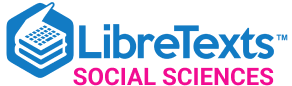
- school Campus Bookshelves
- menu_book Bookshelves
- perm_media Learning Objects
- login Login
- how_to_reg Request Instructor Account
- hub Instructor Commons
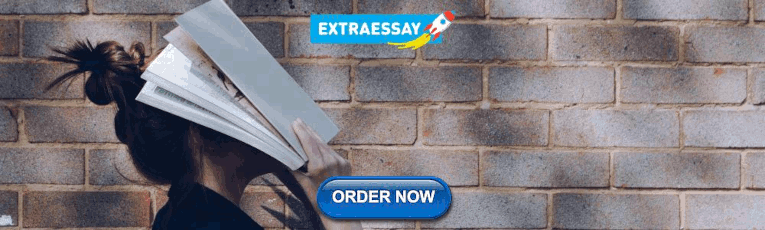
Margin Size
- Download Page (PDF)
- Download Full Book (PDF)
- Periodic Table
- Physics Constants
- Scientific Calculator
- Reference & Cite
- Tools expand_more
- Readability
selected template will load here
This action is not available.
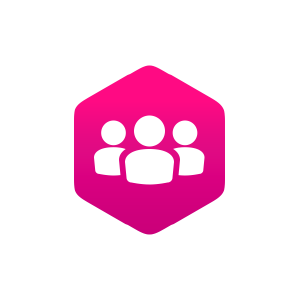
7.1: Anxiety Disorders - Clinical Presentation
- Last updated
- Save as PDF
- Page ID 161382
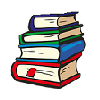
- Alexis Bridley and Lee W. Daffin Jr.
- Washington State University
\( \newcommand{\vecs}[1]{\overset { \scriptstyle \rightharpoonup} {\mathbf{#1}} } \)
\( \newcommand{\vecd}[1]{\overset{-\!-\!\rightharpoonup}{\vphantom{a}\smash {#1}}} \)
\( \newcommand{\id}{\mathrm{id}}\) \( \newcommand{\Span}{\mathrm{span}}\)
( \newcommand{\kernel}{\mathrm{null}\,}\) \( \newcommand{\range}{\mathrm{range}\,}\)
\( \newcommand{\RealPart}{\mathrm{Re}}\) \( \newcommand{\ImaginaryPart}{\mathrm{Im}}\)
\( \newcommand{\Argument}{\mathrm{Arg}}\) \( \newcommand{\norm}[1]{\| #1 \|}\)
\( \newcommand{\inner}[2]{\langle #1, #2 \rangle}\)
\( \newcommand{\Span}{\mathrm{span}}\)
\( \newcommand{\id}{\mathrm{id}}\)
\( \newcommand{\kernel}{\mathrm{null}\,}\)
\( \newcommand{\range}{\mathrm{range}\,}\)
\( \newcommand{\RealPart}{\mathrm{Re}}\)
\( \newcommand{\ImaginaryPart}{\mathrm{Im}}\)
\( \newcommand{\Argument}{\mathrm{Arg}}\)
\( \newcommand{\norm}[1]{\| #1 \|}\)
\( \newcommand{\Span}{\mathrm{span}}\) \( \newcommand{\AA}{\unicode[.8,0]{x212B}}\)
\( \newcommand{\vectorA}[1]{\vec{#1}} % arrow\)
\( \newcommand{\vectorAt}[1]{\vec{\text{#1}}} % arrow\)
\( \newcommand{\vectorB}[1]{\overset { \scriptstyle \rightharpoonup} {\mathbf{#1}} } \)
\( \newcommand{\vectorC}[1]{\textbf{#1}} \)
\( \newcommand{\vectorD}[1]{\overrightarrow{#1}} \)
\( \newcommand{\vectorDt}[1]{\overrightarrow{\text{#1}}} \)
\( \newcommand{\vectE}[1]{\overset{-\!-\!\rightharpoonup}{\vphantom{a}\smash{\mathbf {#1}}}} \)
Learning Objectives
- Describe how generalized anxiety disorder presents.
- Describe how specific phobia presents.
- Describe how agoraphobia presents.
- Describe how social anxiety disorder presents.
- Describe how panic disorder presents.
The hallmark symptoms of anxiety-related disorders are excessive fear and anxiety and related behavioral disturbances. How do we distinguish fear from anxiety? The DSM says that fear is an emotional response to a real or perceived imminent threat which leads to “…surges of autonomic arousal necessary for flight or flight, thoughts of immediate danger, and escape behaviors.” Anxiety, on the other hand, is the anticipation of a future threat leading to, “…muscle tension and vigilance in preparation for future danger and cautious or avoidant behaviors” (APA, 2022, pg. 215). The anxiety disorders differ from one another in the types of objects or situations that lead to fear, anxiety, or avoidance behavior. We will cover generalized anxiety disorder, specific phobia, agoraphobia, social anxiety disorder, and panic disorder.
Generalized Anxiety Disorder
Generalized anxiety disorder is characterized by an underlying excessive anxiety and worry related to a wide range of events or activities and lasting for more days than not for at least six months. While many individuals experience some degree of worry throughout the day, individuals with generalized anxiety disorder experience worry of greater intensity and for longer periods than the average person (APA, 2022). Additionally, they are often unable to control their worry through various coping strategies, which directly interferes with their ability to engage in daily social and occupational tasks. To receive a diagnosis of generalized anxiety disorder, three or more of the following somatic symptoms must be present in adults as well: restlessness, fatigue, difficultly concentrating, irritability, muscle tension, and problems sleeping (APA, 2022; Gelenberg, 2000).
Specific Phobia
Specific phobia is distinguished by fear or anxiety specific to an object or a situation. While the amount of fear or anxiety related to the specific object or situation varies among individuals, it also varies related to the proximity of the object or situation. When individuals are face-to-face with their specific phobia, immediate fear is present, and the phobic object or situation is actively avoided or endured. It should also be noted that these fears are excessive and irrational, often severely impacting one’s daily functioning. The fear, anxiety, or avoidance is persistent, lasting at least six months (APA, 2022).
Individuals can experience multiple specific phobias at the same time. In fact, nearly 75% of individuals with a specific phobia report fear of more than one object and the average individual fears three or more objects or situations (APA, 2022). When making a diagnosis of specific phobia, it is important to identify the stimulus. Among the most diagnosed specific phobias are animals, natural environment (height, storms, water), blood-injection-injury (needles, invasive medical procedures), or situational (airplanes, elevators, enclosed places). In terms of gender differences, women predominantly experience animal, natural environment, and situational specific phobias while blood-injection-injury phobia is experienced by both men and women equally (APA, 2022).
Agoraphobia
Agoraphobia is defined as intense fear or anxiety triggered by two or more of the following: using public transportation such as planes, trains, ships, buses; being in large, open spaces such as parking lots or on bridges; being in enclosed spaces like stores or movie theaters; being in a crowd or standing in line; or being outside of the home alone. The individual fears or avoids these situations because they believe something terrible may occur and due to concern over not being able to escape or help not being available (APA, 2022). Active avoidance of the situations occurs and can be behavioral such as changing daily routines or using delivery to avoid entering a restaurant or cognitive such as using distraction to bear with an agoraphobic situation. The avoidance can result in the person being homebound. The fear or anxiety is out of proportion to the actual danger they pose and has been present for at least six months.
Social Anxiety Disorder
For social anxiety disorder , the anxiety or fear relates to social situations, particularly those in which an individual can be evaluated by others. More specifically, the individual is worried that they will be judged negatively and viewed as stupid, anxious, crazy, boring, or unlikeable, to name a few. Some individuals report feeling concerned that their anxiety symptoms will be obvious to others via blushing, stuttering, sweating, trembling, etc. These fears severely limit an individual’s behavior in social settings and have occurred for six months or more.
To explain social anxiety in greater detail, let’s review the story of Mary. Mary reported the onset of her social anxiety disorder in early elementary school when teachers would call on students to read parts of their textbook aloud. Mary stated that she was fearful of making mistakes while reading and to alleviate this anxiety, she would read several sections ahead of the class to prepare for her turn to read aloud. Despite her preparedness, one day in 5th grade, Mary was called to read, and she stumbled on a few words. While none of her classmates realized her mistake, Mary was extremely embarrassed and reported higher levels of anxiety during future read aloud moments in school. In fact, when she was called upon, Mary stated she would completely freeze up and not talk at all. After a few moments of not speaking, her teacher would skip Mary and ask another student to read her section. It took several years and a very supportive teacher for Mary to begin reading aloud in class again.
Like Mary, individuals with social anxiety disorder report that all or nearly all social situations provoke this intense fear. Some individuals even report significant anticipatory fear days or weeks before a social event is to occur. This anticipatory fear often leads to avoidance of social events in some individuals; others will attend social events with a marked fear of possible threats. Because of these fears, there is a significant impact on one’s social and occupational functioning.
It is important to note that the cognitive interpretation of these social events is often excessive and out of proportion to the actual risk of being negatively evaluated. As we saw in Mary’s case, when she stumbled upon her words while reading to the class, none of her peers even noticed her mistake. Situations in which individuals experience anxiety toward a real threat, such as bullying or ostracizing, would not be diagnosed with social anxiety disorder as the negative evaluation and threat are real.
Panic Disorder
Panic disorder consists of a series of recurrent, unexpected panic attacks coupled with the fear of future panic attacks. A panic attack is defined as a sudden or abrupt surge of fear or impending doom along with at least four physical or cognitive symptoms. Physical symptoms include heart palpitations, sweating, trembling or shaking, shortness of breath, feeling as though they are being choked, chest pain, nausea, dizziness, chills or heat sensations, and numbness/tingling. Cognitive symptoms may consist of feelings of derealization (feelings of unreality) or depersonalization (feelings of being detached from oneself), the fear of losing control or ‘going crazy,’ or the fear of dying (APA, 2022). While symptoms generally peak within a few minutes, it seems much longer for the individual experiencing the panic attack.
There are two key components to panic disorder—the attacks are unexpected , meaning there is nothing that triggers them, and they are recurrent , meaning they occur multiple times. Because these panic attacks occur frequently and are primarily “out of the blue,” they cause significant worry or anxiety in the individual as they are unsure of when the next attack will happen. In contrast to unexpected there are also expected panic attacks, or those that have an obvious trigger. The DSM-5-TR states that presence of expected panic attacks does not rule out the diagnosis of panic disorder as about half of individuals diagnosed with the disorder in the United States and Europe have both types of attacks (APA, 2022).
In some individuals, significant behavioral changes such as fear of leaving their home or attending large events occur as the individual is fearful an attack will happen in one of these situations, causing embarrassment. Additionally, individuals report worry that others will think they are “going crazy” or losing control if they were to observe an individual experiencing a panic attack. Occasionally, an additional diagnosis of agoraphobia is given to an individual with panic disorder if their behaviors meet diagnostic criteria for this disorder as well.
The frequency and intensity of these panic attacks vary widely among individuals. Some people report panic attacks occurring once a week for months on end, others report more frequent attacks multiple times a day, but then experience weeks or months without any attacks. The intensity of symptoms also varies among individuals, with some patients experiencing four or more symptoms (full-symptom) or less than four (limited-symptom. Furthermore, individuals report variability within their panic attack symptoms, with some panic attacks presenting with more symptoms than others. To be diagnosed with panic disorder, the individual must present with more than one unexpected full-symptom panic attack (APA, 2022).
Key Takeaways
You should have learned the following in this section:
- All anxiety disorders share the hallmark symptoms of excessive fear or worry related to behavioral disturbances.
- Generalized anxiety disorder is characterized by an underlying excessive worry related to a wide range of events or activities and an inability to control their worry through coping strategies.
- Specific phobia is characterized by fear or anxiety specific to an object or a situation and individuals can experience fear of more than one object.
- Agoraphobia is characterized by intense fear related to situations in which the individual is in public situations where escape may be difficult and help may not be able to come.
- Social anxiety disorder is characterized by fear or anxiety related to social situations, especially when evaluation by others is possible.
- Panic disorder is characterized by a series of recurrent, unexpected panic attacks coupled with the fear of future panic attacks.
Review Questions
- What is the difference between fear and anxiety?
- What are the key differences between generalized anxiety disorder and agoraphobia?
- Individuals with social anxiety disorder will experience both physical and cognitive symptoms, particularly when presented with social interactions. What are these symptoms?
- What are the common types of specific phobias?
- What are the physical and cognitive symptoms observed during panic disorder?
- What are the key components of panic disorder?

An official website of the United States government
The .gov means it’s official. Federal government websites often end in .gov or .mil. Before sharing sensitive information, make sure you’re on a federal government site.
The site is secure. The https:// ensures that you are connecting to the official website and that any information you provide is encrypted and transmitted securely.
- Publications
- Account settings
Preview improvements coming to the PMC website in October 2024. Learn More or Try it out now .
- Advanced Search
- Journal List
- Wiley Open Access Collection

Co‐morbidity between mood and anxiety disorders: A systematic review and meta‐analysis
Sukanta saha.
1 Queensland Brain Institute, University of Queensland, St Lucia Australia
2 Queensland Centre for Mental Health Research, The Park Centre for Mental Health, Wacol Australia
Carmen C. W. Lim
Danielle l. cannon, lucinda burton, monique bremner, peter cosgrove.
3 Business School, Faculty of Business, Economics and Law, University of Queensland, St Lucia Australia
John J. McGrath
4 National Centre for Register‐based Research, Aarhus University, Aarhus Denmark
Associated Data
All data underlying the meta‐analyses and code can be found at this URL: https://github.com/clim072/NB-SR_MOOD_ANX .
There is consistent evidence that mood disorders often co‐occur with anxiety disorders, however, the strength of the association of these two broad groups of disorders has been challenging to summarize across different studies. The aim was to conduct a meta‐analysis of publications reporting on the pairwise comorbidity between mood and anxiety disorders after sorting into comparable study types. We searched MEDLINE, Embase, CINAHL, Web of Science, and the grey literature for publications between 1980 and 2017 regardless of geographical locations and languages. We meta‐analyzed estimates from original articles after sorting by: (a) broad or narrow diagnostic criteria, (b) study time‐frame, and (c) estimates with or without covariate adjustments. Over 43 000 unique studies were identified through electronic searches, of which 391 were selected for full‐text review. Finally, 171 studies were eligible for inclusion, including 53 articles from additional snowball searching. In general, regardless of variations in diagnosis type, study time‐frame, temporal order, or use of adjustments, there was substantial comorbidity between mood and anxiety disorders. Based on the entire 90 separate meta‐analyses, the median OR was 6.1 (range 1.5–18.7). Of these estimates, all 90 were above 1, and 87 were significantly greater than 1 (i.e., the 95% confidence intervals did not include 1). Fourteen of the 90 pooled estimates had ORs that were greater than 10. This systematic review found robust and consistent evidence of comorbidity between broadly defined mood and anxiety disorders. Clinicians should be vigilant for the prompt identification and treatment of this common type of comorbidity.
1. INTRODUCTION
It is widely recognized that mood and anxiety disorders frequently co‐occur—the presence of one of these two disorders increases the risk of subsequently developing the other (comorbid) disorder. Comorbidity refers to the presence of one or more disorders in relation to an index disorder either within the same time period (concurrent comorbidity) or across different phases of the life span (cumulative comorbidity) (van den Akker et al., 1996 ). The presence of comorbidity between these two disorders is important because anxiety and depressive disorders independently contribute to a significant portion of the global burden of disease, with depression being the second largest contributor to years lived with disability in those 15–44 years of age (Ferrari et al., 2013 ). In addition, comorbidity between mood and anxiety disorders is associated with greater symptom severity (Bernstein, 1991 ; Coryell et al., 1988 ), increased substance use, and suicidal risk (Lewinsohn, Gotlib, et al., 1995 ; Lewinsohn, Rohde, et al., 1995 ; Rohde et al., 2001 ), and treatment resistance compared with those who have either disease in isolation (Brent et al., 1998 ; Emslie et al., 1998 ; Lewinsohn, Gotlib, et al., 1995 ; Melton et al., 2016 ).
Based on recent large studies, both the concurrent and cumulative risk of comorbidity between anxiety and mood disorders is substantial and bidirectional (Moffitt et al., 2007 ; Plana‐Ripoll et al., 2019 ), and exists in individuals of all ages. For example, a birth cohort study from Dunedin found that among those aged 11–32 years, anxiety concurrently occurs in 37% of depression cases, while depression occurs in 32% of anxiety cases (Moffitt et al., 2007 ). Cumulatively, 72% of lifetime anxiety cases had a history of depression, and 48% of lifetime depression cases had anxiety disorders (odds ratio [OR] between 4.7 and 5.5). A recent large Danish register‐based study ( n = 5 940 778) found that those who developed a mood disorder by age 20 had a four‐fold risk of subsequently developing an anxiety disorder (OR = 4.3; 95% CI = 4.1–4.4) (Plana‐Ripoll et al., 2019 ). An analysis of community‐based surveys from 27 countries also showed a very high rate of comorbidity between mood and anxiety disorders; the risk of developing generalized anxiety disorder was about seven times in those with major depression even after 15 years (hazard ratio = 6.6; 95% CI = 5.7–7.7) (McGrath et al., 2020 ).
Previous systematic reviews have provided pooled estimates for selected types of comorbid mood and anxiety disorders (Lemstra et al., 2008 ; Nabavi et al., 2015 ; Pavlova et al., 2015 ; Rytwinski et al., 2013 ; Zavaglia & Bergeron, 2017 ). However, the pooling of estimates related to comorbidity can be challenging because of the heterogeneity in the study design and between‐site variations in the prevalence of the underlying disorders. Estimates of pair‐wise comorbidity can be based on: (a) broad or narrow diagnostic criteria (e.g., any type of depression‐related disorder, specific depression‐related disorder); (b) different time periods and temporal framework (e.g., cumulative comorbidity over one year or a lifetime regardless of the temporal order of the two disorders; estimates based on temporally ordered disorders with prior depression leading to subsequent anxiety or vice versa); and (c) the presentation of unadjusted risk estimates or estimates adjusted for a range of covariates (e.g., sex, age, etc.). There is a need for a more comprehensive systematic review and meta‐analysis that (a) takes into account a comprehensive range of mood and anxiety‐related disorders, and (b) provides pooled estimates based on features related to the period of observation (e.g., last 12 months, lifetime prevalence), and adjustments of covariates, and temporally ordering between disorder pairs.
The aims of the current systematic review are to provide an up‐to‐date list of studies that have examined comorbidity between broadly defined mood‐ and anxiety‐related disorders and to meta‐analyze the risk estimates according to key design features related to the types of prevalence estimates for the two disorders.
2.1. Search strategy and identification of studies
Based on a protocol registered with PROSPERO (Saha et al., 2019 ), this systematic review is PRISMA (Preferred Reporting Items for Systematic Reviews and Meta‐Analyses) (Moher et al., 2015 ; Shamseer et al., 2015 ), and MOOSE (Meta‐analysis of Observational Studies in Epidemiology) (Stroup et al., 2000 ) compliant. A comprehensive search strategy was developed for identifying research publications on comorbidity of mood and anxiety disorders. In the absence of optimized search filters for bibliographic search (Waffenschmidt et al., 2017 ), to design this search algorithm with optimal sensitivity and specificity, different tests were performed to optimize the best combination of terms in different databases. Detailed search strategies are found in the Supporting Information eMethods 1‐2. A panel of experts (JM, SS) independently validated and revised search algorithms in different databases. Studies were identified through four electronic databases namely, PubMed‐Medline, Embase, CINAHL, and Web of Science between January 1, 1980, and December 30, 2017. No restrictions were made regarding the geographical location or language of publications.
2.2. Screening, snowball searching, and data extraction
All potential articles from four database searches were uploaded to a commercial software, Covidence for the management of Title and Abstract (TIAB) screening followed by full‐text scrutiny. Any discrepancy for the screening process was resolved by the consensus of the two reviewers (PRISMA flowchart, Figure 1 ). Articles were screened followed by full‐text scrutiny for final inclusion into the relevant article pool. Articles with LOTE (Language Other Than English) were translated and assessed. We conducted TIAB screening based on inclusion and exclusion criteria (details in Supporting Information eMethods 2) where discrepancies were resolved by consensus among senior authors. This was followed by full‐text scrutiny for the final inclusion of relevant studies. Any discrepancy at this stage was also resolved by the consensus of the two reviewers.

Flow diagram (selection strategy) of included studies
We conducted snowball search for additional articles. The references cited by each relevant article, and grey literature (e.g., systematic reviews, book chaptersetc.) were searched by title, and screened followed by full‐text scrutiny for final inclusion into the relevant article pool. As stated, the final inclusion of the relevant articles from the snowball search was also accomplished by the consensus of two reviewers.
Data were extracted from the final list of the “included” articles and entered into a three‐level database. We collected information on (i) study characteristics (author, year, site etc.), (ii) methodology (sample size used, diagnostic criteria, type of mood and anxiety disorder etc.), and (iii) risk estimates (odds ratios, hazard ratios). In general, we extracted data from studies that provided: (1) both cross‐sectional and temporally ordered risk estimates (e.g., odds ratio, risk ratio), and (2) both unadjusted and adjusted estimates. We also extracted data from studies that provided sufficient information to allow the calculation of odds ratios. These estimates were considered as unadjusted estimates. For uniform presentation, we used pooled odds ratio as our primary risk measure as 95.1% of the studies reported ORs. Additional details of these steps can be found in Supporting Information eMethods 3.1.
2.3. Operationalized features: Diagnostic criteria, sequential filters, and quality reporting scale
In keeping with our previous systematic reviews (McGrath et al., 2004 ; Saha et al., 2005 , 2007 ), and following standard systematic review guidelines (von Elm et al., 2007 ), we used several operationalized features. We used studies with defined diagnostic criteria (e.g., ICD, DSM etc.) for case definitions for both mood and anxiety disorders. Thus, any studies with symptom‐based disorders as well as those with an unclear methodology for any case definitions were excluded. Detailed diagnostic criteria and instruments are shown in Supporting Information eMethods 4.
The second key operationalized feature was to apply a sorting algorithm using a series of sequential filters . When multiple studies overlapped partially or fully in both time and space, we used a sequential filter for delineating discrete estimates. We divided the estimates into three different types (based on study types): lifetime prevalence, period prevalence, and temporally ordered estimates related to respective cross‐sectional, case‐control, or cohort studies. We also divided estimates based on adjusted or unadjusted estimates to avoid adjustment reporting bias (Peters & Mengersen, 2008 ). The aim was to avoid double counting of any study estimates while sorting estimates into meaningful groups to minimize possible biases.
Next, for identifying discrete data from overlapping studies, we used two types of filters, a “study‐level filter” and an “estimate‐level filter”; the former delineated overlapping studies while the latter parsed overlapping estimates between studies (details are in Supporting Information eMethods 3.2 and assessments are in Supporting Information eMethods 5). Using the study‐level filter we were able to exclude 43 papers that were completely overlapping by time and place.
To assess the overall quality of the study estimates, we employed a “Quality Reporting Scale” (QRS). The scale contains 14 criteria based on features that were operationalized, and were based on simple, categorical judgments (criteria met vs. not met) (McGrath et al., 2004 ; Saha et al., 2005 , 2007 ). Detailed criteria and scales are in Supporting Information eMethods 6.
We found that a variety of labels were used for mood‐and anxiety‐related disorders. For computational tractability, we combined similar terms into a “broad” category. For example, we used “broadly defined mood disorder” (henceforth “MOOD”) for any mood disorders. Similarly, we used “broadly defined depressive disorder” (henceforth “DEP”) for depressive disorders, “broadly defined dysthymic disorder” (henceforth “DYS”) for dysthymic disorders, and “broadly defined anxiety disorders” (henceforth “ANX”) for anxiety disorders. Details of these categories with subtypes are available in Supporting Information eMethods 7. We used four broad mood disorders (MOOD, DEP, DYS, BIPOLAR) with seven subtypes of ANX pairs as follows: generalized anxiety disorder (GAD), obsessive‐compulsive disorder (OCD), posttraumatic stress disorder (PTSD), social phobia, specific phobia, panic, and agoraphobia.
2.4. Data analysis and presentation
We reported pooled risk estimates (i.e., odds ratio) using inverse‐variance random‐effects models. Based on expected differences in the prevalence of anxiety‐ and mood‐related disorders between the included studies, we predicted considerable heterogeneity between studies. To quantify this, we used the Q‐statistic which is sensitive to the number of studies especially when the number of studies is small (DerSimonian & Laird, 1986 , 2015 ). We have also included I 2 , a measure of the proportion of total variation in estimates that is due to heterogeneity with values 25%, 50%, and 75% corresponding to low, moderate, and a high degree of heterogeneity, respectively. For disorder pairs with a sufficient number of studies (>10), we visually inspected the funnel plot and used Egger's test for the possibility of publication bias (Egger et al., 1997 ).
Data were presented in both tabular and graphical format (e.g., forest plots, funnel plots). To avoid bias in meta‐analysis, we presented pooled estimates separately for crude (unadjusted) and adjusted models (Peters & Mengersen, 2008 ), and for a lifetime, period prevalence, and temporally ordered estimates. Period prevalence estimates included any estimates above 1‐month (1, 3, 6, and 12 months). In the temporally ordered estimates, we present pairwise risk estimates for both directions.
The “metafor” package in R version 3.6.2 was used to produce pooled estimates, forest and funnel plots. ORs from each study were transformed using natural logarithm, and standard errors (SE) were calculated from the reported confidence intervals (CIs). In studies with missing CIs, reported p‐values were used to calculate SEs. Comorbidity between pairwise disorders was presented as pooled risk estimates using crude OR or adjusted OR (aOR) with not less than three studies for disorder pairs.
The results of the detailed search strategy are shown in Supporting Information eMethods 1‐2 and Figure 1 . We identified 43 419 studies from four electronic databases, of which 390 were screened for full‐text reviews including nine LOTE papers. More than half ( n = 272) were excluded for various reasons (Figure 1 ). We also found 53 papers from citation search of “included” papers and grey literature. Consequently, 171 studies were included in our quantitative synthesis drawn from 37 countries (efigure 1). Using 56 pairs of mood and anxiety disorders, 90 estimates were investigated in the meta‐analysis including 76 unidirectional lifetime and period prevalence risk estimates and 14 temporally ordered bidirectional estimates.
Study characteristics of the 171 studies are presented in Table 1 and etable 1. Table 1 includes 147 studies (eReference 1) that presented lifetime and period prevalence estimates whereas eTable 1 includes 36 studies (eReference 2) that presented temporally ordered estimates.
