Thank you for visiting nature.com. You are using a browser version with limited support for CSS. To obtain the best experience, we recommend you use a more up to date browser (or turn off compatibility mode in Internet Explorer). In the meantime, to ensure continued support, we are displaying the site without styles and JavaScript.
- View all journals
- My Account Login
- Explore content
- About the journal
- Publish with us
- Sign up for alerts
- Open access
- Published: 07 May 2024
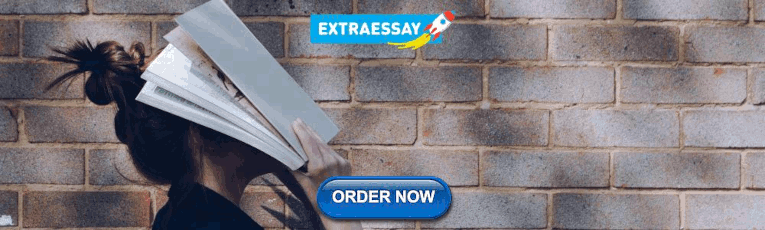
Prediction and causal inference of cardiovascular and cerebrovascular diseases based on lifestyle questionnaires
- Riku Nambo 1 ,
- Shigehiro Karashima 2 ,
- Ren Mizoguchi 3 ,
- Seigo Konishi 3 ,
- Atsushi Hashimoto 3 ,
- Daisuke Aono 3 ,
- Mitsuhiro Kometani 3 ,
- Kenji Furukawa 4 ,
- Takashi Yoneda 3 ,
- Kousuke Imamura 5 &
- Hidetaka Nambo 6
Scientific Reports volume 14 , Article number: 10492 ( 2024 ) Cite this article
211 Accesses
Metrics details
- Health care
- Medical research
Cardiovascular and cerebrovascular diseases (CCVD) are prominent mortality causes in Japan, necessitating effective preventative measures, early diagnosis, and treatment to mitigate their impact. A diagnostic model was developed to identify patients with ischemic heart disease (IHD), stroke, or both, using specific health examination data. Lifestyle habits affecting CCVD development were analyzed using five causal inference methods. This study included 473,734 patients aged ≥ 40 years who underwent specific health examinations in Kanazawa, Japan between 2009 and 2018 to collect data on basic physical information, lifestyle habits, and laboratory parameters such as diabetes, lipid metabolism, renal function, and liver function. Four machine learning algorithms were used: Random Forest, Logistic regression, Light Gradient Boosting Machine, and eXtreme-Gradient-Boosting (XGBoost). The XGBoost model exhibited superior area under the curve (AUC), with mean values of 0.770 (± 0.003), 0.758 ( ± 0.003), and 0.845 ( ± 0.005) for stroke, IHD, and CCVD, respectively. The results of the five causal inference analyses were summarized, and lifestyle behavior changes were observed after the onset of CCVD. A causal relationship from ‘reduced mastication’ to ‘weight gain’ was found for all causal species theory methods. This prediction algorithm can screen for asymptomatic myocardial ischemia and stroke. By selecting high-risk patients suspected of having CCVD, resources can be used more efficiently for secondary testing.
Similar content being viewed by others
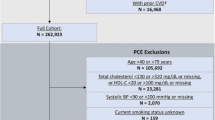
Machine learning and atherosclerotic cardiovascular disease risk prediction in a multi-ethnic population
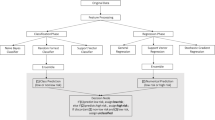
Application of ensemble machine learning algorithms on lifestyle factors and wearables for cardiovascular risk prediction
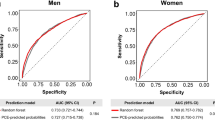
Machine learning reveals sex-specific associations between cardiovascular risk factors and incident atherosclerotic cardiovascular disease
Introduction.
Cardiovascular and cerebrovascular diseases (CCVD) are major causes of death in the Japanese population 1 , 2 . These diseases are classified as ‘symptomatic’ or ‘asymptomatic,’ according to the presence or absence of symptoms. Prevention, early diagnosis, and treatment are important to prevent poor quality of life (QoL) and death 2 , 3 , 4 . In some cases, such as stroke, early treatment may lead to the recovery of neurological functions. Lifestyle modification is an important component of such prevention. Appropriate diet, moderate exercise, and good sleep management can reduce the risk of CCVD. Health check-ups are also crucial. In Japan, specific health checkups focusing on metabolic syndrome have been conducted since 2008 to prevent lifestyle-related diseases 5 . Based on the results of these checkups, specific health guidelines are provided to review lifestyle habits pertaining to exercise, diet, and smoking.
In recent years, artificial intelligence (AI) has attracted significant attention because of its use in preventive medicine. AI methods can automatically identify important patterns in an individual’s clinical data and predict disease onset and prognosis 6 , 7 . Several studies have demonstrated the high accuracy of AI models for stroke and coronary artery disease 8 , 9 . Investigating causal inference in observational studies is also an effective method, as it captures real-world events and behaviors and may provide results that resemble real-world situations compared to experimental studies 10 , 11 .
Several predictive models for CCVD have been proposed, but reports are limited. In addition, there are no reports examining causal relationships among lifestyle factors used for prediction. Therefore, this study aimed to examine how specific lifestyle habits affect the CCVD risk by testing multiple causal relationships. In this study, we developed a predictive model for CCVD using data obtained from metabolic syndrome screening in the general population.
Participant characteristics
A total of 473,734 participants who underwent medical examinations were included in the KMA database. 261,645 individuals were excluded from the dataset because they had at least one missing value. The exclusion criteria were applied, and the remaining patients were classified into stroke (n = 10,713), IHD (n = 20,922), CCVD (n = 3868), and normal (n = 176,586) groups. Table 1 lists the baseline characteristics of each disease and normal group. The IHD, stroke, and CCVD groups showed significant differences in age, female sex ratio, BMI, prevalence of HT, prevalence of DM, prevalence of DL, SBP, DBP, HbA1c, TG, HDL-C, and T-Cho compared to the normal group. Table 2 lists the lifestyle behaviors of the participants based on the questionnaire. There were significant differences in all lifestyle behaviors in the IHD, stroke, and CCVD groups compared with those in the normal group ( P < 0.05, vs. normal group).
Feature importance ranking
Supplementary Figure 1 shows the feature importance ranking for each prediction model using Dataset 2. eGFR, PG, and TG were consistently among the top 10 important features in all four IHD prediction models. MCV and TG were consistently among the top 10 important features in all four stroke prediction models. ALT, Hb, and TG were consistently among the top 10 important features in all four CCVD prediction models. TG consistently ranked in the top 10 important features in all 12 models.
Supplementary Figure 2 depicts the variation in the AUC as features were incrementally added to each prediction model, starting with the highest-ranked feature. For the LGBM, 19 features were chosen for stroke, 17 for IHD, and 20 for CCVD. For the RF model, 19, 15, and 20 features were selected for the stroke, IHD, and CCVD, respectively. In the XGBoost model, 19, 16, and 19 features were selected for the stroke, IHD, and CCVD, respectively. Finally, in the LR model, stroke incorporated four features, IHD incorporated 18 features, and CCVD incorporated two.
Predictive performance
Figure 1 shows the performance metrics of the CCVD predictive models. For Dataset 1, the RF models consistently achieved the highest AUCs, with mean values of 0.729 (SD 0.003), 0.716 (SD 0.003), and 0.809 (SD 0.005) for stroke, IHD, and CCVD, respectively.
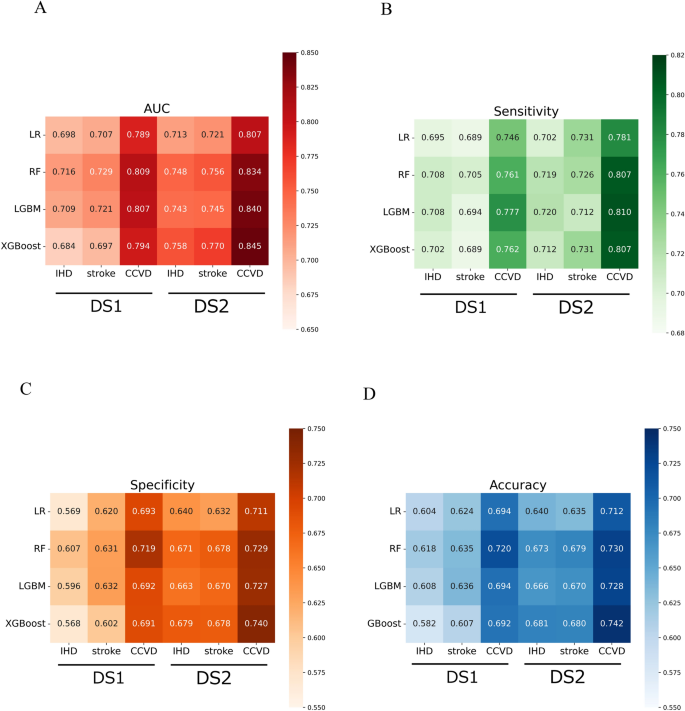
Performance of disease prediction algorithms. Heatmap comparing the area under the receiver operating characteristic curve ( A ), sensitivity ( B ), specificity ( C ), and accuracy ( D ). Counts in each box represent an average of 20 counts. The data set and model combination with the highest AUC and Accuracy was XGBoost in data set 2 for IHD, stroke, and CCVD. The highest sensitivity was for LGBM in Data Set 2 for IHD, for LR and XGBoost in Data Set 2 for stroke, and for LGBM in Data Set 2 for CCVD. The data set and model combination with the highest specificity was XGBoost from Data Set 2 for IHD and CCVD, and RF or XGBoost from Data Set 2 for stroke. LR logistic regression, RF random forest, LGBM light gradient boosting machine, XGBoost eXtreme gradient boosting, IHD ischemic heart disease, CCVD cardiovascular and cerebrovascular diseases, DS1 dataset 1, DS2 dataset 2.
In comparison, when Dataset 2 was analyzed, the XGBoost model exhibited the best AUC. The mean AUCs were 0.770 (SD = 0.003), 0.758 (SD = 0.003), and 0.845 (SD = 0.005) for stroke, IHD, and CCVD, respectively. The prediction model employing Dataset 2 surpassed that employing Dataset 1 for all the accuracy metrics.
Causal inference
Supplementary Figure 3 illustrates the causal network and inferred directions of causality determined using the five causal search methodologies. Supplementary Table 1 shows the score rankings for the lifestyle-related features. First place was “walking speed” with a score of 142. Second place went to “chewing” with a score of 100, and third was “weight gain” with a score of 85. In fourth place were “sleep habits” and “regular exercise” with the same score of 84. The top five rankings were selected as the features for causal inference. The Direct LiNGAM technique visually represents the magnitude of influence of one variable on another using a partial regression coefficient indicated by a connecting arrow. For the three models utilizing NOTEARS, causal arrow strength is denoted as ‘Edge Weight’. However, Bayesian networks do not quantitatively illustrate causality through metrics, such as partial regression coefficients or edge weights.
Figure 2 presents the ensemble analysis results and summarizes the findings of the five causal inference methods. Relationships identified as causal by three or more methods are indicated by arrows and are differentiated using dashed, solid, or bold lines. Importantly, the causal link from DM to DL and the pathway from ‘chewing’ to ‘weight gain’ were consistently supported across all five techniques.
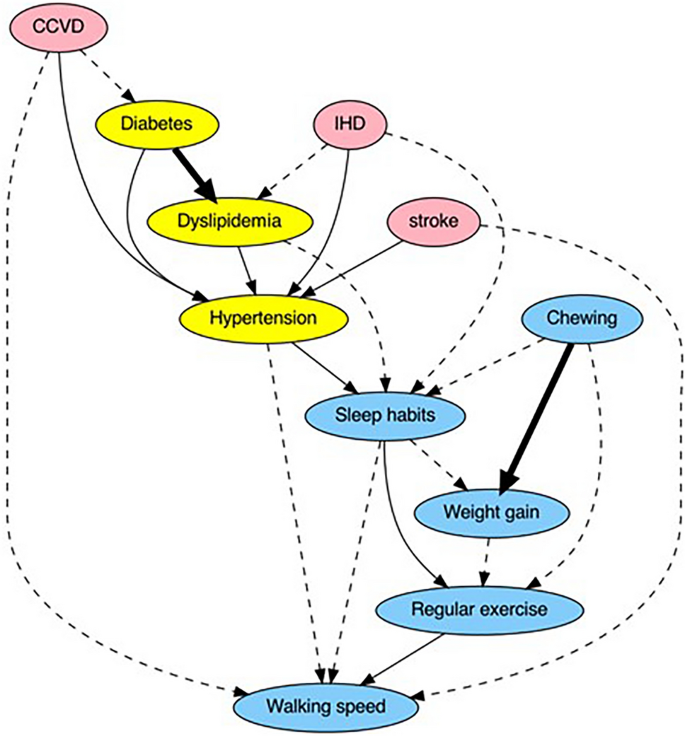
Ensemble causal inference. Analyses were performed for each of the five causal inference models—Direct LiNGAM, NOTESRS, NOTESRS with Lasso, NOTESRS with PyTorch, and Bayesian networks—and the results were integrated. Three of the five models that matched the causal directions are shown as dotted lines, four as solid lines, and five as bold lines. Causal direction indicated by all five causal inference models (bold arrows): the onset of diabetes is causally related to the incidence of dyslipidemia. Decreased chewing ability is associated with increased weight compared to weight around age 20. Causal direction indicated by four of the five causal inference models (solid arrows): Hypertension is causally related to the development of CCVD, IHD, Stroke, diabetes, and dyslipidemia. The development of hypertension is causally related to not getting enough rest with sleep. When people do not get enough sleep, they do not implement a regular exercise routine of at least 30 min twice a week, resulting in a lower walking speed than the average for the same age group of the same sex. IHD ischemic heart disease, CCVD cardiovascular and cerebrovascular disease. Weight gain: Have you gained 10 kg or more since turning 20 kg? Regular exercise: Do you engage in at least 30 min of exercise twice a week for a minimum of 1 year? Walking speed: Do you walk faster than your peers? Chewing: How thoroughly do you chew food during meals? Sleep habits: Do you feel that you get sufficient sleep?
A predictive model including lifestyle questionnaires was developed to diagnose CCVD. There are several reports on cardiovascular disease and stroke prediction models that use lifestyle information 12 , 13 , 14 . Li et al. developed several models to predict cardiovascular disease using a dataset including lifestyle-related items such as smoking, alcohol consumption, dietary patterns, and physical activity for 1,887,710 adults in northwest China and found that the RF prediction model had the best prediction accuracy with an AUC of 0.723 12 . Zhu et al. found the best prediction accuracy for stroke, with an AUC of 0.686, in a dataset of 2147 members of the general population using a model that included sex, age, lifestyle habits, genetic factors, medical history, nasal examination results, and blood sampling results 14 . However, these studies did not examine causality between the features used for prediction.
The precise predictive results of the algorithm are expected to alert individuals and trigger behavioral changes that lead to further guidance and closer examination. Conversely, the algorithm can reduce the financial burden and time wasted by patients with a low predictive risk who would otherwise undergo secondary testing. However, caution should be exercised when implementing this algorithm, as the model does not predict the future development of the disease, but rather indicates the possibility of prior myocardial ischemia or stroke occurrence in health-screening participants who are unaware of their symptoms. Asymptomatic stroke does not present with symptoms such as paralysis or sensory disturbance 15 , whereas asymptomatic myocardial ischemia is characterized by few or no typical symptoms of IHD, such as chest pain, pressure, nausea, and shortness of breath 16 . In practice, these asymptomatic conditions are detected incidentally using electrocardiography, computed tomography, and magnetic resonance imaging (MRI). Moreover, asymptomatic stroke increases the risk of symptomatic stroke and vascular cognitive impairment 17 , 18 , 19 . Asymptomatic myocardial ischemia is more common in the elderly 20 , 21 and patients with DM 22 , 23 , 24 , 25 , and is associated with increased cardiac and all-cause mortality, which is particularly important in the presence of other coronary disease risk factors 26 , 27 , 28 , 29 . A diagnosis of asymptomatic stroke or myocardial ischemia does not imply the need for aggressive invasive treatment. However, intensive treatment of lifestyle-related diseases should be considered 2 , 3 . Choosing patients with a high likelihood of asymptomatic disease for treatment is reasonable to prevent the future development of symptomatic CCVD and may be an economically advantageous approach owing to optimized target selection and prevention of unnecessary treatment costs. Further research on the practical efficiency and health economics of this algorithm is required.
The results of the causal inference, a novel element of this study, are surprising. We expected a causal relationship between worsening lifestyle habits and cerebrovascular diseases via lifestyle-related diseases, similar to the “metabolic domino” proposed by Ito et al. 30 . However, in the present study, behavior was observed to change after the onset of CVD. This result may be attributed to the change in participants’ health awareness after the onset of CCVD and the effectiveness of the National Health Guidance and Health Guidance System. When participants develop CCVD and become aware of its symptoms for the first time, they may become more conscious of the changes they need to make to prevent further exacerbation of the disease and try to improve their lifestyle. It is also not yet clear whether this system is an effective mechanism for encouraging participants to change their behavior for the primary prevention of CCVD.
Fukuma et al. reported no evidence that Japanese government-led national health and health guidance interventions were associated with improvements in cardiovascular risk factors among Japanese working-age men 31 . They evaluated changes in obesity status and cardiovascular risk factors (blood pressure, hemoglobin A1c levels, HDL cholesterol levels) 1 to 4 years after screening, but not stroke, IHD, or CCVD. However, if interventions do not improve cardiovascular risk factors, they will not prevent the development of CCVD either. General health screening programs in other countries have also been reported to be ineffective in reducing mortality from cardiovascular disease 32 , 33 . Inter9914 in Denmark reported that the incidence of IHD, stroke, and total mortality after 10 years was not significantly different between the control groups, even with interventions such as health checkups, lifestyle guidance for five years, and, if necessary, referral to a medical institution 33 . Ensemble causal analysis suggests that the primary prevention system for metabolic syndrome screening and specific health guidance in Japan has not fully achieved its objectives, such as preventing CCVD.
The ensemble causal network revealed significant relationships such as: (i) The causal relationship “the lower the chewing ability, the more weight gain” was found in all five causal inference models. Several studies have suggested that chewing slowly and often during meals is associated with a lower BMI 34 , 35 , 36 . Chewing well is an effective way to reduce the rate of eating and may contribute to a lower risk of obesity 37 , 38 . (ii) Sleep habits are frequently associated with diseases and other lifestyle habits in a causal manner. Short sleep duration and sleep disturbances are associated with adverse cardiometabolic risks such as obesity, HT, type 2 DM, and cardiovascular disease 39 , 40 . IHD is complicated by heart failure (HF). Approximately 75% of patients with heart failure experience sleep disturbance 41 . Obstructive sleep apnea, an obvious risk factor for heart disease, is associated with poor sleep quality, HT, and DL 42 . Thus, sleep may have multifaceted effects on the development of CCVD.
Finally, the observed variability in the causal inference results can be attributed to the occurrence of systematic biases due to confounding factors, such as selection and measurement 43 . Causal inference may be more susceptible to bias due to potential confounding factors when the sample size is small, resulting in variable causal relationships. Shimizu et al. reported a false causal association when analyzing a 1300-sample, six-feature dataset with potential confounding factors using Direct LiNGAM 44 . However, when the sample size of the dataset is large, the causal direction converges to the right in Direct LiNGAM 44 , 45 . There were 200,000 cases in this dataset, which may not have had a significant effect on causal direction. Additionally, a selection bias may have occurred in the population. Nakao et al. reported that those who participated in the health guidance intervention improved more dramatically in both weight and cardiovascular risk factors than those who did not participate 46 . Predictive models and causal relationships may differ significantly depending on the participation rate of health guidance interventions. The rate of specific health guidance provided in Kanazawa City ranged from 21.9 to 35.8%, while the national average implementation rate of specific health guidance was low, ranging from 7.74 to 23.2% 47 . These regional differences may affect the predictive accuracy and causality of the developed algorithms. Finally, lifestyle and medical history information relied on the participant’s responses to the questionnaire. Measurement bias may have existed if the participants misidentified and responded to their health information. Therefore, it is impossible to eliminate all biases when using real-world data. Therefore, we analyzed and integrated the results using five different methods to ensure their robustness in the presence of these variations. None of the models showed any variations in the results, indicating that CCVD affected lifestyle-related diseases or specific lifestyle habits. The ensemble causal network method was more reliable than the single-model causal inference method.
In conclusion, we established a predictive model for CCVD using data from lifestyle questionnaires, physical observations, medical histories, and general laboratory results obtained during health examinations. Using real-world data, we used an ensemble causal network to represent the causal relationships among lifestyle, lifestyle-related diseases, and CCVD. The algorithm can predict whether health checkup participants experience asymptomatic myocardial ischemia and stroke, which may lead to savings in healthcare costs through the efficient use of healthcare resources for secondary health checkups. However, the results of this causal inference should be interpreted with caution. The results should be analyzed in other populations, and the reproducibility of the causal relationships should be confirmed. Further research is required to determine the usefulness of this algorithm in the Japanese health checkup system.
Materials and methods
Study participants.
This study design is a secondary data analysis using community health screening. The study included 473,734 participants aged 40 years or older who underwent community health screening in Kanazawa City between 2009 and 2018. Medical institutions in charge of health checkups were sent identical manuals in accordance with the guidelines of the respective associations and checkups were conducted accordingly. During the checkups, clinicians performed a standard consultation and recorded data on height, weight, waist circumference, blood pressure, biochemical test results, urinalysis, and lifestyle questionnaires 6 . The study was approved by the Ethics Committee of the Kanazawa Medical Association (KMA) (No. 16000003) and the Ethics Committee of Kanazawa University (No. 2019-080) and was conducted in accordance with the Declaration of Helsinki and ethical guidelines for human medical research. All data were anonymized. The Ethics Committee waived the need for informed consent, as this was secondary data use. An opt-out notification form regarding the study was provided on the KMA website ( http://www.kma.jp/kenkyu/kenkyu_index.html ).
The KMA database contains information on various clinical parameters as previously reported 6 . Six variables were collected from the dataset: age, sex, body mass index (BMI), waist circumference, systolic blood pressure (SBP), and diastolic blood pressure (DBP).
Laboratory blood parameters were measured within 24 h of collection using an automated clinical chemistry analyzer. These parameters included plasma glucose (PG), hemoglobin A1c (HbA1c), total cholesterol (T-Cho), triglycerides (TG), low-density lipoprotein cholesterol, high-density lipoprotein cholesterol (HDL-C), serum creatinine, estimated glomerular filtration rate (eGFR), serum uric acid, aspartate aminotransferase, alanine aminotransferase, gamma-glutamyl transpeptidase, white blood cell count, red blood cell count, hemoglobin, hematocrit, mean corpuscular volume (MCV), mean corpuscular hemoglobin, mean corpuscular hemoglobin concentration, and platelet count. The test procedures followed the specimen testing methods recommended by the Japanese Society for Clinical Chemistry.
Standardized questionnaire items for specific health checkups were developed by experts under the initiative of the Japanese Ministry of Health, Labor and Welfare. The questionnaire covered medical history including treatment for hypertension (HT), diabetes (DM), and dyslipidemia (DL). We also inquired whether the patient had previously experienced stroke, ischemic heart disease (IHD), chronic kidney disease (CKD), or anemia. The questionnaire comprised 13 lifestyle-related questions. The details of the questionnaire are presented in Supplementary Table 2 .
Dataset construction
Two datasets were assembled to develop a predictive model based on questionnaire responses. Dataset 1 comprised data from 13 lifestyle-related questionnaire items in addition to age, sex, BMI, waist circumference, SBP, and DBP. Dataset 2 consisted of blood and urine test results from Dataset 1. The definitions of predictive brain disease, IHD, and complications associated with both brain diseases and IHD were derived from a questionnaire.
Statistical analysis for clinical background
Data are expressed as mean (SD) or percentage. The clinical background of each disease group was compared with that of the normal group. Normality was assessed using the Shapiro–Wilk test. Normally distributed data with equal variances were compared using the Student’s t-test, whereas data with unequal variances were compared using Welch’s t-test. P < 0.05 was considered significant. Statistical analyses were performed using Python 3.8.3 programming language (Python Software Foundation, Wilmington, DE, USA), and SciPy 1.5.2.
General process of prediction model construction
The procedure for building and predicting the CCVD model is illustrated in Fig. 3 . Patients with missing data were excluded. The data set was assembled using a stratified extraction method to split the data in a 7:3 ratio for training and testing while maintaining the distribution of it.
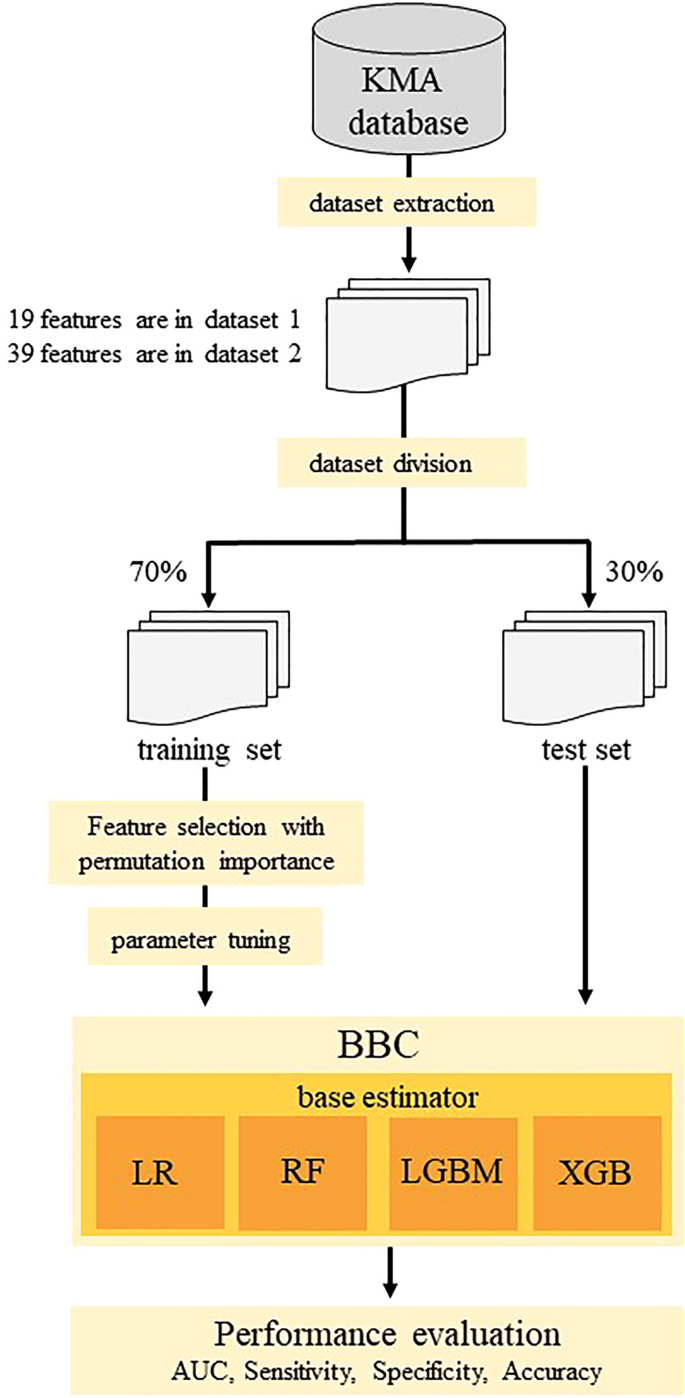
Procedure for developing a diagnostic prediction algorithm. Of the participants enrolled in the KMA database, 261,645 participants with missing data were excluded. Participants were then classified into stroke group (n = 10,713), IHD group (n = 20,922), CCVD group (n = 3868), and normal group (n = 176,586). Dataset 1 consisted of 19 features including age, gender, BMI, waist circumference, SBP, DBP, and 13 lifestyle-related questions. Dataset 2 consisted of 19 variables from dataset 1 and 20 variables including blood tests. The cases were split into training and test data in a 7:3 ratio. The machine learning algorithms (MLAs) used as base estimators were Logistic regression (LR), Random Forest (RF), Light Gradient Boosting Machine (LGBM), and eXtreme-Gradient-Hyperparameter tuning and feature selection by permutation importance were used for each model to optimize MLA performance. A Balanced Bagging Classifier was used to address the imbalance in the number of cases in the CCVD and healthy groups. The prediction performance was evaluated using the area under the receiver operating characteristic curve (AUC), sensitivity, specificity, and accuracy.
The machine learning algorithms used were Light Gradient Boosting Machine (LGBM) 48 , Random Forest (RF) 49 , Logistic regression (LR) 50 , and eXtreme-Gradient-Boosting (XGBoost) 51 . Hyperparameter tuning and feature selection by permutation importance were used for each model to optimize the performance of the machine learning models. Parameter tuning is the process of adjusting external configuration values that control the model’s learning process to maximize the model’s predictive performance. Permutation importance is a method to evaluate the importance of a feature. We randomly swapped the values of certain features, evaluated how they affected the performance of the model, and ranked the features in order of importance. Based on the ranked list of features, the minimum set of features needed to maintain or improve the model’s performance is selected. To address the imbalance in the number of cases in the CCVD and healthy groups, a Balanced Bagging Classifier was used. The Balanced Bagging Classifier is a method that combines bagging and under sampling, and used LGBM, RF, LR, or XGBoost as the base estimator. Youden Index was used to determine the cutoff value for the receiver operating characteristic (ROC) curve. The model-construction process was repeated 20 times. Predictive metrics, such as accuracy, area under the ROC curve (AUC), sensitivity, and specificity, were computed and averaged. For models utilizing Dataset 2, we gauged the significance of the clinical tests via permutation importance 52 and selected the features exhibiting the highest AUC 53 .
Construction and integration of causal inference
Causal inferences were drawn using the Direct Linear Non-Gaussian Acyclic Model (LiNGAM) 44 , Non-combinatorial Optimization via Trace Exponential and Augmented Lagrangian for structure learning (NOTEARS) 54 , NOTEARS with the least absolute shrinkage and selection operator 54 , NOTEARS with PyTorch 54 , and a Bayesian network 55 , 56 . For causal inference, the variables incorporated were CCVD, stroke, IHD, DM, HT, DL, and lifestyle factors. Features were selected based on their importance, and the top five features were identified using permutation importance. The 13 lifestyle items were selected as follows:
Determining feature importance using permutation importance.
The questionnaire items were ranked from 1st to 13th position and scored in descending order: 13 points for 1st place, 12 points for 2nd place, 2 points for 12th place, and 1 point for 13th place.
Scoring was repeated 12 times across the four prediction models and three diseases to achieve cumulative scores. Cumulative scores determined the top five lifestyle items incorporated into the causal inference.
All features used for causal inference were standardized and used in the analysis.
Data availability
De-identified participant data were shared. Please contact Shigehiro Karashima at [email protected]. The data will be restricted by the Kanazawa University IRB depending on the intended use. The data were shared in an Excel file after approval by the Kanazawa University Institutional Review Board.
Code availability
The code used for the analysis was shared at the following link. https://zenodo.org/records/10703345 .
Ministry of Health, Labour and Welfare, Japan. The 2021 Vital Statistics . https://www.mhlw.go.jp/english/database/db-hw/vs01.html (2019).
Miyamoto, S. et al. Japan stroke society guideline 2021 for the treatment of stroke. Int. J. Stroke 17 , 1039–1049 (2022).
Article PubMed PubMed Central Google Scholar
Kimura, K. et al. JCS 2018 guideline on diagnosis and treatment of acute coronary syndrome. Circ. J. 83 , 1085–1196 (2019).
Article CAS PubMed Google Scholar
Maron, D. J. et al. Initial invasive or conservative strategy for stable coronary disease. N. Engl. J. Med. 382 , 1395–1407 (2020).
The Ministry of Health, Labour and Welfare. About Specific Health Screening and Specific Health Guidance . https://www.mhlw.go.jp/stf/seisakunitsuite/bunya/0000161103.html .
Kawakami, M. et al. Explainable machine learning for atrial fibrillation in the general population using a generalized additive. Cross-Sectional study. Circ. Rep. 4 , 73–82 (2021).
Karashima, S. et al. A hyperaldosteronism subtypes predictive model using ensemble learning. Sci. Rep. 13 , 3043 (2023).
Article ADS CAS PubMed PubMed Central Google Scholar
Mobley, B. A., Schechter, E., Moore, W. E., McKee, P. A. & Eichner, J. E. Predictions of coronary artery stenosis by artificial neural network. Artif. Intell. Med. 18 , 187–203 (2000).
Bivard, A., Churilov, L. & Parsons, M. Artificial intelligence for decision support in acute stroke—Current roles and potential. Nat. Rev. Neurol. 16 , 575–585 (2020).
Article PubMed Google Scholar
Prosperi, M. et al. Causal inference and counterfactual prediction in machine learning for actionable healthcare. Nat. Mach. Intell. 2 , 369–375 (2020).
Article Google Scholar
Ohlsson, H. & Kendler, K. S. Applying causal inference methods in psychiatric epidemiology: A review. JAMA Psychiatry 77 , 637–644 (2020).
Li, J. X. et al. Machine learning identifies prominent factors associated with cardiovascular disease: Findings from two million adults in the Kashgar Prospective Cohort Study (KPCS). Glob. Health Res. Policy 7 , 48 (2022).
Article CAS PubMed PubMed Central Google Scholar
Yang, L. et al. Study of cardiovascular disease prediction model based on random forest in eastern China. Sci. Rep. 10 , 5245 (2020).
Zhu, Q. et al. A model for risk prediction of cerebrovascular disease prevalence-Based on community residents aged 40 and above in a City in China. Int. J. Environ. Res. Public Health 18 , 6584 (2021).
Longstreth, W. T. Jr. et al. Lacunar infarcts defined by magnetic resonance imaging of 3660 elderly people: The cardiovascular health study. Arch. Neurol. 55 , 1217–1225 (1998).
Conti, C. R., Bavry, A. A. & Petersen, J. W. Silent ischemia: Clinical relevance. J. Am. Coll. Cardiol. 59 , 435–441 (2012).
Bernick, C. et al. Silent MRI infarcts and the risk of future stroke: The cardiovascular health study. Neurology 57 , 1222–1229 (2001).
Vermeer, S. E. et al. Silent brain infarcts and white matter lesions increase stroke risk in the general population: The Rotterdam scan study. Stroke 34 , 1126–1129 (2003).
Debette, S. et al. Association of MRI markers of vascular brain injury with incident stroke, mild cognitive impairment, dementia, and mortality: The Framingham Offspring Study. Stroke 41 , 600–606 (2010).
Gottlieb, S. O. & Gerstenblith, G. Silent myocardial ischemia in the elderly: Current concepts. Geriatrics 43 , 29–34 (1988).
CAS PubMed Google Scholar
Fleg, J. L. et al. Prevalence and prognostic significance of exercise-induced silent myocardial ischemia detected by thallium scintigraphy and electrocardiography in asymptomatic volunteers. Circulation 81 , 428–436 (1990).
Ambepityia, G. et al. Exertional myocardial ischemia in diabetes: A quantitative analysis of anginal perceptual threshold and the influence of autonomic function. J. Am. Coll. Cardiol. 15 , 72–77 (1990).
Naka, M. et al. Silent myocardial ischemia in patients with non-insulin-dependent diabetes mellitus as judged by treadmill exercise testing and coronary angiography. Am. Heart J. 123 , 46–53 (1992).
Aronow, W. S., Mercando, A. D. & Epstein, S. Prevalence of silent myocardial ischemia detected by 24-hour ambulatory electrocardiography, and its association with new coronary events at 40-month follow-up in elderly diabetic and nondiabetic patients with coronary artery disease. Am. J. Cardiol. 69 , 555–556 (1992).
Nakao, Y. M. et al. Holter monitoring for the screening of cardiac disease in diabetes mellitus: The non-invasive Holter monitoring observation of new cardiac events in diabetics study. Diab. Vasc. Dis. Res. 12 , 396–404 (2015).
Multiple Risk Factor Intervention Trial Research Group. Exercise electrocardiogram and coronary heart disease mortality in the multiple risk factor intervention trial. Multiple risk factor intervention trial research group. Am. J. Cardiol. 55 , 16–24 (1985).
Ekelund, L. G. et al. Coronary heart disease morbidity and mortality in hypercholesterolemic men predicted from an exercise test: The lipid research clinics coronary primary prevention trial. J. Am. Coll. Cardiol. 14 , 556–563 (1989).
Laukkanen, J. A. et al. Exercise-induced silent myocardial ischemia and coronary morbidity and mortality in middle-aged men. J. Am. Coll. Cardiol. 38 , 72–79 (2001).
Gibbons, L. W., Mitchell, T. L., Wei, M., Blair, S. N. & Cooper, K. H. Maximal exercise test as a predictor of risk for mortality from coronary heart disease in asymptomatic men. Am. J. Cardiol. 86 , 53–58 (2000).
Itoh, H. Metabolic domino: New concept in lifestyle medicine. Drugs Today (Barc.) 42 (Suppl C), 9–16 (2006).
Fukuma, S. et al. Association of the National Health Guidance Intervention for obesity and cardiovascular risks with health outcomes among Japanese men. JAMA Intern. Med. 180 (1630), 1637 (2020).
Google Scholar
Krogsbøll, L. T., Jørgensen, K. J., Grønhøj Larsen, C. & Gøtzsche, P. C. General health checks in adults for reducing morbidity and mortality from disease: Cochrane systematic review and meta-analysis. BMJ 345 , e7191 (2012).
Jørgensen, T. et al. Effect of screening and lifestyle counselling on incidence of ischaemic heart disease in general population. Interiors99 randomised trial. BMJ 348 , g3617 (2014).
Fukuda, H. et al. Chewing number is related to incremental increases in body weight from 20 years of age in Japanese middle-aged adults. Gerodontology 30 , 214–219 (2013).
Ekuni, D., Furuta, M., Takeuchi, N., Tomofuji, T. & Morita, M. Self-reports of eating quickly are related to a decreased number of chews until first swallow, total number of chews, and total duration of chewing in young people. Arch. Oral Biol. 57 , 981–986 (2012).
Zhu, Y. & Hollis, J. H. Relationship between chewing behavior and body weight status in fully dentate healthy adults. Int. J. Food Sci. Nutr. 66 , 135–139 (2015).
Zhu, Y. & Hollis, J. H. Increasing the number of chews before swallowing reduces meal size in normal-weight, overweight, and obese adults. J. Acad. Nutr. Diet 114 , 926–931 (2014).
Cassady, B. A., Hollis, J. H., Fulford, A. D., Considine, R. V. & Mattes, R. D. Mastication of almonds: Effects of lipid bioaccessibility, appetite, and hormone response. Am. J. Clin. Nutr. 89 , 794–800 (2009).
St-Onge, M. P. et al. Sleep duration and quality: Impact on lifestyle behaviors and cardiometabolic health: A scientific statement from the American Heart Association. Circulation 134 , e367–e386 (2016).
Zuraikat, F. M., Wood, R. A., Barragán, R. & St-Onge, M. P. Sleep and diet: Mounting evidence of a cyclical relationship. Annu. Rev. Nutr. 41 , 309–332 (2021).
Jorge-Samitier, P., Fernández-Rodrigo, M. T., Juárez-Vela, R., Antón-Solanas, I. & Gea-Caballero, V. Management of hypnotics in patients with insomnia and heart failure during hospitalization: A systematic review. Nurs. Rep. 11 , 373–381 (2021).
Gaines, J., Vgontzas, A. N., Fernandez-Mendoza, J. & Bixler, E. O. Obstructive sleep apnea and the metabolic syndrome: The road to clinically-meaningful phenotyping, improved prognosis, and personalized treatment. Sleep Med. Rev. 42 , 211–219 (2018).
Hernán, M. A. & Robins, J. M. Causal Inference: What If (Chapman & Hall/CRC, 2020).
Shimizu, S. et al. DirectLiNGAM: A direct method for learning a linear non-Gaussian structural equation model. J. Mach. Learn. Res. 12 , 1225–1248 (2011).
MathSciNet Google Scholar
Genin, K. Statistical undecidability in linear, non-Gaussian causal models in the presence of latent confounders. In Proc. Causal Discovery & Causality-Inspired Machine Learning Workshop, NeurIPS (2020).
Nakao, Y. M. et al. Effectiveness of nationwide screening and lifestyle intervention for abdominal obesity and cardiometabolic risks in Japan: The metabolic syndrome and comprehensive lifestyle intervention study on nationwide database in Japan (MetS ACTION-J study). PLoS ONE 13 , e0190862 (2020).
Status of Implementation of Specific Health Check-Ups and Specific Health Guidance . https://www.mhlw.go.jp/stf/seisakunitsuite/bunya/newpage_00043.html.23 . (2021).
Ke, G. et al. LightGBM: A highly efficient gradient boosting decision tree. Adv. Neural Inf. Process. Syst. 1 , 3146–3154 (2017).
Breiman, L. Random forests. Mach. Learn. 45 , 5–32 (2001).
Cox, D. R. The Regression Analysis of Binary Sequences [with Discussion] (1958).
Chen, T. & Guestrin, C. XGBoost: A Scalable Tree Boosting System (2016).
Altmann, A., Toloşi, L., Sander, O. & Lengauer, T. Permutation importance: A corrected feature importance measure. Bioinformatics 26 , 1340–1347 (2010).
Youden, W. J. Index for rating diagnostic tests. Cancer 3 , 32–35 (1950).
Zheng, X., Aragam, B., Ravikumar, P. & Xing, E. P. DAGs with NO TEARS: Continuous optimization for structure learning. Preprint at http://arXiv.org/1803.01422 .
Heckerman, D., Geiger, D. & Chickering, D. M. Learning Bayesian networks: The combination of knowledge and statistical data. Mach. Learn. 20 , 197–243 (1995).
Tsamardinos, I., Brown, L. E. & Aliferis, C. F. The max–min hill-climbing Bayesian network structure learning algorithm. Mach. Learn. 65 , 31–78 (2006).
Download references
Acknowledgements
The authors thank Editage (Tokyo, Japan; www.editage.jp ) for the English language editing.
Author information
Authors and affiliations.
School of Electrical Information Communication Engineering, College of Science and Engineering, Kanazawa University, Kanazawa, Japan
Institute of Liberal Arts and Science, Kanazawa University, Kanazawa, Japan
Shigehiro Karashima
Department of Health Promotion and Medicine of the Future, Kanazawa University, Kanazawa, Japan
Ren Mizoguchi, Seigo Konishi, Atsushi Hashimoto, Daisuke Aono, Mitsuhiro Kometani & Takashi Yoneda
Health Care Center, Japan Advanced Institute of Science and Technology, Nomi, Japan
Kenji Furukawa
Faculty of Electrical, Information and Communication Engineering, Institute of Science and Engineering, Kanazawa University, Kanazawa, Japan
Kousuke Imamura
Institute of Transdisciplinary Sciences, Kanazawa University, Kanazawa, Japan
Hidetaka Nambo
You can also search for this author in PubMed Google Scholar
Contributions
SK and HN designed the study and evaluated and edited the manuscript. SeiK, AH, DA, MK, and TY supervised the consultations. KF collected the data. RN and RM performed the statistical analyses. RN, SK, RM, AH, KI, and HN contributed to the analysis and interpretation of the data. RN, SK, and HN wrote the manuscript. SK, TY, KI, and HN supervised the study. All authors have checked and approved the final version of the manuscript.
Corresponding authors
Correspondence to Shigehiro Karashima or Hidetaka Nambo .
Ethics declarations
Competing interests.
K. Furukawa received lecture fees from Sanofi K.K., Eli Lilly Japan K.K., and Ono Pharmaceutical Co., Ltd. A. The remaining authors declare no conflicts of interest with respect to this research or paper.
Additional information
Publisher's note.
Springer Nature remains neutral with regard to jurisdictional claims in published maps and institutional affiliations.
Supplementary Information
Supplementary figure s1., supplementary figure s2., supplementary figure s3., supplementary information., supplementary tables., rights and permissions.
Open Access This article is licensed under a Creative Commons Attribution 4.0 International License, which permits use, sharing, adaptation, distribution and reproduction in any medium or format, as long as you give appropriate credit to the original author(s) and the source, provide a link to the Creative Commons licence, and indicate if changes were made. The images or other third party material in this article are included in the article's Creative Commons licence, unless indicated otherwise in a credit line to the material. If material is not included in the article's Creative Commons licence and your intended use is not permitted by statutory regulation or exceeds the permitted use, you will need to obtain permission directly from the copyright holder. To view a copy of this licence, visit http://creativecommons.org/licenses/by/4.0/ .
Reprints and permissions
About this article
Cite this article.
Nambo, R., Karashima, S., Mizoguchi, R. et al. Prediction and causal inference of cardiovascular and cerebrovascular diseases based on lifestyle questionnaires. Sci Rep 14 , 10492 (2024). https://doi.org/10.1038/s41598-024-61047-w
Download citation
Received : 14 October 2023
Accepted : 30 April 2024
Published : 07 May 2024
DOI : https://doi.org/10.1038/s41598-024-61047-w
Share this article
Anyone you share the following link with will be able to read this content:
Sorry, a shareable link is not currently available for this article.
Provided by the Springer Nature SharedIt content-sharing initiative
By submitting a comment you agree to abide by our Terms and Community Guidelines . If you find something abusive or that does not comply with our terms or guidelines please flag it as inappropriate.
Quick links
- Explore articles by subject
- Guide to authors
- Editorial policies
Sign up for the Nature Briefing newsletter — what matters in science, free to your inbox daily.

