- Original article
- Open access
- Published: 09 April 2020
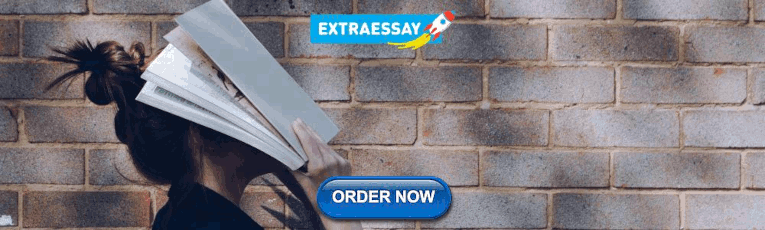
Why does peer instruction benefit student learning?
- Jonathan G. Tullis 1 &
- Robert L. Goldstone 2
Cognitive Research: Principles and Implications volume 5 , Article number: 15 ( 2020 ) Cite this article
85k Accesses
46 Citations
52 Altmetric
Metrics details
In peer instruction, instructors pose a challenging question to students, students answer the question individually, students work with a partner in the class to discuss their answers, and finally students answer the question again. A large body of evidence shows that peer instruction benefits student learning. To determine the mechanism for these benefits, we collected semester-long data from six classes, involving a total of 208 undergraduate students being asked a total of 86 different questions related to their course content. For each question, students chose their answer individually, reported their confidence, discussed their answers with their partner, and then indicated their possibly revised answer and confidence again. Overall, students were more accurate and confident after discussion than before. Initially correct students were more likely to keep their answers than initially incorrect students, and this tendency was partially but not completely attributable to differences in confidence. We discuss the benefits of peer instruction in terms of differences in the coherence of explanations, social learning, and the contextual factors that influence confidence and accuracy.
Significance
Peer instruction is widely used in physics instruction across many universities. Here, we examine how peer instruction, or discussing one’s answer with a peer, affects students’ decisions about a class assignment. Across six different university classes, students answered a question, discussed their answer with a peer, and finally answered the question again. Students’ accuracy consistently improved through discussion with a peer. Our peer instruction data show that students were hesitant to switch away from their initial answer and that students did consider both their own confidence and their partner’s confidence when making their final decision, in accord with basic research about confidence in decision making. More broadly, the data reveal that peer discussion helped students select the correct answer by prompting them to create new knowledge. The benefit to student accuracy that arises when students discuss their answers with a partner is a “process gain”, in which working in a group yields better performance than can be predicted from individuals’ performance alone.
Peer instruction is specific evidence-based instructional strategy that is well-known and widely used, particularly in physics (Henderson & Dancy, 2009 ). In fact, peer instruction has been advocated as a part of best methods in science classrooms (Beatty, Gerace, Leonard, & Dufresne, 2006 ; Caldwell, 2007 ; Crouch & Mazur, 2001 ; Newbury & Heiner, 2012 ; Wieman et al., 2009 ) and over a quarter of university physics professors report using peer instruction (Henderson & Dancy, 2009 ). In peer instruction, instructors pose a challenging question to students, students answer the question individually, students discuss their answers with a peer in the class, and finally students answer the question again. There are variations of peer instruction in which instructors show the class’s distribution of answers before discussion (Nielsen, Hansen-Nygård, & Stav, 2012 ; Perez et al., 2010 ), in which students’ answers are graded for participation or for correctness (James, 2006 ), and in which instructors’ norms affect whether peer instruction offers opportunities for answer-seeking or for sense-making (Turpen & Finkelstein, 2007 ).
Despite wide variations in its implementation, peer instruction consistently benefits student learning. Switching classroom structure from didactic lectures to one centered around peer instruction improves learners’ conceptual understanding (Duncan, 2005 ; Mazur, 1997 ), reduces student attrition in difficult courses (Lasry, Mazur, & Watkins, 2008 ), decreases failure rates (Porter, Bailey-Lee, & Simon, 2013 ), improves student attendance (Deslauriers, Schelew, & Wieman, 2011 ), and bolsters student engagement (Lucas, 2009 ) and attitudes to their course (Beekes, 2006 ). Benefits of peer instruction have been found across many fields, including physics (Mazur, 1997 ; Pollock, Chasteen, Dubson, & Perkins, 2010 ), biology (Knight, Wise, & Southard, 2013 ; Smith, Wood, Krauter, & Knight, 2011 ), chemistry (Brooks & Koretsky, 2011 ), physiology (Cortright, Collins, & DiCarlo, 2005 ; Rao & DiCarlo, 2000 ), calculus (Lucas, 2009 ; Miller, Santana-Vega, & Terrell, 2007 ), computer science (Porter et al., 2013 ), entomology (Jones, Antonenko, & Greenwood, 2012 ), and even philosophy (Butchart, Handfield, & Restall, 2009 ). Additionally, benefits of peer instruction have been found at prestigious private universities, two-year community colleges (Lasry et al., 2008 ), and even high schools (Cummings & Roberts, 2008 ). Peer instruction benefits not just the specific questions posed during discussion, but also improves accuracy on later similar problems (e.g., Smith et al., 2009 ).
One of the consistent empirical hallmarks of peer instruction is that students’ answers are more frequently correct following discussion than preceding it. For example, in introductory computer science courses, post-discussion performance was higher on 70 out of 71 questions throughout the semester (Simon, Kohanfars, Lee, Tamayo, & Cutts, 2010 ). Further, gains in performance from discussion are found on many different types of questions, including recall, application, and synthesis questions (Rao & DiCarlo, 2000 ). Performance improvements are found because students are more likely to switch from an incorrect answer to the correct answer than from the correct answer to an incorrect answer. In physics, 59% of incorrect answers switched to correct following discussion, but only 13% of correct answers switched to incorrect (Crouch & Mazur, 2001 ). Other research on peer instruction shows the same patterns: 41% of incorrect answers are switched to correct ones, while only 18% of correct answers are switched to incorrect (Morgan & Wakefield, 2012 ). On qualitative problem-solving questions in physiology, 57% of incorrect answers switched to correct after discussion, and only 7% of correct answers to incorrect (Giuliodori, Lujan, & DiCarlo, 2006 ).
There are two explanations for improvements in pre-discussion to post-discussion accuracy. First, switches from incorrect to correct answers may be driven by selecting the answer from the peer who is more confident. When students discuss answers that disagree, they may choose whichever answer belongs to the more confident peer. Evidence about decision-making and advice-taking substantiates this account. First, confidence is correlated with correctness across many settings and procedures (Finley, Tullis, & Benjamin, 2010 ). Students who are more confident in their answers are typically more likely to be correct. Second, research examining decision-making and advice-taking indicates that (1) the less confident you are, the more you value others’ opinions (Granovskiy, Gold, Sumpter, & Goldstone, 2015 ; Harvey & Fischer, 1997 ; Yaniv, 2004a , 2004b ; Yaniv & Choshen-Hillel, 2012 ) and (2) the more confident the advisor is, the more strongly they influence your decision (Kuhn & Sniezek, 1996 ; Price & Stone, 2004 ; Sah, Moore, & MacCoun, 2013 ; Sniezek & Buckley, 1995 ; Van Swol & Sniezek, 2005 ; Yaniv, 2004b ). Consequently, if students simply choose their final answer based upon whoever is more confident, accuracy should increase from pre-discussion to post-discussion. This explanation suggests that switches in answers should be driven entirely by a combination of one’s own initial confidence and one’s partner’s confidence. In accord with this confidence view, Koriat ( 2015 ) shows that an individual’s confidence typically reflects the group’s most typically given answer. When the answer most often given by group members is incorrect, peer interactions amplify the selection of and confidence in incorrect answers. Correct answers have no special draw. Rather, peer instruction merely amplifies the dominant view through differences in the individual’s confidence.
In a second explanation, working with others may prompt students to verbalize explanations and verbalizations may generate new knowledge. More specifically, as students discuss the questions, they need to create a common representation of the problem and answer. Generating a common representation may compel students to identify gaps in their existing knowledge and construct new knowledge (Schwartz, 1995 ). Further, peer discussion may promote students’ metacognitive processes of detecting and correcting errors in their mental models. Students create more new knowledge and better diagnostic tests of answers together than alone. Ultimately, then, the new knowledge and improved metacognition may make the correct answer appear more compelling or coherent than incorrect options. Peer discussion would draw attention to coherent or compelling answers, more so than students’ initial confidence alone and the coherence of the correct answer would prompt students to switch away from incorrect answers. Similarly, Trouche, Sander, and Mercier ( 2014 ) argue that interactions in a group prompt argumentation and discussion of reasoning. Good arguments and reasoning should be more compelling to change individuals’ answers than confidence alone. Indeed, in a reasoning task known to benefit from careful deliberation, good arguments and the correctness of the answers change partners’ minds more than confidence in one’s answer (Trouche et al., 2014 ). This explanation predicts several distinct patterns of data. First, as seen in prior research, more students should switch from incorrect answers to correct than vice versa. Second, the intrinsic coherence of the correct answer should attract students, so the likelihood of switching answers would be predicted by the correctness of an answer above and beyond differences in initial confidence. Third, initial confidence in an answer should not be as tightly related to initial accuracy as final confidence is to final accuracy because peer discussion should provide a strong test of the coherence of students’ answers. Fourth, because the coherence of an answer is revealed through peer discussion, student confidence should increase more from pre-discussion to post-discussion when they agree on the correct answers compared to agreeing on incorrect answers.
Here, we examined the predictions of these two explanations of peer instruction across six different classes. We specifically examined whether changes in answers are driven exclusively through the confidence of the peers during discussion or whether the coherence of an answer is better constructed and revealed through peer instruction than on one’s own. We are interested in analyzing cognitive processes at work in a specific, but common, implementation of classroom-based peer instruction; we do not intend to make general claims about all kinds of peer instruction or to evaluate the long-term effectiveness of peer instruction. This research is the first to analyze how confidence in one’s answer relates to answer-switching during peer instruction and tests the impact of peer instruction in new domains (i.e., psychology and educational psychology classes).
Participants
Students in six different classes participated as part of their normal class procedures. More details about these classes are presented in Table 1 . The authors served as instructors for these classes. Across the six classes, 208 students contributed a total of 1657 full responses to 86 different questions.
The instructors of the courses developed multiple-choice questions related to the ongoing course content. Questions were aimed at testing students’ conceptual understanding, rather than factual knowledge. Consequently, questions often tested whether students could apply ideas to new settings or contexts. An example of a cognitive psychology question used is: Which is a fixed action pattern (not a reflex)?
Knee jerks up when patella is hit
Male bowerbirds building elaborate nests [correct]
Eye blinks when air is blown on it
Can play well learned song on guitar even when in conversation
The procedures for peer instruction across the six different classes followed similar patterns. Students were presented with a multiple-choice question. First, students read the question on their own, chose their answer, and reported their confidence in their answer on a scale of 1 “Not at all confident” to 10 “Highly confident”. Students then paired up with a neighbor in their class and discussed the question with their peer. After discussion, students answered the question and reported the confidence for a second time. The course instructor indicated the correct answer and discussed the reasoning for the answer after all final answers had been submitted. Instruction was paced based upon how quickly students read and answered questions. Most student responses counted towards their participation grade, regardless of the correctness of their answer (the last question in each of the cognitive psychology classes was graded for correctness).
There were small differences in procedures between classes. Students in the cognitive psychology classes input their responses using classroom clickers, but those in other classes wrote their responses on paper. Further, students in the cognitive psychology classes explicitly reported their partner’s answer and confidence, while students in other classes only reported the name of their partner (the partners’ data were aligned during data recording). The cognitive psychology students then were required to mention their own answer and their confidence to their partner during peer instruction; students in other classes were not required to tell their answer or their confidence to their peer. Finally, the questions appeared at any point during the class period for the cognitive psychology classes, while the questions typically happened at the beginning of each class for the other classes.
Analytic strategy
Data are available on the OpenScienceFramework: https://mfr.osf.io/render?url=https://osf.io/5qc46/?action=download%26mode=render .
For most of our analyses we used linear mixed-effects models (Baayen, Davidson, & Bates, 2008 ; Murayama, Sakaki, Yan, & Smith, 2014 ). The unit of analysis in a mixed-effect model is the outcome of a single trial (e.g., whether or not a particular question was answered correctly by a particular participant). We modeled these individual trial-level outcomes as a function of multiple fixed effects - those of theoretical interest - and multiple random effects - effects for which the observed levels are sampled out of a larger population (e.g., questions, students, and classes sampled out of a population of potential questions, students, and classes).
Linear mixed-effects models solve four statistical problems involved with the data of peer instruction. First, there is large variability in students’ performance and the difficulty of questions across students and classes. Mixed-effect models simultaneously account for random variation both across participants and across items (Baayen et al., 2008 ; Murayama et al., 2014 ). Second, students may miss individual classes and therefore may not provide data across every item. Similarly, classes varied in how many peer instruction questions were posed throughout the semester and the number of students enrolled. Mixed-effects models weight each response equally when drawing conclusions (rather than weighting each student or question equally) and can easily accommodate missing data. Third, we were interested in how several different characteristics influenced students’ performance. Mixed effects models can include multiple predictors simultaneously, which allows us to test the effect of one predictor while controlling for others. Finally, mixed effects models can predict the log odds (or logit) of a correct answer, which is needed when examining binary outcomes (i.e., correct or incorrect; Jaeger, 2008 ).
We fit all models in R using the lmer() function of the lme4 package (Bates, Maechler, Bolker, & Walker, 2015 ). For each mixed-effect model, we included random intercepts that capture baseline differences in difficulty of questions, in classes, and in students, in addition to multiple fixed effects of theoretical interest. In mixed-effect models with hundreds of observations, the t distribution effectively converges to the normal, so we compared the t statistic to the normal distribution for analyses involving continuous outcomes (i.e., confidence; Baayen, 2008 ). P values can be directly obtained from Wald z statistics for models with binary outcomes (i.e., correctness).
Does accuracy change through discussion?
First, we examined how correctness changed across peer discussion. A logit model predicting correctness from time point (pre-discussion to post-discussion) revealed that the odds of correctness increased by 1.57 times (95% confidence interval (conf) 1.31–1.87) from pre-discussion to post-discussion, as shown in Table 2 . In fact, 88% of students showed an increase or no change in accuracy from pre-discussion to post-discussion. Pre-discussion to post-discussion performance for each class is shown in Table 3 . We further examined how accuracy changed from pre-discussion to post-discussion for each question and the results are plotted in Fig. 1 . The data show a consistent improvement in accuracy from pre-discussion to post-discussion across all levels of initial difficulty.
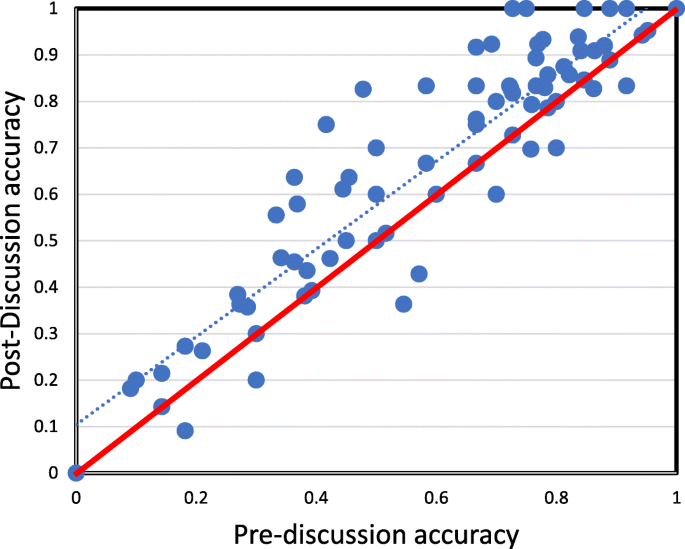
The relationship between pre-discussion accuracy (x axis) and post-discussion accuracy (y axis). Each point represents a single question. The solid diagonal line represents equal pre-discussion and post-discussion accuracy; points above the line indicate improvements in accuracy and points below represent decrements in accuracy. The dashed line indicates the line of best fit for the observed data
We examined how performance increased from pre-discussion to post-discussion by tracing the correctness of answers through the discussion. Figure 2 tracks the percent (and number of items) correct from pre-discussion to post-discussion. The top row shows whether students were initially correct or incorrect in their answer; the middle row shows whether students agreed or disagreed with their partner; the last row show whether students were correct or incorrect after discussion. Additionally, Fig. 2 shows the confidence associated with each pathway. The bottow line of each entry shows the students’ average confidence; in the middle white row, the confidence reported is the average of the peer’s confidence.
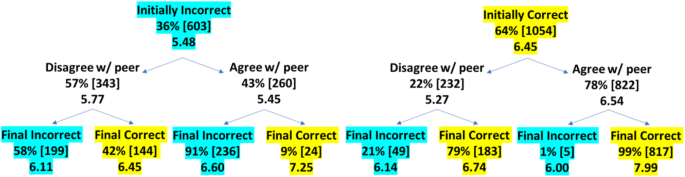
The pathways of answers from pre-discussion (top row) to post-discussion (bottom row). Percentages indicate the portion of items from the category immediately above in that category, the numbers in brackets indicate the raw numbers of items, and the numbers at the bottom of each entry indicate the confidence associated with those items. In the middle, white row, confidence values show the peer’s confidence. Turquoise indicates incorrect answers and yellow indicates correct answers
Broadly, only 5% of correct answers were switched to incorrect, while 28% of incorrect answers were switched to correct following discussion. Even for the items in which students were initially correct but disagreed with their partner, only 21% of answers were changed to incorrect answers after discussion. However, out of the items where students were initially incorrect and disagreed with their partner, 42% were changed to the correct answer.
Does confidence predict switching?
Differences in the amount of switching to correct or incorrect answers could be driven solely by differences in confidence, as described in our first theory mentioned earlier. For this theory to hold, answers with greater confidence must have a greater likelihood of being correct. To examine whether initial confidence is associated with initial correctness, we calculated the gamma correlation between correctness and confidence in the answer before discussion, as shown in the first column of Table 4 . The average gamma correlation between initial confidence and initial correctness (mean (M) = 0.40) was greater than zero, t (160) = 8.59, p < 0.001, d = 0.68, indicating that greater confidence was associated with being correct.
Changing from an incorrect to a correct answer, then, may be driven entirely by selecting the answer from the peer with the greater confidence during discussion, even though most of the students in our sample were not required to explicitly disclose their confidence to their partner during discussion. We examined how frequently students choose the more confident answer when peers disagree. When peers disagreed, students’ final answers aligned with the more confident peer only 58% of the time. Similarly, we tested what the performance would be if peers always picked the answer of the more confident peer. If peers always chose the more confident answer during discussion, the final accuracy would be 69%, which is significantly lower than actual final accuracy (M = 72%, t (207) = 2.59, p = 0.01, d = 0.18). While initial confidence is related to accuracy, these results show that confidence is not the only predictor of switching answers.
Does correctness predict switching beyond confidence?
Discussion may reveal information about the correctness of answers by generating new knowledge and testing the coherence of each possible answer. To test whether the correctness of an answer added predictive power beyond the confidence of the peers involved in discussion, we analyzed situations in which students disagreed with their partner. Out of the instances when partners initially disagreed, we predicted the likelihood of keeping one’s answer based upon one’s own confidence, the partner’s confidence, and whether one’s answer was initially correct. The results of a model predicting whether students keep their answers is shown in Table 5 . For each increase in a point of one’s own confidence, the odds of keeping one’s answer increases 1.25 times (95% conf 1.13–1.38). For each decrease in a point of the partner’s confidence, the odds of keeping one’s answer increased 1.19 times (1.08–1.32). The beta weight for one’s confidence did not differ from the beta weight of the partner’s confidence, χ 2 = 0.49, p = 0.48. Finally, if one’s own answer was correct, the odds of keeping one’s answer increased 4.48 times (2.92–6.89). In other words, the more confident students were, the more likely they were to keep their answer; the more confident their peer was, the more likely they were to change their answer; and finally, if a student was correct, they were more likely to keep their answer.
To illustrate this relationship, we plotted the probability of keeping one’s own answer as a function of the difference between one’s own and their partner’s confidence for initially correct and incorrect answers. As shown in Fig. 3 , at every confidence level, being correct led to equal or more frequently keeping one’s answer than being incorrect.
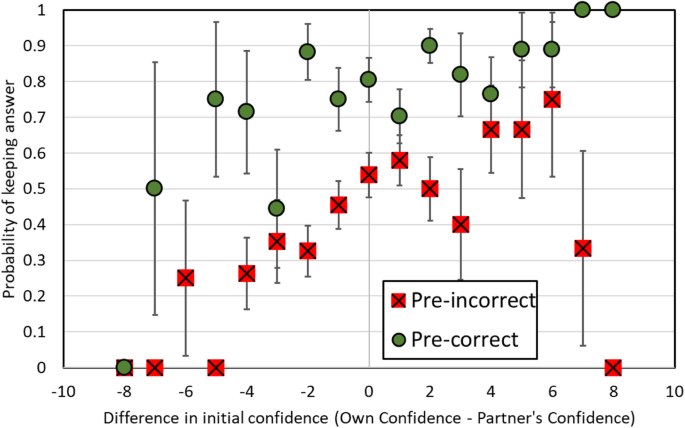
The probability of keeping one’s answer in situations where one’s partner initially disagreed as a function of the difference between partners’ levels of confidence. Error bars indicate the standard error of the proportion and are not shown when the data are based upon a single data point
As another measure of whether discussion allows learners to test the coherence of the correct answer, we analyzed how discussion impacted confidence when partners’ answers agreed. We predicted confidence in answers by the interaction of time point (i.e., pre-discussion versus post-discussion) and being initially correct for situations in which peers initially agreed on their answer. The results, displayed in Table 6 , show that confidence increased from pre-discussion to post-discussion by 1.08 points and that confidence was greater for initially correct answers (than incorrect answers) by 0.78 points. As the interaction between time point and initial correctness shows, confidence increased more from pre-discussion to post-discussion when students were initially correct (as compared to initially incorrect). To illustrate this relationship, we plotted pre-confidence against post-confidence for initially correct and initially incorrect answers when peers agreed (Fig. 4 ). Each plotted point represents a student; the diagonal blue line indicates no change between pre-confidence and post-confidence. The graph reflects that confidence increases more from pre-discussion to post-discussion for correct answers than for incorrect answers, even when we only consider cases where peers agreed.
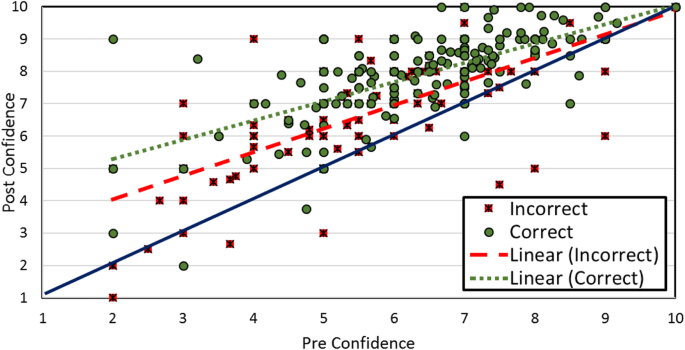
The relationship between pre-discussion and post-discussion confidence as a function of the accuracy of an answer when partners agreed. Each dot represents a student
If students engage in more comprehensive answer testing during discussion than before, the relationship between confidence in their answer and the accuracy of their answer should be stronger following discussion than it is before. We examined whether confidence accurately reflected correctness before and after discussion. To do so, we calculated the gamma correlation between confidence and accuracy, as is typically reported in the literature on metacognitive monitoring (e.g., Son & Metcalfe, 2000 ; Tullis & Fraundorf, 2017 ). Across all students, the resolution of metacognitive monitoring increases from pre-discussion to post-discussion ( t (139) = 2.98, p = 0.003, d = 0.24; for a breakdown of gamma calculations for each class, see Table 4 ). Confidence was more accurately aligned with accuracy following discussion than preceding it. The resolution between student confidence and correctness increases through discussion, suggesting that discussion offers better coherence testing than answering alone.
To examine why peer instruction benefits student learning, we analyzed student answers and confidence before and after discussion across six psychology classes. Discussing a question with a partner improved accuracy across classes and grade levels with small to medium-sized effects. Questions of all difficulty levels benefited from peer discussion; even questions where less than half of students originally answered correctly saw improvements from discussion. Benefits across the spectrum of question difficulty align with prior research showing improvements when even very few students initially know the correct answer (Smith et al., 2009 ). More students switched from incorrect answers to correct answers than vice versa, leading to an improvement in accuracy following discussion. Answer switching was driven by a student’s own confidence in their answer and their partner’s confidence. Greater confidence in one’s answer indicated a greater likelihood of keeping the answer; a partner’s greater confidence increased the likelihood of changing to their answer.
Switching answers depended on more than just confidence: even when accounting for students’ confidence levels, the correctness of the answer impacted switching behavior. Across several measures, our data showed that the correctness of an answer carried weight beyond confidence. For example, the correctness of the answer predicted whether students switched their initial answer during peer disagreements, even after taking the confidence of both partners into account. Further, students’ confidence increased more when partners agreed on the correct answer compared to when they agreed on an incorrect answer. Finally, although confidence increased from pre-discussion to post-discussion when students changed their answers from incorrect to the correct ones, confidence decreased when students changed their answer away from the correct one. A plausible interpretation of this difference is that when students switch from a correct answer to an incorrect one, their decrease in confidence reflects the poor coherence of their final incorrect selection.
Whether peer instruction resulted in optimal switching behaviors is debatable. While accuracy improved through discussion, final accuracy was worse than if students had optimally switched their answers during discussion. If students had chosen the correct answer whenever one of the partners initially chose it, the final accuracy would have been significantly higher (M = 0.80 (SD = 0.19)) than in our data (M = 0.72 (SD = 0.24), t (207) = 6.49, p < 0.001, d = 0.45). While this might be interpreted as “process loss” (Steiner, 1972 ; Weldon & Bellinger, 1997 ), that would assume that there is sufficient information contained within the dyad to ascertain the correct answer. One individual selecting the correct answer is inadequate for this claim because they may not have a compelling justification for their answer. When we account for differences in initial confidence, students’ final accuracy was better than expected. Students’ final accuracy was better than that predicted from a model in which students always choose the answer of the more confident peer. This over-performance, often called “process gain”, can sometimes emerge when individuals collaborate to create or generate new knowledge (Laughlin, Bonner, & Miner, 2002 ; Michaelsen, Watson, & Black, 1989 ; Sniezek & Henry, 1989 ; Tindale & Sheffey, 2002 ). Final accuracy reveals that students did not simply choose the answer of the more confident student during discussion; instead, students more thoroughly probed the coherence of answers and mental models during discussion than they could do alone.
Students’ final accuracy emerges from the interaction between the pairs of students, rather than solely from individuals’ sequestered knowledge prior to discussion (e.g. Wegner, Giuliano, & Hertel, 1985 ). Schwartz ( 1995 ) details four specific cognitive products that can emerge through working in dyads. Specifically, dyads force verbalization of ideas through discussion, and this verbalization facilitates generating new knowledge. Students may not create a coherent explanation of their answer until they engage in discussion with a peer. When students create a verbal explanation of their answer to discuss with a peer, they can identify knowledge gaps and construct new knowledge to fill those gaps. Prior research examining the content of peer interactions during argumentation in upper-level biology classes has shown that these kinds of co-construction happen frequently; over three quarters of statements during discussion involve an exchange of claims and reasoning to support those claims (Knight et al., 2013 ). Second, dyads have more information processing resources than individuals, so they can solve more complex problems. Third, dyads may foster greater motivation than individuals. Finally, dyads may stimulate the creation of new, abstract representations of knowledge, above and beyond what one would expect from the level of abstraction created by individuals. Students need to communicate with their partner; to create common ground and facilitate discourse, dyads negotiate common representations to coordinate different perspectives. The common representations bridge multiple perspectives, so they lose idiosyncratic surface features of individuals’ representation. Working in pairs generates new knowledge and tests of answers that could not be predicted from individuals’ performance alone.
More broadly, teachers often put students in groups so that they can learn from each other by giving and receiving help, recognizing contradictions between their own and others’ perspectives, and constructing new understandings from divergent ideas (Bearison, Magzamen, & Filardo, 1986 ; Bossert, 1988-1989 ; Brown & Palincsar, 1989 ; Webb & Palincsar, 1996 ). Giving explanations to a peer may encourage explainers to clarify or reorganize information, recognize and rectify gaps in understandings, and build more elaborate interpretations of knowledge than they would have alone (Bargh & Schul, 1980 ; Benware & Deci, 1984 ; King, 1992 ; Yackel, Cobb, & Wood, 1991 ). Prompting students to explain why and how problems are solved facilitates conceptual learning more than reading the problem solutions twice without self-explanations (Chi, de Leeuw, Chiu, & LaVancher, 1994 ; Rittle-Johnson, 2006 ; Wong, Lawson, & Keeves, 2002 ). Self-explanations can prompt students to retrieve, integrate, and modify their knowledge with new knowledge; self-explanations can also help students identify gaps in their knowledge (Bielaczyc, Pirolli, & Brown, 1995 ; Chi & Bassock, 1989 ; Chi, Bassock, Lewis, Reimann, & Glaser, 1989 ; Renkl, Stark, Gruber, & Mandl, 1998 ; VanLehn, Jones, & Chi, 1992 ; Wong et al., 2002 ), detect and correct errors, and facilitate deeper understanding of conceptual knowledge (Aleven & Koedinger, 2002 ; Atkinson, Renkl, & Merrill, 2003 ; Chi & VanLehn, 2010 ; Graesser, McNamara, & VanLehn, 2005 ). Peer instruction, while leveraging these benefits of self-explanation, also goes beyond them by involving what might be called “other-explanation” processes - processes recruited not just when explaining a situation to oneself but to others. Mercier and Sperber ( 2019 ) argue that much of human reason is the result of generating explanations that will be convincing to other members of one’s community, thereby compelling others to act in the way that one wants.
Conversely, students receiving explanations can fill in gaps in their own understanding, correct misconceptions, and construct new, lasting knowledge. Fellow students may be particularly effective explainers because they can better take the perspective of their peer than the teacher (Priniski & Horne, 2019 ; Ryskin, Benjamin, Tullis, & Brown-Schmidt, 2015 ; Tullis, 2018 ). Peers may be better able than expert teachers to explain concepts in familiar terms and direct peers’ attention to the relevant features of questions that they do not understand (Brown & Palincsar, 1989 ; Noddings, 1985 ; Vedder, 1985 ; Vygotsky, 1981 ).
Peer instruction may benefit from the generation of explanations, but social influences may compound those benefits. Social interactions may help students monitor and regulate their cognition better than self-explanations alone (e.g., Jarvela et al., 2015 ; Kirschner, Kreijns, Phielix, & Fransen, 2015 ; Kreijns, Kirschner, & Vermeulen, 2013 ; Phielix, Prins, & Kirschner, 2010 ; Phielix, Prins, Kirschner, Erkens, & Jaspers, 2011 ). Peers may be able to judge the quality of the explanation better than the explainer. In fact, recent research suggests that peer instruction facilitates learning even more than self-explanations (Versteeg, van Blankenstein, Putter, & Steendijk, 2019 ).
Not only does peer instruction generate new knowledge, but it may also improve students’ metacognition. Our data show that peer discussion prompted more thorough testing of the coherence of the answers. Specifically, students’ confidences were better aligned with accuracy following discussion than before. Improvements in metacognitive resolution indicate that discussion provides more thorough testing of answers and ideas than does answering questions on one’s own. Discussion facilitates the metacognitive processes of detecting errors and assessing the coherence of an answer.
Agreement among peers has important consequences for final behavior. For example, when peers agreed, students very rarely changed their answer (less than 3% of the time). Further, large increases in confidence occurred when students agreed (as compared to when they disagreed). Alternatively, disagreements likely engaged different discussion processes and prompted students to combine different answers. Whether students weighed their initial answer more than their partner’s initial answer remains debatable. When students disagreed with their partner, they were more likely to stick with their own answer than switch; they kept their own answer 66% of the time. Even when their partner was more confident, students only switched to their partner’s answer 50% of the time. The low rate of switching during disagreements suggests that students weighed their own answer more heavily than their partner’s answer. In fact, across prior research, deciders typically weigh their own thoughts more than the thoughts of an advisor (Harvey, Harries, & Fischer, 2000 ; Yaniv & Kleinberger, 2000 ).
Interestingly, peers agreed more frequently than expected by chance. When students were initially correct (64% of the time), 78% of peers agreed. When students were initially incorrect (36% of the time), peers agreed 43% of the time. Pairs of students, then, agree more than expected by a random distribution of answers throughout the classroom. These data suggest that students group themselves into pairs based upon likelihood of sharing the same answer. Further, these data suggest that student understanding is not randomly distributed throughout the physical space of the classroom. Across all classes, students were instructed to work with a neighbor to discuss their answer. Given that neighbors agreed more than predicted by chance, students seem to tend to sit near and pair with peers that share their same levels of understanding. Our results from peer instruction reveal that students physically locate themselves near students of similar abilities. Peer instruction could potentially benefit from randomly pairing students together (i.e. not with a physically close neighbor) to generate the most disagreements and generative activity during discussion.
Learning through peer instruction may involve deep processing as peers actively challenge each other, and this deep processing may effectively support long-term retention. Future research can examine the persistence of gains in accuracy from peer instruction. For example, whether errors that are corrected during peer instruction stay corrected on later retests of the material remains an open question. High and low-confidence errors that are corrected during peer instruction may result in different long-term retention of the correct answer; more specifically, the hypercorrection effect suggests that errors committed with high confidence are more likely to be corrected on subsequent tests than errors with low confidence (e.g., Butler, Fazio, & Marsh, 2011 ; Butterfield & Metcalfe, 2001 ; Metcalfe, 2017 ). Whether hypercorrection holds for corrections from classmates during peer instruction (rather than from an absolute authority) could be examined in the future.
The influence of partner interaction on accuracy may depend upon the domain and kind of question posed to learners. For simple factual or perceptual questions, partner interaction may not consistently benefit learning. More specifically, partner interaction may amplify and bolster wrong answers when factual or perceptual questions lead most students to answer incorrectly (Koriat, 2015 ). However, for more “intellective tasks,” interactions and arguments between partners can produce gains in knowledge (Trouche et al., 2014 ). For example, groups typically outperform individuals for reasoning tasks (Laughlin, 2011 ; Moshman & Geil, 1998 ), math problems (Laughlin & Ellis, 1986 ), and logic problems (Doise & Mugny, 1984; Perret-Clermont, 1980 ). Peer instruction questions that allow for student argumentation and reasoning, therefore, may have the best benefits in student learning.