Study characteristics of included studies that provided lifetime and period prevalence ( n = 146)
Abbreviations: AGO, agoraphobia with or without panic disorder; ANX, broadly defined anxiety disorder; aOR, adjusted odds ratio; BIPOLAR, bipolar I, II or bipolar disorder; CC, case‐control studies; CS, cross‐sectional studies; DEP, depressive disorder; DYS, dysthymia; GAD, generalized anxiety disorder; MOOD, mood disorder; OCD, obsessive‐compulsive disorder; OR, odds ratio; OVRANX, overanxious disorder; PC, prospective cohort studies; PD, panic disorder; PHOBIA, phobia disorder; PTSD, posttraumatic stress disorder; RR, risk ratio; SAD, separation anxiety disorder; SO, social phobia or social anxiety disorder; SP, specific phobia or simple phobia.
3.1. Pairwise association between mood and anxiety disorders
Estimates from the pairwise associations of mood and anxiety disorders are presented in Tables 2 , ,3, 3 , ,4, 4 , ,5. 5 . Forest plots and associated funnel plots are presented in efigures 2–90 (includes 14 funnel plots). Of these, we presented three forest plots in the main text (Figures 2 , ,3, 3 , ,4). 4 ). Overall, there was substantial comorbidity between various mood and anxiety disorders with a median OR of 6.1 (range 1.5–18.7). For example, those with MOOD had 19 times elevated risk of GAD (Figure 2 ); while those with DEP had 13.8 times elevated risk of GAD after pooling all the unadjusted estimates (Figure 3 ), and 11.7 times elevated risk of GAD after pooling all the adjusted estimates (Figure 4 ).

Forest plot of the random‐effects meta‐analysis of period prevalence comorbidity between broadly defined mood and generalized anxiety disorders (adjusted) (aOR 18.7; 95% CI 16.2, 21.6)

Forest plot of the random‐effects meta‐analysis of period prevalence comorbidity between broadly defined depressive disorder and generalized anxiety disorders (unadjusted) (aOR 13.8; 95% CI 9.9, 19.3)

Forest plot of the random‐effects meta‐analysis of period prevalence comorbidity between broadly defined depressive disorder and generalized anxiety disorders (adjusted) (aOR 11.7; 95% CI 5.2, 26.3)
Pooled estimates for broadly defined mood disorder (MOOD) and anxiety disorders
Abbreviations: ANX, broadly defined anxiety disorder, GAD, generalized anxiety disorder.
Pooled estimates for broadly defined depressive disorder (DEP) and anxiety disorders
Abbreviations: ANX, broadly defined anxiety disorder; GAD, generalized anxiety disorder; OCD, obsessive‐compulsive disorder; PTSD, posttraumatic stress disorder.
Pooled estimates for broadly defined dysthymic disorder (DYS) and anxiety disorders
Abbreviations: ANX, broadly defined anxiety disorder; GAD, generalized anxiety disorder.
Pooled estimates for broadly defined bipolar disorder (BIPOLAR) and anxiety disorder subtypes
Abbreviations: ANX, broadly defined anxiety disorder, GAD, generalized anxiety disorder; OCD, obsessive‐compulsive disorder; PTSD, posttraumatic stress disorder.
3.2. MOOD and anxiety disorder: Lifetime comorbidity
We identified nine studies (eReference 3) for lifetime comorbidity between MOOD and different anxiety disorders (Table 2 ). The pooled OR (95% CI) ranged between 2.4 (1.1, 5.2) for MOOD and specific phobia based on three studies, and 7.7 (3.3, 17.7) for MOOD and ANX based on five studies.
3.3. MOOD and anxiety disorder: Period prevalence comorbidity
We identified 20 studies (eReference 4) for period prevalence comorbidity between MOOD and anxiety disorders (Table 2 ). The crude OR (95% CI) ranged between 9.1 (3.9, 21.3) for MOOD and ANX based on 10 studies, and 18.4 (13.3, 27.2) for MOOD and social phobia based on three studies. The aOR (95% CI) ranged between 5.2 (2.9, 10.0) for MOOD and social phobia based on three studies, and 18.7 (16.2, 21.6) for MOOD and GAD based on five studies.
3.4. DEP and anxiety disorder: Lifetime comorbidity
We identified 48 studies (eReference 5) for lifetime comorbidity between DEP and anxiety disorders (Table 3 ). The crude OR (95% CI) ranged between 3.3 (2.7, 4.0) for DEP and specific phobia based on 14 studies, and 9.0 (5.6, 14.5) for DEP and GAD based on 11 studies. The aOR (95% CI) ranged between 2.4 (1.6, 3.5) for DEP and specific phobia based on seven studies, and 7.4 (4.4, 12.4) for DEP and panic disorder based on 11 studies.
3.5. DEP and anxiety disorder: Period prevalence comorbidity
We identified 68 studies (eReference 6) for period prevalence comorbidity between DEP and anxiety disorders (Table 3 ). The crude OR (95% CI) ranged between 3.7 (2.7, 5.0) for DEP and specific phobia based on eight studies, and 13.8 (9.9, 19.3) for DEP and GAD based on 22 studies. The aOR (95% CI) ranged between 2.9 (2.3, 3.7) for DEP and specific phobia based on three studies, and 11.7 (5.2, 26.3) for DEP and GAD based on 12 studies.
3.6. DYS and anxiety disorder: Lifetime comorbidity
We identified 16 studies (eReference 7) for lifetime comorbidity between DYS and anxiety disorders (Table 4 ). The crude OR (95% CI) ranged between 6.6 (3.8, 11.3) for DYS and OCD based on four studies, and 13.8 (12.3, 15.4) for DYS and GAD based on five studies. The only aOR (95% CI) was between DYS and social phobia: 4.1 (2.1, 8.2) based on five studies.
3.7. DYS and anxiety disorder: Period prevalence comorbidity
We identified 17 studies (eReference 8) for period prevalence comorbidity between any DYS and anxiety disorders (Table 4 ). The crude OR (95% CI) ranged between 6.9 (1.6, 29.3) for DYS and specific phobia based on four studies, and 17.2 (10.4, 28.4) for DYS and PTSD based on three studies. The aOR (95% CI) ranged between 2.3 (0.7, 7.8) for DYS and PTSD based on three studies, and 12.3 (9.8, 15.5) for DYS and GAD based on five studies.
3.8. BIPOLAR and anxiety disorder: Lifetime comorbidity
We identified 28 studies (eReference 9) for lifetime comorbidity between BIPOLAR and anxiety disorders (Table 5 ). The crude OR (95% CI) ranged between 4.4 (2.2, 9.0) for BIPOLAR and social phobia based on six studies, and 7.8 (6.5, 9.4) for BIPOLAR and ANX based on eight studies. The aOR (95% CI) ranged between 3.5 (1.9, 6.6) for BIPOLAR and specific phobia based on four studies, and 8.9 (5.2, 15.3) for BIPOLAR and GAD based on four studies.
3.9. BIPOLAR and anxiety disorder: Period prevalence comorbidity
We identified 14 studies (eReference 10) for period prevalence comorbidity between BIPOLAR and different anxiety disorders (Table 5 ). The crude OR (95% CI) ranged between 4.9 (for BIPOLAR and both ANX (2.0, 12.2) and also specific phobia (4.1, 5.8) based on three studies), and 11.6 (8.9, 15.0) for BIPOLAR and panic disorder based on three studies. The aOR (95% CI) ranged between 3.7 (2.1, 6.5) for BIPOLAR and social phobia based on four studies, and 7.4 (1.6, 34.0) for BIPOLAR and GAD based on three studies.
3.10. Temporally ordered associations between mood‐ and anxiety‐related disorders
Although the majority of these estimates could not be pooled because of low number of studies, there was an elevated risk of comorbidity between different MOOD (as a prior disorder) and different anxiety disorders (as a later disorder), and vice‐versa (etables 2 and 3). For example, those with DEP had 2‐3 times elevated risk of later ANX (aOR = 2.1: 95% CI 1.5, 2.8), those with ANX as a prior disorder had similar risk of developing DEP (aOR = 2.1: 95% CI 1.8, 2.5) (etable 3). The highest risk was observed between DEP as a prior disorder and social phobia as a later disorder (OR = 7.3: 95% CI 6.2, 8.7), while the corresponding risk was about one‐third for those with social phobia as a prior disorder and DEP as a later disorder (OR = 2.5: 95% CI 2.1, 3.1).
3.11. Overall findings, heterogeneity, quality scores and funnel plots
Most of the pooled estimates showed heterogeneity (the Q‐statistic and I 2 are presented with each forest plot). However, it is important to note that this heterogeneity does not detract from the main findings (i.e., there is strong comorbidity between the two disorders). The pooled estimates for all meta‐analyses were above one, and only one of the 76 uni‐directional meta‐analyses and two of the 14 bidirectional meta‐analyses had 95% confidence intervals that included 1 (Table 4 : between DYS and PTSD; and etable 3: between OCD and DEP, and specific phobia and DEP respectively). The Eggers z (test) linked to respective funnel plots ranged between 0.04 ( p = .97) for DEP and GAD (efigure 16), and 1.82 ( p = .07) for DEP and panic disorder (efigure 21), thus not providing evidence of substantial publication bias for the various pooled estimates. The 14 funnel plots (with studies > 10) are presented in efigures. The quality score between studies ranged between 8 and 16 (Table 1 ) with the majority having quality scores in the upper range of the scale (median = 13, interquartile range: 12–15). As most of the meta‐analyses were based on small numbers of contributing studies, and because of the relative lack of variation in the quality scores, additional investigation of the impact of this score on the findings was not undertaken.
4. DISCUSSION
Our systematic review identified 171 articles from 37 countries over the last 38 years. Based on estimates from these studies, our systematic review generated 90 separate meta‐analyses that included 76 unidirectional and 14 temporally ordered bidirectional risk estimates. The median of these pooled estimates (OR) was 6.1 (range 1.5–18.7). Regardless of the specific nature of the mood‐ and anxiety‐related disorders, and regardless of the study design (e.g., diagnostic criteria), the pooled risk estimates of all the 90 meta‐analyses were above one. Of these estimates, 87 were significant (i.e., the 95% CI did not include 1), and 14 of the 90 pooled estimates had ORs that were greater than 10. Based on temporally ordered pooled estimates, we also found that the relationship was bidirectional. Regardless of which of the two disorders arose first, there was an increased risk of subsequently developing the other disorder. To the best of our knowledge, this is the most comprehensive systematic review and meta‐analysis of comorbidity between mood and anxiety disorders.
This study builds on previous systematic reviews by including a comprehensive range of mood and anxiety disorders. In addition, these estimates were sorted by design features related to the period of observation (period, lifetime prevalence etc.), temporal order, and the use of adjustments for covariates. Regardless of the many different types of pairs of disorders, the pooled estimates were uniformly above 1, and often were large (i.e., greater than 10‐fold risk). Overall, we found very strong comorbidity between mood and anxiety disorders, often higher than previously reported pooled estimates. For example, Pavlova et al. ( 2015 ) in a recent review found a three‐fold increased lifetime comorbidity between bipolar and anxiety disorder, whereas we found an elevated odds of about eight‐fold between this pair of disorders. In some disorder pairs (e.g., MOOD and GAD), the pooled estimate was as high as 19 times (aOR = 18.7). However, our estimates were broadly consistent with two studies that were published after the completion of our data extraction: a large register‐based study from Denmark ( n = 5 940 778) (Plana‐Ripoll et al., 2019 ), and a trans‐national analysis that combined individual data from 27 countries ( n = 145 990 survey respondents) (McGrath et al., 2020 ). Overall, the consistent patterns identified by our systematic review, and the findings from these two recent studies, provide convergent evidence of the strong comorbidity between these disorders.
With respect to the bidirectional nature of comorbidity between temporally ordered mood‐ and anxiety‐related disorders, we found that there was a two‐fold risk of developing ANX among those with DEP and vice‐versa. However, these estimates were based on a limited number of studies. Although this bidirectional association was broadly consistent with our recently conducted population‐based studies (McGrath et al., 2020 ; Plana‐Ripoll et al., 2019 ), there was a lack of consistency between these studies regarding the symmetry between the size of the reciprocal risk estimates (i.e., did the effect sizes differ according to order, regardless of the fact that both estimates were substantially above 1 and significant). Bi‐directionality within pairs of comorbid disorders suggests that these disorders may result from shared underlying risk factors (e.g., genetic; Levey et al., 2020 ; Purves et al., 2019 ; and/or shared early life exposures; Kessler et al., 1997 ).
With respect to study design features related to the temporal time‐frame, we found significant associations between MOOD and ANX when we pooled estimates from (a) lifetime, (b) period prevalence, and (c) temporally ordered estimates. The overall pattern of strong comorbidity between mood‐ and anxiety‐ disorders was found in both crude and adjusted estimates, consistent with findings from the recently published WHO World Mental Health Survey study (McGrath et al., 2020 ). We meta‐analyzed a large number of studies that presented a strong association between lifetime comorbidity between mood and anxiety disorders. The highest pooled estimate was found between BIPOLAR and GAD (aOR 8.9) followed by BIPOLAR and OCD (aOR 8.4), BIPOLAR and ANX (aOR 7.7), and DEP and panic disorder (aOR 7.4). Similarly, based on period prevalence estimates, the largest estimate was about 19‐fold between MOOD and GAD (aOR 18.7) followed by MOOD and ANX (aOR 11.8), DYS and GAD (aOR 12.3), and DEP and GAD (aOR 11.7).
4.1. Understanding heterogeneity
Despite parsing the estimates by a range of methodological features, the pooled estimates were largely heterogeneous according to Q‐statistics and I 2 test (although a recent report showed that I 2 is not an absolute measure of heterogeneity) (Borenstein et al., 2017 ). It is important to note that despite the heterogeneity underlying the pooled estimates, we found that the majority of the pooled estimates were large (14 estimates above 10‐fold), and only three had 95% confidence intervals that included 1. Given the imprecise nature of psychiatric epidemiology, some degree of heterogeneity is to be expected in our analyses, even when these studies are based on high quality standardized methods (Gureje, 2009 ; Nabavi et al., 2015 ). Trans‐national studies have found that the prevalence of anxiety and depression varies between sites and across time (related to conflict, natural disaster, access to substances of abuse, cultural issues, availability to treatment services etc.) (Scott et al., 2018 ). Thus, comorbidity based on prevalence estimates will also be expected to vary.
4.2. Understanding comorbidity, and clinical implications
This systematic review provided risk estimates (Odds ratios) as the evidence of comorbidity between mood and anxiety disorders. Although it has the appealing feature of summarizing two numbers (the risk in one group and the risk in the other), it provides no information about the underlying absolute risk (the number of events over number of people). Absolute risk estimates are more useful for clinicians to actively monitor comorbid disease development. For example, recent population based studies (McGrath et al., 2020 ) show that in the first 5 years of receiving a diagnosis of mood disorder more than a quarter of patients develop anxiety disorder. We encourage future studies to provide both relative and absolute risk estimates to better understand the epidemiological landscape of comorbidity.
4.3. Limitations
The systematic review has several important limitations. Firstly, for some studies we had to calculate the estimates based on raw data which may misrepresent true weighted estimates. Ideally, population based studies (e.g., survey data) use weightings that incorporates sample selection, nonresponse and post‐stratification and so forth for the presentation of frequencies and estimates (Kessler et al., 2011 ; Kessler & Ustun, 2004 ). We urge caution in the interpretation of unadjusted estimates. Secondly, to pool estimates, we combined similar disorders into broad categories which may create some heterogeneity between estimates as well as loss of fine‐grain data. For example, we used “broadly defined mood disorder” (MOOD) for disorders such as depression, dysthymia and bipolar disorders. Thirdly, estimates from population surveys were often based on interviews from lay interviewers which may inflate comorbidity. On the other hand, lifetime diagnoses were based on retrospective reports that may underestimate prevalence, and therefore deflate comorbidity structure. Pooled estimates usually minimize this discrepancy. Fourthly, surveys usually do not take into account treatment history, which may interrupt progression of comorbidity, and thus distort comorbidity estimates (Kessler et al., 2011 ). In addition, diagnostic overlap within disorders (e.g., bipolar and depression) also may contribute misleading comorbidity estimates. Finally, despite our endeavor to include as many articles as possible by searching important databases, grey literature, as well as snowballing for additional articles, it is possible that some studies may be missed.
5. CONCLUSIONS
This systematic review provides compelling evidence that there is substantial comorbidity between various mood‐ and anxiety‐related disorders. A recent commentary by Hyman ( 2019 ) emphasized the convergence of evidence from (a) comorbidity studies (such as the current meta‐analysis), and (b) twin and genome‐wide association studies. Different types of mental disorders often have a shared risk architecture, which could underpin the pathogenesis of mental disorders and the patterns of comorbidity seen in the current study (Anttila et al., 2018 ; Kendler et al., 2011 ; Kessler et al., 1996 , 2011 ). From a clinical perspective, it is important to remain vigilant for the prompt identification and treatment of comorbidity between anxiety‐ and depression‐related disorders.
CONFLICT OF INTERESTS
The authors declare that there are no conflict of interests.
AUTHOR CONTRIBUTIONS
Sukanta Saha and John J. McGrath conceived the study. Carmen C. W. Lim, John J. McGrath and Sukanta Saha conducted the analysis. Sukanta Saha, Carmen C. W. Lim, and John J. McGrath drafted and edited the manuscript. All authors contributed to refinement of the study protocol and approved the final manuscript.
Supporting information
Supporting information.
ACKNOWLEDGMENTS
We acknowledge the contribution of Andrea Baker and Thomas Dinala from the Queensland Centre for Mental Health Research for helping with initial screening of the articles. Special thanks to Ann Cude for her logistical help. The project is supported by the Danish National Research Foundation (Niels Bohr Professorship to John McGrath) as well as the John Cade Fellowship APP1056929 (awarded to John McGrath) from the National Health and Medical Research Council, Australia.
Saha S, Lim CCW, Cannon DL, et al. Co‐morbidity between mood and anxiety disorders: A systematic review and meta‐analysis . Depression and Anxiety . 2021; 38 :286–306. 10.1002/da.23113 [ PMC free article ] [ PubMed ] [ CrossRef ] [ Google Scholar ]
PROSPERO registration: PROSPERO 2019 CRD42019080516 Available from: https://www.crd.york.ac.uk/prospero/display_record.php?ID=CRD42019080516
DATA AVAILABILITY STATEMENT
- van den Akker, M. , Buntinx, F. , & Knottnerus, J. A. (1996). Comorbidity or multimorbidity . European Journal of Medical Genetics , 2 ( 2 ), 65–70. 10.3109/13814789609162146 [ CrossRef ] [ Google Scholar ]
- Anttila, V. , Bulik‐Sullivan, B. , Finucane, H. K. , Walters, R. K. , Bras, J. , Duncan, L. , & Murray, R. (2018). Analysis of shared heritability in common disorders of the brain . Science , 360 ( 6395 ), eaap8757. 10.1126/science.aap8757 [ PMC free article ] [ PubMed ] [ CrossRef ] [ Google Scholar ]
- Bernstein, G. A. (1991). Comorbidity and severity of anxiety and depressive disorders in a clinic sample . Journal of the American Academy of Child and Adolescent Psychiatry , 30 ( 1 ), 43–50. 10.1097/00004583-199101000-00007 [ PubMed ] [ CrossRef ] [ Google Scholar ]
- Borenstein, M. , Higgins, J. P. , Hedges, L. V. , & Rothstein, H. R. (2017). Basics of meta‐analysis: I(2) is not an absolute measure of heterogeneity . Research Synthesis Methods , 8 ( 1 ), 5–18. 10.1002/jrsm.1230 [ PubMed ] [ CrossRef ] [ Google Scholar ]
- Brent, D. A. , Kolko, D. J. , Birmaher, B. , Baugher, M. , Bridge, J. , Roth, C. , & Holder, D. (1998). Predictors of treatment efficacy in a clinical trial of three psychosocial treatments for adolescent depression . Journal of the American Academy of Child and Adolescent Psychiatry , 37 ( 9 ), 906–914. 10.1097/00004583-199809000-00010 [ PubMed ] [ CrossRef ] [ Google Scholar ]
- Coryell, W. , Endicott, J. , Andreasen, N. C. , Keller, M. B. , Clayton, P. J. , Hirschfeld, R. M. , & Winokur, G. (1988). Depression and panic attacks: The significance of overlap as reflected in follow‐up and family study data . American Journal of Psychiatry , 145 ( 3 ), 293–300. 10.1176/ajp.145.3.293 [ PubMed ] [ CrossRef ] [ Google Scholar ]
- DerSimonian, R. , & Laird, N. (1986). Meta‐analysis in clinical trials . Controlled Clinical Trials , 7 ( 3 ), 177–188. [ PubMed ] [ Google Scholar ]
- DerSimonian, R. , & Laird, N. (2015). Meta‐analysis in clinical trials revisited . Contemporary Clinical Trials , 45 , 139–145. 10.1016/j.cct.2015.09.002 [ PMC free article ] [ PubMed ] [ CrossRef ] [ Google Scholar ]
- Egger, M. , Davey Smith, G. , Schneider, M. , & Minder, C. (1997). Bias in meta‐analysis detected by a simple, graphical test . British Medical Journal , 315 ( 7109 ), 629–634. 10.1136/bmj.315.7109.629 [ PMC free article ] [ PubMed ] [ CrossRef ] [ Google Scholar ]
- Elm, E. , Altman, D. G. , Egger, M. , Pocock, S. J. , Gotzsche, P. C. , & Vandenbroucke, J. P. (2007). The strengthening the reporting of observational studies in epidemiology (STROBE) statement: Guidelines for reporting observational studies . PLOS Medicine , 4 ( 10 ):e296von. http://www.ncbi.nlm.nih.gov/entrez/query.fcgi?cmd=Retrieve&db=PubMed&dopt=Citation&list_uids=17941714 [ PMC free article ] [ PubMed ] [ Google Scholar ]
- Emslie, G. J. , Weinberg, W. A. , & Mayes, T. L. (1998). Treatment of children with antidepressants: focus on selective serotonin reuptake inhibitors . Depression and Anxiety , 8 ( Suppl. 1 ), 13–17. [ PubMed ] [ Google Scholar ]
- Ferrari, A. J. , Charlson, F. J. , Norman, R. E. , Patten, S. B. , Freedman, G. , Murray, C. J. , & Whiteford, H. A. (2013). Burden of depressive disorders by country, sex, age, and year: findings from the global burden of disease study 2010 . PLOS Medicine , 10 ( 11 ), e1001547. 10.1371/journal.pmed.1001547 [ PMC free article ] [ PubMed ] [ CrossRef ] [ Google Scholar ]
- Gureje, O. (2009). The pattern and nature of mental‐physical comorbidity: Specific or general. In Von Korff M. R., Scott K. M., & Gureje O. (Eds.), Global perspectives on mental‐physical comorbidity in the WHO World Mental Health surveys (first ed., pp. 51–83). Cambridge University Press. [ Google Scholar ]
- Hyman, S. E. (2019). New evidence for shared risk architecture of mental disorders . JAMA Psychiatry , 76 ( 3 ), 235–236. 10.1001/jamapsychiatry.2018.4269 [ PubMed ] [ CrossRef ] [ Google Scholar ]
- Kendler, K. S. , Aggen, S. H. , Knudsen, G. P. , Røysamb, E. , Neale, M. C. , & Reichborn‐Kjennerud, T. (2011). The structure of genetic and environmental risk factors for syndromal and subsyndromal common DSM‐IV axis I and all axis II disorders . American Journal of Psychiatry , 168 ( 1 ), 29–39. 10.1176/appi.ajp.2010.10030340 [ PMC free article ] [ PubMed ] [ CrossRef ] [ Google Scholar ]
- Kessler, R. C. , Davis, C. G. , & Kendler, K. S. (1997). Childhood adversity and adult psychiatric disorder in the US National Comorbidity Survey . Psychological Medicine , 27 ( 5 ), 1101–1119. 10.1017/s0033291797005588 [ PubMed ] [ CrossRef ] [ Google Scholar ]
- Kessler, R. C. , Nelson, C. B. , McGonagle, K. A. , Liu, J. , Swartz, M. , & Blazer, D. G. (1996). Comorbidity of DSM‐III‐R major depressive disorder in the general population: Results from the US National Comorbidity Survey . British Journal of Psychiatry , 168 (Suppl. 30) , 17–30. https://search.ebscohost.com/login.aspx?direct=true&AuthType=ip,athens&db=psyh&AN=1996-05073-002&site=eds-live [ PubMed ] [ Google Scholar ]
- Kessler, R. C. , Ormel, J. , Petukhova, M. , McLaughlin, K. A. , Green, J. G. , Russo, L. J. , & Ustün, T. B. (2011). Development of lifetime comorbidity in the World Health Organization world mental health surveys . Archives of General Psychiatry , 68 ( 1 ), 90–100. 10.1001/archgenpsychiatry.2010.180 [ PMC free article ] [ PubMed ] [ CrossRef ] [ Google Scholar ]
- Kessler, R. C. , & Ustun, T. B. (2004). The World Mental Health (WMH) Survey Initiative Version of the World Health Organization (WHO) Composite International Diagnostic Interview (CIDI) , International Journal of Methods in Psychiatric Research ( 12 ) 2 , 93–120. [ PMC free article ] [ PubMed ] [ Google Scholar ]
