonea path for the new motion planning scheme ( 19 ) with \(\varepsilon =0.001\) and \(\mu =0.1\) . Figures 10 and 11 show the corresponding simulation results, which fully demonstrate the validity of the new motion planning scheme ( 19 ). In particular, the end-effector tracking trajectory closely follows the desired path, and the corresponding maximum error is of the order of \({10}^{-10}\) m or \({10}^{-11}\) m. Joint velocity \(\dot{\theta }\) and the joint angle \(\theta\) obtained through the new scheme ( 19 ) also remain within their respective limits, ensuring that they satisfy the conditions \({\dot{\theta }}^-\le \dot{\theta }(t)\le {\dot{\theta }}^+\) and \(\theta ^-\le \theta (t)\le \theta ^+\) , respectively.
In summary, the simulation results provided in Figs. 8 , 9 , 10 , 11 confirm the validity of the new motion planning scheme ( 19 ) for the PUMA560 robotic arm. Furthermore, these results underscore the effectiveness and practicality of the proposed DTZNN model ( 12 ).
6 Conclusion
In this paper, utilizing the Taylor difference formula ( 9 ) to discretize the CTZNN model ( 5 ), the new DTZNN model ( 12 ) is proposed and studied to address the BCTDLE ( 1 ). Theoretical analysis demonstrates that the proposed DTZNN model ( 12 ) has convergence property and can generate the exact time-dependent solution of ( 1 ). Numerical results indicate the validity and superiority of the proposed DTZNN model ( 12 ) and further point to the SSCE variation being of the \(O(\varepsilon ^5)\) mode. Such a DTZNN model is finally applied to robotic arms, and the related new motion planning scheme ( 19 ) is derived. Simulation results obtained from the PUMA560 robotic arm denote the validity and reliability of the new motion planning scheme ( 19 ) for different desired path tracking examples. The applicability of the proposed DTZNN model ( 12 ) is confirmed as well.
One future research directions involves utilizing the proposed DTZNN model ( 12 ) to solve the BCTDLE ( 1 ) in a noisy environment. Another direction is to explore the application of the proposed DTZNN model ( 12 ) in other tasks of redundant robot manipulators, such as repetitive motions and obstacle avoidance. As a continuation of this paper, further efforts will focus on designing and selecting different activation functions to enhance the robustness of the proposed DTZNN model ( 12 ).
Code or data availability
The code or data were available in this manuscript.
Abdelmalek NN (1977) Minimum L ∞ solution of underdetermined systems of linear equations. J Approx Theory 20(1):57–69
Article MathSciNet Google Scholar
Cichocki A, Ramirez-Angulo J, Unbehauen R (1992) Architectures for analog VLSI implementation of neural networks for solving linear equations with inequality constraints. Proc IEEE Int Symp Circuits Syst 3:1529–1532
Google Scholar
Donoho DL, Tsaig Y, Drori I, Starck JL (2012) Sarse solution of underdetermined systems of linear equations by stagewise orthogonal matching pursuit. IEEE Trans Inf Theory 58(2):1094–1121
Article Google Scholar
Griffiths DF, Higham DJ (2010) Numerical methods for ordinary differential equations initial value problems. Springer, London
Book Google Scholar
Guo D, Zhang Y (2012) Zhang neural network, Getz-Marsden dynamic system, and discrete-time algorithms for time-varying matrix inversion with application to robots’ kinematic control. Neurocomputing 97:22–32
Guo D, Nie Z, Yan L (2017) Novel discrete-time Zhang neural network for time-varying matrix inversion. IEEE Trans Syst Man Cybern: Syst 47(8):2301–2310
Guo D, Li K, Liao B (2018) Bi-criteria minimization with MWVN-INAM type for motion planning and control of redundant robot manipulators. Robotica 36(5):655–675
Guo D, Xu F, Li Z, Nie Z, Shao H (2018) Design, verification, and application of new discrete-time recurrent neural network for dynamic nonlinear equations solving. IEEE Trans Ind Informat 14(9):3936–3945
Huang S, Ma Z, Yu S, Han Y (2022) New discrete-time zeroing neural network for solving time-variant underdetermined nonlinear systems under bound constraint. Neurocomputing 487:214–227