The underlying benefits of peer instruction extend beyond the improvements in accuracy seen from pre-discussion to post-discussion. Peer instruction prompts students to retrieve information from long-term memory, and these practice tests improve long-term retention of information (Roediger III & Karpicke, 2006 ; Tullis, Fiechter, & Benjamin, 2018 ). Further, feedback provided by instructors following peer instruction may guide students to improve their performance and correct misconceptions, which should benefit student learning (Bangert-Drowns, Kulik, & Kulik, 1991 ; Thurlings, Vermeulen, Bastiaens, & Stijnen, 2013 ). Learners who engage in peer discussion can use their new knowledge to solve new, but similar problems on their own (Smith et al., 2009 ). Generating new knowledge and revealing gaps in knowledge through peer instruction, then, effectively supports students’ ability to solve novel problems. Peer instruction can be an effective tool to generate new knowledge through discussion between peers and improve student understanding and metacognition.
Availability of data and materials
As described below, data and materials are available on the OpenScienceFramework: https://mfr.osf.io/render?url=https://osf.io/5qc46/?action=download%26mode=render .
Aleven, V., & Koedinger, K. R. (2002). An effective metacognitive strategy: Learning by doing and explaining with a computer based cognitive tutor. Cognitive Science , 26 , 147–179.
Article Google Scholar
Atkinson, R. K., Renkl, A., & Merrill, M. M. (2003). Transitioning from studying examples to solving problems: Effects of self-explanation prompts and fading worked-out steps. Journal of Educational Psychology , 95 , 774–783.
Baayen, R. H. (2008). Analyzing linguistic data: A practical introduction to statistics . Cambridge: Cambridge University Press.
Baayen, R. H., Davidson, D. J., & Bates, D. M. (2008). Mixed-effects modeling with crossed random effects for subjects and items. Journal of Memory and Language , 59 , 390–412.
Bangert-Drowns, R. L., Kulik, J. A., & Kulik, C.-L. C. (1991). Effects of frequent classroom testing. Journal of Educational Research , 85 , 89–99.
Bargh, J. A., & Schul, Y. (1980). On the cognitive benefit of teaching. Journal of Educational Psychology , 72 , 593–604.
Bates, D., Maechler, M., Bolker, B., & Walker, S. (2015). Fitting linear mixed-effects models using lme4. Journal of Statistical Software , 67 , 1–48.
Bearison, D. J., Magzamen, S., & Filardo, E. K. (1986). Sociocognitive conflict and cognitive growth in young children. Merrill-Palmer Quarterly , 32 (1), 51–72.
Google Scholar
Beatty, I. D., Gerace, W. J., Leonard, W. J., & Dufresne, R. J. (2006). Designing effective questions for classroom response system teaching. American Journal of Physics , 74 (1), 31e39.
Beekes, W. (2006). The “millionaire” method for encouraging participation. Active Learning in Higher Education , 7 , 25–36.
Benware, C. A., & Deci, E. L. (1984). Quality of learning with an active versus passive motivational set. American Educational Research Journal , 21 , 755–765.
Bielaczyc, K., Pirolli, P., & Brown, A. L. (1995). Training in self-explanation and self regulation strategies: Investigating the effects of knowledge acquisition activities on problem solving. Cognition and Instruction , 13 , 221–251.
Bossert, S. T. (1988-1989). Cooperative activities in the classroom. Review of Research in Education , 15 , 225–252.
Brooks, B. J., & Koretsky, M. D. (2011). The influence of group discussion on students’ responses and confidence during peer instruction. Journal of Chemistry Education , 88 , 1477–1484.
Brown, A. L., & Palincsar, A. S. (1989). Guided, cooperative learning and individual knowledge acquisition. In L. B. Resnick (Ed.), Knowing, learning, and instruction: essays in honor of Robert Glaser , (pp. 393–451). Hillsdale: Erlbaum.
Butchart, S., Handfield, T., & Restall, G. (2009). Using peer instruction to teach philosophy, logic and critical thinking. Teaching Philosophy , 32 , 1–40.
Butler, A. C., Fazio, L. K., & Marsh, E. J. (2011). The hypercorrection effect persists over a week, but high-confidence errors return. Psychonomic Bulletin & Review , 18 (6), 1238–1244.
Butterfield, B., & Metcalfe, J. (2001). Errors committed with high confidence are hypercorrected. Journal of Experimental Psychology: Learning, Memory, and Cognition , 27 (6), 1491.
PubMed Google Scholar
Caldwell, J. E. (2007). Clickers in the large classroom: current research and best-practice tips. CBE-Life Sciences Education , 6 (1), 9–20.
Article PubMed PubMed Central Google Scholar
Chi, M., & VanLehn, K. A. (2010). Meta-cognitive strategy instruction in intelligent tutoring systems: How, when and why. Journal of Educational Technology and Society , 13 , 25–39.
Chi, M. T. H., & Bassock, M. (1989). Learning from examples via self-explanations. In L. B. Resnick (Ed.), Knowing, learning, and instruction: Essays in honor of Robert Glaser , (pp. 251–282). Hillsdale: Erlbaum.
Chi, M. T. H., Bassock, M., Lewis, M., Reimann, P., & Glaser, R. (1989). Self-explanations: How students study and use examples in learning to solve problems. Cognitive Science , 13 , 145–182.
Chi, M. T. H., de Leeuw, N., Chiu, M. H., & LaVancher, C. (1994). Eliciting self-explanations improves understanding. Cognitive Science , 18 , 439–477.
Cortright, R. N., Collins, H. L., & DiCarlo, S. E. (2005). Peer instruction enhanced meaningful learning: Ability to solve novel problems. Advances in Physiology Education , 29 , 107–111.
Article PubMed Google Scholar
Crouch, C. H., & Mazur, E. (2001). Peer instruction: Ten years of experience and results. American Journal of Physics , 69 , 970–977.
Cummings, K., & Roberts, S. (2008). A study of peer instruction methods with school physics students. In C. Henderson, M. Sabella, & L. Hsu (Eds.), Physics education research conference , (pp. 103–106). College Park: American Institute of Physics.
Deslauriers, L., Schelew, E., & Wieman, C. (2011). Improved learning in a large-enrollment physics class. Science , 332 , 862–864.
Duncan, D. (2005). Clickers in the classroom: How to enhance science teaching using classroom response systems . San Francisco: Pearson/Addison-Wesley.
Finley, J. R., Tullis, J. G., & Benjamin, A. S. (2010). Metacognitive control of learning and remembering. In M. S. Khine, & I. M. Saleh (Eds.), New science of learning: Cognition, computers and collaborators in education . New York: Springer Science & Business Media, LLC.
Giuliodori, M. J., Lujan, H. L., & DiCarlo, S. E. (2006). Peer instruction enhanced student performance on qualitative problem solving questions. Advances in Physiology Education , 30 , 168–173.
Graesser, A. C., McNamara, D., & VanLehn, K. (2005). Scaffolding deep comprehension strategies through AutoTutor and iSTART. Educational Psychologist , 40 , 225–234.
Granovskiy, B., Gold, J. M., Sumpter, D., & Goldstone, R. L. (2015). Integration of social information by human groups. Topics in Cognitive Science , 7 , 469–493.
Harvey, N., & Fischer, I. (1997). Taking advice: Accepting help, improving judgment, and sharing responsibility. Organizational Behavior and Human Decision Processes , 70 , 117–133.
Harvey, N., Harries, C., & Fischer, I. (2000). Using advice and assessing its quality. Organizational Behavior and Human Decision Processes , 81 , 252–273.
Henderson, C., & Dancy, M. H. (2009). The impact of physics education research on the teaching of introductory quantitative physics in the United States. Physical Review Special Topics: Physics Education Research , 5 (2), 020107.
Jaeger, T. F. (2008). Categorical data analysis: away from ANOVAs (transformation or not) and towards logit mixed models. Journal of Memory and Language , 59 , 434–446.
James, M. C. (2006). The effect of grading incentive on student discourse in peer instruction. American Journal of Physics , 74 (8), 689–691.
Jarvela, S., Kirschner, P., Panadero, E., Malmberg, J., Phielix, C., Jaspers, J., … Jarvenoja, H. (2015). Enhancing socially shared regulation in collaborative learning groups: Designing for CSCL regulation tools. Educational Technology Research and Development , 63 (1), 125e142.
Jones, M. E., Antonenko, P. D., & Greenwood, C. M. (2012). The impact of collaborative and individualized student response system strategies on learner motivation, metacognition, and knowledge transfer. Journal of Computer Assisted Learning , 28 (5), 477–487.
King, A. (1992). Facilitating elaborative learning through guided student-generated questioning. Educational Psychologist , 27 , 111–126.
Kirschner, P. A., Kreijns, K., Phielix, C., & Fransen, J. (2015). Awareness of cognitive and social behavior in a CSCL environment. Journal of Computer Assisted Learning , 31 (1), 59–77.
Knight, J. K., Wise, S. B., & Southard, K. M. (2013). Understanding clicker discussions: student reasoning and the impact of instructional cues. CBE-Life Sciences Education , 12 , 645–654.
Koriat, A. (2015). When two heads are better than one and when they can be worse: The amplification hypothesis. Journal of Experimental Psychology: General , 144 , 934–950. https://doi.org/10.1037/xge0000092 .
Kreijns, K., Kirschner, P. A., & Vermeulen, M. (2013). Social aspects of CSCL environments: A research framework. Educational Psychologist , 48 (4), 229e242.
Kuhn, L. M., & Sniezek, J. A. (1996). Confidence and uncertainty in judgmental forecasting: Differential effects of scenario presentation. Journal of Behavioral Decision Making , 9 , 231–247.
Lasry, N., Mazur, E., & Watkins, J. (2008). Peer instruction: From Harvard to the two-year college. American Journal of Physics , 76 (11), 1066–1069.
Laughlin, P. R. (2011). Group problem solving. Princeton: Princeton University Press.
Book Google Scholar
Laughlin, P. R., Bonner, B. L., & Miner, A. G. (2002). Groups perform better than individuals on letters-to-numbers problems. Organisational Behaviour and Human Decision Processes , 88 , 605–620.
Laughlin, P. R., & Ellis, A. L. (1986). Demonstrability and social combination processes on mathematical intellective tasks. Journal of Experimental Social Psychology, 22, 177–189.
Lucas, A. (2009). Using peer instruction and i-clickers to enhance student participation in calculus. Primus , 19 (3), 219–231.
Mazur, E. (1997). Peer instruction: A user’s manual . Upper Saddle River: Prentice Hall.
Mercier, H., & Sperber, D. (2019). The enigma of reason . Cambridge: Harvard University Press.
Metcalfe, J. (2017). Learning from errors. Annual Review of Psychology , 68 , 465–489.
Michaelsen, L. K., Watson, W. E., & Black, R. H. (1989). Realistic test of individual versus group decision making. Journal of Applied Psychology , 64 , 834–839.
Miller, R. L., Santana-Vega, E., & Terrell, M. S. (2007). Can good questions and peer discussion improve calculus instruction? Primus , 16 (3), 193–203.
Morgan, J. T., & Wakefield, C. (2012). Who benefits from peer conversation? Examining correlations of clicker question correctness and course performance. Journal of College Science Teaching , 41 (5), 51–56.
Moshman, D., & Geil, M. (1998). Collaborative reasoning: Evidence for collective rationality. Thinking and Reasoning, 4, 231–248.
Murayama, K., Sakaki, M., Yan, V. X., & Smith, G. M. (2014). Type I error inflation in the traditional by-participant analysis to metamemory accuracy: A generalized mixed-effects model perspective. Journal of Experimental Psychology: Learning, Memory, and Cognition , 40 , 1287–1306.
Newbury, P., & Heiner, C. (2012). Ready, set, react! getting the most out of peer instruction using clickers. Retrieved October 28, 2015, from http://www.cwsei.ubc.ca/Files/ReadySetReact_3fold.pdf .
Nielsen, K. L., Hansen-Nygård, G., & Stav, J. B. (2012). Investigating peer instruction: how the initial voting session affects students’ experiences of group discussion. ISRN Education , 2012 , article 290157.
Noddings, N. (1985). Small groups as a setting for research on mathematical problem solving. In E. A. Silver (Ed.), Teaching and learning mathematical problem solving , (pp. 345–360). Hillsdale: Erlbaum.
Perret-Clermont, A. N. (1980). Social Interaction and Cognitive Development in Children. London: Academic Press.
Perez, K. E., Strauss, E. A., Downey, N., Galbraith, A., Jeanne, R., Cooper, S., & Madison, W. (2010). Does displaying the class results affect student discussion during peer instruction? CBE Life Sciences Education , 9 , 133–140.
Phielix, C., Prins, F. J., & Kirschner, P. A. (2010). Awareness of group performance in a CSCL-environment: Effects of peer feedback and reflection. Computers in Human Behavior , 26 (2), 151–161.
Phielix, C., Prins, F. J., Kirschner, P. A., Erkens, G., & Jaspers, J. (2011). Group awareness of social and cognitive performance in a CSCL environment: Effects of a peer feedback and reflection tool. Computers in Human Behavior , 27 (3), 1087–1102.
Pollock, S. J., Chasteen, S. V., Dubson, M., & Perkins, K. K. (2010). The use of concept tests and peer instruction in upper-division physics. In M. Sabella, C. Singh, & S. Rebello (Eds.), AIP conference proceedings , (vol. 1289, p. 261). New York: AIP Press.
Porter, L., Bailey-Lee, C., & Simon, B. (2013). Halving fail rates using peer instruction: A study of four computer science courses. In SIGCSE ‘13: Proceedings of the 44th ACM technical symposium on computer science education , (pp. 177–182). New York: ACM Press.
Price, P. C., & Stone, E. R. (2004). Intuitive evaluation of likelihood judgment producers. Journal of Behavioral Decision Making , 17 , 39–57.
Priniski, J. H., & Horne, Z. (2019). Crowdsourcing effective educational interventions. In A. K. Goel, C. Seifert, & C. Freska (Eds.), Proceedings of the 41st annual conference of the cognitive science society . Austin: Cognitive Science Society.
Rao, S. P., & DiCarlo, S. E. (2000). Peer instruction improves performance on quizzes. Advances in Physiological Education , 24 , 51–55.
Renkl, A., Stark, R., Gruber, H., & Mandl, H. (1998). Learning from worked-out examples: The effects of example variability and elicited self-explanations. Contemporary Educational Psychology , 23 , 90–108.
Rittle-Johnson, B. (2006). Promoting transfer: Effects of self-explanation and direct instruction. Child Development , 77 , 1–15.
Roediger III, H. L., & Karpicke, J. D. (2006). Test-enhanced learning: Taking memory tests improves long-term retention. Psychological Science , 17 , 249–255.
Ryskin, R., Benjamin, A. S., Tullis, J. G., & Brown-Schmidt, S. (2015). Perspective-taking in comprehension, production, and memory: An individual differences approach. Journal of Experimental Psychology: General , 144 , 898–915.
Sah, S., Moore, D. A., & MacCoun, R. J. (2013). Cheap talk and credibility: The consequences of confidence and accuracy on advisor credibility and persuasiveness. Organizational Behavior and Human Decision Processes , 121 , 246–255.
Schwartz, D. L. (1995). The emergence of abstract representations in dyad problem solving. The Journal of the Learning Sciences , 4 , 321–354.
Simon, B., Kohanfars, M., Lee, J., Tamayo, K., & Cutts, Q. (2010). Experience report: peer instruction in introductory computing. In Proceedings of the 41st SIGCSE technical symposium on computer science education .
Smith, M. K., Wood, W. B., Adams, W. K., Wieman, C., Knight, J. K., Guild, N., & Su, T. T. (2009). Why peer discussion improves student performance on in-class concept questions. Science , 323 , 122–124.
Smith, M. K., Wood, W. B., Krauter, K., & Knight, J. K. (2011). Combining peer discussion with instructor explanation increases student learning from in-class concept questions. CBE-Life Sciences Education , 10 , 55–63.
Sniezek, J. A., & Buckley, T. (1995). Cueing and cognitive conflict in judge–Advisor decision making. Organizational Behavior and Human Decision Processes , 62 , 159–174.
Sniezek, J. A., & Henry, R. A. (1989). Accuracy and confidence in group judgment. Organizational Behavior and Human Decision Processes , 43 , 1–28.
Son, L. K., & Metcalfe, J. (2000). Metacognitive and control strategies in study-time allocation. Journal of Experimental Psychology: Learning, Memory, and Cognition , 26 , 204–221.
Steiner, I. D. (1972). Group processes and productivity . New York: Academic Press.
Thurlings, M., Vermeulen, M., Bastiaens, T., & Stijnen, S. (2013). Understanding feedback: A learning theory perspective. Educational Research Review , 9 , 1–15.
Tindale, R. S., & Sheffey, S. (2002). Shared information, cognitive load, and group memory. Group Processes & Intergroup Relations , 5 (1), 5–18.
Trouche, E., Sander, E., & Mercier, H. (2014). Arguments, more than confidence, explain the good performance of reasoning groups. Journal of Experimental Psychology: General , 143 , 1958–1971.
Tullis, J. G. (2018). Predicting others’ knowledge: Knowledge estimation as cue-utilization. Memory & Cognition , 46 , 1360–1375.
Tullis, J. G., Fiechter, J. L., & Benjamin, A. S. (2018). The efficacy of learners’ testing choices. Journal of Experimental Psychology: Learning, Memory, and Cognition , 44 , 540–552.
Tullis, J. G., & Fraundorf, S. H. (2017). Predicting others’ memory performance: The accuracy and bases of social metacognition. Journal of Memory and Language , 95 , 124–137.
Turpen, C., & Finkelstein, N. (2007). Understanding how physics faculty use peer instruction. In L. Hsu, C. Henderson, & L. McCullough (Eds.), Physics education research conference , (pp. 204–209). College Park: American Institute of Physics.
Van Swol, L. M., & Sniezek, J. A. (2005). Factors affecting the acceptance of expert advice. British Journal of Social Psychology , 44 , 443–461.
VanLehn, K., Jones, R. M., & Chi, M. T. H. (1992). A model of the self-explanation effect. Journal of the Learning Sciences , 2 (1), 1–59.
Vedder, P. (1985). Cooperative learning: A study on processes and effects of cooperation between primary school children . Westerhaven: Rijkuniversiteit Groningen.
Versteeg, M., van Blankenstein, F. M., Putter, H., & Steendijk, P. (2019). Peer instruction improves comprehension and transfer of physiological concepts: A randomized comparison with self-explanation. Advances in Health Sciences Education , 24 , 151–165.
Vygotsky, L. S. (1981). The genesis of higher mental functioning. In J. V. Wertsch (Ed.), The concept of activity in Soviet psychology , (pp. 144–188). Armonk: Sharpe.
Webb, N. M., & Palincsar, A. S. (1996). Group processes in the classroom. In D. C. Berliner, & R. C. Calfee (Eds.), Handbook of educational psychology , (pp. 841–873). New York: Macmillan Library Reference USA: London: Prentice Hall International.
Wegner, D. M., Giuliano, T., & Hertel, P. (1985). Cognitive interdependence in close relationships. In W. J. Ickes (Ed.), Compatible and incompatible relationships , (pp. 253–276). New York: Springer-Verlag.
Chapter Google Scholar
Weldon, M. S., & Bellinger, K. D. (1997). Collective memory: Collaborative and individual processes in remembering. Journal of Experimental Psychology: Learning, Memory, and Cognition , 23 , 1160–1175.
Wieman, C., Perkins, K., Gilbert, S., Benay, F., Kennedy, S., Semsar, K., et al. (2009). Clicker resource guide: An instructor’s guide to the effective use of personalresponse systems (clickers) in teaching . Vancouver: University of British Columbia Available from http://www.cwsei.ubc.ca/resources/files/Clicker_guide_CWSEI_CU-SEI.pdf .
Wong, R. M. F., Lawson, M. J., & Keeves, J. (2002). The effects of self-explanation training on students’ problem solving in high school mathematics. Learning and Instruction , 12 , 23.
Yackel, E., Cobb, P., & Wood, T. (1991). Small-group interactions as a source of learning opportunities in second-grade mathematics. Journal for Research in Mathematics Education , 22 , 390–408.
Yaniv, I. (2004a). The benefit of additional opinions. Current Directions in Psychological Science , 13 , 75–78.
Yaniv, I. (2004b). Receiving other people’s advice: Influence and benefit. Organizational Behavior and Human Decision Processes , 93 , 1–13.
Yaniv, I., & Choshen-Hillel, S. (2012). Exploiting the wisdom of others to make better decisions: Suspending judgment reduces egocentrism and increases accuracy. Journal of Behavioral Decision Making , 25 , 427–434.
Yaniv, I., & Kleinberger, E. (2000). Advice taking in decision making: Egocentric discounting and reputation formation. Organizational Behavior and Human Decision Processes , 83 , 260–281.
Download references
Acknowledgements
Not applicable.
No funding supported this manuscript.
Author information
Authors and affiliations.
Department of Educational Psychology, University of Arizona, 1430 E. Second St., Tucson, AZ, 85721, USA
Jonathan G. Tullis
Department of Psychology, Indiana University, Bloomington, IN, USA
Robert L. Goldstone
You can also search for this author in PubMed Google Scholar
Contributions
JGT collected some data, analyzed the data, and wrote the first draft of the paper. RLG collected some data, contributed significantly to the framing of the paper, and edited the paper. The authors read and approved the final manuscript.
Authors’ information
JGT: Assistant Professor in Educational Psychology at University of Arizona. RLG: Chancellor’s Professor in Psychology at Indiana University.
Corresponding author
Correspondence to Jonathan G. Tullis .
Ethics declarations
Ethics approval and consent to participate.
The ethics approval was waived by the Indiana University Institutional Review Board (IRB) and the University of Arizona IRB, given that these data are collected as part of normal educational settings and processes.
Consent for publication
No individual data are presented in the manuscript.
Competing interests
The authors declare that they have no competing interests.
Additional information
Publisher’s note.
Springer Nature remains neutral with regard to jurisdictional claims in published maps and institutional affiliations.
Rights and permissions
Open Access This article is licensed under a Creative Commons Attribution 4.0 International License, which permits use, sharing, adaptation, distribution and reproduction in any medium or format, as long as you give appropriate credit to the original author(s) and the source, provide a link to the Creative Commons licence, and indicate if changes were made. The images or other third party material in this article are included in the article's Creative Commons licence, unless indicated otherwise in a credit line to the material. If material is not included in the article's Creative Commons licence and your intended use is not permitted by statutory regulation or exceeds the permitted use, you will need to obtain permission directly from the copyright holder. To view a copy of this licence, visit http://creativecommons.org/licenses/by/4.0/ .
Reprints and permissions
About this article
Cite this article.
Tullis, J.G., Goldstone, R.L. Why does peer instruction benefit student learning?. Cogn. Research 5 , 15 (2020). https://doi.org/10.1186/s41235-020-00218-5
Download citation
Received : 08 October 2019
Accepted : 25 February 2020
Published : 09 April 2020
DOI : https://doi.org/10.1186/s41235-020-00218-5
Share this article
Anyone you share the following link with will be able to read this content:
Sorry, a shareable link is not currently available for this article.
Provided by the Springer Nature SharedIt content-sharing initiative
- Group decisions
- Peer instruction
- Metacognition
- Decision making
You are using an outdated browser. Please upgrade your browser to improve your experience.

Health & Nursing
Courses and certificates.
- Bachelor's Degrees
- View all Business Bachelor's Degrees
- Business Management – B.S. Business Administration
- Healthcare Administration – B.S.
- Human Resource Management – B.S. Business Administration
- Information Technology Management – B.S. Business Administration
- Marketing – B.S. Business Administration
- Accounting – B.S. Business Administration
- Finance – B.S.
- Supply Chain and Operations Management – B.S.
- Accelerated Information Technology Bachelor's and Master's Degree (from the School of Technology)
- Health Information Management – B.S. (from the Leavitt School of Health)
Master's Degrees
- View all Business Master's Degrees
- Master of Business Administration (MBA)
- MBA Information Technology Management
- MBA Healthcare Management
- Management and Leadership – M.S.
- Accounting – M.S.
- Marketing – M.S.
- Human Resource Management – M.S.
- Master of Healthcare Administration (from the Leavitt School of Health)
- Data Analytics – M.S. (from the School of Technology)
- Information Technology Management – M.S. (from the School of Technology)
- Education Technology and Instructional Design – M.Ed. (from the School of Education)
Certificates
- Supply Chain
- Accounting Fundamentals
- View all Business Degrees
Bachelor's Preparing For Licensure
- View all Education Bachelor's Degrees
- Elementary Education – B.A.
- Special Education and Elementary Education (Dual Licensure) – B.A.
- Special Education (Mild-to-Moderate) – B.A.
- Mathematics Education (Middle Grades) – B.S.
- Mathematics Education (Secondary)– B.S.
- Science Education (Middle Grades) – B.S.
- Science Education (Secondary Chemistry) – B.S.
- Science Education (Secondary Physics) – B.S.
- Science Education (Secondary Biological Sciences) – B.S.
- Science Education (Secondary Earth Science)– B.S.
- View all Education Degrees
Bachelor of Arts in Education Degrees
- Educational Studies – B.A.
Master of Science in Education Degrees
- View all Education Master's Degrees
- Curriculum and Instruction – M.S.
- Educational Leadership – M.S.
- Education Technology and Instructional Design – M.Ed.
Master's Preparing for Licensure
- Teaching, Elementary Education – M.A.
- Teaching, English Education (Secondary) – M.A.
- Teaching, Mathematics Education (Middle Grades) – M.A.
- Teaching, Mathematics Education (Secondary) – M.A.
- Teaching, Science Education (Secondary) – M.A.
- Teaching, Special Education (K-12) – M.A.
Licensure Information
- State Teaching Licensure Information
Master's Degrees for Teachers
- Mathematics Education (K-6) – M.A.
- Mathematics Education (Middle Grade) – M.A.
- Mathematics Education (Secondary) – M.A.
- English Language Learning (PreK-12) – M.A.
- Endorsement Preparation Program, English Language Learning (PreK-12)
- Science Education (Middle Grades) – M.A.
- Science Education (Secondary Chemistry) – M.A.
- Science Education (Secondary Physics) – M.A.
- Science Education (Secondary Biological Sciences) – M.A.
- Science Education (Secondary Earth Science)– M.A.
- View all Technology Bachelor's Degrees
- Cloud Computing – B.S.
- Computer Science – B.S.
- Cybersecurity and Information Assurance – B.S.
- Data Analytics – B.S.
- Information Technology – B.S.
- Network Engineering and Security – B.S.
- Software Engineering – B.S.
- Accelerated Information Technology Bachelor's and Master's Degree
- Information Technology Management – B.S. Business Administration (from the School of Business)
- View all Technology Master's Degrees
- Cybersecurity and Information Assurance – M.S.
- Data Analytics – M.S.
- Information Technology Management – M.S.
- MBA Information Technology Management (from the School of Business)
- Full Stack Engineering
- Web Application Deployment and Support
- Front End Web Development
- Back End Web Development
3rd Party Certifications
- IT Certifications Included in WGU Degrees
- View all Technology Degrees
- View all Health & Nursing Bachelor's Degrees
- Nursing (RN-to-BSN online) – B.S.
- Nursing (Prelicensure) – B.S. (Available in select states)
- Health Information Management – B.S.
- Health and Human Services – B.S.
- Psychology – B.S.
- Health Science – B.S.
- Healthcare Administration – B.S. (from the School of Business)
- View all Nursing Post-Master's Certificates
- Nursing Education—Post-Master's Certificate
- Nursing Leadership and Management—Post-Master's Certificate
- Family Nurse Practitioner—Post-Master's Certificate
- Psychiatric Mental Health Nurse Practitioner —Post-Master's Certificate
- View all Health & Nursing Degrees
- View all Nursing & Health Master's Degrees
- Nursing – Education (BSN-to-MSN Program) – M.S.
- Nursing – Leadership and Management (BSN-to-MSN Program) – M.S.
- Nursing – Nursing Informatics (BSN-to-MSN Program) – M.S.
- Nursing – Family Nurse Practitioner (BSN-to-MSN Program) – M.S. (Available in select states)
- Nursing – Psychiatric Mental Health Nurse Practitioner (BSN-to-MSN Program) – M.S. (Available in select states)
- Nursing – Education (RN-to-MSN Program) – M.S.
- Nursing – Leadership and Management (RN-to-MSN Program) – M.S.
- Nursing – Nursing Informatics (RN-to-MSN Program) – M.S.
- Master of Healthcare Administration
- Master of Public Health
- MBA Healthcare Management (from the School of Business)
- Business Leadership (with the School of Business)
- Supply Chain (with the School of Business)
- Accounting Fundamentals (with the School of Business)
- Back End Web Development (with the School of Technology)
- Front End Web Development (with the School of Technology)
- Web Application Deployment and Support (with the School of Technology)
- Full Stack Engineering (with the School of Technology)
- Single Courses
- Course Bundles
Apply for Admission
Admission requirements.
- New Students
- WGU Returning Graduates
- WGU Readmission
- Enrollment Checklist
- Accessibility
- Accommodation Request
- School of Education Admission Requirements
- School of Business Admission Requirements
- School of Technology Admission Requirements
- Leavitt School of Health Admission Requirements
Additional Requirements
- Computer Requirements
- No Standardized Testing
- Clinical and Student Teaching Information
Transferring
- FAQs about Transferring
- Transfer to WGU
- Transferrable Certifications
- Request WGU Transcripts
- International Transfer Credit
- Tuition and Fees
- Financial Aid
- Scholarships
Other Ways to Pay for School
- Tuition—School of Business
- Tuition—School of Education
- Tuition—School of Technology
- Tuition—Leavitt School of Health
- Your Financial Obligations
- Tuition Comparison
- Applying for Financial Aid
- State Grants
- Consumer Information Guide
- Responsible Borrowing Initiative
- Higher Education Relief Fund
FAFSA Support
- Net Price Calculator
- FAFSA Simplification
- See All Scholarships
- Military Scholarships
- State Scholarships
- Scholarship FAQs
Payment Options
- Payment Plans
- Corporate Reimbursement
- Current Student Hardship Assistance
- Military Tuition Assistance
WGU Experience
- How You'll Learn
- Scheduling/Assessments
- Accreditation
- Student Support/Faculty
- Military Students
- Part-Time Options
- Virtual Military Education Resource Center
- Student Outcomes
- Return on Investment
- Students and Gradutes
- Career Growth
- Student Resources
- Communities
- Testimonials
- Career Guides
- Skills Guides
- Online Degrees
- All Degrees
- Explore Your Options
Admissions & Transfers
- Admissions Overview
Tuition & Financial Aid
Student Success
- Prospective Students
- Current Students
- Military and Veterans
- Commencement
- Careers at WGU
- Advancement & Giving
- Partnering with WGU
Peer Learning: Overview, Benefits, and Models
- Classroom Strategies
- See More Tags
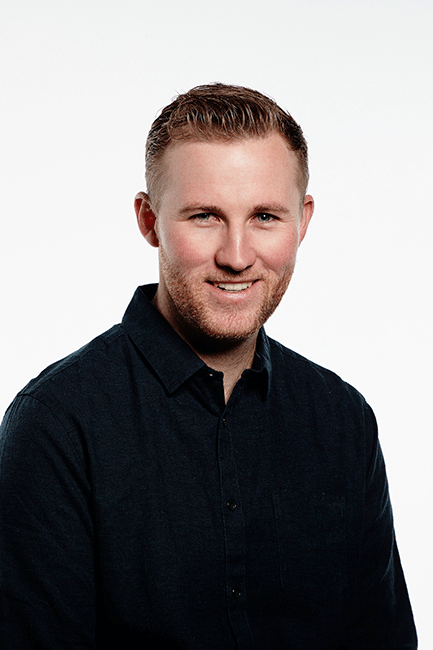
How do K-12 teachers facilitate effective learning? The best teachers do more than just read from a textbook. They understand that there are many different techniques, theories, and teaching models that can give students a well-rounded education that’s foundational to a lifetime of success and continual improvement.
Effective learning happens in many ways. Some students learn well directly from a teacher. Others are skilled independent learners. Yet, one of the most effective active learning techniques is that of peer learning. Put simply, peer learning is when students teach each other. This type of learning aids retention and encourages communication and collaboration.
Learn more about peer learning and how a teaching degree from WGU can prepare you to make a difference in the classroom.
What Is Peer Learning?
Peer learning is an education method that helps students solidify their knowledge by teaching each other. One student tutoring another in a supervised environment can result in better learning and retention. Why? Because to teach another, one must first fully understand a concept themselves. Verbalizing a concept and sharing the information with a peer serves to reinforce the knowledge gained.
Peer learning is best supported by other learning strategies, including the Constructivism Learning Theory and the Connectivism Learning Theory .
Constructivist learning suggests that knowledge is constructed by each individual student. The new concepts they learn are built upon their existing knowledge and beliefs. Constructivism also proposes that learning is an active process and a social activity. These concepts tie in well with peer learning.
Next, there’s Connectivism. Introduced in 2005 by George Siemens, the Connectivism Learning Theory focuses on technology as a critical component of connected learning. Today’s social networks allow rapid information transfer, but not every piece of information is equally helpful or enriching. Siemens suggests that being able to distinguish between important and unimportant information is vital. Even young students today are connected to the world and to each other through online means. An understanding of connectivism is especially helpful for K-12 teachers in the digital age.
Why Is Peer Learning Important?
To thrive in school, in the workplace, and in society, individuals must be able to learn from others and work with them to achieve mutual success. Below are even more reasons why peer learning is important.
Teamwork: Peer learning fosters teamwork, cooperation, patience, and better social skills. In a cooperative peer learning environment, each student’s strengths can serve to complement the group and enhance learning. Becoming skilled at working with and learning from one's peers can start at a young age in the classroom.
Better Feedback : Often, students are not able to recognize the gaps in their own knowledge. But when they learn with their peers, they can see new processes for answering questions and come up with creative, collaborative solutions. Importantly, they will carry these new perspectives, as well as a willingness to seek and accept feedback, with them as they progress in their education.
Supports Diversity: Peer learning fosters diversity and depth in a student’s knowledge and opinions. Learning from peers of different backgrounds, views, and ethnicities fosters an environment of mutual respect, gratitude, and progress. It’s the differences between students that add a richness to the learning environment. Supporting diversity through peer learning is part of culturally responsive teaching .