- Lemstra, M. , Neudorf, C. , D'Arcy, C. , Kunst, A. , Warren, L. M. , & Bennett, N. R. (2008). A systematic review of depressed mood and anxiety by SES in youth aged 10‐15 years . Canadian Journal of Public Health , 99 ( 2 ), 125–129. [ PMC free article ] [ PubMed ] [ Google Scholar ]
- Levey, D. F. , Gelernter, J. , Polimanti, R. , Zhou, H. , Cheng, Z. , Aslan, M. , & Stein, M. B. (2020). Reproducible genetic risk loci for anxiety: Results from ∼200,000 participants in the Million Veteran Program . American Journal of Psychiatry , 177 ( 3 ), 223–232. 10.1176/appi.ajp.2019.19030256 [ PMC free article ] [ PubMed ] [ CrossRef ] [ Google Scholar ]
- Lewinsohn, P. M. , Gotlib, I. H. , & Seeley, J. R. (1995). Adolescent psychopathology: IV. Specificity of psychosocial risk factors for depression and substance abuse in older adolescents . Journal of the American Academy of Child and Adolescent Psychiatry , 34 ( 9 ), 1221–1229. 10.1097/00004583-199509000-00021 [ PubMed ] [ CrossRef ] [ Google Scholar ]
- Lewinsohn, P. M. , Rohde, P. , & Seeley, J. R. (1995). Adolescent psychopathology: III. The clinical consequences of comorbidity . Journal of the American Academy of Child and Adolescent Psychiatry , 34 ( 4 ), 510–519. 10.1097/00004583-199504000-00018 [ PubMed ] [ CrossRef ] [ Google Scholar ]
- McGrath, J. , Lim, C. C. W. , Plana‐Ripoll, O., Y., H. , Agerbo, E. , Momen, N. , & Kessler, R. C. (2020). Comorbidity within mental disorders: A comprehensive analysis based on 145,990 survey respondents from 27 countries . Epidemiology and Psychiatric Sciences , 29 , e153. In press (accepted July 03, 2020). [ PMC free article ] [ PubMed ] [ Google Scholar ]
- McGrath, J. , Saha, S. , Welham, J. , El Saadi, O. , MacCauley, C. , & Chant, D. (2004). A systematic review of the incidence of schizophrenia: the distribution of rates and the influence of sex, urbanicity, migrant status and methodology . BMC Medicine , 2 ( 1 ), 13–35. http://www.ncbi.nlm.nih.gov/entrez/query.fcgi?cmd=Retrieve&db=PubMed&dopt=Citation&list_uids=15115547 [ PMC free article ] [ PubMed ] [ Google Scholar ]
- Melton, T. H. , Croarkin, P. E. , Strawn, J. R. , & McClintock, S. M. (2016). Comorbid anxiety and depressive symptoms in children and adolescents: A systematic review and analysis . Journal of Psychiatric Practice , 22 ( 2 ), 84–98. 10.1097/pra.0000000000000132 [ PMC free article ] [ PubMed ] [ CrossRef ] [ Google Scholar ]
- Moffitt, T. E. , Harrington, H. , Caspi, A. , Kim‐Cohen, J. , Goldberg, D. , Gregory, A. M. , & Poulton, R. (2007). Depression and generalized anxiety disorder: cumulative and sequential comorbidity in a birth cohort followed prospectively to age 32 years . Archives of General Psychiatry , 64 ( 6 ), 651–660. 10.1001/archpsyc.64.6.651 [ PubMed ] [ CrossRef ] [ Google Scholar ]
- Moher, D. , Shamseer, L. , Clarke, M. , Ghersi, D. , Liberati, A. , Petticrew, M. , & Stewart, L. A. (2015). Preferred reporting items for systematic review and meta‐analysis protocols (PRISMA‐P) 2015 statement . Systematic Review , 4 , 1. 10.1186/2046-4053-4-1 [ PMC free article ] [ PubMed ] [ CrossRef ] [ Google Scholar ]
- Nabavi, B. , Mitchell, A. J. , & Nutt, D. (2015). A lifetime prevalence of comorbidity between bipolar affective disorder and anxiety disorders: A meta‐analysis of 52 interview‐based studies of psychiatric population . EBioMedicine , 2 ( 10 ), 1405–1419. 10.1016/j.ebiom.2015.09.006 [ PMC free article ] [ PubMed ] [ CrossRef ] [ Google Scholar ]
- Pavlova, B. , Perlis, R. H. , Alda, M. , & Uher, R. (2015). Lifetime prevalence of anxiety disorders in people with bipolar disorder: A systematic review and meta‐analysis . Lancet Psychiatry , 2 ( 8 ), 710–717. 10.1016/s2215-0366(15)00112-1 [ PubMed ] [ CrossRef ] [ Google Scholar ]
- Peters, J. , & Mengersen, K. (2008). Selective reporting of adjusted estimates in observational epidemiology studies: Reasons and implications for meta‐analyses . Evaluation and the Health Professions , 31 ( 4 ), 370–389. 10.1177/0163278708324438 [ PubMed ] [ CrossRef ] [ Google Scholar ]
- Plana‐Ripoll, O. , Pedersen, C. B. , Holtz, Y. , Benros, M. E. , Dalsgaard, S. , de Jonge, P. , & McGrath, J. J. (2019). Exploring comorbidity within mental disorders among a Danish national population . JAMA Psychiatry , 76 ( 3 ), 259–270. 10.1001/jamapsychiatry.2018.3658 [ PMC free article ] [ PubMed ] [ CrossRef ] [ Google Scholar ]
- Purves, K. L. , Coleman, J. R. I. , Meier, S. M. , Rayner, C. , Davis, K. A. S. , Cheesman, R. , & Eley, T. C. (2019). A major role for common genetic variation in anxiety disorders [published online ahead of print November 20, 2019]. Molecular Psychiatry . 10.1038/s41380-019-0559-1 [ PMC free article ] [ PubMed ] [ CrossRef ]
- Rohde, P. , Clarke, G. N. , Lewinsohn, P. M. , Seeley, J. R. , & Kaufman, N. K. (2001). Impact of comorbidity on a cognitive‐behavioral group treatment for adolescent depression . Journal of the American Academy of Child and Adolescent Psychiatry , 40 ( 7 ), 795–802. 10.1097/00004583-200107000-00014 [ PubMed ] [ CrossRef ] [ Google Scholar ]
- Rytwinski, N. K. , Scur, M. D. , Feeny, N. C. , & Youngstrom, E. A. (2013). The co‐occurrence of major depressive disorder among individuals with posttraumatic stress disorder: a meta‐analysis . Journal of Traumatic Stress , 26 ( 3 ), 299–309. 10.1002/jts.21814 [ PubMed ] [ CrossRef ] [ Google Scholar ]
- Saha, S. , Chant, D. , & McGrath, J. (2007). A systematic review of mortality in schizophrenia: Is the differential mortality gap worsening over time? Archives of General Psychiatry , 64 ( 10 ), 1123–1131. http://www.ncbi.nlm.nih.gov/entrez/query.fcgi?cmd=Retrieve&db=PubMed&dopt=Citation&list_uids=17909124 [ PubMed ] [ Google Scholar ]
- Saha, S. , Chant, D. , Welham, J. , & McGrath, J. (2005). A Systematic Review of the Prevalence of Schizophrenia . PLOS Medicine , 2 ( 5 ), e141. http://www.ncbi.nlm.nih.gov/entrez/query.fcgi?cmd=Retrieve&db=PubMed&dopt=Citation&list_uids=15916472 [ PMC free article ] [ PubMed ] [ Google Scholar ]
- Saha, S. , Lim, C. C. W. , & McGrath, J. (2019). Comorbidity within mental disorders—A protocol for a systematic review and meta‐analysis . Retrieved from https://www.crd.york.ac.uk/prospero/display_record.php?ID=CRD42019080516
- Scott, K. M. , de Jonge, P. , Stein, D. J. , & Kessler, R. C. (2018). Mental disorders around the world: Facts and figures from the WHO World Mental Health Surveys (Vol. 1 , 1st(1–384). Cambridge, UK: Cambridge University Press. [ Google Scholar ]
- Shamseer, L. , Moher, D. , Clarke, M. , Ghersi, D. , Liberati, A. , Petticrew, M. , & Stewart, L. A. (2015). Preferred reporting items for systematic review and meta‐analysis protocols (PRISMA‐P) 2015: Elaboration and explanation . British Medical Journal , 349 , g7647. 10.1136/bmj.g7647 [ PubMed ] [ CrossRef ] [ Google Scholar ]
- Stroup, D. F. , Berlin, J. A. , Morton, S. C. , Olkin, I. , Williamson, G. D. , Rennie, D. , & Thacker, S. B. (2000). Meta‐analysis of observational studies in epidemiology: a proposal for reporting. Meta‐analysis of observational studies in epidemiology (MOOSE) group . Journal of the American Medical Association , 283 ( 15 ), 2008–2012. 10.1001/jama.283.15.2008 [ PubMed ] [ CrossRef ] [ Google Scholar ]
- Waffenschmidt, S. , Hermanns, T. , Gerber‐Grote, A. , & Mostardt, S. (2017). No suitable precise or optimized epidemiologic search filters were available for bibliographic databases . Journal of Clinical Epidemiology , 82 , 112–118. 10.1016/j.jclinepi.2016.08.008 [ PubMed ] [ CrossRef ] [ Google Scholar ]
- Zavaglia, E. , & Bergeron, L. (2017). Systematic review of comorbidity between DSM disorders and depression according to age and sex in youth . Canadian Psychology , 58 ( 2 ), 124–139. 10.1037/cap0000085 [ CrossRef ] [ Google Scholar ]
Anxiety and Related Disorders
- First Online: 06 October 2022
Cite this chapter
- Rosemary Purcell 2 , 3 ,
- Courtney C. Walton 2 , 3 ,
- Claudia L. Reardon 4 &
- Simon M. Rice 2 , 3
995 Accesses
Anxiety is an almost ubiquitous experience in high-performance sport. Managing competitive performance anxiety has been a focus of sport psychology for many decades, but more recently, attention has turned to the experience of clinical forms of anxiety in elite athletes. Research demonstrates that athletes report experiencing anxiety symptoms and disorders at rates similar to those observed in the general population, with a range of both sport-related factors and general risk factors associated with anxiety in this population. Anxiety symptoms and disorders can compromise the affected athlete’s mental well-being and psychosocial functioning, and potentially their performance. Assessment and treatment of anxiety and related conditions (such as obsessive-compulsive disorder) need to consider the individual athlete’s physiology and wider psychosocial contexts. This chapter examines the current knowledge on anxiety disorders in athletes, including how specific types of anxiety (such as generalized anxiety disorder or social anxiety disorder) may manifest in elite athletes, and how treatment options may be impacted by sport-related considerations.
This is a preview of subscription content, log in via an institution to check access.
Access this chapter
- Available as EPUB and PDF
- Read on any device
- Instant download
- Own it forever
- Compact, lightweight edition
- Dispatched in 3 to 5 business days
- Free shipping worldwide - see info
- Durable hardcover edition
Tax calculation will be finalised at checkout
Purchases are for personal use only
Institutional subscriptions
Similar content being viewed by others
Anxiety in Athletes
Anxiety in Sport
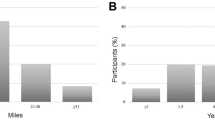
Correlation of Pre-Race Anxiety Using the Generalized Anxiety Disorder 2-Item Scale
Slade T, Johnston A, Oakley Browne MA, Andrews G, Whiteford H. 2007 national survey of mental health and wellbeing: methods and key findings. Aust N Z J Psychiatry. 2009;43(7):594–605.
Article PubMed Google Scholar
Kessler RC, Chiu WT, Demler O, Walters EE. Prevalence, severity, and comorbidity of 12-month DSM-IV disorders in the National Comorbidity Survey Replication. Arch Gen Psychiatry. 2005;62(6):617–27.
Article PubMed PubMed Central Google Scholar
Baxter AJ, Scott KM, Vos T, Whiteford HA. Global prevalence of anxiety disorders: a systematic review and meta-regression. Psychol Med. 2013;43(5):897.
Article CAS PubMed Google Scholar
Rice SM, Gwyther K, Santesteban-Echarri O, et al. Determinants of anxiety in elite athletes: a systematic review and meta-analysis. Br J Sports Med. 2019;53(11):722–30.
Rice SM, Purcell R, De Silva S, et al. The mental health of elite athletes: a narrative systematic review. Sports Med. 2016;46(9):1333–53.
Reardon CL, Gorczynski P, Hainline B, et al. Anxiety disorders in athletes: a clinical review. Adv Psychiatry Behav Health. 2021;1:149–60.
Article Google Scholar
Reardon CL, Hainline B, Aron CM, et al. Mental health in elite athletes: International Olympic Committee consensus statement. Br J Sports Med. 2019;53(11):667–99. https://doi.org/10.1136/bjsports-2019-100715 .
Merikangas KR, He J, Burstein M, et al. Lifetime prevalence of mental disorders in US adolescents: results from the National Comorbidity Study-Adolescent Supplement (NCS-A). J Am Acad Child Adolesc Psychiatry. 2010;49(10):980–9.
Lijster JM, Dierckx B, Utens EM, et al. The age of onset of anxiety disorders. Can J Psychiatr. 2017;62(4):237–46.
Penninx BW, Pine DS, Holmes EA, Reif A. Anxiety disorders. Lancet. 2021;397:914–27.
Remes O, Brayne C, Van Der Linde R, Lafortune L. A systematic review of reviews on the prevalence of anxiety disorders in adult populations. Brain Behav. 2016;6(7):e00497.
Craske MG, Stein MB, Eley TC, et al. Anxiety disorders. Nat Rev Dis Primers. 2017;4(3):17024.
Mellalieu SD, Hanton S, Fletcher D. A competitive anxiety review: recent directions in sport psychology research. Lit Rev Sport Psychol. 2006;2006:1–45.
Google Scholar
Ford JL, Ildefonso K, Jones ML, Arvinen-Barrow M. Sport-related anxiety: current insights. Open Access J Sports Med. 2017;8:205–12.
Schaal K, Tafflet M, Nassif H, et al. Psychological balance in high level athletes: gender-based differences and sport-specific patterns. PLoS One. 2011;6:e19007.
Article CAS PubMed PubMed Central Google Scholar
Somers JM, Goldner EM, Waraich P, et al. Prevalence and incidence studies of anxiety disorders: a systematic review of the literature. Can J Psychiatr. 2006;51:100–13.
Rice SM, Gwyther K, Santestebanecha O, et al. Determinants of anxiety in elite athletes: a systematic review and meta-analysis. Br J Sports Med. 2019;53:722–30.
Gouttebarge V, Aoki H, Verhagen EA, et al. A 12-month prospective cohort study of symptoms of common mental disorders among European professional footballers. Clin J Sport Med. 2017;27:487–92.
Hulley AJ, Hill AJ. Eating disorders and health in elite women distance runners. Int J Eat Disord. 2001;30:312–7.
Reardon CL. Psychiatric comorbidities in sports. Neurol Clin. 2017;35:537–46.
Junge A, Prinz B. Depression and anxiety symptoms in 17 teams of female football players including 10 German first league teams. Br J Sports Med. 2019;53:471–7.
Forsdyke D, Smith A, Jones M, Gledhill A. Psychosocial factors associated with outcomes of sports injury rehabilitation in competitive athletes: a mixed studies systematic review. Br J Sports Med. 2016;50(9):537. https://doi.org/10.1136/bjsports-2015-094850 .
Nixdorf I, Frank R, Beckmann J. Comparison of athletes’ proneness to depressive symptoms in individual and team sports: research on psychological mediators in junior elite athletes. Front Psychol. 2016;7:893.
Purcell R, Gwyther K, Rice SR. Mental health in elite athletes: Increased awareness requires an early intervention framework to respond to athlete needs. Sports Med Open. 2019;5(1):46–54.
Du Preez EJ, Graham KS, Gan TY, Moses B, Ball C, Kuah DE. Depression, anxiety, and alcohol use in elite rugby league players over a competitive season. Clin J Sport Med. 2017;27(6):530.
Junge A, Feddermann-Demont N. Prevalence of depression and anxiety in top-level male and female football players. BMJ Open Sport Exerc Med. 2016;19:e000087.
Li C, Fan R, Sun J, Li G. Risk and protective factors of generalized anxiety disorder of elite collegiate athletes: a cross-sectional study. Front Public Health. 2021;9:607800.
Akesdotter C, Kentta G, Eloranta S, Franck J. The prevalence of mental health problems in elite athletes. Aust J Sci Med Sport. 2020;23(4):329–35.
Gulliver A, Griffiths KM, Mackinnon A, Batterham PJ, Stanimirovic R. The mental health of Australian elite athletes. Aust J Sci Med Sport. 2015;18(3):255–61.
McLoughlin E, Fletcher D, Slavich GM, Arnold R, Moore LJ. Cumulative lifetime stress exposure, depression, anxiety, and well-being in elite athletes: a mixed-method study. Psychol Sport Exerc. 2020;52:101823.
Bandelow B, Michaelis S. Epidemiology of anxiety disorders in the 21st century. Dialogues Clin Neurosci. 2015;17:327–35.
Chang CJ, Putukian M, Aerni G, et al. Mental health issues and psychological factors in athletes: detection, management, effect on performance, and prevention: American Medical Society for Sports Medicine Position Statement. Clin J Sport Med. 2020;30(2):e61–87.
Cameron OG, Hudson CJ. Influence of exercise on anxiety level in patients with anxiety disorders. Psychosomatics. 1986;27:720–3.
American Psychiatric Association. Diagnostic and statistical manual of mental disorders. 5th ed. Arlington: APA; 2013.
Book Google Scholar
Cromer L, Kaier E, Davis J, Stunk K, Stewart SE. OCD in college athletes. Am J Psychiatr. 2017;174(6):595–7.
Bleak JL, Frederick CM. Superstitious behavior in sport: levels of effectiveness and determinants of use in three collegiate sports. J Sport Behav. 1998;21:1–15.
Dömötör Z, Ruíz-Barquín R, Szabo A. Superstitious behavior in sport: a literature review. Scand J Psychol. 2016;57:368–82.
Rupprecht AG, Tran US, Gröpel P. The effectiveness of pre-performance routines in sports: a meta-analysis. Int Rev Sport Exerc Psychol. 2021;2021:1–26.
Cho S, Choi H, Youngsook K. The relationship between perceived coaching behaviors, competitive trait anxiety, and athlete burnout: a cross-sectional study. Int J Environ Res Public Health. 2019;16(8):1424.
Article PubMed Central Google Scholar
Encel K, Mesagno C, Brown H. Facebook use and its relationship with sport anxiety. J Sports Sci. 2017;35(8):756–61.
Gouttebarge V, Bindra A, Blauwet C, et al. International Olympic Committee (IOC) sport mental health assessment tool 1 (SMHAT-1) and sport mental health recognition tool 1 (SMHRT-1): towards better support of athletes’ mental health. Br J Sports Med. 2020;55:30–7.
Spitzer RL, Kroenke K, Williams JBW, et al. A brief measure for assessing generalized anxiety disorder: The GAD-7. Arch Intern Med. 2006;166:1092–7.
Beck AT, Steer RA. Beck anxiety inventory manual. San Antonio: Psychological Corporation; 1993.
Goodman WK, Price LH, Rasmussen SA, et al. The yale-brown obsessive compulsive scale: I. Development, use, and reliability. Arch Gen Psychiatry. 1989;46(11):1006–11.
Smith RE, Smoll FL, Cumming SP, et al. Measurement of multidimensional sport performance anxiety in children and adults: the sport anxiety scale-2. J Sport Exerc Psychol. 2006;28(4):479–501.
Brown TA, Barlow DH. Anxiety and related disorders interview schedule for DSM-5 (ADIS-5). Oxford: Oxford University Press; 2014.
Reardon CL, Factor RM. Sport psychiatry: a systematic review of diagnosis and medical treatment of mental illness in athletes. Sports Med. 2010;40:961–80.
Mountjoy M, Brackenridge C, Arrington M, et al. International Olympic Committee consensus statement: harassment and abuse (non-accidental violence) in sport. Br J Sports Med. 2016;50(17):1019.
Stillman MA, Glick ID, McDuff D, et al. Psychotherapy for mental health symptoms and disorders in elite athletes: a narrative review. Br J Sports Med. 2019;53(12):767–71.
Purcell R, Chevroulet C, Pilkington V, Rice S. What works for mental health in sporting teams? An evidence guide for best practice in mental health promotion and early intervention. Melbourne: Orygen; 2020.
Moreton A, Wahesh E, Schmidt CD. Indirect effect of mindfulness on psychological distress via sleep hygiene in division I college student athletes. J Am Coll Heal. 2020;2020:1–5.
Henriksen K, Hansen J, Larsen CH. Mindfulness and acceptance in sport: How to help athletes perform and thrive under pressure. London: Routledge; 2019.
Mosewich AD, Ferguson LJ, McHugh T-LF, Kowalski KC. Enhancing capacity: integrating self-compassion in sport. J Sport Psychol Action. 2019;10(4):235–43.
Walton CC, Baranoff J, Gilbert P, Kirby J. Self-compassion, social rank, and psychological distress in athletes of varying competitive levels. Psychol Sport Exerc. 2020;50:101733.
Johnston A, McAllister-Williams RH. Psychotropic drug prescribing. In: Currie A, Owen B, editors. Sports psychiatry. Oxford: Oxford University Press; 2016. p. 133–43.
Chapter Google Scholar
Download references
Author information
Authors and affiliations.
Elite Sport and Mental Health, Orygen, Melbourne, VIC, Australia
Rosemary Purcell, Courtney C. Walton & Simon M. Rice
Centre for Youth Mental Health, The University of Melbourne, Melbourne, VIC, Australia
Department of Psychiatry, University of Wisconsin School of Medicine and Public Health, Madison, WI, USA
Claudia L. Reardon
You can also search for this author in PubMed Google Scholar
Corresponding author
Correspondence to Rosemary Purcell .
Editor information
Editors and affiliations, rights and permissions.
Reprints and permissions
Copyright information
© 2022 The Author(s), under exclusive license to Springer Nature Switzerland AG
About this chapter
Purcell, R., Walton, C.C., Reardon, C.L., Rice, S.M. (2022). Anxiety and Related Disorders. In: Reardon, C.L. (eds) Mental Health Care for Elite Athletes. Springer, Cham. https://doi.org/10.1007/978-3-031-08364-8_7
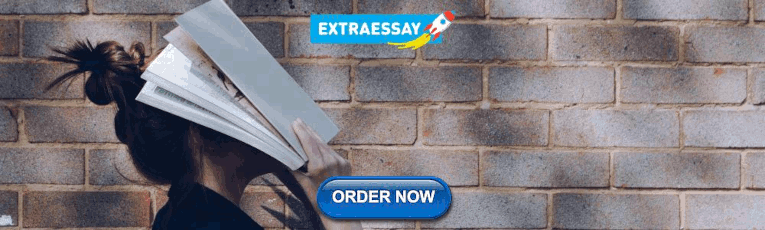
Download citation
DOI : https://doi.org/10.1007/978-3-031-08364-8_7
Published : 06 October 2022
Publisher Name : Springer, Cham
Print ISBN : 978-3-031-08363-1
Online ISBN : 978-3-031-08364-8
eBook Packages : Behavioral Science and Psychology Behavioral Science and Psychology (R0)
Share this chapter
Anyone you share the following link with will be able to read this content:
Sorry, a shareable link is not currently available for this article.
Provided by the Springer Nature SharedIt content-sharing initiative
- Publish with us
Policies and ethics
- Find a journal
- Track your research
Introduction
Chapter outline.
Clive Wearing is an accomplished musician who lost his ability to form new memories when he became sick at the age of 46. While he can remember how to play the piano perfectly, he cannot remember what he ate for breakfast just an hour ago (Sacks, 2007). James Wannerton experiences a taste sensation that is associated with the sound of words. His former girlfriend’s name tastes like rhubarb (Mundasad, 2013). John Nash is a brilliant mathematician and Nobel Prize winner. However, while he was a professor at MIT, he would tell people that the New York Times contained coded messages from extraterrestrial beings that were intended for him. He also began to hear voices and became suspicious of the people around him. Soon thereafter, Nash was diagnosed with schizophrenia and admitted to a state-run mental institution (O’Connor & Robertson, 2002). Nash was the subject of the 2001 movie A Beautiful Mind . Why did these people have these experiences? How does the human brain work? And what is the connection between the brain’s internal processes and people’s external behaviors? This textbook will introduce you to various ways that the field of psychology has explored these questions.
As an Amazon Associate we earn from qualifying purchases.
This book may not be used in the training of large language models or otherwise be ingested into large language models or generative AI offerings without OpenStax's permission.
Want to cite, share, or modify this book? This book uses the Creative Commons Attribution License and you must attribute OpenStax.
Access for free at https://openstax.org/books/psychology/pages/1-introduction
- Authors: Rose M. Spielman, Kathryn Dumper, William Jenkins, Arlene Lacombe, Marilyn Lovett, Marion Perlmutter
- Publisher/website: OpenStax
- Book title: Psychology
- Publication date: Dec 8, 2014
- Location: Houston, Texas
- Book URL: https://openstax.org/books/psychology/pages/1-introduction
- Section URL: https://openstax.org/books/psychology/pages/1-introduction
© Feb 9, 2022 OpenStax. Textbook content produced by OpenStax is licensed under a Creative Commons Attribution License . The OpenStax name, OpenStax logo, OpenStax book covers, OpenStax CNX name, and OpenStax CNX logo are not subject to the Creative Commons license and may not be reproduced without the prior and express written consent of Rice University.
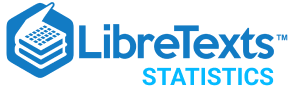
- school Campus Bookshelves
- menu_book Bookshelves
- perm_media Learning Objects
- login Login
- how_to_reg Request Instructor Account
- hub Instructor Commons
Margin Size
- Download Page (PDF)
- Download Full Book (PDF)
- Periodic Table
- Physics Constants
- Scientific Calculator
- Reference & Cite
- Tools expand_more
- Readability
selected template will load here
This action is not available.
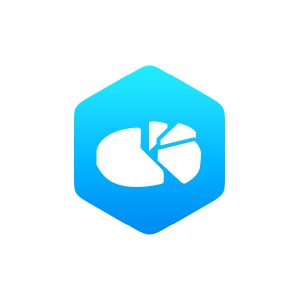
14.7: Practice on Anxiety and Depression
- Last updated
- Save as PDF
- Page ID 22152
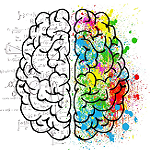
- Michelle Oja
- Taft College
\( \newcommand{\vecs}[1]{\overset { \scriptstyle \rightharpoonup} {\mathbf{#1}} } \)
\( \newcommand{\vecd}[1]{\overset{-\!-\!\rightharpoonup}{\vphantom{a}\smash {#1}}} \)
\( \newcommand{\id}{\mathrm{id}}\) \( \newcommand{\Span}{\mathrm{span}}\)
( \newcommand{\kernel}{\mathrm{null}\,}\) \( \newcommand{\range}{\mathrm{range}\,}\)
\( \newcommand{\RealPart}{\mathrm{Re}}\) \( \newcommand{\ImaginaryPart}{\mathrm{Im}}\)
\( \newcommand{\Argument}{\mathrm{Arg}}\) \( \newcommand{\norm}[1]{\| #1 \|}\)
\( \newcommand{\inner}[2]{\langle #1, #2 \rangle}\)
\( \newcommand{\Span}{\mathrm{span}}\)
\( \newcommand{\id}{\mathrm{id}}\)
\( \newcommand{\kernel}{\mathrm{null}\,}\)
\( \newcommand{\range}{\mathrm{range}\,}\)
\( \newcommand{\RealPart}{\mathrm{Re}}\)
\( \newcommand{\ImaginaryPart}{\mathrm{Im}}\)
\( \newcommand{\Argument}{\mathrm{Arg}}\)
\( \newcommand{\norm}[1]{\| #1 \|}\)
\( \newcommand{\Span}{\mathrm{span}}\) \( \newcommand{\AA}{\unicode[.8,0]{x212B}}\)
\( \newcommand{\vectorA}[1]{\vec{#1}} % arrow\)
\( \newcommand{\vectorAt}[1]{\vec{\text{#1}}} % arrow\)
\( \newcommand{\vectorB}[1]{\overset { \scriptstyle \rightharpoonup} {\mathbf{#1}} } \)
\( \newcommand{\vectorC}[1]{\textbf{#1}} \)
\( \newcommand{\vectorD}[1]{\overrightarrow{#1}} \)
\( \newcommand{\vectorDt}[1]{\overrightarrow{\text{#1}}} \)
\( \newcommand{\vectE}[1]{\overset{-\!-\!\rightharpoonup}{\vphantom{a}\smash{\mathbf {#1}}}} \)
Our first example will focus on variables related to health: mental health. Our hypothesis testing procedure follows the same four-step process as before, starting with our research and null hypotheses.
