
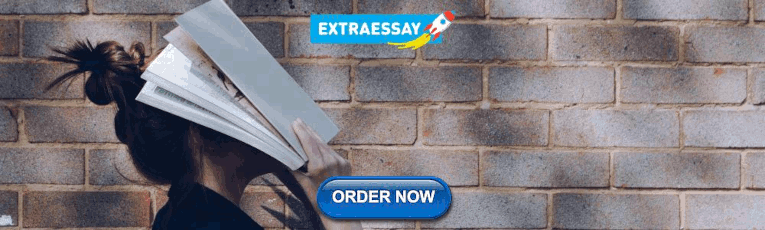
Validity & Reliability In Research
A Plain-Language Explanation (With Examples)
By: Derek Jansen (MBA) | Expert Reviewer: Kerryn Warren (PhD) | September 2023
Validity and reliability are two related but distinctly different concepts within research. Understanding what they are and how to achieve them is critically important to any research project. In this post, we’ll unpack these two concepts as simply as possible.
This post is based on our popular online course, Research Methodology Bootcamp . In the course, we unpack the basics of methodology using straightfoward language and loads of examples. If you’re new to academic research, you definitely want to use this link to get 50% off the course (limited-time offer).
Overview: Validity & Reliability
- The big picture
- Validity 101
- Reliability 101
- Key takeaways
First, The Basics…
First, let’s start with a big-picture view and then we can zoom in to the finer details.
Validity and reliability are two incredibly important concepts in research, especially within the social sciences. Both validity and reliability have to do with the measurement of variables and/or constructs – for example, job satisfaction, intelligence, productivity, etc. When undertaking research, you’ll often want to measure these types of constructs and variables and, at the simplest level, validity and reliability are about ensuring the quality and accuracy of those measurements .
As you can probably imagine, if your measurements aren’t accurate or there are quality issues at play when you’re collecting your data, your entire study will be at risk. Therefore, validity and reliability are very important concepts to understand (and to get right). So, let’s unpack each of them.
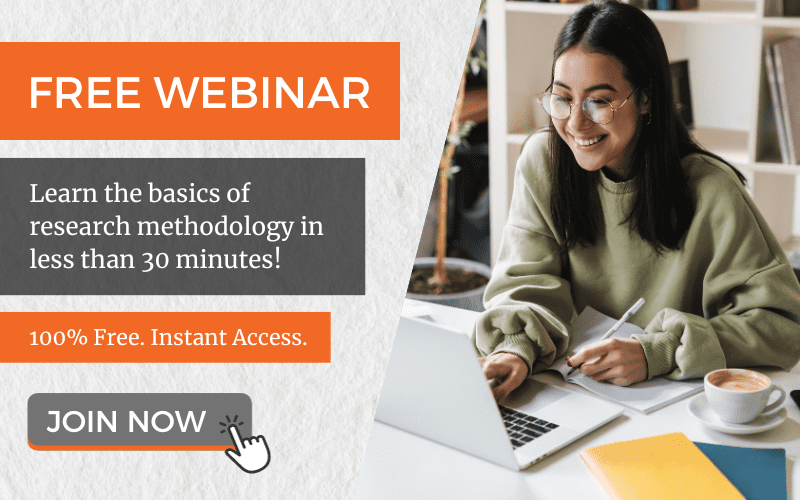
What Is Validity?
In simple terms, validity (also called “construct validity”) is all about whether a research instrument accurately measures what it’s supposed to measure .
For example, let’s say you have a set of Likert scales that are supposed to quantify someone’s level of overall job satisfaction. If this set of scales focused purely on only one dimension of job satisfaction, say pay satisfaction, this would not be a valid measurement, as it only captures one aspect of the multidimensional construct. In other words, pay satisfaction alone is only one contributing factor toward overall job satisfaction, and therefore it’s not a valid way to measure someone’s job satisfaction.
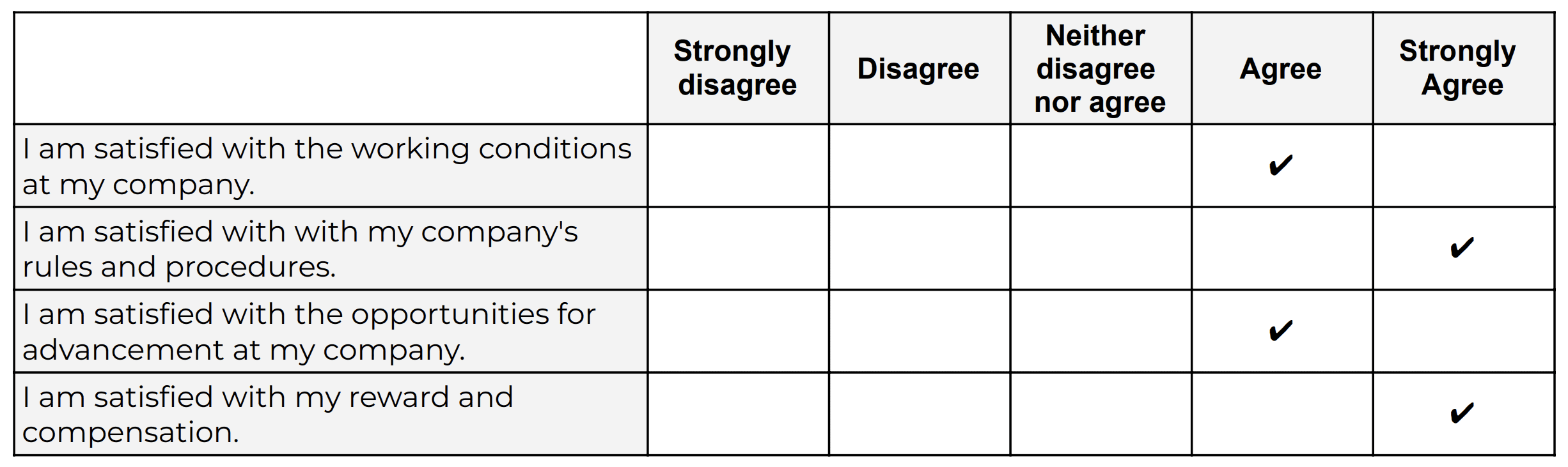
Oftentimes in quantitative studies, the way in which the researcher or survey designer interprets a question or statement can differ from how the study participants interpret it . Given that respondents don’t have the opportunity to ask clarifying questions when taking a survey, it’s easy for these sorts of misunderstandings to crop up. Naturally, if the respondents are interpreting the question in the wrong way, the data they provide will be pretty useless . Therefore, ensuring that a study’s measurement instruments are valid – in other words, that they are measuring what they intend to measure – is incredibly important.
There are various types of validity and we’re not going to go down that rabbit hole in this post, but it’s worth quickly highlighting the importance of making sure that your research instrument is tightly aligned with the theoretical construct you’re trying to measure . In other words, you need to pay careful attention to how the key theories within your study define the thing you’re trying to measure – and then make sure that your survey presents it in the same way.
For example, sticking with the “job satisfaction” construct we looked at earlier, you’d need to clearly define what you mean by job satisfaction within your study (and this definition would of course need to be underpinned by the relevant theory). You’d then need to make sure that your chosen definition is reflected in the types of questions or scales you’re using in your survey . Simply put, you need to make sure that your survey respondents are perceiving your key constructs in the same way you are. Or, even if they’re not, that your measurement instrument is capturing the necessary information that reflects your definition of the construct at hand.
If all of this talk about constructs sounds a bit fluffy, be sure to check out Research Methodology Bootcamp , which will provide you with a rock-solid foundational understanding of all things methodology-related. Remember, you can take advantage of our 60% discount offer using this link.
Need a helping hand?
What Is Reliability?
As with validity, reliability is an attribute of a measurement instrument – for example, a survey, a weight scale or even a blood pressure monitor. But while validity is concerned with whether the instrument is measuring the “thing” it’s supposed to be measuring, reliability is concerned with consistency and stability . In other words, reliability reflects the degree to which a measurement instrument produces consistent results when applied repeatedly to the same phenomenon , under the same conditions .
As you can probably imagine, a measurement instrument that achieves a high level of consistency is naturally more dependable (or reliable) than one that doesn’t – in other words, it can be trusted to provide consistent measurements . And that, of course, is what you want when undertaking empirical research. If you think about it within a more domestic context, just imagine if you found that your bathroom scale gave you a different number every time you hopped on and off of it – you wouldn’t feel too confident in its ability to measure the variable that is your body weight 🙂
It’s worth mentioning that reliability also extends to the person using the measurement instrument . For example, if two researchers use the same instrument (let’s say a measuring tape) and they get different measurements, there’s likely an issue in terms of how one (or both) of them are using the measuring tape. So, when you think about reliability, consider both the instrument and the researcher as part of the equation.
As with validity, there are various types of reliability and various tests that can be used to assess the reliability of an instrument. A popular one that you’ll likely come across for survey instruments is Cronbach’s alpha , which is a statistical measure that quantifies the degree to which items within an instrument (for example, a set of Likert scales) measure the same underlying construct . In other words, Cronbach’s alpha indicates how closely related the items are and whether they consistently capture the same concept .
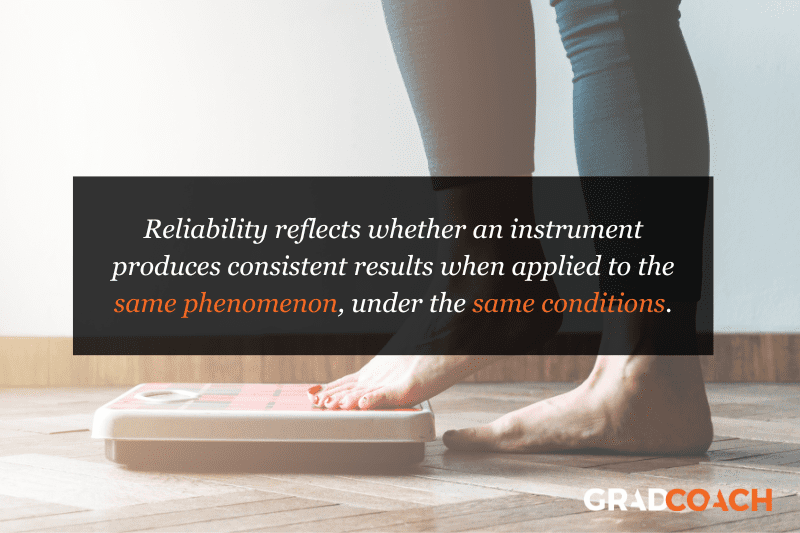
Recap: Key Takeaways
Alright, let’s quickly recap to cement your understanding of validity and reliability:
- Validity is concerned with whether an instrument (e.g., a set of Likert scales) is measuring what it’s supposed to measure
- Reliability is concerned with whether that measurement is consistent and stable when measuring the same phenomenon under the same conditions.
In short, validity and reliability are both essential to ensuring that your data collection efforts deliver high-quality, accurate data that help you answer your research questions . So, be sure to always pay careful attention to the validity and reliability of your measurement instruments when collecting and analysing data. As the adage goes, “rubbish in, rubbish out” – make sure that your data inputs are rock-solid.
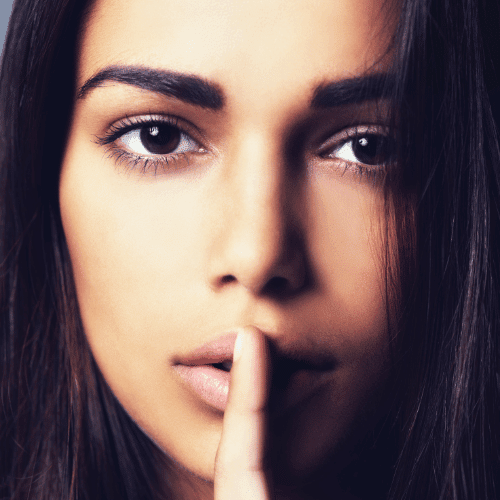
Psst… there’s more!
This post is an extract from our bestselling short course, Methodology Bootcamp . If you want to work smart, you don't want to miss this .
You Might Also Like:
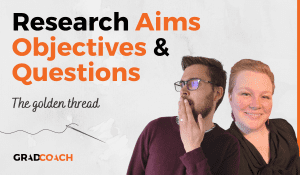
THE MATERIAL IS WONDERFUL AND BENEFICIAL TO ALL STUDENTS.
THE MATERIAL IS WONDERFUL AND BENEFICIAL TO ALL STUDENTS AND I HAVE GREATLY BENEFITED FROM THE CONTENT.
Submit a Comment Cancel reply
Your email address will not be published. Required fields are marked *
Save my name, email, and website in this browser for the next time I comment.
- Print Friendly
- How it works
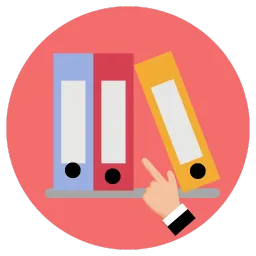
Reliability and Validity – Definitions, Types & Examples
Published by Alvin Nicolas at August 16th, 2021 , Revised On October 26, 2023
A researcher must test the collected data before making any conclusion. Every research design needs to be concerned with reliability and validity to measure the quality of the research.
What is Reliability?
Reliability refers to the consistency of the measurement. Reliability shows how trustworthy is the score of the test. If the collected data shows the same results after being tested using various methods and sample groups, the information is reliable. If your method has reliability, the results will be valid.
Example: If you weigh yourself on a weighing scale throughout the day, you’ll get the same results. These are considered reliable results obtained through repeated measures.
Example: If a teacher conducts the same math test of students and repeats it next week with the same questions. If she gets the same score, then the reliability of the test is high.
What is the Validity?
Validity refers to the accuracy of the measurement. Validity shows how a specific test is suitable for a particular situation. If the results are accurate according to the researcher’s situation, explanation, and prediction, then the research is valid.
If the method of measuring is accurate, then it’ll produce accurate results. If a method is reliable, then it’s valid. In contrast, if a method is not reliable, it’s not valid.
Example: Your weighing scale shows different results each time you weigh yourself within a day even after handling it carefully, and weighing before and after meals. Your weighing machine might be malfunctioning. It means your method had low reliability. Hence you are getting inaccurate or inconsistent results that are not valid.
Example: Suppose a questionnaire is distributed among a group of people to check the quality of a skincare product and repeated the same questionnaire with many groups. If you get the same response from various participants, it means the validity of the questionnaire and product is high as it has high reliability.
Most of the time, validity is difficult to measure even though the process of measurement is reliable. It isn’t easy to interpret the real situation.
Example: If the weighing scale shows the same result, let’s say 70 kg each time, even if your actual weight is 55 kg, then it means the weighing scale is malfunctioning. However, it was showing consistent results, but it cannot be considered as reliable. It means the method has low reliability.
Internal Vs. External Validity
One of the key features of randomised designs is that they have significantly high internal and external validity.
Internal validity is the ability to draw a causal link between your treatment and the dependent variable of interest. It means the observed changes should be due to the experiment conducted, and any external factor should not influence the variables .
Example: age, level, height, and grade.
External validity is the ability to identify and generalise your study outcomes to the population at large. The relationship between the study’s situation and the situations outside the study is considered external validity.
Also, read about Inductive vs Deductive reasoning in this article.
Looking for reliable dissertation support?
We hear you.
- Whether you want a full dissertation written or need help forming a dissertation proposal, we can help you with both.
- Get different dissertation services at ResearchProspect and score amazing grades!
Threats to Interval Validity
Threats of external validity, how to assess reliability and validity.
Reliability can be measured by comparing the consistency of the procedure and its results. There are various methods to measure validity and reliability. Reliability can be measured through various statistical methods depending on the types of validity, as explained below:
Types of Reliability
Types of validity.
As we discussed above, the reliability of the measurement alone cannot determine its validity. Validity is difficult to be measured even if the method is reliable. The following type of tests is conducted for measuring validity.
Does your Research Methodology Have the Following?
- Great Research/Sources
- Perfect Language
- Accurate Sources
If not, we can help. Our panel of experts makes sure to keep the 3 pillars of Research Methodology strong.
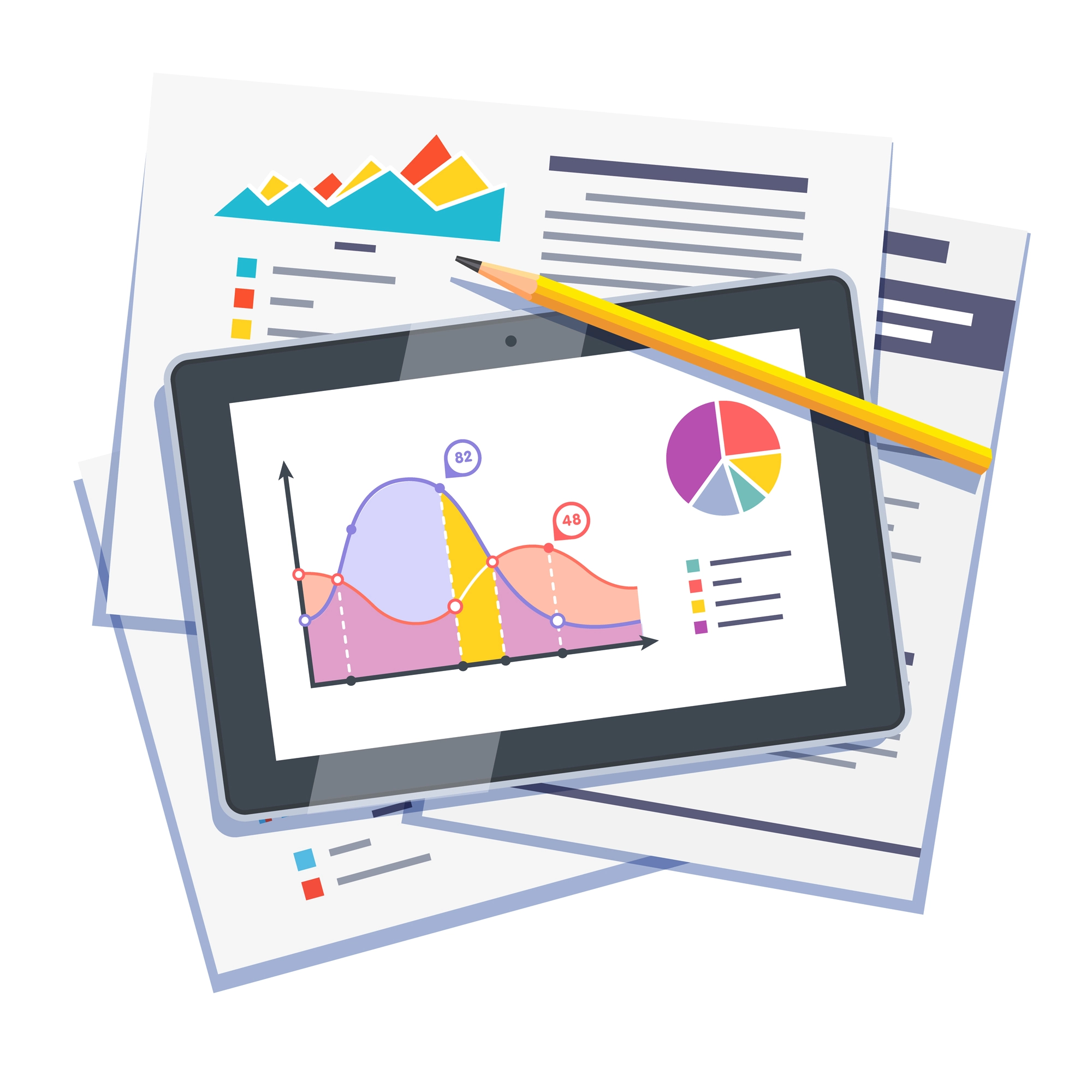
How to Increase Reliability?
- Use an appropriate questionnaire to measure the competency level.
- Ensure a consistent environment for participants
- Make the participants familiar with the criteria of assessment.
- Train the participants appropriately.
- Analyse the research items regularly to avoid poor performance.
How to Increase Validity?
Ensuring Validity is also not an easy job. A proper functioning method to ensure validity is given below:
- The reactivity should be minimised at the first concern.
- The Hawthorne effect should be reduced.
- The respondents should be motivated.
- The intervals between the pre-test and post-test should not be lengthy.
- Dropout rates should be avoided.
- The inter-rater reliability should be ensured.
- Control and experimental groups should be matched with each other.
How to Implement Reliability and Validity in your Thesis?
According to the experts, it is helpful if to implement the concept of reliability and Validity. Especially, in the thesis and the dissertation, these concepts are adopted much. The method for implementation given below:
Frequently Asked Questions
What is reliability and validity in research.
Reliability in research refers to the consistency and stability of measurements or findings. Validity relates to the accuracy and truthfulness of results, measuring what the study intends to. Both are crucial for trustworthy and credible research outcomes.
What is validity?
Validity in research refers to the extent to which a study accurately measures what it intends to measure. It ensures that the results are truly representative of the phenomena under investigation. Without validity, research findings may be irrelevant, misleading, or incorrect, limiting their applicability and credibility.
What is reliability?
Reliability in research refers to the consistency and stability of measurements over time. If a study is reliable, repeating the experiment or test under the same conditions should produce similar results. Without reliability, findings become unpredictable and lack dependability, potentially undermining the study’s credibility and generalisability.
What is reliability in psychology?
In psychology, reliability refers to the consistency of a measurement tool or test. A reliable psychological assessment produces stable and consistent results across different times, situations, or raters. It ensures that an instrument’s scores are not due to random error, making the findings dependable and reproducible in similar conditions.
What is test retest reliability?
Test-retest reliability assesses the consistency of measurements taken by a test over time. It involves administering the same test to the same participants at two different points in time and comparing the results. A high correlation between the scores indicates that the test produces stable and consistent results over time.
How to improve reliability of an experiment?
- Standardise procedures and instructions.
- Use consistent and precise measurement tools.
- Train observers or raters to reduce subjective judgments.
- Increase sample size to reduce random errors.
- Conduct pilot studies to refine methods.
- Repeat measurements or use multiple methods.
- Address potential sources of variability.
What is the difference between reliability and validity?
Reliability refers to the consistency and repeatability of measurements, ensuring results are stable over time. Validity indicates how well an instrument measures what it’s intended to measure, ensuring accuracy and relevance. While a test can be reliable without being valid, a valid test must inherently be reliable. Both are essential for credible research.
Are interviews reliable and valid?
Interviews can be both reliable and valid, but they are susceptible to biases. The reliability and validity depend on the design, structure, and execution of the interview. Structured interviews with standardised questions improve reliability. Validity is enhanced when questions accurately capture the intended construct and when interviewer biases are minimised.
Are IQ tests valid and reliable?
IQ tests are generally considered reliable, producing consistent scores over time. Their validity, however, is a subject of debate. While they effectively measure certain cognitive skills, whether they capture the entirety of “intelligence” or predict success in all life areas is contested. Cultural bias and over-reliance on tests are also concerns.
Are questionnaires reliable and valid?
Questionnaires can be both reliable and valid if well-designed. Reliability is achieved when they produce consistent results over time or across similar populations. Validity is ensured when questions accurately measure the intended construct. However, factors like poorly phrased questions, respondent bias, and lack of standardisation can compromise their reliability and validity.
You May Also Like
What are the different research strategies you can use in your dissertation? Here are some guidelines to help you choose a research strategy that would make your research more credible.
Sampling methods are used to to draw valid conclusions about a large community, organization or group of people, but they are based on evidence and reasoning.
A hypothesis is a research question that has to be proved correct or incorrect through hypothesis testing – a scientific approach to test a hypothesis.
USEFUL LINKS
LEARNING RESOURCES
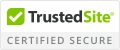
COMPANY DETAILS
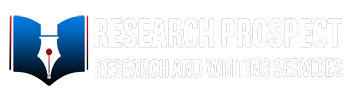
- How It Works
Have a language expert improve your writing
Run a free plagiarism check in 10 minutes, automatically generate references for free.
- Knowledge Base
- Methodology
- Reliability vs Validity in Research | Differences, Types & Examples
Reliability vs Validity in Research | Differences, Types & Examples
Published on 3 May 2022 by Fiona Middleton . Revised on 10 October 2022.
Reliability and validity are concepts used to evaluate the quality of research. They indicate how well a method , technique, or test measures something. Reliability is about the consistency of a measure, and validity is about the accuracy of a measure.
It’s important to consider reliability and validity when you are creating your research design , planning your methods, and writing up your results, especially in quantitative research .
Table of contents
Understanding reliability vs validity, how are reliability and validity assessed, how to ensure validity and reliability in your research, where to write about reliability and validity in a thesis.
Reliability and validity are closely related, but they mean different things. A measurement can be reliable without being valid. However, if a measurement is valid, it is usually also reliable.
What is reliability?
Reliability refers to how consistently a method measures something. If the same result can be consistently achieved by using the same methods under the same circumstances, the measurement is considered reliable.
What is validity?
Validity refers to how accurately a method measures what it is intended to measure. If research has high validity, that means it produces results that correspond to real properties, characteristics, and variations in the physical or social world.
High reliability is one indicator that a measurement is valid. If a method is not reliable, it probably isn’t valid.
However, reliability on its own is not enough to ensure validity. Even if a test is reliable, it may not accurately reflect the real situation.
Validity is harder to assess than reliability, but it is even more important. To obtain useful results, the methods you use to collect your data must be valid: the research must be measuring what it claims to measure. This ensures that your discussion of the data and the conclusions you draw are also valid.
Prevent plagiarism, run a free check.
Reliability can be estimated by comparing different versions of the same measurement. Validity is harder to assess, but it can be estimated by comparing the results to other relevant data or theory. Methods of estimating reliability and validity are usually split up into different types.
Types of reliability
Different types of reliability can be estimated through various statistical methods.
Types of validity
The validity of a measurement can be estimated based on three main types of evidence. Each type can be evaluated through expert judgement or statistical methods.
To assess the validity of a cause-and-effect relationship, you also need to consider internal validity (the design of the experiment ) and external validity (the generalisability of the results).
The reliability and validity of your results depends on creating a strong research design , choosing appropriate methods and samples, and conducting the research carefully and consistently.
Ensuring validity
If you use scores or ratings to measure variations in something (such as psychological traits, levels of ability, or physical properties), it’s important that your results reflect the real variations as accurately as possible. Validity should be considered in the very earliest stages of your research, when you decide how you will collect your data .
- Choose appropriate methods of measurement
Ensure that your method and measurement technique are of high quality and targeted to measure exactly what you want to know. They should be thoroughly researched and based on existing knowledge.
For example, to collect data on a personality trait, you could use a standardised questionnaire that is considered reliable and valid. If you develop your own questionnaire, it should be based on established theory or the findings of previous studies, and the questions should be carefully and precisely worded.
- Use appropriate sampling methods to select your subjects
To produce valid generalisable results, clearly define the population you are researching (e.g., people from a specific age range, geographical location, or profession). Ensure that you have enough participants and that they are representative of the population.
Ensuring reliability
Reliability should be considered throughout the data collection process. When you use a tool or technique to collect data, it’s important that the results are precise, stable, and reproducible.
- Apply your methods consistently
Plan your method carefully to make sure you carry out the same steps in the same way for each measurement. This is especially important if multiple researchers are involved.
For example, if you are conducting interviews or observations, clearly define how specific behaviours or responses will be counted, and make sure questions are phrased the same way each time.
- Standardise the conditions of your research
When you collect your data, keep the circumstances as consistent as possible to reduce the influence of external factors that might create variation in the results.
For example, in an experimental setup, make sure all participants are given the same information and tested under the same conditions.
It’s appropriate to discuss reliability and validity in various sections of your thesis or dissertation or research paper. Showing that you have taken them into account in planning your research and interpreting the results makes your work more credible and trustworthy.
Cite this Scribbr article
If you want to cite this source, you can copy and paste the citation or click the ‘Cite this Scribbr article’ button to automatically add the citation to our free Reference Generator.
Middleton, F. (2022, October 10). Reliability vs Validity in Research | Differences, Types & Examples. Scribbr. Retrieved 31 May 2024, from https://www.scribbr.co.uk/research-methods/reliability-or-validity/
Is this article helpful?
Fiona Middleton
Other students also liked, the 4 types of validity | types, definitions & examples, a quick guide to experimental design | 5 steps & examples, sampling methods | types, techniques, & examples.
- Skip to main content
- Skip to primary sidebar
- Skip to footer
- QuestionPro

- Solutions Industries Gaming Automotive Sports and events Education Government Travel & Hospitality Financial Services Healthcare Cannabis Technology Use Case NPS+ Communities Audience Contactless surveys Mobile LivePolls Member Experience GDPR Positive People Science 360 Feedback Surveys
- Resources Blog eBooks Survey Templates Case Studies Training Help center

Home Market Research
Reliability vs. Validity in Research: Types & Examples
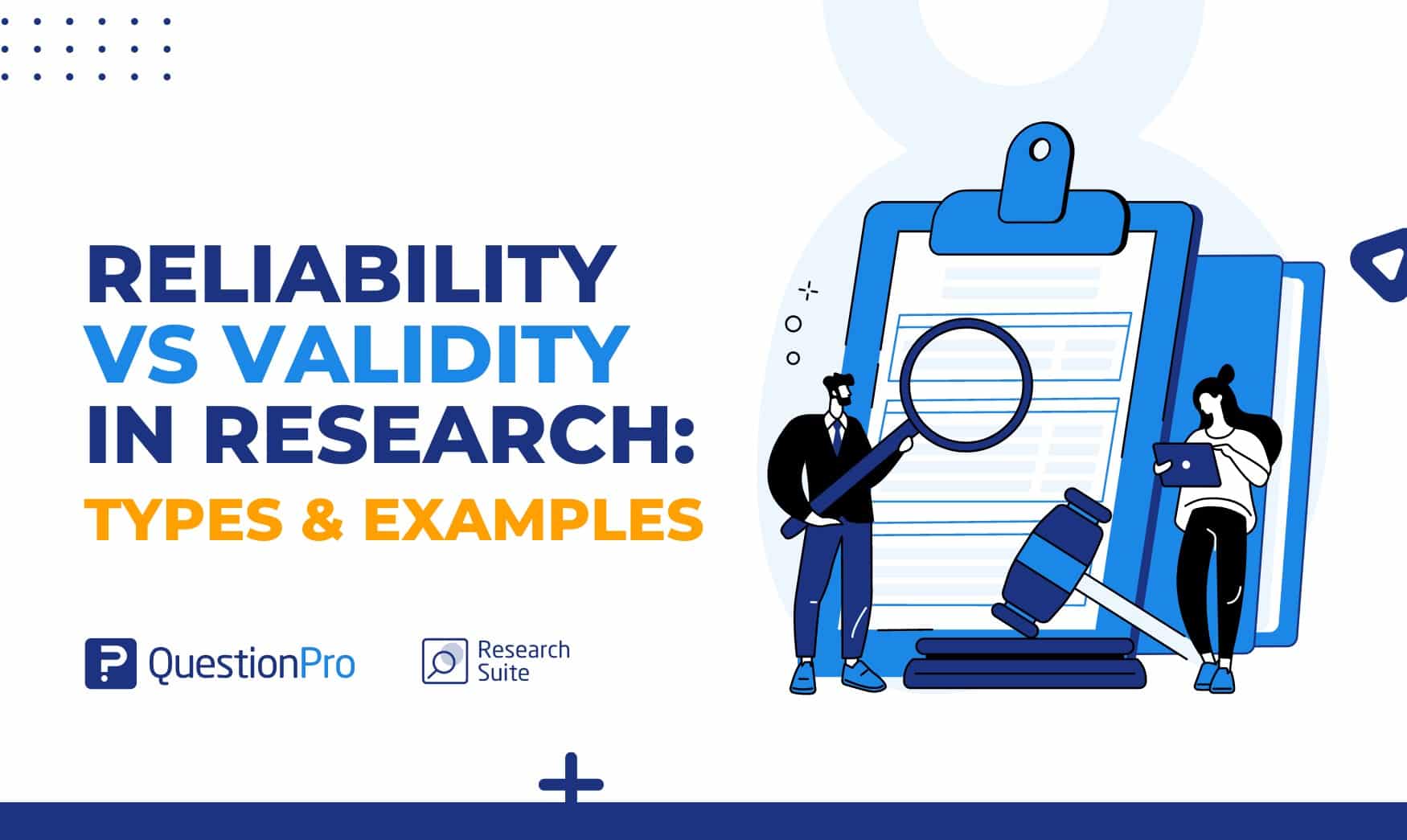
When it comes to research, getting things right is crucial. That’s where the concepts of “Reliability vs Validity in Research” come in.
Imagine it like a balancing act – making sure your measurements are consistent and accurate at the same time. This is where test-retest reliability, having different researchers check things, and keeping things consistent within your research plays a big role.
As we dive into this topic, we’ll uncover the differences between reliability and validity, see how they work together, and learn how to use them effectively.
Understanding Reliability vs. Validity in Research
When it comes to collecting data and conducting research, two crucial concepts stand out: reliability and validity.
These pillars uphold the integrity of research findings, ensuring that the data collected and the conclusions drawn are both meaningful and trustworthy. Let’s dive into the heart of the concepts, reliability, and validity, to comprehend their significance in the realm of research truly.