Kumar R (2022) A Lyapunov-stability-based context-layered recurrent pi-sigma neural network for the identification of nonlinear systems. Appl Soft Comput 122:108836
Kumar R (2022) Memory recurrent Elman neural network-based identification of time-delayed nonlinear dynamical system. IEEE Trans Syst Man Cybern: Syst 53(2):753–762
Kumar R (2023) Double internal loop higher-order recurrent neural network-based adaptive control of the nonlinear dynamical system. Soft Comput 27:17313–17331
Li S, Jin L, Mirza MA (2019) Kinematic control of redundant robot arms using neural networks. Wiley, Hoboken
Li W, Xiao L, Liao B (2019) A finite-time convergent and noise-rejection recurrent neural network and its discretization for dynamic nonlinear equations solving. IEEE Trans Cybern 50(7):3195–3207
Liang XB, Tso SK (2002) Improved upper bound on step-size parameters of discrete-time recurrent neural networks for linear inequality and equation system. IEEE Trans Circuits Syst I Fundam Theory Appl 49(5):695–698
Liao B, Han L, Cao X, Li S, Li J (2024) Double integral?enhanced Zeroing neural network with linear noise rejection for time? Varying matrix inverse. CAAI Trans Intell Technol 9(1):197–210
Lu H, Jin L, Luo X, Liao B, Guo D, Xiao L (2019) RNN for solving perturbed time-varying underdetermined linear system with double bound limits on residual errors and state variables. IEEE Trans Indus Inf 15(11):5931–5942
Ma Z, Guo D (2021) Discrete-time recurrent neural network for solving bound-constrained time-varying underdetermined linear system. IEEE Trans Ind Informat 17(6):3869–3878
Mathews JH, Fink KD (2004) Numerical methods using MATLAB, 4th edn. Prentice-Hall, Upper Saddle River
Morigi S, Sgallari F (2001) A regularizing L-curve Lanczos method for underdetermined linear systems. Appl Math Comput 121(1):55–73
MathSciNet Google Scholar
Neta B, Chun C, Scott M (2014) Basins of attraction for optimal eighth order methods to find simple roots of nonlinear equations. Appl Math Comput 227:567–592
Park K, Chang P, Kim S (1998) The enhanced compact QP method for redundant manipulators using practical inequality constraints. Proc IEEE Int Conf Robot Autom 1:107–114
Sharma JR (2005) A composite third order Newton–Steffensen method for solving nonlinear equations. Appl Math Comput 169(1):242–246
Spedicato E, Xia Z, Zhang L (2000) ABS algorithms for linear equations and optimization. J Comput Appl Math 124(1/2):155–170
Sun M, Liu J (2020) A novel noise-tolerant Zhang neural network for time-varying Lyapunov equation. Adv Diff Equ 1:1–15
Tsiligianni E, Kondi LP, Katsaggelos AK (2015) Preconditioning for underdetermined linear systems with sparse solutions. IEEE Signal Process Lett 22(9):1239–1243
Xia Y, Wang J, Hung DL (1999) Recurrent neural networks for solving linear inequalities and equations. IEEE Trans Circuits Syst I: Fundam Theory Appl 46(4):452–462
Xiao L, Dai J, Lu R, Li S, Li J, Wang S (2020) Design and comprehensive analysis of a noise-tolerant ZNN model with limited-time convergence for time-dependent nonlinear minimization. IEEE Trans Neural Netw Learn Syst 31(12):5339–5348
Xu F, Li Z, Nie Z, Shao H, Guo D (2019) New recurrent neural network for online solution of time-dependent underdetermined linear system with bound constraint. IEEE Trans Ind Informat 15(4):2167–2176
Xu F, Li Z, Nie Z, Shao H, Guo D (2019) Zeroing neural network for solving time-varying linear equation and inequality systems. IEEE Trans Neural Netw Learn Syst 30(8):2346–2357
Zeng J, Lin S, Xu Z (2014) Sparse solution of underdetermined linear equations via adaptively iterative thresholding. Signal Process 97:152–161
Zhang Y, Guo D (2015) Zhang functions and various models. Springer, Germany
Zhang Y, Jin L (2017) Robot manipulator redundancy resolution. Wiley, Hoboken
Zhang Y, Yi C (2011) Zhang neural networks and neural-dynamic method. Nova, New York
Zhang Y, Ge SS, Lee TH (2004) A unified quadratic-programming-based dynamical system approach to joint torque optimization of physically constrained redundant manipulators. IEEE Trans on Syst Man Cybern 34(5):2126–2132
