MINI REVIEW article
Solving the credit assignment problem with the prefrontal cortex.
- Department of Psychology, University of California, Los Angeles, Los Angeles, CA, United States
In naturalistic multi-cue and multi-step learning tasks, where outcomes of behavior are delayed in time, discovering which choices are responsible for rewards can present a challenge, known as the credit assignment problem . In this review, I summarize recent work that highlighted a critical role for the prefrontal cortex (PFC) in assigning credit where it is due in tasks where only a few of the multitude of cues or choices are relevant to the final outcome of behavior. Collectively, these investigations have provided compelling support for specialized roles of the orbitofrontal (OFC), anterior cingulate (ACC), and dorsolateral prefrontal (dlPFC) cortices in contingent learning. However, recent work has similarly revealed shared contributions and emphasized rich and heterogeneous response properties of neurons in these brain regions. Such functional overlap is not surprising given the complexity of reciprocal projections spanning the PFC. In the concluding section, I overview the evidence suggesting that the OFC, ACC and dlPFC communicate extensively, sharing the information about presented options, executed decisions and received rewards, which enables them to assign credit for outcomes to choices on which they are contingent. This account suggests that lesion or inactivation/inhibition experiments targeting a localized PFC subregion will be insufficient to gain a fine-grained understanding of credit assignment during learning and instead poses refined questions for future research, shifting the focus from focal manipulations to experimental techniques targeting cortico-cortical projections.
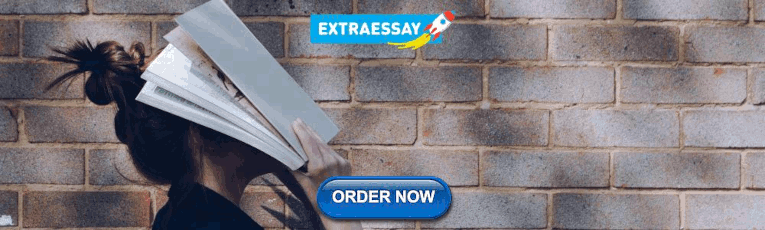
Introduction
When an animal is introduced to an unfamiliar environment, it will explore the surroundings randomly until an unexpected reward is encountered. Reinforced by this experience, the animal will gradually learn to repeat those actions that produced the desired outcome. The work conducted in the past several decades has contributed a detailed understanding of the psychological and neural mechanisms that support such reinforcement-driven learning ( Schultz and Dickinson, 2000 ; Schultz, 2004 ; Niv, 2009 ). It is now broadly accepted that dopamine (DA) signaling conveys prediction errors, or the degree of surprise brought about by unexpected rewards, and interacts with cortical and basal ganglia circuits to selectively reinforce the advantageous choices ( Schultz, 1998a , b ; Schultz and Dickinson, 2000 ; Niv, 2009 ). Yet, in naturalistic settings, where rewards are delayed in time, and where multiple cues are encountered, or where several decisions are made before the outcomes of behavior are revealed, discovering which choices are responsible for rewards can present a challenge, known as the credit assignment problem ( Mackintosh, 1975 ; Rothkopf and Ballard, 2010 ).
In most everyday situations, the rewards are not immediate consequences of behavior, but instead appear after substantial delays. To influence future choices, the teaching signal conveyed by DA release needs to reinforce synaptic events occurring on a millisecond timescale, frequently seconds before the outcomes of decisions are revealed ( Izhikevich, 2007 ; Fisher et al., 2017 ). This apparent difficulty in linking preceding behaviors caused by transient neuronal activity to a delayed feedback has been termed the distal reward or temporal credit assignment problem ( Hull, 1943 ; Barto et al., 1983 ; Sutton and Barto, 1998 ; Dayan and Abbott, 2001 ; Wörgötter and Porr, 2005 ). Credit for the reward delayed by several seconds can frequently be assigned by establishing an eligibility trace, a molecular memory of the recent neuronal activity, allowing modification of synaptic connections that participated in the behavior ( Pan et al., 2005 ; Fisher et al., 2017 ). On longer timescales, or when multiple actions need to be performed sequentially to reach a final goal, intermediate steps themselves can acquire motivational significance and subsequently reinforce preceding decisions, such as in temporal-difference (TD) learning models ( Sutton and Barto, 1998 ).
Several excellent reviews have summarized the accumulated knowledge on mechanisms that link choices and their outcomes through time, highlighting the advantages of eligibility traces and TD models ( Wörgötter and Porr, 2005 ; Barto, 2007 ; Niv, 2009 ; Walsh and Anderson, 2014 ). Yet these solutions to the distal reward problem can impede learning in multi-choice tasks, or when an animal is presented with many irrelevant stimuli prior to or during the delay. Here, I only briefly overview the work on the distal reward problem to highlight potential complications that can arise in credit assignment based on eligibility traces when learning in multi-cue environments. Instead, I focus on the structural (or spatial ) credit assignment problem, requiring animals to select and learn about the most meaningful features in the environment and ignore irrelevant distractors. Collectively, the reviewed evidence highlights a critical role for the prefrontal cortex (PFC) in such contingent learning.
Recent studies have provided compelling support for specialized functions of the orbitofrontal (OFC) and dorsolateral prefrontal (dlPFC) cortices in credit assignment in multi-cue tasks, with fewer experiments targeting the anterior cingulate cortex (ACC). For example, it has seen suggested that the dlPFC aids reinforcement-driven learning by directing attention to task-relevant cues ( Niv et al., 2015 ), the OFC assigns credit for rewards based on the causal relationship between trial outcomes and choices ( Jocham et al., 2016 ; Noonan et al., 2017 ), whereas the ACC contributes to unlearning of action-outcome associations when the rewards are available for free ( Jackson et al., 2016 ). However, this work has similarly revealed shared contributions and emphasized rich and heterogeneous response properties of neurons in the PFC, with different subregions monitoring and integrating the information about the task (i.e., current context, available options, anticipated rewards, as well as delay and effort costs) at variable times within a trial (upon stimulus presentation, action selection, outcome anticipation, and feedback monitoring; ex., Hunt et al., 2015 ; Khamassi et al., 2015 ). In the concluding section, I overview the evidence suggesting that contingent learning in multi-cue environments relies on dynamic cortico-cortical interactions during decision making and outcome valuation.
Solving the Temporal Credit Assignment Problem
When outcomes follow choices after short delays (Figure 1A ), the credit for distal rewards can frequently be assigned by establishing an eligibility trace, a sustained memory of the recent activity that renders synaptic connections malleable to modification over several seconds. Eligibility traces can persist as elevated levels of calcium in dendritic spines of post-synaptic neurons ( Kötter and Wickens, 1995 ) or as a sustained neuronal activity throughout the delay period ( Curtis and Lee, 2010 ) to allow for synaptic changes in response to reward signals. Furthermore, spike-timing dependent plasticity can be influenced by neuromodulator input ( Izhikevich, 2007 ; Abraham, 2008 ; Fisher et al., 2017 ). For example, the magnitude of short-term plasticity can be modulated by DA, acetylcholine and noradrenaline, which may even revert the sign of the synaptic change ( Matsuda et al., 2006 ; Izhikevich, 2007 ; Seol et al., 2007 ; Abraham, 2008 ; Zhang et al., 2009 ). Sustained neural activity has been observed in the PFC and striatum ( Jog et al., 1999 ; Pasupathy and Miller, 2005 ; Histed et al., 2009 ; Kim et al., 2009 , 2013 ; Seo et al., 2012 ; Her et al., 2016 ), as well as the sensory cortices after experience with consistent pairings between the stimuli and outcomes separated by predictable delays ( Shuler and Bear, 2006 ).
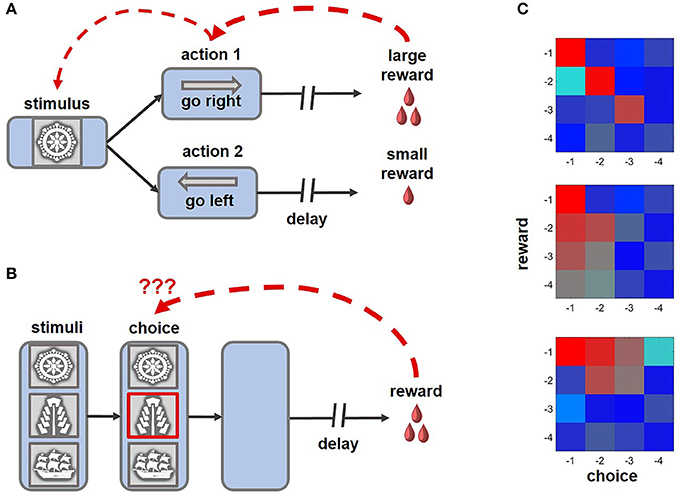
Figure 1 . Example tasks highlighting the challenge of credit assignment and learning strategies enabling animals to solve this problem. (A) An example of a distal reward task that can be successfully learned with eligibility traces and TD rules, where intermediate choices can acquire motivational significance and subsequently reinforce preceding decisions (ex., Pasupathy and Miller, 2005 ; Histed et al., 2009 ). (B) In this version of the task, multiple cues are present at the time of choice, only one of which is meaningful for obtaining rewards. After a brief presentation, the stimuli disappear, requiring an animal to solve a complex structural and temporal credit assignment problem (ex., Noonan et al., 2010 , 2017 ; Niv et al., 2015 ; Asaad et al., 2017 ; while the schematic of the task captures the challenge of credit assignment, note that in some experimental variants of the behavioral paradigm stimuli disappeared before an animal revealed its choice, whereas in others the cues remained on the screen until the trial outcome was revealed). Under such conditions, learning based on eligibility traces is suboptimal, as non-specific reward signals can reinforce visual cues that did not meaningfully contribute, but occurred close, to beneficial outcomes of behavior. (C) On reward tasks, similar to the one shown in (B) , the impact of previous decisions and associated rewards on current behavior can be assessed by performing regression analyses ( Jocham et al., 2016 ; Noonan et al., 2017 ). Here, the color of each cell in a matrix represents the magnitude of the effect of short-term choice and outcome histories, up to 4 trials into the past (red-strong influence; blue-weak influence on the current decision). Top: an animal learning based on the causal relationship between outcomes and choices (i.e., contingent learning). Middle: each choice is reinforced by a combined history of rewards (i.e., decisions are repeated if beneficial outcomes occur frequently). Bottom: the influence of recent rewards spreads to unrelated choices.
On extended timescales, when multiple actions need to be performed sequentially to reach a final goal, the distal reward problem can be solved by assigning motivational significance to intermediate choices that can subsequently reinforce preceding decisions, such as in TD learning models ( Montague et al., 1996 ; Sutton and Barto, 1998 ; Barto, 2007 ). Assigning values to these intervening steps according to expected future rewards allows to break complex temporal credit assignment problems into smaller and easier tasks. There is ample evidence for TD learning in humans and other animals that on the neural level is supported by transfer of DA responses from the time of reward delivery to preceding cues and actions ( Montague et al., 1996 ; Schultz, 1998a , b ; Walsh and Anderson, 2014 ).
Both TD learning and eligibility traces offer elegant solutions to the distal reward problem, and models based on cooperation between these two mechanisms can predict animal behavior as well as neuronal responses to rewards and predictive stimuli ( Pan et al., 2005 ; Bogacz et al., 2007 ). Yet assigning credit based on eligibility traces can be suboptimal when an animal interacts with many irrelevant stimuli prior to or during the delay (Figure 1B ). Under such conditions sensory areas remain responsive to distracting stimuli and the arrival of non-specific reward signals can reinforce intervening cues that did not meaningfully contribute, but occurred close, to the outcome of behavior ( FitzGerald et al., 2013 ; Xu, 2017 ).
The Role of the PFC in Structural Credit Assignment
Several recent studies have investigated the neural mechanisms of appropriate credit assignment in challenging tasks where only a few of the multitude of cues predict rewards reliably. Collectively, this work has provided compelling support for causal contributions of the PFC to structural credit assignment. For example, Asaad et al. (2017) examined the activity of neurons in monkey dlPFC while subjects were performing a delayed learning task. The arrangement of the stimuli varied randomly between trials and within each block either the spatial location or stimulus identity was relevant for solving the task. The monkeys' goal was to learn by trial-and-error to select one of the four options that led to rewards according to current rules. When stimulus identity was relevant for solving the task, neural activity in the dlPFC at the time of feedback reflected both the relevant cue (regardless of its spatial location) and the trial outcome, thus integrating the information necessary for credit assignment. Such responses were strategy-selective: these neurons did not encode cue identity at the time of feedback when it was not necessary for learning in the spatial location task, in which making a saccade to the same position on the screen was reinforced within a block of trials. Previous research has similarly indicated that neurons in the dlPFC respond selectively to behaviorally-relevant and attended stimuli ( Lebedev et al., 2004 ; Markowitz et al., 2015 ) and integrate information about prediction errors, choice values as well as outcome uncertainty prior to trial feedback ( Khamassi et al., 2015 ).