Advertisement
The use of nanotechnology in cardiovascular disease
- Review Article
- Open access
- Published: 16 August 2018
- Volume 8 , pages 1607–1619, ( 2018 )
Cite this article
You have full access to this open access article
- Meera Chandarana 1 ,
- Anthony Curtis 1 &
- Clare Hoskins ORCID: orcid.org/0000-0002-7200-0566 1
22k Accesses
71 Citations
Explore all metrics
Cardiovascular diseases claim a number of lives globally; many of which are preventable. With the increase in diets that consist of high saturated fat, salt, and sugar, people often living sedentary lifestyles, and a rise in cases of obesity, the incidence of cardiovascular disease is increasing. These contributing factors, coupled with more advanced methods of diagnosis, have delivered statistics that clearly show that there is a rising trend in the prevalence of cardiovascular disease. Treatment for cardiovascular diseases is limited currently to oral medicines or invasive surgery. There is a huge gap in this area of medicine for novel therapeutics for improved patient outcomes. Nanotechnology may provide a solution to more effective treatment of disease, with better prognoses and a reduced side effect profile. This review will explore for potential solutions to the limited pharmacological therapies currently on the market and the future that lies ahead for the place of nanotechnology within cardiovascular medicine.
Similar content being viewed by others
Cardiovascular Nanotechnology
Atherosclerosis and Nanotechnology: Diagnostic and Therapeutic Applications
Avoid common mistakes on your manuscript.
Introduction
Cardiovascular diseases (CVDs) are the leading cause of death worldwide, claiming 17.7 million lives in 2015, and this figure is projected to increase to 23.6 million in 2030 (WHO 2017 ). The term ‘cardiovascular diseases’ encompasses, but is not limited to coronary heart disease, deep vein thrombosis, pulmonary embolism, and myocardial infarction (MI), all of which result in ischaemia and tissue death. Of these, MI and heart failure are the major conditions which lead to mortality.
A MI, known commonly as a heart attack, is the result of total occlusion of the major arteries supplying the heart leading to a decrease in blood flow, oxygen, and nutrient supply. Ultimately, this results in myocardial tissue death by apoptosis or necrosis due to coronary occlusion. An MI can manifest as two different forms, ST-elevation MI (STEMI) or non-ST-elevation MI (NSTEMI), dependant on the visible changes on an ECG trace (NICE 2013 ). The current treatment for MI is reperfusion in the shortest period of time following arterial obstruction, which can be achieved through invasive methods such as percutaneous coronary intervention (PCI) or coronary artery bypass graft (CABG), or through pharmacological therapy (Ruiz-Esparza et al. 2013 ).
Heart failure is a clinical disease state that presents with symptoms of breathlessness, peripheral oedema, and fatigue, all of which are a result of reduced cardiac functionality (Ponikowski et al. 2016 ). This form of cardiomyopathy is a state in which the heart muscle is unable to pump efficiently to satisfy the body’s metabolic requirements (Ruiz-Esparza et al. 2013 ). Disease of the coronary artery is the largest contributory factor in the development of heart failure (Fox et al. 2001 ). Heart failure is an umbrella term that refers to a range of various cardiac syndromes, including: left-ventricular dysfunction, valvular, pericardial, or endocardial abnormalities, and irregularity of rhythm (Ponikowski et al. 2016 ). It is estimated that up to 2 million people are living with heart failure in the UK, a figure that is continuously increasing due primarily to the significantly aging population (Mitchell et al. 2015 ). Currently, guidance recommends ACE inhibitors and β-receptor blockers as the first-line treatment for heart failure (NICE 2010 ).
The current statistics regarding the incidence of CVDs highlight the need for new therapies and technological advancements to combat these diseases.
Atherosclerosis is the precursor to several vascular diseases. Atherosclerotic plaques are formed of macrophage cells, fat, cholesterol, and calcium, which become deposited in the vasculature and the growing plaques harden over time. This results in narrowing of the blood vessels, a reduction in blood flow, rupture, and ultimately ischaemia ((Weaver 2013 ) (Fig. 1 ). Histologically, the rupture of plaques, which leads to a cardiovascular event, is a result of poor fibrous plaque caps containing increased concentrations of matrix metalloproteinases (MMPs) that cause weakness in the plaque (Qin et al. 2016 ). Lifestyle factors are eminent contributors to the development of atheromatous plaques. It is widely accepted that consuming foods rich in salt, sugar, and saturated fat greatly increases the risk of suffering from a CVD in later life. Having a high low-density lipoprotein (LDL)-to-high-density lipoprotein (HDL) ratio is also a risk factor as LDLs are deposited on the walls of blood vessels and contribute to the development of plaques. Hypertension, smoking, lack of physical activity, family history, obesity, age, and stress all play a role in the development of CVDs, estimated to cost the economy £19 billion per annum, with the National Health Service (NHS) bearing a burden of £9 billion (British Heart Foundation 2017 ).
Progression of atherosclerotic plaque showing narrowing of the blood vessel
Current treatments for CVDs are focussed on restoring normal blood flow through or around the damaged vasculature and the prevention of recurrent cardiovascular insults. Reduction in further build up and thickening of atherosclerotic plaques, as well as effects on external elastic membranes and fibrous and dense calcium volumes is achieved by statin therapy (Banach et al. 2015 ). Dual antiplatelet therapies using cyclooxygenase inhibitors such as aspirin and P2Y12 inhibitor such as clopidogrel are first-line treatments for prevention of CVDs, which aim to reduce clot formation and platelet aggregation (Kapil et al. 2017 ). There is a requirement for improvement in these treatments, primarily due to the risks associated with taking antiplatelet therapy which significantly adverse side effect profiles, and poor patient compliance (Harrington et al. 2003 ). In addition to this, some patients do not respond well to antiplatelet therapy, which has a negative effect on their long-term prognosis. Studies have demonstrated that patients who have suffered an acute myocardial infarction but have a low response to clopidogrel are at increased risk of recurrent cardiovascular events at follow-up (Geisler et al. 2006 ). This supports the necessity for advancements in technology and an opportunity for nanomedicine (Fig. 2 ).
Use of nanotechnology for cardiovascular disease
Nanotechnologies for treatment
The field of nanomedicine comprises the diagnosis, treatment, and prevention of disease or injury to improve and maintain a good quality of life and health, with use of nanotechnology (Patil et al. 2008 ). For example, a domain of nanomedicine aims to control and manipulate biomacromolecular and supramolecular entities which are vital to human health, encompassing DNA, RNA, cell membranes, and lipid bilayers (Tomalia et al. 2007 ). Nanoparticles, in particular, are widely accepted as having physicochemical properties that improve biological function, such as a high surface energy due to their large surface area to volume ratio, wettability, reactivity, and roughness (Jiang et al. 2017 ). The British Standards Institute defines nanoparticles as microscopic particles with all three dimensions in the nanoscale, ranging from 1 to 100 nm (British Standards Institute 2011 ). Advancements in nanomedicine research have led to better ways to reduce toxicity, prolong the half-life of drugs, and reduce side effects through alteration of nanoparticle properties, whilst maintaining the particle biocompatibility (Gupta and Gupta 2005 ). Targeted drug delivery in treatment of disease using nanoparticles is possible through active or passive means: active targeting requires conjugation of the therapeutic agent to a tissue- or cell-specific ligand, whereas passive targeting involves coupling of the therapeutic agent to a high-molecular-weight polymer that has enhanced permeation and retention to vascular tissue (Patel and Bailey 2007 ).
A diverse range of nanotechnologies have been developed for biomedicine within the past 2 decades, each with their own unique properties and advantages. These include micelles, liposomes, dendrimers, nanoparticles, and the use of nano-coatings (De Jong and Borm 2008 ) (Fig. 3 ). Currently, therapeutic applications of nanotechnology within medicine are focused around cancer treatment and diagnosis (Sanna et al. 2014 ); however, the focus is shifting to other areas of therapeutics, such as cardiovascular medicine (Binsalamah et al. 2012 ) and antimicrobial resistance (Kumar et al. 2018 ). Table 1 summarises the main classes of technologies under exploration in this area.
Nanocarriers for the delivery of therapeutic agents: a micelle, b liposome, and c dendrimer
Liposomes form phospholipid bilayer structures, around 50–200 nm in size, with aqueous cores encapsulated within natural phospholipids, such as cholesterol (Akbarzadeh et al. 2013 ). As they possess qualities of both hydrophilicity and hydrophobicity, liposomes can be used as effective drug delivery systems. There are three classifications of liposomes, based on their size and number of bilayers. Variable size, method of preparation, surface charge, and lipid composition are all contributing factors to the properties displayed by a liposome (Sahoo and Labhasetwar 2003 ). Small unilamellar vesicles (SUV) have a single lipid bilayer; large unilamellar vesicles (LUV) are similar to SUVs, whereas multilamellar vesicles (MLV) have numerous bilayers (Sahoo and Labhasetwar 2003 ). Manipulation of the surface of liposomes can be undertaken via the addition of poly(ethylene glycol) (PEG), giving the carrier ‘stealth’-like properties (Immordino et al. 2006 ). The ‘stealth liposomes’ have a prolonged circulation time, reduced uptake, and reduced clearance by phagocytes or the liver (Sahoo and Labhasetwar 2003 ). Furthermore, antibodies or other targeting moieties can be attached to the liposomal surface to target specific areas (Levchenko et al. 2012 ). Due to the processes of platelet aggregation in myocardial infarction, atherosclerosis, and thrombosis, platelet-targeted liposomal drug delivery may prove to have potential therapeutic applications (Ruiz-Esparza et al. 2013 ). In regard to applications in cardiovascular medicine, liposomes have the potential to be utilised to treat peripheral artery disease and intermitted claudication. Currently, research into the liposomal drug delivery of prostaglandin E-1 (PGE-1), under the trade name Liprostin, is undergoing phase III clinical trials (Bulbake et al. 2017 ). PGE-1 possesses a wide range of pharmacological properties, including vasodilation, inhibition of platelet aggregation and leukocyte adhesion, and anti-inflammatory qualities (Gao et al. 2010 ). Encapsulation of PGE-1 within lipid nanoparticles reduced the degradation of PGE-1 and improved both the prolongation of the drug outcome and the anti-inflammatory effect (Gao et al. 2010 ), exhibiting real potential for PGE-1-loaded lipid nanoparticles in clinical application.
Liposomes have been designed for targeted treatment of thrombosis. Thrombosis, a blockage of the blood vessels, is associated with myocardial infarction (and stroke). Recently, liposomes carrying thrombolytic drug urokinase were reported; these were surface functionalised with a cyclic arginyl-glycyl-aspartic acid (cRGD) peptide (Zhang et al. 2018 ). cRGD peptides selectively bind onto the GPIIb/IIIa receptors on the active platelets conferring targeted delivery. In the cRGD, liposomes demonstrated similar effect to the free drug at 75% less drug dosage in a mouse mesenteric thrombosis model (Zhang et al. 2018 ).
Multiple studies have reported the encapsulation and delivery of berberine (Allijn et al. 2017 ; Lou et al. 2013 ; Sailor 2015 ). Berberine is a small fluorescent isoquinoline quaternary alkaloid which has proven to be useful at high doses as a cardio-protectant (Zhang et al. 2014 ) and for improvement of cardiac function (Huang et al. 2015 ). Berberine-loaded liposomes have been developed and tested for IL-6 secretion inhibition and cardiac function protection against adverse remodelling after MI in C57BL/6J mice (Allijn et al. 2017 ). In vivo, the liposomal berberine formulation significantly preserved cardiac ejection fraction 28 days post-MI by 64% compared with the unloaded liposomes and free drug. This study highlights the potential which liposomal berberine formulation possess as a potential treatment of adverse remodelling after MI.
The use of liposomes is relatively old compared to the polymer-based systems and it is likely that these systems will be clinically applicable at an earlier stage. However, as observed in other liposomal drug delivery applications, there are some disadvantages. These include the lack of long-term stability of these systems resulting in premature drug release, as well. In addition, increased excipient-to-drug ratios are often required making them more expensive and less effective than some of the polymer-based systems.
Polymeric nanoparticles
Polymeric nanoparticles have attracted particular interest in the nanomedicine field, due to the manipulative qualities of the materials and the potential of reabsorption in the body (Jiang et al. 2017 ). The route of delivery of polymeric nanoparticles is dependent on their site of action and the most minimally invasive method of getting them into systemic circulation. Intravenous injection is the most common method of administration; however, oral, mucosal, dermal, or transdermal administration is also viable (Elsabahy and Wooley 2012 ). Polymeric nanoparticles make up a large class of systems. These include solid nanoparticles, amphiphilic nanoparticles (forming micelles, vesicles, and rods), dendrimers, and star-shaped systems. All of these systems possess their own unique architecture and properties (Hoskins et al. 2012 ). In general, polymer-based systems are more cost-effective and easier to manufacture and scale up compared to liposomes with longer stability profiles.
There are a number of non-biodegradable polymers such as poly(methyl methacrylate) (PMMA), poly(acrylamide), poly(styrene), and poly(acrylates), (Banik et al. 2016 ); however, chronic toxicity has been observed in studies using these materials. This has led a focus on biodegradable polymers (Burman et al. 2015 ), examples of which include synthetic polymers such as poly(lactide) (PLA), poly(lactide-co-glycolide) copolymers (PLGA), poly(ε-caprolactone) (PCL), and 17 and poly(amino acids) (Elsabahy and Wooley 2012 ).
Expanded polytetrafluoroethylene (ePTFE) grafts are most commonly used to substitute blood vessels that have been damaged as a result of ischaemia or atherosclerotic plaques, re-establishing regular blood flow. A study was conducted using poly(lactic- co -glycolic acid) (PLGA) nanoparticles covalently bonded to ePTFE surfaces to test their therapeutic effects. Following oxidation of the ePTFE surface using H 2 O 2 /H 2 SO 4 , aminolyzation using 3-aminopropyl triethoxysilane was undertaken. The nanoparticles showed good stability on the ePTFE surface and the biocompatibility of the graft with mouse fibroblasts which demonstrated no substantial cytotoxicity (Al Meslmani et al. 2017 ), suggesting promise for the use of ePTFE for the treatment of CVD by incorporating antithrombotic drugs to the polymer grafts. Korin et al. ( 2012 ) studied the effects of biodegradable poly(lactic- co -glycolic) acid (PLGA) nanoparticles laden with the enzyme tissue plasminogen activator (tPA) which aids in the dispersion of clots. The nanoparticles were designed specifically to disintegrate and release the drug upon encountering high shear stress resulting from the narrowing of the surrounding vasculature (Korin et al. 2012 ). In in vivo studies, the complexes released tPA rapidly, thus dissolving the clot swiftly, which increased the survival rate following an induced pulmonary embolism (Korin et al. 2012 ). Furthermore, the results indicated that lower doses of tPA were required if it was incorporated in the polymeric grafts, suggesting a reduced side effect profile. This research clearly indicates that there are applications for this technology in the treatment of CVDs with the potential to transform cardiovascular medicine.
Quercetin (Qu) encapsulated within polymeric nanoparticles (Giannouli et al. 2018 ) or as solid drug nanoparticles (Kakran et al. 2012 ; Tefas et al. 2015 ) has been the subject of a number of investigations. Qu is an antioxidant drug which has shown to protect against CVDs. One recent study reported a novel poly(lactic- co -glycolic acid) (PLGA) loaded with Qu for atherosclerosis prevention (Giannouli et al. 2018 ). The particles showed promising encapsulation efficiencies and drug release profiles indicating these systems as possible formulations to protect against CVDs (Giannouli et al. 2018 ). Work is ongoing to further explore the in vitro and in vivo potential of these particles.
Micelles are amphiphilic structures with characteristic hydrophobic cores and hydrophilic shells, fashioned from either polymer- or lipid-based amphiphilic molecules (Gupta 2011 ). They form spontaneously due to a reduction in free energy arising from aggregation of the hydrophobic portions from the aqueous surrounding, and the creation of a micelle core stabilised with hydrophilic fragments exposed to the water (Mourya et al. 2011 ). As the concentration of an amphiphile increases in solution, an increase in the free energy of the system occurs due to the unfavourable conditions of the environment, as a result of interactions between water and the hydrophobic section of the amphiphile, which results in a decrease in the system entropy and structuring of the water in solution (Letchford and Burt 2007 ). Micelles are formed as the critical micelle concentration (CMC) is reached (Trinh et al. 2017 ). The formation of micelles at the CMC lessens the number of undesirable interactions with water as the hydrophobic moieties are effectively removed from the aqueous environment. The hydrophobic nature of the core allows for encapsulation of drugs, and the hydrophilic shell increases circulation time and systemic exposure to the micelle. Their small size and physicochemical properties allow for entry into tissues by crossing membranes (Eniola-Adefeso et al. 2012 ). Polymeric nanoparticles possess increased stability compared to low-molecular-weight surfactants and liposomes; this is due to their extremely low excipient:drug ratios. Hence, there is reduced chance of systemic toxicity resulting from the carrier vehicle but also makes them more cost-effective. The synthesis is highly controllable, and they can easily be tailor made to application, i.e., addition of targeting ligands or tracking molecules. Most commonly, the polymers used for micellar drug delivery are comprised of polyester or poly(amino acid) derivatives to form the hydrophobic region (Gaucher et al. 2005 ). Forms of polyester that are approved for use in human applications include poly(lactic acid) (PLA), poly(ɛ-caprolactone) (PCL), and poly(glycolic acid) due to their biocompatibility, biodegradability, and structural properties (Gaucher et al. 2005 ). Reports on the use of polymeric micelles for cardiovascular disease are rapidly emerging in the literature (Ma et al. 2017 ; Chmielowski et al. 2017 ; Singla et al. 2018 ; Wu et al. 2018 ).
An in vitro study demonstrated successfully the use of a block co-polymer micelle formed from poly(ethylene glycol)(PEG)- block -polycation carrying a (PEG- b -P[Asp(DET)]) side chain as a gene delivery vector for treatment of vascular disease, with efficient gene expression and low cytotoxicity in vascular smooth muscle cells (Akagi et al. 2007 ). To fully understand the in vivo outcome of gene transfer, the PEG- b -P[Asp(DET)] micellar formulation was implanted to a rabbit carotid artery and the degree of gene expression of plasmid DNA (pDNA) to the vascular region was determined. The carotid was wounded using a balloon catheter, inducing neointimal hyperplasia, and the encapsulated pDNA was administered. The presence of pDNA was detected using the FLAG sequence (p-MP-FLAG). The artery was stained with an anti-FLAG antibody to confirm the expression of the gene, the results of which confirmed that the micellar formulation was superior in comparison to the controls, homopolymer P[Asp(DET)], and branched polyethyleneimine (BPEI). The control vectors displayed a significant vessel occlusion by thrombus, which the micellar vectors studied did not exhibit (Akagi et al. 2007 ). The results of this study show that limitations with the current technology which uses viral vectors, such as antigenicity and oncogenicity, directs research towards use of non-viral vectors, since the safety and efficacy profiles of nanomicelles are superior (Kagaya et al. 2011 ).
Recently, an intelligent delivery system which responds to the oxidative atherosclerotic plaque microenvironment was reported (Wu et al. 2018 ). Block copolymer micelles were constructed using poly(ethylene glycol) and poly(propylene sulphide) (PEG-PPS). The micelles were used to solubilise andrographolide to decrease inflammatory response and the level of reactive oxygen species (ROS) for atherosclerosis treatment. Andrographolide is a plant-derived compound which exhibits cardiovascular protection effects via downregulation of inducible nitric oxide synthase expression and upregulation of endothelial nitric oxide synthase expression (Kajal et al. 2016 ). The stimuli responsive micelles formed resulted in rapid drug release triggered by the ROS, but also consumed the ROS by itself at the pathologic sites, resulting in the suppression of the pro-inflammatory cytokines (Wu et al. 2018 ). The authors concluded that the novel formulation demonstrated excellent therapeutic effects both in vitro and in vivo, and hence, it may have promising potential against atherosclerosis (Wu et al. 2018 ).
Cationic-mixed polymeric micelles have been developed for targeted delivery of lumbrokinase (LK) for the treatment of thrombosis (Pan et al. 2018 ). LK is a fibrinolytic enzyme originated from earthworm which has shown to possess antithrombotic action (Wang et al. 2013 ). The micelles consisted of a block copolymer of polycaprolactone- b -poly(2-(dimethylamino) ethyl methacrylate) (PCL-PDMAEMA), as well as a block copolymer of methoxy polyethylene glycol- b -polycaprolactone (mPEG-PCL) missed with a block copolymer of polycaprolactone- b -polyethylene glycol with an RGD peptide conjugated (PCL-PEG-RGDfk) forming a treatment known as LKTM (Pan et al. 2018 ). The formulations were tested in vivo in a thrombosis model in mice. The study showed that those mice treated with LKTM exhibited a significantly shorter tail bleeding time compared to those treated with LK alone or the LK micelles without targeting, hence, suggesting that the novel LKTM had effectively reduced the bleeding risk (Pan et al. 2018 ).
Other mixed micelle systems reported include the blending of different Pluronic ® mixtures (F127 &P123) for the solubilisation of olmesartan medoxomil (OLM) (El-Gendy et al. 2017 ). OLM is an antihypertensive drug with poor aqueous solubility which results in low bioavailability (Norwood et al. 2002 ) OLM was loaded into mixed micelles carriers to overcome this physicochemical barrier. Three drugs: Pluronic ® mixture ratios (1:40, 1:50, and 1:60) and various F127:P123 ratios were prepared. The study showed that the mixed micelles were capable of up to 43% release efficiency compared to 35% in the drug suspension (El-Gendy et al. 2017 ).
The term dendrimer stems from the Greek ‘dendron’, meaning tree, accurately reflecting the structure of these repeatedly branched molecules (Crampton and Simanek 2007 ). The uniqueness of dendrimers stems from their multibranched, three-dimensional architecture, coupled with low polydispersity and great functionality (Sherje et al. 2018 ). There are many apparent advantages to the utilisation of dendrimers as non-viral vectors for therapeutic indications, as opposed to other nanotechnologies. Their high solubility, greater stability, minimal immunogenicity, and ability to facilitate effective delivery of drug molecules, DNA, and RNAs make them superior to other viral and non-viral counterparts (Mendes et al. 2017 ). One drawback to dendrimers is that they often possess highly charged exteriors due to their high number of branches at their surface all with their own surface charges; this can often result in either highly cationic or highly anionic nature and, if not fully addressed, can lead to toxicity issues (Jain et al. 2010 ).
The topology of dendrimers is classified as the focal core, multiple peripheral functional groups, and building blocks with a number of repeating units within the inner core (Jang and Aida 2003 ). The focal core within a dendrimer has been exploited to host chemical species within, due to the nanoscale environment generated through extensive branching. Second, dendrimers can display various, multiple functional groups on the periphery, allowing for a variety of interactions between other dendrimers and the surrounding molecular environment. Coupled with this, the repeated units permit flexibility within the dendrimer, allowing for the manipulation and encapsulation of drug molecules within (Jang and Aida 2003 ). The exclusivity of these properties to dendritic molecules gives rise to the possibility for their use as drug delivery systems. The improved efficacy and lower leakage percentages in dendrimers make them more suitable in various formulations, including oral, ocular, and transdermal, since the pharmacokinetic parameters for dendrimers are also promising (Yu et al. 2015 ).
Nitric oxide (NO) is a potent vasodilator, regulator of vascular cell proliferation, and maintains vascular homeostasis. Due to these effects, it can, therefore, play a key role in preventing atherosclerosis (Napoli et al. 2006 ). Studies have shown that there is potential for NO encapsulation within dendrimers as a targeted therapy for damaged tissue. Lu et al. ( 2011 ) reported that NO release was successful from poly(propylene imine) dendrimers, which shows promise in their potential uses as drug delivery systems (Lu et al. 2011 ). Dendrimers with potential as gene delivery agents for the treatment of CVDs have also been investigated, in an effort to control the upregulation of inflammation in targeted sites within insulted vasculature. Studies have shown that dendrimers carrying DNA plasmids enhanced survivability, stability, and viability of the gene within the nucleus (Gothwal et al. 2015 ), demonstrating viability as prospective therapeutic agents.
Recently, a dendrimer composed of poly(glutamic acid) and poly(ethylene glycol) (PEG) (Gn-PEG-Gn) was reported for the delivery of nattokinase (NK) (Zhang et al. 2017 ). NK is a thrombolytic drug, which possessed high safety levels and low side effects, however, extremely sensitive to degradation from the external environment. Hence, careful formulation is required for the drug to be clinically useful. The NK-loaded G3-PEG-G3 dendrimer formed exhibited excellent thrombolytic activity in vitro, and further work is ongoing to elucidate the in vivo potential of this system (Zhang et al. 2017 ).
Another study reported the development of a poly(amidoamine) (PAMAM) dendrimer-based system as drug carriers for combined delivery of ramipril (RAPL) and hydrochlorothiazide (HCTZ) (Singh et al. 2017 ). RAPL is an antihypertensive drug and HCTZ is a diuretics drug, both of which are used in the treatment of high blood pressure (de Leeuw and Birkenhäger 1987 ; Roush and Sica 2016 ). At 0.8% (w/v), dendrimer concentration the RAPL solubility was increased 4.91-fold with amine-terminated dendrimer, whilst the optimal dendrimer for HCTZ solubilisation (3.72-fold) was with carboxy-terminated functional groups. The novel formulations resulted in more rapid dissolution compared to the free drugs. Hybrid formulations showed similar dissolution patterns compared to the single drug-loaded dendrimer. The authors concluded that the formulation strategy held promise for application and more work is ongoing to push this technology forward (Singh et al. 2017 ).
Gel nanoparticles
The least common on the polymer-based particles are the gelated nanoparticles. These nanoparticles form at with increased polymer concentration into hydrogel matrices into which drugs can be incorporated. These systems allow for a greater retardation of drug release and greater long-term stability (Hamidi et al. 2008 ).
Regeneration of the ischaemic heart through utilisation of vascular endothelial growth factor (VEGF)-loaded core/shell nanoparticles and their gelation behaviour was monitored to assess vascular cell regeneration (Oh et al. 2010 ). The core of the gel nanoparticle was constituted from lecithin containing VEGF, with a shell comprising of Pluronic F-127 (poly(ethylene oxide)-poly(propylene oxide)-poly(ethylene oxide) triblock copolymer) (Oh et al. 2010 ). The temperature-induced gel was formed when the nanoparticles were added to a solution containing propylene glycol monocaprylate at body temperature. Gelated and non-gelated nanoparticles were added to the in vivo sample and functional analysis tests were conducted on the myocardial tissue. The results demonstrated that there was a greater improvement to heart function (cardiac output and ejection fraction) with gelated VEGF-loaded core/shell nanoparticles as compared to non-gelated nanoparticulates (Oh et al. 2010 ).
Nano-coated stents
Coronary artery bypass graft (CABG) and percutaneous transluminal coronary angioplasty (PTCA)/percutaneous coronary intervention (PCI) are invasive therapies used commonly within clinical practice to relive blockages in myocardial vasculature. CABG involves major cardiac surgery, whereas PTCA is a non-surgical procedure, whereby an artery is widened using a balloon and, in some instances, is held open using a stent (NICE 2008 ). The stents used are composed of thin wire mesh, which is used to retain sufficient blood flow through the previously occluded artery, thus preserving its patency (Fig. 4 ).
Use of a stent to widen an occluded blood vessel
The overbearing issue with the use of stents in reperfusion of cardiac tissue leads to a significant risk of restenosis within the vasculature. Restenosis occurs due to overdistention of the diseased vessel, resulting in endothelial disruption, internal elastic laminal fracture, and medical dissection (Bennett 2003 ). A number of processes contribute to in-stent stenosis including elastic recoil, relocation of axially positioned plaques, and negative remodelling. Neointima formation is the major cause of in-stent stenosis, whereby platelets, mitogens, and cytokines aggregate. Cell proliferation results and synthesis of the extracellular matrix and collagen lead to the creation of the neointima (Bennett 2003 ).
A method that can be employed to reduce the incidence of in-stent stenosis is the use of drug-eluting stents (DES), which release the incorporated drug to the locality of its placement. The stents can be coated in various polymers, such as poly(lactic- co -glycolic acid) (PLGA), polyethylene glycol (PEG), and polycaprolactone (PCL) (Acharya et al. 2012 ). The use of polyhedral oligomeric silsesquioxane poly(carbonate-urea) urethane (POSS-PCU) polymer with attached anti-CD34 antibodies was studied as a possible nanocomposite polymer to coat bare metal stents, in an attempt to improve endothelial revascularisation (Tan et al. 2013 ). The results of this study indicated that POSS-PCU had advanced biocompatibility and haemo-compatibility in vitro. This is further supported by research into the use of POSS-PCU in the field of heart valve replacements. Synthetic valves made of POSS-PCU nanocomposites have been successfully tested, demonstrating their biocompatibility and biostability against oxidation, hydrolysis, and enzymatic attack in vitro and in vivo (Kidane et al. 2009 ). However, it has also been demonstrated that the use of drug-eluting stents in animal models led to delayed arterial healing and promoted inflammation at the site of the overlap in the implanted stents. Overlapping paclitaxel-eluting stents (PES) showed a greater degree of fibrin deposition and neointima formation, raising concerns over safety in human subjects, drug toxicity, and further systemic complications arising from sites of overlap (Finn et al. 2005 ). Use of POSS-PCU as a nanocomposite coating on stents has been taken forward into human trials. The first-in-man trial (FIM) studied the efficacy and long-term implications of non-polymeric drug-coated stents (DCS) in patients with de novo coronary artery disease, with follow-up times of 4 months, 12 months, and 5 years. Notably, at 4 months, there was a significantly lower rate of lumen loss with the DCS than PES. The results demonstrated no inferiority to PES in the rate of lumen loss, a subclass of neointimal hyperplasia (Costa et al. 2016 ). The use of nano-coatings on stents is being trialled currently in the United States: The COBRA PzF Stenting to Reduce the Duration of Triple Therapy in Patients Treated With Oral Anticoagulation Undergoing Percutaneous Coronary Intervention trial is ongoing and aims to investigate if the COBRA PzF stent with a polyzene-F nano-coating can successfully reduce bleeding compared to DES, through reducing the duration of oral anticoagulation therapy to 14 days, rather than the current 6 months, in patients undergoing PCI (US National Library of Medicine 2018 ). Polyzene-F is a new generation nano-coating based on cobalt–chromium, designed to be highly biocompatible and easily deliverable (CeloNova Biosciences 2018 ).
Stents and drug-eluting stents are one of the most established technologies for cardiovascular disease treatment. However, as discussed, there are problems in their usage, and although nanotechnology has been employed in their coating to reduce occurrence of rejection in vivo and restenosis, there is a move away from these technology towards flexible polymer scaffolds.
Nanoparticles for diagnostic imaging
Nanoparticles also have a place in medicine to fulfil an unmet need in the field of imaging. Nanoparticles offer real potential in medical imaging due to their good bioavailability profiles, versatility of use, and ease of manipulation. Nanoparticles offer adaptable qualities to generate multimodal and multifunctional imaging vehicles with modifiable features, allowing for the integration of contrast materials, ligands, and functional groups (Stendahl and Sinusas 2015 ). Cardiovascular magnetic resonance (CMR) is a non-invasive method for the detection of inflammation: Due to the pathophysiology of coronary heart disease, inflammation is a major complication of the illness, and early detection through the utilisation of these methods can improve prognosis. CMR relies on the observation of changes in myocardial tissue configuration (Bietenbeck et al. 2015 ). Gadolinium shows the composition of plaques, including the details of the fibrous cap, necrotic core (Cai et al. 2005 ), macrophage content, and extent of plaque revascularisation (Kampschulte et al. 2004 ). Furthermore, contrast-enhanced CMR (ceCMR) can be employed for the recognition of myocardial oedema, necrosis, and fibrosis, using gadolinium or fluorine-19 ( 19 F) contrast agents (Friedrich et al. 2009 ). Healthy myocardium clears the contrast agent rapidly, while damaged tissues retain the dye (Bietenbeck et al. 2015 ). T1-weighted imaging established CMR imaging as a technique, whereby gadolinium-contrast agent was detected in > 90% of the myocardium following insult as a result of induced MI in in vivo models, successfully demonstrating the accuracy of CMR as a diagnostic tool (Kim et al. 1996 ). Such advances in diagnostic imaging will also allow a better patient-centred therapy, by clearer identification of atherosclerotic plaques and the extent of atherosclerosis to guide the dosage of statins required to have a better prophylactic effect, for example.
Gold nanoparticles have also shown great promise in both cardiovascular medicine and oncology imaging. Gold is a noble metal and essentially an inert material which is stable and biocompatible (Thakor et al. 2011 ). Gold particulates occur in a variety of shapes and sizes, including rods, spheres, cages, stars, crescents, and prisms, with sizes varying from 1 to 500 nm. Gold particles are excreted renally. The elimination rate through glomerular filtration can be modified by attaching PEG chains to the nanoparticle surface to increase hydrophilicity and circulation time of the nanoparticle (Varna et al. 2018 ). Gold nanoparticles can be used in cardiovascular optical imaging: photoacoustic imaging is based on detection of both ultrasound and optical imaging, whereby light is used a source of excitation and ultrasound detects sound waves generated by the target, generating images of optical absorption (Chen et al. 2014 ).
Future perspectives
Medical technology and treatments for CVDs, in particular, have significantly advanced in an aim to increase the quality of and prolong life. It is imperative to find better solutions for the treatment of CVD as it is estimated that only 15% of current guidelines for practice are supported by high-quality evidence (Califf 2016 ). Better lifestyle, diet, medial intervention, earlier detection, prevention strategies, and improved standard of living have all contributed to better prognoses. A large proportion of all premature deaths under the age of 75, within persons whom are residents of the European Union are due to CVDs. Therefore, it is imperative that solutions are sought quickly to effectively treat this pandemic, with the goal to prevent around 80% of all cardiovascular diseases through the use of nanotechnologies (Letourneur et al. 2014 ). As of 2014, a study showed that there were 122 nanomedicines going through clinical development, of which 17 had made it to phase II or III trials (Letourneur et al. 2014 ). Of those in progress, 70 products were oncology based, 19 for infectious diseases, and only 7 for cardiovascular medicine (Letourneur et al. 2014 ). This highlights the desperate need for greater research and development in this field. There are a number of current, ongoing trials into the therapeutic use of nanotechnology in cardiovascular medicine, notably, the NanoAthero project, funded by the European Commission. The objectives of this phase I trial include successfully undertaking thrombus imaging and treating vulnerable plaques using novel carriers (European Commission 2017 ). Further to this, Habib et al. are currently studying the possibility of diagnosing heart failure using breath samples and sensors based on nanomaterials (US National Library of Medicine 2018 ). Further clinical trials are being conducted in the uses of nanotechnology in drug-eluting stents in comparison to bare metal stents: The research has a participant size of 8000 and thus far has demonstrated the promising results (US National Library of Medicine 2018 ).
The evidence presented within this review demonstrates that nanotechnology does hold potential for application in the treatment of CVD, demonstrated by translational clinical trials. With increasing investment into the field of nanotechnology and suitable infrastructure worldwide, it is only a matter of time before nanomedicines, nanomaterial devices, and other related technologies successfully complete the rigorous clinical trial process and come onto the market. A certainty is that nanotechnology holds promise to improve patient health and well-being, and that any progression on the current therapies will positively impact the lives of patients globally. To have the greatest possible impact in medicine, more in vivo studies will need to be conducted and clinical trials commissioned to fully understand the behaviours of nanoparticulates systemically. As the future in therapeutics is becoming more geared towards personalised medicine, nanotechnology may be best placed to achieve this intended goal to tailor treatment to individual disease states. Furthermore, increasing healthcare costs mean that it is imperative to seek alternatives to the existing pharmacological and surgical management, to curb these exponential increases. However, there is substantial evidence to support the concept that nanotechnology is yet to make its full impact to revolutionise medicine.
Acharya G, Lee C, Lee Y (2012) Optimization of cardiovascular stent against restenosis: factorial design-based statistical analysis of polymer coating conditions. PLoS One 7:e43100
Article CAS Google Scholar
Aisha AFA, Abdulmajid AMS, Ismail Z, Alrokayan SA, Abu-Salah KM (2015) Development of polymeric nanoparticles of Garcinia mangostana xanthones in eudragit RL100/RS100 for anti-colon cancer drug delivery. J Nanomater 16:385 ( Article 2015:ID 701979 )
Google Scholar
Akagi D, Oba M, Koyama H, Nishiyama N, Fukushima S, Miyata T, Nagawa H, Kataoka K (2007) Biocompatible micellar nanovectors achieve efficient gene transfer to vascular lesions without cytotoxicity and thrombus formation. Gene Ther 14:1029–1038
Akbarzadeh A, Rezaei-Sadabady R, Davaran S, Joo S, Zarghami N, Hanifehpour Y, Samiei M, Kouhi M, Nejati-Koshki K (2013) Liposome: classification, preparation, and applications. Nanoscale Res Lett 8:102
Al Meslmani B, Mahmoud G, Bakowsky U (2017) Development of expanded polytetrafluoroethylene cardiovascular graft platform based on immobilization of poly lactic- co -glycolic acid nanoparticles using a wet chemical modification technique. Int J Pharm 529:238–244
Alaarg A, Hamers A, Versloot M, Lobatto M, Mulder WJM, Stroes ESG, Storm G, Metselaar JM (2015) Targeted liposomal drug delivery to inhibit atherosclerotic plaque inflammation. Atherosclerosis. 241:e87
Article Google Scholar
Allijn IE, Czarny SMS, Wang X, Chong SY, Weiler M, da Silva AE, Metselaar JM, Lam CSP, Pastorin G, de Kleijn DPV, Storm G, Wang J-W, Schiffelers RM (2017) Liposome encapsulated berberine treatment attenuates cardiac dysfunction after myocardial infarction. J Control Rel 247:127–133
Banach M, Serban C, Sahebkar A, Mikhailidis D, Ursoniu S, Ray K, Rysz J, Toth P, Muntner P, Mosteoru S, García-García H, Hovingh G, Kastelein J, Serruys P (2015) Impact of statin therapy on coronary plaque composition: a systematic review and meta-analysis of virtual histology intravascular ultrasound studies. BMC Med 13:1–20
Banik BL, Fattahi P, Brown JL (2016) Polymeric nanoparticles: the future of nanomedicine. WIREs Nanomed Nanobiotechnol 8:271–299
Bennett MR (2003) In-stent stenosis: pathology and implications for the development of drug eluting stents. Heart 89:218–224
Bietenbeck M, Florian A, Sechtem U, Yilmaz A (2015) The diagnostic value of iron oxide nanoparticles for imaging of myocardial inflammation—quo vadis? J Cardiovasc Magn Reson 17:1
Binsalamah ZM, Paul A, Prakash S, Shum-Tim D (2012) Nanomedicine in cardiovascular therapy: recent advancements. Expert Rev Cardiovasc Ther 10:805–815
British Heart Foundation (2017) BHF CVD statistics compendium 2017. British Heart Foundation, London, pp 1–123
British Standards Institute (2011) PAS 71:2011: nanoparticles-vocabulary. London: BSI. [Online] https://shop.bsigroup.com/upload/Shop/Download/Nano/PAS71.pdf . Accessed 19 Apr 2018
Bulbake U, Doppalapudi S, Kommineni N, Khan W (2017) Liposomal formulations in clinical use: an updated review. Pharmaceutics 9:12
Burman A, Mukherjee R, Khatta D, Mullick S, Jaggi M, Singh M, Kumar M, Prusthy D, Gupta P, Praveen R, Singh S (2015) Biocompatible, non-biodegradable, non-toxic polymer useful for nanoparticle pharmaceutical compositions. 8,927,023 B2
Cai J, Hatsukami T, Ferguson M, Kerwin W, Saam T, Chu B, Takaya N, Polissar L, Yuan C (2005) In vivo quantitative measurement of intact fibrous cap and lipid-rich necrotic core size in atherosclerotic carotid plaque: comparison of high-resolution, contrast-enhanced magnetic resonance imaging and histology. Circulation 112:3437–3444
Califf R (2016) The future of cardiovascular medicine from the regulatory perspective. J Am Coll Cardiol 68:766–769
CeloNova Biosciences (2018) COBRA PzF nanocoated stent: COBRA PzF stent with polyzene-F nanocoating. [Online]. http://celonova.com/cobra-pzf-nanocoated-stent/ . Accessed 18 Apr 2018
Chan JW, Lewis DR, Petersen LK, Moghe PV, Uhrich KE (2016) Amphiphilic macromolecule nanoassemblies suppress smooth muscle cell proliferation and platelet adhesion. Biomaterials 84:219–229
Chen Y, Yeager D, Emelianov S (2014) Photoacoustic imaging for cancer diagnosis and therapy guidance. Cancer theranostic. Elsevier Inc., New York, pp 139–158
Chmielowski RA, Abdelhamid DS, Faig JJ, Petersen KL, Gardner CR, Uhrich KE, Joseph LB, Moghe PV (2017) Athero-inflammatory nanotherapeutics: ferulic acid-based poly(anhydride-ester) nanoparticles attenuate foam cell formation by regulating macrophage lipogenesis and reactive oxygen species generation. Acta Biomater 57:85–94
Chono S, Tauchi Y, Deguchi Y, Morimoto K (2005) Efficient drug delivery to atherosclerotic lesions and the antiatherosclerotic effect by dexamethasone incorporated into liposomes in atherogenic mice. J Drug Target 13:267–276
Costa R, Abizaid A, Mehran R, Schofer J, Schuler G, Hauptmann K, Magalhães M, Parise H, Grube E (2016) Polymer-free biolimus A9-coated stents in the treatment of de novo coronary lesions. JACC Cardiovasc Interv 9:51–64
Cote B, Carlson LJ, Rao DA, Alani AWG (2015) Combinatorial resveratrol and quercetin polymeric micelles mitigate doxorubicin induced cardiotoxicity in vitro and in vivo. J Control Release 213:128–133
Crampton HL, Simanek EE (2007) Dendrimers as drug delivery vehicles: non-covalent interactions of bioactive compounds with dendrimers. Polym Int 56:489–496
de Leeuw PW, Birkenhäger WH (1987) Short- and long-term effects of ramipril in hypertension. Am J Cardiol 59:D79–D82
De Jong WH, Borm PJA (2008) Drug delivery and nanoparticles: applications and hazards. Int J Nanomed 3:133–149
Durán-Lara E, Guzmán L, John A, Fuentes E, Alarcón M, Palomo I, Santos LS (2013) PAMAM dendrimer derivatives as a potential drug for antithrombotic therapy. Eur J Med Chem 69:601–608
El-Gendy MA, El-Assal MIA, Tadros MI, El-Gazayerly ON (2017) Olmesartan medoxomil-loaded mixed micelles: preparation, characterization and in-vitro evaluation. Future J Pharm Sci 3:90–94
Elsabahy M, Wooley K (2012) Design of polymeric nanoparticles for biomedical delivery applications. Chem Soc Rev 41:2545–2561
Eniola-Adefeso O, Heslinga MJ, Porter TM (2012) Design of nano vectors for therapy and imaging of cardiovascular diseases. Methodist DeBakey Cardiovasc J 8:13–17
European Commission (2017) Nanoathero: nanomedicine for target-specific imaging and treatment of atherosclerosis: development and initial clinical feasibility. [Online]. http://cordis.europa.eu/project/rcn/106699_en.html . Accessed 19 Apr 2018
Fares AR, El Meshad AN, Kassem MAA (2018) Enhancement of dissolution and oral bioavailability of lacidipine via pluronic P123/F127 mixed polymeric micelles: formulation, optimization using central composite design and in vivo bioavailability study. Drug Deliv 25:132–142
Finn A, Kolodgie F, Harnek J, Guerrero L, Acampado E, Tefera K, Skorija K, Weber D, Gold H, Virmani R (2005) Differential response of delayed healing and persistent inflammation at sites of overlapping sirolimus- or paclitaxel-eluting stents. Circulation 112:270–278
Fox K, Cowie M, Wood D, Coats A, Gibbs J, Underwood S, Turner R, Poole-Wilson P, Davies S, Sutton G (2001) Coronary artery disease as the cause of incident heart failure in the population. Eur Heart J 22:228–236
Friedrich M, Sechtem U, Schulz-Menger J, Holmvang G, Alakija P, Cooper L, White J, Abdel-Aty H, Gutberlet M, Prasad S, Aletras A, Laissy J, Paterson I, Filipchuk N, Kumar A, Pauschinger M, Liu P (2009) Cardiovascular magnetic resonance in myocarditis: a JACC white paper. J Am Coll Cardiol 53:1475–1487
Gao Y, Xu P, Chen L, Li Y (2010) Prostaglandin E1 encapsulated into lipid nanoparticles improves its anti-inflammatory effect with low side-effect. Int J Pharm 387:263–271
Gaucher G, Dufresne M, Sant V, Kang N, Maysinger D, Leroux J (2005) Block copolymer micelles: preparation, characterization and application in drug delivery. J Control Release 109:169–188
Geisler T, Langer H, Wydymus M, Gohring K, Zurn C, Bigalke B, Stellos K, May A, Gawaz M (2006) Low response to clopidogrel is associated with cardiovascular outcome after coronary stent implantation. Eur Heart J 27:2420–2425
Giannouli M, Karagkiozaki V, Pappa F, Moutsios I, Gravalidis C, Logothetidis S (2018) Fabrication of quercetin-loaded PLGA nanoparticles via electrohydrodynamic atomization for cardiovascular disease. Mater Today Proc 5:15998–16005
Gothwal A, Kesharwani P, Gupta U, Khan I, Amin MCIM, Banerjee S, Iyer AK (2015) Dendrimers as an effective nanocarrier in cardiovascular disease. Curr Pharm Des 21:4519–4526
Gupta A (2011) Nanomedicine approaches in vascular disease: a review. Nanomed Nanotechnol Biol Med 7:.763–779
Gupta A, Gupta M (2005) Synthesis and surface engineering of iron oxide nanoparticles for biomedical applications. Biomaterials 26:3995–4021
Hamidi M, Azadi A, Rafiei P (2008) Hydrogel nanoparticles in drug delivery. Adv Drug Deliv Rev 60:1638–1649
Harrington R, Hodgson P, Larsen R (2003) Antiplatelet therapy. Circulation 108:45–47
Hoskins C, Thoo-Lin PK, Cheng WP (2012) A review of comb-shaped amphiphilic polymers for hydrophobic drug solubilisation. Ther Deliv 3:59–79
Hosseini H, Li Y, Kanellakis P, Tay C, Cao A, Tipping P, Bobik A, Toh B-H, Kyaw T (2015) Phosphatidylserine liposomes mimic apoptotic cells to attenuate atherosclerosis by expanding polyreactive IgM producing B1a lymphocytes. Cardiovasc Res 106:443–452
Huang Z, Han Z, Ye B, Dai Z, Shan P, Lu Z, Dai K, Wang C, Huang W (2015) Berberine alleviates cardiac ischemia/reperfusion injury by inhibiting excessive autophagy in cardiomyocytes. Eur J Pharmacol 762:1–10
Immordino M, Dosio F, Cattel L (2006) Stealth liposomes: review of the basic science, rationale, and clinical applications, existing and potential. Int J Nanomed 1:297–315
Jain K, Keharwani P, Gupta U, Jain NK (2010) Dendrimer toxicity: lets meet the challenge. Int J Pharm 394:122–142
Jain PP, Leber R, Nagaraj C, Leitinger G, Lehofer B, Olschewski H, Olschewski A, Prassi R, Marsh LM (2014) Liposomal nanoparticles encapsulating iloprost exhibit enhanced vasodilation in pulmonary arteries. Int J Nanomed 9:3249–3261
Jang W-D, Aida T (2003) Dendritic Physical Gels: Structural Parameters for Gelation with Peptide-Core Dendrimers. Macromolecules 36:8461–8469
Jiang W, Rutherford D, Vuong T, Liu H (2017) Nanomaterials for treating cardiovascular diseases: a review. Bioact Mater 2:185–198
Kagaya H, Oba M, Miura Y, Koyama H, Ishii T, Shimada T, Takato T, Kataoka K, Miyata T (2011) Impact of polyplex micelles installed with cyclic RGD peptide as ligand on gene delivery to vascular lesions. Gene Ther 19:61–69
Kajal A, Kishore L, Kaur N, Gollen R, Singh R (2016) Therapeutic agents for the management of atherosclerosis from herbal sources. Beni Suef Univ J Basic Appl Sci 5:156–169
Kakran M, Sahoo NG, Li L, Judeh (2012) Fabrication of quercetin nanoparticles by anti-solvent precipitation method for enhanced dissolution. Powder Technol 223 7:59–64
Kampschulte A, Ferguson M, Kerwin W, Polissar L, Chu B, Saam T, Hatsukami T, Yuan C (2004) Differentiation of intraplaque versus juxtaluminal hemorrhage/thrombus in advanced human carotid atherosclerotic lesions by in vivo magnetic resonance imaging. Circulation 110:3239–3244
Kidane A, Burriesci G, Edirisinghe M, Ghanbari H, Bonhoeffer P, Seifalian A (2009) A novel nanocomposite polymer for development of synthetic heart valve leaflets. Acta Biomater 5:2409–2417
Kim R, Chen E, Lima J, Judd R (1996) Myocardial Gd-DTPA kinetics determine MRI contrast enhancement and reflect the extent and severity of myocardial injury after acute reperfused infarction. Circulation 94:.3318–3326
Kapil N, Datta YH, Alakbarova N, Bershad E, Selim M, Liebeskind DS, Bachour O, Rao GHR, Divani AA (2017) Antiplatelet and Anticoagulant Therapies for Prevention of Ischemic Stroke. Clin Appl Thrombosis/Hemostasis 23:301–318
Korin N, Kanapathipillai M, Matthews B, Crescente M, Brill A, Mammoto T, Ghosh K, Jurek S, Bencherif S, Bhatta D, Coskun A, Feldman C, Wagner DD, Ingber DE (2012) Shear-activated nanotherapeutics for drug targeting to obstructed blood vessels. Science 10:738–742
Kumar M, Curtis A, Hoskins C (2018) Application of nanoparticle technologies in the combat against anti-microbial resistance. Pharmaceutics 10:11
Kuo C-F, Leon L, Chung EJ, Huang R-T, Sontag TJ, Reardon CA, Getz GS, Tirrell M, Fang Y (2014) Inhibition of atherosclerosis-promoting microRNAs via targeted polyelectrolyte complex micelles. J Mater Chem B 2:8142–8153
Letourneur D, Chauvierre C, Perk J (2014) NanoAthero project: a new way to fight cardiovascular diseases. [Online]. https://www.openaccessgovernment.org/wp-content/uploads/2014/05/INSERM-ebook-plus-edit-web.pdf . Accessed 19 Apr 2018