Zhang Y, Li W, Guo D, Ke Z (2013) Different Zhang functions leading to different ZNN models illustrated via time-varying matrix square roots finding. Expert Syst Appl 40(11):4393–4403
Zhang Y, Wang Y, Jin L (2013) Different ZFs leading to various ZNN models illustrated via online solution of time-varying underdetermined systems of linear equations with robotic application. Lect Notes Comput Sci 7952:481–488
Zhang Z, Zheng L, Li L, Deng X, Xiao L, Huang G (2018) A new finite-time varying-parameter convergent-differential neural-network for solving nonlinear and nonconvex optimization problems. Neurocomputing 319:74–83
Zhang Y, Li S, Gui J, Luo X (2018) Velocity-level control with compliance to acceleration-level constraints: a novel scheme for manipulator redundancy resolution. IEEE Trans Ind Informat 14(3):921–930
Zhang Y, Yang M, Huang H, Xiao M, Hu H (2019) New discrete solution model for solving future different-level linear inequality and equality with robot manipulator control. IEEE Trans Ind Informat 15(4):1975–1984
Zhao YB (2013) New and improved conditions for uniqueness of sparsest solutions of underdetermined linear systems. Appl Math Comput 224:58–73
Zhang Y, Shi Y, Xiao L, Mu B (2012) Convergence and stability results of Zhang neural network solving systems of time-varying nonlinear equations. Proc 8th Int Conf Natural Comput 143–147
Zhang Y, Qiu H, Peng C, Shi Y, Tan H (2015) Simply and effectively proved square characteristics of discrete-time zd solving systems of time-varying nonlinear equations. Proc IEEE Int Conf Robot Autom 1457–1462
Cai J, Feng Q, Guo D (2021) New discrete-time zeroing neural network for solving time-varying system of linear equation and inequality. Proc 33rd Chinese Control Decision Conf 3806–3811
Download references
Acknowledgements
The authors would like to thank the editors and reviewers for their time and effort in evaluating this paper and for the constructive comments for the improvement of its presentation and quality.
This work is partly supported by Scientific Research Fund of Hainan University under Grant (KYQD(ZR)23025), Shenzhen Science and Technology Program under Grant (JCYJ20230807093513027), the National Science and Technology Major Project (Grant No. 2022ZD0119900), Shanghai Science and Technology program (Grant No. 22015810300), Hainan Province Science and Technology Special Fund (Grant No. ZDYF2024GXJS003), the Hainan Provincial Natural Science Foundation of China (Grant No. 620QN284), National Natural Science Foundation (Grant Nos. 61976096 and 62373157), and National High-Level Talents Special Support Program (Youth Talent of Technological Innovation of Ten-Thousands Talents Program) (Grant No. C7220060).
Author information
Authors and affiliations.
School of Information and Communication Engineering, Hainan University, Haikou, China
Naimeng Cang, Feng Qiu, Shan Xue, Zehua Jia & Dongsheng Guo
School of System Design and Intelligent Manufacturing, South China University of Technology, Shenzhen, China
School of Automation Science and Engineering, South China University of Technology, Guangzhou, China
Zhijun Zhang
School of Computer Science and Engineering, Sun Yat-sen University, Guangzhou, China
You can also search for this author in PubMed Google Scholar
Contributions
All authors contributed to the study conception and design. Material preparation, data collection and analysis were performed by Naimeng Cang, Dongsheng Guo and Shan Xue. The first draft of the manuscript was written by Naimeng Cang and all authors commented on previous versions of the manuscript. All authors read and approved the final manuscript.
Corresponding author
Correspondence to Dongsheng Guo .
Ethics declarations
Conflict of interest.
The authors have no relevant financial or non-financial interests to disclose
Ethical approval
This paper does not contain any studies with human participants or animals performed by any of the authors.
Consent to participate
Informed consent was obtained from all individual participants included in the study.
