- Business Essentials
- Leadership & Management
- Credential of Leadership, Impact, and Management in Business (CLIMB)
- Entrepreneurship & Innovation
- Digital Transformation
- Finance & Accounting
- Business in Society
- For Organizations
- Support Portal
- Media Coverage
- Founding Donors
- Leadership Team
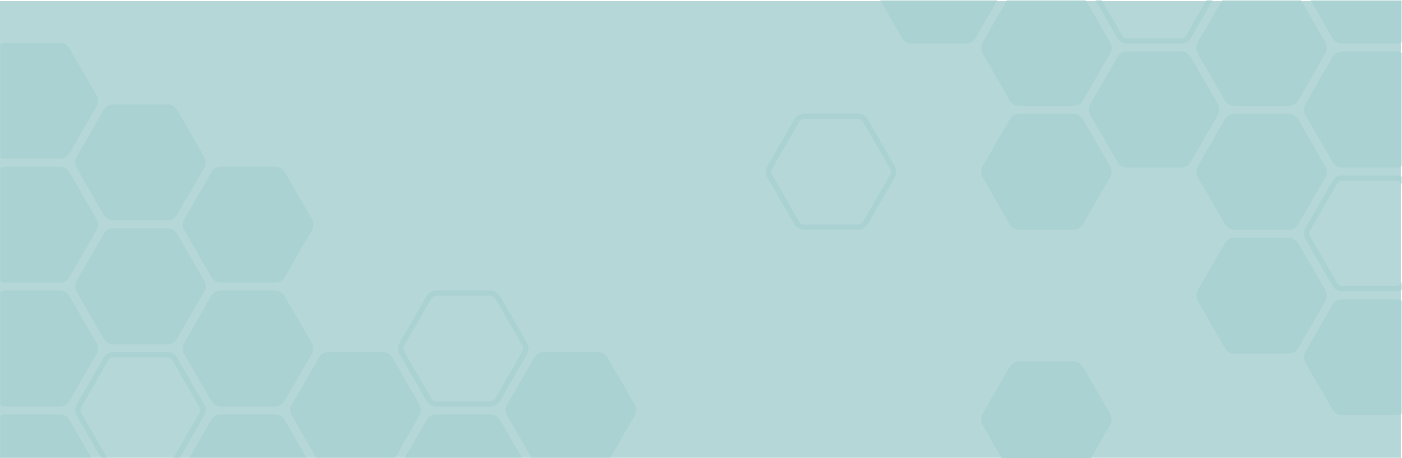
- Harvard Business School →
- HBS Online →
- Business Insights →
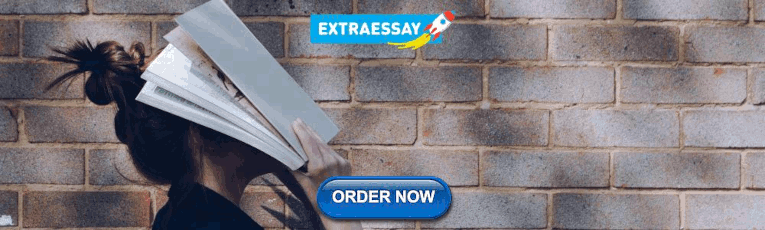
Business Insights
Harvard Business School Online's Business Insights Blog provides the career insights you need to achieve your goals and gain confidence in your business skills.
- Career Development
- Communication
- Decision-Making
- Earning Your MBA
- Negotiation
- News & Events
- Productivity
- Staff Spotlight
- Student Profiles
- Work-Life Balance
- AI Essentials for Business
- Alternative Investments
- Business Analytics
- Business Strategy
- Business and Climate Change
- Design Thinking and Innovation
- Digital Marketing Strategy
- Disruptive Strategy
- Economics for Managers
- Entrepreneurship Essentials
- Financial Accounting
- Global Business
- Launching Tech Ventures
- Leadership Principles
- Leadership, Ethics, and Corporate Accountability
- Leading Change and Organizational Renewal
- Leading with Finance
- Management Essentials
- Negotiation Mastery
- Organizational Leadership
- Power and Influence for Positive Impact
- Strategy Execution
- Sustainable Business Strategy
- Sustainable Investing
- Winning with Digital Platforms
Bad Data Visualization: 5 Examples of Misleading Data
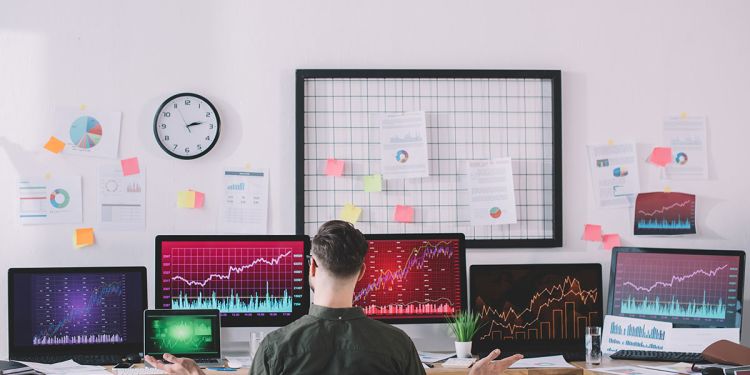
- 28 Jan 2021
Data visualization , the process of creating visual representations of data, offers businesses various benefits. One of the most powerful is the ability to communicate with data to a wider audience both internally and externally. This, in turn, enables more stakeholders to make data-driven decisions .
In recent years, a proliferation of data visualization tools has made it easier than ever for professionals without a data background to create graphics and charts.
While this has primarily been a positive development, it’s likely given rise to one specific problem: poor or otherwise inaccurate data visualization. Just as well-crafted data visualizations benefit organizations that generate and use them, poorly crafted ones create several problems.
Access your free e-book today.
Data Visualization Mistakes to Avoid
When generating data visualizations, it can be easy to make mistakes that lead to faulty interpretation, especially if you’re just starting out. Below are five common mistakes you should be aware of and some examples that illustrate them.
1. Using the Wrong Type of Chart or Graph
There are many types of charts or graphs you can leverage to represent data visually. This is largely beneficial because it allows you to include some variety in your data visualizations. It can, however, prove detrimental if you choose a graph that isn’t well suited to the insights you’re trying to illustrate.
Some graphs and charts work well for communicating specific types of information, but not others. Problems can arise when you try visualizing data using an unsuitable format.
The nature of your data usually dictates the format of your visualization. The most important characteristic is whether the data is qualitative (it describes or categorizes) or quantitative (meaning, it’s measurable). Qualitative data tends to be better suited to bar graphs and pie charts, while quantitative data is best represented in formats like charts and histograms.
2. Including Too Many Variables
The point of generating a data visualization is to tell a story. As such, it’s your job to include as much relevant information as possible—while excluding irrelevant or unnecessary details. Doing so ensures your audience pays attention to the most important data.
For this reason, in conceptualizing your data visualization, you should first seek to identify the necessary variables. The number of variables you select will then inform your visualization’s format. Ask yourself: Which format will help communicate the data in the clearest manner possible?
A pie chart that compares too many variables, for example, will likely make it difficult to see the differences between values. It might also distract the viewer from the point you’re trying to make.

3. Using Inconsistent Scales
If your chart or graph is meant to show the difference between data points, your scale must remain consistent. If your visualization’s scale is inconsistent, it can cause significant confusion for the viewer.
For example, if you generate a pictogram that uses images to represent a measure of data within a bar graph, the images should remain the same size from column to column.

4. Unclear Linear vs. Logarithmic Scaling
The easiest way to understand the difference between a linear scale and a logarithmic one is to look at the axes that each is built on. When a chart is built on a linear scale, the value between any two points along either axis is always equal and unchanging. When a chart is built on a logarithmic scale, the value between any two points along either axis changes according to a particular pattern.
While logarithmic scaling can be an effective means of communicating data, it must be clear that it’s being used in the graphic. When this is unclear, the viewer may, by default, assume they’re looking at a linear scale, which is more common. This can cause confusion and understate your data’s significance.
For example, the two graphics below communicate the same data. The primary difference is that the graphic on the left is built on a linear scale, while the one on the right is built on a logarithmic one.

This isn’t to say logarithmic scaling shouldn’t be used; simply that, when it’s used, it must be clearly stated and communicated to the viewer.
5. Poor Color Choices
Used carefully, color can make it easier for the viewer to understand the data you’re trying to communicate. When used incorrectly, however, it can cause significant confusion. It’s important to understand the story you’re hoping to tell with your data visualization and choose your colors wisely.
Some common issues that arise when incorporating color into your visualizations include:
- Using too many colors, making it difficult for the reader to quickly understand what they’re looking at
- Using familiar colors (for example, red and green) in surprising ways
- Using colors with little contrast
- Not accounting for viewers who may be colorblind
Consider a bar graph that’s meant to show changes in a technology’s adoption rate. Some of the bars indicate increases in adoption, while others indicate decreases. If you use red to represent increases and green to indicate decreases, it might confuse the viewer, who’s likely accustomed to red meaning negative and green meaning positive.
As another example, consider a US map chart that shows virus infection rates from state to state, with colors representing different concentrations of positive cases. Typically, map charts leverage different shades within the same color family. The lighter the shade, the fewer the cases in that state; the darker the shade, the more cases there are. If you go against this assumption and use a darker color to indicate fewer cases, it could confuse the viewer.

The Impact of Poor Data Visualization
While an inaccurate chart may seem like a small error in the grand scheme of your organization, it can have profound repercussions.
By their nature, inaccurate data visualizations lead your audience to have an inaccurate understanding of the data that’s presented in them. This misunderstanding can lead to faulty insights and poor business decisions—all under the guise that they’re backed by data.
Taken to the extreme, a chart or graph that’s improperly formatted could lead to legal or regulatory issues. For example, a misleading data visualization included in a financial report could cause investors to buy or sell shares of company stock.

For these reasons, a firm understanding of data science is an essential skill for professionals. Knowing when data is accurate and complete, and being able to identify discrepancies between numbers and any visualizations created from them, is a must-have in today’s business environment. An online course centered around business analytics can be an effective way to build these skills .
Do you want to further your data literacy? Download our Beginner’s Guide to Data & Analytics to find out how you can leverage data for professional and organizational success.