What Are the Benefits of Peer Learning?
It’s hard to number all the benefits of peer learning, but some of them include new perspectives, more social interaction, and deepened personal learning. See more information on these specific areas below.
New Perspectives for Students: If a student learns exclusively from the teacher, they may only gain one new perspective. Learning from their peers can add numerous helpful perspectives, nuances, and layers to a student’s knowledge.
Social Interaction Makes Studying Fun: By nature, humans are social beings. We long to make connections and be part of a group. The added element of social interaction in peer learning can be exciting and enriching. Students who may be hesitant to interact with the teacher may be more willing to open up to their peers.
Teaching Others Helps Students Learn: Nothing requires you to feel confident in your own knowledge quite like teaching what you know to someone else. As mentioned, peer learning can help students learn and solidify their own knowledge. Effective teaching requires a deeper level of knowledge on a subject.
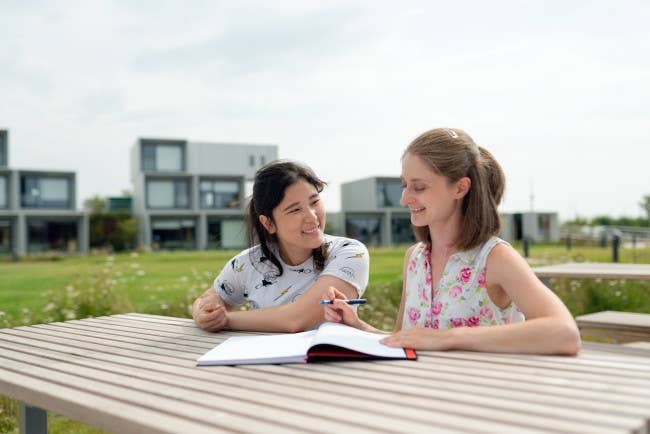
Peer Learning Drawbacks
While there are many benefits to peer learning, there are also some drawbacks, including distraction and lack of respect for feedback.
Working in Groups Can Be Distracting: Learning from your peers can be exciting. However, especially for younger students, that excitement can lead to distraction. When working with their friends, some students can easily get off track, misbehave, and focus on anything but learning.
Students Might Not Respect the Feedback of Their Peers: If a teacher gives feedback, the student is more likely to listen carefully. After all, the teacher is the authority in the classroom and the resident expert on the subject being taught. On the other hand, if one’s peer gives them feedback, it’s easier to disregard it.
Peer Learning Models
Effective peer learning can take place through many different models and strategies. See some of the tried-and-true ways to encourage peer learning.
Proctor Model: In the proctor model, an older or more experienced student teaches a younger or less experienced peer. In an elementary school, this might mean that students from a higher grade level come and teach kindergarteners. It could also entail having a more skilled student within the class teach their classmate.
Discussion Seminars: Discussion seminars are more common at the university level. They’re often held after students learn the material through a lecture or a weekly reading. Through these discussions, students deepen their knowledge and gain additional perspectives.
Peer Support Groups: Sometimes referred to as private study groups, peer support groups are student-led gatherings that are generally held outside of class without teacher support. Peers might meet up to study for a test together or complete a group project.
Peer Assessment Schemes: Peer assessment schemes can be common in writing courses. For instance, an AP English Language teacher might have students read one another’s essays to provide informal feedback.
Collaborative Projects: Assigning students to work on collaborative projects can serve them well for their future endeavors in the workplace and society. These projects teach collaboration, the importance of combining skills, and the need to meet deadlines.
Cascading Groups: Cascading groups is a learning method by which students are split into groups that get either progressively larger or smaller. For instance, students might be encouraged to learn about a distinct topic on their own and then share it with a partner. That partnership would then share their knowledge with another partnership and so forth.
Mentoring: A mentor is someone who has experience in a certain area. They guide a student, training them and teaching them the lessons they once had to learn. Peer tutoring is a form of mentoring. Sometimes students who require extra support are assigned a personal peer mentor who works one-on-one with them to help them succeed.
Reciprocal Teaching: In reciprocal teaching, students must develop the skills of questioning, predicting, summarizing, and clarifying. They teach one another using these techniques. They serve to form a sort of scaffolding for peer-led learning.
Jigsaw Method: In the jigsaw method of peer learning, students are split into groups, with each group given a different topic to study. Then, one student from each group is taken to form a collaborative group where multiple concepts are discussed. If there are eight jigsaw groups, then eight topics will ultimately be discussed in one group.
Discover More Learning Models with WGU
Peer learning is an effective way to facilitate deep learning. It also lends itself to many different approaches. The power of a classroom where students come together is that of collaborative learning. Teachers who implement peer learning strategies in their classroom may see higher levels of student performance, satisfaction, and overall engagement.
If you’re ready to learn new teaching methods and prepare to make a difference in the classroom, check out the WGU School of Education . The programs help teachers learn up-to-date teaching methods for the modern learning environment.
Ready to Start Your Journey?
HEALTH & NURSING
Recommended Articles
Take a look at other articles from WGU. Our articles feature information on a wide variety of subjects, written with the help of subject matter experts and researchers who are well-versed in their industries. This allows us to provide articles with interesting, relevant, and accurate information.
{{item.date}}
{{item.preTitleTag}}
{{item.title}}
The university, for students.
- Student Portal
- Alumni Services
Most Visited Links
- Business Programs
- Student Experience
- Diversity, Equity, and Inclusion
- Student Communities

An official website of the United States government
The .gov means it’s official. Federal government websites often end in .gov or .mil. Before sharing sensitive information, make sure you’re on a federal government site.
The site is secure. The https:// ensures that you are connecting to the official website and that any information you provide is encrypted and transmitted securely.
- Publications
- Account settings
Preview improvements coming to the PMC website in October 2024. Learn More or Try it out now .
- Advanced Search
- Journal List
- Cogn Res Princ Implic
- v.5; 2020 Dec
Why does peer instruction benefit student learning?
Jonathan g. tullis.
1 Department of Educational Psychology, University of Arizona, 1430 E. Second St., Tucson, AZ 85721 USA
Robert L. Goldstone
2 Department of Psychology, Indiana University, Bloomington, IN USA
Associated Data
As described below, data and materials are available on the OpenScienceFramework: https://mfr.osf.io/render?url=https://osf.io/5qc46/?action=download%26mode=render .
In peer instruction, instructors pose a challenging question to students, students answer the question individually, students work with a partner in the class to discuss their answers, and finally students answer the question again. A large body of evidence shows that peer instruction benefits student learning. To determine the mechanism for these benefits, we collected semester-long data from six classes, involving a total of 208 undergraduate students being asked a total of 86 different questions related to their course content. For each question, students chose their answer individually, reported their confidence, discussed their answers with their partner, and then indicated their possibly revised answer and confidence again. Overall, students were more accurate and confident after discussion than before. Initially correct students were more likely to keep their answers than initially incorrect students, and this tendency was partially but not completely attributable to differences in confidence. We discuss the benefits of peer instruction in terms of differences in the coherence of explanations, social learning, and the contextual factors that influence confidence and accuracy.
Significance
Peer instruction is widely used in physics instruction across many universities. Here, we examine how peer instruction, or discussing one’s answer with a peer, affects students’ decisions about a class assignment. Across six different university classes, students answered a question, discussed their answer with a peer, and finally answered the question again. Students’ accuracy consistently improved through discussion with a peer. Our peer instruction data show that students were hesitant to switch away from their initial answer and that students did consider both their own confidence and their partner’s confidence when making their final decision, in accord with basic research about confidence in decision making. More broadly, the data reveal that peer discussion helped students select the correct answer by prompting them to create new knowledge. The benefit to student accuracy that arises when students discuss their answers with a partner is a “process gain”, in which working in a group yields better performance than can be predicted from individuals’ performance alone.
Peer instruction is specific evidence-based instructional strategy that is well-known and widely used, particularly in physics (Henderson & Dancy, 2009 ). In fact, peer instruction has been advocated as a part of best methods in science classrooms (Beatty, Gerace, Leonard, & Dufresne, 2006 ; Caldwell, 2007 ; Crouch & Mazur, 2001 ; Newbury & Heiner, 2012 ; Wieman et al., 2009 ) and over a quarter of university physics professors report using peer instruction (Henderson & Dancy, 2009 ). In peer instruction, instructors pose a challenging question to students, students answer the question individually, students discuss their answers with a peer in the class, and finally students answer the question again. There are variations of peer instruction in which instructors show the class’s distribution of answers before discussion (Nielsen, Hansen-Nygård, & Stav, 2012 ; Perez et al., 2010 ), in which students’ answers are graded for participation or for correctness (James, 2006 ), and in which instructors’ norms affect whether peer instruction offers opportunities for answer-seeking or for sense-making (Turpen & Finkelstein, 2007 ).
Despite wide variations in its implementation, peer instruction consistently benefits student learning. Switching classroom structure from didactic lectures to one centered around peer instruction improves learners’ conceptual understanding (Duncan, 2005 ; Mazur, 1997 ), reduces student attrition in difficult courses (Lasry, Mazur, & Watkins, 2008 ), decreases failure rates (Porter, Bailey-Lee, & Simon, 2013 ), improves student attendance (Deslauriers, Schelew, & Wieman, 2011 ), and bolsters student engagement (Lucas, 2009 ) and attitudes to their course (Beekes, 2006 ). Benefits of peer instruction have been found across many fields, including physics (Mazur, 1997 ; Pollock, Chasteen, Dubson, & Perkins, 2010 ), biology (Knight, Wise, & Southard, 2013 ; Smith, Wood, Krauter, & Knight, 2011 ), chemistry (Brooks & Koretsky, 2011 ), physiology (Cortright, Collins, & DiCarlo, 2005 ; Rao & DiCarlo, 2000 ), calculus (Lucas, 2009 ; Miller, Santana-Vega, & Terrell, 2007 ), computer science (Porter et al., 2013 ), entomology (Jones, Antonenko, & Greenwood, 2012 ), and even philosophy (Butchart, Handfield, & Restall, 2009 ). Additionally, benefits of peer instruction have been found at prestigious private universities, two-year community colleges (Lasry et al., 2008 ), and even high schools (Cummings & Roberts, 2008 ). Peer instruction benefits not just the specific questions posed during discussion, but also improves accuracy on later similar problems (e.g., Smith et al., 2009 ).
One of the consistent empirical hallmarks of peer instruction is that students’ answers are more frequently correct following discussion than preceding it. For example, in introductory computer science courses, post-discussion performance was higher on 70 out of 71 questions throughout the semester (Simon, Kohanfars, Lee, Tamayo, & Cutts, 2010 ). Further, gains in performance from discussion are found on many different types of questions, including recall, application, and synthesis questions (Rao & DiCarlo, 2000 ). Performance improvements are found because students are more likely to switch from an incorrect answer to the correct answer than from the correct answer to an incorrect answer. In physics, 59% of incorrect answers switched to correct following discussion, but only 13% of correct answers switched to incorrect (Crouch & Mazur, 2001 ). Other research on peer instruction shows the same patterns: 41% of incorrect answers are switched to correct ones, while only 18% of correct answers are switched to incorrect (Morgan & Wakefield, 2012 ). On qualitative problem-solving questions in physiology, 57% of incorrect answers switched to correct after discussion, and only 7% of correct answers to incorrect (Giuliodori, Lujan, & DiCarlo, 2006 ).
There are two explanations for improvements in pre-discussion to post-discussion accuracy. First, switches from incorrect to correct answers may be driven by selecting the answer from the peer who is more confident. When students discuss answers that disagree, they may choose whichever answer belongs to the more confident peer. Evidence about decision-making and advice-taking substantiates this account. First, confidence is correlated with correctness across many settings and procedures (Finley, Tullis, & Benjamin, 2010 ). Students who are more confident in their answers are typically more likely to be correct. Second, research examining decision-making and advice-taking indicates that (1) the less confident you are, the more you value others’ opinions (Granovskiy, Gold, Sumpter, & Goldstone, 2015 ; Harvey & Fischer, 1997 ; Yaniv, 2004a , 2004b ; Yaniv & Choshen-Hillel, 2012 ) and (2) the more confident the advisor is, the more strongly they influence your decision (Kuhn & Sniezek, 1996 ; Price & Stone, 2004 ; Sah, Moore, & MacCoun, 2013 ; Sniezek & Buckley, 1995 ; Van Swol & Sniezek, 2005 ; Yaniv, 2004b ). Consequently, if students simply choose their final answer based upon whoever is more confident, accuracy should increase from pre-discussion to post-discussion. This explanation suggests that switches in answers should be driven entirely by a combination of one’s own initial confidence and one’s partner’s confidence. In accord with this confidence view, Koriat ( 2015 ) shows that an individual’s confidence typically reflects the group’s most typically given answer. When the answer most often given by group members is incorrect, peer interactions amplify the selection of and confidence in incorrect answers. Correct answers have no special draw. Rather, peer instruction merely amplifies the dominant view through differences in the individual’s confidence.
In a second explanation, working with others may prompt students to verbalize explanations and verbalizations may generate new knowledge. More specifically, as students discuss the questions, they need to create a common representation of the problem and answer. Generating a common representation may compel students to identify gaps in their existing knowledge and construct new knowledge (Schwartz, 1995 ). Further, peer discussion may promote students’ metacognitive processes of detecting and correcting errors in their mental models. Students create more new knowledge and better diagnostic tests of answers together than alone. Ultimately, then, the new knowledge and improved metacognition may make the correct answer appear more compelling or coherent than incorrect options. Peer discussion would draw attention to coherent or compelling answers, more so than students’ initial confidence alone and the coherence of the correct answer would prompt students to switch away from incorrect answers. Similarly, Trouche, Sander, and Mercier ( 2014 ) argue that interactions in a group prompt argumentation and discussion of reasoning. Good arguments and reasoning should be more compelling to change individuals’ answers than confidence alone. Indeed, in a reasoning task known to benefit from careful deliberation, good arguments and the correctness of the answers change partners’ minds more than confidence in one’s answer (Trouche et al., 2014 ). This explanation predicts several distinct patterns of data. First, as seen in prior research, more students should switch from incorrect answers to correct than vice versa. Second, the intrinsic coherence of the correct answer should attract students, so the likelihood of switching answers would be predicted by the correctness of an answer above and beyond differences in initial confidence. Third, initial confidence in an answer should not be as tightly related to initial accuracy as final confidence is to final accuracy because peer discussion should provide a strong test of the coherence of students’ answers. Fourth, because the coherence of an answer is revealed through peer discussion, student confidence should increase more from pre-discussion to post-discussion when they agree on the correct answers compared to agreeing on incorrect answers.
Here, we examined the predictions of these two explanations of peer instruction across six different classes. We specifically examined whether changes in answers are driven exclusively through the confidence of the peers during discussion or whether the coherence of an answer is better constructed and revealed through peer instruction than on one’s own. We are interested in analyzing cognitive processes at work in a specific, but common, implementation of classroom-based peer instruction; we do not intend to make general claims about all kinds of peer instruction or to evaluate the long-term effectiveness of peer instruction. This research is the first to analyze how confidence in one’s answer relates to answer-switching during peer instruction and tests the impact of peer instruction in new domains (i.e., psychology and educational psychology classes).
Participants
Students in six different classes participated as part of their normal class procedures. More details about these classes are presented in Table 1 . The authors served as instructors for these classes. Across the six classes, 208 students contributed a total of 1657 full responses to 86 different questions.
Descriptions of classes used
The instructors of the courses developed multiple-choice questions related to the ongoing course content. Questions were aimed at testing students’ conceptual understanding, rather than factual knowledge. Consequently, questions often tested whether students could apply ideas to new settings or contexts. An example of a cognitive psychology question used is: Which is a fixed action pattern (not a reflex)?
- Knee jerks up when patella is hit
- Male bowerbirds building elaborate nests [correct]
- Eye blinks when air is blown on it
- Can play well learned song on guitar even when in conversation
The procedures for peer instruction across the six different classes followed similar patterns. Students were presented with a multiple-choice question. First, students read the question on their own, chose their answer, and reported their confidence in their answer on a scale of 1 “Not at all confident” to 10 “Highly confident”. Students then paired up with a neighbor in their class and discussed the question with their peer. After discussion, students answered the question and reported the confidence for a second time. The course instructor indicated the correct answer and discussed the reasoning for the answer after all final answers had been submitted. Instruction was paced based upon how quickly students read and answered questions. Most student responses counted towards their participation grade, regardless of the correctness of their answer (the last question in each of the cognitive psychology classes was graded for correctness).
There were small differences in procedures between classes. Students in the cognitive psychology classes input their responses using classroom clickers, but those in other classes wrote their responses on paper. Further, students in the cognitive psychology classes explicitly reported their partner’s answer and confidence, while students in other classes only reported the name of their partner (the partners’ data were aligned during data recording). The cognitive psychology students then were required to mention their own answer and their confidence to their partner during peer instruction; students in other classes were not required to tell their answer or their confidence to their peer. Finally, the questions appeared at any point during the class period for the cognitive psychology classes, while the questions typically happened at the beginning of each class for the other classes.
Analytic strategy
Data are available on the OpenScienceFramework: https://mfr.osf.io/render?url=https://osf.io/5qc46/?action=download%26mode=render .
For most of our analyses we used linear mixed-effects models (Baayen, Davidson, & Bates, 2008 ; Murayama, Sakaki, Yan, & Smith, 2014 ). The unit of analysis in a mixed-effect model is the outcome of a single trial (e.g., whether or not a particular question was answered correctly by a particular participant). We modeled these individual trial-level outcomes as a function of multiple fixed effects - those of theoretical interest - and multiple random effects - effects for which the observed levels are sampled out of a larger population (e.g., questions, students, and classes sampled out of a population of potential questions, students, and classes).
Linear mixed-effects models solve four statistical problems involved with the data of peer instruction. First, there is large variability in students’ performance and the difficulty of questions across students and classes. Mixed-effect models simultaneously account for random variation both across participants and across items (Baayen et al., 2008 ; Murayama et al., 2014 ). Second, students may miss individual classes and therefore may not provide data across every item. Similarly, classes varied in how many peer instruction questions were posed throughout the semester and the number of students enrolled. Mixed-effects models weight each response equally when drawing conclusions (rather than weighting each student or question equally) and can easily accommodate missing data. Third, we were interested in how several different characteristics influenced students’ performance. Mixed effects models can include multiple predictors simultaneously, which allows us to test the effect of one predictor while controlling for others. Finally, mixed effects models can predict the log odds (or logit) of a correct answer, which is needed when examining binary outcomes (i.e., correct or incorrect; Jaeger, 2008 ).
We fit all models in R using the lmer() function of the lme4 package (Bates, Maechler, Bolker, & Walker, 2015 ). For each mixed-effect model, we included random intercepts that capture baseline differences in difficulty of questions, in classes, and in students, in addition to multiple fixed effects of theoretical interest. In mixed-effect models with hundreds of observations, the t distribution effectively converges to the normal, so we compared the t statistic to the normal distribution for analyses involving continuous outcomes (i.e., confidence; Baayen, 2008 ). P values can be directly obtained from Wald z statistics for models with binary outcomes (i.e., correctness).
Does accuracy change through discussion?
First, we examined how correctness changed across peer discussion. A logit model predicting correctness from time point (pre-discussion to post-discussion) revealed that the odds of correctness increased by 1.57 times (95% confidence interval (conf) 1.31–1.87) from pre-discussion to post-discussion, as shown in Table 2 . In fact, 88% of students showed an increase or no change in accuracy from pre-discussion to post-discussion. Pre-discussion to post-discussion performance for each class is shown in Table 3 . We further examined how accuracy changed from pre-discussion to post-discussion for each question and the results are plotted in Fig. 1 . The data show a consistent improvement in accuracy from pre-discussion to post-discussion across all levels of initial difficulty.
The effect of time point (pre-discussion to post-discussion) on accuracy using a mixed effect logit model
Accuracy before and after discussion by class

The relationship between pre-discussion accuracy (x axis) and post-discussion accuracy (y axis). Each point represents a single question. The solid diagonal line represents equal pre-discussion and post-discussion accuracy; points above the line indicate improvements in accuracy and points below represent decrements in accuracy. The dashed line indicates the line of best fit for the observed data
We examined how performance increased from pre-discussion to post-discussion by tracing the correctness of answers through the discussion. Figure 2 tracks the percent (and number of items) correct from pre-discussion to post-discussion. The top row shows whether students were initially correct or incorrect in their answer; the middle row shows whether students agreed or disagreed with their partner; the last row show whether students were correct or incorrect after discussion. Additionally, Fig. Fig.2 2 shows the confidence associated with each pathway. The bottow line of each entry shows the students’ average confidence; in the middle white row, the confidence reported is the average of the peer’s confidence.

The pathways of answers from pre-discussion (top row) to post-discussion (bottom row). Percentages indicate the portion of items from the category immediately above in that category, the numbers in brackets indicate the raw numbers of items, and the numbers at the bottom of each entry indicate the confidence associated with those items. In the middle, white row, confidence values show the peer’s confidence. Turquoise indicates incorrect answers and yellow indicates correct answers
Broadly, only 5% of correct answers were switched to incorrect, while 28% of incorrect answers were switched to correct following discussion. Even for the items in which students were initially correct but disagreed with their partner, only 21% of answers were changed to incorrect answers after discussion. However, out of the items where students were initially incorrect and disagreed with their partner, 42% were changed to the correct answer.
Does confidence predict switching?
Differences in the amount of switching to correct or incorrect answers could be driven solely by differences in confidence, as described in our first theory mentioned earlier. For this theory to hold, answers with greater confidence must have a greater likelihood of being correct. To examine whether initial confidence is associated with initial correctness, we calculated the gamma correlation between correctness and confidence in the answer before discussion, as shown in the first column of Table 4 . The average gamma correlation between initial confidence and initial correctness (mean (M) = 0.40) was greater than zero, t (160) = 8.59, p < 0.001, d = 0.68, indicating that greater confidence was associated with being correct.
The gamma correlation between accuracy and confidence before and after discussion for each class
a Gamma correlation requires that learners have variance in both confidence and correctness before and after discussion. Degrees of freedom are reduced because many students did not have requisite variation
Changing from an incorrect to a correct answer, then, may be driven entirely by selecting the answer from the peer with the greater confidence during discussion, even though most of the students in our sample were not required to explicitly disclose their confidence to their partner during discussion. We examined how frequently students choose the more confident answer when peers disagree. When peers disagreed, students’ final answers aligned with the more confident peer only 58% of the time. Similarly, we tested what the performance would be if peers always picked the answer of the more confident peer. If peers always chose the more confident answer during discussion, the final accuracy would be 69%, which is significantly lower than actual final accuracy (M = 72%, t (207) = 2.59, p = 0.01, d = 0.18). While initial confidence is related to accuracy, these results show that confidence is not the only predictor of switching answers.
Does correctness predict switching beyond confidence?
Discussion may reveal information about the correctness of answers by generating new knowledge and testing the coherence of each possible answer. To test whether the correctness of an answer added predictive power beyond the confidence of the peers involved in discussion, we analyzed situations in which students disagreed with their partner. Out of the instances when partners initially disagreed, we predicted the likelihood of keeping one’s answer based upon one’s own confidence, the partner’s confidence, and whether one’s answer was initially correct. The results of a model predicting whether students keep their answers is shown in Table 5 . For each increase in a point of one’s own confidence, the odds of keeping one’s answer increases 1.25 times (95% conf 1.13–1.38). For each decrease in a point of the partner’s confidence, the odds of keeping one’s answer increased 1.19 times (1.08–1.32). The beta weight for one’s confidence did not differ from the beta weight of the partner’s confidence, χ 2 = 0.49, p = 0.48. Finally, if one’s own answer was correct, the odds of keeping one’s answer increased 4.48 times (2.92–6.89). In other words, the more confident students were, the more likely they were to keep their answer; the more confident their peer was, the more likely they were to change their answer; and finally, if a student was correct, they were more likely to keep their answer.
Logit mixed-level regression analysis
The results of a logit mixed level regression predicting keeping one's answer from one's own confidence, the peer's confidence, and the correctness of one's initial answer for situations in which peers initially disagreed
To illustrate this relationship, we plotted the probability of keeping one’s own answer as a function of the difference between one’s own and their partner’s confidence for initially correct and incorrect answers. As shown in Fig. 3 , at every confidence level, being correct led to equal or more frequently keeping one’s answer than being incorrect.

The probability of keeping one’s answer in situations where one’s partner initially disagreed as a function of the difference between partners’ levels of confidence. Error bars indicate the standard error of the proportion and are not shown when the data are based upon a single data point
As another measure of whether discussion allows learners to test the coherence of the correct answer, we analyzed how discussion impacted confidence when partners’ answers agreed. We predicted confidence in answers by the interaction of time point (i.e., pre-discussion versus post-discussion) and being initially correct for situations in which peers initially agreed on their answer. The results, displayed in Table 6 , show that confidence increased from pre-discussion to post-discussion by 1.08 points and that confidence was greater for initially correct answers (than incorrect answers) by 0.78 points. As the interaction between time point and initial correctness shows, confidence increased more from pre-discussion to post-discussion when students were initially correct (as compared to initially incorrect). To illustrate this relationship, we plotted pre-confidence against post-confidence for initially correct and initially incorrect answers when peers agreed (Fig. 4 ). Each plotted point represents a student; the diagonal blue line indicates no change between pre-confidence and post-confidence. The graph reflects that confidence increases more from pre-discussion to post-discussion for correct answers than for incorrect answers, even when we only consider cases where peers agreed.
Mixed-level regression analysis of predicting confidence
The results of the mixed level regression predicting confidence in one's answer from the time point (pre- or post- discussion), the correctness of one's answer, and their interaction for situations in which peers initially agreed

The relationship between pre-discussion and post-discussion confidence as a function of the accuracy of an answer when partners agreed. Each dot represents a student
If students engage in more comprehensive answer testing during discussion than before, the relationship between confidence in their answer and the accuracy of their answer should be stronger following discussion than it is before. We examined whether confidence accurately reflected correctness before and after discussion. To do so, we calculated the gamma correlation between confidence and accuracy, as is typically reported in the literature on metacognitive monitoring (e.g., Son & Metcalfe, 2000 ; Tullis & Fraundorf, 2017 ). Across all students, the resolution of metacognitive monitoring increases from pre-discussion to post-discussion ( t (139) = 2.98, p = 0.003, d = 0.24; for a breakdown of gamma calculations for each class, see Table Table4). 4 ). Confidence was more accurately aligned with accuracy following discussion than preceding it. The resolution between student confidence and correctness increases through discussion, suggesting that discussion offers better coherence testing than answering alone.
To examine why peer instruction benefits student learning, we analyzed student answers and confidence before and after discussion across six psychology classes. Discussing a question with a partner improved accuracy across classes and grade levels with small to medium-sized effects. Questions of all difficulty levels benefited from peer discussion; even questions where less than half of students originally answered correctly saw improvements from discussion. Benefits across the spectrum of question difficulty align with prior research showing improvements when even very few students initially know the correct answer (Smith et al., 2009 ). More students switched from incorrect answers to correct answers than vice versa, leading to an improvement in accuracy following discussion. Answer switching was driven by a student’s own confidence in their answer and their partner’s confidence. Greater confidence in one’s answer indicated a greater likelihood of keeping the answer; a partner’s greater confidence increased the likelihood of changing to their answer.
Switching answers depended on more than just confidence: even when accounting for students’ confidence levels, the correctness of the answer impacted switching behavior. Across several measures, our data showed that the correctness of an answer carried weight beyond confidence. For example, the correctness of the answer predicted whether students switched their initial answer during peer disagreements, even after taking the confidence of both partners into account. Further, students’ confidence increased more when partners agreed on the correct answer compared to when they agreed on an incorrect answer. Finally, although confidence increased from pre-discussion to post-discussion when students changed their answers from incorrect to the correct ones, confidence decreased when students changed their answer away from the correct one. A plausible interpretation of this difference is that when students switch from a correct answer to an incorrect one, their decrease in confidence reflects the poor coherence of their final incorrect selection.
Whether peer instruction resulted in optimal switching behaviors is debatable. While accuracy improved through discussion, final accuracy was worse than if students had optimally switched their answers during discussion. If students had chosen the correct answer whenever one of the partners initially chose it, the final accuracy would have been significantly higher (M = 0.80 (SD = 0.19)) than in our data (M = 0.72 (SD = 0.24), t (207) = 6.49, p < 0.001, d = 0.45). While this might be interpreted as “process loss” (Steiner, 1972 ; Weldon & Bellinger, 1997 ), that would assume that there is sufficient information contained within the dyad to ascertain the correct answer. One individual selecting the correct answer is inadequate for this claim because they may not have a compelling justification for their answer. When we account for differences in initial confidence, students’ final accuracy was better than expected. Students’ final accuracy was better than that predicted from a model in which students always choose the answer of the more confident peer. This over-performance, often called “process gain”, can sometimes emerge when individuals collaborate to create or generate new knowledge (Laughlin, Bonner, & Miner, 2002 ; Michaelsen, Watson, & Black, 1989 ; Sniezek & Henry, 1989 ; Tindale & Sheffey, 2002 ). Final accuracy reveals that students did not simply choose the answer of the more confident student during discussion; instead, students more thoroughly probed the coherence of answers and mental models during discussion than they could do alone.
Students’ final accuracy emerges from the interaction between the pairs of students, rather than solely from individuals’ sequestered knowledge prior to discussion (e.g. Wegner, Giuliano, & Hertel, 1985 ). Schwartz ( 1995 ) details four specific cognitive products that can emerge through working in dyads. Specifically, dyads force verbalization of ideas through discussion, and this verbalization facilitates generating new knowledge. Students may not create a coherent explanation of their answer until they engage in discussion with a peer. When students create a verbal explanation of their answer to discuss with a peer, they can identify knowledge gaps and construct new knowledge to fill those gaps. Prior research examining the content of peer interactions during argumentation in upper-level biology classes has shown that these kinds of co-construction happen frequently; over three quarters of statements during discussion involve an exchange of claims and reasoning to support those claims (Knight et al., 2013 ). Second, dyads have more information processing resources than individuals, so they can solve more complex problems. Third, dyads may foster greater motivation than individuals. Finally, dyads may stimulate the creation of new, abstract representations of knowledge, above and beyond what one would expect from the level of abstraction created by individuals. Students need to communicate with their partner; to create common ground and facilitate discourse, dyads negotiate common representations to coordinate different perspectives. The common representations bridge multiple perspectives, so they lose idiosyncratic surface features of individuals’ representation. Working in pairs generates new knowledge and tests of answers that could not be predicted from individuals’ performance alone.
More broadly, teachers often put students in groups so that they can learn from each other by giving and receiving help, recognizing contradictions between their own and others’ perspectives, and constructing new understandings from divergent ideas (Bearison, Magzamen, & Filardo, 1986 ; Bossert, 1988-1989 ; Brown & Palincsar, 1989 ; Webb & Palincsar, 1996 ). Giving explanations to a peer may encourage explainers to clarify or reorganize information, recognize and rectify gaps in understandings, and build more elaborate interpretations of knowledge than they would have alone (Bargh & Schul, 1980 ; Benware & Deci, 1984 ; King, 1992 ; Yackel, Cobb, & Wood, 1991 ). Prompting students to explain why and how problems are solved facilitates conceptual learning more than reading the problem solutions twice without self-explanations (Chi, de Leeuw, Chiu, & LaVancher, 1994 ; Rittle-Johnson, 2006 ; Wong, Lawson, & Keeves, 2002 ). Self-explanations can prompt students to retrieve, integrate, and modify their knowledge with new knowledge; self-explanations can also help students identify gaps in their knowledge (Bielaczyc, Pirolli, & Brown, 1995 ; Chi & Bassock, 1989 ; Chi, Bassock, Lewis, Reimann, & Glaser, 1989 ; Renkl, Stark, Gruber, & Mandl, 1998 ; VanLehn, Jones, & Chi, 1992 ; Wong et al., 2002 ), detect and correct errors, and facilitate deeper understanding of conceptual knowledge (Aleven & Koedinger, 2002 ; Atkinson, Renkl, & Merrill, 2003 ; Chi & VanLehn, 2010 ; Graesser, McNamara, & VanLehn, 2005 ). Peer instruction, while leveraging these benefits of self-explanation, also goes beyond them by involving what might be called “other-explanation” processes - processes recruited not just when explaining a situation to oneself but to others. Mercier and Sperber ( 2019 ) argue that much of human reason is the result of generating explanations that will be convincing to other members of one’s community, thereby compelling others to act in the way that one wants.
Conversely, students receiving explanations can fill in gaps in their own understanding, correct misconceptions, and construct new, lasting knowledge. Fellow students may be particularly effective explainers because they can better take the perspective of their peer than the teacher (Priniski & Horne, 2019 ; Ryskin, Benjamin, Tullis, & Brown-Schmidt, 2015 ; Tullis, 2018 ). Peers may be better able than expert teachers to explain concepts in familiar terms and direct peers’ attention to the relevant features of questions that they do not understand (Brown & Palincsar, 1989 ; Noddings, 1985 ; Vedder, 1985 ; Vygotsky, 1981 ).
Peer instruction may benefit from the generation of explanations, but social influences may compound those benefits. Social interactions may help students monitor and regulate their cognition better than self-explanations alone (e.g., Jarvela et al., 2015 ; Kirschner, Kreijns, Phielix, & Fransen, 2015 ; Kreijns, Kirschner, & Vermeulen, 2013 ; Phielix, Prins, & Kirschner, 2010 ; Phielix, Prins, Kirschner, Erkens, & Jaspers, 2011 ). Peers may be able to judge the quality of the explanation better than the explainer. In fact, recent research suggests that peer instruction facilitates learning even more than self-explanations (Versteeg, van Blankenstein, Putter, & Steendijk, 2019 ).
Not only does peer instruction generate new knowledge, but it may also improve students’ metacognition. Our data show that peer discussion prompted more thorough testing of the coherence of the answers. Specifically, students’ confidences were better aligned with accuracy following discussion than before. Improvements in metacognitive resolution indicate that discussion provides more thorough testing of answers and ideas than does answering questions on one’s own. Discussion facilitates the metacognitive processes of detecting errors and assessing the coherence of an answer.