Levchenko TS, Hartner WC, Torchilin VP (2012) Liposomes in diagnosis a and treatment of cardiovascular disorders. Methodist DeBakey Cardiovasc J 8:36–41
Letchford K, Burt H (2007) A review of the formation and classification of amphiphilic block copolymer nanoparticulate structures: micelles, nanospheres, nanocapsules and polymersomes. Eur J Pharm Biopharm 65:259–69
Lu Y, Sun B, Li C, Schoenfisch M (2011) Structurally diverse nitric oxide-releasing poly(propylene imine) dendrimers. Chem Mater 23:.4227–4233
Luo X, Li J, Guo L, Cheng X, Zhang T, Deng Y (2013) Preparation of berberine hydrochloride long-circulating liposomes by ionophore A23187-mediated ZnSO 4 gradient method. Asian J Pharm Sci 8:261–266
Ma R, Ma Z-G, Zhen C-L, Shen X, Li S-L, Li L, Zheng Y-F, Dong D-L, Sun Z-J (2017) Design, synthesis and characterization of poly(methacrylic acid-niclosamide) and its effect on arterial function. Mater Sci Eng C 77:352–359
Matoba T, Koga J-I, Nakano K, Egashira K, Tsutsui H (2017) Nanoparticle-mediated drug delivery system for atherosclerotic cardiovascular disease. J Cardiol 70:206–211
Mendes LP, Pan J, Torchilin VP (2017) Dendrimers as nanocarriers for nucleic acid and drug delivery in cancer therapy. Molecules 22:E1401
Mitchell P, Marle D, Donkor A, Shote A, McDonagh T, Hardman S, Dargie H, Cleland J (2015) National heart failure audit. National Institute for Cardiovascular Outcomes Research, London, pp 1–72
Mourya VK, Inamdar N, Nawale RB, Kulthe SS (2011) Polymeric micelles: general considerations and their application. Indian J Pharm Ed Res 45:128–138
Mukhametova LI, Zakharyan EM, Karakhanov EA, Gershkovich KB, Varfolomeyev SD (2017) Thrombolytic and fibrinogenolytic properties of bioconjugate streptokinase-polyamidoamine dendrimers in vitro. Thromb Res 154:50–52
Murohara T, Margiotta J, Phillips LM, Paulson JC, DeFrees S, Zalipsky S, Guo LS, Lefer AM (1995) Cardioprotection by liposome-conjugated sialyl Lewisx-oligosaccharide in myocardial ischaemia and reperfusion injury. Cardiovasc Res 30:965–974
Nahar K, Absar S, Gupta N, Kotamraju VR, McMurtry IF, Oka M, Komatsu M, Nozik-Grayck E, Ahsan F (2014) Peptide-coated liposomal fasudil enhances site specific vasodilation in pulmonary arterial hypertension. Mol Pharm 11:4374–4384
Napoli C, de Nigris F, Williams-Ignarro S, Pignalosa O, Sica V, Ignarro LJ (2006) Nitric oxide and atherosclerosis: an update. Nitric Oxide 15:265–79
National Institute for Health and Clinical Excellence (NICE) (2008) Guidance on the use of coronary artery stents. NICE guidelines (TA71)
National Institute for Health and Clinical Excellence (NICE) (2010) Chronic heart failure in adults: management. Guideline NICE (CG108)
National Institute for Health and Clinical Excellence (NICE) (2013) Myocardial infarction: cardiac rehabilitation and prevention of further cardiovascular disease. NICE guideline (CG172)
Norwood D, Branch E, Smith B, Honeywell M (2002) Olmesartan medoxomil for hypertension: a clinical review. P&T Drug Forecast 27:611–618
Oh K, Song J, Yoon S, Park Y, Kim D, Yuk S (2010) Temperature-induced gel formation of core/shell nanoparticles for the regeneration of ischemic heart. J Control Release 146:207–211
Önyüksel H, Ikezaki H, Patel M, Gao X-p, Rubinstein I (1999) A novel formulation of VIP in sterically stabilized micelles amplifies vasodilation in vivo. Pharm Res 16:155–160
Palekar RU, Myerson JW, Schlesinger PH, Sadler JE, Pan H, Wickline SA (2013a) Thrombine targeted liposomes establish a sustained localized anticlotting barrier against acute thrombosis. Mol Pharm 10:4168–4175
Palekar RU, Myerson JW, Schlesinger PH, Pan H, Wickline SA (2013b) Thrombin-inhibiting liposomes as a targeted therapeutic for the treatment of acute thrombosis. FASEB J 27:1
Palekar RU, Jallouk AP, Lanza GM, Pan H, Wickline SA (2015) Molecular imaging of atherosclerosis with nanoparticle-based fluorinated MRI contrast agents. Nanomed (Lond) 10:11
Pan Y, Wang X, Yin Z (2018) Synthesis and evaluation of cationic polymeric micelles as carriers of lumbrokinase for targeted thrombolysis. Asian J Pharm Sci ( In press )
Pang X, Lu Z, Du H, Yang X, Zhai G (2014) Hyaluronic acid-quercetin conjugate micelles: synthesis, characterization, in vitro and in vivo evaluation. Colloid Surface B Biointerface 123:778–786
Patel D, Bailey S (2007) Nanotechnology in cardiovascular medicine. Catheter Cardiovasc Interv 69:643–654
Patil M, Mehta D, Guvva S (2008) Future impact of nanotechnology on medicine and dentistry. J Indian Soc Periodontol 12:34–40
Pazzini C, Marcato PD, Prado LB, Alessio AM, Höehr NF, Montalvão S, Paixão D, Durán N, Annichino-Bizzacchi JM (2015) Polymeric nanoparticles of enoxaparin as a delivery system: in vivo evaluation in normal rats and in a venous thrombosis rat model. J Nanosci Nanotechnol 15:4837–4843
Ponikowski P, Voors A, Anker S, Bueno H, Cleland J, Coats A, Falk V, González-Juanatey J, Harjola V, Jankowska E, Jessup M, Linde C, Nihoyannopoulos P, Parissis J, Pieske B, Riley J, Rosano G, Ruilope L, Ruschitzka F, Rutten F, van der Meer P (2016) 2016 ESC guidelines for the diagnosis and treatment of acute and chronic heart failure. Eur Heart J 37:2129–2200
Qin H, Zhao Y, Zhang J, Pan X, Yang S, Xing D (2016) Inflammation-targeted gold nanorods for intravascular photoacoustic imaging detection of matrix metalloproteinase-2 (MMP 2) in atherosclerotic plaques. Nanomed Nanotechnol Biol Med 12:1765–1774
Roush GC, Sica DA (2016) Diuretics for hypertension: a review and update. Am J Hypertens 29:1130–1137
Ruiz-Esparza G, Flores-Arredondo J, Segura-Ibarra V, Torre-Amione G, Ferrari M, Blanco E, Serda R (2013) The physiology of cardiovascular disease and innovative liposomal platforms for therapy. Int J Nanomed 8:629–640
Sahoo S, Labhasetwar V (2003) Nanotech approaches to drug delivery and imaging. Drug Discov Today 8:1112–1120
Sailor G, Seth AK, Parmer G, Chauhan S, Javia A (2015) Formulation and in vitro evaluation of berberine containing liposome optimized by 3 2 full factorial designs. J Appl Pharm Sci 5:023–028
Sanchez-Gaytan BL, Fay F, Lobatto ME, Tang J, Ouimet M, Kim YT, van der Staay SEM, van Rijs SM, Priem B, Zhang L, Fisher EA, Moore KJ, Langer R, Fayad ZA, Mulder WJM (2015) HDL-mimetic PLGA nanoparticle to target atherosclerosis plaque. Macrophages Bioconj Chem 26:443–451
Sanna V, Pala N, Sechi M (2014) Targeted therapy using nanotechnology: focus on cancer. Int J Nanomed 9:467–483
CAS Google Scholar
Sherje AP, Jadhav M, Dravyakar BR, Kadam D (2018) Dendrimers: a versatile nanocarrier for drug delivery and targeting. Int J Pharm 548:7070–7720
Siddalingappa B, Benson HAE, Brown DH, Batty KT, Chen Y (2015) Stabilization of resveratrol in blood circulation by conjugation to mPEG and mPEG-PLA polymers: investigation of conjugate linker and polymer composition on stability, metabolism, antioxidant activity and pharmacokinetic profile. PLoS One 10:e0118824
Singh MK, Pooja D, Kulhari H, Jain SK, Sistla R, Chauhan AS (2017) Poly(amidoamine) dendrimer-mediated hybrid formulation for combination therapy of ramipril and hydrochlorothiazide. Eur J Pharm Sci 96:84–92
Singla P, Singh O, Chabba S, Mahajan RK (2018) Pluronic-SAILs (surface active ionic liquids) mixed micelles as efficient hydrophobic quercetin drug carriers. J Mol Liq 249:294–303
Stendahl J, Sinusas A (2015) Nanoparticles for cardiovascular imaging and therapeutic delivery, part 1: compositions and features. J Nucl Med 56:1469–1475
Tan A, Farhatnia Y, Goh DGN, de Mel A, Lim J, Teoh S, Malkovskiy A, Chawla R, Rajadas J, Cousins B, Hamblin M, Alavijeh M, Seifalian A (2013) Surface modification of a polyhedral oligomeric silsesquioxane poly(carbonate-urea) urethane (POSS-PCU) nanocomposite polymer as a stent coating for enhanced capture of endothelial progenitor cells. Biointerphases 8:.23
Tefas LR, Tomata I, Achim M, Vlase L (2015) Development and optimization of quercetin loaded PLGA nanoparticles by experimental design. Clujul Med 88:214–223
Thakor A, Jokerst J, Zavaleta C, Massoud T, Gambhir S (2011) Gold nanoparticles: a revival in precious metal administration to patients. Nano Lett 11:4029–4036
Tomalia DA, Reyna LA, Svenson S (2007) Dendrimers as multi-purpose nanodevices for oncology drug delivery and diagnostic imaging. Biochem Soc Trans 35:61–67
Trinh H, Joseph M, Cholkar K, Mitra R, Mitra A (2017) Nanomicelles in diagnosis and drug delivery. In: Mitra A, Cholkar K, Mandal A (eds) Emerging nanotechnologies for diagnostics, drug delivery and medical devices. Elsevier, Amsterdam, Netherlands, pp 45–48
US National Library of Medicine (2018) Randomized trial of COBRA PzF stenting to reduce duration of triple therapy. [Online]. https://clinicaltrials.gov/ct2/show/NCT02594501?term=COBRA+REDUCE&rank=1 . Accessed 18 Apr 2018
Varna M, Xuan H, Fort E (2018) Gold nanoparticles in cardiovascular imaging. Wiley Interdiscip Rev Nanomed Nanobiotechnol 10:1–19
Wan TC, Tosh DK, Du L, Gizewski ET, Jacobson KA, Auchampach JA (2011) Polyamidoamine (PAMAM) dendrimer conjugate specifically activates the A3 adenosine receptor to improve post-ischemic/reperfusion function in isolated mouse hearts. BMC Pharmacol 11:11
Wang KY, Tuli L, Cooper E, Wang N, Liu D (2013) Recombinant protein production of earthworm lumbrokinase for potential antithrombic application. Evid Based Complement Altern Med 2013:783971
Weaver J (2013) PLOS Biology 11(4):e1001533
World Health Organization (WHO) (2017) Cardiovascular diseases (CVDs). [Online]. http://www.who.int/mediacentre/factsheets/fs317/en/ . Accessed 18 Apr 2018
Wu T, Chen X, Wang Y, Xiao H, Peng Y, Lin L, Xia W, Long M, Tao J, Shuai X (2018) Aortic plaque-targeted andrographolide delivery with oxidation-sensitive micelle effectively treats atherosclerosis via simultaneous ROS capture and anti-inflammation. Nanomed Nanotechnol Biol Med 14:2215–2226
Yu M, Jie X, Xu L, Chen C, Shen W, Cao Y, Lian G, Qi R (2015) Recent advances in dendrimer research for cardiovascular diseases. Biomacromolecules 16:2588–2598
Zhai Y, Guo S, Liu C, Yang C, Dou J, Li L, Zhai G (2013) Preparation and in vitro evaluation of apigenin-loaded polymeric micelles. Colloid Surface A Phys Eng Asp 429:24–30. https://doi.org/10.1016/j.colsurfa.2013.03.051
Zhang Y, Yang S, Li M, Iqbal J, Bourantas CV, Mi Q, Yu Y, Li J, Zhao S, Tian N, Chen S (2014) Berberine attenuates adverse left ventricular remodeling and cardiac dysfunction after acute myocardial infarction in rats: role of autophagy. Clin Exp Pharmacol Physiol 41:995–1002
Zhang S-F, Gao C, Lü S, He J, Liu M, Wu C, Liu Y, Zhang X, Liu Z (2017) Synthesis of PEGylated polyglutamic acid peptide dendrimer and its application in dissolving thrombus. Colloid Surface B Biointerface 159:284–292
Zhang M, Li C, Zhou D, Ding C, Jin Y, Tian Q, Meng X, Pu K, Zhu Y (2018) Cyclic RGD functionalized liposomes encapsulating urokinase for thrombolysis. Acta Biomater 70:227–236
Download references
Author information
Authors and affiliations.
School of Pharmacy, Institute of Science and Technology for Medicine, Keele University, Keele, ST5 5BG, UK
Meera Chandarana, Anthony Curtis & Clare Hoskins
You can also search for this author in PubMed Google Scholar
Corresponding author
Correspondence to Clare Hoskins .
Additional information
Publisher’s note.
Springer Nature remains neutral with regard to jurisdictional claims in published maps and institutional affiliations.
Rights and permissions
Open Access This article is distributed under the terms of the Creative Commons Attribution 4.0 International License ( http://creativecommons.org/licenses/by/4.0/ ), which permits unrestricted use, distribution, and reproduction in any medium, provided you give appropriate credit to the original author(s) and the source, provide a link to the Creative Commons license, and indicate if changes were made.
Reprints and permissions
About this article
Chandarana, M., Curtis, A. & Hoskins, C. The use of nanotechnology in cardiovascular disease. Appl Nanosci 8 , 1607–1619 (2018). https://doi.org/10.1007/s13204-018-0856-z
Download citation
Received : 03 May 2018
Accepted : 10 August 2018
Published : 16 August 2018
Issue Date : October 2018
DOI : https://doi.org/10.1007/s13204-018-0856-z
Share this article
Anyone you share the following link with will be able to read this content:
Sorry, a shareable link is not currently available for this article.
Provided by the Springer Nature SharedIt content-sharing initiative
- Nanomedicine
- Cardiovascular disease
- Nanotechnology
- Medical therapeutics
- Find a journal
- Publish with us
- Track your research
Twenty Years of Cardiovascular Complications and Risk Factors in Patients With Type 2 Diabetes: A Nationwide Swedish Cohort Study
Affiliations.
- 1 Institute of Cardiovascular and Medical Sciences, British Heart Foundation Glasgow Cardiovascular Research Centre, UK (N.S., J.M.).
- 2 Department of Molecular and Clinical Medicine (J.B., A.R., E.O., N.B., J.H., K.S., A.R.), Institute of Medicine, University of Gothenburg, Sweden.
- 3 Wallenberg Laboratory for Cardiovascular and Metabolic Research (J.B., A.R., E.O., N.B., A.R.), Institute of Medicine, University of Gothenburg, Sweden.
- 4 Region Västra Götaland, Department of Specialist Medicine, Sahlgrenska University Hospital, Gothenburg, Sweden (B.E.).
- 5 Population Health Research Institute, McMaster University and Hamilton Health Sciences, Hamilton, ON, Canada (H.C.G.).
- 6 Department of Internal Medicine, University of Texas Southwestern Medical Center, and Parkland Health and Hospital System, Dallas (D.K.M.).
- 7 Brigham and Women's Hospital Heart and Vascular Center, Harvard Medical School, Boston, MA (D.B.).
- PMID: 37154040
- DOI: 10.1161/CIRCULATIONAHA.122.063374
Background: The goal of this work was to investigate trends (2001-2019) for cardiovascular events and cardiometabolic risk factor levels in individuals with type 2 diabetes (T2D) and matched control subjects.
Methods: This study included 679 072 individuals with T2D from the Swedish National Diabetes Register and 2 643 800 matched control subjects. Incident outcomes comprised coronary artery disease, acute myocardial infarction, cerebrovascular disease, and heart failure (HF). Trends in time to first event for each outcome were analyzed with Cox regression and standardized incidence rates. In the group with T2D, Cox regression was also used to assess risk factor levels beyond target and outcomes, as well as the relative importance of each risk factor to each model.
Results: Among individuals with T2D, incidence rates per 10 000 person-years in 2001 and 2019 were as follows: acute myocardial infarction, 73.9 (95% CI, 65.4-86.8) and 41.0 (95% CI, 39.5-42.6); coronary artery disease, 205.1 (95% CI, 186.8-227.5) and 80.2 (95% CI, 78.2-82.3); cerebrovascular disease, 83.9 (95% CI, 73.6-98.5) and 46.2 (95% CI, 44.9-47.6); and HF, 98.3 (95% CI, 89.4-112.0) and 75.9 (95% CI, 74.4-77.5). The incidence for HF plateaued around 2013, a trend that then persisted. In individuals with T2D, glycated hemoglobin, systolic blood pressure, estimated glomerular filtration rate, and lipids were independently associated with outcomes. Body mass index alone potentially explained >30% of HF risk in T2D. For those with T2D with no risk factor beyond target, there was no excess cardiovascular risk compared with control subjects except for HF, with increased hazard with T2D even when no risk factor was above target (hazard ratio, 1.50 [95% CI, 1.35-1.67]). Risk for coronary artery disease and cerebrovascular disease increased in a stepwise fashion for each risk factor not within target. Glycated hemoglobin was most prognostically important for incident atherosclerotic events, as was body mass index for incident of HF.
Conclusions: Risk and rates for atherosclerotic complications and HF are generally decreasing among individuals with T2D, although HF incidence has notably plateaued in recent years. Modifiable risk factors within target levels were associated with lower risks for outcomes. This was particularly notable for systolic blood pressure and glycated hemoglobin for atherosclerotic outcomes and body mass index for heart failure.
Keywords: cardiometabolic risk factors; cardiovascular disease; cerebrovascular disorders; coronary artery disease; diabetes mellitus, type 2; heart failure; myocardial infarction.
Publication types
- Research Support, Non-U.S. Gov't
- Atherosclerosis* / complications
- Cerebrovascular Disorders* / complications
- Cerebrovascular Disorders* / diagnosis
- Cerebrovascular Disorders* / epidemiology
- Cohort Studies
- Coronary Artery Disease* / complications
- Coronary Artery Disease* / diagnosis
- Coronary Artery Disease* / epidemiology
- Diabetes Mellitus, Type 2* / diagnosis
- Diabetes Mellitus, Type 2* / epidemiology
- Glycated Hemoglobin
- Heart Failure* / diagnosis
- Heart Failure* / epidemiology
- Heart Failure* / etiology
- Myocardial Infarction* / complications
- Myocardial Infarction* / epidemiology
- Risk Factors
- Sweden / epidemiology
- Open access
- Published: 26 July 2023
Prevalence and knowledge of modifiable cardiovascular diseases risk factors among vulnerable population in Central Tanzania
- Nyasiro Sophia Gibore 1 ,
- Mariam John Munyogwa 2 ,
- Secilia Kapalata Ng’weshemi 2 &
- Ainory Peter Gesase 3
BMC Cardiovascular Disorders volume 23 , Article number: 373 ( 2023 ) Cite this article
1369 Accesses
1 Citations
Metrics details
Cardiovascular diseases (CVDs) are the global public health problem which has been associated with increasing prevalence of modifiable CVDs risk factors. This study aimed to describe the prevalence and knowledge of modifiable CVDs risk factors among vulnerable population of Central Tanzania.
A community-based cross-sectional study design was employed. A total of 749 participants were interviewed. The socio-demographic information and modifiable CVDs risk factors (behavioral and biological) were measured using a modified World Health Organization (WHO) STEPwise approach for chronic disease risk factor surveillance. Knowledge of modifiable CVDs risk factors was measured by comprehensive heart disease knowledge questionnaire. Descriptive statistics were used to describe the knowledge and prevalence of modifiable CVDs risk factors. Logistic regression analysis was used to determine the factors associated with adequate knowledge of CVDs risk factors.
The prevalence of béhavioral risk factors were; current smokers and alcohol consumers were 4.4% and 18.0% respectively, use of raw salt was 43.7%, consumption of fruit/vegetables < 5 days per week was 56.9%. The prevalence of Biological CVDs risk factors was as follows: Overall, 63.5% (33.3% overweight and 29.9% obese) were overweight or obese, 4.5% were diabetic and 43.4% were hypertensive. Only 35.4% of participants had adequate knowledge of CVDs risk factors. Being a male (AOR = 1.44, 95%CI = 1.01–2.06, p < .05), having primary education (AOR = 6.43, 95%CI = 2.39–17.36, p < .0001), being employed (AOR = 1.59, 95%CI = 1.00-2.52, p < .05), ever checked blood pressure (AOR = 0.59, 95%CI = 0.42–0.84, p < .001), family history of hypertension (AOR = 0.38, 95%CI = 0.25–0.57, p < .0001) determined adequate knowledge of CVDs risk factors.
Conclusions
This study has revealed a high prevalence of modifiable CVDs risk factors and low knowledge of CVDs risk factors. Community health promotion interventions to increase population knowledge of CVDs risk factors are recommended for the efficacious reduction of CVDs in the country.
Peer Review reports
Cardiovascular diseases (CVDs) are a group of disorders of the heart and blood vessels that include coronary artery disease, stroke, heart failure, hypertensive heart disease, rheumatic heart disease, cardiomyopathy, valvular heart disease, and peripheral artery disease [ 1 ]. These diseases are common in the general population, mainly among adults population aged < 70 years, with stroke and coronary heart diseases being the main contributors [ 1 , 2 ]. CVDs are the global public health problem, accounting for 32% of all deaths in 2019, with an alarming 80% of these deaths occurring in low- and middle-income countries [ 3 ]. It has been projected that, if appropriate measures are not undertaken, by the year 2030, CVDs will cause more than 23.6 million deaths [ 4 ].
In sub-Saharan Africa most of the population risk of CVDs is fueled by modifiable risk factors, such as smoking, hypertension, diabetes, overweight or obesity, unhealthy diet, being physical inactive, excessive alcohol consumption, raised blood lipids and psychosocial factors [ 5 ]. In Tanzania, increased urbanization, lifestyle changes, lack of awareness and rural to urban movement have been found to increase CVDs risk factors [ 6 ]. The urban population is more vulnerable due to sedentary lifestyles, higher incidence of overweight, obesity, and elevated blood cholesterol levels than rural population [ 7 ]. Studies have shown that alleviating exposure to these risk factors would improve global life expectancy by almost 5 years [ 8 , 9 ].
In order to prevent or delaying the risk of developing CVDs and improve in cardiovascular health, a public health literacy of CVDs risk factors is required [ 10 ]. Studies have shown that health literacy of CVDs risk factors can lead to success in their prevention and control through influencing individual practices towards healthy lifestyle modification, impacting the efficacy of their management and decrease risk of disease complications [ 11 , 12 , 13 ]. It is evident that individuals with inadequate health knowledge have been associated with decreased use of health care services and less likely to engage in health promotion behaviors resulting in overall poor health outcomes [ 12 , 13 ].
Whilst the world health organization is promoting knowledge of behavioral CVDs risk factors [ 1 ]. a systemic review of studies conducted in sub-Saharan Africa (SSA) have reported low levels of knowledge on CVDs risk factors [ 14 ]. In Tanzania a study conducted in rural population of Morogoro Region found only quarter of participants (25.4%) had good knowledge on CVDs risk factors [ 10 ]. Another hospital-based study conducted among companions of outpatients attending a tertiary-level cardiovascular hospital in Dar es Salaam Tanzania found that about 80.0% of study participants had good knowledge of CVDs risks factors [ 15 ]. Despite the increasing in CVDs deaths and their related risk factors in urban population of Tanzania [ 6 ], there is dearth of information regarding public knowledge of CVDs risk factors among urban population. Information on community knowledge regarding CVDs risk factors will serve as the basis for community mobilization for health education intervention strategies for preventing exposure to CVDs risk factors and resulting in reduction of the growing burden of CVDs. This study assessed prevalence and knowledge of modifiable cardiovascular diseases risk factors and factors associated among the vulnerable population in central Tanzania.
Study design and setting
A baseline survey was conducted between March to May, 2021 as part of a community-based cluster randomized controlled trial study on the effectiveness of a community-based lifestyle intervention in reducing the threats of developing CVDs risk factors among vulnerable population in Dodoma City as described in [ 16 ]. The current study analysed the baseline data before the intervention. The survey was conducted in four randomly selected wards (Nkhuhungu, Chang’ombe, Tambukareli and Ipagala). Based to the population projection of the 2017, Dodoma city was projected to have a population of 522,428 in 2022 with the average annual population growth rate of 2.4% [ 17 ]. The city is inhabited by several ethnic groups, this is due to rapid urbanization which has resulted in a large movement of people from all over the country to Dodoma city. However, the predominant indigenous ethnic groups are Gogo, Rangi and Sandawe whose main economic activities include businesses, seasonal agriculture that depends on unimodal rainfall and agro-pastoral activities. The main staple foods include stiff porridge from maize, sorghum or millet served with dried green vegetables and milk in some families.
Study participants
The study participants were male and female adults aged 25–88 years, residents of the selected ward for a period of more than four months, who consented verbally or by writing to participate in the study. Pregnant women, seriously sick and mentally ill individuals were excluded in the study.
Sample size estimation and sampling technique
The sample size was estimated to provide a 90% power to detect a proportional change in body BMI of 20% between the intervention and control groups at an alpha level of 5% and 95% confidence interval, an expected drop-out of 20%, an intercluster correlation of 0.03, and a standard deviation of 0.84, with the ratio of intervention to control of 1:1. The resulting minimum sample size for the study was 400. The study assigned 400 participants in an intervention group and another 400 participants in a control group. Therefore, the total sample size for the study was 800 participants. Simple random sampling using lottery method was used to select four wards. From each ward a systemic sample of 200 households were selected from a list of all households with eligible participants that was identified by the help of respective street leaders. The lottery method was used to select one respondent for households with more than one eligible respondent. If the selected individual in the household was unable to be interviewed, the next household on the list was chosen.
Data collection tools and procedure
Trained research assistants conducted face-to-face interviews using an electronic structured questionnaire which was developed in the open-source toolkit (KoBoToolbox) for data collection and it was accessed through mobile phone. A team of research assistants consisted of the following professionals; registered nurses, nutritionist, public health specialist and laboratory technicians collected the data. They were trained on how to collect data using a mobile phone, as well as on the study instruments and the overall data collection process. Before beginning data collecting, two weeks of training were completed. The questionnaire was adapted from WHO-steps survey [ 18 ] and comprehensive heart disease knowledge questionnaire [ 19 ], pretested and modified to meet the study environment. The following information was collected during the interview: - Socio-demographic information, knowledge of modifiable CVDs risk factors, behavioral characteristics, physical measurements and medical history. STEPS Instrument from WHO STEPS Surveillance manual [ 18 ] collected information on socio-demographic information, behavioral characteristics, physical measurements and medical history while the comprehensive heart disease knowledge questionnaire [ 19 ] was used to measure the knowledge of modifiable CVDs risk factors. To ensure content-related validity, the questionnaire was translated into Kiswahili language by two professional experts in the field of the study who were fluent in both Kiswahili and English. It was further critically evaluated by two other experts in the field so as to establish face validity.
Assessment of knowledge on modifiable CVDs risk factors
Knowledge of modifiable CVDs risk factors was assessed using 11 multiple choice questions related to CVDs risk behaviors and prevention. Knowledge scores were assigned as 1 (one) for each correct answer and 0 (zero) for wrong answer. A percentage score for each participant was calculated by dividing the sum of correct answers to the total number of questions and converted to percentage by multiplying with 100. A score of < 50% was classified as “inadequate knowledge” and a score of ≥ 50% as “adequate knowledge”.
Medical history records and behavioural risk factors Assessment
Participants were asked if they had ever had their blood pressure or blood sugar checked and if they had ever been diagnosed with hypertension or diabetes mellitus. Behavioral risk factors included alcohol consumption, smoking and dietary habits as well as use of raw table salt. Alcohol consumption status was assessed currently, past or never drunk. Smoking habits were assessed currently, past or never smoke. Dietary assessment was based on frequency of consumption of fruits and vegetables in days per week and frequency of use of raw table salt was assessed as never, sometimes or always.
Physical measurements of biological risk factors
Anthropometric measurements taken were weight (kg) and height (cm). Weight was measured to the nearest 0.1 kg using a digital scale (SECA®) and height was taken to the nearest 0.1 cm using a portable stadiometer (SECA®) placed on flat ground [ 20 ]. Height measurements were taken with heels, buttocks and upper back in perpendicular with the stadiometer [ 20 ]. Before taking measurements, participants were coached to stand in the device while having light clothing and with no shoes. Calibration of equipment was performed on a daily basis in accordance with the manufacturer’s instructions. Body mass index (BMI) was calculated using measured weight (in kg) divided by height (in meters-squared). A person was considered to be underweight if BMI < 18.5 kg/m2, normal: BMI 18.5–24.9 kg/m2, overweight: BMI 25.0– 29.9 kg/m2 and obese: BMI ≥ 30.0 kg/m2 [ 20 ]. Blood pressure was measured for three readings using a digital blood pressure (OMRON®) machine, each separated by 5 min of rest in between and with arms elevated at heart level. The average of the second and the third reading was used. Participant was considered hypertensive if had a diastolic blood pressure of ≥ 90 mmHg, and/or systolic blood pressure of ≥ 140 mmHg and/or currently taking blood pressure lowering agent [ 18 , 21 ]. Blood glucose measurement was taken with a digital glucose meter (GlucoPlus) machine following the procedure explained in the user guide manual [ 22 ]. Diabetes was diagnosed using a random blood glucose (RBG) ≥ 11.1 mmol/L and/or fasting blood glucose (FBG) ≥ 7 mmol/L or use of glucose-lowering agents [ 18 , 23 ].
Data analysis
Data were transferred from KoBoToolbox to Statistical Package for Social Sciences software (SPSS) version 26 for cleaning and analysis. Descriptive statistics were used to describe the study sample characteristics and mean for continuous variables. Categorical variables were compared using the Chi square test. Bivariate and multivariate statistics were conducted to assess for factors associated with adequate knowledge of CVDs risks factors. Variables with p ≤ .2 in the bivariate statistics were subjected to multiple logistic regression analysis for inferential analysis to determine the factors that were independently associated with adequate knowledge.
The Hosmer-Lemeshow test was employed to check the model’s fit in order to examine the accuracy of the logistic regression model. The Chi-square had 6 degrees of freedom, a value of 9.917, and a probability of P = .0.1491 which is greater than 0.05 in the test findings, showing that the logistic regression model was fit for the data set. The model explained 0.005 (Cox & Snell R Square) to 0.015 (Nagelkerke R Square) of the variation in the dependent variable, indicating that the logistic model explains 5–15% of the variation between the adequate and inadequate knowledge.
Odds ratios with their corresponding 95% confidence intervals and p-value were reported as measures of association. Statistical significance was accepted at p-value ≤ 0.05 on two-sided test.
Characteristics of study participants
A total of 749 participants were enrolled in this study between March and May 2021. The response rate of 94%. Demographic characteristics of the study participants are described in Table 1 . The age of respondents ranged from 19 to 72 years (mean ± standard deviation = 47.6 ± 14.3 years). More than half of respondents were female (57.9%). Nearly three quarters (71.7%) were married, moreover half (59.3%) had a primary education and majority (65.4%) were self-employed in small to large businesses, casual labor and farming activities.
Table 2 shows the prevalence of modifiable CVDs risk factors among study participants. Only 4.4% and 18.0% of the study participants were current smokers and alcohol drinkers respectively. Less than half (43.7%) of study participants reported to use raw table salt sometimes while about 20% reported to use it always. More than half of participants (56.9%) reported consuming fruit/vegetables less than 5 days per week. Over two-thirds (63.5%) of participants were in the category of overweight and obesity while 4.5% where underweight, 4.5% were diabetic and 43.4% were hypertensive. About 19% and 11% reported having a history of hypertension and diabetes in their family respectively.
Knowledge of modifiable CVDs risk factors
Table 3 summarizes the results of participant’s responses to the 11 questions used to assess knowledge about CVDs risk factors. More than half (59.1%) of participants were aware that physical exercise can lower the CVDs risk. Smoking and second-hand smoke was known by 66.6% of study participants as a CVDs risk. Excessive alcohol intake was identified by 70.9% as a CVDs risk. More than half (58.6%) of participants were not aware that, regular consumption of red meat and sugary food is a CVDs risk. About 50% and 57% knew that, exercising regularly and eating a high fiber diet respectively is not a risk for CVDs risk. Consumption of too much salt was recognized by (62.9%) as a CVDs risk. About 63% were aware that walking and gardening are types of exercise that can lower CVDs risk and about 60% knew that consuming a lot of vegetables and fruits prevents the risk of CVDs. The lowest knowledge score was 0% and this was scored by 13 participants, which indicate that, 2.9% of study participants knew nothing about CVDs risk factors that were asked. The highest score was 100% which indicate that only 1.7% of the study participants had excellent understanding of CVDs risk factors. The mean knowledge score was 42.6%. The overall adequate knowledge score of risk factors for CVDs was 35.4%.
Factors associated with adequate knowledge of modifiable CVDs risk factors
Table 4 shows the results of chi-square analysis of various characteristics by adequate knowledge of CVDs risk factors status. Participants aged above 60 years had a higher likelihood (76.5%, p = .001) of having inadequate knowledge of CVDs risk factors compared to participants in other age groups. Male participants displayed higher rates of adequate knowledge of CVDs risk factors compared to female participants (40.3% vs. 31.8%, p = .016). Regarding marital status, participants who were widowed showed a higher chance (78.0%, p = .007) of having inadequate knowledge of CVDs risk factors compared to other participants in this category. Participants with primary education had a higher likelihood (68.5%, p < .001) of having inadequate knowledge of CVDs risks factors compared to other participants in this education level category. There was no much differences in adequate knowledge among the retired, students and self-employed participants except for the employed who displayed higher rates (51.2%, p = .001) of adequate knowledge of CVDs risk factors compared to the above-mentioned groups. Participants who had never checked their blood pressure had a higher chance (71.2% vs. 60.2%, p = .002) of having inadequate knowledge of CVDs risk factors compared to those who had checked their blood pressure. Likewise, Participants who had never checked their blood glucose showed a higher chance (67.8% vs. 58.9%, p = .014) of having inadequate knowledge of CVDs risk factors than those who had. Participants with a positive family history of hypertension displayed a higher rate (57.4% vs. 30.3%, p < .001) of adequate knowledge of CVDs risk factors compared to those who had no family history of hypertension. Similarly, participants with a positive family history of diabetes displayed a higher rate (50.6% vs. 33.4%, p = .002) of adequate knowledge of CVDs risk factors compared to those who had no family history of diabetes.
The results of logistic regression analysis are presented in Table 5 . Twelve variables were entered into logistic regression model for analysis. Ten variables showed significant association with adequate knowledge of CVDs risk factors. After adjusting for the confounder variables (current alcohol drinking and diabetes) in the multivariate regression model, only six variables (age, sex, education level, occupation, ever checked BP and family history of high BP) remained independently associated with adequate knowledge of CVDs risk factors. When compared to their younger and elderly counterparts, the youth participants knew more about CVD risk factors. Men were more likely to have adequate knowledge of CVDs risk factors compared to women (AOR = 1.44, 95%CI = 1.01–2.06, p < .05). Participants who had primary education were 6 times more likely to have adequate knowledge of CVDs risk factors compared to participants who had no formal education (AOR = 6.43, 95%CI = 2.39–17.36, p < .0001). The employed participants had higher knowledge of CVDs risk factors compared to their counterpart the retired one (AOR = 1.59, 95%CI = 1.00-2.52, p < .05). Participants who had ever checked their blood pressure had higher odds of having adequate knowledge of CVDs risk factors than participants who had not ever checked their blood pressure (AOR = 0.59, 95%CI = 0.42–0.84, p < .001). Likewise, participants who reported to have family history of hypertension had higher odds of having adequate knowledge of CVDs risk factors compared to their counterparts who had not reported family history of hypertension (AOR = 0.38, 95%CI = 0.25–0.57, p < .0001).
The current study designed to assess the prevalence and knowledge of modifiable CVDs risk factors among the exposed population living in urban city of Dodoma, Tanzania’s central region. Overweight and obese, hypertension and low vegetable/fruit consuming were the most prevalent modifiable CVDs risk factors in this study. Overweight and obesity accounted for 63.5% of the total, with 33.6% being overweight and 29.9% being obese. These findings are comparable to those of Ajayi et al.’s study done in four sub-Saharan African adult populations of Nigeria, South Africa, Tanzania, and Uganda, which found that 31% of the adults were overweight and 34% were obese [ 24 ]. The prevalence of overweight was also comparable to that of Muhihi et al.’s study in Morogoro Tanzania, where the figure was 28.5% [ 10 ]. The overall prevalence of overweight and obesity was comparable to that of Kagaruki et al.’s study in Dar es salaam Tanzania, which reported the prevalence of 63.9% [ 25 ]. A high prevalence (75.9%) of overweight and obesity was also reported in the study of Iqbal Fahs et al. among the Lebanese Population [ 26 ]. The higher prevalence seen in this study is probably related to the consumption of unhealth diets combined with sedentary lifestyles and limited physical activity. This could be supported by the fact that in urban setting residence are busy for their earnings, spent much of their day on economic activities and having little time to prepare food of their own choice or preferences so they opt for ready-made food from food selling points. However, this study did not assess dietary quality and pattern, but Kagaruki et al., observed that, the quality of food sold by food selling points and street food vendors may serve as a facilitating or impeding environment for body weight gain or control [ 25 ]. Therefore, the study to assess the household dietary pattern among this community is recommended so as to establish the causal relationship.
The prevalence of hypertension (43.4%) in this study was comparable to that of Kagaruki et al. conducted in Dar es Salaam Tanzania, which reported the prevalence of 42.9% [ 25 ], but lower than other studies conducted in Tanzania [ 10 , 15 , 27 ]. The higher prevalence in this study may be explained by the rapid urbanization of Dodoma city which lead to changes of lifestyle such as eating habits to cope with the modern diets, this can also be reflected by the alarming rate of overweight and obesity in this population. Another possible explanation for the higher prevalence of hypertension in this study could be that, more than half (54.7%) of the study sample were the middle and older age population, female participants were more (57.9%) than men and the fact that females in this age group are past menopause this could have influenced the current prevalence. According to an epidemiological study, men have a higher prevalence of hypertension than women during their young adulthood, while women’s prevalence increases significantly after menopause [ 28 ].
This study documented low (43.1%) intake of fruit/vegetables at least 5 days per week among the study participants. This finding could probably due to insufficient income which may limit purchasing power and food choice in the family. On the other hand, the low intake of fruits and vegetables in this study could imply inadequate knowledge of study participants on the importance of fruits and vegetables in disease prevention and maintaining optimal health. Health program that will include the component of health education on healthy eating behavior will benefit this population. Studies have shown that, the consumption of recommended amount of fruits and vegetables reduces the incidence of type 2 diabetes, risk of cancer diseases and prevent weight gain [ 29 , 30 , 31 ]. The result of this study is similar to what has previously been reported in other part of the country [ 25 , 32 ]. The result also is similar to the study done in South Africa by the South African National Health and Nutrition Survey 2013 which reported low intake of fruits and vegetables among study participants [ 33 ]. The World Health Organization reported that, intake of at least five portions of fruits and vegetables per day reduces the risk of non-communicable diseases and ensures sufficiency daily intake of dietary fiber [ 34 ]. Therefore, the study to assess cardioprotective servings ≥ 5 of fruits and vegetables per day in this study population is recommended so as to understand their portions intake and advice accordingly. The prevalence of diabetes in this study was higher than that of Tanzania national average [ 35 ]. However, it is consistent with other studies of urban site in African populations [ 36 , 37 ]. This variations in the prevalence could be due to change in eating habits and sedentary lifestyle originating from increasing urbanization and economic development. Therefore, health promotion interventions at community level focusing on lifestyle modification could help to reduce the prevalence of the disease.
Only 35.4% of participants had adequate knowledge of modifiable CVDs risk factors while 2.9% of study participants were not able to identify any risk factor. Similarly, the study by Muhihi et al. [ 10 ]. found that 6.9% of study participants were unable to identify any risk factor. The low knowledge of CVDs risk factors in this study is comparable to other community-based studies conducted in African countries [ 38 , 39 ]. The finding of this study is contrary to the study in Dar es Salaam Tanzania which found that 79.7% of study participants had good knowledge of CVDs risk factors [ 15 ]. The variations of the study finding in these two studies could be explained by the difference in study settings and the method used to assess knowledge. The study in Dar es salaam was a hospital-based study involved caretakers of patients attending a tertiary cardiovascular center hospital while the current study was a community-based study involved participants from the household. This could imply that, caretakers of patient attending cardiovascular hospital center could have acquired the knowledge through health education provided to their patients during clinic visits. Studies have reported that, health care providers as sources of information on CVDs risk factors [ 10 , 40 ], unfortunately, individuals who receives knowledge of CVDs risk factors at the health facilities are the one who had already developed or have the risk factors. Therefore, interventions to focus on primary prevention at community level may increase population knowledge which could result in behavior change towards healthy lifestyle and consequently reduce the rising burden of CVDs in Tanzania. In the current situation whereby, the healthcare system in Tanzania is facing the burden of lack of human and financial resources for health, the suggested intervention could serve as a solution to reduce the burden of patients attending to the health facilities looking for CVDs services. Based on methods, the Dar es Salaam study tested risk factor knowledge across five domains (diet, epidemiology, risk factors, medical, and symptoms), whereas the current study assessed knowledge across three domains (diet, risk factors, and medical). This could have influenced the disparity in knowledge levels observed in these two researches. This study suggests doing another research in this cohort utilizing the five domains to evaluate whether there is a substantial change in knowledge level. This study found that the socio-demographic and biological characteristics of study participants were associated with knowledge of CVDs risk factors. The older and younger age participants were less knowledgeable on CVDs risk factors compared to the youth participants. Cognitive decline, which is frequent in the elderly, could explain this. Even after controlling for age and educational level, cognitive impairment can decrease performance across a wide range of cognitive domains, including attention, learning, and memory [ 41 ]. Prior research has indicated that decreases in executive function and memory might hamper clinical communication and compliance, as well as clinical decision-making [ 42 ], which could have contributed to the elderly’s lack of knowledge on CVDs risk factors in this study. People become more vulnerable to CVDs risk factors as they age [ 43 ], as does cognitive impairment [ 41 ]. CVDs are thought to hasten the deterioration of cognitive function, and impairment of cognitive domains raises the incidence of CVDs or worsens their prognosis [ 44 ]. This means that older people with less understanding of CVDs risk factors are more susceptible to CVDs, therefore, enhancing their CVDs knowledge may help to reduce their vulnerability to CVDs [ 45 ]. On the other hand, the young population < 45yrs mostly are still at low risk of CVDs hence less exposed to knowledge on the same compared to the middle aged adult who are at increased risk and some even already developed CVD conditions hence likely to be informed on the CVDs risks.
The finding of higher knowledge of CVDs risk factors among men is similar to the study done in Morogoro, Tanzania [ 10 ]. This is mostly due to men having more education and better wealth, which may lead to easier healthcare access where they can get information related to CVDs risk factors. Another possible explanation could be that men have ample time to listen, watch and read from mass media than women. One study found that more men than women stated that, radio, television and newspapers were their main sources of information on CVDs [ 10 ]. Contrary to this study, the study conducted in Kuwait found higher knowledge of CVDs risk factors among women than men [ 46 ]. This could be due to the high rate of CVDs literacy in their country. Therefore, community literacy on CVDs prevention is highly recommended. In this study the educated and the employed participants had adequate knowledge of CVDs risk factors. The findings of this study coincide to the findings of other studies conducted elsewhere [ 46 , 47 , 48 ]. This could imply that education and employment could help individuals to seek medical care and understand health information presented through various media. This study has found that participants who had ever checked their blood pressure and those who had a family history of hypertension had adequate knowledge on CVDs risk factors. This finding is congruent with other studies [ 10 , 15 ]. This could suggest that, susceptibility to a health problem may lead an individual to seek for health information which may result in acquiring knowledge of a specific risk behaviors.
One of the study’s strengths is that all of the data gathering methods have been validated, translated, pre-tested, and adjusted to fit the study context. The knowledge tool was designed to be easily administered with true/false questions, which is essential when using actual scales to measure knowledge rather than self-reports. This scale has the potential to be a significant tool for both scholars and other practitioners. The tool comprised items with a wide variety of difficulties, resulting in the tool having maximal discrimination power throughout the spectrum of knowledge levels. This was beneficial in identifying knowledge gaps among research participants so that appropriate authorities could adapt health information. The tool is more comprehensive in that it measures knowledge of risk factors and CVDs prevention and it can be used to the majority of adult populations.
One of the weaknesses of the knowledge tool employed in this study is that the authors did not collect all five areas of the original tool (diet, epidemiology, risk factors, medical, and CVD symptoms). The research proposes another study in the same community that will use all five domains to assess if there is a significant difference in knowledge level between the current study and the planned study. Another weakness of this study is that, it did not assess the individuals’ dietary quality and eating habits, physical activity and economic situation which could function as an enabler for the prevalence of CVDs risk factors. Furthermore, the study did not assess the source of knowledge on CVDs risk factors, which could help in identifying the major sources of information for the community and developing novel measures to strengthen them. Despite these limitations the study provides the baseline estimate of the prevalence and understanding of modifiable CVDs risk factors in a large adult population of Dodoma City, where intervention to lower CVDs risk factors possible.
In conclusion, the study has revealed high prevalence of biological (hypertension, diabetes, overweight and obesity) compared to behavioral (alcohol consumption, smoking and use of raw table salt) CVDs risk factors. The overall knowledge of modifiable CVDs risk factors in this study was inadequate. There seems to be a connection between inadequate knowledge of CVDs risk factors and high prevalence of modifiable CVDs risk factors in this study population. This may indicate lack of awareness and individual vulnerability to CVDs. Thus, innovative community educational strategies are desirable to create risk awareness in the community. These strategies will increase self-efficacy among individuals which may result to lifestyle modification and consequently reduce the risk of developing CVDs.
Data Availability
The datasets used and/or analysed during the current study are available from the corresponding author on reasonable request.
Abbreviations
Cardiovascular Diseases
Random Blood Glucose
Fasting Glucose Blood
Body Mass index
Adjusted Odds Ratio
Confidence Interval
Mendis S, Puska P, Norrving B, World Health Organization., World Heart Federation. World Stroke Organization. Global atlas on cardiovascular disease prevention and control. World Health Organization in collaboration with the World Heart Federation and the World Stroke Organization; 2011. p. 155.
Rani M, Nusrat S, Hawken LH. A qualitative study of governance of evolving response to non-communicable diseases in low-and middle- income countries: current status, risks and options. BMC Public Health. 2012;12(1).
WHO. World health statistics 2022: monitoring health for the SDGs, sustainable development goals. Geneva; 2022.
Mendis S, Davis S, Norrving B. Organizational update. Vol. 46, stroke. Lippincott Williams and Wilkins; 2015. p. e123.
Keates AK, Mocumbi AO, Ntsekhe M, Sliwa K, Stewart S. Cardiovascular disease in Africa: epidemiological profile and challenges. Nature Reviews Cardiology. Volume 14. Nature Publishing Group; 2017. pp. 273–93.
Roman WP, Martin HD, Sauli E. Cardiovascular diseases in Tanzania: the burden of modifiable and intermediate risk factors. Journal of Xiangya Medicine. Volume 4. AME Publishing Company; 2019.
Dickie K, Micklesfield LK, Chantler S, Lambert EV, Goedecke JH. Meeting physical activity guidelines is associated with reduced risk for cardiovascular disease in black south african women; a 5.5-year follow-up study. BMC Public Health. 2014;14(1).
World Health Organization. The SuRF report 2: surveillance of chronic disease risk factors: country-level data and comparable estimates. WHO; 2005. p. 91.
Organization WH. Global Health Risks: Mortality and Burden of Disease attributable to selected major risks. World Health Organization; 2009. p. 70.
Muhihi AJ, Anaeli A, Mpembeni RNM, Sunguya BF, Leyna G, Kakoko D et al. Public knowledge of risk factors and warning signs for cardiovascular disease among young and middle-aged adults in rural Tanzania. BMC Public Health. 2020;20(1).
Berkman ND, Sheridan SL, Donahue KE, Halpern DJ, Crotty K. Low Health Literacy and Health Outcomes: An Updated Systematic Review.