About the Author
Home Blog Design Understanding Data Presentations (Guide + Examples)
Understanding Data Presentations (Guide + Examples)
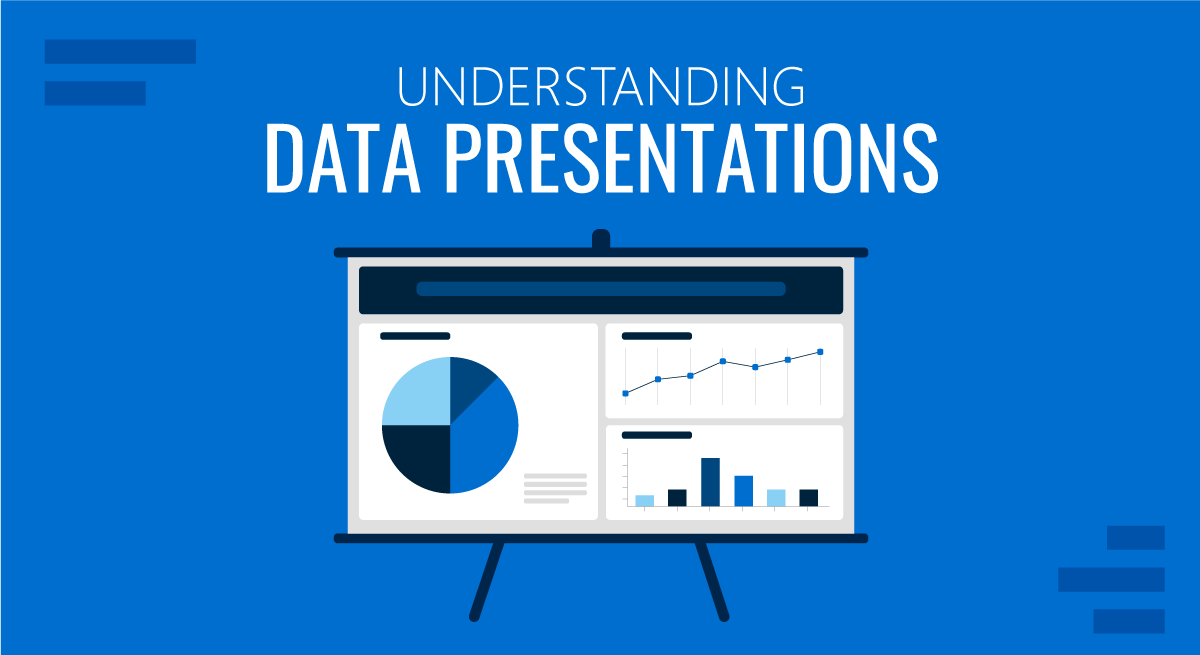
In this age of overwhelming information, the skill to effectively convey data has become extremely valuable. Initiating a discussion on data presentation types involves thoughtful consideration of the nature of your data and the message you aim to convey. Different types of visualizations serve distinct purposes. Whether you’re dealing with how to develop a report or simply trying to communicate complex information, how you present data influences how well your audience understands and engages with it. This extensive guide leads you through the different ways of data presentation.
Table of Contents
What is a Data Presentation?
What should a data presentation include, line graphs, treemap chart, scatter plot, how to choose a data presentation type, recommended data presentation templates, common mistakes done in data presentation.
A data presentation is a slide deck that aims to disclose quantitative information to an audience through the use of visual formats and narrative techniques derived from data analysis, making complex data understandable and actionable. This process requires a series of tools, such as charts, graphs, tables, infographics, dashboards, and so on, supported by concise textual explanations to improve understanding and boost retention rate.
Data presentations require us to cull data in a format that allows the presenter to highlight trends, patterns, and insights so that the audience can act upon the shared information. In a few words, the goal of data presentations is to enable viewers to grasp complicated concepts or trends quickly, facilitating informed decision-making or deeper analysis.
Data presentations go beyond the mere usage of graphical elements. Seasoned presenters encompass visuals with the art of data storytelling , so the speech skillfully connects the points through a narrative that resonates with the audience. Depending on the purpose – inspire, persuade, inform, support decision-making processes, etc. – is the data presentation format that is better suited to help us in this journey.
To nail your upcoming data presentation, ensure to count with the following elements:
- Clear Objectives: Understand the intent of your presentation before selecting the graphical layout and metaphors to make content easier to grasp.
- Engaging introduction: Use a powerful hook from the get-go. For instance, you can ask a big question or present a problem that your data will answer. Take a look at our guide on how to start a presentation for tips & insights.
- Structured Narrative: Your data presentation must tell a coherent story. This means a beginning where you present the context, a middle section in which you present the data, and an ending that uses a call-to-action. Check our guide on presentation structure for further information.
- Visual Elements: These are the charts, graphs, and other elements of visual communication we ought to use to present data. This article will cover one by one the different types of data representation methods we can use, and provide further guidance on choosing between them.
- Insights and Analysis: This is not just showcasing a graph and letting people get an idea about it. A proper data presentation includes the interpretation of that data, the reason why it’s included, and why it matters to your research.
- Conclusion & CTA: Ending your presentation with a call to action is necessary. Whether you intend to wow your audience into acquiring your services, inspire them to change the world, or whatever the purpose of your presentation, there must be a stage in which you convey all that you shared and show the path to staying in touch. Plan ahead whether you want to use a thank-you slide, a video presentation, or which method is apt and tailored to the kind of presentation you deliver.
- Q&A Session: After your speech is concluded, allocate 3-5 minutes for the audience to raise any questions about the information you disclosed. This is an extra chance to establish your authority on the topic. Check our guide on questions and answer sessions in presentations here.
Bar charts are a graphical representation of data using rectangular bars to show quantities or frequencies in an established category. They make it easy for readers to spot patterns or trends. Bar charts can be horizontal or vertical, although the vertical format is commonly known as a column chart. They display categorical, discrete, or continuous variables grouped in class intervals [1] . They include an axis and a set of labeled bars horizontally or vertically. These bars represent the frequencies of variable values or the values themselves. Numbers on the y-axis of a vertical bar chart or the x-axis of a horizontal bar chart are called the scale.
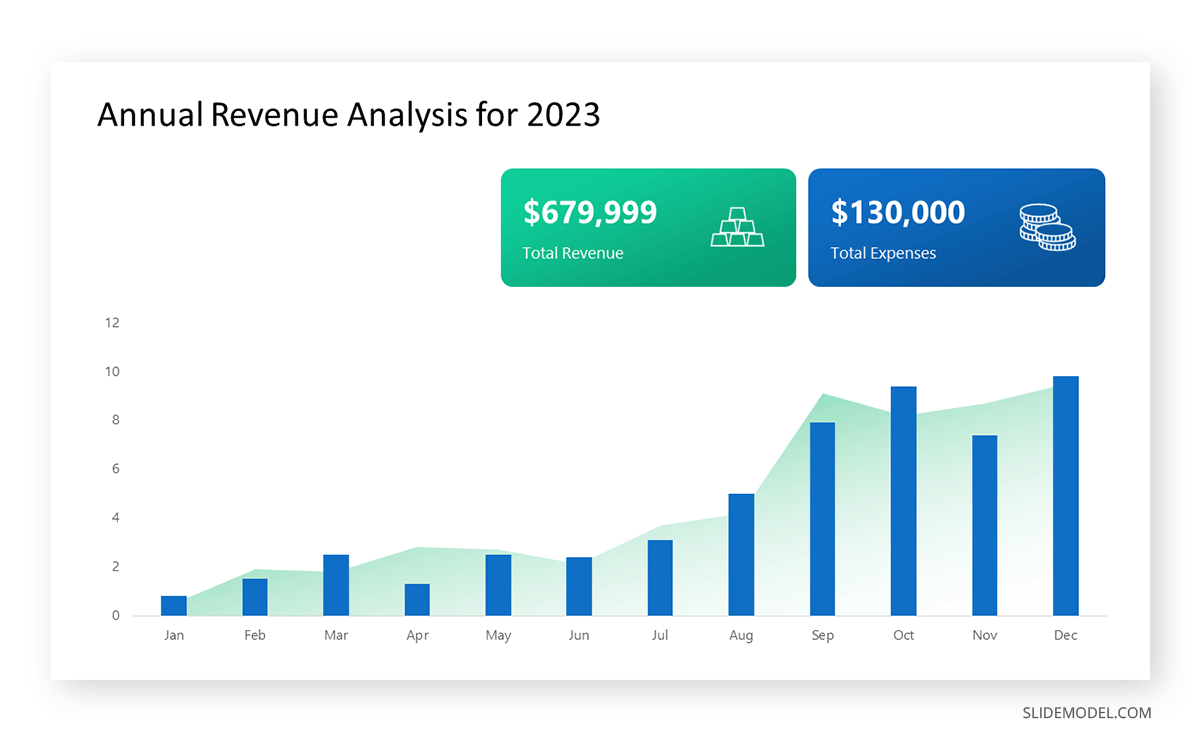
Real-Life Application of Bar Charts
Let’s say a sales manager is presenting sales to their audience. Using a bar chart, he follows these steps.
Step 1: Selecting Data
The first step is to identify the specific data you will present to your audience.
The sales manager has highlighted these products for the presentation.
- Product A: Men’s Shoes
- Product B: Women’s Apparel
- Product C: Electronics
- Product D: Home Decor
Step 2: Choosing Orientation
Opt for a vertical layout for simplicity. Vertical bar charts help compare different categories in case there are not too many categories [1] . They can also help show different trends. A vertical bar chart is used where each bar represents one of the four chosen products. After plotting the data, it is seen that the height of each bar directly represents the sales performance of the respective product.
It is visible that the tallest bar (Electronics – Product C) is showing the highest sales. However, the shorter bars (Women’s Apparel – Product B and Home Decor – Product D) need attention. It indicates areas that require further analysis or strategies for improvement.
Step 3: Colorful Insights
Different colors are used to differentiate each product. It is essential to show a color-coded chart where the audience can distinguish between products.
- Men’s Shoes (Product A): Yellow
- Women’s Apparel (Product B): Orange
- Electronics (Product C): Violet
- Home Decor (Product D): Blue
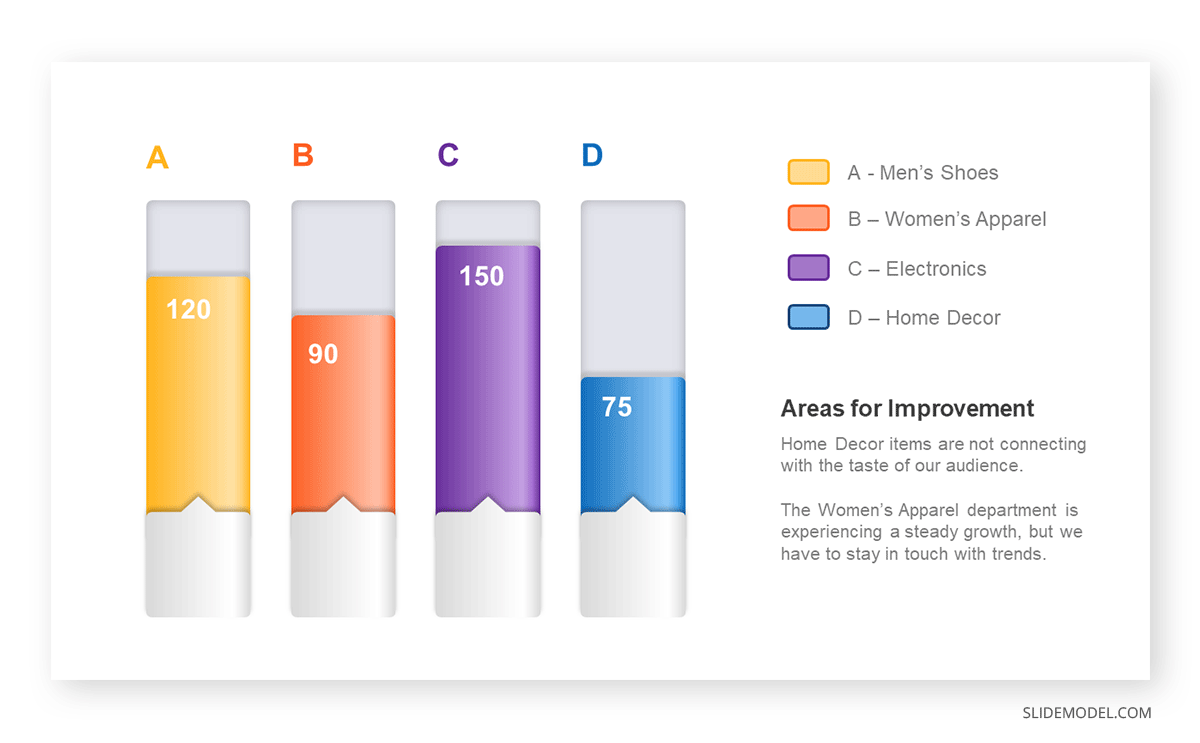
Bar charts are straightforward and easily understandable for presenting data. They are versatile when comparing products or any categorical data [2] . Bar charts adapt seamlessly to retail scenarios. Despite that, bar charts have a few shortcomings. They cannot illustrate data trends over time. Besides, overloading the chart with numerous products can lead to visual clutter, diminishing its effectiveness.
For more information, check our collection of bar chart templates for PowerPoint .
Line graphs help illustrate data trends, progressions, or fluctuations by connecting a series of data points called ‘markers’ with straight line segments. This provides a straightforward representation of how values change [5] . Their versatility makes them invaluable for scenarios requiring a visual understanding of continuous data. In addition, line graphs are also useful for comparing multiple datasets over the same timeline. Using multiple line graphs allows us to compare more than one data set. They simplify complex information so the audience can quickly grasp the ups and downs of values. From tracking stock prices to analyzing experimental results, you can use line graphs to show how data changes over a continuous timeline. They show trends with simplicity and clarity.
Real-life Application of Line Graphs
To understand line graphs thoroughly, we will use a real case. Imagine you’re a financial analyst presenting a tech company’s monthly sales for a licensed product over the past year. Investors want insights into sales behavior by month, how market trends may have influenced sales performance and reception to the new pricing strategy. To present data via a line graph, you will complete these steps.
First, you need to gather the data. In this case, your data will be the sales numbers. For example:
- January: $45,000
- February: $55,000
- March: $45,000
- April: $60,000
- May: $ 70,000
- June: $65,000
- July: $62,000
- August: $68,000
- September: $81,000
- October: $76,000
- November: $87,000
- December: $91,000
After choosing the data, the next step is to select the orientation. Like bar charts, you can use vertical or horizontal line graphs. However, we want to keep this simple, so we will keep the timeline (x-axis) horizontal while the sales numbers (y-axis) vertical.
Step 3: Connecting Trends
After adding the data to your preferred software, you will plot a line graph. In the graph, each month’s sales are represented by data points connected by a line.
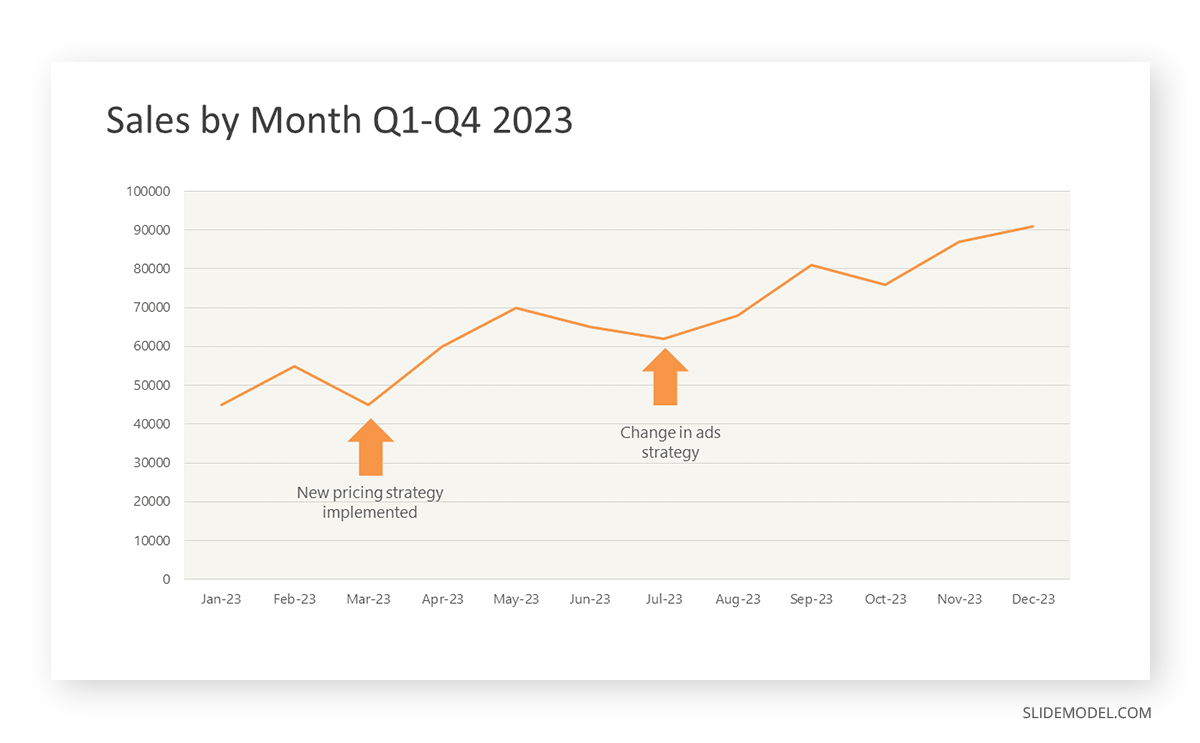
Step 4: Adding Clarity with Color
If there are multiple lines, you can also add colors to highlight each one, making it easier to follow.
Line graphs excel at visually presenting trends over time. These presentation aids identify patterns, like upward or downward trends. However, too many data points can clutter the graph, making it harder to interpret. Line graphs work best with continuous data but are not suitable for categories.
For more information, check our collection of line chart templates for PowerPoint and our article about how to make a presentation graph .
A data dashboard is a visual tool for analyzing information. Different graphs, charts, and tables are consolidated in a layout to showcase the information required to achieve one or more objectives. Dashboards help quickly see Key Performance Indicators (KPIs). You don’t make new visuals in the dashboard; instead, you use it to display visuals you’ve already made in worksheets [3] .
Keeping the number of visuals on a dashboard to three or four is recommended. Adding too many can make it hard to see the main points [4]. Dashboards can be used for business analytics to analyze sales, revenue, and marketing metrics at a time. They are also used in the manufacturing industry, as they allow users to grasp the entire production scenario at the moment while tracking the core KPIs for each line.
Real-Life Application of a Dashboard
Consider a project manager presenting a software development project’s progress to a tech company’s leadership team. He follows the following steps.
Step 1: Defining Key Metrics
To effectively communicate the project’s status, identify key metrics such as completion status, budget, and bug resolution rates. Then, choose measurable metrics aligned with project objectives.
Step 2: Choosing Visualization Widgets
After finalizing the data, presentation aids that align with each metric are selected. For this project, the project manager chooses a progress bar for the completion status and uses bar charts for budget allocation. Likewise, he implements line charts for bug resolution rates.
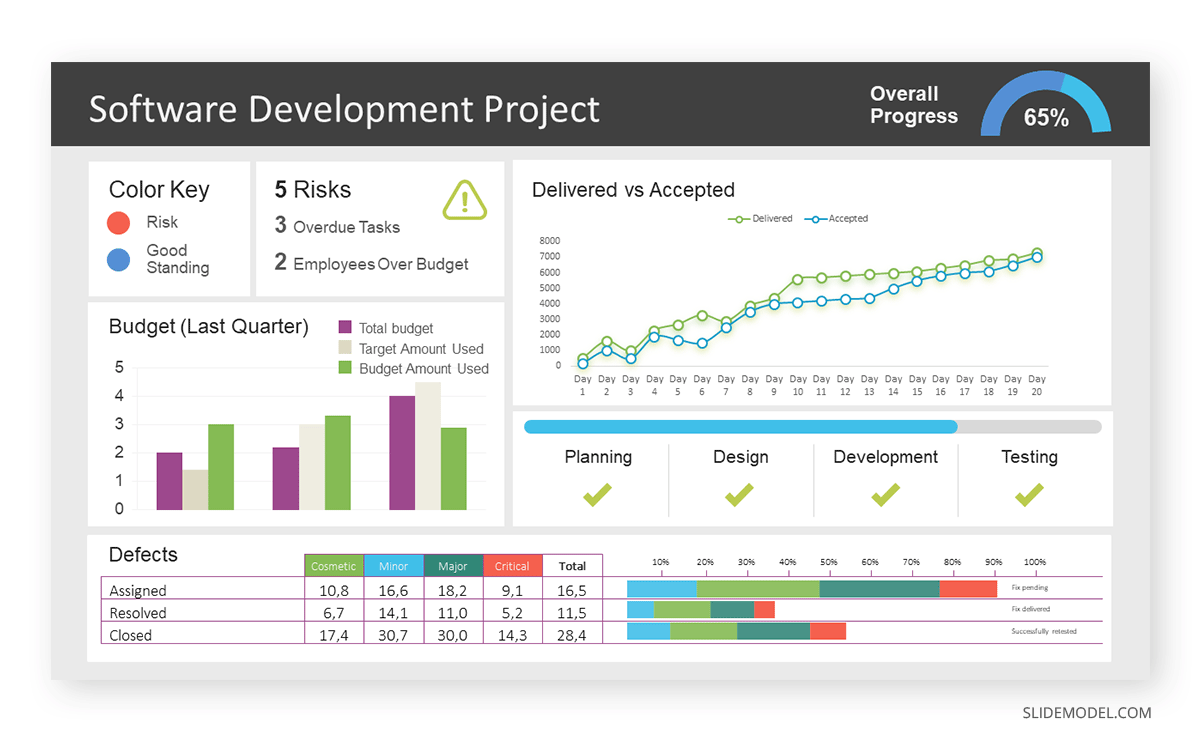
Step 3: Dashboard Layout
Key metrics are prominently placed in the dashboard for easy visibility, and the manager ensures that it appears clean and organized.
Dashboards provide a comprehensive view of key project metrics. Users can interact with data, customize views, and drill down for detailed analysis. However, creating an effective dashboard requires careful planning to avoid clutter. Besides, dashboards rely on the availability and accuracy of underlying data sources.
For more information, check our article on how to design a dashboard presentation , and discover our collection of dashboard PowerPoint templates .
Treemap charts represent hierarchical data structured in a series of nested rectangles [6] . As each branch of the ‘tree’ is given a rectangle, smaller tiles can be seen representing sub-branches, meaning elements on a lower hierarchical level than the parent rectangle. Each one of those rectangular nodes is built by representing an area proportional to the specified data dimension.
Treemaps are useful for visualizing large datasets in compact space. It is easy to identify patterns, such as which categories are dominant. Common applications of the treemap chart are seen in the IT industry, such as resource allocation, disk space management, website analytics, etc. Also, they can be used in multiple industries like healthcare data analysis, market share across different product categories, or even in finance to visualize portfolios.
Real-Life Application of a Treemap Chart
Let’s consider a financial scenario where a financial team wants to represent the budget allocation of a company. There is a hierarchy in the process, so it is helpful to use a treemap chart. In the chart, the top-level rectangle could represent the total budget, and it would be subdivided into smaller rectangles, each denoting a specific department. Further subdivisions within these smaller rectangles might represent individual projects or cost categories.
Step 1: Define Your Data Hierarchy
While presenting data on the budget allocation, start by outlining the hierarchical structure. The sequence will be like the overall budget at the top, followed by departments, projects within each department, and finally, individual cost categories for each project.
- Top-level rectangle: Total Budget
- Second-level rectangles: Departments (Engineering, Marketing, Sales)
- Third-level rectangles: Projects within each department
- Fourth-level rectangles: Cost categories for each project (Personnel, Marketing Expenses, Equipment)
Step 2: Choose a Suitable Tool
It’s time to select a data visualization tool supporting Treemaps. Popular choices include Tableau, Microsoft Power BI, PowerPoint, or even coding with libraries like D3.js. It is vital to ensure that the chosen tool provides customization options for colors, labels, and hierarchical structures.
Here, the team uses PowerPoint for this guide because of its user-friendly interface and robust Treemap capabilities.
Step 3: Make a Treemap Chart with PowerPoint
After opening the PowerPoint presentation, they chose “SmartArt” to form the chart. The SmartArt Graphic window has a “Hierarchy” category on the left. Here, you will see multiple options. You can choose any layout that resembles a Treemap. The “Table Hierarchy” or “Organization Chart” options can be adapted. The team selects the Table Hierarchy as it looks close to a Treemap.
Step 5: Input Your Data
After that, a new window will open with a basic structure. They add the data one by one by clicking on the text boxes. They start with the top-level rectangle, representing the total budget.
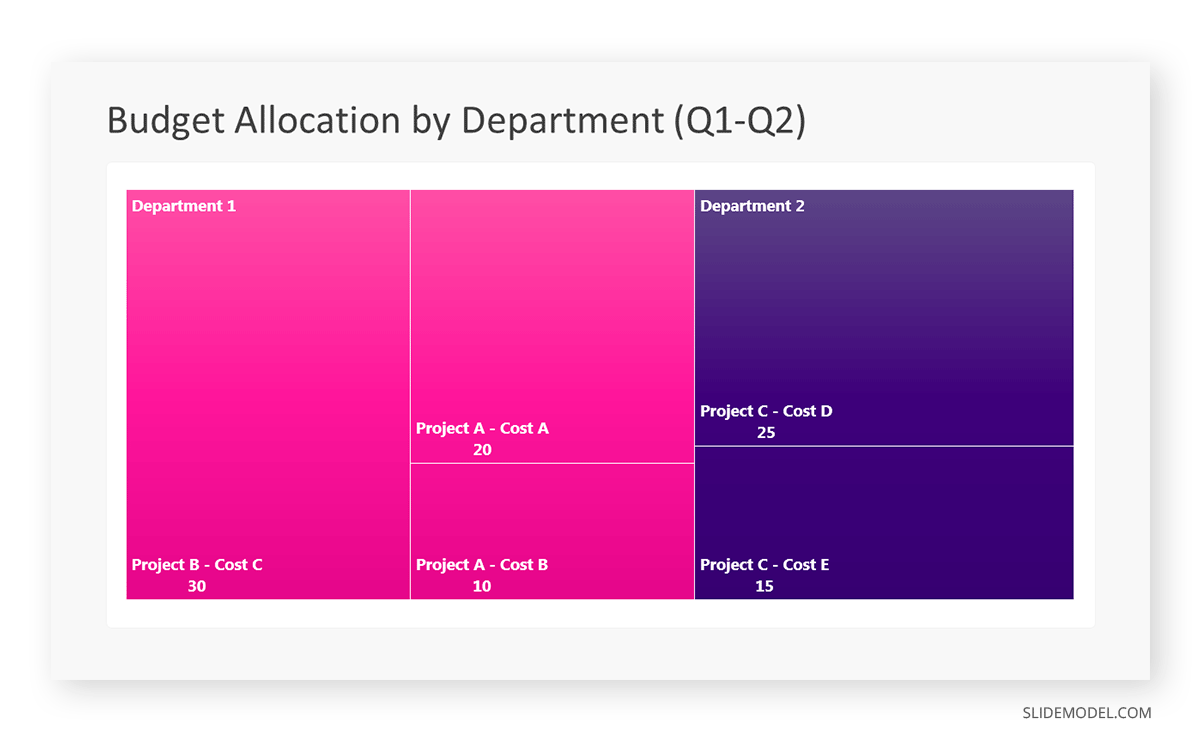
Step 6: Customize the Treemap
By clicking on each shape, they customize its color, size, and label. At the same time, they can adjust the font size, style, and color of labels by using the options in the “Format” tab in PowerPoint. Using different colors for each level enhances the visual difference.
Treemaps excel at illustrating hierarchical structures. These charts make it easy to understand relationships and dependencies. They efficiently use space, compactly displaying a large amount of data, reducing the need for excessive scrolling or navigation. Additionally, using colors enhances the understanding of data by representing different variables or categories.
In some cases, treemaps might become complex, especially with deep hierarchies. It becomes challenging for some users to interpret the chart. At the same time, displaying detailed information within each rectangle might be constrained by space. It potentially limits the amount of data that can be shown clearly. Without proper labeling and color coding, there’s a risk of misinterpretation.
A heatmap is a data visualization tool that uses color coding to represent values across a two-dimensional surface. In these, colors replace numbers to indicate the magnitude of each cell. This color-shaded matrix display is valuable for summarizing and understanding data sets with a glance [7] . The intensity of the color corresponds to the value it represents, making it easy to identify patterns, trends, and variations in the data.
As a tool, heatmaps help businesses analyze website interactions, revealing user behavior patterns and preferences to enhance overall user experience. In addition, companies use heatmaps to assess content engagement, identifying popular sections and areas of improvement for more effective communication. They excel at highlighting patterns and trends in large datasets, making it easy to identify areas of interest.
We can implement heatmaps to express multiple data types, such as numerical values, percentages, or even categorical data. Heatmaps help us easily spot areas with lots of activity, making them helpful in figuring out clusters [8] . When making these maps, it is important to pick colors carefully. The colors need to show the differences between groups or levels of something. And it is good to use colors that people with colorblindness can easily see.
Check our detailed guide on how to create a heatmap here. Also discover our collection of heatmap PowerPoint templates .
Pie charts are circular statistical graphics divided into slices to illustrate numerical proportions. Each slice represents a proportionate part of the whole, making it easy to visualize the contribution of each component to the total.
The size of the pie charts is influenced by the value of data points within each pie. The total of all data points in a pie determines its size. The pie with the highest data points appears as the largest, whereas the others are proportionally smaller. However, you can present all pies of the same size if proportional representation is not required [9] . Sometimes, pie charts are difficult to read, or additional information is required. A variation of this tool can be used instead, known as the donut chart , which has the same structure but a blank center, creating a ring shape. Presenters can add extra information, and the ring shape helps to declutter the graph.
Pie charts are used in business to show percentage distribution, compare relative sizes of categories, or present straightforward data sets where visualizing ratios is essential.
Real-Life Application of Pie Charts
Consider a scenario where you want to represent the distribution of the data. Each slice of the pie chart would represent a different category, and the size of each slice would indicate the percentage of the total portion allocated to that category.
Step 1: Define Your Data Structure
Imagine you are presenting the distribution of a project budget among different expense categories.
- Column A: Expense Categories (Personnel, Equipment, Marketing, Miscellaneous)
- Column B: Budget Amounts ($40,000, $30,000, $20,000, $10,000) Column B represents the values of your categories in Column A.
Step 2: Insert a Pie Chart
Using any of the accessible tools, you can create a pie chart. The most convenient tools for forming a pie chart in a presentation are presentation tools such as PowerPoint or Google Slides. You will notice that the pie chart assigns each expense category a percentage of the total budget by dividing it by the total budget.
For instance:
- Personnel: $40,000 / ($40,000 + $30,000 + $20,000 + $10,000) = 40%
- Equipment: $30,000 / ($40,000 + $30,000 + $20,000 + $10,000) = 30%
- Marketing: $20,000 / ($40,000 + $30,000 + $20,000 + $10,000) = 20%
- Miscellaneous: $10,000 / ($40,000 + $30,000 + $20,000 + $10,000) = 10%
You can make a chart out of this or just pull out the pie chart from the data.
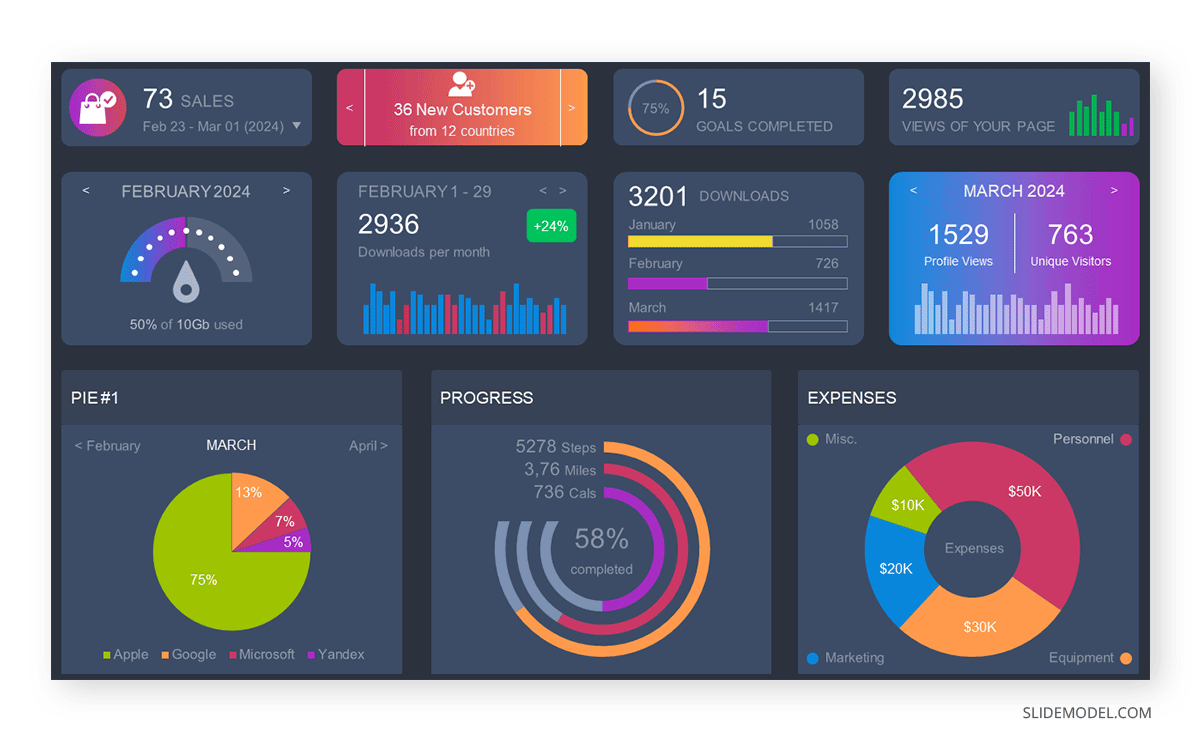
3D pie charts and 3D donut charts are quite popular among the audience. They stand out as visual elements in any presentation slide, so let’s take a look at how our pie chart example would look in 3D pie chart format.
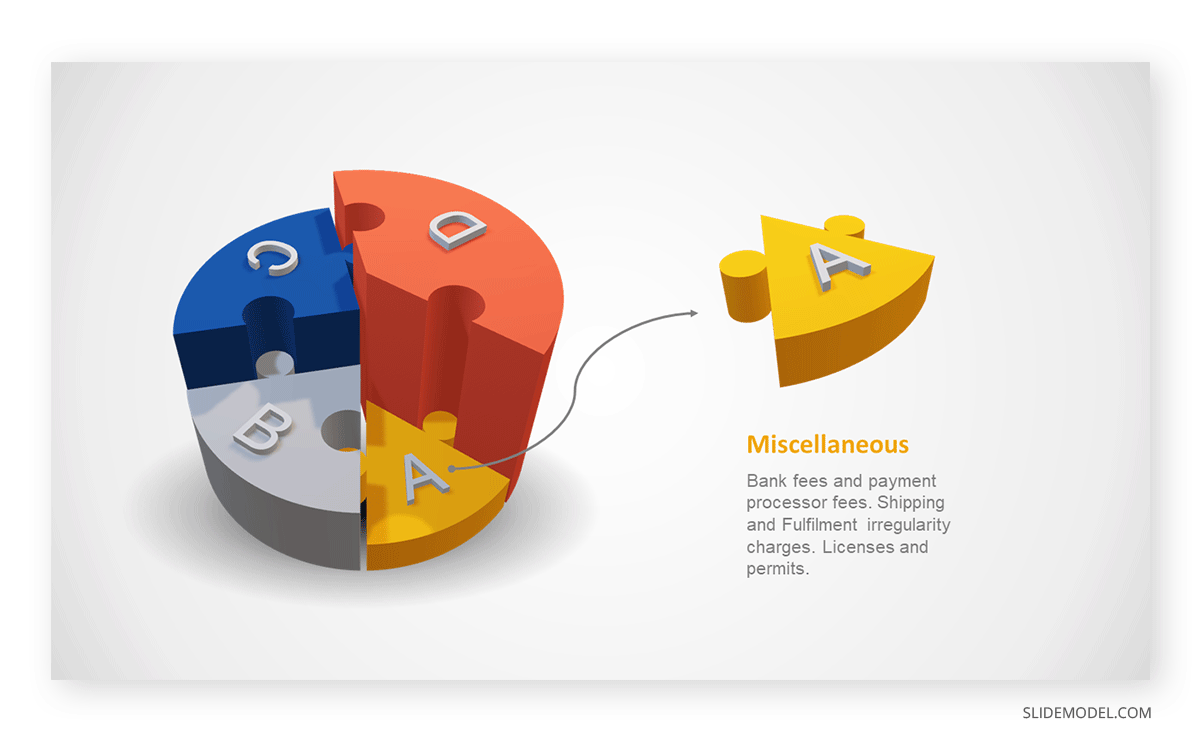
Step 03: Results Interpretation
The pie chart visually illustrates the distribution of the project budget among different expense categories. Personnel constitutes the largest portion at 40%, followed by equipment at 30%, marketing at 20%, and miscellaneous at 10%. This breakdown provides a clear overview of where the project funds are allocated, which helps in informed decision-making and resource management. It is evident that personnel are a significant investment, emphasizing their importance in the overall project budget.
Pie charts provide a straightforward way to represent proportions and percentages. They are easy to understand, even for individuals with limited data analysis experience. These charts work well for small datasets with a limited number of categories.
However, a pie chart can become cluttered and less effective in situations with many categories. Accurate interpretation may be challenging, especially when dealing with slight differences in slice sizes. In addition, these charts are static and do not effectively convey trends over time.
For more information, check our collection of pie chart templates for PowerPoint .
Histograms present the distribution of numerical variables. Unlike a bar chart that records each unique response separately, histograms organize numeric responses into bins and show the frequency of reactions within each bin [10] . The x-axis of a histogram shows the range of values for a numeric variable. At the same time, the y-axis indicates the relative frequencies (percentage of the total counts) for that range of values.
Whenever you want to understand the distribution of your data, check which values are more common, or identify outliers, histograms are your go-to. Think of them as a spotlight on the story your data is telling. A histogram can provide a quick and insightful overview if you’re curious about exam scores, sales figures, or any numerical data distribution.
Real-Life Application of a Histogram
In the histogram data analysis presentation example, imagine an instructor analyzing a class’s grades to identify the most common score range. A histogram could effectively display the distribution. It will show whether most students scored in the average range or if there are significant outliers.
Step 1: Gather Data
He begins by gathering the data. The scores of each student in class are gathered to analyze exam scores.
After arranging the scores in ascending order, bin ranges are set.
Step 2: Define Bins
Bins are like categories that group similar values. Think of them as buckets that organize your data. The presenter decides how wide each bin should be based on the range of the values. For instance, the instructor sets the bin ranges based on score intervals: 60-69, 70-79, 80-89, and 90-100.
Step 3: Count Frequency
Now, he counts how many data points fall into each bin. This step is crucial because it tells you how often specific ranges of values occur. The result is the frequency distribution, showing the occurrences of each group.
Here, the instructor counts the number of students in each category.
- 60-69: 1 student (Kate)
- 70-79: 4 students (David, Emma, Grace, Jack)
- 80-89: 7 students (Alice, Bob, Frank, Isabel, Liam, Mia, Noah)
- 90-100: 3 students (Clara, Henry, Olivia)
Step 4: Create the Histogram
It’s time to turn the data into a visual representation. Draw a bar for each bin on a graph. The width of the bar should correspond to the range of the bin, and the height should correspond to the frequency. To make your histogram understandable, label the X and Y axes.
In this case, the X-axis should represent the bins (e.g., test score ranges), and the Y-axis represents the frequency.
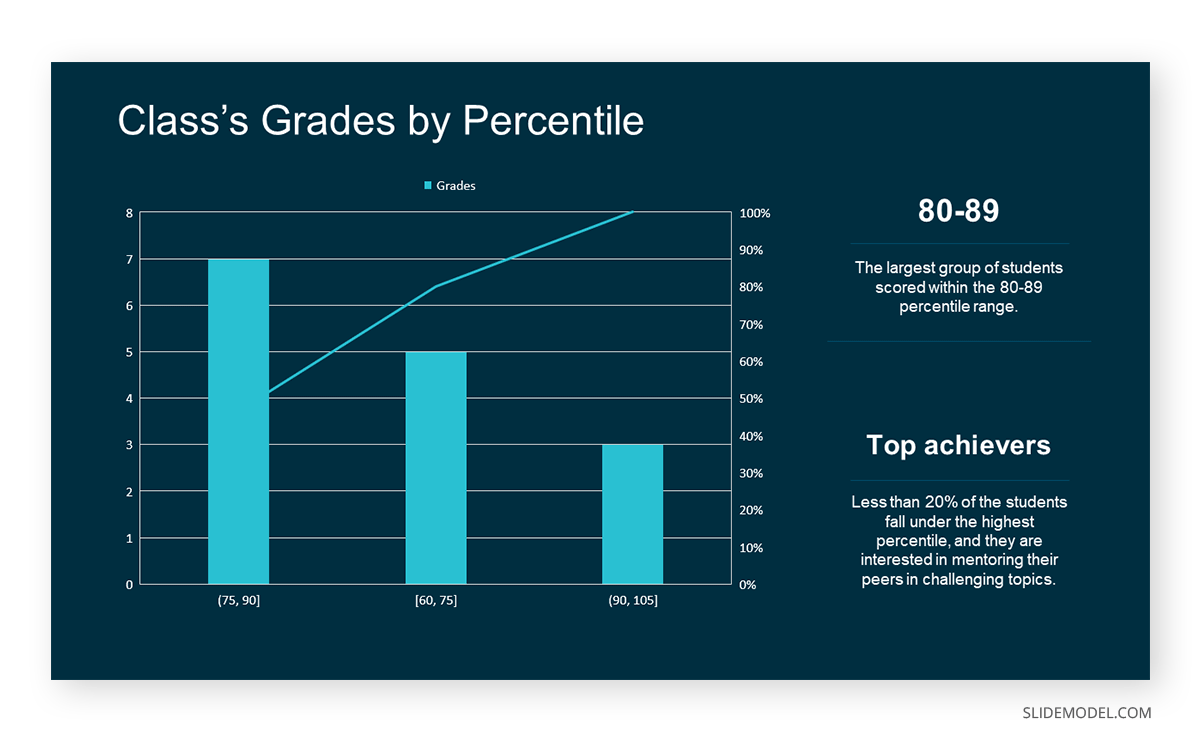
The histogram of the class grades reveals insightful patterns in the distribution. Most students, with seven students, fall within the 80-89 score range. The histogram provides a clear visualization of the class’s performance. It showcases a concentration of grades in the upper-middle range with few outliers at both ends. This analysis helps in understanding the overall academic standing of the class. It also identifies the areas for potential improvement or recognition.
Thus, histograms provide a clear visual representation of data distribution. They are easy to interpret, even for those without a statistical background. They apply to various types of data, including continuous and discrete variables. One weak point is that histograms do not capture detailed patterns in students’ data, with seven compared to other visualization methods.
A scatter plot is a graphical representation of the relationship between two variables. It consists of individual data points on a two-dimensional plane. This plane plots one variable on the x-axis and the other on the y-axis. Each point represents a unique observation. It visualizes patterns, trends, or correlations between the two variables.
Scatter plots are also effective in revealing the strength and direction of relationships. They identify outliers and assess the overall distribution of data points. The points’ dispersion and clustering reflect the relationship’s nature, whether it is positive, negative, or lacks a discernible pattern. In business, scatter plots assess relationships between variables such as marketing cost and sales revenue. They help present data correlations and decision-making.
Real-Life Application of Scatter Plot
A group of scientists is conducting a study on the relationship between daily hours of screen time and sleep quality. After reviewing the data, they managed to create this table to help them build a scatter plot graph:
In the provided example, the x-axis represents Daily Hours of Screen Time, and the y-axis represents the Sleep Quality Rating.
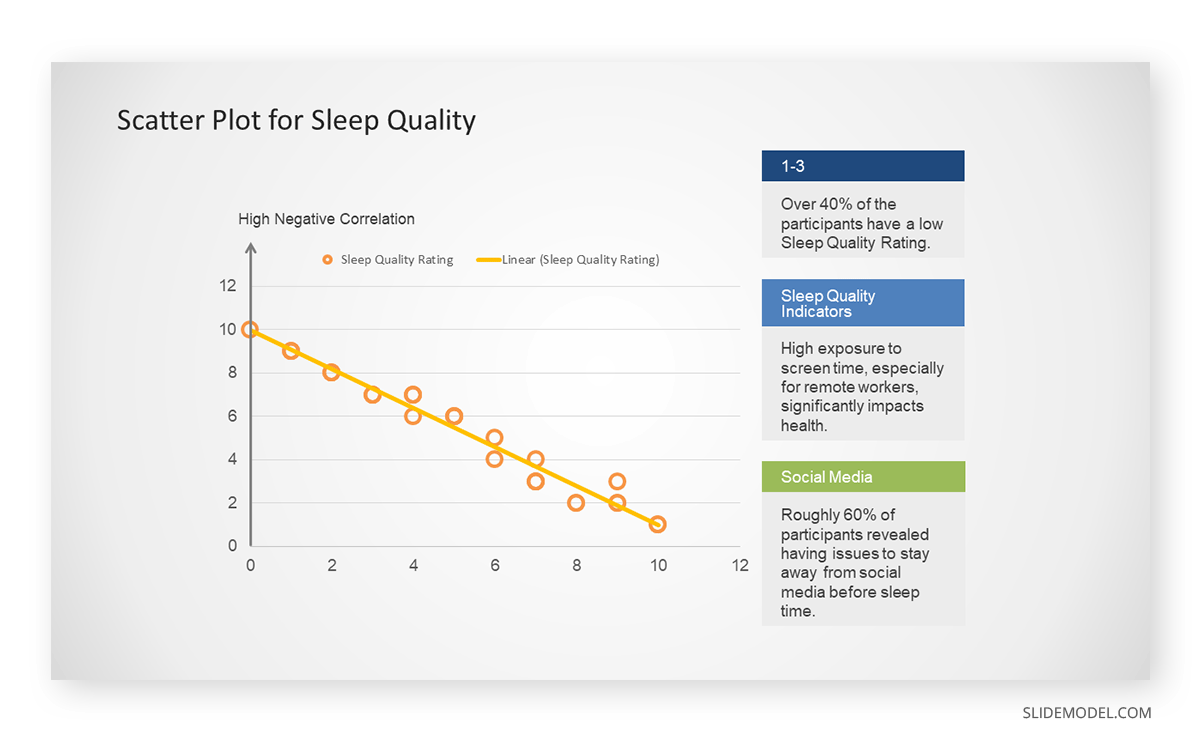
The scientists observe a negative correlation between the amount of screen time and the quality of sleep. This is consistent with their hypothesis that blue light, especially before bedtime, has a significant impact on sleep quality and metabolic processes.
There are a few things to remember when using a scatter plot. Even when a scatter diagram indicates a relationship, it doesn’t mean one variable affects the other. A third factor can influence both variables. The more the plot resembles a straight line, the stronger the relationship is perceived [11] . If it suggests no ties, the observed pattern might be due to random fluctuations in data. When the scatter diagram depicts no correlation, whether the data might be stratified is worth considering.
Choosing the appropriate data presentation type is crucial when making a presentation . Understanding the nature of your data and the message you intend to convey will guide this selection process. For instance, when showcasing quantitative relationships, scatter plots become instrumental in revealing correlations between variables. If the focus is on emphasizing parts of a whole, pie charts offer a concise display of proportions. Histograms, on the other hand, prove valuable for illustrating distributions and frequency patterns.
Bar charts provide a clear visual comparison of different categories. Likewise, line charts excel in showcasing trends over time, while tables are ideal for detailed data examination. Starting a presentation on data presentation types involves evaluating the specific information you want to communicate and selecting the format that aligns with your message. This ensures clarity and resonance with your audience from the beginning of your presentation.
1. Fact Sheet Dashboard for Data Presentation
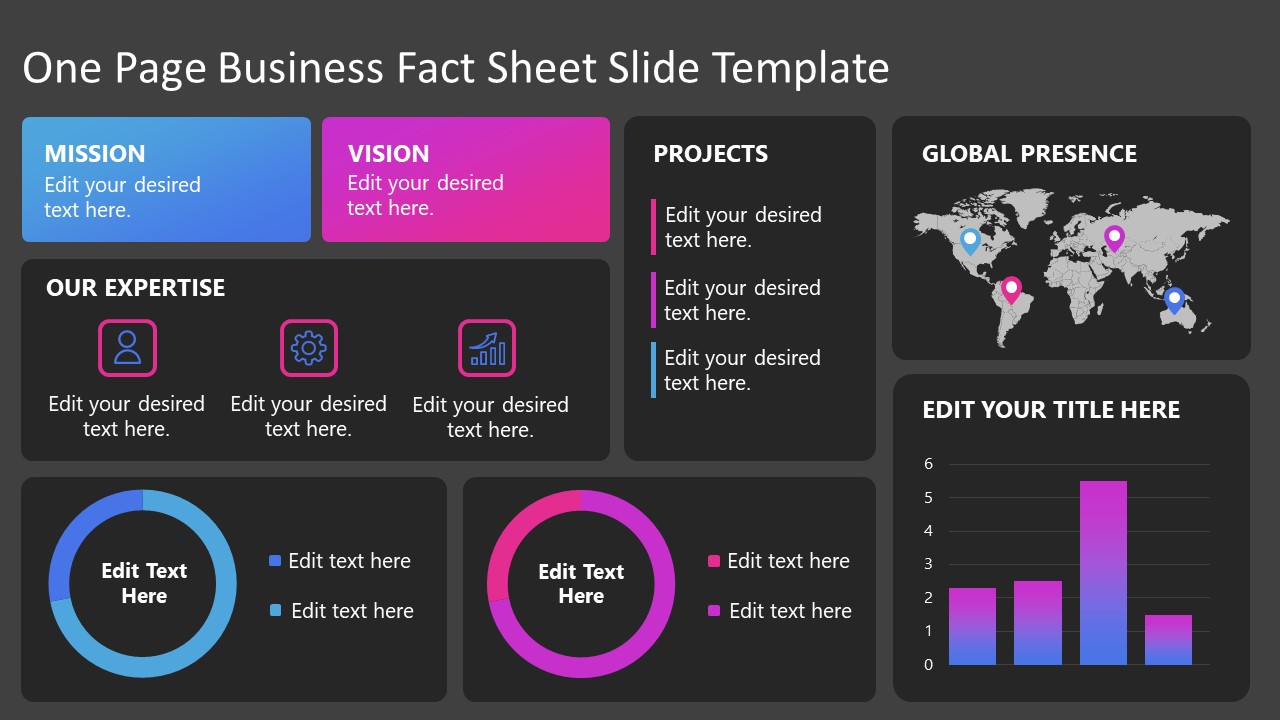
Convey all the data you need to present in this one-pager format, an ideal solution tailored for users looking for presentation aids. Global maps, donut chats, column graphs, and text neatly arranged in a clean layout presented in light and dark themes.
Use This Template
2. 3D Column Chart Infographic PPT Template
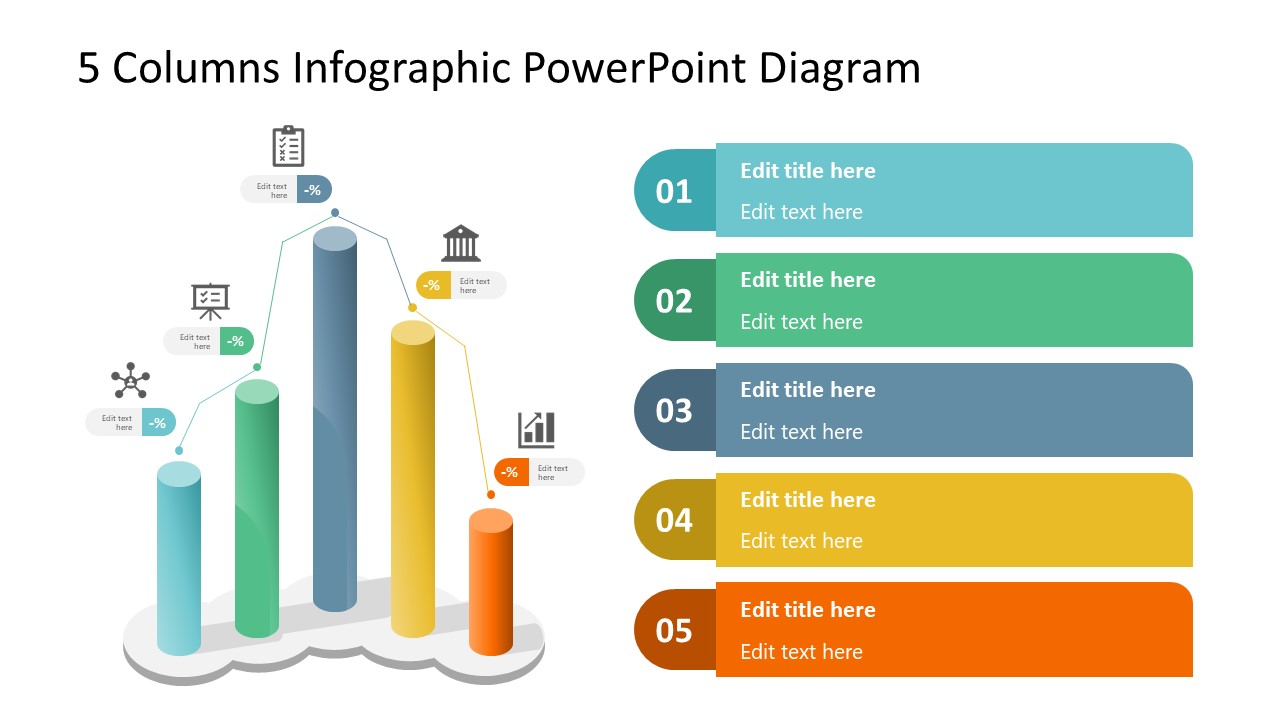
Represent column charts in a highly visual 3D format with this PPT template. A creative way to present data, this template is entirely editable, and we can craft either a one-page infographic or a series of slides explaining what we intend to disclose point by point.
3. Data Circles Infographic PowerPoint Template
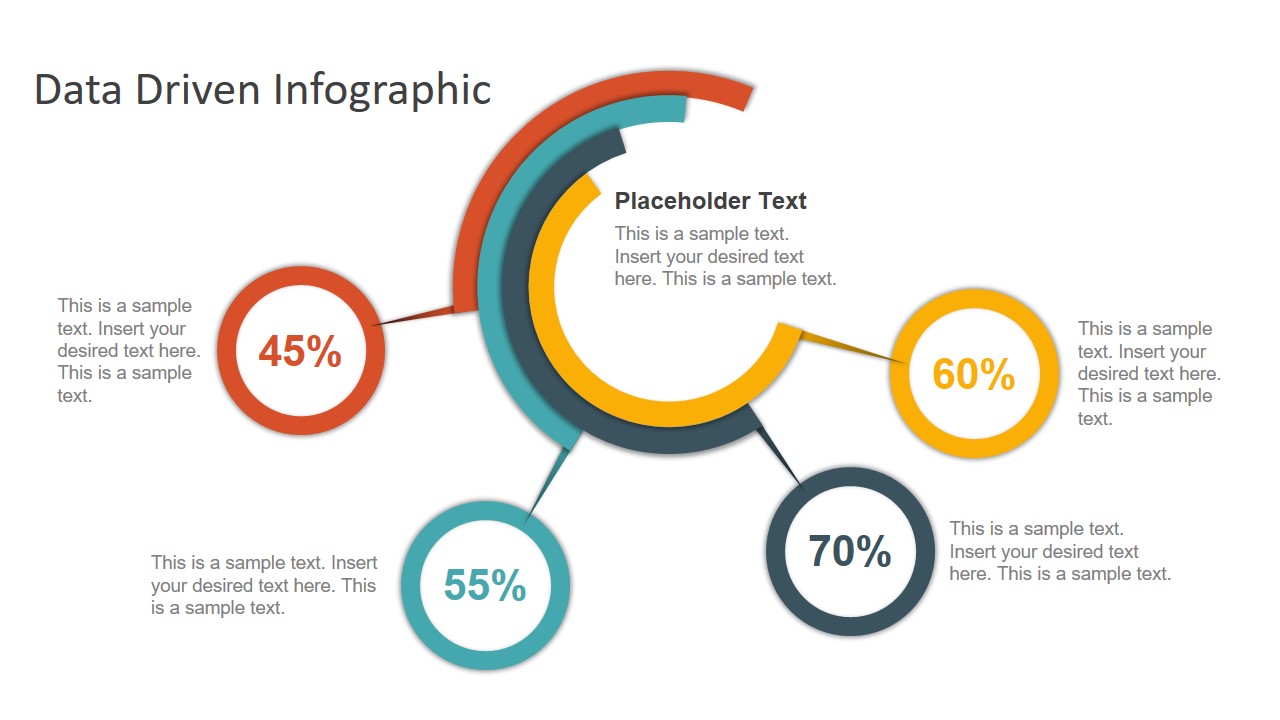
An alternative to the pie chart and donut chart diagrams, this template features a series of curved shapes with bubble callouts as ways of presenting data. Expand the information for each arch in the text placeholder areas.
4. Colorful Metrics Dashboard for Data Presentation
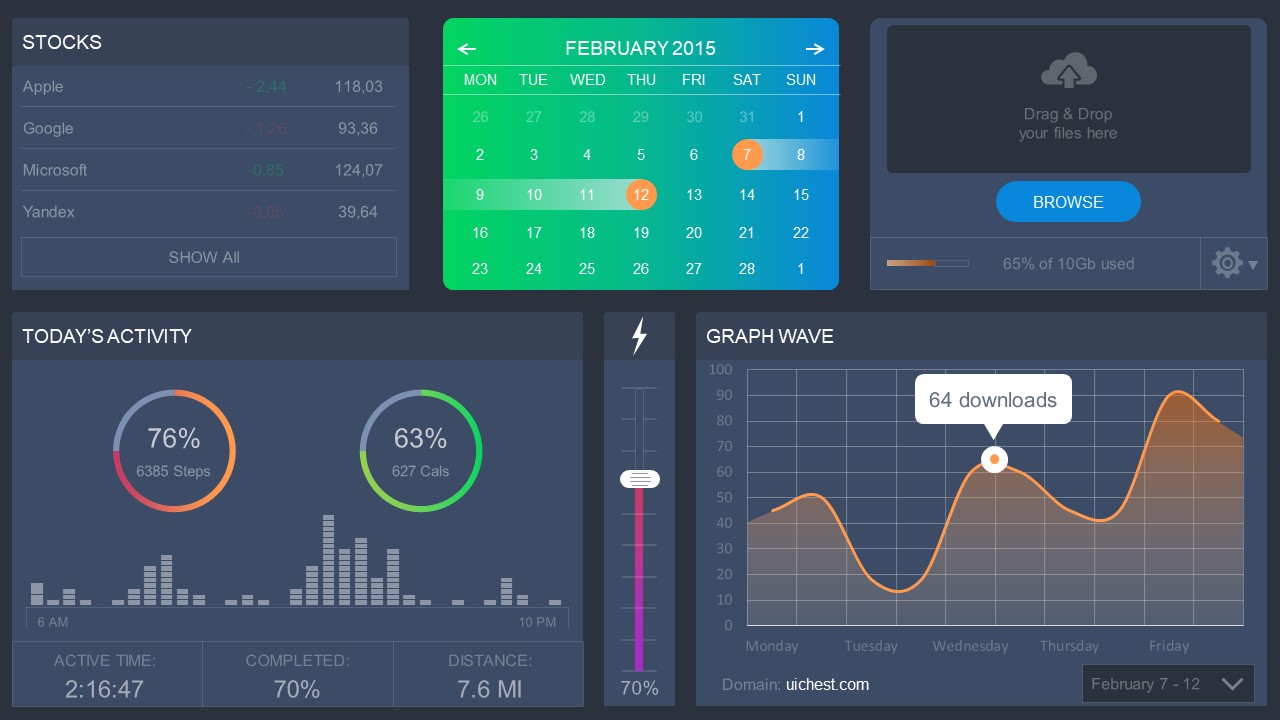
This versatile dashboard template helps us in the presentation of the data by offering several graphs and methods to convert numbers into graphics. Implement it for e-commerce projects, financial projections, project development, and more.
5. Animated Data Presentation Tools for PowerPoint & Google Slides
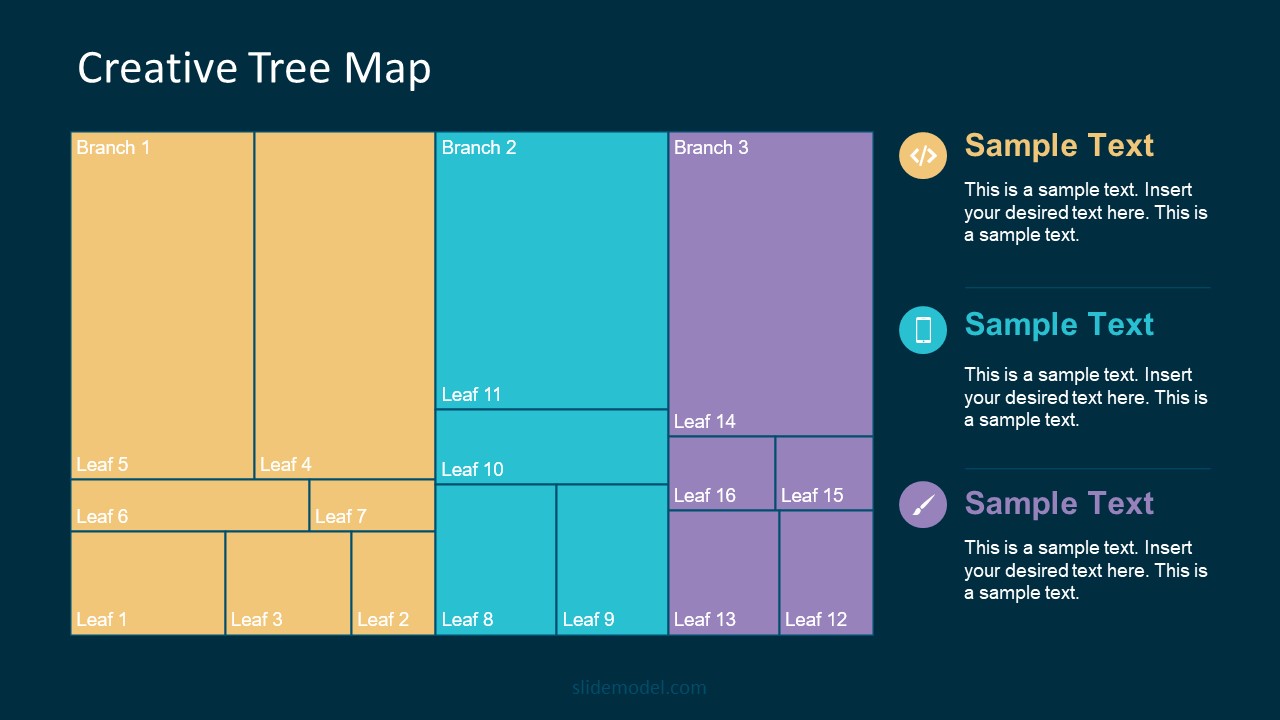
A slide deck filled with most of the tools mentioned in this article, from bar charts, column charts, treemap graphs, pie charts, histogram, etc. Animated effects make each slide look dynamic when sharing data with stakeholders.
6. Statistics Waffle Charts PPT Template for Data Presentations
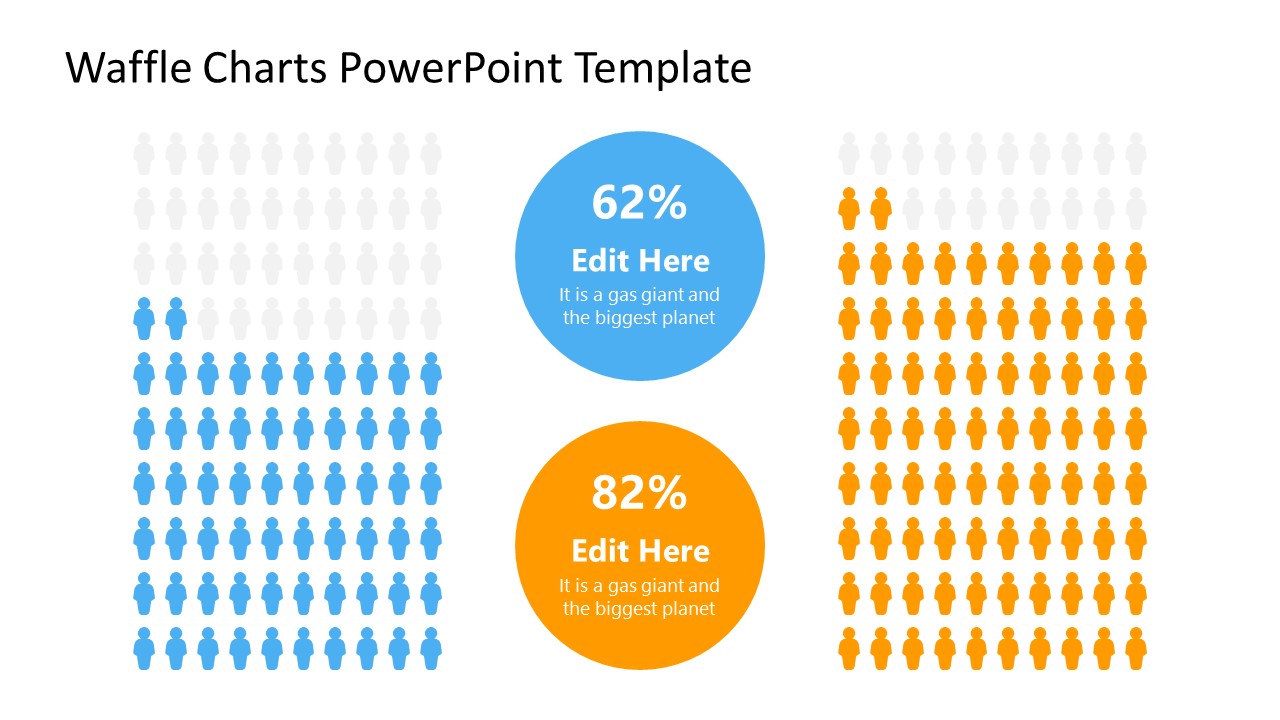
This PPT template helps us how to present data beyond the typical pie chart representation. It is widely used for demographics, so it’s a great fit for marketing teams, data science professionals, HR personnel, and more.
7. Data Presentation Dashboard Template for Google Slides
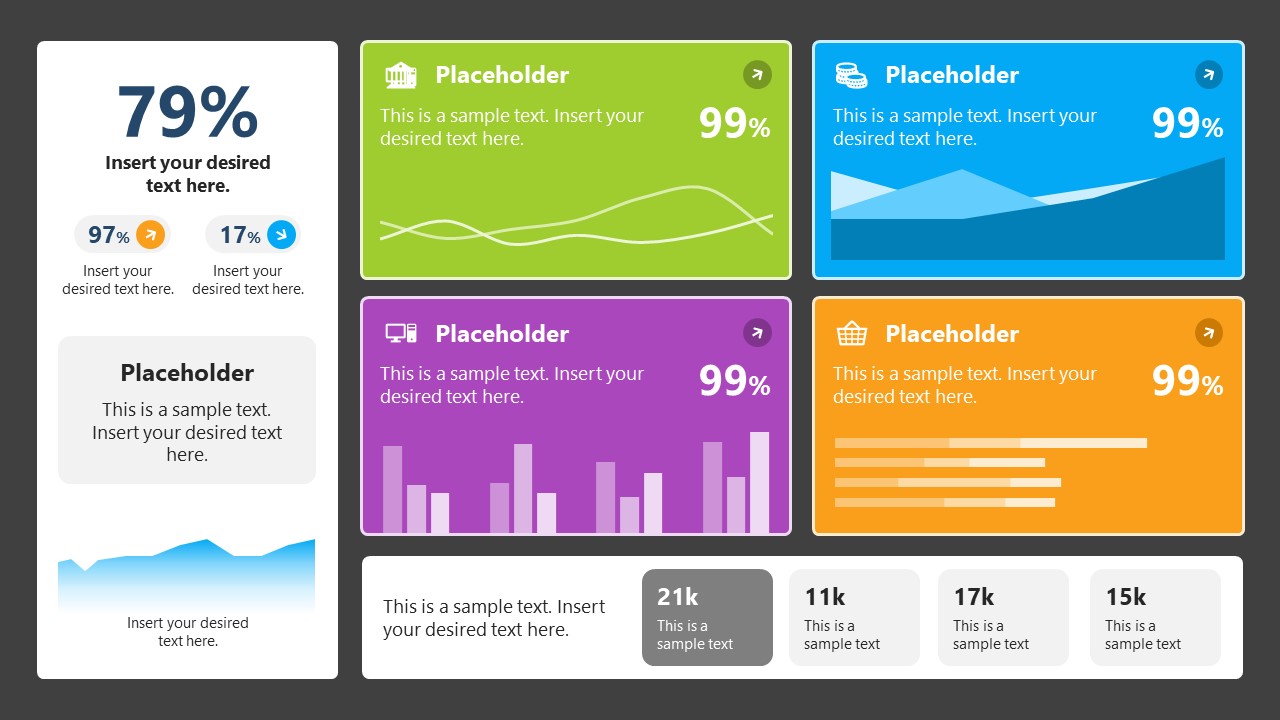
A compendium of tools in dashboard format featuring line graphs, bar charts, column charts, and neatly arranged placeholder text areas.
8. Weather Dashboard for Data Presentation
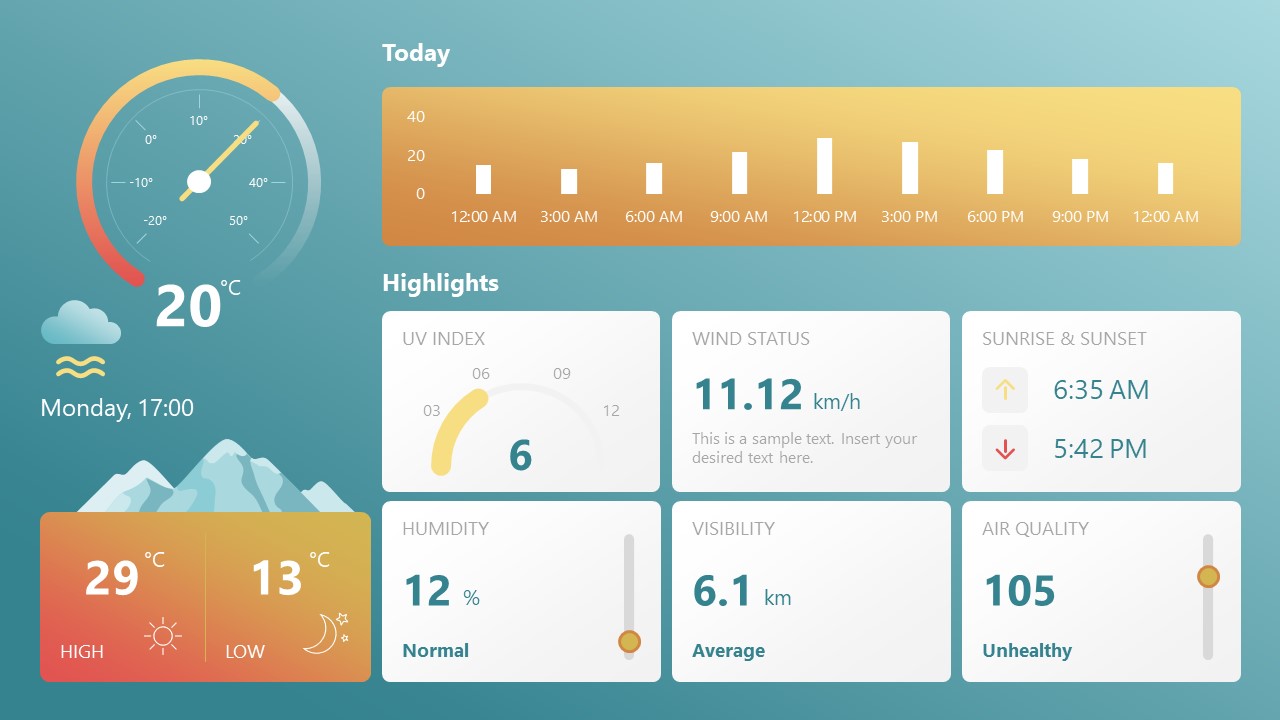
Share weather data for agricultural presentation topics, environmental studies, or any kind of presentation that requires a highly visual layout for weather forecasting on a single day. Two color themes are available.
9. Social Media Marketing Dashboard Data Presentation Template
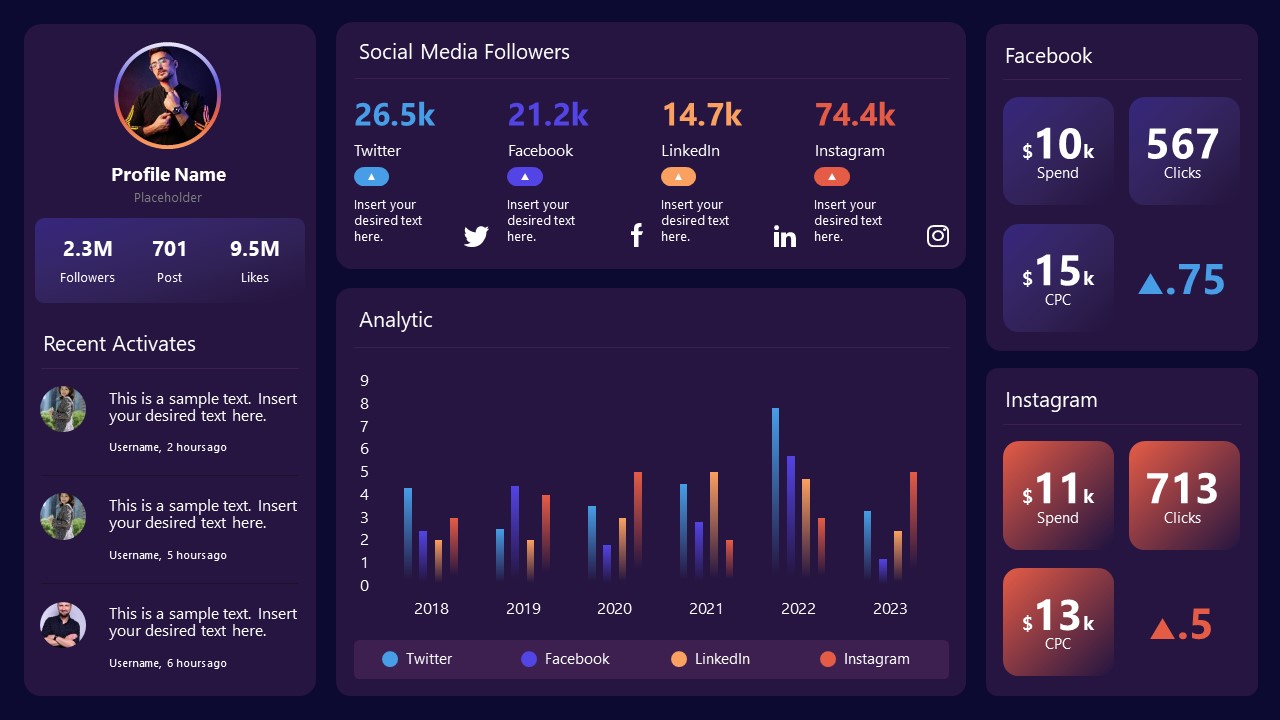
Intended for marketing professionals, this dashboard template for data presentation is a tool for presenting data analytics from social media channels. Two slide layouts featuring line graphs and column charts.
10. Project Management Summary Dashboard Template
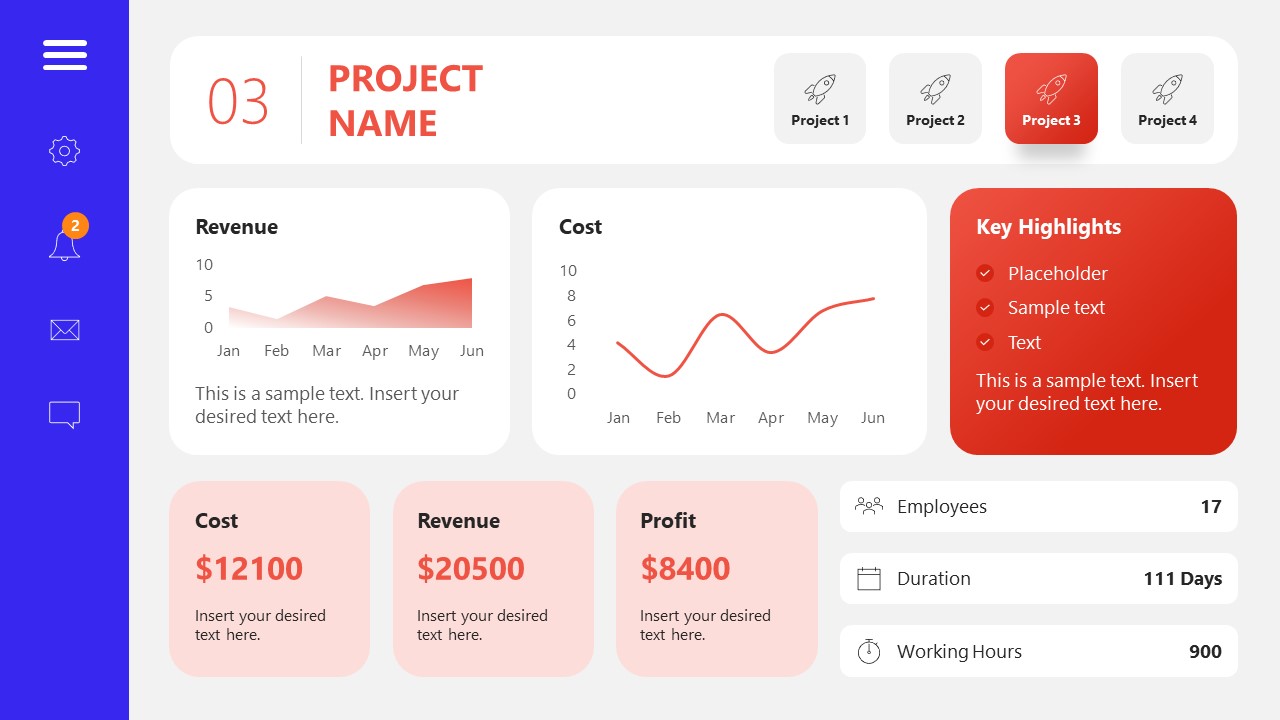
A tool crafted for project managers to deliver highly visual reports on a project’s completion, the profits it delivered for the company, and expenses/time required to execute it. 4 different color layouts are available.
11. Profit & Loss Dashboard for PowerPoint and Google Slides
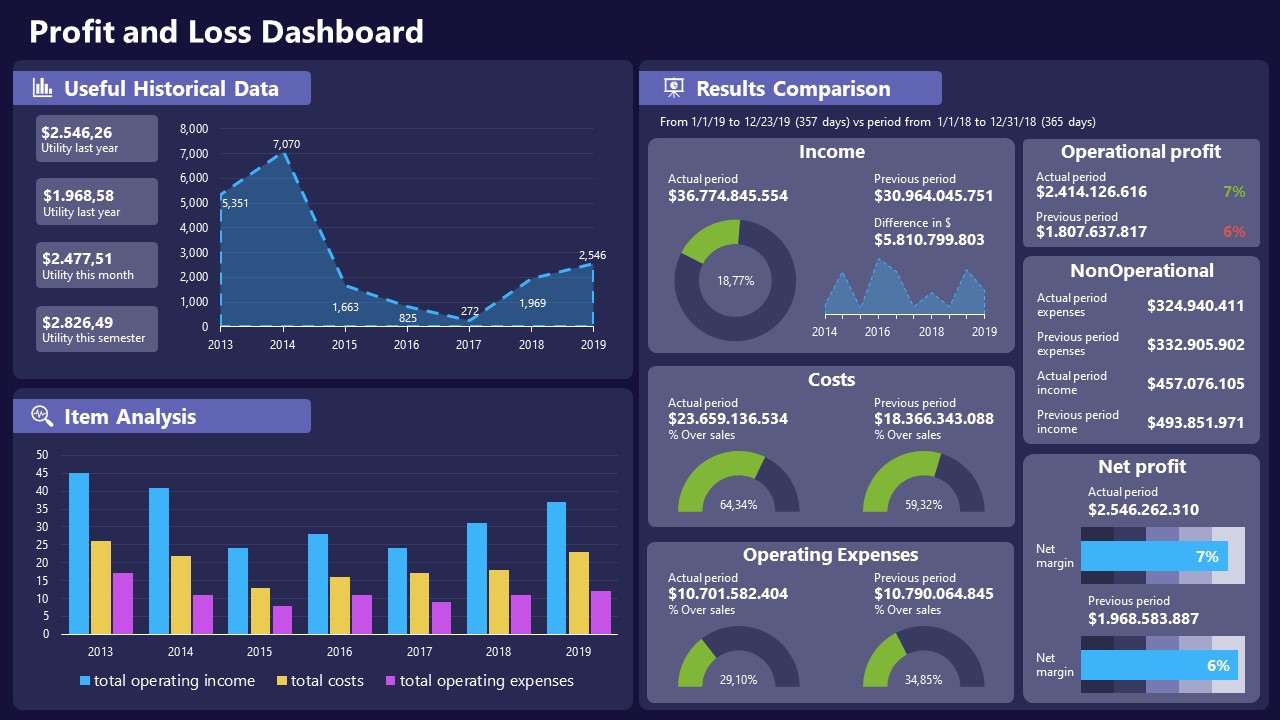
A must-have for finance professionals. This typical profit & loss dashboard includes progress bars, donut charts, column charts, line graphs, and everything that’s required to deliver a comprehensive report about a company’s financial situation.
Overwhelming visuals
One of the mistakes related to using data-presenting methods is including too much data or using overly complex visualizations. They can confuse the audience and dilute the key message.
Inappropriate chart types
Choosing the wrong type of chart for the data at hand can lead to misinterpretation. For example, using a pie chart for data that doesn’t represent parts of a whole is not right.
Lack of context
Failing to provide context or sufficient labeling can make it challenging for the audience to understand the significance of the presented data.
Inconsistency in design
Using inconsistent design elements and color schemes across different visualizations can create confusion and visual disarray.
Failure to provide details
Simply presenting raw data without offering clear insights or takeaways can leave the audience without a meaningful conclusion.
Lack of focus
Not having a clear focus on the key message or main takeaway can result in a presentation that lacks a central theme.
Visual accessibility issues
Overlooking the visual accessibility of charts and graphs can exclude certain audience members who may have difficulty interpreting visual information.
In order to avoid these mistakes in data presentation, presenters can benefit from using presentation templates . These templates provide a structured framework. They ensure consistency, clarity, and an aesthetically pleasing design, enhancing data communication’s overall impact.
Understanding and choosing data presentation types are pivotal in effective communication. Each method serves a unique purpose, so selecting the appropriate one depends on the nature of the data and the message to be conveyed. The diverse array of presentation types offers versatility in visually representing information, from bar charts showing values to pie charts illustrating proportions.
Using the proper method enhances clarity, engages the audience, and ensures that data sets are not just presented but comprehensively understood. By appreciating the strengths and limitations of different presentation types, communicators can tailor their approach to convey information accurately, developing a deeper connection between data and audience understanding.
[1] Government of Canada, S.C. (2021) 5 Data Visualization 5.2 Bar Chart , 5.2 Bar chart . https://www150.statcan.gc.ca/n1/edu/power-pouvoir/ch9/bargraph-diagrammeabarres/5214818-eng.htm
[2] Kosslyn, S.M., 1989. Understanding charts and graphs. Applied cognitive psychology, 3(3), pp.185-225. https://apps.dtic.mil/sti/pdfs/ADA183409.pdf
[3] Creating a Dashboard . https://it.tufts.edu/book/export/html/1870
[4] https://www.goldenwestcollege.edu/research/data-and-more/data-dashboards/index.html
[5] https://www.mit.edu/course/21/21.guide/grf-line.htm
[6] Jadeja, M. and Shah, K., 2015, January. Tree-Map: A Visualization Tool for Large Data. In GSB@ SIGIR (pp. 9-13). https://ceur-ws.org/Vol-1393/gsb15proceedings.pdf#page=15
[7] Heat Maps and Quilt Plots. https://www.publichealth.columbia.edu/research/population-health-methods/heat-maps-and-quilt-plots
[8] EIU QGIS WORKSHOP. https://www.eiu.edu/qgisworkshop/heatmaps.php
[9] About Pie Charts. https://www.mit.edu/~mbarker/formula1/f1help/11-ch-c8.htm
[10] Histograms. https://sites.utexas.edu/sos/guided/descriptive/numericaldd/descriptiven2/histogram/ [11] https://asq.org/quality-resources/scatter-diagram
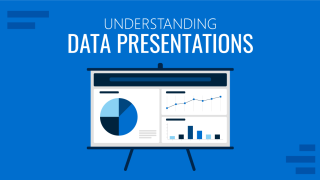
Like this article? Please share
Data Analysis, Data Science, Data Visualization Filed under Design
Related Articles
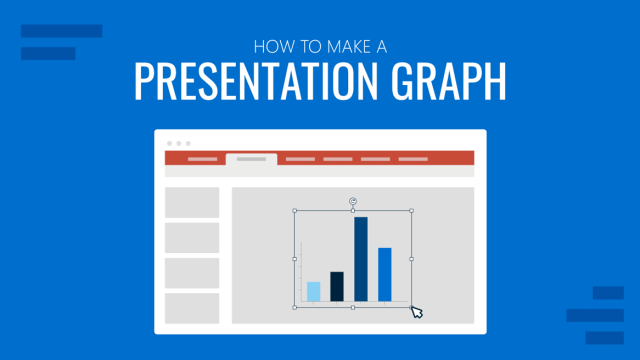
Filed under Design • March 27th, 2024
How to Make a Presentation Graph
Detailed step-by-step instructions to master the art of how to make a presentation graph in PowerPoint and Google Slides. Check it out!
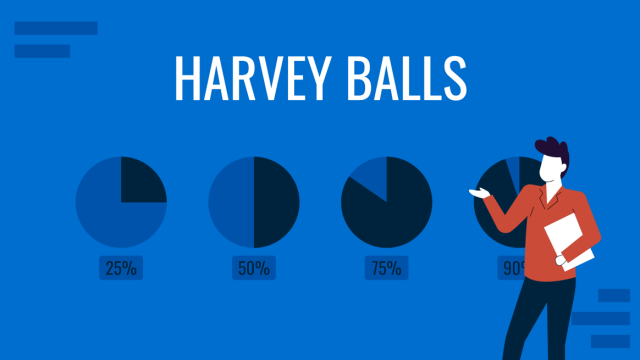
Filed under Presentation Ideas • January 6th, 2024
All About Using Harvey Balls
Among the many tools in the arsenal of the modern presenter, Harvey Balls have a special place. In this article we will tell you all about using Harvey Balls.
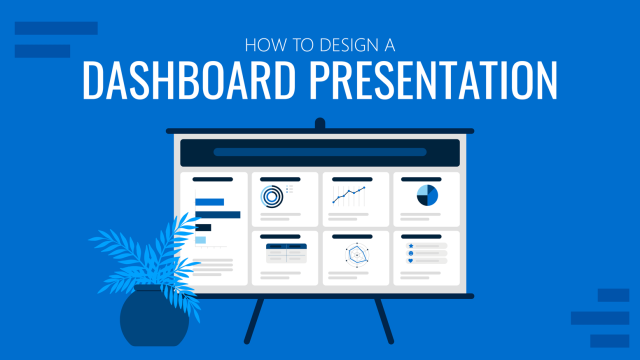
Filed under Business • December 8th, 2023
How to Design a Dashboard Presentation: A Step-by-Step Guide
Take a step further in your professional presentation skills by learning what a dashboard presentation is and how to properly design one in PowerPoint. A detailed step-by-step guide is here!
Leave a Reply
Click through the PLOS taxonomy to find articles in your field.
For more information about PLOS Subject Areas, click here .
Loading metrics
Open Access
Peer-reviewed
Research Article
Poor statistical reporting, inadequate data presentation and spin persist despite editorial advice
Roles Data curation, Formal analysis, Investigation, Methodology, Project administration, Resources, Software, Visualization, Writing – original draft, Writing – review & editing
Affiliations Sydney Medical School, University of Sydney, Sydney, NSW, Australia, Neuroscience Research Australia (NeuRA), Randwick, NSW, Australia