Agreement among peers has important consequences for final behavior. For example, when peers agreed, students very rarely changed their answer (less than 3% of the time). Further, large increases in confidence occurred when students agreed (as compared to when they disagreed). Alternatively, disagreements likely engaged different discussion processes and prompted students to combine different answers. Whether students weighed their initial answer more than their partner’s initial answer remains debatable. When students disagreed with their partner, they were more likely to stick with their own answer than switch; they kept their own answer 66% of the time. Even when their partner was more confident, students only switched to their partner’s answer 50% of the time. The low rate of switching during disagreements suggests that students weighed their own answer more heavily than their partner’s answer. In fact, across prior research, deciders typically weigh their own thoughts more than the thoughts of an advisor (Harvey, Harries, & Fischer, 2000 ; Yaniv & Kleinberger, 2000 ).
Interestingly, peers agreed more frequently than expected by chance. When students were initially correct (64% of the time), 78% of peers agreed. When students were initially incorrect (36% of the time), peers agreed 43% of the time. Pairs of students, then, agree more than expected by a random distribution of answers throughout the classroom. These data suggest that students group themselves into pairs based upon likelihood of sharing the same answer. Further, these data suggest that student understanding is not randomly distributed throughout the physical space of the classroom. Across all classes, students were instructed to work with a neighbor to discuss their answer. Given that neighbors agreed more than predicted by chance, students seem to tend to sit near and pair with peers that share their same levels of understanding. Our results from peer instruction reveal that students physically locate themselves near students of similar abilities. Peer instruction could potentially benefit from randomly pairing students together (i.e. not with a physically close neighbor) to generate the most disagreements and generative activity during discussion.
Learning through peer instruction may involve deep processing as peers actively challenge each other, and this deep processing may effectively support long-term retention. Future research can examine the persistence of gains in accuracy from peer instruction. For example, whether errors that are corrected during peer instruction stay corrected on later retests of the material remains an open question. High and low-confidence errors that are corrected during peer instruction may result in different long-term retention of the correct answer; more specifically, the hypercorrection effect suggests that errors committed with high confidence are more likely to be corrected on subsequent tests than errors with low confidence (e.g., Butler, Fazio, & Marsh, 2011 ; Butterfield & Metcalfe, 2001 ; Metcalfe, 2017 ). Whether hypercorrection holds for corrections from classmates during peer instruction (rather than from an absolute authority) could be examined in the future.
The influence of partner interaction on accuracy may depend upon the domain and kind of question posed to learners. For simple factual or perceptual questions, partner interaction may not consistently benefit learning. More specifically, partner interaction may amplify and bolster wrong answers when factual or perceptual questions lead most students to answer incorrectly (Koriat, 2015 ). However, for more “intellective tasks,” interactions and arguments between partners can produce gains in knowledge (Trouche et al., 2014 ). For example, groups typically outperform individuals for reasoning tasks (Laughlin, 2011 ; Moshman & Geil, 1998 ), math problems (Laughlin & Ellis, 1986 ), and logic problems (Doise & Mugny, 1984; Perret-Clermont, 1980 ). Peer instruction questions that allow for student argumentation and reasoning, therefore, may have the best benefits in student learning.
The underlying benefits of peer instruction extend beyond the improvements in accuracy seen from pre-discussion to post-discussion. Peer instruction prompts students to retrieve information from long-term memory, and these practice tests improve long-term retention of information (Roediger III & Karpicke, 2006 ; Tullis, Fiechter, & Benjamin, 2018 ). Further, feedback provided by instructors following peer instruction may guide students to improve their performance and correct misconceptions, which should benefit student learning (Bangert-Drowns, Kulik, & Kulik, 1991 ; Thurlings, Vermeulen, Bastiaens, & Stijnen, 2013 ). Learners who engage in peer discussion can use their new knowledge to solve new, but similar problems on their own (Smith et al., 2009 ). Generating new knowledge and revealing gaps in knowledge through peer instruction, then, effectively supports students’ ability to solve novel problems. Peer instruction can be an effective tool to generate new knowledge through discussion between peers and improve student understanding and metacognition.
Acknowledgements
Not applicable.
Authors’ contributions
JGT collected some data, analyzed the data, and wrote the first draft of the paper. RLG collected some data, contributed significantly to the framing of the paper, and edited the paper. The authors read and approved the final manuscript.
Authors’ information
JGT: Assistant Professor in Educational Psychology at University of Arizona. RLG: Chancellor’s Professor in Psychology at Indiana University.
No funding supported this manuscript.
Availability of data and materials
Ethics approval and consent to participate.
The ethics approval was waived by the Indiana University Institutional Review Board (IRB) and the University of Arizona IRB, given that these data are collected as part of normal educational settings and processes.
Consent for publication
No individual data are presented in the manuscript.
Competing interests
The authors declare that they have no competing interests.
Publisher’s Note
Springer Nature remains neutral with regard to jurisdictional claims in published maps and institutional affiliations.
- Aleven V, Koedinger KR. An effective metacognitive strategy: Learning by doing and explaining with a computer based cognitive tutor. Cognitive Science. 2002; 26 :147–179. doi: 10.1207/s15516709cog2602_1. [ CrossRef ] [ Google Scholar ]
- Atkinson RK, Renkl A, Merrill MM. Transitioning from studying examples to solving problems: Effects of self-explanation prompts and fading worked-out steps. Journal of Educational Psychology. 2003; 95 :774–783. doi: 10.1037/0022-0663.95.4.774. [ CrossRef ] [ Google Scholar ]
- Baayen, R. H. (2008). Analyzing linguistic data: A practical introduction to statistics . Cambridge: Cambridge University Press.
- Baayen RH, Davidson DJ, Bates DM. Mixed-effects modeling with crossed random effects for subjects and items. Journal of Memory and Language. 2008; 59 :390–412. doi: 10.1016/j.jml.2007.12.005. [ CrossRef ] [ Google Scholar ]
- Bangert-Drowns RL, Kulik JA, Kulik C-LC. Effects of frequent classroom testing. Journal of Educational Research. 1991; 85 :89–99. doi: 10.1080/00220671.1991.10702818. [ CrossRef ] [ Google Scholar ]
- Bargh JA, Schul Y. On the cognitive benefit of teaching. Journal of Educational Psychology. 1980; 72 :593–604. doi: 10.1037/0022-0663.72.5.593. [ CrossRef ] [ Google Scholar ]
- Bates D, Maechler M, Bolker B, Walker S. Fitting linear mixed-effects models using lme4. Journal of Statistical Software. 2015; 67 :1–48. doi: 10.18637/jss.v067.i01. [ CrossRef ] [ Google Scholar ]
- Bearison DJ, Magzamen S, Filardo EK. Sociocognitive conflict and cognitive growth in young children. Merrill-Palmer Quarterly. 1986; 32 (1):51–72. [ Google Scholar ]
- Beatty ID, Gerace WJ, Leonard WJ, Dufresne RJ. Designing effective questions for classroom response system teaching. American Journal of Physics. 2006; 74 (1):31e39. doi: 10.1119/1.2121753. [ CrossRef ] [ Google Scholar ]
- Beekes W. The “millionaire” method for encouraging participation. Active Learning in Higher Education. 2006; 7 :25–36. doi: 10.1177/1469787406061143. [ CrossRef ] [ Google Scholar ]
- Benware CA, Deci EL. Quality of learning with an active versus passive motivational set. American Educational Research Journal. 1984; 21 :755–765. doi: 10.3102/00028312021004755. [ CrossRef ] [ Google Scholar ]
- Bielaczyc K, Pirolli P, Brown AL. Training in self-explanation and self regulation strategies: Investigating the effects of knowledge acquisition activities on problem solving. Cognition and Instruction. 1995; 13 :221–251. doi: 10.1207/s1532690xci1302_3. [ CrossRef ] [ Google Scholar ]
- Bossert ST. Cooperative activities in the classroom. Review of Research in Education. 1988; 15 :225–252. [ Google Scholar ]
- Brooks BJ, Koretsky MD. The influence of group discussion on students’ responses and confidence during peer instruction. Journal of Chemistry Education. 2011; 88 :1477–1484. doi: 10.1021/ed101066x. [ CrossRef ] [ Google Scholar ]
- Brown AL, Palincsar AS. Guided, cooperative learning and individual knowledge acquisition. In: Resnick LB, editor. Knowing, learning, and instruction: essays in honor of Robert Glaser. Hillsdale: Erlbaum; 1989. pp. 393–451. [ Google Scholar ]
- Butchart S, Handfield T, Restall G. Using peer instruction to teach philosophy, logic and critical thinking. Teaching Philosophy. 2009; 32 :1–40. doi: 10.5840/teachphil20093212. [ CrossRef ] [ Google Scholar ]
- Butler AC, Fazio LK, Marsh EJ. The hypercorrection effect persists over a week, but high-confidence errors return. Psychonomic Bulletin & Review. 2011; 18 (6):1238–1244. doi: 10.3758/s13423-011-0173-y. [ PubMed ] [ CrossRef ] [ Google Scholar ]
- Butterfield B, Metcalfe J. Errors committed with high confidence are hypercorrected. Journal of Experimental Psychology: Learning, Memory, and Cognition. 2001; 27 (6):1491. [ PubMed ] [ Google Scholar ]
- Caldwell JE. Clickers in the large classroom: current research and best-practice tips. CBE-Life Sciences Education. 2007; 6 (1):9–20. doi: 10.1187/cbe.06-12-0205. [ PMC free article ] [ PubMed ] [ CrossRef ] [ Google Scholar ]
- Chi M, VanLehn KA. Meta-cognitive strategy instruction in intelligent tutoring systems: How, when and why. Journal of Educational Technology and Society. 2010; 13 :25–39. [ Google Scholar ]
- Chi MTH, Bassock M. Learning from examples via self-explanations. In: Resnick LB, editor. Knowing, learning, and instruction: Essays in honor of Robert Glaser. Hillsdale: Erlbaum; 1989. pp. 251–282. [ Google Scholar ]
- Chi MTH, Bassock M, Lewis M, Reimann P, Glaser R. Self-explanations: How students study and use examples in learning to solve problems. Cognitive Science. 1989; 13 :145–182. doi: 10.1207/s15516709cog1302_1. [ CrossRef ] [ Google Scholar ]
- Chi MTH, de Leeuw N, Chiu MH, LaVancher C. Eliciting self-explanations improves understanding. Cognitive Science. 1994; 18 :439–477. [ Google Scholar ]
- Cortright RN, Collins HL, DiCarlo SE. Peer instruction enhanced meaningful learning: Ability to solve novel problems. Advances in Physiology Education. 2005; 29 :107–111. doi: 10.1152/advan.00060.2004. [ PubMed ] [ CrossRef ] [ Google Scholar ]
- Crouch CH, Mazur E. Peer instruction: Ten years of experience and results. American Journal of Physics. 2001; 69 :970–977. doi: 10.1119/1.1374249. [ CrossRef ] [ Google Scholar ]
- Cummings K, Roberts S. A study of peer instruction methods with school physics students. In: Henderson C, Sabella M, Hsu L, editors. Physics education research conference. College Park: American Institute of Physics; 2008. pp. 103–106. [ Google Scholar ]
- Deslauriers L, Schelew E, Wieman C. Improved learning in a large-enrollment physics class. Science. 2011; 332 :862–864. doi: 10.1126/science.1201783. [ PubMed ] [ CrossRef ] [ Google Scholar ]
- Duncan D. Clickers in the classroom: How to enhance science teaching using classroom response systems. San Francisco: Pearson/Addison-Wesley; 2005. [ Google Scholar ]
- Finley JR, Tullis JG, Benjamin AS. Metacognitive control of learning and remembering. In: Khine MS, Saleh IM, editors. New science of learning: Cognition, computers and collaborators in education. New York: Springer Science & Business Media, LLC; 2010. [ Google Scholar ]
- Giuliodori MJ, Lujan HL, DiCarlo SE. Peer instruction enhanced student performance on qualitative problem solving questions. Advances in Physiology Education. 2006; 30 :168–173. doi: 10.1152/advan.00013.2006. [ PubMed ] [ CrossRef ] [ Google Scholar ]
- Graesser AC, McNamara D, VanLehn K. Scaffolding deep comprehension strategies through AutoTutor and iSTART. Educational Psychologist. 2005; 40 :225–234. doi: 10.1207/s15326985ep4004_4. [ CrossRef ] [ Google Scholar ]
- Granovskiy B, Gold JM, Sumpter D, Goldstone RL. Integration of social information by human groups. Topics in Cognitive Science. 2015; 7 :469–493. doi: 10.1111/tops.12150. [ PMC free article ] [ PubMed ] [ CrossRef ] [ Google Scholar ]
- Harvey N, Fischer I. Taking advice: Accepting help, improving judgment, and sharing responsibility. Organizational Behavior and Human Decision Processes. 1997; 70 :117–133. doi: 10.1006/obhd.1997.2697. [ CrossRef ] [ Google Scholar ]
- Harvey N, Harries C, Fischer I. Using advice and assessing its quality. Organizational Behavior and Human Decision Processes. 2000; 81 :252–273. doi: 10.1006/obhd.1999.2874. [ PubMed ] [ CrossRef ] [ Google Scholar ]
- Henderson C, Dancy MH. The impact of physics education research on the teaching of introductory quantitative physics in the United States. Physical Review Special Topics: Physics Education Research. 2009; 5 (2):020107. doi: 10.1103/PhysRevSTPER.5.020107. [ CrossRef ] [ Google Scholar ]
- Jaeger TF. Categorical data analysis: away from ANOVAs (transformation or not) and towards logit mixed models. Journal of Memory and Language. 2008; 59 :434–446. doi: 10.1016/j.jml.2007.11.007. [ PMC free article ] [ PubMed ] [ CrossRef ] [ Google Scholar ]
- James MC. The effect of grading incentive on student discourse in peer instruction. American Journal of Physics. 2006; 74 (8):689–691. doi: 10.1119/1.2198887. [ CrossRef ] [ Google Scholar ]
- Jarvela S, Kirschner P, Panadero E, Malmberg J, Phielix C, Jaspers J, Koivuniemi M, Jarvenoja H. Enhancing socially shared regulation in collaborative learning groups: Designing for CSCL regulation tools. Educational Technology Research and Development. 2015; 63 (1):125e142. doi: 10.1007/s11423-014-9358-1. [ CrossRef ] [ Google Scholar ]
- Jones ME, Antonenko PD, Greenwood CM. The impact of collaborative and individualized student response system strategies on learner motivation, metacognition, and knowledge transfer. Journal of Computer Assisted Learning. 2012; 28 (5):477–487. doi: 10.1111/j.1365-2729.2011.00470.x. [ CrossRef ] [ Google Scholar ]
- King A. Facilitating elaborative learning through guided student-generated questioning. Educational Psychologist. 1992; 27 :111–126. doi: 10.1207/s15326985ep2701_8. [ CrossRef ] [ Google Scholar ]
- Kirschner PA, Kreijns K, Phielix C, Fransen J. Awareness of cognitive and social behavior in a CSCL environment. Journal of Computer Assisted Learning. 2015; 31 (1):59–77. doi: 10.1111/jcal.12084. [ CrossRef ] [ Google Scholar ]
- Knight JK, Wise SB, Southard KM. Understanding clicker discussions: student reasoning and the impact of instructional cues. CBE-Life Sciences Education. 2013; 12 :645–654. doi: 10.1187/cbe.13-05-0090. [ PMC free article ] [ PubMed ] [ CrossRef ] [ Google Scholar ]
- Koriat A. When two heads are better than one and when they can be worse: The amplification hypothesis. Journal of Experimental Psychology: General. 2015; 144 :934–950. doi: 10.1037/xge0000092. [ PubMed ] [ CrossRef ] [ Google Scholar ]
- Kreijns K, Kirschner PA, Vermeulen M. Social aspects of CSCL environments: A research framework. Educational Psychologist. 2013; 48 (4):229e242. doi: 10.1080/00461520.2012.750225. [ CrossRef ] [ Google Scholar ]
- Kuhn LM, Sniezek JA. Confidence and uncertainty in judgmental forecasting: Differential effects of scenario presentation. Journal of Behavioral Decision Making. 1996; 9 :231–247. doi: 10.1002/(SICI)1099-0771(199612)9:4<231::AID-BDM240>3.0.CO;2-L. [ CrossRef ] [ Google Scholar ]
- Lasry N, Mazur E, Watkins J. Peer instruction: From Harvard to the two-year college. American Journal of Physics. 2008; 76 (11):1066–1069. doi: 10.1119/1.2978182. [ CrossRef ] [ Google Scholar ]
- Laughlin PR. Group problem solving. Princeton: Princeton University Press; 2011. [ Google Scholar ]
- Laughlin PR, Bonner BL, Miner AG. Groups perform better than individuals on letters-to-numbers problems. Organisational Behaviour and Human Decision Processes. 2002; 88 :605–620. doi: 10.1016/S0749-5978(02)00003-1. [ CrossRef ] [ Google Scholar ]
- Laughlin PR, Ellis AL. Demonstrability and social combination processes on mathematical intellective tasks. Journal of Experimental Social Psychology. 1986; 22 :177–189. doi: 10.1016/0022-1031(86)90022-3. [ CrossRef ] [ Google Scholar ]
- Lucas A. Using peer instruction and i-clickers to enhance student participation in calculus. Primus. 2009; 19 (3):219–231. doi: 10.1080/10511970701643970. [ CrossRef ] [ Google Scholar ]
- Mazur E. Peer instruction: A user’s manual. Upper Saddle River: Prentice Hall; 1997. [ Google Scholar ]
- Mercier H, Sperber D. The enigma of reason. Cambridge: Harvard University Press; 2019. [ Google Scholar ]
- Metcalfe J. Learning from errors. Annual Review of Psychology. 2017; 68 :465–489. doi: 10.1146/annurev-psych-010416-044022. [ PubMed ] [ CrossRef ] [ Google Scholar ]
- Michaelsen LK, Watson WE, Black RH. Realistic test of individual versus group decision making. Journal of Applied Psychology. 1989; 64 :834–839. doi: 10.1037/0021-9010.74.5.834. [ CrossRef ] [ Google Scholar ]
- Miller RL, Santana-Vega E, Terrell MS. Can good questions and peer discussion improve calculus instruction? Primus. 2007; 16 (3):193–203. doi: 10.1080/10511970608984146. [ CrossRef ] [ Google Scholar ]
- Morgan JT, Wakefield C. Who benefits from peer conversation? Examining correlations of clicker question correctness and course performance. Journal of College Science Teaching. 2012; 41 (5):51–56. [ Google Scholar ]
- Moshman D, Geil M. Collaborative reasoning: Evidence for collective rationality. Thinking and Reasoning. 1998; 4 :231–248. doi: 10.1080/135467898394148. [ CrossRef ] [ Google Scholar ]
- Murayama K, Sakaki M, Yan VX, Smith GM. Type I error inflation in the traditional by-participant analysis to metamemory accuracy: A generalized mixed-effects model perspective. Journal of Experimental Psychology: Learning, Memory, and Cognition. 2014; 40 :1287–1306. [ PubMed ] [ Google Scholar ]
- Newbury P, Heiner C. Ready, set, react! getting the most out of peer instruction using clickers. 2012. [ Google Scholar ]
- Nielsen KL, Hansen-Nygård G, Stav JB. Investigating peer instruction: how the initial voting session affects students’ experiences of group discussion. ISRN Education. 2012; 2012 :article 290157. doi: 10.5402/2012/290157. [ CrossRef ] [ Google Scholar ]
- Noddings N. Small groups as a setting for research on mathematical problem solving. In: Silver EA, editor. Teaching and learning mathematical problem solving. Hillsdale: Erlbaum; 1985. pp. 345–360. [ Google Scholar ]
- Perret-Clermont AN. Social Interaction and Cognitive Development in Children. London: Academic Press; 1980. [ Google Scholar ]
- Perez KE, Strauss EA, Downey N, Galbraith A, Jeanne R, Cooper S, Madison W. Does displaying the class results affect student discussion during peer instruction? CBE Life Sciences Education. 2010; 9 :133–140. doi: 10.1187/cbe.09-11-0080. [ PMC free article ] [ PubMed ] [ CrossRef ] [ Google Scholar ]
- Phielix C, Prins FJ, Kirschner PA. Awareness of group performance in a CSCL-environment: Effects of peer feedback and reflection. Computers in Human Behavior. 2010; 26 (2):151–161. doi: 10.1016/j.chb.2009.10.011. [ CrossRef ] [ Google Scholar ]
- Phielix C, Prins FJ, Kirschner PA, Erkens G, Jaspers J. Group awareness of social and cognitive performance in a CSCL environment: Effects of a peer feedback and reflection tool. Computers in Human Behavior. 2011; 27 (3):1087–1102. doi: 10.1016/j.chb.2010.06.024. [ CrossRef ] [ Google Scholar ]
- Pollock SJ, Chasteen SV, Dubson M, Perkins KK. The use of concept tests and peer instruction in upper-division physics. In: Sabella M, Singh C, Rebello S, editors. AIP conference proceedings. New York: AIP Press; 2010. p. 261. [ Google Scholar ]
- Porter L, Bailey-Lee C, Simon B. SIGCSE ‘13: Proceedings of the 44th ACM technical symposium on computer science education. New York: ACM Press; 2013. Halving fail rates using peer instruction: A study of four computer science courses; pp. 177–182. [ Google Scholar ]
- Price PC, Stone ER. Intuitive evaluation of likelihood judgment producers. Journal of Behavioral Decision Making. 2004; 17 :39–57. doi: 10.1002/bdm.460. [ CrossRef ] [ Google Scholar ]
- Priniski JH, Horne Z. Crowdsourcing effective educational interventions. In: Goel AK, Seifert C, Freska C, editors. Proceedings of the 41st annual conference of the cognitive science society. Austin: Cognitive Science Society; 2019. [ Google Scholar ]
- Rao SP, DiCarlo SE. Peer instruction improves performance on quizzes. Advances in Physiological Education. 2000; 24 :51–55. doi: 10.1152/advances.2000.24.1.51. [ PubMed ] [ CrossRef ] [ Google Scholar ]
- Renkl A, Stark R, Gruber H, Mandl H. Learning from worked-out examples: The effects of example variability and elicited self-explanations. Contemporary Educational Psychology. 1998; 23 :90–108. doi: 10.1006/ceps.1997.0959. [ PubMed ] [ CrossRef ] [ Google Scholar ]
- Rittle-Johnson B. Promoting transfer: Effects of self-explanation and direct instruction. Child Development. 2006; 77 :1–15. doi: 10.1111/j.1467-8624.2006.00852.x. [ PubMed ] [ CrossRef ] [ Google Scholar ]
- Roediger HL, III, Karpicke JD. Test-enhanced learning: Taking memory tests improves long-term retention. Psychological Science. 2006; 17 :249–255. doi: 10.1111/j.1467-9280.2006.01693.x. [ PubMed ] [ CrossRef ] [ Google Scholar ]
- Ryskin R, Benjamin AS, Tullis JG, Brown-Schmidt S. Perspective-taking in comprehension, production, and memory: An individual differences approach. Journal of Experimental Psychology: General. 2015; 144 :898–915. doi: 10.1037/xge0000093. [ PubMed ] [ CrossRef ] [ Google Scholar ]
- Sah S, Moore DA, MacCoun RJ. Cheap talk and credibility: The consequences of confidence and accuracy on advisor credibility and persuasiveness. Organizational Behavior and Human Decision Processes. 2013; 121 :246–255. doi: 10.1016/j.obhdp.2013.02.001. [ CrossRef ] [ Google Scholar ]
- Schwartz DL. The emergence of abstract representations in dyad problem solving. The Journal of the Learning Sciences. 1995; 4 :321–354. doi: 10.1207/s15327809jls0403_3. [ CrossRef ] [ Google Scholar ]
- Simon B, Kohanfars M, Lee J, Tamayo K, Cutts Q. Proceedings of the 41st SIGCSE technical symposium on computer science education. 2010. Experience report: peer instruction in introductory computing. [ Google Scholar ]
- Smith MK, Wood WB, Adams WK, Wieman C, Knight JK, Guild N, Su TT. Why peer discussion improves student performance on in-class concept questions. Science. 2009; 323 :122–124. doi: 10.1126/science.1165919. [ PubMed ] [ CrossRef ] [ Google Scholar ]
- Smith MK, Wood WB, Krauter K, Knight JK. Combining peer discussion with instructor explanation increases student learning from in-class concept questions. CBE-Life Sciences Education. 2011; 10 :55–63. doi: 10.1187/cbe.10-08-0101. [ PMC free article ] [ PubMed ] [ CrossRef ] [ Google Scholar ]
- Sniezek JA, Buckley T. Cueing and cognitive conflict in judge–Advisor decision making. Organizational Behavior and Human Decision Processes. 1995; 62 :159–174. doi: 10.1006/obhd.1995.1040. [ CrossRef ] [ Google Scholar ]
- Sniezek JA, Henry RA. Accuracy and confidence in group judgment. Organizational Behavior and Human Decision Processes. 1989; 43 :1–28. doi: 10.1016/0749-5978(89)90055-1. [ CrossRef ] [ Google Scholar ]
- Son LK, Metcalfe J. Metacognitive and control strategies in study-time allocation. Journal of Experimental Psychology: Learning, Memory, and Cognition. 2000; 26 :204–221. [ PubMed ] [ Google Scholar ]
- Steiner ID. Group processes and productivity. New York: Academic Press; 1972. [ Google Scholar ]
- Thurlings M, Vermeulen M, Bastiaens T, Stijnen S. Understanding feedback: A learning theory perspective. Educational Research Review. 2013; 9 :1–15. doi: 10.1016/j.edurev.2012.11.004. [ CrossRef ] [ Google Scholar ]
- Tindale RS, Sheffey S. Shared information, cognitive load, and group memory. Group Processes & Intergroup Relations. 2002; 5 (1):5–18. doi: 10.1177/1368430202005001535. [ CrossRef ] [ Google Scholar ]
- Trouche E, Sander E, Mercier H. Arguments, more than confidence, explain the good performance of reasoning groups. Journal of Experimental Psychology: General. 2014; 143 :1958–1971. doi: 10.1037/a0037099. [ PubMed ] [ CrossRef ] [ Google Scholar ]
- Tullis JG. Predicting others’ knowledge: Knowledge estimation as cue-utilization. Memory & Cognition. 2018; 46 :1360–1375. doi: 10.3758/s13421-018-0842-4. [ PubMed ] [ CrossRef ] [ Google Scholar ]
- Tullis JG, Fiechter JL, Benjamin AS. The efficacy of learners’ testing choices. Journal of Experimental Psychology: Learning, Memory, and Cognition. 2018; 44 :540–552. [ PubMed ] [ Google Scholar ]
- Tullis JG, Fraundorf SH. Predicting others’ memory performance: The accuracy and bases of social metacognition. Journal of Memory and Language. 2017; 95 :124–137. doi: 10.1016/j.jml.2017.03.003. [ CrossRef ] [ Google Scholar ]
- Turpen, C., & Finkelstein, N. (2007). Understanding how physics faculty use peer instruction. In L. Hsu, C. Henderson, & L. McCullough (Eds.), Physics education research conference , (pp. 204–209). College Park: American Institute of Physics.
- Van Swol LM, Sniezek JA. Factors affecting the acceptance of expert advice. British Journal of Social Psychology. 2005; 44 :443–461. doi: 10.1348/014466604X17092. [ PubMed ] [ CrossRef ] [ Google Scholar ]
- VanLehn K, Jones RM, Chi MTH. A model of the self-explanation effect. Journal of the Learning Sciences. 1992; 2 (1):1–59. doi: 10.1207/s15327809jls0201_1. [ CrossRef ] [ Google Scholar ]
- Vedder P. Cooperative learning: A study on processes and effects of cooperation between primary school children. Westerhaven: Rijkuniversiteit Groningen; 1985. [ Google Scholar ]
- Versteeg M, van Blankenstein FM, Putter H, Steendijk P. Peer instruction improves comprehension and transfer of physiological concepts: A randomized comparison with self-explanation. Advances in Health Sciences Education. 2019; 24 :151–165. doi: 10.1007/s10459-018-9858-6. [ PMC free article ] [ PubMed ] [ CrossRef ] [ Google Scholar ]
- Vygotsky LS. The genesis of higher mental functioning. In: Wertsch JV, editor. The concept of activity in Soviet psychology. Armonk: Sharpe; 1981. pp. 144–188. [ Google Scholar ]
- Webb NM, Palincsar AS. Group processes in the classroom. In: Berliner DC, Calfee RC, editors. Handbook of educational psychology. New York: Macmillan Library Reference USA: London: Prentice Hall International; 1996. pp. 841–873. [ Google Scholar ]
- Wegner DM, Giuliano T, Hertel P. Cognitive interdependence in close relationships. In: Ickes WJ, editor. Compatible and incompatible relationships. New York: Springer-Verlag; 1985. pp. 253–276. [ Google Scholar ]
- Weldon MS, Bellinger KD. Collective memory: Collaborative and individual processes in remembering. Journal of Experimental Psychology: Learning, Memory, and Cognition. 1997; 23 :1160–1175. [ PubMed ] [ Google Scholar ]
- Wieman C, Perkins K, Gilbert S, Benay F, Kennedy S, Semsar K, et al. Clicker resource guide: An instructor’s guide to the effective use of personalresponse systems (clickers) in teaching. Vancouver: University of British Columbia; 2009. [ Google Scholar ]
- Wong RMF, Lawson MJ, Keeves J. The effects of self-explanation training on students’ problem solving in high school mathematics. Learning and Instruction. 2002; 12 :23. doi: 10.1016/S0959-4752(01)00027-5. [ CrossRef ] [ Google Scholar ]
- Yackel E, Cobb P, Wood T. Small-group interactions as a source of learning opportunities in second-grade mathematics. Journal for Research in Mathematics Education. 1991; 22 :390–408. doi: 10.2307/749187. [ CrossRef ] [ Google Scholar ]
- Yaniv I. The benefit of additional opinions. Current Directions in Psychological Science. 2004; 13 :75–78. doi: 10.1111/j.0963-7214.2004.00278.x. [ CrossRef ] [ Google Scholar ]
- Yaniv I. Receiving other people’s advice: Influence and benefit. Organizational Behavior and Human Decision Processes. 2004; 93 :1–13. doi: 10.1016/j.obhdp.2003.08.002. [ CrossRef ] [ Google Scholar ]
- Yaniv I, Choshen-Hillel S. Exploiting the wisdom of others to make better decisions: Suspending judgment reduces egocentrism and increases accuracy. Journal of Behavioral Decision Making. 2012; 25 :427–434. doi: 10.1002/bdm.740. [ CrossRef ] [ Google Scholar ]
- Yaniv I, Kleinberger E. Advice taking in decision making: Egocentric discounting and reputation formation. Organizational Behavior and Human Decision Processes. 2000; 83 :260–281. doi: 10.1006/obhd.2000.2909. [ PubMed ] [ CrossRef ] [ Google Scholar ]

Peer to Peer Learning – Examples, Benefits & Strategies
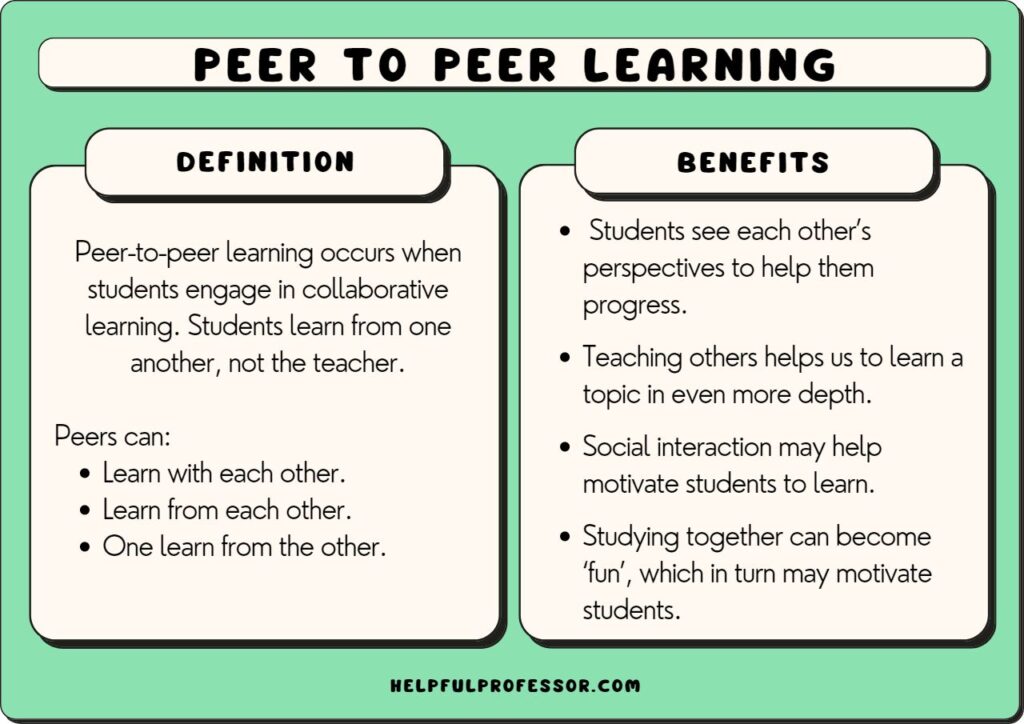
Peer-to-peer learning occurs when students engage in collaborative learning .
- Learn with each other.
- Learn from each other.
- One learn from the other.
Peers should:
- Both be students.
- Each get something educationally beneficial out of the collaboration.
- Be equals either in terms of ability level or status as ‘students’.
There is no one peer learning strategy .
Any strategy involving the collaboration of peers in a learning situation could be called ‘peer learning’.
Below are 9 peer to peer learning examples.
9 Examples & Types of Peer to Peer Learning
1. proctor model..
The proctor model involves senior students tutoring junior students.
The senior student can be:
- An older student from a higher grade level: In this instance, the older student benefits from the peer tutoring scenario because they consolidate knowledge they already know (‘the best way to learn is to teach’). They may also undertake the task to develop mentorship and leadership skills .
- A more skilled student helping a less skilled student in the same class: In this instance, the students may be the same age level and in the same class. However, one student is significantly more advanced than the other. This student acts as the ‘ more knowledgeable other ’, helping bring the other students up to their level. The more skilled student may benefit from this scenario by refining their knowledge and being able to apply it in their explanations.
2. Discussion seminars.
Discussion seminars are common in higher education. They usually occur following a lecture or prepared study (such as a weekly reading).
The purpose of the discussion seminar is for peers to talk together in a group about the topic they have just learned about.
Discussion seminars tend to be unstructured and designed to have students jump in with thoughts or contributions when they feel they have something important to add.