The activity within the dlPFC has been linked to structural credit assignment through selective attention and representational learning ( Niv et al., 2015 ). Under conditions of reward uncertainty and unknown relevant task features, human participants opt for computational efficiency and engage in a serial-hypothesis-testing strategy ( Wilson and Niv, 2011 ), selecting one cue and its anticipated outcome as the main focus of their behavior, and updating the expectations associated exclusively with that choice upon feedback receipt ( Akaishi et al., 2016 ). Niv and colleagues tested participant on a three-armed bandit task, where relevant stimulus dimensions (i.e., shape, color or texture) predicting outcome probabilities changed between block of trials ( Niv et al., 2015 ). In such multidimensional environment, reinforcement-driven learning was aided by attentional control mechanisms that engaged the dlPFC, intraparietal cortex, and precuneus.
In many tasks, the credit for outcomes can be assigned according to different rules: based on the causal relationship between rewards and choices (i.e., contingent learning), their temporal proximity (i.e., when the reward is received shortly after a response), or their statistical relationship (when an action has been executed frequently before beneficial outcomes; Jocham et al., 2016 ; Figure 1C ). The analyses presented in papers discussed above did not allow for the dissociation between these alternative strategies of credit assignment. By testing human participants on a task with continuous stimulus presentation, instead of a typical trial-by-trial structure, Jocham et al. (2016) demonstrated that the tendency to repeat choices that were immediately followed by rewards and causal learning operate in parallel. In this experiment, activity within another subregion of the PFC, the OFC, was associated with contingent learning. Complementary work in monkeys revealed that the OFC contributes causally to credit assignment ( Noonan et al., 2010 ): animals with OFC lesions were unable to associate a reward with the choice on which it was contingent and instead relied on temporal and statistical learning rules. In another recent paper, Noonan and colleagues (2017) extended these observations to humans, demonstrating causal contributions of the OFC to credit assignment across species. The participants were tested on a three-choice probabilistic learning task. The three options were presented simultaneously and maintained on the screen until the outcome of a decision was revealed, thus requiring participants to ignore irrelevant distractors. Notably, only patients with lateral OFC lesions displayed any difficulty in learning the task, whereas damage to the medial OFC or dorsomedial PFC preserved contingent learning mechanisms. However, it is presently unknown whether lesions to the dlPFC or ACC affect such causal learning.
In another test of credit assignment in learning, contingency degradation, the subjects are required to track causal relationships between the stimuli or actions and rewards. During contingency degradation sessions, the animals are still reinforced for responses, but rewards are also available for free. After experiencing non-contingent rewards, control subjects reliably decrease their choices of the stimuli. However, lesions to both the ACC and OFC inhibit contingency degradation ( Jackson et al., 2016 ). Taken together, these observations demonstrate causal contributions of the PFC to appropriate credit assignment in multi-cue environments.
Cooperation Between PFC Subregions Supports Contingent Learning in Multi-Cue Tasks
Despite the segregation of temporal and structural aspects of credit assignment in earlier sections of this review, in naturalistic settings the brains frequently need to tackle both problems simultaneously. Here, I overview the evidence favoring a network perspective, suggesting that dynamic cortico-cortical interactions during decision making and outcome valuation enable adaptive solutions to complex spatio-temporal credit assignment problems. It has been previously suggested that feedback projections from cortical areas occupying higher levels of processing hierarchy, including the PFC, can aid in attribution of outcomes to individual decisions by implementing attention-gated reinforcement learning ( Roelfsema and van Ooyen, 2005 ). Similarly, recent theoretical work has shown that even complex multi-cue and multi-step problems can be solved by an extended cascade model of synaptic memory traces, in which the plasticity is modulated not only by the activity within a population of neurons, but also by feedback about executed decisions and resulting rewards ( Urbanczik and Senn, 2009 ; Friedrich et al., 2010 , 2011 ). Contingent learning, according to these models, can be supported by the communication between neurons encoding available options, committed choices and outcomes of behavior during decision making and feedback monitoring. For example, at the time of outcome valuation, information about recent choices can be maintained as a memory trace in the neuronal population involved in action selection or conveyed by an efference copy from an interconnected brain region ( Curtis and Lee, 2010 ; Khamassi et al., 2011 , 2015 ). Similarly, reinforcement feedback is likely communicated as a global reward signal (ex., DA release) as well as projections from neural populations engaged in performance monitoring, such as those within the ACC ( Friedrich et al., 2010 ; Khamassi et al., 2011 ). The complexity of reciprocal and recurrent projections spanning the PFC ( Barbas and Pandya, 1989 ; Felleman and Van Essen, 1991 ; Elston, 2000 ) may enable this network to implement such learning rules, integrating the information about the task, executed decisions and performance feedback.
In many everyday decisions, the options are compared across multiple features simultaneously (ex., by considering current context, needs, available reward types, as well as delay and effort costs). Neurons in different subregions of the PFC exhibit rich response properties, signaling these features of the task at various time epochs within a trial. For example, reward selectivity in response to predictive stimuli emerges earlier in the OFC and may then be passed to the dlPFC that encodes both the expected outcome and the upcoming choice ( Wallis and Miller, 2003 ). Similarly, on trials where options are compared based on delays to rewards, choices are dependent on interactions between the OFC and dlPFC ( Hunt et al., 2015 ). Conversely, when effort costs are more meaningful for decisions, it is the ACC that influences choice-related activity in the dlPFC ( Hunt et al., 2015 ). The OFC is required not only for the evaluation of stimuli, but also more complex abstract rules, based on rewards they predict ( Buckley et al., 2009 ). While both the OFC and dlPFC encode abstract strategies (ex., persisting with recent choices or shifting to a new response), such signals appear earlier in the OFC and may be subsequently conveyed to the dlPFC where they are combined with upcoming response (i.e., left vs. right saccade) encoding ( Tsujimoto et al., 2011 ). Therefore, the OFC may be the first PFC subregion to encode task rules and/or potential rewards predicted by sensory cues; via cortico-cortical projections, this information may be subsequently communicated to the dlPFC or ACC ( Kennerley et al., 2009 ; Hayden and Platt, 2010 ) to drive strategy-sensitive response planning.
The behavioral strategy that the animal follows is influenced by recent reward history ( Cohen et al., 2007 ; Pearson et al., 2009 ). If its choices are reinforced frequently, the animal will make similar decisions in the future (i.e., exploit its current knowledge). Conversely, unexpected omission of expected rewards can signal a need for novel behaviors (i.e., exploration). Neurons in the dlPFC carry representations of planned as well as previous choices, anticipate outcomes, and jointly encode the current decisions and their consequences following feedback ( Seo and Lee, 2007 ; Seo et al., 2007 ; Tsujimoto et al., 2009 ; Asaad et al., 2017 ). Similarly, the ACC tracks trial-by-trial outcomes of decisions ( Procyk et al., 2000 ; Shidara and Richmond, 2002 ; Amiez et al., 2006 ; Quilodran et al., 2008 ) as well as reward and choice history ( Seo and Lee, 2007 ; Kennerley et al., 2009 , 2011 ; Sul et al., 2010 ; Kawai et al., 2015 ) and signals errors in outcome prediction ( Kennerley et al., 2009 , 2011 ; Hayden et al., 2011 ; Monosov, 2017 ). At the time of feedback, neurons in the OFC encode committed choices, their values and contingent rewards ( Tsujimoto et al., 2009 ; Sul et al., 2010 ). Notably, while the OFC encodes the identity of expected outcomes and the value of the chosen option after the alternatives are presented to an animal, it does not appear to encode upcoming decisions ( Tremblay and Schultz, 1999 ; Wallis and Miller, 2003 ; Padoa-Schioppa and Assad, 2006 ; Sul et al., 2010 ; McDannald et al., 2014 ), therefore it might be that feedback projections from the dlPFC or ACC are required for such activity to emerge at the time of reward feedback.
To capture the interactions between PFC subregions in reinforcement-driven learning, Khamassi and colleagues have formulated a computation model in which action values are stored and updated in the ACC and then communicated to the dlPFC that decides which action to trigger ( Khamassi et al., 2011 , 2013 ). This model relies on meta-learning principles ( Doya, 2002 ), flexibly adjusting the exploration-exploitation parameter based on performance history and variability in the environment that are monitored by the ACC. The explore-exploit parameter then influences action-selection mechanisms in the dlPFC, prioritizing choice repetition once the rewarded actions are discovered and encouraging switching between different options when environmental conditions change. In addition to highlighting the dynamic interactions between the dlPFC and ACC in learning, the model similarly offers an elegant solution to the credit assignment problem by restricting value updating only to those actions that were selected on a given trial. This is implemented by requiring the prediction error signals in the ACC to coincide with a motor efference copy sent by the premotor cortex. The model is endorsed with an ability to learn meta-values of novel objects in the environment based on the changes in the average reward that follow the presentation of such stimuli. While the authors proposed that such meta-value learning is implemented by the ACC, it is plausible that the OFC also plays a role in this process based on its contributions to stimulus-outcome and state learning ( Wilson et al., 2014 ; Zsuga et al., 2016 ). Intriguingly, this model could reproduce monkey behavior and neural responses on two tasks: four-choice deterministic and two-choice probabilistic paradigms, entailing a complex spatio-temporal credit assignment problem as the stimuli disappeared from the screen prior to action execution and outcome presentation ( Khamassi et al., 2011 , 2013 , 2015 ). Model-based analyses of neuronal responses further revealed that information about prediction errors, action values and outcome uncertainty is integrated both in the dlPFC and ACC, but at different timepoints: before trial feedback in the dlPFC and after feedback in the ACC ( Khamassi et al., 2015 ).
Collectively, these findings highlight the heterogeneity of responses in each PFC subregion that differ in temporal dynamics within a single trial and suggest that the cooperation between the OFC, ACC and dlPFC may support flexible, strategy- and context-dependent choices. This network perspective further suggests that individual PFC subregions may be less specialized in their functions than previously thought. For example, in primates both the ACC and dlPFC participate in decisions based on action values ( Hunt et al., 2015 ; Khamassi et al., 2015 ). And more recently, it has been demonstrated that the OFC is involved in updating action-outcome values as well ( Fiuzat et al., 2017 ). Analogously, while it has been proposed that the OFC is specialized for stimulus-outcome and ACC for action-outcome learning ( Rudebeck et al., 2008 ), lesions to the ACC have been similarly reported to impair stimulus-based reversal learning ( Chudasama et al., 2013 ), supporting shared contributions of the PFC subregions to adaptive behavior. Indeed, these brain regions communicate extensively, sharing the information about presented options, executed decisions and received rewards (Figure 2 ), which can enable them to assign credit for outcomes to choices on which they are contingent ( Urbanczik and Senn, 2009 ; Friedrich et al., 2010 , 2011 ). Attention-gated learning likely relies on the cooperation between PFC subregions as well: for example, coordinated and synchronized activity between the ACC and dlPFC aids in goal-directed attentional shifting and prioritization of task-relevant information ( Womelsdorf et al., 2014 ; Oemisch et al., 2015 ; Voloh et al., 2015 ).
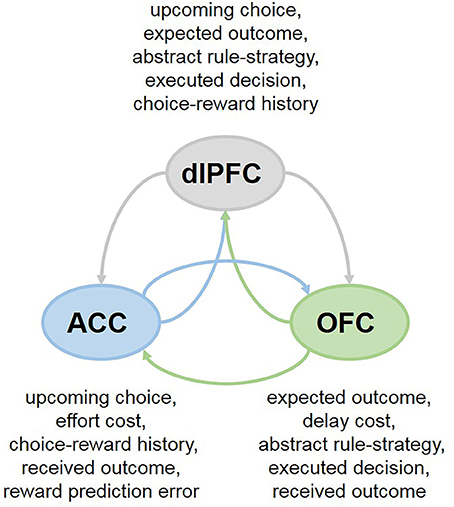
Figure 2 . Cooperation between PFC subregions in multi-cue tasks. In many everyday decisions, the options are compared across multiple features simultaneously (ex., by considering current context, needs, available reward types, as well as delay and effort costs). Neurons in different subregions of the PFC exhibit rich response properties, integrating many aspects of the task at hand. The OFC, ACC and dlPFC communicate extensively, sharing the information about presented options, executed decisions and received rewards, which can enable them to assign credit for outcomes to choices on which they are contingent.