Roles Investigation, Methodology, Project administration, Writing – review & editing
Affiliations Neuroscience Research Australia (NeuRA), Randwick, NSW, Australia, University of New South Wales, Randwick, NSW, Australia
Roles Funding acquisition, Investigation, Methodology, Project administration, Writing – review & editing
* E-mail: [email protected]
Roles Conceptualization, Formal analysis, Investigation, Methodology, Project administration, Resources, Software, Visualization, Writing – review & editing
- Joanna Diong,
- Annie A. Butler,
- Simon C. Gandevia,
- Martin E. Héroux
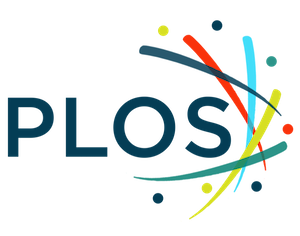
- Published: August 15, 2018
- https://doi.org/10.1371/journal.pone.0202121
- Reader Comments
The Journal of Physiology and British Journal of Pharmacology jointly published an editorial series in 2011 to improve standards in statistical reporting and data analysis. It is not known whether reporting practices changed in response to the editorial advice. We conducted a cross-sectional analysis of reporting practices in a random sample of research papers published in these journals before (n = 202) and after (n = 199) publication of the editorial advice. Descriptive data are presented. There was no evidence that reporting practices improved following publication of the editorial advice. Overall, 76-84% of papers with written measures that summarized data variability used standard errors of the mean, and 90-96% of papers did not report exact p-values for primary analyses and post-hoc tests. 76-84% of papers that plotted measures to summarize data variability used standard errors of the mean, and only 2-4% of papers plotted raw data used to calculate variability. Of papers that reported p-values between 0.05 and 0.1, 56-63% interpreted these as trends or statistically significant. Implied or gross spin was noted incidentally in papers before (n = 10) and after (n = 9) the editorial advice was published. Overall, poor statistical reporting, inadequate data presentation and spin were present before and after the editorial advice was published. While the scientific community continues to implement strategies for improving reporting practices, our results indicate stronger incentives or enforcements are needed.
Citation: Diong J, Butler AA, Gandevia SC, Héroux ME (2018) Poor statistical reporting, inadequate data presentation and spin persist despite editorial advice. PLoS ONE 13(8): e0202121. https://doi.org/10.1371/journal.pone.0202121
Editor: Bart O. Williams, Van Andel Institute, UNITED STATES
Received: April 28, 2018; Accepted: July 27, 2018; Published: August 15, 2018
Copyright: © 2018 Diong et al. This is an open access article distributed under the terms of the Creative Commons Attribution License , which permits unrestricted use, distribution, and reproduction in any medium, provided the original author and source are credited.
Data Availability: All relevant data are within the paper and its Supporting Information files.
Funding: This work is supported by the National Health and Medical Research Council ( https://www.nhmrc.gov.au/ ), APP1055084. The funders had no role in study design, data collection and analysis, decision to publish, or preparation of the manuscript.
Competing interests: The authors have declared that no competing interests exist.
Introduction
The accurate communication of scientific discovery depends on transparent reporting of methods and results. Specifically, information on data variability and results of statistical analyses are required to make accurate inferences.
The quality of statistical reporting and data presentation in scientific papers is generally poor. For example, one third of clinical trials in molecular drug interventions and breast cancer selectively report outcomes [ 1 ], 60-95% of biomedical research papers report statistical analyses that are not pre-specified or are different to published analysis plans [ 2 ], and one third of all graphs published in the prestigious Journal of the American Medical Association cannot be interpreted unambiguously [ 3 ]. In addition, reported results may differ from the actual statistical results. For example, distorted interpretation of statistically non-siginficant results (i.e. spin) is present in more than 40% of clinical trial reports [ 4 ].
Many reporting guidelines (e.g. the Consolidated Standards of Reporting Trials; CONSORT [ 5 ]) have been developed, endorsed and mandated by key journals to improve the quality of research reporting. Furthermore, journals have published editorial advice to advocate better reporting standards [ 6 – 9 ]. Nevertheless, it is arguable whether reporting standards have improved substantially [ 10 – 12 ].
In response to the poor quality of statistical reporting and data presentation in physiology and pharmacology, the Journal of Physiology published an editorial series to provide authors with clear, non-technical guidance on best-practice standards for data analysis, data presentation and reporting of results. Co-authored by the Journal of Physiology’s senior statistics editor and a medical statistician, the editorial series by Drummond and Vowler was jointly published in 2011 under a non-exclusive licence in the Journal of Physiology, the British Journal of Pharmacology, as well as several other journals. (The editorial series was simultaneously published, completely or in part, in Experimental Physiology, Advances in Physiology Education, Microcirculation, the British Journal of Nutrition, and Clinical and Experimental Pharmacology and Physiology.) The key recommendations by Drummond and Vowler include instructions to (1) report variability of continuous outcomes using standard deviations instead of standard errors of the mean, (2) report exact p-values for primary analyses and post-hoc tests, and (3) plot raw data used to calculate variability [ 13 – 15 ]. These recommendations were made so authors would implement them in future research reports. However, it is not known whether reporting practices in these journals have improved since the publication of this editorial advice.
We conducted a cross-sectional analysis of research papers published in the Journal of Physiology and the British Journal of Pharmacology to assess reporting practices. Specifically, we assessed statistical reporting, data presentation and spin in a random sample of papers published in the four years before and four years after the editorial advice by Drummond and Vowler was published.
Materials and methods
Pubmed search and eligibility criteria.
All papers published in the Journal of Physiology and the British Journal of Pharmacology in the years 2007-2010 and 2012-2015 and indexed on PubMed were extracted using the search strategy: (J Physiol[TA] OR Br J Pharmacol[TA]) AND yyyy: yyyy[DP] NOT (editorial OR review OR erratum OR comment OR rebuttal OR crosstalk). Papers were excluded if they were editorials, reviews, erratums, comments, rebuttals, or part of the Journal of Physiology’s Crosstalk correspondence series. Of the eligible papers, a random sample of papers published in the four years before the 2011 editorial advice by Drummond and Vowler was published (2007-2010) and four years after (2012-2015) was extracted ( S1 File ), and full-text PDFs were obtained.
Question development and pilot testing
Ten questions and scoring criteria were developed to assess statistical reporting, data presentation and spin in the text and figures of the extracted papers. Questions assessing statistical reporting in the text (Q1-5) determined if and how written measures that summarize variability were defined, and if exact p-values were reported for primary analyses and post-hoc tests. Questions assessing data presentation in figures (Q6-8) determined if and how plotted measures that summarize variability were defined, and if raw data used to calculate the variability were plotted. Questions assessing the presence of spin (Q9-10) determined if p-values between 0.05 and 0.1 were interpreted as trends or statistically significant.
A random sample of 20 papers before and 20 papers after the editorial advice was used to assess the clarity of the scoring instructions and scoring agreement between raters. These papers were separate from those included in the full audit. All papers were independently scored by three raters (AAB, JD, MEH). Scores that differed between raters were discussed to reach agreement by consensus. The wording of the questions, scoring criteria, and scoring instructions were refined to avoid different interpretations by raters. The questions are shown in Fig 1 . Scoring criteria and additional details of the questions are provided in the scoring information sheets in the supporting information ( S2 File ).
- PPT PowerPoint slide
- PNG larger image
- TIFF original image
Counts and proportions of papers that fulfilled scoring criteria for each question before (white) and after (gray) the editorial advice was published. Abbreviations are SEM: standard error of the mean, ANOVA: analysis of variance.
https://doi.org/10.1371/journal.pone.0202121.g001
Data collection, outcomes and analysis
Each rater (AAB, JD, MEH, SCG) had to score and extract data independently from 50 papers before and 50 papers after the editorial advice was published. For each rater, papers from the 2007-2010 and 2012-2015 periods were audited in an alternating pattern to avoid an order or block effect. One rater unintentionally audited an additional 2007-2010 paper, and another rater unintentionally audited a 2007-2010 paper instead of a 2012-2015 paper. Thus, data from a random sample of 202 papers before and 199 papers after the editorial advice were analysed. When scoring was completed, papers that were difficult or ambiguous to score (less than 5% of all papers) were reviewed by all raters and scoring determined by consensus.
It was difficult to score some papers unambiguously on some of the scoring criteria. For example in question 3, it was sometimes difficult to determine what a paper’s primary analyses, main effects and interactions were, in order to determine whether p-values for these were reported or implied. When raters could not unambiguously interpret the data, either individually or as a team, we scored papers to give authors the benefit of doubt.
Counts and proportions of papers that fulfilled the scoring criteria for each question were calculated; no statistical tests were performed. Descriptive data are reported. All data processing and analysis were performed using Python (v3.5). Raw data, computer analysis code and result are available in the supporting information ( S3 File ).
The random sample of audited papers was reasonably representative of the number of papers published each year in the Journal of Physiology and the British Journal of Pharmacology in the two periods of interest ( Table 1 ).
https://doi.org/10.1371/journal.pone.0202121.t001
The proportions of audited papers that fulfilled the scoring criteria are presented in Fig 1 . The figure shows there is no substantial difference in statistical reporting, data presentation or the presence of spin after the editorial advice was published. Overall, 76-84% of papers with written measures that summarized data variability used standard errors of the mean, and 90-96% of papers did not report exact p-values for primary analyses and post-hoc tests. 76-84% of papers that plotted measures to summarize variability used standard errors of the mean, and only 2-4% of papers plotted raw data used to calculate variability.
Of papers that reported p-values between 0.05 and 0.1, 56-63% interpreted such p-values as trends or statistically significant. Examples of such interpretations include:
- “A P < 0.05 level of significance was used for all analyses. […] As a result, further increases in the tidal P oes /VT (by 2.64 and 1.41 cmH 2 O l −1 , P = 0.041) and effort–displacement ratios (by 0.22 and 0.13 units, P = 0.060) were consistently greater during exercise …” (PMID 18687714)
- “The level of IL-15 mRNA tended to be lower in vastus lateralis than in triceps (P = 0.07) ( Fig 1A )” (PMID 17690139)
- “… where P < 0.05 indicates statistical significance […] was found to be slightly smaller than that of basal cells (−181 ± 21 pA, n = 7, P27–P30) but the difference was not quite significant (P = 0.05)” (PMID 18174213)
- “… resting activity of A5 neurons was marginally but not significantly higher in toxin-treated rats (0.9 ± 0.2vs. 1.8 ± 0.5 Hz, P = 0.068)” (PMID 22526887)
- “… significantly smaller than with fura-6F alone (P = 0.009), and slightly smaller than with fura-6F and EGTA (P = 0.08).” (PMID 18832426)
- “The correlation becomes only marginally significant if the single experiment with the largest effect is removed (r = 0.41, P = 0.057, n = 22).” (PMID 17916607)
Implied or gross spin (i.e. spin other than interpreting p-values between 0.05 and 0.1 as trends or statistically significant) was noted incidentally in papers before (n = 10) and after (n = 9) the editorial advice was published. Examples of statements where implied or gross spin was present include:
- “However, analysis of large spontaneous events (>50 pA in four of six cells) ( Fig 1G–1I ) showed the frequency to be increased from 0.4 ± 0.1 Hz to 0.8 ± 0.2 Hz (P < 0.05) ( Fig 1G and 1H ) and the amplitude by 20.3 ± 15.6 pA (P > 0.1) …” (PMID 24081159)
- “… whereas there was only a non-significant trend in the older group.” (no p-value, PMID 18469848)
Post-hoc analyses revealed audit results were comparable between raters ( S4 File ). Additional post-hoc analyses revealed audit results were relatively consistent across years and journals ( S5 File ). A notable exception was the British Journal of Pharmacology and its lower rate of reporting p-values (3-27% lower; question 3) and exact p-values for main analyses (8-22% lower; question 4).
In 2011 the Journal of Physiology and the British Journal of Pharmacology jointly published editorial advice on best practice standards for statistical reporting and data presentation [ 13 ]. These recommendations were reiterated in the Journals’ Instructions to Authors. Our cross-sectional analysis shows there was no substantial improvement in statistical reporting and data presentation in the four years after publication of this editorial advice.
Our results confirm that the quality of statistical reporting is generally poor. We found that ∼80% of papers that plotted error bars used standard error of the mean. In line with this, a systematic review of 703 papers published in key physiology journals revealed 77% of papers plotted bar graphs with standard error of the mean [ 16 ]. Similarly, one of the authors (MH) audited all 2015 papers published in the Journal of Neurophysiology and found that in papers with error bars, 65% used standard error of the mean and ∼13% did not define their error bars. That audit also revealed ∼42% of papers did not report exact p-values and ∼57% of papers with p-values between 0.05 and 0.1 interpreted these p-values as trends or statistically significant [ 12 ]. Our current study found that ∼93% of papers included non-exact p-values and ∼60% of papers with p-values between 0.05 and 0.1 reported these with spin. It is unfortunate that authors adopt practices that distort the interpretation of results and mislead readers into viewing results more favorably. This problem was recently highlighted by a systematic review on the prevalence of spin in the biomedical literature [ 17 ]. Spin was present in 35% of randomized control trials with significant primary outcomes, 60% of randomized controls with non-significant primary outcomes, 84% of non-randomized trials and 86% of observational studies. Overall, these results highlight the sheer magnitude of the problem: poor statistical reporting and questionable interpretation of results are truly common practice for many scientists.
Our findings also broadly agree with other observational data on the ineffectiveness of statistical reporting guidelines in biomedical and clinical research. For example, the CONSORT guidelines for the reporting of randomized controlled trials are widely supported and mandated by key medical journals, but the quality of statistical reporting and data presentation in randomized trial reports remains inadequate [ 18 – 20 ]. A scoping audit of papers published by American Physiological Society journals in 1996 showed most papers mistakenly reported standard errors of the mean as estimates of variability, not as estimates of uncertainty [ 21 ]. Consequently, in 2004 the Society published editorial guidelines to improve statistical reporting practices [ 22 ]. These guidelines instructed authors to report variability using standard deviations, and report uncertainty about scientific importance using confidence intervals. However, the authors of the guidelines audited papers published before and after their implementation and found no improvement in the proportion of papers reporting standard errors of the mean, standard deviations, confidence intervals, and exact p-values [ 10 ]. Likewise, in 1999 and 2001 the American Psychological Association published guidelines instructing authors to report effect sizes and confidence intervals [ 23 , 24 ]. Once again, an audit of papers published before and after the guidelines were implemented found no improvement in the proportion of figures with error bars defined as standard errors of the mean (43-59%) or worse, with error bars that were not defined (29-34%) [ 25 ].
One example where editorial instructions improved reporting practices occurred in public health. In the mid-80’s the American Journal of Public Health had an influential editor who advocated and enforced the use of confidence intervals rather than p-values. An audit of papers published before and during the tenure of this editor found that the reliance on p-values to interpret findings dropped from 63% to 5% and the reporting of confidence intervals increased from 10% to 54% [ 26 ]. However, few authors referred to confidence intervals when interpreting results. In psychology, when editors of Memory & Cognition and the Journal of Consulting and Clinical Psychology enforced the use of confidence intervals and effect sizes, the use of these statistics increased to some extent, even though long-term use was not maintained [ 27 ]. These examples provide evidence that editors with training in statistical intepretation may enforce editorial instructions more successfully, even if author understanding does not necessarily improve.
Why are reporting practices not improving? The pressure to publish may be partly to blame. Statistically significant findings that are visually and numerically clean are easier to publish. Thus, it should come as no surprise that p-values between 0.05 and 0.1 are interpreted as trends or statistically significant, and that researchers use standard errors of the mean to plot and report results. There is also a cultural component to these practices. The process of natural selection ensures that practices associated with higher publication rates are transmitted from one generation of successful researchers to the next [ 28 ]. Unfortunately, some of these practices include poor reporting practices. As was recently highlighted by Goodman [ 29 ], conventions die hard, even if they contribute to irreproducible research. In the article, citing a government report on creating change within a system, Goodman highlights that “culture will trump rules, standards and control strategies every single time”. Thus, researchers will often opt for reporting practices that make their papers look like others in their field, conscious or not that these reporting practices are inadequate and not in line with published reporting guidelines. A final contributing factor is that many researchers continue to misunderstand key statistical concepts, such as measures of variability and uncertainty, inferences made from independent and repeated-measures study designs, and error bars and how they reflect statistical significance [ 30 ]. This partly explains the resistance to statistical innovations and robust reporting practices [ 27 ].
The recent reproducibility crisis has seen all levels of the scientific community implement new strategies to improve how science is conducted and reported. For example, journals have introduced article series to promote awareness [ 9 , 31 ] and adopted more stringent reporting guidelines [ 32 , 33 ]. Whole disciplines have also taken steps to tackle these issues. For example, the Academy of Medical Sciences partnered with the Biotechnology and Biological Sciences Research Council, the Medical Research Council and the Wellcome Trust to host a symposium on improving reproducibility and reliability of biomedical research [ 34 ]. Funding bodies have also acted. For example, the NIH launched training modules to educate investigators on topics such as bias, blinding and experimental design [ 35 ], and the Wellcome Trust published guidelines on research integrity and good research practice [ 36 ]. Other initiatives include the Open Science Framework, which facilitates open collaboration [ 37 ], and the Transparency and Openness Promotion guidelines, which were developed to improve reproducibility in research and have been endorsed by many key journals [ 38 ]. To improve research practices, these initiatives aim to raise awareness of the issues, educate researchers and provide tools to implement the various recommendations. While the enduring success of these initiatives remains to be determined, we remain hopeful for the future. There is considerable momentum throughout science, and many leaders from various disciplines have stepped up to lead the way.
In summary, reporting practices have not improved despite published editorial advice. Journals and other members of the scientific community continue to advocate and implement strategies for change, but these have only had limited success. Stronger incentives, better education and widespread enforcement are needed for enduring improvements in reporting practices to occur.
Supporting information
S1 file. random paper selection..
Python code and PubMed search results used to randomly select papers for the audit. See the included README.txt file for a full description.
https://doi.org/10.1371/journal.pone.0202121.s001
S2 File. Scoring information sheets.
Scoring criteria and details of questions 1-10.
https://doi.org/10.1371/journal.pone.0202121.s002
S3 File. Data and code.
Comma-separated-values (CSV) file of raw scores for questions 1-10 and the Python files used to analyse the data. See the included README.txt file for a full description.
https://doi.org/10.1371/journal.pone.0202121.s003
S4 File. Audit results across raters.
Comparison of audit results across raters indicates the scoring criteria were applied uniformly across raters.
https://doi.org/10.1371/journal.pone.0202121.s004
S5 File. Audit results across years and journals.
Comparison of audit results for each year and journal. The British Journal of Pharmacology consistently had lower reporting rates of p-values for main analyses, and exact p-values for main analyses.
https://doi.org/10.1371/journal.pone.0202121.s005
Acknowledgments
We thank Dr Gordon Drummond for reviewing a draft of the manuscript.
- View Article
- PubMed/NCBI
- Google Scholar
- 24. American Psychological Association. Publication manual of the American Psychological Association. American Psychological Association; 2001. Available from: http://www.apa.org/pubs/books/4200061.aspx .
- 25. Cumming G, Fidler F, Leonard M, Kalinowski P, Christiansen A, Kleinig A, et al. Statistical reform in psychology: is anything changing?; 2007. Available from: https://www.jstor.org/stable/40064724 .
- 31. Nature Special. Challenges in irreproducible research; 2014. Available from: https://www.nature.com/collections/prbfkwmwvz/ .
- 34. The Academy of Medical Sciences. Reproducibility and reliability of biomedical research; 2016. Available from: https://acmedsci.ac.uk/policy/policy-projects/reproducibility-and-reliability-of-biomedical-research .
- 35. National Institutes of Health. Clearinghouse for training modules to enhance data reproducibility; 2014. Available from: https://www.nigms.nih.gov/training/pages/clearinghouse-for-training-modules-to-enhance-data-reproducibility.aspx .
- 36. Wellcome Trust. Research practice; 2005. Available from: https://wellcome.ac.uk/what-we-do/our-work/research-practice .