A teacher may present the students with a stimulus question or object. The students use that stimulus as an entry point into a discussion of the nuances, contradictions and features of the topic at hand.
For discussion seminars to be successful, teachers need to create a safe, comfortable space where students feel free to speak up in front of their peers.
3. Peer Support Groups.
Peer support groups are also known as private study groups. These tend not to have a teacher’s presence and are often organized by peers themselves.
Common peer study groups take place during free time, after school or on weekends.
A peer study group can be beneficial for motivating students in the lead-up to exams or assignment due dates.
Students who work together can stave off distraction, boredom and frustration. Peers can push each other past difficulties and mind blocks. When studying with peers, a student has people to bounce ideas off and provide support and explanations.
4. Peer Assessment Schemes.
Peer assessment schemes involve having students look over each other’s work and give feedback to one another.
The benefits of peer assessment schemes involve:
- Being able to see how other students have gone about the task.
- Getting insight into the cognitive processes and study strategies other students used.
- Learning diplomatic skills .
- A requirement to think critically about how to address a topic or task.
However, peer assessment schemes usually cannot be used for summative or formal assessments which require stringent quality control checks.
5. Collaborative Projects
Collaborative projects are common in science lab work. They involve getting students together to work on a problem that has been presented to them by the teacher.
Collaborative projects are very popular in 21st Century approaches such as problem based learning and problem posing education .
When students work together on longer-term projects, additional benefits may arise such as:
- Negotiation skills.
- Skill sharing capabilities.
- Setting and meeting deadlines.
- Interdependence (‘we sink or swim together’)
Collaborative projects can involve groups from as small as pairs up to large group collaborations.
Read Also: Collaborate vs Cooperative Learning
6. Cascading Groups.
Cascading groups involve placing students in groups that are either successively smaller or successively larger:
- Successively smaller: The class starts out as a large group then splits in half for a follow-up activity. Then, those two groups split into halves again, and then again, until students end up in pairs or as individuals.
- Successively larger: Often called ‘think-pair-share’, this method involves starting out as an individual, then pairing up, then going into a group of 4, then 8, and so on.
A cascading group lesson has several benefits:
- In successively smaller groups, students can nominate areas of a topic they want to specialize in. They start with a general overview in the large group, then become experts on their small piece of the pie when they pair off to work alone.
- The successively smaller groups method also provides students with the chance to get support in larger groups to build up their knowledge before peeling off to work alone.
- In successively larger groups, students start off with their own thoughts which they then contribute to the larger group. As the groups get larger, students can pick up other students’ ideas and perspectives and build their knowledge more and more ‘from the ground up’.
7. Workplace mentoring.
Workplace mentoring involves having people in a workplace to pair up to support one another.
This can involve:
- Mentor-Mentee Relationship: A more established member of the workplace team mentors a new member of the team. This method closely mirrors the situated learning approach, whereby an apprentice is slowly absorbed into the workplace by observing their peers go about their work.
- Peer Support: On a regular basis, peers will watch one another go about their work to provide and receive tips and help on how to do the tasks more effectively or efficiently.
8. Reciprocal teaching.
Reciprocal teaching involves having students develop skills in scaffolding their peers’ learning. It has four skills that students should develop:
- Questioning: ask each other questions to test knowledge.
- Predicting: ask each other to predict answers based on limited knowledge.
- Summarizing: ask each other to sum something up in shorter terms.
- Clarifying: ask for help when you’re not sure about something.
With these four skills, students can develop the metacognitive skills to support one another in learning scenarios.
9. Expert Jigsaw Method
The expert jigsaw method involves getting students into two successive groups:
- Session 1: In the first instance, each group focuses on a different aspect of a topic.
- Session 2: Then, students peel off and re-form new groups. Each new group should have one member of each of the previous groups. This ensures that every group has one expert on a specific aspect of the topic. These new groups then go about a task, knowing there is breadth of knowledge amongst the group members.
Theoretical Foundation
Peer support in learning is underpinned by the sociocultural theory of education . The theory holds that learning and development can be progressed faster through social interactions .
This theory’s key proponents include Lev Vygotsky, Barbara Rogoff and Jerome Bruner.
Central aspects of the theory include:
- Scaffolding : Students can learn better when support is provided by a ‘more knowledgeable other’. When a student’s skills have developed sufficiently, the support is removed.
- Language Acquisition: Through social interaction, students develop the domain specific language required to discuss topics like mathematics, history, etc.
- Multiple Perspectives: By interacting with others, we see things from their perspectives which can open up new understandings about topics.
For more on the sociocultural approach, read my full post on the sociocultural theory of education .
Related Concept: Peer Mediation of Disputes
Benefits and Challenges (Pros and Cons) of Peer to Peer Learning
- Students see each other’s perspectives to help them progress their knowledge.
- Teaching others helps us to learn a topic in even more depth.
- Social interaction may help motivate students to learn.
- Studying together can become ‘fun’, which in turn may encourage students to continue to focus on the topic for longer.
- Working in groups can be distracting for students, especially if some members of the group are not as focused as others.
- Some students work better in silence or isolation where they have time to think and focus.
- Students with sensory or behavioral challenges may struggle in peer-to-peer interactions.
- Students need to be explicitly taught group work and self-regulation skills before group work is a success.
- Students may not respect the critical feedback that their peers provide .
Final Thoughts
Teaching, learning from and interacting with peers is an incredibly useful strategy.
I’ve found that no matter how hard I try, sometimes a child is far better at explaining an idea to another child than I’ll ever be. They just have the capacity to speak to each other at the same level!
Peer interactions are incredibly important for learning in classrooms and the workplace.
References and Further Reading
All citations below are in APA format :
Boud, D., Cohen, R., & Sampson, J. (2014). Peer learning in higher education: Learning from and with each other . London: Routledge.
Keenan, C. (2014). Mapping student-led peer learning in the UK . York: Higher Education Academy.
O’donnell, A. M., & King, A. (2014). Cognitive perspectives on peer learning . London: Routledge.
Riese, H., Samara, A., & Lillejord, S. (2012). Peer relations in peer learning. International Journal of Qualitative Studies in Education , 25 (5), 601-624.
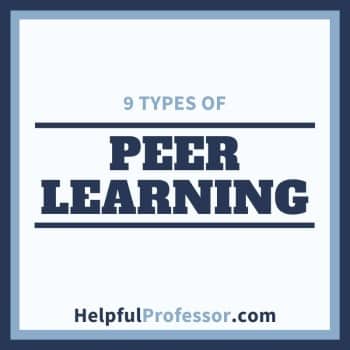
Chris Drew (PhD)
Dr. Chris Drew is the founder of the Helpful Professor. He holds a PhD in education and has published over 20 articles in scholarly journals. He is the former editor of the Journal of Learning Development in Higher Education. [Image Descriptor: Photo of Chris]
- Chris Drew (PhD) https://helpfulprofessor.com/author/chris-drew-phd/ 15 Self-Actualization Examples (Maslow's Hierarchy)
- Chris Drew (PhD) https://helpfulprofessor.com/author/chris-drew-phd/ Forest Schools Philosophy & Curriculum, Explained!
- Chris Drew (PhD) https://helpfulprofessor.com/author/chris-drew-phd/ Montessori's 4 Planes of Development, Explained!
- Chris Drew (PhD) https://helpfulprofessor.com/author/chris-drew-phd/ Montessori vs Reggio Emilia vs Steiner-Waldorf vs Froebel
1 thought on “Peer to Peer Learning – Examples, Benefits & Strategies”
Helpful info.
Leave a Comment Cancel Reply
Your email address will not be published. Required fields are marked *
Change Password
Your password must have 8 characters or more and contain 3 of the following:.
- a lower case character,
- an upper case character,
- a special character
Password Changed Successfully
Your password has been changed
- Sign in / Register
Request Username
Can't sign in? Forgot your username?
Enter your email address below and we will send you your username
If the address matches an existing account you will receive an email with instructions to retrieve your username
Peer Instruction
- Jennifer K. Knight
- Cynthia J. Brame
Department of Molecular, Cellular, and Developmental Biology, University of Colorado, Boulder, CO 80309
Search for more papers by this author
*Address correspondence to: Cynthia J. Brame ( E-mail Address: [email protected] ).
Center for Teaching and Department of Biological Sciences, Vanderbilt University, Nashville, TN 37203
Peer instruction, a form of active learning, is generally defined as an opportunity for peers to discuss ideas or to share answers to questions in an in-class environment, where they also have opportunities for further interactions with their instructor. When implementing peer instruction, instructors have many choices to make about group design, assignment format, and grading, among others. Ideally, these choices can be informed by research about the impact of these components of peer instruction on student learning. This essay describes an online, evidence-based teaching guide published by CBE—Life Sciences Education at http://lse.ascb.org/evidence-based-teaching-guides/peer-instruction . The guide provides condensed summaries of key research findings organized by teaching choices, summaries of and links to research articles and other resources, and actionable advice in the form of a checklist for instructors. In addition to describing key features of the guide, this essay also identifies areas in which further empirical studies are warranted.
INTRODUCTION
Peer instruction is a well-researched active-learning technique that has been widely adopted in college science classes. In peer instruction, the instructor poses a question with discrete options and gives students the chance to consider and record their answers individually, often by voting using clickers. Students then discuss their answers with neighbors, explaining their reasoning, before being given a chance to vote again. Finally, the instructor discusses the answer to the question, often soliciting input from the class. While instructors vary the exact implementation of this process—sometimes eliminating the individual voting process, sometimes using colored cards or a show of hands instead of clickers—the general process is an adaptation of the think–pair–share technique ( Crouch and Mazur, 2001 ).
Peer instruction can improve students’ conceptual understanding and problem-solving skills, an effect that has been observed in multiple disciplines, in courses at different levels, and with different instructors (for a review, see Vickrey et al. , 2015 ). Student response to peer instruction is generally positive; students report that the technique helps them learn course material and that the immediate feedback it provides is valuable.
Peer instruction’s value as a teaching approach is unsurprising, as it incorporates many elements known to promote learning. It is a form of cooperative learning, which has been shown to increase student achievement, persistence, and attitudes toward science (e.g., Johnson and Johnson, 2009 ). The peer instruction cycle provides opportunities for all the elements that social interdependence theory identify as necessary for cooperative learning: individual action; positive interdependence, wherein individual success is enhanced by the success of other group members; promotive interaction, or actions by individuals to help other group members’ efforts; and group processing ( Johnson and Johnson, 2009 ). It explicitly incorporates opportunities for students to explain their reasoning and engage in argumentation, practices that help students integrate new information with existing knowledge and revise their mental models (e.g., Chi et al. , 1994 ). In addition, as with many types of informal cooperative learning, peer instruction provides opportunities for formative assessment with immediate feedback and thus incorporates opportunities for students to be metacognitive, monitoring their understanding and reflecting on misunderstanding ( McDonnell and Mullally, 2016 ).
In implementing peer instruction, instructors have many choices to make that can impact students’ experience. In this article, we describe an evidence-based teaching guide that condenses, summarizes, and provides actionable advice from research findings (including many articles from CBE—Life Sciences Education ). It can be accessed at http://lse.ascb.org/evidence-based-teaching-guides/peer-instruction . The guide has several features intended to help instructors: a landing page that indicates starting points for instructors ( Figure 1 ), syntheses of observations from the literature, summaries of and links to selected papers ( Figure 2 ), and an instructor checklist that details recommendations and points to consider. The guide is meant to aid instructors as they implement peer instruction and may also benefit researchers new to this area. Some of the questions that serve to organize the guide are highlighted below.
FIGURE 1. Screenshot of the landing page of the guide, which provides readers with an overview of choice points.
FIGURE 2. Screenshot showing a summary of research findings and representative article summaries for one element of peer instruction.
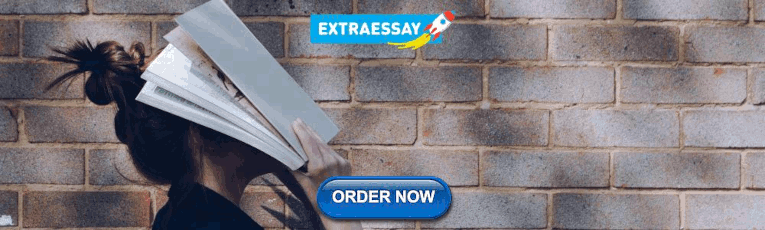
WHAT TYPES OF QUESTIONS SHOULD BE USED?
There are a few clear recommendations about the types of questions that are particularly beneficial in peer instruction. First, questions should be challenging enough to provoke interest and discussion, and the greatest gains are seen with the most difficult questions ( Knight et al. , 2013 ; Zingaro and Porter, 2014 ). Importantly, question difficulty is not necessarily defined by the level of cognitive activity a student engages in to answer the question (e.g., Bloom’s application vs. evaluation levels). Questions that require lower-order cognitive skills can promote as robust peer discussion as those that require higher-order skills, with discussions on both potentially leading to conceptual change ( Knight et al. , 2013 ; Lemons and Lemons, 2013 ). Further, questions that uncover misconceptions can have particular benefits ( Modell et al. , 2005 ), in that they expose students to a commonly held incorrect idea and then give them opportunity to discover why that idea is incorrect.
Are there question types or formats that are particularly effective at helping students meet particular types of outcomes? For example, do questions that ask students to illustrate their ideas, or constructively build theoretical models, impact student learning?
What combinations of question cognitive level (e.g., Bloom’s level) and difficulty help promote self-efficacy, conceptual change, and conceptual understanding? Do different “levels” of questions promote some of these outcomes over others?
WHAT INSTRUCTIONAL PRACTICES PROMOTE PRODUCTIVE PEER INTERACTIONS?
Incentives for students to participate in peer instruction increase student engagement. Low-stakes grading incentives, in which correct and incorrect answers receive equal or very similar credit, result in more robust exchanges of reasoning and more equitable contribution of all group members to the discussion, whereas high-stakes grading incentives tend to lead to dominance of the discussion by a single group member (e.g., James, 2006 , and others within the Accountability section of the guide). Social incentives can also impact peer discussion. For example, randomly calling on groups to explain reasoning for an answer rather than asking for volunteers increases exchanges of reasoning during peer discussion ( Knight et al. , 2016 ).
Instructor cues that encourage students to explain their reasoning influence both student behavior and the classroom norms that students perceive. Thus, these cues can have a large impact on the nature of peer discussion ( Turpen and Finkelstein, 2010 , and others in the Instructional Cues section of the guide). Specifically, instructor language that encourages students to explain their reasoning can lead to higher-quality peer discussion and greater use of scientific argumentation moves ( Knight et al. , 2013 ). Further, instructor-led discussion of the answer after peer discussion provides clear benefits, particularly for weaker students and on more difficult questions ( Smith et al. , 2009 , 2011 ; Zingaro and Porter, 2014 ).
One common practice may have unintended negative consequences. Traditional implementation of peer instruction involves displaying the histogram of student responses after students answer individually but before peer discussion. Several lines of work suggest that this practice may bias students toward the most common answer and reduce the value of peer discussion ( Perez et al. , 2010 ). Thus, instructors may choose to prompt peer discussion that focuses on reasoning before showing the response histogram, and only use the histogram as a summary of student choices after students have shared their reasoning.
One of the steps that is most commonly omitted during peer instruction is the individual response ( Turpen and Finkelstein, 2009 ). Students have been reported to prefer the inclusion of individual thinking time, and it appears to increase discussion time ( Nicol and Boyle, 2003 ; Nielsen et al. , 2014 ). What is the role of this step in promoting productive peer discussion? Can objective measures of student learning be applied to determine its efficacy? ( Vickrey et al. , 2015 ).
Several studies indicate that students prefer to use personal response devices during peer instruction but that their use does not appear to impact students’ learning when compared with other reporting methods (such as a show of hands or colored cards). The role of anonymity and its potential relationship to stereotype threat has not been investigated, however. Can peer instruction induce stereotype threat, and if so, can the effect be mitigated by an anonymous reporting device or by other instructor interventions?
Further, stereotype threat is most relevant when people are working at the edge of their ability ( O’Brien and Crandall 2003 ), and it therefore seems more likely to be a factor for more difficult peer instruction questions. While active-learning approaches have generally been shown to be particularly effective for students from underrepresented groups (e.g., Eddy and Hogan, 2014 ), investigating the nuanced effects within particular groups of students can help instructors make effective choices ( Eddy et al. , 2015 ). Can personal response devices, which afford anonymity, have particular value for more difficult questions?
WHAT CHALLENGES ARE ASSOCIATED WITH PEER INSTRUCTION?
Finally, it is important to note that there can be challenges to implementing peer instruction. As noted earlier, instructors implement peer instruction differently, leading to classroom norms that can work to enhance or detract from student learning and affect student perceptions. Further, students have many different kinds of discussions during peer instruction, not all focused on the topic and not all centered around the concepts instructors intend. By its very nature, peer instruction allows exposure to others’ ideas, which can lead to better understanding but also potentially to shared misconceptions, an effect that may be enhanced among students who feel less confident in the classroom. Thus, the peer discussion part of each clicker question cycle is truly the key to successful peer instruction. Perhaps due to the reasons cited above, peer instruction does not uniformly improve students’ course grades. However, it clearly improves students’ use of reasoning and argumentation skills ( Knight et al. , 2013 , 2016 ), which may contribute to student learning in nonobvious ways. Avoiding the pitfalls discussed in this article and maximizing the benefits of peer instruction require that instructors carefully construct challenging questions and intentionally promote classroom norms that value reasoning and argumentation.
ACKNOWLEDGMENTS
We acknowledge and thank Adele Wolfson and Kristy Wilson for their thoughtful review. We also thank William Pierce and Thea Clarke for their efforts in producing the Evidence-Based Teaching Guide website.
- Chi, M. T. H., de Leeuw, N., Chiu, M-H., & Lavancher, C. ( 1994 ). Eliciting self-explanations improves understanding . Cognitive Science , 18 , 439–477. Google Scholar
- Crouch, C. H., & Mazur, E. ( 2001 ). Peer instruction: Ten years of experience and results . American Journal of Physics , 69 , 970. 10.1119/1.1374249 Google Scholar
- Eddy, S. L., Brownell, S. E., Thummaphan, P., Lan, M-C., & Wenderoth, M. P. ( 2015 ). Caution, student experience may vary: Social identities impact a student’s experience in peer discussions . CBE—Life Sciences Education , 14 , ar45. Link , Google Scholar
- Eddy, S. L., & Hogan, K. A. ( 2014 ). Getting under the hood: How and for whom does increasing course structure work . CBE—Life Sciences Education , 13 , 453–468. Link , Google Scholar
- James, M. C. ( 2006 ). The effect of grading incentive on student discourse in peer instruction . American Journal of Physics , 74 , 689. Google Scholar
- Johnson, D. W., & Johnson, R. T. ( 2009 ). An educational psychology success story: Social interdependence theory and cooperative learning . Educational Research , 38 , 365–379. Google Scholar
- Knight, J. K., Wise, S. B., & Sieke, S. ( 2016 ). Group random call can positively affect student in-class clicker discussions . CBE—Life Sciences Education , 15 (4), ar56. Link , Google Scholar
- Knight, J. K., Wise, S. B., & Southard, K. M. ( 2013 ). Understanding clicker discussions: Student reasoning and the impact of instructional cues . CBE—Life Sciences Education , 12 , 645–654. Link , Google Scholar
- Lemons, P. P., & Lemons, J. D. ( 2013 ). Questions for assessing higher-order cognitive skills: It’s not just Bloom’s . CBE—Life Sciences Education , 12 , 47–58. Link , Google Scholar
- McDonnell, L., & Mullally, M. ( 2016 ). Research and teaching: Teaching students how to check their work while solving problems in genetics . Journal of College Science Teaching , 46 , 68–75. Google Scholar
- Modell, H., Michael, J., & Wenderoth, M. P. ( 2005 ). Helping the learner to learn: The role of uncovering misconceptions . The American Biology Teacher , 67 , 20. Google Scholar
- Nicol, D. J., & Boyle, J. T. ( 2003 ). Peer instruction versus class-wide discussion in large classes: A comparison of two interaction methods in the wired classroom . Studies in Higher Education , 28 , 458–473. Google Scholar
- Nielsen, K. L., Hansen, G., & Stav, J. B. ( 2014 ). How the initial thinking period affects student argumentation during peer instruction: Students’ experiences versus observations . Studies in Higher Education , 3 , 1–15. Google Scholar
- O’Brien, L. T., & Crandall, C. S. ( 2003 ). Stereotype threat and arousal: Effects on women’s math performance . Personality and Social Psychology Bulletin , 29 , 782–789. Medline , Google Scholar
- Perez, K. E., Strauss, E. A., Downey, N., Galbraith, A., Jeanne, R., & Cooper, S. ( 2010 ). Does displaying the class results affect student discussion during peer instruction . CBE—Life Sciences Education , 9 , 133–140. Link , Google Scholar
- Smith, M. K., Wood, W. B., Adams, W. K., Wieman, C., Knight, J. K., Guild, N., & Su, T. T. ( 2009 ). Why peer discussion improves student performance on in-class concept questions . Science , 323 , 122–124. Medline , Google Scholar
- Smith, M. K., Wood, W. B., Krauter, K., & Knight, J. K. ( 2011 ). Combining peer discussion with instructor explanation increases student learning from in-class concept questions . CBE—Life Sciences Education , 10 , 55. Link , Google Scholar
- Turpen, C., & Finkelstein, N. D. ( 2009 ). Not all interactive engagement is the same: Variations in physics professors’ implementation of peer instruction . Physical Review Special Topics–Physics Education Research , 5 , 020101. Google Scholar
- Turpen, C., & Finkelstein, N. D. ( 2010 ). The construction of different classroom norms during peer instruction: Students perceive differences . Physical Review Special Topics–Physics Education Research , 6 , 020123. Google Scholar
- Vickrey, T., Rosploch, K., Rahmanian, R., Pilarz, M., & Stains, M. ( 2015 ). Research-based implementation of peer instruction: A literature review . CBE—Life Sciences Education , 14 , es3. Link , Google Scholar
- Zingaro, D., & Porter, L. ( 2014 ). Peer instruction in computing: The value of instructor intervention . Computers & Education , 71 , 87–96. Google Scholar
- Tab-Meta Key: A Model for Exam Review 30 January 2024 | Journal of College Science Teaching, Vol. 53, No. 1
- Differentiated Instruction as a Viable Framework for Meeting the Needs of Diverse Adult Learners in Health Professions Education 28 June 2023 | Medical Science Educator, Vol. 33, No. 4
- Incorporating Technologies to Achieve Active Learning in Large Engineering Classrooms 19 May 2023
- Simplifying Texts for Easier Comprehension in an Introductory Computer Science Course: An Evaluation of Rewordify 28 July 2023
- The Future of Higher Education: Identifying Current Educational Problems and Proposed Solutions 3 December 2022 | Education Sciences, Vol. 12, No. 12
- Student Response Systems in Online Nursing Education 1 Dec 2022 | Nursing Clinics of North America, Vol. 57, No. 4
- Reactions of Computer Science Students to Peer Instruction Activities in Class 1 Dec 2022
- Implementing Design Thinking and Peer Instruction in an Online Environment to Develop Tourism Undergraduates’ English Language Skills 1 March 2023 | Journal of Language and Cultural Education, Vol. 10, No. 2
- Review of Trends in Peer Instruction: Bibliometric Mapping Analysis and Systematic Review 13 January 2022 | Journal of Learning and Teaching in Digital Age, Vol. 7, No. 1
- 2022 | Education and Information Technologies, Vol. 27, No. 4
- 2022 | Academic Psychiatry, Vol. 46, No. 4
- Applying Peer Instruction to Computer Science Students Using Non-native Language: A Study with Undergraduate Students 5 Dec 2021
- Connecting Activity Implementation Characteristics to Student Buy-In Toward and Utilization of Formative Assessments Within Undergraduate Biology Courses 8 June 2021 | Journal for STEM Education Research, Vol. 4, No. 3
- Group Size and Peer Learning: Peer Discussions in Different Group Size Influence Learning in a Biology Exercise Performed on a Tablet With Stylus 10 November 2021 | Frontiers in Education, Vol. 6
- An agile approach to teach introductory programming in the hybrid classroom 1 Jul 2021
- Learning by Creating: Making Games in a Political Science Course 10 December 2020 | PS: Political Science & Politics, Vol. 54, No. 2
- The Impact of Peer Instruction on Ninth Grade Students’ Trigonometry Knowledge 1 January 2021 | Bolema: Boletim de Educação Matemática, Vol. 35, No. 69
- Guiding the use of collective feedback displayed on heatmaps to reduce group conformity and improve learning in Peer Instruction 19 June 2020 | Journal of Computer Assisted Learning, Vol. 36, No. 6
- Meeting the Needs of A Changing Landscape: Advances and Challenges in Undergraduate Biology Education 13 May 2020 | Bulletin of Mathematical Biology, Vol. 82, No. 5
- Peer Interaction in Active Learning Biology 24 February 2020
- 2020 | Informação & Informação, Vol. 25, No. 3
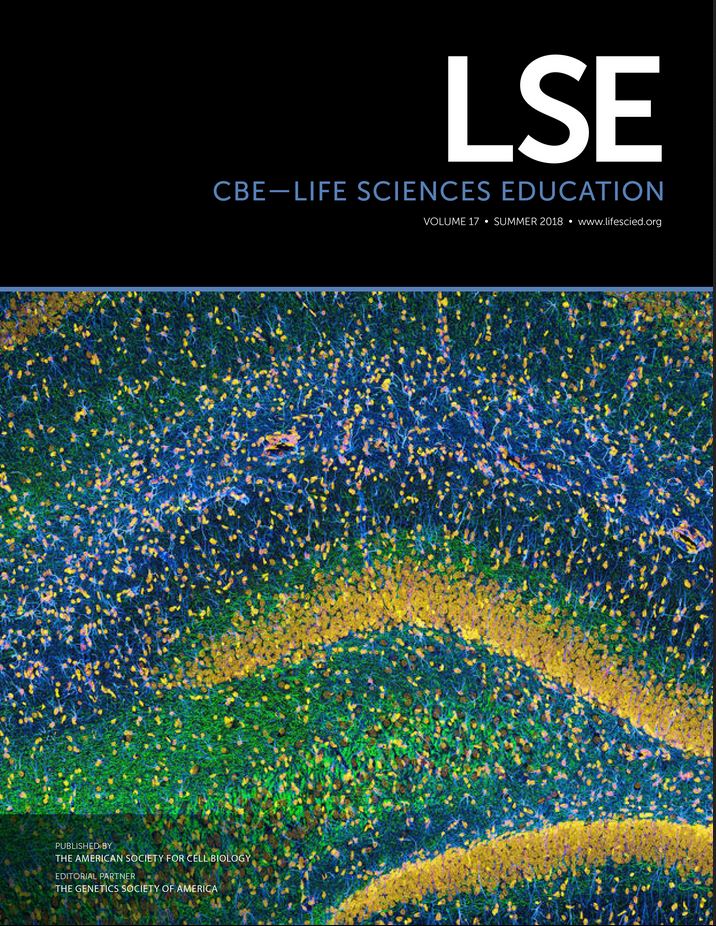
© 2018 J. K. Knight and C. J. Brame. CBE—Life Sciences Education © 2018 The American Society for Cell Biology. This article is distributed by The American Society for Cell Biology under license from the author(s). It is available to the public under an Attribution–Noncommercial–Share Alike 3.0 Unported Creative Commons License (http://creativecommons.org/licenses/by-nc-sa/3.0).
Your browser is unsupported
We recommend using the latest version of IE11, Edge, Chrome, Firefox or Safari.
Center for the Advancement of Teaching Excellence
Peer feedback on your teaching.
Crystal Tse, CATE Associate Director June 13, 2022
WHAT? Heading link Copy link
Good instruction involves using data to assess the impact of, and inform improvements to your teaching, known as “reflective teaching” (Brookfield 2017) .
One aspect of reflective teaching is peer feedback. The best known form of peer feedback is peer teaching observations, but it can take on many forms.
Peers (colleagues, mentors, and other instructors) can give you valuable feedback on different facets of your teaching:
- Instruction in the classroom: This is where a colleague will visit one of your class sessions and provide feedback on your teaching strategies demonstrated in the session.
- Course materials: Your peers can also review your course syllabus, assessments (e.g., assignments, tests, quizzes), and materials you use during course delivery (e.g., powerpoint slides, assigned readings, videos)
- Learning management system course site (i.e., Blackboard) and online teaching materials: If you are teaching portions of, or all of your course online, a peer can review your course site (e.g., organization and design of the course) as well as your use of educational technology and opportunities you have provided for students to interact with you and each other.
- Teaching portfolio: Your peer can also provide feedback on broader aspects of your teaching beyond the classroom (e.g., teaching statement, student evaluations, scholarly teaching activity, etc.)
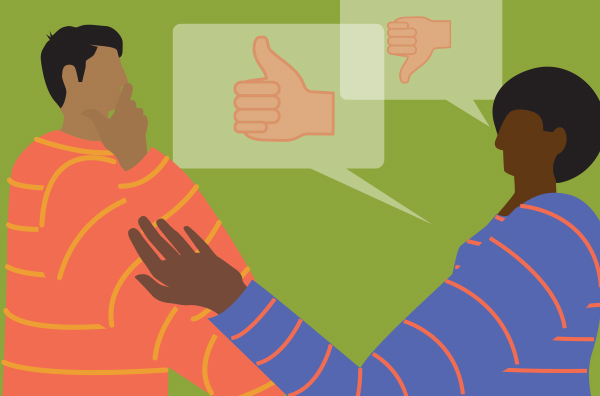
In this teaching guide, you will learn more about:
- Benefits of getting feedback from peers on your teaching
- Best practices and recommendations for getting peer feedback
- Different instruments for conducting peer observations
- Principles of giving and receiving effective feedback
WHY? Heading link Copy link
Engaging in peer review of teaching comes with several benefits..
- Gain a sense of community: Instructors may often feel as if they are teaching and dealing with the challenges of this work in isolation. Soliciting and giving feedback to your peers can help to break down silos and create community by sharing things that have worked well and lessons learned with your colleagues (Hutchings, 1996).
- Go beyond student course evaluations for feedback: Recent research has called into question the validity of, and common practice of solely using course evaluations to measure teaching effectiveness (see Spooren et al., 2013 and Stark & Freishtat, 2014 for a review). It is important to consider collecting data from different sources to ensure that all the feedback you are collecting reveals a similar story and outcomes. You may also consider reviewing your student course evaluations with a peer, with a focus on qualitative feedback, to gain another perspective on the feedback you are getting from students. See CATE’s teaching guide on Student Feedback for further reading on this topic.
- Observing your peers can also help enhance your teaching: If you take on the role as a peer reviewer, observing your colleague’s teaching techniques can spark new ideas for your own teaching. One way to do this is engaging in teaching squares , a program that typically involves a group of 4 instructors (in the same or different disciplines) who visit each other’s classes during the semester (Haave, 2018). The instructors meet regularly to discuss their observations – not to provide evaluative feedback but to gather ideas that they might want to implement in their own courses.
HOW? Heading link Copy link
Best practices/recommendations:.
- Set goals for using peer feedback. How do you intend on using this feedback? For example, will this feedback be used informally to improve your teaching? To provide evidence of your growth and excellence as an instructor for promotion and advancement purposes? To be used with your department on setting norms for what effective teaching looks like?
- How do you and your department define effective teaching? The approach to peer feedback on teaching varies widely by department and college, and there isn’t a one-size-fits-all approach. One question to consider is how your department defines effective teaching – what are your department’s expectations on teaching? Consider looking to the literature and evidence-based teaching practices to create this definition. Department members may need to come to consensus, establish expectations, and develop a classroom observation instrument together as a department. By creating a shared definition of effective teaching and the process by which to provide feedback, you ensure that the process is fair, rigorous, and constructive for the instructor.
- Evaluative and non-evaluative peer feedback: Expectations should be set in the department prior to peer review about how the peer observation report will be used. Peer review of teaching can be non-evaluative, where the focus is on improving and developing one’s teaching, or evaluative, where materials are used for promotion and tenure and advancement decisions. Questions to consider are: Who will own the final peer review report? What will the report and feedback be used for?
- What other sources of data do you want to collect? A classroom observation provides just one snapshot of your course during one semester of your teaching. Consider doing a classroom observation more than once, and conducting observations across multiple courses and over time, to help you reflect on how you have developed your teaching. The evaluation of teaching should be holistic and include multiple forms of data (feedback from students and peers, self-assessment, and looking at the education research literature) that tell a coherent narrative about your teaching.
Example Peer Observation Protocol Heading link Copy link
Example peer observation protocol.
The peer observation protocol will differ by department and/or college, depending on their departmental norms and processes that are in place. We show one example of a peer observation protocol with best practices and recommendations adapted from the University of Oregon , Indiana University Bloomington , UC Berkeley , and Vanderbilt University . Think about how you might adapt this protocol to suit the needs of your teaching and/or your department.
Pre-classroom observation meeting
Meet with your peer prior to the classroom observation. During this meeting, you can orient your peer to the course and your goals for the observation:
- A specific teaching technique, or an issue arising in the classroom?
- How you have engaged with students, or your assessment practices?
- How you have handled technology in the classroom (online or in-person)?
- What are the intended learning objectives of the course
- How is the physical classroom space structured?
- How does the class session fit with the rest of the course?
- Prior knowledge and experience with the course content
- Motivation to take your course
- Concerns or expectations about taking your course
- General interests and academic and career goals
- Theories used to inform your teaching and teaching practices you typically use
- Your strengths and areas where you want to make improvements
Classroom observation
When taking on the role as the observer, we recommend you:
- Use a structured observation instrument (please see the next section for examples of instruments). Having structure will help guide your observations and feedback that you want to note down for your peer.
- Arrive at the class session ahead of time to ensure you are prepared and ready to take notes, and stay for the whole class session.
- Don’t intervene during the class session – be an impartial observer.
During the class session, what do you want to observe and take note of? Here are some examples:
- Course alignment: Does the course content match students’ prior knowledge and skills? Are the course activities and assessments aligned with the course learning objectives?
- Instructor to student interaction: Does the instructor foster a welcoming classroom climate? Does the instructor provide students opportunities to ask questions and contribute to the class?
- Student engagement: Does the instructor use multiple methods of student engagement (individual and/or group activities)? Do students actively participate in class by asking questions or interacting with other students?
- Organization: Does the instructor explicitly state the purpose of each class activity? Does the instructor help to prepare students for the next class session?