Anxiety and depression are often reported to be highly linked (or “co-morbid”). We will test whether higher scores on an anxiety scale are related to higher scales on a depression scale, lower scores on the depression scale, or not related to scores on a depression scale (meaning that the two variables do not vary together). We have scores on an anxiety scale and a depression scale for a group of 10 people. Because both scales are measured by numbers, this means that we have two different quantitative variables for the same people; perfect for a correlational statistical analysis!
Step 1: State the Hypotheses
For a research hypothesis for correlation analyses, we are predicting that there will be a positive linear relationship or a negative linear relationship. A positive linear relationship means that the two variables vary in the same direction (when one goes up, the other also goes up), while a negative linear relationship means that the two variables vary in opposite directions (when one goes up, the other goes down). We keep the word "linear" in the hypotheses to keep reminding ourselves that Pearson's r can only detect linear relationships. If we expect something else, then Pearson's r is not the correct analysis.
Okay, let's start with a research hypothesis. If the two variables are co-morbid, do you think that the would vary in the same direction or in opposite directions? Use that to determine your research hypothesis.
Example \(\PageIndex{1}\)
What is a research hypothesis for this scenario?
The research hypothesis should be: There is positive linear relationship between anxiety scores and depression scores.
Look back at the research hypothesis above in Example \(\PageIndex{1}\). Does it include the names of both variables (what was measured)? Yes. Does it state whether the relationship will be positive or negative? Yes. Does it include the phrase "linear relationship"? Yes. Yay, we have all of the important parts of a research hypothesis! Does it say anything about means? No, which is fine; correlations aren't comparing means. Although knowing the means and standard deviations of each variable might be interesting, that descriptive information is not really necessary to test whether the two variables are linearly related.
Let's move on to the null hypothesis. What do you think that will look like?
Example \(\PageIndex{2}\)
What is a null hypothesis for this scenario?
The null hypothesis should be: There is no linear relationship between anxiety scores and depression scores..
Notice that the variables are included again, and the word "linear".
Step 2: Find the Critical Values
The critical values for correlations come from the Table of Critical Values of r , which looks very similar to the \(t\)-table. Just like our \(t\)-table, the row is determined by our degrees of freedom. For correlations, we have \(N – 2\) degrees of freedom, rather than \(N – 1\) (why this is the case is not important). For our example, we have 10 people.
We were not given any information about the lev el of significance at which we should test our hypothesis, so we will assume \(α = 0.05\). From the table, we can see that at p = .05 level, the critical value of r Critical = 0.576. Thus, if our calculated correlation is greater than 0.576, it will be statistically significant and we would reject the null hypothesis. This is a rather high bar (remember, the guideline for a strong relation is \(r\) = 0.50); this is because we have so few people. Larger samples make it easier to find significant relations.
Step 3: Calculate the Test Statistic
We have laid out our hypotheses and the criteri a we will use to assess them, so now we can move on to our test statistic. Before we do that, we should first create a scatterplot of the data to make sure that the most likely form of our relation is in fact linear. Figure \(\PageIndex{2}\) below shows our data plotted out. Dr. MO is not convinced that there's a strong linear relationship, but there doesn't seem to be a strong curvilinear relationship, either. Dr. Foster thinks that the dots are, in fact, linearly related, so Pearson’s \(r\) is appropriate.
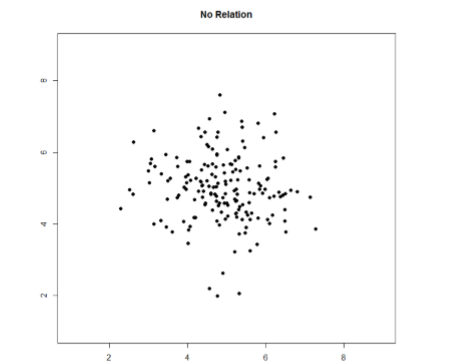
The data we gather from our participants is shown in Table \(\PageIndex{1}\). The sum for each variable is also provided so that it's easy to calculate the mean.
It's easiest to calculate the formula that we're using for correlations by using a table to find the difference from the mean for each participant and multiplying them. Let's start by finding the means.
Exercise \(\PageIndex{1}\)
What is the average depression score? What is the average anxiety score?
\[ \displaystyle \bar{X_D} = \dfrac{\sum X}{N} = \dfrac{31.63}{10} = 3.163 = 3.16 \nonumber \] \[ \displaystyle \bar{X_A} = \dfrac{\sum X}{N} = \dfrac{36.39}{10} = 3.639 =3.64 \nonumber \]
Now that you've done that, let's put the means and the standard deviations in Table \(\PageIndex{2}\). We can learn a littlebit about the two variables from this information alone. It looks like the participants might be more anxious than they are depressed. This conclusion is very tentative, however, for two reasons. First, we didn't conduct a t-test to see if the means are statistically significantly different from each other. Second, we don't actually know that the two scales have the same range! Maybe the Depression Scale ranges from 1-5, and the Anxiety Scale ranges from 0-10; we don't really have enough information to make any final determines about the means with only this table. However, it also appears that there is more variability in the Depression Scale than the Anxiety Scale, but again, we don't know that for sure unless we do some other statistical analyses.
But since we're trying to conduct a correlational analysis:
\[ r= \cfrac{ \left( \cfrac{\sum ((x_{Each} - \bar{X_x})*(y_{Each} - \bar{X_y}) ) }{(N-1)}\right) } {(s_x * s_y)} \nonumber \]
let's get going by filling in Table \(\PageIndex{3}\). Because we were provided the standard deviations, we do not need columns to square the differences from the means so those columns are not included in Table \(\PageIndex{3}\).
Example \(\PageIndex{3}\)
Fill in Table \(\PageIndex{3}\). Keep the negative signs!
Now it's Table \(\PageIndex{4}\):
The bottom row is the sum of each column. The difference scores for Depression sum to 0.03 and the differences scores fo Anxiety sum to -0.1; both of these are very close to 0 so, given rounding error, everything looks right so far. If you had a spreadsheet conduct all of your computations, your sums of the differences will be slightly different. However, it all sorta washes out because the final sum of the products is 2.22 no matter how many decimals you save.
Okay, let's look at the formula again to see what information we might still need:
\[ r= \cfrac{ \left( \cfrac{\sum ((x_{Each} - \bar{X_x})*(y_{Each} - \bar{X_y}) ) }{(N-1)}\right) } {(s_x \times s_y)} \nonumber \]
Hey, it looks like we have all of the numbers that we need! But let's replace the "x" and "y" with D for Depression and A for Anxiety. That gives us:
\[ r= \cfrac{ \left( \cfrac{\sum ((D_{Each} - \bar{X_D})*(A_{Each} - \bar{X_A}) ) }{(N-1)}\right) } {(s_D \times s_A)} \nonumber \]
Example \(\PageIndex{4}\)
Use the information provided and that we've calculated to complete the correlational analysis:
\[ r_{Filled In}= \cfrac{ \left( \cfrac{2.22 }{(10-1)}\right) } {(0.94 \times 0.38 )} \nonumber \]
It's pretty crazy, but all of that mess at the very top of the formula (the numerator of the numerator) is what we did in the table, so it boils down to 2.22. After that, it's pretty easy!
\[ r_{Parentheses}= \cfrac{ \left( \cfrac{2.22 }{9}\right) } {0.36} \nonumber \]
\[ r_{Divide}= \dfrac{ 0.25 } {0.36} \nonumber \]
\[ r = 0.69 \nonumber \].
If you use a spreadsheet to keep all of the decimal points, you might end up with r=0.68 or if you round differently you could get r=0.70. Since we're learning the process right now, these are all close enough.
So our calculated correlation between anxiety and depression is \(r=0.69\), which is a strong, positive correlation. Now we need to compare it to our critical value to see if it is also statistically significant.
Step 4: Make a Decision
Our critical value was r Critical = 0.576 and our calculated value was \(r Calc = 0.69\). Our calculated value was larger than our critical value, so we can reject the null hypothesis because this is still true:
(Critical \(<\) Calculated) \(=\) Reject null \(=\) There is a linear relationship. \(= p<.05 \)
(Critical \(>\) Calculated) \(=\) Retain null \(=\) There is not a linear relationship. \(= p>.05\)
The statistical sentence is similar to what we've been doing, so let's try that now:
Example \(\PageIndex{6}\)
What is the statistical sentence for the results for our correlational analysis?
The statistical sentence is: r(8)=0.69, p<.05
Because the Degrees of Freedom are N-2 for Pearson's r.
Okay, we're ready for the final write-up! Because our r = 0.69, a positive number, we can say that the linear relationship is positive.
Example \(\PageIndex{7}\)
Write up your conclusion. Don't forget to include the four requirements for reporting results !
"We hypothesized that there would be a positive linear relationship between depression scores (M=3.16) and anxiety scores (M=3.64). This hypothesis was supported (r(8)=0.69, p < .05). As depression scores increase, anxiety scores also increase. With our sample, depression and anxiety do appear to be co-morbid."
As we will discover in the next chapter, we can also add a sentence about predictions: "Based on these results, we can use a participant's depression score to predict their anxiety score."
Even though we are dealing with a very different type of data, our process of hypothesis testing has remained unchanged.
Let's try that again, but use the full Sum of Products table to calculate the standard deviations, too.
Faculty Resources
Assignments.
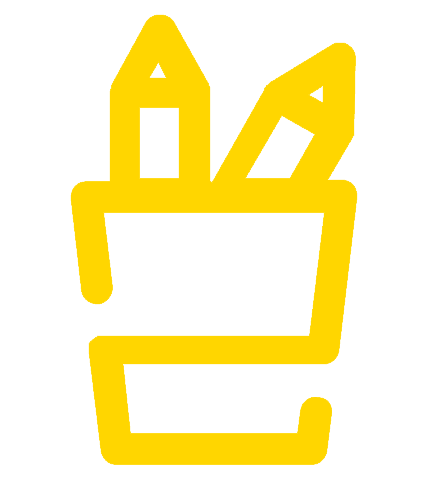
The assignments in this course are openly licensed, and are available as-is, or can be modified to suit your students’ needs. Selected answer keys are available to faculty who adopt Waymaker, OHM, or Candela courses with paid support from Lumen Learning. This approach helps us protect the academic integrity of these materials by ensuring they are shared only with authorized and institution-affiliated faculty and staff.
If you import this course into your learning management system (Blackboard, Canvas, etc.), the assignment will automatically be loaded into the assignment tool, where they may be adjusted, or edited there.
The assignment and discussion explanations are linked out to Google docs, so that they can be easily adjusted and customized for your own personal use. Assignments also come with rubrics and pre-assigned point values that may easily be edited or removed. Some of the discussions and assignments are larger, more time-consuming projects, so we recommend carefully reviewing them and including only the pieces that work well for your course . To do this, we recommend that you:
- Review the discussion and assignment instructions contained within the google doc.
- Make a copy of the google doc to make any personal edits or changes. Note that if you do make changes, you’ll want to replace the URL in the assignment page with the URL to your edited version.
- If you’d prefer, paste your specific instructions and rubric directly inside of your discussion or assignment page within the LMS (so that it no longer links out to the google doc.)
Note that each module contains at least one discussion and one assignment, with the discussion encouraging reflection and engagement with classmates about the material, and the assignment consisting of a somewhat larger task that has students create a product related to the content. Note that oftentimes the “assignments” are also housed inside of discussion forums so that students can share their finished products with their classmates. Because of this, you may want to select the option inside of your discussion forum to prevent students from seeing responses from others until they have first submitted their own.
- Assignments with Solutions. Provided by : Lumen Learning. License : CC BY: Attribution
- Pencil Cup. Authored by : IconfactoryTeam. Provided by : Noun Project. Located at : https://thenounproject.com/term/pencil-cup/628840/ . License : CC BY: Attribution
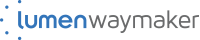
Thank you for visiting nature.com. You are using a browser version with limited support for CSS. To obtain the best experience, we recommend you use a more up to date browser (or turn off compatibility mode in Internet Explorer). In the meantime, to ensure continued support, we are displaying the site without styles and JavaScript.
- View all journals
- My Account Login
- Explore content
- About the journal
- Publish with us
- Sign up for alerts
- Open access
- Published: 08 February 2024
Disease clusters subsequent to anxiety and stress-related disorders and their genetic determinants
- Xin Han ORCID: orcid.org/0000-0003-2009-8503 1 , 2 , 3 na1 ,
- Qing Shen ORCID: orcid.org/0000-0002-7214-4797 4 , 5 , 6 na1 ,
- Can Hou ORCID: orcid.org/0000-0002-6903-6007 2 , 3 ,
- Huazhen Yang 2 , 3 ,
- Wenwen Chen 2 , 3 ,
- Yu Zeng 2 , 3 ,
- Yuanyuan Qu 2 , 3 ,
- Chen Suo 7 , 8 ,
- Weimin Ye ORCID: orcid.org/0000-0002-6859-4648 9 ,
- Fang Fang ORCID: orcid.org/0000-0002-3310-6456 6 ,
- Unnur A. Valdimarsdóttir ORCID: orcid.org/0000-0001-5382-946X 6 , 10 , 11 na2 &
- Huan Song ORCID: orcid.org/0000-0003-3845-8079 2 , 3 , 10 na2
Nature Communications volume 15 , Article number: 1209 ( 2024 ) Cite this article
2415 Accesses
1 Citations
1 Altmetric
Metrics details
- Epidemiology
- Psychiatric disorders
Anxiety/stress-related disorders have been associated with multiple diseases, whereas a comprehensive assessment of the structure and interplay of subsequent associated diseases and their genetic underpinnings is lacking. Here, we first identify 136, out of 454 tested, medical conditions associated with incident anxiety/stress-related disorders attended in specialized care using a population-based cohort from the nationwide Swedish Patient Register, comprising 70,026 patients with anxiety/stress-related disorders and 1:10 birth year- and sex-matched unaffected individuals. By combining findings from the comorbidity network and disease trajectory analyses, we identify five robust disease clusters to be associated with a prior diagnosis of anxiety/stress-related disorders, featured by predominance of psychiatric disorders, eye diseases, ear diseases, cardiovascular diseases, and skin and genitourinary diseases. These five clusters and their featured diseases are largely validated in the UK Biobank. GWAS analyses based on the UK Biobank identify 3, 33, 40, 4, and 16 significantly independent single nucleotide polymorphisms for the link to the five disease clusters, respectively, which are mapped to several distinct risk genes and biological pathways. These findings motivate further mechanistic explorations and aid early risk assessment for cluster-based disease prevention among patients with newly diagnosed anxiety/stress-related disorders in specialized care.
Similar content being viewed by others
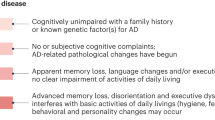
Alzheimer’s disease risk reduction in clinical practice: a priority in the emerging field of preventive neurology
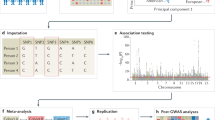
Genome-wide association studies
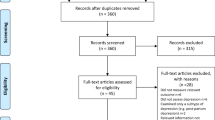
The serotonin theory of depression: a systematic umbrella review of the evidence
Introduction.
Anxiety and stress-related disorders are among the most common mental disorders, with a regional variation in prevalence globally (i.e., from 5.3% to 10.4%) 1 and a pooled lifetime prevalence of ~12.9% 2 . With shared clinical symptoms and neurobiological features, anxiety and stress-related disorders are considered highly correlated―they are historically in the same diagnosis category 3 , with similarities in genetic architecture demonstrated in familial coaggregation 4 , 5 and genome-wide association analysis (GWAS) studies 6 .
Individuals with anxiety and stress-related disorders usually follow an intermittent recurring symptom episode throughout life and a as result, experience impaired mental and physical functioning, increased rates of disability, and higher-than-expected mortality 7 , 8 . The causes of the excess disability and mortality include a considerable range of medical conditions, such as other psychiatric disorders (e.g., depression) 9 , metabolic diseases 10 , 11 , cardiovascular disease 12 , 13 , 14 , autoimmune disease 15 , 16 , and infections 17 . However, previous studies have mostly focused on specific groups of diseases and, to the best of our knowledge, no comprehensive assessment of disease clusters arising subsequent to anxiety and stress-related disorders has been conducted.
Although still early on, the scant literature suggests a role of stress-related genetic loci in the development of cardiovascular disease 18 and mortality 19 after exposure to terrorist attacks or social adversity. Likewise, a recent GWAS study revealed that both anxiety and stress-related disorders are genetically correlated with multiple obesity-related phenotypes 6 , promoting studies on the genetic basis of adverse health consequences after anxiety and stress-related disorders. However, as a wide range of medical conditions have been associated with a prior diagnosis of anxiety and stress-related disorders, exploration of patients’ genetic susceptibilities to a single disease has limited clinical implications. The recent advances in human disease network methodology, e.g., disease trajectory 20 and comorbidity network 21 , provide new means to comprehensively summarize the possible sets of diseases (i.e., disease clusters with close temporal or non-temporal relationships) following a predetermined phenotype 22 , 23 . Furthermore, with the notion that disease located in the same cluster should have shared or linked biological mechanisms, the identification of cluster-specific, instead of diseases-specific, genetic variants, has the potential to realize the prevention of a general further health decline among patients with anxiety or stress-related disorders.
Therefore, taking advantage of the nationwide population and health registers in Sweden as well as the community-based health records and genetic information available in the UK Biobank, we aimed to identify major clusters of subsequent medical conditions after a diagnosis of anxiety and stress-related disorders. We further aimed to elucidate the underlying genetic determinants associated with those identified disease clusters.
Baseline characteristics
Based on the specialized diagnoses from the nationwide Swedish Patient Register, we first included a Swedish cohort comprising 70,026 patients at first diagnosis of anxiety or stress-related disorders from 2001 to 2016 and ten randomly selected birth year- and sex-matched unaffected individuals per patient using incidence density sampling ( N = 700,260), without history of other psychiatric disorders and severe somatic diseases, as the exploratory dataset (Fig. 1 , Supplementary Fig. 1 , Supplementary Data 1 ). To validate the identified disease clusters in the Swedish cohort, we constructed a UK cohort (i.e., the validation dataset) based on the UK Biobank, including 23,365 patients diagnosed with anxiety or stress-related disorders between 1997 and 2019 from inpatient and primary care, and 233,596 unaffected participants individually matched by sex and year of birth (Supplementary Fig. 2 ). The Swedish and the UK cohorts were both overrepresented by females (62.7% and 67.3% respectively), with a similar median age at diagnosis for anxiety and stress-related disorders (Table 1 ). The median follow-up time was 7.1 and 13.3 years in the Swedish cohort and UK cohort, respectively. Patients in both cohorts had lower educational and income levels than their matched unaffected individuals.
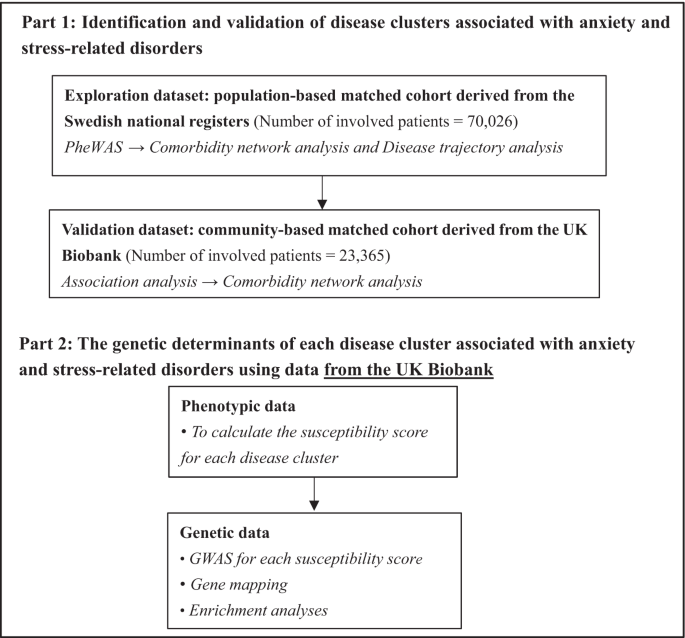
PheWAS phenome-wide association study, GWAS Genome-wide association study.
Identification of associated disease clusters
In the Swedish cohort, 183 medical conditions (among 454 tested) had a prevalence of ≥0.5% and 136 were positively associated with a prior diagnosis of anxiety or stress-related disorders (Supplementary Data 2 ). The top HRs were noted for other psychiatric disorders, including personality and behavior disorder (HR [95% confidence intervals]: 16.7 [15.0–18.5]), sedatives or hypnotics abuse (16.0 [14.1–18.2]), and other mood disorder (13.4 [12.1–14.9]). Somatic medical conditions with the highest HRs were other headache syndromes (2.8 [2.6–3.0]), irritable bowel syndrome (2.6 [2.3–2.8]), and other functional intestinal disorders (2.3 [2.2–2.4]). In addition, one negative association was noted between anxiety/stress-related disorders and varicose veins of lower extremities (0.93 [0.89–0.98]).
Subsequently, we identified 433 and 97 disease pairs to construct a comorbidity network and disease trajectory after anxiety or stress-related disorders, respectively (details of the disease pair identification in Supplementary Fig. 3 and results in Supplementary Data 3 - 4 ). The analysis of the comorbidity network identified seven modules, characterized by their predominant components related to psychiatric disorders, eye diseases, ear diseases, cardiovascular disease, genitourinary diseases, musculoskeletal diseases, and cerebrovascular diseases (Fig. 2A ). Figure 2B shows an overview of the disease trajectories after anxiety or stress-related disorders. The medical conditions that were listed immediately after anxiety or stress-related disorders in chronological order (i.e., D1) included disorders of sense organs, genitourinary diseases, cardiovascular disease, and psychiatric disorders.
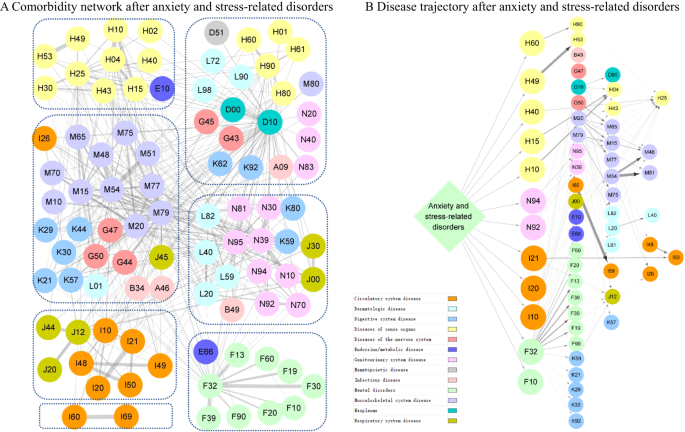
Each node represents a medical condition, and the combined ICD-10 codes are shown within the circle, while the color of the node indicates the category of the corresponding medical condition. The color of the link represents the strength of comorbidity association, measured by odd ratio. Definition of combined ICD-10 codes can be found in Supplementary Data 1 . A Comorbidity network after anxiety and stress-related disorders. The network was partitioned into seven main modules using Louvain algorithm, and nodes belonging to the same group were gathered together using blue dashes. B Disease trajectory after anxiety and stress-related disorders. The first affected diseases (D1) by anxiety and stress-related disorders included disorders of sense organs, genitourinary diseases, cardiovascular diseases, and psychiatric disorders.
We determined stable disease clusters by merging the findings from the comorbidity network and disease trajectory analyses together (Fig. 3 ), which consequently led to five clusters including 31 medical conditions (Fig. 4 A, B). Cluster 1 denoted a link from anxiety or stress-related disorders to depression and alcohol abuse, and further to obesity and several other psychiatric disorders. Cluster 2 and Cluster 3 denoted a link to eye and ear diseases. Cluster 4 included mainly cardiovascular disease, with a direct link to hypertensive disorders, ischemic heart diseases, and angina pectoris. Cluster 5 was predominated by genitourinary diseases as the first affected medical conditions, and further linked to skin diseases.
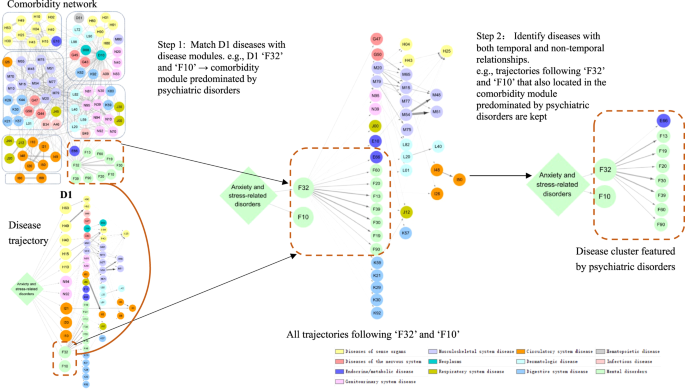
Each node represents a medical condition, and the combined ICD-10 codes are shown within the circle, while the color of the node indicates the category of the corresponding medical condition. Definition of combined ICD-10 codes can be found in Supplementary Data 1 . D1 disease 1.