Joshi C, Jayasinghe UW, Parker S, Mar C, Del, Russell G, Lloyd J et al. Does health literacy affect patients’ receipt of preventative primary care? A multilevel analysis. BMC Fam Pract. 2014;15(1).
Lim S, Beauchamp A, Dodson S, O’Hara J, McPhee C, Fulton A, et al. Health literacy and fruit and vegetable intake in rural Australia. Public Health Nutr. 2017;20(15):2680–4.
Article PubMed PubMed Central Google Scholar
Boateng D, Wekesah F, Browne JL, Agyemang C, Agyei-Baffour P, De-Graft Aikins A et al. Knowledge and awareness of and perception towards cardiovascular disease risk in sub-saharan Africa: a systematic review. PLoS ONE. 2017;12(12).
Pallangyo P, Misidai N, Komba M, Mkojera Z, Swai HJ, Hemed NR, et al. Knowledge of cardiovascular risk factors among caretakers of outpatients attending a tertiary cardiovascular center in Tanzania: a cross-sectional survey. BMC Cardiovascular Disorders. Volume 20. BioMed Central Ltd; 2020.
Gibore NS, Munyogwa MJ, Ng’weshemi SK, Gesase AP. Effect of community-based lifestyle education intervention to reduce cardiovascular diseases risk factors among vulnerable population in Dodoma city, Tanzania: a cluster randomized controlled trial study protocol. Biol Methods Protoc. 2021;6(1).
Aguma JB, Economist PT, Officer SD, Project. Dodoma City Outer Ring Road (110. 2Km) Upgrading Project, Dodoma Region Countries: Rap Summary for the Proposed Upgrading of the Dodoma City Outer Ring Road (110. 2Km) To Bitumen Standard, Dodoma Region, Tanzania. 2018;1–13.
World Health Organization. WHO STEPS surveillance manual:STEPwise Approach to non communicable Disease risk factors surveillance. World Health Organization; 2020.
Bergman HE, Reeve BB, Moser RP, Scholl S, Klein WMP. Development of a comprehensive heart disease knowledge questionnaire. Am J Heal Educ. 2011;42(2):74–87.
Article Google Scholar
WHO Expert Committee on Physical Status. WHO the use and interpretation of anthropometry. Geneva, Switzerland; 1995.
Chobanian AV, Bakris GL, Black HR, Cushman WC, Green LA, Izzo JL et al. Seventh report of the Joint National Committee on Prevention, Detection, Evaluation, and Treatment of High Blood Pressure. Vol. 42, Hypertension. 2003. p. 1206–52.
Aspirateur DUL, Garantie L. Blood Glucose Complete Monitoring Systemm Système de surveillance de la Glycémie User’s Manuall Guide d’utilisation [Internet]. Owner ’ s Manual Guide d ’ utilisation. 2009 [cited 2020 May 25]. Available from: https://www.glucoplus.ca/images/glucoplusmanuel.pdf .
American Diabetes Association. Standards of Medical Care in Diabetes—2022 abridged for primary care providers. Diabetes Care. 2022;40(1):10–3.
Google Scholar
Ajayi IOO, Adebamowo C, Adami HO, Dalal S, Diamond MB, Bajunirwe F, et al. Urban-rural and geographic differences in overweight and obesity in four sub-saharan african adult populations: a multi-country cross-sectional study. BMC Public Health. 2016;16(1):1–13.
Kagaruki GB, Mahande MJ, Kimaro GD, Ngadaya ES, Mary Mayige T, Selemani M, et al. Prevalence and correlates of cardio-metabolic risk factors among regular street food consumers in dar es salaam, Tanzania. Diabetes. Metab Syndr Obes Targets Ther. 2021;14:1011–24.
Fahs I, Khalife Z, Malaeb D, Iskandarani M, Salameh P. The Prevalence and Awareness of Cardiovascular Diseases Risk Factors among the Lebanese Population: A Prospective Study Comparing Urban to Rural Populations. 2017.
Diarz EJ, Leyaro BJ, Kivuyo SL, Ngowi BJ, Msuya SE, Mfinanga SG et al. Red meat consumption and its association with hypertension and hyperlipidaemia among adult Maasai pastoralists of Ngorongoro Conservation Area, Tanzania. PLoS ONE. 2020;15(6).
Benjamin EJ, Muntner P, Alonso A, Bittencourt MS, Callaway CW, Carson AP, et al. Heart Disease and Stroke Statistics-2019 update: a Report from the American Heart Association. Circulation. 2019;139:56–28.
Miller V, Mente A, Dehghan M, Rangarajan S, Zhang X, Swaminathan S, et al. Fruit, vegetable, and legume intake, and cardiovascular disease and deaths in 18 countries (PURE): a prospective cohort study. Lancet. 2017;390(10107):2037–49.
Article PubMed Google Scholar
Boeing H, Bechthold A, Bub A, Ellinger S, Haller D, Kroke A, et al. Critical review: vegetables and fruit in the prevention of chronic diseases. Eur J Nutr. 2012;51:637–63.
Article CAS PubMed PubMed Central Google Scholar
Gonzalez CA, Lujan-Barroso L, Bueno-De-Mesquita HB, Jenab M, Duell EJ, Agudo A, et al. Fruit and vegetable intake and the risk of gastric adenocarcinoma: a reanalysis of the european prospective investigation into cancer and nutrition (EPIC-EURGAST) study after a longer follow-up. Int J Cancer. 2012;131(12):2910–9.
Article CAS PubMed Google Scholar
Zubery D, Kimiywe J, Martin HD. Prevalence of overweight and obesity, and its associated factors among health-care workers, teachers, and bankers in Arusha City, Tanzania. Diabetes. Metab Syndr Obes Targets Ther. 2021;14:455–65.
Shisana O, South A, Department of Health., Human Sciences Research Council., South African Medical Research Council. The south African National Health and Nutrition Examination Survey: SANHANES-1. HSRC Press; 2013. p. 401.
Agudo A. Measuring intake of fruit and vagetables: background paper for Joint FAO/WHO workshop on Fruit and Vegetable for Health. Kobe, Japan; 2004.
Mwangome M, Geubbels E, Klatser P, Dieleman M. Perceptions on diabetes care provision among health providers in rural Tanzania: a qualitative study. Health Policy Plan. 2017;32(3):418–29.
PubMed Google Scholar
Holmes MD, Dalal S, Sewram V, Diamond MB, Adebamowo SN, Ajayi IO, et al. Consumption of processed food dietary patterns in four african populations. Public Health Nutr. 2018;21(8):1529–37.
Seck SM, Dia DG, Doupa D, Diop-Dia A, Thiam I, Ndong M et al. Diabetes Burden in Urban and Rural Senegalese populations: a cross-sectional study in 2012. Int J Endocrinol. 2015;2015.
Kaddumukasa M, Kayima J, Kaddumukasa MN, Ddumba E, Mugenyi L, Pundik S et al. Knowledge, attitudes and perceptions of stroke: a cross-sectional survey in rural and urban Uganda. BMC Res Notes. 2015;8(1).
Donkor ES, Owolabi MO, Bampoh P, Aspelund T, Gudnason V. Community awareness of stroke in Accra, Ghana. BMC Public Health. 2014;14(1).
Awosan K, Taofeek Olalekan MTO, Ibrahim M. Awareness and prevalence of risk factors of coronary heart disease among teachers and bankers in Sokoto, Nigeria. 2013.
Eshkoor SA, Hamid TA, Mun CY, Ng CK. Mild cognitive impairment and its management in older people. Clin Interv Aging. 2015;10:687–93.
Greaves D, Psaltis PJ, Ross TJ, Davis D, Smith AE, Boord MS, et al. Cognitive outcomes following coronary artery bypass grafting: a systematic review and meta-analysis of 91,829 patients. Int J Cardiol. 2019;289:43–9.
Rodgers JL, Jones J, Bolleddu SI, Vanthenapalli S, Rodgers LE, Shah K, et al. Cardiovascular risks associated with gender and aging. Volume 6. Journal of Cardiovascular Development and Disease. MDPI; 2019.
Zuo W, Wu J. The interaction and pathogenesis between cognitive impairment and common cardiovascular diseases in the elderly. Ther Adv Chronic Dis. 2022;13:1–12.
Truong D, Xiaoming Liu R, Yu J, Jasper. Mixed methods research in tourism and hospitality journals. Int J Contemp Hosp Manag. 2020;32(4):1563–79.
Awad A, Al-Nafisi H. Public knowledge of cardiovascular disease and its risk factors in Kuwait: a cross-sectional survey. BMC Public Health. Volume 14. BioMed Central Ltd.; 2014.
Aminde LN, Takah N, Ngwasiri C, Noubiap JJ, Tindong M, Dzudie A et al. Population awareness of cardiovascular disease and its risk factors in Buea, Cameroon. BMC Public Health. 2017;17(1).
Wahab KW, Kayode OO, Musa OI. Knowledge of stroke risk factors among Nigerians at high risk. J Stroke Cerebrovasc Dis. 2015;24(1):125–9.
Download references
Acknowledgements
Authors would like to thank the study participants and research assistant for their contribution to the study, as well as current and past investigators and staff. Authors wish to thank The University of Dodoma for supporting the research team to execute this research project.
This work was supported by the University of Dodoma as a contextual plan for the award of Junior Academic Staff Research (JAS) Project of the University of Dodoma. The fund has no any role in any of the stages from study design to submission of the paper for publication.
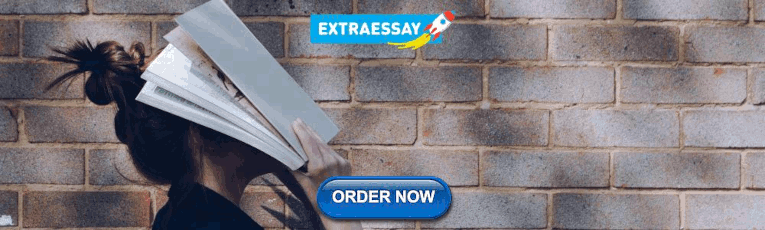
Author information
Authors and affiliations.
Department of Public Health and Community Nursing, The University of Dodoma, P.O. Box 395, Dodoma, Tanzania
Nyasiro Sophia Gibore
Department of Community Medicine, The University of Dodoma, P.O. Box 395, Dodoma, Tanzania
Mariam John Munyogwa & Secilia Kapalata Ng’weshemi
Department of Anatomy and Histology, The University of Dodoma, P.O. Box 395, Dodoma, Tanzania
Ainory Peter Gesase
You can also search for this author in PubMed Google Scholar
Contributions
N.S.G conceived the study. N.S.G, M.J.M and S.K.N designed the study and collected data. N.S.G analyzed, interpreted data, and prepared the first draft of the manuscript. A.P.G provided expert guidance to the design of the study and scientific review of the manuscript. All authors read and approved the final version of the manuscript for submission.
Corresponding author
Correspondence to Nyasiro Sophia Gibore .
Ethics declarations
Competing interests.
The authors declare that they have no competing interests.
Ethics approval and informed consent
The study was approved by the University of Dodoma, Institutional Research Review and Ethical committee (Ref. No. CB.299/308/2020) and it was conducted according to the Declaration of Helsinki. Permission to conduct research was sought from Dodoma Regional Commissioner, Dodoma City Council Director and local leaders of all wards involved in the study. The research subjects gave informed consent to this study after being briefed on the study. To ensure confidentiality and maintain anonymity, data collection was done individually in a special prepared room in their ward, codes instead of names were used to identifying participants.
Consent for publication
Not Applicable.
Additional information
Publisher’s note.
Springer Nature remains neutral with regard to jurisdictional claims in published maps and institutional affiliations.
Rights and permissions
Open Access This article is licensed under a Creative Commons Attribution 4.0 International License, which permits use, sharing, adaptation, distribution and reproduction in any medium or format, as long as you give appropriate credit to the original author(s) and the source, provide a link to the Creative Commons licence, and indicate if changes were made. The images or other third party material in this article are included in the article’s Creative Commons licence, unless indicated otherwise in a credit line to the material. If material is not included in the article’s Creative Commons licence and your intended use is not permitted by statutory regulation or exceeds the permitted use, you will need to obtain permission directly from the copyright holder. To view a copy of this licence, visit http://creativecommons.org/licenses/by/4.0/ . The Creative Commons Public Domain Dedication waiver ( http://creativecommons.org/publicdomain/zero/1.0/ ) applies to the data made available in this article, unless otherwise stated in a credit line to the data.
Reprints and permissions
About this article
Cite this article.
Gibore, N.S., Munyogwa, M.J., Ng’weshemi, S.K. et al. Prevalence and knowledge of modifiable cardiovascular diseases risk factors among vulnerable population in Central Tanzania. BMC Cardiovasc Disord 23 , 373 (2023). https://doi.org/10.1186/s12872-023-03408-3
Download citation
Received : 05 April 2023
Accepted : 19 July 2023
Published : 26 July 2023
DOI : https://doi.org/10.1186/s12872-023-03408-3
Share this article
Anyone you share the following link with will be able to read this content:
Sorry, a shareable link is not currently available for this article.
Provided by the Springer Nature SharedIt content-sharing initiative
- Cardiovascular diseases risk factors
- Modifiable risk factors
- Biological risk factors
- Behavioral risk factors
- Knowledge of risk factors
BMC Cardiovascular Disorders
ISSN: 1471-2261
- Submission enquiries: [email protected]
- General enquiries: [email protected]
- Introduction
- Conclusions
- Article Information
ICH indicates ideal cardiovascular health.
eTable 1. Ideal Cardiovascular Health Domains as Implemented in REGARDS, Including Criteria and Scoring
eTable 2. Association Among Race, Individual and Community Stressors, and Ideal Cardiovascular Health Overall
eTable 3. Association Among Race, Individual and Community Stressors, and Ideal Cardiovascular Health for Men
eTable 4. Association Among Race, Individual and Community Stressors, and Ideal Cardiovascular Health for Women
Data Sharing Statement
See More About
Sign up for emails based on your interests, select your interests.
Customize your JAMA Network experience by selecting one or more topics from the list below.
- Academic Medicine
- Acid Base, Electrolytes, Fluids
- Allergy and Clinical Immunology
- American Indian or Alaska Natives
- Anesthesiology
- Anticoagulation
- Art and Images in Psychiatry
- Artificial Intelligence
- Assisted Reproduction
- Bleeding and Transfusion
- Caring for the Critically Ill Patient
- Challenges in Clinical Electrocardiography
- Climate and Health
- Climate Change
- Clinical Challenge
- Clinical Decision Support
- Clinical Implications of Basic Neuroscience
- Clinical Pharmacy and Pharmacology
- Complementary and Alternative Medicine
- Consensus Statements
- Coronavirus (COVID-19)
- Critical Care Medicine
- Cultural Competency
- Dental Medicine
- Dermatology
- Diabetes and Endocrinology
- Diagnostic Test Interpretation
- Drug Development
- Electronic Health Records
- Emergency Medicine
- End of Life, Hospice, Palliative Care
- Environmental Health
- Equity, Diversity, and Inclusion
- Facial Plastic Surgery
- Gastroenterology and Hepatology
- Genetics and Genomics
- Genomics and Precision Health
- Global Health
- Guide to Statistics and Methods
- Hair Disorders
- Health Care Delivery Models
- Health Care Economics, Insurance, Payment
- Health Care Quality
- Health Care Reform
- Health Care Safety
- Health Care Workforce
- Health Disparities
- Health Inequities
- Health Policy
- Health Systems Science
- History of Medicine
- Hypertension
- Images in Neurology
- Implementation Science
- Infectious Diseases
- Innovations in Health Care Delivery
- JAMA Infographic
- Law and Medicine
- Leading Change
- Less is More
- LGBTQIA Medicine
- Lifestyle Behaviors
- Medical Coding
- Medical Devices and Equipment
- Medical Education
- Medical Education and Training
- Medical Journals and Publishing
- Mobile Health and Telemedicine
- Narrative Medicine
- Neuroscience and Psychiatry
- Notable Notes
- Nutrition, Obesity, Exercise
- Obstetrics and Gynecology
- Occupational Health
- Ophthalmology
- Orthopedics
- Otolaryngology
- Pain Medicine
- Palliative Care
- Pathology and Laboratory Medicine
- Patient Care
- Patient Information
- Performance Improvement
- Performance Measures
- Perioperative Care and Consultation
- Pharmacoeconomics
- Pharmacoepidemiology
- Pharmacogenetics
- Pharmacy and Clinical Pharmacology
- Physical Medicine and Rehabilitation
- Physical Therapy
- Physician Leadership
- Population Health
- Primary Care
- Professional Well-being
- Professionalism
- Psychiatry and Behavioral Health
- Public Health
- Pulmonary Medicine
- Regulatory Agencies
- Reproductive Health
- Research, Methods, Statistics
- Resuscitation
- Rheumatology
- Risk Management
- Scientific Discovery and the Future of Medicine
- Shared Decision Making and Communication
- Sleep Medicine
- Sports Medicine
- Stem Cell Transplantation
- Substance Use and Addiction Medicine
- Surgical Innovation
- Surgical Pearls
- Teachable Moment
- Technology and Finance
- The Art of JAMA
- The Arts and Medicine
- The Rational Clinical Examination
- Tobacco and e-Cigarettes
- Translational Medicine
- Trauma and Injury
- Treatment Adherence
- Ultrasonography
- Users' Guide to the Medical Literature
- Vaccination
- Venous Thromboembolism
- Veterans Health
- Women's Health
- Workflow and Process
- Wound Care, Infection, Healing
Get the latest research based on your areas of interest.
Others also liked.
- Download PDF
- X Facebook More LinkedIn
Hines AL , Albert MA , Blair JP, et al. Neighborhood Factors, Individual Stressors, and Cardiovascular Health Among Black and White Adults in the US : The Reasons for Geographic and Racial Differences in Stroke (REGARDS) Study . JAMA Netw Open. 2023;6(9):e2336207. doi:10.1001/jamanetworkopen.2023.36207
Manage citations:
© 2024
- Permissions
Neighborhood Factors, Individual Stressors, and Cardiovascular Health Among Black and White Adults in the US : The Reasons for Geographic and Racial Differences in Stroke (REGARDS) Study
- 1 Department of Health Behavior and Policy, Virginia Commonwealth University School of Medicine, Richmond, Virginia
- 2 Department of Medicine, University of California, San Francisco, San Francisco
- 3 Department of Biostatistics, University of Alabama at Birmingham, Birmingham
- 4 Department of Medicine, Johns Hopkins School of Medicine, Baltimore, Maryland
- 5 Department of Medicine, University of Mississippi Medical Center, Jackson
Question Do neighborhood factors (physical and social environments) and individual stressors (discrimination) mediate racial differences in cardiovascular health?
Findings In this cross-sectional study of 7720 participants, Black adults had poorer cardiovascular health than White adults. Neighborhood physical environment and safety attenuated racial differences in total ICH scores by 5% and 6%, respectively, while discrimination attenuated these differences by 11%.
Meaning Findings of this study suggest that racial differences in cardiovascular health are attenuated by varying experiences with neighborhood physical and social environments as well as by lived personal experiences with discrimination; approaches to improve ICH that target neighborhood factors and discrimination by gender and race are warranted.
Importance Chronic stress has been posited to contribute to racial disparities in cardiovascular health. Investigation of whether neighborhood- and individual-level stressors mediate this disparity is needed.
Objective To examine whether racial differences in ideal cardiovascular health (ICH) are attenuated by experiences with neighborhood- and individual-level stressors within a racially and geographically diverse population sample.
Design, Setting, and Participants This cross-sectional study examined data from 7720 participants in the Reasons for Geographic and Racial Differences in Stroke (REGARDS) Study who completed the second in-home visit (2013-2016). The REGARDS study is a population-based, longitudinal study of 30 239 non-Hispanic Black and non-Hispanic White adults aged 45 years or older at baseline (2003-2007). Data for the present study were analyzed from June to July 2021 and in March 2022.
Exposures Neighborhood physical environment (eg, excessive noise, violence; scored from 7-28, with higher scores indicating more problems), neighborhood safety (scored as very safe, safe, or not safe), neighborhood social cohesion (eg, shared values; scored from 5-25, with higher scores indicating higher cohesion), perceived stress (eg, coping; scored from 0-16, with higher scores indicating greater perceived stress), and the experience of discrimination (yes or no).
Main Outcomes and Measures Ideal cardiovascular health (ICH), measured as a composite of 4 health behaviors (cigarette smoking, diet, physical activity, body mass index) and 3 health factors (blood pressure, cholesterol, and glucose levels).
Results The sample included 7720 participants (mean [SD] age, 71.9 [8.3] years; 4390 women [56.9%]; 2074 Black participants [26.9%]; and 5646 White participants [73.1%]). Black participants compared with White participants reported higher perceived stress (mean [SD] score, 3.2 [2.8] vs 2.8 [2.7]) and more often reported discrimination (77.0% vs 24.0%). Black participants also reported poorer neighborhood physical environment (mean [SD] score, 11.2 [3.8] vs 9.8 [2.9]) and social cohesion (mean [SD] score, 15.5 [2.0] vs 15.7 [1.9]) and more often reported their neighborhoods were unsafe (54.7% vs 24.3%). The odds of having a high total ICH score (ie, closer to ideal) were lower for Black adults compared with White adults, both overall (adjusted odds ratio [AOR], 0.53; 95% CI, 0.45-0.61) and by gender (men: AOR, 0.73 [95% CI, 0.57-0.93]; women: AOR, 0.45 [95% CI, 0.37-0.54]). In mediation analyses, the racial disparity in total ICH score was attenuated by neighborhood physical environment (5.14%), neighborhood safety (6.27%), neighborhood social cohesion (1.41%), and discrimination (11.01%). In stratified analyses, the factors that most attenuated the racial disparity in total ICH scores were neighborhood safety among men (12.32%) and discrimination among women (14.37%). Perceived stress did not attenuate the racial disparity in total ICH scores.
Conclusions and Relevance In this cross-sectional study of Black and White US adults aged 45 years and older, neighborhood-level factors, including safety and physical and social environments, and individual-level factors, including discrimination, attenuated racial disparities in cardiovascular health. Interventional approaches to improve ICH that separately target neighborhood context and discrimination by gender and race are warranted.
In the US, Black adults have earlier onset of cardiovascular risk factors and poorer overall cardiovascular health compared with White adults. 1 , 2 Psychosocial stress and stress-related coping are associated with increased cardiovascular disease (CVD) risk and may contribute to these health disparities. 3 The weathering framework (the idea that persistent exposure to socioeconomic disadvantage, marginalization, and discrimination is associated with premature health deterioration) 4 , 5 and allostatic load (dysregulated or heightened endocrine, inflammatory, and autonomic regulatory system responses resulting from chronic stress) 4 describe how lifetime cumulative psychosocial stress (hereafter, stress) related to structural and interpersonal racism converge with health care factors to render Black Americans vulnerable to psychological and physiological responses, leading to premature, stress-related illness and mortality. Across the life span, Black people, and Black women in particular, have higher levels of allostatic load related to differential lived social experiences compared with White and male counterparts. 5
The health equity framework for social determinants of health posits that the sociopolitical and economic context shapes social position through neighborhood environment, social and community context, and lived personal experiences of discrimination, which, ultimately, contribute to cardiovascular health inequities. 6 For example, racial residential segregation—a manifestation of structural racism in the sociopolitical and economic context—has been associated with incident hypertension 7 and CVD. 8 In the Multi-Ethnic Study of Atherosclerosis, 9 racial residential segregation was associated with cardiometabolic risk among Black participants but not among non-Hispanic White and Hispanic persons after adjusting for income. This association suggests that both individual and contextual economics are associated with CVD among Black people. 10 A critical feature of racial residential segregation is resource disinvestment that results in neighborhood disadvantage, 11 including concerns about crime, 12 and fewer physical resources in the built environment, such as supermarkets 13 , 14 ; these factors have unfavorable implications for stress and cardiovascular risk. Aspects of the neighborhood social environment, such as social cohesion or a sense of connectedness, have also been strongly associated with inflammatory stress biomarkers related to cardiovascular risk among non-Hispanic Black residents. 15 Other contributing factors include perceptions of neighborhood safety that draw on both built and social attributes to influence health outcomes. 16 The association between individuals’ self-reported experiences with racial discrimination and cardiovascular outcomes has been reported in the literature. 17 , 18 In addition, perceived stress and discrimination have been associated with health behaviors, such as lower medication adherence, among Black patients with hypertension. 19 , 20
Few studies have characterized the implications of multiple levels of simultaneous stressors for racial differences in cardiovascular health. A large cohort study of US Black adults in the South found that those experiencing higher levels of multiple stress measures were less likely to achieve ideal cardiovascular health (ICH). 21 Ideal cardiovascular health is a composite of 4 health behaviors (cigarette smoking, diet, physical activity, body mass index) and 3 health factors (blood pressure, cholesterol and glucose levels). One study examining cumulative psychosocial stress and ICH among older female health professionals found worse ICH outcomes among Black women compared with White women. 22 Those authors suggested that future studies should include more diverse populations, including men, participants other than health care workers, and younger participants, in addition to alternative measures of stress, including physical and social neighborhood environments. 22 In an analysis of data from 3 cohort studies, low neighborhood social cohesion, which may elicit a chronic stress response, was also not associated with cardiovascular health. 23 Investigating chronic stressors at both the neighborhood and individual levels may help identify which stressors are most salient for cardiovascular health and thus aid in the development of targeted interventions.
The purpose of this study was to assess whether neighborhood factors (physical environment, safety, and social cohesion) and individual stressors (perceived stress and discrimination) attenuate racial differences in ICH. We hypothesized that neighborhood factors and individual stressors would attenuate racial differences in ICH and that the observed associations would vary by gender given the well-documented disproportionate levels of stress experienced by women relative to men. 5 , 24
We analyzed cross-sectional data from the Reasons for Geographic and Racial Differences in Stroke (REGARDS) Study, a population-based, longitudinal study of 30 239 Black and White adults aged 45 years or older at baseline from 2003 to 2007. 25 The present study used data from the REGARDS second in-home visit (2013-2016; n = 16 150 participants), which included a psychosocial questionnaire. Individuals missing data on ICH score components (n = 6603), neighborhood factors (physical environment, safety, and social cohesion; n = 1037), or individual stressors (perceived stress and discrimination; n = 790) were excluded, resulting in a final sample size of 7720 participants ( Figure ). Distributions of key demographics and neighborhood- and individual-level stressors were similar between persons included and excluded from the current study except for experiences with discrimination, which were reported more often by excluded individuals (91.6% of Black individuals and 74.4% of White individuals). This secondary data analysis was approved by the Virginia Commonwealth University Institutional Review Board. The REGARDS Study obtained written informed consent from all study participants and was approved by the University of Alabama at Birmingham Institutional Review Board. This study followed the Strengthening the Reporting of Observational Studies in Epidemiology ( STROBE ) reporting guideline.
Ideal cardiovascular health was calculated as a composite measure of 7 components: 4 health behaviors (cigarette smoking, diet, physical activity, body mass index) and 3 health factors (blood pressure, cholesterol and glucose levels). 26 Each component was categorized 27 , 28 and given a score of 2 for ideal, 1 for intermediate, and 0 for poor (eTable 1 in Supplement 1 ). Component scores were summed (range, 0-14) and used to categorize overall total ICH as poor (0-4), intermediate (5-9), or ideal (≥10).
Race is a social construct. In this study, the race variable represents an indicator of shared lived experiences within sociopolitical and economic contexts in the US. Participants self-reported their race and ethnicity. Individuals who identified as non-Hispanic Black or non-Hispanic White were eligible to participate in the REGARDS study. 25
The neighborhood physical environment provides the broader structural context for individual supports (or lack thereof) that affect health, including daily stress. 12 , 29 Participants rated potential problems in their neighborhood (excessive noise, heavy or speeding traffic, inadequate food shopping, lack of parks or playgrounds, trash or litter, no or poorly maintained sidewalks, violence) from 1 (not really a problem) to 4 (very serious problem) 12 (Cronbach α = 0.73-0.83). 30 Items were summed for a total score (range, 7-28), with a higher score indicating more problems. Participants rated their neighborhood’s safety separately on a scale of 1 (very safe) to 5 (not safe at all). Due to the small sample, these categories were collapsed to 3 levels for analytical purposes (1, very safe; 2, safe; and ≥3, unsafe).
The neighborhood social environment (ie, social cohesion) has been noted as a potential stress buffer and describes support provided through social interactions 31 and other organizational assets. Participants rated the following statements on a scale of 1 (strongly agree) to 5 (strongly disagree): (1) people around here are willing to help their neighbors; (2) this is a close-knit neighborhood; (3) people in this neighborhood can be trusted; (4) people in this neighborhood generally do not get along; and (5) people in this neighborhood do not share the same values (Cronbach α = 0.80). 32 Items 4 and 5 were reverse-coded; the total score was summed (range, 5-25), with higher scores indicating higher social cohesion.
Participants rated their perceived stress via the 4-item Perceived Stress Scale (0, never to 4, very often): (1) feelings of controlling important things in life; (2) feeling confident about their ability to handle personal problems; (3) not coping; and (4) feelings of encountering difficulties that they could not overcome within the past month (Cronbach α = 0.74). 33 Items 3 and 4 were reverse-coded; the total score was summed (range, 0-16), with higher scores indicating higher perceived stress.
Participants rated lifetime discrimination “due to their race, ethnicity, or color” across settings (eg, at school, getting medical care, from the police) via the Experiences of Discrimination scale, which ranges from 0 (have not experienced discrimination) to 3 (have experienced discrimination ≥4 times; Cronbach α = 0.77 to 0.81). 34 In our analysis, we created a binary variable (did not experience discrimination [score of 0] vs experienced discrimination [score ≥1]) based on the frequency distribution of responses.
Demographic data included in the analysis were gender (man, woman), age (in years), highest educational attainment, annual household income, and marital status (married, divorced, widowed, separated, never married). We estimated the means for continuous variables and percentages for categorical variables and compared across racial groups using t tests and Cochran-Mantel-Haenszel χ 2 tests, respectively. Due to the natural ordering of the poor, intermediate, and ideal ICH categories, we used multivariable ordinal logistic regression models to evaluate racial differences in ICH, adjusting for stressors and demographics overall and stratified by gender. 35 Probabilities modeled were cumulated over the lower ordered values, ie, poor ICH compared with intermediate and ideal ICH. A higher odds ratio reflected a better total ICH score, or closer to ideal, compared with the poor ICH reference group.
To test our hypothesis that the racial difference in ICH was explained by neighborhood factors and individual stressors, we performed a mediation analysis with bootstrapping for each characteristic of interest: neighborhood physical environment, neighborhood safety, neighborhood social cohesion, perceived stress, and discrimination. Using the difference method approach to quantify attenuation, we estimated the association of race (acknowledging that race is a proxy for lived experiences of structural racism) with total ICH scores using estimated coefficient of race in models without and with each neighborhood factor and individual stressor separately. 36 , 37 The extent to which each variable attenuated the racial differences in ICH was calculated as the ratio of the change in association compared with a reference model after adjustment for sociodemographics. Bootstrapping with a random seed and 1000 replications was used to estimate 95% CIs. Similar analyses were stratified by gender, with the extent to which each variable attenuated the racial differences in ICH calculated separately. 38 We also estimated a model including all characteristics of interest compared with the reference model. P < .05 was considered to be statistically significant. Data were analyzed from June to July 2021 and in March 2022 using SAS, version 9.4 (SAS Institute, Inc).
This study included data from 7720 participants from the REGARDS study (mean [SD] age, 71.9 [8.3] years; 4390 women [56.9%] and 3330 men [43.1%]; 2074 Black participants [26.9%] and 5646 White participants [73.1%]) ( Table 1 ). Compared with White participants, Black participants were younger and more often had a lower level of educational attainment, an annual household income less than $34 000, and were unmarried. Black participants also reported higher levels of perceived stress and discrimination and poorer neighborhood physical, social, and safety characteristics.
Table 2 displays total and component ICH scores and categorizations (ideal, intermediate, and poor) by race and gender. Overall, Black individuals had lower mean (SD) total ICH scores than White individuals (6.7 [2.0] vs 7.7 [2.1]; P < .001). Compared with Black women, Black men had better mean (SD) total ICH scores (7.1 [2.0] vs 6.6 [1.9]; P < .001) and a higher proportion of individuals with ICH scores that were considered ideal (ie, a score of 2) (11.7% vs 7.0%; P < .001). White men and women had similar mean (SD) total ICH scores (7.7 [2.0] vs 7.7 [2.1]) as well as proportions of individuals with ideal ICH components (19.1% vs 21.3%), but they varied with respect to score components. For example, more White men achieved ideal physical activity than White women (32.4% vs 23.5%), but more White women achieved an ideal body mass index than White men (34.9% and 26.5%). Compared with Black men, White men had better mean (SD) total ICH scores (7.7 [2.1] vs 7.1 [2.0]) and a higher proportion with ideal ICH components (19.1% vs 11.7%) compared with Black men. Physical activity and cholesterol scores were similar between Black and White men (proportions with ideal ICH components: physical activity, 25.7% and 23.5%; cholesterol, 41.2% and 39.3%). White women had better ICH over all comparisons compared with Black women. For example, mean [SD] total ICH scores were 7.7 [2.1] for White women and 6.6 [1.9] in Black women.
Table 3 shows the associations between race, neighborhood factors, and individual stressors and ICH in a fully adjusted model. Black participants had lower odds of having a high total ICH score compared with their White counterparts (adjusted odds ratio [AOR], 0.53; 95% CI, 0.45-0.61). Higher neighborhood social cohesion (AOR, 1.05; 95% CI, 1.03-1.08) was associated with higher odds of having a high total ICH score. In contrast, higher perceived stress was associated with 4% lower odds (AOR, 0.96; 95% CI, 0.94-0.98) of having a high total ICH score in the fully adjusted model.
In analyses stratified by gender ( Table 3 ), Black men had 27% lower odds of having a high total ICH score (AOR, 0.73; 95% CI, 0.57-0.93) compared with White men in the fully adjusted model. Among women, Black women had 55% lower odds of having a higher total ICH score (AOR, 0.45; 95% CI, 0.37-0.54) compared with White women in the fully adjusted model. Higher educational level was associated with ICH. For example, compared with having less than a high school education, having a college education was associated with higher total ICH score for men (AOR, 2.88; 95% CI, 1.90-4.38) and women (AOR 2.32; 95% CI, 1.69-3.18). Compared with being single, being married (AOR, 1.56; 95% CI, 1.13-2.16) or divorced (AOR, 1.78; 95% CI, 1.27-2.49) was associated with higher odds of having a higher total ICH score in women. Social cohesion was associated with higher odds of having a higher total ICH score (AOR, 1.07; 95% CI, 1.03-1.11) among women. Perceived stress (AOR, 0.95; 95% CI, 0.92-0.97) and discrimination (AOR, 0.80; 95% CI, 0.68-0.95) were associated with lower odds of having a higher total ICH score among women. Stepwise models adding characteristics individually are provided in eTables 2 to 4 in Supplement 1 .
The neighborhood- and individual-level factors that attenuated the racial differences in ICH scores among Black and White participants are presented in Table 4 . In the reference model that was adjusted for sociodemographic variables, Black participants had lower total ICH scores overall (β = −0.70; 95% CI, −0.81 to −0.59), among men (β = −0.36; 95% CI, −0.53 to −0.18), and among women (β = −0.88; 95% CI, −1.01 to −0.74) compared with White participants. The overall difference in total ICH scores between Black and White participants was attenuated by neighborhood physical environment (5.14%; 95% CI, 3.27%-7.15%), neighborhood safety (6.27%; 95% CI, 2.46%-10.05%), social cohesion (1.41%; 95% CI, 0.48%-2.60%), and discrimination (11.01%; 95% CI, 3.22%-19.15%). Among men, the racial difference in total ICH scores was attenuated by neighborhood physical environment (5.59%; 95% CI, 1.51%-10.23%), neighborhood safety (12.32%; 95% CI, 2.08%-22.42%), and neighborhood social cohesion (4.95%; 95% CI, 1.24%-9.26%). Among women, the racial difference in total ICH scores was attenuated by neighborhood physical environment (4.81%; 95% CI, 2.27%-7.32%), neighborhood safety (4.38%; 95%, 0.33%-8.98%), and discrimination (14.37%; 95% CI, 6.50%-22.23%). In the model including all characteristics of interest, the racial difference in total ICH scores was attenuated overall (12.27%; 95% CI, 3.58%-20.86%) and among women (13.68%; 95% CI, 4.51%-23.01%) but not among men (9.15; 95% CI, −17.60% to 36.67%).
This study sought to characterize the role of neighborhood-level factors and individual-level stressors in racial differences in ICH among Black and White individuals. We found that Black participants had a higher prevalence of stressors, such as stress-related neighborhood physical environment, neighborhood safety, perceived stress, and discrimination, and lower levels of neighborhood social cohesion. 32 Consistent with our hypothesis grounded in the social determinants of health framework 6 —that disproportionate exposures to stressors underlie racial differences in cardiovascular health—neighborhood physical environment, safety, and social cohesion and individual experiences with racial discrimination (and not perceived stress, which is a noncontextual measure of general stress) partially mediated the association between race and ICH, together attenuating approximately 12% of the observed racial difference in the fully adjusted model. Moreover, the proportions of the racial differences mediated by specific stressors, such as neighborhood safety and discrimination, ranged from approximately 1% to 14% and varied by gender.
Previous studies have indicated that women have higher rates of ICH than men 39 - 41 ; however, this study offers further nuance suggesting gender differences by race. Within race groups, we found no difference in ICH between White men and White women, while Black men had better ICH compared with Black women. Further investigation is warranted to understand these unexpected gender associations. Racial differences in ICH persisted in within-gender comparisons. Whereas Black men had 27% lower odds of having a higher total ICH score than White men, Black women had 55% lower odds than White women—more than double the racial gap among men. A previous study found a similar racial disparity among female health professionals after adjusting for cumulative stress. 22 The current analysis also offers new insight regarding ICH disparities among men, who typically have a higher incidence of cardiovascular disease but have better anticipated outcomes than women after acute cardiovascular events. 42
Demographic correlates of ICH also differed by gender, illuminating psychosocial implications. Education level was associated with ICH in a seemingly dose-dependent association, such that the odds of having a higher total ICH score increased with each ordinal level of educational attainment. Educational attainment is strongly associated with health outcomes among White individuals. 43 Education may be a more salient factor in ICH among Black people and women, 44 who reap diminishing returns—earning less than White men at every education level 45 —and subsequently, experience poorer health care, 46 subjective health, 47 and cardiovascular outcomes. 48 Black women, specifically, earn less than men and White women, 49 which may be reflected in the ICH outcomes in this study. We found that higher income was related to ICH among women but not men in this study. In another example, marital status, frequently operationalized as a proxy of social support, was not associated with ICH among men despite previous evidence of marriage’s protection against poor health 50 and cardiovascular outcomes 51 in this group. On the other hand, our finding that being married or divorced was associated with higher total ICH scores among women is consistent with previous work suggesting some economic benefits (especially among White women) and, consequently, health benefits for women related to marriage vs being single 52 (even if the marriage has dissolved). In this way, social support may be operationalized differently within the context of healthy cardiovascular aging among women and men. It should be further noted that benefits of marriage may not be conferred uniformly across racial groups, in that Black women are less likely to marry at all, marry later in life, and have higher rates of marital instability compared with White and Hispanic women. 53
Among men, the racial difference in ICH was partially explained by neighborhood physical environment (an inventory of problematic disorder, resources, and violence), safety, and social cohesion (sentiments of community trust and shared values). Neighborhood disorder has been associated with crime and violence, which may collectively influence mental health outcomes. 12 , 29 A growing body of literature indicates that exposure to violence (reflected in our neighborhood measure) may be associated with hypertension and other cardiovascular outcomes. 54 Over the life span, men represent a greater proportion of perpetrators and victims of violent crime compared with women, although gender differences in violence appear to be decreasing over time 55 and are reduced at higher levels of disadvantage. 56 Despite these slowly shifting trends, community violence presents a more relevant threat and, thus, a more prominent stressor among men relative to women. Moreover, neighborhood disadvantage increases the exposure to peer violence for both men and women. 57 For Black individuals, whose communities are policed more often, 58 threats of fatal police encounters, which occur at rates nearly 3 to 5 times that of their White counterparts, 59 , 60 serve as an additional type of potential violent stressor. Indeed, studies examining the outcomes of the killing of unarmed Black people related to neighborhood policing have found adverse mental health implications for Black communities. 61 - 63 Additionally, circumstances of heightened police presence may spark vigilant anticipatory coping (a strategy used to prepare for possible mistreatment related to race 64 ), which is associated with increased hypertension and obesity risk among Black individuals 65 , 66 and large arterial elasticity among boys. 67 Vigilant coping against racial discrimination may help to buffer against depression 68 but exacerbate hypertension risk 69 among Black individuals. In this study, discrimination was not associated with racial differences in ICH among men. Indeed, it is possible that the disproportionate threat of physical harm to Black men could be perceived as a form of discrimination, thereby subsuming the discrimination construct as measured. More research is needed to understand the implications of violence and neighborhood policing as a neighborhood stressor for cardiovascular outcomes. Additionally, neighborhood disadvantage is associated with diminished access to healthy food options and inability to safely perform physical activity. 12 - 14 Of note, social cohesion helped to explain racial differences in ICH among men only. This finding suggests that neighborhood social conditions constituting a more communal level of social support rather than solely that of the familial level may help to counter some of the stress faced by Black men, with implications for improved ICH.
Discrimination explained 14.37% of the racial disparity in ICH among women only. Experiences with discrimination have been associated with increased cardiovascular risk factors, such as obesity and hypertension. 70 , 71 Discrimination may be particularly detrimental to the health outcomes of Black women due to social disadvantage in both race and gender domains. 72 Experiencing racial discrimination may also reduce the effectiveness of interventions to improve cardiometabolic health within this group. 73 Black women have higher levels of chronic stress over the life span as measured by allostatic load, which is likely related to the disadvantages of this dual identity. 5 , 24 One category of unique discrimination experiences by Black women—gendered racial microaggressions 74 —has been associated with poorer mental health and self-reported health. Additional studies are required to understand the role of these unique discrimination experiences in cardiovascular health. Moreover, it is unclear how common coping mechanisms, such as the superwoman schema (a frame describing the associations between stress and health among African American women), 75 impact cardiovascular outcomes, although possible pathways have been described. 76 Taken together, understanding racial and gender differences in ICH should be considered intersectionally through the “interconnected nature of social categorizations, such as race, class, and gender,” which may “create overlapping and interdependent systems of discrimination or disadvantage.” 77
We believe that this study offers new insight regarding the role of varying stressors on racial and gender disparities in ICH. A study strength is the large, population-based cohort of Black and White individuals with rigorously assessed physiological measures and validated measures of neighborhood attributes and individual stressors, including discrimination.
Nonetheless, the findings of this study should be interpreted within the context of the following limitations. First, this study is cross-sectional and thus causation cannot be determined. Second, because a higher proportion of individuals excluded from the analysis experienced discrimination regardless of race, the association between discrimination and ICH warrants further investigation. Third, we discuss stressors in terms of neighborhood factors and individual stressors (ie, lived personal experience) to convey interpretable opportunities for intervention through clinical and population health strategies with the understanding that these constructs are interrelated. Finally, the ICH construct does not capture health in totality. Despite these limitations, evaluating the differential implications of stressors for cardiovascular health by race is important given that CVD is the greatest contributor to racial disparities in premature mortality in the US. 78
In this cross-sectional study that used a population-based sample of middle-aged and older adults, Black adults had poorer ICH than their White counterparts, with larger racial differences noted among women than men. Neighborhood factors helped to attenuate racial disparities in ICH, especially among men, while discrimination attenuated differences among women only. Further work is needed to explore these associations in other diverse populations, particularly differential associations between discrimination and ICH by gender. From a public health standpoint, these data suggest that interventional approaches that separately target neighborhood factors and discrimination by gender and race are warranted. For example, addressing unique stressors that tie into the superwoman schema could be more consequential in Black women, whereas addressing the impact of neighborhood violence may be differentially more important for Black men.
Accepted for Publication: August 23, 2023.
Published: September 29, 2023. doi:10.1001/jamanetworkopen.2023.36207
Open Access: This is an open access article distributed under the terms of the CC-BY License . © 2023 Hines AL et al. JAMA Network Open .
Corresponding Author: Anika L. Hines, PhD, MPH, Virginia Commonwealth University School of Medicine, 830 E Main St, Room 4-116, PO Box 980430, Richmond, VA 23298 ( [email protected] ).
Author Contributions: Ms Blair had full access to all of the data in the study and takes responsibility for the integrity of the data and the accuracy of the data analysis.
Concept and design: Hines, Albert.
Acquisition, analysis, or interpretation of data: Hines, Blair, Crews, Cooper, Long, Carson.
Drafting of the manuscript: Hines, Albert, Long.
Critical review of the manuscript for important intellectual content: All authors.
Statistical analysis: Hines, Blair, Long.
Administrative, technical, or material support: Hines, Long.
Supervision: Hines, Albert.
Conflict of Interest Disclosures: Dr Hines reported receiving grants from the National Heart, Lung, and Blood Institute and the American Heart Association during the conduct of the study. Dr Crews reported receiving grants from Baxter International, Inc, and Somatus, Inc and consulting fees from Bayer Advisory Group outside the submitted work. Dr Cooper reported serving on the Pfizer, Inc, Clinical Trials Diversity Advisory Board; receiving grants from Johnson & Johnson, Merck & Co, Inc; and receiving personal fees from Baxter International, Inc, outside the submitted work. Dr Long reported receiving grants from the National Institute of Neurological Disorders and Stroke (NINDS) and the National Institute on Aging (NIA) during the conduct of the study; and grants from Amgen, Inc, outside the submitted work. Dr Carson reported receiving grants from the National Institutes of Health (NIH) and the Centers for Disease Control and Prevention during the conduct of the study and grants from Amgen, Inc, outside the submitted work. No other disclosures were reported.
Funding/Support: This research was supported by cooperative agreement U01 NS041588 cofunded by NINDS and the NIA, NIH.
Role of the Funder/Sponsor: Representatives of the NINDS were involved in the review of the manuscript but were not directly involved in the collection, management, analysis, or interpretation of the data. Otherwise, the funders had no role in the design and conduct of the study; collection, management, analysis, and interpretation of the data; preparation, review, or approval of the manuscript; and decision to submit the manuscript for publication.
Disclaimer: The content is solely the responsibility of the authors and does not necessarily represent the official views of the NINDS or the NIA.
Data Sharing Statement: See Supplement 2 .
Additional Contributions: The authors thank the investigators, staff, and participants of the Reasons for Geographic and Racial Differences in Stroke (REGARDS) study for their valuable contributions.
Additional Information: A full list of participating REGARDS investigators and institutions can be found at: https://www.uab.edu/soph/regardsstudy/ .
- Register for email alerts with links to free full-text articles
- Access PDFs of free articles
- Manage your interests
- Save searches and receive search alerts
- Search Menu
- Advance Articles
- Editor's Choice
- Braunwald's Corner
- ESC Guidelines
- EHJ Dialogues
- Issue @ a Glance Podcasts
- CardioPulse
- Weekly Journal Scan
- European Heart Journal Supplements
- Year in Cardiovascular Medicine
- Asia in EHJ
- Most Cited Articles
- ESC Content Collections
- Author Guidelines
- Submission Site
- Why publish with EHJ?
- Open Access Options
- Submit from medRxiv or bioRxiv
- Author Resources
- Self-Archiving Policy
- Read & Publish
- Advertising and Corporate Services
- Advertising
- Reprints and ePrints
- Sponsored Supplements
- Journals Career Network
- About European Heart Journal
- Editorial Board
- About the European Society of Cardiology
- ESC Publications
- War in Ukraine
- ESC Membership
- ESC Journals App
- Developing Countries Initiative
- Dispatch Dates
- Terms and Conditions
- Journals on Oxford Academic
- Books on Oxford Academic