- Tips & Tricks
- PowerPoint Templates
- Training Programs
- Free E-Courses
5 Small Mistakes To Avoid While Presenting Data
Home > Presentation of Data Page > Presenting Data Mistakes
Small mistakes are like bad breath. Your friends won’t point them out to you and neither can you find them out yourself. Find the small mistakes people make in a data presentation and learn how to avoid them.
Small mistakes in presenting data go unnoticed:
It is easy to correct big mistakes in your presentations, because they are easily noticeable. Small mistakes are difficult to identify, but they irritate the audience. Here are the 5 common mistakes made while presenting data and the ways to solve them.
1. Retaining the small fonts of Excel in PowerPoint slides
Most presenters have the habit of sticking charts directly from excel sheet to PowerPoint. They don’t bother to increase the font size of the numbers (which is usually 10 when you stick a chart from excel sheet).
Want to know how hard it is to read that small font size from a distance?
Try reading the legend on the slide below:
Solution to issue with font size:
Increase the font size of text and numbers in the chart to at least 18 to 20. This is the minimum acceptable font size even in a small conference room.
2. Using images in chart background
Some presentation gurus advise putting pictures in the chart background for building an emotional connection with the audience. Here is the result:
Wow! That definitely strikes an emotional chord. But, where is the graph?
Remember, the fancy presentation methods used for presenting on culture and philosophy don’t work in business presentations. Remove all chart backgrounds. Let your audience understand your chart.
Related: 2 Creative Info graphic Examples
3. Flaunting thoroughness with footnotes
Some presenters boast the thoroughness of their research and the ‘correctness’ of their observation by using slides like this:
The disclaimers and footnotes occupy half the slide in font size 6. This immediately puts a distance between the presenter and the audience.
Solution to complex tables:
- Remove footnotes from your slides. Include them in your handouts if you must
- Don’t display raw data. Write your conclusions on your slides.
- Avoid using large tables on a slide. Table larger than 2X2 is quite difficult to follow on a slide during presentations.
4. Showing lack of sensitivity while writing text and digits
Some presenters don’t care about audience convenience while writing their text on slides. Here is a quick list of small mistakes and their solution:
- Don’t write vertical text on the Y axis or slanting text on the X axis. Your audience can’t read them easily. If you have a lot of text to write on the x- axis of your column chart, use bar chart instead.
- Don’t write large numbers like 56798745.08 on your charts or tables. Express the big numbers in Millions or Thousands. Round off the decimals while using big numbers. Use space between digits for easy reading. Ex: 12 000 or 12,000 is easy to read instead of 12000.
- Use wide intervals for your Y axis if you want to show gridlines. Avoid showing minor gridlines at all cost.
- Order your lists by groups or by alphabetical order for easy reference.
5. Showing lack of preparedness by reading numbers
A lot of presenters lose audience trust because they don’t familiarize themselves with the charts and numbers on their slides. They try to ‘figure out’ their charts along with the audience. They read the numbers from the slide instead of talking about what the numbers mean.
Solution to reading numbers when presenting data:
- Rehearse your charts at least 3 times before presenting them to the audience.
- Practice the custom animations used in your charts.
- Provide insights from the numbers.
Summary of small mistakes to avoid while presenting data:
- Retaining the small fonts of Excel in PowerPoint slides
- Using images in chart background
- Flaunting thoroughness with footnotes
- Showing lack of audience sensitivity while writing text and digits
- Showing lack of preparedness by reading numbers
Avoid these small mistakes and you will succeed in presenting data with purpose.
FRelated: Top 5 Presentation Design Mistakes
Return to Top of Presenting Data Mistakes Page
Share these tips & tutorials
Get 25 creative powerpoint ideas mini course & members-only tips & offers. sign up for free below:.
- Slide Design Training
- Design Services
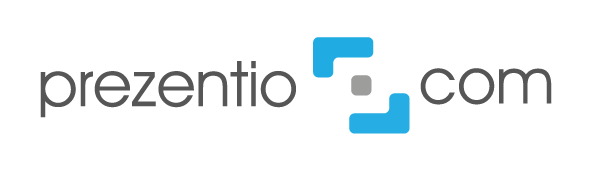
Five Data Chart Mistakes to Avoid in Presentation
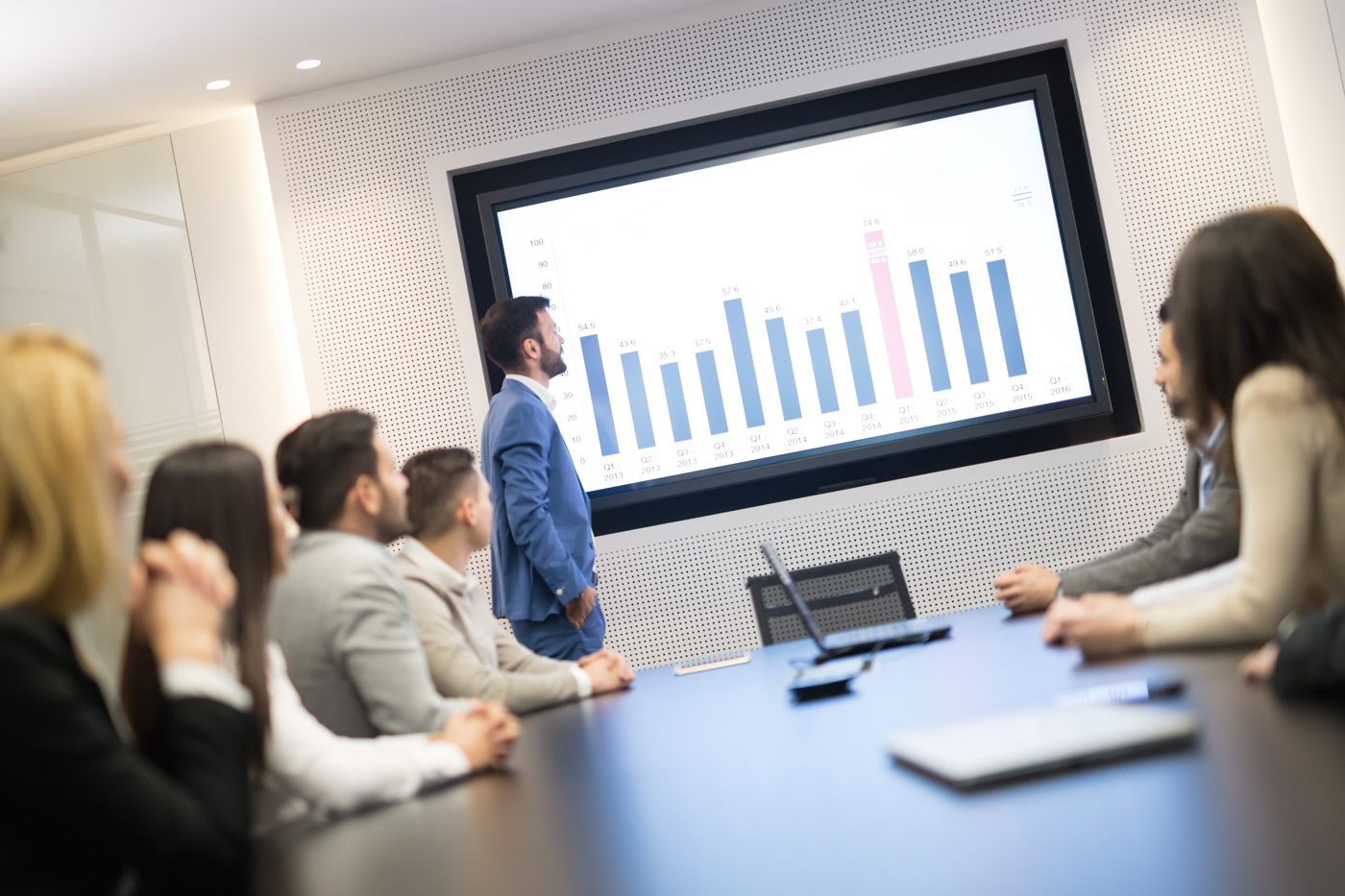
Are you presenting data graphs a lot on your slides? Make sure you are not failing into one of the data visualization traps. Here are the most common mistakes I see people do when they present various Excel charts to PowerPoint.
How to avoid those typical data visualization mistakes? I am sharing a few quick tips how you can handle those mistakes using only PowerPoint tools.
This is a fragment of our training workshops. For more details see our Online Trainings on Presentation Design.
1. Inappropriate data chart
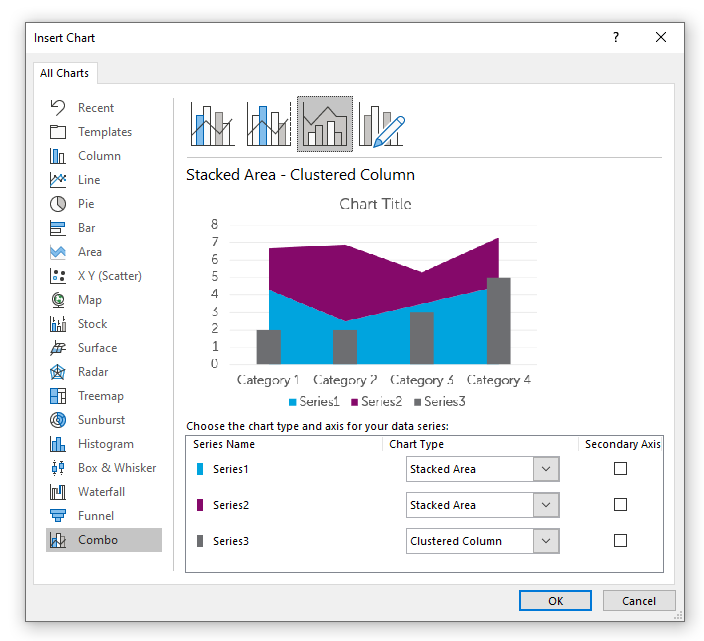
PowerPoint offers a dozen of data charts you can choose from. Recent addition (from Office365) include fancy looking Sunburst, Funnel or finally Waterfall chart.
However for most of presentation purposes you will need to use a few basic charts or their versions: Bar (or column) chart, Pie chart (also doughnut or donut one), and Line chart (or area chart). Each of them fits a different purpose:
Line Charts and Area Charts
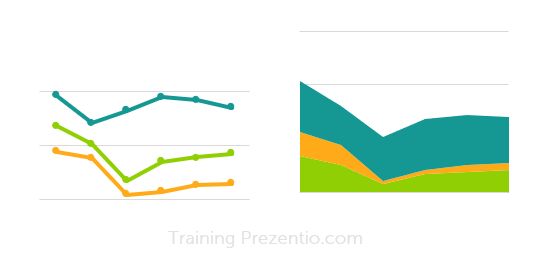
Line chart is best for showing data change over time, For example showing sales trends, website traffic over last days or global warming temperatures over decades.
Mistakes I have seen involve using line charts with categorical Y axes (e.g. a specific product). Another thing to avoid using line chart are showing too many variables on one chart.
Pie and Doughnut Charts
Those are nice looking charts on shape of circle, that are meant to represent data that compose one whole. Typically a market share or any kind of percentage data e.g. a survey answers distribution.
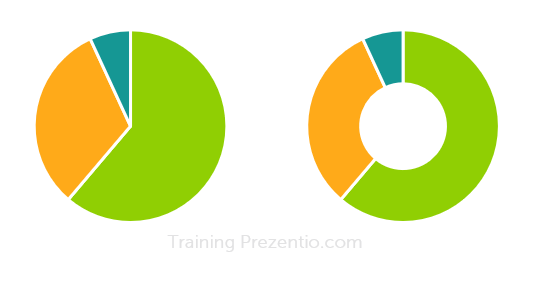
Logical error in a pie chart is when percentage values do not sum up to 100%.
Visual mistakes I see a lot is using pie charts with way to many categories. From design point of view, to keep readability I’d recommended showing max 6 categories in a pie chart. And order them by value from biggest to smallest. If you have more categories, group last 7+ into one category “others”. And if needed, show this category on other chart.
Column and Bar Charts
Those charts represent data value by size of a vertical or horizontal bar. They are suitable to compare multiple categories e.g. sales of various products. Unlike line or pie charts, you can show here also more variables and chart will be still readable.
One thing I would suggest is to use color coding with some business logic behind. Use various colors if you want to underline that variables are different. Apply only 1 color if you want to focus more on values than on variety of categories.
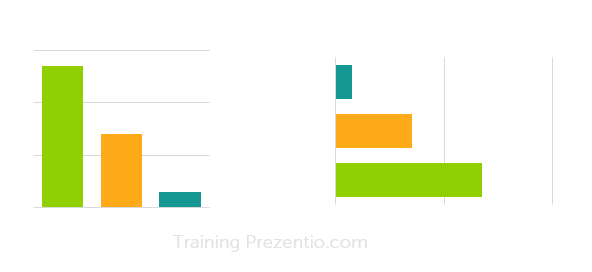
How to choose chart to your data
If the focus of your presentation is to show outcomes in an understandable way, and not to do exploratory data analysis, then it’s better to use those classical data chart. Avoid fancy new chart, that may not be that easy to comprehend by your audience.
Choosing one of those basic data graphs, consider what you want to show:
- Is it a share from some whole part? Then consider Pie or Doughnut chart.
- Is it a trend over a time ? Then Line chart is your obvious choice.
- Or do you compare several items? Then go for Bar chart.
To learn more about data charts I recommend book by Nancy Duarte: Data Story or Say it with a Charts by Gene Zelazny .
2. Unclear reading flow of a data slide
When you are designing a slide with data presentation, indicate clearly how the reader should look at it, where to start and where to follow.
Is the chart title the first thing a reader should look at? Or is it a trend line? A specific data value or outlier you want to highlight? Don’t let reader guess what’s the most important part.
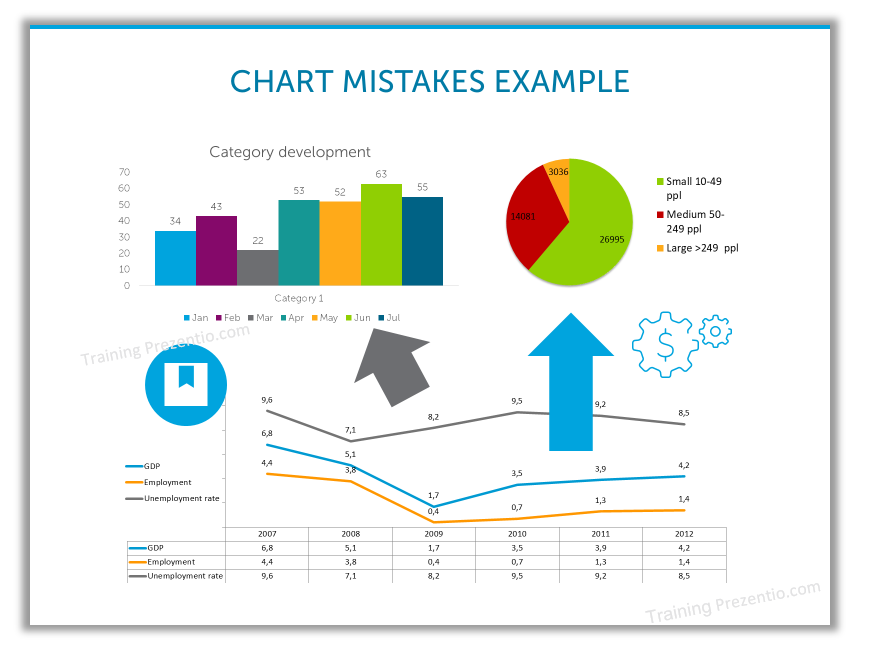
How to tackle reading flow mistake?
Use design elements to lead an eye of a reader – using color contrast, font size or some highlighter shapes – arrow, oval. Remember, natural way for reading the slide is from left to right, from top to bottom (at least in western culture). People will tend to read the slide this way, unless you show the the other way.
Therefore take case your chart objects are aligned for a logical reading flow. This will ensure your slide will be easy to read.
3. Too many data presented on a slide
You may be tempted to show all details of your data analysis, how you got these results, exploring and presenting many data nuances. However ask yourself: Is this a crucial information also for my audience? Presentation should focus on the final result of your analysis and explain it properly.
Imagine there is a limited attention span and people can stay highly focused for a few minutes only. How to do want to spend those precious minutes of their time?
How to tackle data overload issue?
Think twice, whether you need to present all details that led to your final data analysis outcomes. Does the audience need to hear it all? Maybe some parts can be put into appendix materials only?
4. Chart slide too detailed and decorated
It’s good idea to enrich a graph with colors, icons or other additional element. However, each element should have a function. It should add value and not be a mere decoration.
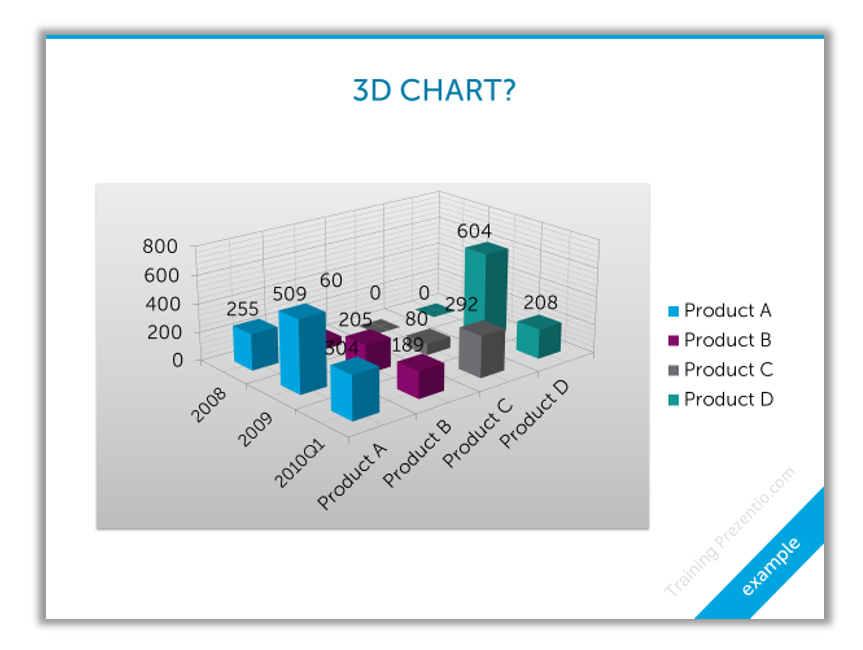
This is another frequent trap in data visualization – having a graph visual with too many too many additional elements. Or using fancy graphs that are not intuitive to understand – this maybe the case of 3D bar charts, or radial charts overuse instead of simple bar chart, if your audience is not used to such charts.
Reading data should not be a quest for a reader.
Cure: Remember “less is often more”. Prefer simplicity over sophistication. If your chart is getting too complex, consider splitting it into more charts. Show one variable at a time. Use color coding to highlight only the most important parts. Consider not showing some data labels if they are essential for data understanding.
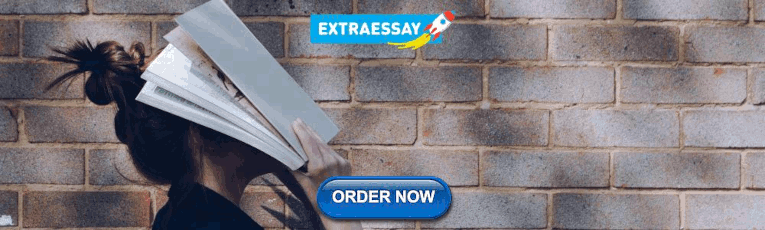
5. Unclear presentation goal
This may not be a strictly visual mistake, but it influences all data presentation challenges I mentioned above. You may have super looking data charts, clear to understand, but if your audience asks at the end “Nice, but what is it good for?”, then the presentation may be wasted time for you and audience. Therefore, think about it before you start making any data visuals.
When putting together a presentation, make sure you know what is your goal, what you want to achieve by showing your data. What’s the story and morale behind data?
Cure: Define the goal of your talk before preparing the presentation. Write it on a paper, white board in the office to have it in the front of your eyes when working on slides content. State it at the presentation beginning, too, so your reader knows what to expect.
Consider structuring your presentation using 4MAT questions model or AIDA flow (Attention, Interest, Desire, Action) – here’s article with using AIDA slide examples .
Summing up data presentation issues
These were a few of examples where data presentation can go wrong. Realizing those risk areas you can think how to tackle them properly. There may be more, of course. A lot depends on your context – are you presenting to experts or novice audience, are you a skilled professional speaker or are you starting the path of presenting.
You don’t need to be a data science Ph.D. expert to use data charts properly. In data visualization, a lot can be done using a few rules of graphical design (contrast, alignment, or consistency). With proper use of colors and PowerPoint shapes can create visually attractive data plots. You can enrich them with additional elements that will help a reader in faster interpretation of data categories.
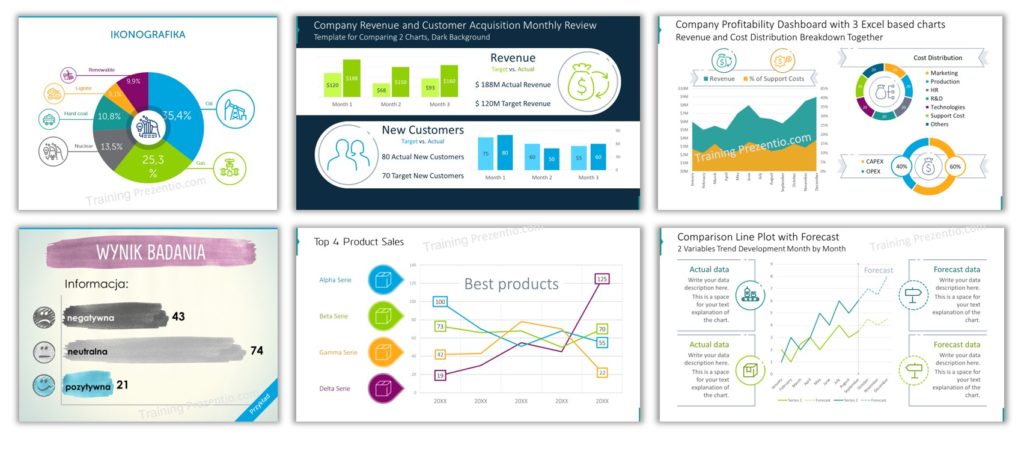
If you want more learn more, check my data presentation training where I share more ways you can enhance data charts and make your presentation slides attractive.
Need a further help in slide redesign – reach out to me , I will be glad to talk.
Peter Concept visualization nerd :), Slide Design Trainer & Designer