What is reliability?
Reliability refers to the consistency and dependability of the data collection process. It’s like having a steady hand that produces the same result each time it reaches for a task.
In the research context, reliability is all about ensuring that if you were to repeat the same study using the same reliable measurement technique, you’d end up with the same results. It’s like having multiple researchers independently conduct the same experiment and getting outcomes that align perfectly.
Imagine you’re using a thermometer to measure the temperature of the water. You have a reliable measurement if you dip the thermometer into the water multiple times and get the same reading each time. This tells you that your method and measurement technique consistently produce the same results, whether it’s you or another researcher performing the measurement.
What is validity?
On the other hand, validity refers to the accuracy and meaningfulness of your data. It’s like ensuring that the puzzle pieces you’re putting together actually form the intended picture. When you have validity, you know that your method and measurement technique are consistent and capable of producing results aligned with reality.
Think of it this way; Imagine you’re conducting a test that claims to measure a specific trait, like problem-solving ability. If the test consistently produces results that accurately reflect participants’ problem-solving skills, then the test has high validity. In this case, the test produces accurate results that truly correspond to the trait it aims to measure.
In essence, while reliability assures you that your data collection process is like a well-oiled machine producing the same results, validity steps in to ensure that these results are not only consistent but also relevantly accurate.
Together, these concepts provide researchers with the tools to conduct research that stands on a solid foundation of dependable methods and meaningful insights.
Types of Reliability
Let’s explore the various types of reliability that researchers consider to ensure their work stands on solid ground.
High test-retest reliability
Test-retest reliability involves assessing the consistency of measurements over time. It’s like taking the same measurement or test twice – once and then again after a certain period. If the results align closely, it indicates that the measurement is reliable over time. Think of it as capturing the essence of stability.
Inter-rater reliability
When multiple researchers or observers are part of the equation, interrater reliability comes into play. This type of reliability assesses the level of agreement between different observers when evaluating the same phenomenon. It’s like ensuring that different pairs of eyes perceive things in a similar way.
Internal reliability
Internal consistency dives into the harmony among different items within a measurement tool aiming to assess the same concept. This often comes into play in surveys or questionnaires, where participants respond to various items related to a single construct. If the responses to these items consistently reflect the same underlying concept, the measurement is said to have high internal consistency.
Types of validity
Let’s explore the various types of validity that researchers consider to ensure their work stands on solid ground.
Content validity
It delves into whether a measurement truly captures all dimensions of the concept it intends to measure. It’s about making sure your measurement tool covers all relevant aspects comprehensively.
Imagine designing a test to assess students’ understanding of a history chapter. It exhibits high content validity if the test includes questions about key events, dates, and causes. However, if it focuses solely on dates and omits causation, its content validity might be questionable.
Construct validity
It assesses how well a measurement aligns with established theories and concepts. It’s like ensuring that your measurement is a true representation of the abstract construct you’re trying to capture.
Criterion validity
Criterion validity examines how well your measurement corresponds to other established measurements of the same concept. It’s about making sure your measurement accurately predicts or correlates with external criteria.
Differences between reliability and validity in research
Let’s delve into the differences between reliability and validity in research.
While both reliability and validity contribute to trustworthy research, they address distinct aspects. Reliability ensures consistent results, while validity ensures accurate and relevant results that reflect the true nature of the measured concept.
Example of Reliability and Validity in Research
In this section, we’ll explore instances that highlight the differences between reliability and validity and how they play a crucial role in ensuring the credibility of research findings.
Example of reliability
Imagine you are studying the reliability of a smartphone’s battery life measurement. To collect data, you fully charge the phone and measure the battery life three times in the same controlled environment—same apps running, same brightness level, and same usage patterns.
If the measurements consistently show a similar battery life duration each time you repeat the test, it indicates that your measurement method is reliable. The consistent results under the same conditions assure you that the battery life measurement can be trusted to provide dependable information about the phone’s performance.
Example of validity
Researchers collect data from a group of participants in a study aiming to assess the validity of a newly developed stress questionnaire. To ensure validity, they compare the scores obtained from the stress questionnaire with the participants’ actual stress levels measured using physiological indicators such as heart rate variability and cortisol levels.
If participants’ scores correlate strongly with their physiological stress levels, the questionnaire is valid. This means the questionnaire accurately measures participants’ stress levels, and its results correspond to real variations in their physiological responses to stress.
Validity assessed through the correlation between questionnaire scores and physiological measures ensures that the questionnaire is effectively measuring what it claims to measure participants’ stress levels.
In the world of research, differentiating between reliability and validity is crucial. Reliability ensures consistent results, while validity confirms accurate measurements. Using tools like QuestionPro enhances data collection for both reliability and validity. For instance, measuring self-esteem over time showcases reliability, and aligning questions with theories demonstrates validity.
QuestionPro empowers researchers to achieve reliable and valid results through its robust features, facilitating credible research outcomes. Contact QuestionPro to create a free account or learn more!
LEARN MORE FREE TRIAL
MORE LIKE THIS
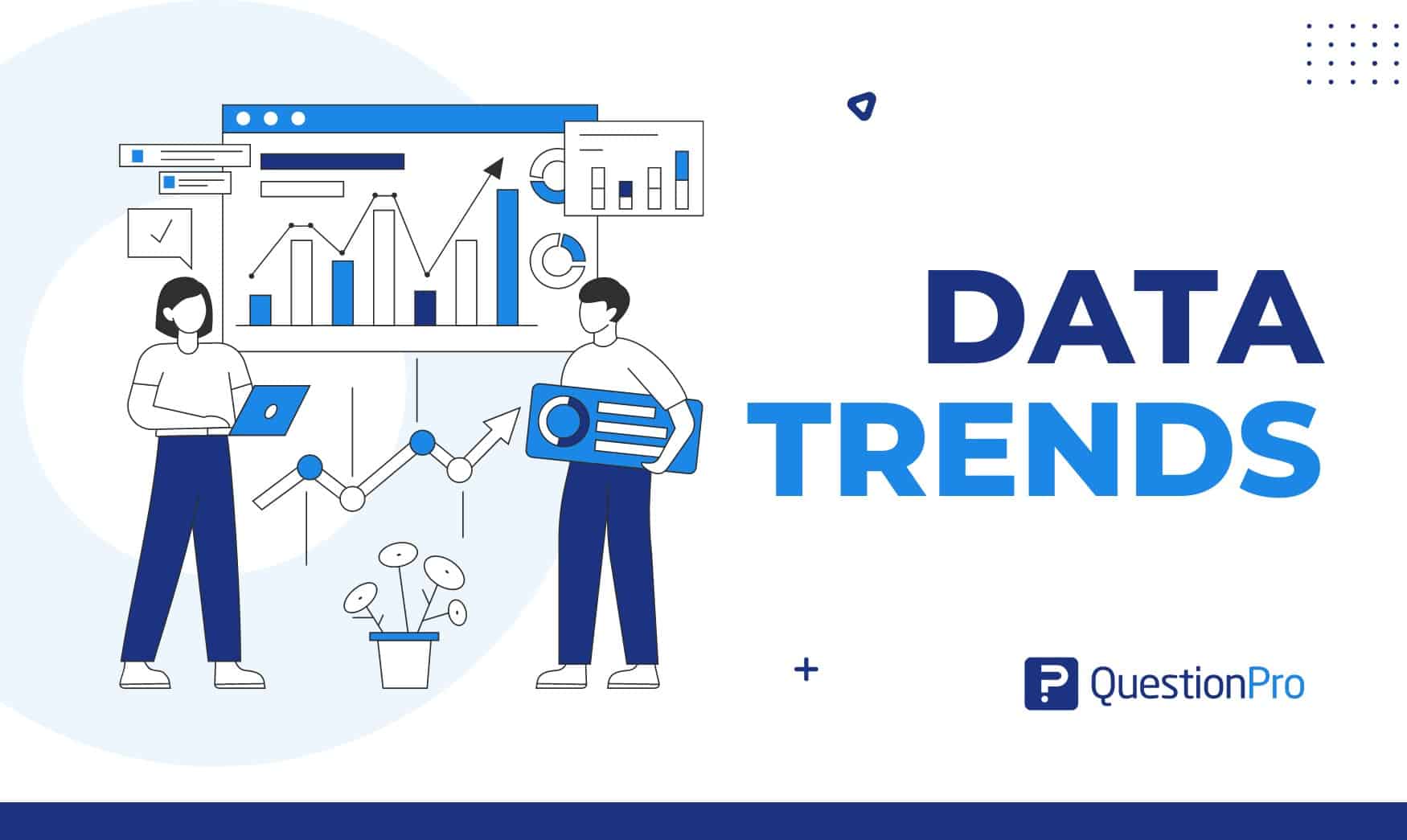
Top 8 Data Trends to Understand the Future of Data
May 30, 2024
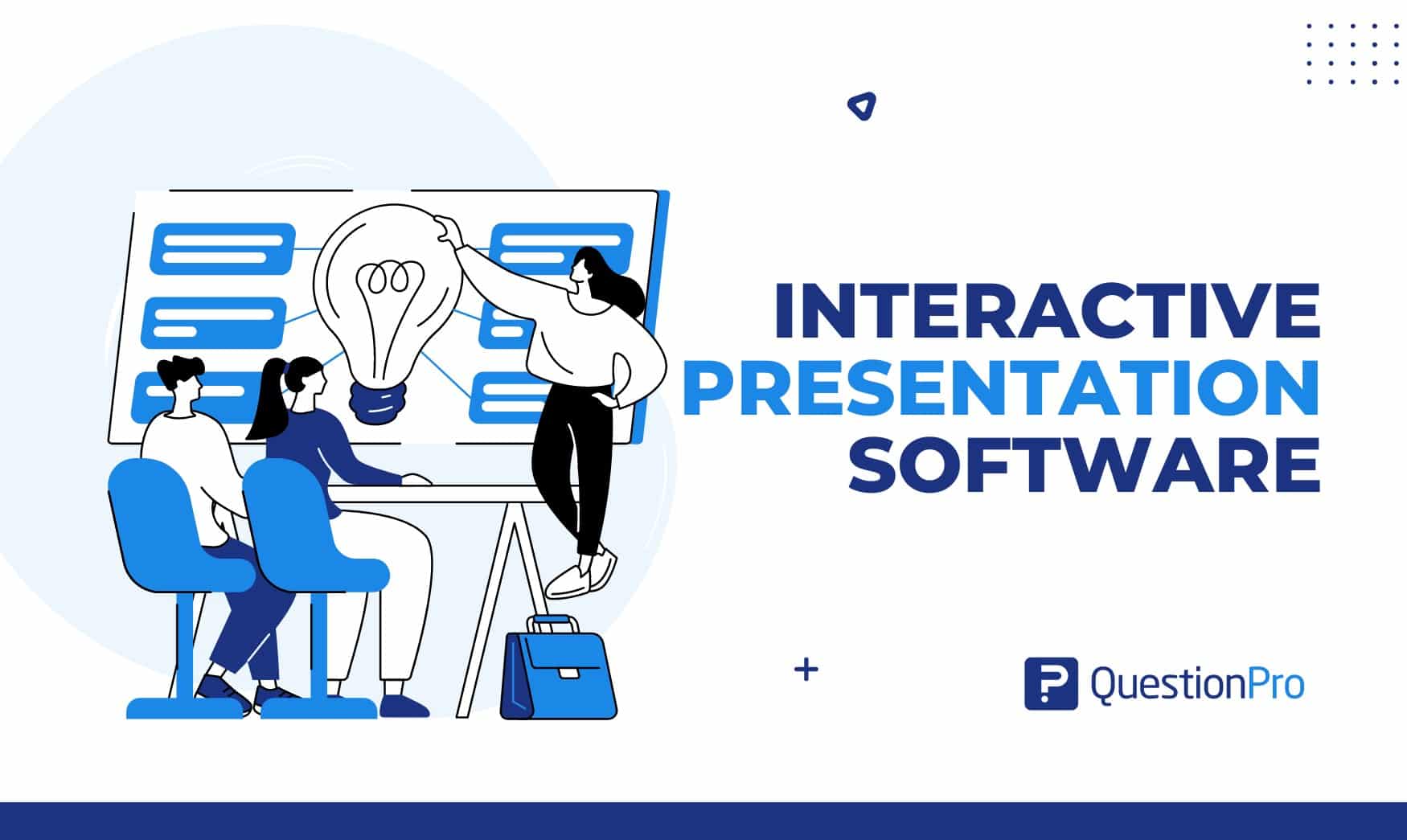
Top 12 Interactive Presentation Software to Engage Your User
May 29, 2024
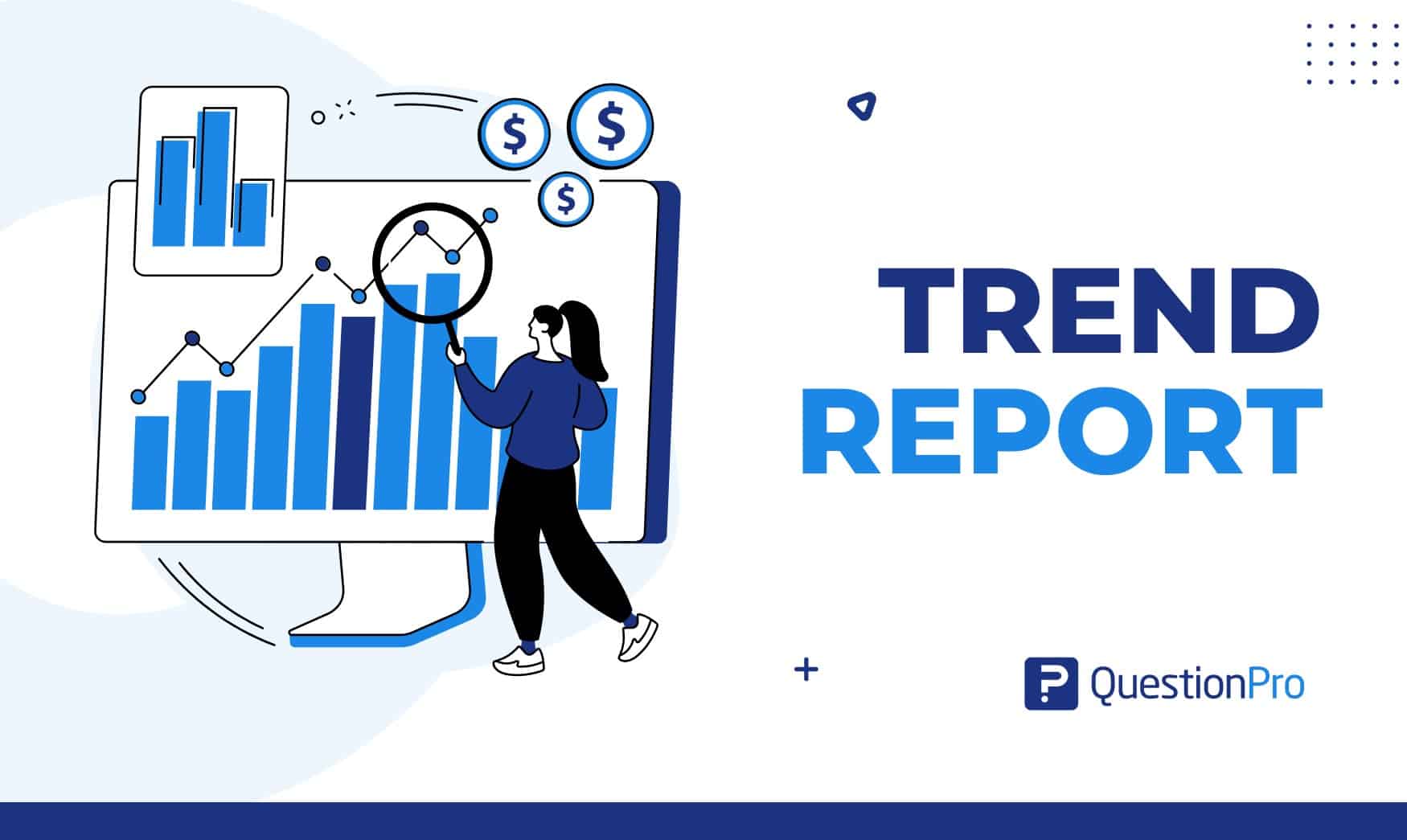
Trend Report: Guide for Market Dynamics & Strategic Analysis
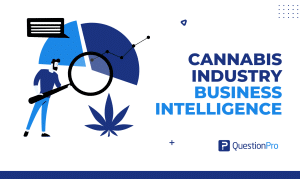
Cannabis Industry Business Intelligence: Impact on Research
May 28, 2024
Other categories
- Academic Research
- Artificial Intelligence
- Assessments
- Brand Awareness
- Case Studies
- Communities
- Consumer Insights
- Customer effort score
- Customer Engagement
- Customer Experience
- Customer Loyalty
- Customer Research
- Customer Satisfaction
- Employee Benefits
- Employee Engagement
- Employee Retention
- Friday Five
- General Data Protection Regulation
- Insights Hub
- Life@QuestionPro
- Market Research
- Mobile diaries
- Mobile Surveys
- New Features
- Online Communities
- Question Types
- Questionnaire
- QuestionPro Products
- Release Notes
- Research Tools and Apps
- Revenue at Risk
- Survey Templates
- Training Tips
- Uncategorized
- Video Learning Series
- What’s Coming Up
- Workforce Intelligence
- Reliability vs Validity in Research: Types & Examples

In everyday life, we probably use reliability to describe how something is valid. However, in research and testing, reliability and validity are not the same things.
When it comes to data analysis, reliability refers to how easily replicable an outcome is. For example, if you measure a cup of rice three times, and you get the same result each time, that result is reliable.
The validity, on the other hand, refers to the measurement’s accuracy. This means that if the standard weight for a cup of rice is 5 grams, and you measure a cup of rice, it should be 5 grams.
So, while reliability and validity are intertwined, they are not synonymous. If one of the measurement parameters, such as your scale, is distorted, the results will be consistent but invalid.
Data must be consistent and accurate to be used to draw useful conclusions. In this article, we’ll look at how to assess data reliability and validity, as well as how to apply it.
Read: Internal Validity in Research: Definition, Threats, Examples
What is Reliability?
When a measurement is consistent it’s reliable. But of course, reliability doesn’t mean your outcome will be the same, it just means it will be in the same range.
For example, if you scored 95% on a test the first time and the next you score, 96%, your results are reliable. So, even if there is a minor difference in the outcomes, as long as it is within the error margin, your results are reliable.
Reliability allows you to assess the degree of consistency in your results. So, if you’re getting similar results, reliability provides an answer to the question of how similar your results are.
What is Validity?
A measurement or test is valid when it correlates with the expected result. It examines the accuracy of your result.
Here’s where things get tricky: to establish the validity of a test, the results must be consistent. Looking at most experiments (especially physical measurements), the standard value that establishes the accuracy of a measurement is the outcome of repeating the test to obtain a consistent result.
Read: What is Participant Bias? How to Detect & Avoid It
For example, before I can conclude that all 12-inch rulers are one foot, I must repeat the experiment several times and obtain very similar results, indicating that 12-inch rulers are indeed one foot.
Most scientific experiments are inextricably linked in terms of validity and reliability. For example, if you’re measuring distance or depth, valid answers are likely to be reliable.
But for social experiences, one isn’t the indication of the other. For example, most people believe that people that wear glasses are smart.
Of course, I’ll find examples of people who wear glasses and have high IQs (reliability), but the truth is that most people who wear glasses simply need their vision to be better (validity).
So reliable answers aren’t always correct but valid answers are always reliable.
How Are Reliability and Validity Assessed?
When assessing reliability, we want to know if the measurement can be replicated. Of course, we’d have to change some variables to ensure that this test holds, the most important of which are time, items, and observers.
If the main factor you change when performing a reliability test is time, you’re performing a test-retest reliability assessment.
Read: What is Publication Bias? (How to Detect & Avoid It)
However, if you are changing items, you are performing an internal consistency assessment. It means you’re measuring multiple items with a single instrument.
Finally, if you’re measuring the same item with the same instrument but using different observers or judges, you’re performing an inter-rater reliability test.
Assessing Validity
Evaluating validity can be more tedious than reliability. With reliability, you’re attempting to demonstrate that your results are consistent, whereas, with validity, you want to prove the correctness of your outcome.
Although validity is mainly categorized under two sections (internal and external), there are more than fifteen ways to check the validity of a test. In this article, we’ll be covering four.
First, content validity, measures whether the test covers all the content it needs to provide the outcome you’re expecting.
Suppose I wanted to test the hypothesis that 90% of Generation Z uses social media polls for surveys while 90% of millennials use forms. I’d need a sample size that accounts for how Gen Z and millennials gather information.
Next, criterion validity is when you compare your results to what you’re supposed to get based on a chosen criteria. There are two ways these could be measured, predictive or concurrent validity.
Read: Survey Errors To Avoid: Types, Sources, Examples, Mitigation
Following that, we have face validity . It’s how we anticipate a test to be. For instance, when answering a customer service survey, I’d expect to be asked about how I feel about the service provided.
Lastly, construct-related validity . This is a little more complicated, but it helps to show how the validity of research is based on different findings.
As a result, it provides information that either proves or disproves that certain things are related.
Types of Reliability
We have three main types of reliability assessment and here’s how they work:
1) Test-retest Reliability
This assessment refers to the consistency of outcomes over time. Testing reliability over time does not imply changing the amount of time it takes to conduct an experiment; rather, it means repeating the experiment multiple times in a short time.
For example, if I measure the length of my hair today, and tomorrow, I’ll most likely get the same result each time.
A short period is relative in terms of reliability; two days for measuring hair length is considered short. But that’s far too long to test how quickly water dries on the sand.
A test-retest correlation is used to compare the consistency of your results. This is typically a scatter plot that shows how similar your values are between the two experiments.
If your answers are reliable, your scatter plots will most likely have a lot of overlapping points, but if they aren’t, the points (values) will be spread across the graph.
Read: Sampling Bias: Definition, Types + [Examples]
2) Internal Consistency
It’s also known as internal reliability. It refers to the consistency of results for various items when measured on the same scale.
This is particularly important in social science research, such as surveys, because it helps determine the consistency of people’s responses when asked the same questions.
Most introverts, for example, would say they enjoy spending time alone and having few friends. However, if some introverts claim that they either do not want time alone or prefer to be surrounded by many friends, it doesn’t add up.
These people who claim to be introverts or one this factor isn’t a reliable way of measuring introversion.
Internal reliability helps you prove the consistency of a test by varying factors. It’s a little tough to measure quantitatively but you could use the split-half correlation .
The split-half correlation simply means dividing the factors used to measure the underlying construct into two and plotting them against each other in the form of a scatter plot.
Introverts, for example, are assessed on their need for alone time as well as their desire to have as few friends as possible. If this plot is dispersed, likely, one of the traits does not indicate introversion.
3) Inter-Rater Reliability
This method of measuring reliability helps prevent personal bias. Inter-rater reliability assessment helps judge outcomes from the different perspectives of multiple observers.
A good example is if you ordered a meal and found it delicious. You could be biased in your judgment for several reasons, perception of the meal, your mood, and so on.
But it’s highly unlikely that six more people would agree that the meal is delicious if it isn’t. Another factor that could lead to bias is expertise. Professional dancers, for example, would perceive dance moves differently than non-professionals.
Read: What is Experimenter Bias? Definition, Types & Mitigation
So, if a person dances and records it, and both groups (professional and unprofessional dancers) rate the video, there is a high likelihood of a significant difference in their ratings.
But if they both agree that the person is a great dancer, despite their opposing viewpoints, the person is likely a great dancer.
Types of Validity
Researchers use validity to determine whether a measurement is accurate or not. The accuracy of measurement is usually determined by comparing it to the standard value.
When a measurement is consistent over time and has high internal consistency, it increases the likelihood that it is valid.
1) Content Validity
This refers to determining validity by evaluating what is being measured. So content validity tests if your research is measuring everything it should to produce an accurate result.
For example, if I were to measure what causes hair loss in women. I’d have to consider things like postpartum hair loss, alopecia, hair manipulation, dryness, and so on.
By omitting any of these critical factors, you risk significantly reducing the validity of your research because you won’t be covering everything necessary to make an accurate deduction.
Read: Data Cleaning: 7 Techniques + Steps to Cleanse Data
For example, a certain woman is losing her hair due to postpartum hair loss, excessive manipulation, and dryness, but in my research, I only look at postpartum hair loss. My research will show that she has postpartum hair loss, which isn’t accurate.
Yes, my conclusion is correct, but it does not fully account for the reasons why this woman is losing her hair.
2) Criterion Validity
This measures how well your measurement correlates with the variables you want to compare it with to get your result. The two main classes of criterion validity are predictive and concurrent.
3) Predictive validity
It helps predict future outcomes based on the data you have. For example, if a large number of students performed exceptionally well in a test, you can use this to predict that they understood the concept on which the test was based and will perform well in their exams.
4) Concurrent validity
On the other hand, involves testing with different variables at the same time. For example, setting up a literature test for your students on two different books and assessing them at the same time.
You’re measuring your students’ literature proficiency with these two books. If your students truly understood the subject, they should be able to correctly answer questions about both books.
5) Face Validity
Quantifying face validity might be a bit difficult because you are measuring the perception validity, not the validity itself. So, face validity is concerned with whether the method used for measurement will produce accurate results rather than the measurement itself.
If the method used for measurement doesn’t appear to test the accuracy of a measurement, its face validity is low.
Here’s an example: less than 40% of men over the age of 20 in Texas, USA, are at least 6 feet tall. The most logical approach would be to collect height data from men over the age of twenty in Texas, USA.
However, asking men over the age of 20 what their favorite meal is to determine their height is pretty bizarre. The method I am using to assess the validity of my research is quite questionable because it lacks correlation to what I want to measure.
6) Construct-Related Validity
Construct-related validity assesses the accuracy of your research by collecting multiple pieces of evidence. It helps determine the validity of your results by comparing them to evidence that supports or refutes your measurement.
7) Convergent validity
If you’re assessing evidence that strongly correlates with the concept, that’s convergent validity .
8) Discriminant validity
Examines the validity of your research by determining what not to base it on. You are removing elements that are not a strong factor to help validate your research. Being a vegan, for example, does not imply that you are allergic to meat.
How to Ensure Validity and Reliability in Your Research
You need a bulletproof research design to ensure that your research is both valid and reliable. This means that your methods, sample, and even you, the researcher, shouldn’t be biased.
- Ensuring Reliability
To enhance the reliability of your research, you need to apply your measurement method consistently. The chances of reproducing the same results for a test are higher when you maintain the method you’re using to experiment.
For example, you want to determine the reliability of the weight of a bag of chips using a scale. You have to consistently use this scale to measure the bag of chips each time you experiment.
You must also keep the conditions of your research consistent. For instance, if you’re experimenting to see how quickly water dries on sand, you need to consider all of the weather elements that day.
So, if you experimented on a sunny day, the next experiment should also be conducted on a sunny day to obtain a reliable result.
Read: Survey Methods: Definition, Types, and Examples
- Ensuring Validity
There are several ways to determine the validity of your research, and the majority of them require the use of highly specific and high-quality measurement methods.
Before you begin your test, choose the best method for producing the desired results. This method should be pre-existing and proven.
Also, your sample should be very specific. If you’re collecting data on how dogs respond to fear, your results are more likely to be valid if you base them on a specific breed of dog rather than dogs in general.
Validity and reliability are critical for achieving accurate and consistent results in research. While reliability does not always imply validity, validity establishes that a result is reliable. Validity is heavily dependent on previous results (standards), whereas reliability is dependent on the similarity of your results.
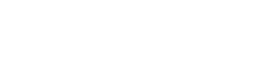
Connect to Formplus, Get Started Now - It's Free!
- concurrent validity
- examples of research bias
- predictive reliability
- research analysis
- research assessment
- validity of research
- busayo.longe
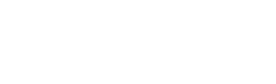
You may also like:
How to do a Meta Analysis: Methodology, Pros & Cons
In this article, we’ll go through the concept of meta-analysis, what it can be used for, and how you can use it to improve how you...

Selection Bias in Research: Types, Examples & Impact
In this article, we’ll discuss the effects of selection bias, how it works, its common effects and the best ways to minimize it.
Research Bias: Definition, Types + Examples
Simple guide to understanding research bias, types, causes, examples and how to avoid it in surveys
Simpson’s Paradox & How to Avoid it in Experimental Research
In this article, we are going to look at Simpson’s Paradox from its historical point and later, we’ll consider its effect in...
Formplus - For Seamless Data Collection
Collect data the right way with a versatile data collection tool. try formplus and transform your work productivity today..
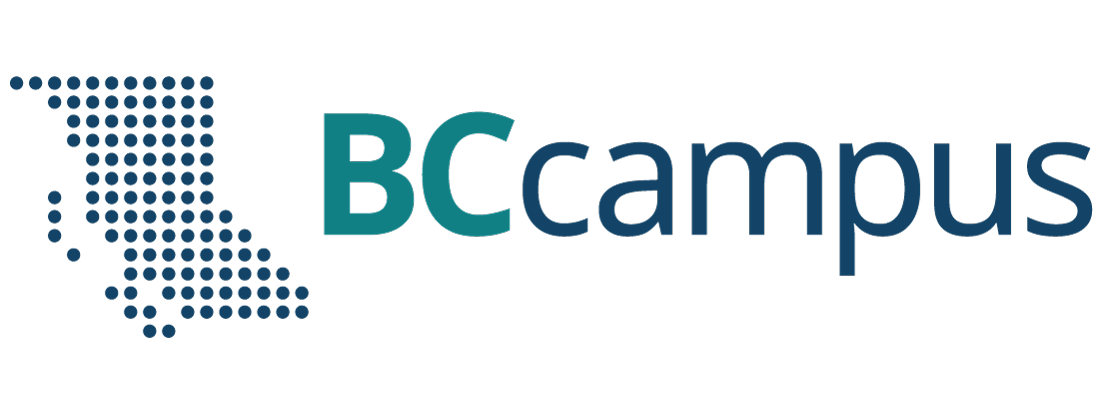
Want to create or adapt books like this? Learn more about how Pressbooks supports open publishing practices.
Chapter 5: Psychological Measurement
Reliability and Validity of Measurement
Learning Objectives
- Define reliability, including the different types and how they are assessed.
- Define validity, including the different types and how they are assessed.
- Describe the kinds of evidence that would be relevant to assessing the reliability and validity of a particular measure.
Again, measurement involves assigning scores to individuals so that they represent some characteristic of the individuals. But how do researchers know that the scores actually represent the characteristic, especially when it is a construct like intelligence, self-esteem, depression, or working memory capacity? The answer is that they conduct research using the measure to confirm that the scores make sense based on their understanding of the construct being measured. This is an extremely important point. Psychologists do not simply assume that their measures work. Instead, they collect data to demonstrate that they work. If their research does not demonstrate that a measure works, they stop using it.
As an informal example, imagine that you have been dieting for a month. Your clothes seem to be fitting more loosely, and several friends have asked if you have lost weight. If at this point your bathroom scale indicated that you had lost 10 pounds, this would make sense and you would continue to use the scale. But if it indicated that you had gained 10 pounds, you would rightly conclude that it was broken and either fix it or get rid of it. In evaluating a measurement method, psychologists consider two general dimensions: reliability and validity.
Reliability
Reliability refers to the consistency of a measure. Psychologists consider three types of consistency: over time (test-retest reliability), across items (internal consistency), and across different researchers (inter-rater reliability).
Test-Retest Reliability
When researchers measure a construct that they assume to be consistent across time, then the scores they obtain should also be consistent across time. Test-retest reliability is the extent to which this is actually the case. For example, intelligence is generally thought to be consistent across time. A person who is highly intelligent today will be highly intelligent next week. This means that any good measure of intelligence should produce roughly the same scores for this individual next week as it does today. Clearly, a measure that produces highly inconsistent scores over time cannot be a very good measure of a construct that is supposed to be consistent.
Assessing test-retest reliability requires using the measure on a group of people at one time, using it again on the same group of people at a later time, and then looking at test-retest correlation between the two sets of scores. This is typically done by graphing the data in a scatterplot and computing Pearson’s r . Figure 5.2 shows the correlation between two sets of scores of several university students on the Rosenberg Self-Esteem Scale, administered two times, a week apart. Pearson’s r for these data is +.95. In general, a test-retest correlation of +.80 or greater is considered to indicate good reliability.