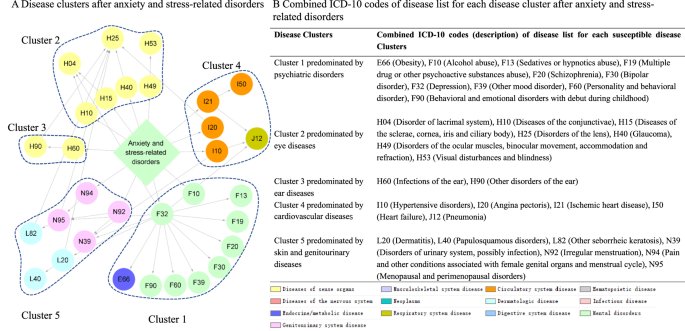
A Disease clusters after anxiety and stress-related disorders. Each node represents a medical condition, and the combined ICD-10 codes are shown within the circle, while the color of the node indicates the category of the corresponding medical condition. One disease cluster was defined as D1 and its subsequent diseases from the disease trajectory in Fig. 2A , which belonged the same module in the comorbidity network in Fig. 2B . The network contains five disease clusters, and nodes belonging to the same disease cluster were grouped together using blue dashes. B Combined ICD-10 codes of disease list for each disease cluster after anxiety and stress-related disorders. This table lists combined ICD-10 codes for each disease cluster. Definition of combined ICD-10 codes can be found in Supplementary Data 1 .
Among the 31 medical conditions identified from the Swedish cohort, 19 medical conditions were validated in the UK cohort (i.e., statistically significantly associated with a prior diagnosis of anxiety or stress-related disorders, Supplementary Data 5 ). When validating the disease clusters, we identified 170 possible disease pairs and selected 55 disease pairs to be included in the comorbidity network analysis (Supplementary Data 6 ). All five disease clusters were replicated in the UK cohort, although the cluster predominated by psychiatric disorders and ear diseases from the Swedish cohort was found to be merged as one cluster in the UK cohort (Supplementary Table 1 ).
Genetic determinants of associated disease clusters
To identify the potential genetic determinants for each disease cluster among patients with anxiety or stress-related disorders, we first calculated five cluster-specific quantitative scores as an index of individual’s susceptibility to each disease cluster, and then performed GWAS analyses for the five susceptibility scores separately, among individuals from the UK cohort with eligible genotyping data ( n = 27,781, Supplementary Fig. 2 ), using mixed linear model (MLM)-based models. GWAS analyses identified three, 33, 40, 4, and 16 independent single nucleotide polymorphisms (SNPs) for clusters featured by psychiatric disorders, eye diseases, ear diseases, cardiovascular diseases, and skin and genitourinary diseases, respectively (Table 2 ). The full lists of the SNPs, their mapped genes, and enriched biological pathways are presented in Supplementary Data 7 – 9 . According to the genomic inflation analysis results of Linkage Disequilibrium (LD) score regression, we found little indication for confounding effects in the GWAS of the five disease clusters (Supplementary Table 2 ). We found 20 (e.g., AP000304.12, ATP5O, MRPS6 ), 362 (e.g., ZFAND1, CHMP4C, SNX16 ), 440 (e.g., C10orf88, PSTK, TEX36 ), 30 (e.g., EFNA5, VAV3, SLC25A24 ) and 229 (e.g., BCHE, ZBBX, OR6S1 ) mapped genes for the five associated disease clusters, which were then associated with several enrichment biologic pathways topped by GO:0098660 inorganic ion transmembrane transport, WP5224 2q37 copy number variation syndrome, GO:0048545 response to steroid hormone, M5884 NABA CORE MATRISOME, and GO:0097484 dendrite extension, respectively. Based on information from FUMA and GeneCards, we found that several cluster-specific genes have been previously associated with individual psychiatric or somatic traits in the disease cluster (e.g., PRPF38B for angina pectoris and myocardial infarction, Supplementary Data 10 ).
When comparing genes and pathways crossing different disease clusters, we found several common genes (e.g., AGAP1, AOAH, C8orf59 for both clusters featured by eye and ear diseases, Fig. 5A ), although no common pathways. Further protein–protein interaction (PPI) analysis identified ten MCODE components (e.g., MCODE components featured by pathways of ‘Transmembrane signal transduction’ and ‘Signaling by G protein-coupled receptor (GPCR)’, Fig. 5B ) shared by disease clusters of eye, ear and skin and genitourinary diseases.
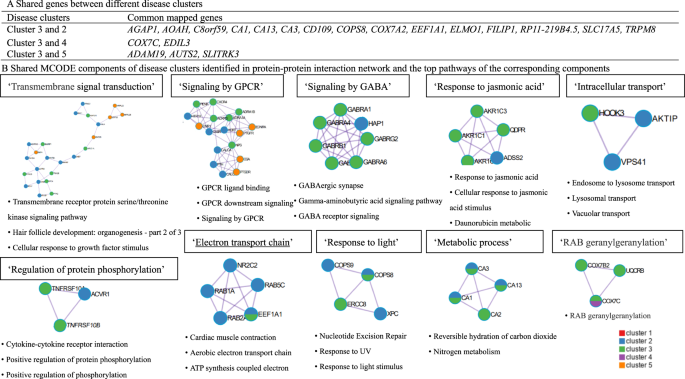
A Common gene between different disease clusters. B Shared MCODE components of disease clusters identified in protein–protein interaction network and the top pathways of the corresponding components, where each node represents a protein with a pie chart encoding its origin. SNPs: single nucleotide polymorphisms. Cluster 1 predominated by psychiatric disorders, Cluster 2 predominated by eye diseases, Cluster 3 predominated by ear diseases, Cluster 4 predominated by cardiovascular diseases, Cluster 5 predominated by skin and genitourinary diseases. GWAS genome-wide association study, GPCR G Protein Coupled Receptors, MCODE Molecular Complex Detection algorithm.
In sensitivity analyses using the disease clusters and their component medical conditions that can be validated in the UK cohort alone (Table 2 and Supplementary Data 11 ), we found some identical genetic determinants. Specifically, for the cluster predominated by eye diseases, 11 independent SNPs (e.g., rs578045293, rs574810100, rs192296901) and 115 mapped genes (e.g., DMRTA2, FAF1, CDKN2C ) were identified in both genetic analyses. For the cluster predominated by ear diseases, five independent SNPs (e.g., rs113248357, rs193072412, rs556283981), 183 mapped genes (e.g., RHCE, TMEM57, LDLRAP1 ), and two biological pathways (i.e., R-HSA-3700989 Transcriptional Regulation by TP53, R-HSA-1475029 Reversible hydration of carbon dioxide) were identified in both.
Furthermore, comparing the mapped genes for each disease cluster among individuals with anxiety/stress-related disorders ( n = 27,781) to those obtained among individuals without anxiety/stress-related disorders ( n = 452,148, Supplementary Data 12 ), we found only a small proportion of overlapping genes (i.e., 5 and 2 genes for clusters predominated by ear diseases and cardiovascular disease, respectively), indicating that few identified genetic hits were driven by the disease clusters only.
Subgroup analyses
We found largely similar disease clusters when using the entire Swedish cohort, although the clusters dominated by eye diseases, ear diseases, and cardiovascular disease were merged into one cluster (Supplementary Fig. 4 and Supplementary Table 3 ). When exploring the disease clusters following anxiety and stress-related disorders separately, we found an additional disease cluster predominated by cerebrovascular diseases for both disorders and another cluster predominated by musculoskeletal diseases for stress-related disorders (Supplementary Figs. 5 , 6 ). We obtained similar disease clusters among females as in the main analysis, although two clusters predominated by cerebrovascular diseases and digestive diseases were also identified (Supplementary Fig. 7 ). The cluster predominated by ear diseases was not identified but a disease cluster predominated by musculoskeletal diseases was noted among males (Supplementary Fig. 8 ).
Leveraging the nationwide health registers in Sweden and the large community-based UK Biobank, our study, revealed the most comprehensive picture of subsequent disease clusters following a diagnosis of anxiety and stress-related disorders. The five distinct disease clusters, featured by psychiatric disorders, eye diseases, ear diseases, cardiovascular diseases, and skin and genitourinary diseases, were discovered in the Swedish cohort and validated in the UK cohort. Furthermore, based on individual-level genotyping data in the UK cohort, we identified several distinct genetic determinants for the five disease clusters, as well as genetic components involved in the GPCR signaling pathway that were shared between multiple disease clusters. With novel attempts to conceptualize associated disease clusters, these findings shed light on the biological basis, both commonly and specifically, towards further diverse health consequences after a diagnosis of anxiety and stress-related disorders, which could aid mechanistic explorations, and facilitate risk surveillance (e.g., precise risk assessment) and management (e.g., development of targeted interventions) for health decline prevention among patients with newly diagnosed anxiety and stress-related disorders.
Over the past decade, accumulated evidence suggests a positive link between anxiety or stress-related disorders and a number of medical conditions. Previous studies have, however often relied on small samples 24 , with incomplete follow-up 25 , and focused on a single outcome/disease 11 , 26 . Our study, therefore complements the knowledge gaps through a data-driven approach of including virtually all medical conditions after the diagnosis of anxiety and stress-related disorders. As a result, we managed to identify five key clusters of disease associated with a prior diagnosis of anxiety or stress-related disorders (with component diseases in the same system or across different systems), considering the temporal order and high intrinsic connectivity between diseases and with validation across two populations. Although evidence on the risk of these disease clusters after anxiety and stress-related disorders was scarce, our findings gain support from previous studies reporting associations between anxiety or stress-related disorders and individual diseases. For instance, anxiety and stress-related disorders have previously been reported to co-occur with other psychiatric disorders (e.g., major depressive episode, bipolar disorder, and alcohol dependence) 27 . Additionally, a population-based cohort study covering 5.9 million people in Denmark reported increased risks of 31 somatic medical conditions, including cardiovascular disease, vision problems, and hearing problems, following a prior diagnosis of neurotic disorders (including anxiety and stress-related disorders) 28 . In our previous population-based cohort study in Sweden, we also noted risk increases of 16 specific cardiovascular diseases among patients with stress-related disorders 12 . In addition, several diseases in disease clusters predominated by skin and genitourinary diseases have been reported to be associated with anxiety or stress-related disorders, such as urinary infection 29 , irregular menstruation 30 , and premenstrual syndrome 31 . We found largely similar disease clusters following a diagnosis of anxiety and stress-related disorders, with an additional cluster of musculoskeletal diseases noted among patients with stress-related disorders. Several studies have reported similar associations between post-traumatic stress disorder (PTSD) and arthritis, although using self-reported data 32 , 33 . Furthermore, we found little role of age and sex in the identification of most disease clusters, indicating that the risk of developing these disease clusters is independent of age and sex.
Prior attempts to illustrate disease networks include a recent Danish study based on national data from inpatient and outpatient care, which established a browser presenting the disease trajectories both before and after a target disease of interest 34 . Using this browser, we found that some key diseases in our study, such as cardiovascular diseases identified subsequent to stress-related disorders, were listed prior to stress-related disorders. However, as the comparability of these two studies is limited (due to the different research purposes and study designs), the inconsistent results do not necessarily invalidate each other.
With the notion that diseases with high levels of connectivity (i.e., in a disease cluster) may share common pathological mechanisms with possibly the same affected genes and biological pathways, we consider it is reasonable to focus on disease clusters, instead of each individual disease, for the purpose of genetic determinant identification and for the future development of disease prevention strategies. Despite the lack of comparable results from studies of similar design, the findings of our cluster-specific genetic analyses were in line with prior studies. For instance, some mapped genes for susceptibility to the disease cluster featured by psychiatric disorders (e.g., AMOTL1, CWC15, KDM4D ) were reported to be associated with mood disorders 35 as well as attention deficit hyperactivity disorder (ADHD) and conduct disorder 36 . IQCB1 and GOLGB1 , the genes identified for the disease cluster dominated by eye diseases, have been associated with corneal resistance factor (a measure of the biomechanical properties of the cornea) 37 , while CHDH and FILIP1L have been associated with ocular axial length 38 . Additionally, some mapped genes for the disease cluster dominated by cardiovascular disease have been demonstrated as risk genes for diastolic blood pressure ( COL23A1 and PHYKPL ) 39 or stroke ( COL23A1 , PHYKPL , and ADAMTS2 ) 40 , 41 . Nevertheless, we found several risk genes (e.g., ATP5O, POLQ, RHCE ) and biological pathways not discussed in the existing literature, mainly involved in the regulation of molecular transport, cellular metabolic processes, and the structure and function of proteins. Particularly, it is also notable that, except for genes and pathways that were linked to a specific disease cluster, we identified the biological components related to the signaling of GPCR that may contribute to the development of multiple disease clusters (i.e., clusters featured by eye, ear, and skin and genitourinary diseases). GPCR signaling has been widely reported to play a role in responses to stress 42 , inflammatory response 43 , and development and drug targets for multiple diseases 44 (e.g., dry eye disease 45 , allergic conjunctivitis 46 , and urinary tract infection 47 ), which may indicate the key shared pathways and mechanisms linking anxiety and stress-related disorders to subsequent sequelae. Collectively, if verified, the findings of our study might provide additional insights into why patients diagnosed with anxiety and stress-related disorders face a general health decline, with large variations in developed disease outcomes. Regardless, our measure of susceptibility score to a disease cluster rather than to a single disease, might limit the comparison of findings between the present study and previous studies.
Our efforts to identify of disease clusters and their genetic determinants were conceptual and were largely based on accumulating evidence of the existence of disease networks and their shared biological mechanisms 20 , 21 , 48 , with the potential to aid in the development of cost-effective health promotion strategies for this vulnerable population. For instance, medications indicated for the genes/pathways within each disease cluster could be further tested for effectiveness in reducing risks of further disease development among individuals with anxiety and stress-related disorders. Other major strengths of our study include the inclusion of two large population- and community-based cohorts with long and complete follow-up data collected prospectively and independently, which largely minimized information and selection biases, and enabled the ascertainment of disease clusters using data from two distinct populations. Furthermore, the combination of disease trajectory and comorbidity network analyses to ascertain disease clusters enhanced the reliability of the connectivity and temporal order between disease pairs in each disease cluster. Last, the availability of enriched phenotypic and genotypic data, together with the application of comprehensive analytic strategies, including PheWAS, disease network analysis, and genetic analysis, for the first time, led to a comprehensive illustration of health consequences in relation to anxiety and stress-related disorders from phenotypic to genetic levels. This analytic strategy could be applied as a pipeline for studying comorbidities of other phenotypes.
Several limitations should be acknowledged. First, given the lack of complete primary care data in the Swedish Patient Register and the UK Biobank, as well as the lack of outpatient care data in the UK Biobank, we might have underestimated the number of patients with anxiety and stress-related disorders as well as the number of studied medical conditions, primarily the milder forms of these diseases. Therefore, disease cluster identification based on a more comprehensive data source to validate the findings of the present study is warranted. Second, although we excluded patients with a history of other psychiatric disorders and severe somatic diseases and started the follow-up from six months after the index date, we cannot rule out the possibility that some pre-existing diseases other than anxiety and stress-related disorders might have contributed to the identified disease clusters. Third, the identification of disease clusters relied on the results of association analyses (i.e., PheWAS analysis). Although confirmed by using two methods and validated in the UK cohort, the lack of data on important confounders in the health register data (e.g., lifestyle and environmental factors) can raise the concern of residual confounding. This also applies to the noted negative association of anxiety/stress-related disorders with varicose veins of lower extremities. With few supportive data from existing literature, such a finding needs to be validated in future studies. Last, our findings may not be generalized to other populations with non-European ancestry or different healthcare systems than in Sweden and the UK.
In conclusion, based on detailed phenotypic and genetic analyses of two large-scale cohorts, we identified five distinct disease clusters subsequent to an inpatient/outpatient diagnosis of anxiety and stress-related disorders, featured by other psychiatric disorders, eye diseases, ear diseases, cardiovascular diseases, and skin and genitourinary diseases as predominant diseases in each cluster. We further identified a list of genetic variants and biological pathways linking anxiety and stress-related disorders, specifically or commonly, to those identified disease clusters, contributing to a better understanding of the underlying mechanisms.
Study design
The analytic process included two parts, namely phenotypic and genetic analyses (Fig. 1 ). In the phenotypic analysis, we undertook a phenome-wide association study (PheWAS), followed by both comorbidity network analysis and disease trajectory analysis to determine robust disease clusters (i.e., associated diseases with both temporal and non-temporal relationships) following a diagnosis of anxiety or stress-related disorders in the Swedish cohort (i.e., the exploratory dataset). To validate identified disease clusters and the diseases they entailed, we performed similar analyses in the UK cohort of similar study designs (i.e., the validation dataset). Regarding the genetic analyses based on individual-level genotyping data of the UK cohort, we first calculated a cluster-specific susceptibility score, which was designated as a quantitative index of individuals’ susceptibility to a specific disease cluster, and then performed GWAS analysis, gene mapping, and enrichment analysis to identify risk genes and biological pathways that may count for the pathogenesis of such a disease cluster after anxiety or stress-related disorders.
Swedish cohort
The Swedish Patient Register includes nearly complete health records of inpatient care since 1987 and outpatient specialist care since 2001 in Sweden 49 . By cross-linkage to the Total Population Register using the unique Swedish personal identification numbers, we included all Swedish-born individuals residing from 2001 to 2016 in Sweden and excluded those with any pre-existing psychiatric disorders or history of severe somatic diseases at the time of diagnosis determined by the Charlson Comorbidity Index before 2001 50 , leading to a study population of 8,456,485 (Supplementary Fig. 1 ). We focused on Swedish-born individuals in the present study to reduce the heterogeneity in genetic background as well as other sociodemographic factors, including differential health-seeking behaviors. Among these, we identified all individuals who received a first primary diagnosis in specialized care of anxiety or stress-related disorders from 2001 to 2016 ( N = 212,767, 63.3% with anxiety disorder), and a set of ten unaffected individuals randomly selected from the study base per exposed patient, individually matched on sex and birth year using incidence density sampling ( N = 2,127,670), without history of other psychiatric disorders and severe somatic diseases. The diagnostic date of anxiety or stress-related disorders was used as the index date for the start of follow-up of both the exposed patients and their matched unaffected individuals.
To minimize the concern of reverse causality, we followed all participants of the Swedish cohort for all medical conditions from 6 months after the index date until death, first diagnosis of anxiety or stress-related disorders (for matched unexposed individuals), emigration, or the end of the study period (i.e., 31 December 2016), whichever occurred first.
Ascertainment of anxiety and stress-related disorders and subsequent medical conditions
In the Swedish cohort, we defined anxiety or stress-related disorders as any first specialist care diagnosis in an inpatient or outpatient hospital visit, where these disorders were identified as the primary discharge diagnosis, according to the Swedish Patient Register, using the 10th Swedish revision of the International Classification of Diseases (ICD-10) codes (anxiety: F40 and F41, stress-related disorder: F43) (Supplementary Table 1 ). In the PheWAS, medical conditions refer to any disease or health outcomes recorded in the Patient Register comprising inpatient and outpatient diagnoses. We ascertained medical conditions through the primary diagnosis from the Patient Register, using the 3-digit ICD-10 codes (A00 to N99) (Supplementary Table 1 ). The diagnostic codes for most common diseases in the Patient Register have been validated, showing a satisfactory accuracy with positive predicted values [PPV] of 85–95% for most common diseases 49 , 81% for social anxiety disorder 51 , and 75–90% for PTSD 52 . We obtained the highest level of education and income at the year of index date from the Swedish Longitudinal Integration Database for Health Insurance and Labor Market 53 .
The validation dataset (UK cohort) was constructed based on the UK Biobank, using a similar design (Supplementary Fig. 2 ). The UK Biobank (UKB) is a community-based cohort study that enrolled half a million participants aged 40–69 at recruitment between 2006 and 2010 across England, Scotland, and Wales. Details of the study design are described elsewhere 54 . The inpatient hospital data, obtained from the Hospital Episode Statistics database, the Scottish Morbidity Record, and the Patient Episode Database, cover all UK Biobank participants since 1997 55 . The primary care data, provided by various general practitioner computer system suppliers, cover ~45% of participants since 1985 55 . We first excluded individuals who had withdrawn from the UK Biobank ( n = 108) or had conflicting information ( n = 1), leaving 502,398 eligible participants (Supplementary Fig. 2 ). Among these, we constructed a matched cohort, including patients with newly inpatient/primary care diagnosed anxiety or stress-related disorders between January 1, 1997 and December 31, 2019 ( N = 23,365) who had no history of severe somatic diseases or other psychiatric disorders, and up to ten unaffected individuals for each patient who were randomly selected and individually matched by sex and year of birth using incidence density sampling ( N = 233,596). The diagnostic date of anxiety or stress-related disorders was used as the index date for the start of follow up of the exposed and matched unaffected individuals.
We followed all participants of the UK cohort for all medical conditions from 6 months after the index date until death, first diagnosis of anxiety or stress-related disorders (for matched unexposed individuals), loss to follow-up 56 , or the end of the study period (i.e., 31 December 2019), whichever occurred first.
In the UK cohort, we defined a new diagnosis of anxiety or stress-related disorder as a first primary diagnosis based on the inpatient and primary care data, using the ICD-10 codes for the inpatient hospital data (Supplementary Data 1 ) and the version 2 and version 3 read codes (i.e., Read v2 and Read v3) for the primary care data (Supplementary Table 4 ). Medical conditions were ascertained from the primary and secondary diagnoses through the inpatient hospital data (Supplementary Data 1 ). Information on the highest educational level and Townsend Deprivation Index (proxy for socioeconomic status, with a higher index score indicating a higher degree of deprivation) 57 were collected at recruitment through questionnaires.
Statistical analyses
The age distribution differed between the Swedish cohort and the UK cohort (median age at diagnosis 32 versus 52). To facilitate validation between the two cohorts, we selected a sub-cohort of the Swedish cohort, namely participants with age >second tertile (median age at index date = 51, N = 70,026, Table 1 ), and used this sub-cohort as the exploration dataset throughout the main analyses. A sensitivity analysis was performed using the entire Swedish cohort with all age groups.
Exploration of associated disease clusters in the Swedish cohort
In the exploration dataset (Swedish cohort), we identified a total of 454 medical conditions diagnosed six months after the diagnosis of anxiety or stress-related disorders. To ensure statistical power, we included only medical conditions with a prevalence ≥0.5% among patients with anxiety or stress-related disorders. We performed a PheWAS to investigate the associations between anxiety or stress-related disorders and each medical condition, using Cox regression models stratified by matching variables (i.e., sex and birth year) with adjustment for highest education and income. Individuals with a prior diagnosis of the studied medical condition were excluded, when estimating the association with each medical condition. Only medical conditions with statistically significant positive associations, after adjusting for multiple testing (hazard ratio [HR] > 1, and false discovery rate [FDR] adjusted p value [i.e., q value] <0.05), were included in the following analyses.
Among the identified medical conditions from the PheWAS, we constructed all possible disease pairs as disease 1 (D1) and disease 2 (D2) pairs and only analyzed disease pairs that co-occurred with a prevalence ≥0.25% among patients with anxiety or stress-related disorders. To ensure comorbidity strength, we calculated the relative risk (RR) and Pearson’s correlation ( Φ -correlation) for each disease pair. For each disease pair, a sub-cohort was formed through excluding patients with a history of D1 and D2 before their index date (i.e., the diagnosis date of anxiety or stress-related disorders). The formulas for RR and Φ -correlation were calculated using the following formulas:
Where \({C}_{{ij}}\) is the number of patients affected by both D1 and D2, and \({N}_{{ij}}\) is the number of individuals in the sub-cohort, while \({C}_{i}\) and \({C}_{j}\) are the number of patients affected by D1 and D2 respectively. For both RR and Φ -correlation measures, the significance of RR = 0 and Φ = 0 can be both determined using z-test (given large sample size in our study). The corresponding z-score for RR and Φ -correlation were calculated using the following formula 21 , 58 :
P values were then calculated using the z-score and adjusted for the issue of multiple testing. Only disease pairs with strong comorbidity strength (i.e., RR > 1, Φ -correlation > 0, and q value < 0.05) were included in the comorbidity network and disease trajectory analyses.
In the comorbidity network analysis, we used logistic regression to determine the magnitude of association between the disease pairs with strong comorbidity strength (i.e., significant non-temporal relationship). Disease pairs with confirmed positive association (i.e., odds ratio [OR] > 1 and q value < 0.05) were selected to construct a comorbidity network. The comorbidity network was then subdivided into different comorbidity modules with high intrinsic connectivity determined by the Louvain clustering algorithm 59 . For disease trajectory analysis, binomial tests were used to assess the temporal direction (i.e., D1 → D2 or D2 → D1 among D1D2 pairs) among disease pairs with strong comorbidity strength (i.e., significant temporal relationship). For each disease pair with a determined temporal order, we constructed a nested case-control dataset in the sub-cohort, by considering D2 as outcome and D1 as exposure. For each patient with D2, at most two controls were matched by sex and birth year using intensity density sampling. to confirm the magnitude of the association between the disease pair, we then used conditional logistic regression by adjusting for education level and Townsend Deprivation Index. We then included the disease pairs with positive associations (OR > 1 and q value < 0.05) to construct the disease trajectory.
As disease trajectory analysis is designed to visualize sequential disease progression while comorbidity network analysis captures disease groups with high intrinsic connectivity, the combined use of those two data-driven approaches can theoretically lead to the identification of more reliable disease clusters (i.e., groups of diseases with both temporal and non-temporal relationships). Thus, based on results from the aforementioned disease trajectory and comorbidity network analyses, we defined disease clusters as the first layer diseases (D1) and their subsequent diseases in a disease trajectory that were also located within the same comorbidity module (Fig. 3 ). For example, in the disease trajectories, out of all diseases in the first layer, “F32” and “F10” were located in one comorbidity module (i.e., the module predominated by psychiatric disorders) derived from the comorbidity network. We then found the following diseases of “F32” and “F10” in the trajectories which were also in such a comorbidity module to constitute a disease cluster (i.e., the disease cluster featured by psychiatric disorders including “E66”, “F10”, “F13”, “F19”, “F20”, “F30”, “F32”, “F39”, “F60”, and “F90”).
Validation of associated disease clusters in the UK cohort
To validate the identified disease clusters in the UK cohort, we used Cox models to assess the associations between anxiety and stress-related disorders and each medical condition of the identified disease cluster, comparing affected patients to their matched unaffected individuals. The models were stratified by matching variables (i.e., sex and birth year) and adjusted for highest educational level and Townsend Deprivation Index. Only medical conditions with statistically significant positive associations were included in the comorbidity network analysis (same as described above) to construct disease clusters in the UK cohort. Trajectory analysis was not performed in the UK cohort due to the lack of complete primary care and outpatient data.
Genetic determinants of associated disease clusters using data from the UK Biobank
In the UK cohort, a cluster-specific “susceptibility score” was calculated to quantify the subsequent risk of each disease cluster for each patient with anxiety or stress-related disorder. The susceptibility score was defined as an individual person’s number of diagnosed diseases included in each disease cluster, according to the inpatient hospital data.