- Presentation: Is the instructor’s pace and volume of speaking appropriate to the course content? Does the instructor vary lecturing with active learning strategies?
- Assessment of student learning (e.g., student coursework and artifacts)
- Student course evaluations (paying attention to qualitative comments for common themes)
- Student academic advising and other teaching service activities
- Awards and recognition of teaching
- Engagement in teaching professional development (e.g., attending CATE programming)
- Engagement in scholarly teaching activities (i.e., conducting research on teaching and student learning)
Post-classroom observation meeting
Consider setting up a meeting after the classroom observation to talk about how the class session went. Bring your own questions and reflections on how you believe the course session went to this meeting to see if there are any differences or commonalities, and to provide a starting point for your discussion.
- What does your peer think went well?
- What areas do they recommend that you work on?
- With your peer, create goals and next steps you want to take in your teaching
Feedback letter/report
As the reviewer, you may be asked to write a feedback letter or report based on the classroom observation that can be placed in the instructor’s file, or to be used for feedback to improve teaching depending on your departmental norms. When writing this letter or report, consider doing the following:
- Outline the steps taken in the peer review process and instrument(s) used to conduct the observation
- Use information collected from the observation instrument you used to summarize the instructor’s teaching approach
- Provide the instructor with constructive and actionable feedback
Example observation instruments Heading link Copy link
Example observation instruments.
When selecting, adapting, or creating your peer observation instrument, consider what will work best for you for the kind of feedback you wish to receive – what questions do you have about your teaching? What are your departmental norms for how observations are typically conducted? Observation instruments vary in their structure, and can be based on a rubric or checklists of teaching strategies, measure the frequency of student and instructor behaviors, or be open-ended.
Rubric-based
University of Kansas Benchmarks for Teaching Effectiveness : This rubric aims to provide an instructor with holistic feedback on their teaching by including a range of activities and sources of data on teaching, such as alignment of course goals and instructional practices, creating motivating and inclusive learning climates, reflecting on how instructors’ teaching practices have changed over time, and involvement in student advising and scholarly teaching activities.
In addition to using it as a peer observation/review instrument, it can also be used as a self-reflection tool for instructors. The creators of this rubric encourage departments to modify the rubric as needed for their specific contexts.
Checklist-based
You can create or adapt a checklist of teaching strategies and behaviors, where your peer can look for evidence of the use of these strategies during the classroom observation.
For example, the CATE Inclusive Teaching Toolkit contains several different checklists specific to teaching strategies for inclusive teaching, building community, and culturally-responsive instruction.
You can use an open-ended observation instrument, that is based on answering specific questions or addressing specific themes on teaching. Here are some examples:
- UIC LAS Sample peer teaching evaluation review questions : Resource from UIC’s College of LAS with example questions you can answer during a peer observation.
- University of Oregon : This instrument contains specific evidence-based teaching practices (see a References list at the bottom of the document). As the reviewer, you would perform a “fact-based” observation, recording what instructors and students do with specific examples, and adding comments with your feedback and suggestions for improvement.
- University of California, Los Angeles : You can address specific themes on teaching (e.g., student engagement, class organization) in this tool. There is also a fact-based supplementary tool where you can note observations for every 5-10 minute segment of the class by looking for specific teaching strategies used. These notes can then be used to populate the open-ended section when giving the instructor feedback.
Frequency of behaviors
The Classroom Observation Protocol for Undergraduate STEM (COPUS) (Smith et al., 2013) is an observation instrument used in real-time to give you data on the frequency and range of teaching practices happening in the classroom. The COPUS was designed for undergraduate STEM teaching, but can be used for non-STEM classrooms as well to collect information on teaching practices being used. After a brief training period (1.5 hours) to use the tool, as an observer you can note what students are doing (e.g., listening, problem-solving, asking questions) and what instructors are doing (e.g., lecturing, writing, posing clicker questions) during the class session.
The GORP tool (Stains et al., 2018) is an app you can download to integrate COPUS onto mobile and desktop devices, giving you immediate access to quantifiable data.
The Protocol for Advancing Inclusive Teaching Efforts (PAITE) is another teaching observation instrument used in real-time to give you data on different observable inclusive teaching strategies. Observers can become trained on the PAITE observation codes (e.g., using diverse examples, incorporating growth mindset language, ensuring equitable participation, etc.) and practice coding with provided vignettes. The resource includes an observation template form, a data visualization tool for observers to create visualizations of inclusive teaching practices, and a post-observation report template.
Considerations for Your Course Context Heading link Copy link
Considerations for your course context.
When making your plan to get peer feedback on your teaching, consider the following questions:
- What questions do you want answered about your teaching? Are you looking for support on troubleshooting a specific issue arising in the classroom? Feedback on a new teaching strategy you are using? Depending on the questions you have about your teaching, what peer observation instruments (existing, or that you would like to adapt) make most sense for you?
- University of Kansas: How to conduct a peer review of online teaching
- Penn State: A Peer Review Guide for Online Courses at Penn State
- University of Oregon Peer Review template for courses across all modalities (in-person, online, etc)
- How much time and resources do you have? Engaging in the peer review process can take a significant amount of time (e.g., choosing an observation instrument, meeting with your colleague prior to the classroom observation). Consider doing this once or twice during the semester that works best for your schedule.
- Who would you like to ask to provide feedback? Instructors typically ask for feedback on their teaching from those in their department, but you can also consider asking a colleague from a different department to provide feedback (see the teaching squares model). Consider asking a peer who is familiar with your course content, or has significant teaching experience. Importantly, consider asking a colleague whom you trust and who will be able to provide you with constructive feedback on your teaching (Bandy, 2015).
Giving and Receiving Effective Feedback Heading link Copy link
Giving and receiving effective feedback.
When serving as a peer reviewer, how should you frame your feedback to your peer so that this feedback is heard and understood as well as possible?
Principles of Effective Feedback
Consider how your comments will best serve to develop your colleague’s teaching. Your feedback should be:
- Prioritized. Prioritize comments that will serve to develop your colleague receiving feedback. For example, what do they need to work on most? How will your feedback help them improve upon for example, specific teaching strategies, classroom management, or the design of their formative and summative assessments?
- Specific rather than general. Giving feedback with concrete examples will help your colleague reflect on their teaching practices and behaviors, whereas feedback that may be more general or vague may be confusing and difficult to implement in a practical way. What are a few concrete changes that the instructor can implement right away in their course, or the next time they offer the course?
- Descriptive rather evaluative. We encourage you to avoid judgmental terms such as “good” or “bad”, and focus on the teaching content or practices. When giving feedback, refer to what the instructor is doing, rather than suggesting reasons for their actions.
- Balanced. Provide both positive feedback on things that the instructor is doing well, in addition to suggestions for improvement.
- Manageable. The feedback you provide should not overwhelm your colleague. Focus on a few key areas that the instructor can work on and realistically change in their next offering of the course.
Types of Feedback
There are a few different types of feedback you can give to your peer, including warm, cool, and hard feedback (taken from the School Reform Initiative resource ):
- Warm : Warm feedback highlights your peer’s strengths in teaching. It recognizes specific strategies, actions, or behaviors that you saw your peer engage in in their classroom. Example: “I appreciated how you engaged with your students using a variety of polling and group activities.”
- Cool: Cool feedback analyzes and probes your peer’s teaching. Example: “I think I know your intention for this learning activity, but I’m not sure that was what the actual outcome was for your students.”
- Hard: Hard feedback presents challenges for your peer and is meant to extend what your peer is doing. Example: “Who does this learning activity benefit? Who might this activity present barriers to? How might you change the activity to reach all students?”
Giving and Receiving Feedback
When giving feedback to a peer, consider:
- Giving positive feedback first. Let your peer know both their strengths and areas for improvement. Positive feedback will allow your peer to continue to build on their existing teaching skills.
- Focusing on behaviors that are observable and changeable. How will your feedback serve your peer in making concrete changes to their instruction that will benefit students?
- Prioritizing comments that will serve to enhance your peer’s teaching.
When receiving feedback from a peer, consider:
- Focusing on the positive feedback. It may be challenging or upsetting to receive critical feedback. Allow yourself the time and space to process this feedback.
- Confirming your hearing of specific suggestions. Do you understand your peer’s feedback and suggestions? Do you know what your next steps are, if you are to make any changes to your teaching practice?
- Prioritizing the feedback based on importance and feasibility. Taking into consideration your time and resources, think about what is most important to you, and what is realistic to change in your teaching (now, or in the next offering of the course).
CITING THIS GUIDE Heading link Copy link
Citing this guide.
Tse, Crystal (2022). “Peer Feedback on Your Teaching.” Center for the Advancement of Teaching Excellence at the University of Illinois Chicago. Retrieved [today’s date] from https://teaching.uic.edu/resources/teaching-guides/reflective-teaching-guides/peer-feedback-on-your-teaching/
ADDITIONAL RESOURCES Heading link Copy link
Peer review best practices and example procedures:
- Guide to peer review (University of California, Berkeley)
- Best practices for peer review of teaching (Vanderbilt University)
- Peer review of teaching process and general guidelines (Indiana University Bloomington)
- Peer review of teaching resources and example instruments (University of Minnesota)
Example checklist of teaching strategies and self-assessment tool:
- Teaching Self-Reflection Tool (pgs 6-7) and Skills Checklist (Thomas Jefferson University): Open-ended questions and a checklist of teaching practices
REFERENCES Heading link Copy link
Bandy, J. (2015). Peer Review of Teaching . Vanderbilt University Center for Teaching.
Bernstein, D. J., Jonson, J., & Smith, K. (2000). “ An Examination of the Implementation of Peer Review of Teaching .” New Directions for Teaching and Learning, 83, 73-86
Brookfield, S. (2017). Becoming a Critically Reflective Teacher. (2nd ed.). Jossey Bass.
Hutchings, P. (1996). The peer collaboration and review of teaching . American Council of Learned Societies (ACLS) Occasional Paper No. 33.
Smith, M., Jones, F., Gilbert, S., & Wieman, C. (2013). The classroom observation protocol for undergraduate stem (COPUS): A new instrument to characterize university STEM classroom practices . CBE-Life Sciences Education, 12(4), 618-727
Spooren, P., Brock, B., & Mortelmans, D. (2013). On the validity of student evaluation of teaching the state of the art . Review of Educational Research, 83(4), 598-642.
Stains, M., et al. (2018). Anatomy of STEM teaching in North American universities . Science, 359(6383), 1468-1470.
Stark, P. B., & Freishtat, R. (2014). An evaluation of course evaluations . ScienceOpen Research.
- Request a Consultation
- Workshops and Virtual Conversations
- Technical Support
- Course Design and Preparation
- Observation & Feedback
Teaching Resources
Planning and Guiding In-Class Peer Review
Resource overview.
How to plan and guide in-class peer review.
Incorporating peer review into your course can help your students become better writers, readers, and collaborators. However, peer review must be planned and guided carefully.
The following suggestions for planning and guiding peer review are based on our approach to peer review. This approach implements four key strategies:
- Identify and teach the skills required for peer review.
- Teach peer review as an essential part of the writing process.
- Present peer review as an opportunity for students to learn how to write for an audience.
- Define the role of the peer-reviewer as that of a reader, not an evaluator.
These tips are are organized in four areas:
Before the Semester Starts
During the semester and before the first peer-review session, during and after peer-review sessions, peer review is challenging work.
The Center for Teaching and Learning provides sample worksheets (Peer Review Worksheet for Thesis-Driven Essay) that may be adapted to suit various types of courses and genres of writing.
1. Determine how peer review will fit into the course.
A. Decide which writing assignments will include a peer-review session. Given the time that is required to conduct peer-review sessions successfully (see below), in undergraduate courses, peer review will work best with papers of 5 pages or less. Instructors who want to incorporate peer-review sessions for longer papers will have to ask students to complete part of the work outside of class (e.g. reading peers’ papers and preparing written comments); such an approach is likely to be more successful if students first practice peer review during class, with the guidance of the instructor.
B. Decide when peer-review sessions will occur. The ideal time for peer review is after students have written a complete draft of a paper, but while there is still time for substantial revision.
Each peer-review session will require at least one class period. While it is possible to complete a session in one hour, a one-and-one-half hour class period is preferable (see below for a detailed discussion of how to structure peer-review sessions).
As you look over your course schedule, make time for a “mock” peer-review session before you ask students to review one another’s writing, so that they can learn to identify and begin practicing the skills necessary for peer review. Before the semester begins, furthermore, you should find a short sample paper that will serve as the focus of the “mock” peer review. You can also write this short paper yourself (for more detailed suggestions on how to set up a mock peer-review session, see below).
Instructors should schedule the first peer-review session early in the semester to give students time to get to know one another and to develop peer-review skills. The atmosphere of trust and mutual respect that is necessary for the success of peer-review sessions does not develop instantaneously. Ideally, the first peer-review session should focus on a short piece of writing, such as a paragraph or two, so that students develop comfort with giving and receiving feedback before taking on the task of reading longer papers.
2. Design peer-review worksheets that students will complete during each peer-review session.
These worksheets should include specific tasks that reviewers should complete during the session. The guidance you provide on the worksheets should help students stay “on task” during the session and should help them discern the amount of commenting that is desirable.
The role of the peer-reviewer should be that of a reader, not an evaluator or grader. Do not replicate the grading criteria when designing these worksheets. Your students will not necessarily be qualified to apply these criteria effectively, and they may feel uncomfortable if they are given the responsibility to pronounce an overall judgment on their peers’ work.
Peer-review worksheets should ask the reviewer to begin by offering a positive comment about the paper. After that point, the peer-reviewer role in commenting should be descriptive: each reviewer should describe his response to the paper. For example, a peer-reviewer might write: “I found this description very clear” or “I do not understand how this point relates to your thesis.” The worksheet should give students specific tasks to complete when recording their response to a paper (Nilson 2003). Where evaluation is required, it should be based on the reviewer’s impressions as a reader. Examples of specific tasks include:
- Indicate which parts of the paper the reader finds most or least effective, and why
- Identify or rephrase the thesis
- List the major points of support or evidence
- Indicate sentences or paragraphs that seem out of order, incompletely explained, or otherwise in need of revision
Performing these tasks should enable each peer-reviewer to provide the writer with a written response that will help the writer determine which parts of the paper are effective as is, and which are unclear, incomplete, or unconvincing.
Do not require students to tell the writer how to revise the paper. Advanced undergraduates, students who have been meeting in peer-review groups for an extended time, and graduate students may be able to handle adding more directive responses (e.g. suggesting that the writer make specific changes).
3. During the course-planning process, think carefully about the kind of comments that you will provide students when you review drafts and grade papers.
With your comments, you can model for your students the qualities you would like to see reflected in their comments as peer-reviewers. For example, you can give them examples of comments that are descriptive and specific.
4. Decide whether and how you will grade students’ contributions to peer-review sessions.
One way to communicate to students the importance of peer review and the skills it requires is to grade their contributions to the peer-review process. If you do grade students’ performances in peer review, you will need to decide ahead of time what exactly you will be grading and what criteria you will use to judge their achievement. Furthermore, you might decide to use a straightforward -√/ √/ √+ system, or you might assign a point-value to different aspects of the work required for peer review. You should then decide how to incorporate each peer-review score into the course grade or into the grade earned for each paper.
The following example illustrates a point-system approach to grading student performance in peer review:
Brought 2 copies of paper to class: 5 pts Provided peers with specific, constructive written feedback: 0-5 pts Participated actively in discussion of each paper: 0-5 pts Wrote specific response to peers’ feedback: 0-5 pts Total score for each peer-review session: 0-20 pts.
This example makes it clear that those students who do not bring a draft to be peer-reviewed would nevertheless earn points by acting as reviewers of their peers’ work. Of course, if you use such a point-system, you will need to explain to the students the criteria by which you judge their performance in each category. Providing students with graded examples will help to clarify these criteria.
Whatever you decide regarding whether and how you will grade each student’s performance in peer review, you should observe and evaluate what students are doing during peer review so that you can give them some feedback and suggestions for improvement throughout the semester (see below for further suggestions on how to observe and evaluate peer review).
1. Hold a “mock” peer-review session.
First, copy and distribute a brief sample paper. You can either use a paper submitted by a student in an earlier semester (block-out the name and ask the student’s permission to distribute the paper) or write a sample paper yourself, approximating a draft that would be typical of students in your course. Next, ask students to take 5 minutes to read the paper and 10 minutes to write some comments, using a peer-review worksheet. If time allows, you can ask students to work in groups of 3-4 to produce written comments; if you do so, give them an additional 5-10 minutes for group discussion.
After students have produced written comments individually or as a group, use a document camera or overhead projector to display a blank peer-review worksheet. Then, ask students to present their reviewing comments to the class and use these to write comments on the displayed worksheet. When necessary, follow-up with questions that help the students phrase their comments in more specific and constructive ways. For example, if a student comments, “I like the first paragraph,” you might ask, “can you tell the writer what you find effective or appealing about that paragraph? And why?” Your aim should be to help students understand that the point of their comments should be to describe their experience as readers with specific language, not to praise or condemn their peers or to tell the peer how they would write the paper. Note that while students often hesitate to give specific feedback to a writer face-to-face, they may actually be overly critical when critiquing something written by a writer who is not present. Therefore, it might be helpful to direct students to construct their comments as if the writer were indeed in the room, listening.
2. Teach students how to think about, respond to, and use comments by peer-reviewers.
Just as your students will need to learn and practice the skills involved in providing constructive feedback on their peers’ writing, they will also need to learn how to respond, as writers, to the feedback they receive. Therefore, you might consider including in the “mock” peer-review session, described above, an exercise in which you ask your students to put themselves in the position of the writer and come up with a plan for revision based on the comments that they and their classmates have formulated in response to the sample paper.
Students must learn how to approach a peer-review session with an open mind (and a thick skin, perhaps). Often, undergraduate students go into a peer-review session thinking that their papers are essentially “done” and need to be edited or changed only slightly. Thus they “hear” only those responses that confirm this view and they end up making very few changes to their papers after the peer-review session and before submitting the final draft to the instructor. Alternatively, they can become so discouraged by what they view as a negative response from a peer that they are not able to discern what is useful about those responses.
To help students resist the understandable temptation to become either discouraged or defensive during the peer-review session and to help them focus on listening carefully to their peers’ comments, it is useful to institute a rule that prohibits writers from speaking when peer-reviewers are offering feedback. An exception might be made in a case in which the writer does not understand a reviewer’s comments and needs to ask for more information.
In addition, instructors should require each writer to respond in writing to their peers’ comments. This written response can be recorded directly on the peer-review worksheet, or it can take the form of an informal letter (addressed to the peer-reviewers). Alternatively, instructors might require each writer to sketch out a plan for revision that 1) indicates any changes she will make in response to the reviewers’ comments and 2) explains any decisions she has made to disregard a specific comment or suggestion.The point of such writing exercises is to ask students to take their peers’ comments seriously and to think carefully about how readers respond to the choices they have made in their writing–even if that means determining that they will decide not to make changes based on those comments.
3. Assign three students to each peer-review group: maintain the same groups throughout the semester.
With groups of three, each student will be reviewing the papers of two peers during each peer-review session, but each group will discuss three papers (for detailed instructions on how to structure each session, see below).
It is best to assign students to groups, rather than to have them define the groups themselves. Students often want to form groups with friends, which may actually create difficulties. As you may want to explain to your students, it can be more difficult to provide honest feedback to a writer when that writer is a friend. Moreover, assigning students to the groups will allow the instructor to ensure that the groups are heterogeneous in terms of, for example, student ability, gender, race, and academic major. Such heterogeneity can enhance student learning in groups (Millis 2002).
Maintaining the groups throughout the semester will help your students build the trust that is necessary for peer review to be successful (Millis 2002). You should only reassign students to another group in the rare case when one or two group members drop the course. You should encourage your students to speak with you if they find that their peer-review groups are not functioning as well as desired, but you should also make it clear that you are interested in helping them find ways to work together to solve whatever problems have surfaced.
4. Ask each student to bring 2 copies of his or her paper to class on the designated day.
You can tell students that these copies are required, but if they do not bring copies of their own paper to class, they should come to class anyway, so that they can act as reviewers of other students’ papers.
1. Structure each peer-review session: give students clear instructions and time limits.
To start each session, distribute peer-review worksheets (see above), explain how students should complete the worksheets, set time limits, and ask each group to designate one person as a time-keeper to make sure that the group stays on schedule.
Peer-review sessions can be accomplished during one-hour classes, but instructors may find that a 90-minute class is preferable. If you teaching a one-hour or 50 minute class, consider asking students to read their peers’ papers before coming to class, then spending the first 10 minutes reviewing the paper and writing comments.
The following is a peer-review schedule that can work in a 90-minute class.
I. When papers are around three pages long, peer-reviewers should spend about 20-25 minutes reading and reviewing each paper: 15 minutes reading the paper (tell students to read each paper twice) and 5-10 minutes writing comments. You should lengthen the time limit when necessary, for instance when papers are longer or when they are written in a foreign language. This schedule will mean that during the first 45-50 minutes of class, each student will be reading and writing comments on papers written by two peers.
II. After all 3 students have finished commenting on the two papers submitted by their peers, the group should then devote 5-10 minutes to a “discussion” of each paper (spending a total of 15-30 minutes discussing three papers). During this discussion, the 2 reviewers should present spoken feedback to the writer. If reviewers feel uncomfortable with providing spoken feedback, they might start by reading their written comments out loud to the writer. Doing so can produce the added benefit of helping the reviewers clarify their written comments. As noted above, the writer of the paper should not speak during this discussion, except perhaps to ask a clarifying question.
2. Take an active role in observing the progress of each group and offering guidance when appropriate.
Even with clear instructions, peer-review sessions can go awry. Circulate throughout the session to make sure that the groups stay focused. Listen carefully to the spoken feedback, and use questions to help students make their comments as specific and descriptive as possible. For example, if you hear a student saying, “I was confused by the third paragraph,” you might prompt them to say more by asking, “Can you tell the writer where you got lost?” or “What word or phrase confused you? Why?” Students will soon learn to supply such details themselves.
Paying attention to how the groups are functioning overall can help you determine whether you need to give additional guidance to the class as a whole. For example, you might tell students that you noticed that many groups seem to be rushing through the spoken feedback period for each paper, and that even reviewers who wrote detailed and constructive comments on the worksheet are giving only cursory responses when speaking to the writer (e.g. “I thought you did a good job,” or “Your paper was interesting”). You might then remind them that they do not need to present an overall judgment of the paper, but they should try to say something specific that can help the writer revise the paper.
3. Have each student submit the completed peer-review worksheets when they turn-in the final drafts of their papers.
Whether or not you are grading the responses that reviewers and writers write on the peer-review worksheets, you should read the completed worksheets to get a sense of what students are actually doing during the peer-review sessions and how they are responding to one another’s comments. Having the students turn in the worksheets also helps you communicate to them that you are taking the peer-review process seriously. Instructors should also give students feedback on their performance during peer review so that they know what they are doing well and what they should try to improve upon.
4. Regularly assess how the peer-review sessions are going; seek and incorporate student input.
You should review completed peer-review worksheets when you grade papers not only to evaluate individual student performance, but also to gauge the success of the peer-review sessions and to determine what you might do to improve them.
Are students writing thoughtful comments that provide an adequate amount of detail? If not, spend some time in class before the next peer-review session giving students suggestions for how to phrase comments in a specific, constructive way.
Are students using the peer-review worksheets to develop thoughtful responses to peer comments? Are they coming up with plans for revision that take into account at least some of their peers’ comments? Again, if needed, give your students additional guidance and in-class activities that will lead them through the process of identifying potential aspects needing revision and coming up with a plan for revision that takes into account peer comments.
Around midterm, ask students to complete anonymous evaluation forms that include questions such as, “What is the most important insight that I have learned as a result of the peer-review process?” and “What can be done (by the instructor or by students, or both) to make the peer-review sessions run more smoothly?”
Be prepared to hear that the peer-review sessions are not functioning as well as you believe they are, and be open to making changes that incorporate your students’ observations and ideas. In other words, model the same open-mindedness to revision that you want them to display as writers during peer review.
Instructors who ask their students to review their peers’ writing should recall how difficult it is–even after years of experience–to accomplish with efficiency the tasks involved in responding to student writing: reading drafts of papers (usually multiple papers at one sitting), quickly discerning each draft’s strengths and most pressing problems, then formulating specific and well written comments that will help the writer improve the paper. It can also be difficult, even for experienced writers, to respond effectively to the comments they receive from reviewers of their work. It is essential, then, that you plan carefully the guidance you will give your students on how to conduct and utilize peer review, and that you give them a chance to reflect on the process.
Bean, John C. (2001). Engaging Ideas: The Professor’s Guide to Integrating Writing, Critical Thinking, and Active Learning in the Classroom . San Francisco: Jossey-Bass.
Gottschalk, Katherine and Keith Hjortshoj (2004). “What Can You Do with Student Writing?” In The Elements of Teaching Writing: A Resource for Instructors in All Disciplines . Boston: Bedford/St. Martin’s.
Millis, Barbara J. (2002). “ Enhancing Learning-and More! Through Collaborative Learning. IDEA Paper 38 . The IDEA Center.
Nilson, Linda. (2003). “Improving Student Peer Feedback.” College Teaching, 51 (1 ), p. 34-38.
Have suggestions?
If you have suggestions of resources we might add to these pages, please contact us:
[email protected] (314) 935-6810 Mon - Fri, 8:30 a.m. - 5:00 p.m.
- Our Mission
A Framework for Teaching Students How to Peer Edit
Giving meaningful feedback on a peer’s work doesn’t come naturally to students. Try these tips to help students hone their editing skills.
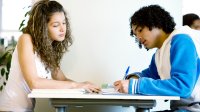
Too often, asking students to edit each other’s writing results in superficial commentary. Many students are uncertain about how to provide meaningful feedback on a peer’s work.
One way to make peer review more effective is by scaffolding it, or breaking down the practice into several classes where students critique each other’s work in a more focused, incremental manner. Scaffolding allows students to identify and address a single type of error in an allotted time period. While it is a valuable process for all students, it is especially useful for English-language learners and learning-support students, who benefit from breaking tasks and information into more manageable components.
Deconstruct Constructive Criticism
Students need to learn how to give and receive criticism in a productive and respectful manner. Before embarking on a class-wide peer review activity, teachers might underscore the importance of responses that are forthright and civil. Mastering the art of giving valuable feedback that doesn’t offend will benefit students in nearly every professional and personal relationship they maintain.
Start by breaking down the two words: constructive and criticism . What do these words mean by themselves? What synonyms might apply to each word? Ask students to think of examples of ways they might offer constructive criticism on a peer’s writing. They can be as simple as “Remember to capitalize proper nouns” or “Restate your thesis in your final paragraph.” Underscore to students that the criticism must be specific and helpful. “Good job!” doesn’t suffice. Write their responses on one or two poster boards, and place them where students can see them and refer back to them throughout the process.
Provide samples of criticism for students to emulate. You may want to advise learners to attach positive feedback with constructive criticism. For example, “Your hook poses a good question, but it contains several grammar errors” or “You inserted this quotation correctly.”
As there is no definitive guide to constructive criticism, teachers and students are encouraged to discuss what constitutes responsible feedback to find a definition and standards that best suit the class.
Set Clear Plans
In the same way that instruction often demands that educators create the assessment first, teachers should prepare for the peer review at the beginning of any writing assignment. A scaffolded peer review can be time-consuming, so consider the length of the writing assignment to be assessed when making a determination about the class time required.
Before assigning writing, consider what writing skills you want your students to learn, review, or practice. The objectives will vary by class, and they should be articulated to students from the outset. Some teachers may have the class focus on writing an effective thesis, incorporating quotations, or adding in-text citations. In other classes, the objective may be reviewing capitalization or comma usage. Identify the skills that students are expected to practice writing and finding in each other’s papers.
Facilitate the Process
Scaffolding the peer review provides an opportunity for students to read a piece multiple times to assess different elements of writing. First the class reviews the objective as a whole group. Then peer pairs review their individual writing with a focus on the defined learning objective.
Some students may be reluctant to criticize peers’ work. Consider choosing peer-review partners instead of letting the students pick. This might cut down on students’ being fearful of offending their friends. Also, if the debrief period is generating little discussion, ask students to debrief with their partners as opposed to in front of the class. Give students a set of debrief prompts to focus their discussion, such as “Discuss the corrections you made.”
Encourage students to refer to the posters regarding constructive criticism examples, especially if someone has given an impolite criticism.
Debrief as a Class
After the pair reviews, debrief by discussing the findings as a class. The debrief can be an open-ended session in which the teacher encourages students to ask questions and voice misunderstandings about both writing and critiquing. The debrief can also be more structured and incorporate specific questions, such as “What is a challenge an editor or peer reviewer might face?” or “What is one element of your writing you wish to improve upon?” The debrief can also take the form of a small writing assignment, such as a reflective paragraph on the peer review process in which students summarize what they have learned as an editor and proofreader.
We want our students to be proficient writers and thinkers. Reviewing a peer’s work can help young people better understand the often difficult process of writing by challenging them to adopt a dynamic new role as critic.
- Research article
- Open access
- Published: 12 April 2024
Feedback sources in essay writing: peer-generated or AI-generated feedback?
- Seyyed Kazem Banihashem 1 , 2 ,
- Nafiseh Taghizadeh Kerman 3 ,
- Omid Noroozi 2 ,
- Jewoong Moon 4 &
- Hendrik Drachsler 1 , 5
International Journal of Educational Technology in Higher Education volume 21 , Article number: 23 ( 2024 ) Cite this article
3370 Accesses
13 Altmetric
Metrics details
Peer feedback is introduced as an effective learning strategy, especially in large-size classes where teachers face high workloads. However, for complex tasks such as writing an argumentative essay, without support peers may not provide high-quality feedback since it requires a high level of cognitive processing, critical thinking skills, and a deep understanding of the subject. With the promising developments in Artificial Intelligence (AI), particularly after the emergence of ChatGPT, there is a global argument that whether AI tools can be seen as a new source of feedback or not for complex tasks. The answer to this question is not completely clear yet as there are limited studies and our understanding remains constrained. In this study, we used ChatGPT as a source of feedback for students’ argumentative essay writing tasks and we compared the quality of ChatGPT-generated feedback with peer feedback. The participant pool consisted of 74 graduate students from a Dutch university. The study unfolded in two phases: firstly, students’ essay data were collected as they composed essays on one of the given topics; subsequently, peer feedback and ChatGPT-generated feedback data were collected through engaging peers in a feedback process and using ChatGPT as a feedback source. Two coding schemes including coding schemes for essay analysis and coding schemes for feedback analysis were used to measure the quality of essays and feedback. Then, a MANOVA analysis was employed to determine any distinctions between the feedback generated by peers and ChatGPT. Additionally, Spearman’s correlation was utilized to explore potential links between the essay quality and the feedback generated by peers and ChatGPT. The results showed a significant difference between feedback generated by ChatGPT and peers. While ChatGPT provided more descriptive feedback including information about how the essay is written, peers provided feedback including information about identification of the problem in the essay. The overarching look at the results suggests a potential complementary role for ChatGPT and students in the feedback process. Regarding the relationship between the quality of essays and the quality of the feedback provided by ChatGPT and peers, we found no overall significant relationship. These findings imply that the quality of the essays does not impact both ChatGPT and peer feedback quality. The implications of this study are valuable, shedding light on the prospective use of ChatGPT as a feedback source, particularly for complex tasks like argumentative essay writing. We discussed the findings and delved into the implications for future research and practical applications in educational contexts.
Introduction
Feedback is acknowledged as one of the most crucial tools for enhancing learning (Banihashem et al., 2022 ). The general and well-accepted definition of feedback conceptualizes it as information provided by an agent (e.g., teacher, peer, self, AI, technology) regarding aspects of one’s performance or understanding (e.g., Hattie & Timplerely, 2007 ). Feedback serves to heighten students’ self-awareness concerning their strengths and areas warranting improvement, through providing actionable steps required to enhance performance (Ramson, 2003 ). The literature abounds with numerous studies that illuminate the positive impact of feedback on diverse dimensions of students’ learning journey including increasing motivation (Amiryousefi & Geld, 2021 ), fostering active engagement (Zhang & Hyland, 2022 ), promoting self-regulation and metacognitive skills (Callender et al., 2016 ; Labuhn et al., 2010 ), and enriching the depth of learning outcomes (Gan et al., 2021 ).
Normally, teachers have primarily assumed the role of delivering feedback, providing insights into students’ performance on specific tasks or their grasp of particular subjects (Konold et al., 2004 ). This responsibility has naturally fallen upon teachers owing to their expertise in the subject matter and their competence to offer constructive input (Diezmann & Watters, 2015 ; Holt-Reynolds, 1999 ; Valero Haro et al., 2023 ). However, teachers’ role as feedback providers has been challenged in recent years as we have witnessed a growth in class sizes due to the rapid advances in technology and the widespread use of digital technologies that resulted in flexible and accessible education (Shi et al., 2019 ). The growth in class sizes has translated into an increased workload for teachers, leading to a pertinent predicament. This situation has directly impacted their capacity to provide personalized and timely feedback to each student, a capability that has encountered limitations (Er et al., 2021 ).
In response to this challenge, various solutions have emerged, among which peer feedback has arisen as a promising alternative instructional approach (Er et al., 2021 ; Gao et al., 2024 ; Noroozi et al., 2023 ; Kerman et al., 2024 ). Peer feedback entails a process wherein students assume the role of feedback providers instead of teachers (Liu & Carless, 2006 ). Involving students in feedback can add value to education in several ways. First and foremost, research indicates that students delve into deeper and more effective learning when they take on the role of assessors, critically evaluating and analyzing their peers’ assignments (Gielen & De Wever, 2015 ; Li et al., 2010 ). Moreover, involving students in the feedback process can augment their self-regulatory awareness, active engagement, and motivation for learning (e.g., Arguedas et al., 2016 ). Lastly, the incorporation of peer feedback not only holds the potential to significantly alleviate teachers’ workload by shifting their responsibilities from feedback provision to the facilitation of peer feedback processes but also nurtures a dynamic learning environment wherein students are actively immersed in the learning journey (e.g., Valero Haro et al., 2023 ).