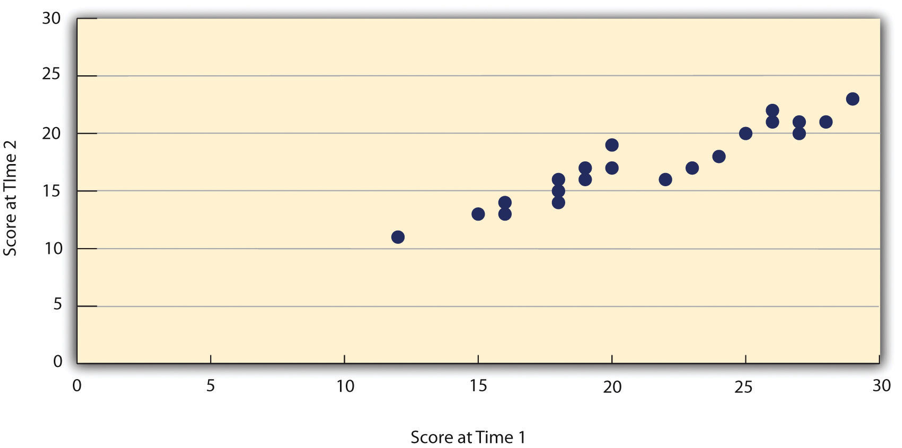
Again, high test-retest correlations make sense when the construct being measured is assumed to be consistent over time, which is the case for intelligence, self-esteem, and the Big Five personality dimensions. But other constructs are not assumed to be stable over time. The very nature of mood, for example, is that it changes. So a measure of mood that produced a low test-retest correlation over a period of a month would not be a cause for concern.
Internal Consistency
A second kind of reliability is internal consistency , which is the consistency of people’s responses across the items on a multiple-item measure. In general, all the items on such measures are supposed to reflect the same underlying construct, so people’s scores on those items should be correlated with each other. On the Rosenberg Self-Esteem Scale, people who agree that they are a person of worth should tend to agree that that they have a number of good qualities. If people’s responses to the different items are not correlated with each other, then it would no longer make sense to claim that they are all measuring the same underlying construct. This is as true for behavioural and physiological measures as for self-report measures. For example, people might make a series of bets in a simulated game of roulette as a measure of their level of risk seeking. This measure would be internally consistent to the extent that individual participants’ bets were consistently high or low across trials.
Like test-retest reliability, internal consistency can only be assessed by collecting and analyzing data. One approach is to look at a split-half correlation . This involves splitting the items into two sets, such as the first and second halves of the items or the even- and odd-numbered items. Then a score is computed for each set of items, and the relationship between the two sets of scores is examined. For example, Figure 5.3 shows the split-half correlation between several university students’ scores on the even-numbered items and their scores on the odd-numbered items of the Rosenberg Self-Esteem Scale. Pearson’s r for these data is +.88. A split-half correlation of +.80 or greater is generally considered good internal consistency.
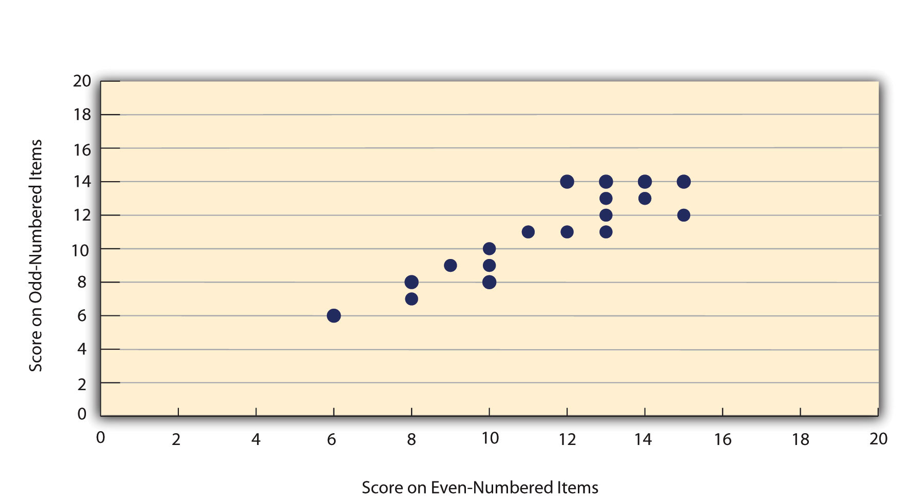
Perhaps the most common measure of internal consistency used by researchers in psychology is a statistic called Cronbach’s α (the Greek letter alpha). Conceptually, α is the mean of all possible split-half correlations for a set of items. For example, there are 252 ways to split a set of 10 items into two sets of five. Cronbach’s α would be the mean of the 252 split-half correlations. Note that this is not how α is actually computed, but it is a correct way of interpreting the meaning of this statistic. Again, a value of +.80 or greater is generally taken to indicate good internal consistency.
Interrater Reliability
Many behavioural measures involve significant judgment on the part of an observer or a rater. Inter-rater reliability is the extent to which different observers are consistent in their judgments. For example, if you were interested in measuring university students’ social skills, you could make video recordings of them as they interacted with another student whom they are meeting for the first time. Then you could have two or more observers watch the videos and rate each student’s level of social skills. To the extent that each participant does in fact have some level of social skills that can be detected by an attentive observer, different observers’ ratings should be highly correlated with each other. Inter-rater reliability would also have been measured in Bandura’s Bobo doll study. In this case, the observers’ ratings of how many acts of aggression a particular child committed while playing with the Bobo doll should have been highly positively correlated. Interrater reliability is often assessed using Cronbach’s α when the judgments are quantitative or an analogous statistic called Cohen’s κ (the Greek letter kappa) when they are categorical.
Validity is the extent to which the scores from a measure represent the variable they are intended to. But how do researchers make this judgment? We have already considered one factor that they take into account—reliability. When a measure has good test-retest reliability and internal consistency, researchers should be more confident that the scores represent what they are supposed to. There has to be more to it, however, because a measure can be extremely reliable but have no validity whatsoever. As an absurd example, imagine someone who believes that people’s index finger length reflects their self-esteem and therefore tries to measure self-esteem by holding a ruler up to people’s index fingers. Although this measure would have extremely good test-retest reliability, it would have absolutely no validity. The fact that one person’s index finger is a centimetre longer than another’s would indicate nothing about which one had higher self-esteem.
Discussions of validity usually divide it into several distinct “types.” But a good way to interpret these types is that they are other kinds of evidence—in addition to reliability—that should be taken into account when judging the validity of a measure. Here we consider three basic kinds: face validity, content validity, and criterion validity.
Face Validity
Face validity is the extent to which a measurement method appears “on its face” to measure the construct of interest. Most people would expect a self-esteem questionnaire to include items about whether they see themselves as a person of worth and whether they think they have good qualities. So a questionnaire that included these kinds of items would have good face validity. The finger-length method of measuring self-esteem, on the other hand, seems to have nothing to do with self-esteem and therefore has poor face validity. Although face validity can be assessed quantitatively—for example, by having a large sample of people rate a measure in terms of whether it appears to measure what it is intended to—it is usually assessed informally.
Face validity is at best a very weak kind of evidence that a measurement method is measuring what it is supposed to. One reason is that it is based on people’s intuitions about human behaviour, which are frequently wrong. It is also the case that many established measures in psychology work quite well despite lacking face validity. The Minnesota Multiphasic Personality Inventory-2 (MMPI-2) measures many personality characteristics and disorders by having people decide whether each of over 567 different statements applies to them—where many of the statements do not have any obvious relationship to the construct that they measure. For example, the items “I enjoy detective or mystery stories” and “The sight of blood doesn’t frighten me or make me sick” both measure the suppression of aggression. In this case, it is not the participants’ literal answers to these questions that are of interest, but rather whether the pattern of the participants’ responses to a series of questions matches those of individuals who tend to suppress their aggression.
Content Validity
Content validity is the extent to which a measure “covers” the construct of interest. For example, if a researcher conceptually defines test anxiety as involving both sympathetic nervous system activation (leading to nervous feelings) and negative thoughts, then his measure of test anxiety should include items about both nervous feelings and negative thoughts. Or consider that attitudes are usually defined as involving thoughts, feelings, and actions toward something. By this conceptual definition, a person has a positive attitude toward exercise to the extent that he or she thinks positive thoughts about exercising, feels good about exercising, and actually exercises. So to have good content validity, a measure of people’s attitudes toward exercise would have to reflect all three of these aspects. Like face validity, content validity is not usually assessed quantitatively. Instead, it is assessed by carefully checking the measurement method against the conceptual definition of the construct.
Criterion Validity
Criterion validity is the extent to which people’s scores on a measure are correlated with other variables (known as criteria ) that one would expect them to be correlated with. For example, people’s scores on a new measure of test anxiety should be negatively correlated with their performance on an important school exam. If it were found that people’s scores were in fact negatively correlated with their exam performance, then this would be a piece of evidence that these scores really represent people’s test anxiety. But if it were found that people scored equally well on the exam regardless of their test anxiety scores, then this would cast doubt on the validity of the measure.
A criterion can be any variable that one has reason to think should be correlated with the construct being measured, and there will usually be many of them. For example, one would expect test anxiety scores to be negatively correlated with exam performance and course grades and positively correlated with general anxiety and with blood pressure during an exam. Or imagine that a researcher develops a new measure of physical risk taking. People’s scores on this measure should be correlated with their participation in “extreme” activities such as snowboarding and rock climbing, the number of speeding tickets they have received, and even the number of broken bones they have had over the years. When the criterion is measured at the same time as the construct, criterion validity is referred to as concurrent validity ; however, when the criterion is measured at some point in the future (after the construct has been measured), it is referred to as predictive validity (because scores on the measure have “predicted” a future outcome).
Criteria can also include other measures of the same construct. For example, one would expect new measures of test anxiety or physical risk taking to be positively correlated with existing measures of the same constructs. This is known as convergent validity .
Assessing convergent validity requires collecting data using the measure. Researchers John Cacioppo and Richard Petty did this when they created their self-report Need for Cognition Scale to measure how much people value and engage in thinking (Cacioppo & Petty, 1982) [1] . In a series of studies, they showed that people’s scores were positively correlated with their scores on a standardized academic achievement test, and that their scores were negatively correlated with their scores on a measure of dogmatism (which represents a tendency toward obedience). In the years since it was created, the Need for Cognition Scale has been used in literally hundreds of studies and has been shown to be correlated with a wide variety of other variables, including the effectiveness of an advertisement, interest in politics, and juror decisions (Petty, Briñol, Loersch, & McCaslin, 2009) [2] .
Discriminant Validity
Discriminant validity , on the other hand, is the extent to which scores on a measure are not correlated with measures of variables that are conceptually distinct. For example, self-esteem is a general attitude toward the self that is fairly stable over time. It is not the same as mood, which is how good or bad one happens to be feeling right now. So people’s scores on a new measure of self-esteem should not be very highly correlated with their moods. If the new measure of self-esteem were highly correlated with a measure of mood, it could be argued that the new measure is not really measuring self-esteem; it is measuring mood instead.
When they created the Need for Cognition Scale, Cacioppo and Petty also provided evidence of discriminant validity by showing that people’s scores were not correlated with certain other variables. For example, they found only a weak correlation between people’s need for cognition and a measure of their cognitive style—the extent to which they tend to think analytically by breaking ideas into smaller parts or holistically in terms of “the big picture.” They also found no correlation between people’s need for cognition and measures of their test anxiety and their tendency to respond in socially desirable ways. All these low correlations provide evidence that the measure is reflecting a conceptually distinct construct.
Key Takeaways
- Psychological researchers do not simply assume that their measures work. Instead, they conduct research to show that they work. If they cannot show that they work, they stop using them.
- There are two distinct criteria by which researchers evaluate their measures: reliability and validity. Reliability is consistency across time (test-retest reliability), across items (internal consistency), and across researchers (interrater reliability). Validity is the extent to which the scores actually represent the variable they are intended to.
- Validity is a judgment based on various types of evidence. The relevant evidence includes the measure’s reliability, whether it covers the construct of interest, and whether the scores it produces are correlated with other variables they are expected to be correlated with and not correlated with variables that are conceptually distinct.
- The reliability and validity of a measure is not established by any single study but by the pattern of results across multiple studies. The assessment of reliability and validity is an ongoing process.
- Practice: Ask several friends to complete the Rosenberg Self-Esteem Scale. Then assess its internal consistency by making a scatterplot to show the split-half correlation (even- vs. odd-numbered items). Compute Pearson’s r too if you know how.
- Discussion: Think back to the last college exam you took and think of the exam as a psychological measure. What construct do you think it was intended to measure? Comment on its face and content validity. What data could you collect to assess its reliability and criterion validity?
- Cacioppo, J. T., & Petty, R. E. (1982). The need for cognition. Journal of Personality and Social Psychology, 42 , 116–131. ↵
- Petty, R. E, Briñol, P., Loersch, C., & McCaslin, M. J. (2009). The need for cognition. In M. R. Leary & R. H. Hoyle (Eds.), Handbook of individual differences in social behaviour (pp. 318–329). New York, NY: Guilford Press. ↵
The consistency of a measure.
The consistency of a measure over time.
The consistency of a measure on the same group of people at different times.
Consistency of people’s responses across the items on a multiple-item measure.
Method of assessing internal consistency through splitting the items into two sets and examining the relationship between them.
A statistic in which α is the mean of all possible split-half correlations for a set of items.
The extent to which different observers are consistent in their judgments.
The extent to which the scores from a measure represent the variable they are intended to.
The extent to which a measurement method appears to measure the construct of interest.
The extent to which a measure “covers” the construct of interest.
The extent to which people’s scores on a measure are correlated with other variables that one would expect them to be correlated with.
In reference to criterion validity, variables that one would expect to be correlated with the measure.
When the criterion is measured at the same time as the construct.
when the criterion is measured at some point in the future (after the construct has been measured).
When new measures positively correlate with existing measures of the same constructs.
The extent to which scores on a measure are not correlated with measures of variables that are conceptually distinct.
Research Methods in Psychology - 2nd Canadian Edition Copyright © 2015 by Paul C. Price, Rajiv Jhangiani, & I-Chant A. Chiang is licensed under a Creative Commons Attribution-NonCommercial-ShareAlike 4.0 International License , except where otherwise noted.
Share This Book

An official website of the United States government
The .gov means it’s official. Federal government websites often end in .gov or .mil. Before sharing sensitive information, make sure you’re on a federal government site.
The site is secure. The https:// ensures that you are connecting to the official website and that any information you provide is encrypted and transmitted securely.
- Publications
- Account settings
Preview improvements coming to the PMC website in October 2024. Learn More or Try it out now .
- Advanced Search
- Journal List
- J Family Med Prim Care
- v.4(3); Jul-Sep 2015
Validity, reliability, and generalizability in qualitative research
Lawrence leung.
1 Department of Family Medicine, Queen's University, Kingston, Ontario, Canada
2 Centre of Studies in Primary Care, Queen's University, Kingston, Ontario, Canada
In general practice, qualitative research contributes as significantly as quantitative research, in particular regarding psycho-social aspects of patient-care, health services provision, policy setting, and health administrations. In contrast to quantitative research, qualitative research as a whole has been constantly critiqued, if not disparaged, by the lack of consensus for assessing its quality and robustness. This article illustrates with five published studies how qualitative research can impact and reshape the discipline of primary care, spiraling out from clinic-based health screening to community-based disease monitoring, evaluation of out-of-hours triage services to provincial psychiatric care pathways model and finally, national legislation of core measures for children's healthcare insurance. Fundamental concepts of validity, reliability, and generalizability as applicable to qualitative research are then addressed with an update on the current views and controversies.
Nature of Qualitative Research versus Quantitative Research
The essence of qualitative research is to make sense of and recognize patterns among words in order to build up a meaningful picture without compromising its richness and dimensionality. Like quantitative research, the qualitative research aims to seek answers for questions of “how, where, when who and why” with a perspective to build a theory or refute an existing theory. Unlike quantitative research which deals primarily with numerical data and their statistical interpretations under a reductionist, logical and strictly objective paradigm, qualitative research handles nonnumerical information and their phenomenological interpretation, which inextricably tie in with human senses and subjectivity. While human emotions and perspectives from both subjects and researchers are considered undesirable biases confounding results in quantitative research, the same elements are considered essential and inevitable, if not treasurable, in qualitative research as they invariable add extra dimensions and colors to enrich the corpus of findings. However, the issue of subjectivity and contextual ramifications has fueled incessant controversies regarding yardsticks for quality and trustworthiness of qualitative research results for healthcare.
Impact of Qualitative Research upon Primary Care
In many ways, qualitative research contributes significantly, if not more so than quantitative research, to the field of primary care at various levels. Five qualitative studies are chosen to illustrate how various methodologies of qualitative research helped in advancing primary healthcare, from novel monitoring of chronic obstructive pulmonary disease (COPD) via mobile-health technology,[ 1 ] informed decision for colorectal cancer screening,[ 2 ] triaging out-of-hours GP services,[ 3 ] evaluating care pathways for community psychiatry[ 4 ] and finally prioritization of healthcare initiatives for legislation purposes at national levels.[ 5 ] With the recent advances of information technology and mobile connecting device, self-monitoring and management of chronic diseases via tele-health technology may seem beneficial to both the patient and healthcare provider. Recruiting COPD patients who were given tele-health devices that monitored lung functions, Williams et al. [ 1 ] conducted phone interviews and analyzed their transcripts via a grounded theory approach, identified themes which enabled them to conclude that such mobile-health setup and application helped to engage patients with better adherence to treatment and overall improvement in mood. Such positive findings were in contrast to previous studies, which opined that elderly patients were often challenged by operating computer tablets,[ 6 ] or, conversing with the tele-health software.[ 7 ] To explore the content of recommendations for colorectal cancer screening given out by family physicians, Wackerbarth, et al. [ 2 ] conducted semi-structure interviews with subsequent content analysis and found that most physicians delivered information to enrich patient knowledge with little regard to patients’ true understanding, ideas, and preferences in the matter. These findings suggested room for improvement for family physicians to better engage their patients in recommending preventative care. Faced with various models of out-of-hours triage services for GP consultations, Egbunike et al. [ 3 ] conducted thematic analysis on semi-structured telephone interviews with patients and doctors in various urban, rural and mixed settings. They found that the efficiency of triage services remained a prime concern from both users and providers, among issues of access to doctors and unfulfilled/mismatched expectations from users, which could arouse dissatisfaction and legal implications. In UK, a care pathways model for community psychiatry had been introduced but its benefits were unclear. Khandaker et al. [ 4 ] hence conducted a qualitative study using semi-structure interviews with medical staff and other stakeholders; adopting a grounded-theory approach, major themes emerged which included improved equality of access, more focused logistics, increased work throughput and better accountability for community psychiatry provided under the care pathway model. Finally, at the US national level, Mangione-Smith et al. [ 5 ] employed a modified Delphi method to gather consensus from a panel of nominators which were recognized experts and stakeholders in their disciplines, and identified a core set of quality measures for children's healthcare under the Medicaid and Children's Health Insurance Program. These core measures were made transparent for public opinion and later passed on for full legislation, hence illustrating the impact of qualitative research upon social welfare and policy improvement.
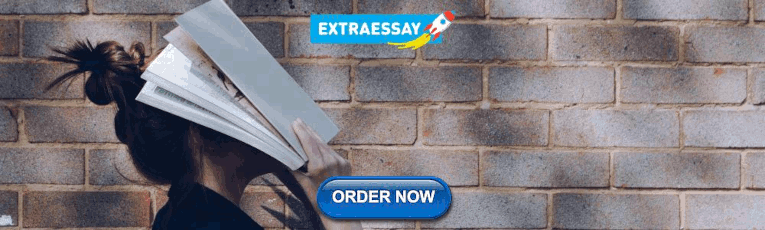
Overall Criteria for Quality in Qualitative Research
Given the diverse genera and forms of qualitative research, there is no consensus for assessing any piece of qualitative research work. Various approaches have been suggested, the two leading schools of thoughts being the school of Dixon-Woods et al. [ 8 ] which emphasizes on methodology, and that of Lincoln et al. [ 9 ] which stresses the rigor of interpretation of results. By identifying commonalities of qualitative research, Dixon-Woods produced a checklist of questions for assessing clarity and appropriateness of the research question; the description and appropriateness for sampling, data collection and data analysis; levels of support and evidence for claims; coherence between data, interpretation and conclusions, and finally level of contribution of the paper. These criteria foster the 10 questions for the Critical Appraisal Skills Program checklist for qualitative studies.[ 10 ] However, these methodology-weighted criteria may not do justice to qualitative studies that differ in epistemological and philosophical paradigms,[ 11 , 12 ] one classic example will be positivistic versus interpretivistic.[ 13 ] Equally, without a robust methodological layout, rigorous interpretation of results advocated by Lincoln et al. [ 9 ] will not be good either. Meyrick[ 14 ] argued from a different angle and proposed fulfillment of the dual core criteria of “transparency” and “systematicity” for good quality qualitative research. In brief, every step of the research logistics (from theory formation, design of study, sampling, data acquisition and analysis to results and conclusions) has to be validated if it is transparent or systematic enough. In this manner, both the research process and results can be assured of high rigor and robustness.[ 14 ] Finally, Kitto et al. [ 15 ] epitomized six criteria for assessing overall quality of qualitative research: (i) Clarification and justification, (ii) procedural rigor, (iii) sample representativeness, (iv) interpretative rigor, (v) reflexive and evaluative rigor and (vi) transferability/generalizability, which also double as evaluative landmarks for manuscript review to the Medical Journal of Australia. Same for quantitative research, quality for qualitative research can be assessed in terms of validity, reliability, and generalizability.
Validity in qualitative research means “appropriateness” of the tools, processes, and data. Whether the research question is valid for the desired outcome, the choice of methodology is appropriate for answering the research question, the design is valid for the methodology, the sampling and data analysis is appropriate, and finally the results and conclusions are valid for the sample and context. In assessing validity of qualitative research, the challenge can start from the ontology and epistemology of the issue being studied, e.g. the concept of “individual” is seen differently between humanistic and positive psychologists due to differing philosophical perspectives:[ 16 ] Where humanistic psychologists believe “individual” is a product of existential awareness and social interaction, positive psychologists think the “individual” exists side-by-side with formation of any human being. Set off in different pathways, qualitative research regarding the individual's wellbeing will be concluded with varying validity. Choice of methodology must enable detection of findings/phenomena in the appropriate context for it to be valid, with due regard to culturally and contextually variable. For sampling, procedures and methods must be appropriate for the research paradigm and be distinctive between systematic,[ 17 ] purposeful[ 18 ] or theoretical (adaptive) sampling[ 19 , 20 ] where the systematic sampling has no a priori theory, purposeful sampling often has a certain aim or framework and theoretical sampling is molded by the ongoing process of data collection and theory in evolution. For data extraction and analysis, several methods were adopted to enhance validity, including 1 st tier triangulation (of researchers) and 2 nd tier triangulation (of resources and theories),[ 17 , 21 ] well-documented audit trail of materials and processes,[ 22 , 23 , 24 ] multidimensional analysis as concept- or case-orientated[ 25 , 26 ] and respondent verification.[ 21 , 27 ]
Reliability
In quantitative research, reliability refers to exact replicability of the processes and the results. In qualitative research with diverse paradigms, such definition of reliability is challenging and epistemologically counter-intuitive. Hence, the essence of reliability for qualitative research lies with consistency.[ 24 , 28 ] A margin of variability for results is tolerated in qualitative research provided the methodology and epistemological logistics consistently yield data that are ontologically similar but may differ in richness and ambience within similar dimensions. Silverman[ 29 ] proposed five approaches in enhancing the reliability of process and results: Refutational analysis, constant data comparison, comprehensive data use, inclusive of the deviant case and use of tables. As data were extracted from the original sources, researchers must verify their accuracy in terms of form and context with constant comparison,[ 27 ] either alone or with peers (a form of triangulation).[ 30 ] The scope and analysis of data included should be as comprehensive and inclusive with reference to quantitative aspects if possible.[ 30 ] Adopting the Popperian dictum of falsifiability as essence of truth and science, attempted to refute the qualitative data and analytes should be performed to assess reliability.[ 31 ]
Generalizability
Most qualitative research studies, if not all, are meant to study a specific issue or phenomenon in a certain population or ethnic group, of a focused locality in a particular context, hence generalizability of qualitative research findings is usually not an expected attribute. However, with rising trend of knowledge synthesis from qualitative research via meta-synthesis, meta-narrative or meta-ethnography, evaluation of generalizability becomes pertinent. A pragmatic approach to assessing generalizability for qualitative studies is to adopt same criteria for validity: That is, use of systematic sampling, triangulation and constant comparison, proper audit and documentation, and multi-dimensional theory.[ 17 ] However, some researchers espouse the approach of analytical generalization[ 32 ] where one judges the extent to which the findings in one study can be generalized to another under similar theoretical, and the proximal similarity model, where generalizability of one study to another is judged by similarities between the time, place, people and other social contexts.[ 33 ] Thus said, Zimmer[ 34 ] questioned the suitability of meta-synthesis in view of the basic tenets of grounded theory,[ 35 ] phenomenology[ 36 ] and ethnography.[ 37 ] He concluded that any valid meta-synthesis must retain the other two goals of theory development and higher-level abstraction while in search of generalizability, and must be executed as a third level interpretation using Gadamer's concepts of the hermeneutic circle,[ 38 , 39 ] dialogic process[ 38 ] and fusion of horizons.[ 39 ] Finally, Toye et al. [ 40 ] reported the practicality of using “conceptual clarity” and “interpretative rigor” as intuitive criteria for assessing quality in meta-ethnography, which somehow echoed Rolfe's controversial aesthetic theory of research reports.[ 41 ]
Food for Thought
Despite various measures to enhance or ensure quality of qualitative studies, some researchers opined from a purist ontological and epistemological angle that qualitative research is not a unified, but ipso facto diverse field,[ 8 ] hence any attempt to synthesize or appraise different studies under one system is impossible and conceptually wrong. Barbour argued from a philosophical angle that these special measures or “technical fixes” (like purposive sampling, multiple-coding, triangulation, and respondent validation) can never confer the rigor as conceived.[ 11 ] In extremis, Rolfe et al. opined from the field of nursing research, that any set of formal criteria used to judge the quality of qualitative research are futile and without validity, and suggested that any qualitative report should be judged by the form it is written (aesthetic) and not by the contents (epistemic).[ 41 ] Rolfe's novel view is rebutted by Porter,[ 42 ] who argued via logical premises that two of Rolfe's fundamental statements were flawed: (i) “The content of research report is determined by their forms” may not be a fact, and (ii) that research appraisal being “subject to individual judgment based on insight and experience” will mean those without sufficient experience of performing research will be unable to judge adequately – hence an elitist's principle. From a realism standpoint, Porter then proposes multiple and open approaches for validity in qualitative research that incorporate parallel perspectives[ 43 , 44 ] and diversification of meanings.[ 44 ] Any work of qualitative research, when read by the readers, is always a two-way interactive process, such that validity and quality has to be judged by the receiving end too and not by the researcher end alone.
In summary, the three gold criteria of validity, reliability and generalizability apply in principle to assess quality for both quantitative and qualitative research, what differs will be the nature and type of processes that ontologically and epistemologically distinguish between the two.
Source of Support: Nil.
Conflict of Interest: None declared.
Reliability In Psychology Research: Definitions & Examples
Saul Mcleod, PhD
Editor-in-Chief for Simply Psychology
BSc (Hons) Psychology, MRes, PhD, University of Manchester
Saul Mcleod, PhD., is a qualified psychology teacher with over 18 years of experience in further and higher education. He has been published in peer-reviewed journals, including the Journal of Clinical Psychology.
Learn about our Editorial Process
Olivia Guy-Evans, MSc
Associate Editor for Simply Psychology
BSc (Hons) Psychology, MSc Psychology of Education
Olivia Guy-Evans is a writer and associate editor for Simply Psychology. She has previously worked in healthcare and educational sectors.
On This Page:
Reliability in psychology research refers to the reproducibility or consistency of measurements. Specifically, it is the degree to which a measurement instrument or procedure yields the same results on repeated trials. A measure is considered reliable if it produces consistent scores across different instances when the underlying thing being measured has not changed.
Reliability ensures that responses are consistent across times and occasions for instruments like questionnaires . Multiple forms of reliability exist, including test-retest, inter-rater, and internal consistency.
For example, if people weigh themselves during the day, they would expect to see a similar reading. Scales that measured weight differently each time would be of little use.
The same analogy could be applied to a tape measure that measures inches differently each time it is used. It would not be considered reliable.
If findings from research are replicated consistently, they are reliable. A correlation coefficient can be used to assess the degree of reliability. If a test is reliable, it should show a high positive correlation.
Of course, it is unlikely the same results will be obtained each time as participants and situations vary. Still, a strong positive correlation between the same test results indicates reliability.
Reliability is important because unreliable measures introduce random error that attenuates correlations and makes it harder to detect real relationships.
Ensuring high reliability for key measures in psychology research helps boost the sensitivity, validity, and replicability of studies. Estimating and reporting reliable evidence is considered an important methodological practice.
There are two types of reliability: internal and external.
- Internal reliability refers to how consistently different items within a single test measure the same concept or construct. It ensures that a test is stable across its components.
- External reliability measures how consistently a test produces similar results over repeated administrations or under different conditions. It ensures that a test is stable over time and situations.
Some key aspects of reliability in psychology research include:
- Test-retest reliability : The consistency of scores for the same person across two or more separate administrations of the same measurement procedure over time. High test-retest reliability suggests the measure provides a stable, reproducible score.
- Interrater reliability : The level of agreement in scores on a measure between different raters or observers rating the same target. High interrater reliability suggests the ratings are objective and not overly influenced by rater subjectivity or bias.
- Internal consistency reliability : The degree to which different test items or parts of an instrument that measure the same construct yield similar results. Analyzed statistically using Cronbach’s alpha, a high value suggests the items measure the same underlying concept.
Test-Retest Reliability
The test-retest method assesses the external consistency of a test. Examples of appropriate tests include questionnaires and psychometric tests. It measures the stability of a test over time.
A typical assessment would involve giving participants the same test on two separate occasions. If the same or similar results are obtained, then external reliability is established.
Here’s how it works:
- A test or measurement is administered to participants at one point in time.
- After a certain period, the same test is administered again to the same participants without any intervention or treatment in between.
- The scores from the two administrations are then correlated using a statistical method, often Pearson’s correlation.
- A high correlation between the scores from the two test administrations indicates good test-retest reliability, suggesting the test yields consistent results over time.
This method is especially useful for tests that measure stable traits or characteristics that aren’t expected to change over short periods.
The disadvantage of the test-retest method is that it takes a long time for results to be obtained. The reliability can be influenced by the time interval between tests and any events that might affect participants’ responses during this interval.
Beck et al. (1996) studied the responses of 26 outpatients on two separate therapy sessions one week apart, they found a correlation of .93 therefore demonstrating high test-restest reliability of the depression inventory.
This is an example of why reliability in psychological research is necessary, if it wasn’t for the reliability of such tests some individuals may not be successfully diagnosed with disorders such as depression and consequently will not be given appropriate therapy.
The timing of the test is important; if the duration is too brief, then participants may recall information from the first test, which could bias the results.
Alternatively, if the duration is too long, it is feasible that the participants could have changed in some important way which could also bias the results.
The test-retest method assesses the external consistency of a test. This refers to the degree to which different raters give consistent estimates of the same behavior. Inter-rater reliability can be used for interviews.
Inter-Rater Reliability
Inter-rater reliability, often termed inter-observer reliability, refers to the extent to which different raters or evaluators agree in assessing a particular phenomenon, behavior, or characteristic. It’s a measure of consistency and agreement between individuals scoring or evaluating the same items or behaviors.
High inter-rater reliability indicates that the findings or measurements are consistent across different raters, suggesting the results are not due to random chance or subjective biases of individual raters.
Statistical measures, such as Cohen’s Kappa or the Intraclass Correlation Coefficient (ICC), are often employed to quantify the level of agreement between raters, helping to ensure that findings are objective and reproducible.
Ensuring high inter-rater reliability is essential, especially in studies involving subjective judgment or observations, as it provides confidence that the findings are replicable and not heavily influenced by individual rater biases.
Note it can also be called inter-observer reliability when referring to observational research. Here, researchers observe the same behavior independently (to avoid bias) and compare their data. If the data is similar, then it is reliable.
Where observer scores do not significantly correlate, then reliability can be improved by:
- Train observers in the observation techniques and ensure everyone agrees with them.
- Ensuring behavior categories have been operationalized. This means that they have been objectively defined.
For example, if two researchers are observing ‘aggressive behavior’ of children at nursery they would both have their own subjective opinion regarding what aggression comprises.
In this scenario, they would be unlikely to record aggressive behavior the same, and the data would be unreliable.
However, if they were to operationalize the behavior category of aggression, this would be more objective and make it easier to identify when a specific behavior occurs.
For example, while “aggressive behavior” is subjective and not operationalized, “pushing” is objective and operationalized. Thus, researchers could count how many times children push each other over a certain duration of time.
Internal Consistency Reliability
Internal consistency reliability refers to how well different items on a test or survey that are intended to measure the same construct produce similar scores.
For example, a questionnaire measuring depression may have multiple questions tapping issues like sadness, changes in sleep and appetite, fatigue, and loss of interest. The assumption is that people’s responses across these different symptom items should be fairly consistent.
Cronbach’s alpha is a common statistic used to quantify internal consistency reliability. It calculates the average inter-item correlations among the test items. Values range from 0 to 1, with higher values indicating greater internal consistency. A good rule of thumb is that alpha should generally be above .70 to suggest adequate reliability.