The quality control contains two parts. For quality control on individuals from the UK Biobank, we removed individuals with non-European ancestry, inconsistent sex, or sex chromosome aneuploidy. For quality control on individual level of genetic data, we first removed SNPs with imputation quality score <0.8, minor allele frequency < 0.001, or deviations from Hardy-Weinberg equilibrium ( p < 1 × 10 −10 ) 60 . We removed SNPs in the extended major histocompatibility complex (MHC) region (chr6: 25–34 Mb), considering the long-range linkage disequilibrium (LD) and special genetic architecture in this region. After standard GWAS quality control on the individual-level genotyping data 60 , we included 22,781 patients (out of the 23,365 patients) and 13,225,429 variants for further analysis.
To assess the association between SNPs and five susceptibility scores (as a continuous variable) respectively, we used mixed linear model (MLM)-based models for GWAS analysis, adjusted for sex, birth year, genotyping array, and the first ten principal components 61 . Independent significant SNPs with p < 5 × 10 −8 were identified for each genomic locus. The inflation of GWAS analyses was tested by LD score regression 62 . Together with surrounding genomic loci that were identified based on LD structure at r 2 ≥ 0.6, all the SNPs were further mapped to identify potential genes using the web-based platform FUMA ( http://fuma.ctglab.nl/ ) 63 . The strategies for gene mapping included positional mapping, expression quantitative trait loci mapping based on the GTEx v8 Project 64 , and Chromatin interaction mapping 65 , 66 . Further, these mapped genes were included in the gene-set enrichment analyses based on Metascape ( https://metascape.org/ ) to identify underlying biological pathways for each disease cluster with the following ontology sources: KEGG Pathway, GO Biological Processes, Reactome Gene Sets, Canonical Pathways, CORUM, WikiPathways, and PANTHER Pathway 67 . To further investigate genetic overlap across disease clusters, the abovementioned mapping genes were included in the PPI network enrichment analysis to identify protein network components using the Molecular Complex Detection (MCODE) algorithm based on Metascape 68 based on the following genomic interaction databases: STRING 69 , BioGrid 70 , OmniPath 71 , and InWeb_IM 72 . To test whether these identified cluster-specific genes are associated with any of the individual diseases in each disease cluster, we first conducted hypergeometric tests using the function of “GENE2FUNC” in the website platform ‘FUMA’. As an alternative approach serving a similar purpose, we searched GeneCards, a gene database providing all annotated and predicted human genes ( https://www.genecards.org/ ), to identify traits that have been previously associated with the top 20 identified genes of each disease cluster.
In a sensitivity analysis, we repeated the genetic analysis merely for the disease clusters (and their components) that can be validated from the UK cohort. Additionally, to test whether the identified genes were primarily driven by the studied disease clusters, independent of the prior anxiety/stress-related disorders, we conducted additional GWAS analyses for those disease clusters among individuals without anxiety/stress-related disorders ( n = 452,148).
As we used the advanced age group (i.e., >second tertile) of the Swedish cohort in the main analysis, we repeated the phenotypic analyses in the entire Swedish cohort ( N = 216,727). To assess whether the results would differ between anxiety and stress-related disorders, we constructed two independent matched cohorts for anxiety and stress-related disorders, separately, and repeated the main analyses to identify disease clusters associated with anxiety disorder and stress-related disorder exclusively. To explore the role of sex in disease clusters, we performed analyses separately for males and females.
The phenotypic analyses were conducted using SAS 9.4 (SAS Institute), R (Version 4.0.2), Python (Version 3.8), and Cytoscape (Version 3.8.0). PLINK (Version 1.9) and GCTA (Version 1.24) were used for genetic analyses. For multiple testing, a q value < 0.05 was considered statistically significant. This study was approved by the Ethical Vetting Board in Stockholm, Sweden (DNRs 2012/1814-31/4 and 2015/1062-32), the NHS National Research Ethics Service (16/NW/0274), and the Biomedical Research Ethics Committee of West China Hospital (2019-1171). The requirement of informed consent for Swedish participants is waived in register-based studies in Sweden, and all the participants in the UK Biobank provided written informed consent before data collection.
Reporting summary
Further information on research design is available in the Nature Portfolio Reporting Summary linked to this article.
Data availability
The raw data from Swedish registers are protected and not available due to Swedish law. The raw data from the UK Biobank ( http://www.ukbiobank.ac.uk/ ) are available to all researchers upon making an application. Part of this research was conducted using the UK Biobank Resource under Application 54803. Other data or platforms are available to all researchers: FUMA ( http://fuma.ctglab.nl/ ), Metascape ( https://metascape.org/ ), GeneCards ( https://www.genecards.org/ ).
Code availability
As the codes are highly specific to our curated database and may not be universally applicable to others, all codes associated with the current submission are available and can be requested by contacting the corresponding authors.
Baxter, A. J., Scott, K. M., Vos, T. & Whiteford, H. A. Global prevalence of anxiety disorders: a systematic review and meta-regression. Psychol. Med. 43 , 897–910 (2013).
Article CAS PubMed Google Scholar
Steel, Z. et al. The global prevalence of common mental disorders: a systematic review and meta-analysis 1980-2013. Int. J. Epidemiol. 43 , 476–493 (2014).
Article PubMed PubMed Central Google Scholar
Williamson, J. B., Jaffee, M. S. & Jorge, R. E. Posttraumatic stress disorder and anxiety-related conditions. Continuum 27 , 1738–1763 (2021).
PubMed Google Scholar
Telman, L. G. E., van Steensel, F. J. A., Maric, M. & Bogels, S. M. What are the odds of anxiety disorders running in families? A family study of anxiety disorders in mothers, fathers, and siblings of children with anxiety disorders. Eur. Child Adolesc. Psychiatry 27 , 615–624 (2018).
Article PubMed Google Scholar
Hettema, J. M., Neale, M. C. & Kendler, K. S. A review and meta-analysis of the genetic epidemiology of anxiety disorders. Am. J. Psychiatry 158 , 1568–1578 (2001).
Meier, S. M. et al. Genetic variants associated with anxiety and stress-related disorders: a genome-wide association study and mouse-model study. JAMA Psychiatry 76 , 924–932 (2019).
Baxter, A. J., Vos, T., Scott, K. M., Ferrari, A. J. & Whiteford, H. A. The global burden of anxiety disorders in 2010. Psychol. Med. 44 , 2363–2374 (2014).
Olatunji, B. O., Cisler, J. M. & Tolin, D. F. Quality of life in the anxiety disorders: a meta-analytic review. Clin. Psychol. Rev. 27 , 572–581 (2007).
Choi, K. W., Kim, Y. K. & Jeon, H. J. Comorbid anxiety and depression: clinical and conceptual consideration and transdiagnostic treatment. Adv. Exp. Med. Biol. 1191 , 219–235 (2020).
Michopoulos, V., Vester, A. & Neigh, G. Posttraumatic stress disorder: a metabolic disorder in disguise? Exp. Neurol. 284 , 220–229 (2016).
Rosenbaum, S. et al. The prevalence and risk of metabolic syndrome and its components among people with posttraumatic stress disorder: a systematic review and meta-analysis. Metabolism 64 , 926–933 (2015).
Song, H. et al. Stress related disorders and risk of cardiovascular disease: population-based, sibling controlled cohort study. BMJ 365 , l1255 (2019).
Batelaan, N. M., Seldenrijk, A., Bot, M., van Balkom, A. J. & Penninx, B. W. Anxiety and new onset of cardiovascular disease: critical review and meta-analysis. Br. J. Psychiatry 208 , 223–231 (2016).
Emdin, C. A. et al. Meta-analysis of anxiety as a risk factor for cardiovascular disease. Am. J. Cardiol. 118 , 511–519 (2016).
Song, H. et al. Association of stress-related disorders with subsequent autoimmune disease. JAMA 319 , 2388–2400 (2018).
Bookwalter, D. B. et al. Posttraumatic stress disorder and risk of selected autoimmune diseases among US military personnel. BMC Psychiatry 20 , 23 (2020).
Song, H. et al. Stress related disorders and subsequent risk of life-threatening infections: population based sibling controlled cohort study. BMJ 367 , l5784 (2019).
Holman, E. A. Acute stress and cardiovascular health: is there an ACE gene connection? J. Trauma Stress 25 , 592–597 (2012).
Cole, S. W. et al. Computational identification of gene-social environment interaction at the human IL6 locus. Proc. Natl Acad. Sci. USA 107 , 5681–5686 (2010).
Article ADS CAS PubMed PubMed Central Google Scholar
Jensen, A. B. et al. Temporal disease trajectories condensed from population-wide registry data covering 6.2 million patients. Nat. Commun. 5 , 4022 (2014).
Article ADS CAS PubMed Google Scholar
Hidalgo, C. A., Blumm, N., Barabasi, A. L. & Christakis, N. A. A dynamic network approach for the study of human phenotypes. PLoS Comput. Biol. 5 , e1000353 (2009).
Hou, C. et al. Medical conditions associated with coffee consumption: disease-trajectory and comorbidity network analyses of a prospective cohort study in UK Biobank. Am. J. Clin. Nutr. 116 , 730–740 (2022).
Article CAS PubMed PubMed Central Google Scholar
Han, X. et al. Disease trajectories and mortality among individuals diagnosed with depression: a community-based cohort study in UK Biobank. Mol. Psychiatry 26 , 6736–6746 (2021).
Kubzansky, L. D., Koenen, K. C., Jones, C. & Eaton, W. W. A prospective study of posttraumatic stress disorder symptoms and coronary heart disease in women. Health Psychol. 28 , 125–130 (2009).
Burg, M. M. et al. Risk for incident hypertension associated with posttraumatic stress disorder in military veterans and the effect of posttraumatic stress disorder treatment. Psychosom. Med. 79 , 181–188 (2017).
Roy, S. S., Foraker, R. E., Girton, R. A. & Mansfield, A. J. Posttraumatic stress disorder and incident heart failure among a community-based sample of US veterans. Am. J. Public Health 105 , 757–763 (2015).
McGrath, J. J. et al. Comorbidity within mental disorders: a comprehensive analysis based on 145 990 survey respondents from 27 countries. Epidemiol. Psychiatr. Sci. 29 , e153 (2020).
Article MathSciNet CAS PubMed PubMed Central Google Scholar
Momen, N. C. et al. Association between mental disorders and subsequent medical conditions. N. Engl. J. Med. 382 , 1721–1731 (2020).
Jiang, T. et al. Posttraumatic stress disorder and incident infections: a nationwide cohort study. Epidemiology 30 , 911–917 (2019).
Kim, T. et al. Associations of mental health and sleep duration with menstrual cycle irregularity: a population-based study. Arch. Womens Ment. Health 21 , 619–626 (2018).
Jung, S. J. et al. Posttraumatic stress disorder and development of premenstrual syndrome in a longitudinal cohort of women. Arch. Womens Ment. Health 22 , 535–539 (2019).
Weisberg, R. B. et al. Nonpsychiatric illness among primary care patients with trauma histories and posttraumatic stress disorder. Psychiatr. Serv. 53 , 848–854 (2002).
Lauterbach, D., Vora, R. & Rakow, M. The relationship between posttraumatic stress disorder and self-reported health problems. Psychosom. Med. 67 , 939–947 (2005).
Siggaard, T. et al. Disease trajectory browser for exploring temporal, population-wide disease progression patterns in 7.2 million Danish patients. Nat. Commun. 11 , 4952 (2020).
Thompson, A. G. et al. Genome-wide association study of behavioural and psychiatric features in human prion disease. Transl. Psychiatry 5 , e552 (2015).
Anney, R. J. et al. Conduct disorder and ADHD: evaluation of conduct problems as a categorical and quantitative trait in the international multicentre ADHD genetics study. Am. J. Med. Genet. B Neuropsychiatr. Genet. 147B , 1369–1378 (2008).
He, W. et al. Association of novel loci with keratoconus susceptibility in a multitrait genome-wide association study of the UK Biobank database and Canadian longitudinal study on aging. JAMA Ophthalmol. 140 , 568–576 (2022).
Cheng, C. Y. et al. Nine loci for ocular axial length identified through genome-wide association studies, including shared loci with refractive error. Am. J. Hum. Genet. 93 , 264–277 (2013).
Gouveia, M. H. et al. Trans-ethnic meta-analysis identifies new loci associated with longitudinal blood pressure traits. Sci. Rep. 11 , 4075 (2021).
Kumar, A. et al. Association of SUMOylation pathway genes with stroke in a genome-wide association study in India. Neurology 97 , e345–e356 (2021).
Arning, A. et al. A genome-wide association study identifies a gene network of ADAMTS genes in the predisposition to pediatric stroke. Blood 120 , 5231–5236 (2012).
Hauger, R. L. et al. Molecular and cell signaling targets for PTSD pathophysiology and pharmacotherapy. Neuropharmacology 62 , 705–714 (2012).
Ge, Y. J. et al. Anti-inflammatory signaling through G protein-coupled receptors. Acta Pharmacol. Sin. 41 , 1531–1538 (2020).
Sriram, K. & Insel, P. A. G protein-coupled receptors as targets for approved drugs: how many targets and how many drugs? Mol. Pharmacol. 93 , 251–258 (2018).
C.T. Development America, I. A study of RX-10045 in the treatment of dry eye disease. https://ClinicalTrials.gov/show/NCT01675570 (2012).
C.T. Development America, I. Evaluation of the onset and duration of action of RX-10045 in allergic conjunctivitis. https://ClinicalTrials.gov/show/NCT01639846 (2012).
Chen, P., Li, B. & Ou-Yang, L. Role of estrogen receptors in health and disease. Front. Endocrinol. 13 , 839005 (2022).
Article Google Scholar
Barabasi, A. L., Gulbahce, N. & Loscalzo, J. Network medicine: a network-based approach to human disease. Nat. Rev. Genet. 12 , 56–68 (2011).
Ludvigsson, J. F. et al. External review and validation of the Swedish national inpatient register. BMC Public Health 11 , 450 (2011).
Quan, H. et al. Coding algorithms for defining comorbidities in ICD-9-CM and ICD-10 administrative data. Med. Care 43 , 1130–1139 (2005).
Vilaplana-Perez, A. et al. Validity and reliability of social anxiety disorder diagnoses in the Swedish National Patient Register. BMC Psychiatry 20 , 242 (2020).
Hollander, A. C. et al. Validation study of randomly selected cases of PTSD diagnoses identified in a Swedish regional database compared with medical records: is the validity sufficient for epidemiological research? BMJ Open 9 , e031964 (2019).
Longitudinell Integrationsdatabas för Sjukförsäkrings- och Arbetsmarknadsstudier (LISA). Statistiska Centralbyrån, (Statistiska Centralbyrån, 2018).
Fry, A. et al. Comparison of sociodemographic and health-related characteristics of UK Biobank participants with those of the general population. Am. J. Epidemiol. 186 , 1026–1034 (2017).
Sudlow, C. et al. UK biobank: an open access resource for identifying the causes of a wide range of complex diseases of middle and old age. PLoS Med. 12 , e1001779 (2015).
Carney, R. M., Freedland, K. E., Eisen, S. A., Rich, M. W. & Jaffe, A. S. Major depression and medication adherence in elderly patients with coronary artery disease. Health Psychol. 14 , 88–90 (1995).
Townsend, P., Phillimore, P. & Beattie, A. Health and Deprivation: Inequality and the North (Routledge, 1988).
Katz, D., Baptista, J., Azen, S. & Pike, M. Obtaining confidence intervals for the risk ratio in cohort studies. Biometrics 34 , 469–474 (1978).
Meo, P. D., Ferrara, E., Fiumara, G. & Provetti, A. Generalized Louvain method for community detection in large networks. In Proc. 11th International Conference on Intelligent Systems Design and Applications , 88–93 (2011).
Choi, S. W., Mak, T. S. & O’Reilly, P. F. Tutorial: a guide to performing polygenic risk score analyses. Nat. Protocols 15 , 2759–2772 (2020).
Jiang, L. et al. A resource-efficient tool for mixed model association analysis of large-scale data. Nat. Genet. 51 , 1749–1755 (2019).
Bulik-Sullivan, B. K. et al. LD Score regression distinguishes confounding from polygenicity in genome-wide association studies. Nat. Genet. 47 , 291–295 (2015).
Watanabe, K., Taskesen, E., van Bochoven, A. & Posthuma, D. Functional mapping and annotation of genetic associations with FUMA. Nat. Commun. 8 , 1826 (2017).
Article ADS PubMed PubMed Central Google Scholar
Consortium, G. T. The GTEx Consortium atlas of genetic regulatory effects across human tissues. Science 369 , 1318–1330 (2020).
Wang, D. et al. Comprehensive functional genomic resource and integrative model for the human brain. Science 362 , eaat8464 (2018).
Schmitt, A. D. et al. A compendium of chromatin contact maps reveals spatially active regions in the human genome. Cell Rep. 17 , 2042–2059 (2016).
Zhou, Y. et al. Metascape provides a biologist-oriented resource for the analysis of systems-level datasets. Nat. Commun. 10 , 1523 (2019).
Bader, G. D. & Hogue, C. W. An automated method for finding molecular complexes in large protein interaction networks. BMC Bioinform. 4 , 2 (2003).
Szklarczyk, D. et al. STRING v11: protein–protein association networks with increased coverage, supporting functional discovery in genome-wide experimental datasets. Nucleic Acids Res. 47 , D607–D613 (2018).
Article PubMed Central Google Scholar
Stark, C. et al. BioGRID: a general repository for interaction datasets. Nucleic Acids Res. 34 , D535–D539 (2006).
Türei, D., Korcsmáros, T. & Saez-Rodriguez, J. OmniPath: guidelines and gateway for literature-curated signaling pathway resources. Nat. Methods 13 , 966–967 (2016).
Li, T. et al. A scored human protein-protein interaction network to catalyze genomic interpretation. Nat. Methods 14 , 61–64 (2017).
Download references
Acknowledgements
We thank the team members and colleagues involved in the West China Biomedical Big Data Center- UK Biobank project for their support. This work is supported by the National Natural Science Foundation of China (No. 81971262 to H.S.), 1.3.5 project for disciplines of excellence, West China Hospital, Sichuan University (No. ZYYC21005 to H.S.), EU Horizon2020 Research and Innovation Action Grant (847776 to U.V. and F.F.), Consolidator grant from the European Research Council (726413 to U.V.), the Fundamental Research Funds for Central Universities (No. 20826041F4144 to X.H.), the Outstanding Clinical Discipline Project of Shanghai Pudong (No.PWYgy2021-02 to Q.S.) and the Fundamental Research Funds for the Central Universities (to Q.S.). This research has been conducted using the UK Biobank Resource under Application 54803. This work uses data provided by patients and collected by the NHS as part of their care and support. This research used data assets made available by National Safe Haven as part of the Data and Connectivity National Core Study, led by Health Data Research UK in partnership with the Office for National Statistics and funded by UK Research and Innovation (grant ref: MC_PC_20029 and MC_PC_20058).
Author information
These authors contributed equally: Xin Han, Qing Shen.
These authors jointly supervised this work: Unnur A Valdimarsdóttir, Huan Song.
Authors and Affiliations
Mental Health Center, West China Hospital, Sichuan University, Chengdu, China
West China Biomedical Big Data Center, West China Hospital, Sichuan University, Chengdu, China
Xin Han, Can Hou, Huazhen Yang, Wenwen Chen, Yu Zeng, Yuanyuan Qu & Huan Song
Med-X Center for Informatics, Sichuan University, Chengdu, China
Clinical Research Center for Mental Disorders, Shanghai Pudong New Area Mental Health Center, Tongji University School of Medicine, Shanghai, China
Institute for Advanced Study, Tongji University, Shanghai, China
Institute of Environmental Medicine, Karolinska Institutet, Stockholm, Sweden
Qing Shen, Fang Fang & Unnur A. Valdimarsdóttir
Department of Epidemiology & Ministry of Education Key Laboratory of Public Health Safety, School of Public Health, Fudan University, Shanghai, China
Taizhou Institute of Health Sciences, Fudan University, Taizhou, China
Department of Medical Epidemiology and Biostatistics, Karolinska Institutet, Stockholm, Sweden
Center of Public Health Sciences, Faculty of Medicine, University of Iceland, Reykjavík, Iceland
Unnur A. Valdimarsdóttir & Huan Song
Department of Epidemiology, Harvard T H Chan School of Public Health, Boston, MA, USA
Unnur A. Valdimarsdóttir
You can also search for this author in PubMed Google Scholar
Contributions
H.S. and U.A.V. were responsible for the study concept and design. X.H., Q.S., H.Y., W.C., Y.Z., Y.Q. and W.Y. did the data and project management. X.H., Q.S. and C.H. did the data cleaning and analysis. X.H., Q.S., C.S., H.S., U.A.V. and F.F. interpreted the data. X.H., Q.S., U.A.V., F.F. and H.S. drafted the manuscript. All authors approved the final manuscript as submitted and agree to be accountable for all aspects of the work. The corresponding author attests that all listed authors meet authorship criteria and that no others meeting the criteria have been omitted.
Corresponding author
Correspondence to Huan Song .
Ethics declarations
Competing interests.
The authors declare no competing interests.
Peer review
Peer review information.
Nature Communications thanks Amaia Calderón-Larrañaga and the other, anonymous, reviewer(s) for their contribution to the peer review of this work. A peer review file is available.
Additional information
Publisher’s note Springer Nature remains neutral with regard to jurisdictional claims in published maps and institutional affiliations.
Supplementary information
Supplementary information, peer review file, description of additional supplementary files, supplementary data 1-12, reporting summary, rights and permissions.
Open Access This article is licensed under a Creative Commons Attribution 4.0 International License, which permits use, sharing, adaptation, distribution and reproduction in any medium or format, as long as you give appropriate credit to the original author(s) and the source, provide a link to the Creative Commons licence, and indicate if changes were made. The images or other third party material in this article are included in the article’s Creative Commons licence, unless indicated otherwise in a credit line to the material. If material is not included in the article’s Creative Commons licence and your intended use is not permitted by statutory regulation or exceeds the permitted use, you will need to obtain permission directly from the copyright holder. To view a copy of this licence, visit http://creativecommons.org/licenses/by/4.0/ .
Reprints and permissions
About this article
Cite this article.
Han, X., Shen, Q., Hou, C. et al. Disease clusters subsequent to anxiety and stress-related disorders and their genetic determinants. Nat Commun 15 , 1209 (2024). https://doi.org/10.1038/s41467-024-45445-2
Download citation
Received : 01 May 2023
Accepted : 23 January 2024
Published : 08 February 2024
DOI : https://doi.org/10.1038/s41467-024-45445-2
Share this article
Anyone you share the following link with will be able to read this content:
Sorry, a shareable link is not currently available for this article.
Provided by the Springer Nature SharedIt content-sharing initiative
By submitting a comment you agree to abide by our Terms and Community Guidelines . If you find something abusive or that does not comply with our terms or guidelines please flag it as inappropriate.
Quick links
- Explore articles by subject
- Guide to authors
- Editorial policies
Sign up for the Nature Briefing newsletter — what matters in science, free to your inbox daily.

Module 4: Mood Disorders
3rd edition as of July 2023
Module Overview
In Module 4, we will discuss matters related to mood disorders to include their clinical presentation, epidemiology, comorbidity, etiology, and treatment options. Our discussion will cover major depressive disorder, persistent depressive disorder (formerly Dysthymia), bipolar I disorder, bipolar II disorder, and cyclothymic disorder. We will also cover major depressive, manic, and hypomanic episodes. Be sure you refer Modules 1-3 for explanations of key terms (Module 1), an overview of the various models to explain psychopathology (Module 2), and descriptions of several therapies (Module 3). Note that this module will cover two chapters from the DSM 5-TR; namely, Bipolar and Related Disorders and Depressive Disorders.
Module Outline
4.1. Clinical Presentation – Depressive Disorders
4.2. clinical presentation – bipolar and related disorders, 4.3. epidemiology, 4.4. comorbidity, 4.5. etiology.
- 4.6. Treatment
Module Learning Outcomes
- Describe how depressive disorders present.
- Describe how bipolar and related disorders present.
- Describe the epidemiology of mood disorders.
- Describe comorbidity in relation to mood disorders.
- Describe the etiology of mood disorders.
- Describe treatment options for mood disorders.
Section Learning Objectives
- Distinguish the two distinct groups of mood disorders.
- Identify and describe the two types of depressive disorders.
- Classify symptoms of depression.
- Describe premenstrual dysphoric disorder.
4.1.1. Distinguishing Mood Disorders
Within mood disorders are two distinct groups—individuals with depressive disorders and individuals with bipolar disorders. The key difference between the two mood disorder groups is episodes of mania/hypomania. More specifically, in bipolar I disorder, the individual experiences a manic episode that “may have been preceded by and may be followed by hypomanic or major depressive episodes” (APA, 2022, pg. 139) whereas for bipolar II disorder, the individual has experienced in the past or is currently experiencing a hypomanic episode and has experienced in the past or is currently experiencing a major depressive episode. In contrast, individuals presenting with a depressive disorder have never experienced a manic or hypomanic episode.
4.1.2. Types of Depressive Disorders
The two most common types of depressive disorders are major depressive disorder (MDD) and persistent depressive disorder (PDD). Persistent depressive disorder , which in the DSM-5 now includes the diagnostic categories of dysthymia and chronic major depression, is a continuous and chronic form of depression. While the symptoms of PDD are very similar to MDD, they are usually less acute, as symptoms tend to ebb and flow over a long period (i.e., more than two years). Major depressive disorder , on the other hand, has discrete episodes lasting at least two weeks in which there are substantial changes in affect, cognition, and neurovegetative functions (APA, 2022, pg. 177).
It should be noted that after a careful review of the literature, premenstrual dysphoric disorder , was moved from “Criteria Sets and Axes Provided for Future Study” in the DSM-IV to Section II of DSM-5 as the disorder was confirmed as a “specific and treatment-responsive form of depressive disorder that begins sometime following ovulation and remits within a few days of menses and has a marked impact on functioning” (APA, 2022, pg. 177).
The DSM-5 also added a new diagnosis, disruptive mood dysregulation disorder (DMDD), for children up to 12 years of age, to deal with the potential for overdiagnosis and treatment of bipolar disorder in children, both in the United States and internationally. Children with DMDD present with persistent irritability and frequent episodes of extreme behavioral dyscontrol and so develop unipolar, not bipolar, depressive disorders or anxiety disorders as they move into adolescence and adulthood.
For a discussion of DMDD, please visit our sister book, Behavioral Disorders of Childhood:
https://opentext.wsu.edu/behavioral-disorders-childhood/
4.1.3. Symptoms Associated with Depressive Disorders
When making a diagnosis of depression, there are a wide range of symptoms that may be present. These symptoms can generally be grouped into four categories: mood, behavioral, cognitive, and physical symptoms.