- SUGGESTED TOPICS
- The Magazine
- Newsletters
- Managing Yourself
- Managing Teams
- Work-life Balance
- The Big Idea
- Data & Visuals
- Reading Lists
- Case Selections
- HBR Learning
- Topic Feeds
- Account Settings
- Email Preferences
Five Presentation Mistakes Everyone Makes
- Nancy Duarte
Learn from the most common traps.
We all know what it’s like to sit through a bad presentation. We can easily spot the flaws — too long, too boring, indecipherable, what have you — when we watch others speak. The thing is, when we take the stage ourselves, many of us fall into the same traps.

- ND Nancy Duarte is a best-selling author with thirty years of CEO-ing under her belt. She’s driven her firm, Duarte, Inc., to be the global leader behind some of the most influential messages and visuals in business and culture. Duarte, Inc., is the largest design firm in Silicon Valley, as well as one of the top woman-owned businesses in the area. Nancy has written six best-selling books, four have won awards, and her new book, DataStory: Explain Data and Inspire Action Through Story , is available now. Follow Duarte on Twitter: @nancyduarte or LinkedIn .
Partner Center
Data Collection, Presentation and Analysis
- First Online: 25 May 2023
Cite this chapter
- Uche M. Mbanaso 4 ,
- Lucienne Abrahams 5 &
- Kennedy Chinedu Okafor 6
596 Accesses
This chapter covers the topics of data collection, data presentation and data analysis. It gives attention to data collection for studies based on experiments, on data derived from existing published or unpublished data sets, on observation, on simulation and digital twins, on surveys, on interviews and on focus group discussions. One of the interesting features of this chapter is the section dealing with using measurement scales in quantitative research, including nominal scales, ordinal scales, interval scales and ratio scales. It explains key facets of qualitative research including ethical clearance requirements. The chapter discusses the importance of data visualization as key to effective presentation of data, including tabular forms, graphical forms and visual charts such as those generated by Atlas.ti analytical software.
This is a preview of subscription content, log in via an institution to check access.
Access this chapter
- Available as EPUB and PDF
- Read on any device
- Instant download
- Own it forever
- Durable hardcover edition
- Dispatched in 3 to 5 business days
- Free shipping worldwide - see info
Tax calculation will be finalised at checkout
Purchases are for personal use only
Institutional subscriptions
Bibliography
Abdullah, M. F., & Ahmad, K. (2013). The mapping process of unstructured data to structured data. Proceedings of the 2013 International Conference on Research and Innovation in Information Systems (ICRIIS) , Malaysia , 151–155. https://doi.org/10.1109/ICRIIS.2013.6716700
Adnan, K., & Akbar, R. (2019). An analytical study of information extraction from unstructured and multidimensional big data. Journal of Big Data, 6 , 91. https://doi.org/10.1186/s40537-019-0254-8
Article Google Scholar
Alsheref, F. K., & Fattoh, I. E. (2020). Medical text annotation tool based on IBM Watson Platform. Proceedings of the 2020 6th international conference on advanced computing and communication systems (ICACCS) , India , 1312–1316. https://doi.org/10.1109/ICACCS48705.2020.9074309
Cinque, M., Cotroneo, D., Della Corte, R., & Pecchia, A. (2014). What logs should you look at when an application fails? Insights from an industrial case study. Proceedings of the 2014 44th Annual IEEE/IFIP International Conference on Dependable Systems and Networks , USA , 690–695. https://doi.org/10.1109/DSN.2014.69
Gideon, L. (Ed.). (2012). Handbook of survey methodology for the social sciences . Springer.
Google Scholar
Leedy, P., & Ormrod, J. (2015). Practical research planning and design (12th ed.). Pearson Education.
Madaan, A., Wang, X., Hall, W., & Tiropanis, T. (2018). Observing data in IoT worlds: What and how to observe? In Living in the Internet of Things: Cybersecurity of the IoT – 2018 (pp. 1–7). https://doi.org/10.1049/cp.2018.0032
Chapter Google Scholar
Mahajan, P., & Naik, C. (2019). Development of integrated IoT and machine learning based data collection and analysis system for the effective prediction of agricultural residue/biomass availability to regenerate clean energy. Proceedings of the 2019 9th International Conference on Emerging Trends in Engineering and Technology – Signal and Information Processing (ICETET-SIP-19) , India , 1–5. https://doi.org/10.1109/ICETET-SIP-1946815.2019.9092156 .
Mahmud, M. S., Huang, J. Z., Salloum, S., Emara, T. Z., & Sadatdiynov, K. (2020). A survey of data partitioning and sampling methods to support big data analysis. Big Data Mining and Analytics, 3 (2), 85–101. https://doi.org/10.26599/BDMA.2019.9020015
Miswar, S., & Kurniawan, N. B. (2018). A systematic literature review on survey data collection system. Proceedings of the 2018 International Conference on Information Technology Systems and Innovation (ICITSI) , Indonesia , 177–181. https://doi.org/10.1109/ICITSI.2018.8696036
Mosina, C. (2020). Understanding the diffusion of the internet: Redesigning the global diffusion of the internet framework (Research report, Master of Arts in ICT Policy and Regulation). LINK Centre, University of the Witwatersrand. https://hdl.handle.net/10539/30723
Nkamisa, S. (2021). Investigating the integration of drone management systems to create an enabling remote piloted aircraft regulatory environment in South Africa (Research report, Master of Arts in ICT Policy and Regulation). LINK Centre, University of the Witwatersrand. https://hdl.handle.net/10539/33883
QuestionPro. (2020). Survey research: Definition, examples and methods . https://www.questionpro.com/article/survey-research.html
Rajanikanth, J. & Kanth, T. V. R. (2017). An explorative data analysis on Bangalore City Weather with hybrid data mining techniques using R. Proceedings of the 2017 International Conference on Current Trends in Computer, Electrical, Electronics and Communication (CTCEEC) , India , 1121-1125. https://doi/10.1109/CTCEEC.2017.8455008
Rao, R. (2003). From unstructured data to actionable intelligence. IT Professional, 5 , 29–35. https://www.researchgate.net/publication/3426648_From_Unstructured_Data_to_Actionable_Intelligence
Schulze, P. (2009). Design of the research instrument. In P. Schulze (Ed.), Balancing exploitation and exploration: Organizational antecedents and performance effects of innovation strategies (pp. 116–141). Gabler. https://doi.org/10.1007/978-3-8349-8397-8_6
Usanov, A. (2015). Assessing cybersecurity: A meta-analysis of threats, trends and responses to cyber attacks . The Hague Centre for Strategic Studies. https://www.researchgate.net/publication/319677972_Assessing_Cyber_Security_A_Meta-analysis_of_Threats_Trends_and_Responses_to_Cyber_Attacks
Van de Kaa, G., De Vries, H. J., van Heck, E., & van den Ende, J. (2007). The emergence of standards: A meta-analysis. Proceedings of the 2007 40th Annual Hawaii International Conference on Systems Science (HICSS’07) , USA , 173a–173a. https://doi.org/10.1109/HICSS.2007.529
Download references
Author information
Authors and affiliations.
Centre for Cybersecurity Studies, Nasarawa State University, Keffi, Nigeria
Uche M. Mbanaso
LINK Centre, University of the Witwatersrand, Johannesburg, South Africa
Lucienne Abrahams
Department of Mechatronics Engineering, Federal University of Technology, Owerri, Nigeria
Kennedy Chinedu Okafor
You can also search for this author in PubMed Google Scholar
Rights and permissions
Reprints and permissions
Copyright information
© 2023 The Author(s), under exclusive license to Springer Nature Switzerland AG
About this chapter
Mbanaso, U.M., Abrahams, L., Okafor, K.C. (2023). Data Collection, Presentation and Analysis. In: Research Techniques for Computer Science, Information Systems and Cybersecurity. Springer, Cham. https://doi.org/10.1007/978-3-031-30031-8_7
Download citation
DOI : https://doi.org/10.1007/978-3-031-30031-8_7
Published : 25 May 2023
Publisher Name : Springer, Cham
Print ISBN : 978-3-031-30030-1
Online ISBN : 978-3-031-30031-8
eBook Packages : Engineering Engineering (R0)
Share this chapter
Anyone you share the following link with will be able to read this content:
Sorry, a shareable link is not currently available for this article.
Provided by the Springer Nature SharedIt content-sharing initiative
- Publish with us
Policies and ethics
- Find a journal
- Track your research
Misleading Statistics Examples – Discover The Potential For Misuse of Statistics & Data In The Digital Age
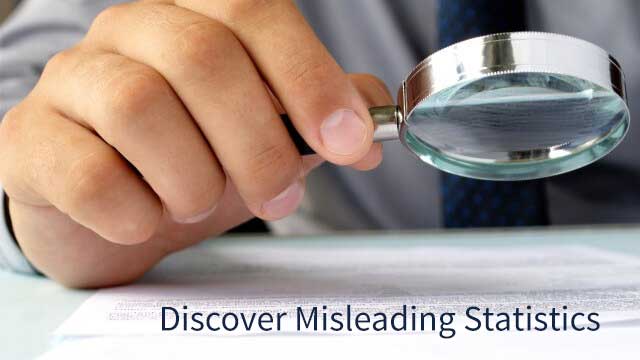
Table of Contents
1) What Is A Misleading Statistic?
2) Are Statistics Reliable?
3) Misleading Statistics Examples In Real Life
4) How Can Statistics Be Misleading
5) How To Avoid & Identify The Misuse Of Statistics?
Statistical analyses have historically been a stalwart of the high-tech and advanced business industries, and today they are more important than ever. With the rise of advanced technology and globalized operations, statistical analyses grant businesses insight into solving the extreme uncertainties of the market. Studies foster informed decision-making, sound judgments, and actions carried out on the weight of evidence, not assumptions.
As businesses are often forced to follow a difficult-to-interpret market roadmap, statistical methods can help with the planning that is necessary to navigate a landscape filled with potholes, pitfalls, and hostile competition. Statistical studies can also assist in the marketing of goods or services, and in understanding each target market’s unique value drivers. In the digital age, these capabilities are only further enhanced and harnessed through the implementation of advanced technology and business intelligence software . If all this is true, what is the problem with statistics?
Actually, there is no problem per se – but there can be. Statistics are infamous for their ability and potential to exist as misleading and bad data . To get this journey started, let's look at a misleading statistics definition.
What Is A Misleading Statistic?
Misleading statistics refers to the misuse of numerical data either intentionally or by error. The results provide deceiving information that creates false narratives around a topic. Misuse of statistics often happens in advertisements, politics, news, media, and others.
Given the importance of data in today’s rapidly evolving digital world, it is important to be familiar with the basics of misleading statistics and oversight. As an exercise in due diligence, we will review some of the most common forms of misuse of statistics, and various alarming (and sadly, common) misleading statistics examples from public life.
Are Statistics Reliable?
73.6% of statistics are false . Really? No, of course, it’s a made-up number (even though such a study would be interesting to know – but again, could have all the flaws it tries at the same time to point out). Statistical reliability is crucial in order to ensure the precision and validity of the analysis. To make sure the reliability is high, there are various techniques to perform – the first of them being the control tests, which should have similar results when reproducing an experiment in similar conditions. These controlling measures are essential and should be part of any experiment or survey – unfortunately, that isn’t always the case.
While numbers don’t lie, they can in fact be used to mislead with half-truths. This is known as the “misuse of statistics.” It is often assumed that the misuse of statistics is limited to those individuals or companies seeking to gain profit from distorting the truth, be it economics, education, or mass media.
However, the telling of half-truths through study is not only limited to mathematical amateurs. A 2009 investigative survey by Dr. Daniele Fanelli from The University of Edinburgh found that 33.7% of scientists surveyed admitted to questionable research practices, including modifying results to improve outcomes, subjective data interpretation, withholding analytical details, and dropping observations because of gut feelings…. Scientists!
While numbers don’t always have to be fabricated or misleading, it is clear that even society’s most trusted numerical gatekeepers are not immune to the carelessness and bias that can arise with statistical interpretation processes. There are different ways in which statistics can be misleading that we will detail later. The most common one is of course correlation versus causation, which always leaves out another (or two or three) factors that are the actual causation of the problem. Drinking tea increases diabetes by 50%, and baldness raises the cardiovascular disease risk up to 70%! Did we forget to mention the amount of sugar put in the tea or the fact that baldness and old age are related – just like cardiovascular disease risks and old age?
So, can statistics be manipulated? They sure can. Do numbers lie? You can be the judge.
Misleading Statistics Examples In Real Life
Now that we’ve put the misuse of statistics in context, let’s look at various digital age examples of statistics that are misleading across five distinct, but related, spectrums: media and politics, news, advertising, science, and healthcare. While certain topics listed here are likely to stir emotion depending on one’s point of view, their inclusion is for data demonstration purposes only.
1) Examples of misleading statistics in politics
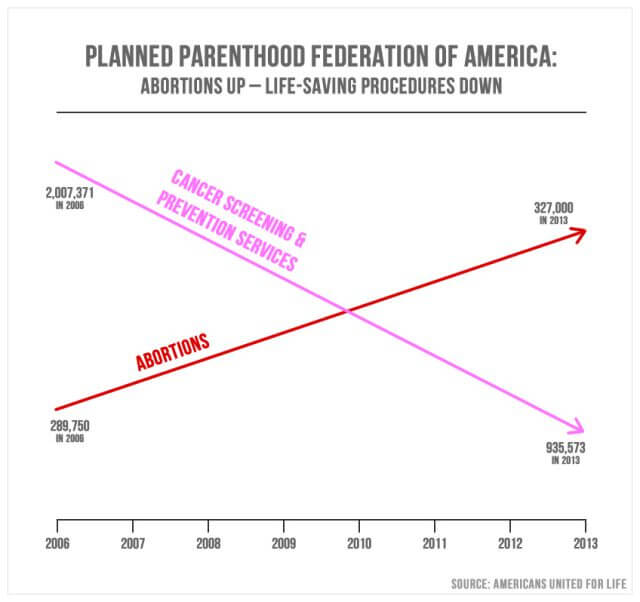
Misleading statistics in politics are quite common. On Sept. 29, 2015, Republicans from the U.S. Congress questioned Cecile Richards, the president of Planned Parenthood, regarding the misappropriation of $500 million in annual federal funding. The above graph/chart was presented as a point of emphasis.
Representative Jason Chaffetz of Utah explained: “In pink, that’s the reduction in the breast exams, and the red is the increase in the abortions. That’s what’s going on in your organization.”
Based on the structure of the chart, it does in fact appear to show that the number of abortions since 2006 experienced substantial growth, while the number of cancer screenings substantially decreased. The intent is to convey a shift in focus from cancer screenings to abortion. The chart points appear to indicate that 327,000 abortions are greater in inherent value than 935,573 cancer screenings. Yet, closer examination will reveal that the chart has no defined y-axis. This means that there is no definable justification for the placement of the visible measurement lines.
Politifact, a fact-checking advocacy website, reviewed Rep. Chaffetz’s numbers via a comparison with Planned Parenthood’s own annual reports. Using a clearly defined scale, here is what the information looks like:

And like this with another valid scale:
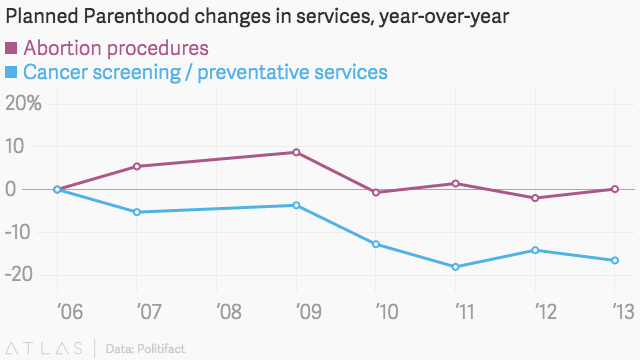
Once placed within a clearly defined scale, it becomes evident that while the amount of cancer screenings has in fact decreased, it still far outnumbers the amount of abortion procedures performed yearly. As such, this is a great misleading statistics example, and some could argue bias considering that the chart originated not from the Congressman, but from Americans United for Life, an anti-abortion group. This is just one of many examples of misleading statistics in the media and politics.
2) Examples of misleading statistics in healthcare
Just like other industries or areas that we will cover on this list of examples, the healthcare industry is not free of the misuse of statistics. Although this controversy happened around 1996, the case of Purdue Pharma and their highly addictive drug OxyContin is still affecting thousands of American citizens and has already taken the lives of thousands of others to this date, all due to the misuse of statistics as a marketing tactic.
The case started when the giant pharmaceutical company, Purdue Pharma, launched its new product OxyContin, which they advertised as a safe, non-addictive opioid that was highly effective for pain relief. Once hearing this statement, doctors were skeptical, as they knew how dangerously addictive opioids could be to treat chronicle pain.
Amongst various videos of “success cases” of patients, merchandising, and unethical messaging included in Purdue’s marketing strategy to advertise OxyContin as a safe drug, there was a very interesting graph, used to prove to doctors that the drug was non-addictive because it stayed on the patient’s blood over time avoiding symptoms of withdrawal. The problem was, the graph, which is depicted below, was built with a y-axis on a logarithmic scale instead of a linear one, making it look like the rate of change is smaller than it actually is.
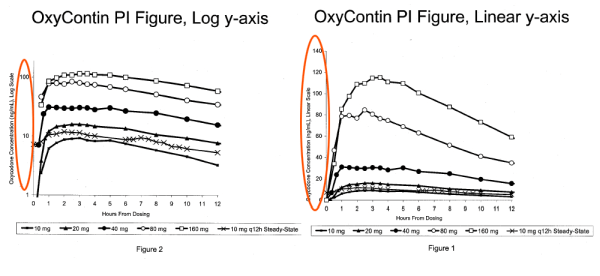
It was this unethical and misleading graph, which was also FDA approved, that helped in initiating one of the biggest health crises in the US, opioid addiction. Although in 2007 the company was forced to pay a $600 million fine for its criminal actions, the consequences of this are still seen to date. This a sad representation of how dangerous misinformation can be.
3) Misleading COVID statistics
This list of misleading statistics fallacy examples would not be complete without referencing the COVID-19 pandemic. During the initial stages of COVID, the general public was forced to consume scientific information in the form of data visualizations to stay informed about the current developments of the virus. But this didn’t come easy. The lack of statistical literacy from the public, paired with the fact that organizations didn’t always share accurate statistical information, lead to widespread misrepresentation of data.
Christopher Engledowl & Travis Weiland wrote an insightful article called “Data (Mis)representation and COVID-19: Leveraging Misleading Data Visualizations For Developing Statistical Literacy Across Grades 6–16”. There, they speak about two use cases in which COVID-19 information was used in a misleading way. Let’s look at one of them closely.
In May 2020, around 5 months after COVID-19 started spreading around the world, the US Georgia Department of Public Health posted a chart that aimed at showing the top 5 counties that had the highest COVID-19 cases in the past 15 days and the number of cases over time.
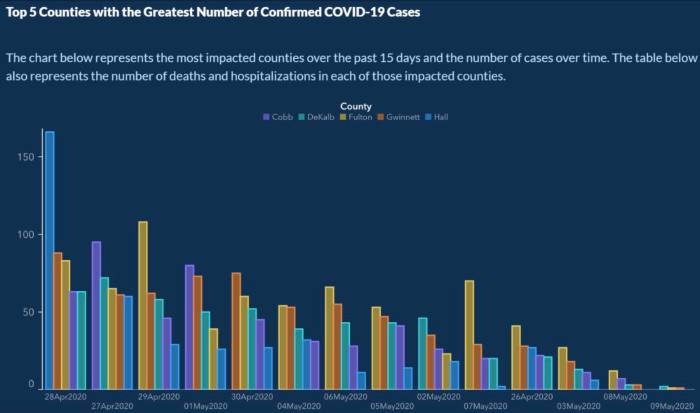
Source : Vox
Now, if we take a closer look at this chart we can find a few mistakes that make the information very misleading. First of all, the X-axis does not have a label, even though according to the chart, it is meant to show the number of cases over time, this doesn't happen.
Another issue, and maybe the worst of them all, is that the dates under the bars are not ordered chronologically. Instead, we see the dates between April and May interspersed with the aim of making viewers of this graph believe that the cases are gradually decreasing. This is paired with the fact that counties are not always depicted in the same order, but instead in descending order of cases. This is with the same aim of making it seem like the cases are dropping.
The graph generated a big controversy on social media, especially on Twitter, where users pointed out that the Georgia Health Department had repeatedly used misleading statistics during the COVID-19 outbreak. As an answer to the issue, Candice Broce , the communications director for Giorgia’s Governor. Brian Kemp's said: "The x-axis was set up that way to show descending values to more easily demonstrate peak values and counties on those dates, our mission failed. We apologize. It is fixed". The graph was later republished with organized dates and counties. You can see the updated version below.
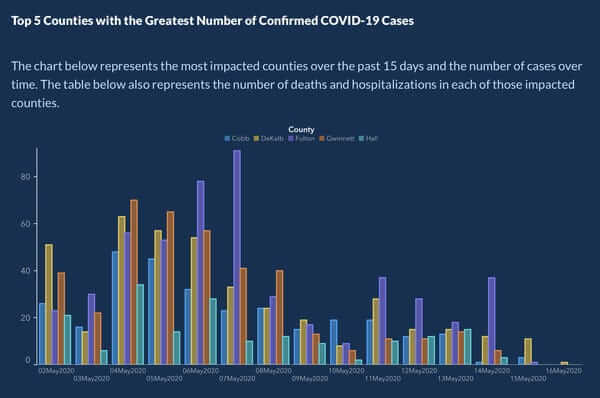
Source: Business Insider
This is one of the many controversial examples of misleading COVID statistics that illustrates the authorities' responsibility to inform their audience accurately. In critical scenarios such as a global pandemic, this becomes even more important as misinformation can lead to a higher spread and more deaths. To avoid situations like this, there is a bunch of healthcare analytics software that assists analysts and average users in the creation of stunning and accurate visualizations for their data.
4) Misleading statistics in advertising
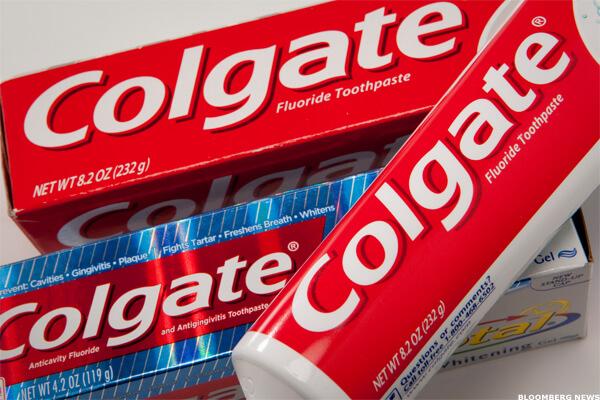
Next, in our list of bad statistics examples, we have the case of a popular toothpaste brand. In 2007, Colgate was ordered by the Advertising Standards Authority (ASA) of the U.K. to abandon their claim: “More than 80% of Dentists recommend Colgate.” The slogan in question was positioned on an advertising billboard in the U.K. and was deemed to be in breach of U.K. advertising rules.
The claim, which was based on surveys of dentists and hygienists carried out by the manufacturer, was found to be misrepresentative as it allowed the participants to select one or more toothpaste brands. The ASA stated that the claim “… would be understood by readers to mean that 80 percent of dentists recommend Colgate over and above other brands, and the remaining 20 percent would recommend different brands.”
The ASA continued, “Because we understood that another competitor’s brand was recommended almost as much as the Colgate brand by the dentists surveyed, we concluded that the claim misleadingly implied 80 percent of dentists recommend Colgate toothpaste in preference to all other brands.” The ASA also claimed that the scripts used for the survey informed the participants that the study was being performed by an independent research company, which was inherently false.
Based on the misuse techniques we covered, it is safe to say that this sleight off-hand technique by Colgate is a clear example of misleading statistics in advertising, and would fall under faulty polling and outright bias.
5) Misleading statistics examples in the news
Misuse of statistics is present everywhere and news outlets are no exception. American network Fox News has been under scrutiny several times throughout the years for showing misleading statistics graphs that seem to purposely portray a conclusion that is not accurate. The most recent case happened not too long ago in September 2021. During one of Fow New’s broadcasts, anchor Tucker Carlson displayed a graph saying that the number of Americans identifying as Christians had collapsed over the last decade.