An alpha of .90 for a depression questionnaire, for example, means there is a high average correlation between respondents’ scores on the different symptom items.
This suggests all the items are measuring the same underlying construct (depression) in a consistent manner. It taps the unidimensionality of the scale – evidence it is measuring one thing.
If some items were unrelated to others, the average inter-item correlations would be lower, resulting in a lower alpha. This would indicate the presence of multiple dimensions in the scale, rather than a unified single concept.
So, in summary, high internal consistency reliability evidenced through high Cronbach’s alpha provides support for the fact that various test items successfully tap into the same latent variable the researcher intends to measure. It suggests the items meaningfully cohere together to reliably measure that construct.
Split-Half Method
The split-half method assesses the internal consistency of a test, such as psychometric tests and questionnaires.
There, it measures the extent to which all parts of the test contribute equally to what is being measured.
The split-half approach provides another method of quantifying internal consistency by taking advantage of the natural variation when a single test is divided in half.
It’s somewhat cumbersome to implement but avoids limitations associated with Cronbach’s alpha. However, alpha remains much more widely used in practice due to its relative ease of calculation.
- A test or questionnaire is split into two halves, typically by separating even-numbered items from odd-numbered items, or first-half items vs. second-half.
- Each half is scored separately, and the scores are correlated using a statistical method, often Pearson’s correlation.
- The correlation between the two halves gives an indication of the test’s reliability. A higher correlation suggests better reliability.
- To adjust for the test’s shortened length (because we’ve split it in half), the Spearman-Brown prophecy formula is often applied to estimate the reliability of the full test based on the split-half reliability.
The reliability of a test could be improved by using this method. For example, any items on separate halves of a test with a low correlation (e.g., r = .25) should either be removed or rewritten.
The split-half method is a quick and easy way to establish reliability. However, it can only be effective with large questionnaires in which all questions measure the same construct. This means it would not be appropriate for tests that measure different constructs.
For example, the Minnesota Multiphasic Personality Inventory has sub scales measuring differently behaviors such as depression, schizophrenia, social introversion. Therefore the split-half method was not be an appropriate method to assess reliability for this personality test.
Validity vs. Reliability In Psychology
In psychology, validity and reliability are fundamental concepts that assess the quality of measurements.
- Validity refers to the degree to which a measure accurately assesses the specific concept, trait, or construct that it claims to be assessing. It refers to the truthfulness of the measure.
- Reliability refers to the overall consistency, stability, and repeatability of a measurement. It is concerned with how much random error might be distorting scores or introducing unwanted “noise” into the data.
A key difference is that validity refers to what’s being measured, while reliability refers to how consistently it’s being measured.
An unreliable measure cannot be truly valid because if a measure gives inconsistent, unpredictable scores, it clearly isn’t measuring the trait or quality it aims to measure in a truthful, systematic manner. Establishing reliability provides the foundation for determining the measure’s validity.
A pivotal understanding is that reliability is a necessary but not sufficient condition for validity.
It means a test can be reliable, consistently producing the same results, without being valid, or accurately measuring the intended attribute.
However, a valid test, one that truly measures what it purports to, must be reliable. In the pursuit of rigorous psychological research, both validity and reliability are indispensable.
Ideally, researchers strive for high scores on both -Validity to make sure you’re measuring the correct construct and reliability to make sure you’re measuring it accurately and precisely. The two qualities are independent but both crucial elements of strong measurement procedures.
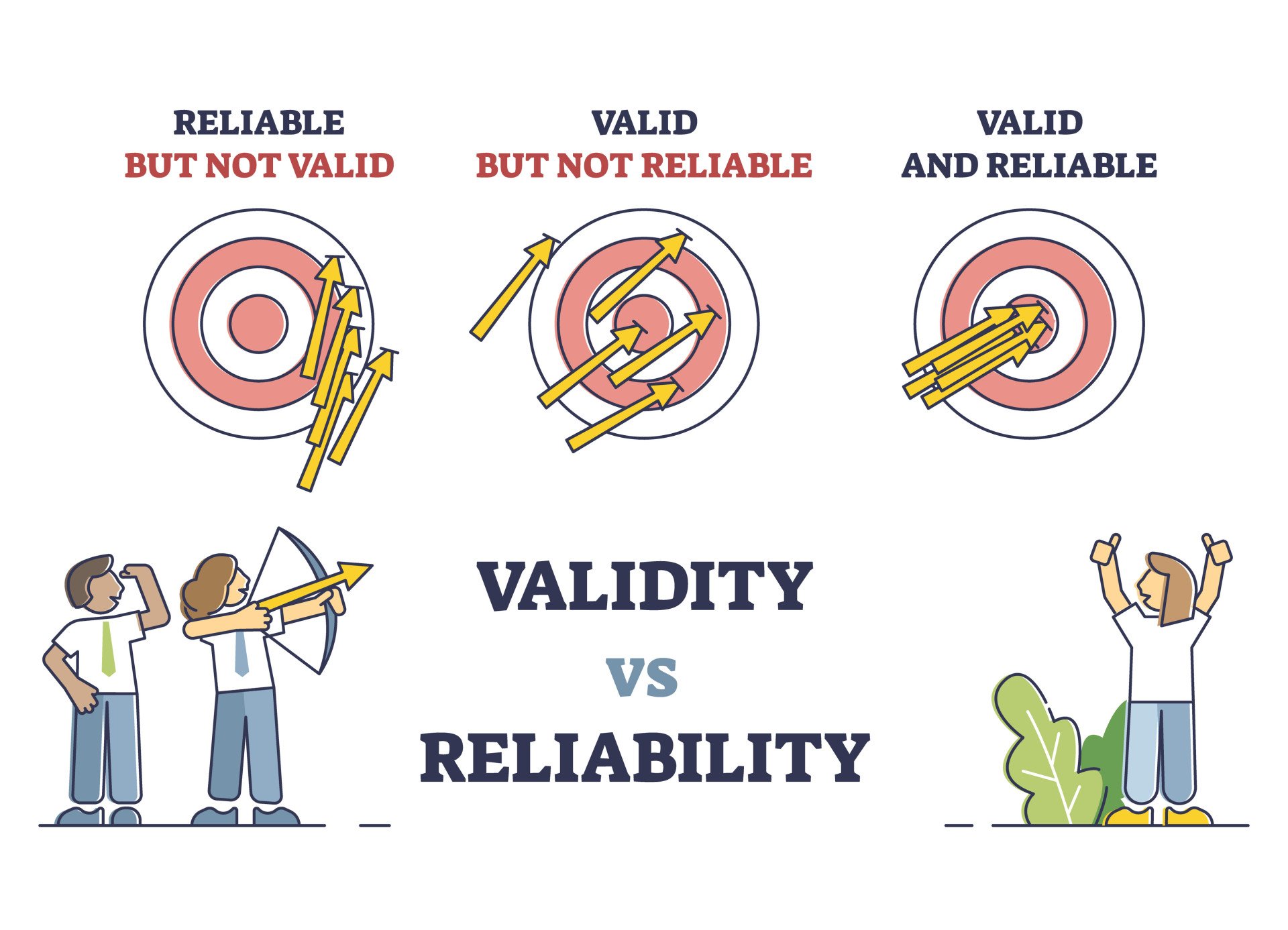
Beck, A. T., Steer, R. A., & Brown, G. K. (1996). Manual for the beck depression inventory The Psychological Corporation. San Antonio , TX.
Clifton, J. D. W. (2020). Managing validity versus reliability trade-offs in scale-building decisions. Psychological Methods, 25 (3), 259–270. https:// doi.org/10.1037/met0000236
Guttman, L. (1945). A basis for analyzing test-retest reliability. Psychometrika, 10 (4), 255–282. https://doi.org/10.1007/BF02288892
Hathaway, S. R., & McKinley, J. C. (1943). Manual for the Minnesota Multiphasic Personality Inventory . New York: Psychological Corporation.
Jannarone, R. J., Macera, C. A., & Garrison, C. Z. (1987). Evaluating interrater agreement through “case-control” sampling. Biometrics, 43 (2), 433–437. https://doi.org/10.2307/2531825
LeBreton, J. M., & Senter, J. L. (2008). Answers to 20 questions about interrater reliability and interrater agreement. Organizational Research Methods, 11 (4), 815–852. https://doi.org/10.1177/1094428106296642
Watkins, M. W., & Pacheco, M. (2000). Interobserver agreement in behavioral research: Importance and calculation. Journal of Behavioral Education, 10 , 205–212

Related Articles
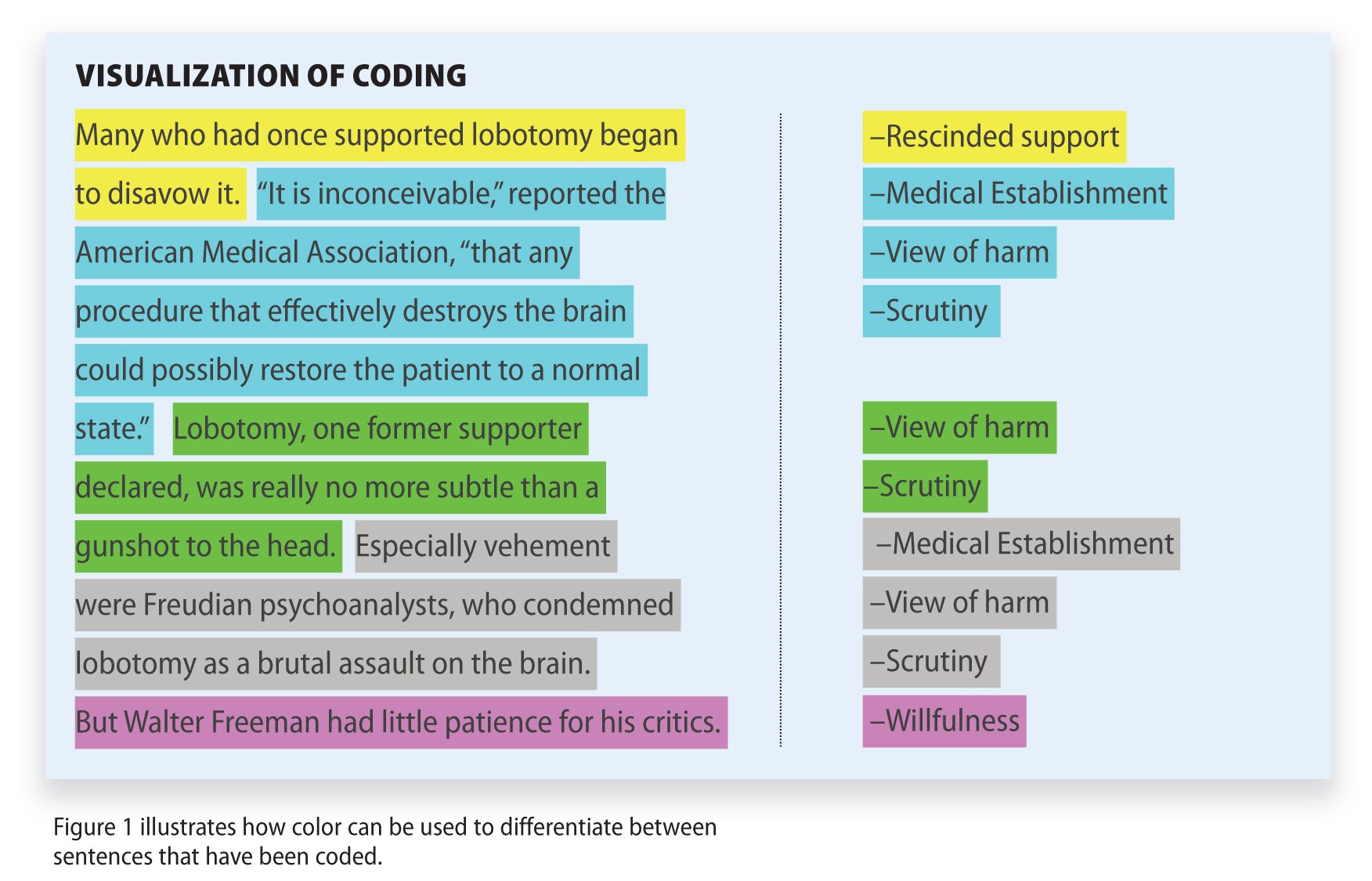
Research Methodology
Qualitative Data Coding
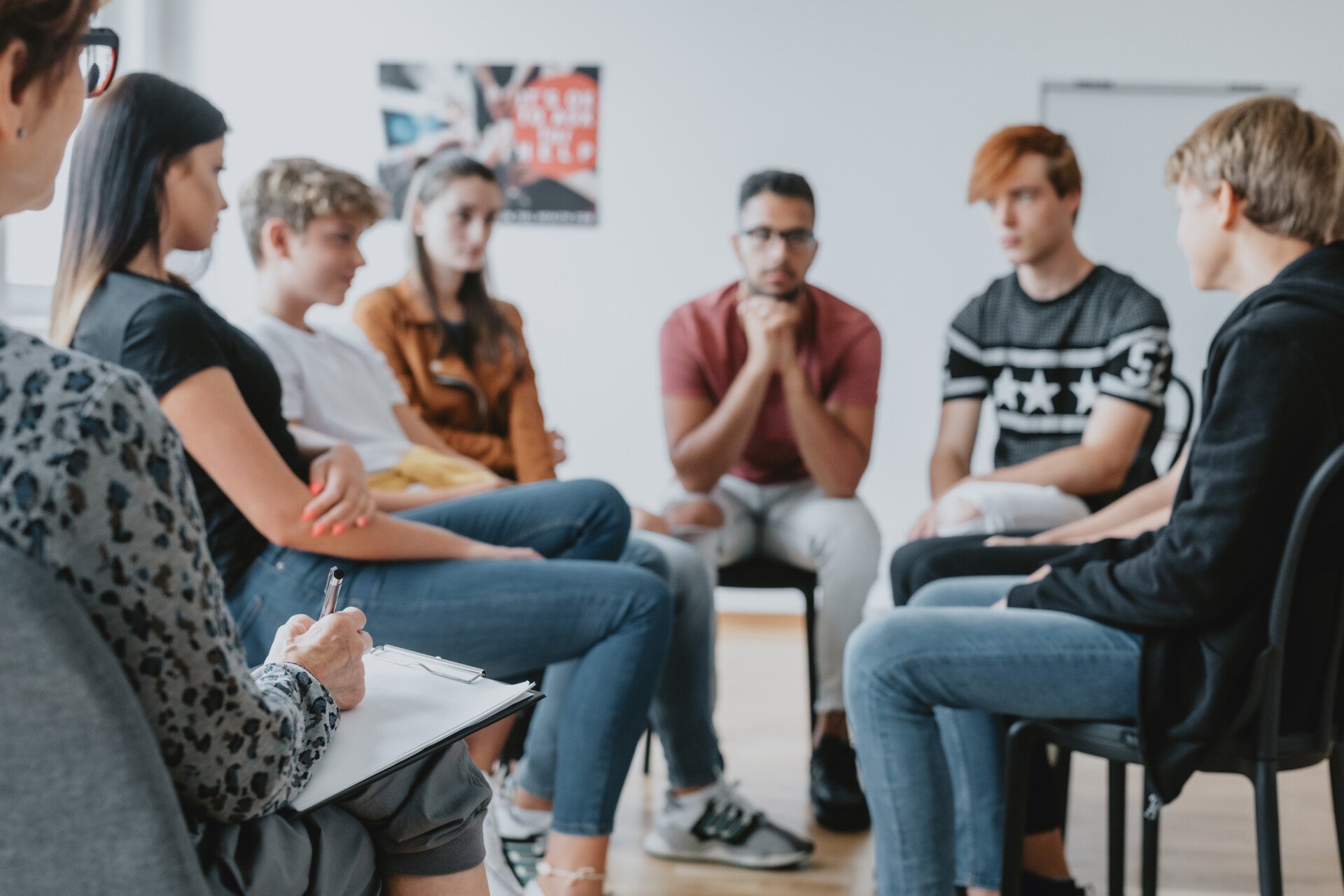
What Is a Focus Group?
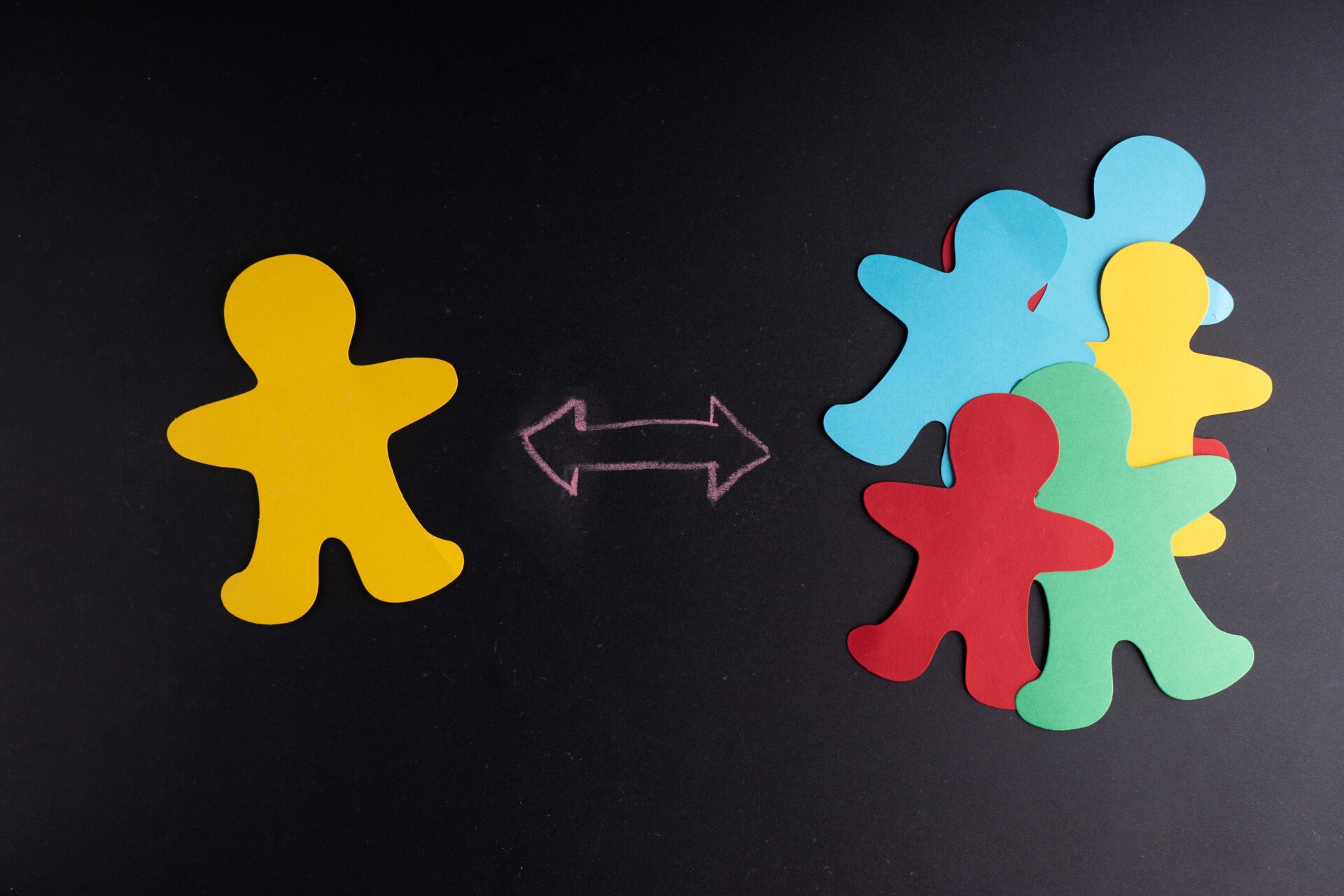
Cross-Cultural Research Methodology In Psychology
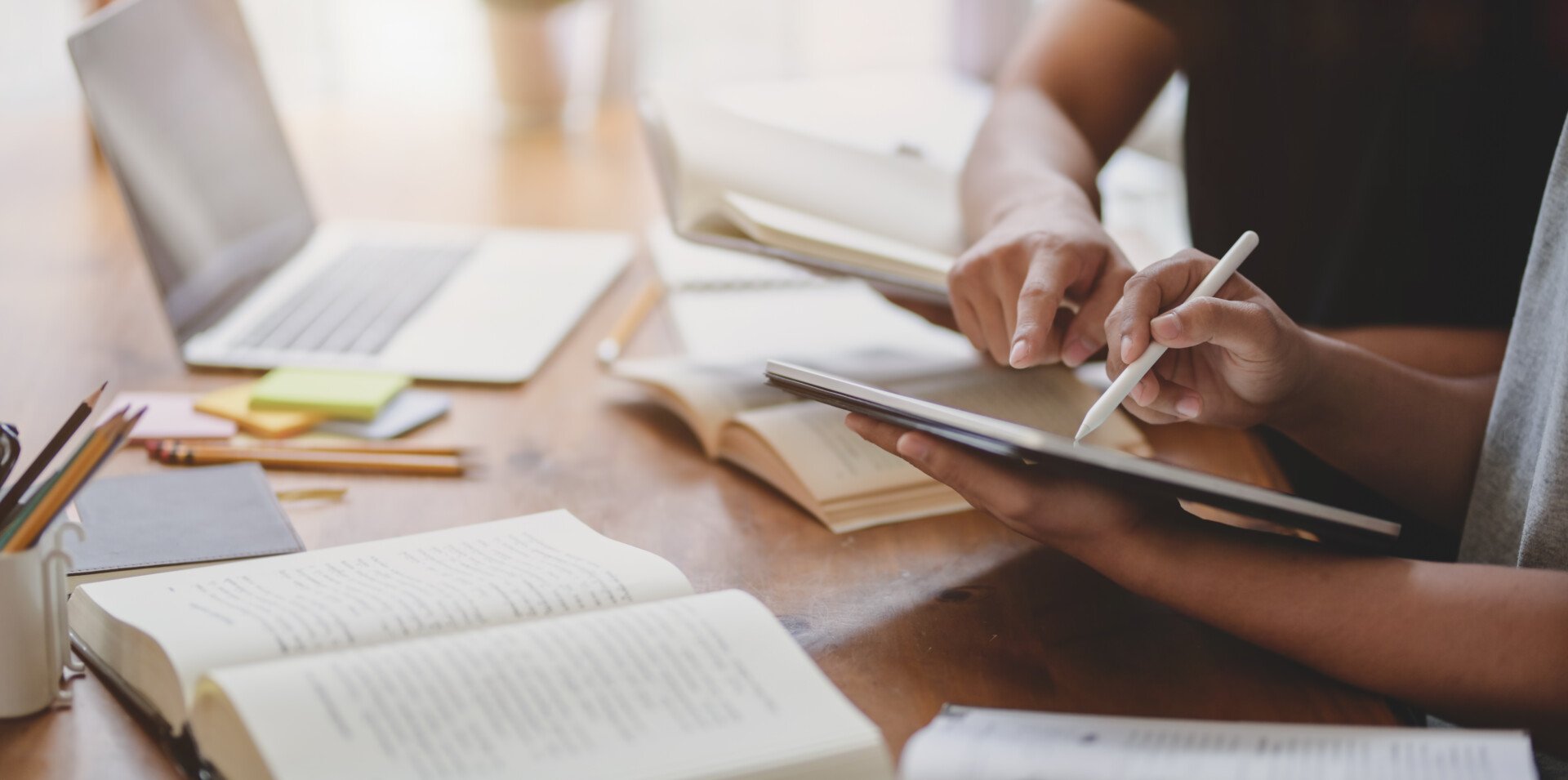
What Is Internal Validity In Research?
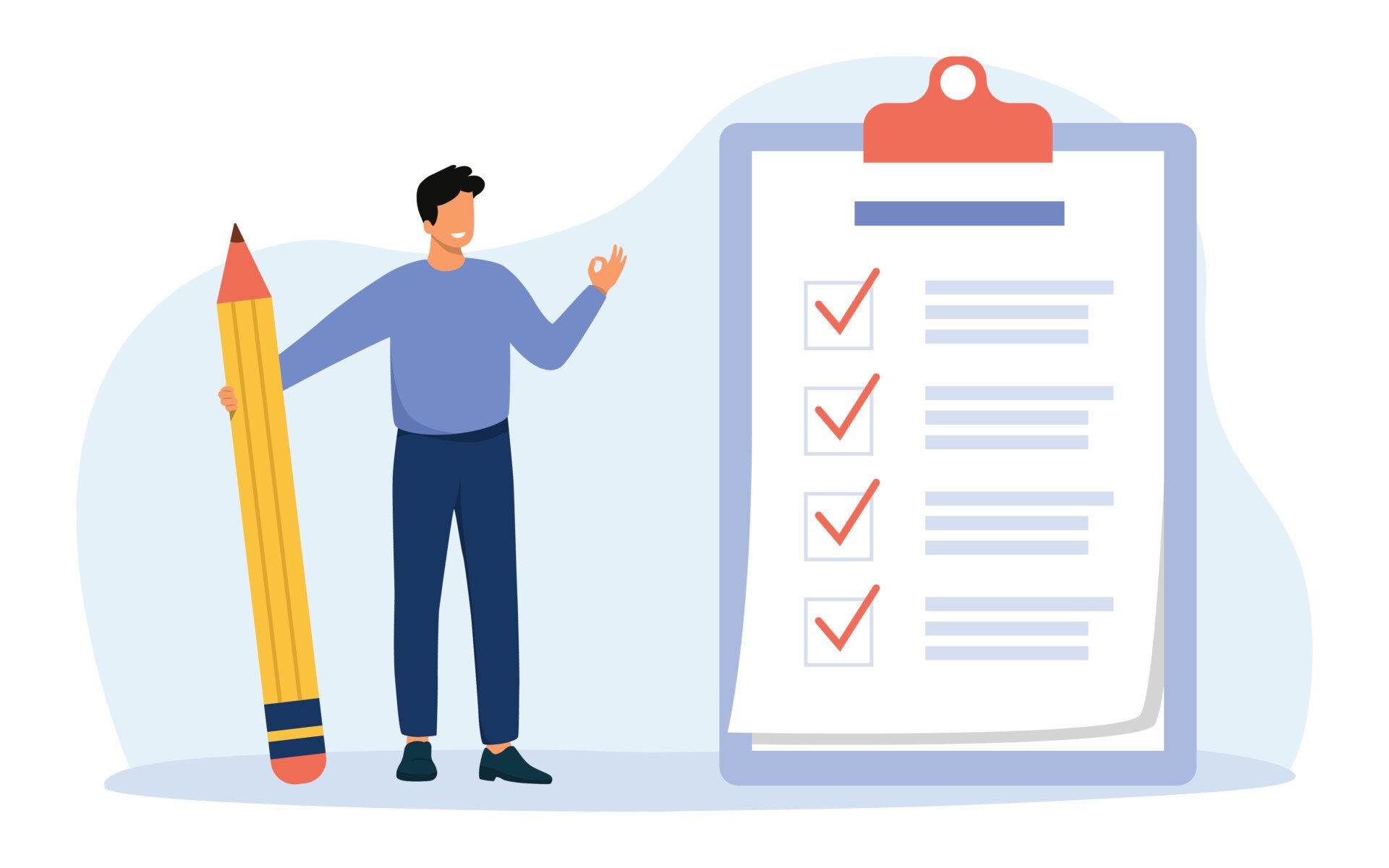
Research Methodology , Statistics
What Is Face Validity In Research? Importance & How To Measure
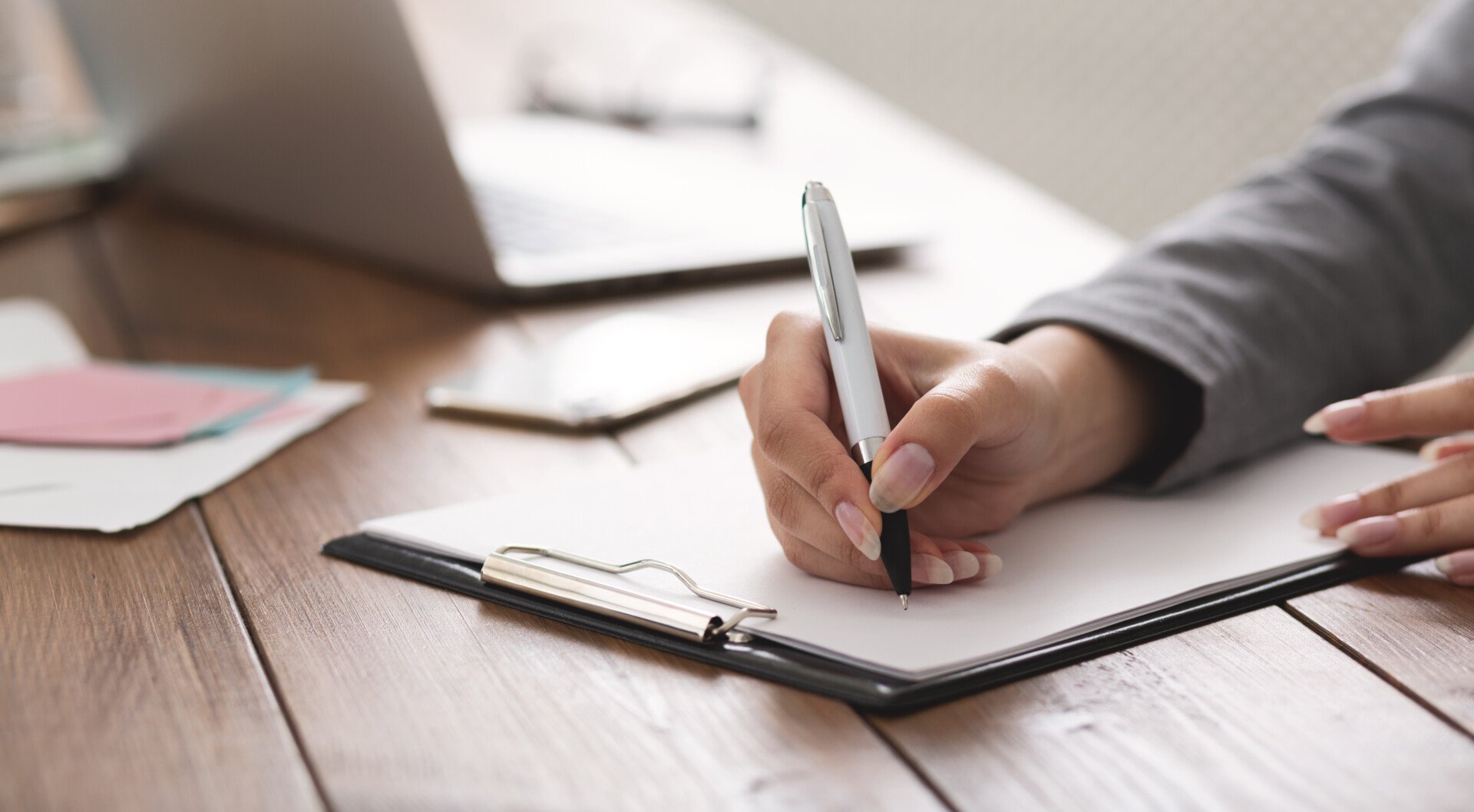
Criterion Validity: Definition & Examples
- Privacy Policy

Home » Reliability – Types, Examples and Guide
Reliability – Types, Examples and Guide
Table of Contents

Reliability
Definition:
Reliability refers to the consistency, dependability, and trustworthiness of a system, process, or measurement to perform its intended function or produce consistent results over time. It is a desirable characteristic in various domains, including engineering, manufacturing, software development, and data analysis.
Reliability In Engineering
In engineering and manufacturing, reliability refers to the ability of a product, equipment, or system to function without failure or breakdown under normal operating conditions for a specified period. A reliable system consistently performs its intended functions, meets performance requirements, and withstands various environmental factors, stress, or wear and tear.
Reliability In Software Development
In software development, reliability relates to the stability and consistency of software applications or systems. A reliable software program operates consistently without crashing, produces accurate results, and handles errors or exceptions gracefully. Reliability is often measured by metrics such as mean time between failures (MTBF) and mean time to repair (MTTR).
Reliability In Data Analysis and Statistics
In data analysis and statistics, reliability refers to the consistency and repeatability of measurements or assessments. For example, if a measurement instrument consistently produces similar results when measuring the same quantity or if multiple raters consistently agree on the same assessment, it is considered reliable. Reliability is often assessed using statistical measures such as test-retest reliability, inter-rater reliability, or internal consistency.
Research Reliability
Research reliability refers to the consistency, stability, and repeatability of research findings . It indicates the extent to which a research study produces consistent and dependable results when conducted under similar conditions. In other words, research reliability assesses whether the same results would be obtained if the study were replicated with the same methodology, sample, and context.
What Affects Reliability in Research
Several factors can affect the reliability of research measurements and assessments. Here are some common factors that can impact reliability:
Measurement Error
Measurement error refers to the variability or inconsistency in the measurements that is not due to the construct being measured. It can arise from various sources, such as the limitations of the measurement instrument, environmental factors, or the characteristics of the participants. Measurement error reduces the reliability of the measure by introducing random variability into the data.