Article Contents
Introduction, conclusions, acknowledgements.
- < Previous
Hot topics and trends in cardiovascular research

- Article contents
- Figures & tables
- Supplementary Data
Diane Gal, Bart Thijs, Wolfgang Glänzel, Karin R Sipido, Hot topics and trends in cardiovascular research, European Heart Journal , Volume 40, Issue 28, 21 July 2019, Pages 2363–2374, https://doi.org/10.1093/eurheartj/ehz282
- Permissions Icon Permissions
Comprehensive data on research undertaken in cardiovascular medicine can inform the scientific community and can support policy building. We used the publication output from 2004 to 2013 and the 2014 references to these documents, to identify research topics and trends in the field of cardiovascular disease.
Text fragments were extracted from the titles and abstracts of 478 000 publications using natural language processing. Through machine-learning algorithms, these text fragments combined to identify specific topics across all publications. A second method, which included cross-references, assigned each publication document to a specific cluster. Experts named the topics and document clusters based on various outputs from these semi-automatic methods. We identified and labelled 175 cardiovascular topics and 20 large document clusters, with concordance between the approaches. Overarching, strongly growing topics in clinical and population sciences are evidence-based guidance for treatment, research on outcomes, prognosis, and risk factors. ‘Hot’ topics include novel treatments in valve disease and in coronary artery disease, and imaging. Basic research decreases its share over time but sees substantial growth of research on stem cells and tissue engineering, as well as in translational research. Inflammation, biomarkers, metabolic syndrome, obesity, and lipids are hot topics across population, clinical and basic research, supporting integration across the cardiovascular field.
Growth in clinical and population research emphasizes improving patient outcomes through novel treatments, risk stratification, and prevention. Translation and innovation redefine basic research in cardiovascular disease. Medical need, funding and publishing policies, and scientific opportunities are potential drivers for these evolutions.
Current policies for public funding of health research increasingly focus on innovation, with a final goal to improve health outcomes. 1 To support policies, roadmaps are established, for example for diabetes 2 and respiratory 3 diseases. In the USA, the joint Academies developed a document to guide national policy in health 4 with a dedicated document for cardiovascular medicine 5 that includes general directions for research. In Europe, building a roadmap for cardiovascular research is one of the tasks of the ERA-CVD network. 6 Expert opinion guides the exercise but a macro and global-level overview of past cardiovascular research can enrich the debate and strengthen the basis for recommendations. The breadth of cardiovascular research is astounding, 7 with research undertaken across a variety of institutions and with each piece of research having its own scope/focus or topic. It is thus challenging to review and summarize all the research that has been undertaken.
Identifying all the relevant research is the first hurdle to overcome, then classifying or identifying topics of research is the next significant hurdle. Journal classification systems offer little assistance, as they are not granular enough to identify more specific topics within broader fields. Thesauri or medical dictionaries, such as PubMed or the International Classification of Diseases (ICD), do not offer an overview of time-dependent changes in topics or changing concepts.
Identifying key topics using semi-automatic approaches based on text analysis is an alternative solution that takes advantage of recent developments in high-level informatics. As this is not reliant on a predefined classification, it may result in different outcomes. Various methods use natural language processing (NLP) to extract topics or clusters from text. For example, the bibliometric community has compared the results when varying methods are applied to a set of astronomy publications, focusing on the importance having topic expert input throughout the process. 8 The recent CardioScape project analysed abstracts of 2476 research projects awarded 2010–12 as published by funding bodies. The authors assigned research project to topics, based on the abstract text, using a semi-automatic process that tested and trained the data to more quickly allocate abstracts to a topic than depending solely on expert review. They produced a detailed taxonomy or classification of cardiovascular research based on the list of topics of the European Society of Cardiology, creating a hierarchical list of over 600 topics. 9
Here, we aim to identify topics in published cardiovascular research and their evolution between 2004 and 2013, assessing whether they have appeared, disappeared, or changed over time. In a comprehensive approach, we use a combination of existing methods for text mining, network analysis, and clustering, and further develop these tools to handle a large dataset of >400 000 publications.
In our study, we use two different and complementary approaches. A first one detects topics across the collection of publications, counting number of documents, and relations between topics. A second one maps document networks into clusters with an identifiable subject of research. These approaches are described here in brief, with more detail provided in the Supplementary material online .
Data sources
The dataset includes the reference, abstract, address, and citation data for 478 006 cardiovascular publications from 2004 to 2013, including 2014 references to these documents, using an expert informed search strategy and references to core cardiovascular journals, as previously published. 7 The documents span across >5000 journals, and include cardiovascular publications in leading general journals in medical and life sciences ( Supplementary material online , Table S1 ). We obtained the data from Clarivate Analytics Web of Science Core Collection (WoS) through a custom data license held by ECOOM, KU Leuven.
Text pre-processing
We took all titles and abstracts of the above publications, and extracted the noun phrases (text fragments of various lengths) using the NLP framework developed at Stanford. 10 Supplementary material online , Figure S1 illustrates the subsequent data flow for the analysis.
Topic modelling
For this approach, we applied latent Dirichlet allocation (LDA) 11 to the above-mentioned text fragments from the titles and abstracts of all publications. This LDA approach groups the text fragments to identify topics and allocates documents to topics. In this approach, a document contributes to several topics. Of note, general terms or terms that are used frequently across the majority of documents are filtered out as part of the methodology, resulting in groups of highly specific text fragments and, consequently, topics, as illustrated in Supplementary material online , Figure S2 .
At least three cardiovascular experts (listed in the Acknowledgements section) named each topic based on a set of the top 40 text fragments representing a topic. Further rounds of cross-review validated and consolidated the naming process. A final review of all topics ensured naming consistency across the topics and allowed for additional expert-based classification as clinical, basic, or population research.
We then calculated the number of documents that contributed to a topic, using probability analysis in LDA. Furthermore, we calculated the co-occurrence of topics in the publications, and visualized the outcome of this network analysis using VOSViewer ( www.vosviewer.com ). 12
Document clustering
For this second approach, the dataset was reduced to two periods, and we analysed the cardiovascular publications from 2006 to 2008 and those from 2011 to 2013, separately. For each time period, we then calculated the similarities between documents based on the noun phrase text fragments from the titles and abstracts of all publications and based on the references in these publications, using adapted cosine calculations and a hybrid document clustering algorithm, as previously described. 13 We then applied the Louvain 14 community detection algorithm to identify clusters of similar documents. For this method, each document is only located in one cluster. Subsequently, we applied the DrL/OpenOrd algorithm 15 to map and visualize the documents and clusters. We used R 16 in a high-powered cloud-based parallelized computing environment for all operations.
We identified and described the core documents, 13 the most common text fragments, as well as, the most highly cited documents and the most productive authors in each cluster, to name the clusters. For each document cluster, we identified the most highly representative topics from the LDA topic model.
Evolution of cardiovascular topics—trends and ‘hot’ topics
We identified 175 topics, listed alphabetically in Supplementary material online , Table S2 . This list groups specific topics within areas such as atherosclerosis, coronary artery disease, arrhythmias, heart failure, and their evolution over time.
For a visual and comprehensive overview, we prepared a map of the topics and their interrelation, based on co-occurrence within publications using a network analysis ( Figure 1 A ). This map identifies different categories of research: population (at the top, blue), clinical (left, green/yellow), and basic research (right, red). Large topics in each category define overarching interests such as Evidence-guided-treatment and Outcomes and prognosis in clinical research, and Epidemiology of CVD and risk factors in population research, topics that have seen large growth in numbers of publications since 2004 ( Figure 1 B ). Cell signalling and gene transcription is a central topic for basic research, with modest growth ( Figure 1 B ).