Functional connectivity within the PFC can support contingent learning on shorter timescales (ex., across trials within the same task), when complex rules or stimulus-action-outcome mappings are switching frequently ( Duff et al., 2011 ; Johnson et al., 2016 ). Under such conditions, the same stimuli can carry different meaning depending on task context or due to changes in the environment (ex., serial discrimination-reversal problems) and the PFC neurons with heterogeneous response properties may be better targets for modification, allowing the brain to exert flexible, rapid and context-sensitive control over behavior ( Asaad et al., 1998 ; Mansouri et al., 2006 ). Indeed, it has been shown that rule and reversal learning induce plasticity in OFC synapses onto the dorsomedial PFC (encompassing the ACC) in rats ( Johnson et al., 2016 ). When motivational significance of reward-predicting cues fluctuates frequently, neuronal responses and synaptic connections within the PFC tend to update more rapidly (i.e., across block of trials) compared to subcortical structures and other cortical regions ( Padoa-Schioppa and Assad, 2008 ; Morrison et al., 2011 ; Xie and Padoa-Schioppa, 2016 ; Fernández-Lamo et al., 2017 ; Saez et al., 2017 ). Similarly, neurons in the PFC promptly adapt their responses to incoming information based on the recent history of inputs ( Freedman et al., 2001 ; Meyers et al., 2012 ; Stokes et al., 2013 ). Critically, changes in the PFC activity closely track behavioral performance ( Mulder et al., 2003 ; Durstewitz et al., 2010 ), and interfering with neural plasticity within this brain area prevents normal responses to contingency degradation ( Swanson et al., 2015 ).
When the circumstances are stable overall and the same cues or actions remain reliable predictors of rewards, long-range connections between the PFC, association and sensory areas can support contingent learning on prolonged timescales. Neurons in the lateral intraparietal area demonstrate larger post-decisional responses and enhanced learning following choices that predict final outcomes of sequential behavior in a multi-step and -cue task ( Gersch et al., 2014 ). Such changes in neuronal activity likely rely on information about task rules conveyed by the PFC directly or via interactions with neuromodulatory systems. These hypotheses could be tested in future work.
In summary, dynamic interactions between subregions of the PFC can support contingent learning in multi-cue environments. Furthermore, via feedback projections, the PFC can guide plasticity in other cortical areas associated with sensory and motor processing ( Cohen et al., 2011 ). This account suggests that lesion experiments targeting a localized PFC subregion will be insufficient to gain fine-grained understanding of credit assignment during learning and instead poses refined questions for future research, shifting the focus from focal manipulations to experimental techniques targeting cortico-cortical projections. To gain novel insights into functional connectivity between PFC subregions, it will be critical to assess neural correlates of contingent learning in the OFC, ACC, and dlPFC simultaneously in the context of the same task. In humans, functional connectivity can be assessed by utilizing coherence, phase synchronization, Granger causality and Bayes network approaches ( Bastos and Schoffelen, 2016 ; Mill et al., 2017 ). Indeed, previous studies have linked individual differences in cortico-striatal functional connectivity to reinforcement-driven learning ( Horga et al., 2015 ; Kaiser et al., 2017 ) and future work could focus on examining cortico-cortical interactions in similar paradigms. To probe causal contributions of projections spanning the PFC, future research may benefit from designing multi-cue tasks for rodents and taking advantage of recently developed techniques (i.e., chemo- and opto-genetic targeting of projection neurons followed by silencing of axonal terminals to achieve pathway-specific inhibition; Deisseroth, 2010 ; Sternson and Roth, 2014 ) that afford increasingly precise manipulations of cortico-cortical connectivity. It should be noted, however, that most experiments to date have probed the contributions of the PFC to credit assignment in primates, and functional specialization across different subregions may be even less pronounced in mice and rats. Finally, as highlighted throughout this review, the recent progress in understanding the neural mechanisms of credit assignment has relied on introduction of more complex tasks, including multi-cue and probabilistic choice paradigms. While such tasks better mimic the naturalistic problems that the brains have evolved to solve, they also produce behavioral patterns that are more difficult to analyze and interpret ( Scholl and Klein-Flügge, 2017 ). As such, computational modeling of the behavior and neuronal activity may prove especially useful in future work on credit assignment.
Author Contributions
The author confirms being the sole contributor of this work and approved it for publication.
This work was supported by UCLA's Division of Life Sciences Recruitment and Retention fund (Izquierdo), as well as the UCLA Distinguished University Fellowship (Stolyarova).
Conflict of Interest Statement
The author declares that the research was conducted in the absence of any commercial or financial relationships that could be construed as a potential conflict of interest.
Acknowledgments
The author thanks her mentor Dr. Alicia Izquierdo for helpful feedback and critiques on the manuscript, and Evan E. Hart, as well as the members of the Center for Brains, Minds and Machines and Lau lab for stimulating conversations on the topic.
Abraham, W. C. (2008). Metaplasticity: tuning synapses and networks for plasticity. Nat. Rev. Neurosci. 9:387 doi: 10.1038/nrn2356
PubMed Abstract | CrossRef Full Text | Google Scholar
Akaishi, R., Kolling, N., Brown, J. W., and Rushworth, M. (2016). Neural mechanisms of credit assignment in a multicue environment. J. Neurosci. 36, 1096–1112. doi: 10.1523/JNEUROSCI.3159-15.2016
Amiez, C., Joseph, J. P., and Procyk, E. (2006). Reward encoding in the monkey anterior cingulate cortex. Cereb. Cortex 16, 1040–1055. doi: 10.1093/cercor/bhj046
Asaad, W. F., Lauro, P. M., Perge, J. A., and Eskandar, E. N. (2017). Prefrontal neurons encode a solution to the credit assignment problem. J. Neurosci. 37, 6995–7007. doi: 10.1523/JNEUROSCI.3311-16.2017.
Asaad, W. F., Rainer, G., and Miller, E. K. (1998). Neural activity in the primate prefrontal cortex during associative learning. Neuron 21, 1399–1407. doi: 10.1016/S0896-6273(00)80658-3
Barbas, H., and Pandya, D. N. (1989). Architecture and intrinsic connections of the prefrontal cortex in the rhesus monkey. J. Comp. Neurol. 286, 353–375 doi: 10.1002/cne.902860306
Barto, A. G. (2007). Temporal difference learning. Scholarpedia J. 2:1604. doi: 10.4249/scholarpedia.1604
CrossRef Full Text | Google Scholar
Barto, A. G., Sutton, R. S., and Anderson, C. W. (1983). “Neuronlike adaptive elements that can solve difficult learning control problems,” in IEEE Transactions on Systems, Man, and Cybernetics, SMC-13 , 834–846
Google Scholar
Bastos, A. M., and Schoffelen, J. M. (2016). A tutorial review of functional connectivity analysis methods and their interpretational pitfalls. Front. Syst. Neurosci . 9:175. doi: 10.3389/fnsys.2015.00175
Bogacz, R., McClure, S. M., Li, J., Cohen, J. D., and Montague, P. R. (2007). Short-term memory traces for action bias in human reinforcement learning. Brain Res. 1153, 111–121. doi: 10.1016/j.brainres.2007.03.057
Buckley, M. J., Mansouri, F. A., Hoda, H., Mahboubi, M., Browning, P. G. F., Kwok, S. C., et al. (2009). Dissociable components of rule-guided behavior depend on distinct medial and prefrontal regions. Science 325, 52–58. doi: 10.1126/science.1172377
Chudasama, Y., Daniels, T. E., Gorrin, D. P., Rhodes, S. E., Rudebeck, P. H., and Murray, E. A. (2013). The role of the anterior cingulate cortex in choices based on reward value and reward contingency. Cereb Cortex 23, 2884–2898. doi: 10.1093/cercor/bhs266
Cohen, J. D., McClure, S. M., and Yu, A. J. (2007). Should I stay or should I go? How the human brain manages the trade-off between exploitation and exploration. Philos. Trans. R. Soc. Lond. Ser. B Biol. Sci. 362, 933–942. doi: 10.1098/rstb.2007.2098
Cohen, M. X., Wilmes, K., and Vijver, I. v. (2011). Cortical electrophysiological network dynamics of feedback learning. Trends Cogn. Sci. 15, 558–566. doi: 10.1016/j.tics.2011.10.004
Curtis, C. E., and Lee, D. (2010). Beyond working memory: the role of persistent activity in decision making. Trends Cogn. Sci. 14, 216–222. doi: 10.1016/j.tics.2010.03.006
Dayan, P., and Abbott, L. F. (2001). Theoretical Neuroscience: Computational and Mathematical Modeling of Neural Systems. Cambridge, MA: MIT Press.
Deisseroth, K. (2010). Optogenetics. Nat. Methods 8, 26–29. doi: 10.1038/nmeth.f.324
Doya, K. (2002). Metalearning and neuromodulation. Neural. Netw. 15, 495–506. doi: 10.1016/S0893-6080(02)00044-8
Duff, A., Sanchez Fibla, M., and Verschure, P. F. M. J. (2011). A biologically based model for the integration of sensory–motor contingencies in rules and plans: a prefrontal cortex based extension of the distributed adaptive control architecture. Brain Res. Bull. 85, 289–304. doi: 10.1016/j.brainresbull.2010.11.008
Durstewitz, D., Vittoz, N. M., Floresco, S. B., and Seamans, J. K. (2010). Abrupt transitions between prefrontal neural ensemble states accompany behavioral transitions during rule learning. Neuron 66, 438–448. doi: 10.1016/j.neuron.2010.03.029
Elston, G. N. (2000). Pyramidal cells of the frontal lobe: all the more spinous to think with. J. Neurosci. 20:RC95. Available online at: http://www.jneurosci.org/content/20/18/RC95.long
PubMed Abstract | Google Scholar
Felleman, D. J., and Van Essen, D. C. (1991). Distributed hierarchical processing in the primate cerebral cortex. Cereb. Cortex 1, 1–47. doi: 10.1093/cercor/1.1.1
Fernández-Lamo, I., Delgado-García, J. M., and Gruart, A. (2017). When and where learning is taking place: multisynaptic changes in strength during different behaviors related to the acquisition of an operant conditioning task by behaving rats. Cereb. Cortex 14, 1–13. doi: 10.1093/cercor/bhx011
CrossRef Full Text
Fisher, S. D., Robertson, P. B., Black, M. J., Redgrave, P., Sagar, M. A., Abraham, W. C., et al. (2017). Reinforcement determines the timing dependence of corticostriatal synaptic plasticity in vivo . Nat. Commun. 8:334. doi: 10.1038/s41467-017-00394-x
FitzGerald, T. H. B., Friston, K. J., and Dolan, R. J. (2013). Characterising reward outcome signals in sensory cortex. NeuroImage 83, 329–334. doi: 10.1016/j.neuroimage.2013.06.061
Fiuzat, E. C., Rhodes, S. E., and Murray, E. A. (2017). The role of orbitofrontal-amygdala interactions in updating action-outcome valuations in macaques. J. Neurosci. 37, 2463–2470. doi: 10.1523/JNEUROSCI.1839-16.2017
Freedman, D. J., Riesenhuber, M., Poggio, T., and Miller, E. K. (2001). Categorical representation of visual stimuli in the primate prefrontal cortex. Science 291, 312–316. doi: 10.1126/science.291.5502.312
Friedrich, J., Urbanczik, R., and Senn, W. (2010). Learning spike-based population codes by reward and population feedback. Neural. Comput. 22, 1698–1717. doi: 10.1162/neco.2010.05-09-1010
Friedrich, J., Urbanczik, R., and Senn, W. (2011). Spatio-temporal credit assignment in neuronal population learning. PLoS Comput. Biol. 7:e1002092. doi: 10.1371/journal.pcbi.1002092
Gersch, T. M., Foley, N. C., Eisenberg, I., and Gottlieb, J. (2014). Neural correlates of temporal credit assignment in the parietal lobe. PloS One , 9:e88725. doi: 10.1371/journal.pone.0088725
Hayden, B. Y., Heilbronner, S. R., Pearson, J. M., and Platt, M. L. (2011). Surprise signals in anterior cingulate cortex: neuronal encoding of unsigned reward prediction errors driving adjustment in behavior. J. Neurosci. 31, 4178–4187. doi: 10.1523/JNEUROSCI.4652-10.2011
Hayden, B. Y., and Platt, M. L. (2010). Neurons in anterior cingulate cortex multiplex information about reward and action. J. Neurosci. 30, 3339–3346. doi: 10.1523/JNEUROSCI.4874-09.2010
Her, E. S., Huh, N., Kim, J., and Jung, M. W. (2016). Neuronal activity in dorsomedial and dorsolateral striatum under the requirement for temporal credit assignment. Sci. Rep. 6:27056. doi: 10.1038/srep27056
Histed, M. H., Pasupathy, A., and Miller, E. K. (2009). Learning substrates in the primate prefrontal cortex and striatum: sustained activity related to successful actions. Neuron 63, 244–253. doi: 10.1016/j.neuron.2009.06.019
Horga, G., Maia, T. V., Marsh, R., Hao, X., Xu, D., Duan, Y., et al. (2015). Changes in corticostriatal connectivity during reinforcement learning in humans. Hum. Brain Mapp. 36, 793–803. doi: 10.1002/hbm.22665
Hull, C. (1943). Principles of Behavior . New York, NY: Appleton-Century-Crofts.