4.1.3.1. Mood. While clinical depression can vary in its presentation among individuals, most, if not all individuals with depression will report significant mood disturbances such as a depressed mood for most of the day and/or feelings of anhedonia , which is the loss of interest in previously interesting activities.
4.1.3.2. Behavioral. Behavioral issues such as decreased physical activity and reduced productivity—both at home and work—are often observed in individuals with depression. This is typically where a disruption in daily functioning occurs as individuals with depressive disorders are unable to maintain their social interactions and employment responsibilities.
4.1.3.3. Cognitive. It should not come as a surprise that there is a serious disruption in cognitions as individuals with depressive disorders typically hold a negative view of themselves and the world around them. They are quick to blame themselves when things go wrong, and rarely take credit when they experience positive achievements. Individuals with depressive disorders often feel worthless, which creates a negative feedback loop by reinforcing their overall depressed mood. They also report difficulty concentrating on tasks, as they are easily distracted from outside stimuli. This assertion is supported by research that has found individuals with depression perform worse than those without depression on tasks of memory, attention, and reasoning (Chen et al., 2013). Finally, thoughts of suicide and self-harm do occasionally occur in those with depressive disorders ( Note – this will be discussed in more detail in Section 4.3 ).
4.1.3.4. Physical. Changes in sleep patterns are common in those experiencing depression with reports of both hypersomnia and insomnia. Hypersomnia , or excessive sleeping, often impacts an individual’s daily functioning as they spend the majority of their time sleeping as opposed to participating in daily activities (i.e., meeting up with friends or getting to work on time). Reports of insomnia are also frequent and can occur at various points throughout the night to include difficulty falling asleep, staying asleep, or waking too early with the inability to fall back asleep before having to wake for the day. Although it is unclear whether symptoms of fatigue or loss of energy are related to insomnia issues, the fact that those experiencing hypersomnia also report symptoms of fatigue suggests that these symptoms are a component of the disorder rather than a secondary symptom of sleep disturbance.
Additional physical symptoms, such as a change in weight or eating behaviors, are also observed. Some individuals who are experiencing depression report a lack of appetite, often forcing themselves to eat something during the day. On the contrary, others overeat, often seeking “comfort foods,” such as those high in carbohydrates. Due to these changes in eating behaviors, there may be associated changes in weight.
Finally, psychomotor agitation or retardation, which is the purposeless or slowed physical movement of the body (i.e., pacing around a room, tapping toes, restlessness, etc.) is also reported in individuals with depressive disorders.
4.1.4. Diagnostic Criteria and Features for Depressive Disorders
4.1.4.1. Major depressive disorder (MDD). According to the DSM-5-TR (APA, 2022), to meet the criteria for a diagnosis of major depressive disorder, an individual must experience at least five symptoms across the four categories discussed above, and at least one of the symptoms is either 1) a depressed mood most of the day, almost every day, or 2) loss of interest or pleasure in all, or most, activities, most of the day, almost every day. These symptoms must be present for at least two weeks and cause clinically significant distress or impairment in important areas of functioning such as social and occupational. The DSM-5 cautions that responses to a significant loss (such as the death of a loved one, financial ruin, and discovery of a serious medical illness or disability), can lead to many of the symptoms described above (i.e., intense sadness, rumination about the loss, insomnia, etc.) but this may be the normal response to such a loss. Though the individual’s response resembles a major depressive episode, clinical judgment should be utilized in making any diagnosis and be based on the clinician’s understanding of the individual’s personal history and cultural norms related to how members should express distress in the context of loss.
4.1.4.2. Persistent depressive disorder (PDD). For a diagnosis of persistent depressive disorder, an individual must experience a depressed mood for most of the day, for more days than not, for at least two years. (APA, 2022) . This feeling of a depressed mood is also accompanied by two or more additional symptoms, to include changes in appetite, insomnia or hypersomnia, low energy or fatigue, low self-esteem, feelings of hopelessness, and poor concentration or difficulty with decision making. The symptoms taken together cause clinically significant distress or impairment in important areas of functioning such as social and occupational and these impacts can be as great as or greater than MDD. The individual may experience a temporary relief of symptoms; however, the individual will not be without symptoms for more than two months during this two-year period.
Making Sense of the Disorders
In relation to depressive disorders, note the following:
- Diagnosis MDD …… if symptoms have been experienced for at least two weeks and can be regarded as severe
- Diagnosis PDD … if the symptoms have been experienced for at least two years and are not severe
4.1.4.3. Premenstrual dysphoric disorder. In terms of premenstrual dysphoric disorder, the DSM-5-TR states in the majority of menstrual cycles, at least five symptoms must be present in the final week before the onset of menses, being improving with a few days after menses begins, and disappear or become negligible in the week postmenses. Individuals diagnosed with premenstrual dysphoric disorder must have one or more of the following: increased mood swings, irritability or anger, depressed mood, or anxiety/tension. Additionally, they must have one or more of the following to reach a total of five symptoms: anhedonia, difficulty concentrating, lethargy, changes in appetite, hypersomnia or insomnia, feelings of being overwhelmed or out of control, and/or experience breast tenderness or swelling. The symptoms lead to issues at work or school (i.e., decreased productivity and efficiency), within relationships (i.e., discord in the intimate partner relationship or with children, friends, or other family members), and with usual social activities (i.e., avoidance of the activities).
Key Takeaways
You should have learned the following in this section:
- Mood disorder fall into one of two groups – depressive or bipolar disorders – with the key distinction between the two being episodes of mania/hypomania.
- Symptoms of depression fall into one of four categories – mood, behavioral, cognitive, and physical.
- Persistent Depressive Disorder shares symptoms with Major Depressive Disorder though they are usually not as severe and ebb and flow over a period of at least two years.
- Premenstrual dysphoric disorder presents as mood lability, irritability, dysphoria, and anxiety symptoms occurring often during the premenstrual phase of the cycle and remit around the beginning of menses or shortly thereafter.
Section 4.1 Review Questions
- What are the different categories of mood disorder symptoms? Identify the symptoms within each category.
- What are the key differences in a major depression and a persistent depressive disorder diagnosis?
- What is premenstrual dysphoric disorder?
- Distinguish the forms bipolar disorder takes.
- Contrast a manic episode with a hypomanic episode.
- Define cyclothymic disorder.
4.2.1. Distinguishing Bipolar I and II Disorders
According to the DSM-5-TR (APA, 2022), there are two types of bipolar disorder- bipolar I and bipolar II. A diagnosis of bipolar I disorder is made when there is at least one manic episode. This manic episode can be preceded by and/or followed by a hypomanic or major depressive episode, however, diagnostic criteria for a manic episode is the only criteria that needs to be met for a bipolar I diagnosis. A diagnosis of bipolar II Disorder is made when there is a current or history of a hypomanic episode and a current or past major depressive episode. Descriptions of both manic and hypomanic episodes follow below.
In relation to bipoloar I and II disorders, note the following:
- Diagnosis bipolar I disorder …. if an individual has ever experienced a manic episode
- Diagnosis bipolar II disorder … if the criteria has only been met for a hypomanic episode
4.2.2. Manic and Hypomanic Episodes
4.2.2.1. Manic episode. The key feature of a manic episode is a specific period in which an individual reports abnormal, persistent, or expansive irritable mood for nearly all day, every day, for at least one week (APA, 2022). Additionally, the individual will display increased activity or energy during this same time. With regards to mood, an individual in a manic episode will appear excessively happy, often engaging haphazardly in sexual or interpersonal interactions. They also display rapid shifts in mood, also known as mood lability , ranging from happy, neutral, to irritable. At least three of the symptoms described below (four if the mood is only irritable) must be present and represent a noticeable change in the individual’s typical behavior.
Inflated self-esteem or grandiosity (Criterion B1) is present during a manic episode. Occasionally these inflated self-esteem levels can appear delusional. For example, individuals may believe they are friends with a celebrity, do not need to abide by laws, or even perceive themselves as God. They also engage in multiple overlapping new projects (Criteria B6 and 7), often initiated with no prior knowledge about the topic, and engaged in at unusual hours of the day.
Despite the increased activity level, individuals experiencing a manic episode also require a decreased need for sleep (Criterion B2), sleeping as little as a few hours a night yet still feeling rested. Reduced need for sleep may also be a precursor to a manic episode, suggesting that a manic episode is to begin imminently. It is not uncommon for those experiencing a manic episode to be more talkative than usual. It can be difficult to follow their conversation due to the quick pace of their talking, as well as tangential storytelling. Additionally, they can be difficult to interrupt in conversation, often disregarding the reciprocal nature of communication (Criterion B3). If the individual is more irritable than expansive, speech can become hostile and they engage in tirades, particularly if they are interrupted or not allowed to engage in an activity they are seeking out (APA, 2022).
Based on their speech pattern, it should not be a surprise that racing thoughts and flights of ideas (Criterion B4) also present during manic episodes. Because of these rapid thoughts, speech may become disorganized or incoherent. Finally, individuals experiencing a manic episode are distractable (Criterion B5).
4.2.2.2. Hypomanic episode. As mentioned above, for a bipolar II diagnosis, an individual must report symptoms consistent with a major depressive episode and at least one hypomanic episode. An individual with bipolar II disorder must not have a history of a manic episode—if there is a history of mania, the diagnosis will be diagnosed with bipolar I. A hypomanic episode is like a manic episode in that the individual will experience abnormally and persistently elevated, expansive, or irritable mood and energy levels, however, the behaviors are not as extreme as in mania. Additionally, behaviors consistent with a hypomanic episode must be present for at least four days, compared to the one week in a manic episode.
Take note of the following in relation to manic and hypomanic episodes:
- A manic episode is severe enough to cause impairments in social or occupational functioning and can lead to hospitalization to prevent harm to self or others.
- A hypomanic episode is NOT severe enough to cause such impairments or hospitalization.
4.2.3. Cyclothymic Disorder
Notably, there is a subclass of individuals who experience numerous periods with hypomanic symptoms that do not meet the criteria for a hypomanic episode and mild depressive symptoms (i.e., do not fully meet criteria for a major depressive episode). These individuals are diagnosed with cyclothymic disorder (APA, 2022). Presentation of these symptoms occur for two or more years and are typically interrupted by periods of normal mood not lasting more than two months at a time. The symptoms cause clinically significant distress or impairment in important areas of functioning, such as social and occupational. While only a small percentage of the population develops cyclothymic disorder, it can eventually progress into bipolar I or bipolar II disorder (Zeschel et al., 2015).
- An individual is diagnosed with bipolar I disorder if they have ever experienced a manic episode and are diagnosed with bipolar II disorder if the criteria has only been met for a hypomanic episode.
- A manic episode is characterized by a specific period in which an individual reports abnormal, persistent, or expansive irritable mood for nearly all day, every day, for at least one week.
- A hypomanic episode is characterized by abnormally and persistently elevated, expansive, or irritable mood and energy levels, though not as extreme as in mania, and must be present for at least four days. It is also not severe enough to cause impairments or hospitalization.
- Cyclothymic disorder includes periods of hypomanic and mild depressive symptoms without meeting the criteria for a depressive episode which lasts two or more years and is interrupted by periods of normal moods.
Section 4.2 Review Questions
- What is the difference between bipolar I and II disorder?
- What are the key diagnostic differences between a hypomanic and manic episode?
- What is cyclothymic disorder?
- Describe the epidemiology of depressive disorders.
- Describe the epidemiology of bipolar disorders.
- Describe the epidemiology of suicidality.
4.3.1. Depressive Disorders
According to the DSM-5-TR (APA, 2022), the 12-month prevalence rate for major depressive disorder is approximately 7% within the United States. Recall that DSM-5 persistent depressive disorder is a blend of DSM-IV dysthymic disorder and chronic major depressive disorder. The prevalence rate for DSM-IV dysthymic disorder is much lower than MDD, with a 0.5% rate among adults in the United States, while DSM-IV chronic major depressive disorder is 1.5%.
As well, individuals in the 18- to 29- year-old age bracket report the highest rates of MDD than any other age group. Women experience about twofold higher rates than men of MDD, especially between menarche and menopause (APA, 2022). The estimated lifetime prevalence for major depressive disorder in women is 21.3% compared to 12.7% in men (Nolen-Hoeksema, 2001). Regrading DSM-IV dysthymic disorder and chronic major depressive disorder, the prevalence among women is 1.5 and 2 times greater than the prevalence for men for each of these diagnoses, respectively (APA, 2022).
4.3.2. Bipolar Disorders
The 12-month prevalence of bipolar I disorder in the United States is 1.5% and did not differ statistically between men and women. In contrast, bipolar II disorder has a prevalence rate of 0.8% in the United States and 0.3% internationally (APA, 2022) and some clinical samples suggest it is more common in women, with approximately 80-90% of individuals with rapid-cycling episodes being women (Bauer & Pfenning, 2005). Childbirth may be a specific trigger for a hypomanic episode, occurring in 10-20% of women in nonclinical settings and most often in the early postpartum period.
4.3.3. Suicidality
Individuals with a depressive disorder have a 17-fold increased risk for suicide over the age- and sex-adjusted general population rate. Features associated with an increased risk for death by suicide include anhedonia, living alone, being single, disconnecting socially, having access to a firearm, early life adversity, sleep disturbance, feelings of hopelessness, and problems with decision making. Women attempt suicide at a higher rate though men are more likely to complete suicide. Finally, the premenstrual phase is considered a risk period for suicide by some (APA, 2022).
In terms of bipolar disorders, the lifetime risk of suicide is estimated to be 20- to 30- fold greater than in the general population and 5-6% of individuals with bipolar disorder die by suicide. Like depressive disorders, women attempt suicide at a higher rate though lethal suicide is more common in men with bipolar disorder. About 1/3 of individuals with bipolar II disorder report a lifetime history of suicide attempt, which is similar in bipolar I disorder, though lethality of attempts is higher in individuals with bipolar II (APA, 2022).
- Major depressive disorder is experienced by about 7% of the population in the United States, afflicting young adults and women the most.
- Bipolar I disorder afflicts 1.5% and bipolar II disorder afflicts 0.8% of the U.S. population with bipolar II affecting women more than men and no gender difference being apparent for bipolar I.
- Individuals with a depressive disorder have a 17-fold increased risk for suicide while the lifetime risk of suicide for an individual with a bipolar disorder is estimated to be 20- to 30- fold greater than in the general population and 5-6% of individuals with bipolar disorder die by suicide.
Section 4.3 Review Questions
- What are the prevalence rates of the mood disorders?
- What gender differences exist in the rate of occurrence of mood disorders?
- How do depressive and bipolar disorders compare in terms of suicidality (attempts and lethality)?
- Describe the comorbidity of depressive disorders.
- Describe the comorbidity of bipolar disorders.
4.4.1. Depressive Disorders
Studies exploring depression symptoms among the general population show a substantial pattern of comorbidity between depression and other mental disorders, particularly substance use disorders (Kessler, Berglund, et al., 2003). Nearly three-fourths of participants with lifetime MDD in a large-scale research study also met the criteria for at least one other DSM disorder (Kessler, Berglund, et al., 2003). MDD has been found to co-occur with substance-related disorders, panic disorder, generalized anxiety disorder, PTSD, OCD, anorexia, bulimia, and borderline personality disorder. Gender differences do exist within comorbidities such that women report comorbid anxiety disorders, bulimia, and somatoform disorders while men report comorbid alcohol and substance abuse. In contrast, those with PDD are at higher risk for psychiatric comorbidity in general and for anxiety disorders, substance use disorders, and personality disorders in particular (APA, 2022).
Given the extent of comorbidity among individuals with MDD, researchers have tried to identify which disorder precipitated the other. The majority of studies found that most depression cases occur secondary to another mental health disorder, meaning that the onset of depression is a direct result of the onset of another disorder (Gotlib & Hammen, 2009).
4.4.2. Bipolar Disorders
Those with bipolar I disorder typically have a history of three or more mental disorders. The most frequent comorbid disorders include anxiety disorders, alcohol use disorder, other substance use disorder, and ADHD, along with borderline, schizotypal, and antisocial personality disorder.
Bipolar II disorder is more often than not associated with one or more comorbid mental disorders, with anxiety disorders being the most common (38% with social anxiety, 36% with specific phobia, and 30% having generalized anxiety). As with bipolar I, substance use disorders are common with alcohol use (42%) leading the way, followed by cannabis use (20%). Premenstrual syndrome and premenstrual dysphoric disorder are common in women with bipolar II disorder especially (APA, 2022).
Finally, cyclothymic disorder has been found to be comorbid with substance-related disorders and sleep disorders.
- Depressive disorders have a high comorbidity with substance use disorders, anxiety disorders, and some personality disorders.
- Bipolar disorders have a high comorbidity with anxiety disorders and substance abuse disorders while cyclothymic disorder is comorbid with substance-related disorders and sleep disorders.
Section 4.4 Review Questions
- What are common comorbidities for the depressive disorders?
- What are common comorbidities for bipolar disorders?
- Describe the biological causes of mood disorders.
- Describe the cognitive causes of mood disorders.
- Describe the behavioral causes of mood disorders.
- Describe the sociocultural causes of mood disorders.
4.5.1. Biological
Research throughout the years continues to provide evidence that depressive disorders have some biological cause. While it does not explain every depressive case, it is safe to say that some individuals may at least have a predisposition to developing a depressive disorder. Among the biological factors are genetic factors, biochemical factors, and brain structure.
4.5.1.1. Genetics. Like with any disorder, researchers often explore the prevalence rate of depressive disorders among family members to determine if there is some genetic component, whether it be a direct link or a predisposition. If there is a genetic predisposition to developing depressive disorders, one would expect a higher rate of depression within families than that of the general population. Research supports this with regards to depressive disorders as there is nearly a 30% increase in relatives diagnosed with depression compared to 10% of the general population (Levinson & Nichols, 2014). Similarly, there is an elevated prevalence among first-degree relatives for both Bipolar I and Bipolar II disorders as well.
Another way to study the genetic component of a disorder is via twin studies. One would expect identical twins to have a higher rate of the disorder as opposed to fraternal twins, as identical twins share the same genetic make-up, whereas fraternal twins only share roughly 50%, similar to that of siblings. A large-scale study found that if one identical twin was diagnosed with depression, there was a 46% chance their identical twin was diagnosed with depression. In contrast, the rate of a depression diagnosis in fraternal twins was only 20%. Despite the fraternal twin rate still being higher than that of a first-degree relative, this study provided enough evidence that there is a strong genetic link in the development of depression (McGuffin et al., 1996).
More recently, scientists have been studying depression at a molecular level, exploring possibilities of gene abnormalities as a cause for developing a depressive disorder. While much of the research is speculation due to sampling issues and low power, there is some evidence that depression may be tied to the 5-HTT gene on chromosome 17, as this is responsible for the activity of serotonin (Jansen et al., 2016).
Bipolar disorders share a similar genetic predisposition to that of major depressive disorder. Twin studies within bipolar disorder yielded concordance rates for identical twins at as high as 72%, yet the range for fraternal twins, siblings, and other close relatives ranged from 5-15%. It is important to note that both percentages are significantly higher than that of the general population, suggesting a strong genetic component within bipolar disorder (Edvardsen et al., 2008). The DSM-5-TR more recently reports heritability estimates around 90% in some twin studies and the risk of bipolar disorder being around 1% in the general population compared to 5-10% in a first-degree relative (APA, 2022).
4.5.1.2. Biochemical. As you will read in the treatment section, there is strong evidence of a biochemical deficit in depression and bipolar disorders. More specifically, low activity levels of norepinephrine and serotonin, have long been documented as contributing factors to developing depressive disorders. This relationship was discovered accidentally in the 1950s when MAOIs were given to tuberculosis patients, and miraculously, their depressive moods were also improved. Soon thereafter, medical providers found that medications used to treat high blood pressure by causing a reduction in norepinephrine also caused depression in their patients (Ayd, 1956).
While these initial findings were premature in the identification of how neurotransmitters affected the development of depressive features, they did provide insight as to what neurotransmitters were involved in this system. Researchers are still trying to determine exact pathways; however, it does appear that both norepinephrine and serotonin are involved in the development of symptoms, whether it be between the interaction between them, or their interaction on other neurotransmitters (Ding et al., 2014).
Due to the close nature of depression and bipolar disorder, researchers initially believed that both norepinephrine and serotonin were implicated in the development of bipolar disorder; however, the idea was that there was a drastic increase in serotonin during mania episodes. Unfortunately, research supports the opposite. It is believed that low levels of serotonin and high levels of norepinephrine may explain mania episodes (Soreff & McInnes, 2014). Despite these findings, additional research within this area is needed to conclusively determine what is responsible for the manic episodes within bipolar disorder.
4.5.1.3. Endocrine system. As you may know, the endocrine system is a collection of glands responsible for regulating hormones, metabolism, growth and development, sleep, and mood, among other things. Some research has implicated hormones, particularly cortisol , a hormone released as a stress response, in the development of depression (Owens et al., 2014). Additionally, melatonin , a hormone released when it is dark outside to assist with the transition to sleep, may also be related to depressive symptoms, particularly during the winter months.
4.5.1.4. Brain anatomy. Seeing as neurotransmitters have been implicated in the development of depressive disorders, it should not be a surprise that various brain structures have also been identified as contributors to mood disorders. While exact anatomy and pathways are yet to be determined, research studies implicate the prefrontal cortex , the hippocampus , and the amygdala . More specifically, drastic changes in blood flow throughout the prefrontal cortex have been linked with depressive symptoms. Similarly, a smaller hippocampus, and consequently, fewer neurons, has also been linked to depressive symptoms. Finally, heightened activity and blood flow in the amygdala, the brain area responsible for our fight or flight response, is also consistently found in individuals with depressive symptoms.
Abnormalities in several brain structures have also been identified in individuals with bipolar disorder; however, what or why these structures are abnormal has yet to be determined. Researchers continue to focus on areas of the basal ganglia and cerebellum, which appear to be much smaller in individuals with bipolar disorder compared to the general public. Additionally, there appears to be a decrease in brain activity in regions associated with regulating emotions, as well as an increase in brain activity among structures related to emotional responsiveness (Houenou et al., 2011). Additional research is still needed to determine precisely how each of these brain structures may be implicated in the development of bipolar disorder.
4.5.2. Cognitive
The cognitive model, arguably the most conclusive model with regards to depressive disorders, focuses on the negative thoughts and perceptions of an individual. One theory often equated with the cognitive model of depression is learned helplessness. Coined by Martin Seligman (1975), learned helplessness was developed based on his laboratory experiment involving dogs. In this study, Seligman restrained dogs in an apparatus and routinely shocked them regardless of their behavior. The following day, the dogs were placed in a similar apparatus; however, this time they were not restrained and there was a small barrier placed between the “shock” floor and the “safe” floor. What Seligman observed was that despite the opportunity to escape the shock, the dogs flurried for a bit, and then ultimately laid down and whimpered while being shocked.
Based on this study, Seligman concluded that the animals essentially learned that they were unable to avoid the shock the day prior, and therefore, learned that they were helpless in preventing the shocks. When they were placed in a similar environment but had the opportunity to escape the shock, their learned helplessness carried over, and they continued to believe they were unable to escape the shock.
This study has been linked to humans through research on attributional style (Nolen-Hoeksema, Girgus & Seligman, 1992). There are two types of attributional styles—positive and negative. A negative attributional style focuses on the internal, stable , and global influence of daily lives, whereas a positive attributional style focuses on the external, unstable, and specific influence of the environment. Research has found that individuals with a negative attributional style are more likely to experience depression. This is likely due to their negative interpretation of daily events. For example, if something bad were to happen to them, they would conclude that it is their fault (internal), bad things always happen to them (stable), and bad things happen all day to them. Unfortunately, this maladaptive thinking style often takes over an individual’s daily view, thus making them more vulnerable to depression.
In addition to attributional style, Aaron Beck also attributed negative thinking as a precursor to depressive disorders (Beck, 2002, 1991, 1967). Often viewed as the grandfather of Cognitive-Behavioral Therapy, Beck went on to coin the terms—maladaptive attitudes, cognitive triad, errors in thinking, and automatic thoughts—all of which combine to explain the cognitive model of depressive disorders.
Maladaptive attitudes , or negative attitudes about oneself, others, and the world around them are often present in those with depressive symptoms. These attitudes are inaccurate and often global. For example, “If I fail my exam, the world will know I’m stupid.” Will the entire world really know you failed your exam? Not likely. Because you fail the exam, are you stupid? No. Individuals with depressive symptoms often develop these maladaptive attitudes regarding everything in their life, indirectly isolating themselves from others. The cognitive triad also plays into the maladaptive attitudes in that the individual interprets these negative thoughts about their experiences, themselves , and their futures.
Cognitive distortions, also known as errors in thinking , are a key component in Beck’s cognitive theory. Beck identified 15 errors in thinking that are most common in individuals with depression (see the end of the module). Among the most common are catastrophizing, jumping to conclusions, and overgeneralization. I always like to use my dad (first author’s dad) as an example for overgeneralization. Whenever we go to the grocery store, he always comments about how whatever line he chooses, at every store, it is always the slowest line. Does this happen every time he is at the store? I’m doubtful, but his error in thinking leads to him believing this is true.
Finally, automatic thoughts , or the constant stream of negative thoughts, also leads to symptoms of depression as individuals begin to feel as though they are inadequate or helpless in a given situation. While some cognitions are manipulated and interpreted negatively, Beck stated that there is another set of negative thoughts that occur automatically. Research studies have continually supported Beck’s maladaptive thoughts, attitudes, and errors in thinking as fundamental issues in those with depressive disorders (Lai et al., 2014; Possel & Black, 2014). Furthermore, as you will see in the treatment section (Section 4.5), cognitive strategies are among the most effective forms of treatment for depressive disorders.
4.5.3. Behavioral
The behavioral model explains depression as a result of a change in the number of rewards and punishments one receives throughout their life. This change can come from work, intimate relationships, family, or even the environment in general. Among the most influential in the field of depression is Peter Lewinsohn. He stated depression occurred in most people due to reduced positive rewards in their life. Because they were not positively rewarded, their constructive behaviors occurred more infrequently until they stop engaging in the behavior completely (Lewinsohn et al., 1990; 1984). An example of this is a student who keeps receiving bad grades on their exam despite studying for hours. Over time, the individual will reduce the amount of time they are studying, thus continuing to earn poor grades.
4.5.4. Sociocultural
In the sociocultural theory, the role of family and one’s social environment play a substantial role in the development of depressive disorders. There are two sociocultural views: the family-social perspective and the multi-cultural perspective.