Rater/Observer Bias
In studies involving subjective assessments or ratings, the biases or subjective judgments of the raters or observers can affect reliability. If different raters interpret and evaluate the same phenomenon differently, it can lead to inconsistencies in the ratings, resulting in lower inter-rater reliability.
Participant Factors
Characteristics or factors related to the participants themselves can influence reliability. For example, factors such as fatigue, motivation, attention, or mood can introduce variability in responses, affecting the reliability of self-report measures or performance assessments.
Instrumentation
The quality and characteristics of the measurement instrument can impact reliability. If the instrument lacks clarity, has ambiguous items or instructions, or is prone to measurement errors, it can decrease the reliability of the measure. Poorly designed or unreliable instruments can introduce measurement error and decrease the consistency of the measurements.
Sample Size
Sample size can affect reliability, especially in studies where the reliability coefficient is based on correlations or variability within the sample. A larger sample size generally provides more stable estimates of reliability, while smaller samples can yield less precise estimates.
Time Interval
The time interval between test administrations can impact test-retest reliability. If the time interval is too short, participants may recall their previous responses and answer in a similar manner, artificially inflating the reliability coefficient. On the other hand, if the time interval is too long, true changes in the construct being measured may occur, leading to lower test-retest reliability.
Content Sampling
The specific items or questions included in a measure can affect reliability. If the measure does not adequately sample the full range of the construct being measured or if the items are too similar or redundant, it can result in lower internal consistency reliability.
Scoring and Data Handling
Errors in scoring, data entry, or data handling can introduce variability and impact reliability. Inaccurate or inconsistent scoring procedures, data entry mistakes, or mishandling of missing data can affect the reliability of the measurements.
Context and Environment
The context and environment in which measurements are obtained can influence reliability. Factors such as noise, distractions, lighting conditions, or the presence of others can introduce variability and affect the consistency of the measurements.
Types of Reliability
There are several types of reliability that are commonly discussed in research and measurement contexts. Here are some of the main types of reliability:
Test-Retest Reliability
This type of reliability assesses the consistency of a measure over time. It involves administering the same test or measure to the same group of individuals on two separate occasions and then comparing the results. If the scores are similar or highly correlated across the two testing points, it indicates good test-retest reliability.
Inter-Rater Reliability
Inter-rater reliability examines the degree of agreement or consistency between different raters or observers who are assessing the same phenomenon. It is commonly used in subjective evaluations or assessments where judgments are made by multiple individuals. High inter-rater reliability suggests that different observers are likely to reach the same conclusions or make consistent assessments.
Internal Consistency Reliability
Internal consistency reliability assesses the extent to which the items or questions within a measure are consistent with each other. It is commonly measured using techniques such as Cronbach’s alpha. High internal consistency reliability indicates that the items within a measure are measuring the same construct or concept consistently.
Parallel Forms Reliability
Parallel forms reliability assesses the consistency of different versions or forms of a test that are intended to measure the same construct. Two equivalent versions of a test are administered to the same group of individuals, and the scores are compared to determine the level of agreement between the forms.
Split-Half Reliability
Split-half reliability involves splitting a measure into two halves and examining the consistency between the two halves. It can be done by dividing the items into odd-even pairs or by randomly splitting the items. The scores from the two halves are then compared to assess the degree of consistency.
Alternate Forms Reliability
Alternate forms reliability is similar to parallel forms reliability, but it involves administering two different versions of a test to the same group of individuals. The two forms should be equivalent and measure the same construct. The scores from the two forms are then compared to assess the level of agreement.
Applications of Reliability
Reliability has several important applications across various fields and disciplines. Here are some common applications of reliability:
Psychological and Educational Testing
Reliability is crucial in psychological and educational testing to ensure that the scores obtained from assessments are consistent and dependable. It helps to determine the accuracy and stability of measures such as intelligence tests, personality assessments, academic exams, and aptitude tests.
Market Research
In market research, reliability is important for ensuring consistent and dependable data collection. Surveys, questionnaires, and other data collection instruments need to have high reliability to obtain accurate and consistent responses from participants. Reliability analysis helps researchers identify and address any issues that may affect the consistency of the data.
Health and Medical Research
Reliability is essential in health and medical research to ensure that measurements and assessments used in studies are consistent and trustworthy. This includes the reliability of diagnostic tests, patient-reported outcome measures, observational measures, and psychometric scales. High reliability is crucial for making valid inferences and drawing reliable conclusions from research findings.
Quality Control and Manufacturing
Reliability analysis is widely used in industries such as manufacturing and quality control to assess the reliability of products and processes. It helps to identify and address sources of variation and inconsistency, ensuring that products meet the required standards and specifications consistently.
Social Science Research
Reliability plays a vital role in social science research, including fields such as sociology, anthropology, and political science. It is used to assess the consistency of measurement tools, such as surveys or observational protocols, to ensure that the data collected is reliable and can be trusted for analysis and interpretation.
Performance Evaluation
Reliability is important in performance evaluation systems used in organizations and workplaces. Whether it’s assessing employee performance, evaluating the reliability of scoring rubrics, or measuring the consistency of ratings by supervisors, reliability analysis helps ensure fairness and consistency in the evaluation process.
Psychometrics and Scale Development
Reliability analysis is a fundamental step in psychometrics, which involves developing and validating measurement scales. Researchers assess the reliability of items and subscales to ensure that the scale measures the intended construct consistently and accurately.
Examples of Reliability
Here are some examples of reliability in different contexts:
Test-Retest Reliability Example: A researcher administers a personality questionnaire to a group of participants and then administers the same questionnaire to the same participants after a certain period, such as two weeks. The scores obtained from the two administrations are highly correlated, indicating good test-retest reliability.
Inter-Rater Reliability Example: Multiple teachers assess the essays of a group of students using a standardized grading rubric. The ratings assigned by the teachers show a high level of agreement or correlation, indicating good inter-rater reliability.
Internal Consistency Reliability Example: A researcher develops a questionnaire to measure job satisfaction. The researcher administers the questionnaire to a group of employees and calculates Cronbach’s alpha to assess internal consistency. The calculated value of Cronbach’s alpha is high (e.g., above 0.8), indicating good internal consistency reliability.
Parallel Forms Reliability Example: Two versions of a mathematics exam are created, which are designed to measure the same mathematical skills. Both versions of the exam are administered to the same group of students, and the scores from the two versions are highly correlated, indicating good parallel forms reliability.
Split-Half Reliability Example: A researcher develops a survey to measure self-esteem. The survey consists of 20 items, and the researcher randomly divides the items into two halves. The scores obtained from each half of the survey show a high level of agreement or correlation, indicating good split-half reliability.
Alternate Forms Reliability Example: A researcher develops two versions of a language proficiency test, which are designed to measure the same language skills. Both versions of the test are administered to the same group of participants, and the scores from the two versions are highly correlated, indicating good alternate forms reliability.
Where to Write About Reliability in A Thesis
When writing about reliability in a thesis, there are several sections where you can address this topic. Here are some common sections in a thesis where you can discuss reliability:
Introduction :
In the introduction section of your thesis, you can provide an overview of the study and briefly introduce the concept of reliability. Explain why reliability is important in your research field and how it relates to your study objectives.
Theoretical Framework:
If your thesis includes a theoretical framework or a literature review, this is a suitable section to discuss reliability. Provide an overview of the relevant theories, models, or concepts related to reliability in your field. Discuss how other researchers have measured and assessed reliability in similar studies.
Methodology:
The methodology section is crucial for addressing reliability. Describe the research design, data collection methods, and measurement instruments used in your study. Explain how you ensured the reliability of your measurements or data collection procedures. This may involve discussing pilot studies, inter-rater reliability, test-retest reliability, or other techniques used to assess and improve reliability.
Data Analysis:
In the data analysis section, you can discuss the statistical techniques employed to assess the reliability of your data. This might include measures such as Cronbach’s alpha, Cohen’s kappa, or intraclass correlation coefficients (ICC), depending on the nature of your data and research design. Present the results of reliability analyses and interpret their implications for your study.
Discussion:
In the discussion section, analyze and interpret the reliability results in relation to your research findings and objectives. Discuss any limitations or challenges encountered in establishing or maintaining reliability in your study. Consider the implications of reliability for the validity and generalizability of your results.
Conclusion:
In the conclusion section, summarize the main points discussed in your thesis regarding reliability. Emphasize the importance of reliability in research and highlight any recommendations or suggestions for future studies to enhance reliability.
Importance of Reliability
Reliability is of utmost importance in research, measurement, and various practical applications. Here are some key reasons why reliability is important:
- Consistency : Reliability ensures consistency in measurements and assessments. Consistent results indicate that the measure or instrument is stable and produces similar outcomes when applied repeatedly. This consistency allows researchers and practitioners to have confidence in the reliability of the data collected and the conclusions drawn from it.
- Accuracy : Reliability is closely linked to accuracy. A reliable measure produces results that are close to the true value or state of the phenomenon being measured. When a measure is unreliable, it introduces error and uncertainty into the data, which can lead to incorrect interpretations and flawed decision-making.
- Trustworthiness : Reliability enhances the trustworthiness of measurements and assessments. When a measure is reliable, it indicates that it is dependable and can be trusted to provide consistent and accurate results. This is particularly important in fields where decisions and actions are based on the data collected, such as education, healthcare, and market research.
- Comparability : Reliability enables meaningful comparisons between different groups, individuals, or time points. When measures are reliable, differences or changes observed can be attributed to true differences in the underlying construct, rather than measurement error. This allows for valid comparisons and evaluations, both within a study and across different studies.
- Validity : Reliability is a prerequisite for validity. Validity refers to the extent to which a measure or assessment accurately captures the construct it is intended to measure. If a measure is unreliable, it cannot be valid, as it does not consistently reflect the construct of interest. Establishing reliability is an important step in establishing the validity of a measure.
- Decision-making : Reliability is crucial for making informed decisions based on data. Whether it’s evaluating employee performance, diagnosing medical conditions, or conducting research studies, reliable measurements and assessments provide a solid foundation for decision-making processes. They help to reduce uncertainty and increase confidence in the conclusions drawn from the data.
- Quality Assurance : Reliability is essential for maintaining quality assurance in various fields. It allows organizations to assess and monitor the consistency and dependability of their processes, products, and services. By ensuring reliability, organizations can identify areas of improvement, address sources of variation, and deliver consistent and high-quality outcomes.
Limitations of Reliability
Here are some limitations of reliability:
- Limited to consistency: Reliability primarily focuses on the consistency of measurements and findings. However, it does not guarantee the accuracy or validity of the measurements. A measurement can be consistent but still systematically biased or flawed, leading to inaccurate results. Reliability alone cannot address validity concerns.
- Context-dependent: Reliability can be influenced by the specific context, conditions, or population under study. A measurement or instrument that demonstrates high reliability in one context may not necessarily exhibit the same level of reliability in a different context. Researchers need to consider the specific characteristics and limitations of their study context when interpreting reliability.
- Inadequate for complex constructs: Reliability is often based on the assumption of unidimensionality, which means that a measurement instrument is designed to capture a single construct. However, many real-world phenomena are complex and multidimensional, making it challenging to assess reliability accurately. Reliability measures may not adequately capture the full complexity of such constructs.
- Susceptible to systematic errors: Reliability focuses on minimizing random errors, but it may not detect or address systematic errors or biases in measurements. Systematic errors can arise from flaws in the measurement instrument, data collection procedures, or sample selection. Reliability assessments may not fully capture or address these systematic errors, leading to biased or inaccurate results.
- Relies on assumptions: Reliability assessments often rely on certain assumptions, such as the assumption of measurement invariance or the assumption of stable conditions over time. These assumptions may not always hold true in real-world research settings, particularly when studying dynamic or evolving phenomena. Failure to meet these assumptions can compromise the reliability of the research.
- Limited to quantitative measures: Reliability is typically applied to quantitative measures and instruments, which can be problematic when studying qualitative or subjective phenomena. Reliability measures may not fully capture the richness and complexity of qualitative data, limiting their applicability in certain research domains.
Also see Reliability Vs Validity
About the author
Muhammad Hassan
Researcher, Academic Writer, Web developer
You may also like

Validity – Types, Examples and Guide

Alternate Forms Reliability – Methods, Examples...

Construct Validity – Types, Threats and Examples

Internal Validity – Threats, Examples and Guide

Reliability Vs Validity

Internal Consistency Reliability – Methods...
Reliability and validity: Importance in Medical Research
Affiliations.
- 1 Al-Nafees Medical College,Isra University, Islamabad, Pakistan.
- 2 Fauji Foundation Hospital, Foundation University Medical College, Islamabad, Pakistan.
- PMID: 34974579
- DOI: 10.47391/JPMA.06-861
Reliability and validity are among the most important and fundamental domains in the assessment of any measuring methodology for data-collection in a good research. Validity is about what an instrument measures and how well it does so, whereas reliability concerns the truthfulness in the data obtained and the degree to which any measuring tool controls random error. The current narrative review was planned to discuss the importance of reliability and validity of data-collection or measurement techniques used in research. It describes and explores comprehensively the reliability and validity of research instruments and also discusses different forms of reliability and validity with concise examples. An attempt has been taken to give a brief literature review regarding the significance of reliability and validity in medical sciences.
Keywords: Validity, Reliability, Medical research, Methodology, Assessment, Research tools..
Publication types
- Biomedical Research*
- Reproducibility of Results
- Open access
- Published: 26 May 2024
The sense of coherence scale: psychometric properties in a representative sample of the Czech adult population
- Martin Tušl 1 ,
- Ivana Šípová 2 ,
- Martin Máčel 2 ,
- Kristýna Cetkovská 2 &
- Georg F. Bauer 1
BMC Psychology volume 12 , Article number: 293 ( 2024 ) Cite this article
279 Accesses
Metrics details
Sense of coherence (SOC) is a personal resource that reflects the extent to which one perceives the world as comprehensible, manageable, and meaningful. Decades of empirical research consistently show that SOC is an important protective resource for health and well-being. Despite the extensive use of the 13-item measure of SOC, there remains uncertainty regarding its factorial structure. Additionally, a valid and reliable Czech version of the scale is lacking. Therefore, the present study aims to examine the psychometric properties of the SOC-13 scale in a representative sample of Czech adults.
An online survey was completed by 498 Czech adults (18–86 years old) between November 2021 and December 2021. We used confirmatory factor analysis to examine the factorial structure of the scale. Further, we examined the variations in SOC based on age and gender, and we tested the criterion validity of the scale using the short form of the Mental Health Continuum (MHC) scale and the Generalized Anxiety Disorder (GAD) scale as mental health outcomes.
SOC-13 showed an acceptable one- and three-factor fit only with specified residual covariance between items 2 and 3. We tested alternative short versions by systematically removing poorly performing items. The fit significantly improved for all shorter versions with SOC-9 having the best psychometric properties with a clear one-factorialstructure. We found that SOC increases with age and males score higher than females. SOC showed a moderately strong positive correlation with MHC, and a moderately strong negative correlation with GAD. These findings were similar for all tested versions supporting the criterion validity of the SOC scale.
Our findings suggest that shortened versions of the SOC-13 scale have better psychometric properties than the original 13-item version in the Czech adult population. Particularly, SOC-9 emerges as a viable alternative, showing comparable reliability and validity as the 13-item version and a clear one-factorial structure in our sample.
Peer Review reports
Sense of coherence (SOC) was introduced by the sociologist Aaron Antonovsky as the main pillar of his salutogenic theory, which explains how individuals cope with stressors and stay healthy even in case of adverse life situations [ 1 ]. SOC is a personal resource defined as a global orientation to life determining the degree to which one perceives life as comprehensible, manageable, and meaningful [ 2 ]. A strong SOC enables individuals to cope with stressors and manage tension, thus moving to the ease-end of the ease/disease continuum [ 2 , 3 ]. A person’s strength of SOC can be measured with the Orientation to Life Questionnaire commonly referred to as the SOC scale [ 4 ]. The original version is composed of 29 items (SOC-29) and Antonovsky recommended 13 items for the short version of the scale (SOC-13). To date, both versions of the scale have been used across diverse populations in at least 51 languages and 51 countries [ 5 ]. Studies have consistently shown that SOC correlates strongly with different health and well-being outcomes [ 6 , 7 ] and quality of life measures [ 8 ]. In the context of the recent COVID-19 pandemic, SOC has been identified as the most important protective resource in relation to mental health [ 9 ]. Regarding individual differences, SOC has been shown to strengthen over the life course [ 10 ], males usually score higher than females [ 11 ], and some studies indicate that SOC increases with the level of education [ 12 ]. However, despite the extensive evidence on the criterion validity of the scale, there is still a lack of clarity about its underlying factor structure and dimensionality.
The SOC scale was conceptualized as unidimensional suggesting that SOC in its totality, as a global orientation, influences the movement along the ease/dis-ease continuum [ 2 ]. However, the structure of the scale is rather multidimensional as each item is composed of multiple elements. Antonovsky developed the scale according to the facet theory [ 13 , 14 ] which assumes that social phenomena are best understood when they are seen as multidimensional. Facet theory involves the construction of a mapping sentence which consists of the facets and the sentence linking the facets together [ 15 ]. The SOC scale is composed of five facets: (i) the response mode (comprehensibility, manageability, meaningfulness); (ii) the modality of stimulus (instrumental, cognitive, affective), (iii) its source (internal, external, both), (iv) the nature of the demand it poses (concrete, diffuse, affective), (v) and its time reference (past, present, future). For example, item 3 “Has it happened that people whom you counted on disappointed you?” is a manageability item that can be described with the mapping sentence as follows: "Respondent X responds to an instrumental stimulus (“counted on”), which originated from the external environment (“people”), and which poses a diffuse demand (“disappointed”) being in the past (“has it happened”)." Although each item can be categorized along the SOC component comprehensibility, manageability, or meaningfulness, the items also share elements from the other four facets with items within the same, but also within the other SOC components (see 2, Chap. 4 for details). As Antonovsky states [ 2 , p. 87]: “The SOC facet pulls the items apart; the other facets push them together.”
Thus, the multi-facet nature of the scale can create difficulties in identifying the three theorized SOC components using statistical methods such as factor analysis. In fact, both the unidimensional and the three-dimensional SOC-13 rarely yield an acceptable fit without specifying residual covariance between single items (see 5 for an overview). This has been further exemplified in a recent study which examined the dimensionality of SOC-13 using a network perspective. The authors were unable to identify a clear structure and concluded that SOC is composed of multiple elements that are deeply linked and not necessarily distinct [ 16 ]. As a result, several researchers have suggested modified [ 17 ] or abbreviated versions of the scale, such as SOC-12 [ 18 , 19 ], SOC-11 [ 20 , 21 , 22 ], or SOC-9 [ 23 ], which have empirically shown a better factorial structure. This prompts the general question, whether an alternative short version should be preferred over the 13-item version. In fact, looking into the original literature [ 2 ], it is not clear why Antonovsky chose specifically these 13 items from the 29-item scale. We will address this question with the Czech version of the SOC-13 scale.
Salutogenesis in the Czech Republic
Salutogenesis and the SOC scale were introduced to the Czech audience in the early 90s by a Czech psychologist Jaro Křivohlavý. His work included the Czech translation of the SOC-29 scale [ 24 ] and the application of the concept in research on resilience [ 25 ] and behavioral medicine [ 26 ]. Unfortunately, the early Czech translation of the scale by Křivohlavý is not available electronically, nor could we locate it in library repositories. Later studies examined SOC-29 in relation to resilience [ 27 , 28 ] and self-reported health [ 29 , 30 ], however, it is not clear which translation of SOC-29 the authors used in the studies. A new Czech translation of the SOC-13 scale has recently been developed by the authors of this paper to examine the protective role of SOC for mental health during the COVID-19 crisis [ 31 ]. In line with earlier studies [ 9 ], SOC was identified as an important protective resource for individual mental health. This recent Czech translation of the SOC-13 scale [ 31 ] is the subject of the present study.
Present study
Our study aims to investigate the psychometric properties of the SOC-13 scale within a representative sample of the Czech adult population. Specifically, we will examine the factorial structure of the SOC-13 scale to understand its underlying dimensions and evaluate its internal consistency to ensure its reliability as a measure of SOC. Additionally, we aim to assess criterion validity by examining the scale’s association with established measures of positive and negative mental health outcomes - the Mental Health Continuum [ 32 ] and Generalized Anxiety Disorder [ 33 ]. We anticipate a strong correlation between these measures and the SOC construct [ 6 ]. Furthermore, we will investigate demographic variations in SOC, considering factors such as age, gender, and education. Understanding these variations will provide valuable insights into the applicability of the SOC-13 scale across different population subgroups. Finally, we will explore whether alternative short versions of the SOC scale should be preferred over the 13-item version. This analysis will help determine the most efficient version of the SOC scale for future research.
Study design and data collection
Our study design is a cross-sectional online survey of the Czech adult population. We contracted a professional agency DataCollect ( www.datacollect.cz ) to collect data from a representative sample for our study. Participants were recruited using quota sampling. The inclusion criteria were: being of adult age (18+), speaking the Czech language, and having permanent residence in the Czech Republic. Exclusion criteria related to study participation were predetermined to minimize the risk of biases in the collected data. The order of items in all measures was randomized and we implemented two attention checks in the questionnaire (e.g. “Please, choose option number 2”). Participants were excluded if they did not finish the survey, completed the survey in less than five minutes, did not pass the attention checks, or gave the same answer to more than 10 consecutive items. Data collection was conducted via the online platform Survey Monkey between November 2021 and December 2021.
Translation into the Czech language
Translation of the SOC scale was carried out by the authors of the paper with the help of a qualified translator. We followed the translation guidelines provided on the website of the Society for Research and Theory on Salutogenesis ( www.stars-society.org ), where the original English version of the SOC scale is available for download. Two translations were conducted independently, then compared and checked for differences. Based on this comparison, the agreed version of the scale was back translated into English by a Czech-English translator. The final version was checked for resemblance to the original version in content and in form. Although we used only the short version of the scale in our study (i.e., SOC-13), the translation included the full SOC-29 scale. The Czech translation of the full SOC scale is available as supplementary material.
Sense of coherence. We used the short version of the Orientation to Life Questionnaire [ 3 ] to assess SOC. The measure consists of 13 items evaluated on a 7-point Likert-type scale with different response options. Five items measure comprehensibility (e.g., “Does it happen that you experience feelings that you would rather not have to endure?”), four items measure manageability (e.g., “Has it happened that people whom you counted on disappointed you?”), and four items measure meaningfulness (e.g., “Do you have the feeling that you really don’t care about what is going on around you?”). In our sample, Cronbach’s alpha for the full scale was α = 0.88, for comprehensibility α = 0.76, manageability α = 0.72, and meaningfulness α = 0.70.
Mental health continuum - short form (MHC-SF; 32). This scale consists of 14 items that capture three dimensions of well-being: (i) emotional (e.g. “During the past month, how often did you feel interested in life?”); (ii) social (e.g. “During the past month, how often did you feel that the way our society works makes sense to you?”); (iii) psychological (e.g. “During the past month, how often did you feel confident to think or express your own ideas and opinions?”). The items assess the experiences the participants had over the past two weeks, the response options ranged from 1 (never) to 6 (every day). Internal consistency of the scale was α = 0.90.
Generalized anxiety disorder (GAD; 33). The scale consists of seven items that measure symptoms of anxiety over the past two weeks. Sample items include, e.g. “Over the past two weeks, how often have you been bothered by the following problems?” (i) “feeling nervous, anxious, or on edge”, (ii) “worrying too much about different things”, (iii) “becoming easily annoyed or irritable”. The response options ranged from 0 (not at all) to 3 (almost every day). Internal consistency of the scale was α = 0.92.
Sociodemographic characteristics included age, gender, and level of education (i.e., primary/vocational, secondary, tertiary).
Analytical procedure
Data analysis was conducted in R [ 34 ]. For confirmatory factor analysis, we used the cfa function of the lavaan package 0.6–16 [ 35 ]. We compared a one-factor model of SOC-13 to a correlated three-factor model (correlated latent factors comprehensibility, manageability, and meaningfulness) and a bi-factor model (general SOC dimension and specific dimensions comprehensibility, manageability, meaningfulness). Based on the empirical findings we further assessed the fit of alternative shorter versions of the SOC scale. We assessed the model fit using the comparative-fit index (CFI), Tucker-Lewis index (TLI), root mean square error of approximation (RMSEA), and standardized root mean square residual (SRMR) with the conventional cut-off values. The goodness-of-fit values for CFI and TLI surpassing 0.90 indicate an acceptable fit and exceeding 0.95 a good fit [ 36 ]. A value under 0.08 for RMSEA and SRMR indicates a good fit [ 37 ]. Nested models were compared using chi-square difference tests and the Bayesian Information Criterion (BIC). Models with lower BIC values should be preferred over models with higher BIC values [ 38 ]. All models were fitted using maximum likelihood estimation.
Further, we used the cor function of the stats package 4.3.2 [ 34 ] for Pearson correlation analysis to explore the association between SOC-13 and age, the t.test function of the same package for between groups t-test for differences based on gender, and the aov function with posthoc tests of the same package for one-way between-subjects ANOVA to test for differences based on level of education. To examine the criterion validity of the scale, we used the cor function for Pearson correlation analysis to examine the associations between SOC-13, MHC-SF, and GAD. We conducted the same analyses for the alternative short versions of the scale.
Participants
The median survey completion time was 11 min. In total, 676 participants started the survey and 557 completed it. Of those, 56 were excluded due to exclusion criteria. One additional respondent was excluded because of dubious responses on demographic items (e.g., 100 years old and a student), and two respondents were excluded for not meeting the inclusion criteria (under 18 years old). The final sample included N = 498 participants. Of those, 53.4% were female, the average age was 49 years ( SD = 16.6; range = 18–86), 43% had completed primary, 35% secondary, and 22% tertiary education. The sample is a good representation of the Czech adult population Footnote 1 with regard to gender (51% females), age ( M = 50 years), and education level (44% primary, 33% secondary, 18% tertiary). Representativeness was tested using chi-squared test which yielded non-significant results for all domains.
Descriptive statistics
In Table 1 , we present an inter-item correlation matrix along with skewness, kurtosis, means and standard deviations of single items for SOC-13. Item correlations ranged from r = 0.07 (items 2 and 4) to r = 0.67 (items 8 and 9). Strong and moderately strong correlations were found also across the three SOC dimensions (e.g., r = 0.77 comprehensibility and manageability).
- Confirmatory factor analysis
A one-factor model showed inadequate fit to the data [χ2(65) = 338.2, CFI = 0.889, TLI = 0.867, RMSEA = 0.092, SRMR = 0.062]. Based on existing evidence [ 6 ], we specified residual covariance between items 2 and 3 and tested a modified one-factor model. The model showed an acceptable fit to the data [χ2(64) = 242.6, CFI = 0.927, TLI = 0.911, RMSEA = 0.075, SRMR = 0.050], and it was superior to the one-factor model (Δχ2 = 95.5, Δ df = 1, p < 0.001).
A correlated three-factor model showed an acceptable fit considering CFI and SRMR [χ2(63) = 286.6, CFI = 0.909, TLI = 0.885, RMSEA = 0.085, SRMR = 0.058]. The model was superior to the one-factor model (Δχ2 = 51.5, Δ df = 2, p < 0.001), however, it was inferior to the modified one-factor model (ΔBIC = -56). We further tested a modified three-factor model with residual covariance between items 2 and 3 which showed an acceptable fit to the data based on CFI and TLI and a good fit based on RMSEA and SRMR [χ2(62) = 191.7, CFI = 0.947, TLI = 0.932, RMSEA = 0.066, SRMR = 0.046]. The model was superior to the three-factor model (Δχ2 = 97.1, Δ df = 1, p < 0.001) as well as to the modified one-factor model (Δχ2 = 50.9, Δ df = 3, p < 0.001). See Fig. 1 for a detailed illustration of the model.
Finally, we tested a bi-factor model with one general SOC factor and three specific factors (comprehensibility, manageability, meaningfulness), however, the model was not identified.
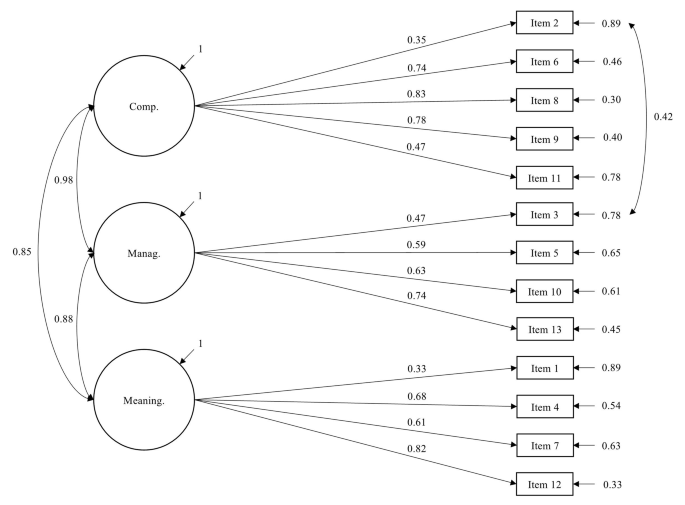
Correlated three-factor model of SOC-13 with residual covariance between item 2 and item 3
Alternative short versions of the SOC scale
We further tested the fit of alternative shorter versions of the SOC scale by systematically removing poorly performing items. In SOC-12, item 2 was excluded (“Has it happened in the past that you were surprised by the behavior of people whom you thought you knew well?”). This item measures comprehensibility, hence SOC-12 has even distribution of items for each dimension (i.e., comprehensibility, manageability, meaningfulness). Item 2 has previously been identified as problematic [ 6 ] and also in our sample it did not perform well in any of the fitted SOC-13 models (i.e., low factor loading and explained variance). A one-factor SOC-12 model showed an acceptable fit to the data based on CFI and TLI and a good fit based on RMSEA and SRMR [χ2(54) = 221.1, CFI = 0.927, RMSEA = 0.079, SRMR = 0.048]. A correlated three-factor model showed an acceptable fit based on CFI and TLI and a good fit based on RMSEA and SRMR [χ2(52) = 171.1, CFI = 0.948, TLI = 0.932, RMSEA = 0.069 SRMR = 0.043]. The model was superior to the one-factor model (Δχ2 = 50, Δ df = 3, p < 0.001). Bi-factor model was not identified.
In SOC-11, we removed item 3 (“Has it happened that people whom you counted on disappointed you?”), which measures manageability. The item had the lowest factor loading and the lowest explained variance in the one-factor SOC-12. A one-factor SOC-11 model showed a good fit to the data [χ2 (44) = 138.5, CFI = 0.955, TLI = 0.944, RMSEA = 0.066, SRMR = 0.038]. A correlated three-factor model was identified but not acceptable due to covariance between comprehensibility and manageability higher than 1 (i.e., Heywood case; 39).
In SOC-10, we removed item 1 (“Do you have the feeling that you don’t really care about what goes on around you?”), which measures meaningfulness. The item had the lowest factor loading and the lowest explained variance in one-factor SOC-11. A one-factor SOC-10 model showed a good fit to the data [χ2 (35) = 126.6, CFI = 0.956, TLI = 0.943, RMSEA = 0.072, SRMR = 0.039]. As in the case of SOC-11, a correlated three-factor model was identified but not acceptable due to covariance between comprehensibility and manageability higher than 1.
Finally, in SOC-9, we removed item 11 (“When something happened, have you generally found that… you overestimated or underestimated its importance / you saw the things in the right proportion”), which measures comprehensibility. The item had the lowest factor loading and the lowest explained variance in one-factor SOC-10. SOC-9 has an even distribution of three items for each dimension. A one-factor model showed a good fit to the data [χ2 (27) = 105.6, CFI = 0.959, TLI = 0.946, RMSEA = 0.076, SRMR = 0.038]. As in the previous models, a correlated three-factor model was identified but not acceptable due to covariance between comprehensibility and manageability higher than 1. See Fig. 2 for an illustration of one-factor SOC-9 model. Detailed results of the confirmatory factor analysis are shown in Table 2 . In Table 3 , we present the items of the SOC-13 (and SOC-9) scale with details about their facet structure.
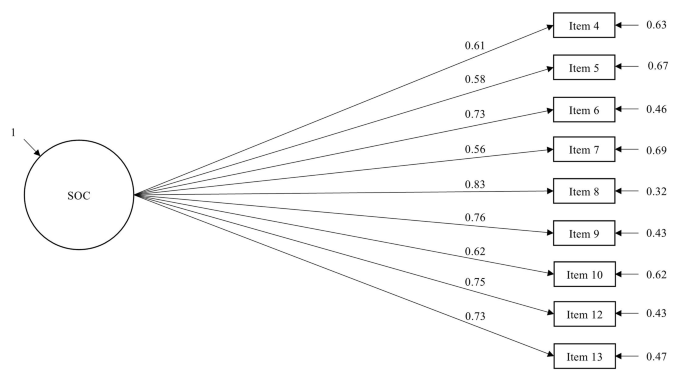
One-factor model of SOC-9
Differences by gender, age, and education
Correlation analysis indicated that SOC-13 increases with age ( r = 0.32, p < 0.001), this finding was identical for all alternative short versions of the SOC scale (see Table 2 ). Further, the results of the two-tailed t-test showed that males ( M = 4.8, SD = 1.08) had a significantly higher SOC-13 score [ t (497) = 3.06, p = 0.002, d = 0.27] than females ( M = 4.5, SD = 1.07). A one-way between-subjects ANOVA did not show any significant effect of level of education on SOC-13 score [F(2, 497) = 1.78, p = 0.169, η p 2 = 0.022]. These results were similar for all alternative short versions of the SOC scale.