Consent for publication
The authors affirm that human research participants provided informed consent for publication of the images in all figures.
Additional information
Publisher's note.
Springer Nature remains neutral with regard to jurisdictional claims in published maps and institutional affiliations.
Rights and permissions
Open Access This article is licensed under a Creative Commons Attribution 4.0 International License, which permits use, sharing, adaptation, distribution and reproduction in any medium or format, as long as you give appropriate credit to the original author(s) and the source, provide a link to the Creative Commons licence, and indicate if changes were made. The images or other third party material in this article are included in the article’s Creative Commons licence, unless indicated otherwise in a credit line to the material. If material is not included in the article’s Creative Commons licence and your intended use is not permitted by statutory regulation or exceeds the permitted use, you will need to obtain permission directly from the copyright holder. To view a copy of this licence, visit http://creativecommons.org/licenses/by/4.0/ .
Reprints and permissions
About this article
Cang, N., Qiu, F., Xue, S. et al. New discrete-time zeroing neural network for solving time-dependent linear equation with boundary constraint. Artif Intell Rev 57 , 140 (2024). https://doi.org/10.1007/s10462-024-10746-x
Download citation
Accepted : 06 March 2024
Published : 13 May 2024
DOI : https://doi.org/10.1007/s10462-024-10746-x
Share this article
Anyone you share the following link with will be able to read this content:
Sorry, a shareable link is not currently available for this article.
Provided by the Springer Nature SharedIt content-sharing initiative
- Discrete-time zeroing neural network
- Time-dependent linear equation
- Boundary constraint
- Taylor difference formula
- Robotic arm
- Find a journal
- Publish with us
- Track your research
- SI SWIMSUIT
- SI SPORTSBOOK
- BEARDIGEST+
Can Bears Supporting Coaching Cast Give Caleb Williams a Chance?
Gene chamberlain | may 12, 2024.
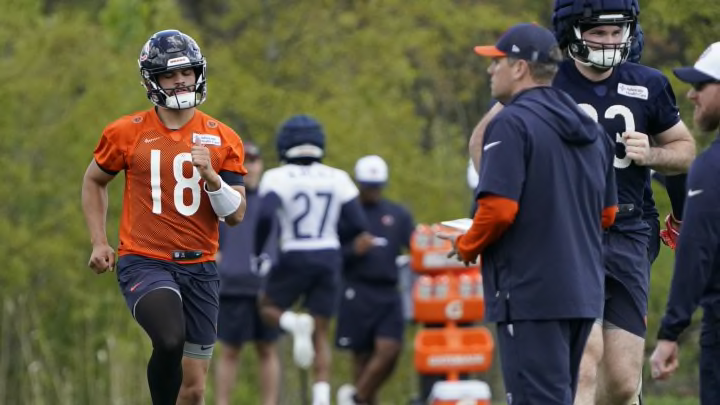
There is the popular narrative about how the Bears flopped at trying to develop their last two first-round quarterbacks, Justin Fields and Mitchell Trubisky.
Then there is the great fear they're about to do the same with a group of relatively inexperienced assistant coaches under offensive coordinator Shane Waldron, who hasn't exactly had experience developing QBs at the root level in the NFL.
Fields and Trubisky had varying degrees of supporting experience with coaching staffs, but both lacked necessary offensive talent support in various ways.
What is definitely true is Trubisky never left Chicago and suddenly had his talent start showing through to the point where he started playing much better in another system when given a chance. He has more interceptions than TD passes, a far worse quarterback rating and much lower yards per pass attempt since leaving. Meanwhile, his completion percentage has been about the same.
Time will tell with Fields, who brought the Bears back only a conditional sixth-round pick in the trade with Pittsburgh.
The important aspect of all this is how it pertains to the new quarterback, Caleb Williams.
Can the Bears get this one right? Do they have the right plan and supporting staff?
Five Guys pic.twitter.com/1RRtJR4jkj — Chicago Bears (@ChicagoBears) May 11, 2024
For comparison's sake, they've got more experience on the staff at working with quarterbacks than C.J. Stroud had on the staff of offensive coordinator Bobby Slowik.