Main areas and organization of research focus. ( A ) Visual presentation of the topics in 2013 and how they relate to each other, based on how often the topics are included in the same publication. Each circle represents one topic and each group of topics is highlighted in a separate colour; the most similar documents and clusters are located closer to each other based on VOSviewer mapping. ( B ) Evolution of overarching topics.
More focused ‘hot’ topics that experienced a large growth in number of publications are presented in Figure 2 .

Topics with large growth. For population research, the eight topics that increased more than two-fold in volume are shown; for clinical research, 27 topics increased more than two-fold and 10 of these are presented; for basic research only two topics had more than a two-fold increase, and the top 8 growers are presented. Overarching topics are shown in Figure 1 B .
In population research, risk factors with research on metabolic syndrome, lipids, diabetes, physical activity, and mental health are prominent. In clinical research, patient management after myocardial infarction (MI) and outside the hospital are leading topics, but the true ‘hot’ topic was aortic valve disease that saw a surge of interest, related to transaortic valve repair, starting 2008. Though still small in numbers, heart failure research and stem cells saw substantial growth. This last clinical topic complements the major hot topics in basic research, on stem cells and cardiac repair and tissue engineering. In basic research, increasing translational output in metabolic syndrome and diabetes use mostly mouse models. Focused topics are organelle studies on mitochondria and endoplasmic reticulum.
Table 1 complements the fast growing topics of Figure 2 with additional leading 2013 topics. Most of these also have grown since 2004, but two topics, even if large, seem to have lost momentum, i.e. longitudinal studies on blood pressure, and basic research in cardiac electrophysiology.
Large topics in 2013
PCOS, polycystic ovary syndrome.
Only four topics in clinical, and none in population research, saw a decrease, whereas seven topics in basic research saw a decline in output ( Figure 3 A ). Across all topics, the growth in publication output, measured as the number of documents in 2013 divided by the number of documents in 2004, was significantly larger in clinical and population research topics than in basic research topics ( Figure 3 B ).