Criterion validity
We found a moderately strong positive correlation ( r = 0.61, p < 0.001) between SOC-13 and the positive mental health measure MHC, and a moderately strong negative correlation between SOC-13 and the negative mental health measure GAD ( r = -0.68, p < 0.001). These findings were similar for all alternative short versions of the SOC scale (see Table 4 ).
Our study examined the psychometric properties of the SOC-13 scale and its alternative short versions SOC-12, SOC-11, SOC-10, and SOC-9 in a representative sample of the Czech adult population. In line with existing studies [ 40 ], we found that SOC increases with age and that males score higher than females. In contrast to some prior findings [ 12 ], we did not find any significant differences in SOC based on the level of education. Further, we tested criterion validity using both positive and negative mental health outcomes (i.e., MHC and GAD). SOC had a strong positive correlation with MHC and a strong negative correlation with GAD, thus adding to the evidence about the criterion validity of the scale [ 6 , 40 ].
Analysis of the factor structure showed that a one-factor SOC-13 had an inadequate fit to our data, however, an acceptable fit was achieved for a modified one-factor model with specified residual covariance between item 2 (“Has it happened in the past that you were surprised by the behavior of people whom you thought you knew well?”) and item 3 (“Has it happened that people whom you counted on disappointed you?”). A correlated three factor model with latent factors comprehensibility, manageability, and meaningfulness showed a better fit than the one factor-model. However, it was also necessary to specify residual covariance between item 2 and item 3 to reach an acceptable fit for all fit indices. A recent Slovenian study [ 41 ] found a similar result and several prior studies (see 6 for an overview) have noted that items 2 and 3 of the SOC-13 scale are problematic. Although the items pertain to different SOC dimensions (item 2 to comprehensibility, item 3 to manageability), multiple studies [e.g., 20 , 42 , 43 ] have reported moderately strong correlation between them and this is also the case in our study ( r = 0.5, p < 0.001). The two items aptly illustrate the facet theory behind the scale construction as the SOC component represents only one building block of each item. Although items 2 and 3 theoretically pertain to different SOC components, they share the same elements from the other four facets (i.e., modality, source, demand, and time) which is reflected in the similarity of their wording. Therefore, they will necessarily share residual variance and this needs to be specified to achieve a good model fit. Drageset and Haugan [ 18 ] explain this similarity in that the people whom we know well are usually the ones that we count on, and feeling disappointed and surprised by the behavior of people we know well is closely related. Therefore, it should be theoretically justifiable to specify residual covariance between item 2 and item 3 as a possible solution to improve the fit. As we could show in our sample, the model fit significantly improved for both one-factor and three-factor solutions.
In addition, we examined the fit of alternative short versions of the SOC scale by systematically removing single items that performed poorly. First, in line with previous studies [ 6 ], we addressed the issue of residual covariance in SOC-13 by removing item 2, examining the factor structure of SOC-12. The remaining 12 items were equally distributed within the three SOC components with four items per each component. Interestingly, a one-factor model reached an acceptable fit and the fit further improved for a correlated three-factor model with latent factors of comprehensibility, manageability, and meaningfulness. Although correlated three-factor models were superior to one-factor models, we observed extreme covariances between latent variables, especially in case of comprehensibility and manageability (cov = 0.98). This suggests that the SOC components are not empirically separable and that, indeed, SOC is rather a one-dimensional global orientation with multiple components that are dynamically interrelated as Antonovsky proposed [ 2 ]. This notion was supported in a recent study that explored the dimensionality of the scale using a network perspective [ 16 ]. Our examination of SOC-11, SOC-10 and SOC-9 provided further support for a one-factor structure of the scale. All shorter versions yielded a good one-dimensional fit, however, we could not identify a correlated three-factor model fit due to the Heywood case. This refers to the situation when a solution that otherwise is satisfactory produces communality greater than one explained by the latent factor, which implies that the residual variance of the variable is negative [ 39 ]. In our case, this was true for the latent factors comprehensibility and manageability. However, we demonstrated that we could attain a good one-dimensional fit for all alternative short versions of SOC, and, importantly, they all showed comparable reliability and validity metrics to their longer counterpart SOC-13. In particular, SOC-9 shows very good fit indices and it performs equally well in validity analyses as SOC-13. Given these findings and existing evidence [ 5 ], we propose that future investigations may consider utilizing the SOC-9 scale instead of the SOC-13. It is interesting to point out that the majority of items that were removed for the shorter versions of the scale are negatively worded or reverse-scored (expect for item 11). This is in line with the latest research suggesting that such items can cause problems in model identification as they create additional method factors [ 44 , 45 , 46 ].
Finally, it is important to highlight that Antonovsky did not provide any information about the selection of the 13 items for the short version of the SOC scale [ 2 ]. For example, a detailed examination of the facet structure reveals that none of the items included in SOC-13 refers to future which is part of facet referring to time (i.e., past, present, future). Hence, considering the absence of explicit criteria for item selection in the SOC-13 scale, it would be interesting to gather data from diverse populations utilizing the full SOC-29 scale. Subsequently, through exploratory factor analysis, researchers could derive a new, theory- and empirical-driven, short version of the SOC scale.
Strengths and limitations
A clear strength of our study is that our findings are based on a representative sample that accurately reflects the Czech adult population. Moreover, we implemented rigorous data cleaning procedures, meticulously excluding participants who provided potentially careless or low-quality responses. By doing so, we ensured that our conclusions are based on high-quality data and that they are generalizable to our target population of Czech adults. Finally, we conducted a thorough back-translation procedure to achieve an accurate Czech version of the SOC scale and we carried out systematic testing of different short versions of the SOC scale.
However, our study also has some limitations. First, our conclusions are based on data from a culturally specific country and they may not be generalizable to other populations. It is important to note, however, that most of our findings are in line with multiple existing studies which supports the validity of our conclusions. Second, the data were collected during a later stage of the COVID-19 pandemic, which may have impacted particularly the mental health outcomes we used for criterion validity. It would be worthwhile to investigate whether the data replicate in our population outside of this exceptional situation. Third, it should be noted that we did not examine test-retest reliability of the scale due to the cross-sectional design of our study. Finally, self-reported data are subject to common method biases such as social desirability, recall bias, or consistency motive [ 47 ]. We aimed to minimize this risk by implementing various strategies in the questionnaire, such as randomization of items and the use of disqualifying items (e.g. “Please, choose option number 2”) to disqualify careless answers.
Our study contributes to decades of ongoing research on SOC, the main pillar of the theory of salutogenesis. In line with existing research, we found evidence for the validity of the SOC as a construct, but we could not identify a clear factorial structure of the SOC-13 scale. However, following Antonovsky’s conception of the scale, we believe it is theoretically sound to aim for a one-factor solution of the scale and we could show that this is possible with shorter versions of the SOC scale. We particularly recommend using the SOC-9 scale in future research which shows an excellent one-factor fit and validity indices comparable to SOC-13. Finally, since Antonovsky does not explain how he selected the items of the SOC-13 scale, it would be interesting to examine the possibility of developing a new one-dimensional short version based on exploratory factor analysis of the original SOC-29 scale.
Data availability
The datasets used and analyzed during the current study and the R code used for the statistical analysis are available as supplementary material.
www.czso.cz .
Antonovsky A. The salutogenic model as a theory to guide health promotion. Health Promot Int. 1996;11(1).
Antonovsky A. Unraveling the mystery of Health how people manage stress and stay well. Jossey-Bass; 1987.
Antonovsky A. Health stress and coping. Jossey-Bass; 1979.
Antonovsky A. The structure and Properties of the sense of coherence scale. Soc Sci Med. 1993;36(6):125–733.
Article Google Scholar
Eriksson M, Contu P. The sense of coherence: Measurement issues. The Handbook of Salutogenesis. Springer International Publishing; 2022. pp. 79–91.
Eriksson M. The sense of coherence: the Concept and its relationship to Health. The Handbook of Salutogenesis. Springer International Publishing; 2022. pp. 61–8.
Eriksson M, Lindström B. Antonovsky’s sense of coherence scale and the relation with health: a systematic review. J Epidemiol Community Health (1978). 2006;60(5):376–81.
Eriksson M, Lindström B. Antonovsky’s sense of coherence scale and its relation with quality of life: a systematic review. J Epidemiol Community Health. 2007;61(11):938–44.
Article PubMed PubMed Central Google Scholar
Mana A, Super S, Sardu C, Juvinya Canal D, Moran N, Sagy S. Individual, social and national coping resources and their relationships with mental health and anxiety: A comparative study in Israel, Italy, Spain, and the Netherlands during the Coronavirus pandemic. Glob Health Promot [Internet]. 2021;28(2):17–26.
Silverstein M, Heap J. Sense of coherence changes with aging over the second half of life. Adv Life Course Res. 2015;23:98–107.
Article PubMed Google Scholar
Rivera F, García-Moya I, Moreno C, Ramos P. Developmental contexts and sense of coherence in adolescence: a systematic review. J Health Psychol. 2013;18(6):800–12.
Volanen SM, Lahelma E, Silventoinen K, Suominen S. Factors contributing to sense of coherence among men and women. Eur J Public Health [Internet]. 2004;14(3):322–30.
Guttman L. Measurement as structural theory. Psychometrika. 1971;3(4):329–47.
Guttman R, Greenbaum CW. Facet theory: its development and current status. Eur Psychol. 1998;3(1):13–36.
Shye S. Theory Construction and Data Analysis in the behavioral sciences. San Francisco: Jossey-Bass; 1978.
Google Scholar
Portoghese I, Sardu C, Bauer G, Galletta M, Castaldi S, Nichetti E, Petrocelli L, Tassini M, Tidone E, Mereu A, Contu P. A network perspective to the measurement of sense of coherence (SOC): an exploratory graph analysis approach. Current Psychology. 2024;12:1-3.
Bachem R, Maercker A. Development and psychometric evaluation of a revised sense of coherence scale. Eur J Psychol Assess. 2016;34(3):206–15.
Drageset J, Haugan G. Psychometric properties of the orientation to Life Questionnaire in nursing home residents. Scand J Caring Sci. 2016;30(3):623–30.
Kanhai J, Harrison VE, Suominen AL, Knuuttila M, Uutela A, Bernabé E. Sense of coherence and incidence of periodontal disease in adults. J Clin Periodontol. 2014;41(8):760–5.
Naaldenberg J, Tobi H, van den Esker F, Vaandrager L. Psychometric properties of the OLQ-13 scale to measure sense of coherence in a community-dwelling older population. Health Qual Life Outcomes. 2011;9.
Luyckx K, Goossens E, Apers S, Rassart J, Klimstra T, Dezutter J et al. The 13-item sense of coherence scale in Dutch-speaking adolescents and young adults: structural validity, age trends, and chronic disease. Psychol Belg. 2012;52(4):351–68.
Lerdal A, Opheim R, Gay CL, Moum B, Fagermoen MS, Kottorp A. Psychometric limitations of the 13-item sense of coherence scale assessed by Rasch analysis. BMC Psychol. 2017;5(1).
Klepp OM, Mastekaasa A, Sørensen T, Sandanger I, Kleiner R. Structure analysis of Antonovsky’s sense of coherence from an epidemiological mental health survey with a brief nine-item sense of coherence scale. Int J Methods Psychiatr Res. 2007;16(1):11–22.
Křivohlavý J. Sense of coherence: methods and first results. II. Sense of coherence and cancer. Czechoslovak Psychol. 1990;34:511–7.
Křivohlavý J. Nezdolnost v pojetí SOC. Czechoslovak Psychol. 1990;34(6).
Křivohlavý J. Salutogenesis and behavioral medicine. Cas Lek Cesk. 1990;126(36):1121–4.
Kebza V, Šolcová I. Hlavní Koncepce psychické odolnosti. Czechoslovak Psychol. 2008;52(1):1–19.
Šolcová I, Blatný M, Kebza V, Jelínek M. Relation of toddler temperament and perceived parenting styles to adult resilience. Czechoslovak Psychol. 2016;60(1):61–70.
Šolcová I, Kebza V, Kodl M, Kernová V. Self-reported health status predicting resilience and burnout in longitudinal study. Cent Eur J Public Health. 2017;25(3):222–7.
Šolcová I, Kebza V. Subjective health: current state of knowledge and results of two Czech studies. Czechoslovak Psychol. 2006;501:1–15.
Šípová I, Máčel M, Zubková A, Tušl M. Association between coping resources and mental health during the COVID-19 pandemic: a cross-sectional study in the Czech Republic. Int J Environ Health Res. 2022;1–9.
Keyes CLM. The Mental Health Continuum: from languishing to flourishing in life. J Health Soc Behav. 2002;43(2):207–22.
Löwe B, Decker O, Müller S, Brähler E, Schellberg D, Herzog W, et al. Validation and standardization of the generalized anxiety disorder screener (GAD-7) in the General Population. Med Care. 2008;46(3):266–74.
R Core Team. R: a language and environment for statistical computing. Vienna, Austria: R Foundation for Statistical Computing; 2022.
Rosseel Y. Lavaan: an R Package for Structural equation modeling. J Stat Softw. 2012;48(2):1–36.
Bentler PM, Bonett DG. Significance tests and goodness of fit in the analysis of covariance structures. Psychol Bull. 1980;88(3):588–606.
Beauducel A, Wittmann WW. Simulation study on fit indexes in CFA based on data with slightly distorted simple structure. Struct Equ Model. 2005;12(1):41–75.
Raftery AE. Bayesian model selection in Social Research. Sociol Methodol. 1995;25:111–63.
Farooq R. Heywood cases: possible causes and solutions. Int J Data Anal Techniques Strategies. 2022;14(1):79.
Eriksson M, Lindström B. Validity of Antonovsky’s sense of coherence scale: a systematic review. J Epidemiol Community Health (1978). 2005;59(6):460–6.
Stern B, Socan G, Rener-Sitar K, Kukec A, Zaletel-Kragelj L. Validation of the Slovenian version of short sense of coherence questionnaire (SOC-13) in multiple sclerosis patients. Zdr Varst. 2019;58(1):31–9.
PubMed PubMed Central Google Scholar
Bernabé E, Tsakos G, Watt RG, Suominen-Taipale AL, Uutela A, Vahtera J, et al. Structure of the sense of coherence scale in a nationally representative sample: the Finnish Health 2000 survey. Qual Life Res. 2009;18(5):629–36.
Sardu C, Mereu A, Sotgiu A, Andrissi L, Jacobson MK, Contu P. Antonovsky’s sense of coherence scale: cultural validation of soc questionnaire and socio-demographic patterns in an Italian Population. Clin Pract Epidemiol Mental Health. 2012;8:1–6.
Chyung SY, Barkin JR, Shamsy JA. Evidence-based Survey Design: the Use of negatively worded items in surveys. Perform Improv. 2018;57(3):16–25.
Suárez-Alvarez J, Pedrosa I, Lozano LM, García-Cueto E, Cuesta M, Muñiz J. Using reversed items in likert scales: a questionable practice. Psicothema. 2018;30(2):149–58.
PubMed Google Scholar
van Sonderen E, Sanderman R, Coyne JC. Ineffectiveness of reverse wording of questionnaire items: let’s learn from cows in the rain. PLoS ONE. 2013;8(7).
Podsakoff PM, MacKenzie SB, Lee JY, Podsakoff NP. Common method biases in behavioral research: a critical review of the literature and recommended remedies. J Appl Psychol. 2003;88(5):879–903.
Download references
Acknowledgements
The authors would like to thank to the team of Center of Salutogenesis at the University of Zurich for their helpful comments on the adapted version of the SOC scale.
MT received funding from the European Union’s Horizon 2020 research and innovation program under the Marie Skłodowska-Curie grant agreement No 801076, through the SSPH + Global PhD Fellowship Program in Public Health Sciences (GlobalP3HS) of the Swiss School of Public Health. Data collection was supported by the Charles University Strategic Partnerships Fund 2021. The University of Zurich Foundation supported the contribution of GB.
Author information
Authors and affiliations.
Division of Public and Organizational Health, Center of Salutogenesis, Epidemiology, Biostatistics and Prevention Institute, University of Zurich, Hirschengraben 84, Zurich, 8001, Switzerland
Martin Tušl & Georg F. Bauer
Department of Psychology, Faculty of Arts, Charles University, Prague, Czech Republic
Ivana Šípová, Martin Máčel & Kristýna Cetkovská
You can also search for this author in PubMed Google Scholar
Contributions
All authors contributed to the conception and design of the study. MT wrote the manuscript, conducted data analysis, and contributed to data collection. MM and IS conducted data collection, contributed to data analysis, interpretation of results, edited and commented on the manuscript. KC and GB contributed to interpretation of results, edited and commented on the manuscript. All authors have read and approved the final manuscript.
Corresponding author
Correspondence to Martin Tušl .
Ethics declarations
Ethics approval and consent to participate.
The study was conducted in accordance with the general principles of the Declaration of Helsinki and with the ethical principles defined by the university and by the national law ( https://cuni.cz/UK-5317.html ). Informed consent was obtained from all participants prior to the completion of the survey. Participation was voluntary and participants could withdraw from the study at any time without any consequences. For anonymous online surveys in adult population no ethical review by an ethics committee was necessary under national law and university rules. See: https://www.muni.cz/en/about-us/organizational-structure/boards-and-committees/research-ethics-committee/evaluation-request .
Consent for publication
Not applicable.
Competing interests
The authors declare no competing interests.
Additional information
Publisher’s note.
Springer Nature remains neutral with regard to jurisdictional claims in published maps and institutional affiliations.
Electronic supplementary material
Below is the link to the electronic supplementary material.
Supplementary Material 1
Supplementary material 2, supplementary material 3, rights and permissions.
Open Access This article is licensed under a Creative Commons Attribution 4.0 International License, which permits use, sharing, adaptation, distribution and reproduction in any medium or format, as long as you give appropriate credit to the original author(s) and the source, provide a link to the Creative Commons licence, and indicate if changes were made. The images or other third party material in this article are included in the article’s Creative Commons licence, unless indicated otherwise in a credit line to the material. If material is not included in the article’s Creative Commons licence and your intended use is not permitted by statutory regulation or exceeds the permitted use, you will need to obtain permission directly from the copyright holder. To view a copy of this licence, visit http://creativecommons.org/licenses/by/4.0/ . The Creative Commons Public Domain Dedication waiver ( http://creativecommons.org/publicdomain/zero/1.0/ ) applies to the data made available in this article, unless otherwise stated in a credit line to the data.
Reprints and permissions
About this article
Cite this article.
Tušl, M., Šípová, I., Máčel, M. et al. The sense of coherence scale: psychometric properties in a representative sample of the Czech adult population. BMC Psychol 12 , 293 (2024). https://doi.org/10.1186/s40359-024-01805-7
Download citation
Received : 22 March 2023
Accepted : 21 May 2024
Published : 26 May 2024
DOI : https://doi.org/10.1186/s40359-024-01805-7
Share this article
Anyone you share the following link with will be able to read this content:
Sorry, a shareable link is not currently available for this article.
Provided by the Springer Nature SharedIt content-sharing initiative
- Salutogenesis
- Sense of coherence
- Psychometrics
- Czech adult population
- Mental health
BMC Psychology
ISSN: 2050-7283
- General enquiries: [email protected]
Enhancing student engagement through emerging technology integration in STEAM learning environments
- Open access
- Published: 24 May 2024
Cite this article
You have full access to this open access article
- Mirjana Maričić ORCID: orcid.org/0000-0001-8447-7735 1 &
- Zsolt Lavicza ORCID: orcid.org/0000-0002-3701-5068 2
244 Accesses
Explore all metrics
Emerging technologies can potentially transform education through student engagement. The aim of our study is threefold. Firstly, we aspired to examine the validity and reliability of Reeve and Tsengs’ 4-construct (emotional, behavioral, cognitive, and agentic) engagement scale (EBCA scale). Secondly, we aimed to examine whether and to what extent the integration of emerging technology through virtual simulations (VS) in STEAM activities can improve students’ perceived engagement. Thirdly, we strived to examine how the order of integration of VS in STEAM activities affects students’ perceived engagement. A cross-over research design was used. 84 primary school students (9–10 years old) were assigned to one of the following conditions: STA (science + technology + art); SA (science + art); STA + SA; and SA + STA. The results showed that the 4-factor EBCA scale model is aligned and fits the overall sample well. It was also observed that the longer students are involved in STEAM activities, the better their perceived engagement is, and the more they work on VS, the more they develop the values of attentive listening, directing attention, and investing effort in learning. The order of integration of VS affects perceived engagement, and students who learn with them first perceive engagement better. One of the implications of our study is to examine the metric characteristics of the EBCA scale on different samples as well. Other recommendations are stated in the discussion.
Similar content being viewed by others
Social Learning Theory—Albert Bandura
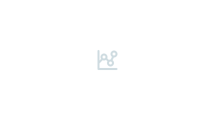
AI and education: the importance of teacher and student relations
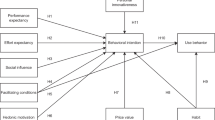
Students’ Acceptance of ChatGPT in Higher Education: An Extended Unified Theory of Acceptance and Use of Technology
Avoid common mistakes on your manuscript.
1 Introduction
Emerging technologies could have the potential to transform the education system and are currently considered one of the most engaging ways for students to learn the content of various scientific disciplines (Anđić et al., 2024 ; Chen & Chu, 2024 ; Leavy et al., 2023 ; Moreno-Guerrero et al., 2021 ). The role of emerging technologies within STEAM education is not fully understood due to the lack of quality theoretical frameworks, practitioner knowledge, and empirical evidence in educational research (Leavy et al., 2023 ). In particular, little is known about the potential of integrating certain emerging technologies, like virtual simulations (VS), into STEAM learning environments (Thisgaard & Makransky, 2017 ). A limited number of studies found on this topic dealt with determining the contribution of VS, mainly to the development of the following variables: student achievement, scientific inquiry, reasoning and scientific process skills, interest, goals toward STEM-related careers, STEM awareness, and students’ perceptions of STEM activities (D’Angelo et al., 2014 ; Sarı et al., 2020 ; Thisgaard & Makransky, 2017 ). These studies, as well as the meta-analysis by the authors Perignat and Katz-Buonincontro ( 2018 ), which analyzed 44 studies on the topic of identifying the intention of STEAM approaches, singled out the promotion of student engagement as a basic feature of STEAM. Student engagement largely determines all other teaching and learning outcomes. However, this variable was not tested in the mentioned studies but was observed as an indirect construct without determining the method of its measurement. Through indirect observation, it was noticed that the development of student engagement is influenced by instructional practice, the structure of lectures, as well as the interactions of participants in the teaching process (Nicol & Macfarlane-Dick, 2006 ; Wang et al., 2015 ). Given the limited amount of research on this topic and certain methodological ambiguities, it remains important that educators communicate their classroom teaching experiences regarding student engagement (Barlow & Brown, 2020 ). It is particularly recommended to consider factors relevant to the development of this variable (such as instructional practice and content delivery methods) as well as instruments that will directly measure student engagement within STEAM learning environments (Barlow & Brown, 2020 ). Identified research gaps (1. a limited number of studies as well as a lack of understanding of the role and potential of certain emerging technologies, like VS within STEAM education; 2. a lack of research on this topic within which student engagement was measured directly with an appropriate instrument) served as the basis for the implementation of our research. For these purposes, we selected primary school students and the emotional, behavioral, cognitive, and agentic EBCA scale for assessing student engagement as a 4-component construct developed by the authors Reeve and Tseng ( 2011 ). Given that this instrument is intended for students of higher education, we had to modify or adapt it to the needs of our study and check its metric characteristics. With this in mind, we have set a threefold aim. Firstly, we aspired to examine the validity and reliability of the EBCA engagement scale. Secondly, we aimed to examine whether and to what extent the integration of VS in STEAM activities can improve students’ perceived engagement. Thirdly, we strived to examine whether and how the order of integration of VS in STEAM activities affects students’ perceived engagement.
2 Emerging technology integration into STEAM environment
According to the latest reports (Leavy et al., 2023 ), emerging technologies are defined as tools or software that could have the potential to radically transform the current state of education and thus enable more creative and engaging ways of learning and teaching (Leavy et al., 2023 ; Sosa et al., 2017 ). According to this, the following technologies are currently considered “emerging” for education: artificial intelligence (AI), big data, learning analytics, immersive technologies such as virtual reality (VR), augmented reality (AR), and mixed reality (MR), virtual labs and simulations (VS), serious games, robotics, the internet of things, hardware with sensors, wearable devices, and drones (Leavy et al., 2023 ). Integrating emerging technologies into STEAM activities is considered one of the most engaging ways for students to learn content from various scientific disciplines (Anđić et al., 2022, 2023 ; Janković et al., 2023 ; Moreno-Guerrero et al., 2021 ). The ‘T’ letter in the STEAM acronym is primarily used for solving various engineering challenges, programming, or designing computer graphics. These activities aim to research and design active, innovative, creative solutions and create artifacts (digital or otherwise) by balancing technical expertise with artistic vision and expressing knowledge and skills in the global world (Glancy, 2014 ; Jones, 2014 ). Its value, as well as the value of using technology, primarily has long-term aspirations. They are reflected in the transition from a consumer to a producer society, i.e., training the young generations to provide socio-technical contributions using simpler examples in the STEAM classroom and, in the future, complex and useful ones for society (Boy, 2013 ). Within the STEAM approach, the shift from knowledge consumption to production via emerging technology-enabled tools in a collaborative environment is a way for students to contribute to the closer community, learn from each other, and acquire skills in these areas that are necessary for the future (Boy, 2013 ).
In a recent meta-analysis, Leavy et al. ( 2023 ) reviewed and analyzed 43 qualitative empirical studies on the topic of identifying emerging technologies that are used to strengthen STEAM education. These papers are classified into the following categories: (1) AR/VR/MR; (2) Programming and Robotics; (3) Maker Movement; and (4) Other Technological Applications. The general importance of the integration of the mentioned emerging technologies into the STEAM approach is reflected in the development of 21st -century skills such as creativity, persistence, and problem solving, attitudes towards computing, creative thinking, and learning in this way through promoting engagement, collaborative problem solving, hands-on learning experiences, and providing strong motivation to promote equity (Leavy et al., 2023 ). However, the authors state that this meta-analysis is limited in providing insight into how emerging technologies can transform and influence learning due to the lack of quality theoretical frameworks, practitioner knowledge, and empirical evidence. Also, bearing in mind that this field is developing, there is a possibility that not all examples of good practice are included due to poor dissemination and recording of the results. Furthermore, we will list a few examples of papers that were not included in this meta-analysis.
In a study by Laut et al. ( 2015 ), STEM activities are empowered with robotics to develop and understand the connection between biology and engineering. The students were given the task of completing their biomimetic robotic fish through STEM and letting the finished product be verified at the New York Aquarium to observe the fish’s response in biology. The results of this study showed that robotics can strengthen STEM learning and contribute to students’ understanding of the connection between these two disciplines. In the study by Techakosit and Nilsook ( 2018 ), the contribution of the integration of AR within STEM activities to the development of STEM literacy was examined. The results showed that, for learning the STEM contents, imagination, design ability, finding information, and using STEM’s basic abilities to solve problems were very important skills that students develop while learning in this way. Further, Chen and Huang ( 2020 ) investigated the contribution of serious game-based learning to the strengthening of STEAM activities from the aspect of improving achievement and reducing the cognitive load when working with primary school students (13–14 years old). The results of this study showed that game-based learning can strengthen STEAM activities and contribute to the development of student achievements and the reduction of cognitive load. Emerging technologies have the potential to initiate inevitable and necessary changes in the educational system through redefining and reshaping teaching that is consistent with STEAM principles (Leavy et al., 2023 ). What is needed to utilize and maximize the opportunities of these technologies within the STEAM approach is a reconceptualization of school programs, teaching, learning, and assessment methods (Meletiou-Mavrotheris, 2019 ). We will further look at the potential of integrating certain emerging technologies into the STEAM approach, i.e., we will focus on the integration of VS in the STEAM learning environment.
3 Integration of virtual simulations into STEAM environment
Virtual simulations (VS) imply computer modeling of reality, i.e., computer-based representations of real or hypothesized scientific phenomena and processes, with which students, in an interactive way in a virtual environment, become familiar with the mental models of scientists and construct their own to understand and explain certain scientific phenomena (Falloon, 2019 ; Sanina et al., 2020 ; Zhang, 2014 ). They offer the possibility of observing scientific processes visible and invisible to the naked eye, as well as the possibility of visualizing abstract, less abstract, and non-abstract concepts (e.g., electrons, molecules, light rays) (Maričić et al., 2023 ; Olympiou et al., 2013 ). The basic intention of this visualization is reflected in the transformation of abstract phenomena, i.e., theoretical-conceptual constructions into perceptual representations, to build a bridge between the students’ understanding of those concepts in the natural environment and the mechanism of their actual functioning (Sanina et al., 2020 ). In addition, VS offers the possibility of simplifying the investigated phenomenon or process by highlighting the target elements being observed and removing complexity, or it can be modified to a simpler or shorter time frame to more easily interpret certain natural phenomena (de Jong et al., 2013 ; Maričić et al., 2023 ). VS appear in the form of computer-based animations such as models, simulations, and experiments (Falloon, 2019 ). All these forms offer students the opportunity to enter a micro-virtual world where they can manipulate virtual equipment, materials, and variables of interest and immediately access the obtained results (Scalise et al., 2011 ; Wen et al., 2020 ). Through virtual models, simulations, and experiments, students can observe and investigate those natural phenomena and processes that are not easy to observe and investigate in real-life circumstances (Zhang, 2014 ). In addition to the above, they can be more manageable, more flexible, safer, more profitable, and faster to implement than real hands-on activities (Wen et al., 2020 ).
When working on VS, students encounter two processes: transformation and regulation. In the process of transformation, students produce direct information by forming hypotheses, designing experiments, and concluding. Through the process of regulation, students connect the variables, conditions, and events presented in the problem, identify key variables, and visualize the conditions of the simulation (Lim, 2004 ; Sarı et al., 2020 ). As a result of these processes, we can notice that VS can play an active role in the STEAM learning environment in terms of supporting the research process and providing modeling opportunities (Sarı et al., 2020 ). These processes can then be carried out through real hands-on activities, which include the integration of other disciplines such as engineering, art, and mathematics. Within them, research support is strengthened through previous manipulation of the phenomenon or process in virtual conditions, visualization of the invisible, simplification of reality, regulation of the time frame, and manipulation of the variable of interest, while the modeling process can be performed more faithfully and creatively through the design of active, innovative, creative solutions using knowledge of engineering and mathematics and the creation of artifacts through balancing real material with artistic vision. Thus, VS can strengthen and support STEAM learning, and students can express their skills and knowledge through different disciplines.
Although VS are considered promising emerging technologies that can support STEAM learning, very little is known about their potential in research practice (Thisgaard & Makransky, 2017 ). In a meta-analysis by D’Angelo et al. ( 2014 ), which dealt with determining the contribution of VS within the STEM approach, 59 studies were reviewed. The results of this meta-analysis showed that VS can strengthen STEM activities in terms of student achievement, scientific inquiry, reasoning skills, and non-cognitive outcomes. Although this meta-analysis showed that VS can strengthen STEM learning, the authors state that it is necessary to carry out more research to gain insight into the benefits of VS within the STEM domain. The research by Thisgaard and Makransky ( 2017 ) examined the contribution of VS to students’ knowledge of evolution, interest, and whether simulations could catalyze STEM academic and career development. High school students (18 years old) were supposed to identify an unknown animal found on the beach through VS while investigating various aspects of natural selection and genetics through video displays of genetic links and 3D visualization of a population of a species on an island. The results of this study showed that VS can strengthen STEM learning in terms of developing student interest and goals toward STEM-related careers. Sarı et al. ( 2020 ) analyzed the contribution of VS within STEM activities to the development of students’ scientific process skills, STEM awareness, and views on activities. Second-year undergraduate students participated in the research. The results showed that VS can strengthen STEM learning from the perspective of these variables and that students believe that STEM activities provide numerous advantages, such as designing and developing engineering products, conducting experiments, and reducing errors.
The contribution of VS was examined within STEM learning, focusing mainly on the following variables: student achievement, scientific inquiry, reasoning and scientific process skills, interest, goals toward STEM-related careers, STEM awareness, and students’ perceptions of STEM activities (D’Angelo et al., 2014 ; Sarı et al., 2020 ; Thisgaard & Makransky, 2017 ). These studies, as well as the meta-analysis by Perignat and Katz-Buonincontro ( 2018 ), which reviewed 44 studies on the topic of STEAM approaches (i.e., identifying the purpose of STEAM education, definitions of STEAM acronyms, and definitions of ‘A’ in STEAM), single out the engagement of students as the basic feature of STEAM education within these disciplines. However, student engagement was not tested in these studies but was observed as an indirect construct without determining the method of its measurement. In the next section, we will focus on this variable.