Hunt, L. T., Behrens, T. E. J., Hosokawa, T., Wallis, J. D., and Kennerley, S. W. (2015). Capturing the temporal evolution of choice across prefrontal cortex. eLife 4:e11945. doi: 10.7554/eLife.11945
Izhikevich, E. M. (2007). Solving the distal reward problem through linkage of STDP and dopamine signaling. Cereb. Cortex 17, 2443–2452. doi: 10.1093/cercor/bhl152
Jackson, S. A. W., Horst, N. K., Pears, A., Robbins, T. W., and Roberts, A. C. (2016). Role of the perigenual anterior cingulate and orbitofrontal cortex in contingency learning in the marmoset. Cereb. Cortex 26, 3273–3284. doi: 10.1093/cercor/bhw067
Jocham, G., Brodersen, K. H., Constantinescu, A. O., Kahn, M. C., Ianni, A. M., Walton, M. E., et al. (2016). Reward-guided learning with and without causal attribution. Neuron 90, 177–190. doi: 10.1016/j.neuron.2016.02.018
Jog, M. S., Kubota, Y., Connolly, C. I., Hillegaart, V., and Graybiel, A. M. (1999). Building neural representations of habits. Science 286, 1745–1749. doi: 10.1126/science.286.5445.1745
Johnson, C. M., Peckler, H., Tai, L. H., and Wilbrecht, L. (2016). Rule learning enhances structural plasticity of long-range axons in frontal cortex. Nat. Commun. 7:10785. doi: 10.1038/ncomms10785
Kaiser, R. H., Treadway, M. T., Wooten, D. W., Kumar, P., Goer, F., Murray, L., et al. (2017). Frontostriatal and dopamine markers of individual differences in reinforcement learning: a multi-modal investigation. Cereb. Cortex . doi: 10.1093/cercor/bhx281. [Epub ahead of print].
Kawai, T., Yamada, H., Sato, N., Takada, M., and Matsumoto, M. (2015). Roles of the lateral habenula and anterior cingulate cortex in negative outcome monitoring and behavioral adjustment in nonhuman primates. Neuron 88, 792–804. doi: 10.1016/j.neuron.2015.09.030
Kennerley, S. W., Behrens, T. E. J., and Wallis, J. D. (2011). Double dissociation of value computations in orbitofrontal and anterior cingulate neurons. Nat. Neurosci. 14, 1581–1589. doi: 10.1038/nn.2961
Kennerley, S. W., Dahmubed, A. F., Lara, A. H., and Wallis, J. D. (2009). Neurons in the frontal lobe encode the value of multiple decision variables. J. Cogn. Neurosci. 21, 1162–1178. doi: 10.1162/jocn.2009.21100
Khamassi, M., Enel, P., Dominey, P. F., and Procyk, E. (2013). Medial prefrontal cortex and the adaptive regulation of reinforcement learning parameters. Prog. Brain Res. 202, 441–464. doi: 10.1016/B978-0-444-62604-2.00022-8
Khamassi, M., Lallée, S., Enel, P., Procyk, E., and Dominey, P. F. (2011). Robot cognitive control with a neurophysiologically inspired reinforcement learning model. Front Neurorobot 5:1. doi: 10.3389/fnbot.2011.00001
Khamassi, M., Quilodran, R., Enel, P., Dominey, P. F., and Procyk, E. (2015). Behavioral regulation and the modulation of information coding in the lateral prefrontal and cingulate cortex. Cereb. Cortex 25, 3197–3218. doi: 10.1093/cercor/bhu114
Kim, H., Lee, D., and Jung, M. W. (2013). Signals for previous goal choice persist in the dorsomedial, but not dorsolateral striatum of rats. J. Neurosci. 33, 52–63. doi: 10.1523/JNEUROSCI.2422-12.2013
Kim, H., Sul, J. H., Huh, N., Lee, D., and Jung, M. W. (2009). Role of striatum in updating values of chosen actions. J. Neurosci. 29, 14701–14712. doi: 10.1523/JNEUROSCI.2728-09.2009
Kötter, R., and Wickens, J. (1995). Interactions of glutamate and dopamine in a computational model of the striatum. J. Comput. Neurosci. 2, 195–214. doi: 10.1007/BF00961434
Lebedev, M. A., Messinger, A., Kralik, J. D., and Wise, S. P. (2004). Representation of attended versus remembered locations in prefrontal cortex. PLoS Biol. 2:e365. doi: 10.1371/journal.pbio.0020365
Mackintosh, N. J. (1975). Blocking of conditioned suppression: role of the first compound trial. J. Exp. Psychol. 1, 335–345. doi: 10.1037/0097-7403.1.4.335
Mansouri, F. A., Matsumoto, K., and Tanaka, K. (2006). Prefrontal cell activities related to monkeys' success and failure in adapting to rule changes in a Wisconsin Card Sorting Test analog. J. Neurosci. 26, 2745–2756. doi: 10.1523/JNEUROSCI.5238-05.2006
Markowitz, D. A., Curtis, C. E., and Pesaran, B. (2015). Multiple component networks support working memory in prefrontal cortex. Proc. Natl. Acad. Sci. U.S.A. 112, 11084–11089. doi: 10.1073/pnas.1504172112
Matsuda, Y., Marzo, A., and Otani, S. (2006). The presence of background dopamine signal converts long-term synaptic depression to potentiation in rat prefrontal cortex. J. Neurosci. 26, 4803–4810. doi: 10.1523/JNEUROSCI.5312-05.2006
McDannald, M. A., Esber, G. R., Wegener, M. A., Wied, H. M., Liu, T.-L., Stalnaker, T. A., et al. (2014). Orbitofrontal neurons acquire responses to “valueless” Pavlovian cues during unblocking. eLife 3:e02653. doi: 10.7554/eLife.02653
Meyers, E. M., Qi, X. L., and Constantinidis, C. (2012). Incorporation of new information into prefrontal cortical activity after learning working memory tasks. Proc. Natl. Acad. Sci. U.S.A. 109, 4651–4656. doi: 10.1073/pnas.1201022109
Mill, R. D., Bagic, A., Bostan, A., Schneider, W., and Cole, M. W. (2017). Empirical validation of directed functional connectivity. Neuroimage 146, 275–287. doi: 10.1016/j.neuroimage.2016.11.037
Monosov, I. E. (2017). Anterior cingulate is a source of valence-specific information about value and uncertainty. Nat. Commun. 8:134. doi: 10.1038/s41467-017-00072-y
Montague, P. R., Dayan, P., and Sejnowski, T. J. (1996). A framework for mesencephalic dopamine systems based on predictive Hebbian learning. J. Neurosci. 16, 1936–1947.
Morrison, S. E., Saez, A., Lau, B., and Salzman, C. D. (2011). Different time courses for learning-related changes in amygdala and orbitofrontal cortex. Neuron 71, 1127–1140. doi: 10.1016/j.neuron.2011.07.016
Mulder, A. B., Nordquist, R. E., Orgüt, O., and Pennartz, C. M. A. (2003). Learning-related changes in response patterns of prefrontal neurons during instrumental conditioning. Behav. Brain Res. 146, 77–88. doi: 10.1016/j.bbr.2003.09.016
Niv, Y. (2009). Reinforcement learning in the brain. J. Math. Psychol. 53, 139–154. doi: 10.1016/j.jmp.2008.12.005
Niv, Y., Daniel, R., Geana, A., Gershman, S. J., Leong, Y. C., Radulescu, A., et al. (2015). Reinforcement learning in multidimensional environments relies on attention mechanisms. J. Neurosci. 35, 8145–8157. doi: 10.1523/JNEUROSCI.2978-14.2015
Noonan, M. P., Chau, B. K. H., Rushworth, M. F. S., and Fellows, L. K. (2017). Contrasting effects of medial and lateral orbitofrontal cortex lesions on credit assignment and decision-making in humans. J. Neurosci . 37, 7023–7035. doi: 10.1523/JNEUROSCI.0692-17.2017
Noonan, M. P., Walton, M. E., Behrens, T. E., Sallet, J., Buckley, M. J., and Rushworth, M. F. (2010). Separate value comparison and learning mechanisms in macaque medial and lateral orbitofrontal cortex. Proc. Natl. Acad. Sci. U.S.A. 107, 20547–20252. doi: 10.1073/pnas.1012246107
Oemisch, M., Westendorff, S., Everling, S., and Womelsdorf, T. (2015). Interareal spike-train correlations of anterior cingulate and dorsal prefrontal cortex during attention shifts. J. Neurosci. 35, 13076–13089. doi: 10.1523/JNEUROSCI.1262-15.2015
Padoa-Schioppa, C., and Assad, J. A. (2006). Neurons in the orbitofrontal cortex encode economic value. Nature 441, 223–226 doi: 10.1038/nature04676
Padoa-Schioppa, C., and Assad, J. A. (2008). The representation of economic value in the orbitofrontal cortex is invariant for changes of menu. Nat. Neurosci. 11, 95–102. doi: 10.1038/nn2020
Pan, W. X., Schmidt, R., Wickens, J. R., and Hyland, B. I. (2005). Dopamine cells respond to predicted events during classical conditioning: evidence for eligibility traces in the reward-learning network. J. Neurosci. 25, 6235–6242. doi: 10.1523/JNEUROSCI.1478-05.2005
Pasupathy, A., and Miller, E. K. (2005). Different time courses of learning-related activity in the prefrontal cortex and striatum. Nature 433, 873–876. doi: 10.1038/nature03287
Pearson, J. M., Hayden, B. Y., Raghavachari, S., and Platt, M. L. (2009). Neurons in posterior cingulate cortex signal exploratory decisions in a dynamic multioption choice task. Curr. Biol. 19, 1532–1537. doi: 10.1016/j.cub.2009.07.048
Procyk, E., Tanaka, Y. L., and Joseph, J. P. (2000). Anterior cingulate activity during routine and non-routine sequential behaviors in macaques. Nat. Neurosci. 3, 502–508. doi: 10.1038/74880
Quilodran, R., Rothe, M., and Procyk, E. (2008). Behavioral shifts and action valuation in the anterior cingulate cortex. Neuron 57, 314–325. doi: 10.1016/j.neuron.2007.11.031
Roelfsema, P. R., and van Ooyen, A. (2005). Attention-gated reinforcement learning of internal representations for classification. Neural. Comput. 17, 2176–2214. doi: 10.1162/0899766054615699
Rothkopf, C. A., and Ballard, D. H. (2010). Credit assignment in multiple goal embodied visuomotor behavior. Front. Psychol. 1:173. doi: 10.3389/fpsyg.2010.00173
Rudebeck, P. H., Behrens, T. E., Kennerley, S. W., Baxter, M. G., Buckley, M. J., Walton, M. E., et al. (2008). Frontal cortex subregions play distinct roles in choices between actions and stimuli. J. Neurosci. 28, 13775–13785. doi: 10.1523/JNEUROSCI.3541-08.2008
Saez, R. A., Saez, A., Paton, J. J., Lau, B., and Salzman, C. D. (2017). Distinct roles for the amygdala and orbitofrontal cortex in representing the relative amount of expected reward. Neuron 95, 70.e3–77.e3. doi: 10.1016/j.neuron.2017.06.012
Scholl, J., and Klein-Flügge, M. (2017). Understanding psychiatric disorder by capturing ecologically relevant features of learning and decision-making. Behav Brain Res . doi: 10.1016/j.bbr.2017.09.050. [Epub ahead of print].