Source : www.rawstory.com
In the image above, we can see a graph showing 77% of Christian Americans in 2009, a number that decreased to 65% in 2019. Now, if the issue here is not obvious enough, we can see that the Y-axis in this chart starts from 58% and ends at 78%, making the 12% drop from 2009 to 2019 look way more significant than it actually is.
As mentioned, this is not the only time Fox News has been criticized because of these situations. Examples of misuse of statistics in the media are very common. Columbia Journalism School professor Bill Grueskin even made a lesson to its students about the topic and used several misleading charts from the US news show as an example of what not to do when presenting data. Grueskin shared some of these insightful examples of misleading statistics in the news in a Twitter thread that became very popular. We took a very obvious one to show you below.
For the presidential run of 2012, the news network showed the graph below where we see a pie chart displaying a total of 193% which is clearly wrong and misleading as the total should be 100%.

Source: Bill Grueskin
6) Misleading statistics in the media
Television is not the only media platform that can provide examples of bad statistics in the news. In 2006, The Times, a popular UK newspaper, printed a story about how they were the leading paper both online and in print in the UK. The article, titled “The Times leaves the rest behind” started by displaying a graphic of the exponential growth of The Times website visitors from 2004 to 2006. Which saw an increase of millions of visitors in just a couple of years, so far, everything looks normal.
The issue comes with the second graph that is displayed in the article, in which we see a comparison of full-price sales between The Times and one of its biggest competitors, the Daily Telegraph. At a glance, the chart makes you believe that The Times has twice as many full-price subscriptions as its competitor. However, a closer look shows that the X-axis starts at 420,000 instead of 0. Making the difference between the two publications a lot bigger than what it actually is, which is just 10%.

Source : statisticshowto.com
Now, you might argue that The Times is telling the truth, as they are actually leading over their competitors. Regardless, many people will look at the graph and get a different idea of what the actual difference is, which is an unethical and dangerous practice. Just like we saw with Fox News examples, the manipulation of the axes can completely change the way the information on a graph is perceived. We will discuss this specific case in more detail later in the post.
7) Misleading statistics in science
Much like abortion, global warming is another politically charged topic that is likely to arouse emotions. It also happens to be a topic that is vigorously endorsed by both opponents and proponents via studies. Let’s take a look at some of the evidence for and against.
It is generally agreed upon that the global mean temperature in 1998 was 58.3 degrees Fahrenheit. This is according to NASA’s Goddard Institute for Space Studies . In 2012, the global mean temperature was measured at 58.2 degrees. It is, therefore, argued by global warming opponents that, as there was a 0.1-degree decrease in the global mean temperature over a 14-year period, global warming is disproved.
The below graph is the one most often referenced to disprove global warming. It demonstrates the change in air temperature (Celsius) from 1998 to 2012.

It is worth mentioning that 1998 was one of the hottest years on record due to an abnormally strong El Niño wind current. It is also worth noting that, as there is a large degree of variability within the climate system, temperatures are typically measured with at least a 30-year cycle. The below chart expresses the 30-year change in global mean temperatures.
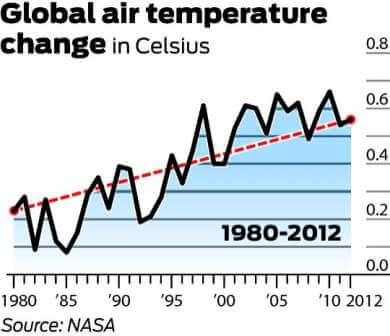
And now have a look at the trend from 1900 to 2012:
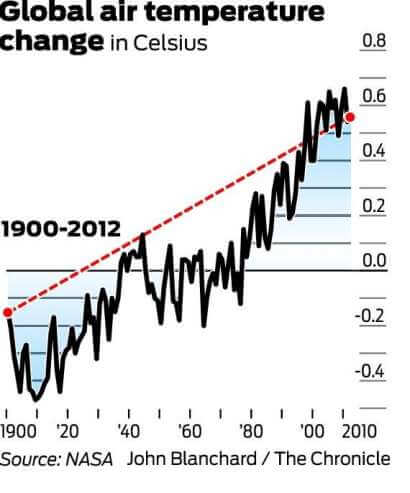
While the long-term data may appear to reflect a plateau, it clearly paints a picture of gradual warming. Therefore, using the first graph, and only the first graph, to disprove global warming is a perfect misleading statistics example.
8) Misleading crime statistics
Rather its politicians trying to make a point for their own interest or just someone not understanding the information behind the graphs and charts they create, crime statistics are not free of being misleading. A controversial representation of this happened in 2014 when a graph depicting the number of murders committed using firearms in Florida from 1990 to 2010 was published in the context of the “Stand Your Ground” law, enacted in 2005 to give people the right to use deadly force for self-defense.
At a first glance, the graph, which is displayed below, shows a descending trend that starts the year the law was enacted, concluding that “Stand Your Grown” is responsible for the apparent drop in the number of murders committed using firearms in the years after it was implemented. However, when taking a closer look at the graph, we can see that the y-axis is reversed, starting with the highest numbers at the bottom and reaching 0 at the top.
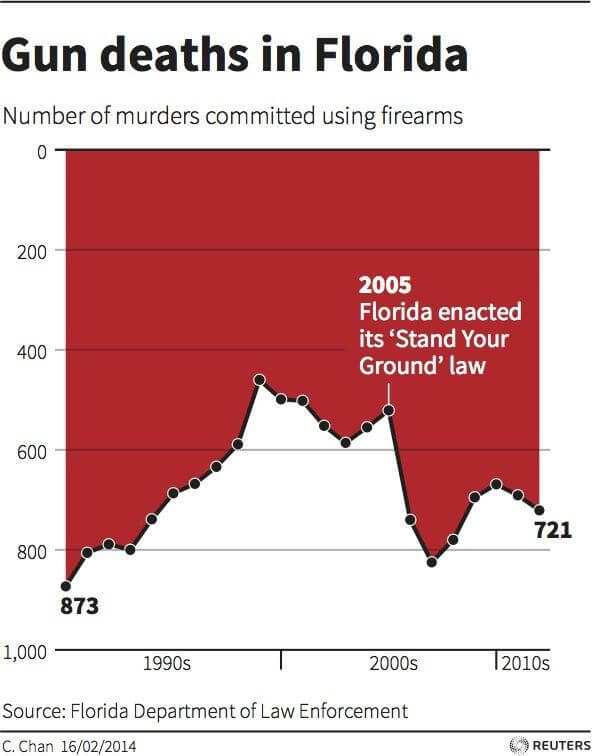
Source : livescience.com
Taking that into account, what the graph is actually showing is an increase in deaths using firearms after the law was enacted. Now, as we learned throughout this post, we can’t say with certainty that the law caused the rise in deaths as there are other factors that could influence that number. There is also no evidence to say that the Florida Law Enforcement Department was purposely deceiving the public. However, at the time this graph was published, many media publications interpreted the graph as if the deaths dropped, showing how damaging the misuse of graphs and numbers can be.
How Can Statistics Be Misleading
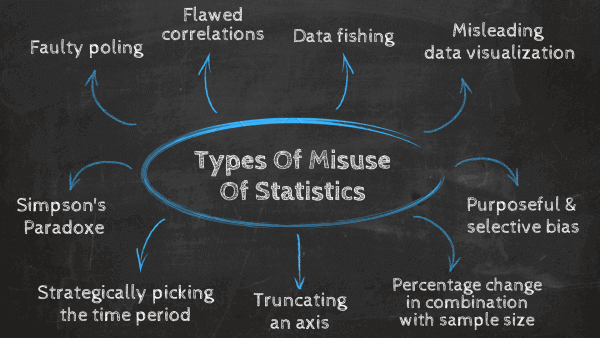
Remember, misuse of statistics can be accidental or purposeful. While a malicious intent to blur lines with misleading statistics will surely magnify bias, the intent is not necessary to create misunderstandings. The misuse of statistics is a much broader problem that now permeates multiple industries and fields of study. Here are a few potential mishaps that commonly lead to misuse:
1) Faulty polling
The manner in which questions are phrased can have a huge impact on the way an audience answers them. Specific wording patterns have a persuasive effect and induce respondents to answer in a predictable manner. For example, on a poll seeking tax opinions, let’s look at the two potential questions:
- Do you believe that you should be taxed so other citizens don’t have to work?
- Do you think that the government should help those people who cannot find work?
These two questions are likely to provoke far different responses, even though they deal with the same topic of government assistance. These are examples of “loaded questions.”
A more accurate way of wording the question would be, “Do you support government assistance programs for unemployment?” or, (even more neutrally) “What is your point of view regarding unemployment assistance?”
The latter two examples of the original questions eliminate any inference or suggestion from the poller, and thus, are significantly more impartial. Another unfair method of polling is to ask a question, but precede it with a conditional statement or a statement of fact. Staying with our example, that would look like this: “Given the rising costs to the middle class, do you support government assistance programs?”
A good rule of thumb is to always take polling with a grain of salt and to try to review the questions that were actually presented. They provide great insight, often more so than the answers.
2) Flawed correlations
The problem with correlations is this: if you measure enough variables, eventually it will appear that some of them correlate. As one out of twenty will inevitably be deemed significant without any direct correlation, studies can be manipulated (with enough data) to prove a correlation that does not exist or that is not significant enough to prove causation.
To illustrate this point further, let’s assume that a study has found a correlation between an increase in car accidents in the state of New York in the month of June (A), and an increase in bear attacks in the state of New York in the month of June (B).
That means there will likely be six possible explanations:
- Car accidents (A) cause bear attacks (B)
- Bear attacks (B) cause car accidents (A)
- Car accidents (A) and bear attacks (B) partly cause each other
- Car accidents (A) and bear attacks (B) are caused by a third factor (C)
- Bear attacks (B) are caused by a third factor (C) which correlates to car accidents (A)
- The correlation is only chance
Any sensible person would easily identify the fact that car accidents do not cause bear attacks. Each is likely a result of a third factor, that being: an increased population, due to the high tourism season in the month of June. It would be preposterous to say that they cause each other... and that is exactly why it is our example. It is easy to see a correlation.
But, what about causation? What if the measured variables were different? What if it was something more believable, like Alzheimer’s and old age? Clearly, there is a correlation between the two, but is there causation? Many would falsely assume, yes, solely based on the strength of the correlation. Tread carefully, for either knowingly or ignorantly, correlation hunting will continue to exist within statistical studies.
3) Data fishing
This misleading data example is also referred to as “data dredging” (and related to flawed correlations). It is a data mining technique where extremely large volumes of data are analyzed for the purpose of discovering relationships between different points. Seeking a relationship between data isn’t a misuse per se, however, doing so without a hypothesis is.
Data dredging is a self-serving technique often employed for the unethical purpose of circumventing traditional data mining techniques, in order to seek additional conclusions that do not exist. This is not to say that there is no proper use of data mining, as it can in fact lead to surprise outliers and interesting analyses. However, more often than not, data dredging is used to assume the existence of relationships without further study.
Oftentimes, data fishing results in studies that are highly publicized due to their important or outlandish findings. These studies are very soon contradicted by other important or outlandish findings. These false correlations often leave the general public very confused and searching for answers regarding the significance of causation and correlation.
Likewise, another common practice with data is omission, meaning that after looking at a large data set of answers, you only pick the ones that are supporting your views and findings and leave out those that contradict them. As mentioned at the beginning of this article, it has been shown that a third of the scientists admitted that they had questionable research practices, including withholding analytical details and modifying results...! But then again, we are facing a study that could itself fall into this 33% of questionable practices, faulty polling, and selective bias... It becomes hard to believe any analysis!
4) Misleading data visualization
Insightful graphs and charts include a very basic, but essential, grouping of elements. Whatever the types of graphs and charts you choose to use, it must convey:
- The scales used
- The starting value (zero or otherwise)
- The method of calculation (e.g., dataset and time period)
Absent these elements, visual data representations should be viewed with a grain of salt, taking into account the common data visualization mistakes one can make. Intermediate data points should also be identified and context is given if it would add value to the information presented. With the increasing reliance on intelligent solution automation for variable data point comparisons, best practices (i.e., design and scaling) should be implemented prior to comparing data from different sources, datasets, times, and locations.
5) Purposeful and selective bias
The next of our most common examples for misuse of statistics and misleading data is, perhaps, the most serious. Purposeful bias is the deliberate attempt to influence findings without even feigning professional accountability. Bias is most likely to take the form of data omissions or adjustments to prove a specific point.
The selective bias is slightly more discreet for those who do not read the small lines. It usually falls down on the sample of people surveyed. For instance, the nature of the group of people surveyed: asking a class of college students about the legal drinking age, or a group of retired people about the elderly care system. You will end up with a statistical error called “selective bias”. To avoid this issue, you should always pick a random sample of people whose background may or may not be related to the topic of the survey.
Businesses and analysts are exposed to making biases when a single person is doing an entire analysis. Whether this person notices or not, they might be providing an inaccurate or manipulated picture to confirm a specific conclusion. This can lead to poor decision-making due to misinformation.
6) Percentage change in combination with a small sample size
Another way of creating misleading statistics, also linked with the choice of sample discussed above, is the size of said sample. When an experiment or a survey is led on a totally not significant sample size, not only will the results be unusable, but the way of presenting them - namely as percentages - will be totally misleading.
Asking a question to a sample size of 20 people, where 19 answers "yes" (=95% say for yes) versus asking the same question to 1,000 people and 950 answers "yes" (=95% as well): the validity of the percentage is clearly not the same. Providing solely the percentage of change without the total numbers or sample size will be totally misleading. xkdc's comic illustrates this very well, to show how the "fastest-growing" claim is a totally relative marketing speech:
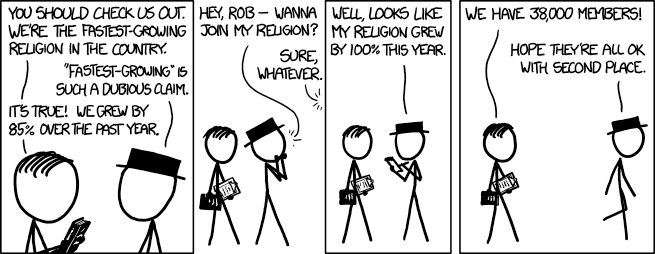
Likewise, the needed sample size is influenced by the kind of question you ask, the statistical significance you need (clinical study vs business study), and the statistical technique. If you perform a quantitative analysis, sample sizes under 200 people are usually invalid.
7) Truncating an axis
Truncating an axis is another way in which statistics can be misleading. When creating a graph to portray a statistic, it is natural to assume that the X and Y axes start at zero. Truncating axes means doing the opposite. For example, starting the axes in a predefined value so that it will affect the way the graph is perceived to achieve a certain conclusion. This technique is often used in politics to exaggerate a result that would otherwise be much less interesting.
Let’s put this into perspective with an example of the misuse of statistics in advertising. The image below shows a graph advertising KFC’s crispy chicken twister wrap and comparing its calories with other brands with a similar product. As we can see, the X axes here start from 590 instead of zero. This makes it appear that KFC’s wrap has half the calories as the ones from Taco Bell, Burger King, or Wendy’s when is actually just 70 calories less.
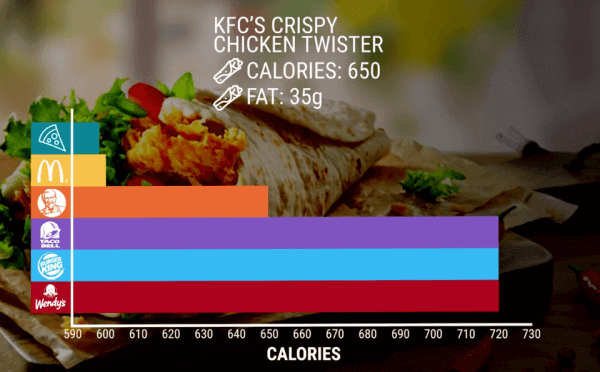
Source : Reddit “Data Is Ugly”
This is a clear situation in which the axes are manipulated to show a specific result that is misleading. Truncating axes is a very dangerous false statistics practice, as it can help create wrong narratives around important topics. In this case, it can create the wrong idea of a product being healthier than it actually is.
8) Strategically picking the time period
Another common misuse of statistics is strategically picking the time period to show a result. This is a case of misleading statistics that can be done purposely, to achieve a specific result, or accidentally. For example, picking only a good-performing month to build a sales report will portray a misleading picture of the overall sales performance.
Purposely or not, the time periods we choose to portray will affect the way viewers perceive the data. For instance, showing a value for 3 months can show radically different trends than showing it over a year. The image below is a great example of this misleading practice. You can see a graph that shows the UK National debt from 1995 to 2016. If you see this graph, you would obviously think the UK’s national debt is higher than ever.

Source : www.economicshelp.org
However, when you look at a longer time period such as 1910 to 2015 (image below) we realize that the debt is actually very low comparing it to other years. Making this a clear example of how the time period that we chose to portray can significantly change the way people will perceive the information.
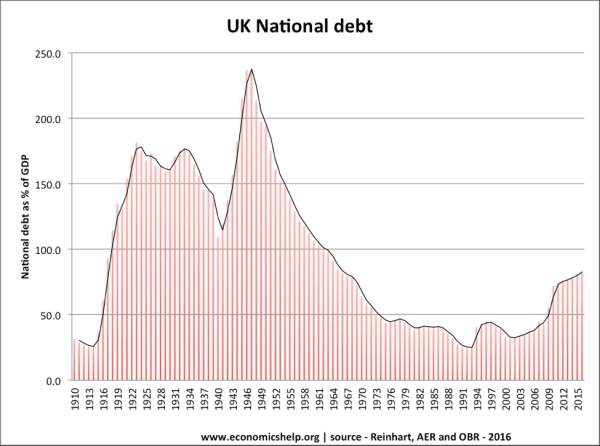
9) Simpson’s Paradox
According to a definition by the Stanford Encyclopedia of Philosophy, a Simpson’s Paradox is a statistical phenomenon where “an association between two variables in a population emerges, disappears or reverses when the population is divided into subpopulations”. Now, you might be wondering, how can this be misleading?
Well, a Simpson’s Paradox can happen when an analyst doesn’t look at the complete scope of the data. When this paradox goes unnoticed, it can significantly influence the way the data is interpreted, leaving room to believe a certain conclusion or assumption is an absolute truth, when it could change by looking at it from a different perspective.
Let’s explain this better with an example . Imagine you are in need of risky emergency surgery and have to choose between going to hospitals A or B to get it. Doing a quick research, you find that 900 out of 1000 patients that went into surgery at Hospital A survived, while 800 out of 1000 survived at Hospital B. Now, the obvious answer is going for option A. However, when considering other factors such as the health conditions in which patients arrived at the hospitals we can drive other conclusions. For instance, of 100 patients that arrived in poor condition in Hospital A, 30 survived. On the other side, of 400 patients that arrived in poor condition at Hospital B, 210 survived at a survival rate of 52.5%.
While initially, the trend was going towards choosing option A, when grouping surviving patients considering other variables the trend changed to option B. This is a Simpson’s Paradox at its finest, and it happens when the data hides a conditional variable that can significantly influence the results.
How To Avoid & Identify The Misuse Of Statistics?
Now that we’ve looked at examples and common cases of misuse of statistics, you might be wondering, how do I avoid all of this? A first good thing would be, of course, to stand in front of an honest survey/experiment/research – pick the one you have beneath your eyes –, that has applied the correct techniques of collection and interpretation of data . But you cannot know until you ask yourself a couple of questions and analyze the results you have in between your hands.
Who is doing the research?
As an entrepreneur and former consultant, Mark Suster advises in an article , you should wonder who did the primary research of said analysis. Independent university study group, lab-affiliated research team, consulting company? From there naturally stems the question: who paid them? As no one works for free, it is always interesting to know who sponsors the research. Likewise, what are the motives behind it? What did the scientist or statisticians try to figure out? Finally, how big was the sample set, and who was part of it? How inclusive was it?
These are important questions to ponder and answer before spreading everywhere skewed or biased results – even though it happens all the time, because of amplification. A typical example of amplification often happens with newspapers and journalists, who take one piece of data and need to turn it into headlines – thus often out of its original context. No one buys a magazine where it states that next year, the same thing is going to happen in XYZ market as this year – even though it is true. Editors, clients, and people want something new, not something they know; that’s why we often end up with an amplification phenomenon that gets echoed and more than it should.
Is the sample size big enough?
As we mentioned earlier, the sample size is of utmost importance when it comes to deciding the worth of a study or its results. If the sample size of the study is too small to prove its conclusion then you should be responsible enough and not use these results as an absolute truth as this paves the way for future misinformation. If you still want to use the data to make a point, you can make sure to mention the small sample size as a disclaimer. That said, a bigger sample size is always better, as it highlights statistical differences more accurately.
Is the language being used objective and formal?
The power of words is huge, therefore, carefully looking at the way a study is written is another great practice to assess its quality. No matter how good a study might be, if it's not written using objective and formal language, then it is at risk to mislead. Researchers should not allow their values, their bias, or their views to impact their research, analysis, or findings, therefore, looking at the way questions and findings are formulated is a good practice.
Are the visuals being used correctly?
If you are the one performing the analysis, for instance generating reports for your job, you can ask yourself a few relevant questions to avoid using misleading statistics. For example, are visualizations representing the data accurately? (labels are clear, axes begin at 0, right chart type, etc) Is the research represented honestly and in an impartial manner? What information is missing from this data? You can also ask someone external to your research to look at the data, someone biased to the topic that can confirm your results are not misleading.
As you saw throughout this post, illustrated with some insightful bad statistics examples, using data in a misleading way is very easy. If you follow all the steps mentioned above, you should be able to make a clear analysis and correct use of data.
Misuse of Statistics - A Summary
To the question "can statistics be manipulated?", we can address 8 methods often used - on purpose or not - that skew the analysis and the results. Here are common types of misuse of statistics:
- Faulty polling
- Flawed correlations
- Data fishing
- Misleading data visualization
- Purposeful and selective bias
- Using percentage change in combination with a small sample size
- Truncating an axis
- Strategically picking the time period
Now that you know them, it will be easier to spot them and question all the stats that are given to you every day. Likewise, in order to ensure you keep a certain distance to the studies and surveys you read, remember the questions to ask yourself - who researched and why, who paid for it, and what was the sample.
Transparency and Data-Driven Business Solutions
While it is quite clear that statistical data has the potential to be misused, it can also ethically drive market value in the digital world. Big data has the ability to provide digital age businesses with a roadmap for efficiency and transparency, and eventually, profitability. Advanced technology solutions like online reporting software can enhance statistical data models, and provide digital age businesses with a step up on their competition.
Whether for market intelligence, customer experience, or business reporting, the future of data is now. Take care to apply data responsibly, ethically, and visually, and watch your transparent corporate identity grow. Revisit this insightful list of bad statistics examples from time to time to remind you of the importance of using data in a proper way!
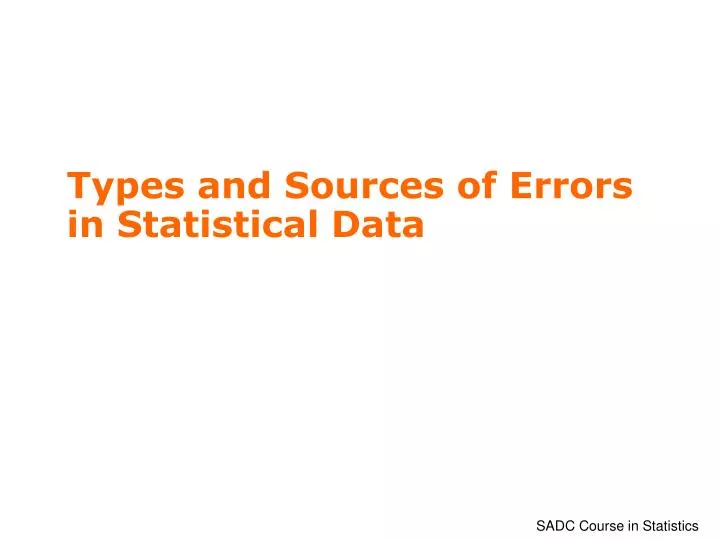
Types and Sources of Errors in Statistical Data
Jun 30, 2012
620 likes | 2.35k Views
Types and Sources of Errors in Statistical Data. Types of Errors. In general, there are two types of errors: a. non-sampling errors and b. sampling errors.
Share Presentation
- data quality assurance systems
- different samples
- whole population
- factors affecting sampling error
- public awareness activities
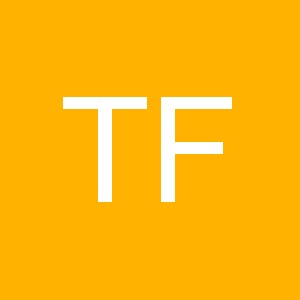
Presentation Transcript
Types of Errors • In general, there are two types of errors: a. non-sampling errors and b. sampling errors. • It is important for a researcher to be aware of these errors, in particular non-sampling errors, so that they can be either minimised or eliminated from the data collected.
Non-sampling errors • These are errors that arise during the course of all data collection activities. • In summary, they have the following characteristics: • exist in both sample surveys and censuses data. • difficult to measure .
Sources of non-sampling errors Non-sampling errors arise from: • defects in the sampling frame. • failure to identify the target population. • non response. • responses given by respondents. • data processing and • reporting, among others.
Defects in the sampling frame • This result in coverage errors. • These occur when there is an omission, duplication or wrongful inclusion of units in the sampling frame. • Omissions are referred to as ‘under coverage’ while duplications and wrongful inclusions are called ‘over coverage’. • These errors are caused by defects such as inaccuracy, incompleteness, duplication, inadequacy and out of date sampling frames. • Coverage errors may also occur in field operations, that is, when an enumerator misses several households or persons during the interviewing process.
Failure to Identify Target Population • This occurs when the target population is not clearly defined through the use of imprecise definitions or concepts or when the survey population does not reflect the target population due to an inadequate sampling frame and poor coverage rules.
Response • They result from the data that have been requested, provided, received or recorded incorrectly. • They may occur as a result of inefficiencies with the questionnaire, the interviewer, the respondent or the survey process.
a. Poor questionnaire design • The content and wording of the questionnaire may be misleading and the layout of the questionnaire may make it difficult to accurately record responses. • As a rule, questions in questionnaire should not be loaded, double-barrelled, misleading or ambiguous, and should be directly relevant to the objectives of the survey. • It is essential to pilot test questionnaires to identify questionnaire flow and question wording problems, and allow sufficient time for improvements to be made to the questionnaire.
Poor questionnaire design – cont’d • The questionnaire should then be re-tested to ensure changes made do not introduce other problems.
b. Interviewer bias • An interviewer may influence the way a respondent answers survey questions. • To prevent this, interviewers must be trained to remain neutral throughout the interviewing process and must pay close attention to the way they ask each question.
c. Respondent errors • These arise through the respondent providing inaccurate or wrong information. • They occur because of memory biases or respondents giving inaccurate or false information when they believe that they are protecting their personal interests or integrity. • They can also arise from the way the respondent interprets the questionnaire and the wording of the answer that the respondent gives. • Careful questionnaire design and effective questionnaire testing can overcome these problems to some extent.
d. Problems with the survey process • Errors can also occur because of problems with the actual survey process such as using proxy responses, that is, taking answers from someone other than the respondent or lacking control over the survey procedure.
Non-Response • Non-response results when data is not collected from respondents. • The proportion of these non-respondents in the sample is called the non-response rate. • Non-response can be either total or partial. • Total non-response or unit non-response can arise if a respondent cannot be contacted (because the sampling frame is incomplete or out-of-dated) or the respondent is not at home or is unable to respond because of language difficulties or illness or out rightly refuses to answer any questions or the dwelling unit is vacant. • Other respondents may indicate that they simply don't have the time to complete the interview or survey form.
Non-response - cont’d • When conducting surveys it is important to document information on why a respondent has not responded. • Partial non-response or item non-response can occur when a respondent replies to some but not all questions of the survey. • This can arise due to memory problems, inadequate information or an inability to answer a particular question/section of the questionnaire. • A respondent may refuse to answer if; a. they find questions particularly sensitive, or if b. they have been asked too many questions.
Non-response - cont’d • To reduce non-response, the following approaches can be used: • care should be taken in questionnaire design through the use of simple questions. • pilot testing of the questionnaire. • explaining survey purposes and uses. • assuring confidentiality of responses. • public awareness activities including discussions with key organisations and interest groups, news releases, media interview and articles.
Processing • These occur at various stages of data processing such as data cleaning, data capture and editing. • Data cleaning involves taking preliminary checks before entering the data onto the processing system. • Coder bias is usually a result of poor training or incomplete instructions, variability in coder performance and data entry errors.
Processing – cont’d • Inadequate checking and quality management at this stage can introduce data loss (where data is not entered into the system) and data duplication (where the same data is entered into the system more than once) thus introducing errors in data. • To minimise these errors, processing staff should be given adequate training, instructions and realistic workloads.
Time Period Bias • This occurs when a survey is conducted during an unrepresentative time period. • Survey timing is thus important and failure to recognise this introduces errors in data.
Analysis and Estimation • Analysis errors include any errors that occur when using wrong analytical tools or when preliminary results are used instead of the final ones. • Errors that occur during the publication of the data results are also considered as analysis errors. • Estimation errors occur when inappropriate or inaccurate weights are used in the estimation procedure thus introducing errors to the data. • They also occur when wrong estimators are selected by the analyst.
Reducing non-sampling errors • Can be minimised by adopting any of the following approaches: • using an up-to-date and accurate sampling frame. • careful selection of the time the survey is conducted. • planning for follow up of non-respondents. • careful questionnaire design. • providing thorough training and periodic retraining of interviewers and processing staff.
Reducing non-sampling errors – cont’d - designing good systems to capture errors that occur during the process of collecting data, sometimes called Data Quality Assurance Systems.
Sampling error • Refer to the difference between the estimate derived from a sample survey and the 'true' value that would result if a census of the whole population were taken under the same conditions. • These are errors that arise because data has been collected from a part, rather than the whole of the population. • Because of the above, sampling errors are restricted to sample surveys only unlike non-sampling errors that can occur in both sample surveys and censuses data.
Sampling errors – cont’d • There are no sampling errors in a census because the calculations are based on the entire population. • They are measurable from the sample data in the case of probability sampling. • More will be discussed in detail in more advanced modules of the training programme.
Factors Affecting Sampling Error It is affected by a number of factors including: a. sample size. • In general, larger sample sizes decrease the sampling error, however this decrease is not directly proportional. • As a rough rule of the thumb, you need to increase the sample size fourfold to halve the sampling error but bear in mind that non sampling errors are likely to increase with large samples. b. the sampling fraction. • this is of lesser influence but as the sample size increases as a fraction of the population, the sampling error should decrease.
Factors Affecting Sampling Error – cont’d c. the variability within the population. • More variable populations give rise to larger errors as the samples or the estimates calculated from different samples are more likely to have greater variation. • The effect of variability within the population can be reduced by the use of stratification that allows explaining some of the variability in the population. d. sample design. • An efficient sampling design will help in reducing sampling error.
Characteristics of the sampling error • generally decreases in magnitude as the sample size increases (but not proportionally). • depends on the variability of the characteristic of interest in the population. • can be accounted for and reduced by an appropriate sample plan. • can be measured and controlled in probability sample surveys.
Reducing sampling error • If sampling principles are applied carefully within the constraints of available resources, sampling error can be kept to a minimum.
Sources • http://www.nss.gov.au/nss/home.nsf/SurveyDesignDoc/4354A8928428F834CA2571AB002479CE?OpenDocument • http://www.statcan.ca/english/edu/power/ch6/nonsampling/nonsampling.htm • http://www.statcan.ca/english/edu/power/ch6/sampling/sampling.htm
- More by User
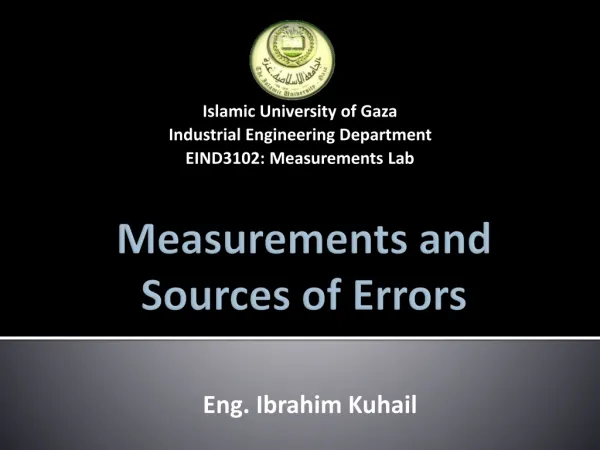
Measurements and Sources of Errors
Islamic University of Gaza Industrial Engineering Department EIND3102: Measurements Lab. Eng. Ibrahim Kuhail. Measurements and Sources of Errors. Metrology.
1.44k views • 28 slides
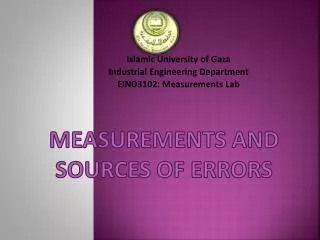
Measurements and Sources of Errors. Islamic University of Gaza Industrial Engineering Department EIND3102: Measurements Lab. Metrology.
680 views • 32 slides
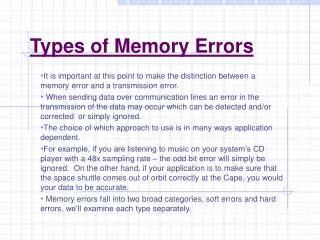
Types of Memory Errors
Types of Memory Errors. It is important at this point to make the distinction between a memory error and a transmission error. When sending data over communication lines an error in the transmission of the data may occur which can be detected and/or corrected or simply ignored.
569 views • 28 slides
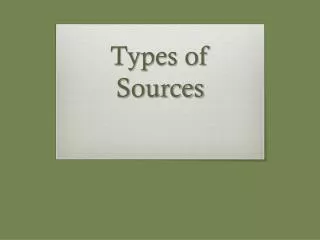
Types of Sources
Types of Sources. “Mind walk”. Not a journal – use a separate piece of paper Fold your paper in half – hot dog style? On the left: “Mind Walk” (think about) all the activities you were involved in in the past 24 hours List as many activities as you can remember on the left side of the paper.
234 views • 10 slides
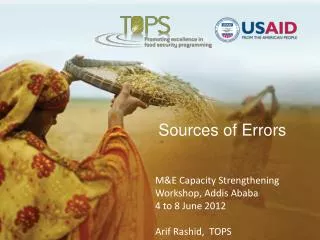
Sources of Errors
Sources of Errors. M&E Capacity Strengthening Workshop, Addis Ababa 4 to 8 June 2012 Arif Rashid, TOPS. Type of Data. Routine Monitoring Data (Output and Process). Annual Monitoring/ Evaluation Survey Data. Slide # 1. Routine Monitoring Data. M&E Officer. Technicians.
435 views • 14 slides
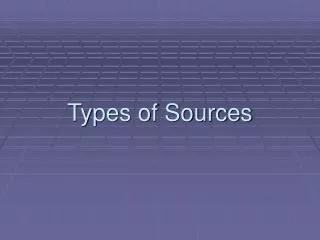
Types of Sources. Add to academic vocabulary. 5. primary source- firsthand information about an event, a place, or time period Draw a picture to represent primary source 6. secondary source- information from someone who did not witness an event Draw a picture to represent secondary source.
324 views • 11 slides
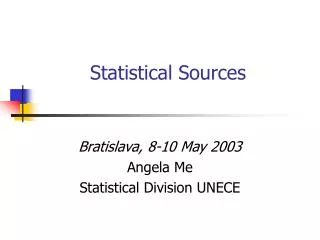
Statistical Sources
Statistical Sources. Bratislava, 8-10 May 2003 Angela Me Statistical Division UNECE. Methods of data collection. Census Surveys Specialized survey On-going survey Administrative records/registers. Advantages It provides data for small areas Data are not affected by sampling errors
799 views • 18 slides
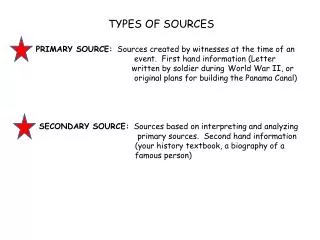
TYPES OF SOURCES
TYPES OF SOURCES. PRIMARY SOURCE: Sources created by witnesses at the time of an event. First hand information (Letter written by soldier during World War II, or original plans for building the Panama Canal). SECONDARY SOURCE: Sources based on interpreting and analyzing
350 views • 11 slides
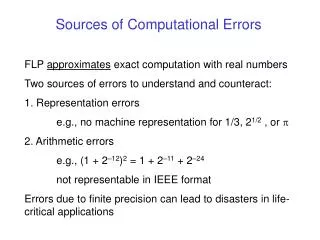
Sources of Computational Errors
Sources of Computational Errors. FLP approximates exact computation with real numbers Two sources of errors to understand and counteract: 1. Representation errors e.g., no machine representation for 1/3, 2 1/2 , or p 2. Arithmetic errors e.g., (1 + 2 –12 ) 2 = 1 + 2 –11 + 2 –24
519 views • 20 slides
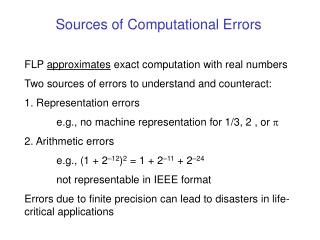
Sources of Computational Errors. FLP approximates exact computation with real numbers Two sources of errors to understand and counteract: 1. Representation errors e.g., no machine representation for 1/3, 2 , or p 2. Arithmetic errors e.g., (1 + 2 –12 ) 2 = 1 + 2 –11 + 2 –24
268 views • 13 slides
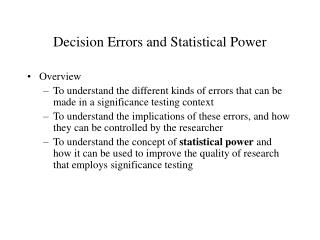
Decision Errors and Statistical Power
Decision Errors and Statistical Power. Overview To understand the different kinds of errors that can be made in a significance testing context To understand the implications of these errors, and how they can be controlled by the researcher
332 views • 13 slides
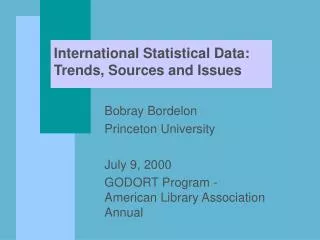
International Statistical Data: Trends, Sources and Issues
International Statistical Data: Trends, Sources and Issues. Bobray Bordelon Princeton University July 9, 2000 GODORT Program - American Library Association Annual. We will examine:. Examples of Commercial Publishers When one should turn to commercial publisher What to look for.
434 views • 30 slides
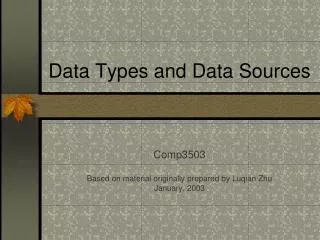
Data Types and Data Sources
Data Types and Data Sources. Comp3503 Based on material originally prepared by Luqian Zhu January, 2003. 1 Datum, 10 Data. A datum - any position or element in relation to which others are determined Data is the plural noun for datum, but commonly data is used for both singular and plural.
299 views • 13 slides
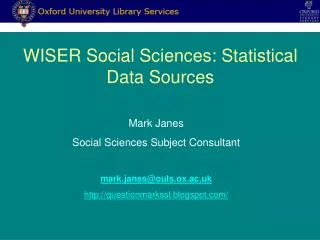
WISER Social Sciences: Statistical Data Sources
WISER Social Sciences: Statistical Data Sources. Mark Janes Social Sciences Subject Consultant [email protected] http://questionmarkssl.blogspot.com/. Question: What is data?. We asked 6 people … Respondent A: social surveys Respondent B: statistical series
292 views • 18 slides
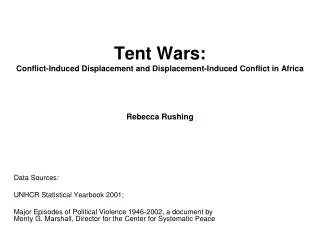
Data Sources: UNHCR Statistical Yearbook 2001;
Tent Wars: Conflict-Induced Displacement and Displacement-Induced Conflict in Africa Rebecca Rushing. Data Sources: UNHCR Statistical Yearbook 2001; Major Episodes of Political Violence 1946-2002, a document by Monty G. Marshall, Director for the Center for Systematic Peace.
196 views • 9 slides
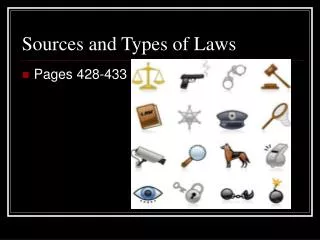
Sources and Types of Laws
Sources and Types of Laws. Pages 428-433. Good Laws. -Characteristics of Good Laws **Why? Fairness Reasonable Understandable Enforceable. Source of Our Laws. -American System - Declaration of Independence - Constitution. -Ancient Law Systems
401 views • 10 slides
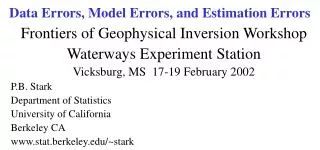
Data Errors, Model Errors, and Estimation Errors
Data Errors, Model Errors, and Estimation Errors. Frontiers of Geophysical Inversion Workshop Waterways Experiment Station Vicksburg, MS 17-19 February 2002 P.B. Stark Department of Statistics University of California Berkeley CA www.stat.berkeley.edu/~stark. Acknowledgements.
494 views • 33 slides
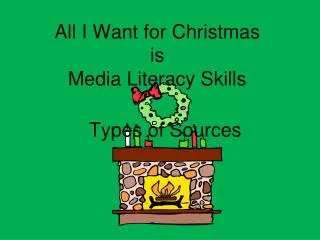
All I Want for Christmas is Media Literacy Skills. Types of Sources. Common Reference Sources. Common Reference Sources. Comparison. Specific Examples. Secondary. Primary. Evaluation of Print. Author’s Purpose Persuade Inform Entertain. P (persuade).
409 views • 18 slides
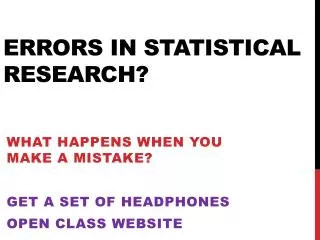
Errors in statistical research?
Errors in statistical research?. What happens when you make a mistake? Get a set of headphones Open class website. Growth in a Time of Debt Carmen M. Reinhart, Kenneth S. Rogoff. Growth in a Time of Debt Carmen M. Reinhart and Kenneth S. Rogoff NBER Working Paper No. 15639 January 2010
425 views • 21 slides
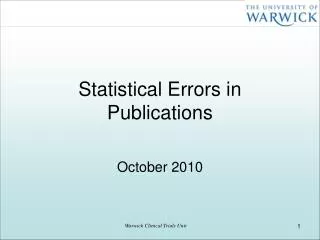
Statistical Errors in Publications
Statistical Errors in Publications. October 2010. OVERVIEW Greater emphasis on sections dealing with: Design; Sample size; Statistical methodology; Results (Presentation/Interpretation); Discussion/Conclusion. SAMPLE PAPERS
505 views • 35 slides
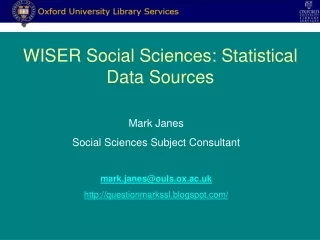
190 views • 18 slides
Failed to get upload status for /mnt/data/*filename*