BEST SUPPORTING CAST EVER FOR A NO. 1 OVERALL QB IN THE NFL
WRAPPING UP BEARS ROOKIE MINICAMP
INJURIES CAST A SHADOW OVER BEARS ROOKIE MINICAMP
ROME ODUNZE CONFIRMS RACE TO 1,000 YARDS IS ON
It's obvious GM Ryan Poles surrounded Williams with necessary talent. No quarterback drafted first overall ever came to a team with three wide receivers the caliber of DJ Moore, Keenan Allen and Rome Odunze, and with tight ends and backs who can help in the passing game.
The question is whether he has the supporting coaching staff to succeed.
Team Williams
Waldron has three years experience at his job, which is more than most new OCs will have.
Can Bears supporting coaching fast give Caleb Williams a chance to develop? https://t.co/NwfS3SWAeC pic.twitter.com/C5zc9np9jk — BearDigest (@BearsOnMaven) May 13, 2024
"For me, also being able to lean on (QB coach) Kerry Joseph and the rest of the offensive staff, to me this is always a collaborative effort and it's going to take all of us to help him along the way and everyone has great individual strengths that they can bring to the table," Waldron said. "So for me, it's helping him with the big picture of the game, understanding the offense, understanding situational football and then Kerry being a great technician and making sure we're always working on the fundamentals and that belief in the basics.
"And then Thomas with the passing game and everything he can bring there and his prior experiences to lean on. So it's a collaborative effort, we'll all work at it together."
Waldron never really developed Jared Goff with the Rams. That happened when he was coaching other positions. But he does great credit for turning around the career of an older QB, Seattle's Geno Smith.
Joseph, a CFL QB with two years experience as an assistant quarterback coach, calls Waldon the right kind of coordinator to lead this development project because of his adaptability.
"He's been around different quarterbacks," Joseph said. "Shane has been around Goff. Even before the Rams, he's been around other quarterbacks. And then he comes to Seattle, he has Russell Wilson, then he has Geno Smith, Drew Lock.
"So he's been able to adjust to the guys on the field and I think that’s very important."
The ability to adapt as a coach is one thing coach Matt Eberflus stressed when looking for a coordinator.
"That's what coaching is about and that's one thing that I love about working with Shane, because I'm an extension of him, of getting that information into that quarterback room," Joseph said.
most expensive WR rooms in 2024: 1. SF - $55.0M 2. SEA - $54.7M 3. DAL - $51.2M 4. MIA - $48.8M 5. CHI - $45.6M 6. LV - $42.1M 7. LAR - $40.9M 8. TB - $40.9M 9. TEN - $40.5M 10. CIN - $39.3M 11. JAX - $39.0M 12. CLE - $38.9M 13. WAS - $38.6M 14. DEN - $35.9M 15. MIN - $34.6M 16.… — Warren Sharp (@SharpFootball) May 12, 2024
Experience with a No. 1
Passing game coordinator Thomas Brown has one year experience as an offensive coordinator last year in Carolina, working with the No. 1 pick in the draft, Bryce Young. Prior to that, he worked on the Rams staff with Waldron but never was a quarterbacks guru. His expertise was with running backs.
The experience with Young last year is being counted on heavily in his work with Williams.
"We talked about the experience last year, being able to deal with the No. 1 overall draft pick but also a rookie and some of the highs and lows about that," Brown said. "But also, just my familiarity working with Shane, being with him in LA before in 2020.
"To bring that prior knowledge, plus my own kinda spin of things as far as the attention and process of it, be more behind the scenes, letting he and Kerry kinda take charge."
Brown also had a little past experience with Williams himself. He recruited former USC running back MarShawn Lloyd while coaching at South Carolina.
"I think Caleb was a freshman or a sophomore (in high school)," Brown said. "I was at DeMatha recruiting MarShawn and someone mentioned a quarterback’s doing pretty good at a school down the road (Gonzaga).
"I hopped in my car and drove over and just laid eyes on him for a quick second, knowing I probably wouldn't have a shot down the road because I was planning on moving on from college football before he graduated from high school."
Throwing Numbers at the Problem
It's not just those three coaches involved with Williams, either.