Unequal growth of research output across categories. ( A ) Topics that saw a decrease of >5%, i.e. 4/102 clinical and 7/50 basic research topics. ( B ) Average growth in each category. Each dot presents a topic; the values are the fractional growth, i.e. the number of documents in 2013 divided by the number of documents in 2004. Kruskal–Wallis followed by Dunn’s test for multiple comparisons; *** P < 0.0001 basic vs. clinical and vs. population.
When considering the overall output and growth of publications across the categories of population, clinical and basic research, the data suggest that the share of basic research publications is declining.
Document clusters define large research areas and trends
The size of topics represents the activity within each of these—documents contribute to more than one topic. In a complementary approach, we examined how documents group together based on the similarity of their text and of their references, whereby each document can belong to one cluster only, effectively dividing the total publication output into different areas. The hybrid clustering algorithm was applied to two datasets, i.e. the publications from 2006 to 2008 and 2011 to 2013.
In each period, 10 large clusters emerged, accounting for >90% of all documents.
To identify trends, we compare the two periods and examine the evolution over time ( Figure 4 ). In the graph legends, emerging areas are marked by green triangle, decreasing ones with a red triangle. Risk scoring in the population and related patient management are the leading areas, growing over time (top position). In 2011–13, a large cluster emerges that relates to gene and stem-cell therapy, including research on inducible pluripotent stem cells. Documents within this cluster include research on ischaemic heart disease and arrhythmias. Haemodynamics and biomechanics are another emerging area that includes documents on atherosclerosis and vascular diseases such as aneurysms, but also heart failure and assist devices. Aortic valve disease is a newly defined area in 2011–13. Imaging also becomes very prominent as an area in its own right. Whereas in 2006–08, hypertension was a defined area, this is no longer identifiable in 2011–13.

Distribution of document clusters in 2006–08 and in 2011–13. ( A ) In 2006–08, the 10 largest clusters represent 93% of the total publication output in this period. ( B ) In 2011–13, the 10 largest clusters represent 92% of the total publication output in this period. The colour codes for similar clusters are maintained across the periods. However, some clusters are present in only one period. The clusters are arranged by size, reading clockwise from the top, and the legends arranged accordingly. Red triangles mark clusters that disappeared and green triangles emerging clusters.
For the last period, we also examined the structure and interrelation of clusters, using a graphical rendering, giving insight in the size, composition, and presence of subclusters ( Figure 5 ).

Document clusters’ map 2011–13. A visual presentation of documents in clusters and subclusters: the most similar documents and clusters are located closer to each other, based on the DrL two-dimensional mapping layout technique.
In this force-directed DrL graph layout, the documents and clusters are mapped to minimize the distance between the most similar documents and maximize the distance between non-linked documents. This produces a two-dimensional co-ordinate layout where the documents closest to each other share the most similarities since they share common text fragments and references. Conversely, documents and clusters on the edges of the graph have the least similarity to other documents or clusters.
Cluster 2 on gene and stem cells is dense and separate, yet touches and interacts with Cluster 5 [acute coronary syndrome (ACS) and MI]. Cluster 9 on imaging is spread out in subclusters at different locations, including one near Cluster 5 (ACS and MI), and one near Cluster 4 (heart failure). Cluster 8 (arrhythmias) is also split with one part closer to heart failure, another to anticoagulation and atrial fibrillation.
Further naming the subclusters is presently beyond reach, as it would require a lot of expert input and resources. However, linking the clusters and the topics adds granularity to the larger research areas and provides internal methodological validation of the cluster naming.
Table 2 presents the most highly associated topics in the ten largest document clusters in each period. Overall, agreement with the LDA topics is high and provides more detail on the research contained in the clusters. E.g., the cluster ‘Haemodynamics’ is now showing different areas of focus, i.e. in congenital disease, aortic, and valvular diseases; the topic ‘Arrhythmias’ is more populated with device research in the second time period compared to the first.
Cluster names and topics present within clusters
AF, atrial fibrillation; ANS, autonomic nervous system; BP, blood pressure; CABG, coronary artery bypass grafting; CRT, cardiac resynchronization therapy; CT, computed tomography; CV, cardiovascular; DES, drug-eluting stent; ECG, electrocardiogram; HF, heart failure; LV, left ventricular; NOAC, new oral anticoagulant; PTCI, percutaneous transluminal coronary intervention; RV, right ventricle; STEMI, ST elevated myocardial infarction.
The method for identification of topics in cardiovascular publication output allowed the visualization and evaluation of trends in cardiovascular research. Over a 10-year period significant shifts occur.
Identification of cardiovascular research topics through natural language processing
In cardiovascular research, topics are generally predefined in a taxonomy that can be hierarchical and/or matrix structured. The CardioScape project approach (see Introduction section) was well suited to its purpose of the analysis of 2476 project abstracts in a single time period and using an existing taxonomy has the advantage of recognizable areas of research. The bottom-up approach used here lent itself well to analysis of much larger numbers of documents and generated a topic list that represents the interests from the community during the period under study.
A recent study by the WHO working to identify cardiovascular disease research output from random sets of publications from PubMed required a significant amount of expert-based review of only a small proportion of the published articles. 17 The current approach was more comprehensive in coverage of the field, but despite reliance on advanced automated analysis, experts still had an important role in interpreting and linking concepts to validate the results.
In the current naming of topics and clusters, experts frequently used terms that connect to a classic hierarchical list in the field, including major diseases, and recognizing clinical, population, and basic discovery research. Nevertheless, the approach uncovered specific emerging areas of research such as transcatheter aortic valve implantation (TAVI), topics consistent with broad trends, such as risk stratification and evidence-based guidance, and innovation (gene and stem cell research). Some of these terms would not appear in a classic taxonomy and thus the NLP approach offers novel insights.
The present study was not attempting to classify all research but to capture and identify the most common and evolving topics over time in the cardiovascular field by using a comprehensive set of cardiovascular publications across some 5000 journals.
Emphasis on improving clinical care and risk assessment
The most represented and fast growing topics across the documents are evidence-based guidance for treatment and research on outcomes and prognosis. These result underscore the attention given to guidelines and evidence based medicine (EBM). 18–23 Part of this research is likely to represent the large number of clinical trials taking place in the cardiovascular field, 24 which over time have had a significant effect on the reduction of mortality from CVD due to establishing the effectiveness and safety of a number of drugs and medical interventions in cardiovascular disease. 25 The presence of policy related topics, such as the topics on quality of care and health economics likewise supports the focus on implementation research and a shift of focus from reducing acute mortality to care in chronic disease.
Growth of research on risk factors emphasizes the importance of preventative medicine, evident in both the topics analysis and the document cluster analysis. However, some specific blood pressure studies declined over time, perhaps reflecting the change in focus on the single risk factor of ‘blood pressure’ to a multivariable spectrum and newly identified risk factors. We have also previously shown that hypertension has moved more closely to clinical cardiovascular research over time. 26
Smaller topics illustrate crosstalk with non-cardiovascular diseases, because of shared risk factors or common methods used in research or occurrence of cardiovascular complications. The latter is particularly evident in two topics that focus on cardiovascular complications in pregnancy and in cancer.
Innovation and translation in clinical and basic science
Major diseases such as ischaemic heart disease and arrhythmias, remain present over time but shifts can be seen. There is for example, a larger focus on atrial fibrillation, in particular embolic risk, on novel treatments, such as stem cells in heart failure, and transcatheter aortic valve interventions as a dominant element within the topic of valvular heart disease. 19 Imaging is present in several topics but emerges as a cluster in its own right in the document analysis. Many of these changes are driven by technological innovation and translation.
Basic research as a whole saw its share decline, but with interesting shifts in content. Although the topic analysis and mapping identifies basic research topics as a category, there are complementarities across categories. Stem cell research, tissue engineering, and biomechanical factors saw rapid growth and are also present in clinical topics. This also applies to inflammation and diabetes. Animal models for disease are rapidly growing topics consistent with growth of translational research.
An analysis of the countries of authorship of the publications in the emerging clusters of discovery research shows that the USA leads in the number and share of publications (30%+), followed mostly by Germany, or the UK or Italy. However, for the large document cluster on genes and stem cells in 2011–13, the second most productive country is China, contributing 17.5% of the publications in this cluster (Supplementary material online, Figure S3 ).
Interestingly, inflammation, biomarkers, metabolic syndrome, obesity, and lipids are hot topics with growing research output in population, clinical and basic research, indicating integration and crosstalk across the spectrum of cardiovascular research.
Drivers of change
Technology and opportunity-driven scientific interest, but also strategic choices and funding policies are likely to influence trends in research. CardioScape studied public and charity funding in the years 2010–12 and describes major investments in clinical research. Yet the share of publication output globally for clinical research appears to be substantially larger than the share of funding for clinical research reported in CardioScape. This could be explained by clinical research funded by other sources, such as industry or local funding, which are not included in the CardioScape analysis. Also, the present data represent global output. Major research investments in China, and the emphasis on clinical research in the USA, can contribute to some of the global trends.
The slower growth in basic science could reflect a slower growth in investment. This can be absolute or relative towards the increasing costs of advanced research methodology. Another reason could be editorial pressure for more comprehensive papers that may reduce quantity to the benefit of rich content in individual papers.
Finally, growing translational research may blur the boundaries between basic and clinical research and lead to an apparent slower growth in discovery research.
Policy perspectives
Policy development is a forward looking exercise. In health research, medical needs identified by health data and expert opinion, are an important consideration. 27 Past research output helps to identify areas that may need more investment. Research funders also use input from society. 28 When assessing current priorities in cardiovascular research for the Dutch 28 and British 29 Heart Foundations we can see that research into heart failure and arrhythmias are common across their top priorities. Focus on healthy lifestyles is a top priority in the Dutch Heart Foundation as well as in the US vision and strategic agenda. 4 , 5 At the macro-level, the data presented here indicate that some of the main issues presented in these research agendas are actively pursued but others less so.
Study limitations
Limitations of studying research topics have been addressed in the bibliometric field. 8 The reliance of expert input is a limitation and potential source of bias that we tried to minimize by using mixed panels.
The current approach was not sufficiently granular to extract recent emerging topics that contain a limited number of documents. In addition, publication output is somewhat delayed vs. actual research and experts may be aware of ongoing research with still limited output. In this case, the method and dataset can be used to interrogate about specific developments (see Supplementary material online , Table S3 for data on micro-RNA and personalized medicine).
As the data set ends in 2013, very recent developments are not covered. This relates to the methodological complexity. Web of Science data including 2014 references were available mid-2015, the cardiovascular publications dataset was complete in 2016 and algorithms for analysis including re-iterative expert review required another 18 months. A similar time lag is seen in other studies that rely on data mining and processing. 9 Congress abstracts could be considered as a source to identify emerging topics but have several limitations. They are of a different nature than papers and the scope of a congress shapes content of selected abstracts. We provide a complementary survey of 3000 abstracts from the 2018 congress of the European Society of Cardiology, illustrating the strong presence of clinical research at this event, within the topics of Clusters 1 and 3–7 of Table 2 ( Supplementary material online , Figure S4 ). Two emerging topics were cardio-oncology and digital health, each representing however <25 abstracts.
In the present analysis, quality and impact of studies in a particular domain were not evaluated, though highly cited papers were part of the cluster identification. In their analysis of poorly cited papers covering 165 000 papers in 1997–2008, Ranasinghe et al . 30 noted the highest percentage of poorly cited papers in the clinical and population research category. Nevertheless, as they and others 31 have noted, citations are not the only parameter to assess impact, in particular in clinical medicine.
Identification of leading research topics and trends illustrates the emphasis on improving clinical medicine, and the growing interest in risk stratification and preventive medicine. Translation and innovation redefine cardiovascular research. Linking the present data with the insights of the professional community and of funders and society, may contribute to the building of a future research roadmap.
The authors thank to the following experts for their review of the text fragments and input into the names of the topics: Dr Matthew Amoni, Dr Peter Haemers, Prof Sian Harding, Dr Frederik Helsen, Prof Gerd Heusch, Prof Tatiana Kuznetsova, Prof Tobias Op‘t Hof, Prof Frank Rademakers, Dr Sander Trenson, Dr Bert Vandenberk, and Dr Maarten Vanhaverbeke.
D.G. had a PhD Fellowship through KU Leuven.
Conflict of interest: K.R.S. is Past Editor-in-Chief of Cardiovascular Research (2013–17). W.G. is Editor-in-Chief of Scientometrics .
Directorate-General for Research and Innovation. Europe’s future: open innovation, open science, open to the world. Reflections of the Research, Innovation and Science Policy Experts (RISE) High Level Group . Brussels : European Commission ; 2017 . p. 228 .
Google Scholar
Google Preview
Welcome to DIAMAP. DIAMAP—Roadmap for Diabetes Research in Europe. http://www.diamap.eu/ (3 December 2013 ).
European Respiratory Roadmap—Recommendations for the Future of Respiratory Medicine. https://www.ersnet.org/publications/european-respiratory-roadmap (22 January 2018 ).
Committee on Global Health and the Future of the United States, Board on Global Health, Health and Medicine Division, National Academies of Sciences, Engineering, and Medicine. Washington, DC : National Academies Press ; 2017 p. 360.
Fuster V , Frazer J , Snair M , Vedanthan R , Dzau V. The Future Role of the United States in Global Health: emphasis on Cardiovascular Disease . J Am Coll Cardiol 2017 ; 70 : 3140 – 3156 .
ERA-Net on Cardiovascular Diseases—ERA-CVD. http://www.era-cvd.eu (22 January 2018 ).
Gal D , Glänzel W , Sipido KR. Mapping cross-border collaboration and communication in cardiovascular research from 1992 to 2012 . Eur Heart J . 2017 ; 38 : 1249 – 1258 .
Velden T , Boyack KW , Gläser J , Koopman R , Scharnhorst A , Wang S. Comparison of topic extraction approaches and their results . Scientometrics 2017 ; 111 : 1169 – 1221 .
Pries AR , Naoum A , Habazettl H , Dunkel M , Preissner R , Coats CJ , Tornada A , Orso F , Van de Werf F , Wood DA , Van de Werf F , Wood DA , O'Kelly S , Craven J , Coats A , Sipido K , De Backer D , Wallentin L , Hasenfuss G , della Sala L , Leggeri I , Wood DA , Van de Werf F , Jaarsma T , Elliott P , Pries AR , Madonna R , Kjeldsen K , Maggioni AP , Franco OH , Hills S , Pugliese F , De Bacquer D. CardioScape mapping the cardiovascular funding landscape in Europe . Eur Heart J 2018 ; 39 : 2423 – 2430 .
Chen D , Manning CD. A fast and accurate dependency parser using neural networks. Proceedings of the 2014 Conference on Empirical Methods in Natural Language Processing (EMNLP) . Doha, Qatar : The Association for Computational Linguistics ; 2014 . pp. 740 – 750 .
Blei DM , Ng AY , Jordan MI. Latent Dirichlet allocation . J Mach Learn Res 2003 ; 3 : 993 – 1022 .
van EN , Waltman L. Citation-based clustering of publications using CitNetExplorer and VOSviewer . Scientometrics 2017 ; 111 : 1053 – 1070 .
Glänzel W , Thijs B. Using hybrid methods and ‘core documents’ for the representation of clusters and topics: the astronomy dataset . Scientometrics 2017 ; 111 : 1071 – 1087 .
Blondel VD , Guillaume J-L , Lambiotte R , Lefebvre E. Fast unfolding of communities in large networks . J Stat Mech Theory Exp 2008 ; 2008 : P10008.
Boyack KW , Klavans R. Creation of a highly detailed, dynamic, global model and map of science: creation of a highly detailed, dynamic, global model and map of science . J Assoc Inf Sci Technol 2014 ; 65 : 670 – 685 .
RStudio Team. RStudio: Integrated Development Environment for R . Boston, MA : RStudio, Inc .; 2015 .
Myers L , Mendis S. Cardiovascular disease research output in WHO priority areas between 2002 and 2011 . J Epidemiol Glob Health 2014 ; 4 : 23 – 28 .
Neumann F-J , Sousa-Uva M , Ahlsson A , Alfonso F , Banning AP , Benedetto U , Byrne RA , Collet J-P , Falk V , Head SJ , Jüni P , Kastrati A , Koller A , Kristensen SD , Niebauer J , Richter DJ , Seferovic PM , Sibbing D , Stefanini GG , Windecker S , Yadav R , Zembala MO ; ESC Scientific Document Group. 2018 ESC/EACTS Guidelines on myocardial revascularization . Eur Heart J 2019 ; 40 : 87 – 165 .
Genereux P , Head SJ , Wood DA , Kodali SK , Williams MR , Paradis J-M , Spaziano M , Kappetein AP , Webb JG , Cribier A , Leon MB. Transcatheter aortic valve implantation 10-year anniversary: review of current evidence and clinical implications . Eur Heart J 2012 ; 33 : 2388 – 2398 .
Collins R , Reith C , Emberson J , Armitage J , Baigent C , Blackwell L , Blumenthal R , Danesh J , Smith GD , DeMets D , Evans S , Law M , MacMahon S , Martin S , Neal B , Poulter N , Preiss D , Ridker P , Roberts I , Rodgers A , Sandercock P , Schulz K , Sever P , Simes J , Smeeth L , Wald N , Yusuf S , Peto R. Interpretation of the evidence for the efficacy and safety of statin therapy . Lancet 2016 ; 388 : 2532 – 2561 .
Eerenberg ES , Kamphuisen PW , Sijpkens MK , Meijers JC , Buller HR , Levi M. Reversal of rivaroxaban and dabigatran by prothrombin complex concentrate: a randomized, placebo-controlled, crossover study in healthy subjects . Circulation 2011 ; 124 : 1573 – 1579 .
Razzouk L , Farkouh ME. Imaging outcomes in cardiovascular clinical trials . Nat Rev Cardiol 2009 ; 6 : 524 – 531 .
Brown ML , Gersh BJ , Holmes DR , Bailey KR , Sundt TM III . From randomized trials to registry studies: translating data into clinical information . Nat Clin Pract Cardiovasc Med 2008 ; 5 : 613 – 620 .
Clinical Trials in Cardiology/Vascular Diseases. https://www.centerwatch.com/clinical-trials/listings/therapeutic-area/1/cardiology-vascular-diseases/ (13 April 2018 ).
Solomon SD , Pfeffer MA. The future of clinical trials in cardiovascular medicine . Circulation 2016 ; 133 : 2662 – 2670 .
Gal D , Thijs B , Glänzel W , Sipido KR. A changing landscape in cardiovascular research publication output: bridging the translational gap . J Am Coll Cardiol 2018 ; 71 : 1584 – 1589 .
Chalmers I , Bracken MB , Djulbegovic B , Garattini S , Grant J , Gülmezoglu AM , Howells DW , Ioannidis JPA , Oliver S. How to increase value and reduce waste when research priorities are set . Lancet 2014 ; 383 : 156 – 165 .
Onderzoeksagenda Hartstichting. https://www.hartstichting.nl/wetenschappelijk-onderzoek/onderzoeksagenda-hartstichting (13 April 2018 ).
British Heart Foundation Research Strategy 2015 –2020. http://extras.bhf.org.uk/research-strategy/site/index.html (13 April 2018).
Ranasinghe I , Shojaee A , Bikdeli B , Gupta A , Chen R , Ross JS , Masoudi F , Spertus JA , Nallamothu BK , Krumholz HM. Poorly cited articles in peer-reviewed cardiovascular journals from 1997-2007: analysis of 5-year citation rates . Circulation 2015 ; 131 : 1755 – 1762 .
Nallamothu BK , Lüscher TF. Moving from impact to influence: measurement and the changing role of medical journals . Eur Heart J 2012 ; 33 : 2892 – 2896 .
- cardiovascular system
- basic science research
Supplementary data
Email alerts, more on this topic, related articles in pubmed, citing articles via, looking for your next opportunity, affiliations.
- Online ISSN 1522-9645
- Print ISSN 0195-668X
- Copyright © 2024 European Society of Cardiology
- About Oxford Academic
- Publish journals with us
- University press partners
- What we publish
- New features
- Open access
- Institutional account management
- Rights and permissions
- Get help with access
- Accessibility
- Media enquiries
- Oxford University Press
- Oxford Languages
- University of Oxford
Oxford University Press is a department of the University of Oxford. It furthers the University's objective of excellence in research, scholarship, and education by publishing worldwide
- Copyright © 2024 Oxford University Press
- Cookie settings
- Cookie policy
- Privacy policy
- Legal notice
This Feature Is Available To Subscribers Only
Sign In or Create an Account
This PDF is available to Subscribers Only
For full access to this pdf, sign in to an existing account, or purchase an annual subscription.
Masks Strongly Recommended but Not Required in Maryland, Starting Immediately
Due to the downward trend in respiratory viruses in Maryland, masking is no longer required but remains strongly recommended in Johns Hopkins Medicine clinical locations in Maryland. Read more .
- Vaccines
- Masking Guidelines
- Visitor Guidelines
Cardiovascular Research
Research topics, cardiovascular research topics, heart rhythm and arrhythmias.
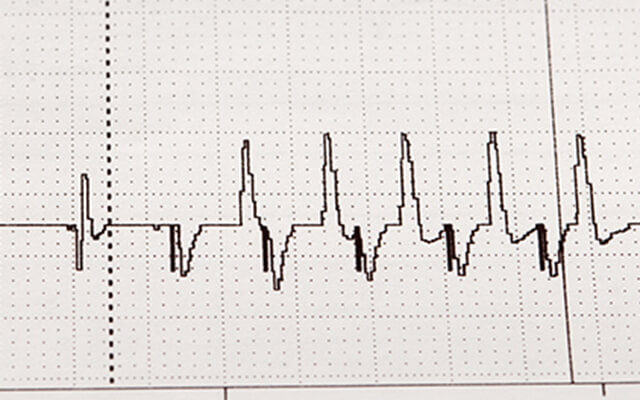
HIV and Heart Disease
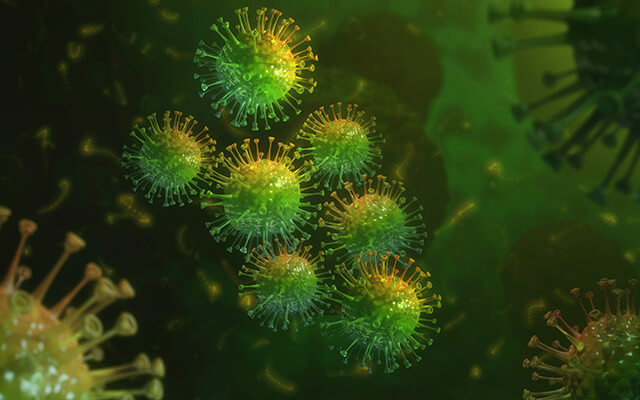
Hypertension
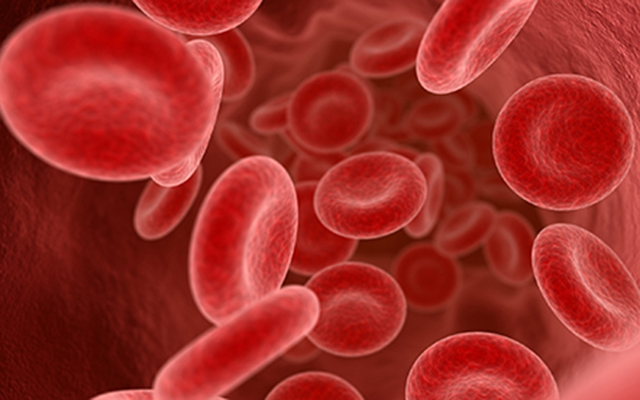
Interventional Cardiology
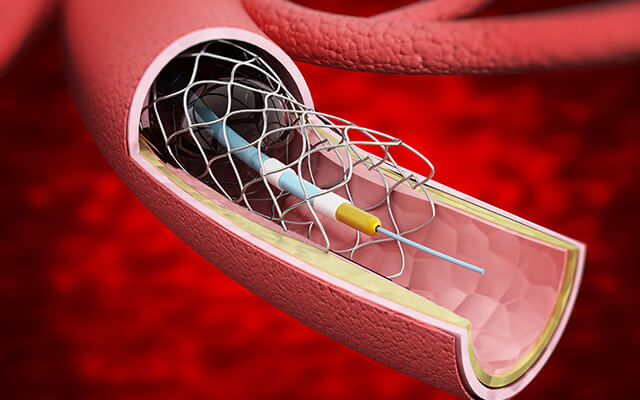
Myocardial Biology/Heart Failure
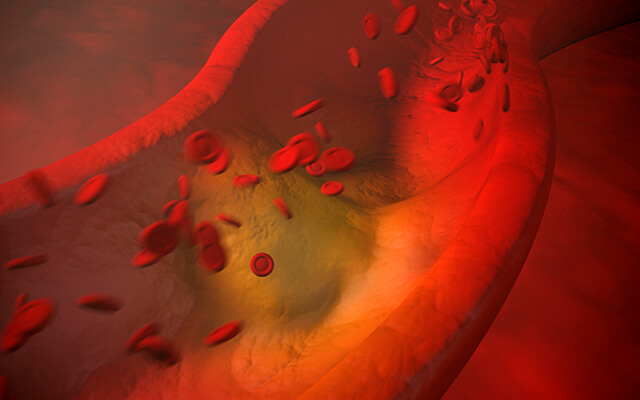
Myocarditis
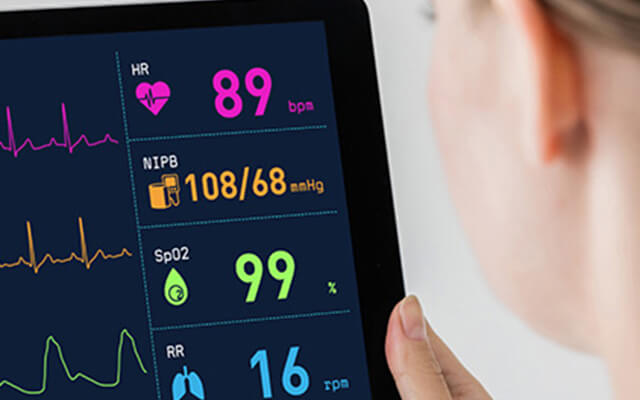
Precision Medicine
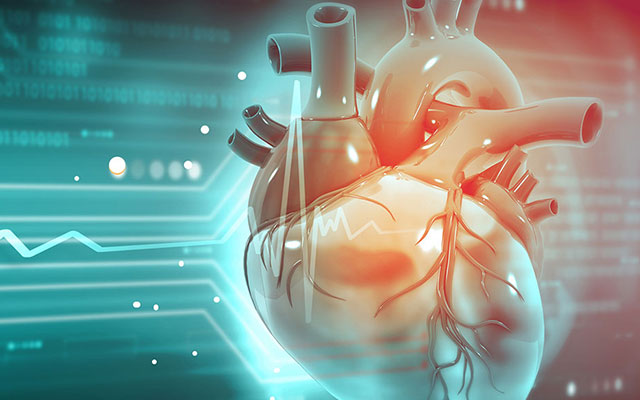
Preventive Cardiology
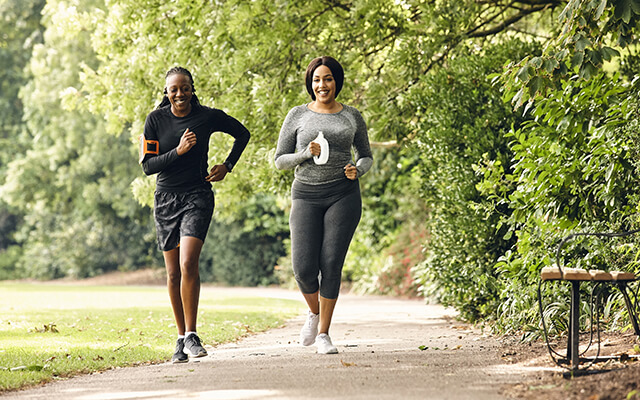
Stem cell and Regenerative Biology
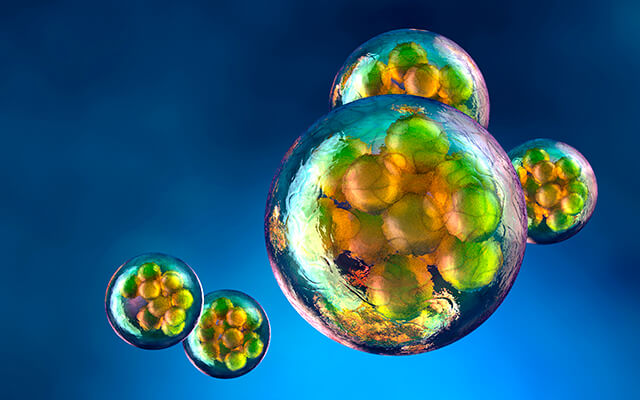
Women and Heart Disease
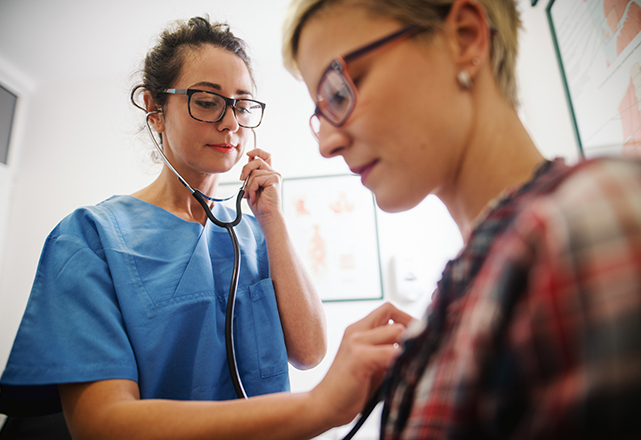
Breakthrough Discoveries Core Lab
The Johns Hopkins Core Lab provides access to Small Animal Cardiovascular Phenotyping and Model Core.