4.5.4.1. Family-social perspective. Similar to that of the behavioral theory, the family-social perspective of depression suggests that depression is related to the unavailability of social support. This is often supported by research studies that show separated and divorced individuals are three times more likely to experience depressive symptoms than those that are married or even widowed (Schultz, 2007). While many factors lead a couple to separate or end their marriage, some relationships end due to a spouse’s mental health issues, particularly depressive symptoms. Depressive symptoms have been positively related to increased interpersonal conflicts, reduced communication, and intimacy issues, all of which are often reported in causal factors leading to a divorce (Najman et al., 2014).
The family-social perspective can also be viewed oppositely, with stress and marital discord leading to increased rates of depression in one or both spouses (Nezlek et al., 2000). While some research indicates that having children provides a positive influence in one’s life, it can also lead to stress both within the individual, as well as between partners due to division of work and discipline differences. Studies have shown that women who had three or more young children, and also lacked a close confidante and outside employment, were more likely than other mothers to become depressed (Brown, 2002).
4.5.4.2. Multi-cultural perspective. While depression is experienced across the entire world, one’s cultural background may influence what symptoms of depression are presented. Common depressive symptoms such as feeling sad, lack of energy, anhedonia, difficulty concentrating, and thoughts of suicide are a hallmark in most societies; other symptoms may be more specific to one’s nationality. More specifically, individuals from non-Western countries (China and other Asian countries) often focus on the physical symptoms of depression—tiredness, weakness, sleep issues—and less of an emphasis on the cognitive symptoms.
Within the United States, many researchers have explored potential differences across ethnic or racial groups in both rates of depression, as well as presenting symptoms of those diagnosed with depression. These studies continually fail to identify any significant differences between ethnic and racial groups; however, one major study has identified a difference in the rate of recurrence of depression in Hispanic and African Americans (Gonzalez et al., 2010). While the exact reason for this is unclear, researchers propose a lack of treatment opportunities as a possible explanation. According to Gonzalez and colleagues (2010), approximately 54% of depressed white Americans seek out treatment, compared to the 34% and 40% Hispanic and African Americans, respectively. The fact that there is a large discrepancy in the use of treatment between white Americans and minority Americans suggests that these individuals are not receiving the effective treatment necessary to resolve the disorder, thus leaving them more vulnerable for repeated depressive episodes.
4.5.4.3. Gender differences . As previously discussed, there is a significant difference between gender and rates of depression, with women twice as likely to experience an episode of depression than men (Schuch et al., 2014). There are a few speculations as to why there is such an imbalance in the rate of depression across genders.
The first theory, artifact theory, suggests that the difference between genders is due to clinician or diagnostic systems being more sensitive to diagnosing women with depression than men. While women are often thought to be more “emotional,” easily expressing their feelings and more willing to discuss their symptoms with clinicians and physicians, men often withhold their symptoms or will present with more traditionally “masculine” symptoms of anger or aggression. While this theory is a possible explanation for the gender differences in the rate of depression, research has failed to support this theory, suggesting that men and women are equally likely to seek out treatment and discuss their depressive symptoms (McSweeney, 2004; Rieker & Bird, 2005).
The second theory, hormone theory , suggests that variations in hormone levels trigger depression in women more than men (Graziottin & Serafini, 2009). While there is biological evidence supporting the changes in hormone levels during various phases of the menstrual cycle and their impact on women’s ability to integrate and process emotional information, research fails to support this theory as the reason for higher rates of depression in women (Whiffen & Demidenko, 2006).
The third theory, life stress theory, suggests that women are more likely to experience chronic stressors than men, thus accounting for their higher rate of depression (Astbury, 2010). Women face increased risk for poverty, lower employment opportunities, discrimination, and poorer quality of housing than men, all of which are strong predictors of depressive symptoms (Garcia-Toro et al., 2013).
The fourth theory, gender roles theory, suggests that social and or psychological factors related to traditional gender roles also influence the rate of depression in women. For example, men are often encouraged to develop personal autonomy, seek out activities that interest them, and display achievement-oriented goals; women are encouraged to empathize and care for others, often fostering an interdependent functioning, which may cause women to value the opinion of others more highly than their male counterparts do.
The final theory, rumination theory , suggests that women are more likely than men to ruminate, or intently focus, on their depressive symptoms, thus making them more vulnerable to developing depression at a clinical level (Nolen-Hoeksema, 2012). Several studies have supported this theory and shown that rumination of negative thoughts is positively related to an increase in depression symptoms (Hankin, 2009).
While many theories try to explain the gender discrepancy in depressive episodes, no single theory has produced enough evidence to fully explain why women experience depression more than men. Due to the lack of evidence, gender differences in depression remains one of the most researched topics within the subject of depression, while simultaneously being the least understood phenomena within clinical psychology.
- In terms of biological explanations for depressive disorders, there is evidence that rates of depression are higher among identical twins (the same is true for bipolar disorders), that the 5-HTT gene on chromosome 17 may be involved in depressive disorders, that norepinephrine and serotonin affect depressive (both being low) and bipolar disorders (low serotonin and high norepinephrine), the hormones cortisol and melatonin affect depression, and several brain structures are implicated in depression (prefrontal cortex, hippocampus, and amygdala) and bipolar disorder (basal ganglia and cerebellum).
- In terms of cognitive explanations, learned helplessness, attributional style, and maladaptive attitudes to include the cognitive triad, errors in thinking, and automatic thoughts, help to explain depressive disorders.
- Behavioral explanations center on changes in the rewards and punishments received throughout life.
- Sociocultural explanations include the family-social perspective and multi-cultural perspective.
- Women are twice as likely to experience depression and this could be due to women being more likely to be diagnosed than men (called the artifact theory), variations in hormone levels in women (hormone theory), women being more likely to experience chronic stressors (life stress theory), the fostering of an interdependent functioning in women (gender roles theory), and that women are more likely to intently focus on their symptoms (rumination theory).
Section 4.5 Review Questions
- How do twin studies explain the biological causes of mood disorders?
- What brain structures are implicated in the development of mood disorders? Discuss their role.
- What is learned helplessness? How has this concept been used to study the development and maintenance of mood disorders?
- What is the cognitive triad?
- What are common cognitive distortions observed in individuals with mood disorders?
- What are the identified theories that are used to explain the gender differences in mood disorder development?
4.6. Treatment of Mood Disorders
- Describe treatment options for depressive disorders.
- Describe treatment options for bipolar disorders.
- Determine the efficacy of treatment options for depressive disorders.
- Determine the efficacy of treatment options for bipolar disorders.
4.6.1. Depressive Disorders
Given that Major Depressive Disorder is among the most frequent and debilitating psychiatric disorders, it should not be surprising that the research on this disorder is quite extensive. Among its treatment options, the most efficacious ones include antidepressant medications, Cognitive-Behavioral Therapy (CBT; Beck et al., 1979), Behavioral Activation (BA; Jacobson et al., 2001), and Interpersonal Therapy (IPT; Klerman et al., 1984). Although CBT is the most widely known and used treatment for Major Depressive Disorder, there is minimal evidence to support one treatment modality over the other; treatment is generally dictated by therapist competence, availability, and patient preference (Craighhead & Dunlop, 2014).
4.6.1.1. Psychopharmacology – Antidepressant medications. Antidepressants are often the most common first-line attempt at treatment for MDD for a few reasons. Oftentimes an individual will present with symptoms to their primary caregiver (a medical doctor) who will prescribe them some line of antidepressant medication. Medication is often seen as an “easier” treatment for depression as the individual can take the medication at their home, rather than attending weekly therapy sessions; however, this also leaves room for adherence issues as a large percentage of individuals fail to take prescription medication as indicated by their physician. Given the biological functions of neurotransmitters and their involvement in maintaining depressive symptoms, it makes sense that this is an effective type of treatment.
Within antidepressant medications, there are a few different classes, each categorized by their structural or functional relationships. It should be noted that no specific antidepressant medication class or medication have been proven to be more effective in treating MDD than others (APA, 2010). In fact, many patients may try several different types of antidepressant medications until they find one that is effective, with minimal side effects.
4.6.1.2. Psychopharmacology – Selective serotonin reuptake inhibitors (SSRIs) . SSRIs are among the most common medications used to treat depression due to their relatively benign side effects. Additionally, the required dose to reach therapeutic levels is low compared to the other medication options. Possible side effects from SSRIs include but are not limited to nausea, insomnia, and reduced sex drive.
SSRIs improve depression symptoms by blocking the reuptake of norepinephrine and/or serotonin in presynaptic neurons, thus allowing more of these neurotransmitters to be available for postsynaptic neurons. While this is the general mechanism through which all SSRI’s work, there are minor biological differences among different types of medications within the SSRI family. These small differences are beneficial to patients in that there are a few treatment options to maximize medication benefits and minimize side effects.
4.6.1.3. Psychopharmacology – Tricyclic antidepressants. Although originally developed to treat schizophrenia, tricyclic antidepressants were adapted to treat depression after failing to manage symptoms of schizophrenia (Kuhn, 1958). The term tricyclic came from the molecular shape of the structure: three rings.
Tricyclic antidepressants are like SSRIs in that they work by affecting brain chemistry, altering the number of neurotransmitters available for neurons. More specifically, they block the absorption or reuptake of serotonin and norepinephrine, thus increasing their availability for postsynaptic neurons. While effective, tricyclic antidepressants have been increasingly replaced by SSRIs due to their reduced side effects. However, tricyclic antidepressants have been shown to be more effective in treating depressive symptoms in individuals who have not been able to achieve symptom reduction via other pharmacological approaches.
While the majority of the side effects are minimal – dry mouth, blurry vision, constipation, others can be serious such as sexual dysfunction, tachycardia, cognitive and/or memory impairment. Due to the potential impact on the heart, tricyclic antidepressants should not be used in cardiac patients as they may exacerbate cardiac arrhythmias (Roose & Spatz, 1999).
4.6.1.4. Psychopharmacology – Monoamine oxidase inhibitors (MAOIs). The use of MAOIs as a treatment for depression began serendipitously as patients in the early 1950s reported reduced depression symptoms while on the medication to treat tuberculosis. Research studies confirmed that MAOIs were effective in treating depression in adults outside the treatment of tuberculosis. Although still prescribed, they are not typically first-line medications due to their safety concerns with hypertensive crises. Because of this, individuals on MAOIs have strict diet restrictions to reduce their risk of hypertensive crises (Shulman, Herrman & Walker, 2013).
How do MAOIs work? In basic terms, monoamine oxidase is released in the brain to remove excess neurotransmitters norepinephrine, serotonin, and dopamine. MAOIs essentially prevent the monoamine oxidase (hence the name monoamine oxidase inhibitors ) from removing these neurotransmitters, thus resulting in an increase in these brain chemicals (Shulman, Herman & Walker, 2013). As previously discussed, norepinephrine, serotonin, and dopamine are all involved in the biological mechanisms of maintaining depressive symptoms.
While these drugs are effective, they come with serious side effects. In addition to the hypertensive episodes, they can also cause nausea, headaches, drowsiness, involuntary muscle jerks, reduced sexual desire, weight gain, etc. (APA, 2010). Despite these side effects, studies have shown that individuals prescribed MAOIs for depression have a treatment response rate of 50-70% (Krishnan, 2007). Overall, despite their effectiveness, MAOIs are likely the best treatment for late-stage, treatment-resistant depression patients who have exhausted other treatment options (Krishnan, 2007).
It should be noted that occasionally, antipsychotic medications are used for individuals with MDD; however, these are limited to individuals presenting with psychotic features.
4.6.1.5. Psychotherapy – Cognitive behavioral therapy (CBT). CBT was founded by Aaron Beck in the 1960s and is a widely practiced therapeutic tool used to treat depression (and other disorders as well). The basics of CBT involve what Beck called the cognitive triangle — cognitions (thoughts), behaviors, and emotions. Beck believed that these three components are interconnected, and therefore, affect one another. It is believed that CBT can improve emotions in depressed patients by changing both cognitions (thoughts) and behaviors, which in return enhances mood. Common cognitive interventions with CBT include thought monitoring and recording, identifying cognitive errors, examining evidence supporting/negating cognitions, and creating rational alternatives to maladaptive thought patterns. Behavioral interventions of CBT include activity planning, pleasant event scheduling, task assignments, and coping-skills training.
CBT generally follows four phases of treatment:
- Phase 1: Increasing pleasurable activities. Similar to behavioral activation (see below), the clinician encourages the patient to identify and engage in activities that are pleasurable to the individual. The clinician can help the patient to select the activity, as well as help them plan when they will engage in that activity.
- Phase 2: Challenging automatic thoughts. During this stage, the clinician provides psychoeducation about the negative automatic thoughts that can maintain depressive symptoms. The patient will learn to identify these thoughts on their own during the week and maintain a thought journal of these cognitions to review with the clinician in session.
- Phase 3: Identifying negative thoughts. Once the individual is consistently able to identify these negative thoughts on a daily basis, the clinician can help the patient identify how these thoughts are maintaining their depressive symptoms. It is at this point that the patient begins to have direct insight as to how their cognitions contribute to their disorder.
- Phase 4: Changing thoughts. The final stage of treatment involves challenging the negative thoughts the patient has been identifying in the last two phases of treatment and replacing them with positive thoughts.
4.6.1.6. Psychotherapy – Behavioral activation (BA). BA is similar to the behavioral component of CBT in that the goal of treatment is to alleviate depression and prevent future relapse by changing an individual’s behavior. Founded by Ferster (1973), as well as Lewinsohn and colleagues (Lewinsohn, 1974; Lewinsohn, Biglan, & Zeiss, 1976), the goal of BA is to increase the frequency of behaviors so that individuals have opportunities to experience greater contact with sources of reward in their lives. To do this, the clinician assists the patient by developing a list of pleasurable activities that they can engage in outside of treatment (i.e., going for a walk, going shopping, having dinner with a friend). Additionally, the clinician assists the patient in identifying their negative behaviors—crying, sleeping in, avoiding friends—and monitoring them so that they do not impact the outcome of their pleasurable activities. Finally, the clinician works with the patient on effective social skills. By minimizing negative behaviors and maximizing pleasurable activities, the individual will receive more positive reward and reinforcement from others and their environment, thus improving their overall mood.
4.6.1.7. Psychotherapy – Interpersonal therapy (IPT). IPT was developed by Klerman, Weissman, and colleagues in the 1970s as a treatment arm for a pharmacotherapy study of depression (Weissman, 1995). The treatment was created based on data from post-World War II individuals who expressed a substantial impact on their psychosocial life events. Klerman and colleagues noticed a significant relationship between the development of depression and complicated bereavement, role disputes, role transitions, and interpersonal deficits in these individuals (Weissman, 1995). The idea behind IPT is that depressive episodes compromise interpersonal functioning, which makes it difficult to manage stressful life events. The basic mechanism of IPT is to establish effective strategies to manage interpersonal issues, which in return, will ameliorate depressive symptoms.
There are two main principles of IPT. First, depression is a common medical illness with a complex and multi-determined etiology. Since depression is a medical illness, it is also treatable and not the patient’s fault. Second, depression is connected to a current or recent life event. The goal of IPT is to identify the interpersonal problem that is related to the depressive symptoms and solve this crisis so the patient can improve their life situation while relieving depressive symptoms.
4.6.1.8. Multimodal treatment. While both pharmacological and psychological treatment alone is very effective in treating depression, a combination of the two treatments may offer additional benefits, particularly in the maintenance of wellness. Additionally, multimodal treatment options may be helpful for individuals who have not achieved wellness in a single modality.
Multimodal treatments can be offered in three different ways: concurrently, sequentially, or within a stepped manner (McGorry et al., 2010). With a stepped manner treatment, pharmacological therapy is often used initially to treat depressive symptoms. Once the patient reports some relief in symptoms, the psychosocial treatment is added to address the remaining symptoms. While all three methods are effective in managing depressive symptoms, matching patients to their treatment preferences may produce better outcomes than clinician-driven treatment decisions.
4.6.2. Bipolar Disorder
4.6.2.1. Psychopharmacology. Unlike treatment for MDD, there is some controversy regarding effective treatment of bipolar disorder. One suggestion is to treat bipolar disorder aggressively with mood stabilizers such as Lithium or Depakote as these medications do not induce pharmacological mania/hypomania. These mood stabilizers are occasionally combined with antidepressants later in treatment only if absolutely necessary (Ghaemi, Hsu, Soldani & Goodwin, 2003). Research has shown that mood stabilizers are less potent in treating depressive symptoms, and therefore, the combination approach is believed to help manage both the manic and depressive episodes (Nivoli et al., 2011).
The other treatment option is to forgo the mood stabilizer and treat symptoms with newer antidepressants early in treatment. Unfortunately, large scale research studies have not shown great support for this method (Gijsman, Geddes, Rendell, Nolen, & Goodwin, 2004; Moller, Grunze & Broich, 2006). Antidepressants often trigger a manic or hypomanic episode in bipolar patients. Because of this, the first-line treatment option for bipolar disorder is mood stabilizers, particularly Lithium.
4.6.2.2. Psychological treatment. Although psychopharmacology is the first and most widely used treatment for bipolar disorders, occasionally psychological interventions are also paired with medication as psychotherapy alone is not a sufficient treatment option. Majority of psychological interventions are aimed at medication adherence, as many bipolar patients stop taking their mood stabilizers when they “feel better” (Advokat et al., 2014). Social skills training and problem-solving skills are also helpful techniques to address in the therapeutic setting as individuals with bipolar disorder often struggle in this area.
4.6.3. Outcome of Treatment
4.6.3.1. Depressive treatment. As we have discussed, major depressive disorder has a variety of treatment options, all found to be efficacious. However, research suggests that while psychopharmacological interventions are more effective in rapidly reducing symptoms, psychotherapy, or even a combined treatment approach, are more effective in establishing long-term relief of symptoms.
Rates of relapse for major depressive disorder are often associated with individuals whose onset was at a younger age (particularly adolescents), those who have already experienced multiple major depressive episodes, and those with more severe symptomology, especially those presenting with severe suicidal ideation and psychotic features (APA, 2022).
4.6.3.2. Bipolar treatment. Lithium and other mood stabilizers are very effective in managing symptoms of patients with bipolar disorder. Unfortunately, it is the adherence to the medication regimen that is often the issue with these patients. Bipolar patients often desire the euphoric highs that are associated with manic and hypomanic episodes, leading them to forgo their medication. A combination of psychopharmacology and psychotherapy aimed at increasing the rate of adherence to medical treatment may be the most effective treatment option for bipolar I and II disorder.
- Treatment of depressive disorders include psychopharmacological options such as anti-depressant mediations, SSRIs, tricyclic antidepressants, and MAOIs and/or psychotherapy options to include CBT, behavioral activation (BA), and interpersonal therapy (IPT). A combination of the two main approaches often works best, especially in relation to maintenance of wellness.
- Treatment of bipolar disorder involves mood stabilizers such as Lithium and psychological interventions with the goal of medication adherence, as well as social skills training and problem-solving skills.
- Regarding depression, psychopharmacological interventions are more effective in rapidly reducing symptoms, while psychotherapy, or even a combined treatment approach, is more effective in establishing long-term relief of symptoms.
- A combination of psychopharmacology and psychotherapy aimed at increasing the rate of adherence to medical treatment may be the most effective treatment option for bipolar I and II disorder.
Section 4.6 Review Questions
- Discuss the effectiveness of the different pharmacological treatments for mood disorders.
- What are the four phases of CBT? How do they address symptoms of mood disorder?
- What is ITP and what are its main treatment strategies?
- What are the effective treatment options for bipolar disorder?
Module Recap
That concludes our discussion of mood disorders. You should now have a good understanding of the two major types of mood disorders – depressive and bipolar disorders. Be sure you are clear on what makes them different from one another in terms of their clinical presentation, epidemiology, comorbidity, and etiology. This will help you with understanding treatment options and their efficacy.
3rd edition

Share This Book
- Increase Font Size
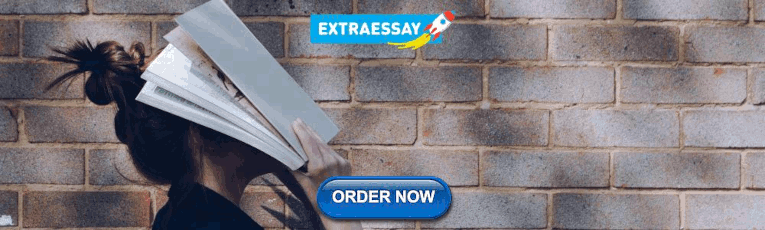
IMAGES
VIDEO
COMMENTS
1. There is a high comorbidity rate within the anxiety-related disorders. What other disorders commonly occur with specific anxiety related disorders? 2. What anxiety-related disorder has a high comorbidity with medical symptoms? 7.4 Etiology Section 7.4 Learning Objectives • Describe the biological causes of anxiety disorders.
A panic attack is defined as a sudden or abrupt surge of fear or impending doom along with at least four physical or cognitive symptoms (ex. sweating, chest pain, nausea, chills, trembling, etc.) 7.2. Epidemiology. 7.2. Epidemiology. Prevalence of specific phobias. 7-9% in the US. 2:1 ratio of females to males.
Generalized anxiety disorder is characterized by an underlying excessive anxiety and worry related to a wide range of events or activities and lasting for more days than not for at least six months. While many individuals experience some degree of worry throughout the day, individuals with generalized anxiety disorder experience worry of ...
Anxiety, on the other hand, is the anticipation of a future threat leading to, "…muscle tension and vigilance in preparation for future danger and cautious or avoidant behaviors" (APA, 2022, pg. 215). The anxiety disorders differ from one another in the types of objects or situations that lead to fear, anxiety, or avoidance behavior.
7.1 - Clinical Presentation 7.2 - Epidemiology 7.3 - Comorbidity 7.4 - Etiology 7.5 - Treatment Learn with flashcards, games, and more — for free.
Module 7: Anxiety Disorders 7.1. Clinical Presentation. The hallmark symptoms of anxiety-related disorders are excessive fear and anxiety and related behavioral disturbances. The DSM says that fear is an emotional response to a real or perceived imminent threat which leads to "…surges of autonomic arousal necessary for flight or flight, thoughts of immediate danger, and escape behaviors ...
In Chapter 4, we will discuss matters related to anxiety disorders including their clinical presentation, epidemiology, comorbidity, treatment options, and etiology. Our discussion will include Panic Disorder, Generalized Anxiety Disorder, Specific Phobias, Social Anxiety Disorder, and Agoraphobia. Be sure you refer to Chapters 1-3 for ...
The findings revealed a 19% concurrent comorbidity between these disorders, and in 65% of the cases, social phobia preceded major depressive disorder by at least 2 years. In addition, initial presentation with social phobia was associated with a 5.7-fold increased risk of developing major depressive disorder. These associations between anxiety ...
View Psych 12.1-2.docx from PSY 1101 at Sinclair Community College. 1. Based on the descriptions in the NOBA reading assignment on Anxiety and Related Disorders, list the main symptoms of each of the
A taxometric investigation of the latent structure of social anxiety disorder in outpatients with anxiety and mood disorders. Depression and Anxiety , 23 , 190 - 199 . doi: 10.1002/da.20158 Google Scholar
The aims of the current systematic review are to provide an up‐to‐date list of studies that have examined comorbidity between broadly defined mood‐ and anxiety‐related disorders and to meta‐analyze the risk estimates according to key design features related to the types of prevalence estimates for the two disorders. 2.1.
This chapter examines the anxiety disorders commonly encountered in elite sport, namely generalized anxiety disorder (GAD), panic disorder and social anxiety disorder, as well as the related conditions of obsessive-compulsive disorder (OCD) and performance anxiety. The risk factors for these symptoms or disorders in elite athletes are examined ...
15.1 What Are Psychological Disorders? 15.2 Diagnosing and Classifying Psychological Disorders; 15.3 Perspectives on Psychological Disorders; 15.4 Anxiety Disorders; 15.5 Obsessive-Compulsive and Related Disorders; 15.6 Posttraumatic Stress Disorder; 15.7 Mood Disorders; 15.8 Schizophrenia; 15.9 Dissociative Disorders; 15.10 Personality ...
Describes how anxiety disorders present. Describes the epidemiology, etiology, and treatment of anxiety disorders. Describes comorbidity in relation to an…
Dr. Foster thinks that the dots are, in fact, linearly related, so Pearson's r r is appropriate. Figure 14.7.2 14.7. 2: Scatterplot of Anxiety and Depression (CC-BY-NC-SA Foster et al. from An Introduction to Psychological Statistics) The data we gather from our participants is shown in Table 14.7.1 14.7. 1.
b. Intense anxiety. c. Avoidance of places that could cause physical sensations. d. Agoraphobia. Based on the descriptions in the NOBA reading assignment on Anxiety and Related Disorders, list the main symptoms of Post-traumatic Stress Disorder. a. Flashbacks. b. Numb emotionally. c. Hypervigilant to surroundings. d. Jumpy. e. Quick to anger. f.
About 8 of every 100 women (or 8%) develop PTSD sometime in their lives compared with about 4 of every 100 men (or 4%). Women are 2x more likely to develop PTSD than men, and children can also develop PTSD. Children and Teens: 15% to 43% of girls and 14% to 43% of boys go through at least one trauma.
Then create a simple "case study" about an anxiety disorder and share reflections from the Ted talk. Discussion: Anxiety Disorders Infographic Assignment. Learn about what OER is, and create a short infographic/flyer about one of the anxiety disorders. Module 5: Obsessive Compulsive Disorder and Stressor Related Disorders: Discussion: OCD ...
Anxiety and stress-related disorders are among the most common mental disorders, with a regional variation in prevalence globally (i.e., from 5.3% to 10.4%) 1 and a pooled lifetime prevalence of ...
Characterized by a pattern of stable traits which are inflexible, pervasive, and lead to significant distress or impairment. Paraphilic Disorders. Characterized by recurrent and intense sexual fantasies that can cause harm to the individual or others; includes exhibitionism, voyeurism, and sexual sadism. 3.2.3.
View Assignment 7 Feeding Disorders Case Paper.docx from NURS 663 at Maryville University. 1 Assignment 7.1: Feeding Disorders Case Paper Rebecca J. Houston Maryville University Nurs 663: ... There are also concerns of anxiety with descriptions of Jill being more anxious ... Assessment questions about Jill having any prior Pica related to her ...
3rd edition as of July 2023. Module Overview. In Module 4, we will discuss matters related to mood disorders to include their clinical presentation, epidemiology, comorbidity, etiology, and treatment options. Our discussion will cover major depressive disorder, persistent depressive disorder (formerly Dysthymia), bipolar I disorder, bipolar II ...