4 Involvment/engagement theory
The understanding of the concept of student engagement was contributed by Astin ( 1984 ), who studied student development for more than 20 years. Instead of the term engagement, Atkin uses the term involvement and focuses on college students. According to him, student involvement refers to the amount of physical and psychological energy that students devote to the academic experience (Astin, 1984 ). This determination is based on the following five postulates, shown in Fig. 1 .
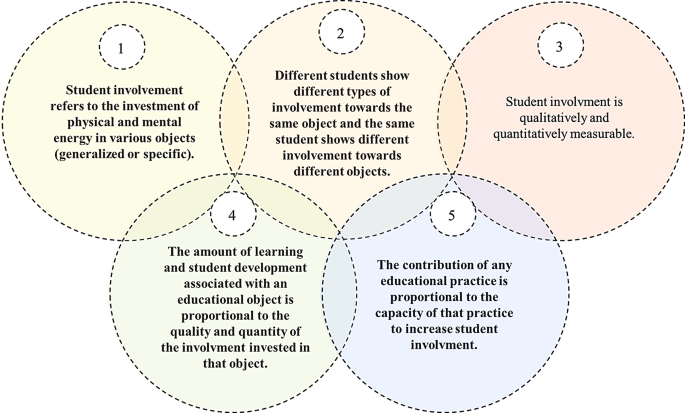
Postulates of student involvment
Atkin’s definition was later expanded by the director of the National Survey of Student Engagement, George Kuh, who states that engagement, in addition to the investment of physical and mental energy of the participants in the educational process, also represents the effort of the institution that it invests in using an effective educational performance (Axelson & Flick, 2010 ). Later, the determinations of student engagement became more and more complex, taking into account different aspects of education, but what they all have in common is that an educational institution with an educational system is not only a place where acquired knowledge is transferred from individual to individual but also a place where different types of relationships develop. These relationships exist between the participants in the educational process (the social component) as well as between the participants and the learning object (the intellectual component), and they are characterized by a certain emotional flow. Bearing that in mind, according to modern understandings, engagement is defined as a state of emotional, social, and intellectual readiness for learning, which is characterized by curiosity, participation, and the drive to learn more (Abla & Fraumeni, 2019 ). These connections can be observable, like visible behavior, but also unobservable, like internal attitudes. With that in mind, the authors Fredricks et al. ( 2004 ) identified three different types of engagement: emotional, behavioral, and cognitive, while Reeve and Tseng ( 2011 ) described a fourth type: agentic engagement (see Fig. 2 ).
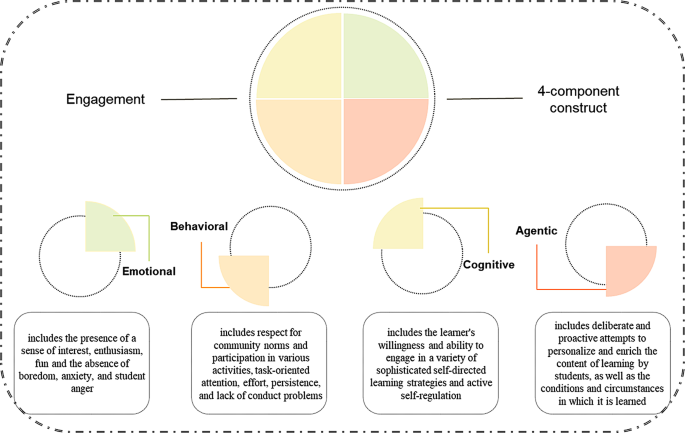
Engagement as a 4-component construct
Therefore, engagement can be defined as a multi-dimensional construct. Within STEAM education, it has been observed that this variable largely determines all other teaching and learning outcomes for students (Barlow & Brown, 2020 ; Hong et al., 2020 ; Khamhaengpol et al., 2021 ). As previously stated, student engagement in these studies was not directly measured but was observed as an indirect construct. Through indirect observation, it was noticed that the development of student engagement is primarily influenced by instructional practice, the structure of lectures (and exams), as well as the interactions of participants in the teaching process (Nicol & Macfarlane-Dick, 2006 ; Wang et al., 2015 ). However, given the limited amount of research on this topic and certain methodological ambiguities within it, it remains important that instructors and educators consider and communicate their practical classroom teaching experiences regarding student engagement (Barlow & Brown, 2020 ). In doing so, it is particularly recommended to take into account factors important for the development of this variable, including the structure of lectures, content delivery methods, and student interactions, as well as instruments that will measure this variable in a direct way within the STEAM learning environments (Barlow & Brown, 2020 ).
5 Purpose of the study
Based on a detailed review of the literature, the following research gaps were identified: (1) a limited number of studies as well as a poor understanding of the role and potential of certain emerging technologies like VS within STEAM education; (2) a lack of research on this topic in which student engagement was directly measured with an appropriate instrument. To fulfill the mentioned research gaps, we decided to conduct this study. For these purposes, we selected primary school students and the scale for measuring emotional, cognitive, behavioral, and agentic engagement—the EBCA scale by Reeve and Tseng ( 2011 ). As the EBCA scale is intended for high school students, we had to modify it, adapt it to the needs of our research, and check its metric characteristics. With this in mind, we have set a threefold aim. Firstly, we aspired to examine the validity and reliability of the EBCA engagement scale. Secondly, we aimed to examine whether and to what extent the integration of VS in STEAM activities can improve students’ perceived engagement. Thirdly, we strived to examine whether and how the order of integration of VS in STEAM activities affects students’ perceived engagement. The following research questions arise from the stated three-fold aim:
Can the EBCA engagement scale be used validly and reliably in the primary school context?
Whether and to what extent the integration of VS in STEAM activities can improve students’ perceived engagement?
Whether and how the order of integration of VS in STEAM activities affects students’ perceived engagement?
6 Methodology
6.1 research design.
The research was carried out according to the cross-over research design (Crowder & Hand, 2017 ; Hughes et al., 2022 ), in which the students of the experimental groups undergo all STEAM (STA and SA) learning conditions but only in a different order. The research design is shown in Fig. 3 .
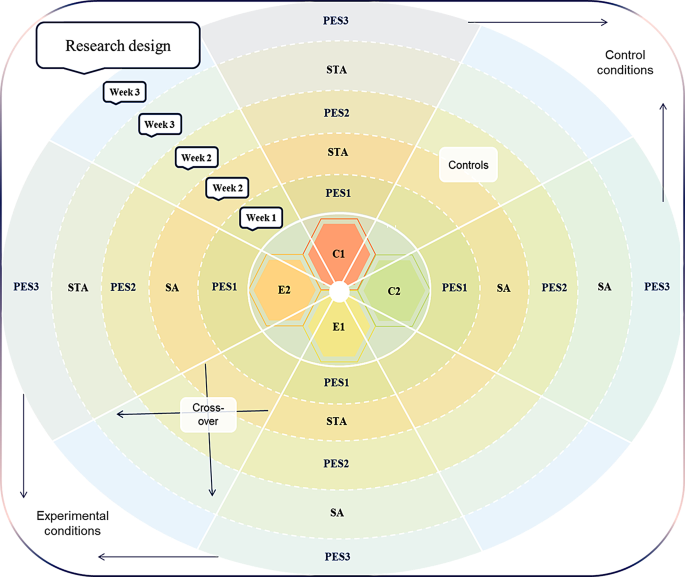
Research design
This Pre—Post—Post-Delayed engagement assessment design was used to collect measurement outcomes before, during and after the intervention (Craig et al., 2012 ). Such a design allowed us to gain insight into whether and to what extent the integration of VS into STEAM activities can improve students’ perceived engagement, as well as how the order of VS integration in STEAM activities affects students’ perceived engagement.
For this research, schools from the district were recruited, and classes of 3rd -grade students that were available to the researcher were selected. A convenience sampling method was applied. The students in the selected classes were given a pre-engagement scale (PES1) to determine the level of their previous perceived engagement in science classes. Those classes of students who showed an approximate perception of previous engagement in the classes were retained in the research. PES1 was used as one of the criteria for equalizing the groups (and as a covariate in the analysis of the results). Selected classes of students were then randomly assigned to one of the STEAM conditions: STA (science, technology, and art) or SA (science and art). Through the combination of these STEAM conditions and the usage of cross-over design, four groups were formed: two control (C1 and C2) and two experimental (E1 and E2): C1 - STA + STA, C2 - SA + SA, E1 - STA + SA, and E2 - SA + STA. After the formation of the groups, the first lesson was held in C1 and E1 (STA lesson), and C2 and E2 (SA lesson). Then the students were given a post-engagement scale (PES2) to determine the level of their perceived engagement after participating in the first part of the intervention. Next week, the second lesson was held in C1 and E2 (STA lesson) and C2 and E1 (SA lesson). After the end of the second lesson, the students were given a delayed post-engagement scale (PES3) to establish their level of perceived engagement after participating in the second part of the intervention.
6.2 Intervention
For the implementation of STEAM activities, the science content Magnetism was selected. The first lesson included the following concepts: what is a magnet, the shapes of a magnet, the poles of a magnet, the lines of force of a magnetic field, attraction and repulsion, and action through different environments. The second lesson included the following concepts: magnetization, magnetic field strength, natural and artificial magnets (make an artificial magnet), and the effect of magnets in different environments (make a boat). These scientific contents are strengthened and integrated with the contents of art: landscape and abstract art (in the first lesson, abstract art , and the second lesson, landscape ). In addition to the concepts of abstract art and landscape, elements of visual art are also integrated into the lessons to introduce a science concept. These elements included the following: observing works of art, painting examples of abstract art and landscapes, and creating original works of art that also present scientific concepts about magnetism. Through the integration of the content of the sciences and arts with technology, the STA condition was formed. Technology integration referred to the introduction of VS (from the JavaLab series) on magnetism to strengthen the understanding of the scientific concepts of these contents. VS offers the possibility of visualizing those abstract concepts that students cannot see with the naked eye, such as the lines of force of the magnetic field and their behavior during the approaching of the same and different poles of the magnet, the concept of magnetization, the formation of domains within metals, and their orientation. By integrating the content of the sciences and arts (without technology), the SA condition was formed. Basic STEAM conditions are shown in Fig. 3 .
By combining the STA and SA conditions through a cross-over design, two more conditions were formed - STA + SA and SA + STA. STEAM activities will be briefly described below.
6.2.1 STA and SA conditions
All students were introduced to the intervention in the same way. They were told the story of the shepherd Magnus - how the ore magnetite was discovered and how the term magnetism came about. During this conversation, students were shown an example of this ore.
STA condition : Lesson 1 – The students were then shown paintings from the series Magnetic North: Imagining Canada in paintings by seven famous Canadian painters (abstract). Through a conversation with the researcher about the paintings, the displayed techniques, and the fascinating name of the entire collection of these works, they came up with the term magnetism. This term is then connected to the term from the story of the shepherd Magnus. Then, through hands-on activities, the students went over the following concepts: what is a magnet, the shapes of a magnet, the poles of a magnet, the lines of force of a magnetic field, attraction and repulsion, and the action of a magnet in different environments. Through VS from the JavaLab series about magnets, students strengthened their knowledge about magnetism, magnetic fields, magnet poles, and magnetic field lines of force. After this part, students were introduced to the concept abstract art. They were shown paintings by famous abstract artists, such as Clyfford Still. The students discussed the paintings and communicated what impressed them, i.e., what was magnetic about them. After that, with the usage of different art materials and media, the students were placed in a position to create their magnetic abstract work. Then, through the main activity, students had to create an original 2D artwork that integrates elements of science and art. The students painted their abstract work of art with magnets through the property of magnets acting through different environments .
Lesson 2 - The students were shown paintings from the series Magnetic North: Imagining Canada in paintings by Canadian artists, but this time the landscape ones. The researcher introduced the students to the concept of magnetism through a conversation about the paintings, the techniques shown, and the fascinating name of the collection of these works. This concept is connected with the concept from the story of the shepherd Magnus. The students then went through the following concepts through hands-on activities: magnetization, magnetic field strength, natural and artificial magnets (make an artificial magnet), and the effect of magnets in different environments (make a boat). Through VS, students strengthened their knowledge of magnetism and magnetization, magnetic fields, and natural and artificial magnets. After this, students were introduced to the concept of landscape. They were shown paintings by famous landscape painters from the Barbizon School. The students discussed the paintings and communicated what impressed them, i.e., what was magnetic about them. After that, with the usage of different art materials and media, the students were placed in a position to create their own magnetic landscape. Then the main activity was introduced, in which the students had to create an original 3D artwork that integrates elements of science and art. The idea was to create an original 3D interactive landscape—an image of a landscape in which a part of the artwork is integrated, which can be moved by a magnet and make it interactive.
SA condition : This condition included the integration of science and art in both lessons, but without technology, i.e. all those elements (in the same order) from the STA condition were represented here, only without the usage of VS.
STA + SA condition : Within this condition, the first lesson was performed under the STA condition, while the second was carried out under the SA condition (without technology).
SA + STA condition : This condition implied that the first lesson was performed according to the SA condition, while the second was carried out according to the STA condition.
6.3 Sampling
84 3rd -grade students (9–10 years old, M = 9.643, SD = 0.482) from two primary schools in Eastern Europe participated in the research. The classes were recruited from schools attended by students with a diverse body: students from national minorities and different ethnic backgrounds, as well as students who learn according to the IEP. In the research, those classes of students that showed an approximate perception of previous engagement on PES1 and those students within those classes who filled out all three PESs were retained. For this research, four classes of 3rd -grade students were recruited and randomly assigned to one of the STEAM conditions. The random distribution in our research was performed so that already-formed classes were randomly assigned to one of the four STEAM conditions (in each of 21 students). Teacher bias was excluded by introducing a trained researcher into the intervention. Including all students in both STA and SA conditions allowed us to monitor the impact of the order of VS integration on students’ perceived engagement.
6.4 Data collection
Data in this research were collected using a previously created instrument, the EBCA scale, for assessing students’ perceived emotional, behavioral, cognitive, and agentic engagement by Reeve and Tseng ( 2011 ). Given that this scale is intended to measure the perceived engagement of high school students, we had to adapt it to the needs of our study to successfully assess the perceived engagement of primary school students. These adaptations were also reflected in the slight modification of the items, which resulted in the creation of three scales: PES1, PES2, and PES3 (for example, on PES1, the items are directed to the state before the implementation of the intervention, on PES2, the items are directed to the state immediately after the first part of the intervention, and on PES3, the items are directed to the state after the implementation of the intervention). Before conducting the research, permission was requested from the author to adapt the scale. Adaptation resulted in several rounds of revision in which some items were excluded. During this process, experts in the field of methodology were consulted, as well as teachers with work experience spanning over 10 years, as the first assessors of the validity of the scale. The revised scale was adapted for 84 primary school students, and for the second round of checking construct validity and reliability, confirmatory factor analysis (CFA) was performed. The scale consists of four blocks, of which the emotional block has four items, the behavioral block has five items, the cognitive block has five items (the original has eight, i.e., three items from this block were excluded), and the agentic block has five items. These items are intended to assess four different types of students’ perceived engagement. Within emotional engagement, the following values were monitored: enjoyment, fun, interest, and curiosity. As part of the behavioral engagement, the following values are followed: careful listening, paying attention, trying hard, careful listening about new topics, and trying hard when starting something new. Within cognitive engagement, the following values were monitored: relating to prior knowledge, relating to personal experience, connecting different ideas into a meaningful whole, creating own examples to understand the concepts, and reviewing what was done. Within agentic engagement, these values are followed: asking questions to make the class active and lively; informing the teacher about personal interests; informing the teacher about the need to improve achievement; informing the teacher about preferences; and suggesting ideas for class improvement. The obtained results for each type of engagement, as well as the discussion about them, will be shown in the next two sections, but in such a way that these data follow each part of our threefold aim.
7.1 First part: Construct validity and reliability of the EBCA scale
The skewness and kurtosis values for PES1, PES2 and PES3 are between -2 and + 2 which shows that the data is normally distributed (Byrne, 2010 ; Hair et al., 2010 ). Kaiser-Meyer-Olkin (KMO) and Bartlett’s Test of Sphericity tests were used to determine the suitability of the data for confirmatory factor analysis (CFA). The KMO and Bartlett’s Test of Sphericity values for PES1, PES2 and PES3 were found to be statistically significant ( p < .000). It was ensured that the sample size was sufficient for data analysis (Tabachnick & Fidell, 2007 ) (see Table 1 ).
The obtained values were accepted as an indication that CFA could be performed. IBM SPSS AMOS program was used for CFA. In the upcoming paragraphs, we will present the CFA results for each scale.
Within CFA results, we monitored the values of various fit indices, which are primarily used to assess the fit of the model to the data. As a result of the analysis conducted on 19 items, the RMSEA values for PES1, PES2 and PES3 were found to be within acceptable range. Fit indices for PES1 show that this scale fits the overall sample well (χ 2 (140, N = 84) = 183.437, p = .008; CFI = 0.977, TLI = 0.972, RMSEA = 0.061, SRMR = 0.076). Covariance of error terms based on modification indices (MI > 20) was created for six pairs which improved the model. The final model is shown in Fig. 4 .
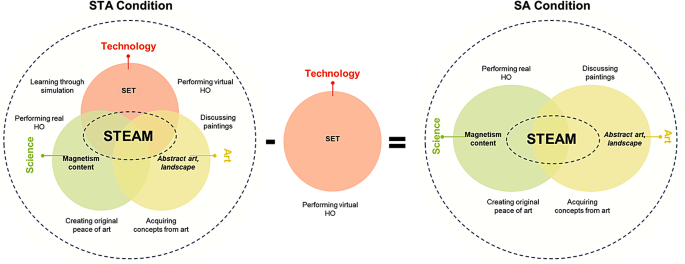
STA and SA conditions
Convergent validity and composite reliability (CR) of PES1 are also good. All factor loadings have a value above 0.60 (Fig. 4 ). Average variance extracted (AVE) values are above 0.05, and CR values are above 0.70 for all constructs (Hair et al., 2017 ). Cronbach alpha (CA) values are also above 0.70. Discriminative validity of the scale is good - the square root of AVE values (bold values) are higher than inter-variable values (below bold values) (Fornell & Larcker, 1981 ) (Table 2 ).
Fit indices for PES2 show that this scale fits the overall sample also well (χ 2 (146, N = 84) = 197.611, p = .003, CFI = 0.939, TLI = 0.929, RMSEA = 0.065, SRMR = 0.065) (Fig. 5 ).
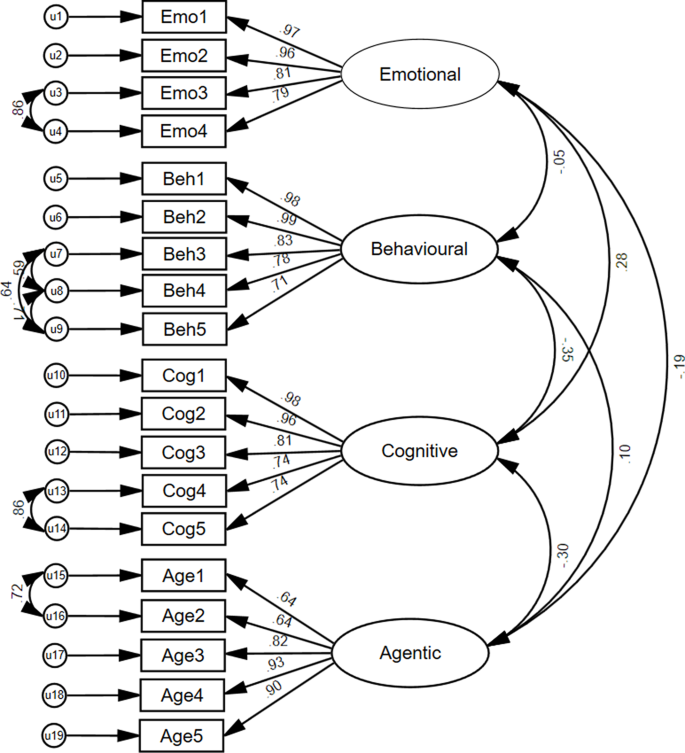
Measurement model of PES1
Convergent validity and composite reliability (CR) of PES2 are also good. Factor loadings are above 0.60 (Fig. 5 ), AVE values are above 0.05, CR and CA values are above 0.70 for all constructs. The discriminative validity of the scale is good - the square root of AVE values is higher than inter-variable values (Table 3 ).
Fit indices for PES3 show that this scale fits the overall sample well (χ 2 (145, N = 84) = 189.682, p = .007, CFI = 0.948, TLI = 0.939, RMSEA = 0.061, SRMR = 0.064). Covariance of error terms based on modification indices (for one pair - MI > 20) was created for one pair which improved the model. The final model is shown in Fig. 6 .
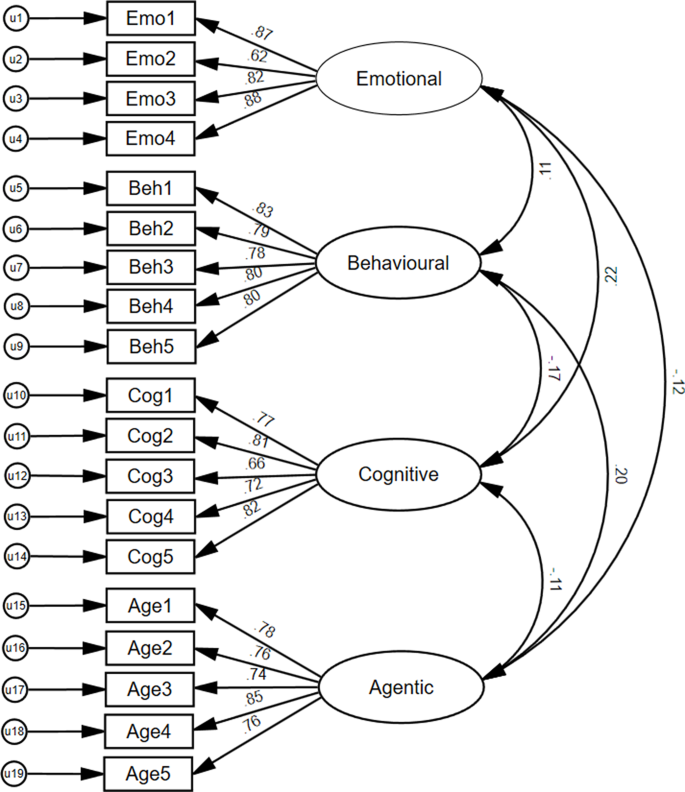
Measurement model of PES2
Convergent validity and composite reliability (CR) of PES3 are also good. Factor loadings are above 0.60 (Fig. 6 ), AVE values are above 0.05, CR and CA values are above 0.70 for all constructs. The discriminative validity of the scale is good - the square root of AVE values is higher than inter-variable values (Table 4 ).
Since the data showed a normal distribution, parametric tests were used for further analysis. A repeated measures ANOVA was used to determine the difference in student-perceived engagement between the three different time points. The ANOVA and ANCOVA analysis where used to determine whether there was a difference in the students’ perceived engagement between different STEAM conditions at PES1, PES2 and PES3. An independent t-test was used to determine whether there was a difference in the order of VS integration. These analyzes cover the second and third parts of the aim Fig. 7 .
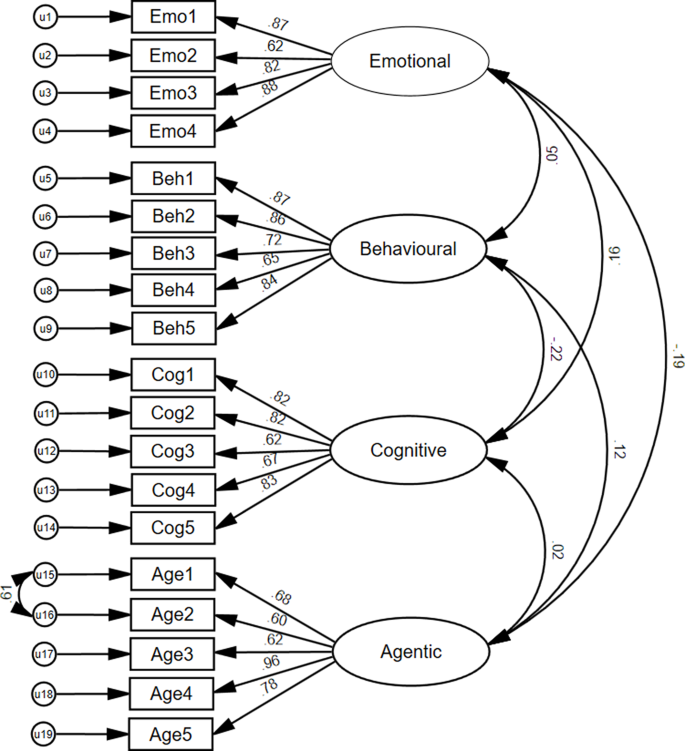
Measurement model of PES3
7.2 Second part - contribution of the VS in STEAM activities
One-factor ANOVA analysis of repeated measures compared the difference in students’ perceived engagement between three different time points - PES1, PES2 and PES3. The results of this analysis for all groups indicate a significant influence of time for all types of engagement, i.e. that the level of perceived emotional, behavioral, cognitive and agentic engagement changed significantly during these three-time points (Table 5 ).
These differences were further processed, to establish between which time points within each type of engagement there was a significant difference. These results are shown in (Table 6 ).
Based on these results, it can be observed that there are significant differences between PES1 and PES2, as well as PES1 and PES3 within each type of engagement, while significant differences between PES2 and PES3 exist within behavioral, cognitive and agentic engagement.
Further analyses considered the differences between the groups. ANOVA analysis found that there was no significant difference in PES1 in terms of perceived engagement ( F (3, 80) = 0.484, p = .695, C1 M = 3.386, SD = 0.299; C2 M = 3.429, SD = 0.375; E1 M = 3.470, SD = 0.405; E2 M = 3.512, SD = 0.320). PES1 scores served as a covariate for ANCOVA analysis.
ANCOVA analysis found that there was a significant difference on PES2 regarding perceived engagement ( F (3, 79) = 6.980, p = .000, ηp 2 = 0.210, covariate under control F (3, 79) = 27.407, p = .000, ηp 2 = 0.258). Through further analysis, we tried to determine within which type of engagement and between which groups this difference exists. The results showed that there was a difference in behavioral engagement ( F (3, 79) = 3.835, p = .013, ηp 2 = 0.127, covariate controlled F (3, 79) = 94.359, p = .000, ηp 2 =. 544) between the STA and SA condition ( p = .024) and the STA and SA + STA condition ( p = .034), where the students of the C1 group ( M = 4.324, SD = 0.171) showed significantly better results compared to the students of C2 ( M = 4.076, SD = 0.462) and E2 groups ( M = 4.124, SD = 0.449).
ANCOVA analysis found that there was a significant difference in PES3 regarding perceived engagement ( F (3, 79) = 7.977, p = .000, ηp 2 = 0.233, controlled covariate F (3, 79) = 19.732, p = .000, ηp 2 = 0.200). Through further analysis, we tried to determine within which type of engagement and between which groups this difference exists. The results showed that there was a difference in terms of behavioral engagement ( F (3, 79) = 5.031, p = .003, ηp 2 = 0.160, covariate under control F (3, 79) = 82.300, p = .000, ηp 2 =. 510) between the STA and SA conditions ( p = .003), where the students of the C1 group ( M = 4.419, SD = 0.374) showed significantly better results compared to the C2 students ( M = 4.124, SD = 0.403).
7.3 Third part - contribution of the VS integration order
The results of independent t-test showed that the p -value is close to the significance threshold t (40) = 1.753, p = .087, but does not exceed it. The students of the E1 group ( M = 4.279, SD = 0.170) showed better results compared to the students of the E2 group ( M = 4.183, SD = 0.184), which indicates that the VS integration in the first part of STEAM intervention contributes to a greater extent to the development of student perceived engagement compared to integration of VS in the second part of STEAM intervention.
8 Discussion
8.1 first part: construct validity and reliability of the ebca scale.
The results of our research show that the 4-factor engagement scale model is aligned, i.e., it fits the overall sample well. It should be noted that for the PES1 and PES3 scales, the covariance of the error term was created based on the modification indices for some pairs, which further improved their model fit. In view of this, it is suggested to check the fitness of the model on another sample and, if necessary, make modifications or remove certain items from the scale. Similar results were found in the research of Ritoša et al. ( 2020 ), in which the model fit of three constructs of engagement was checked: emotional, behavioral, and cognitive (the construct of emotional disaffection was added to the scale) on a sample of students from preschool (ages 6–7). After the modifications based on the modification indices, this scale showed a good fit. The engagement scale with all four constructs was tested in the research of Maričić et al. ( 2023 ) on a sample of primary school students (10–11 years old) and in the research by Zainuddin et al. ( 2020 ) on a sample of students from secondary school (16 years old), but regardless of the modifications that were made by the needs of the research, the model fit was not checked. The model fit of the original scale was checked by authors Reeve and Tseng ( 2011 ) on a sample of students from high school (over 16 years old). The 4-factor model proved to be adequate. Regarding the convergent validity, reliability, and discriminative validity of the engagement scales (PES1, PES2, and PES3), good results were obtained in our study, and this shows that the engagement scale in this form can be validly and reliably used in an educational context when working with primary school students (ages 9–10). Similar results were observed in the research of Ritoša et al. ( 2020 ). It is important to indicate that our results are limited in terms of generalization because the model fit was checked on a smaller sample of students aged 9–10 from Eastern Europe. The modified EBCA scale should be tested in work with students of different grade levels and from different ethnic and cultural backgrounds, which will improve the generalization of the results and affect their applicability on a more global level.
8.2 Second part - contribution of the VS in STEAM activities
The results further show that the level of perceived emotional, behavioral, cognitive, and agentic engagement changes significantly over time, i.e., the longer students are involved in STEAM activities, the better their perceived engagement is. As noticed in previous studies through indirect observation, the STEAM approach can enhance student engagement (Hong et al., 2020 ; Khamhaengpol et al., 2021 ). Our study deepens these observations as it provides results generated as a product of direct measurement of this variable. Observed differences are greatest within agentic, then emotional, behavioral, and finally cognitive engagement between all three time points. These observations are consistent with observations from previous studies indicating that agentic engagement offers great potential in terms of enhancing learning (Reeve & Tseng, 2011 ). Students of all groups perceived this type of engagement the best over time because, during the intervention, an atmosphere was created in which they were free to ask questions, express their opinions, follow their interests, and make suggestions. Agentic engagement is proactive, intentional, and purposeful; it offers opportunities to enrich the learning process by making it more personal, interesting, challenging, and valuable for students; and it develops a constructive contribution to the planning and flow of teaching activities in which students have a say. In order to develop this type of engagement, teachers should provide students with autonomy support, i.e., they need to create classroom conditions in which students feel free to express opinions, pursue interests, and ask questions (Maričić et al., 2023 ; Reeve & Tseng, 2011 ). STEAM activities offer that possibility and leave enough space for an optimal level of personalization of the learning process by students, which is very important for improving their perceptions of learning. Our results indicate that the longer the students were engaged in STEAM activities, the more they developed the values of actively asking questions, communicating their interests, the need to improve achievement as well as suggestions for improving learning, the feeling of enjoyment, fun, interest, curiosity, and finally the values of careful listening, focus, and investing effort. In previous research, it was shown that teachers who work with students from higher schools to a significantly greater extent (disproportionately) activate the components of cognitive engagement, while teachers who work with students of lower school age to a significantly greater extent (disproportionately) activate the components of behavioral engagement (Greene et al., 2004 ; Reeve & Tseng, 2011 ). The results of our study are not in line with the aforementioned because it was shown that our STEAM activities in students over time activate the components of all four types of engagement so that none of them is disproportionate to the others. Also, when we compare them, we notice that the agentic, behavioral, and emotional components are only slightly more activated over time than the cognitive ones. A similar pattern was observed in the research of Ritoša et al. ( 2020 ), where preschool children showed a higher level of emotional, behavioral, and cognitive engagement, but in approximate proportions. This is most likely related to the nature of the STEAM activities and the first student participation in them, where the other three types of engagement slightly prevailed. This problem should be further and more deeply examined in future studies.
In addition to the above, the results of our research show that the integration of VS into STEAM activities over time significantly contributes to the development of students’ perceived engagement compared to STEAM activities without technology (SA condition). Similar results were observed in the meta-analysis by Leavy et al. ( 2023 ), in which it was stated that emerging technologies have the potential to increase student engagement, as well as in the study by Katyara et al. ( 2023 ), in which it was shown that the integration of different technologies into learning activities can enrich this process and significantly increase different types of student engagement. Over time, in our study, emerging technology primarily encouraged the development of agentic, behavioral, emotional, and finally cognitive engagement. This shows us that the implementation of VS develops the values of personalization, enrichment of content, and learning conditions, then the values of participation in activities, attention to tasks, investment of effort, perseverance, and absence of behavioral problems, and finally the feeling of joy, fun, interest, and curiosity in the students. Kahu et al. ( 2015 ) found that positive emotions associated with the topic, such as interest, fun, and enthusiasm, come from learning that is integrated with life experience, as well as the intersection between learning materials and students’ work and experience. STEAM’s technology-enhanced approach offers it all. Considering that the research on this topic is limited, it is recommended to investigate this issue more deeply and further through a longitudinal study, which can provide significant insights into the contribution of emerging technologies to the development of different types of student engagement over a longer period of time. These data would indicate the potential of emerging technologies in maintaining student engagement as well as in the development of different types of student engagement, considering the time frame and acquired experience in STEAM activities.