Despite the advantages of peer feedback, furnishing high-quality feedback to peers remains a challenge. Several factors contribute to this challenge. Primarily, generating effective feedback necessitates a solid understanding of feedback principles, an element that peers often lack (Latifi et al., 2023 ; Noroozi et al., 2016 ). Moreover, offering high-quality feedback is inherently a complex task, demanding substantial cognitive processing to meticulously evaluate peers’ assignments, identify issues, and propose constructive remedies (King, 2002 ; Noroozi et al., 2022 ). Furthermore, the provision of valuable feedback calls for a significant level of domain-specific expertise, which is not consistently possessed by students (Alqassab et al., 2018 ; Kerman et al., 2022 ).
In recent times, advancements in technology, coupled with the emergence of fields like Learning Analytics (LA), have presented promising avenues to elevate feedback practices through the facilitation of scalable, timely, and personalized feedback (Banihashem et al., 2023 ; Deeva et al., 2021 ; Drachsler, 2023 ; Drachsler & Kalz, 2016 ; Pardo et al., 2019 ; Zawacki-Richter et al., 2019 ; Rüdian et al., 2020 ). Yet, a striking stride forward in the field of educational technology has been the advent of a novel Artificial Intelligence (AI) tool known as “ChatGPT,” which has sparked a global discourse on its potential to significantly impact the current education system (Ray, 2023 ). This tool’s introduction has initiated discussions on the considerable ways AI can support educational endeavors (Bond et al., 2024 ; Darvishi et al., 2024 ).
In the context of feedback, AI-powered ChatGPT introduces what is referred to as AI-generated feedback (Farrokhnia et al., 2023 ). While the literature suggests that ChatGPT has the potential to facilitate feedback practices (Dai et al., 2023 ; Katz et al., 2023 ), this literature is very limited and mostly not empirical leading us to realize that our current comprehension of its capabilities in this regard is quite restricted. Therefore, we lack a comprehensive understanding of how ChatGPT can effectively support feedback practices and to what degree it can improve the timeliness, impact, and personalization of feedback, which remains notably limited at this time.
More importantly, considering the challenges we raised for peer feedback, the question is whether AI-generated feedback and more specifically feedback provided by ChatGPT has the potential to provide quality feedback. Taking this into account, there is a scarcity of knowledge and research gaps regarding the extent to which AI tools, specifically ChatGPT, can effectively enhance feedback quality compared to traditional peer feedback. Hence, our research aims to investigate the quality of feedback generated by ChatGPT within the context of essay writing and to juxtapose its quality with that of feedback generated by students.
This study carries the potential to make a substantial contribution to the existing body of recent literature on the potential of AI and in particular ChatGPT in education. It can cast a spotlight on the quality of AI-generated feedback in contrast to peer-generated feedback, while also showcasing the viability of AI tools like ChatGPT as effective automated feedback mechanisms. Furthermore, the outcomes of this study could offer insights into mitigating the feedback-related workload experienced by teachers through the intelligent utilization of AI tools (e.g., Banihashem et al., 2022 ; Er et al., 2021 ; Pardo et al., 2019 ).
However, there might be an argument regarding the rationale for conducting this study within the specific context of essay writing. Addressing this potential query, it is crucial to highlight that essay writing stands as one of the most prevalent yet complex tasks for students (Liunokas, 2020 ). This task is not without its challenges, as evidenced by the extensive body of literature that indicates students often struggle to meet desired standards in their essay composition (e.g., Bulqiyah et al., 2021 ; Noroozi et al., 2016 ;, 2022 ; Latifi et al., 2023 ).
Furthermore, teachers frequently express dissatisfaction with the depth and overall quality of students’ essay writing (Latifi et al., 2023 ). Often, these teachers lament that their feedback on essays remains superficial due to the substantial time and effort required for critical assessment and individualized feedback provision (Noroozi et al., 2016 ;, 2022 ). Regrettably, these constraints prevent them from delving deeper into the evaluation process (Kerman et al., 2022 ).
Hence, directing attention towards the comparison of peer-generated feedback quality and AI-generated feedback quality within the realm of essay writing bestows substantial value upon both research and practical application. This study enriches the academic discourse and informs practical approaches by delivering insights into the adequacy of feedback quality offered by both peers and AI for the domain of essay writing. This investigation serves as a critical step in determining whether the feedback imparted by peers and AI holds the necessary caliber to enhance the craft of essay writing.
The ramifications of addressing this query are noteworthy. Firstly, it stands to significantly alleviate the workload carried by teachers in the process of essay evaluation. By ascertaining the viability of feedback from peers and AI, teachers can potentially reduce the time and effort expended in reviewing essays. Furthermore, this study has the potential to advance the quality of essay compositions. The collaboration between students providing feedback to peers and the integration of AI-powered feedback tools can foster an environment where essays are not only better evaluated but also refined in their content and structure.With this in mind, we aim to tackle the following key questions within the scope of this study:
RQ1. To what extent does the quality of peer-generated and ChatGPT-generated feedback differ in the context of essay writing?
Rq2. does a relationship exist between the quality of essay writing performance and the quality of feedback generated by peers and chatgpt, context and participant.
This study was conducted in the academic year of 2022–2023 at a Dutch university specializing in life sciences. In total, 74 graduate students from food sciences participated in this study in which 77% of students were female ( N = 57) and 23% were male ( N = 17).
Study design and procedure
This empirical study has an exploratory nature and it was conducted in two phases. An online module called “ Argumentative Essay Writing ” (AEW) was designed to be followed by students within the Brightspace platform. The purpose of the AEW module was to improve students’ essay writing skills by engaging them in a peer learning process where students were invited to provide feedback on each other’s essays. After designing the module, the study was implemented in two weeks and followed in two phases.
In week one (phase one), students were asked to write an essay on given topics. The topics for the essay were controversial and included “ Scientists with affiliations to the food industry should abstain from participating in risk assessment processes ”, “ powdered infant formula must adhere to strict sterility standards ”, and “ safe food consumption is the responsibility of the consumer ”. The given controversial topics were directly related to the course content and students’ area of study. Students had time for one week to write their essays individually and submit them to the Brightspace platform.
In week two (phase two), students were randomly invited to provide two sets of written/asynchronous feedback on their peers’ submitted essays. We gave a prompt to students to be used for giving feedback ( Please provide feedback to your peer and explain the extent to which she/he has presented/elaborated/justified various elements of an argumentative essay. What are the problems and what are your suggestions to improve each element of the essay? Your feedback must be between 250 and 350 words ). To be able to engage students in the online peer feedback activity, we used the FeedbackFruits app embedded in the Brightspace platform. FeedbackFruits functions as an external educational technology tool seamlessly integrated into Brightspace, aimed at enhancing student engagement via diverse peer collaboration approaches. Among its features are peer feedback, assignment evaluation, skill assessment, automated feedback, interactive videos, dynamic documents, discussion tasks, and engaging presentations (Noroozi et al., 2022 ). In this research, our focus was on the peer feedback feature of the FeedbackFruits app, which empowers teachers to design tasks that enable students to offer feedback to their peers.
In addition, we used ChatGPT as another feedback source on peers’ essays. To be consistent with the criteria for peer feedback, we gave the same feedback prompt question with a minor modification to ChatGPT and asked it to give feedback on the peers’ essays ( Please read and provide feedback on the following essay and explain the extent to which she/he has presented/elaborated/justified various elements of an argumentative essay. What are the problems and what are your suggestions to improve each element of the essay? Your feedback must be between 250 and 350 words ).
Following this design, we were able to collect students’ essay data, peer feedback data, and feedback data generated by ChatGPT. In the next step, we used two coding schemes to analyze the quality of the essays and feedback generated by peers and ChatGPT.
Measurements
Coding scheme to assess the quality of essay writing.
In this study, a coding scheme proposed by Noroozi et al. ( 2016 ) was employed to assess students’ essay quality. This coding system was constructed based on the key components of high-quality essay composition, encompassing eight elements: introduction pertaining to the subject, taking a clear stance on the subject, presenting arguments in favor of the chosen position, providing justifications for the arguments supporting the position, counter-arguments, justifications for counter-arguments, responses to counter-arguments, and concluding with implications. Each element in the coding system is assigned a score ranging from zero (indicating the lowest quality level) to three (representing the highest quality level). The cumulative scores across all these elements were aggregated to determine the overall quality score of the student’s written essays. Two experienced coders in the field of education collaborated to assess the quality of the written essays, and their agreement level was measured at 75% (Cohen’s Kappa = 0.75 [95% confidence interval: 0.70–0.81]; z = 25.05; p < 0.001), signifying a significant level of consensus between the coders.
Coding scheme to assess the quality of feedback generated by peers and ChatGPT
To assess the quality of feedback provided by both peers and ChatGPT, we employed a coding scheme developed by Noroozi et al. ( 2022 ). This coding framework dissects the characteristics of feedback, encompassing three key elements: the affective component, which considers the inclusion of emotional elements such as positive sentiments like praise or compliments, as well as negative emotions such as anger or disappointment; the cognitive component, which includes description (a concise summary of the essay), identification (pinpointing and specifying issues within the essay), and justification (providing explanations and justifications for the identified issues); and the constructive component, which involves offering recommendations, albeit not detailed action plans for further enhancements. Ratings within this coding framework range from zero, indicating poor quality, to two, signifying good quality. The cumulative scores were tallied to determine the overall quality of the feedback provided to the students. In this research, as each essay received feedback from both peers and ChatGPT, we calculated the average score from the two sets of feedback to establish the overall quality score for the feedback received, whether from peers or ChatGPT. The same two evaluators were involved in the assessment. The inter-rater reliability between the evaluators was determined to be 75% (Cohen’s Kappa = 0.75 [95% confidence interval: 0.66–0.84]; z = 17.52; p < 0.001), showing a significant level of agreement between them.
The logic behind choosing these coding schemes was as follows: Firstly, from a theoretical standpoint, both coding schemes were developed based on robust and well-established theories. The coding scheme for evaluating essay quality draws on Toulmin’s argumentation model ( 1958 ), a respected framework for essay writing. It encompasses all elements essential for high-quality essay composition and aligns well with the structure of essays assigned in the chosen course for this study. Similarly, the feedback coding scheme is grounded in prominent works on identifying feedback features (e.g., Nelson & Schunn, 2009 ; Patchan et al., 2016 ; Wu & Schunn, 2020 ), enabling the identification of key features of high-quality feedback (Noroozi et al., 2022 ). Secondly, from a methodological perspective, both coding schemes feature a transparent scoring method, mitigating coder bias and bolstering the tool’s credibility.
To ensure the data’s validity and reliability for statistical analysis, two tests were implemented. Initially, the Levene test assessed group homogeneity, followed by the Kolmogorov-Smirnov test to evaluate data normality. The results confirmed both group homogeneity and data normality. For the first research question, gender was considered as a control variable, and the MANCOVA test was employed to compare the variations in feedback quality between peer feedback and ChatGPT-generated feedback. Addressing the second research question involved using Spearman’s correlation to examine the relationships among original argumentative essays, peer feedback, and ChatGPT-generated feedback.
The results showed a significant difference in feedback quality between peer feedback and ChatGPT-generated feedback. Peers provided feedback of higher quality compared to ChatGPT. This difference was mainly due to the descriptive and identification of the problem features of feedback. ChatGPT tended to produce more extensive descriptive feedback including a summary statement such as the description of the essay or taken action, while students performed better in pinpointing and identifying the issues in the feedback provided (see Table 1 ).
A comprehensive list featuring selected examples of feedback generated by peers and ChatGPT is presented in Fig 1 . This table additionally outlines examples of how the generated feedback was coded based on the coding scheme to assess the quality of feedback.
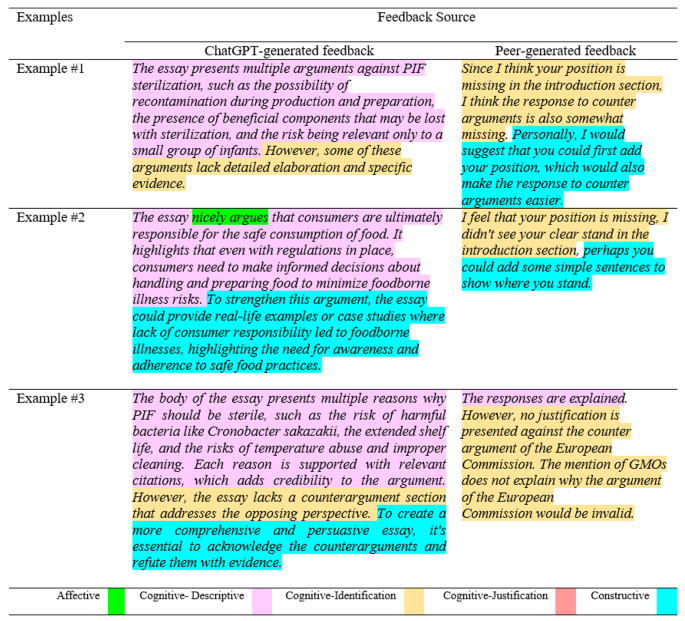
A comparative list of selected examples of peer-generated and ChatGPT-generated feedback
Overall, the results indicated that there was no significant relationship between the quality of essay writing and the feedback generated by peers and ChatGPT. However, a positive correlation was observed between the quality of the essay and the affective feature of feedback generated by ChatGPT, while a negative relationship was observed between the quality of the essay and the affective feature of feedback generated by peers. This finding means that as the quality of the essay improves, ChatGPT tends to provide more affective feedback, while peers tend to provide less affective feedback (see Table 2 ).
This study was an initial effort to explore the potential of ChatGPT as a feedback source in the context of essay writing and to compare the extent to which the quality of feedback generated by ChatGPT differs from the feedback provided by peers. Below we discuss our findings for each research question.
Discussion on the results of RQ1
For the first research question, the results revealed a disparity in feedback quality when comparing peer-generated feedback to feedback generated by ChatGPT. Peer feedback demonstrated higher quality compared to ChatGPT-generated feedback. This discrepancy is attributed primarily to variations in the descriptive and problem-identification features of the feedback.
ChatGPT tended to provide more descriptive feedback, often including elements such as summarizing the content of the essay. This inclination towards descriptive feedback could be related to ChatGPT’s capacity to analyze and synthesize textual information effectively. Research on ChatGPT further supports this notion, demonstrating the AI tool’s capacity to offer a comprehensive overview of the provided content, therefore potentially providing insights and a holistic perspective on the content (Farrokhnia et al., 2023 ; Ray, 2023 ).
ChatGPT’s proficiency in providing extensive descriptive feedback could be seen as a strength. It might be particularly valuable for summarizing complex arguments or providing comprehensive overviews, which could aid students in understanding the overall structure and coherence of their essays.
In contrast, students’ feedback content entailed high quality regarding identifying specific issues and areas for improvement. Peers outperformance compared to ChatGPT in identifying problems within the essays could be related to humans’ potential in cognitive skills, critical thinking abilities, and contextual understanding (e.g., Korteling et al., 2021 ; Lamb et al., 2019 ). This means that students, with their contextual knowledge and critical thinking skills, may be better equipped to identify issues within the essays that ChatGPT may overlook.
Furthermore, a detailed look at the findings of the first research question discloses that the feedback generated by ChatGPT comprehensively encompassed all essential components characterizing high-quality feedback, including affective, cognitive, and constructive dimensions (Kerman et al., 2022 ; Patchan et al., 2016 ). This comprehensive observation could be an indication of the fact that ChatGPT-generated feedback could potentially serve as a viable source of feedback. This observation is supported by previous studies where a positive role for AI-generated feedback and automated feedback in enhancing educational outcomes has been recognized (e.g., Bellhäuser et al., 2023 ; Gombert et al., 2024 ; Huang et al., 2023 ; Xia et al., 2022 ).
Finally, an overarching look at the results of the first research question suggests a potential complementary role for ChatGPT and students in the feedback process. This means that using these two feedback sources together creates a synergistic relationship that could result in better feedback outcomes.
Discussion on the results of RQ2
Results for the second research question revealed no observations of a significant correlation between the quality of the essays and the quality of the feedback generated by both peers and ChatGPT. These findings carry a consequential implication, suggesting that the inherent quality of the essays under scrutiny exerts negligible influence over the quality of feedback furnished by both students and the ChatGPT.
In essence, these results point to a notable degree of independence between the writing prowess exhibited in the essays and the efficacy of the feedback received from either source. This disassociation implies that the ability to produce high-quality essays does not inherently translate into a corresponding ability to provide equally insightful feedback, neither for peers nor for ChatGPT. This decoupling of essay quality from feedback quality highlighted the multifaceted nature of these evaluative processes, where proficiency in constructing a coherent essay does not necessarily guarantee an equally adept capacity for evaluating and articulating constructive commentary on peers’ work.
The implications of these findings are both intriguing and defy conventional expectations, as they deviate somewhat from the prevailing literature’s stance. The existing body of scholarly work generally posits a direct relationship between the quality of an essay and the subsequent quality of generated feedback (Noroozi et al., 2016 ;, 2022 ; Kerman et al., 2022 ; Vale Haro et al., 2023 ). This line of thought contends that essays of inferior quality might serve as a catalyst for more pronounced error detection among students, encompassing grammatical intricacies, depth of content, clarity, and coherence, as well as the application of evidence and support. Conversely, when essays are skillfully crafted, the act of pinpointing areas for enhancement becomes a more complex task, potentially necessitating a heightened level of subject comprehension and nuanced evaluation.
However, the present study’s findings challenge this conventional wisdom. The observed decoupling of essay quality from feedback quality suggests a more nuanced interplay between the two facets of assessment. Rather than adhering to the anticipated pattern, wherein weaker essays prompt clearer identification of deficiencies, and superior essays potentially render the feedback process more challenging, the study suggests that the process might be more complex than previously thought. It hints at a dynamic in which the act of evaluating essays and providing constructive feedback transcends a simple linear connection with essay quality.
These findings, while potentially unexpected, are an indication of the complex nature of essay assignments and feedback provision highlighting the complexity of cognitive processes that underlie both tasks, and suggesting that the relationship between essay quality and feedback quality is not purely linear but influenced by a multitude of factors, including the evaluator’s cognitive framework, familiarity with the subject matter, and critical analysis skills.
Despite this general observation, a closer examination of the affective features within the feedback reveals a different pattern. The positive correlation between essay quality and the affective features present in ChatGPT-generated feedback could be related to ChatGPT’s capacity to recognize and appreciate students’ good work. As the quality of the essay increases, ChatGPT might be programmed to offer more positive and motivational feedback to acknowledge students’ progress (e.g., Farrokhnia et al., 2023 ; Ray, 2023 ). In contrast, the negative relationship between essay quality and the affective features in peer feedback may be attributed to the evolving nature of feedback from peers (e.g., Patchan et al., 2016 ). This suggests that as students witness improvements in their peers’ essay-writing skills and knowledge, their feedback priorities may naturally evolve. For instance, students may transition from emphasizing emotional and affective comments to focusing on cognitive and constructive feedback, with the goal of further enhancing the overall quality of the essays.
Limitations and implications for future research and practice
We acknowledge the limitations of this study. Primarily, the data underpinning this investigation was drawn exclusively from a singular institution and a solitary course, featuring a relatively modest participant pool. This confined scope inevitably introduces certain constraints that need to be taken into consideration when interpreting the study’s outcomes and generalizing them to broader educational contexts. Under this constrained sampling, the findings might exhibit a degree of contextual specificity, potentially limiting their applicability to diverse institutional settings and courses with distinct curricular foci. The diverse array of academic environments, student demographics, and subject matter variations existing across educational institutions could potentially yield divergent patterns of results. Therefore, while the current study’s outcomes provide insights within the confines of the studied institution and course, they should be interpreted and generalized with prudence. Recognizing these limitations, for future studies, we recommend considering a large-scale participant pool with a diverse range of variables, including individuals from various programs and demographics. This approach would enrich the depth and breadth of understanding in this domain, fostering a more comprehensive comprehension of the complex dynamics at play.
In addition, this study omitted an exploration into the degree to which students utilize feedback provided by peers and ChatGPT. That is to say that we did not investigate the effects of such feedback on essay enhancements in the revision phase. This omission inherently introduces a dimension of uncertainty and places a constraint on the study’s holistic understanding of the feedback loop. By not addressing these aspects, the study’s insights are somewhat partial, limiting the comprehensive grasp of the potential influences that these varied feedback sources wield on students’ writing enhancement processes. An analysis of the feedback assimilation patterns and their subsequent effects on essay refinement would have unveiled insights into the practical utility and impact of the feedback generated by peers and ChatGPT.
To address this limitation, future investigations could be structured to encompass a more thorough examination of students’ feedback utilization strategies and the resulting implications for the essay revision process. By shedding light on the complex interconnection between feedback reception, its integration into the revision process, and the ultimate outcomes in terms of essay improvement, a more comprehensive understanding of the dynamics involved could be attained.
Furthermore, in this study, we employed identical question prompts for both peers and ChatGPT. However, there is evidence indicating that ChatGPT is sensitive to how prompts are presented to it (e.g., Cao et al., 2023 ; White et al., 2023 ; Zuccon & Koopman, 2023 ). This suggests that variations in the wording, structure, or context of prompts might influence the responses generated by ChatGPT, potentially impacting the comparability of its outputs with those of peers. Therefore, it is essential to carefully consider and control for prompt-related factors in future research when assessing ChatGPT’s performance and capabilities in various tasks and contexts.
In addition, We acknowledge that ChatGPT can potentially generate inaccurate results. Nevertheless, in the context of this study, our examination of the results generated by ChatGPT did not reveal a significant inaccuracies that would warrant inclusion in our findings.
From a methodological perspective, we reported the interrater reliability between the coders to be 75%. While this level of agreement was statistically significant, signifying the reliability of our coders’ analyses, it did not reach the desired level of precision. We acknowledge this as a limitation of the study and suggest enhancing interrater reliability through additional coder training.
In addition, it is worth noting that the advancement of Generative AI like ChatGPT, opens new avenues in educational feedback mechanisms. Beyond just generating feedback, these AI models have the potential to redefine how feedback is presented and assimilated. In the realm of research on adaptive learning systems, the findings of this study also echo the importance of adaptive learning support empowered by AI and ChatGPT (Rummel et al., 2016 ). It can pave the way for tailored educational experiences that respond dynamically to individual student needs. This is not just about the feedback’s content but its delivery, timing, and adaptability. Further exploratory data analyses, such as sequential analysis and data mining, may offer insights into the nuanced ways different adaptive learning supports can foster student discussions (Papamitsiou & Economides, 2014 ). This involves dissecting the feedback dynamics, understanding how varied feedback types stimulate discourse, and identifying patterns that lead to enhanced student engagement.
Ensuring the reliability and validity of AI-empowered feedback is also crucial. The goal is to ascertain that technology-empowered learning support genuinely enhances students’ learning process in a consistent and unbiased manner. Given ChatGPT’s complex nature of generating varied responses based on myriad prompts, the call for enhancing methodological rigor through future validation studies becomes both timely and essential. For example, in-depth prompt validation and blind feedback assessment studies could be employed to meticulously probe the consistency and quality of ChatGPT’s responses. Also, comparative analysis with different AI models can be useful.
From an educational standpoint, our research findings advocate for the integration of ChatGPT as a feedback resource with peer feedback within higher education environments for essay writing tasks since there is a complementary role potential for pee-generated and ChatGPT-generated feedback. This approach holds the potential to alleviate the workload burden on teachers, particularly in the context of online courses with a significant number of students.
This study contributes to and adds value to the young existing but rapidly growing literature in two distinct ways. From a research perspective, this study addresses a significant void in the current literature by responding to the lack of research on AI-generated feedback for complex tasks like essay writing in higher education. The research bridges this gap by analyzing the effectiveness of ChatGPT-generated feedback compared to peer-generated feedback, thereby establishing a foundation for further exploration in this field. From a practical perspective of higher education, the study’s findings offer insights into the potential integration of ChatGPT as a feedback source within higher education contexts. The discovery that ChatGPT’s feedback quality could potentially complement peer feedback highlights its applicability for enhancing feedback practices in higher education. This holds particular promise for courses with substantial enrolments and essay-writing components, providing teachers with a feasible alternative for delivering constructive feedback to a larger number of students.
Data availability
The data is available upon a reasonable request.
Alqassab, M., Strijbos, J. W., & Ufer, S. (2018). Training peer-feedback skills on geometric construction tasks: Role of domain knowledge and peer-feedback levels. European Journal of Psychology of Education , 33 (1), 11–30. https://doi.org/10.1007/s10212-017-0342-0 .
Article Google Scholar
Amiryousefi, M., & Geld, R. (2021). The role of redressing teachers’ instructional feedback interventions in EFL learners’ motivation and achievement in distance education. Innovation in Language Learning and Teaching , 15 (1), 13–25. https://doi.org/10.1080/17501229.2019.1654482 .
Arguedas, M., Daradoumis, A., & Xhafa Xhafa, F. (2016). Analyzing how emotion awareness influences students’ motivation, engagement, self-regulation and learning outcome. Educational Technology and Society , 19 (2), 87–103. https://www.jstor.org/stable/jeductechsoci.19.2.87 .
Google Scholar
Banihashem, S. K., Noroozi, O., van Ginkel, S., Macfadyen, L. P., & Biemans, H. J. (2022). A systematic review of the role of learning analytics in enhancing feedback practices in higher education. Educational Research Review , 100489. https://doi.org/10.1016/j.edurev.2022.100489 .
Banihashem, S. K., Dehghanzadeh, H., Clark, D., Noroozi, O., & Biemans, H. J. (2023). Learning analytics for online game-based learning: A systematic literature review. Behaviour & Information Technology , 1–28. https://doi.org/10.1080/0144929X.2023.2255301 .
Bellhäuser, H., Dignath, C., & Theobald, M. (2023). Daily automated feedback enhances self-regulated learning: A longitudinal randomized field experiment. Frontiers in Psychology , 14 , 1125873. https://doi.org/10.3389/fpsyg.2023.1125873 .
Bond, M., Khosravi, H., De Laat, M., Bergdahl, N., Negrea, V., Oxley, E., & Siemens, G. (2024). A meta systematic review of artificial intelligence in higher education: A call for increased ethics, collaboration, and rigour. International Journal of Educational Technology in Higher Education , 21 (4), 1–41. https://doi.org/10.1186/s41239-023-00436-z .
Bulqiyah, S., Mahbub, M., & Nugraheni, D. A. (2021). Investigating writing difficulties in Essay writing: Tertiary Students’ perspectives. English Language Teaching Educational Journal , 4 (1), 61–73. https://doi.org/10.12928/eltej.v4i1.2371 .
Callender, A. A., Franco-Watkins, A. M., & Roberts, A. S. (2016). Improving metacognition in the classroom through instruction, training, and feedback. Metacognition and Learning , 11 (2), 215–235. https://doi.org/10.1007/s11409-015-9142-6 .
Cao, J., Li, M., Wen, M., & Cheung, S. C. (2023). A study on prompt design, advantages and limitations of chatgpt for deep learning program repair. arXiv Preprint arXiv:2304 08191 . https://doi.org/10.48550/arXiv.2304.08191 .
Dai, W., Lin, J., Jin, F., Li, T., Tsai, Y. S., Gasevic, D., & Chen, G. (2023). Can large language models provide feedback to students? A case study on ChatGPT. https://doi.org/10.35542/osf.io/hcgzj .
Darvishi, A., Khosravi, H., Sadiq, S., Gašević, D., & Siemens, G. (2024). Impact of AI assistance on student agency. Computers & Education , 210 , 104967. https://doi.org/10.1016/j.compedu.2023.104967 .
Deeva, G., Bogdanova, D., Serral, E., Snoeck, M., & De Weerdt, J. (2021). A review of automated feedback systems for learners: Classification framework, challenges and opportunities. Computers & Education , 162 , 104094. https://doi.org/10.1016/j.compedu.2020.104094 .
Diezmann, C. M., & Watters, J. J. (2015). The knowledge base of subject matter experts in teaching: A case study of a professional scientist as a beginning teacher. International Journal of Science and Mathematics Education , 13 , 1517–1537. https://doi.org/10.1007/s10763-014-9561-x .
Drachsler, H. (2023). Towards highly informative learning analytics . Open Universiteit. https://doi.org/10.25656/01:26787 .
Drachsler, H., & Kalz, M. (2016). The MOOC and learning analytics innovation cycle (MOLAC): A reflective summary of ongoing research and its challenges. Journal of Computer Assisted Learning , 32 (3), 281–290. https://doi.org/10.1111/jcal.12135 .
Er, E., Dimitriadis, Y., & Gašević, D. (2021). Collaborative peer feedback and learning analytics: Theory-oriented design for supporting class-wide interventions. Assessment & Evaluation in Higher Education , 46 (2), 169–190. https://doi.org/10.1080/02602938.2020.1764490 .
Farrokhnia, M., Banihashem, S. K., Noroozi, O., & Wals, A. (2023). A SWOT analysis of ChatGPT: Implications for educational practice and research. Innovations in Education and Teaching International , 1–15. https://doi.org/10.1080/14703297.2023.2195846 .
Gan, Z., An, Z., & Liu, F. (2021). Teacher feedback practices, student feedback motivation, and feedback behavior: How are they associated with learning outcomes? Frontiers in Psychology , 12 , 697045. https://doi.org/10.3389/fpsyg.2021.697045 .
Gao, X., Noroozi, O., Gulikers, J. T. M., Biemans, H. J., & Banihashem, S. K. (2024). A systematic review of the key components of online peer feedback practices in higher education. Educational Research Review , 100588. https://doi.org/10.1016/j.edurev.2023.100588 .
Gielen, M., & De Wever, B. (2015). Scripting the role of assessor and assessee in peer assessment in a wiki environment: Impact on peer feedback quality and product improvement. Computers & Education , 88 , 370–386. https://doi.org/10.1016/j.compedu.2015.07.012 .
Gombert, S., Fink, A., Giorgashvili, T., Jivet, I., Di Mitri, D., Yau, J., & Drachsler, H. (2024). From the Automated Assessment of Student Essay Content to highly informative feedback: A case study. International Journal of Artificial Intelligence in Education , 1–39. https://doi.org/10.1007/s40593-023-00387-6 .
Hattie, J., & Timperley, H. (2007). The power of feedback. Review of Educational Research , 77 (1), 81–112. https://doi.org/10.3102/003465430298487 .
Holt-Reynolds, D. (1999). Good readers, good teachers? Subject matter expertise as a challenge in learning to teach. Harvard Educational Review , 69 (1), 29–51. https://doi.org/10.17763/haer.69.1.pl5m5083286l77t2 .
Huang, A. Y., Lu, O. H., & Yang, S. J. (2023). Effects of artificial intelligence–enabled personalized recommendations on learners’ learning engagement, motivation, and outcomes in a flipped classroom. Computers & Education , 194 , 104684. https://doi.org/10.1016/j.compedu.2022.104684 .
Katz, A., Wei, S., Nanda, G., Brinton, C., & Ohland, M. (2023). Exploring the efficacy of ChatGPT in analyzing Student Teamwork Feedback with an existing taxonomy. arXiv Preprint arXiv . https://doi.org/10.48550/arXiv.2305.11882 .
Kerman, N. T., Noroozi, O., Banihashem, S. K., Karami, M., & Biemans, H. J. (2022). Online peer feedback patterns of success and failure in argumentative essay writing. Interactive Learning Environments , 1–13. https://doi.org/10.1080/10494820.2022.2093914 .
Kerman, N. T., Banihashem, S. K., Karami, M., Er, E., Van Ginkel, S., & Noroozi, O. (2024). Online peer feedback in higher education: A synthesis of the literature. Education and Information Technologies , 29 (1), 763–813. https://doi.org/10.1007/s10639-023-12273-8 .
King, A. (2002). Structuring peer interaction to promote high-level cognitive processing. Theory into Practice , 41 (1), 33–39. https://doi.org/10.1207/s15430421tip4101_6 .
Konold, K. E., Miller, S. P., & Konold, K. B. (2004). Using teacher feedback to enhance student learning. Teaching Exceptional Children , 36 (6), 64–69. https://doi.org/10.1177/004005990403600608 .
Korteling, J. H., van de Boer-Visschedijk, G. C., Blankendaal, R. A., Boonekamp, R. C., & Eikelboom, A. R. (2021). Human-versus artificial intelligence. Frontiers in Artificial Intelligence , 4 , 622364. https://doi.org/10.3389/frai.2021.622364 .
Labuhn, A. S., Zimmerman, B. J., & Hasselhorn, M. (2010). Enhancing students’ self-regulation and mathematics performance: The influence of feedback and self-evaluative standards. Metacognition and Learning , 5 , 173–194. https://doi.org/10.1007/s11409-010-9056-2 .
Lamb, R., Firestone, J., Schmitter-Edgecombe, M., & Hand, B. (2019). A computational model of student cognitive processes while solving a critical thinking problem in science. The Journal of Educational Research , 112 (2), 243–254. https://doi.org/10.1080/00220671.2018.1514357 .
Latifi, S., Noroozi, O., & Talaee, E. (2023). Worked example or scripting? Fostering students’ online argumentative peer feedback, essay writing and learning. Interactive Learning Environments , 31 (2), 655–669. https://doi.org/10.1080/10494820.2020.1799032 .
Li, L., & Liu, X. (2010). Steckelberg. Assessor or assessee: How student learning improves by giving and receiving peer feedback. British Journal of Educational Technology , 41 (3), 525–536. https://doi.org/10.1111/j.1467-8535.2009.00968.x .
Liu, N. F., & Carless, D. (2006). Peer feedback: The learning element of peer assessment. Teaching in Higher Education , 11 (3), 279–290. https://doi.org/10.1080/13562510600680582 .
Liunokas, Y. (2020). Assessing students’ ability in writing argumentative essay at an Indonesian senior high school. IDEAS: Journal on English language teaching and learning. Linguistics and Literature , 8 (1), 184–196. https://doi.org/10.24256/ideas.v8i1.1344 .
Nelson, M. M., & Schunn, C. D. (2009). The nature of feedback: How different types of peer feedback affect writing performance. Instructional Science , 37 , 375–401. https://doi.org/10.1007/s11251-008-9053-x .
Noroozi, O., Banihashem, S. K., Taghizadeh Kerman, N., Parvaneh Akhteh Khaneh, M., Babayi, M., Ashrafi, H., & Biemans, H. J. (2022). Gender differences in students’ argumentative essay writing, peer review performance and uptake in online learning environments. Interactive Learning Environments , 1–15. https://doi.org/10.1080/10494820.2022.2034887 .