I tried to get this error by logging in from a different device, but I couldn’t get it. Could the problem here be that GPT cannot get download permission to the browser? Have you checked your settings? Or have you tried from another browser?
I have the same problem. I do have a colleague that is not having the same issue, but she is on Windows 10 and I am on Windows 11. Wonder if that has something to do with it.
Yes, I tried to use several browsers. I also tried it from my phone - from a mobile app and a mobile browser. And it’s always the same mistake. In addition to the application, there is a different name for the error, but, in general, you can’t download anything anyway.
Probably not. I’ve seen in some discussions how people have tried different systems like macOS and got the same error.
Having the same issue and can’t seem to find any working solutions on the web
i have the same problem any solved for this ?
same problem - using Mac OSX. Previously I was successfully generating .docx but today was trying to generate PDFs and experienced this problem. Then I went back to test .docx generation and that failed with the same error as well: “Failed to get upload status…”
You can use Google Collab Notebook to run Python code to download the file to Google Drive.
Don’t forget to replace the root URL in the code (ChatGPT 4.0 will give you the root URL of “sandbox” that should be replaced).

Cheers for the suggestion @TFox69 I received an error
File [“”](https doesn’t allow links so I’m writing to break up the link ://localhost:8080/#), line 12 f.write(response.content) ^
IndentationError: expected an indented block after ‘with’ statement on line 11
I have the same issue with a custom GPT created for a team. Now won’t download any files created by the GPT, with the same “failed to get upload status” that you get. I replicated the prompts in GPT 4o and it works OK so maybe a bug in standard GPT 4 right now.
Not sure how to get developers to notice but this pretty much kills any Custom GPT where my team needs to get files from it.
I copied your code completely, replacing the link to the presentation with mine, created by ChatGPT for the sandbox, but still nothing happened - it creates a document on GoogleDrive, but there is no way to open and view it. Maybe I didn’t understand something in the code and need to add something else, so if so, then I would be glad if you wrote it.
The fact is that I myself am sitting on 4o, but there is a mistake. Apparently, in 4 standard, in 4o, it gives the same errors.
Thanks for your suggested solution! But, after I tried, i notice that downloaded file by using this way can’t be opened or processed correctly.
I have the same issue now. It was working fine otherwise.
Is there any solution?
I am having the same issue with a CSV file I want to download via 4o. I get the exact same error no matter what browser I try it on.
I am having the same issue right now. Can’t download a .docx file.
likewise, I have this issue also.
i have the same, free user might be the reason?
I am running Windows 11 Home beta and I am having the same problems you are experiencing… Could be Windows 11, but then what can I do with only 1 machine… Think going through the trouble of setting up a virtual machine running Windows 10 would help?
Related Topics
Can we help find anything?
No suggestions.
Suggested Searches
Popular Keyword
Search history, recommended search.
Select your province
*Based on your intended shipping destination/store pick-up location
Please confirm your selection. The page will be reloaded to display the corresponding prices.
We're here for you
Welcome to Samsung Support
Popular searches.
- Galaxy S9 - Insert a microSD Card or Remove it (SM-G960W)
- Which Canadian banks are supported on Samsung Pay?
- Can you wash tennis shoes or sneakers in your Samsung washer?
related search
- Live Translation
- Circle to Search
- How to find model number
- Samsung account
- Washer and Dryer
- Oven cleaning
- Refrigerator cleaning
Product Support
Select a model, how to find model code.
Need some help locating your model number? Select your product from the menus below and we'll show you where your number is.
It may be quicker to check for a solution here
Still can't find the answer you're looking for? Click next to e-mail us
How to enter the unlock code
Unlocking your Galaxy phone lets you use your device with a different provider and network. Disclaimer: When you purchase a Samsung phone from a carrier, your phone is locked to their network for a specified period of time according to the contract. You must contact your carrier to find out the conditions of your contract and obtain an unlock code.
Back up and restore your data
When you back up and restore your content using the storage options on your Galaxy device, you will be able to download the file again.
Update the phone number associated with your Samsung account
Please follow this process before updating to One UI 6.1 Your Samsung account holds a lot of important personal information, so it is protected with two-step verification. You'll receive a text message containing a code on your mobile device to confirm that it is you logging into the account. If your mobile number has changed, and you can't receive the text, you'll need to change the phone number on your account.
Find additional information
Setting up your galaxy device, warranty information, premium care service, screen replacement pricing, request repair service, buy authorized samsung parts, visual support, smartthings support, news & alerts, bespoke upgrade care, download manuals, sign language support, door to door repair service, samsung service: terms & conditions, windows information, samsung members community, maintenance mode, interactive tv simulator, protection & peace of mind, contact info, online support, call support.
1-800-SAMSUNG
Face to Face Support
Printers support.
The coding for Contact US > Call > View more function. And this text is only displayed on the editor page, please do not delet this component from Support Home. Thank you

A .gov website belongs to an official government organization in the United States.
A lock ( ) or https:// means you've safely connected to the .gov website. Share sensitive information only on official, secure websites.
- About Cannabis
- Health Effects
- Cannabis FAQs
- Facts and Stats
- Journal Articles
- MMWR Articles
- Related Websites
Cannabis Facts and Stats
At a glance.
A variety of information sources are available to monitor the prevalence and trends of cannabis use in the United States. The resources below cover cannabis-related issues, including data around use, emergency department visits, substance use and misuse, policy measures, and other related tools.
- Cannabis is the most commonly used federally illegal drug in the United States; 52.5 million people, or about 19% of Americans, used it at least once in 2021. 1
- Recent research estimated that approximately 3 in 10 people who use cannabis have cannabis use disorder. 2
- The risk of developing cannabis use disorder is even greater for people who begin to use it before age 18. 3
- Cannabis use directly affects the parts of the brain responsible for memory, learning, attention, decision-making, coordination, emotion, and reaction time. 4 5
- Infants, children, and teens (who still have developing brains) are especially susceptible to the adverse effects of cannabis. 4 5
- Long-term or frequent cannabis use has been linked to increased risk of psychosis or schizophrenia in some users. 6
- Using cannabis during pregnancy may increase the person's risk for pregnancy complications. Pregnant and breastfeeding persons should avoid cannabis. 7
National Surveys That Collect Information About Cannabis Use
Cdc sponsored surveys.
Behavioral Risk Factor Surveillance System (BRFSS)
World's largest, continuously conducted telephone survey that tracks health behaviors, chronic diseases, and preventive health practices among noninstitutionalized adults in the United States.
Youth Risk Behavior Surveillance System (YRBSS)
Monitors six categories of priority health risk behaviors, including cannabis use, among high school youth at national, state, and local levels.
Pregnancy Risk Assessment Monitoring System (PRAMS)
Collects state-specific, population-based data on cannabis use before, during, and shortly after pregnancy.
National Health and Nutrition Examination Survey (NHANES)
Assesses the health and nutritional status of adults and children, aged 12 years and older, in the United States. The survey is unique in that it combines interviews and physical examinations. Voluntary drug use questions ask lifetime cannabis use, age of first use, age when starting to use cannabis regularly, amount used, frequency of use, and time since last use. These data are available from 2005-2007 survey period onward.
Other National Surveys
National Survey on Drug Use and Health (NSDUH)
Ongoing and long-term system, sponsored by the Substance Abuse and Mental Health Services Administration (SAMHSA) NSDUH is the primary source of information on the prevalence, patterns, and consequences of alcohol, tobacco, and illegal drug use and abuse in the general U.S. civilian noninstitutionalized population, ages 12 and older.
Monitoring the Future Survey
Ongoing and long-term system, sponsored by the National Institute on Drug Abuse (NIDA) that collects data on the behaviors, attitudes, and values regarding substance use of American teens, college students, and adults. Each year a total of approximately 50,000 students in 8th, 10th, and 12th grades are surveyed about substance use, including cannabis, and a subset are sent follow-up questionnaires through age 45 years.
National Drug Early Warning System (NDEWS)
NDEWS monitors drug use trends in 12 sentinel communities across the United States. Sentinel Site profiles describing drug abuse trends and emerging issues are available on NDEWS website.
National Programs That Collect Information About Cannabis Policies
Alcohol Policy Information System (APIS)
A policy monitoring system sponsored by the National Institute on Alcohol Abuse and Alcoholism (NIAA) that provides detailed information on a wide variety of alcohol-related policies in the United States at both state and federal levels. The system was expanded in 2016 to include policies related to legalizing the cultivation, sale, or use of cannabis for prohibitions and restrictions on such practices.
State Cannabis Policy Enactment Database
A policy monitoring system sponsored by the National Conference of State Legislatures that provides up-to-date information on cannabis legislation that has been enacted in the 50 states, District of Columbia, and its territories. The database is sortable by state, topic, keyword, and primary sponsor.
- Substance Abuse and Mental Health Services Administration. Key substance use and mental health indicators in the United States: Results from the 2021 National Survey on Drug Use and Health (HHS Publication No. PEP22-07-01-005, NSDUH Series H-57). Center for Behavioral Health Statistics and Quality, Substance Abuse and Mental Health Services Administration. 2022. https://www.samhsa.gov/data/report/2021-nsduh-annual-national-report . Accessed on February 9, 2024.
- Hasin DS, Saha TD, Kerridge BT, et al. Prevalence of marijuana use disorders in the United States between 2001-2002 and 2012-2013. JAMA Psychiatry. 2015 Dec;72(12):1235-1242. doi: 10.1001/jamapsychiatry.2015.1858.
- Winters KC, Lee C-YS. Likelihood of developing an alcohol and cannabis use disorder during youth: Association with recent use and age. Drug Alcohol Depend. 2008;92(1-3):239-247. doi: 10.1016/j.drugalcdep.2007.08.005.
- National Academies of Sciences, Engineering, and Medicine. The health effects of cannabis and cannabinoids: the current state of evidence and recommendations for research. Washington, DC: The National Academies Press; 2017. https://nap.nationalacademies.org/catalog/24625/the-health-effects-of-cannabis-and-cannabinoids-the-current-state. Accessed February 8, 2024.
- Giedd JN. The teen brain: Insights from neuroimaging. J Adolesc Health. 2008;42(4):335–343. doi: 10.1016/j.jadohealth.2008.01.007.
- Volkow ND, Swanson JM, Evins AE, et al. Effects of cannabis use on human behavior, including cognition, motivation, and psychosis: A review. JAMA Psychiatry. 2016 Mar;73(3):292-297. doi: 10.1001/jamapsychiatry.2015.3278.
- Ryan SA, Ammerman SD, O’Connor ME, et al. Marijuana use during pregnancy and breastfeeding: Implications for neonatal and childhood outcomes. Pediatrics. 2018;142(3):e20181889. doi: 10.1542/peds.2018-1889.
Cannabis and Public Health
Cannabis—which can also be called marijuana —is the most commonly used federally illegal drug in the United States.

An official website of the United States government
The .gov means it’s official. Federal government websites often end in .gov or .mil. Before sharing sensitive information, make sure you’re on a federal government site.
The site is secure. The https:// ensures that you are connecting to the official website and that any information you provide is encrypted and transmitted securely.
- Publications
- Account settings
Preview improvements coming to the PMC website in October 2024. Learn More or Try it out now .
- Advanced Search
- Journal List
- Korean J Anesthesiol
- v.70(3); 2017 Jun
Statistical data presentation
1 Department of Anesthesiology and Pain Medicine, Dongguk University Ilsan Hospital, Goyang, Korea.
Sangseok Lee
2 Department of Anesthesiology and Pain Medicine, Sanggye Paik Hospital, Inje University College of Medicine, Seoul, Korea.
Data are usually collected in a raw format and thus the inherent information is difficult to understand. Therefore, raw data need to be summarized, processed, and analyzed. However, no matter how well manipulated, the information derived from the raw data should be presented in an effective format, otherwise, it would be a great loss for both authors and readers. In this article, the techniques of data and information presentation in textual, tabular, and graphical forms are introduced. Text is the principal method for explaining findings, outlining trends, and providing contextual information. A table is best suited for representing individual information and represents both quantitative and qualitative information. A graph is a very effective visual tool as it displays data at a glance, facilitates comparison, and can reveal trends and relationships within the data such as changes over time, frequency distribution, and correlation or relative share of a whole. Text, tables, and graphs for data and information presentation are very powerful communication tools. They can make an article easy to understand, attract and sustain the interest of readers, and efficiently present large amounts of complex information. Moreover, as journal editors and reviewers glance at these presentations before reading the whole article, their importance cannot be ignored.
Introduction
Data are a set of facts, and provide a partial picture of reality. Whether data are being collected with a certain purpose or collected data are being utilized, questions regarding what information the data are conveying, how the data can be used, and what must be done to include more useful information must constantly be kept in mind.
Since most data are available to researchers in a raw format, they must be summarized, organized, and analyzed to usefully derive information from them. Furthermore, each data set needs to be presented in a certain way depending on what it is used for. Planning how the data will be presented is essential before appropriately processing raw data.
First, a question for which an answer is desired must be clearly defined. The more detailed the question is, the more detailed and clearer the results are. A broad question results in vague answers and results that are hard to interpret. In other words, a well-defined question is crucial for the data to be well-understood later. Once a detailed question is ready, the raw data must be prepared before processing. These days, data are often summarized, organized, and analyzed with statistical packages or graphics software. Data must be prepared in such a way they are properly recognized by the program being used. The present study does not discuss this data preparation process, which involves creating a data frame, creating/changing rows and columns, changing the level of a factor, categorical variable, coding, dummy variables, variable transformation, data transformation, missing value, outlier treatment, and noise removal.
We describe the roles and appropriate use of text, tables, and graphs (graphs, plots, or charts), all of which are commonly used in reports, articles, posters, and presentations. Furthermore, we discuss the issues that must be addressed when presenting various kinds of information, and effective methods of presenting data, which are the end products of research, and of emphasizing specific information.
Data Presentation
Data can be presented in one of the three ways:
–as text;
–in tabular form; or
–in graphical form.
Methods of presentation must be determined according to the data format, the method of analysis to be used, and the information to be emphasized. Inappropriately presented data fail to clearly convey information to readers and reviewers. Even when the same information is being conveyed, different methods of presentation must be employed depending on what specific information is going to be emphasized. A method of presentation must be chosen after carefully weighing the advantages and disadvantages of different methods of presentation. For easy comparison of different methods of presentation, let us look at a table ( Table 1 ) and a line graph ( Fig. 1 ) that present the same information [ 1 ]. If one wishes to compare or introduce two values at a certain time point, it is appropriate to use text or the written language. However, a table is the most appropriate when all information requires equal attention, and it allows readers to selectively look at information of their own interest. Graphs allow readers to understand the overall trend in data, and intuitively understand the comparison results between two groups. One thing to always bear in mind regardless of what method is used, however, is the simplicity of presentation.

Values are expressed as mean ± SD. Group C: normal saline, Group D: dexmedetomidine. SBP: systolic blood pressure, DBP: diastolic blood pressure, MBP: mean blood pressure, HR: heart rate. * P < 0.05 indicates a significant increase in each group, compared with the baseline values. † P < 0.05 indicates a significant decrease noted in Group D, compared with the baseline values. ‡ P < 0.05 indicates a significant difference between the groups.
Text presentation
Text is the main method of conveying information as it is used to explain results and trends, and provide contextual information. Data are fundamentally presented in paragraphs or sentences. Text can be used to provide interpretation or emphasize certain data. If quantitative information to be conveyed consists of one or two numbers, it is more appropriate to use written language than tables or graphs. For instance, information about the incidence rates of delirium following anesthesia in 2016–2017 can be presented with the use of a few numbers: “The incidence rate of delirium following anesthesia was 11% in 2016 and 15% in 2017; no significant difference of incidence rates was found between the two years.” If this information were to be presented in a graph or a table, it would occupy an unnecessarily large space on the page, without enhancing the readers' understanding of the data. If more data are to be presented, or other information such as that regarding data trends are to be conveyed, a table or a graph would be more appropriate. By nature, data take longer to read when presented as texts and when the main text includes a long list of information, readers and reviewers may have difficulties in understanding the information.
Table presentation
Tables, which convey information that has been converted into words or numbers in rows and columns, have been used for nearly 2,000 years. Anyone with a sufficient level of literacy can easily understand the information presented in a table. Tables are the most appropriate for presenting individual information, and can present both quantitative and qualitative information. Examples of qualitative information are the level of sedation [ 2 ], statistical methods/functions [ 3 , 4 ], and intubation conditions [ 5 ].
The strength of tables is that they can accurately present information that cannot be presented with a graph. A number such as “132.145852” can be accurately expressed in a table. Another strength is that information with different units can be presented together. For instance, blood pressure, heart rate, number of drugs administered, and anesthesia time can be presented together in one table. Finally, tables are useful for summarizing and comparing quantitative information of different variables. However, the interpretation of information takes longer in tables than in graphs, and tables are not appropriate for studying data trends. Furthermore, since all data are of equal importance in a table, it is not easy to identify and selectively choose the information required.
For a general guideline for creating tables, refer to the journal submission requirements 1) .
Heat maps for better visualization of information than tables
Heat maps help to further visualize the information presented in a table by applying colors to the background of cells. By adjusting the colors or color saturation, information is conveyed in a more visible manner, and readers can quickly identify the information of interest ( Table 2 ). Software such as Excel (in Microsoft Office, Microsoft, WA, USA) have features that enable easy creation of heat maps through the options available on the “conditional formatting” menu.
All numbers were created by the author. SBP: systolic blood pressure, DBP: diastolic blood pressure, MBP: mean blood pressure, HR: heart rate.
Graph presentation
Whereas tables can be used for presenting all the information, graphs simplify complex information by using images and emphasizing data patterns or trends, and are useful for summarizing, explaining, or exploring quantitative data. While graphs are effective for presenting large amounts of data, they can be used in place of tables to present small sets of data. A graph format that best presents information must be chosen so that readers and reviewers can easily understand the information. In the following, we describe frequently used graph formats and the types of data that are appropriately presented with each format with examples.
Scatter plot
Scatter plots present data on the x - and y -axes and are used to investigate an association between two variables. A point represents each individual or object, and an association between two variables can be studied by analyzing patterns across multiple points. A regression line is added to a graph to determine whether the association between two variables can be explained or not. Fig. 2 illustrates correlations between pain scoring systems that are currently used (PSQ, Pain Sensitivity Questionnaire; PASS, Pain Anxiety Symptoms Scale; PCS, Pain Catastrophizing Scale) and Geop-Pain Questionnaire (GPQ) with the correlation coefficient, R, and regression line indicated on the scatter plot [ 6 ]. If multiple points exist at an identical location as in this example ( Fig. 2 ), the correlation level may not be clear. In this case, a correlation coefficient or regression line can be added to further elucidate the correlation.

Bar graph and histogram
A bar graph is used to indicate and compare values in a discrete category or group, and the frequency or other measurement parameters (i.e. mean). Depending on the number of categories, and the size or complexity of each category, bars may be created vertically or horizontally. The height (or length) of a bar represents the amount of information in a category. Bar graphs are flexible, and can be used in a grouped or subdivided bar format in cases of two or more data sets in each category. Fig. 3 is a representative example of a vertical bar graph, with the x -axis representing the length of recovery room stay and drug-treated group, and the y -axis representing the visual analog scale (VAS) score. The mean and standard deviation of the VAS scores are expressed as whiskers on the bars ( Fig. 3 ) [ 7 ].

By comparing the endpoints of bars, one can identify the largest and the smallest categories, and understand gradual differences between each category. It is advised to start the x - and y -axes from 0. Illustration of comparison results in the x - and y -axes that do not start from 0 can deceive readers' eyes and lead to overrepresentation of the results.
One form of vertical bar graph is the stacked vertical bar graph. A stack vertical bar graph is used to compare the sum of each category, and analyze parts of a category. While stacked vertical bar graphs are excellent from the aspect of visualization, they do not have a reference line, making comparison of parts of various categories challenging ( Fig. 4 ) [ 8 ].

A pie chart, which is used to represent nominal data (in other words, data classified in different categories), visually represents a distribution of categories. It is generally the most appropriate format for representing information grouped into a small number of categories. It is also used for data that have no other way of being represented aside from a table (i.e. frequency table). Fig. 5 illustrates the distribution of regular waste from operation rooms by their weight [ 8 ]. A pie chart is also commonly used to illustrate the number of votes each candidate won in an election.

Line plot with whiskers
A line plot is useful for representing time-series data such as monthly precipitation and yearly unemployment rates; in other words, it is used to study variables that are observed over time. Line graphs are especially useful for studying patterns and trends across data that include climatic influence, large changes or turning points, and are also appropriate for representing not only time-series data, but also data measured over the progression of a continuous variable such as distance. As can be seen in Fig. 1 , mean and standard deviation of systolic blood pressure are indicated for each time point, which enables readers to easily understand changes of systolic pressure over time [ 1 ]. If data are collected at a regular interval, values in between the measurements can be estimated. In a line graph, the x-axis represents the continuous variable, while the y-axis represents the scale and measurement values. It is also useful to represent multiple data sets on a single line graph to compare and analyze patterns across different data sets.
Box and whisker chart
A box and whisker chart does not make any assumptions about the underlying statistical distribution, and represents variations in samples of a population; therefore, it is appropriate for representing nonparametric data. AA box and whisker chart consists of boxes that represent interquartile range (one to three), the median and the mean of the data, and whiskers presented as lines outside of the boxes. Whiskers can be used to present the largest and smallest values in a set of data or only a part of the data (i.e. 95% of all the data). Data that are excluded from the data set are presented as individual points and are called outliers. The spacing at both ends of the box indicates dispersion in the data. The relative location of the median demonstrated within the box indicates skewness ( Fig. 6 ). The box and whisker chart provided as an example represents calculated volumes of an anesthetic, desflurane, consumed over the course of the observation period ( Fig. 7 ) [ 9 ].

Three-dimensional effects
Most of the recently introduced statistical packages and graphics software have the three-dimensional (3D) effect feature. The 3D effects can add depth and perspective to a graph. However, since they may make reading and interpreting data more difficult, they must only be used after careful consideration. The application of 3D effects on a pie chart makes distinguishing the size of each slice difficult. Even if slices are of similar sizes, slices farther from the front of the pie chart may appear smaller than the slices closer to the front ( Fig. 8 ).

Drawing a graph: example
Finally, we explain how to create a graph by using a line graph as an example ( Fig. 9 ). In Fig. 9 , the mean values of arterial pressure were randomly produced and assumed to have been measured on an hourly basis. In many graphs, the x- and y-axes meet at the zero point ( Fig. 9A ). In this case, information regarding the mean and standard deviation of mean arterial pressure measurements corresponding to t = 0 cannot be conveyed as the values overlap with the y-axis. The data can be clearly exposed by separating the zero point ( Fig. 9B ). In Fig. 9B , the mean and standard deviation of different groups overlap and cannot be clearly distinguished from each other. Separating the data sets and presenting standard deviations in a single direction prevents overlapping and, therefore, reduces the visual inconvenience. Doing so also reduces the excessive number of ticks on the y-axis, increasing the legibility of the graph ( Fig. 9C ). In the last graph, different shapes were used for the lines connecting different time points to further allow the data to be distinguished, and the y-axis was shortened to get rid of the unnecessary empty space present in the previous graphs ( Fig. 9D ). A graph can be made easier to interpret by assigning each group to a different color, changing the shape of a point, or including graphs of different formats [ 10 ]. The use of random settings for the scale in a graph may lead to inappropriate presentation or presentation of data that can deceive readers' eyes ( Fig. 10 ).