If we consider the results obtained by comparing all four different conditions and groups (while eliminating the time factor), we can also see that STEAM activities enhanced by VS contribute to the development of student-perceived engagement to a greater extent. These differences are significant in terms of behavioral engagement, where it was shown that the constant integration of VS (through both lessons, STA) within STEAM activities significantly contributes to the development of this type of engagement compared to the STEAM condition without VS integration (SA) and the STEAM condition with partial VS integration (only within the second lesson, SA + STA). A similar observation was made in the research by Garcia-Martinez et al. ( 2021 ), in which it was shown that the integration of technology into teaching not only changes the way students learn but also changes their learning behaviors and performance in the long run. Similar results were also observed in the research by Katyara et al. ( 2023 ), where it was noticed that the integration of technology in learning activities contributes to the greatest extent to the development of behavioral engagement. These facts are explained from the perspective of various opportunities and benefits that technologies provide to the development of this type of engagement, such as the following: they make the learners more actively involved in the learning process and encourage them to invest more efforts; reduce the dominance of the teacher; enable students to independently participate in more self-regulating learning activities; therefore, help them to develop self-reliance, persistence, and attention (Katyara et al., 2023 ; Maričić et al., 2023 ; Zinan & Sai, 2017 ). This indicates that students who learn content with STEAM-embedded technology tools develop the values of active involvement, attentive listening, persistence, focus, and investing effort to a much greater extent. These facts can be justified by the benefits that VS offer in terms of learning. While the students were learning through them, they were able to visualize abstract concepts - those that they failed to see through real hands-on experiments such as the lines of force of a magnetic field, their behavior during the approach of the same and different poles of a magnet, the concept of magnetization, the formation of domains within metals, and their orientation, which encouraged them to listen carefully, direct their attention, and put in extra effort when working on VS. Thus, students were significantly more actively involved in STEAM learning activities, which had the greatest impact on the development of behavioral engagement. Such results should be discussed in future research from the Technology Acceptance Model (TAM) theory perspective, which would indicate the extent to which students (and teachers) accept this type of technology as well as their future intentions regarding the usage of VS in teaching. In addition to the above, it is suggested that different types of engagement should be correlated with other variables, such as student achievement and motivation, to see their connection and consider other important components of the teaching process.
8.3 Third part - contribution of the VS integration order
Our results also reveal that the integration of VS at the beginning (in the first STEAM lesson) contributes to a greater extent to the development of students’ perceived engagement compared to the integration of VS at the end (within the second STEAM lesson). Similar results were observed in the research of Hughes et al. ( 2022 ), which examined the order of arts integration within STEAM activities. The results showed that students who studied life and physical science contents first with the integration of art in STEAM activities showed better results compared to those students who studied those contents in a different order. The order of technology integration can be seen as a significant predictor of student engagement in STEAM activities. Students who first learned with STEAM activities in which VS was integrated showed better results in agentic, behavioral, emotional, and cognitive engagement after the first lesson. These data show us that after the first lesson, the students were significantly more enterprising, behaviorally and effectively active, and invested more mental effort in the learning process, which prepared and encouraged them to continue learning about these contents. Also, the integration of another discipline within STEAM activities at the very beginning of the intervention significantly expands the students’ horizons, which leads to multimodal representation of contents, the generation of new ideas, and a more creative approach to learning (Hughes et al., 2022 ). Students learned scientific concepts about magnetism through demonstration, performing real hands-on experiments, and creating original works of art (that present scientific concepts), but also through VS, i.e., through different modalities. This leads us to the potential conclusion that the integration of VS within the first STEAM lesson prepared the students for the initial conceptualization and visualization of abstract concepts, which gave them a valid basis and later facilitated the continuation of learning the same content. These activities particularly influenced the development of agentic and behavioral engagement, i.e., they strengthened the student’s optimal personalization and enrichment of the learning process through participation, attention, effort, and persistence. Given that within the groups, approximate mean values were observed in terms of all types of student engagement, we can note that multimodal representation of contents greatly influenced the development of emotional and cognitive engagement as well, i.e., it stimulated the development of a positive emotional state and cognitive functions in students. This has been demonstrated by several STEAM studies, which confirmed that this approach prepares students for learning and reduces cognitive load (expands the working memory space) because abstract concepts become much more accessible through multiple modalities of representation, which also affects the regulation of conceptual inconsistency (Campbell et al., 2018 ; Maričić et al., 2022a , b ; Wahyuningsih et al., 2020 ). VS offer exactly that possibility - through visualization. Such results should be discussed in future research from the perspective of cognitive load theory, which can shed more light on the contribution of VS to students’ cognitive potentials and their connection with different types of engagement.
9 Conculsion, contribution, implications and limitations
9.1 conclusions.
Based on the analysis of our results, we can conclude that the 4-factor EBCA scale model is aligned and fits the overall sample well, i.e., the engagement scale in this form can be validly and reliably used in an educational context when working with primary school students. STEAM activities can support student-perceived engagement, and the longer students are involved in STEAM activities, the better their perceived engagement is. Over time, this type of learning has the greatest impact on the development of agentic engagement (but not disproportionately compared to other types of engagement). VS emerging technology has the potential to significantly enhance students’ perceived engagement, and the more they work on VS, the more they develop the values of attentive listening, directing attention, and investing effort in learning. When we eliminate the time factor and only compare different STEAM conditions, we can also conclude that STEAM technology-enhanced activities can contribute to the development of student-perceived engagement to a greater extent compared to non-technology ones. This contribution is significant in terms of behavioral engagement, which was achieved through VS integration within STEAM lessons. The order of integration of VS also improves perceived engagement, and students who learn with them first perceive all types of engagement better.
9.2 Contribution
Assessment of student engagement in education is of exceptional importance, especially for educators and practitioners, because it has been shown through various observations that it greatly affects all other teaching and learning outcomes of students, and that aspect can improve teaching performance and make it more personal and interesting to them. The modified EBCA scale can be used as a valid and reliable instrument for these purposes in working with primary school students;
Based on the assessment of student engagement with the use of the modified EBCA scale, teachers can adjust, dose, and adapt their teaching style, motivational support, and instructional guidance to the needs of students and thereby improve learning. In our study, it was shown that autonomy support, i.e., classroom conditions in which students feel free to express opinions, pursue interests, and ask questions, greatly influences the development of both agentic and all other types of engagement, which has the potential to transform and strengthen learning and bring it closer to students;
In addition to the above, the use of this scale in the assessment of student engagement can show teachers how students emotionally, behaviorally, cognitively, and agentically experience teaching activities, i.e., how they react, how they behave, how they learn, and what they undertake within the teaching process, which can direct them and help them in further adequately designing STEAM lessons according to the needs and interests of the children. Our study offers clear insights into this, as well as an example of a STEAM activity that can support teaching practice from this aspect;
In previous studies, it was confirmed that teachers who work with students of lower school age focus more on activating the behavioral components of engagement, while teachers who work with higher school students focus more on activating the cognitive components of engagement (Birch & Ladd, 1997 ; Greene et al., 2004 ; Reeve & Tseng, 2011 ), which did not prove to be the best in teaching practice. Assessment of student engagement using the EBCA scale can help teachers focus on redesigning teaching activities, i.e., on balancing and equally activating all types of student engagement, because in this way all components important for the learning process and students themselves can be ensured. The results of our study confirm that.
9.3 Implicationas for future studies and limitations
Given that our study is limited in terms of the generalization of the results because the model fit of the engagement scale was checked on a smaller sample of students aged 9–10 from Eastern Europe, the modified EBCA scale could be used for the same purposes in work with students of different primary grade levels and from different ethnic and cultural backgrounds, which will improve the generalization of the results and affect their applicability on a more global level;
Within our study, only one variable was tested: student engagement and it is recommended that its number be expanded (for example, variables achievement and motivation could be tested) and correlated with student engagement. In this sense, the modified EBCA scale can be used to assess whether and to what extent different types of engagement can predict student achievement and their motivation to learn. In this way, it is possible to discover which type of engagement predicts to the greatest extent student achievements and motivation, which is essential for teaching practice;
Given that in our study only VS was tested within STEAM activities, it is suggested to integrate and test other emerging technologies as well, from the perspective of student engagement. It is also recommended to investigate this issue more deeply through a longitudinal study, which would indicate the potential of emerging technologies in maintaining student engagement as well as in the development of different types of engagement considering the time frame and acquired experience in STEAM activities. Also, it is desirable to connect and discuss the results obtained in those studies from the perspectives of cognitive load theory and TAM theory and address the changes in education that STEAM enhanced with different emerging technologies can bring.
Code availability
(software application or custom code): Not applicable.
Data availability
(data transparency): All data and materials as well as software applications or custom code support published claims and comply with field standards. The data generated during and/or analyzed during the current study are available from the corresponding author on reasonable request.
Abla, C., & Fraumeni, B. R. (2019). Student engagement: Evidence-based strategies to boost academic and socialemotional results . McREL International.
Anđić, B., Ulbrich, E., Dana-Picard, T., Cvjetićanin, S., Petrović, F., Lavicza, Z., & Maričić, M. (2023). A Phenomenography Study of STEM teachers’ conceptions of using three-Dimensional modeling and Printing (3DMP) in teaching. Journal of Science Education and Technology , 32 (1), 45–60. https://doi.org/10.1007/s10956-022-10005-0 .
Article Google Scholar
Anđić, B., Lavicza, Z., Vučković, D., Maričić, M., Ulbrich, E., Cvjetićanin, S., & Petrović, F. (2023a). 3D printers as a learning tool for cooperative learning in an inclusive classroom. International Journal of Disability Development and Education . https://doi.org/10.1080/1034912X.2023.2223495 .
Anđić, B., Maričić, M., Weinhandl, R., Mumzu, F., Schmidthaler, E., & Lavicza, Z. (2024). Longitudinal study of metaphors changes in secondary School teachers’ beliefs about 3D modeling and Printing. Education and Information Technologies . https://doi.org/10.1007/s10639-023-12408-x .
Astin, A. (1984). Student involvement: A development theory for higher education. Journal of College Student Development , 40 , 518–529.
Google Scholar
Axelson, R. D., & Flick, A. (2010). Defining student engagement. Change: The Magazine of Higher Learning , 43 (1), 38–43. https://doi.org/10.1080/00091383.2011.533096 .
Barlow, A., & Brown, S. (2020). Correlations between modes of student cognitive engagement and instructional practices in undergraduate STEM courses. International Journal of STEM Education . https://doi.org/10.1186/s40594-020-00214-7 .
Birch, S. H., & Ladd, G. W. (1997). The student–teacher relationship and children’s early school adjustment. Journal of School Psychology , 35 , 61–79. https://doi.org/10.1016/S0022-4405(96)00029-5 .
Boy, G. A. (2013). From STEM to STEAM: toward a human-centred education, creativity & learning thinking. In Proceedings of the 31st European conference on cognitive ergonomics (Vol. 3, pp. 1–7). https://doi.org/10.1145/2501907.2501934
Byrne, B. M. (2010). Structural equation modeling with Amos: Basic concepts, applications, and Programming (2nd ed.). Taylor and Francis Group.
Campbell, C., Speldewinde, C., Howitt, C., & MacDonald, A. (2018). STEM practice in the early years. Creative Education , 9 (01), 11. https://doi.org/10.4236/ce.2018.91002 .
Chen, C. H., & Chu, Y. R. (2024). VR-assisted inquiry-based learning to promote students’ science learning achievements, sense of presence, and global perspectives. Education and Information Technologies . https://doi.org/10.1007/s10639-024-12620-3 .
Chen, C. C., & Huang, P. H. (2020). The effects of STEAM-based mobile learning on learning achievement and cognitive load. Interactive Learning Environments . https://doi.org/10.1080/10494820.2020.1761838 .
Craig, P., Cooper, C., Gunnell, D., Haw, S., Lawson, K., Macintyre, S., Ogilvie, D., Petticrew, M., Reeves, B., Sutton, M., & Thompson, S. (2012). Using natural experiments to evaluate population health interventions: New Medical Research Council guidance. Journal of Epidemiology Community Health , 66 (12), 1182–1186. https://doi.org/10.1136/jech-2011-200375 .
Crowder, M. J., & Hand, D. J. (2017). Analysis of repeated measures . Routledge.
D’Angelo, C., Rutstein, D., Harris, C., Bernard, R., Borokhovski, E., & Haertel, G. (2014). Simulations for STEM learning: Systematic review and Meta-analysis . SRI International.
Falloon, G. (2019). Using simulations to teach young students science concepts: An experiential learning theoretical analysis. Computers & Education , 135 , 138–159. https://doi.org/10.1016/j.compedu.2019.03.001 .
Fornell, C., & Larcker, D. F. (1981). Evaluating structural equation models with unobservable variables and measurement error. Journal of Marketing Research , 18 (1), 39–50. https://doi.org/10.2307/3151312 .
Fredricks, J. A., Blumenfeld, P. C., & Paris, A. H. (2004). School engagement: Potential of the concept, state of the evidence. Review of Educational Research , 74 (1), 59–109. https://doi.org/10.3102/00346543074001059 .
Garcia-Martinez, J. A., Fuentes-Abeledo, E. J., & Rodriguez-Machado, E. R. (2021). Attitudes towards the use of ICT in Costa Rican University students: The influence of sex, academic performance, and training in technology. Sustainability , 13 . https://doi.org/10.3390/su13010282 .
Glancy, M. A. W. (2014). Examination of integrated STEM curricula as a means toward quality K-12 engineering education (Research to practice). ASEE annual Conference and Expositio. https://doi.org/10.18260/1-2-20446
Greene, B. A., Miller, R. B., Crowson, M., Duke, B. L., & Akey, K. L. (2004). Predicting high school students’ cognitive engagement and achievement: Contributions of classroom perceptions and motivation. Contemporary Educational Psychology , 29 , 462–482. https://doi.org/10.1016/j.cedpsych.2004.01.006 .
Hair, J. F., Black, W. C., Babin, B. J., & Anderson, R. E. (2010). Multivariate Data Analysis: A Global Perspective (7th ed).). Prentice Hall.
Hair, J. F., Sarstedt, M., Ringle, C. M., & Gudergan, S. P. (2017). Advanced issues in partial least squares structural equation modeling . Sage Publications Inc.
Hong, J. C., Ye, J. H., Ho, Y. J., & Ho, H. Y. (2020). Developing Inquiry and hands-on learning model to guide STEAM lesson planning for Kindergarten Children. Journal of Baltic Science Education , 19 , 908–922.
Hughes, S. B., Corrigan, W. M., Grove, D., Andersen, B. S., & Wong, T. J. (2022). Integrating arts with STEM and leading with STEAM to increase science learning with equity for emerging bilingual learners in the United States. International Journal of STEM Education, 9 . https://doi.org/10.1186/s40594-022-00375-7
Janković, A., Maričić, M., & Cvjetićanin, S. (2023). Comparing Science Success of Primary School students in the Gamified Learning Environment via Kahoot and Quizizz. Journal of Computers in Education . https://doi.org/10.1007/s40692-023-00266-y .
Jones, V. R. (2014). Teaching STEAM: 21st century skills. Child Technology Engineering, 18 (4), 11–13.
Kang, N. H. (2019). A review of the effect of integrated STEM or STEAM (science, technology, engineering, arts, and mathematics) education in South Korea. Asia-Pacific Science Education . https://doi.org/10.1186/s41029-019-0034-y .
Kahu, E. R., Stephens, C. V., Leach, L., & Zepke, N. (2015). Linking academic emotions and student engagement: Mature-aged distance students’ transition to university. Journal of Further and Higher Education, 39 (4), 481–497. https://doi.org/10.1080/0309877X.2014.895305
Katyara, P., Dahri, K. H., Muhiuddin, G., & Shabroz, S. (2023). Impact of Technology On Student’s Engagement in different dimensions: Cognitive, behavioral, reflective and social Engagement. Webology , 19 (3), 3451–3464.
Khamhaengpol, A., Sriprom, M., & Chuamchaitrakool, P. (2021). Development of STEAM activity on nanotechnology to determine basic science process skills and engineering design process for high school students. Thinking Skills and Creativity , 39 . https://doi.org/10.1016/j.tsc.2021.100796 .
Laut, J., Bartolini, T., & Porfiri, M. (2015). Bioinspiring an interest in STEM. IEEE Transactions on Education , 58 (1), 48–55. https://doi.org/10.1109/TE.2014.232453 .
Leavy, A. M., Dick, L., Meletiou-Mavrotheris, M., Paparistodemou, E., & Stylianou, E. (2023). The prevalence and use of emerging technologies in STEAM education: A systematic review of the literature. Journal of Computer Assisted Learning . https://doi.org/10.1111/jcal.12806 .
Lim, B. R. (2004). Challenges and issues in designing inquiry on the web. British Journal of Educational Technology , 35 (5), 627–643. https://doi.org/10.1111/j.0007-1013.2004.00419.x .
Maričić, M., Cvjetićanin, S., Adamov, J., Olić Ninković, S., & Anđić, B. (2022a). How do Direct and Indirect hands-on instructions strengthened by the self-explanation Effect promote learning? Evidence from Motion Content. Research in Science Education . https://doi.org/10.1007/s11165-022-10054-w .
Maričić, M., Cvjetićanin, S., Andevski, M., & Anđić, B. (2022b). Effects of Withholding Answers Coupled with Physical Manipulation on Students’ Learning of Magnetism-related Science Content. Research in Science and Technological Education . https://doi.org/10.1080/02635143.2022.2066648 .
Maričić, M., Cvjetićanin, S., Anđić, B., Marić, M., & Petojević, A. (2023). Using instructive simulations to teach young students simple Science concepts: Evidence from electricity content. Journal of Research on Technology in Education . https://doi.org/10.1080/15391523.2023.2196460 .
Meletiou-Mavrotheris, M. (2019). Augmented reality in STEAM education. In M. Peters, & R. Heraud (Eds.), Encyclopedia of educational innovation . Springer. https://doi.org/10.1007/978-981-13-2262-4_128-1 .
Moreno-Guerrero, A. J., Soler-Costa, R., Marín-Marín, J., & López-Belmonte, J. (2021). Flipped learning and good teaching practices in secondary education. Comunicar , 29 (68), 1–11. https://doi.org/10.3916/C68-2021-09 .
Nicol, D. J., & Macfarlane-Dick, D. (2006). Formative assessment and selfregulated learning: A model and seven principles of good feedback practice. Studies in Higher Education , 31 (2), 199–218. http://doi.org/10. 1002/j.2168-9830.2010.tb01056.x.
Olympiou, G., Zacharias, Z., & de Jong, T. (2013). Making the invisible visible: Enhancing students’ conceptual understanding by introducing representations of abstract objects in a simulation. Instructional Science , 41 (3), 575–596. https://doi.org/10.1007/s11251-012-9245-2 .
Perignat, E. (2018). J. Katz-Buonincontro (Ed.), STEAM in practice and research: An integrative literature review. Thinking Skills and Creativity https://doi.org/10.1016/j.tsc.2018.10.002 .
Reeve, J., & Tseng, C. M. (2011). Agency as a fourth aspect of students’ engagement during learning activities. Contemporary Educational Psychology , 36 (4), 257–267. https://doi.org/10.1016/j.cedpsych.2011.05.002 .
Ritoša, A., Danielsson, H., Sjöman, M., Almqvist, L., & Granlund, M. (2020). Assessing School Engagement – Adaptation and Validation of Engagement Versus Disaffection with Learning: Teacher report in the Swedish Educational Context. Frontiers in Education , 5 . https://doi.org/10.3389/feduc.2020.521972 .
Sanina, A., Kutergina, E., & Balashov, A. (2020). The Co-Creative approach to digital simulation games in social science education. Computers & Education , 149 . https://doi.org/10.1016/j.compedu.2020.103813 .
Sarı, U., Duygu, E., Şen, Ö. F., & Kırındı, T. (2020). The Effect of STEM Education on scientific process skills and STEM awareness in Simulation Based Inquiry Learning Environment. Journal of Turkish Science Education , 17 (3), 387–405. https://doi.org/10.36681/tused.2020.34 .
Scalise, K., Timms, M., Moorjani, A., Clark, L., Holtermann, K., & Irvin, P. S. (2011). Student learning in science simulations: Design features that promote learning gains. Journal of Research in Science Teaching , 48 (9), 1050–1078. https://doi.org/10.1002/tea.20437 .
Sosa, N. E., Salinas, J., & de Benito, B. (2017). Emerging technologies (ETs) in education: A systematic review of the literature published between 2006 and 2016. International Journal of Emerging Technologies in Learning , 12 (5). https://doi.org/10.3991/ijet.v12i05.6939 .
Tabachnick, B. G., & Fidell, L. S. (2007). Experimental designs using ANOVA (Vol. 724). Thomson/Brooks/Cole.
Techakosit, S., & Nilsook, P. (2018). The development of STEM literacy using the learning process of Scientific Imagineering through AR. International Journal of Emerging Technologies in Learning . https://doi.org/10.3991/ijet.v13i01.7664 .
Thisgaard, M., & Makransky, G. (2017). Virtual learning simulations in high school: Effects on cognitive and non-cognitive outcomes and implications on the development of STEM academic and career choice. Frontiers in Psychology , 8 . https://doi.org/10.3389/fpsyg.2017.00805 .
Wahyuningsih, S., Nurjanah, N. E., Rasmani, U. E. E., Hafdah, R., Pudyaningtyas, A. R., & Syamsuddin, M. M. (2020). STEAM learning in early childhood education: A literature review. International Journal of Pedagogy and Teacher Education , 4 (1), 33–44.
Wang, X., Yang, D., Wen, M., Koedinger, K., & Rosé, C. P. (2015). Investigating how student ’s cognitive behavior in MOOC discussion forums affect learning gains. International Conference on Educational Data Mining . https://files.eric.ed.gov/fulltext/ED560568.pdf .
Wen, C. T., Liu, C. C., Chang, H. Y., Chang, C. J., Chang, M. H., Chiang, F., Yang, S. H., & Hwang, C. W., F. K (2020). Students guided inquiry with simulation and its relation to school science achievement and scientific literacy. Computers & Education , 149. https://doi.org/10.1016/j.compedu.2020.103830 .
Zainuddin, Z., Shujahat, M., Haruna, H., & Wah Chu, S. K. (2020). The role of gamified e-quizzes on student learning and engagement: An interactive gamification solution for a formative assessment system. Computers & Education , 145. https://doi.org/10.1016/j.compedu.2019.103729 .
Zhang, M. (2014). Who are interested in online science simulations? Tracking a trend of digital divide in internet use. Computers & Education , 76 , 205–214. https://doi.org/10.1016/j.compedu.2014.04.001 .
Zinan, W., & Sai, G. T. B. (2017). Students’ perceptions of their ict-based College English Course in China: A Case Study. Teaching English with Technology , 17 (3), 53–76.
Download references
Acknowledgements
The authors thank to the Project that supported the realization of this research, as well as the students who participated in the research.
This research was supported by; by the TransEET Project, funded by the European Union HORIZON-WIDERA − 2021- ACCESS − 03 − 01. Project, licensed under [grant number 101078875], and by the Quality of the education system of Serbia in the European perspective Project, licensed under [grant number: 179010].
Open access funding provided by Johannes Kepler University Linz.
Author information
Authors and affiliations.
Faculty of Education in Sombor, Department of Sciences and Management in Education, University of Novi Sad, Podgorička 4, Sombor, 25000, Republic of Serbia
Mirjana Maričić
Linz School of Education, Department of STEM Education Science Park 5, Johannes Kepler University, Altenberger Straße 69, Linz, 4040, Austria
Zsolt Lavicza
You can also search for this author in PubMed Google Scholar
Corresponding author
Correspondence to Zsolt Lavicza .
Ethics declarations
Ethical approval.
All procedures followed were in accordance with the ethical standards and principles of the conducting a research of the School of Education, University of Novi Sad and School of Education, Johannes Kepler University in Linz. An ethical approval was not mandatory (necessary for the type of collected data (i.e. anonymous questionnaire data) at the time when the study was conducted.
Consent for publication
All authors have read and approved the final version of the article.
Informed consent
For the realization of the research permission (consent) was sought from primary school principals, school pedagogues and psychologists, as well as teachers from selected classes, parents of children as well as the children themselves. Participation in the study was voluntary. The data was collected, saved, and analyzed anonymously.
Research involving human participants - rights
The study involved human participants ( N = 84 3rd grade students, aged 9–10) who voluntarily chose to participate in the research. All research participants are guaranteed anonymity.
Conflict of interest
The authors declare that they have no conflict of interest to disclose.
Additional information
Publisher’s note.
Springer Nature remains neutral with regard to jurisdictional claims in published maps and institutional affiliations.
Rights and permissions
Open Access This article is licensed under a Creative Commons Attribution 4.0 International License, which permits use, sharing, adaptation, distribution and reproduction in any medium or format, as long as you give appropriate credit to the original author(s) and the source, provide a link to the Creative Commons licence, and indicate if changes were made. The images or other third party material in this article are included in the article’s Creative Commons licence, unless indicated otherwise in a credit line to the material. If material is not included in the article’s Creative Commons licence and your intended use is not permitted by statutory regulation or exceeds the permitted use, you will need to obtain permission directly from the copyright holder. To view a copy of this licence, visit http://creativecommons.org/licenses/by/4.0/ .
Reprints and permissions
About this article
Maričić, M., Lavicza, Z. Enhancing student engagement through emerging technology integration in STEAM learning environments. Educ Inf Technol (2024). https://doi.org/10.1007/s10639-024-12710-2
Download citation
Received : 02 December 2023
Accepted : 10 April 2024
Published : 24 May 2024
DOI : https://doi.org/10.1007/s10639-024-12710-2
Share this article
Anyone you share the following link with will be able to read this content:
Sorry, a shareable link is not currently available for this article.
Provided by the Springer Nature SharedIt content-sharing initiative
- Emerging technologies
- Virtual simulations
- STEAM learning environment
- Find a journal
- Publish with us
- Track your research
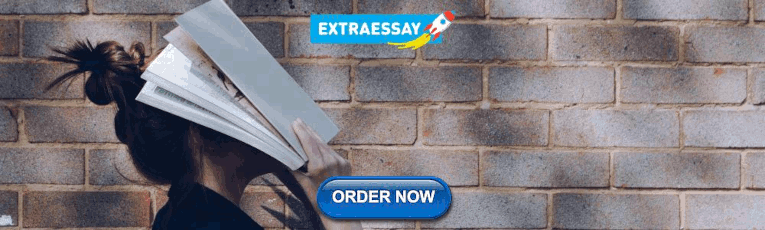
IMAGES
VIDEO
COMMENTS
Reliability is about the consistency of a measure, and validity is about the accuracy of a measure.opt. It's important to consider reliability and validity when you are creating your research design, planning your methods, and writing up your results, especially in quantitative research. Failing to do so can lead to several types of research ...
Reliability and validity are criteria by which researchers assess measurement quality. Measuring a person or item involves assigning scores to represent an attribute. This process creates the data that we analyze. However, to provide meaningful research results, that data must be good.
As with validity, reliability is an attribute of a measurement instrument - for example, a survey, a weight scale or even a blood pressure monitor. But while validity is concerned with whether the instrument is measuring the "thing" it's supposed to be measuring, reliability is concerned with consistency and stability.
Reliability refers to the consistency of the measurement. Reliability shows how trustworthy is the score of the test. If the collected data shows the same results after being tested using various methods and sample groups, the information is reliable. If your method has reliability, the results will be valid. Example: If you weigh yourself on a ...
Revised on 10 October 2022. Reliability and validity are concepts used to evaluate the quality of research. They indicate how well a method, technique, or test measures something. Reliability is about the consistency of a measure, and validity is about the accuracy of a measure. It's important to consider reliability and validity when you are ...
Reliability is a measure of the stability or consistency of test scores. You can also think of it as the ability for a test or research findings to be repeatable. For example, a medical thermometer is a reliable tool that would measure the correct temperature each time it is used. In the same way, a reliable math test will accurately measure ...
Example 1: In an experiment, a researcher manipulates the independent variable (e.g., a new drug) and controls for other variables to ensure that any observed effects on the dependent variable (e.g., symptom reduction) are indeed due to the manipulation. This establishes internal validity.
Example of Reliability and Validity in Research. In this section, we'll explore instances that highlight the differences between reliability and validity and how they play a crucial role in ensuring the credibility of research findings. Example of reliability; Imagine you are studying the reliability of a smartphone's battery life measurement.
For example, looking at a 4th grade math test consisting of problems in which students have to add and multiply, most people would agree that it has strong face validity (i.e., it looks like a math test). On the other hand, content validity evaluates how well a test represents all the aspects of a topic. Assessing content validity is more ...
However, in research and testing, reliability and validity are not the same things. When it comes to data analysis, reliability refers to how easily replicable an outcome is. For example, if you measure a cup of rice three times, and you get the same result each time, that result is reliable. The validity, on the other hand, refers to the ...
Reliability is a key concept in research that measures how consistent and trustworthy the results are. In this article, you will learn about the four types of reliability in research: test-retest, inter-rater, parallel forms, and internal consistency. You will also find definitions and examples of each type, as well as tips on how to improve reliability in your own research.
In psychology research, validity refers to the extent to which a test or measurement tool accurately measures what it's intended to measure. It ensures that the research findings are genuine and not due to extraneous factors. Validity can be categorized into different types, including construct validity (measuring the intended abstract trait), internal validity (ensuring causal conclusions ...
Reliability is consistency across time (test-retest reliability), across items (internal consistency), and across researchers (interrater reliability). Validity is the extent to which the scores actually represent the variable they are intended to. Validity is a judgment based on various types of evidence.
Validity. Validity is defined as the extent to which a concept is accurately measured in a quantitative study. For example, a survey designed to explore depression but which actually measures anxiety would not be considered valid. The second measure of quality in a quantitative study is reliability, or the accuracy of an instrument.In other words, the extent to which a research instrument ...
A good example of the process used in assessing inter-rater reliability is the scores of judges for a skating com-petition. The level of consistency across all judges in the scores given to skating participants is the measure of inter-rater reliability. An example in research is when researchers are asked to give a score for the relevancy of
Whether the research question is valid for the desired outcome, the choice of methodology is appropriate for answering the research question, the design is valid for the methodology, the sampling and data analysis is appropriate, and finally the results and conclusions are valid for the sample and context. In assessing validity of qualitative ...
Reliability in psychology research refers to the reproducibility or consistency of measurements. Specifically, it is the degree to which a measurement instrument or procedure yields the same results on repeated trials. A measure is considered reliable if it produces consistent scores across different instances when the underlying thing being measured has not changed.
Reliability refers to the consistency, dependability, and trustworthiness of a system, process, or measurement to perform its intended function or produce consistent results over time. It is a desirable characteristic in various domains, including engineering, manufacturing, software development, and data analysis. Reliability In Engineering.
Reliability and validity are among the most important and fundamental domains in the assessment of any measuring methodology for data-collection in a good research. Validity is about what an instrument measures and how well it does so, whereas reliability concerns the truthfulness in the data obtained and the degree to which any measuring tool ...
Validity, Reliability, Quantitative Res earch. JEL Codes: C12, C15, C42. Validity and eliability of the scales used in research are essential. that enable the re search to yield beneficialresults ...
3.1 INTRODUCTION. In Chapter 2, the study's aims of exploring how objects can influence the level of construct validity of a Picture Vocabulary Test were discussed, and a review conducted of the literature on the various factors that play a role as to how the validity level can be influenced. In this chapter validity and reliability are ...
Taherdoost [36] revealed "face validity is the degree to which a measure appears to be related to a specific construct, in the judgment of nonexperts such as test takers" and the clarity of the ...
Validity and reliability or trustworthiness are fundamental issues in scientific research whether. it is qualitative, quantitative, or mixed research. It is a necessity for researchers to describe ...
Our findings suggest that shortened versions of the SOC-13 scale have better psychometric properties than the original 13-item version in the Czech adult population. Particularly, SOC-9 emerges as a viable alternative, showing comparable reliability and validity as the 13-item version and a clear one-factorial structure in our sample.
Emerging technologies can potentially transform education through student engagement. The aim of our study is threefold. Firstly, we aspired to examine the validity and reliability of Reeve and Tsengs' 4-construct (emotional, behavioral, cognitive, and agentic) engagement scale (EBCA scale). Secondly, we aimed to examine whether and to what extent the integration of emerging technology ...
2.7. Validity and reliability. Attention was paid to minimize the chance of obtaining higher errors in this study. Hence, reliability and validity tests, which help detect the presence or absence of these errors, were carried out. To that end, the researcher conducted pilot and reliability tests to meet all reliability and validity standards.