They have offensive assistant/quarterbacks and receivers coaches Ryan Griffin and Robbie Picazo. Both have the same title. Griffin hasn't coached before and was still under contract as a quarterback for the Skorpions Varese of the Italian Football League.
Picazo came with Waldron from the Seahawks, where he had been one year after coaching for a year with Houston. In neither place was Picazo a quarterbacks coach, but he has coaching experience in college back to 2013 working with quarterbacks, running backs and receivers.
By Comparison
The group assembled by the Bears is not the most inexperienced to support a rookie quarterback. Nor is it the most experienced.
In fact, among the six QBs drafted in the top 12 this year, it might be the third most experienced in terms of dealing specifically with quarterbacks.
King and Queen. pic.twitter.com/C2djVWNkJs — Bears History (@ChiBearsHistory) May 12, 2024
- In Washington, defensive-side head coach Dan Quinn has Kliff Kingsbury as offensive coordinator and as a former head coach in the NFL and at Texas Tech to work with Jared Daniels. Kingsbury's experience with QBs can't be questioned. It's a good thing he is so experienced because he's backed by Tavita Pritchard as quarterback coach and he hasn't done this before in the NFL. Also, his assistant quarterbacks coach is David Blough, who has been an NFL backup and not coaching until now. Passing game coordinator Brian Johnson was a quarterbacks coach two years with the Eagles and an offensive coordinator in the past both in college and the NFL for one year in Philadelphia.
- New England defensive-side head coach Jerod Mayo has Alex Van Pelt as offensive coordinator. He has been an offensive coordinator and QB coach for the Browns, a QB coach with the Packers and Buccaneers and Buffalo. Their QB coach is Tim McCartney, who has one year of QB coaching experience with Denver in 2019. Also, they have former Giants head coach Ben McAdoo, who has been an offensive coordinator with Carolina and quarterbacks coach with Jacksonville.
- Atlanta QB Michael Penix Jr. has the advantage of watching while Kirk Cousins plays, but he's not supposed to develop immediately. Head coach Raheem Morris has been a head coach before but has more of a defensive background than offensive. His offensive coordinator is Zac Robinson, the former passing game coordinator and assistant QB coach with the Rams who is getting his first chance as an OC. QB coach is TJ Yates, the former NFL backup who was an assistant quarterbacks coach with Houston in 2020. One big asset they have is veteran offensive coach Ken Zampese, who has been with numerous teams and helped with QB developement.
- Vikings coach Kevin O'Connell hasn't developed a quarterback yet, either, although he is a former offensive coordinator and that helps when dealing with J.J. McCarthy. His OC, Wes Phillips, has been with the team since 2022 and has plenty of time to focus on QB development because O'Connell's expertise is in play calling. Their quarterback coach is inexperienced Josh McCown, who was a Panthers assistant last year but has plenty of experience as a player. They also have an assistant quarterback coach in Grant Udinski and passing game coordinator Brian Angelichio on a staff very well supported for a young player.
- Denver has the most experience, although it's debatable whether they've actually succesfully developed any QB since Drew Brees almost two decades ago. Sean Payton was said to be a QB whisperer in New Orleans but it was mainly because he had Brees. He hasn't had success with Russell Wilson, that's for sure. Also on their staff is Joe Lombardi as offensive coordinator, a long-time assistant and coordinator for Payton. The quarterbacks coach, Davis Webb, is lacking much experience with only last year on the job. Passing game coordinator John Morton has more background as a receiver scoach. However, they have the experience of Pete Carmichael on staf as a senior offensive assistant after spending 14 years with the Saints as a coordinator and QB coach.
The bottom line will be how Waldron leads this group of relatively inexperienced assistants and whether Williams pays enough attention to fundamentals to make it all work.
"I think for me, the things we’re pouring into him right now is just the understanding of the big picture of the game and all the intricacies and the nuances of, first of all, between college and the NFL and being able to start with that ground floor approach and build that repertoire of his up as we’re going," Waldron said.
It's going to be a process and they've barely begun.