Schultz, W. (1998a). Predictive reward signal of dopamine neurons. J. Neurophysiol. 80, 1–27. doi: 10.1152/jn.1998.80.1.1
Schultz, W. (1998b). The phasic reward signal of primate dopamine neurons. Adv. Pharmacol. 42, 686–690. doi: 10.1016/S1054-3589(08)60841-8
Schultz, W. (2004). Neural coding of basic reward terms of animal learning theory, game theory, microeconomics and behavioural ecology. Curr. Opin. Neurobiol. 14, 139–147. doi: 10.1016/j.conb.2004.03.017
Schultz, W., and Dickinson, A. (2000). Neuronal coding of prediction errors. Ann. Rev. Neurosci. 23, 473–500. doi: 10.1146/annurev.neuro.23.1.473
Seo, H., Barraclough, D. J., and Lee, D. (2007). Dynamic signals related to choices and outcomes in the dorsolateral prefrontal cortex. Cerebral Cortex 17(Suppl. 1), i110–i117. doi: 10.1093/cercor/bhm064
Seo, H., and Lee, D. (2007). Temporal filtering of reward signals in the dorsal anterior cingulate cortex during a mixed-strategy game. J. Neurosci. 27, 8366–8377. doi: 10.1523/JNEUROSCI.2369-07.2007
Seo, M., Lee, E., and Averbeck, B. B. (2012). Action selection and action value in frontal-striatal circuits. Neuron 74, 947–960. doi: 10.1016/j.neuron.2012.03.037
Seol, G. H., Ziburkus, J., Huang, S., Song, L., Kim, I. T., Takamiya, K., et al. (2007). Neuromodulators control the polarity of spike-timing-dependent synaptic plasticity. Neuron 55, 919–929. doi: 10.1016/j.neuron.2007.08.013
Shidara, M., and Richmond, B. J. (2002). Anterior cingulate: single neuronal signals related to degree of reward expectancy. Science 296, 1709–1711. doi: 10.1126/science.1069504
Shuler, M. G., and Bear, M. F. (2006). Reward timing in the primary visual cortex. Science 311, 1606–1609. doi: 10.1126/science.1123513
Sternson, S. M., and Roth, B. L. (2014). Chemogenetic tools to interrogate brain functions. Ann. Rev. Neurosci. 37, 387–407. doi: 10.1146/annurev-neuro-071013-014048
Stokes, M. G., Kusunoki, M., Sigala, N., Nili, H., Gaffan, D., and Duncan, J. (2013). Dynamic coding for cognitive control in prefrontal cortex. Neuron 78, 364–375. doi: 10.1016/j.neuron.2013.01.039
Sul, J. H., Kim, H., Huh, N., Lee, D., and Jung, M. W. (2010). Distinct roles of rodent orbitofrontal and medial prefrontal cortex in decision making. Neuron 66, 449–460. doi: 10.1016/j.neuron.2010.03.033
Sutton, R. S., and Barto, A. G. (1998). Reinforcement Learning: An Introduction Vol. 1 Cambridge: MIT press
Swanson, A. M., Allen, A. G., Shapiro, L. P., and Gourley, S. L. (2015). GABAAα1-mediated plasticity in the orbitofrontal cortex regulates context-dependent action selection. Neuropsychopharmacology 40, 1027–1036. doi: 10.1038/npp.2014.292
Tremblay, L., and Schultz, W. (1999). Relative reward preference in primate orbitofrontal cortex. Nature 398, 704–708. doi: 10.1038/19525
Tsujimoto, S., Genovesio, A., and Wise, S. P. (2009). Monkey orbitofrontal cortex encodes response choices near feedback time. J. Neurosci. 29, 2569–2574. doi: 10.1523/JNEUROSCI.5777-08.2009
Tsujimoto, S., Genovesio, A., and Wise, S. P. (2011). Comparison of strategy signals in the dorsolateral and orbital prefrontal cortex. J. Neurosci. 31, 4583–4592. doi: 10.1523/JNEUROSCI.5816-10.2011
Urbanczik, R., and Senn, W. (2009). Reinforcement learning in populations of spiking neurons. Nat. Neurosci. 12, 250–252. doi: 10.1038/nn.2264
Voloh, B., Valiante, T. A., Everling, S., and Womelsdorf, T. (2015). Theta-gamma coordination between anterior cingulate and prefrontal cortex indexes correct attention shifts. Proc. Natl. Acad. Sci. U.S.A. 112, 8457–8462. doi: 10.1073/pnas.1500438112
Wallis, J. D., and Miller, E. K. (2003). Neuronal activity in primate dorsolateral and orbital prefrontal cortex during performance of a reward preference task. Eur. J. Neurosci. 18, 2069–2081. doi: 10.1046/j.1460-9568.2003.02922.x
Walsh, M. M., and Anderson, J. R. (2014). Navigating complex decision spaces: problems and paradigms in sequential choice. Psychol. Bull. 140, 466–486. doi: 10.1037/a0033455
Wilson, R. C., and Niv, Y. (2011). Inferring relevance in a changing world. Front Hum. Neurosci. 5:189. doi: 10.3389/fnhum.2011.00189
Wilson, R. C., Takahashi, Y. K., Schoenbaum, G., and Niv, Y. (2014). Orbitofrontal cortex as a cognitive map of task space. Neuron 81, 267–279. doi: 10.1016/j.neuron.2013.11.005
Womelsdorf, T., Ardid, S., Everling, S., and Valiante, T. A. (2014). Burst firing synchronizes prefrontal and anterior cingulate cortex during attentional control. Curr. Biol. 24, 2613–2621. doi: 10.1016/j.cub.2014.09.046
Wörgötter, F., and Porr, B. (2005). Temporal sequence learning, prediction, and control: a review of different models and their relation to biological mechanisms. Neural. Comput. 17, 245–319. doi: 10.1162/0899766053011555
Xie, J., and Padoa-Schioppa, C. (2016). Neuronal remapping and circuit persistence in economic decisions. Nat. Neurosci. 19, 855–861. doi: 10.1038/nn.4300
Xu, Y. (2017). Reevaluating the sensory account of visual working memory storage. Trends Cogn. Sci. 21, 794–815 doi: 10.1016/j.tics.2017.06.013
Zhang, J. C., Lau, P.-M., and Bi, G.-Q. (2009). Gain in sensitivity and loss in temporal contrast of STDP by dopaminergic modulation at hippocampal synapses. Proc. Natl. Acad. Sci. U.S.A. 106, 13028–13033 doi: 10.1073/pnas.0900546106
Zsuga, J., Biro, K., Tajti, G., Szilasi, M. E., Papp, C., Juhasz, B., et al. (2016). ‘Proactive’ use of cue-context congruence for building reinforcement learning's reward function. BMC Neurosci. 17:70. doi: 10.1186/s12868-016-0302-7
Keywords: orbitofrontal, dorsolateral prefrontal, anterior cingulate, learning, reward, reinforcement, plasticity, behavioral flexibility
Citation: Stolyarova A (2018) Solving the Credit Assignment Problem With the Prefrontal Cortex. Front. Neurosci . 12:182. doi: 10.3389/fnins.2018.00182
Received: 27 September 2017; Accepted: 06 March 2018; Published: 27 March 2018.
Reviewed by:
Copyright © 2018 Stolyarova. This is an open-access article distributed under the terms of the Creative Commons Attribution License (CC BY) . The use, distribution or reproduction in other forums is permitted, provided the original author(s) and the copyright owner are credited and that the original publication in this journal is cited, in accordance with accepted academic practice. No use, distribution or reproduction is permitted which does not comply with these terms.
*Correspondence: Alexandra Stolyarova, [email protected]
Disclaimer: All claims expressed in this article are solely those of the authors and do not necessarily represent those of their affiliated organizations, or those of the publisher, the editors and the reviewers. Any product that may be evaluated in this article or claim that may be made by its manufacturer is not guaranteed or endorsed by the publisher.
Towards Practical Credit Assignment for Deep Reinforcement Learning
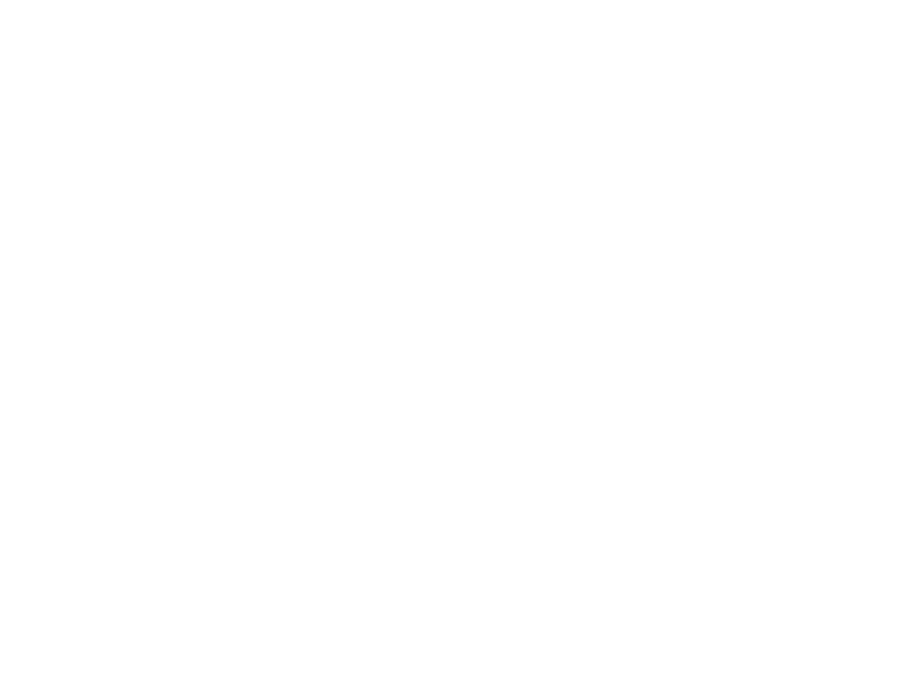
Credit assignment is a fundamental problem in reinforcement learning , the problem of measuring an action's influence on future rewards. Improvements in credit assignment methods have the potential to boost the performance of RL algorithms on many tasks, but thus far have not seen widespread adoption. Recently, a family of methods called Hindsight Credit Assignment (HCA) was proposed, which explicitly assign credit to actions in hindsight based on the probability of the action having led to an observed outcome. This approach is appealing as a means to more efficient data usage, but remains a largely theoretical idea applicable to a limited set of tabular RL tasks, and it is unclear how to extend HCA to Deep RL environments. In this work, we explore the use of HCA-style credit in a deep RL context. We first describe the limitations of existing HCA algorithms in deep RL, then propose several theoretically-justified modifications to overcome them. Based on this exploration, we present a new algorithm, Credit-Constrained Advantage Actor-Critic (C2A2C), which ignores policy updates for actions which don't affect future outcomes based on credit in hindsight, while updating the policy as normal for those that do. We find that C2A2C outperforms Advantage Actor-Critic (A2C) on the Arcade Learning Environment (ALE) benchmark, showing broad improvements over A2C and motivating further work on credit-constrained update rules for deep RL methods.

Vyacheslav Alipov
Riley Simmons-Edler
Nikita Putintsev
Pavel Kalinin
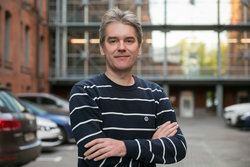
Dmitry Vetrov
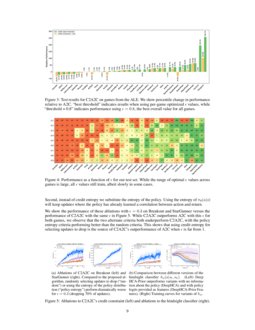
Related Research
Hindsight credit assignment, from credit assignment to entropy regularization: two new algorithms for neural sequence prediction, learning guidance rewards with trajectory-space smoothing, pairwise weights for temporal credit assignment, counterfactual credit assignment in model-free reinforcement learning, variance reduced advantage estimation with δ hindsight credit assignment, direct advantage estimation.
Please sign up or login with your details
Generation Overview
AI Generator calls
AI Video Generator calls
AI Chat messages
Genius Mode messages
Genius Mode images
AD-free experience
Private images
- Includes 500 AI Image generations, 1750 AI Chat Messages, 30 AI Video generations, 60 Genius Mode Messages and 60 Genius Mode Images per month. If you go over any of these limits, you will be charged an extra $5 for that group.
- For example: if you go over 500 AI images, but stay within the limits for AI Chat and Genius Mode, you'll be charged $5 per additional 500 AI Image generations.
- Includes 100 AI Image generations and 300 AI Chat Messages. If you go over any of these limits, you will have to pay as you go.
- For example: if you go over 100 AI images, but stay within the limits for AI Chat, you'll have to reload on credits to generate more images. Choose from $5 - $1000. You'll only pay for what you use.
Out of credits
Refill your membership to continue using DeepAI
Share your generations with friends
What is the "credit assignment" problem in Deep Learning?