An official website of the United States government
The .gov means it’s official. Federal government websites often end in .gov or .mil. Before sharing sensitive information, make sure you’re on a federal government site.
The site is secure. The https:// ensures that you are connecting to the official website and that any information you provide is encrypted and transmitted securely.
- Publications
- Account settings
Preview improvements coming to the PMC website in October 2024. Learn More or Try it out now .
- Advanced Search
- Journal List
- Comput Intell Neurosci
- v.2022; 2022

Cardiovascular Disease Detection using Ensemble Learning
Abdullah alqahtani.
1 College of Computer Engineering and Sciences, Prince Sattam Bin Abdulaziz University, AlKharj, Saudi Arabia
Shtwai Alsubai
Mohemmed sha, lucia vilcekova.
2 Faculty of Management, Comenius University in Bratislava, Odbojárov 10, 82005 Bratislava 25, Slovakia
Talha Javed
3 Department of Computer Science, COMSATS University, Sahiwal, Pakistan
Associated Data
The Cardiovascular Disease data used to support the findings of this study are included in the article.
One of the most challenging tasks for clinicians is detecting symptoms of cardiovascular disease as earlier as possible. Many individuals worldwide die each year from cardiovascular disease. Since heart disease is a major concern, it must be dealt with timely. Multiple variables affecting health, such as excessive blood pressure, elevated cholesterol, an irregular pulse rate, and many more, make it challenging to diagnose cardiac disease. Thus, artificial intelligence can be useful in identifying and treating diseases early on. This paper proposes an ensemble-based approach that uses machine learning (ML) and deep learning (DL) models to predict a person's likelihood of developing cardiovascular disease. We employ six classification algorithms to predict cardiovascular disease. Models are trained using a publicly available dataset of cardiovascular disease cases. We use random forest (RF) to extract important cardiovascular disease features. The experiment results demonstrate that the ML ensemble model achieves the best disease prediction accuracy of 88.70%.
1. Introduction
The human heart is the body's most important organ, responsible for pumping blood to every body region. The circulatory system comprises arteries, veins, and capillaries, all of which connect to the heart [ 1 ]. The heart is one of the body's most essential organs, yet it is vulnerable to illness and injury. Because of its danger to human life and the illnesses and injuries it causes, its presence cannot be ignored. Heart disease affects the heart's pumping systems, causing it to malfunction [ 2 ]. Cardiovascular disease is a category of ailments that are caused by heart problems. Cardiovascular disease may be diagnosed by shortness of breath, physical weakness, swollen feet, and exhaustion. High cholesterol, smoking, sedentary lifestyles, and high blood pressure are a few risk factors for cardiovascular disease [ 3 ]. The leading cause of mortality, according to the World Health Organization [ 4 ], is cardiovascular disease, which kills 18 million people a year, or about 32% of all fatalities. The most common kind of heart disease is coronary artery disease. As a result, heart disease and stroke are major public health concerns.
Clinicians often employ angiography to diagnose cardiovascular disease. In underdeveloped nations, where diagnostic technologies, physicians, and other resources are few, this diagnosis process is time-consuming and costly since it requires examining many variables. Heart disease has become one of the most critical medical topics in recent years as the mortality toll from the cardiovascular disease continues to climb. Prediction aids in the early detection of disease and the most effective treatment. The use of ML in medical diagnostics is proliferating. This may be partly ascribed to improving the categorization and identification of illnesses, giving data to help medical professionals discover and diagnose diseases, sustaining human health, and decreasing the mortality rate. When determining the likelihood of a disease incidence, ML classification approaches are often used [ 5 , 6 ]. This work's goal is a classification model that can predict cardiovascular disease.
Making predictions based on experience has become a central goal of ML research. The neural network is one of the most common methods of machine learning. This is the first step in supervised learning, in which a model is built using labeled data, and its performance is assessed using test data. Classification and regression difficulties are common in supervised learning. This is the second form of learning, where the data is not labeled, and the model attempts to uncover the hidden patterns in the data. Data exploration results generate inferences about the nature of any concealed knowledge. Unsupervised learning is shown by the clustering method [ 7 ]. Reinforcement learning is the third form, which does not employ labeled data and does not link the conclusions to the data. An environment's intelligent agents [ 8 ] focus on this research. Use a real-world dataset of patients with cardiovascular illness to construct a classification model. This ML method is often used to predict the likelihood of illness occurrence [ 5 , 6 ]. To forecast the class of fresh samples, a model learned from training data is used [ 9 , 10 ]. Categorizing data into classes is also a supervised learning notion [ 10 ].
This work provides contributions to detecting cardiovascular disease more effectively and efficiently. Below are the major contributions of this paper.
- Proposed ensemble learning method for detecting cardiovascular disease including several classifiers in voting-based decision-making.
- Compare the proposed method to traditional machine learning approaches such as RF, K-nearest neighbor (KNN), multi-layer perceptron (MLP), and State-of-the-art research to determine the efficacy of the proposed approach.
- Experimental results show a 1.47% accuracy improvement compared to earlier state-of-the-art research and standard machine learning algorithms for the proposed approach.
Section 2 provides an overview of the relevant literature. Details regarding the proposed approach and the dataset are provided in section 3 , and the outcomes and comparisons are provided in Section 4 in the comparative part. Finally, Section 5 concludes this work and provides a detailed description of future research.
2. Literature Review
Machine learning has sparked widespread interest in various fields, including health and medicine [ 11 ]. Researchers have proposed numerous viable approaches to detecting cardiac disease using machine learning. Consequently, studies on developing medical applications employing various machine learning algorithms and approaches have been published.
According to [ 12 ], machine learning may be used to measure and predict people's cognitive health using intelligent home sensors that have been built. A total of 179 individuals were viewed as volunteers by the respondents. We found an r = 0.79 association between the automated task quality evaluation ratings and the direct observation scores. Participants' cognitive health was predicted using machine learning approaches with an AUC of 0.64.
According to [ 13 ], machine learning may be used to detect cardiovascular illness based on a patient's clinical data. The RF model was the most accurate, with an accuracy rate of 86.60%. Motarwar et al. [ 14 ] developed an algorithmic system to predict heart disease risk. Their findings, at 95.08%, discovered that RF was the most accurate. Multiple algorithms, including Decision Tree (DT), NB, KNN, and RF, were used by Sabarish and Parvati [ 5 ]. According to the data, the KNN algorithm has the highest accuracy rate (90.7%). Additionally, a CNN model was used to detect images of Chinese herbal medication, achieving a 71% total accuracy. With an incredible 96.7% success rate, the usage of ANN for lung cancer detection was also found [ 15 ].
Some studies look at ways to improve the status quo and develop new classifiers. Using Natural Language Processing (NLP) to train and assess a depression prediction model, for example, was proposed in research by [ 16 ]. According to [ 17 ], a neural network was proposed for predicting diabetes, with a prediction accuracy of 87.3%. Compared to its baseline performance of 89% and 81%, the proposed approach enhanced the neural network's performance by 91% in the training and 86% in the testing.
It was hypothesized in [ 18 ] that the Particle Swarm Optimization (PSO) approach might be used to enhance a neural network's accuracy further. The research was based on a dataset that included 303 healthy and ill people cases. There were 72 features in the dataset, but only 13 of them were employed in the feature selection process using PSO. The PSO was utilized for feature selection and ranking after preprocessing the dataset. From a pool of thirteen options, ranking results indicated the eight most valuable options for improving neural network training accuracy using a feedforward backpropagation approach. According to the study's findings, the hybrid PSO-FFBP neural network was 91.94% accurate in predicting heart illness. It was also shown that diabetic retinopathy might be predicted using an upgraded version of the DNN using the Grey Wolf Optimization (GWO) algorithm and PCA. A questionnaire was developed by Nowshad et al. [ 19 ] in the Sylhet area of Bangladesh, where they visited many local hospitals and health care facilities to collect data. Their dataset has 564 occurrences and 18 characteristics. The SVM was the most accurate, with a 91% success rate.
Using machine learning approaches to identify key traits, Mohan et al. [ 20 ] have created a method for improving cardiovascular disease prediction accuracy. The proposed mixed RF and linear model method were 88.7% accurate in predicting heart disease. Au et al. [ 21 ] have presented a hybrid approach for diagnosing cardiac disease. Logistic regression was shown to have an accuracy rate of 89% when predicting heart disease. Authors in [ 22 ] proposed a hybrid model for predicting coronary artery disease. They used three machine learning algorithms: RF, DT, and a mix of the two to create the model. The hybrid model was the most accurate, with an accuracy of 88.7%.
According to [ 23 ], they used standalone machine learning approaches to detect heart disease. They used different ensemble learning models to improve the accuracy of a prediction model. They used sleep disorder, stress mismanagement, and pollution factors as features.
For this study, we utilize data on cardiovascular disease. This dataset has around 70000 patients and 11 characteristics, which we believe is the third research to use. Also, we use various machine learning algorithms and deep learning algorithms to find the best one for predicting cardiovascular disease.
3. Proposed Approach
This section describes the methods to predict cardiovascular disease. Figure 1 depicts the proposed approach comprising six phases. The proposed approach begins with the selection of a dataset for the experiment. The Cardiovascular Disease dataset is used for experiments in this research. The preprocessing stage includes many steps that must be completed before model training. The features extraction approach is then utilized to determine the relevance of the features, and finally, several machine learning and deep learning classifiers are employed for experiments. Deep learning methods are also being evaluated in this study to detect cardiovascular disease. To identify the existence of cardiovascular disease, four prior ML classifiers are used: RF, KNN, DT, and Extreme Gradient Boosting (XGB). The ability of deep learning models on a particular dataset is tested using two deep neural network classifiers.

A proposed approach for cardiovascular disease detection.
3.1. Dataset Selection
The Kaggle repository's Cardiovascular Disease dataset ( https://www.kaggle.com/datasets/sulianova/cardiovascular-disease-dataset ) is utilized in this research. There are 70,000 samples in the dataset and 13 attributes. 70% data is utilized for training models, while the remaining 30% is used to assess the model's performance. Table 1 shows the description of the features and explains each feature in-depth.
Dataset features description.
3.2. Data Preprocessing and Corelation Analysis
Machine learning relies on data preprocessing to assess data quality and retrieves critical information that can influence the learning model's performance. Before training a model, preprocessing is essential. During the preprocessing stage, addressing diverse dataset characteristics, handling missing values, scaling, and standardization are dealt with during the preprocessing stage. This includes managing small, huge, and noisy datasets, overcoming overfitting, dealing with class imbalance, and label encoding. This work recommends using preprocessing approaches such as eliminating anomalies (outliers) and applying a standard scaler to the dataset to demonstrate the model's efficiency and acquire an acceptable and dependable accuracy for disease prediction. We employ a standard scaler to normalize the data within a predetermined range. It operates by altering characteristics like its distribution to reach a mean of 0 and a standard deviation of 1. Equation ( 1 ) defines a standard scaler, where S is the standardized form of z i .
ML models make better decisions depending on how labels are used. The Label Encoder transforms the target labels into numeric forms so that machines can read them for improved disease diagnosis. We also made the following changes to the dataset to identify better the characteristics that most affect cardiovascular disease:
- Body Mass Index (BMI) combines weight and height into a single characteristic that evaluates body fat percentage depending on height and weight.
- To make the dataset more understandable, features are modified while the information is preserved. The gender feature is converted to binary, and the age is translated from days to years in this dataset.
- Drop the dataset's year, height, weight, and id columns.
- Checking the maximum and minimum of quartiles to identify outliers and remove the rows that have outliers.
Pearson's coefficient (PCC) is used to get the corelationship between the characteristics to remove nonessential, duplicate, and redundant features from the data, as shown in Figure 2 . The correlation coefficient might be anywhere from −1 to 1. The features are negatively correlated if the value is close to −1; if the value is close to 1, the features are tightly coupled and considerably influence model performance. We set the threshold values to 0.85% to determine the PCC; if the correlation value is above the threshold, the feature is ignored; if the correlation value is below the threshold, the feature is kept. All characteristics are the same after the feature corelation analysis.

Corelation feature matrix.
3.3. Feature Extraction
It is necessary to select essential features that aid in classification. Its goal is to minimize dimensionality by identifying the most informative feature that may help the models perform better. We found that the RF feature selection approach identifies the essential features. It is also a prominent feature selection approach in machine learning methods, and it is worked well in real-world scenarios.
3.4. Classification Models
We propose a machine learning-based ensemble model (XGB, KNN, and DT) and an ML and DL-based stacked model (ML ensemble model, DNN, and KDNN) to improve the classification results. We applied four machine learning methodologies for our experiment: RF, KNN, DT, XGB, and two deep learning models, DNN and KDNN. This section shows how to use the machine learning ensemble classifier and DL method to identify cardiovascular disease and evaluate our approach's success effectively.
3.5. Machine Learning Ensemble Classifier
Researchers have used ensemble learning to solve a range of machine learning problems [ 24 , 25 ]. Because each dataset contains a wide range of problems, an ensemble learning approach is used to distinguish different diseases and categorize data appropriately. Every classifier in the ensemble estimates a class label whenever a new data point comes in. The class label that is predicted by the majority of the classifiers, or the class label with the most votes, is the one that is used for that instance. Try to improve performance by using a mix of ML-classifiers and different ways to vote [ 26 ]. These strategies are better than the classic single-learning approach for better generalization results.
The proposed ML ensemble classifier takes the predictions of several classifiers and uses a weighted majority method to develop the results. After each classification model has been fine-tuned, the best results are reported. The method in the equation is used to get the most votes in equation ( 2 ).
- K-Nearest Neighbour (KNN) is the essential machine learning approach for regression and classification. The data is used in KNN computations, which utilize similarity measurements (e.g., distance function) to describe new points. The KNN calculation relies on the similarity of the items being compared. In KNN, classification is determined by the majority vote of its immediate neighbors. The class with the most neighbors is labeled on the data point [ 27 ]. As the number of nearest neighbors increases, the choice of k and the accuracy may improve. We used the default parameter settings given by the sklearn library.
- Extreme Gradient Boosting helps to improve performance and use of memory resources. This ensemble learning approach uses XGBoost to improve the performance of classification. The XGBoost classifier is used to improve the accuracy of classification. XGBoost is a machine learning algorithm used on a large-scale dataset. It uses gradient descent to optimize the loss function and returns a prediction as a boosting ensemble of weak classification trees [ 28 ]. This model is unique in combining weak ML algorithms with an agile approach to learning. Gradient boosting is one of the few models of its sort. Gradient boosting uses the residual error to change the previous prediction to optimize the loss function. With XGBoost, the loss function is regularized to offer an objective function for assessing the model's performance, which is defined by X ′ ⊕ = Loss ⊕ + Ω ′ ⊕ . (3)
- Equation ( 3 ) indicates the parameters that may be inferred from the available data by adding oplus. The model's complexity is measured by the loss function (Loss) and the regularization term (Omega′). The XGBoost algorithm parameters utilized in this research are as follows: The maximum depth parameter is used by the XGBoost model. 6 is the highest depth that may be reached. Booster is gbtree, eta value is 0.3 in addition to the following parameters: scale position weight is 1, min-children-weight is also 1, and finally, the booster is 1 and all other parameters remain default.
- Decision Tree may be used to sort categorical and numerical data alike. It resembles a tree in terms of structure. When working with medical data, DT is the most popular approach. Creating and analyzing a tree-shaped graph is a cinch. As one of the most effective and extensively utilized methods for regulating learning, we turned to the DT classification method [ 29 ]. Building a stable decision tree for a specific data collection is straightforward. This model also used the default parameters while training on the given dataset.
3.6. Deep Learning Classifier
Deep neural networks have been used as DL-based classifiers (DNN). Recurrent neural network (RNN) models such as LSTM have also been employed, although LSTM did not perform well on the given dataset because of the abundance of numeric input. We used DL-based classifiers to evaluate cardiovascular disease detection and classification performance to provide a thorough study. The input layer with a ReLU activation function of 10 dimensions and a unit value of 16 is followed by three dense layers, each with a unit value of 12, 8, and 4, and a fully-connected layer with a sigmoid activation function associated directly with a specific value that the model is trying to predict; this model is called the DNN model. A total of 176 parameters make up the input layer. This layer has 204 parameters, each with an individual value of 12. One hundred four parameters with eight units are set in the second hidden layer, and 36 parameters with four in the final hidden layer. The learning rate of the DNN model is 0.001, and the loss is calculated using Binary cross-entropy in the DNN model.
It is possible to learn all the parameters, and the proposed model performed well on the test data. To prevent overfitting, we used a neural network known as KDNN, a robust medical disease detection approach. When we reduced the size of the dataset by removing outliers, we applied an effective medical disease detection Neural Network (KDNN) to control overfitting. The Keras Deep Neural Network (KDNN) model employs an Adam optimizer with Binary cross-entropy to compute the loss and simplify fine-tuning. The proposed KDNN model has a single input layer as its architecture. Finally, a single, fully connected layer is revealed after many hidden layers. Finally, a single, fully connected layer is revealed after many hidden layers. The 132 parameters are presented in the input layer. One hundred thirty parameters are buried in the following layer. The third hidden layer has 88 parameters, while the final hidden layer has 54 parameters with 411 training parameters. This fine-tuned KDDN model is successfully trained and tested on the Cardiovascular Disease dataset.
4. Results and Discussion
This section briefly describes the results and compares the baseline methods. This study's major objective is to examine the classification performance of recommended machine learning and deep learning classifiers for cardiovascular disease detection. The experiments for this study are done with the Cardiovascular Disease dataset. Both machine learning and deep learning are used in this study. The experiments are done in two different ways. Machine learning models are used in the first step to getting better results. We devised an ML-based ensemble model that performed well on the given dataset. This is done to get more reliable results. In the second step, we conducted experiments with deep learning approaches. Because there is not much data to work with, the deep learning models did not do well, so we made a stacked ensemble model to get better results from deep learning. This study used several measures of performance: accuracy, precision, recall, F1 score, confusion matrix, and area under the ROC curve (AUC-ROC score). The dataset is split into two parts: 70% of the data is used to train the model, and 30% is used to test the model. Python 3 and a Jupyter notebook running on an i5-8300H laptop with 16 GB RAM and a GTX 1050 2 GB VRAM are used to simulate the proposed model.
4.1. Experimental Results
Table 2 demonstrates the results of the machine learning classifiers on the Cardiovascular Disease dataset to identify the existence of cardiovascular disease. RF, KNN, DT, and XGB are employed in this research. Table 2 demonstrates that, compared to other machine learning models, the RF classifier obtained the best accuracy rate of 88.65%. RF's precision, recall, F1, and AUC scores are 90.03%, 80.03%, 80.02%, and 92%, respectively. We also plot the confusion matrix of the RF classifier. Figure 3 shows the confusion matrix of the RF classifier, where diagonal values are accurately classified by the RF classifier and nondiagonal are misclassified by the RF classifier. In addition, Figure 4 shows the ROC Curve plot with the ROC score of 92%, representing that the RF classifier performed very well on the Cardiovascular Disease dataset.

Confusion matrix of random forest classifier.

ROC AUC curve of random forest classifier.
Machine learning classifier performance (%) for cardiovascular disease detection.
The second stage of the experiments shows the deep learning model results. The deep learning model results are presented in Table 3 . Both the deep learning models performed exceptionally well. Both models' accuracy score is almost the same but less than the machine learning model because the deep learning model performed well on a large amount of data. The DNN model gets the highest accuracy than the KDNN model. The accuracy, precision, recall, F1 score, and ROC AUC score of the DNN model are 87.59%, 97.77%, 76.27%, 65.54%, and 91.50%. We also computed the loss of the DNN model, which is 30.19%. The training and validation loss of the DNN model is shown in Figure 5 while Figure 6 shows the training and validation accuracy of the DNN model on 400 epochs.

Training and validation loss of DNN model.

Training and validation accuracy of DNN model.
Deep learning classifier performance (%) for cardiovascular disease detection.
The confusion matrix of the DNN model is presented in Figure 7 , and the ROC AUC curve plot is shown in Figure 8 .

Confusion matrix of DNN model.

ROC AUC curve of DNN model.
A machine learning ensemble classifier and an ML and DL stacked classifier are used to get the final findings in this study. This classifier has the greatest accuracy of 88.70% among all other classifiers. However, the lack of data meant that the stacked classifier did not perform effectively. ML Ensemble has an 88.02% precision and recall rate, an 88.01% F1 score, and a 93% ROC AUC. The confusion matrix and AUC ROC of the proposed ML Ensemble classifier are shown in Figures Figures9 9 and and10. 10 . The results show that the proposed ML ensemble classifier can efficiently detect the presence of cardiovascular disease from the Cardiovascular Disease dataset.

Confusion matrix of ML Ensemble model.

ROC AUC curve of ML ensemble model.
Table 4 provides the results for the ensemble ML model consisting of ML and DL stacked classifiers to detect cardiovascular disease. The stacked classifier achieved the accuracy of 86.49%, Precision of 87.32%, Recall of 86.02%, F1 score of 86.01%, and ROC-ACU of 99% while the ML ensemble model achieved the accuracy of 88.70%, Precision of 88.02%, Recall of 88.02%, F1 score of 88.01%, and ROC-ACU of 99%. To sum up, the ML model achieved the best results in detecting cardiovascular disease.
ML ensemble and DL stacked classifier performance (%) to detect cardiovascular disease.
4.2. Comparative Analysis
The comparative analysis of the proposed and baseline approaches is presented in Table 5 . We broadly compare with two baseline research studies [ 30 , 31 ]. The experimental setting of the proposed and baseline studies are almost the same. This research used an ML Ensemble method to detect cardiovascular disease.
Comparison of proposed and baseline approaches classifiers performance to detect cardiovascular disease.
In Table 5 , we compare this study's findings to our baseline method. Alfaidi et al. used various ML models (LR, RF, DT, NB, KNN, SVM, and MLP) and achieved the highest result from the MLP model with 87.23% accuracy [ 30 ]. Arroyo and Delima used two Artificial neural networks, and five ML models (LR, DT, RF, SVM, and KNN) [ 31 ]. This study obtained the highest accuracy using the GA-ANN model, 73.43%. The proposed approach used a majority voting approach and created an ML Ensemble model, which obtained the highest accuracy compared with the baseline results. The ML Ensemble model obtained an accuracy score of 88.70%. The accuracy gain of 1.47% using the ML Ensemble model shows that the proposed ML Ensemble performed efficiently to detect cardiovascular disease.
5. Conclusion
This study proposed ensemble-based machine and deep learning approaches to predict cardiovascular disease. For this study, we used 70000 patients' data with various forms of cardiovascular disease. The accuracy of the models was used as a measure of their performance. In addition, we selected additional informative characteristics that influence the models' performance. According to the results, the ML Ensemble model was the most accurate in predicting cardiovascular disease. We have added a few procedures to get the dataset ready for analysis. We may employ various strategies to find the best features for our future work. For a better and more accurate assessment, additional datasets may be employed. Finally, deep learning and reinforcement learning approaches may tackle the prediction issue to detect cardiovascular disease more efficiently.
Data Availability
Conflicts of interest.
The authors declare that they have no conflicts of interest.
American College of Cardiology
Site improvements in progress., thank you for your patience..
This site is undergoing maintenance from Friday, May 10 until Tuesday, May 21 to modernize and improve the College's digital infrastructure. Please visit JACC.org for access to clinical guidelines and related resources, as well as the latest science and research from all 10 JACC journals. For assistance during this period, please reach out to the ACC Member Care team at [email protected] . Please be aware that our customer service may be limited until our systems are fully restored.
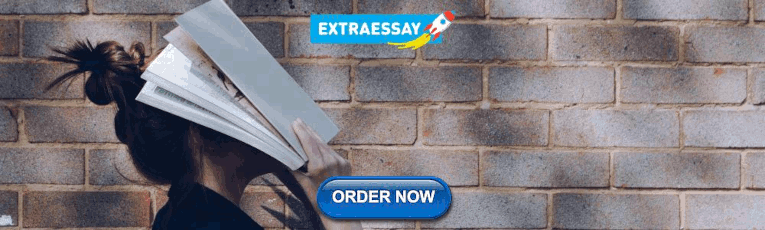
IMAGES
VIDEO
COMMENTS
Cardiovascular diseases (CVDs), principally ischemic heart disease (IHD) and stroke, are the leading cause of. global mortality and a major contributor to disability. This paper reviews the ...
Cardiovascular disease is the leading cause of death worldwide, and the global prevalence of cardiovascular disease increased every year. Cardiovascular disease (CVD) is a complex multifactorial disease. ... This thesis studies the possible determinants for imaging markers of cardiovascular disease risk, including carotid intima media thickness ...
The purpose of this dissertation was to examine factors associated with cardiovascular disease (CVD) risk among emerging adults in college aged 18-25 years. CVD risks that develop during this period often persist into adulthood making it an ideal time to target CVD prevention.
Abstract. Cardiovascular disease has become a growing global and public health concern among non-communicable diseases (NCDs). The purpose of the study was to focus on the increasing prevalence of the risk factors of cardiovascular diseases (CVD), irrespective of age and gender, and its effect on public health worldwide.
Introduction. The thesis of this essay is that the longstanding emphasis on cardiovascular disease (CVD) has at last yielded to a mounting force behind cardiovascular health (CVH), manifest in adoption by the American Heart Association (AHA) of its 2020 Impact Goal: "By 2020, to improve the CVH of all Americans by 20% while reducing deaths from CVDs and stroke by 20%." 1 It is proposed ...
2 Division of Cardiovascular Disease, Department of Medicine, University of Alabama at Birmingham, Lyons Harrison Research Building 306, 1900 University BLVD, Birmingham, AL, 35294, USA. [email protected]. 3 Section of Cardiology, Birmingham Veterans Affairs Medical Center, Birmingham, AL, USA. [email protected].
Patients with coronary artery disease & congestive heart failure need to be on guided medical therapy for recovery from primary event as well as for prevention of further cardiovascular events. Cardiac Rehabilitation programs have shown improvements in secondary prevention of cardiovascular events; however, no clinical studies are available to show
Introduction. Cardiovascular disease remains a major cause of premature mortality and rising health care costs across the world ().In China, the prevalence rate of cardiovascular disease (CVD) increased significantly by 14.7% from 1990 to 2016 (), which was likely to increase substantially in pace with population growth and aging.Among the major drivers of CVD, atherogenic dyslipidemia is one ...
communication of cardiovascular risk becomes a challenge. The overall aim of the thesis was to investigate the publics' perspective of cardiovascular risk information through a multi-method approach, including how individuals perceive risk, factors affecting an underestimation of risk, how cardiovascular risk communication affects
Introduction. Thought of broadly, the management of patients with stable coronary artery disease (CAD) involves significant complexity including the diagnosis of CAD, assessment of associated risk, treatment, and monitoring of symptoms during follow up. 1 To provide sufficient focus in this month's topic review in Circulation: Cardiovascular Quality and Outcomes, we have concentrated on the ...
LOUIS. Department of Mathematics. Program in Statistics Survival Analysis of Cardiovascular Diseases by Yuanxin Hu A thesis presented to the Graduate School of Arts & Sciences. of Washington University in. partial fulfillment of the requirements for the degree of Master of Arts December 2013 Saint Louis, Missouri. ii.
Cardiovascular and cerebrovascular diseases (CCVD) are prominent mortality causes in Japan, necessitating effective preventative measures, early diagnosis, and treatment to mitigate their impact.
Cardiovascular diseases claim a number of lives globally; many of which are preventable. With the increase in diets that consist of high saturated fat, salt, and sugar, people often living sedentary lifestyles, and a rise in cases of obesity, the incidence of cardiovascular disease is increasing. These contributing factors, coupled with more advanced methods of diagnosis, have delivered ...
Evolution of cardiovascular topics—trends and 'hot' topics. We identified 175 topics, listed alphabetically in Supplementary material online, Table S2. This list groups specific topics within areas such as atherosclerosis, coronary artery disease, arrhythmias, heart failure, and their evolution over time.
Background: The goal of this work was to investigate trends (2001-2019) for cardiovascular events and cardiometabolic risk factor levels in individuals with type 2 diabetes (T2D) and matched control subjects. Methods: This study included 679 072 individuals with T2D from the Swedish National Diabetes Register and 2 643 800 matched control subjects.
3) Machine Learning algorithms allowed us to analyze clinical data, draw. relationships between diagnostic variables, design the predictive model, and. tests it against the new case. The predictive model achieved an accuracy of 89.4. percent using RandomForest Classifier's default setting to predict heart diseases.
This prediction is an area that is widely researched. Our paper is part of the research on the detection and prediction of heart disease. It is based on the application of Machine Learning ...
Background Cardiovascular diseases (CVDs) are the global public health problem which has been associated with increasing prevalence of modifiable CVDs risk factors. This study aimed to describe the prevalence and knowledge of modifiable CVDs risk factors among vulnerable population of Central Tanzania. Methods A community-based cross-sectional study design was employed. A total of 749 ...
Conclusion: Study findings support the use of the Reynolds Risk score rather than Framingham risk calculators when calculating cardiovascular risk among women, especially among those with low-intermediate cardiovascular risk per the ATP III model who may benefit from treatment with a statin for primary prevention in the setting of an elevated C ...
Key Points. Question Do neighborhood factors (physical and social environments) and individual stressors (discrimination) mediate racial differences in cardiovascular health?. Findings In this cross-sectional study of 7720 participants, Black adults had poorer cardiovascular health than White adults. Neighborhood physical environment and safety attenuated racial differences in total ICH scores ...
Introduction. Current policies for public funding of health research increasingly focus on innovation, with a final goal to improve health outcomes. 1 To support policies, roadmaps are established, for example for diabetes 2 and respiratory 3 diseases. In the USA, the joint Academies developed a document to guide national policy in health 4 with a dedicated document for cardiovascular medicine ...
Cardiovascular Stem Cell Program. Center for Health Technology and Innovation. CardioScience Webinar Series. Home Heart and Vascular Institute Cardiovascular Research. Research Topics. Cardiovascular Research Topics. Heart Rhythm and Arrhythmias. HIV and Heart Disease. Hypertension. Imaging.
This paper proposes an ensemble-based approach that uses machine learning (ML) and deep learning (DL) models to predict a person's likelihood of developing cardiovascular disease. We employ six classification algorithms to predict cardiovascular disease. Models are trained using a publicly available dataset of cardiovascular disease cases.
Minnesota State University, Mankato
The heart/brain connection is impacted by factors across the levels of the person's biology, psychology and social environment to ultimately impart cumulative cardiovascular disease risk. Therefore, when treating cardiovascular disease, considering the entire person is needed to identify the best course of intervention. In many cases, such an ...