Happy Mother’s Day to Mama Bear herself 🐻⬇️ pic.twitter.com/I21VNn91Xl — Just Another Year Chicago: Bears (@OfficialJAYCHI) May 12, 2024
Twitter: BearDigest@BearsOnMaven
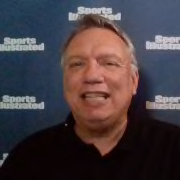
GENE CHAMBERLAIN
BearDigest.com publisher Gene Chamberlain has covered the Chicago Bears full time as a beat writer since 1994 and prior to this on a part-time basis for 10 years. He covered the Bears as a beat writer for Suburban Chicago Newspapers, the Daily Southtown, Copley News Service and has been a contributor for the Daily Herald, the Associated Press, Bear Report, CBS Sports.com and The Sporting News. He also has worked a prep sports writer for Tribune Newspapers and Sun-Times newspapers.
Follow BearsOnMaven
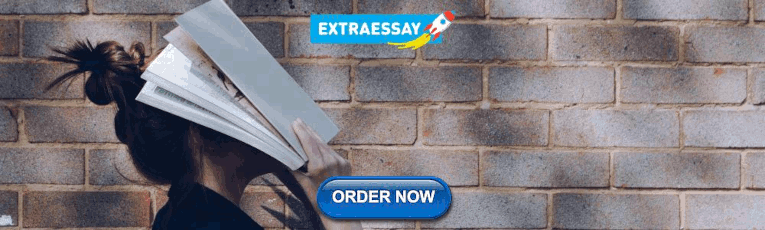
IMAGES
VIDEO
COMMENTS
Abstract. Problem solving is the process of developing a sequence of actions to achieve a goal. This broad definition admits all goal-directed artificial intelligence programs to the ranks of ...
Planning. Problem Solving vs. Planning: A simple planning agent is very similar to problem-solving agents in that it constructs plans that achieve its goals, and then executes them. The limitations of the problem-solving approach motivates the design of planning systems. To solve a planning problem using a state-space search approach we would ...
11 PLANNING In which we see how an agent can take advantage of the structure of a problem to construct complex plans of action. The task of coming up with a sequence of actions that will achieve a goal is called planning. We have seen two examples of planning agents so far: the search-based problem-solving
Planning Solving Logically Speci ed Problems Step by Step Wolfram Burgard, Bernhard Nebel, and Martin Riedmiller Albert-Ludwigs-Universit at Freiburg August 2, 2011 Contents 1 Planning vs. problem solving 2 Planning in the situation calculus 3 STRIPS formalism 4 Non-linear planning 5 The POP algorithm 6 Graphplan 7 Heuristic search planning
Reasoning, Planning, and Problem-Solving. Checking the weather forecast then planning what to wear to work doesn't seem like a serious challenge as you age. That's because your brain has prepared you to solve everything from the problems of your daily routine to deep conundrums. Your brain incorporates all available information from your ...
This chapter discusses problem-solving techniques and reviews the concept of planning. Generally, planning means deciding on a course of action before acting. A plan is, thus, a representation of a course of action. It can be an unordered list of goals, such as a grocery list, but usually a plan has an implicit ordering of its goals; for ...
Balance divergent and convergent thinking. Ask problems as questions. Defer or suspend judgement. Focus on "Yes, and…" rather than "No, but…". According to Carella, "Creative problem solving is the mental process used for generating innovative and imaginative ideas as a solution to a problem or a challenge.
The McKinsey guide to problem solving. Become a better problem solver with insights and advice from leaders around the world on topics including developing a problem-solving mindset, solving problems in uncertain times, problem solving with AI, and much more.
When we do problem definition well in classic problem solving, we are demonstrating the kind of empathy, at the very beginning of our problem, that design thinking asks us to approach. When we ideate—and that's very similar to the disaggregation, prioritization, and work-planning steps—we do precisely the same thing, and often we use ...
Distributed problem solving involves the collective effort of multiple problems solvers to combine their knowledge, information, and capabilities so as to develop solutions to problems that each could not have solved as well (if at all) alone. The challenge in distributed problem solving is thus in marshalling the distributed capabilities in ...
16 PLANNING, PLAYING AND PROBLEM -SOLVING Planning care is a complex process that involves all kinds of thinking, playing with ideas, trying them out and learning from experience. Thinking is a distinctive characteristic of mankind and is considered to distinguish humans from other animals. How do children learn
Finding a suitable solution for issues can be accomplished by following the basic four-step problem-solving process and methodology outlined below. Step. Characteristics. 1. Define the problem. Differentiate fact from opinion. Specify underlying causes. Consult each faction involved for information. State the problem specifically.