I was watching a very interesting video with Yoshua Bengio where he is brainstorming with his students. In this video they seem to make a distinction between “credit assignment” vs gradient descent vs back-propagation. From the conversation it seems that the credit assignment problem is associated with “backprop” rather than gradient descent. I was trying to understand why that happened. Perhaps what would be helpful was if there was a very clear definition of “credit assignment” (specially in the context of Deep Learning and Neural Networks).
What is "the credit assignment problem:?
and how is it related to training/learning and optimization in Machine Learning (specially in Deep Learning).
From the discussion I would have defined as:
The function that computes the value(s) used to update the weights. How this value is used is the training algorithm but the credit assignment is the function that processes the weights (and perhaps something else) to that will later be used to update the weights.
That is how I currently understand it but to my surprise I couldn’t really find a clear definition on the internet. This more precise definition might be possible to be extracted from the various sources I found online:
https://www.youtube.com/watch?v=g9V-MHxSCcsWhat is the “credit assignment” problem in Machine Learning and Deep Learning?
How Auto-Encoders Could Provide Credit Assignment in Deep Networks via Target Propagation https://arxiv.org/pdf/1407.7906.pdf
Yoshua Bengio – Credit assignment: beyond backpropagation ( https://www.youtube.com/watch?v=z1GkvjCP7XA )
Learning to solve the credit assignment problem ( https://arxiv.org/pdf/1906.00889.pdf )
Cross-posted:
What is the "credit assignment" problem in Deep Learning?
https://www.reddit.com/r/MachineLearning/comments/cp54zi/what_is_the_credit_assignment_problem_in_machine/ ?
https://stats.stackexchange.com/questions/421741/what-is-the-credit-assignment-problem-in-machine-learning-and-deep-learning
https://www.quora.com/What-means-credit-assignment-when-talking-about-learning-in-neural-networks
If a sequence ends in a terminal state with a high reward, how do we determine which of the actions in that sequence were responsible for it?
This is the credit assignment problem
A robot will normally perform many actions and generate a reward a credit assignment problem is when the robot cannot define which of the actions has generated the best reward
The “Credit Assignment” Problem
I’m in state 43, reward = 0, action = 2
“ “ “ in state 39,reward = 0, action = 4
“ “ “ in state 10 reward = 0, action = 1
“ “ “ in state 21, reward = 0, action = 1
“ “ “in state 13,reward = 0 ,action = 2
“ “ “in state 26, reward = 100
I got to a state with a big reward but which of my actions along the way actually helped me get it?
This is the Credit Assignment problem.
NTRS - NASA Technical Reports Server
Available downloads, related records.
Credit report errors are more common than you think. Here's how to dispute one

Nearly half of all credit reports may contain errors, some of them costly to your credit score, according to a new watchdog report.
Two consumer groups, Consumer Reports and WorkMoney, invited more than 4,300 volunteers to check their credit reports for accuracy, as a sort of performance review of the three major credit agencies: Equifax , Experian and TransUnion .
Here’s what the y found :
◾ One-quarter of the consumers were unable to access their credit reports, which are supposed to be available to all.
◾ Among those who read their reports, 44% found errors.
Learn more: Best personal loans
◾ Of the errors, 27% were potentially damaging to the consumer’s credit.
The findings, released in late April, suggest that American consumers would be wise to read their reports. The credit agencies allow free access to them on a site called AnnualCreditReport.com .
“People don’t volunteer to be a part of this system, and a lot of decisions are made about you based on what’s in your account,” said Lisa Gill , an investigative reporter at Consumer Reports.
Related story: What information is on your credit report? Here's what I found when I read my own.
Credit scores are part of 'being able to afford life'
Credit reports factor into a dizzying array of consumer transactions. If you want to rent an apartment, buy a house, take a new job, or negotiate a better rate on a car loan, insurance premium, or cellphone contract, your credit score may determine your success. People with weak credit may get turned down, or penalized with higher rates and more stringent terms.
“It’s such an important part of people being able to afford life,” said Carrie Joy Grimes , CEO of WorkMoney, a nonprofit that helps consumers with their finances. “This is not a partisan issue. This is everyone in America.”
Consumer advocates acknowledge that it has probably never been easier to access your credit score, a metric of creditworthiness that ranges from 300 to 850, and the report upon which the score is based.
Long ago, consumers weren’t permitted to read their credit reports. Americans eventually gained the right to see their credit dossier, typically for a $15 fee. A 2003 law guaranteed access to free credit reports once a year . Today, consumers may see their reports once a week.
Industry leaders say they want consumers to read their reports, and to help credit agencies spot and fix potential errors.
“The consumer reporting industry shares the same goal as consumers, advocates, and regulators when it comes to credit reports: they should be accurate and complete,” the Consumer Data Industry Association, a group that represents the credit bureaus, said about the new report in a statement to USA TODAY.
“The nationwide credit reporting agencies are working diligently across the financial ecosystem to achieve that goal,” the statement said. “The entire business model of these companies is predicated on accuracy: when the information is accurate, everyone wins.”
Credit agency complaints nearly doubled in a year
Consumer watchdogs say the credit bureaus should do a better job.
Credit agencies are the most common subject of complaints filed to the federal Consumer Financial Protection Bureau, according to research by the U.S. Public Interest Research Group (PIRG). Complaints about credit reporting nearly doubled between 2021 and 2022, the nonprofit found.
Most complaints concerned allegations of improper use of credit reports, errors, or problems with getting an agency to correct mistakes, said Teresa Murray , consumer watchdog director at PIRG.
The credit bureaus “have not served consumers well for decades,” Murray said. “It’s gotten better, but it’s still a huge problem.”
Complaints are increasing, in part, because consumers have better access to their credit reports and are more keenly aware of them, she said. The massive Equifax data breach in 2017 raised public awareness of credit reports and their vulnerability.
In the Consumer Reports investigation, 872 consumers said they found errors in their credit reports about financial information: accounts they didn’t recognize, payments wrongly reported as late or missed, and debt-collection efforts of which they were not aware, among other issues.
'I had no idea this could happen'
One consumer volunteer in the watchdog study was Tammy Chambers, 55, of Tacoma, Washington.
When Chambers reviewed her Experian credit report, earlier this year, she found four delinquent loans totaling more than $2,000. None of them were hers.
Chambers said an identity thief took out the consumer installment loans in her name more than a year ago. When the loans went delinquent, her credit score sank from nearly 800 to “maybe 520,” she said. She spent months working with the loan company and the credit agency, trying to get the debts removed, to no avail.
“I did my due diligence,” she said. “I had no idea this could happen.”
Chambers finally got the debts expunged this spring. According to Consumer Reports, most of the fault lay not with Experian but with the company that issued the loans, which kept reporting them on Chambers’ account long after she had filed disputes.
Hundreds of other consumers in the watchdog study found mistaken personal information, including incorrect addresses and wrong or misspelled names.
Errors on credit reports can unfairly damage your credit score
Financial errors involving delinquent accounts are worrisome, consumer advocates say, because they can unfairly damage your credit score.
“Anything that’s reporting debt and collections that’s not yours, that is going to pull that score down 30, 40, 50 points, sometimes more,” Gill said.
An unfamiliar name or address or account on a credit report, meanwhile, “can be a signal of identity theft,” Gill said.
Roughly one-quarter of consumers in the watchdog study were unable to access their credit reports in the first place.
Many couldn’t get past the screening questions, which asked them to identify a familiar car loan or home mortgage on a multiple-choice list. Others got past those questions only to hit an error message, saying their credit reports were unavailable.
If you're concerned about your credit report, consumer advocates offer these tips:
Freeze your credit
Freezing your credit means no one can open a new account in your name. It’s free, and a great way to combat identity theft, Murray said.
PIRG offers a step-by-step guide .
One downside: If you want to open a new account, you will have to temporarily unfreeze your credit.
Read your credit reports
Consumers should review their credit reports at least once a year, looking for errors and anything unfamiliar, Murray said.
“If you want to overachieve, stagger them,” she said: Read a report from a different agency every four months.
Report any errors
If you find an error on a credit report, especially something that could affect your credit score or signal possible identity theft, you should report it.
“The bureaus are responsible for providing accurate information on the report,” Gill said.
You can report errors at any of the three credit bureau websites. If the error is on a specific account, consider contacting that company directly.
If necessary, file a complaint
If you find an error in a credit report, give the credit bureau time to fix it. If that doesn’t work, Murray said, file a complaint with the CFPB.
Complaints matter, Murray said: The more of them the agency receives, the more likely policymakers will step in to make it easier for future consumers to review and correct their credit reports.
Daniel de Visé covers personal finance for USA TODAY.
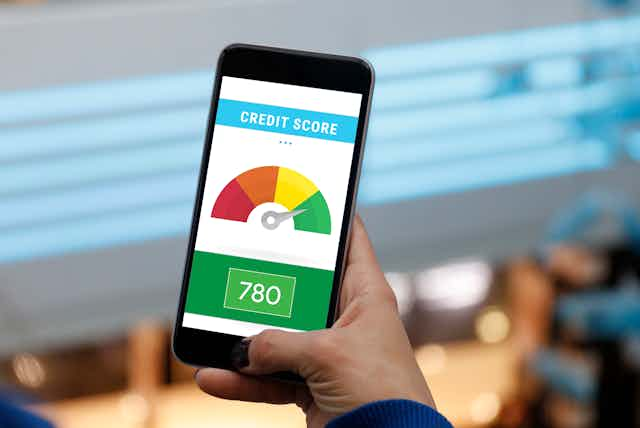
How do credit scores work? 2 finance professors explain how lenders choose who gets loans and at what interest rate
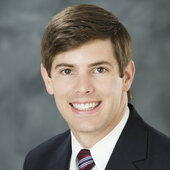
Assistant Professor of Finance, Mississippi State University
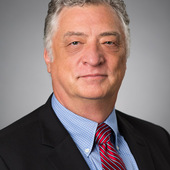
Professor of Finance, Mississippi State University
Disclosure statement
Tom Miller Jr. is affiliated with Consumers' Research, a consumer advocacy organization founded in 1929.
D. Brian Blank does not work for, consult, own shares in or receive funding from any company or organisation that would benefit from this article, and has disclosed no relevant affiliations beyond their academic appointment.
Mississippi State University provides funding as a member of The Conversation US.
View all partners
With the cost of borrowing money to buy a home or a car inching ever higher, understanding who gets access to credit, and at what interest rate, is more important for borrowers’ financial health than ever. Lenders base those decisions on the borrowers’ credit scores.
To learn more about credit scores, The Conversation consulted with two finance scholars. Brian Blank is an assistant professor of finance at Mississippi State University with expertise related to how firms allocate capital , as well as the role of credit in mortgage lending . His colleague at Mississippi State, Tom Miller Jr. , is a finance professor who has written a book on consumer lending , in addition to providing his expertise to policymakers.
Credit scoring assesses the likelihood of default
Lenders stay in business when borrowers pay back loans.
Some borrowers consistently make prompt payments, while others are slow to repay, and still others default – meaning they do not pay back the money they borrowed. Lenders have a strong business incentive to separate loans that will be paid back from loans that might be paid back.
So how do lenders distinguish between good borrowers and risky ones? They rely on various proprietary credit scoring systems that use past borrower repayment history and other factors to predict the likelihood of future repayment. The three organizations that monitor credit scores in the U.S. are Transunion , Experian and Equifax .
Although 26 million of 258 million credit-eligible Americans lack a credit score , anyone who has ever opened a credit card or other credit account, like a loan, has one. Most people don’t have a credit score before turning 18 , which is usually the age applicants can begin opening credit cards in their own name. However, some people still have no credit later in life if they don’t have any accounts for reporting agencies to assess.
Credit scores simply summarize how well individuals repay debt over time. Based on that repayment behavior, the credit scoring system assigns people a single number ranging from 300 to 850 . A credit score ranging from 670 to 739 is generally considered to be good, a score in the range of 580 to 669 would be judged fair, and a score less than 579 is classified poor, or subprime.
The two most important factors in credit scores are how promptly past debts have been paid and the amount the individual owes on current debt. The score also takes into account the mix and length of credit, in addition to how new it is.
Credit scores can help lenders decide what interest rate to offer consumers. And they can affect banks’ decisions concerning access to mortgages, credit cards and auto loans.