Owing to the lack of space, we could not discuss all types of graphs, but have focused on describing graphs that are frequently used in scholarly articles. We have summarized the commonly used types of graphs according to the method of data analysis in Table 3 . For general guidelines on graph designs, please refer to the journal submission requirements 2) .
Conclusions
Text, tables, and graphs are effective communication media that present and convey data and information. They aid readers in understanding the content of research, sustain their interest, and effectively present large quantities of complex information. As journal editors and reviewers will scan through these presentations before reading the entire text, their importance cannot be disregarded. For this reason, authors must pay as close attention to selecting appropriate methods of data presentation as when they were collecting data of good quality and analyzing them. In addition, having a well-established understanding of different methods of data presentation and their appropriate use will enable one to develop the ability to recognize and interpret inappropriately presented data or data presented in such a way that it deceives readers' eyes [ 11 ].
<Appendix>
Output for presentation.
Discovery and communication are the two objectives of data visualization. In the discovery phase, various types of graphs must be tried to understand the rough and overall information the data are conveying. The communication phase is focused on presenting the discovered information in a summarized form. During this phase, it is necessary to polish images including graphs, pictures, and videos, and consider the fact that the images may look different when printed than how appear on a computer screen. In this appendix, we discuss important concepts that one must be familiar with to print graphs appropriately.
The KJA asks that pictures and images meet the following requirement before submission 3)
“Figures and photographs should be submitted as ‘TIFF’ files. Submit files of figures and photographs separately from the text of the paper. Width of figure should be 84 mm (one column). Contrast of photos or graphs should be at least 600 dpi. Contrast of line drawings should be at least 1,200 dpi. The Powerpoint file (ppt, pptx) is also acceptable.”
Unfortunately, without sufficient knowledge of computer graphics, it is not easy to understand the submission requirement above. Therefore, it is necessary to develop an understanding of image resolution, image format (bitmap and vector images), and the corresponding file specifications.
Resolution is often mentioned to describe the quality of images containing graphs or CT/MRI scans, and video files. The higher the resolution, the clearer and closer to reality the image is, while the opposite is true for low resolutions. The most representative unit used to describe a resolution is “dpi” (dots per inch): this literally translates to the number of dots required to constitute 1 inch. The greater the number of dots, the higher the resolution. The KJA submission requirements recommend 600 dpi for images, and 1,200 dpi 4) for graphs. In other words, resolutions in which 600 or 1,200 dots constitute one inch are required for submission.
There are requirements for the horizontal length of an image in addition to the resolution requirements. While there are no requirements for the vertical length of an image, it must not exceed the vertical length of a page. The width of a column on one side of a printed page is 84 mm, or 3.3 inches (84/25.4 mm ≒ 3.3 inches). Therefore, a graph must have a resolution in which 1,200 dots constitute 1 inch, and have a width of 3.3 inches.
Bitmap and Vector
Methods of image construction are important. Bitmap images can be considered as images drawn on section paper. Enlarging the image will enlarge the picture along with the grid, resulting in a lower resolution; in other words, aliasing occurs. On the other hand, reducing the size of the image will reduce the size of the picture, while increasing the resolution. In other words, resolution and the size of an image are inversely proportionate to one another in bitmap images, and it is a drawback of bitmap images that resolution must be considered when adjusting the size of an image. To enlarge an image while maintaining the same resolution, the size and resolution of the image must be determined before saving the image. An image that has already been created cannot avoid changes to its resolution according to changes in size. Enlarging an image while maintaining the same resolution will increase the number of horizontal and vertical dots, ultimately increasing the number of pixels 5) of the image, and the file size. In other words, the file size of a bitmap image is affected by the size and resolution of the image (file extensions include JPG [JPEG] 6) , PNG 7) , GIF 8) , and TIF [TIFF] 9) . To avoid this complexity, the width of an image can be set to 4 inches and its resolution to 900 dpi to satisfy the submission requirements of most journals [ 12 ].
Vector images overcome the shortcomings of bitmap images. Vector images are created based on mathematical operations of line segments and areas between different points, and are not affected by aliasing or pixelation. Furthermore, they result in a smaller file size that is not affected by the size of the image. They are commonly used for drawings and illustrations (file extensions include EPS 10) , CGM 11) , and SVG 12) ).
Finally, the PDF 13) is a file format developed by Adobe Systems (Adobe Systems, CA, USA) for electronic documents, and can contain general documents, text, drawings, images, and fonts. They can also contain bitmap and vector images. While vector images are used by researchers when working in Powerpoint, they are saved as 960 × 720 dots when saved in TIFF format in Powerpoint. This results in a resolution that is inappropriate for printing on a paper medium. To save high-resolution bitmap images, the image must be saved as a PDF file instead of a TIFF, and the saved PDF file must be imported into an imaging processing program such as Photoshop™(Adobe Systems, CA, USA) to be saved in TIFF format [ 12 ].
1) Instructions to authors in KJA; section 5-(9) Table; https://ekja.org/index.php?body=instruction
2) Instructions to Authors in KJA; section 6-1)-(10) Figures and illustrations in Manuscript preparation; https://ekja.org/index.php?body=instruction
3) Instructions to Authors in KJA; section 6-1)-(10) Figures and illustrations in Manuscript preparation; https://ekja.org/index.php?body=instruction
4) Resolution; in KJA, it is represented by “contrast.”
5) Pixel is a minimum unit of an image and contains information of a dot and color. It is derived by multiplying the number of vertical and horizontal dots regardless of image size. For example, Full High Definition (FHD) monitor has 1920 × 1080 dots ≒ 2.07 million pixel.
6) Joint Photographic Experts Group.
7) Portable Network Graphics.
8) Graphics Interchange Format
9) Tagged Image File Format; TIFF
10) Encapsulated PostScript.
11) Computer Graphics Metafile.
12) Scalable Vector Graphics.
13) Portable Document Format.
- Get 7 Days Free
VBI Vaccines Announces Poster Presentation at 2024 ASCO Annual Meeting Highlighting New Interim Phase 2b Data from VBI-1901 in Recurrent Glioblastoma Patients
VBI Vaccines Inc. (Nasdaq: VBIV) (VBI), a biopharmaceutical company driven by immunology in the pursuit of powerful prevention and treatment of disease, today announced that new interim tumor response data from the ongoing randomized, controlled Phase 2b study of VBI-1901, the Company’s cancer vaccine immunotherapeutic candidate in recurrent glioblastoma (GBM), were accepted for poster presentation at the 2024 American Society of Clinical Oncology (ASCO) Annual Meeting.
The presentation at ASCO will provide an update to the encouraging data previously shared at the World Vaccine Congress Washington in April , including additional data from those initially evaluable patients, as well as data from new patients who have since been randomized into the Phase 2b study.
Presentation Details
- Title: Randomized Phase 2b trial of a CMV vaccine immunotherapeutic candidate (VBI-1901) in recurrent glioblastomas
- Date: Saturday, June 1, 2024
- Poster Session: Central Nervous System Tumors
- Poster Session Time: 9:00 AM – 12:00 PM CDT
Phase 2b Study Design Multi-center, randomized, controlled, open-label study in up to 60 patients with first recurrent GBM
- Intradermal VBI-1901 + GM-CSF: 10 µg dose every 4 weeks until clinical disease progression
- Monotherapy standard-of-care: either intravenous carmustine or oral lomustine, every 6 weeks until disease progression or intolerable toxicity
- Safety and tolerability
- Overall survival (OS) – median and overall
- Tumor response rate (TRR)
- Progression-free survival (PFS)
- Immunologic responses
- Reduction in corticosteroid use relative to baseline
- Change in quality of life compared to baseline
The U.S. Food and Drug Administration (FDA) has considered demonstration of a statistically significant improvement in overall survival relative to a randomized control arm to be clinically significant and has recognized this as criteria to support the approval of new oncology drugs. 1
For more information about the Phase 2b study, visit clinicaltrials.gov and reference trial identifier: NCT03382977.
About GBM and VBI-1901
Scientific literature suggests CMV infection is prevalent in multiple solid tumors, including glioblastoma (GBM). GBM is among the most common and aggressive malignant primary brain tumors in humans. In the U.S. alone, more than 12,000 new cases are diagnosed each year. The current standard of care for treating GBM is surgical resection, followed by radiation and chemotherapy. Even with aggressive treatment, GBM progresses rapidly and has a high mortality.
VBI-1901 is a novel cancer vaccine immunotherapeutic candidate developed using VBI’s enveloped virus-like particle (eVLP) technology to target two highly immunogenic cytomegalovirus (CMV) antigens, gB and pp65. The FDA has granted VBI-1901 Fast Track Designation and Orphan Drug Designation for the treatment of recurrent glioblastoma. These designations are intended to provide certain benefits to drug developers, including more frequent meetings with the FDA, and Accelerated Approval and Priority Review, if relevant criteria are met, among other benefits.
About VBI Vaccines Inc.
VBI Vaccines Inc. (“VBI”) is a biopharmaceutical company driven by immunology in the pursuit of powerful prevention and treatment of disease. Through its innovative approach to virus-like particles (“VLPs”), including a proprietary enveloped VLP (“eVLP”) platform technology and a proprietary mRNA-launched eVLP (“MLE”) platform technology, VBI develops vaccine candidates that mimic the natural presentation of viruses, designed to elicit the innate power of the human immune system. VBI is committed to targeting and overcoming significant infectious diseases, including hepatitis B, coronaviruses, and cytomegalovirus (CMV), as well as aggressive cancers including glioblastoma (GBM). VBI is headquartered in Cambridge, Massachusetts, with research operations in Ottawa, Canada, and a research and manufacturing site in Rehovot, Israel.
Website Home: http://www.vbivaccines.com/ News and Resources: http://www.vbivaccines.com/news-and-resources/ Investors: http://www.vbivaccines.com/investors/
References:
1. Oncology Center of Excellence, Center for Drug Evaluation and Research (CDER) and Center for Biologics Evaluation and Research (CBER) at the Food and Drug Administration. Clinical Trial Endpoints for the Approval of Cancer Drugs and Biologics; Guidance for Industry. FDA.gov. December, 2018
Cautionary Statement on Forward-looking Information
Certain statements in this press release that are forward-looking and not statements of historical fact are forward-looking statements within the meaning of the safe harbor provisions of the Private Securities Litigation Reform Act of 1995 and are forward-looking information within the meaning of Canadian securities laws (collectively, “forward-looking statements”). The Company cautions that such forward-looking statements involve risks and uncertainties that may materially affect the Company’s results of operations. Such forward-looking statements are based on the beliefs of management as well as assumptions made by and information currently available to management. Actual results could differ materially from those contemplated by the forward-looking statements as a result of certain factors, including but not limited to, the Company’s ability to regain and maintain compliance with the listing standards of the Nasdaq Capital Market, the Company’s ability to satisfy all of the conditions to the consummation of the transactions with Brii Biosciences, the Company’s ability to comply with its obligations under its loan agreement with K2 HealthVentures, the impact of general economic, industry or political conditions in the United States or internationally; the impact of the COVID-19 endemic on our clinical studies, manufacturing, business plan, and the global economy; the ability to successfully manufacture and commercialize PreHevbrio/PreHevbri; the ability to establish that potential products are efficacious or safe in preclinical or clinical trials; the ability to establish or maintain collaborations on the development of pipeline candidates and the commercialization of PreHevbrio/PreHevbri; the ability to obtain appropriate or necessary regulatory approvals to market potential products; the ability to obtain future funding for developmental products and working capital and to obtain such funding on commercially reasonable terms; the Company’s ability to manufacture product candidates on a commercial scale or in collaborations with third parties; changes in the size and nature of competitors; the ability to retain key executives and scientists; and the ability to secure and enforce legal rights related to the Company’s products. A discussion of these and other factors, including risks and uncertainties with respect to the Company, is set forth in the Company’s filings with the SEC and the Canadian securities authorities, including its Annual Report on Form 10-K filed with the SEC on April 16, 2024, and filed with the Canadian security authorities at sedarplus.ca on April 16, 2024, as may be supplemented or amended by the Company’s Quarterly Reports on Form 10-Q and Current Reports on Form 8-K. Given these risks, uncertainties and factors, you are cautioned not to place undue reliance on such forward-looking statements, which are qualified in their entirety by this cautionary statement. All such forward-looking statements made herein are based on our current expectations and we undertake no duty or obligation to update or revise any forward-looking statements for any reason, except as required by law.
VBI Nicole Anderson Director, Corporate Communications & IR (617) 830-3031 x124 [email protected]
View source version on businesswire.com: https://www.businesswire.com/news/home/20240522640304/en/
Market Updates
Ai is booming, but consumer spending is slowing. which will prevail in the stock market, what’s happening in the markets this week, is the era of volatility-suppressing policies possibly over, 5 undervalued stocks that crushed earnings for q1 2024, what does nvidia’s stock split mean for investors, after earnings, is home depot stock a buy, a sell, or fairly valued, after earnings, is baidu stock a buy, a sell, or fairly valued, why stocks are hitting record highs—and what could send them back to earth, stock picks, 2 wide-moat stocks to consider, live nation: breakup sought by department of justice probably wouldn’t affect fair value much, after earnings, is applied materials stock a buy, sell, or fairly valued, the best energy stocks to buy, snowflake earnings: mixed news, but signs of stability, nvidia earnings: ai demand smashes expectations again, after earnings, is walmart stock a buy, a sell, or fairly valued, target earnings: margins hold up, but top line constrained by weak discretionary spending, sponsor center.
Advertisement
Supported by
Trump Leads in 5 Key States, as Young and Nonwhite Voters Express Discontent With Biden
A new set of Times/Siena polls, including one with The Philadelphia Inquirer, reveal an erosion of support for the president among young and nonwhite voters upset about the economy and Gaza.
- Share full article
THE NEW YORK TIMES
THE PHILADELPHIA INQUIRER
SIENA COLLEGE POLL
April 28 to May 9
If the 2024 presidential election
were held today , who would you
vote for if the candidates were
Joe Biden and Donald Trump ?
Pennsylvania
Margin of error
If the 2024 presidential election were held today, who would you vote for if the candidates were Joe Biden and Donald Trump ?

By Nate Cohn
Donald J. Trump leads President Biden in five crucial battleground states, a new set of polls shows , as a yearning for change and discontent over the economy and the war in Gaza among young, Black and Hispanic voters threaten to unravel the president’s Democratic coalition.
The surveys by The New York Times, Siena College and The Philadelphia Inquirer found that Mr. Trump was ahead among registered voters in a head-to-head matchup against Mr. Biden in five of six key states: Michigan, Arizona, Nevada, Georgia and Pennsylvania. Mr. Biden led among registered voters in only one battleground state, Wisconsin.
[You can find the full results of the polls, including the exact questions that were asked, here . You can see answers to common questions about our polling process here .]
The race was closer among likely voters. Mr. Trump led in five states as well, but Mr. Biden edged ahead in Michigan while trailing only narrowly in Wisconsin and Pennsylvania. While Mr. Biden won all six of those states in 2020, victories in Pennsylvania, Michigan and Wisconsin would be enough for him to win re-election, provided he won everywhere else he did four years ago.
The results were similar in a hypothetical matchup that included minor-party candidates and the independent candidate Robert F. Kennedy Jr., who won an average of 10 percent of the vote across the six states and drew roughly equally from the two major-party candidates.

The findings are mostly unchanged since the last series of Times/Siena polls in battleground states in November. Since then, the stock market has gained 25 percent, Mr. Trump’s criminal trial in Manhattan has started, and the Biden campaign has unleashed tens of millions of dollars in advertisements across the battleground states.
The polls offer little indication that any of these developments have helped Mr. Biden, hurt Mr. Trump or quelled the electorate’s discontent. Instead, the surveys show that the cost of living, immigration, Israel’s war in Gaza and a desire for change continue to be a drag on the president’s standing. While Mr. Biden benefited from a burst of momentum in the wake of his State of the Union address in March, he continues to trail in the average of national and battleground state polls.
How support for the candidates differ
between registered and likely voters
The findings reveal widespread dissatisfaction with the state of the country and serious doubts about Mr. Biden’s ability to deliver major improvements to American life. A majority of voters still desire the return to normalcy promised by Mr. Biden in the last campaign, but voters in battleground states remain particularly anxious, unsettled and itching for change. Nearly 70 percent of voters say that the country’s political and economic systems need major changes — or even to be torn down entirely.
Only a sliver of Mr. Biden’s supporters — just 13 percent — believe that the president would bring major changes in his second term, while even many of those who dislike Mr. Trump grudgingly acknowledge that he would shake up an unsatisfying status quo.
The sense that Mr. Biden would do little to improve the nation’s fortunes has helped erode his standing among young, Black and Hispanic voters, who usually represent the foundation of any Democratic path to the presidency. The Times/Siena polls found that the three groups wanted fundamental changes to American society, not just a return to normalcy, and few believed that Mr. Biden would make even minor changes that would be good for the country.
Mr. Trump and Mr. Biden are essentially tied among 18-to-29-year-olds and Hispanic voters, even though each group gave Mr. Biden more than 60 percent of their vote in 2020. Mr. Trump also wins more than 20 percent of Black voters — a tally that would be the highest level of Black support for any Republican presidential candidate since the enactment of the Civil Rights Act of 1964.
The polls suggest that Mr. Trump’s strength among young and nonwhite voters has at least temporarily upended the electoral map, with Mr. Trump surging to a significant lead in Arizona, Georgia and Nevada — relatively diverse Sun Belt states where Black and Hispanic voters propelled Mr. Biden to signature victories in the 2020 election.
Mr. Biden nonetheless remains within striking distance. He has maintained most of his support among older and white voters, who are much less likely to demand fundamental changes to the system and far likelier to say that democracy is the most important issue for their vote. As a result, Mr. Biden is more competitive in the three relatively white Northern swing states: Michigan, Pennsylvania and Wisconsin.
The economy and the cost of living, however, remain the most important issues for one-quarter of voters — and a significant drag on Mr. Biden’s prospects. More than half of voters still believe that the economy is “poor,” down merely a single percentage point since November despite cooling inflation, an end to rate hikes and significant stock market gains.
Nearly 40 percent of Mr. Trump’s supporters said that the economy or the cost of living was the most important issue in the election, among them Jennifer Wright, a registered nurse in Sterling Heights, Mich. She supported Mr. Trump in 2016 and 2020, and to her the election comes down to one question: “Who is the best candidate who is going to help me be in a financial situation to retire?”
“Even me, as a registered nurse, I’m buying Kroger brand or store brand. I’m not buying Jif. We’ve all had to cut back,” she said.
The Biden administration’s insistence that the economy is faring well has fallen flat for many voters, including Jacob Sprague, 32, who works as a systems engineer in Reno, Nev. He says that he voted for Mr. Biden in 2020 but will not be doing so this time.
“It is concerning to me when I keep seeing press come out of the White House where they keep saying the economy is good,” Mr. Sprague said. “That’s really weird because I’m paying more on taxes and more on groceries and more on housing and more on fuel. So that doesn’t feel good.”
With less than six months to go until the election, there is still time for an improving economy to lift Mr. Biden’s standing. Historically, polls at this early stage have not been necessarily indicative of the outcome, and Mr. Trump’s breakthrough among traditionally Democratic young, Black and Hispanic voters may not rest on a solid foundation. His strength is concentrated among irregular, disengaged voters who do not pay close attention to politics and may not yet be tuned into the race. They may be prone to shift their views as the race gets underway.
In a finding that will frustrate Democrats, even as it presents opportunity for Mr. Biden, nearly 20 percent of voters blame him more than they do Mr. Trump for the Supreme Court’s decision in 2022 to overturn Roe v. Wade. They may be the kind of voters that the Biden campaign hopes to persuade as the campaign heats up.
The polls showed that abortion loomed as one of Mr. Trump’s biggest vulnerabilities. On average, 64 percent of voters in battleground states said that abortion should be always or mostly legal, including 44 percent of Mr. Trump’s own supporters.
In recent weeks, the Biden campaign has sought to emphasize Mr. Trump’s support for the Supreme Court justices who overturned Roe v. Wade. For now, though, voters preferred Mr. Biden over Mr. Trump to handle the issue of abortion by 11 points, 49 to 38 percent.
A bigger challenge for Mr. Biden than disengaged voters may ultimately be the disaffected and the disillusioned — those who desire fundamental changes to American society, or who believe that the political and economic systems need to be torn down altogether. Not long ago, these anti-system voters might have been reliably Democratic, but Mr. Trump’s anti-establishment populist brand of conservatism has flipped the usual political dynamic.
Seventy percent of voters believe that Mr. Trump will either bring major changes to the political or economic system or tear down the systems altogether, compared with 24 percent who expect the same from Mr. Biden. And while many voters express deep reservations about Mr. Trump personally, 43 percent of voters believe that he will bring good changes to the country, compared with 35 percent who think the changes will be bad.
Most Americans think the system
needs to change …
Which comes closest to your view about
the political and economic system in America,
even if none are exactly right?
The system needs ...
... no changes 2%
Don’t know/
declined to say 2%
… and they think that Donald Trump
would bring more change …
If [this candidate] won the election, do you think
nothing would change, there would be minor
changes to how things work, there would be
major changes to how things work, or he would
tear down the system completely?
would change
Minor changes
declined to say 4%
… but they are split on whether that
change would be good or bad.
Do you think the changes that [this candidate]
would make would be good for the country
or bad for the country, or neither good nor bad?
or very good
or very bad
declined to say 5%
Most Americans think the system needs to change …
Which comes closest to your view about the political and economic
system in America, even if none are exactly right?
... major changes
... minor changes
… and they think that Donald Trump would bring more change …
If [this candidate] won the election, do you think nothing would change,
there would be minor changes to how things work, there would be major
changes to how things work, or he would tear down the system completely?
… but they are split on whether that change would be good or bad.
Do you think the changes that [this candidate] would make would be good
for the country or bad for the country, or neither good nor bad?
Mr. Trump fares especially well among those who believe that the political and economic systems ought to be torn down, a group that represents about 15 percent of registered voters. He leads among these anti-system voters by 32 points, and the tear-it-down voters are especially likely to have defected from the president. In contrast, Mr. Biden retains nearly all of his 2020 supporters who believe only minor changes are necessary.
These change voters are not necessarily demanding a more ideologically progressive agenda. In the last Times/Siena poll of the same states, 11 percent of registered voters thought that Mr. Biden was not progressive or liberal enough. And while many liberal or progressive voters want major changes, relatively few of those voters are defecting from Mr. Biden.
Instead, Mr. Biden’s losses are concentrated among moderate and conservative Democratic-leaning voters, who nonetheless think that the system needs major changes or to be torn down altogether. Mr. Trump wins just 2 percent of Mr. Biden’s “very liberal” 2020 voters who think the system at least needs major changes, compared with 16 percent of those who are moderate or conservative.
One exception is Israel’s war in Gaza, an issue on which most of Mr. Biden’s challenge appears to come from his left. Around 13 percent of the voters who say they voted for Mr. Biden last time, but do not plan to do so again, said that his foreign policy or the war in Gaza was the most important issue to their vote. Just 17 percent of those voters reported sympathizing with Israel over the Palestinians.
Gerard Willingham, 30, works as a web administrator and lives in Riverdale, Ga. He voted for Mr. Biden in 2020, but he plans to vote for a third-party candidate in November because of the president’s response to the conflict in Gaza, the issue about which he cares most right now.
“I think it’s made quite a bit of difference in that it made me more heavily than in the past push toward voting for a third party, even if I feel that the candidates almost 100 percent won’t win,” Mr. Willingham said. “It’s starting to reach into my moral conscience, I guess.”
Mr. Trump’s trial in Manhattan, on charges that he falsified business records related to a hush-money payment to cover up an affair with the adult film star Stormy Daniels, was already underway when the polls began in late April. However, the survey offered little indication that the trial had damaged the former president’s political fortunes, at least so far. Just 29 percent of voters in battleground states said that they were paying “a lot” of attention to Mr. Trump’s legal woes, and 35 percent thought that the trial was likely to end in a conviction.
Alyce McFadden contributed reporting.
Here are the key things to know about how these polls were conducted:
We spoke with 4,097 registered voters in Arizona, Georgia, Michigan, Nevada, Pennsylvania and Wisconsin from April 28 to May 9, 2024.
Our polls are conducted by telephone, using live interviewers, in both English and Spanish. Nearly 95 percent of respondents were contacted on a cellphone for this poll. You can see the exact questions that were asked and the order in which they were asked here .
Voters are selected for the survey from a list of registered voters. The list contains information on the demographic characteristics of every registered voter, allowing us to make sure we reach the right number of voters of each party, race and region. For this set of polls, we placed nearly 500,000 calls to about 410,000 voters.
To further ensure that the results reflect the entire voting population, not just those willing to take a poll, we give more weight to respondents from demographic groups underrepresented among survey respondents, like people without a college degree. You can see more information about the characteristics of our respondents and the weighted sample on the methodology page , under “Composition of the Sample.”
When the states are joined together, the margin of sampling error among registered voters is plus or minus 1.8 percentage points. Each state poll has a margin of error ranging from plus or minus 3.6 points in Pennsylvania to plus or minus 4.6 points in Georgia. In theory, this means that the results should reflect the views of the overall population most of the time, though many other challenges create additional sources of error. When computing the difference between two values — such as a candidate’s lead in a race — the margin of error is twice as large.
You can see full results and a detailed methodology here . If you want to read more about how and why we conduct our polls, you can see answers to frequently asked questions and submit your own questions here .
The New York Times/Philadelphia Inquirer/Siena College poll of Pennsylvania was funded by a grant from The Lenfest Institute for Journalism. The poll was designed and conducted independently from the institute.
Nate Cohn is The Times’s chief political analyst. He covers elections, public opinion, demographics and polling. More about Nate Cohn
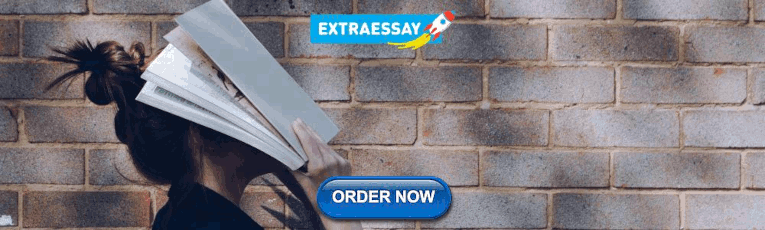
IMAGES
VIDEO
COMMENTS
TheJoelTruth. While a good presentation has data, data alone doesn't guarantee a good presentation. It's all about how that data is presented. The quickest way to confuse your audience is by ...
Data visualization, the process of creating visual representations of data, offers businesses various benefits.One of the most powerful is the ability to communicate with data to a wider audience both internally and externally. This, in turn, enables more stakeholders to make data-driven decisions.. In recent years, a proliferation of data visualization tools has made it easier than ever for ...
Distorting Data Presentation: Using scales or formats that misrepresent the data. Inaccurate Data Descriptions: Text that doesn't match the data can lead to confusion.
Common Mistakes in Data Presentation Stephen Few September 4, 2004 I'm going to take you on a short stream-of-consciousness tour through a few of the most common and sometimes downright amusing problems that I see in my work as a data presentation consultant. This article is the second of a five-part series on the fundamentals of effective data ...
Data presentations go beyond the mere usage of graphical elements. Seasoned presenters encompass visuals with the art of data storytelling, so the speech skillfully connects the points through a narrative that resonates with the audience. Depending on the purpose - inspire, persuade, inform, support decision-making processes, etc. - is the ...
1Mistake 1: Too much data, too little story. One of the most common data presentation mistakes is to overwhelm your audience with too much data, charts, tables, and numbers, without telling a ...
Despite demonstration of widespread statistical and data presentation errors in medical articles, increasing the use of statistical reviewers has been slow . A recent survey found that only 23% of the top biomedical journals reported that they routinely employed statistical review for all original research articles . Introduction of specialist ...
At Comet.ml, we help data scientists and machine learning engineers to automatically track their datasets, code, experiments and results creating efficiency, visibility and reproducibility ...
Each of these unethical data visualization examples has the potential to derail your strategic efforts and take you down an informational dead end. Take heed of these poorly arranged visuals and you will know exactly what to look for when analyzing your business's most invaluable data. 1. Truncated Y-axis.
1. Purpose and audience. Be the first to add your personal experience. 2. Data quality and accuracy. Be the first to add your personal experience. 3. Design and layout. Be the first to add your ...
Start with a Clear Message. If we begin our tour at the very inception of the data presentation process — the point when someone first begins to determine what to present and how — the first common mistake is complete ignorance of the message that ought to be communicated. Before you can determine how to effectively present a message you ...
Questions assessing data presentation in figures (Q6-8) determined if and how plotted measures that summarize variability were defined, and if raw data used to calculate the variability were plotted. Questions assessing the presence of spin (Q9-10) determined if p-values between 0.05 and 0.1 were interpreted as trends or statistically significant.
Summary of small mistakes to avoid while presenting data: Retaining the small fonts of Excel in PowerPoint slides. Using images in chart background. Flaunting thoroughness with footnotes. Showing lack of audience sensitivity while writing text and digits. Showing lack of preparedness by reading numbers.
1. Inappropriate data chart. PowerPoint offers a dozen of data charts you can choose from. Recent addition (from Office365) include fancy looking Sunburst, Funnel or finally Waterfall chart. However for most of presentation purposes you will need to use a few basic charts or their versions: Bar (or column) chart, Pie chart (also doughnut or ...
We can easily spot the flaws — too long, too boring, indecipherable, what have you — when we watch others speak. The thing is, when we take the stage ourselves, many of us fall into the same ...
These include collecting, analyzing, and reporting data. In each of these aspects, errors can and do occur. In this work, we first discuss the importance of focusing on statistical and data errors to continually improve the practice of science. We then describe underlying themes of the types of errors and postulate contributing factors.
Abstract. This chapter covers the topics of data collection, data presentation and data analysis. It gives attention to data collection for studies based on experiments, on data derived from existing published or unpublished data sets, on observation, on simulation and digital twins, on surveys, on interviews and on focus group discussions.
Data were summarised with counts and percentages of papers that fulfilled the scoring criteria for each audit item. As it was not directly relevant to the audit, risk of bias was not assessed. Data processing and analysis were performed in Python (v3.9). Raw data and summary results are provided (underlying data: Audit Results).
Data, which often are numbers and figures, are better presented in tables and graphics, while the interpretation are better stated in text. By doing so, we do not need to repeat the values of HbA 1c in the text (which will be illustrated in tables or graphics), and we can interpret the data for the readers. However, if there are too few variables, the data can be easily described in a simple ...
5) Purposeful and selective bias. The next of our most common examples for misuse of statistics and misleading data is, perhaps, the most serious. Purposeful bias is the deliberate attempt to influence findings without even feigning professional accountability.
Presentation Transcript. Types of Errors • In general, there are two types of errors: a. non-sampling errors and b. sampling errors. • It is important for a researcher to be aware of these errors, in particular non-sampling errors, so that they can be either minimised or eliminated from the data collected. Non-sampling errors • These are ...
I have the same issue with a custom GPT created for a team. Now won't download any files created by the GPT, with the same "failed to get upload status" that you get. I replicated the prompts in GPT 4o and it works OK so maybe a bug in standard GPT 4 right now. Not sure how to get developers to notice but this pretty much kills any Custom ...
Questions assessing data presentation in figures (Q6-8) determined if and how plotted measures that summarize variability were defined, and if raw data used to calculate the variability were plotted. ... Overall, 76-84% of papers with written measures that summarized data variability used standard errors of the mean, and 90-96% of papers did ...
Visit claude.ai! Claude is a family of large language models developed by Anthropic and designed to revolutionize the way you interact with AI. Claude excels at a wide variety of tasks involving language, reasoning, analysis, coding, and more. Our models are highly capable, easy to use, and can be customized to suit your needs.
Online Support. There are a number of a different ways of contacting us via Live Chat, Text, Email and more. Chat Support: 24/7. Please note: If you are unable to access chat, please click here. Learn more Chat with Us.
Custom copilot is pre-populated with information from the file/folder selection. The copilot has a default folder name, branding, description, sources you've selected, and other fields already. You can keep these fields and parameters as-is, or easily update them. Customize the identity with a name change. Customize the grounding knowledge.
Fast facts. Cannabis is the most commonly used federally illegal drug in the United States; 52.5 million people, or about 19% of Americans, used it at least once in 2021. 1. Recent research estimated that approximately 3 in 10 people who use cannabis have cannabis use disorder. 2. The risk of developing cannabis use disorder is even greater for ...
In this article, the techniques of data and information presentation in textual, tabular, and graphical forms are introduced. Text is the principal method for explaining findings, outlining trends, and providing contextual information. A table is best suited for representing individual information and represents both quantitative and ...
VBI Vaccines Announces Poster Presentation at 2024 ASCO Annual Meeting Highlighting New Interim Phase 2b Data from VBI-1901 in Recurrent Glioblastoma Patients.
Donald J. Trump leads President Biden in five crucial battleground states, a new set of polls shows, as a yearning for change and discontent over the economy and the war in Gaza among young, Black ...