In the subject of AI, planning refers to determining a sequence of actions that are known to achieve a particular objective when performed. Problem solving is finding a plan for a task in an abstract domain. A problem is difficult if an appropriate sequence of steps to solve it is not known. This chapter discusses the overall planning task from ...
The problem specification also contains the Table of Connections (TOG), an indication of when each operator is likely to be useful. 2 Planning and Problem Solving 29 The heart of GPS, and an area where it has influenced almost every planning system since, is the way it chooses which operator to apply in any given state by using means-ends ...
A manager's primary challenge is to solve problems creatively. While drawing from a variety of academic disciplines, and to help managers respond to the challenge of creative problem solving, principles of management have long been categorized into the four major functions of planning, organizing, leading, and controlling (the P-O-L-C framework).
In insight problem-solving, the cognitive processes that help you solve a problem happen outside your conscious awareness. 4. Working backward. Working backward is a problem-solving approach often ...
Ensuring that you plan for the roll-out of a solution is one of the most important problem solving steps. Without adequate planning or oversight, it can prove impossible to measure success or iterate further if the problem was not solved. 6. Solution implementation. This is what we were waiting for!
Planning vs problem solving. Situation calculus. Plan-space planning. Lecture 10 • 1. We are going to switch gears a little bit now. In the first section of the class, we talked about problem solving, and search in general, then we did logical representations. The motivation that I gave for doing the problem solving stuff was that you might ...
6. Discovery & Action Dialogue (DAD) One of the best approaches is to create a safe space for a group to share and discover practices and behaviors that can help them find their own solutions. With DAD, you can help a group choose which problems they wish to solve and which approaches they will take to do so.
Problem Solving and Planning involves a series of interactive exchanges between the EI and caregiver focusing on decision-making about a specific target, routine, or area of concern. ... on their impressions, compare expectations with performance, analyze or make inferences, or evaluate the current plan. When problem solving, EIs must remember ...
They use formal languages like STRIPS (Stanford Research Institute Problem Solver) to represent actions and states. Classical Planning agents explore different action sequences using search algorithms to find a plan that achieves a predefined goal. Examples include puzzle-solving tasks, logistical planning, and robotics tasks with no uncertainty.
Problem solving is the process of diminution or abolishment of the divergence. Planning represents this process of finding out the necessary steps. In contrast to a task, the necessary steps to take that transform the original state into the final state are not yet known and must still be figured out. The steps are represented in a Plan.
According to this framework, four main processes are involved in problem solving: "exploring and understanding", "representing and formulating", "planning and executing", and "monitoring and reflecting". The process of "exploring and understanding" involves interaction with the problem environment to gain information.
Create a Results Strategy and an Implementation Plan. The Results Strategy is a working road map and a problem-solving tool that guides the allocation of time and resources.
With the rapid development of New-IT (e.g., Internet of Things, Cloud Computing, Big Data, Digital Twin, Artificial Intelligence, etc.), an increasing number of scholars are introducing digital twin into process planning, which could significantly increase machining efficiency and effectively ensure the quality of parts during the machining process (Hänel et al., 2020; S. Liu et al., 2021c ...
Read reviews, compare customer ratings, see screenshots, and learn more about Goolee. Download Goolee and enjoy it on your iPhone, iPad, and iPod touch. Unlock Your Inner Genius with Goolee: Elevate Your Problem-Solving Skills to New Heights! Are you ready to take your thinking to the next level? Introducing Goolee - the ultimate challenge ...
Recently, continuous- and discrete-time models of a zeroing neural network (ZNN) have been developed to provide online solutions for the time-dependent linear equation (TDLE) with boundary constraint. This paper presents a novel approach to address the bound-constrained TDLE (BCTDLE) problem by proposing a new discrete-time ZNN (DTZNN) model. The proposed DTZNN model is designed using the ...
For comparison's sake, they've got more experience on the staff at working with quarterbacks than C.J. Stroud had on the staff of offensive coordinator Bobby Slowik. BEST SUPPORTING CAST EVER FOR ...