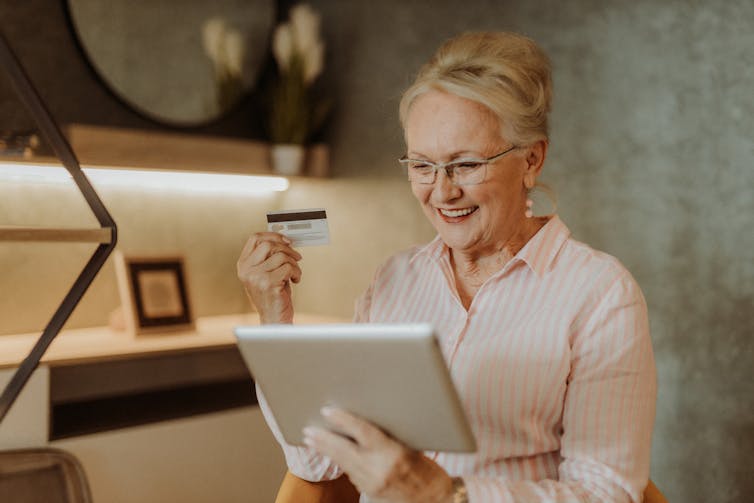
Recent improvements in consumer credit scores
Average credit scores in the United States have risen from 688 in 2005 to 716 as of August of 2021 . They stayed steady at that level through 2022 .
While credit card debt is at a record high , the average consumer was using just over a fourth of the revolving credit to which they had access as of September 2022.
As of 2021, nearly half of U.S. consumers had scores considered very good – meaning in the range of 740 to 799 – or excellent (800-850). Six in 10 Americans have a score above 700 , consistent with the general trend of record-setting credit scores of the past few years. These trends might, in part, reflect new programs that are designed to note when individuals pay bills like rent and utilities on time, which can help boost scores .
During the first quarter of 2023, people taking out new mortgages had an average credit score of 765, which is one point lower than a year ago but still higher than the pre-pandemic average of 760.
Credit score evolution from the 1980s to the 2020s
Developed in the late 1950s, the first credit scores – FICO scores – were created to build a computerized, objective measure to help lenders make lending decisions. Before then, bankers relied on commercial credit reporting, the same system merchants used to evaluate the creditworthiness of potential customers based on relationships and subjective evaluation .
The FICO credit scoring system was enhanced over the 1960s and ‘70s, and lenders grew to trust computerized credit evaluation systems. Credit scores really began to exert an influence on American borrowers beginning in the 1980s as FICO become widely used .
A major goal of the credit score is to expand the pool of potential borrowers while minimizing the overall default rate of the pool. In this way, lenders can maximize the number of loans they make. Still, credit scores are imperfect predictors, likely because most credit models assume that consumers will continue to act in the same way in the future as they have in the past. In addition, some believe that various risk factors make credit scores imperfect . Credit modelers, however, continue to make progress by making continuous technological innovations . Even FinTech lenders, which strive to go beyond traditional credit models , heavily rely on credit scores to set their interest rates.
Recently, “Buy Now, Pay Later” accounts have been added to credit scoring, while medical debt has been removed .
Credit scores might seem scary but can be useful
Borrowers with poor or limited credit have challenges building more positive credit histories and good credit scores. This challenge is particularly important because credit scores have become more widely used than ever because of the increasing availability of data and growing precision of credit models.
The availability of additional data results in more precise estimates of credit scoring , which can improve access to credit for consumers who repay bills consistently over time. These so-called “boost programs” factor in other payments that consumers routinely make on a monthly schedule. Think of the number of bills that you auto pay. Boost programs add points to your credit score for the bills that you pay consistently.
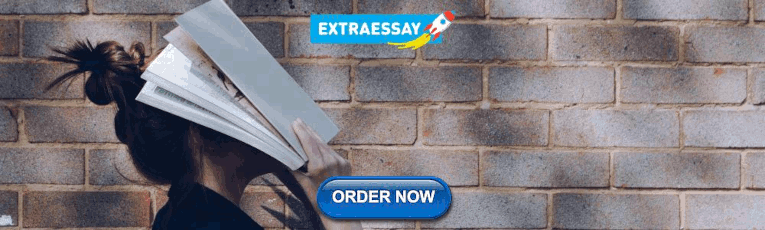
You can improve your credit score by making wise decisions
Two of the most important ways to improve credit scores are paying bills on time and ensuring that your credit report accurately reflects your payment history. Simply avoiding default is not enough. Timely payments are necessary. Someone who pays the bills every three months is “caught up” every quarter. But that consumer is 90 days delinquent four times a year. Being 90 days delinquent alarms creditors. So, someone who pays the bills every month will have a higher credit score at the end of the year.
Having more credit accounts can also positively affect your credit score because having these accounts shows that many lenders find you creditworthy. As a result, you might benefit from leaving credit accounts open if you make the wise decision not to access that credit. Warning! You must not use that extra credit access to spend more money and accumulate more debt. That decision is unwise.
Why? Because managing the ratio of debt to income is also critical to a good credit score . Debt-to-income ratios of 36% or less generally indicate individuals who have income to put toward savings, which is what all lenders are looking to see and one of the best ways to improve your credit.
- Interest rates
- Personal finance
- Credit scores
- Credit reporting
- Credit card debt
- Credit cards
- U.S. consumers

Chief Operating Officer (COO)

Technical Assistant - Metabolomics

Data Manager

Director, Social Policy

Head, School of Psychology
Credit Assignment
- Reference work entry
- First Online: 01 January 2017
- Cite this reference work entry
- Claude Sammut 3
Structural credit assignment ; Temporal credit assignment
When a learning system employs a complex decision process, it must assign credit or blame for the outcomes to each of its decisions. Where it is not possible to directly attribute an individual outcome to each decision, it is necessary to apportion credit and blame between each of the combinations of decisions that contributed to the outcome. We distinguish two cases in the credit assignment problem. Temporal credit assignment refers to the assignment of credit for outcomes to actions. Structural credit assignment refers to the assignment of credit for actions to internal decisions. The first subproblem involves determining when the actions that deserve credit were taken and the second involves assigning credit to the internal structure of actions (Sutton 1984 ).
Consider the problem of learning to balance a pole that is hinged on a cart (Michie and Chambers 1968 ; Anderson and Miller 1991 ). The cart...
This is a preview of subscription content, log in via an institution to check access.
Access this chapter
- Available as PDF
- Read on any device
- Instant download
- Own it forever
- Available as EPUB and PDF
- Durable hardcover edition
- Dispatched in 3 to 5 business days
- Free shipping worldwide - see info
Tax calculation will be finalised at checkout
Purchases are for personal use only
Institutional subscriptions
Recommended Reading
Albus JS (1975) A new approach to manipulator control: the cerebellar model articulation controller (CMAC). J Dyn Syst Measur Control Trans ASME 97(3):220–227
Article MATH Google Scholar
Anderson CW, Miller WT (1991) A set of challenging control problems. In: Miller W, Sutton RS, Werbos PJ (eds) Neural networks for control. MIT Press, Cambridge
Google Scholar
Atkeson C, Schaal S, Moore A (1997) Locally weighted learning. AI Rev 11:11–73
Banerjee B, Liu Y, Youngblood GM (eds) (2006) Proceedings of the ICML workshop on “structural knowledge transfer for machine learning, Pittsburgh
Barto A, Sutton R, Anderson C (1983) Neuron-like adaptive elements that can solve difficult learning control problems. IEEE Trans Syst Man Cybern SMC-13:834–846
Article Google Scholar
Benson S, Nilsson NJ (1995) Reacting, planning and learning in an autonomous agent. In: Furukawa K, Michie D, Muggleton S (eds) Machine intelligence, vol 14. Oxford University Press, Oxford
Bertsekas DP, Tsitsiklis J (1996) Neuro-dynamic programming. Athena Scientific, Nashua
MATH Google Scholar
Caruana R (1997) Multitask learning. Mach Learn 28:41–75
Dejong G, Mooney R (1986) Explanation-based learning: an alternative view. Mach Learn 1:145–176
Goldberg DE (1989) Genetic algorithms in search, optimization and machine learning. Addison-Wesley Longman Publishing, Boston
Grefenstette JJ (1988) Credit assignment in rule discovery systems based on genetic algorithms. Mach Learn 3(2–3):225–245
Hinton G, Rumelhart D, Williams R (1985) Learning internal representation by back-propagating errors. In: Rumelhart D, McClelland J, Group TPR (eds) Parallel distributed computing: explorations in the microstructure of cognition, vol 1. MIT Press, Cambridge, pp 31–362
Holland J (1986) Escaping brittleness: the possibilities of general-purpose learning algorithms applied to parallel rule-based systems. In: Michalski RS, Carbonell JG, Mitchell TM (eds) Machine learning: an artificial intelligence approach, vol 2. Morgan Kaufmann, Los Altos
Laird JE, Newell A, Rosenbloom PS (1987) SOAR: an architecture for general intelligence. Artif Intell 33(1):1–64
Mahadevan S (2009) Learning representation and control in Markov decision processes: new frontiers. Found Trends Mach Learn 1(4):403–565
Michie D, Chambers R (1968) Boxes: an experiment in adaptive control. In: Dale E, Michie D (eds) Machine intelligence, vol 2. Oliver and Boyd, Edinburgh
Minsky M (1961) Steps towards artificial intelligence. Proc IRE 49:8–30
Article MathSciNet Google Scholar
Mitchell TM, Keller RM, Kedar-Cabelli ST (1986) Explanation based generalisation: a unifying view. Mach Learn 1:47–80
Mitchell TM, Utgoff PE, Banerji RB (1983) Learning by experimentation: acquiring and refining problem-solving heuristics. In: Michalski R, Carbonell J, Mitchell T (eds) Machine kearning: an artificial intelligence approach. Tioga, Palo Alto
Moore AW (1990) Efficient memory-based learning for robot control. Ph.D. thesis, UCAM-CL-TR-209, Computer Laboratory, University of Cambridge, Cambridge
Niculescu-mizil A, Caruana R (2007) Inductive transfer for Bayesian network structure learning. In: Proceedings of the 11th international conference on AI and statistics (AISTATS 2007), San Juan
Reid MD (2004) Improving rule evaluation using multitask learning. In: Proceedings of the 14th international conference on inductive logic programming, Porto, pp 252–269
Reid MD (2007) DEFT guessing: using inductive transfer to improve rule evaluation from limited data. Ph.D. thesis, School of Computer Science and Engineering, The University of New South Wales, Sydney
Rosenblatt F (1962) Principles of neurodynamics: perceptrons and the theory of Brain mechanics. Spartan Books, Washington, DC
Samuel A (1959) Some studies in machine learning using the game of checkers. IBM J Res Develop 3(3):210–229
Silver D, Bakir G, Bennett K, Caruana R, Pontil M, Russell S et al (2005) NIPS workshop on “inductive transfer: 10 years later”, Whistler
Sutton R (1984) Temporal credit assignment in reinforcement learning. Ph.D. thesis, Department of Computer and Information Science, University of Massachusetts, Amherst
Sutton R, Barto A (1998) Reinforcement learning: an introduction. MIT Press, Cambridge
Taylor ME, Stone P (2009) Transfer learning for reinforcement learning domains: a survey. J Mach Learn Res 10:1633–1685
MathSciNet MATH Google Scholar
Wang X, Simon HA, Lehman JF, Fisher DH (1996) Learning planning operators by observation and practice. In: Proceedings of the second international conference on AI planning systems (AIPS-94), Chicago, pp 335–340
Watkins C (1989) Learning with delayed rewards. Ph.D. thesis, Psychology Department, University of Cambridge, Cambridge
Watkins C, Dayan P (1992) Q-learning. Mach Learn 8(3–4):279–292
Download references
Author information
Authors and affiliations.
The University of New South Wales, Sydney, NSW, Australia
Claude Sammut
You can also search for this author in PubMed Google Scholar
Editor information
Editors and affiliations.
Faculty of Information Technology, Monash University, Melbourne, VIC, Australia
Geoffrey I. Webb
Rights and permissions
Reprints and permissions
Copyright information
© 2017 Springer Science+Business Media New York
About this entry
Cite this entry.
Sammut, C. (2017). Credit Assignment. In: Sammut, C., Webb, G.I. (eds) Encyclopedia of Machine Learning and Data Mining. Springer, Boston, MA. https://doi.org/10.1007/978-1-4899-7687-1_185
Download citation
DOI : https://doi.org/10.1007/978-1-4899-7687-1_185
Published : 14 April 2017
Publisher Name : Springer, Boston, MA
Print ISBN : 978-1-4899-7685-7
Online ISBN : 978-1-4899-7687-1
eBook Packages : Computer Science Reference Module Computer Science and Engineering
Share this entry
Anyone you share the following link with will be able to read this content:
Sorry, a shareable link is not currently available for this article.
Provided by the Springer Nature SharedIt content-sharing initiative
- Publish with us
Policies and ethics
- Find a journal
- Track your research

An official website of the United States government
Here's how you know
Official websites use .gov A .gov website belongs to an official government organization in the United States.