Noroozi, O., Biemans, H., & Mulder, M. (2016). Relations between scripted online peer feedback processes and quality of written argumentative essay. The Internet and Higher Education , 31, 20-31. https://doi.org/10.1016/j.iheduc.2016.05.002
Noroozi, O., Banihashem, S. K., Biemans, H. J., Smits, M., Vervoort, M. T., & Verbaan, C. L. (2023). Design, implementation, and evaluation of an online supported peer feedback module to enhance students’ argumentative essay quality. Education and Information Technologies , 1–28. https://doi.org/10.1007/s10639-023-11683-y .
Papamitsiou, Z., & Economides, A. A. (2014). Learning analytics and educational data mining in practice: A systematic literature review of empirical evidence. Journal of Educational Technology & Society , 17 (4), 49–64. https://doi.org/10.2307/jeductechsoci.17.4.49 . https://www.jstor.org/stable/ .
Pardo, A., Jovanovic, J., Dawson, S., Gašević, D., & Mirriahi, N. (2019). Using learning analytics to scale the provision of personalised feedback. British Journal of Educational Technology , 50 (1), 128–138. https://doi.org/10.1111/bjet.12592 .
Patchan, M. M., Schunn, C. D., & Correnti, R. J. (2016). The nature of feedback: How peer feedback features affect students’ implementation rate and quality of revisions. Journal of Educational Psychology , 108 (8), 1098. https://doi.org/10.1037/edu0000103 .
Ramsden, P. (2003). Learning to teach in higher education . Routledge.
Ray, P. P. (2023). ChatGPT: A comprehensive review on background, applications, key challenges, bias, ethics, limitations and future scope. Internet of Things and Cyber-Physical Systems , 3 , 121–154. https://doi.org/10.1016/j.iotcps.2023.04.003 .
Rüdian, S., Heuts, A., & Pinkwart, N. (2020). Educational Text Summarizer: Which sentences are worth asking for? In DELFI 2020 - The 18th Conference on Educational Technologies of the German Informatics Society (pp. 277–288). Bonn, Germany.
Rummel, N., Walker, E., & Aleven, V. (2016). Different futures of adaptive collaborative learning support. International Journal of Artificial Intelligence in Education , 26 , 784–795. https://doi.org/10.1007/s40593-016-0102-3 .
Shi, M. (2019). The effects of class size and instructional technology on student learning performance. The International Journal of Management Education , 17 (1), 130–138. https://doi.org/10.1016/j.ijme.2019.01.004 .
Article MathSciNet Google Scholar
Toulmin, S. (1958). The uses of argument . Cambridge University Press.
Valero Haro, A., Noroozi, O., Biemans, H. J., Mulder, M., & Banihashem, S. K. (2023). How does the type of online peer feedback influence feedback quality, argumentative essay writing quality, and domain-specific learning? Interactive Learning Environments , 1–20. https://doi.org/10.1080/10494820.2023.2215822 .
White, J., Fu, Q., Hays, S., Sandborn, M., Olea, C., Gilbert, H., & Schmidt, D. C. (2023). A prompt pattern catalog to enhance prompt engineering with chatgpt. arXiv preprint arXiv:2302.11382 . https://doi.org/10.48550/arXiv.2302.11382 .
Wu, Y., & Schunn, C. D. (2020). From feedback to revisions: Effects of feedback features and perceptions. Contemporary Educational Psychology , 60 , 101826. https://doi.org/10.1016/j.cedpsych.2019.101826 .
Xia, Q., Chiu, T. K., Zhou, X., Chai, C. S., & Cheng, M. (2022). Systematic literature review on opportunities, challenges, and future research recommendations of artificial intelligence in education. Computers and Education: Artificial Intelligence , 100118. https://doi.org/10.1016/j.caeai.2022.100118 .
Zawacki-Richter, O., Marín, V. I., Bond, M., & Gouverneur, F. (2019). Systematic review of research on artificial intelligence applications in higher education–where are the educators? International Journal of Educational Technology in Higher Education , 16 (1), 1–27. https://doi.org/10.1186/s41239-019-0171-0 .
Zhang, Z. V., & Hyland, K. (2022). Fostering student engagement with feedback: An integrated approach. Assessing Writing , 51 , 100586. https://doi.org/10.1016/j.asw.2021.100586 .
Zuccon, G., & Koopman, B. (2023). Dr ChatGPT, tell me what I want to hear: How prompt knowledge impacts health answer correctness. arXiv preprint arXiv:2302 .13793. https://doi.org/10.48550/arXiv.2302.13793 .
Download references
No funding has been received for this research.
Author information
Authors and affiliations.
Open Universiteit, Heerlen, The Netherlands
Seyyed Kazem Banihashem & Hendrik Drachsler
Wageningen University and Research, Wageningen, The Netherlands
Seyyed Kazem Banihashem & Omid Noroozi
Ferdowsi University of Mashhad, Mashhad, Iran
Nafiseh Taghizadeh Kerman
The University of Alabama, Tuscaloosa, USA
Jewoong Moon
DIPE Leibniz Institute, Goethe University, Frankfurt, Germany
Hendrik Drachsler
You can also search for this author in PubMed Google Scholar
Contributions
S. K. Banihashem led this research experiment. N. T. Kerman contributed to the data analysis and writing. O. Noroozi contributed to the designing, writing, and reviewing the manuscript. J. Moon contributed to the writing and revising the manuscript. H. Drachsler contributed to the writing and revising the manuscript.
Corresponding author
Correspondence to Seyyed Kazem Banihashem .
Ethics declarations
Declaration of ai-assisted technologies in the writing process.
The authors used generative AI for language editing and took full responsibility.
Competing interests
The authors declare that they have no competing interests.
Additional information
Publisher’s note.
Springer Nature remains neutral with regard to jurisdictional claims in published maps and institutional affiliations.
Rights and permissions
Open Access This article is licensed under a Creative Commons Attribution 4.0 International License, which permits use, sharing, adaptation, distribution and reproduction in any medium or format, as long as you give appropriate credit to the original author(s) and the source, provide a link to the Creative Commons licence, and indicate if changes were made. The images or other third party material in this article are included in the article’s Creative Commons licence, unless indicated otherwise in a credit line to the material. If material is not included in the article’s Creative Commons licence and your intended use is not permitted by statutory regulation or exceeds the permitted use, you will need to obtain permission directly from the copyright holder. To view a copy of this licence, visit http://creativecommons.org/licenses/by/4.0/ .
Reprints and permissions
About this article
Cite this article.
Banihashem, S.K., Kerman, N.T., Noroozi, O. et al. Feedback sources in essay writing: peer-generated or AI-generated feedback?. Int J Educ Technol High Educ 21 , 23 (2024). https://doi.org/10.1186/s41239-024-00455-4
Download citation
Received : 20 November 2023
Accepted : 18 March 2024
Published : 12 April 2024
DOI : https://doi.org/10.1186/s41239-024-00455-4
Share this article
Anyone you share the following link with will be able to read this content:
Sorry, a shareable link is not currently available for this article.
Provided by the Springer Nature SharedIt content-sharing initiative
- AI-generated feedback
- Essay writing
- Feedback sources
- Higher education
- Peer feedback
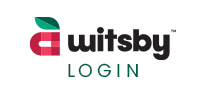
The Power of Peers
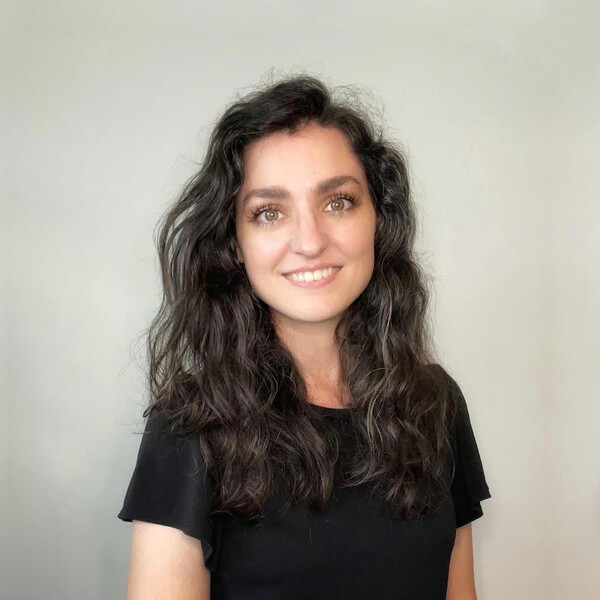
Unpacking Levels of Learning
Building nuanced understanding, positive outcomes of peer learning.
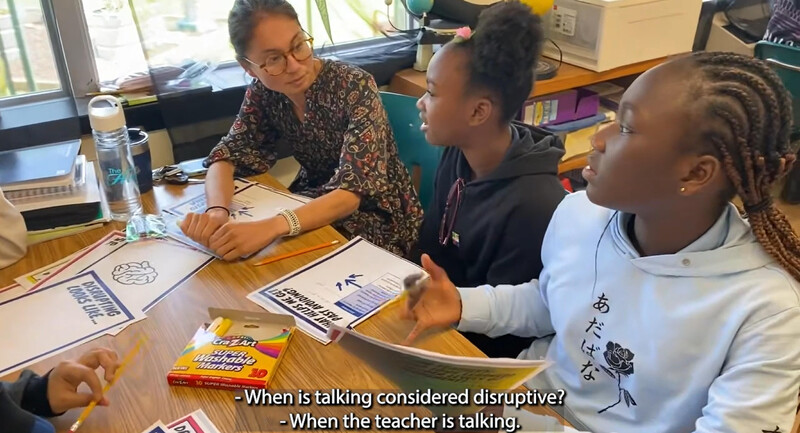
“We are learning about ‘how do animals adapt to the coldness?’ Like polar bears—they’re adapted to cold winter and ice and stuff. The frog adapted by camouflaging themselves. Same thing with the lizard camouflaging itself.”
Learning from peers creates environments where knowledge is constructed collaboratively, enhancing the learning experience for everyone involved.
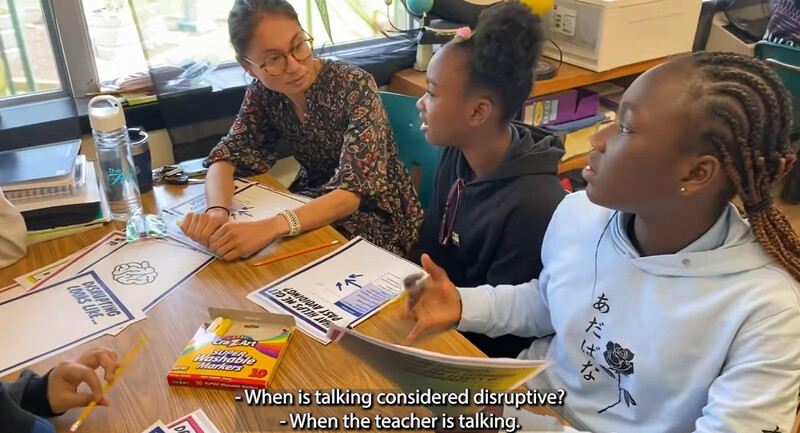
“This actually helped me learn a lot because, you know, you hear big fancy words from adults and you understand it, but you don’t realize how simple it really is until you talk to the kids about it. They lay [a concept] down in front of you…That helped me get a deeper understanding of it.”

Show & Tell / Peer Tutoring Strategies
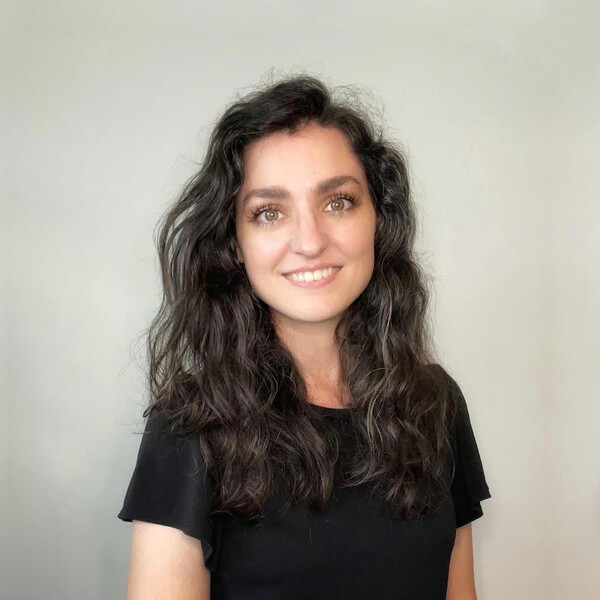
Jessica Comola is an editor with Educational Leadership magazine.
ASCD is dedicated to professional growth and well-being.
Let's put your vision into action., related blogs.
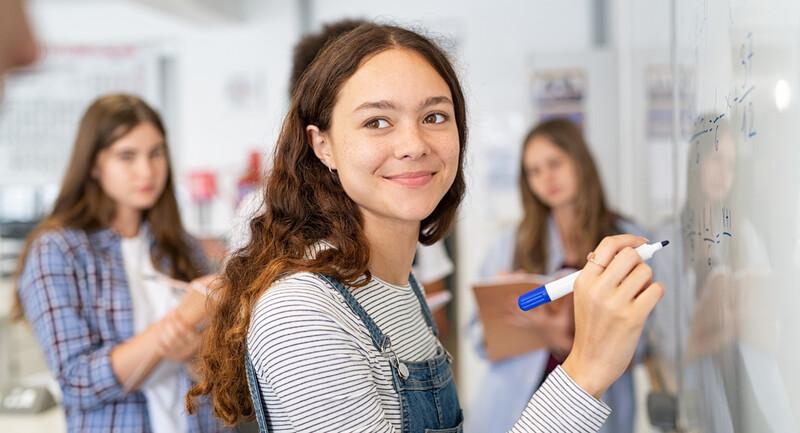
4 Ways to Boost Math Instruction
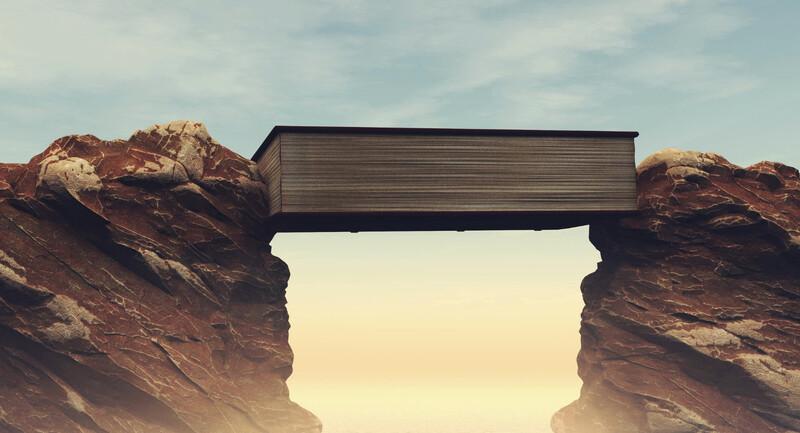
Storytelling Can Bridge Cultural Gaps
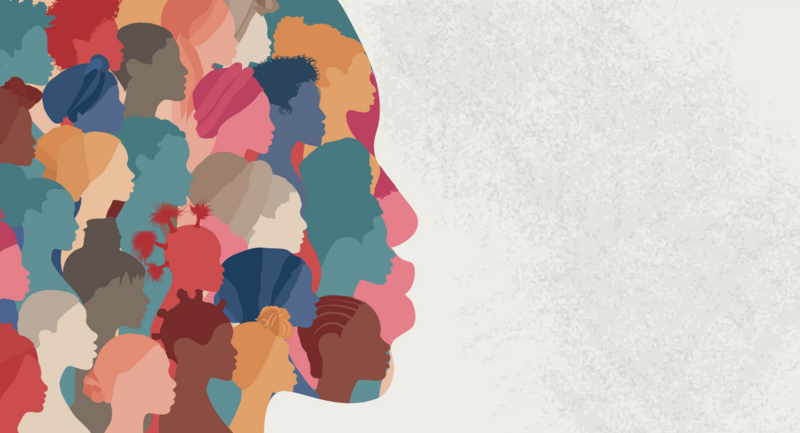
Confronting the Uncomfortable: Strategies to Teach About Enslavement
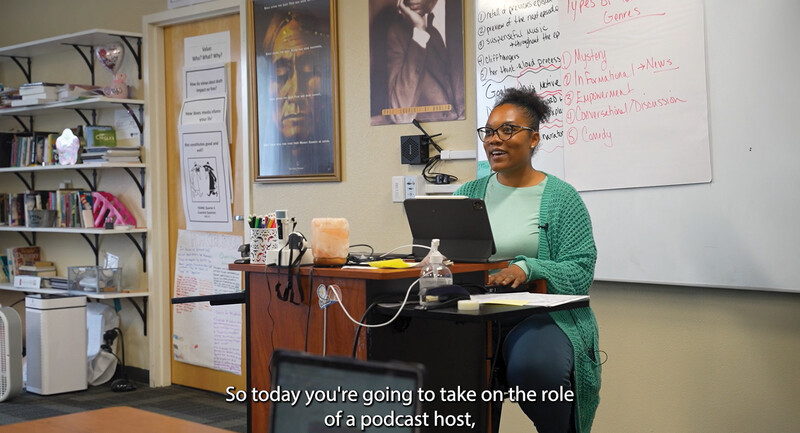
Today, You’re a Podcast Host
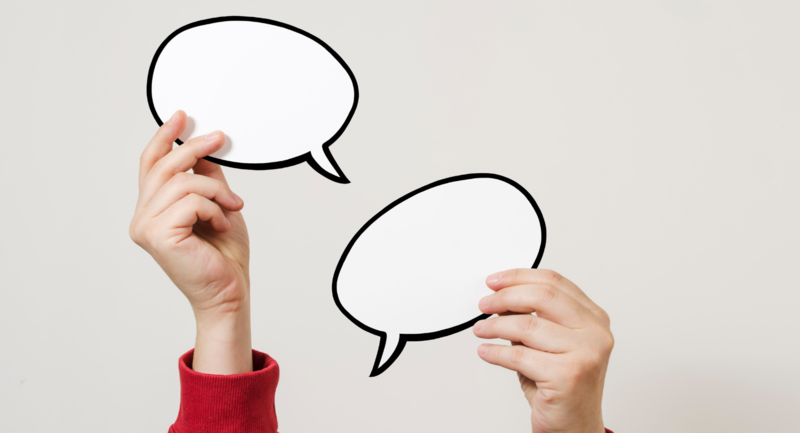
Young Minds, Big Thinking
Philippine E-Journals
Home ⇛ international social science review ⇛ vol. 2 no. 1 (2020), developing essay writing skills of als learners through peer teaching.
Luisa U. Maliao | Mirasol A. Muñez
The main purpose of this study is to establish if peer teaching is a useful instructional intervention to promote shared learning between peers, with learners as peer teacher and peer learner in Alternative Learning System East 1 District specifically in the three sessions of the District ALS Coordinator and two Mobile Teachers. Learners provide as skilled teachers to produce significant learning probability and enhance ability in essay writing with peer learners. Findings revealed a positive result on the use of peer teaching in essay writing as an instructional intervention. The researchers assess the pre-test and the post-test outcome of the learners and identified the definite criterion where the learner finds it difficult to organize in writing an essay. The title of the essay was taken from the lessons discussed in every session. All learners were given thirty- minutes bound to time in writing an essay. On the other hand, the researchers evaluate the learner’s outcome base on the revised rubric. The researchers choose this instructional intervention to help improve and boost the writing skills of the low achiever learner. The researchers use T-Test to evaluate the results of the pre and post-test after using the intervention. The positive effect of this study would facilitate the development of the writing skills in every learner. Repercussion and expectation of peer teaching as a technique in improving learners' capability in writing an essay are discussed.
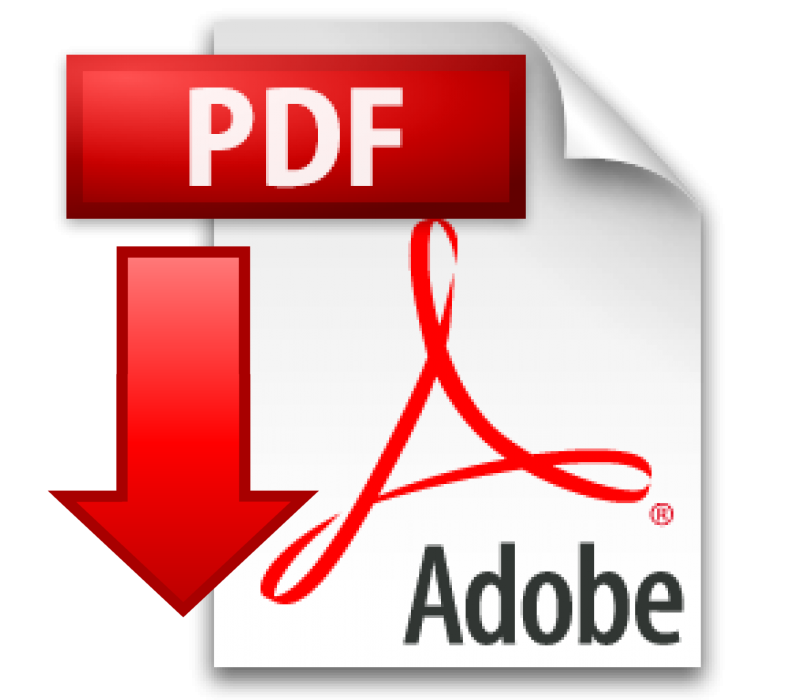
Share Article:

- Citation Generator
- ">Indexing metadata
- Print version

Copyright © 2024 KITE E-Learning Solutions | Exclusively distributed by CE-Logic | Terms and Conditions
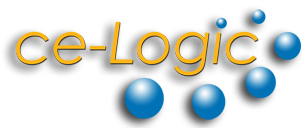

100742 - Year of the Peer: Peer Soars in 2024
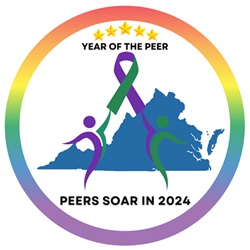
The Mission of Virginia’s Year of the Peer initiative is to celebrate champions of recovery, unify Peer Recovery Specialists, and build bridges of understanding with clinical colleagues, community partners, and organizations across Virginia. We envision Virginia as an integrated recovery-oriented system of care where Peer Specialists are valued for being champions, building connection, and offering support to individuals with mental health and substance use challenges.
Fee Information
Location information.
The Office of Recovery Services will cover lodging expenses for a limited number of individuals that are 75+ miles from the conference hotel location (110 Shenandoah Ave NW Roanoke, VA 24016), one way. Please note that there are a limited number of slots and not everyone eligible for this opportunity will be chosen. Individuals that meet the lodging eligibility criteria will need to submit their registration before June 15th. After that date, all conference attendees will be responsible for their own lodging costs.
Please note that EVERYONE will need to register at the $50 registration fee. If attendees cancel registrations after July 15th, your payment method will be charged a $30 cancellation fee.
100742 - 566703
For the most up-to-date information related to this program, please visit: YOTP: Peers SOAR in 2024
Additional Information: A block of lodging rooms has been reserved at the Hotel Ronaoke and Conference Center at $183.00 per night plus applicable taxes
Refund and Cancellation Policy: Refund requests must be received 14 calendar days prior to the program start date. A $30 administrative fee will be deducted from all refunds. Requests should be sent by email or by initiating a drop request through the student portal in our online registration system. As an alternative to a refund, you may send a substitute at no additional cost. Please contact us at 540-231-5182 or e-mail [email protected] to request a substitution. Please note: refunds will not be issued for no-shows or for cancellations received on or after the program start date. In the unlikely event that this program is cancelled or postponed due to insufficient enrollments or unforeseen circumstances, the university will fully refund registration fees but cannot be held responsible for any other expenses, including cancellation or change charges assessed by airlines, hotels, travel agencies, or other organizations.
Anyone requesting special requirements for this program should contact [email protected] .
Session Time-Out
100742 - 566703 - year of the peer: peer soars in 2024, fees starting at, privacy policy, cookie policy.
Cookie policy
This statement explains how we use cookies on our website. For information about what types of personal information will be gathered when you visit the website, and how this information will be used, please see our privacy policy.
How we use cookies
All of our web pages use "cookies". A cookie is a small file of letters and numbers that we place on your computer or mobile device if you agree. These cookies allow us to distinguish you from other users of our website, which helps us to provide you with a good experience when you browse our website and enables us to improve our website.
Types of cookies we use
We use the following types of cookies:
- Strictly necessary cookies - these are essential in to enable you to move around the websites and use their features. Without these cookies the services you have asked for, such as signing in to your account, cannot be provided.
- Performance cookies - these cookies collect information about how visitors use a website, for instance which pages visitors go to most often. We use this information to improve our websites and to aid us in investigating problems raised by visitors. These cookies do not collect information that identifies a visitor.
- Functionality cookies - these cookies allow the website to remember choices you make and provide more personal features. For instance, a functional cookie can be used to remember the items that you have placed in your shopping cart. The information these cookies collect may be anonymized and they cannot track your browsing activity on other websites.
Most web browsers allow some control of most cookies through the browser settings. To find out more about cookies, including how to see what cookies have been set and how to manage and delete them please visit http://www.allaboutcookies.org/ .
Specific cookies we use
The list below identify the cookies we use and explain the purposes for which they are used. We may update the information contained in this section from time to time.
- JSESSIONID: This cookie is used by the application server to identify a unique user's session.
- registrarToken: This cookie is used to remember items that you have added to your shopping cart
- locale: This cookie is used to remember your locale and language settings.
- cookieconsent_status: This cookie is used to remember if you've already dismissed the cookie consent notice.
- _ga_UA-########: These cookies are used to collect information about how visitors use our site. We use the information to compile reports and to help us improve the website. The cookies collect information in an anonymous form, including the number of visitors to the website, where visitors have come to the site from and the pages they visited. This anonymized visitor and browsing information is stored in Google Analytics.
Changes to our Cookie Statement
Any changes we may make to our Cookie Policy in the future will be posted on this page.
Information Literacy Competency Standards for Higher Education
Journal title, journal issn, volume title.
The Information Literacy Competency Standards for Higher Education (originally approved in January 2000) were rescinded by the ACRL Board of Directors on June 25, 2016, at the 2016 ALA Annual Conference in Orlando, Florida, which means they are no longer in force.
Description
Item.page.type, item.page.format, collections.
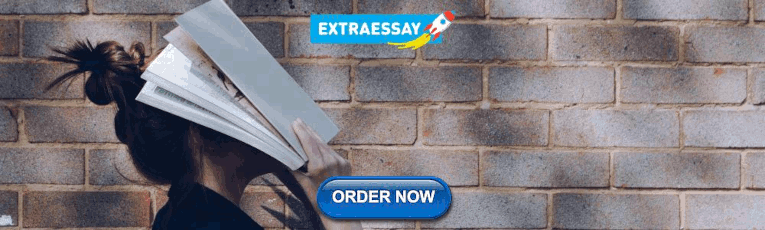
COMMENTS
In peer instruction, instructors pose a challenging question to students, students answer the question individually, students work with a partner in the class to discuss their answers, and finally students answer the question again. A large body of evidence shows that peer instruction benefits student learning. To determine the mechanism for these benefits, we collected semester-long data from ...
Peer learning is an education method that helps students solidify their knowledge by teaching each other. One student tutoring another in a supervised environment can result in better learning and retention. ... For instance, an AP English Language teacher might have students read one another's essays to provide informal feedback. ...
Peer teaching involves one or more students teaching other students in a particular subject area and builds on the belief that "to teach is to learn twice" (Whitman, 1998).". "Peer teaching can enhance learning by enabling learners to take responsibility for reviewing, organizing, and consolidating existing knowledge and material ...
Peer instruction benefits not just the specific questions posed during discussion, but also improves accuracy on later similar problems (e.g., Smith et al., 2009 ). One of the consistent empirical hallmarks of peer instruction is that students' answers are more frequently correct following discussion than preceding it.
"Peer learning" OR "peer instruction" OR "peer-to-peer learning" OR "cooperative learning" OR "collaborative learning" Lthese terms are used somewhat interchangeably in titles and abstracts. Facilitat* Lsome sources will describe the instructor's role in a peer learning classroom as facilitation
In peer teaching, students learn in groups by teaching other students in situations that are planned and directed by a teacher. Peer teaching is not a new method and is based on Bandura's social learning theory, Piaget's cognitive development theory, and Vygotsky's social constructivist learning theory (Iserbyt, Madou, Vergauwen, & Behets, 2011 ...
The term 'Peer Teaching' itself means the sharing of knowledge, ideas and experiences among participants. Beyond this, it is described as way of moving beyond independence to interdependent or mutual learning (Boud, 1988). David Boud (2012) mentioned that "Peer Teaching is …show more content….
University teachers do not comprise the view of peer teaching necessarily resulting in greater academic achievement gains or deep learning. University teachers identify and esteem other pedagogical benefits such as improving students': critical thinking, learning autonomy, motivation, collaborative and communicative skills.
Collaboration and Conversation with Peers. Self-study 'provides fertile ground for investigating and developing your knowledge about teaching with evidence that is immediate and personal,' according to Samaras and Freese (Citation 2006, p. 43).They also describe it as 'multiple and multifaceted … using different theories, various research methods, with numerous purposes' (p. 46).
Introduction. Professional conversations about teaching and learning can support the development of teaching practice in Higher Education (Ashgar & Pilkington, Citation 2018; Roxå & Mårtensson, Citation 2009).Professional dialogue is noted as a space for professional learning where professionals listen carefully (ibid) and can invoke reflection and thinking about practice.
Peer support groups are also known as private study groups. These tend not to have a teacher's presence and are often organized by peers themselves. Common peer study groups take place during free time, after school or on weekends. A peer study group can be beneficial for motivating students in the lead-up to exams or assignment due dates.
Peer instruction, a form of active learning, is generally defined as an opportunity for peers to discuss ideas or to share answers to questions in an in-class environment, where they also have opportunities for further interactions with their instructor. When implementing peer instruction, instructors have many choices to make about group design, assignment format, and grading, among others ...
This paper reports on the feedback of a case study on peer teaching acti vity in a third year unive r-. sity mathematics course. Th e objective of the peer -teaching activity was to motivate ...
Summary. This Element traces the evolution of peer assessment in writing instruction and illustrates how peer assessment can be used to promote the teaching and learning of writing in various sociocultural and educational contexts. Specifically, this Element aims to present a critical discussion of the major themes and research findings in the ...
Leverage peer feedback to improve your teaching: Peer review can help to increase critical reflection of teaching and can motivate and encourage you to experiment with new teaching methods. Research has shown that instructors who participate in peer review incorporate more active learning strategies in their courses, increase the quality of their feedback to students, and report enjoying ...
The Center for Teaching and Learning provides sample worksheets (Peer Review Worksheet for Thesis-Driven Essay) that may be adapted to suit various types of courses and genres of writing. Before the Semester Starts 1. Determine how peer review will fit into the course. A. Decide which writing assignments will include a peer-review session.
Scaffolding the peer review provides an opportunity for students to read a piece multiple times to assess different elements of writing. First the class reviews the objective as a whole group. Then peer pairs review their individual writing with a focus on the defined learning objective. Some students may be reluctant to criticize peers' work.
Peer feedback is introduced as an effective learning strategy, especially in large-size classes where teachers face high workloads. However, for complex tasks such as writing an argumentative essay, without support peers may not provide high-quality feedback since it requires a high level of cognitive processing, critical thinking skills, and a deep understanding of the subject.
In the video that accompanies Douglas Fisher and Nancy Frey's October Show & Tell column, we see the protégé effect in action as high schoolers engage in cross-age tutoring with elementary school students on the topic of engagement. For all students in the video, younger and older, this peer learning approach not only enhances academic understanding, but also nurtures crucial social and ...
The peer correction and teacher correction technique was found productive in teaching writing through action research as a whole. Journal of NELTA, Vol. 17 No. 1-2, December 2012, Page 70-82 DOI ...
The quest to improve teaching on a wide scale is an enduring challenge globally. Yet demonstrable improvement in teaching quality is both elusive and slow. In this essay, I explore some of the complexities that contribute to the slow pace of change, including: the slippage between teachers and teaching as the object of improvement; the poorly ...
Learners provide as skilled teachers to produce significant learning probability and enhance ability in essay writing with peer learners. Findings revealed a positive result on the use of peer teaching in essay writing as an instructional intervention. The researchers assess the pre-test and the post-test outcome of the learners and identified ...
Background Therapeutic Radiographers (RT) and Speech and Language Therapists (SLT) work closely together in caring for people with head and neck cancer and need a strong understanding of each others' roles. Peer teaching has been shown to be one of the most effective methods of teaching; however, no studies to date, have involved RT and SLT students. This research aims to establish the ...
We show that peer effects estimates obtained using our approach can differ significantly from classical estimates (where effort is approximated) if the network includes isolated students. Applying our approach to data on high school students in the United States, we find that peer effect estimates relying on GPA as a proxy for effort are 40% ...
Year of the Peer: Peer Soars in 2024. For the most up-to-date information related to this program, please visit: YOTP: Peers SOAR in 2024 Additional Information: A block of lodging rooms has been reserved at the Hotel Ronaoke and Conference Center at $183.00 per night plus applicable taxes Refund and Cancellation Policy: Refund requests must be received 14 calendar days prior to the program ...
The Information Literacy Competency Standards for Higher Education (originally approved in January 2000) were rescinded by the ACRL Board of Directors on June 25, 2016, at the 2016 ALA Annual Conference in Orlando, Florida, which means they are no longer in force.
This study investigated the relationships between maternal, paternal, teacher, and peer social support, behavioral engagement, and school burnout among Finnish lower secondary student athletes ( n = 209) and regular students ( n = 156) using cross-sectional questionnaire data collected in Grade 7. Structural equation modeling revealed positive associations between social support and student ...
Pathways for Advancing Careers and Education (PACE) Evaluation and Health Profession Opportunity Grants (HPOG 1.0) Impact Study: Joint Nine-Year Follow-Up Analysis Plan ... These pages feature resources, publications, and tools to provide information on Peer TA initiatives that are sponsored through the U.S. Department of Health and Human ...
Peer teaching involves interdependence as students participate as both teachers and learners who give and receive as they help each other gain knowledge and understanding (Barkley, Cross, & Major, 2005). Peer teaching has been researched as an effective method to enhance students' academic performance.
"Beyond the Bus: Rosa Parks' Lifelong Struggle for Justice" Biographer Jeanne Theoharis, professor of political science at Brooklyn College of the City University of New York, describes in this article written for the Library of Congress Magazine, vol. 4 no. 2 (March-April 2015):16-18, the recently acquired Rosa Parks Papers and how they shed new light on Parks and her activism.