Secure .gov websites use HTTPS A lock ( Lock A locked padlock ) or https:// means you've safely connected to the .gov website. Share sensitive information only on official, secure websites.
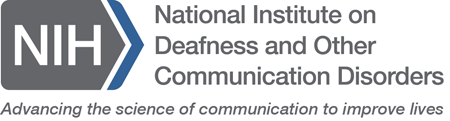
Voice, Speech, and Language
- Developmental Language Disorder
Diseases and Conditions
- Apraxia of Speech
- Auditory Processing Disorder
- Autism Spectrum Disorder: Communication Problems in Children
- Recurrent Respiratory Papillomatosis or Laryngeal Papillomatosis
- Spasmodic Dysphonia
- Vocal Fold Paralysis
More Health Information
- Clinical Studies: Information for the Public and Potential Volunteers
- MedlinePlus Consumer Health Database — at nlm.nih.gov
Communication Resources
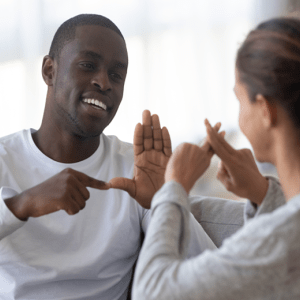
Information specialists can answer your questions in English or Spanish.
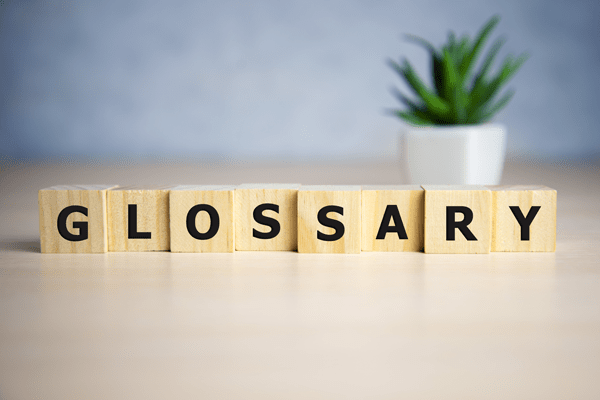
Our glossary provides definitions of medical words used on the NIDCD website.
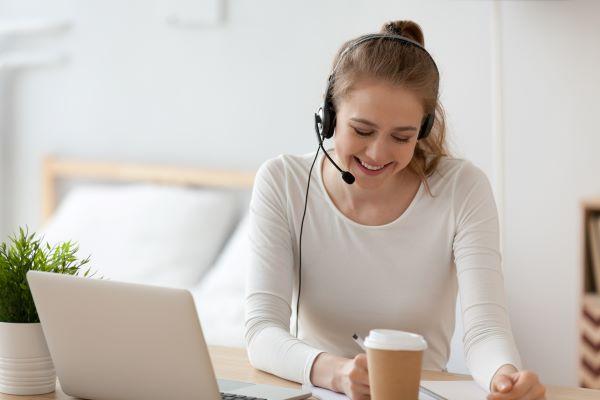
Order free publications. Find organizations. Get content for your site and much more.
Voice, Speech, and Language in the News: NIDCD-Supported Research
- May 8, 2024 Take care of your voice - NIH News in Health
- April 8, 2024 NYU researchers develop neural decoding that can give back lost speech
- March 19, 2024 Brain recordings in people before surgery reveal how all minds plan what to say before speaking
Related Topics
- Nearly 1 in 12 Children Ages 3–17 Has a Disorder Related to Voice, Speech, Lang…
- Prevent Voice Problems: Stay Hydrated (Multimedia content)
- Statistics and Epidemiology
- Developmental Milestones in Children Through Age 5 - Centers for Disease Contro…
Help | Advanced Search
Computer Science > Machine Learning
Title: credit assignment for trained neural networks based on koopman operator theory.
Abstract: Credit assignment problem of neural networks refers to evaluating the credit of each network component to the final outputs. For an untrained neural network, approaches to tackling it have made great contributions to parameter update and model revolution during the training phase. This problem on trained neural networks receives rare attention, nevertheless, it plays an increasingly important role in neural network patch, specification and verification. Based on Koopman operator theory, this paper presents an alternative perspective of linear dynamics on dealing with the credit assignment problem for trained neural networks. Regarding a neural network as the composition of sub-dynamics series, we utilize step-delay embedding to capture snapshots of each component, characterizing the established mapping as exactly as possible. To circumvent the dimension-difference problem encountered during the embedding, a composition and decomposition of an auxiliary linear layer, termed minimal linear dimension alignment, is carefully designed with rigorous formal guarantee. Afterwards, each component is approximated by a Koopman operator and we derive the Jacobian matrix and its corresponding determinant, similar to backward propagation. Then, we can define a metric with algebraic interpretability for the credit assignment of each network component. Moreover, experiments conducted on typical neural networks demonstrate the effectiveness of the proposed method.
Submission history
Access paper:.
- Other Formats
References & Citations
- Google Scholar
- Semantic Scholar
BibTeX formatted citation

Bibliographic and Citation Tools
Code, data and media associated with this article, recommenders and search tools.
- Institution
arXivLabs: experimental projects with community collaborators
arXivLabs is a framework that allows collaborators to develop and share new arXiv features directly on our website.
Both individuals and organizations that work with arXivLabs have embraced and accepted our values of openness, community, excellence, and user data privacy. arXiv is committed to these values and only works with partners that adhere to them.
Have an idea for a project that will add value for arXiv's community? Learn more about arXivLabs .
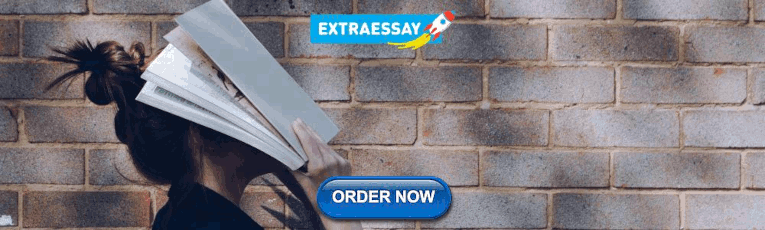
IMAGES
VIDEO
COMMENTS
The credit assignment problem (CAP) is a fundamental challenge in reinforcement learning. It arises when an agent receives a reward for a particular action, but the agent must determine which of its previous actions led to the reward. In reinforcement learning, an agent applies a set of actions in an environment to maximize the overall reward.
The (temporal) credit assignment problem (CAP) (discussed in Steps Toward Artificial Intelligence by Marvin Minsky in 1961) is the problem of determining the actions that lead to a certain outcome. For example, in football, at each second, each football player takes an action.
In its simplest form, the credit assignment problem refers to the difficulty of assigning credit in complex networks. Updating weights using the gradient of the objective function, $\nabla_WF(W)$, has proven to be an excellent means of solving the credit assignment problem in ANNs. A question that systems neuroscience faces is whether the brain ...
Figure 1.Example tasks highlighting the challenge of credit assignment and learning strategies enabling animals to solve this problem. (A) An example of a distal reward task that can be successfully learned with eligibility traces and TD rules, where intermediate choices can acquire motivational significance and subsequently reinforce preceding decisions (ex., Pasupathy and Miller, 2005 ...
The credit assignment problem concerns determining how the success of a system's overall performance is due to the various contributions of the system's components (Minsky, 1963). "In playing a complex game such as chess or checkers, or in writing a computer program, one has a definite success criterion - the game is won or lost.
Determining that action is the problem of temporal credit assignment. Although the actions are directly responsible for the outcome of a trial, the internal process for choosing the action indirectly affects the outcome. Assigning credit or blame to those internal processes that lead to the choice of action is the structural credit assignment ...
Credit assignment is a fundamental problem in reinforcement learning, the problem of measuring an action's influence on future rewards. Explicit credit assignment methods have the potential to boost the performance of RL algorithms on many tasks, but thus far remain impractical for general use. Recently, a family of methods called Hindsight Credit Assignment (HCA) was proposed, which ...
The Credit Assignment Problem (CAP) refers to the longstanding challenge of Reinforcement Learning (RL) agents to associate actions with their long-term consequences. Solving the CAP is a crucial step towards the successful deployment of RL in the real world since most decision problems provide feedback that is noisy, delayed, and with little or no information about the causes. These ...
To solve the credit assignment problem is to devise means of building relevant internal representations; that is to say, to decide which state I µ, hid of hidden units is to be associated with a given pattern I µ, vis of visible units. Type Chapter Information
Credit assignment is a fundamental problem in reinforcement learning, the problem of measuring an action's influence on future rewards. Improvements in credit assignment methods have the potential to boost the performance of RL algorithms on many tasks, but thus far have not seen widespread adoption. Recently, a family of methods called ...
The credit assignment problem in reinforcement learning [Minsky,1961,Sutton,1985,1988] is concerned with identifying the contribution of past actions on observed future outcomes. Of par-ticular interest to the reinforcement-learning (RL) problem [Sutton and Barto,1998] are observed
Credit assignment is necessary for any form of associative learning, but it is more challenging when the causal environmental feature is ephemeral and so no longer present when the outcome is revealed (this is the temporal credit-assignment problem) or when multiple potentially relevant features are concurrently present (the structural credit ...
Credit assignment is a fundamental problem in reinforcement learning, the problem of measuring an action's influence on future rewards. Explicit credit assignment methods have the potential to boost the performance of RL algorithms on many tasks, but thus far remain impractical for general
This is the credit assignment problem. Example1: A robot will normally perform many actions and generate a reward a credit assignment problem is when the robot cannot define which of the actions has generated the best reward. Example2: The "Credit Assignment" Problem. I'm in state 43, reward = 0, action = 2. " " " in state 39,reward ...
the credit assignment problem. 2 LEARNING FEEDBACK WEIGHTS THROUGH PERTURBATIONS We use the following notation. Let x 2Rm represent an input vector. Let an Nhidden-layer network be given by y^ = f(x) 2Rp. This is composed of a set of layer-wise summation and non-linear activations hi= fi(hi 1) = ˙ Wihi 1; for hidden layer states hi 2Rn
Assigning credit or blame for each of those actions individually is known as the (temporal) Credit Assignment Problem (CAP) . The CAP is particularly relevant for real-world tasks, where we need to learn effective policies from small, limited training datasets.
effects. The problem of learning to associate actions with distant, future outcomes is known as the temporal Credit Assignment Problem (CAP): to distribute the credit of success among the multitude of decisions involved (Minsky, 1961). Overall, the influence that an action has on an outcome represents knowledge in
This equivalence shows how an often neglected issue in multi-agent systems is equivalent to a well-known deficiency in multi-time-step learning and lays the basis for solving time-extended multi-agent problems, where both credit assignment problems are present. Document ID. 20040068179. Acquisition Source. Ames Research Center.
The assignment problem is a fundamental combinatorial optimization problem. In its most general form, the problem is as follows: The problem instance has a number of agents and a number of tasks. Any agent can be assigned to perform any task, incurring some cost that may vary depending on the agent-task assignment.
Learning to solve the credit assignment problem. Benjamin James Lansdell, Prashanth Ravi Prakash, Konrad Paul Kording. Backpropagation is driving today's artificial neural networks (ANNs). However, despite extensive research, it remains unclear if the brain implements this algorithm. Among neuroscientists, reinforcement learning (RL) algorithms ...
Credit scores are part of 'being able to afford life' Credit reports factor into a dizzying array of consumer transactions. If you want to rent an apartment, buy a house, take a new job, or ...
Credit scores simply summarize how well individuals repay debt over time. Based on that repayment behavior, the credit scoring system assigns people a single number ranging from 300 to 850. A ...
Determining that action is the problem of temporal credit assignment. Although the actions are directly responsible for the outcome of a trial, the internal process for choosing the action indirectly affects the outcome. Assigning credit or blame to those internal processes that lead to the choice of action is the structural credit assignment ...
Diseases and Conditions. Aphasia. Apraxia of Speech. Auditory Processing Disorder. Autism Spectrum Disorder: Communication Problems in Children. Developmental Language Disorder. Dysphagia. Hoarseness. Recurrent Respiratory Papillomatosis or Laryngeal Papillomatosis.
integration of two different signals, and may thus provide a realistic solution to the credit assignment problem. Thus we implement a network that learns to use feedback signals trained with reinforcement learning via a global reward signal. We mathematically analyze the model, and compare its capabilities
Credit assignment problem of neural networks refers to evaluating the credit of each network component to the final outputs. For an untrained neural network, approaches to tackling it have made great contributions to parameter update and model revolution during the training phase. This problem on trained neural networks receives rare attention ...